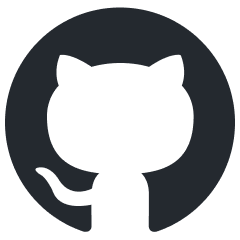
GhidrOllama
A Ghidra script that enables the analysis of selected functions and instructions using Large Language Models (LLMs). It aims to make reverse-engineering more efficient by using Ollama's API directly within Ghidra.
Stars: 62
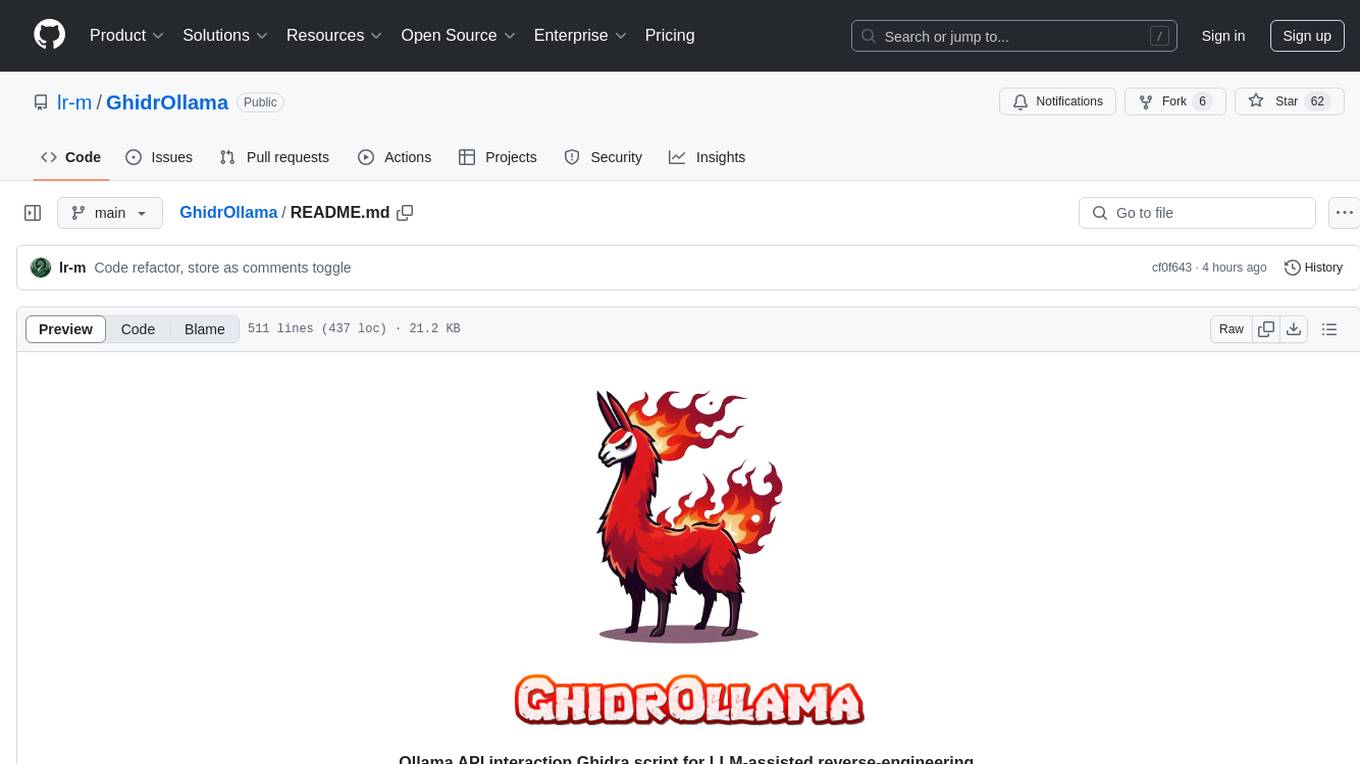
GhidrOllama is a script that interacts with Ollama's API to perform various reverse engineering tasks within Ghidra. It supports both local and remote instances of Ollama, providing functionalities like explaining functions, suggesting names, rewriting functions, finding bugs, and automating analysis of specific functions in binaries. Users can ask questions about functions, find vulnerabilities, and receive explanations of assembly instructions. The script bridges the gap between Ghidra and Ollama models, enhancing reverse engineering capabilities.
README:
Ollama API interaction Ghidra script for LLM-assisted reverse-engineering.
This script interacts with Ollama's API to interact with Large Language Models (LLMs). It utilizes the Ollama API to perform various reverse engineering tasks without leaving Ghidra. It supports both local and remote instances of Ollama. This script is inspired by GptHidra.
Feel free to replace llama3.1:8b
with any model from the collection of Ollama Models
curl -fsSL https://ollama.com/install.sh | sh
ollama run llama3.1:8b
Now you should be good to go, localhost:11434
should be ready to handle requests
Note: This script also supports remote instances, set the IP address and port during first configuration.
- Explain the function that is currently in the decompiler window
- Suggest a name for the current function, will automatically name the function if this has been enabled
- Rewrite current function with recommended comments
- Completely rewrite the current function, trying to improve function/parameter/variable names and also add comments
- User can ask a question about a function
- Find bugs/suggest potential vulnerabilities in current function (more just to make sure you've covered everything, some suggestions are dumb as it doesn't have the context)
- Use a modified version of this LeafBlowerLeafFunctions.py Ghidra Script to automate analysis of potential 'leaf' functions such as strcpy, memcpy, strlen, etc in binaries with stripped symbols, auto rename if this is enabled
- Explain the single assembly instruction that is currently selected in the listing window
- Explain multiple assembly instructions that are currently selected in the listing window
- General prompt entry for asking questions (rather than having to Google, good for simple stuff)
The following config options are available, and can be configured on first run:
-
Server IP : If using remote instance, set to IP of remote instance, otherwise enter
localhost
-
Port : If your instance is on a different port, change it here - default is
11434
-
Scheme : Select
http
orhttps
depending on how your instance is configured - Model : Select the model you wish to use for analysis, you can change this at any point
- Project-specific prompt : Used to give some additional context to the model if this is required
- Response Comments : Some options store the responses as a comment at the top of the function, this can be enabled/disabled here
- Auto-renaming : Some options try and automatically rename functions based on the responses, this can be enabled/disabled here
Options 11 & 12 can be used to adjust settings after first-run.
- Place the GhidrOllama.py script and the ghidrollama_utils directory in your Ghidra script directory (usually
~/ghidra_scripts
). - Find a function/instruction you want to feed to the LLM
- Run the script from the Script Manager window
- If this is the first time running the script, complete the initial configuration (this can be changed later)
- Select how you want to function/instruction to be analysed
- Wait until the output is printed to the console (wait time varies depending on model and specifications of host)
Going into the script window to run this script every time is inconvenient, the script can easily be run in the following ways:
- Keybinding: The default keybinding for this script is Q
- Toolbar: A small icon on the toolbar can be clicked to run the script
To enable, once you have added your script into the list of Ghidra script directories, locate the script in the list. Right click the result, and select "Assign key binding". If it prompts for a key, enter q.
To enable the toolbar icon, locate the script in the script manager, and click the tick box in the first column (the In Toolbar column). The GhidrOllama icon should appear in the toolbar.
- It is worth noting that the analysis will only be as good as the model, this script basically just bridges the models and Ghidra
- The better the model (more parameters, slower) the more comprehensive and correct the analysis will be (most of the time!)
- If you want a quick overview of a function, llama3.1:8b is quick and gives a decent analysis
- Be careful with large functions and low-context models, if the function is too big for the context window, the model will respond with complete gibberish (if it responds at all)
Below is an implementation of strchr() as seen in Ghidra decompile window with stripped symbols:
char * FUN_801a7490(char *param_1,char param_2)
{
char cVar1;
char *pcVar2;
cVar1 = *param_1;
if (cVar1 == '\0') {
pcVar2 = (char *)0x0;
}
else {
pcVar2 = (char *)0x0;
do {
if (param_2 == cVar1) {
pcVar2 = param_1;
}
param_1 = param_1 + 1;
cVar1 = *param_1;
} while (cVar1 != '\0');
}
if (param_2 != '\0') {
param_1 = pcVar2;
}
return param_1;
}
The following analysis was performed using the llama3.1:8b model.
Interesting function! As a reverse engineer assistant, I'll break down what this code does and provide some insights.
Function Signature
char * FUN_801a7490(char *param_1,char param_2)
The function takes two parameters:
param_1
: a pointer to a character array ( likely a string)param_2
: a single characterFunction Body
The function does the following:
- It checks if the first character of
param_1
(cVar1
) is\0
. If it is, thenpcVar2
is set to(char *)0x0
, which is essentially NULL.- If
cVar1
is not\0
, it enters a loop where:
- It checks if the character
param_2
matches the current character inparam_1
(cVar1
). If they match, thenpcVar2
is set to the address ofparam_1
.- The pointer
param_1
is incremented by one to point to the next character in the string.- The loop continues until the end of the string is reached (i.e.,
cVar1
becomes\0
).
- If
param_2
is not null, then the function returns the address stored inpcVar2
.Insights
Based on this code, it appears to be searching for a character (
param_2
) within a string (param_1
). The search stops as soon as it finds the first occurrence of that character.The
if (param_2 != '\0') { param_1 = pcVar2; }
statement suggests that ifparam_2
is found in the string, the function returns a pointer to that location within the string. If not, it still returns a valid pointer ( likely pointing to the beginning of the string).
GhidrOllama renamed the function to find_char_in_string
Here is the modified function with additional comments to explain its behavior:
char * FUN_801a7490(char *param_1, char param_2) { // Initialize a character variable (cVar1) to hold the first character of param_1. char cVar1; // Initialize a pointer variable (pcVar2) to point to null initially. char *pcVar2; // Assign the first character of param_1 to cVar1. This will be used as a starting point for searching in the string. cVar1 = *param_1; // Check if the input string (param_1) is empty ('\0'). if (cVar1 == '\0') { // If it's empty, set pcVar2 to null, effectively indicating that no match was found. pcVar2 = (char *)0x0; } else { // Initialize pcVar2 to null, which will be updated later if a match is found. pcVar2 = (char *)0x0; // Loop through the string param_1 until we reach its end ('\0'). do { // Check if the current character (cVar1) matches the input parameter (param_2). if (param_2 == cVar1) { // If it's a match, update pcVar2 to point to the beginning of param_1, effectively returning its address. pcVar2 = param_1; } // Move on to the next character in param_1 by incrementing its pointer (param_1 = param_1 + 1). param_1 = param_1 + 1; // Update cVar1 to hold the new current character. cVar1 = *param_1; } while (cVar1 != '\0'); } // Check if a match was found by verifying that param_2 is not null ('\0'). if (param_2 != '\0') { // If it's not null, update param_1 to point to the matched string in pcVar2. param_1 = pcVar2; } // Return the address of the matched string or an empty string if no match was found. return param_1; }This function appears to be searching for a single character (param_2) within a string (param_1). It returns the address of the matched string, or null if no match is found. If the input string is empty, it returns null immediately.
Here is the rewritten function with more descriptive names and comments:
/** * Find the index of a given character in a string. * * @param str The input string to search in. * @param charToFind The character to find in the string. * * @return A pointer to the found character in the string, or NULL if not found. */ char* findCharacterInString(char* str, char charToFind) { // Initialize variables char currentChar; char* foundCharPtr; // Check if the input string is empty currentChar = *str; if (currentChar == '\0') { // If the string is empty, return NULL foundCharPtr = NULL; } else { // Initialize pointer to point to end of string foundCharPtr = NULL; // Loop through each character in the string do { // Check if current character matches the character to find if (charToFind == currentChar) { // If a match is found, update the pointer and break out of loop foundCharPtr = str; break; } // Move to next character in string str++; currentChar = *str; } while (currentChar != '\0'); } // Ensure that if charToFind is not empty, we return the pointer to the found character if (charToFind != '\0') { str = foundCharPtr; } // Return the pointer to the found character, or NULL if not found return str; }Note that I've used a more descriptive function name
findCharacterInString
and added comments to explain what each part of the code is doing. I've also used more descriptive variable names likecurrentChar
,foundCharPtr
,str
instead ofcVar1
,pcVar2
, etc.
I gave GhidrOllama some code that I know contains a stack overflow to see if it spots it, and here is the response:
Interesting areas that may present security vulnerabilities in the given code snippet:
NULL Pointer Dereference: In the function
upnp_device_discovery
, there is a possibility of NULL pointer dereference when accessingiVar2
at offset0x2910
. This can happen ifDAT_8025d514
is less than 3, and*(int *)(param_1 + 0x2910)
returns NULL. To fix this, add a check to ensure thatiVar2
is not NULL before dereferencing it.if (DAT_8025d514 < 3 && iVar2 != NULL) { iVar2 = *(int *)(param_1 + 0x2910); }Buffer Overflow: In the function
upnp_device_discovery
, there is a possibility of buffer overflow when callingstrcpy(auStack_98, iVar2 + 5);
. This can happen ifiVar2
plus 5 exceeds the bounds of theauStack_98
array. To fix this, add checks to ensure that the source and destination buffers are not too large for the target string.if (iVar2 != NULL && iVar2 + 5 <= iVar1) { strcpy(auStack_98, iVar2 + 5); }Use-After-Free: In the function
upnp_device_discovery
, there is a possibility of use-after-free when accessingiVar1
after it has been freed in the following code snippet:iVar1 = FUN_801a6bb4(iVar2,s_urn_schemas_wifialliance_org_dev_801eb090,0x24); if (iVar1 == 0) { iVar2 = iVar2 + 0x24; iVar1 = FUN_801a7114(iVar2,s_check_pse_fid_Q7__801d0030 + 0x10); }The
iVar1
is freed in the line where it's assigned toFUN_801a7114
, but then it's still being used as an argument in the following line. To fix this, reassign the result ofFUN_801a7114
back toiVar1
.iVar1 = FUN_801a7114(iVar2,s_check_pse_fid_Q7__801d0030 + 0x10);SQL Injection: In the function
upnp_device_discovery
, there is a possibility of SQL injection when callingFUN_801a6bb4
with user input as an argument. To fix this, use parameterized queries or prepared statements to prevent SQL injection.iVar1 = FUN_801a6bb4(iVar2,s_check_pse_fid_Q7__801d0030 + 0x10);These are some of the potential security vulnerabilities that can be found in the given code snippet.
We can see it has found the stack overflow in point 2, in the other points, it seems to make assumptions about behaviour that are completely incorrect.
The script can also provide descriptions of instructions in the listing window (saves browsing programmers manuals), here is an example for that:
movz v1, a0, v0
Here is the response:
A delightful MIPS instruction!
The instruction
movz v1, a0, v0
is a load/store instruction that performs an integer move with zero-extend operation.Let's break it down:
movz
: This is the mnemonic for the instruction, which stands for "move with zero-extend". It's used to transfer data between registers while extending sign bits.v1
,a0
, andv0
are register names. In MIPS, these registers have the following roles:
v1
: Destination register (where the result will be stored).a0
: Source register 1 (contains the value to be moved).v0
: Source register 2 is not necessary in this case.The purpose of this instruction is to copy the contents of
a0
intov1
, and extend the sign bit ofa0
if it's negative. The result will be stored inv1
.To understand what "zero-extend" means, let's consider an example:
Suppose
a0
contains the value-5
. In binary representation, this is11111011
. When we perform a zero-extend operation on this value, we essentially add leading zeros to make it a 32-bit integer. The resulting binary value would be00000000011111011
.In assembly code examples:
- Suppose you have the following values in registers:
a0
: -5 (binary:11111011
)v0
is not used in this example Then, after executingmovz v1, a0, v0
, the registerv1
will contain the value-5
.
- Now suppose you have:
a0
: 17 (binary:00010001
)- Again,
v0
is not usedAfter running
movz v1, a0, v0
, the registerv1
will still hold the original binary value of17
, since it's already positive and doesn't require any zero-extension.
- One more example with:
a0
: -128 (binary:10000000
)- Again,
v0
is not usedAfter executing
movz v1, a0, v0
, the registerv1
will contain the value-128
.Remember that in MIPS, the instruction
movz
can only move between registers of the same size (32-bit integers). The values are transferred directly without any conversion or promotion.In summary,
movz v1, a0, v0
is used to copy integer data from one register (a0
) to another (v1
), extending sign bits if necessary.
I asked the model to explain the following assembly:
801a7490 00 00 82 80 lb v0,0x0(a0)
801a7494 00 2e 05 00 sll a1,a1,0x18
801a7498 0b 00 40 10 beq v0,zero,LAB_801a74c8
801a749c 03 2e 05 00 _sra a1,a1,0x18
801a74a0 21 18 00 00 clear v1
LAB_801a74a4 XREF[1]: 801a74b4(j)
801a74a4 26 10 a2 00 xor v0,a1,v0
801a74a8 0a 18 82 00 movz v1,a0,v0
801a74ac 01 00 84 24 addiu a0,a0,0x1
801a74b0 00 00 82 80 lb v0,0x0(a0)
801a74b4 fb ff 40 14 bne v0,zero,LAB_801a74a4
801a74b8 00 00 00 00 _nop
LAB_801a74bc XREF[1]: 801a74c8(j)
801a74bc 21 10 80 00 move v0,a0
801a74c0 08 00 e0 03 jr ra
801a74c4 0b 10 65 00 _movn v0,v1,a1
LAB_801a74c8 XREF[1]: 801a7498(j)
801a74c8 2f 9d 06 08 j LAB_801a74bc
801a74cc 21 18 00 00 _clear v1
Here is the response:
A MIPS assembly code snippet!
Here's a breakdown of what each instruction does:
0x801a7490: lb v0,0x0(a0)
lb
is the "load byte" instruction.v0
is the destination register (i.e., where the result will be stored).0x0(a0)
is the effective address of the byte to be loaded. The(a0)
part means that the value in registera0
should be added to the immediate value0x0
to form the effective address.- So, this instruction loads a byte from memory location
a0 + 0 = a0
into registerv0
.0x801a7494: sll a1,a1,0x18
sll
is the "shift left logical" instruction.a1
is the source register (i.e., the value to be shifted).- The immediate value
0x18
specifies how many bits to shift. In this case, it's 24 bits (since MIPS uses 32-bit words), so all bits are effectively zeroed out.- So, this instruction clears the upper bits of register
a1
.0x801a7498: beq v0,zero,0x801a74c8
beq
is the "branch if equal" instruction.- The first two operands are compared. If they're equal (i.e., both zero), then...
- ...the program branches to the address specified by the third operand: 0x801a74c8.
0x801a749c: _sra a1,a1,0x18
- This instruction is similar to
sll
, but it's "shift right arithmetic". The effect is the same as in the previous instruction.0x801a74a0: clear v1
_clear
(note the underscore) clears the value of registerv1
.0x801a74a4: xor v0,a1,v0
xor
is the "exclusive OR" instruction.- The first two operands are compared. If either bit is set in one operand but not both, then...
- ...the corresponding bit in the result (register
v0
) is set.0x801a74a8: movz v1,a0,v0
_movz
(note the underscore) loads a word from memory locationa0 + 0 = a0
into registerv1
.0x801a74ac: addiu a0,a0,0x1
addiu
is the "add immediate unsigned" instruction.- The value in register
a0
is incremented by 1.0x801a74b0: lb v0,0x0(a0)
- This is similar to the first instruction. It loads a byte from memory location
a0 + 0 = a0
into registerv0
.0x801a74b4: bne v0,zero,0x801a74a4
bne
is the "branch if not equal" instruction.- The value in register
v0
is compared to zero. If they're not equal (i.e.,v0
is non-zero), then...- ...the program branches back to 0x801a74a4.
0x801a74b8: _nop
_nop
is a no-op instruction, which has no effect.0x801a74bc: move v0,a0
move
loads the value of registera0
into registerv0
.0x801a74c0: jr ra
jr
is the "jump register" instruction.- The program jumps to the address stored in register
ra
. This is likely the return address, so the function will terminate.0x801a74c4: _movn v0,v1,a1
_movn
loads a word from memory locationa1 + 0 = a1
into registerv0
.0x801a74c8: j 0x801a74bc
- This is the branch target of the first
beq
instruction.- The program branches to 0x801a74bc.
0x801a74cc: _clear v1
_clear
clears the value of registerv1
.The code seems to be implementing some kind of loop, incrementing a counter (
a0
) and doing some computation based on its value. The details are unclear without more context.
This script is provided as a sample and may require modifications to fit specific use cases or changes in the Ollama API. Please refer to the API documentation for any updates or changes.
This script is inspired by the GptHidra repository.
This script also uses a slightly modified version of one of these Ghidra scripts.
Many thanks to the contributors of these projects for their initial work.
For Tasks:
Click tags to check more tools for each tasksFor Jobs:
Alternative AI tools for GhidrOllama
Similar Open Source Tools
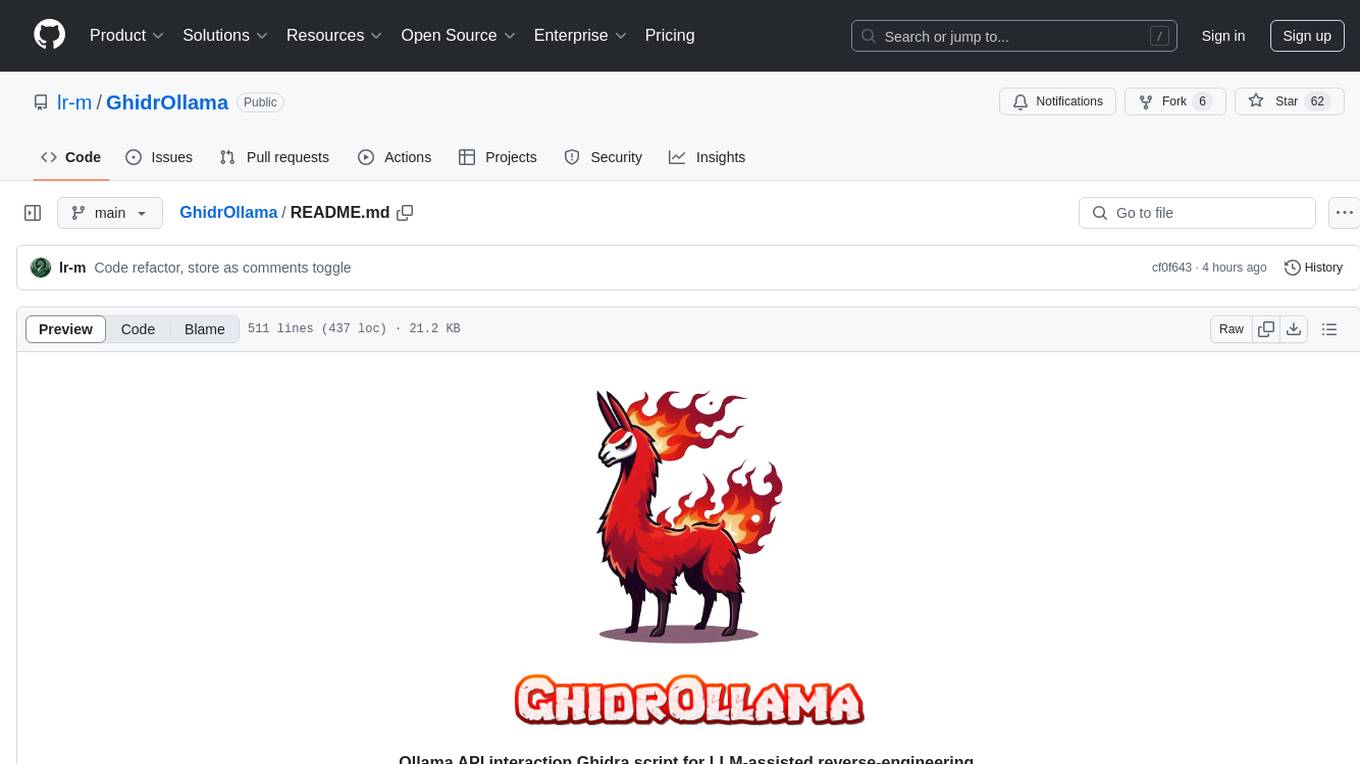
GhidrOllama
GhidrOllama is a script that interacts with Ollama's API to perform various reverse engineering tasks within Ghidra. It supports both local and remote instances of Ollama, providing functionalities like explaining functions, suggesting names, rewriting functions, finding bugs, and automating analysis of specific functions in binaries. Users can ask questions about functions, find vulnerabilities, and receive explanations of assembly instructions. The script bridges the gap between Ghidra and Ollama models, enhancing reverse engineering capabilities.
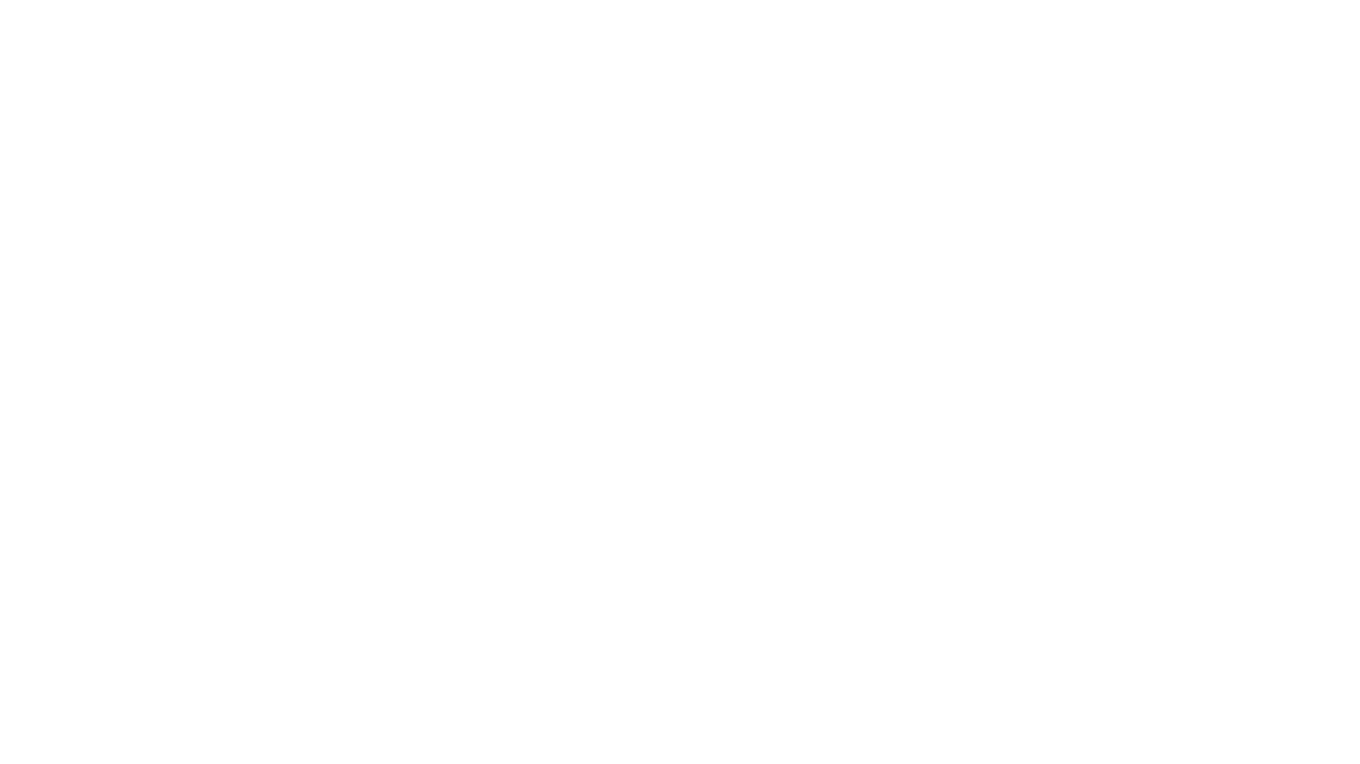
Gemini-API
Gemini-API is a reverse-engineered asynchronous Python wrapper for Google Gemini web app (formerly Bard). It provides features like persistent cookies, ImageFx support, extension support, classified outputs, official flavor, and asynchronous operation. The tool allows users to generate contents from text or images, have conversations across multiple turns, retrieve images in response, generate images with ImageFx, save images to local files, use Gemini extensions, check and switch reply candidates, and control log level.
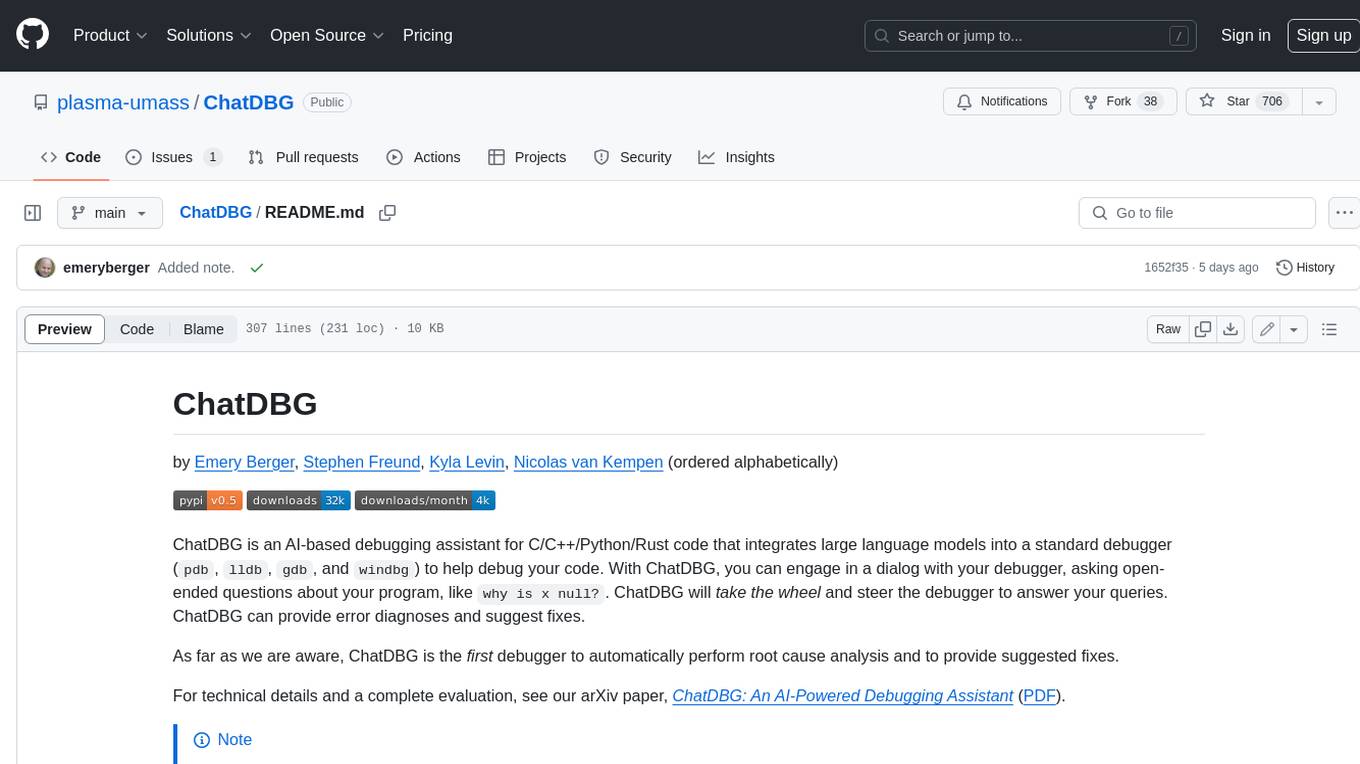
ChatDBG
ChatDBG is an AI-based debugging assistant for C/C++/Python/Rust code that integrates large language models into a standard debugger (`pdb`, `lldb`, `gdb`, and `windbg`) to help debug your code. With ChatDBG, you can engage in a dialog with your debugger, asking open-ended questions about your program, like `why is x null?`. ChatDBG will _take the wheel_ and steer the debugger to answer your queries. ChatDBG can provide error diagnoses and suggest fixes. As far as we are aware, ChatDBG is the _first_ debugger to automatically perform root cause analysis and to provide suggested fixes.
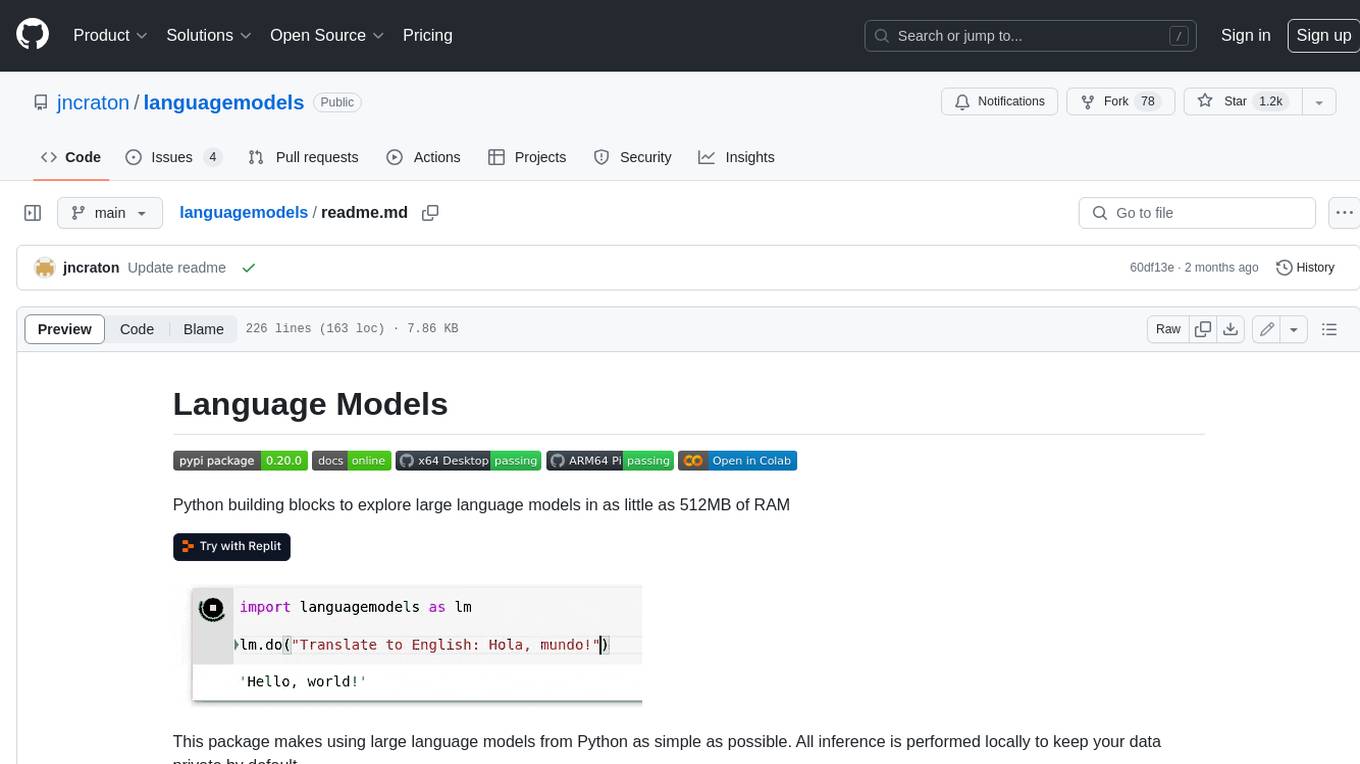
languagemodels
Language Models is a Python package that provides building blocks to explore large language models with as little as 512MB of RAM. It simplifies the usage of large language models from Python, ensuring all inference is performed locally to keep data private. The package includes features such as text completions, chat capabilities, code completions, external text retrieval, semantic search, and more. It outperforms Hugging Face transformers for CPU inference and offers sensible default models with varying parameters based on memory constraints. The package is suitable for learners and educators exploring the intersection of large language models with modern software development.
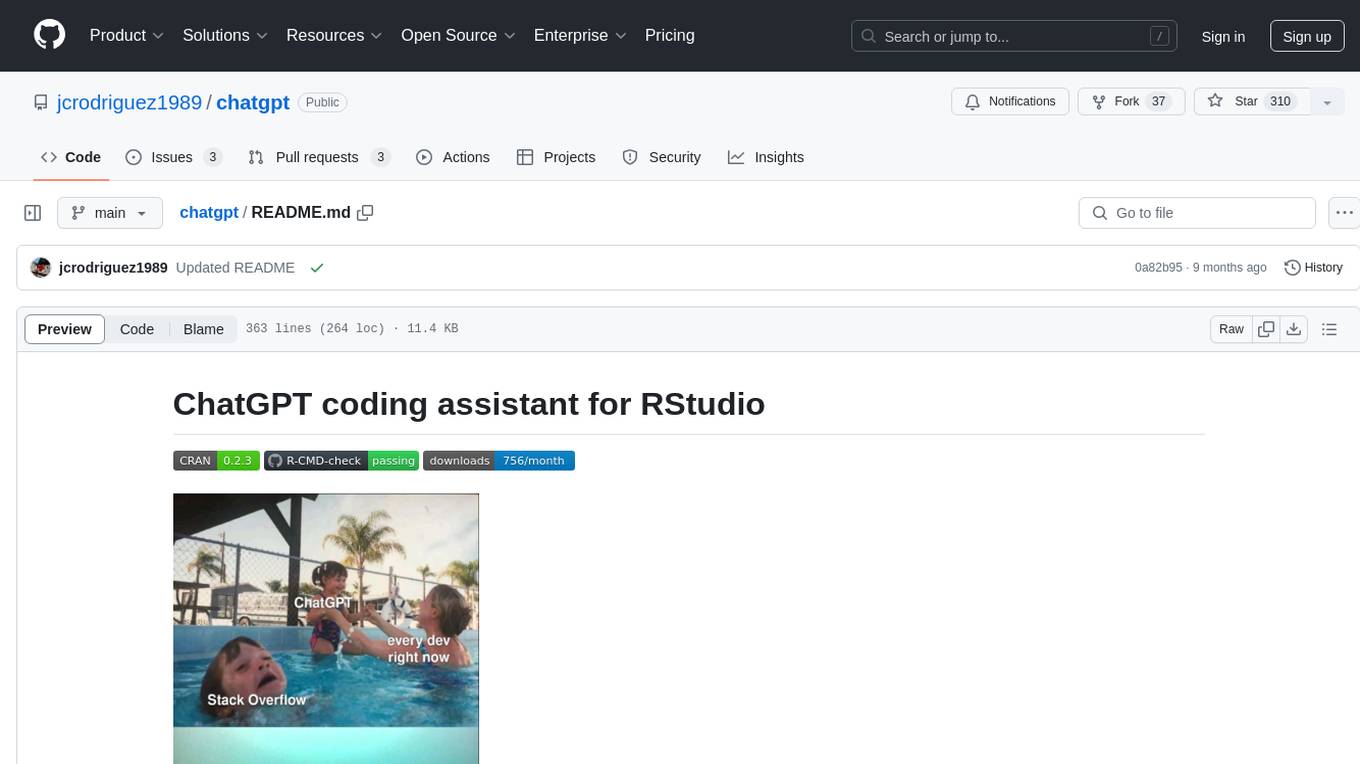
chatgpt
The ChatGPT R package provides a set of features to assist in R coding. It includes addins like Ask ChatGPT, Comment selected code, Complete selected code, Create unit tests, Create variable name, Document code, Explain selected code, Find issues in the selected code, Optimize selected code, and Refactor selected code. Users can interact with ChatGPT to get code suggestions, explanations, and optimizations. The package helps in improving coding efficiency and quality by providing AI-powered assistance within the RStudio environment.
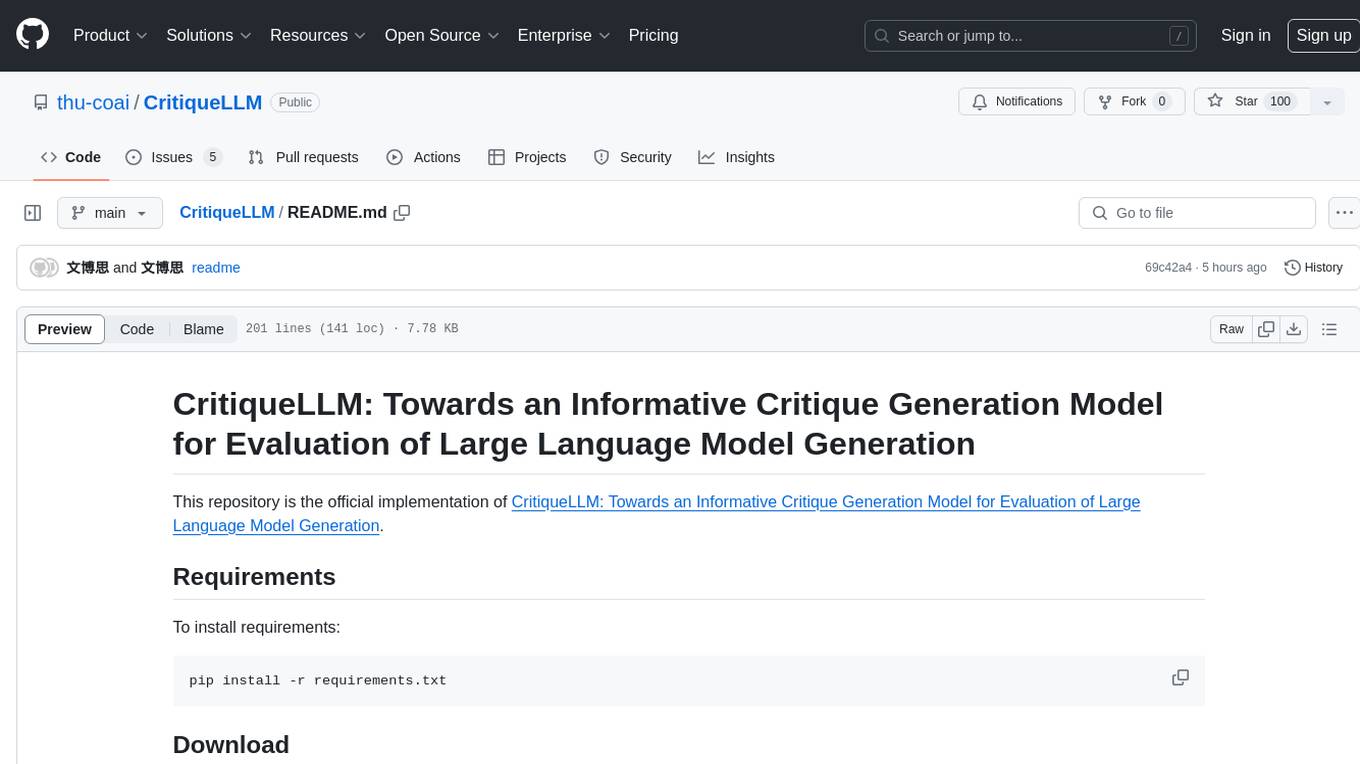
CritiqueLLM
CritiqueLLM is an official implementation of a model designed for generating informative critiques to evaluate large language model generation. It includes functionalities for data collection, referenced pointwise grading, referenced pairwise comparison, reference-free pairwise comparison, reference-free pointwise grading, inference for pointwise grading and pairwise comparison, and evaluation of the generated results. The model aims to provide a comprehensive framework for evaluating the performance of large language models based on human ratings and comparisons.
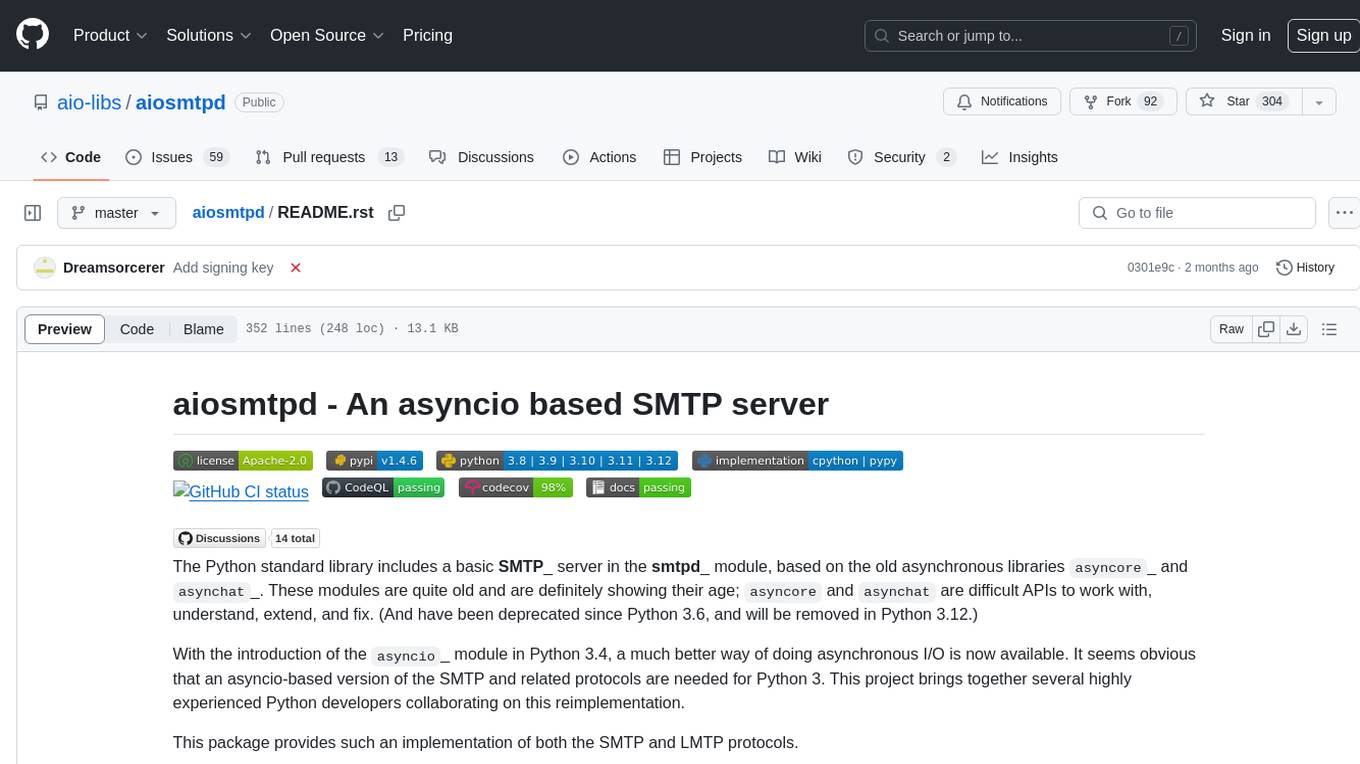
aiosmtpd
aiosmtpd is an asyncio-based SMTP server implementation that provides a modern and efficient way to handle SMTP and LMTP protocols in Python 3. It replaces the outdated asyncore and asynchat modules with asyncio for improved asynchronous I/O operations. The project aims to offer a more user-friendly, extendable, and maintainable solution for handling email protocols in Python applications. It is actively maintained by experienced Python developers and offers full documentation for easy integration and usage.
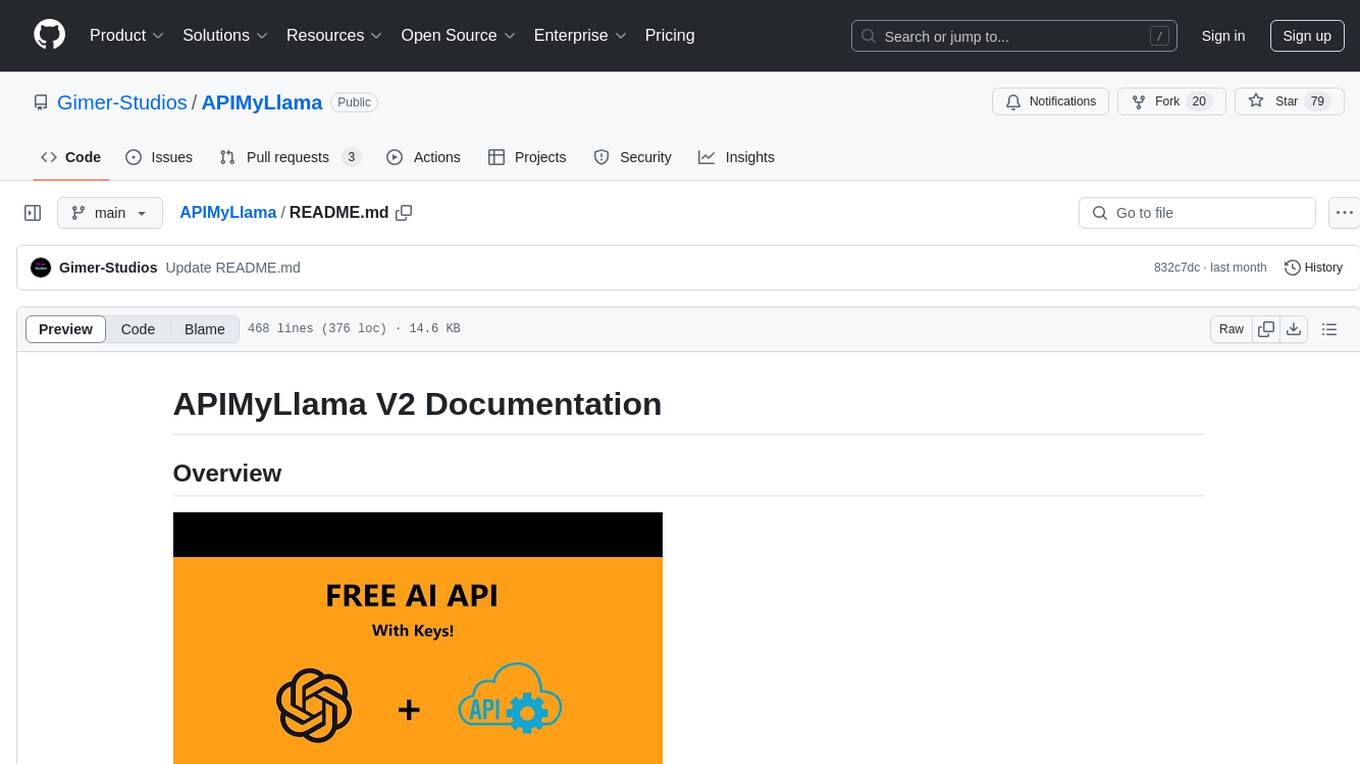
APIMyLlama
APIMyLlama is a server application that provides an interface to interact with the Ollama API, a powerful AI tool to run LLMs. It allows users to easily distribute API keys to create amazing things. The tool offers commands to generate, list, remove, add, change, activate, deactivate, and manage API keys, as well as functionalities to work with webhooks, set rate limits, and get detailed information about API keys. Users can install APIMyLlama packages with NPM, PIP, Jitpack Repo+Gradle or Maven, or from the Crates Repository. The tool supports Node.JS, Python, Java, and Rust for generating responses from the API. Additionally, it provides built-in health checking commands for monitoring API health status.
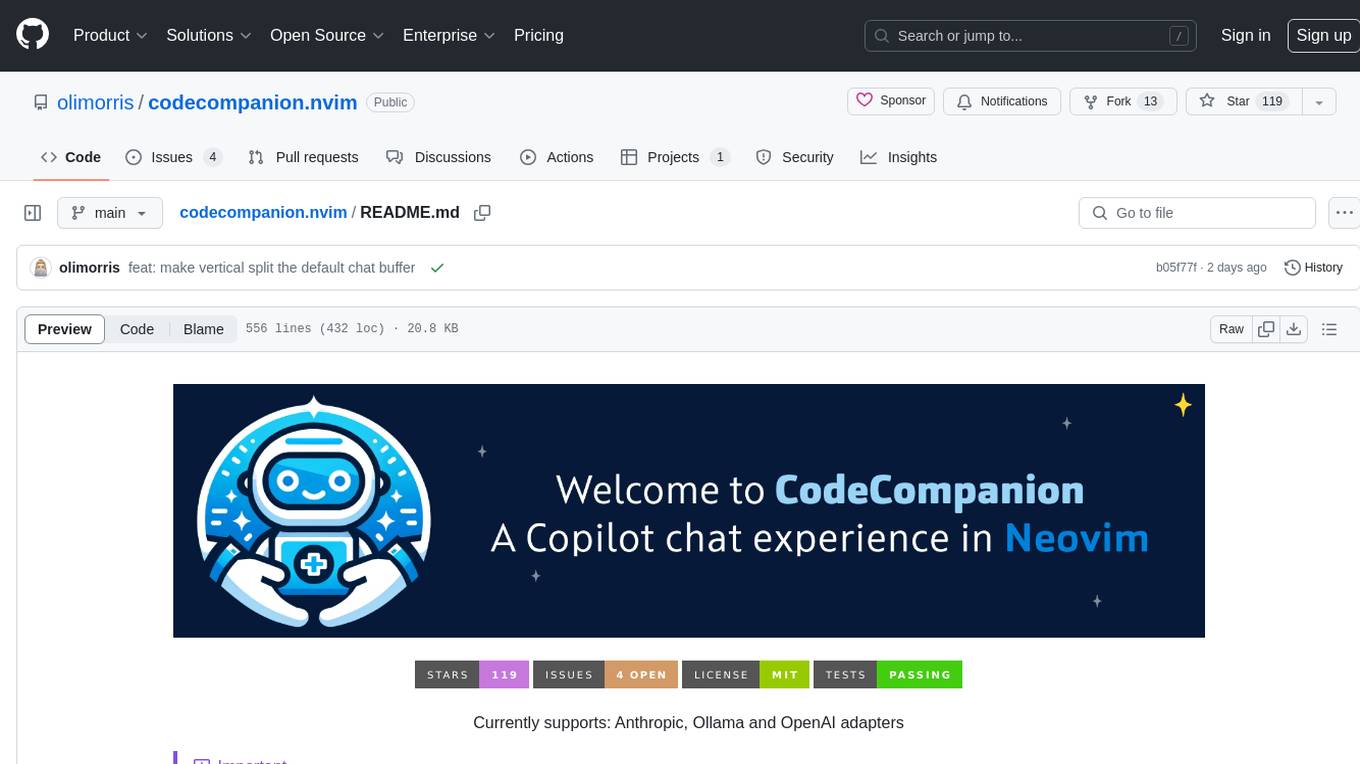
codecompanion.nvim
CodeCompanion.nvim is a Neovim plugin that provides a Copilot Chat experience, adapter support for various LLMs, agentic workflows, inline code creation and modification, built-in actions for language prompts and error fixes, custom actions creation, async execution, and more. It supports Anthropic, Ollama, and OpenAI adapters. The plugin is primarily developed for personal workflows with no guarantees of regular updates or support. Users can customize the plugin to their needs by forking the project.
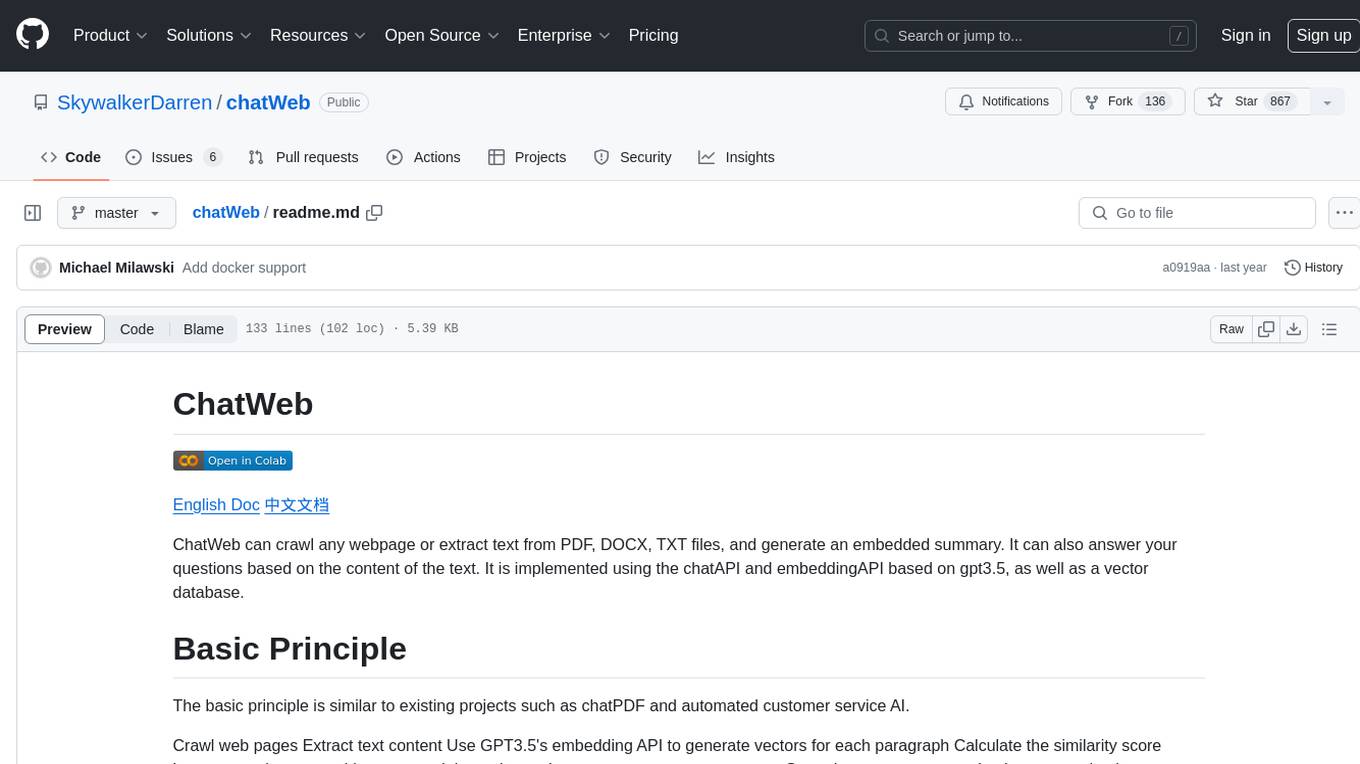
chatWeb
ChatWeb is a tool that can crawl web pages, extract text from PDF, DOCX, TXT files, and generate an embedded summary. It can answer questions based on text content using chatAPI and embeddingAPI based on GPT3.5. The tool calculates similarity scores between text vectors to generate summaries, performs nearest neighbor searches, and designs prompts to answer user questions. It aims to extract relevant content from text and provide accurate search results based on keywords. ChatWeb supports various modes, languages, and settings, including temperature control and PostgreSQL integration.
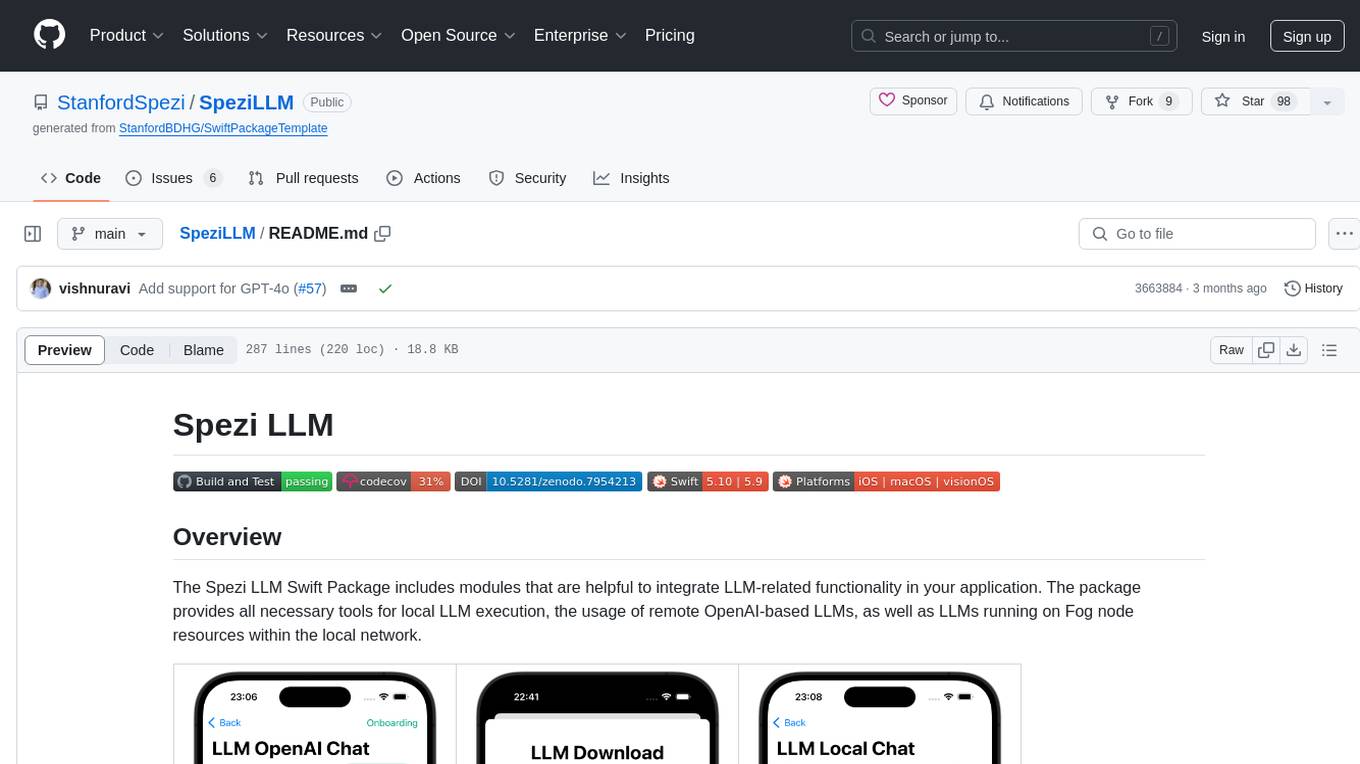
SpeziLLM
The Spezi LLM Swift Package includes modules that help integrate LLM-related functionality in applications. It provides tools for local LLM execution, usage of remote OpenAI-based LLMs, and LLMs running on Fog node resources within the local network. The package contains targets like SpeziLLM, SpeziLLMLocal, SpeziLLMLocalDownload, SpeziLLMOpenAI, and SpeziLLMFog for different LLM functionalities. Users can configure and interact with local LLMs, OpenAI LLMs, and Fog LLMs using the provided APIs and platforms within the Spezi ecosystem.
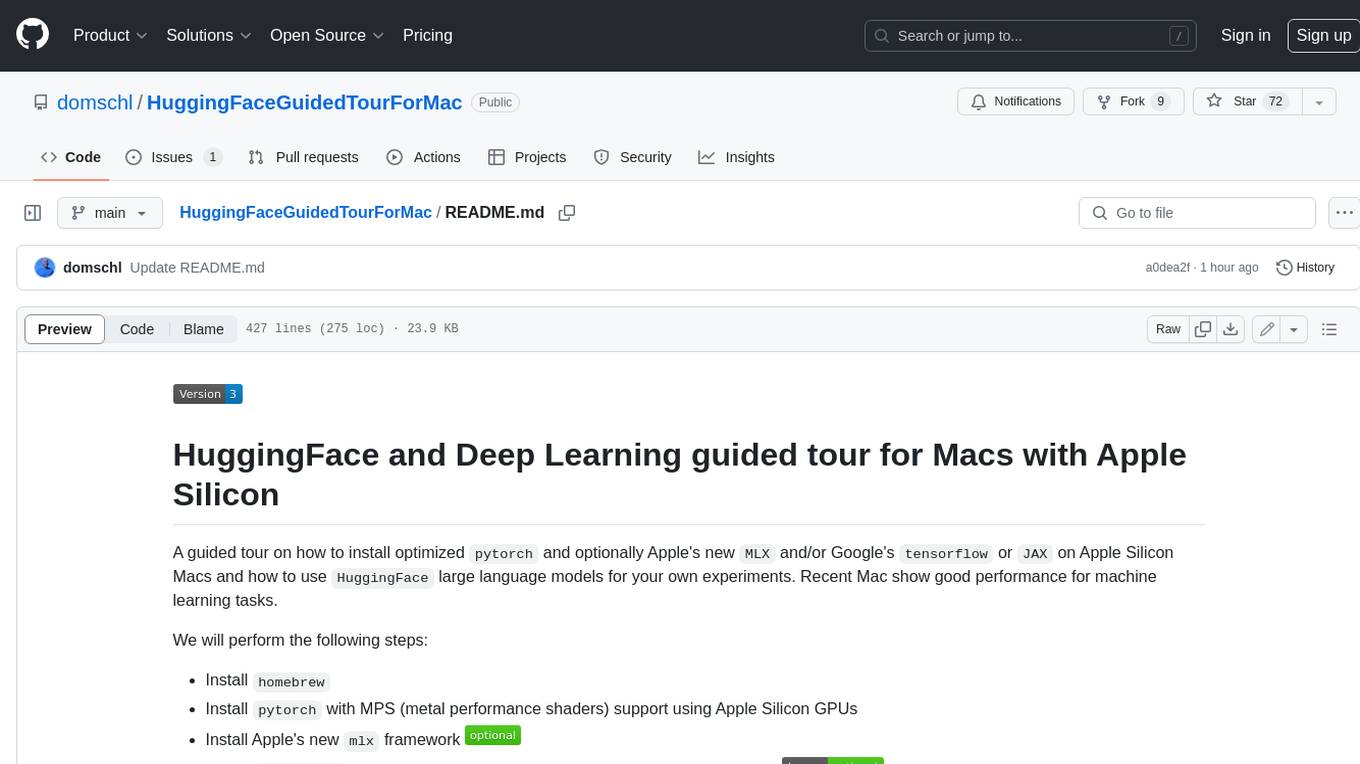
HuggingFaceGuidedTourForMac
HuggingFaceGuidedTourForMac is a guided tour on how to install optimized pytorch and optionally Apple's new MLX, JAX, and TensorFlow on Apple Silicon Macs. The repository provides steps to install homebrew, pytorch with MPS support, MLX, JAX, TensorFlow, and Jupyter lab. It also includes instructions on running large language models using HuggingFace transformers. The repository aims to help users set up their Macs for deep learning experiments with optimized performance.
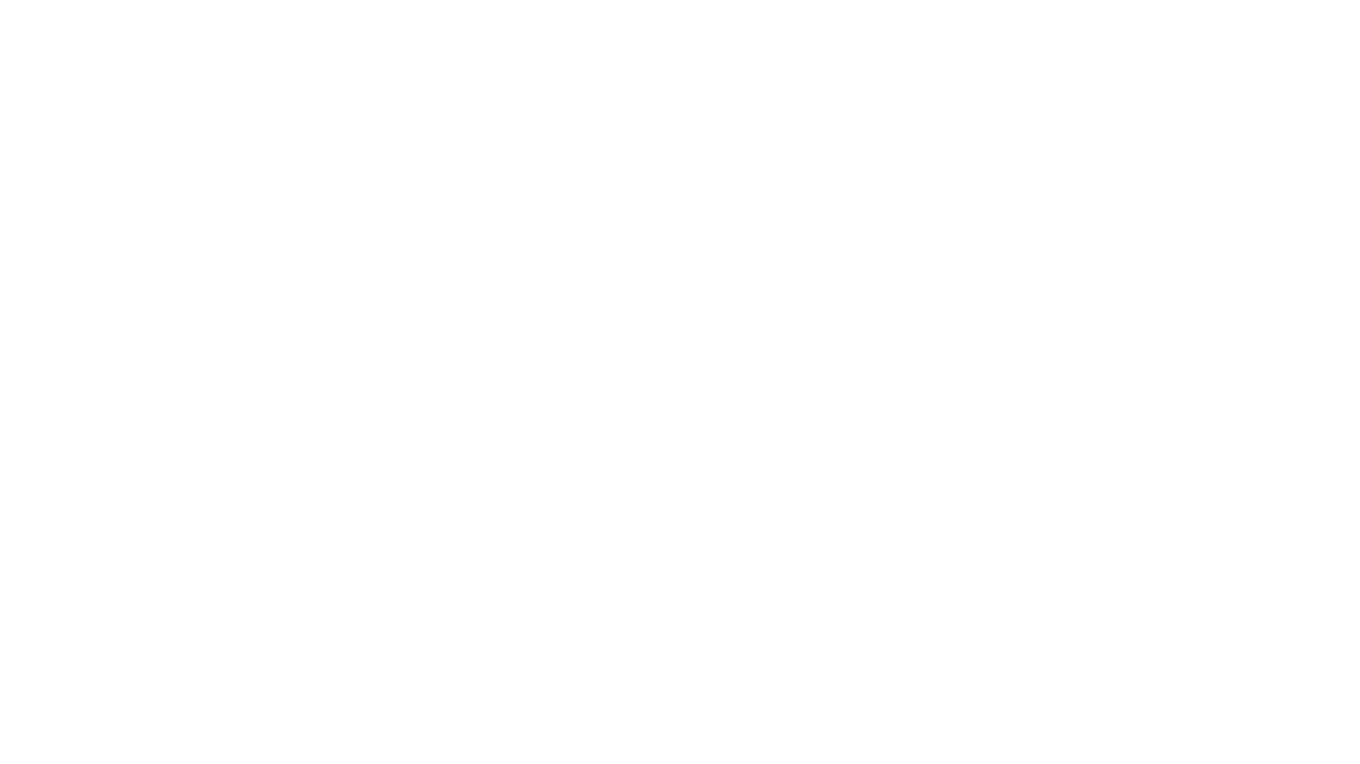
aiomisc
aiomisc is a Python library that provides a collection of utility functions and classes for working with asynchronous I/O in a more intuitive and efficient way. It offers features like worker pools, connection pools, circuit breaker pattern, and retry mechanisms to make asyncio code more robust and easier to maintain. The library simplifies the architecture of software using asynchronous I/O, making it easier for developers to write reliable and scalable asynchronous code.
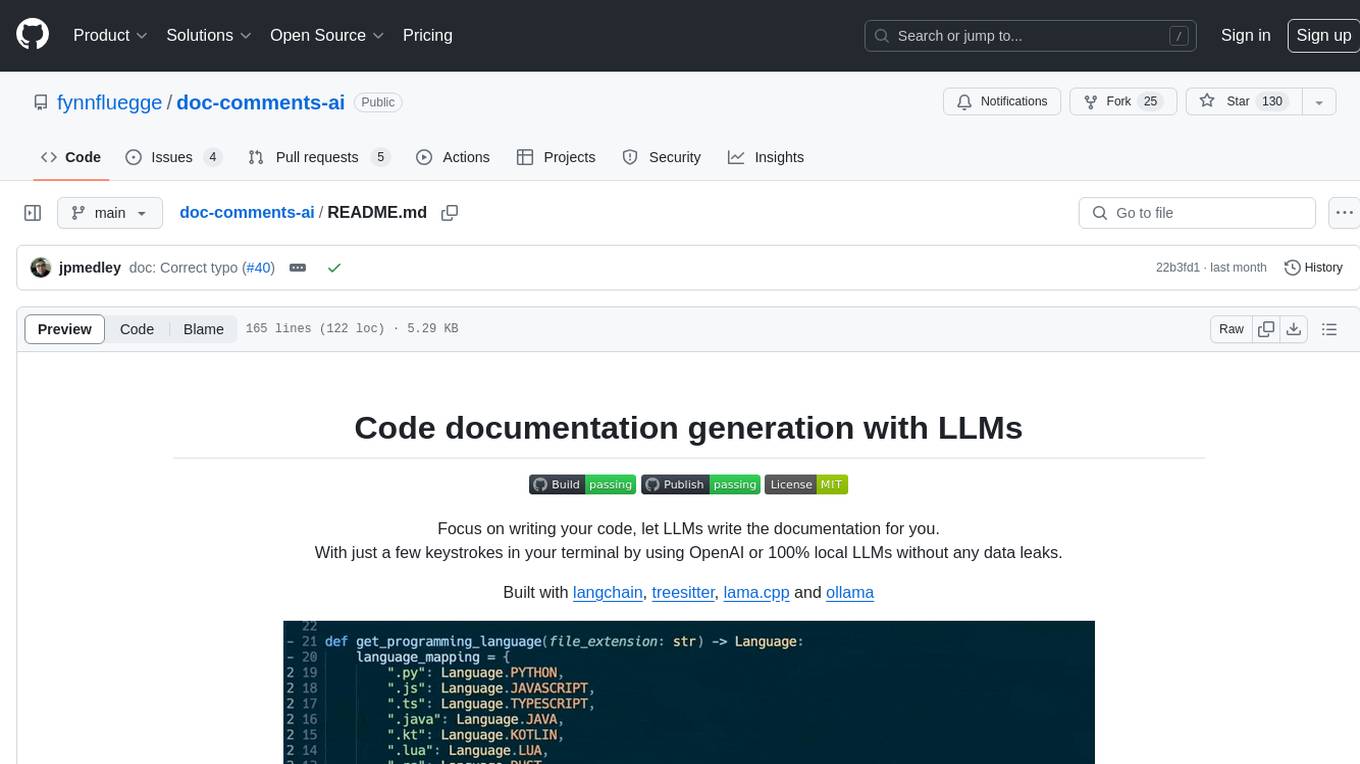
doc-comments-ai
doc-comments-ai is a tool designed to automatically generate code documentation using language models. It allows users to easily create documentation comment blocks for methods in various programming languages such as Python, Typescript, Javascript, Java, Rust, and more. The tool supports both OpenAI and local LLMs, ensuring data privacy and security. Users can generate documentation comments for methods in files, inline comments in method bodies, and choose from different models like GPT-3.5-Turbo, GPT-4, and Azure OpenAI. Additionally, the tool provides support for Treesitter integration and offers guidance on selecting the appropriate model for comprehensive documentation needs.
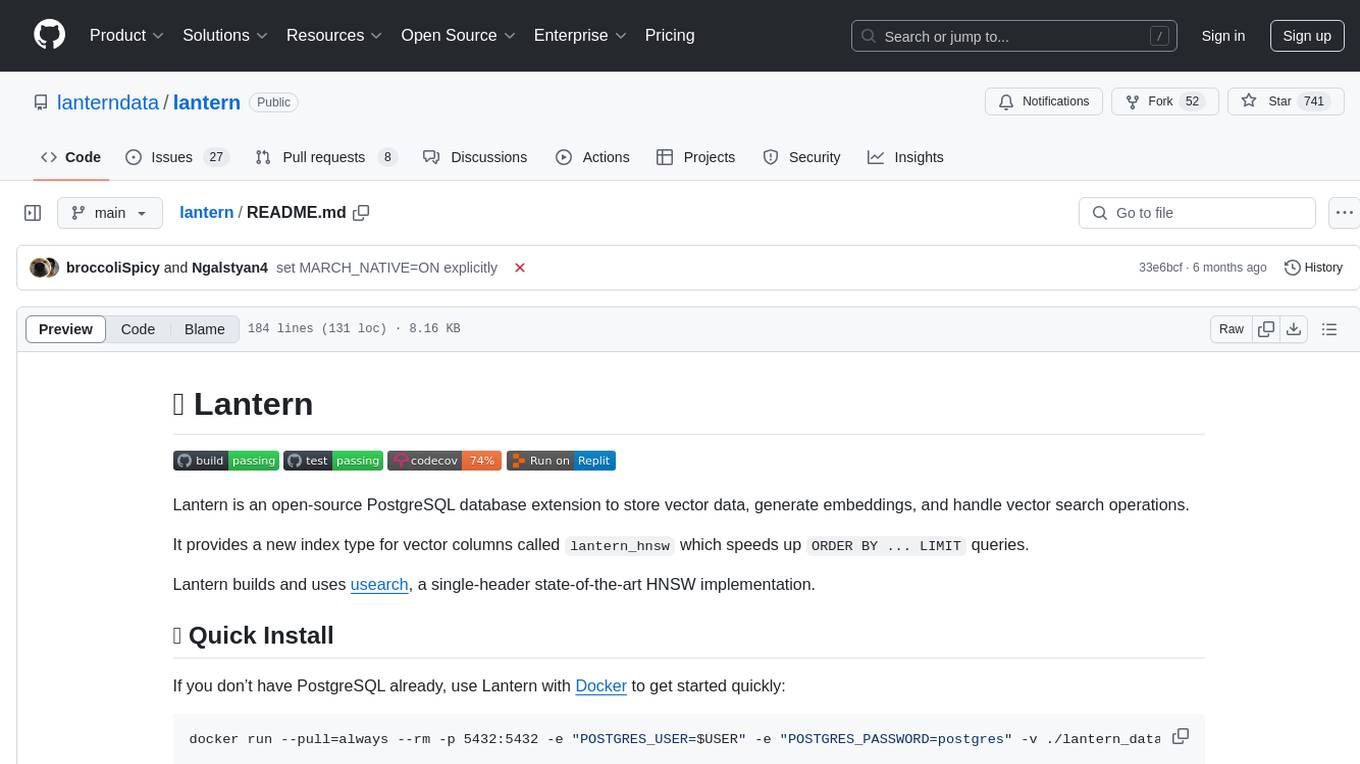
lantern
Lantern is an open-source PostgreSQL database extension designed to store vector data, generate embeddings, and handle vector search operations efficiently. It introduces a new index type called 'lantern_hnsw' for vector columns, which speeds up 'ORDER BY ... LIMIT' queries. Lantern utilizes the state-of-the-art HNSW implementation called usearch. Users can easily install Lantern using Docker, Homebrew, or precompiled binaries. The tool supports various distance functions, index construction parameters, and operator classes for efficient querying. Lantern offers features like embedding generation, interoperability with pgvector, parallel index creation, and external index graph generation. It aims to provide superior performance metrics compared to other similar tools and has a roadmap for future enhancements such as cloud-hosted version, hardware-accelerated distance metrics, industry-specific application templates, and support for version control and A/B testing of embeddings.
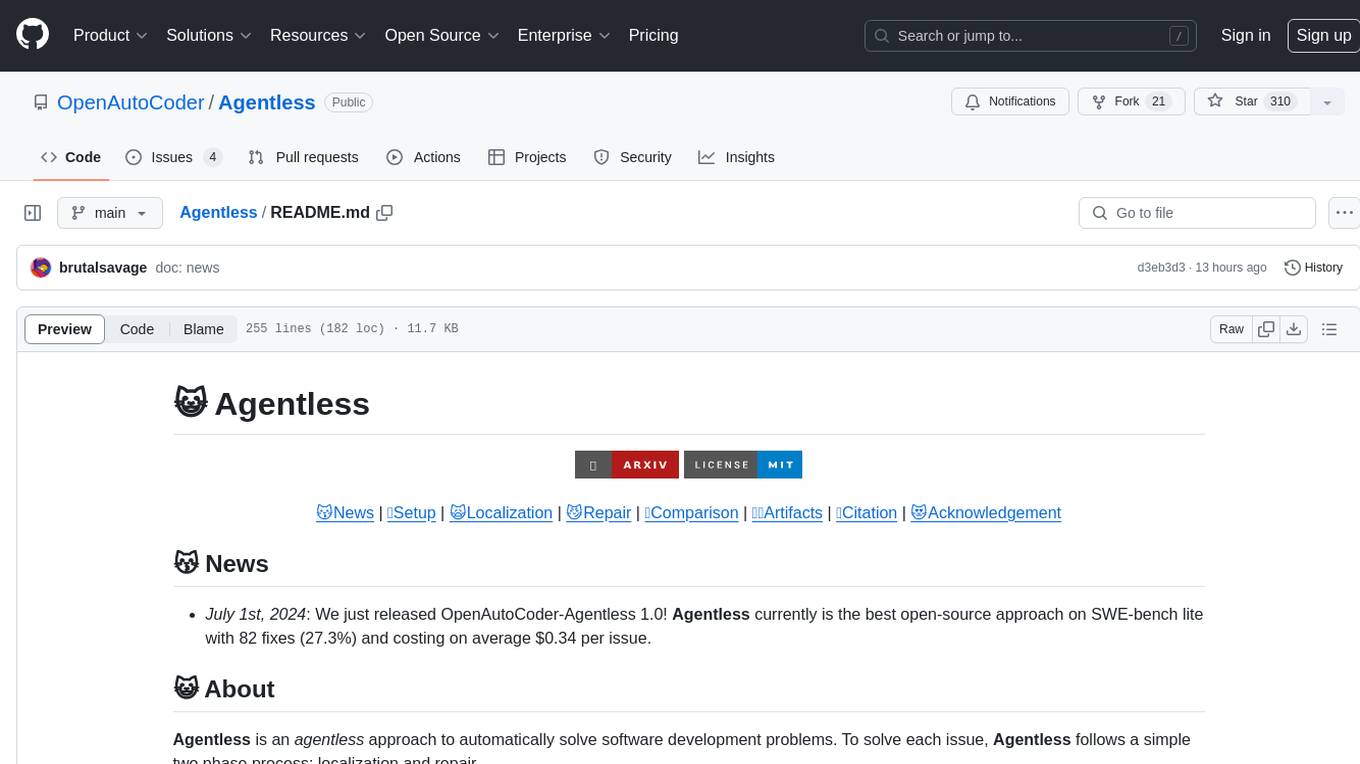
Agentless
Agentless is an open-source tool designed for automatically solving software development problems. It follows a two-phase process of localization and repair to identify faults in specific files, classes, and functions, and generate candidate patches for fixing issues. The tool is aimed at simplifying the software development process by automating issue resolution and patch generation.
For similar tasks
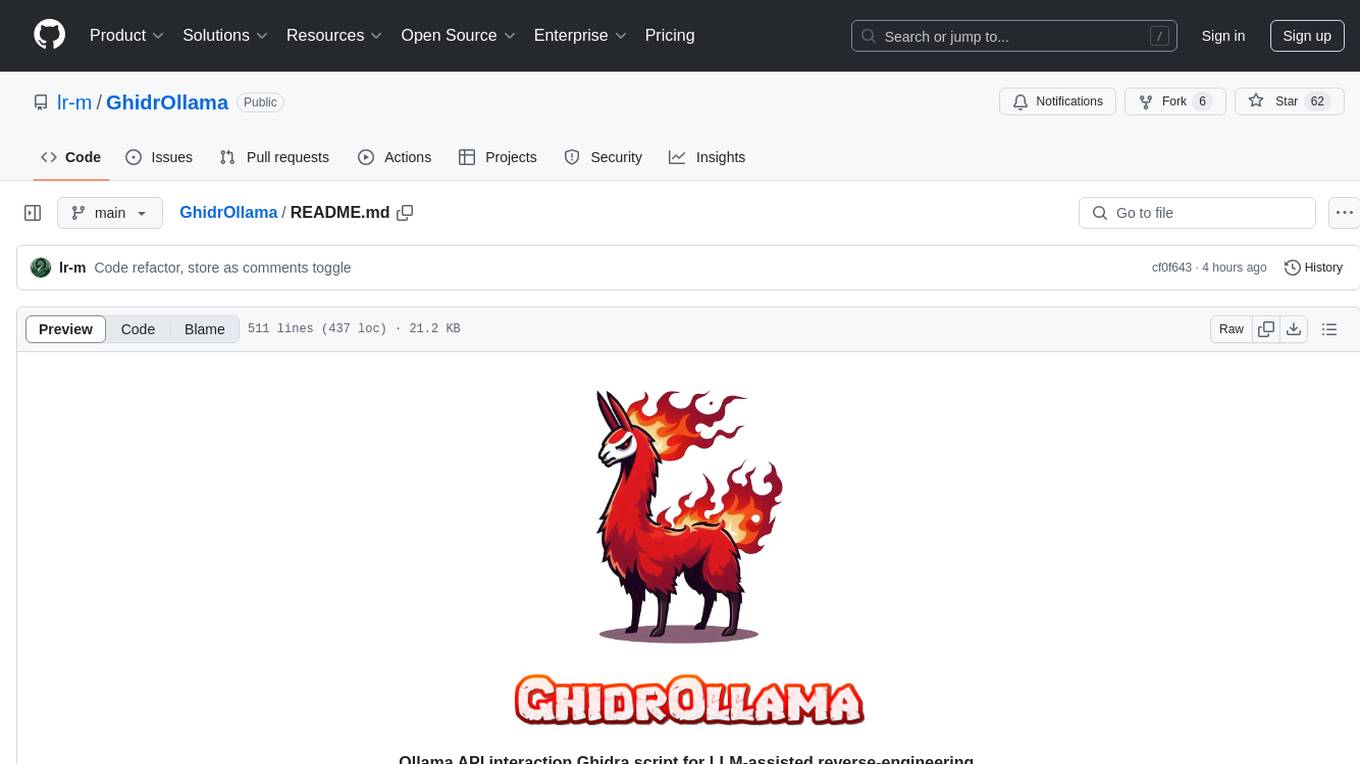
GhidrOllama
GhidrOllama is a script that interacts with Ollama's API to perform various reverse engineering tasks within Ghidra. It supports both local and remote instances of Ollama, providing functionalities like explaining functions, suggesting names, rewriting functions, finding bugs, and automating analysis of specific functions in binaries. Users can ask questions about functions, find vulnerabilities, and receive explanations of assembly instructions. The script bridges the gap between Ghidra and Ollama models, enhancing reverse engineering capabilities.
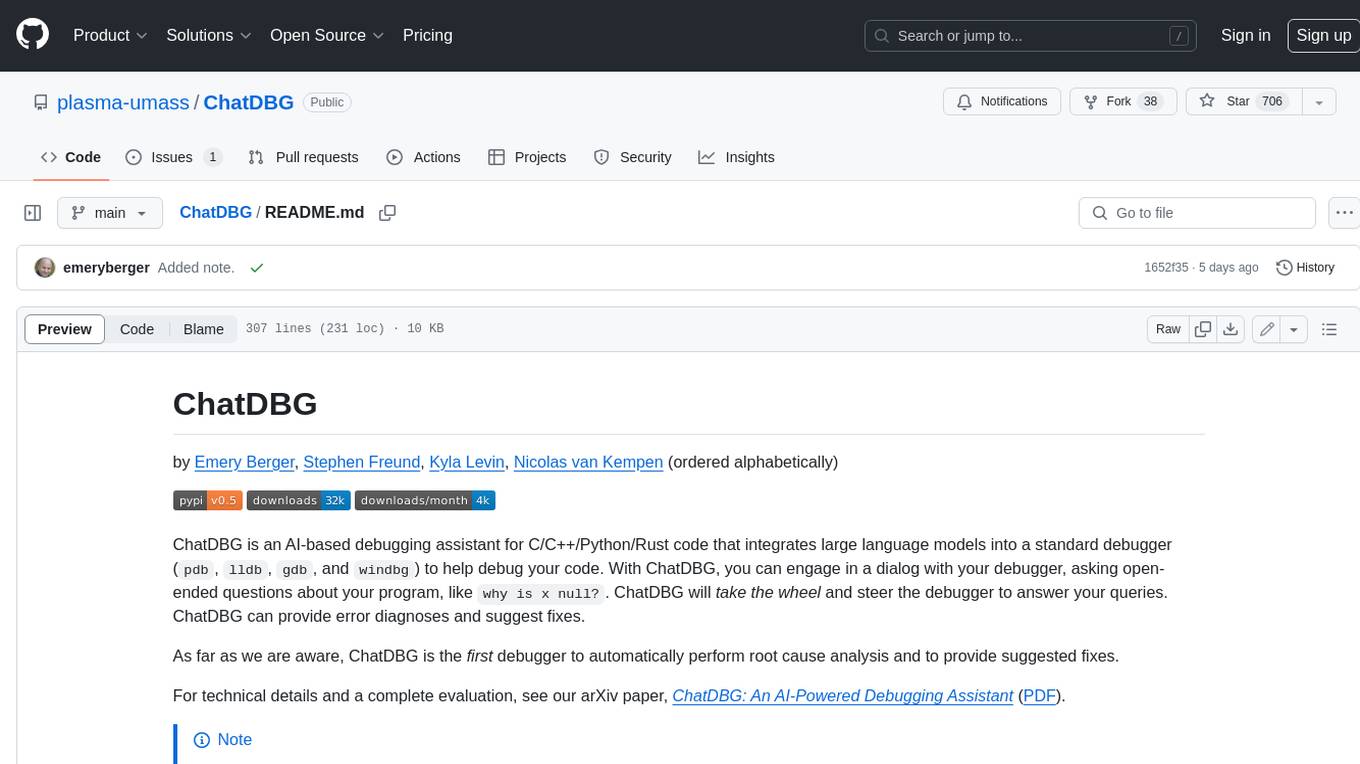
ChatDBG
ChatDBG is an AI-based debugging assistant for C/C++/Python/Rust code that integrates large language models into a standard debugger (`pdb`, `lldb`, `gdb`, and `windbg`) to help debug your code. With ChatDBG, you can engage in a dialog with your debugger, asking open-ended questions about your program, like `why is x null?`. ChatDBG will _take the wheel_ and steer the debugger to answer your queries. ChatDBG can provide error diagnoses and suggest fixes. As far as we are aware, ChatDBG is the _first_ debugger to automatically perform root cause analysis and to provide suggested fixes.
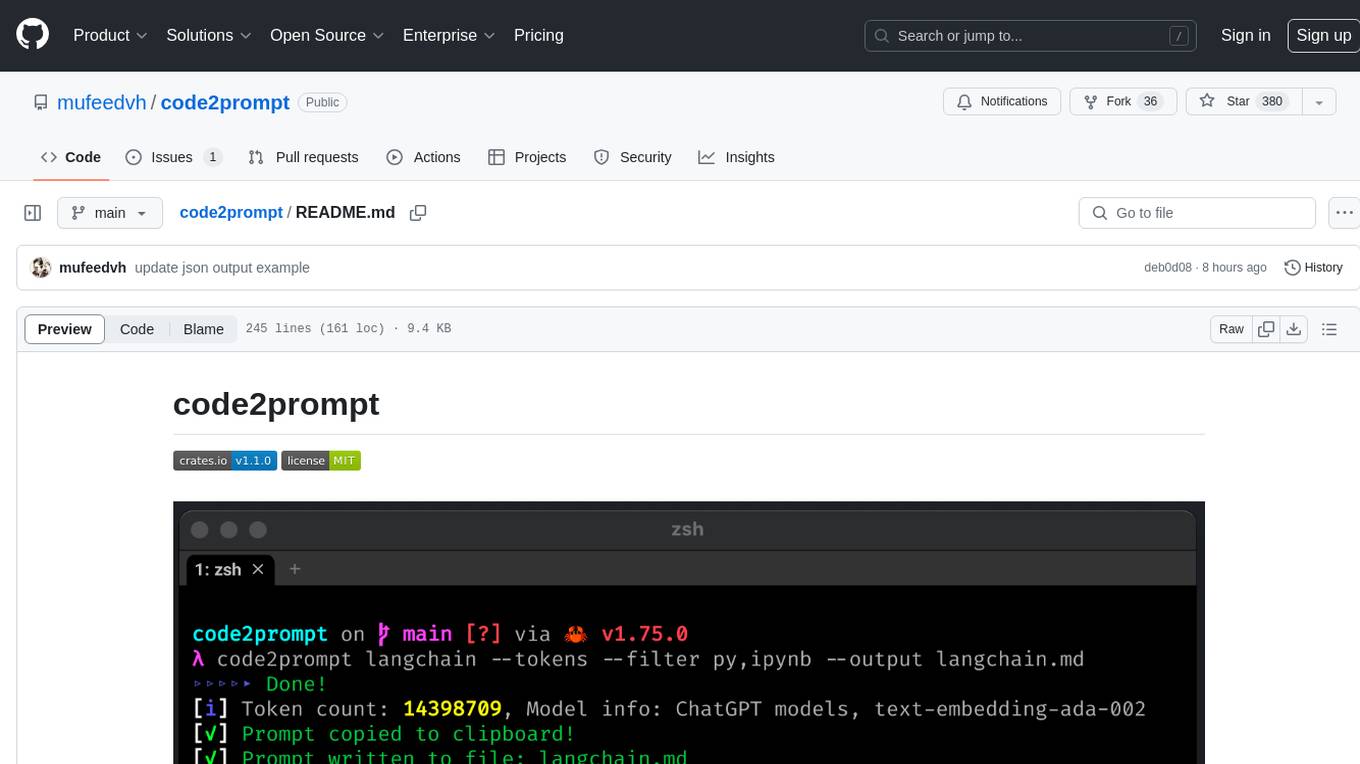
code2prompt
code2prompt is a command-line tool that converts your codebase into a single LLM prompt with a source tree, prompt templating, and token counting. It automates generating LLM prompts from codebases of any size, customizing prompt generation with Handlebars templates, respecting .gitignore, filtering and excluding files using glob patterns, displaying token count, including Git diff output, copying prompt to clipboard, saving prompt to an output file, excluding files and folders, adding line numbers to source code blocks, and more. It helps streamline the process of creating LLM prompts for code analysis, generation, and other tasks.
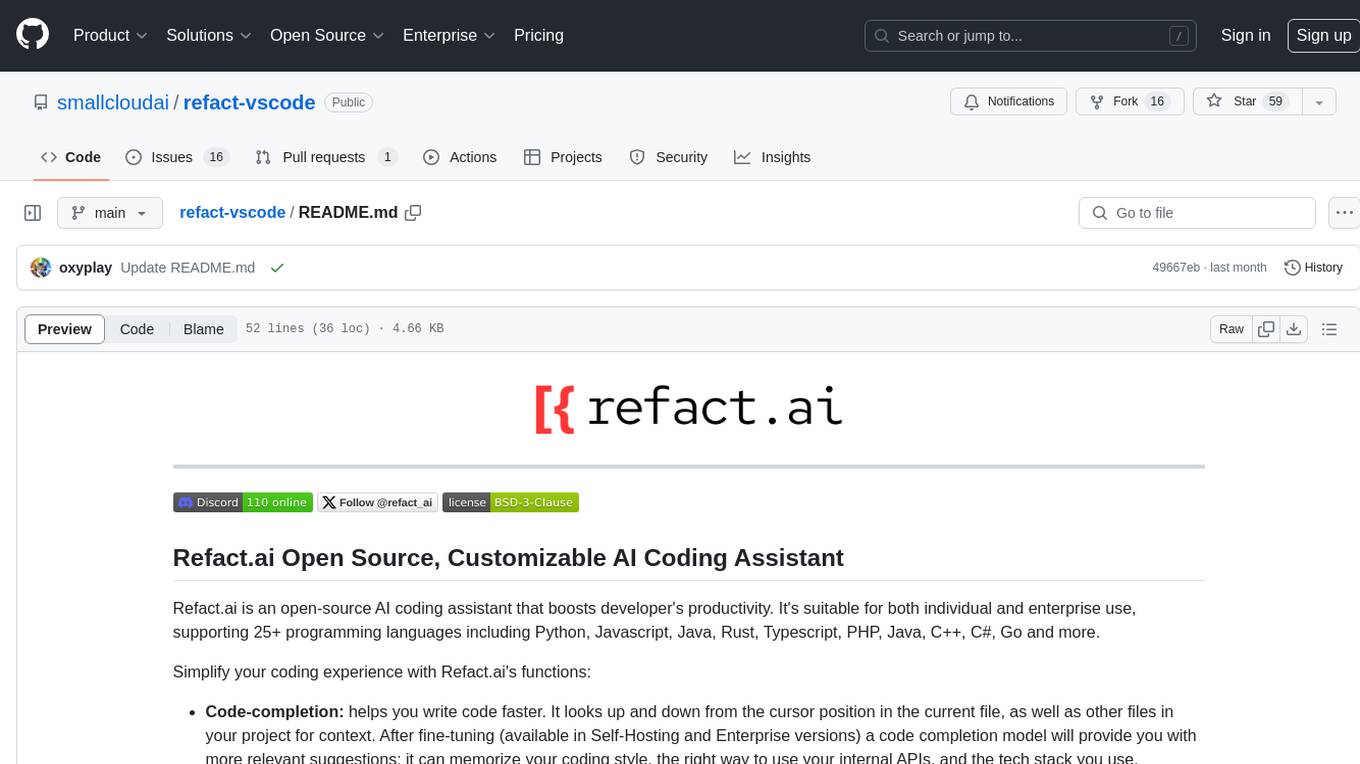
refact-vscode
Refact.ai is an open-source AI coding assistant that boosts developer's productivity. It supports 25+ programming languages and offers features like code completion, AI Toolbox for code explanation and refactoring, integrated in-IDE chat, and self-hosting or cloud version. The Enterprise plan provides enhanced customization, security, fine-tuning, user statistics, efficient inference, priority support, and access to 20+ LLMs for up to 50 engineers per GPU.
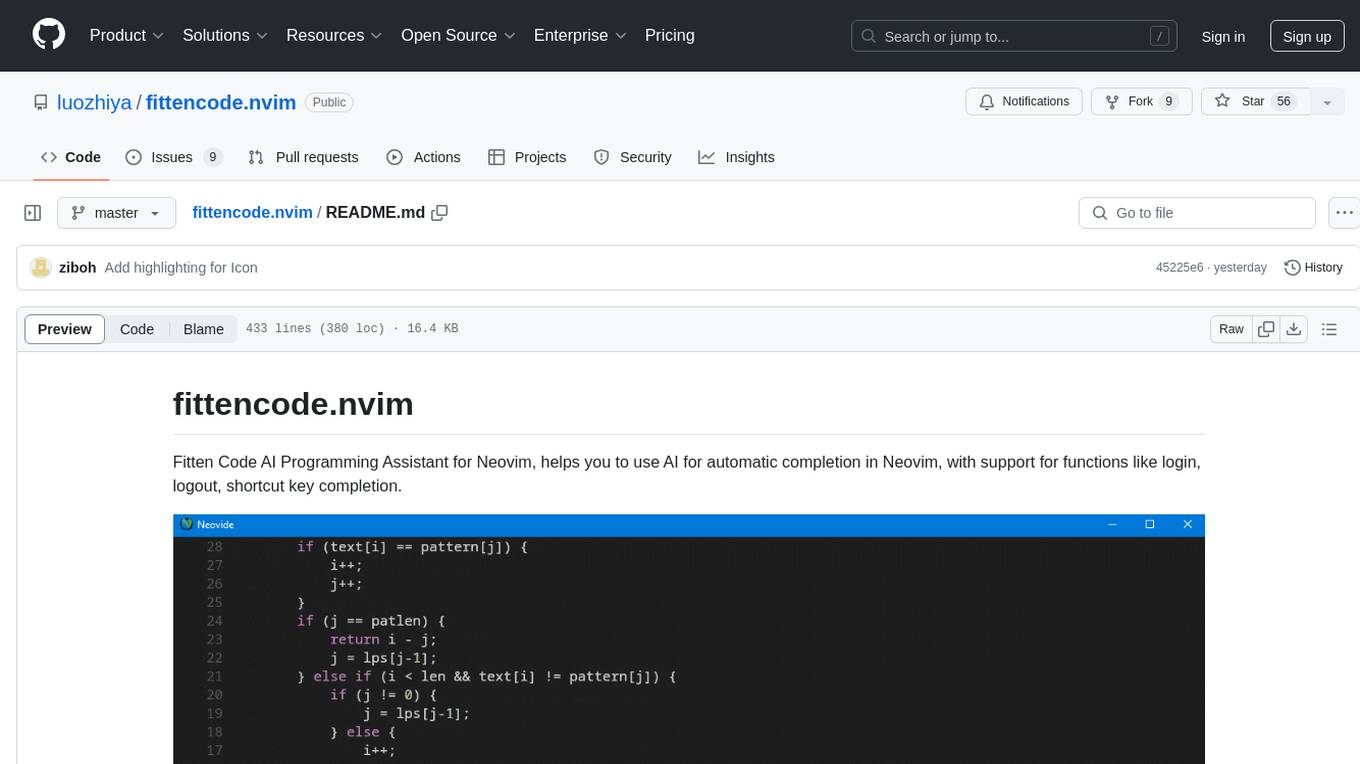
fittencode.nvim
Fitten Code AI Programming Assistant for Neovim provides fast completion using AI, asynchronous I/O, and support for various actions like document code, edit code, explain code, find bugs, generate unit test, implement features, optimize code, refactor code, start chat, and more. It offers features like accepting suggestions with Tab, accepting line with Ctrl + Down, accepting word with Ctrl + Right, undoing accepted text, automatic scrolling, and multiple HTTP/REST backends. It can run as a coc.nvim source or nvim-cmp source.
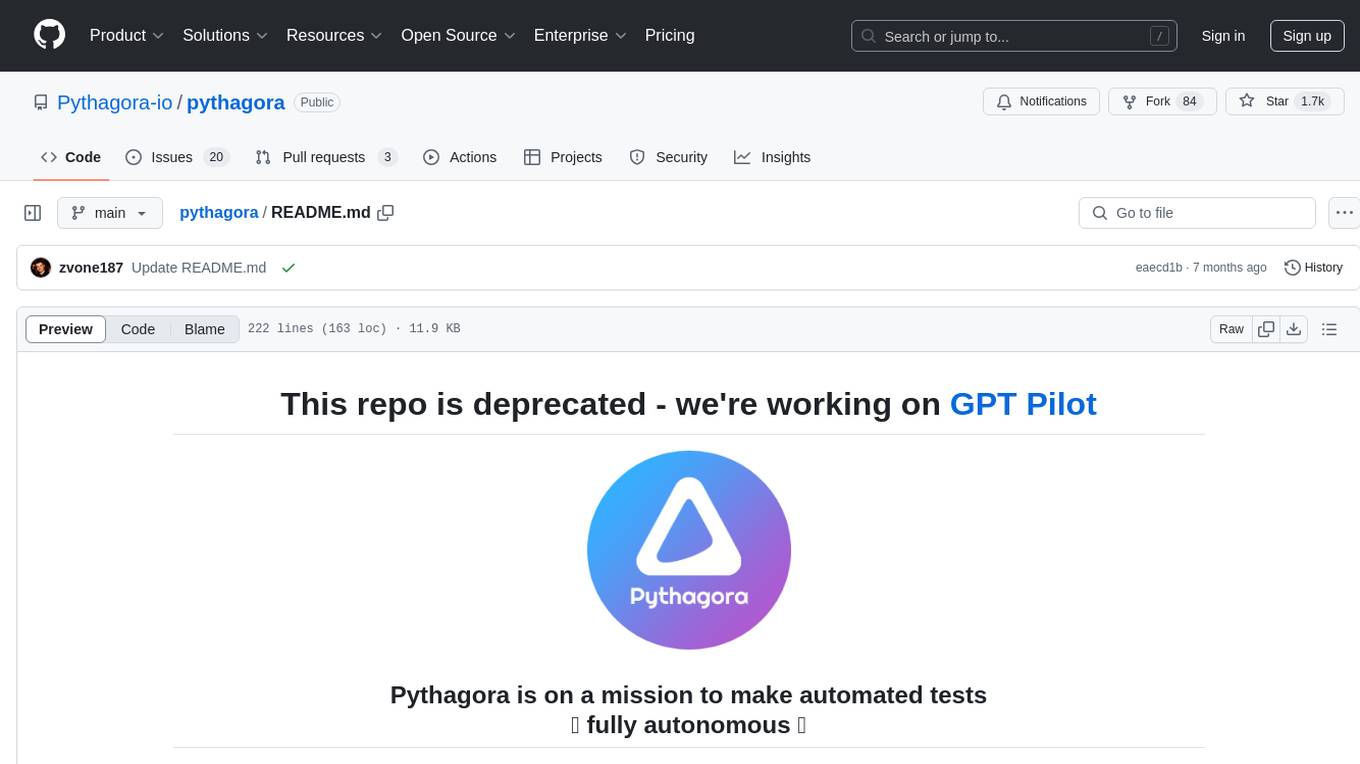
pythagora
Pythagora is an automated testing tool designed to generate unit tests using GPT-4. By running a single command, users can create tests for specific functions in their codebase. The tool leverages AST parsing to identify related functions and sends them to the Pythagora server for test generation. Pythagora primarily focuses on JavaScript code and supports Jest testing framework. Users can expand existing tests, increase code coverage, and find bugs efficiently. It is recommended to review the generated tests before committing them to the repository. Pythagora does not store user code on its servers but sends it to GPT and OpenAI for test generation.
For similar jobs
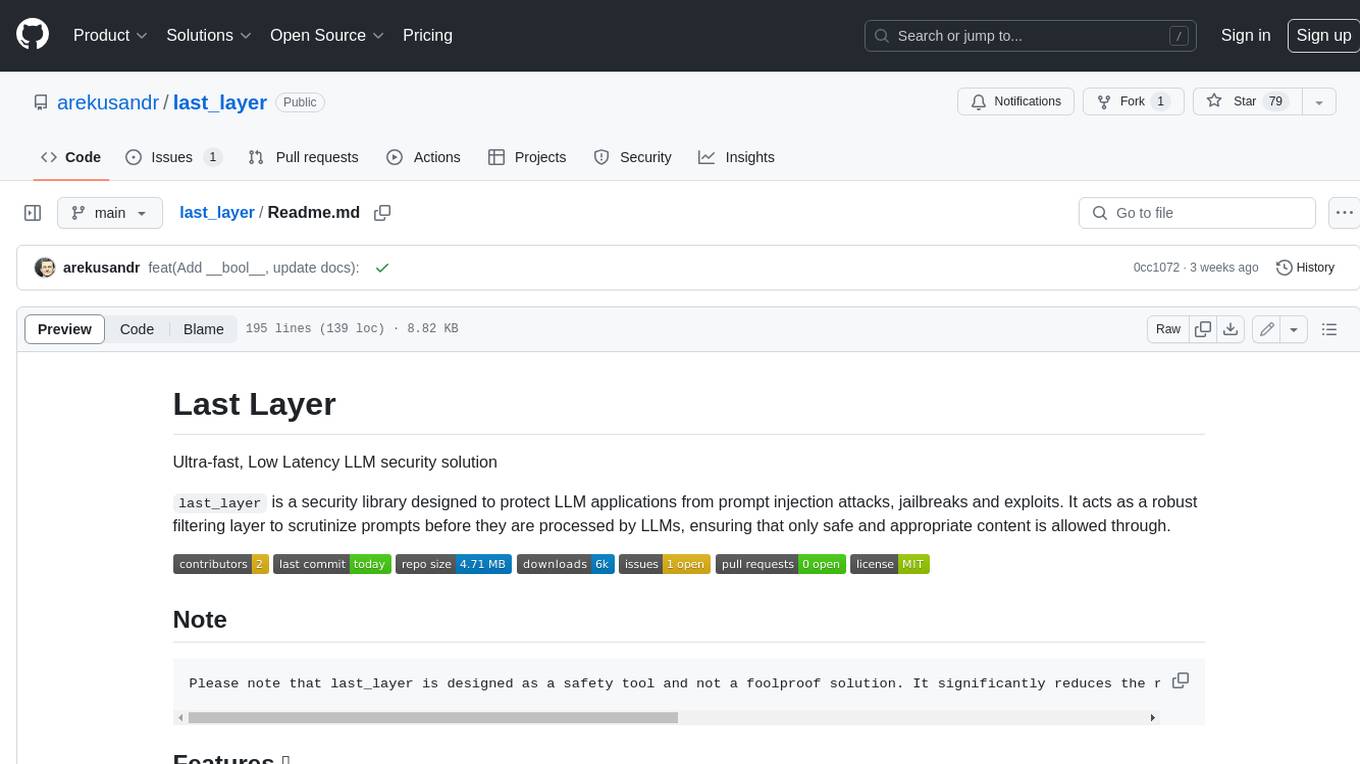
last_layer
last_layer is a security library designed to protect LLM applications from prompt injection attacks, jailbreaks, and exploits. It acts as a robust filtering layer to scrutinize prompts before they are processed by LLMs, ensuring that only safe and appropriate content is allowed through. The tool offers ultra-fast scanning with low latency, privacy-focused operation without tracking or network calls, compatibility with serverless platforms, advanced threat detection mechanisms, and regular updates to adapt to evolving security challenges. It significantly reduces the risk of prompt-based attacks and exploits but cannot guarantee complete protection against all possible threats.
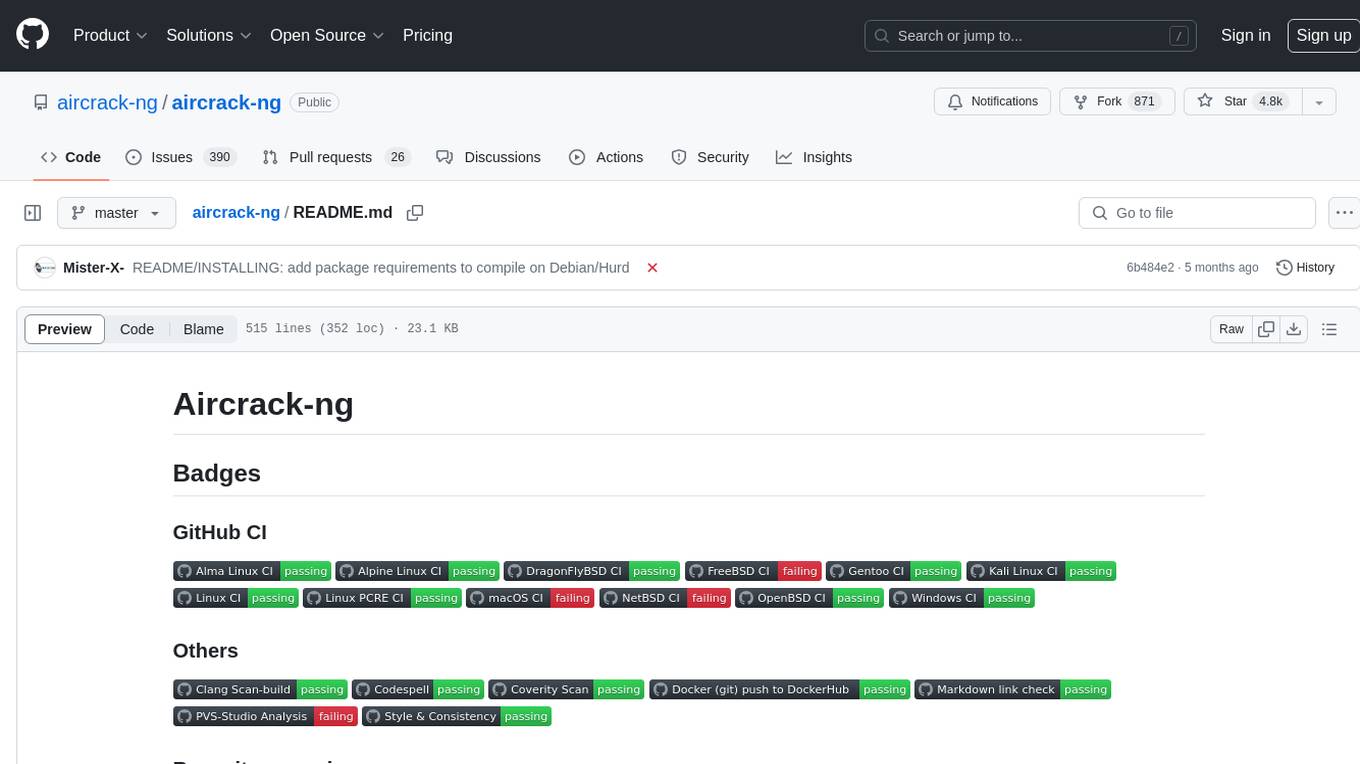
aircrack-ng
Aircrack-ng is a comprehensive suite of tools designed to evaluate the security of WiFi networks. It covers various aspects of WiFi security, including monitoring, attacking (replay attacks, deauthentication, fake access points), testing WiFi cards and driver capabilities, and cracking WEP and WPA PSK. The tools are command line-based, allowing for extensive scripting and have been utilized by many GUIs. Aircrack-ng primarily works on Linux but also supports Windows, macOS, FreeBSD, OpenBSD, NetBSD, Solaris, and eComStation 2.
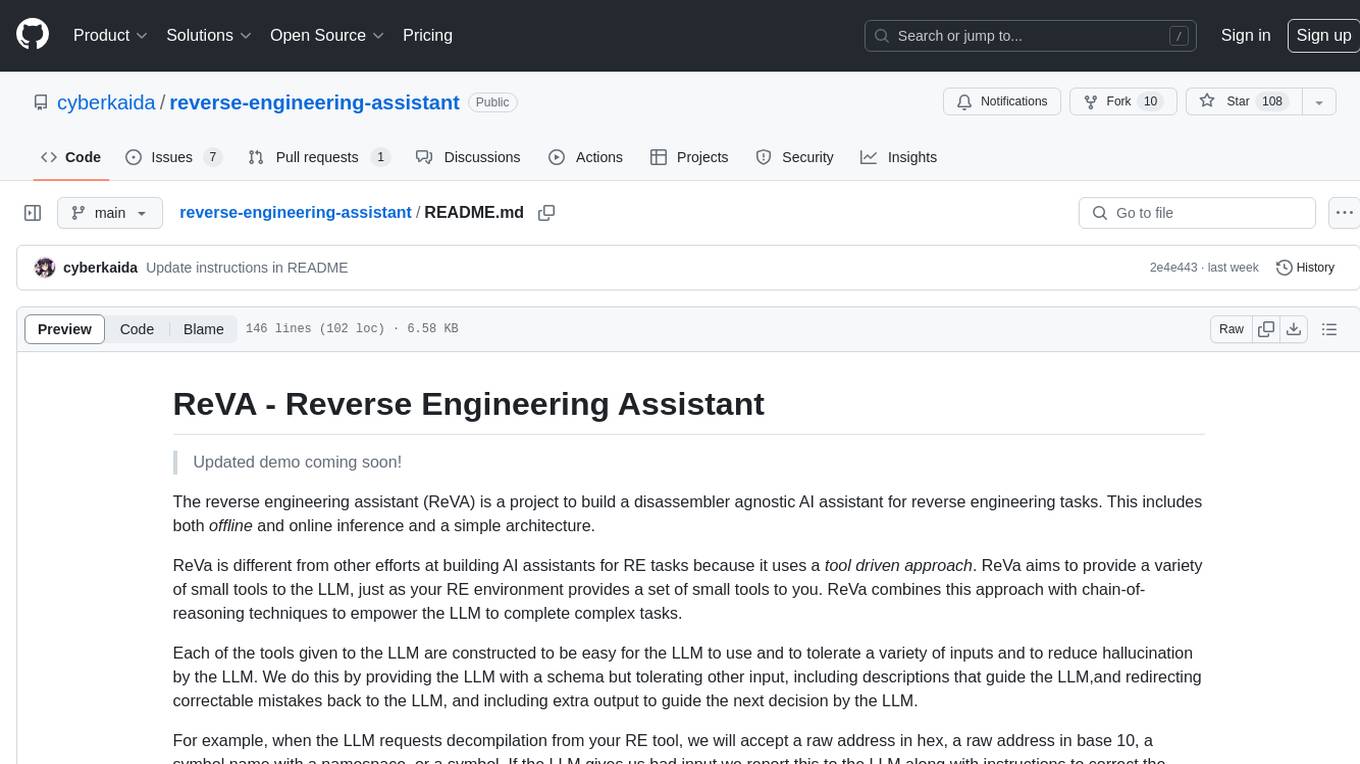
reverse-engineering-assistant
ReVA (Reverse Engineering Assistant) is a project aimed at building a disassembler agnostic AI assistant for reverse engineering tasks. It utilizes a tool-driven approach, providing small tools to the user to empower them in completing complex tasks. The assistant is designed to accept various inputs, guide the user in correcting mistakes, and provide additional context to encourage exploration. Users can ask questions, perform tasks like decompilation, class diagram generation, variable renaming, and more. ReVA supports different language models for online and local inference, with easy configuration options. The workflow involves opening the RE tool and program, then starting a chat session to interact with the assistant. Installation includes setting up the Python component, running the chat tool, and configuring the Ghidra extension for seamless integration. ReVA aims to enhance the reverse engineering process by breaking down actions into small parts, including the user's thoughts in the output, and providing support for monitoring and adjusting prompts.
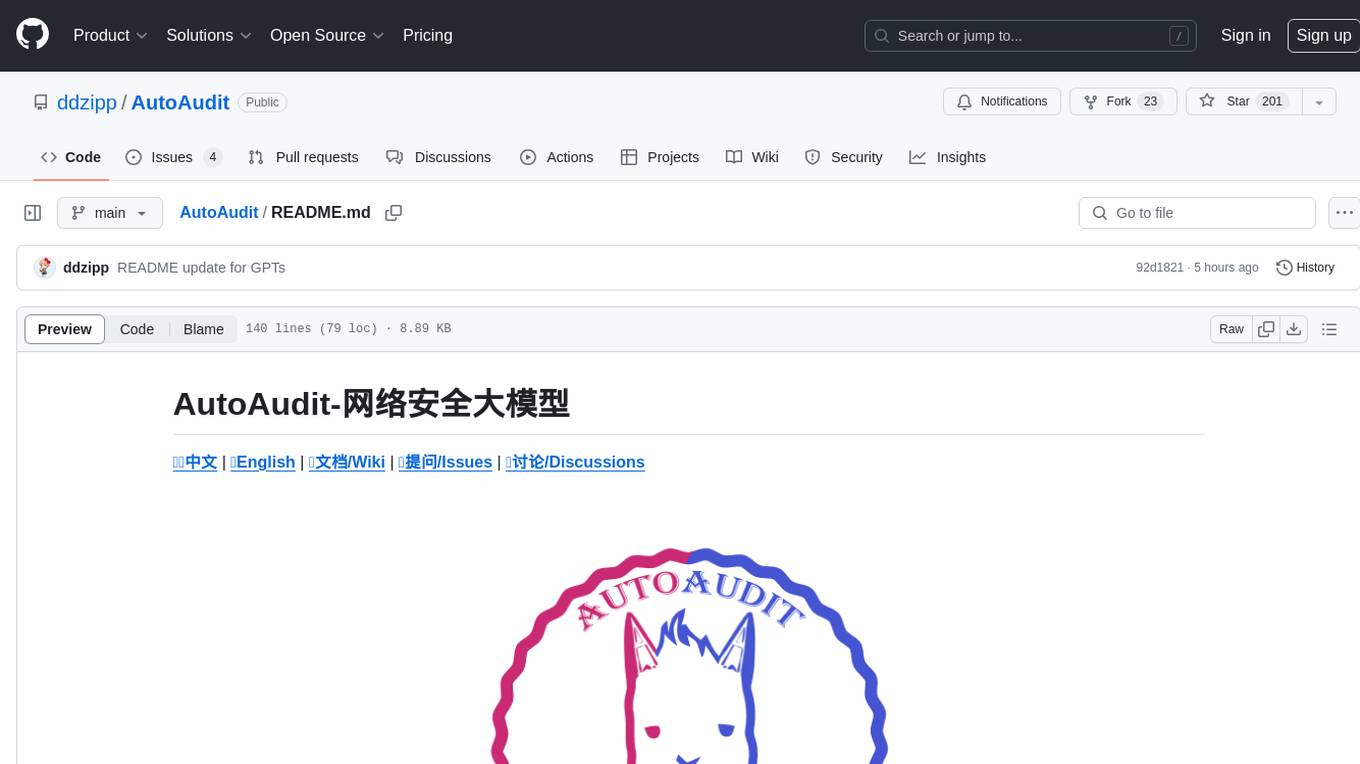
AutoAudit
AutoAudit is an open-source large language model specifically designed for the field of network security. It aims to provide powerful natural language processing capabilities for security auditing and network defense, including analyzing malicious code, detecting network attacks, and predicting security vulnerabilities. By coupling AutoAudit with ClamAV, a security scanning platform has been created for practical security audit applications. The tool is intended to assist security professionals with accurate and fast analysis and predictions to combat evolving network threats.
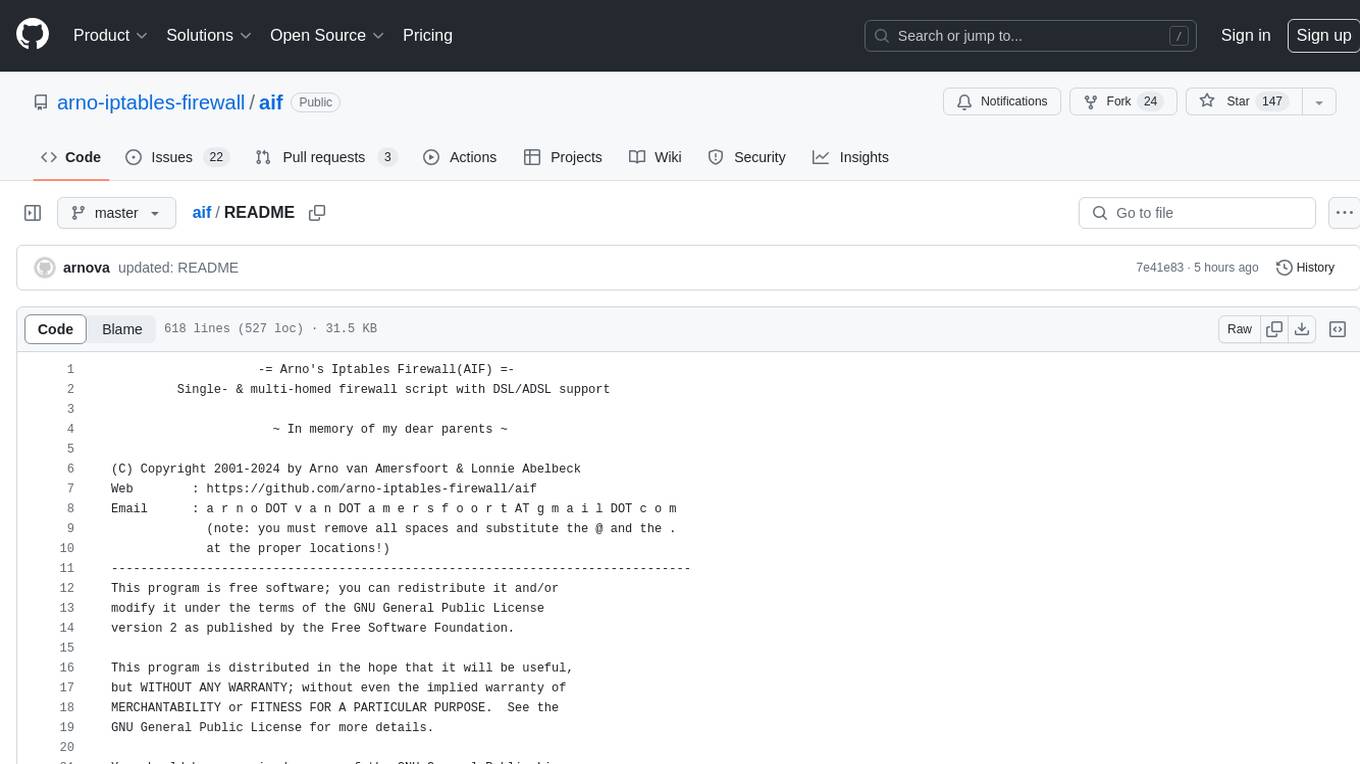
aif
Arno's Iptables Firewall (AIF) is a single- & multi-homed firewall script with DSL/ADSL support. It is a free software distributed under the GNU GPL License. The script provides a comprehensive set of configuration files and plugins for setting up and managing firewall rules, including support for NAT, load balancing, and multirouting. It offers detailed instructions for installation and configuration, emphasizing security best practices and caution when modifying settings. The script is designed to protect against hostile attacks by blocking all incoming traffic by default and allowing users to configure specific rules for open ports and network interfaces.
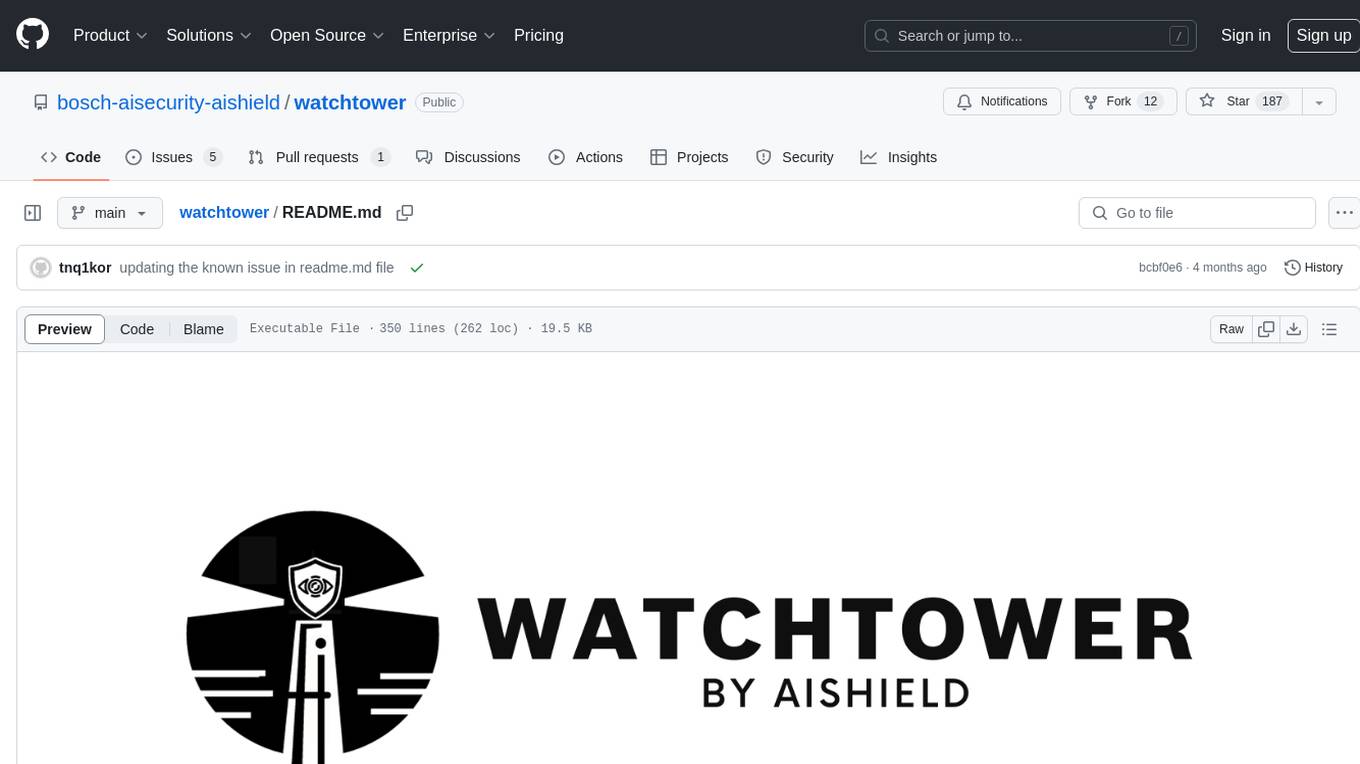
watchtower
AIShield Watchtower is a tool designed to fortify the security of AI/ML models and Jupyter notebooks by automating model and notebook discoveries, conducting vulnerability scans, and categorizing risks into 'low,' 'medium,' 'high,' and 'critical' levels. It supports scanning of public GitHub repositories, Hugging Face repositories, AWS S3 buckets, and local systems. The tool generates comprehensive reports, offers a user-friendly interface, and aligns with industry standards like OWASP, MITRE, and CWE. It aims to address the security blind spots surrounding Jupyter notebooks and AI models, providing organizations with a tailored approach to enhancing their security efforts.
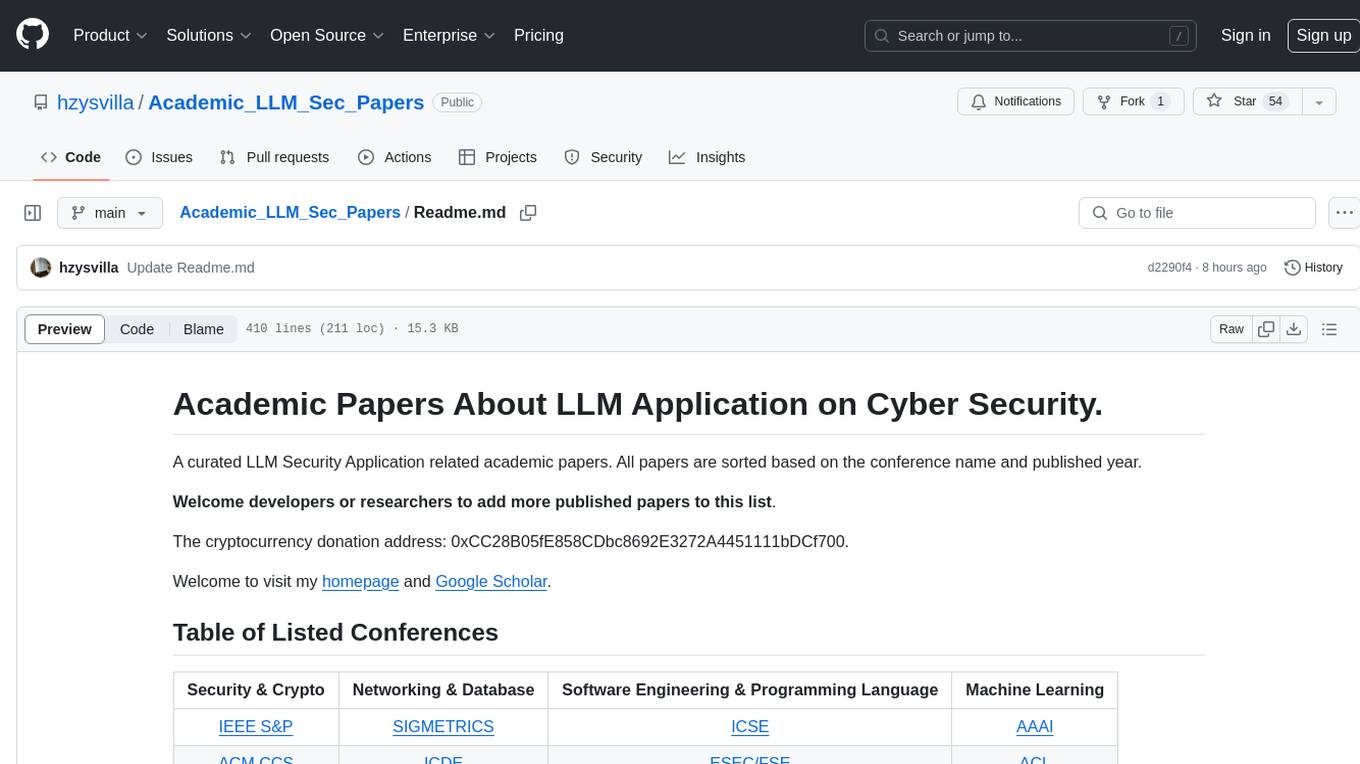
Academic_LLM_Sec_Papers
Academic_LLM_Sec_Papers is a curated collection of academic papers related to LLM Security Application. The repository includes papers sorted by conference name and published year, covering topics such as large language models for blockchain security, software engineering, machine learning, and more. Developers and researchers are welcome to contribute additional published papers to the list. The repository also provides information on listed conferences and journals related to security, networking, software engineering, and cryptography. The papers cover a wide range of topics including privacy risks, ethical concerns, vulnerabilities, threat modeling, code analysis, fuzzing, and more.
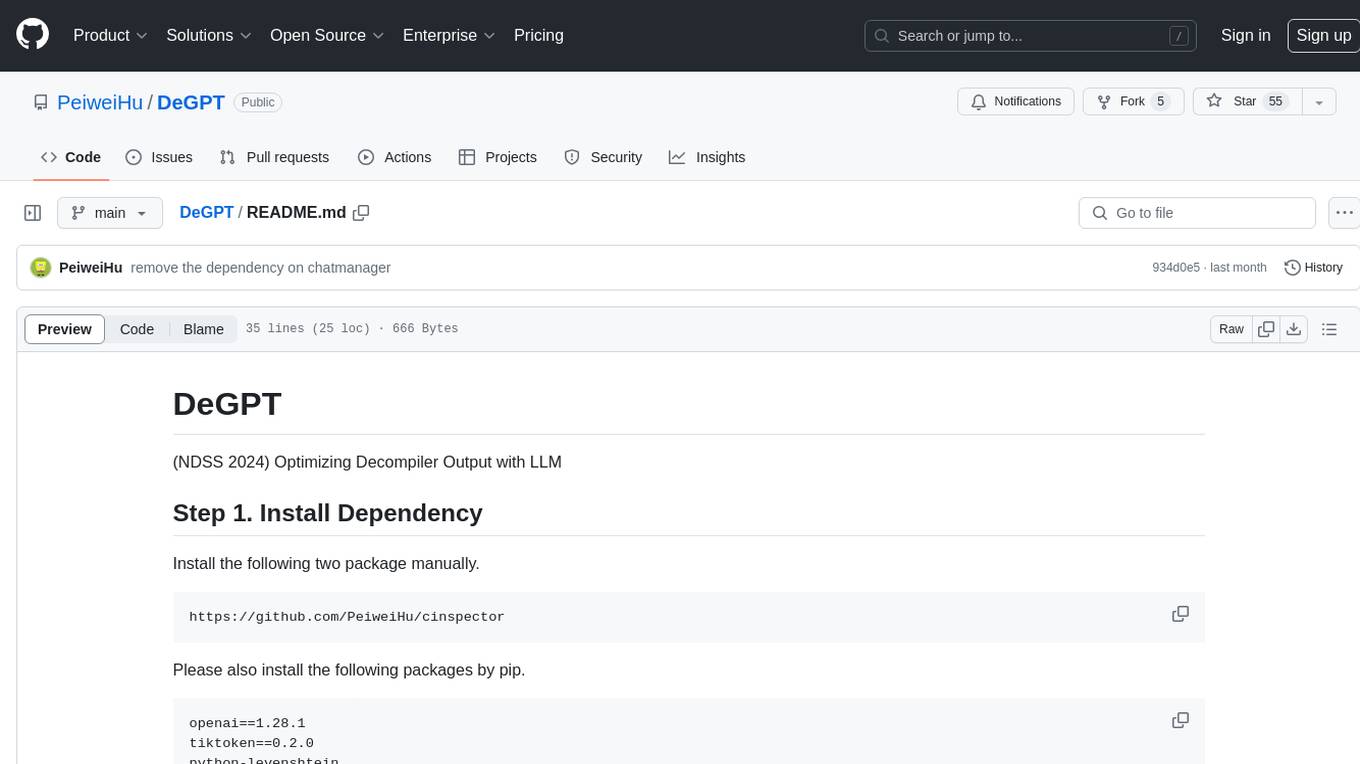
DeGPT
DeGPT is a tool designed to optimize decompiler output using Large Language Models (LLM). It requires manual installation of specific packages and setting up API key for OpenAI. The tool provides functionality to perform optimization on decompiler output by running specific scripts.