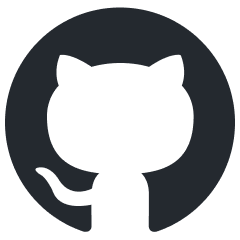
fastfit
FastFit ⚡ When LLMs are Unfit Use FastFit ⚡ Fast and Effective Text Classification with Many Classes
Stars: 183
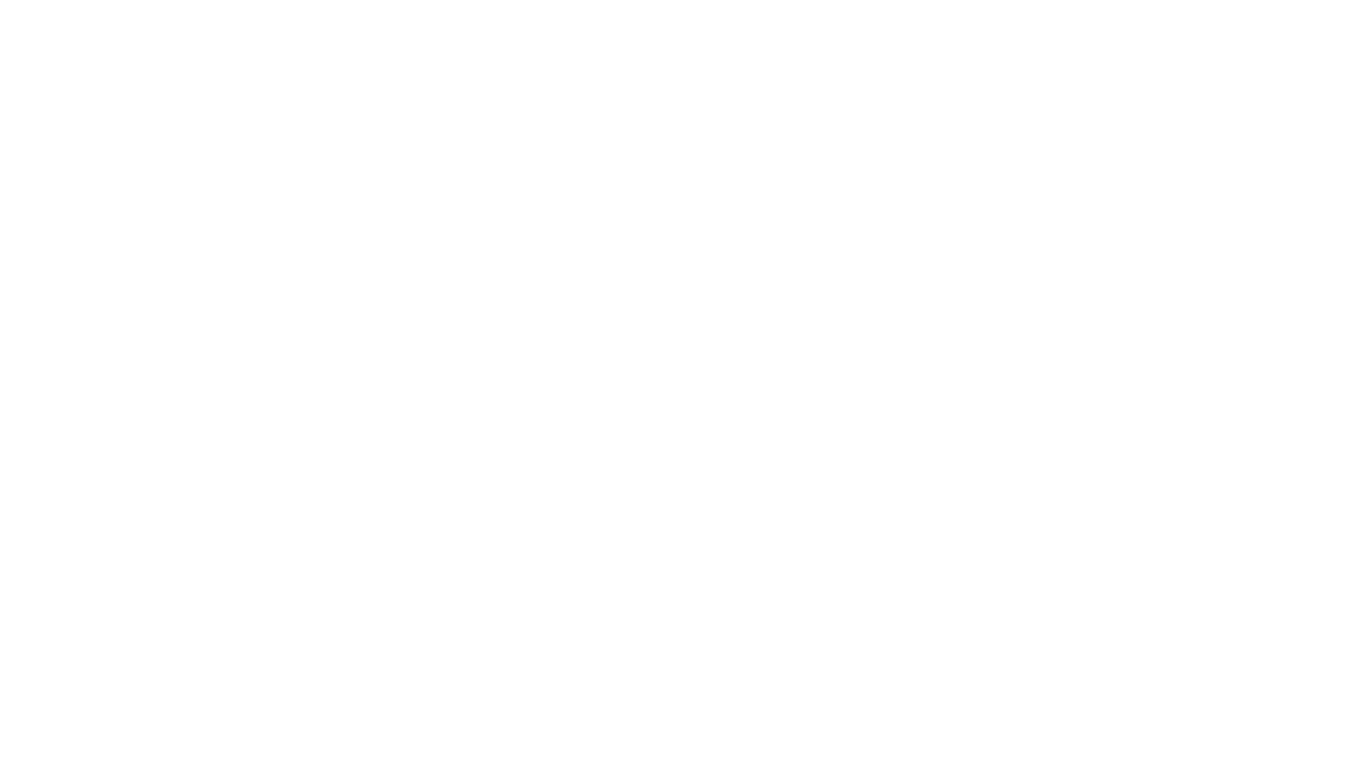
FastFit is a Python package designed for fast and accurate few-shot classification, especially for scenarios with many semantically similar classes. It utilizes a novel approach integrating batch contrastive learning and token-level similarity score, significantly improving multi-class classification performance in speed and accuracy across various datasets. FastFit provides a convenient command-line tool for training text classification models with customizable parameters. It offers a 3-20x improvement in training speed, completing training in just a few seconds. Users can also train models with Python scripts and perform inference using pretrained models for text classification tasks.
README:
FastFit, a method, and a Python package design to provide fast and accurate few-shot classification, especially for scenarios with many semantically similar classes. FastFit utilizes a novel approach integrating batch contrastive learning and token-level similarity score. Compared to existing few-shot learning packages, such as SetFit, Transformers, or few-shot prompting of large language models via API calls, FastFit significantly improves multi-class classification performance in speed and accuracy across FewMany, our newly curated English benchmark, and Multilingual datasets. FastFit demonstrates a 3-20x improvement in training speed, completing training in just a few seconds.
Our package provides a convenient command-line tool train_fastfit
to train text classification models. This tool comes with a variety of configurable parameters to customize your training process.
Before running the training script, ensure you have Python installed along with our package and its dependencies. If you haven't already installed our package, you can do so using pip:
pip install fast-fit
To run the training script with custom configurations, use the train_fastfit
command followed by the necessary arguments similar to huggingface training args with few additions relevant for fast-fit.
Here's an example of how to use the run_train
command with specific settings:
train_fastfit \
--model_name_or_path "sentence-transformers/paraphrase-mpnet-base-v2" \
--train_file $TRAIN_FILE \
--validation_file $VALIDATION_FILE \
--output_dir ./tmp/try \
--overwrite_output_dir \
--report_to none \
--label_column_name label\
--text_column_name text \
--num_train_epochs 40 \
--dataloader_drop_last true \
--per_device_train_batch_size 32 \
--per_device_eval_batch_size 64 \
--evaluation_strategy steps \
--max_text_length 128 \
--logging_steps 100 \
--dataloader_drop_last=False \
--num_repeats 4 \
--save_strategy no \
--optim adafactor \
--clf_loss_factor 0.1 \
--do_train \
--fp16 \
--projection_dim 128
Upon execution, train_fastfit
will start the training process based on your parameters and output the results, including logs and model checkpoints, to the designated directory.
You can simply run it with your python
from datasets import load_dataset
from fastfit import FastFitTrainer, sample_dataset
# Load a dataset from the Hugging Face Hub
dataset = load_dataset("FastFit/banking_77")
dataset["validation"] = dataset["test"]
# Down sample the train data for 5-shot training
dataset["train"] = sample_dataset(dataset["train"], label_column="label", num_samples_per_label=5)
trainer = FastFitTrainer(
model_name_or_path="sentence-transformers/paraphrase-mpnet-base-v2",
label_column_name="label",
text_column_name="text",
num_train_epochs=40,
per_device_train_batch_size=32,
per_device_eval_batch_size=64,
max_text_length=128,
dataloader_drop_last=False,
num_repeats=4,
optim="adafactor",
clf_loss_factor=0.1,
fp16=True,
dataset=dataset,
)
model = trainer.train()
results = trainer.evaluate()
print("Accuracy: {:.1f}".format(results["eval_accuracy"] * 100))
Then the model can be saved:
model.save_pretrained("fast-fit")
Then you can use the model for inference
from fastfit import FastFit
from transformers import AutoTokenizer, pipeline
model = FastFit.from_pretrained("fast-fit")
tokenizer = AutoTokenizer.from_pretrained("sentence-transformers/paraphrase-mpnet-base-v2")
classifier = pipeline("text-classification", model=model, tokenizer=tokenizer)
print(classifier("I love this package!"))
Optional Arguments:
-
-h, --help
: Show this help message and exit. -
--num_repeats NUM_REPEATS
: The number of times to repeat the queries and docs in every batch. (default: 1) -
--proj_dim PROJ_DIM
: The dimension of the projection layer. (default: 128) -
--clf_loss_factor CLF_LOSS_FACTOR
: The factor to scale the classification loss. (default: 0.1) -
--pretrain_mode [PRETRAIN_MODE]
: Whether to do pre-training. (default: False) -
--inference_type INFERENCE_TYPE
: The inference type to be used. (default: sim) -
--rep_tokens REP_TOKENS
: The tokens to use for representation when calculating the similarity in training and inference. (default: all) -
--length_norm [LENGTH_NORM]
: Whether to normalize by length while considering pad (default: False) -
--mlm_factor MLM_FACTOR
: The factor to scale the MLM loss. (default: 0.0) -
--mask_prob MASK_PROB
: The probability of masking a token. (default: 0.0) -
--model_name_or_path MODEL_NAME_OR_PATH
: Path to pretrained model or model identifier from huggingface.co/models (default: None) -
--config_name CONFIG_NAME
: Pretrained config name or path if not the same as model_name (default: None) -
--tokenizer_name TOKENIZER_NAME
: Pretrained tokenizer name or path if not the same as model_name (default: None) -
--cache_dir CACHE_DIR
: Where do you want to store the pretrained models downloaded from huggingface.co (default: None) -
--use_fast_tokenizer [USE_FAST_TOKENIZER]
: Whether to use one of the fast tokenizer (backed by the tokenizers library) or not. (default: True) -
--no_use_fast_tokenizer
: Whether to use one of the fast tokenizer (backed by the tokenizers library) or not. (default: False) -
--model_revision MODEL_REVISION
: The specific model version to use (can be a branch name, tag name, or commit id). (default: main) -
--use_auth_token [USE_AUTH_TOKEN]
: Will use the token generated when runningtransformers-cli login
(necessary to use this script with private models). (default: False) -
--ignore_mismatched_sizes [IGNORE_MISMATCHED_SIZES]
: Will enable to load a pretrained model whose head dimensions are different. (default: False) -
--load_from_FastFit [LOAD_FROM_FASTFIT]
: Will load the model from the trained model directory. (default: False) -
--task_name TASK_NAME
: The name of the task to train on: custom (default: None) -
--metric_name METRIC_NAME
: The name of the task to train on: custom (default: accuracy) -
--dataset_name DATASET_NAME
: The name of the dataset to use (via the datasets library). (default: None) -
--dataset_config_name DATASET_CONFIG_NAME
: The configuration name of the dataset to use (via the datasets library). (default: None) -
--max_seq_length MAX_SEQ_LENGTH
: The maximum total input sequence length after tokenization. Sequences longer than this will be truncated, sequences shorter will be padded. (default: 128) -
--overwrite_cache [OVERWRITE_CACHE]
: Overwrite the cached preprocessed datasets or not. (default: False) -
--pad_to_max_length [PAD_TO_MAX_LENGTH]
: Whether to pad all samples tomax_seq_length
. If False, will pad the samples dynamically when batching to the maximum length in the batch. (default: True) -
--no_pad_to_max_length
: Whether to pad all samples tomax_seq_length
. If False, will pad the samples dynamically when batching to the maximum length in the batch. (default: False) -
--max_train_samples MAX_TRAIN_SAMPLES
: For debugging purposes or quicker training, truncate the number of training examples to this value if set. (default: None) -
--max_eval_samples MAX_EVAL_SAMPLES
: For debugging purposes or quicker training, truncate the number of evaluation examples to this value if set. (default: None) -
--max_predict_samples MAX_PREDICT_SAMPLES
: For debugging purposes or quicker training, truncate the number of prediction examples to this value if set. (default: None) -
--train_file TRAIN_FILE
: A csv or a json file containing the training data. (default: None) -
--validation_file VALIDATION_FILE
: A csv or a json file containing the validation data. (default: None) -
--test_file TEST_FILE
: A csv or a json file containing the test data. (default: None) -
--custom_goal_acc CUSTOM_GOAL_ACC
: If set, save the model every this number of steps. (default: None) -
--text_column_name TEXT_COLUMN_NAME
: The name of the column in the datasets containing the full texts (for summarization). (default: None) -
--label_column_name LABEL_COLUMN_NAME
: The name of the column in the datasets containing the labels. (default: None) -
--max_text_length MAX_TEXT_LENGTH
: The maximum total input sequence length after tokenization for text. (default: 32) -
--max_label_length MAX_LABEL_LENGTH
: The maximum total input sequence length after tokenization for label. (default: 32) -
--pre_train [PRE_TRAIN]
: The path to the pretrained model. (default: False) -
--added_tokens_per_label ADDED_TOKENS_PER_LABEL
: The number of added tokens to add to every class. (default: None) -
--added_tokens_mask_factor ADDED_TOKENS_MASK_FACTOR
: How much of the added tokens should be consisted of mask tokens embedding. (default: 0.0) -
--added_tokens_tfidf_factor ADDED_TOKENS_TFIDF_FACTOR
: How much of the added tokens should be consisted of tfidf tokens embedding. (default: 0.0) -
--pad_query_with_mask [PAD_QUERY_WITH_MASK]
: Whether to pad the query with the mask token. (default: False) -
--pad_doc_with_mask [PAD_DOC_WITH_MASK]
: Whether to pad the docs with the mask token. (default: False) -
--doc_mapper DOC_MAPPER
: The source for mapping docs to augmented docs (default: None) -
--doc_mapper_type DOC_MAPPER_TYPE
: The type of doc mapper (default: file) -
--output_dir OUTPUT_DIR
: The output directory where the model predictions and checkpoints will be written. (default: None) -
--overwrite_output_dir [OVERWRITE_OUTPUT_DIR]
: Overwrite the content of the output directory. Use this to continue training if output_dir points to a checkpoint directory. (default: False) -
--do_train [DO_TRAIN]
: Whether to run training. (default: False) -
--do_eval [DO_EVAL]
: Whether to run eval on the dev set. (default: False) -
--do_predict [DO_PREDICT]
: Whether to run predictions on the test set. (default: False) -
--evaluation_strategy {no,steps,epoch}
: The evaluation strategy to use. (default: no) -
--prediction_loss_only [PREDICTION_LOSS_ONLY]
: When performing evaluation and predictions, only returns the loss. (default: False) -
--per_device_train_batch_size PER_DEVICE_TRAIN_BATCH_SIZE
: Batch size per GPU/TPU core/CPU for training. (default: 8) -
--per_device_eval_batch_size PER_DEVICE_EVAL_BATCH_SIZE
: Batch size per GPU/TPU core/CPU for evaluation. (default: 8) -
--per_gpu_train_batch_size PER_GPU_TRAIN_BATCH_SIZE
: Deprecated, the use of--per_device_train_batch_size
is preferred. Batch size per GPU/TPU core/CPU for training. (default: None) -
--per_gpu_eval_batch_size PER_GPU_EVAL_BATCH_SIZE
: Deprecated, the use of--per_device_eval_batch_size
is preferred. Batch size per GPU/TPU core/CPU for evaluation. (default: None) -
--gradient_accumulation_steps GRADIENT_ACCUMULATION_STEPS
: Number of updates steps to accumulate before performing a backward/update pass. (default: 1) -
--eval_accumulation_steps EVAL_ACCUMULATION_STEPS
: Number of predictions steps to accumulate before moving the tensors to the CPU. (default: None) -
--eval_delay EVAL_DELAY
: Number of epochs or steps to wait for before the first evaluation can be performed, depending on the evaluation_strategy. (default: 0) -
--learning_rate LEARNING_RATE
: The initial learning rate for AdamW. (default: 5e-05) -
--weight_decay WEIGHT_DECAY
: Weight decay for AdamW if we apply some. (default: 0.0) -
--adam_beta1 ADAM_BETA1
: Beta1 for AdamW optimizer (default: 0.9) -
--adam_beta2 ADAM_BETA2
: Beta2 for AdamW optimizer (default: 0.999) -
--adam_epsilon ADAM_EPSILON
: Epsilon for AdamW optimizer. (default: 1e-08) -
--max_grad_norm MAX_GRAD_NORM
: Max gradient norm. (default: 1.0) -
--num_train_epochs NUM_TRAIN_EPOCHS
: Total number of training epochs to perform. (default: 3.0) -
--max_steps MAX_STEPS
: If > 0: set the total number of training steps to perform. Override num_train_epochs. (default: -1) -
--lr_scheduler_type {linear,cosine,cosine_with_restarts,polynomial,constant,constant_with_warmup}
: The scheduler type to use. (default: linear) -
--warmup_ratio WARMUP_RATIO
: Linear warmup over warmup_ratio fraction of total steps. (default: 0.0) -
--warmup_steps WARMUP_STEPS
: Linear warmup over warmup_steps. (default: 0) -
--log_level {debug,info,warning,error,critical,passive}
: Logger log level to use on the main node. Possible choices are the log levels as strings: 'debug', 'info', 'warning', 'error', and 'critical', plus a 'passive' level which doesn't set anything and lets the application set the level. Defaults to 'passive'. (default: passive) -
--log_level_replica {debug,info,warning,error,critical,passive}
: Logger log level to use on replica nodes. Same choices and defaults aslog_level
(default: passive) -
--log_on_each_node [LOG_ON_EACH_NODE]
: When doing a multinode distributed training, whether to log once per node or just once on the main node. (default: True) -
--no_log_on_each_node
: When doing a multinode distributed training, whether to log once per node or just once on the main node. (default: False) -
--logging_dir LOGGING_DIR
: Tensorboard log dir. (default: None) -
--logging_strategy {no,steps,epoch}
: The logging strategy to use. (default: steps) -
--logging_first_step [LOGGING_FIRST_STEP]
: Log the first global_step (default: False) -
--logging_steps LOGGING_STEPS
: Log every X updates steps. (default: 500) -
--logging_nan_inf_filter [LOGGING_NAN_INF_FILTER]
: Filter nan and inf losses for logging. (default: True) -
--no_logging_nan_inf_filter
: Filter nan and inf losses for logging. (default: False) -
--save_strategy {no,steps,epoch}
: The checkpoint save strategy to use. (default: steps) -
--save_steps SAVE_STEPS
: Save checkpoint every X updates steps. (default: 500)
For Tasks:
Click tags to check more tools for each tasksFor Jobs:
Alternative AI tools for fastfit
Similar Open Source Tools
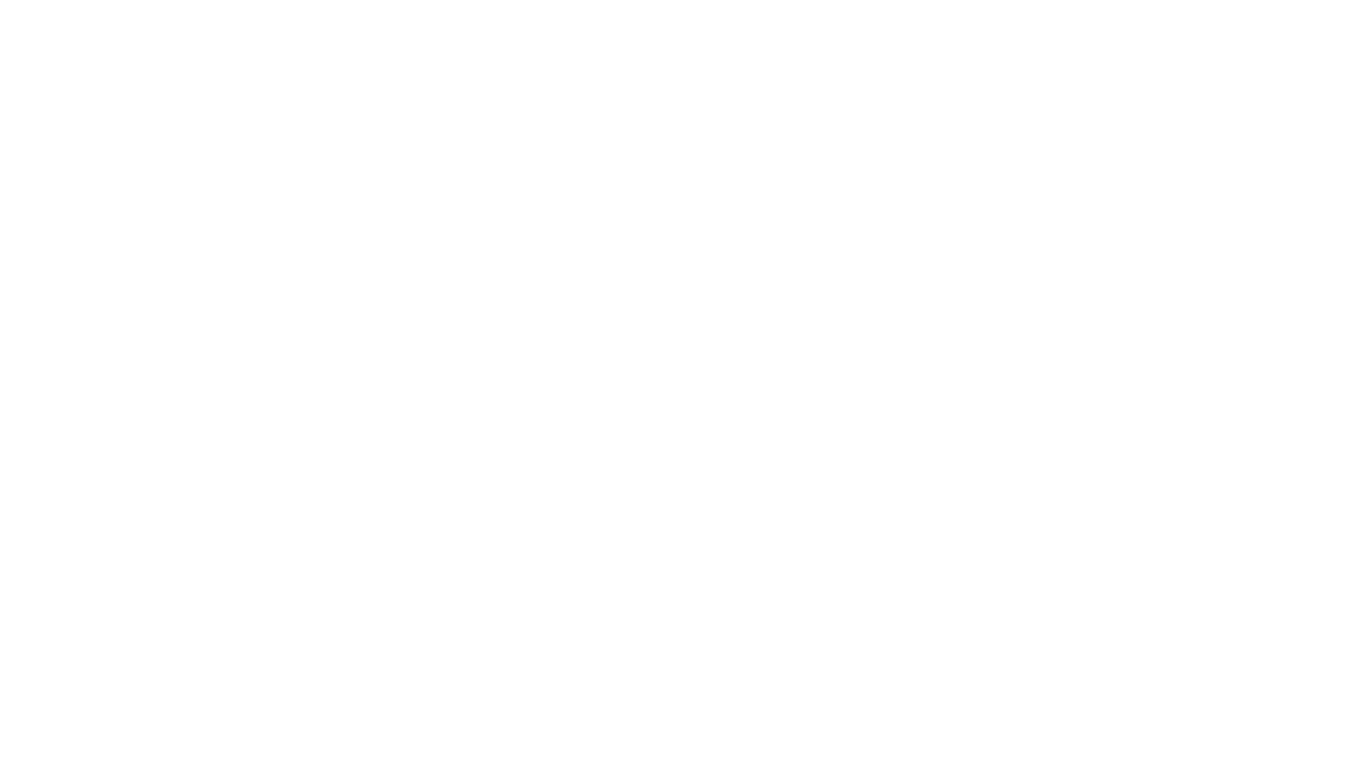
fastfit
FastFit is a Python package designed for fast and accurate few-shot classification, especially for scenarios with many semantically similar classes. It utilizes a novel approach integrating batch contrastive learning and token-level similarity score, significantly improving multi-class classification performance in speed and accuracy across various datasets. FastFit provides a convenient command-line tool for training text classification models with customizable parameters. It offers a 3-20x improvement in training speed, completing training in just a few seconds. Users can also train models with Python scripts and perform inference using pretrained models for text classification tasks.
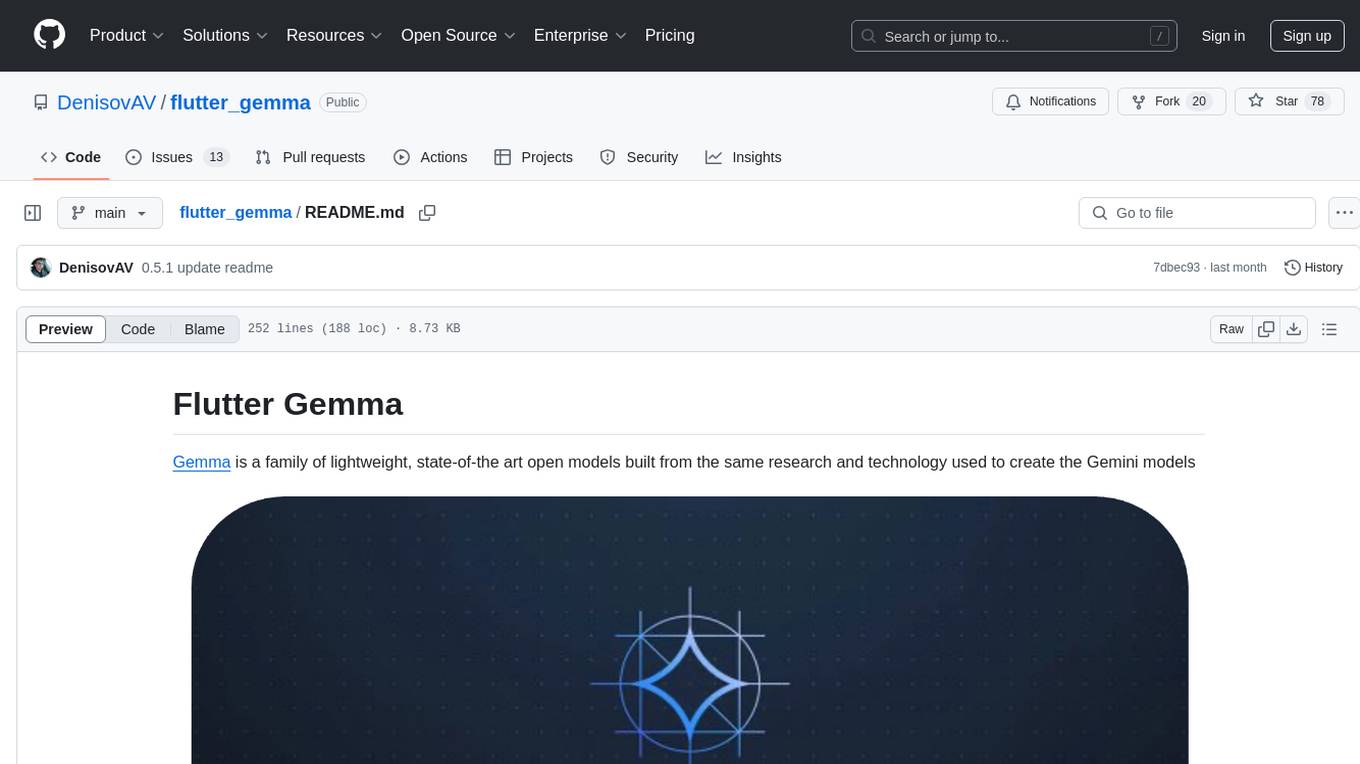
flutter_gemma
Flutter Gemma is a family of lightweight, state-of-the art open models that bring the power of Google's Gemma language models directly to Flutter applications. It allows for local execution on user devices, supports both iOS and Android platforms, and offers LoRA support for tailored AI behavior. The tool provides a simple interface for integrating Gemma models into Flutter projects, enabling advanced AI capabilities without relying on external servers. Users can easily download pre-trained Gemma models, fine-tune them for specific use cases, and customize behavior using LoRA weights. The tool supports model and LoRA weight management, model initialization, response generation, and chat scenarios, with considerations for model size, LoRA weights, and production app deployment.
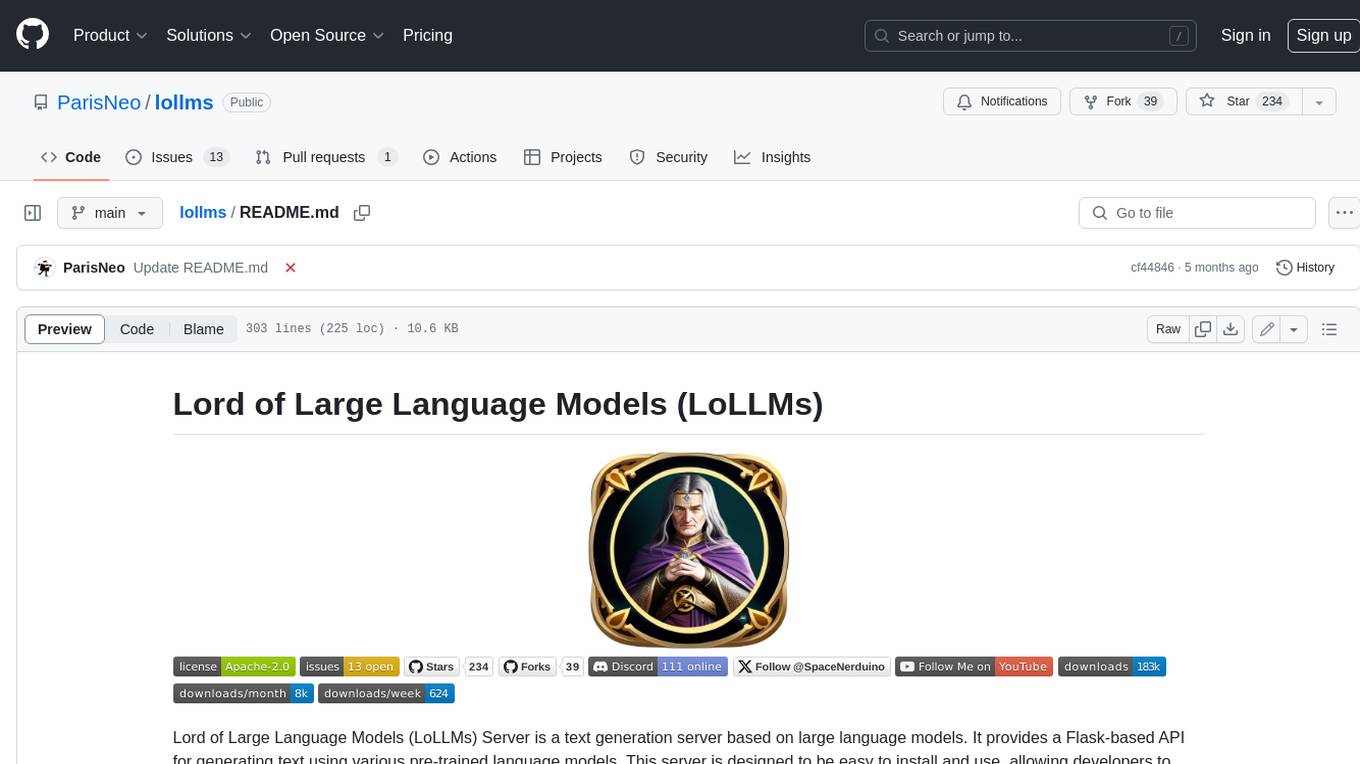
lollms
LoLLMs Server is a text generation server based on large language models. It provides a Flask-based API for generating text using various pre-trained language models. This server is designed to be easy to install and use, allowing developers to integrate powerful text generation capabilities into their applications.
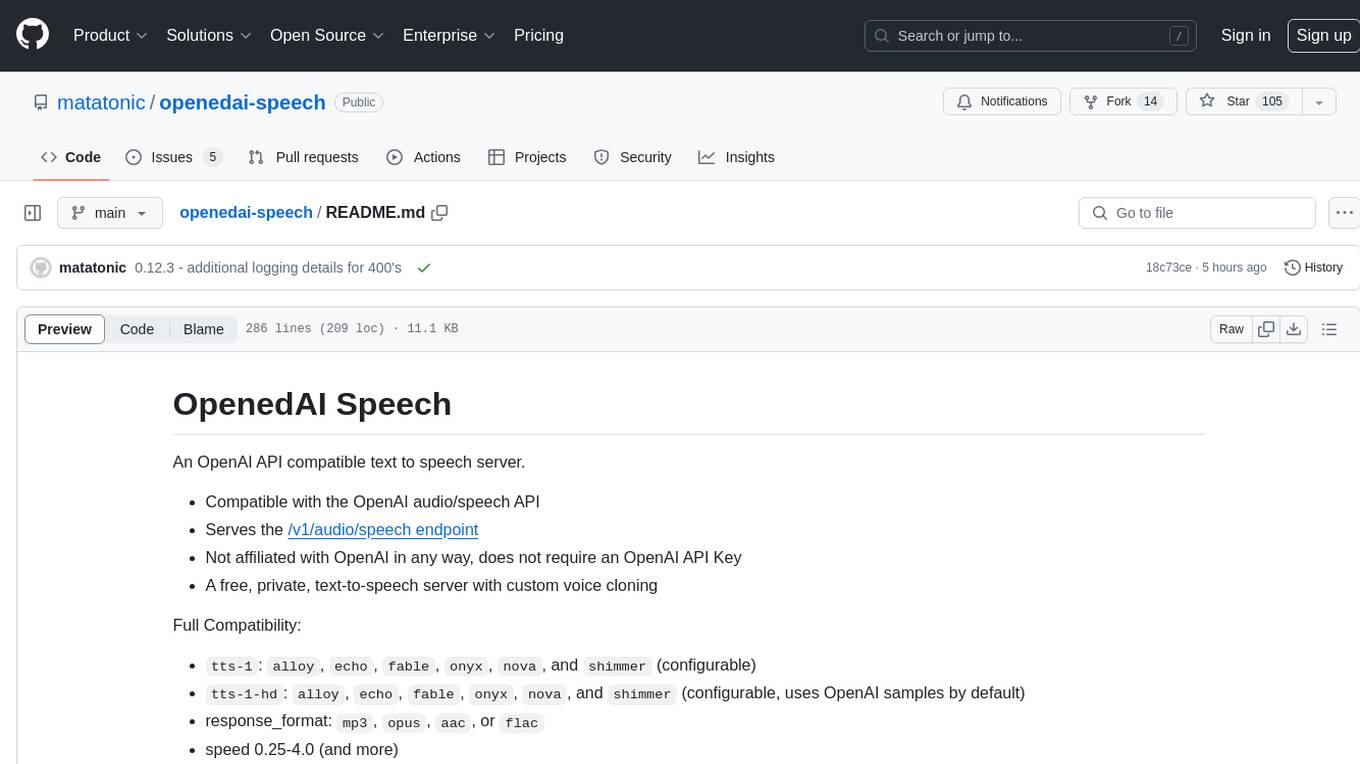
openedai-speech
OpenedAI Speech is a free, private text-to-speech server compatible with the OpenAI audio/speech API. It offers custom voice cloning and supports various models like tts-1 and tts-1-hd. Users can map their own piper voices and create custom cloned voices. The server provides multilingual support with XTTS voices and allows fixing incorrect sounds with regex. Recent changes include bug fixes, improved error handling, and updates for multilingual support. Installation can be done via Docker or manual setup, with usage instructions provided. Custom voices can be created using Piper or Coqui XTTS v2, with guidelines for preparing audio files. The tool is suitable for tasks like generating speech from text, creating custom voices, and multilingual text-to-speech applications.
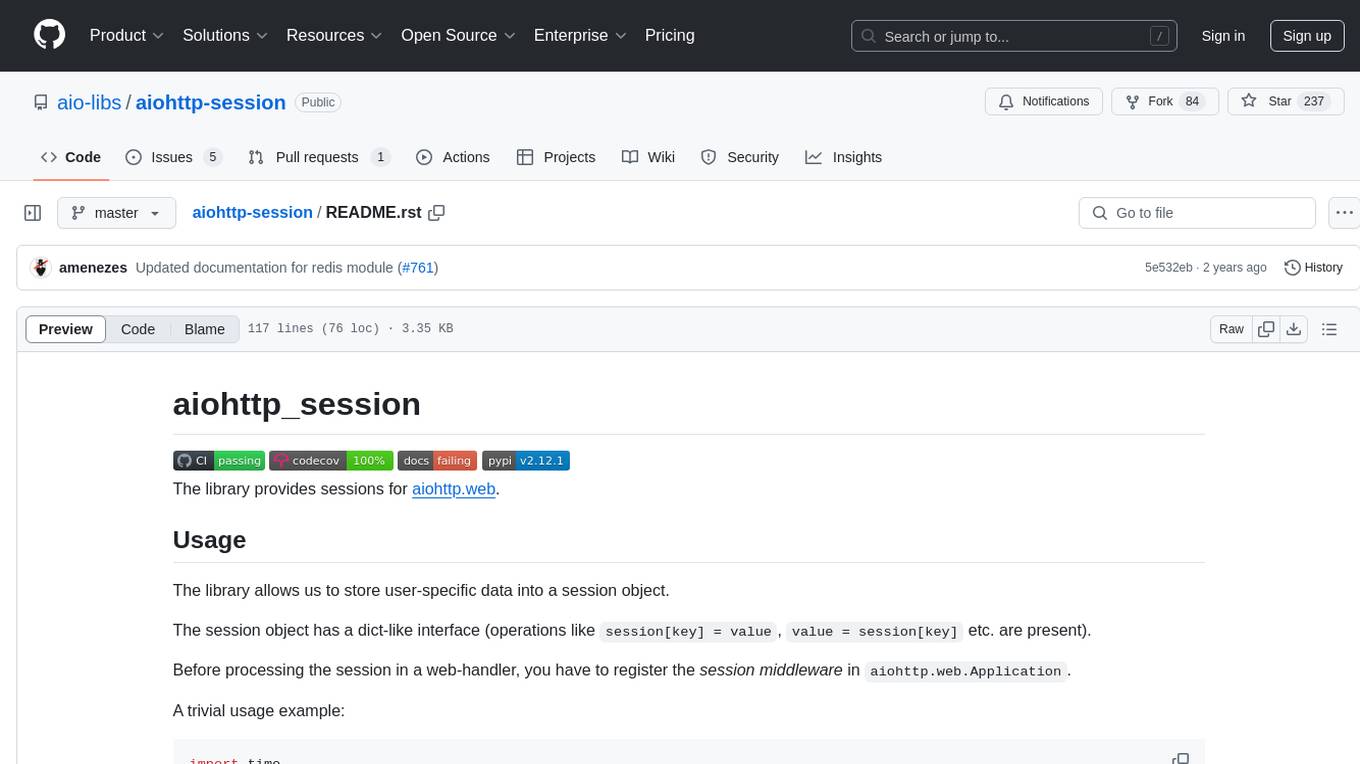
aiohttp-session
aiohttp_session is a Python library that provides session management for aiohttp.web applications. It allows storing user-specific data in session objects with a dict-like interface. The library offers different session storage options, including SimpleCookieStorage for testing, EncryptedCookieStorage for secure data storage, and RedisStorage for storing data in Redis. Users can easily integrate session management into their aiohttp.web applications by registering the session middleware. The library is designed to simplify session handling and enhance the security of web applications.
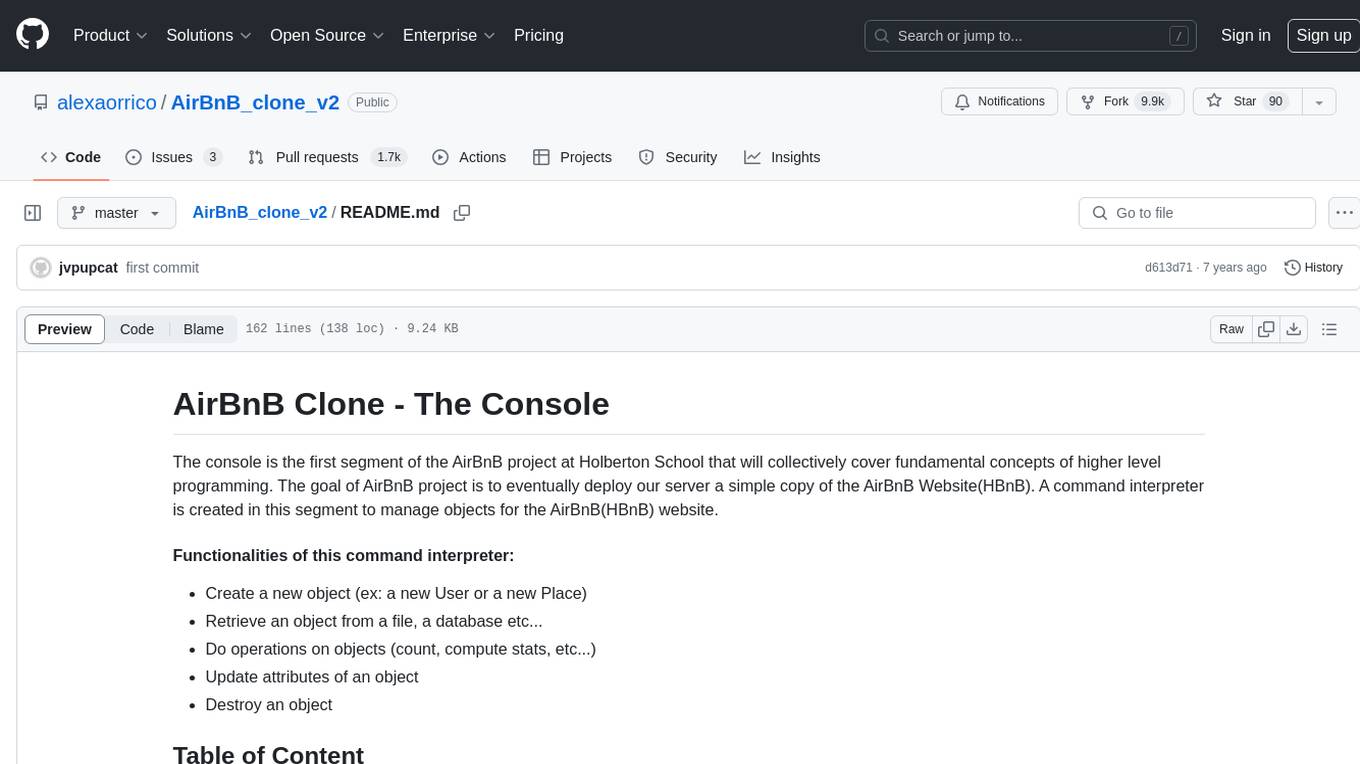
AirBnB_clone_v2
The AirBnB Clone - The Console project is the first segment of the AirBnB project at Holberton School, aiming to cover fundamental concepts of higher level programming. The goal is to deploy a server as a simple copy of the AirBnB Website (HBnB). The project includes a command interpreter to manage objects for the AirBnB website, allowing users to create new objects, retrieve objects, perform operations on objects, update object attributes, and destroy objects. The project is interpreted/tested on Ubuntu 14.04 LTS using Python 3.4.3.
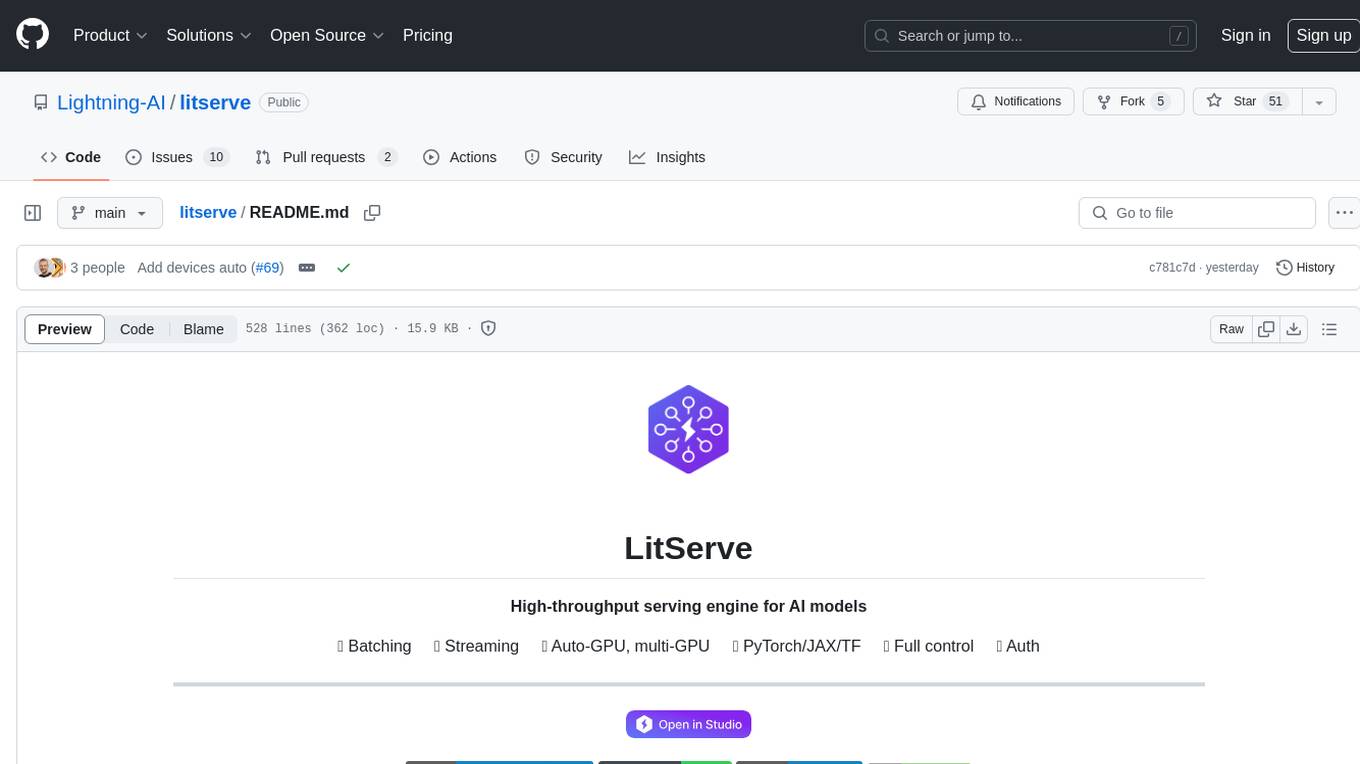
litserve
LitServe is a high-throughput serving engine for deploying AI models at scale. It generates an API endpoint for a model, handles batching, streaming, autoscaling across CPU/GPUs, and more. Built for enterprise scale, it supports every framework like PyTorch, JAX, Tensorflow, and more. LitServe is designed to let users focus on model performance, not the serving boilerplate. It is like PyTorch Lightning for model serving but with broader framework support and scalability.
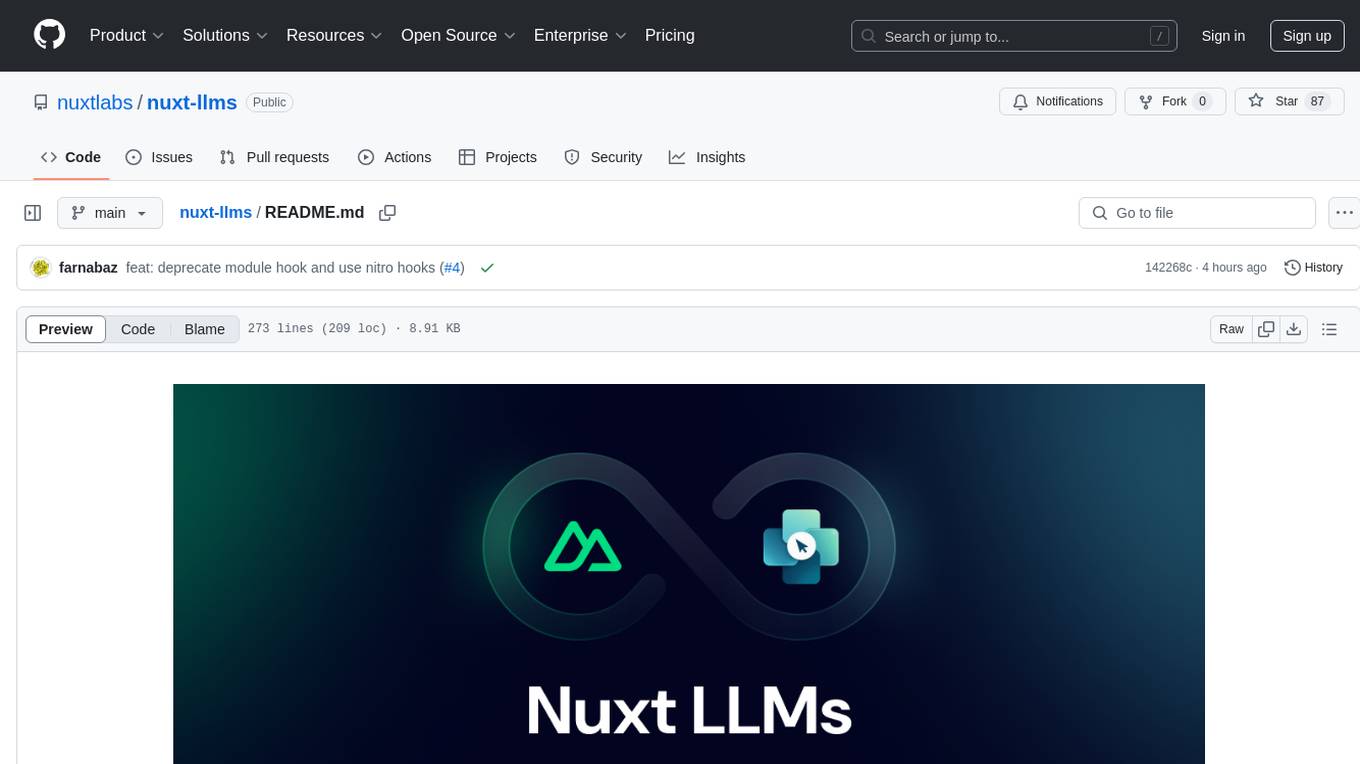
nuxt-llms
Nuxt LLMs automatically generates llms.txt markdown documentation for Nuxt applications. It provides runtime hooks to collect data from various sources and generate structured documentation. The tool allows customization of sections directly from nuxt.config.ts and integrates with Nuxt modules via the runtime hooks system. It generates two documentation formats: llms.txt for concise structured documentation and llms_full.txt for detailed documentation. Users can extend documentation using hooks to add sections, links, and metadata. The tool is suitable for developers looking to automate documentation generation for their Nuxt applications.
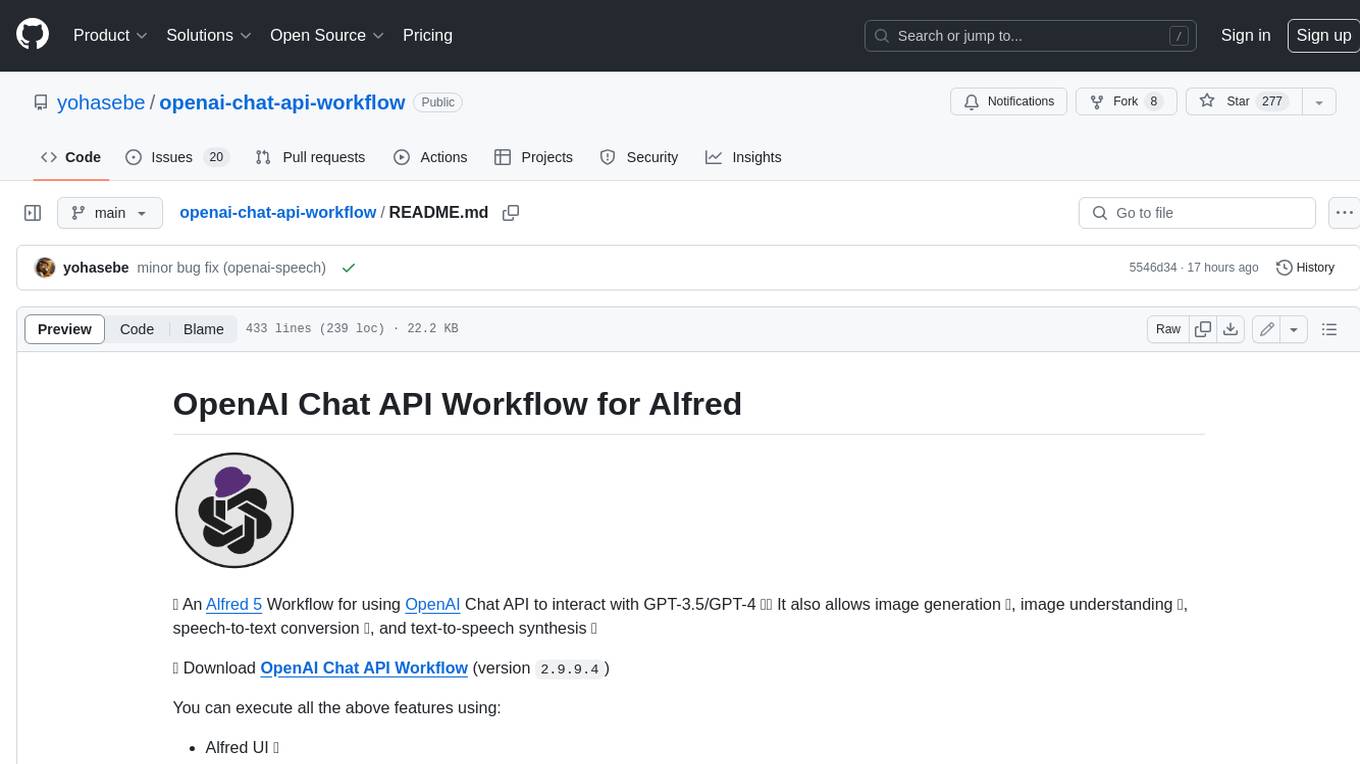
openai-chat-api-workflow
**OpenAI Chat API Workflow for Alfred** An Alfred 5 Workflow for using OpenAI Chat API to interact with GPT-3.5/GPT-4 🤖💬 It also allows image generation 🖼️, image understanding 👀, speech-to-text conversion 🎤, and text-to-speech synthesis 🔈 **Features:** * Execute all features using Alfred UI, selected text, or a dedicated web UI * Web UI is constructed by the workflow and runs locally on your Mac 💻 * API call is made directly between the workflow and OpenAI, ensuring your chat messages are not shared online with anyone other than OpenAI 🔒 * OpenAI does not use the data from the API Platform for training 🚫 * Export chat data to a simple JSON format external file 📄 * Continue the chat by importing the exported data later 🔄
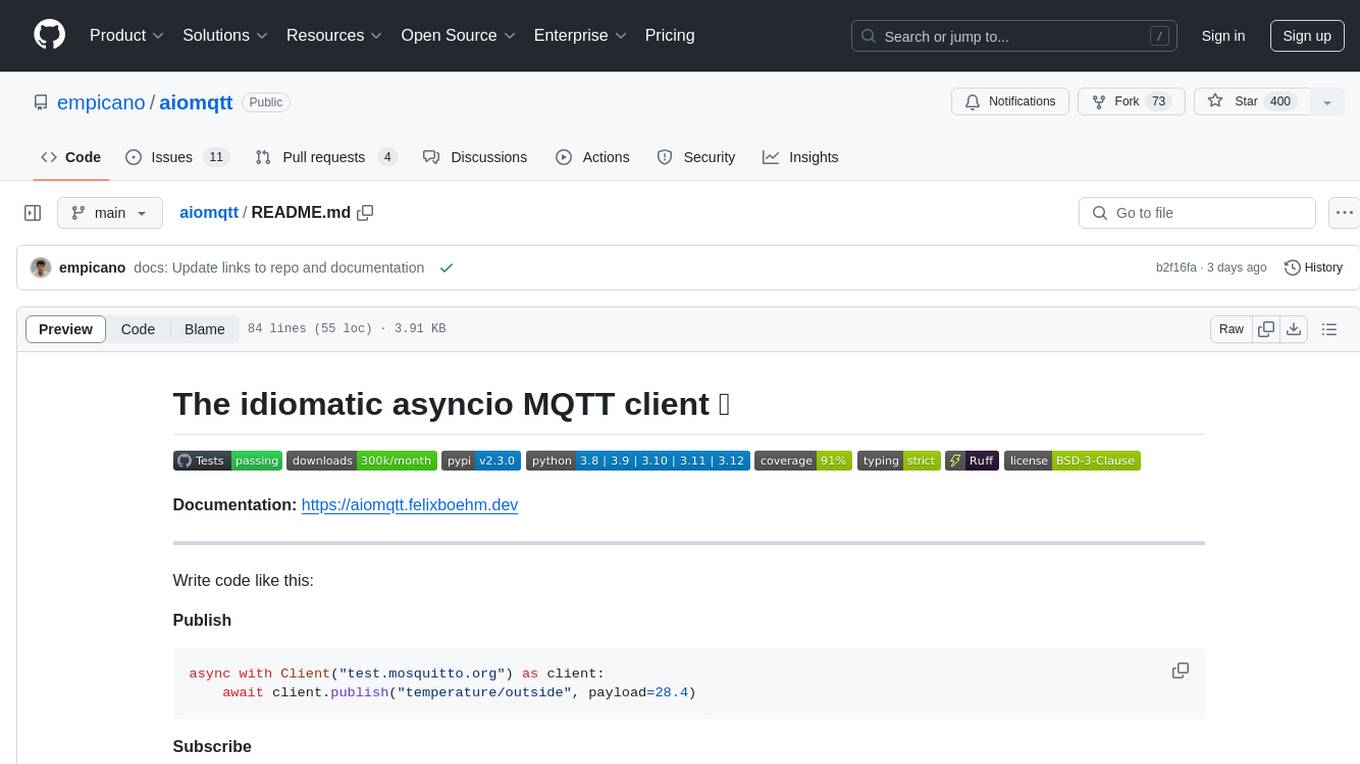
aiomqtt
aiomqtt is an idiomatic asyncio MQTT client that allows users to interact with MQTT brokers using asyncio in Python. It eliminates the need for callbacks and return codes, providing a more streamlined experience. The tool supports MQTT versions 5.0, 3.1.1, and 3.1, and offers graceful disconnection handling. It is fully type-hinted, making it easier to work with. Users can publish and subscribe to MQTT topics with ease, making it a versatile tool for MQTT communication in Python.
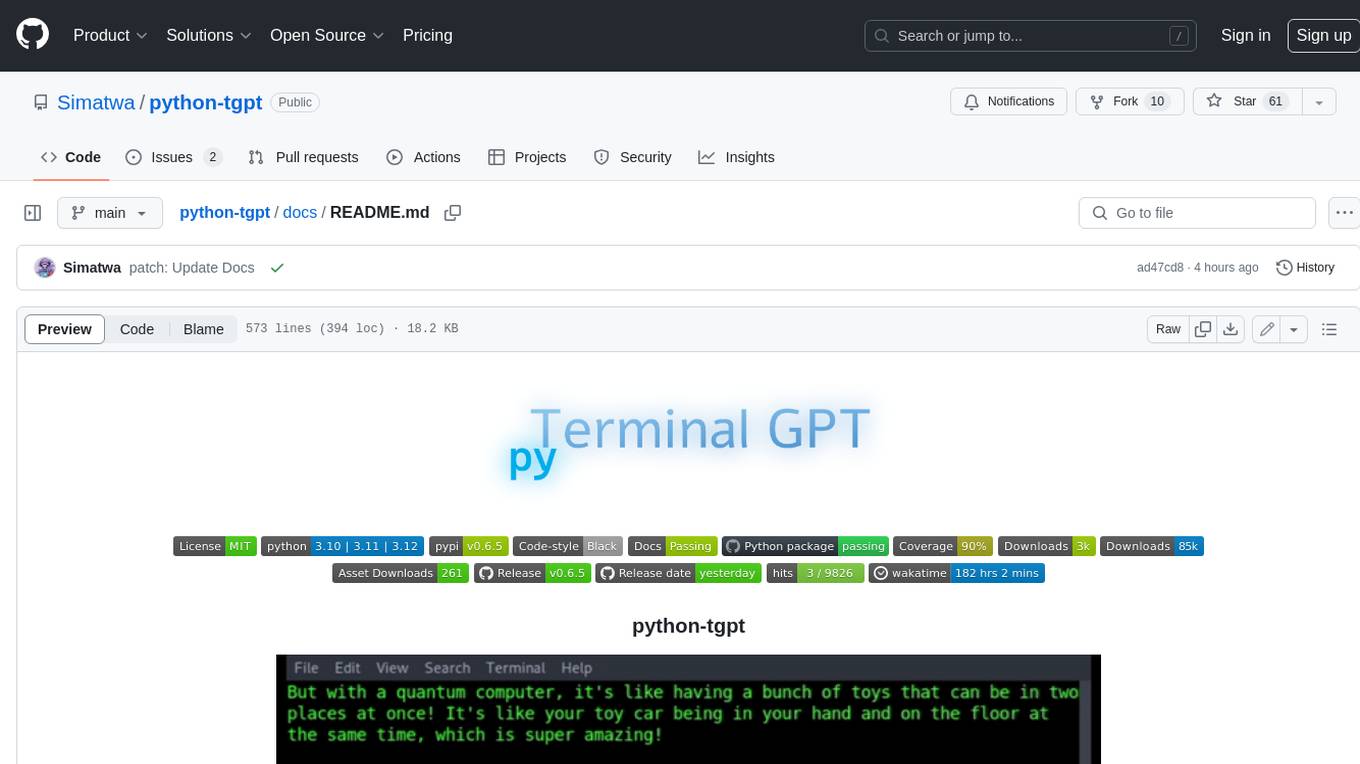
python-tgpt
Python-tgpt is a Python package that enables seamless interaction with over 45 free LLM providers without requiring an API key. It also provides image generation capabilities. The name _python-tgpt_ draws inspiration from its parent project tgpt, which operates on Golang. Through this Python adaptation, users can effortlessly engage with a number of free LLMs available, fostering a smoother AI interaction experience.
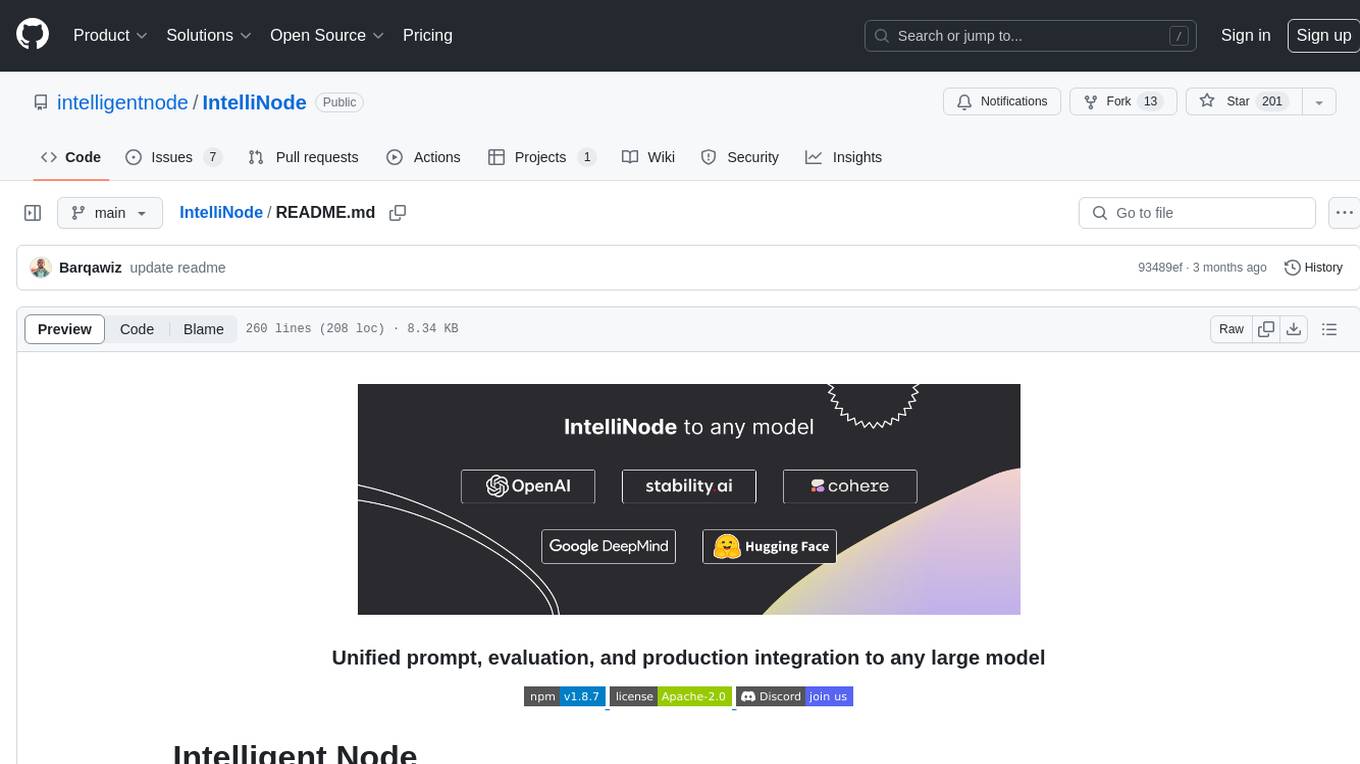
IntelliNode
IntelliNode is a javascript module that integrates cutting-edge AI models like ChatGPT, LLaMA, WaveNet, Gemini, and Stable diffusion into projects. It offers functions for generating text, speech, and images, as well as semantic search, multi-model evaluation, and chatbot capabilities. The module provides a wrapper layer for low-level model access, a controller layer for unified input handling, and a function layer for abstract functionality tailored to various use cases.
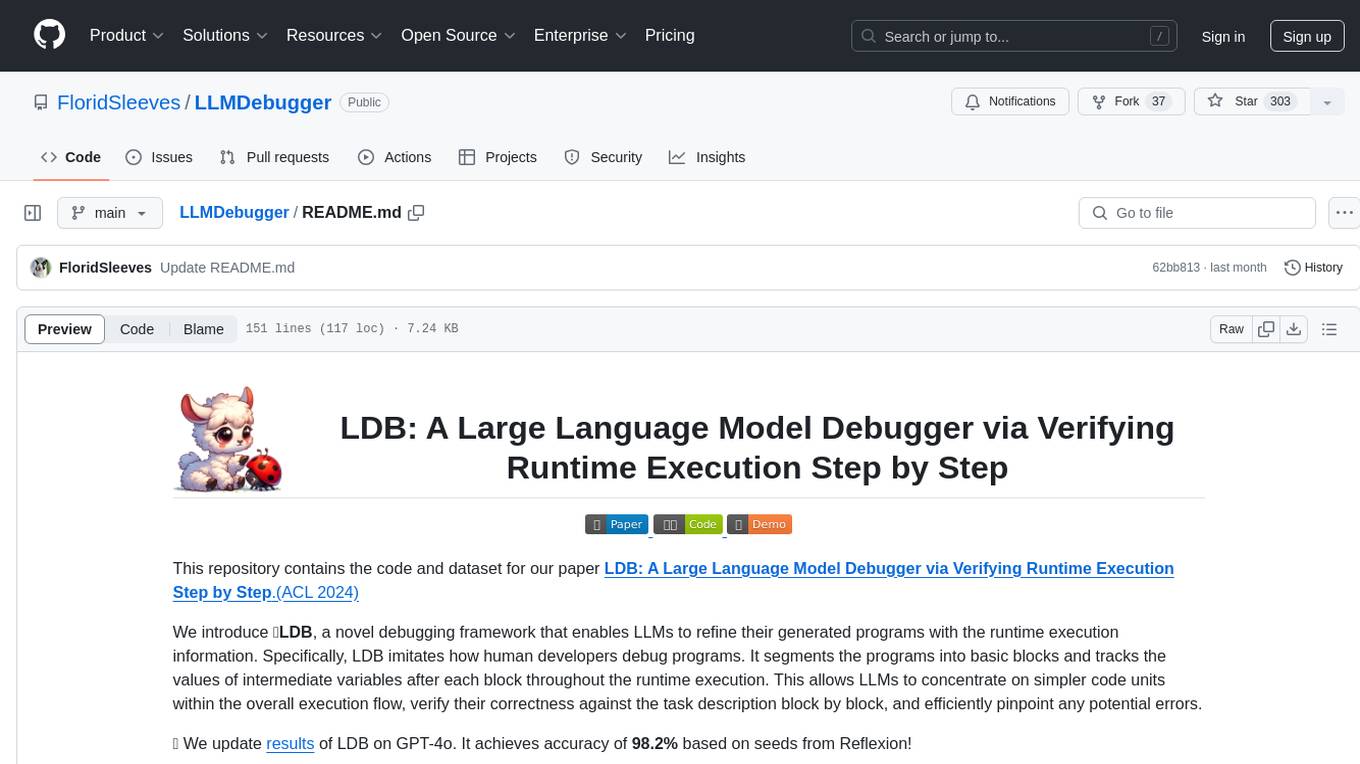
LLMDebugger
This repository contains the code and dataset for LDB, a novel debugging framework that enables Large Language Models (LLMs) to refine their generated programs by tracking the values of intermediate variables throughout the runtime execution. LDB segments programs into basic blocks, allowing LLMs to concentrate on simpler code units, verify correctness block by block, and pinpoint errors efficiently. The tool provides APIs for debugging and generating code with debugging messages, mimicking how human developers debug programs.
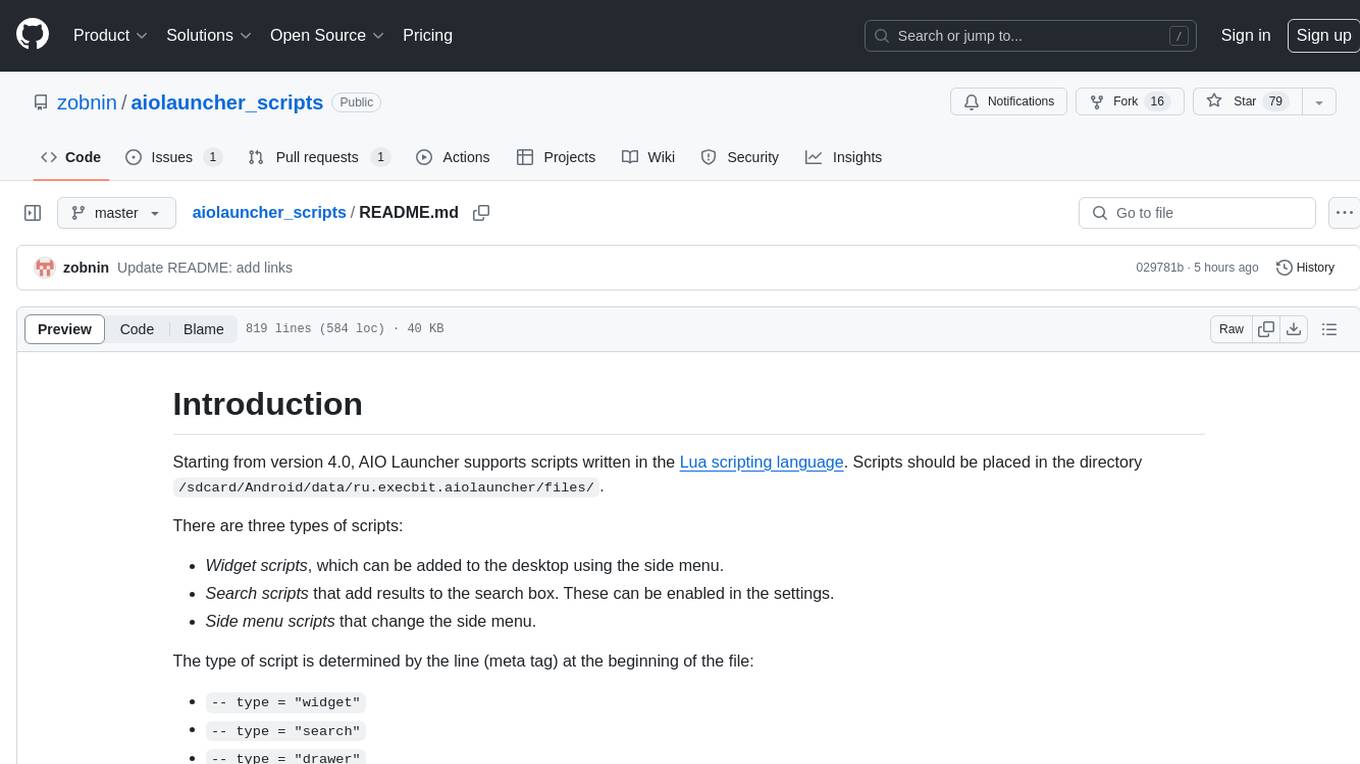
aiolauncher_scripts
AIO Launcher Scripts is a collection of Lua scripts that can be used with AIO Launcher to enhance its functionality. These scripts can be used to create widget scripts, search scripts, and side menu scripts. They provide various functions such as displaying text, buttons, progress bars, charts, and interacting with app widgets. The scripts can be used to customize the appearance and behavior of the launcher, add new features, and interact with external services.
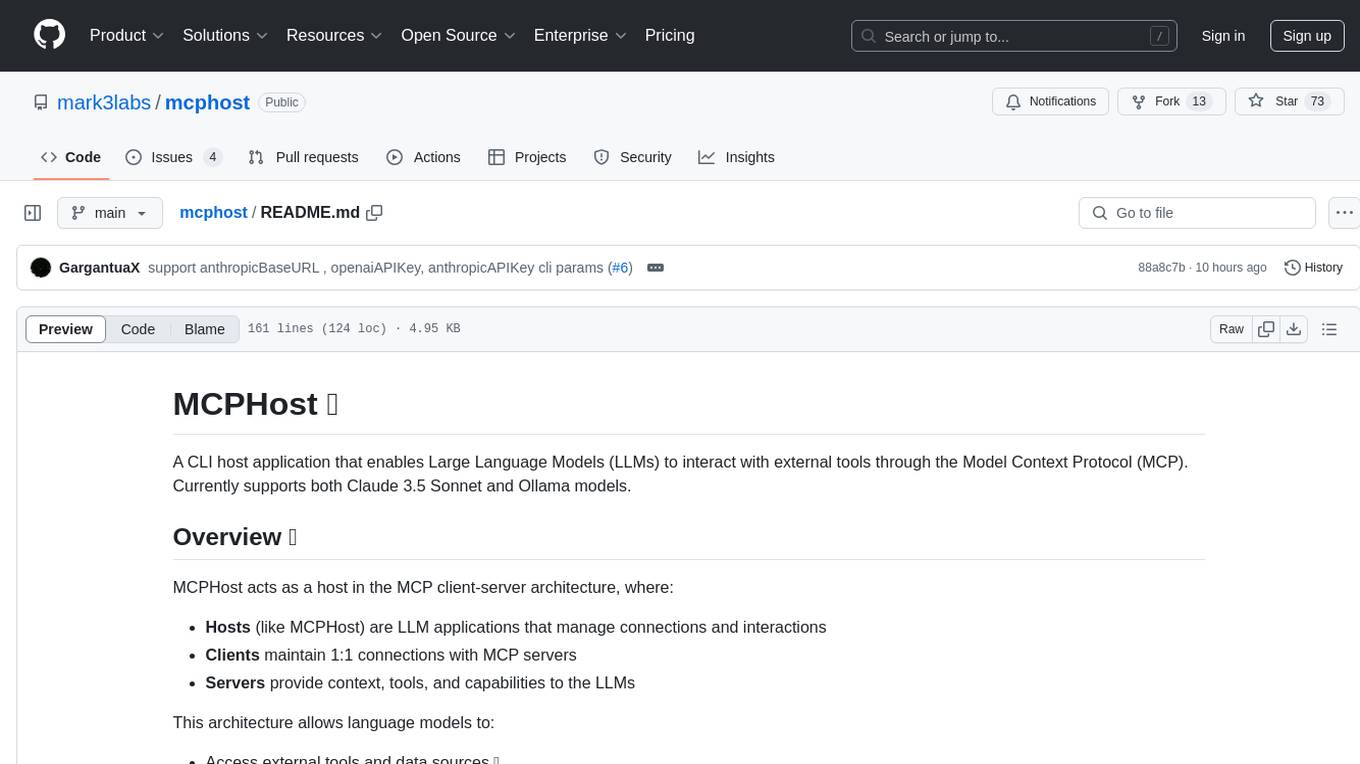
mcphost
MCPHost is a CLI host application that enables Large Language Models (LLMs) to interact with external tools through the Model Context Protocol (MCP). It acts as a host in the MCP client-server architecture, allowing language models to access external tools and data sources, maintain consistent context across interactions, and execute commands safely. The tool supports interactive conversations with Claude 3.5 Sonnet and Ollama models, multiple concurrent MCP servers, dynamic tool discovery and integration, configurable server locations and arguments, and a consistent command interface across model types.
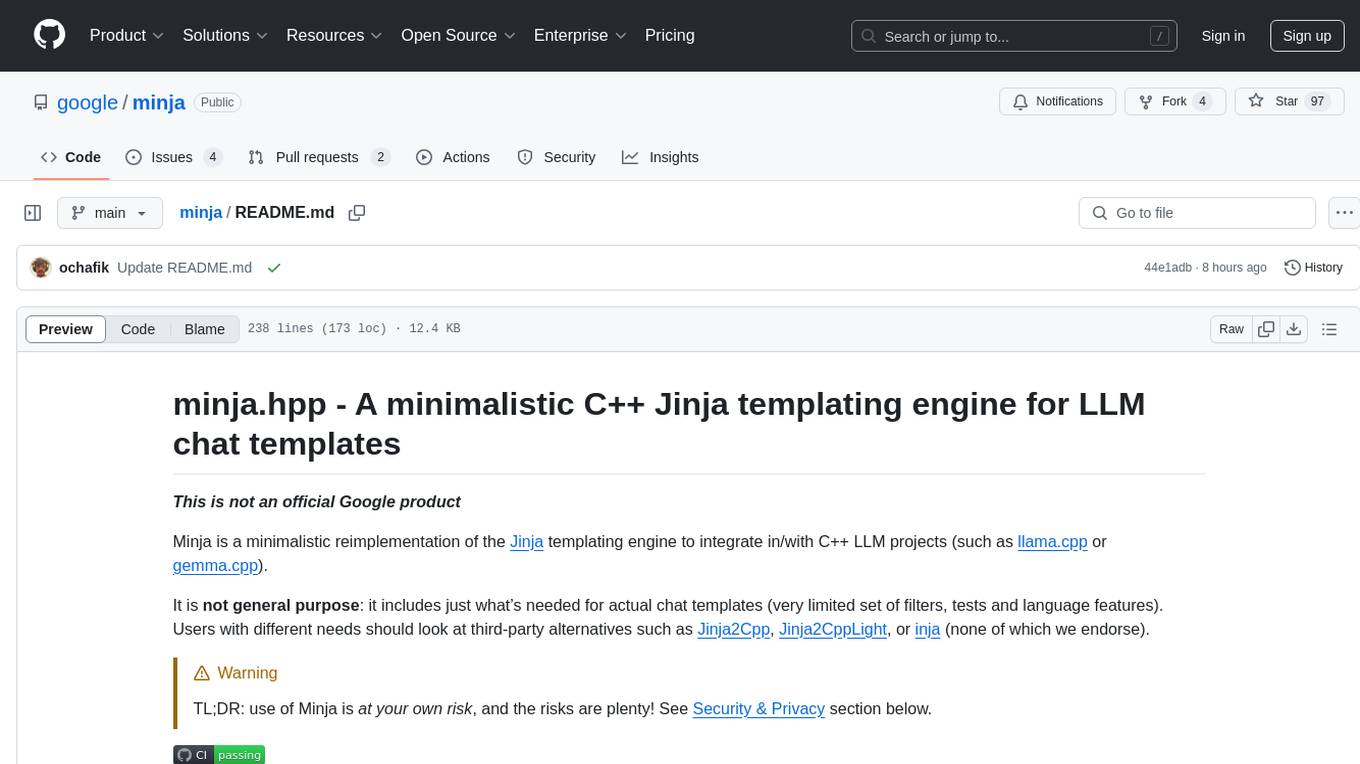
minja
Minja is a minimalistic C++ Jinja templating engine designed specifically for integration with C++ LLM projects, such as llama.cpp or gemma.cpp. It is not a general-purpose tool but focuses on providing a limited set of filters, tests, and language features tailored for chat templates. The library is header-only, requires C++17, and depends only on nlohmann::json. Minja aims to keep the codebase small, easy to understand, and offers decent performance compared to Python. Users should be cautious when using Minja due to potential security risks, and it is not intended for producing HTML or JavaScript output.
For similar tasks
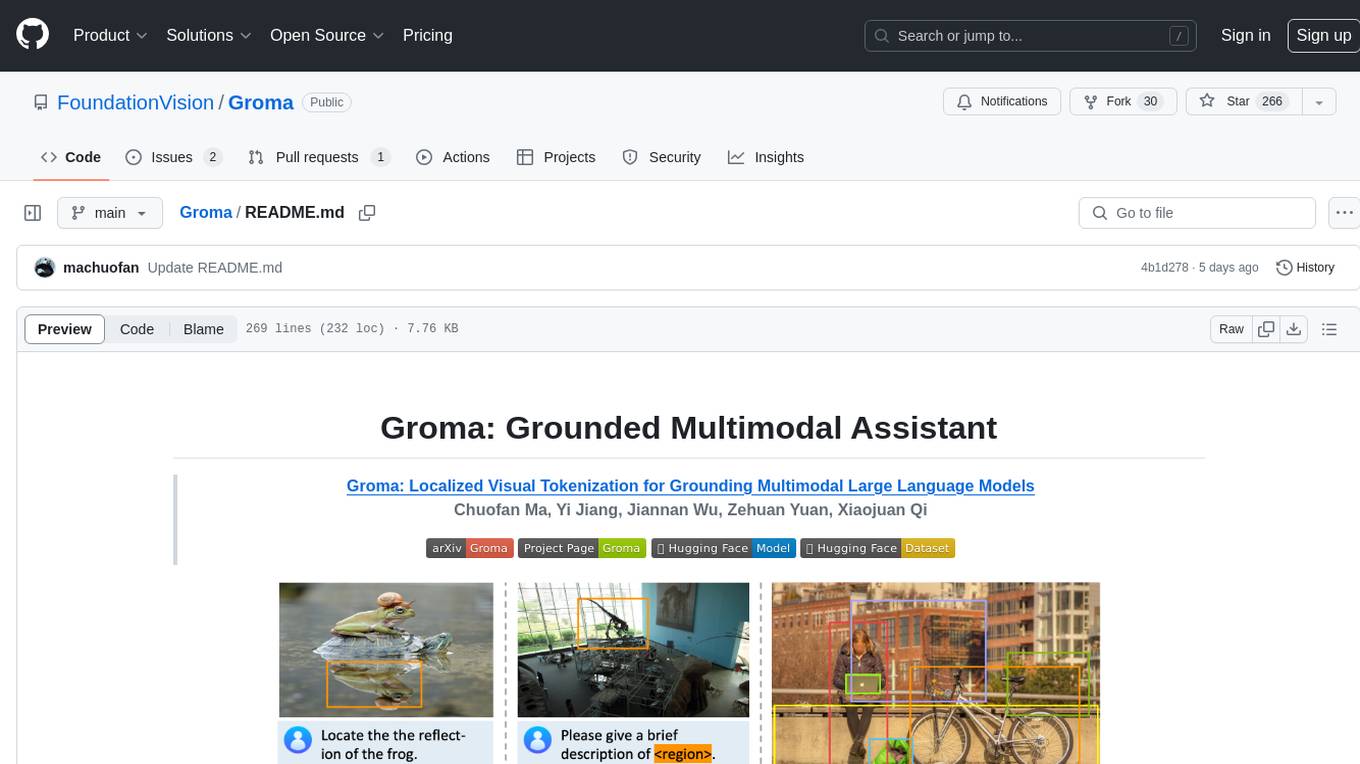
Groma
Groma is a grounded multimodal assistant that excels in region understanding and visual grounding. It can process user-defined region inputs and generate contextually grounded long-form responses. The tool presents a unique paradigm for multimodal large language models, focusing on visual tokenization for localization. Groma achieves state-of-the-art performance in referring expression comprehension benchmarks. The tool provides pretrained model weights and instructions for data preparation, training, inference, and evaluation. Users can customize training by starting from intermediate checkpoints. Groma is designed to handle tasks related to detection pretraining, alignment pretraining, instruction finetuning, instruction following, and more.
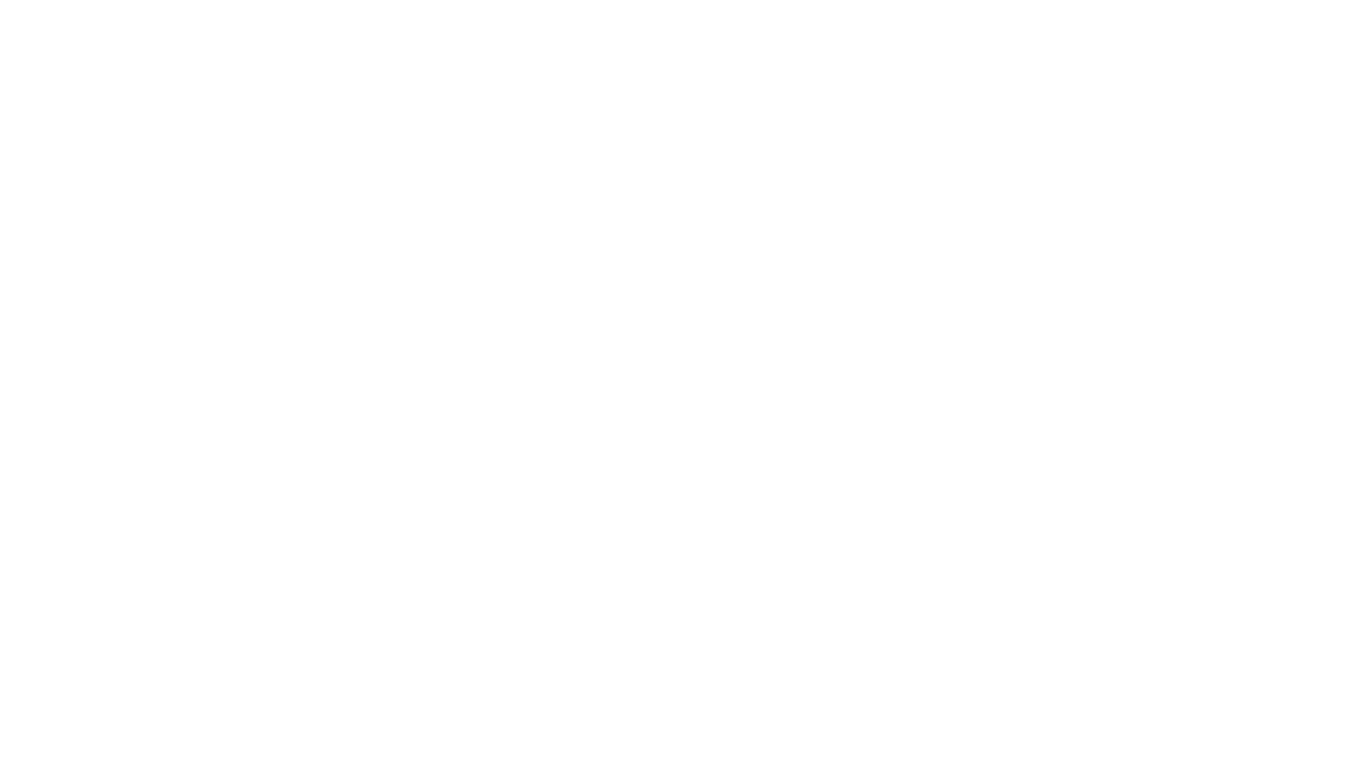
fastfit
FastFit is a Python package designed for fast and accurate few-shot classification, especially for scenarios with many semantically similar classes. It utilizes a novel approach integrating batch contrastive learning and token-level similarity score, significantly improving multi-class classification performance in speed and accuracy across various datasets. FastFit provides a convenient command-line tool for training text classification models with customizable parameters. It offers a 3-20x improvement in training speed, completing training in just a few seconds. Users can also train models with Python scripts and perform inference using pretrained models for text classification tasks.
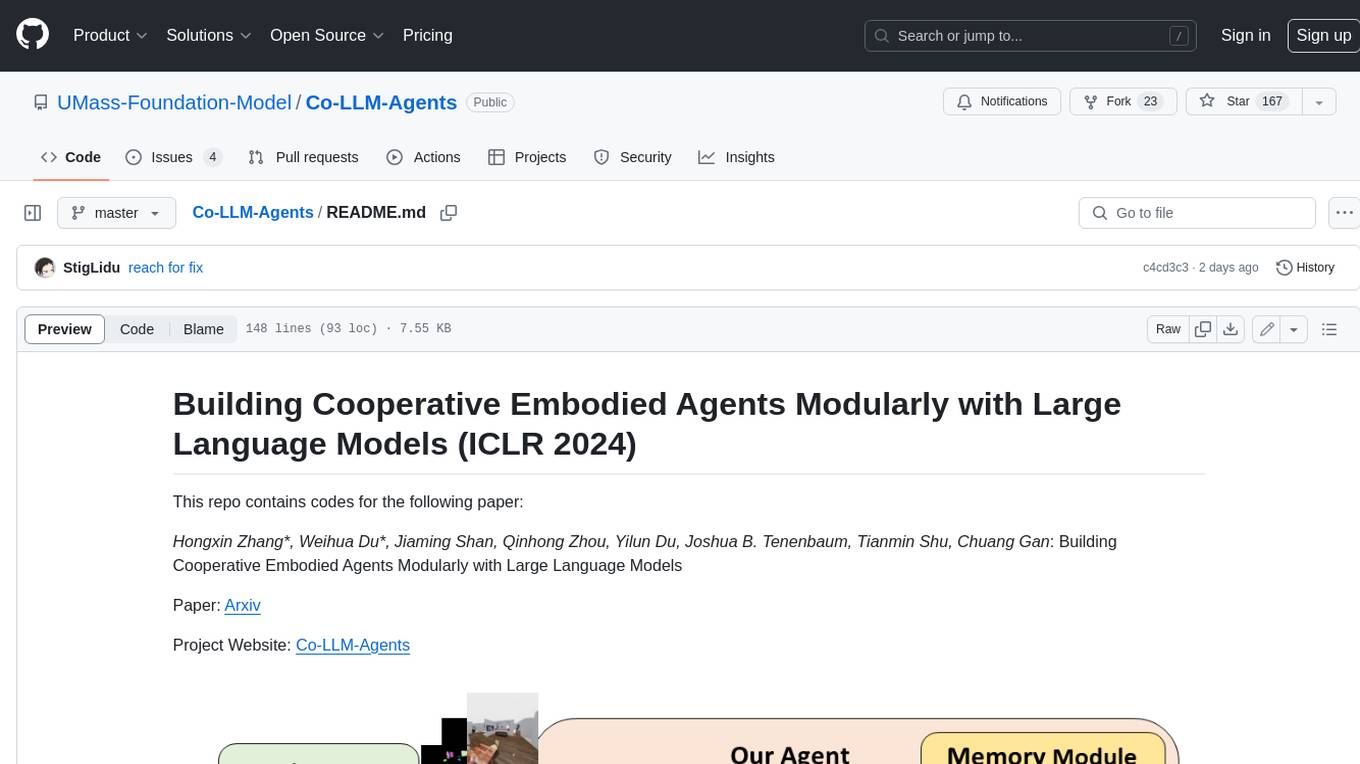
Co-LLM-Agents
This repository contains code for building cooperative embodied agents modularly with large language models. The agents are trained to perform tasks in two different environments: ThreeDWorld Multi-Agent Transport (TDW-MAT) and Communicative Watch-And-Help (C-WAH). TDW-MAT is a multi-agent environment where agents must transport objects to a goal position using containers. C-WAH is an extension of the Watch-And-Help challenge, which enables agents to send messages to each other. The code in this repository can be used to train agents to perform tasks in both of these environments.
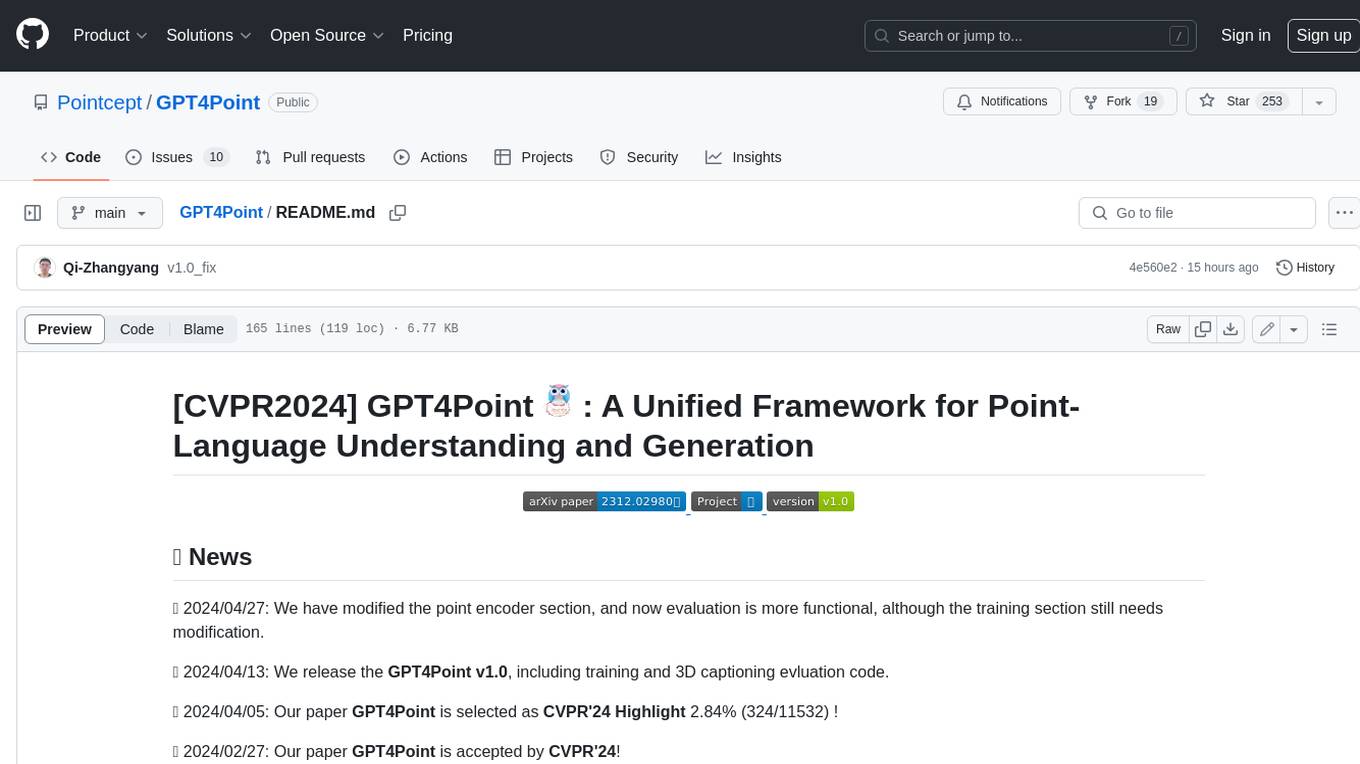
GPT4Point
GPT4Point is a unified framework for point-language understanding and generation. It aligns 3D point clouds with language, providing a comprehensive solution for tasks such as 3D captioning and controlled 3D generation. The project includes an automated point-language dataset annotation engine, a novel object-level point cloud benchmark, and a 3D multi-modality model. Users can train and evaluate models using the provided code and datasets, with a focus on improving models' understanding capabilities and facilitating the generation of 3D objects.
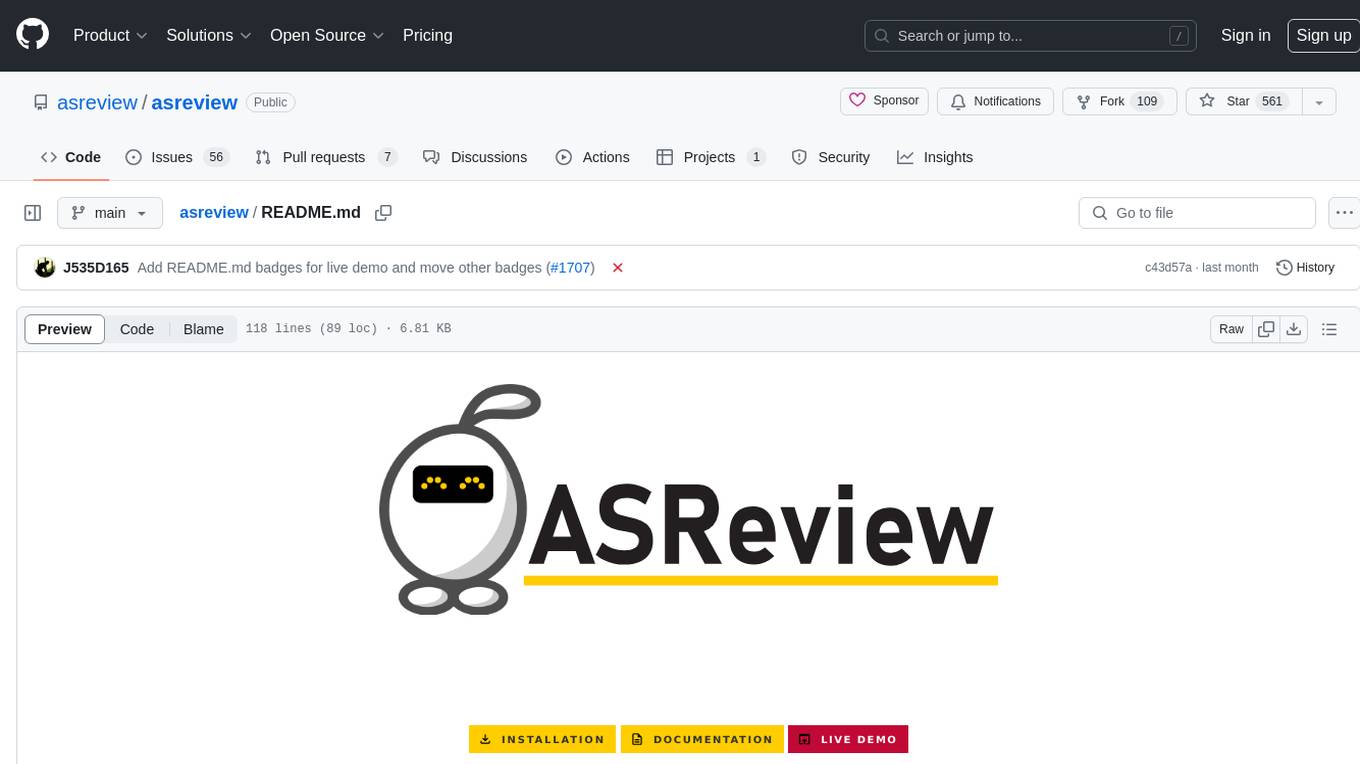
asreview
The ASReview project implements active learning for systematic reviews, utilizing AI-aided pipelines to assist in finding relevant texts for search tasks. It accelerates the screening of textual data with minimal human input, saving time and increasing output quality. The software offers three modes: Oracle for interactive screening, Exploration for teaching purposes, and Simulation for evaluating active learning models. ASReview LAB is designed to support decision-making in any discipline or industry by improving efficiency and transparency in screening large amounts of textual data.
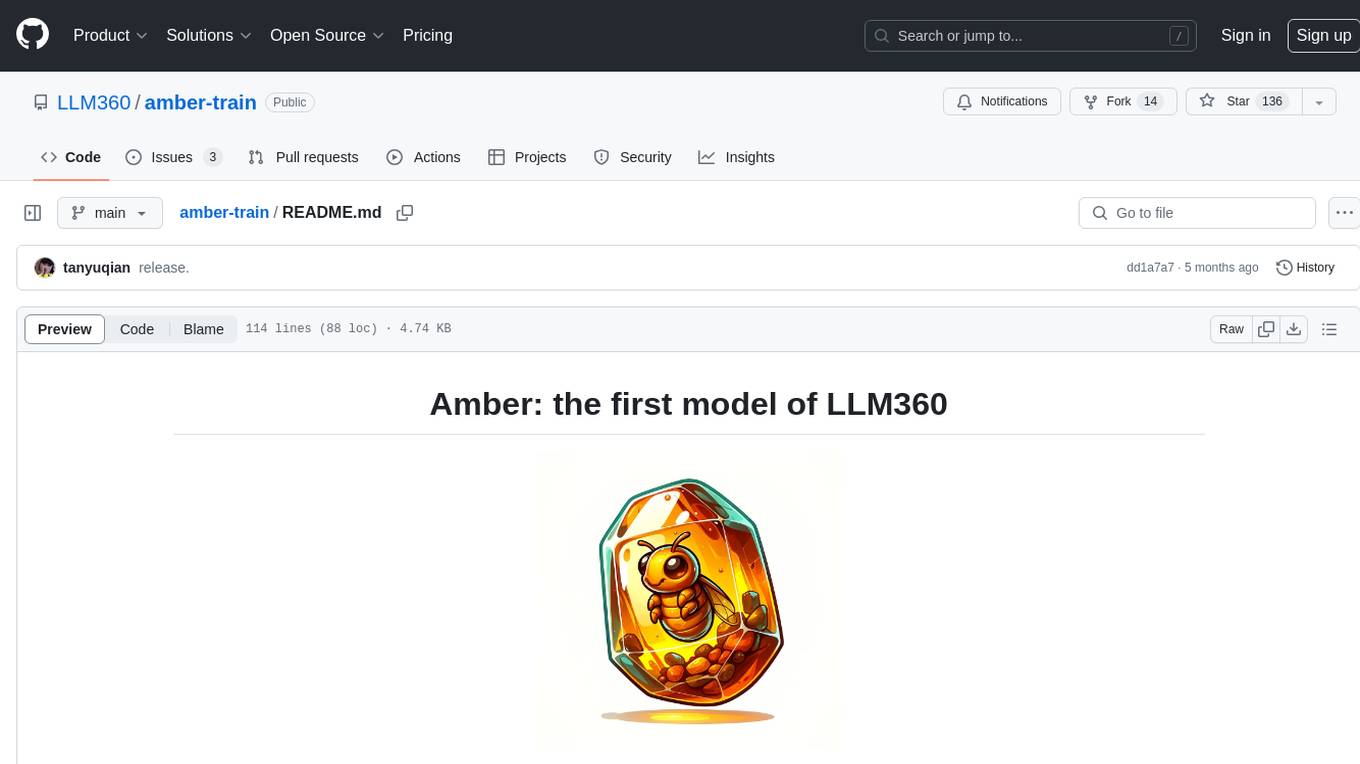
amber-train
Amber is the first model in the LLM360 family, an initiative for comprehensive and fully open-sourced LLMs. It is a 7B English language model with the LLaMA architecture. The model type is a language model with the same architecture as LLaMA-7B. It is licensed under Apache 2.0. The resources available include training code, data preparation, metrics, and fully processed Amber pretraining data. The model has been trained on various datasets like Arxiv, Book, C4, Refined-Web, StarCoder, StackExchange, and Wikipedia. The hyperparameters include a total of 6.7B parameters, hidden size of 4096, intermediate size of 11008, 32 attention heads, 32 hidden layers, RMSNorm ε of 1e^-6, max sequence length of 2048, and a vocabulary size of 32000.
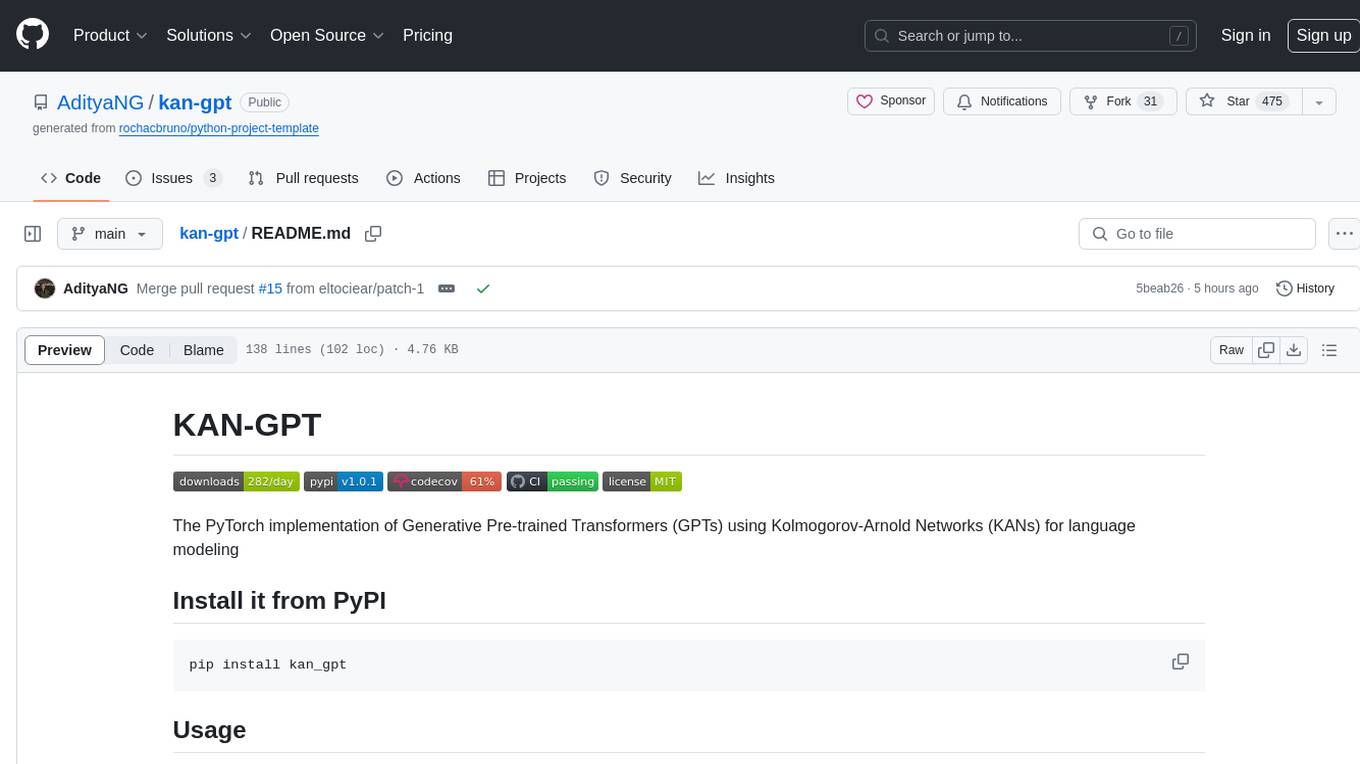
kan-gpt
The KAN-GPT repository is a PyTorch implementation of Generative Pre-trained Transformers (GPTs) using Kolmogorov-Arnold Networks (KANs) for language modeling. It provides a model for generating text based on prompts, with a focus on improving performance compared to traditional MLP-GPT models. The repository includes scripts for training the model, downloading datasets, and evaluating model performance. Development tasks include integrating with other libraries, testing, and documentation.
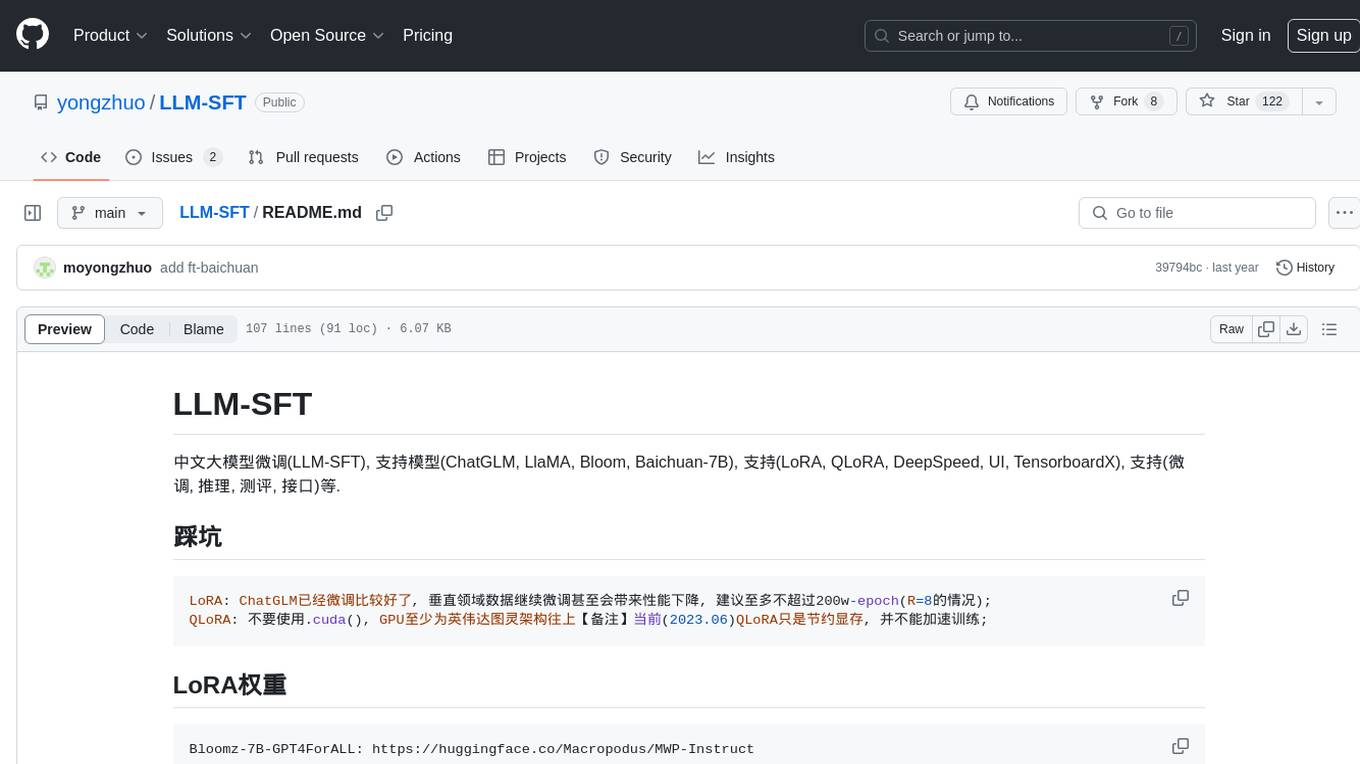
LLM-SFT
LLM-SFT is a Chinese large model fine-tuning tool that supports models such as ChatGLM, LlaMA, Bloom, Baichuan-7B, and frameworks like LoRA, QLoRA, DeepSpeed, UI, and TensorboardX. It facilitates tasks like fine-tuning, inference, evaluation, and API integration. The tool provides pre-trained weights for various models and datasets for Chinese language processing. It requires specific versions of libraries like transformers and torch for different functionalities.
For similar jobs
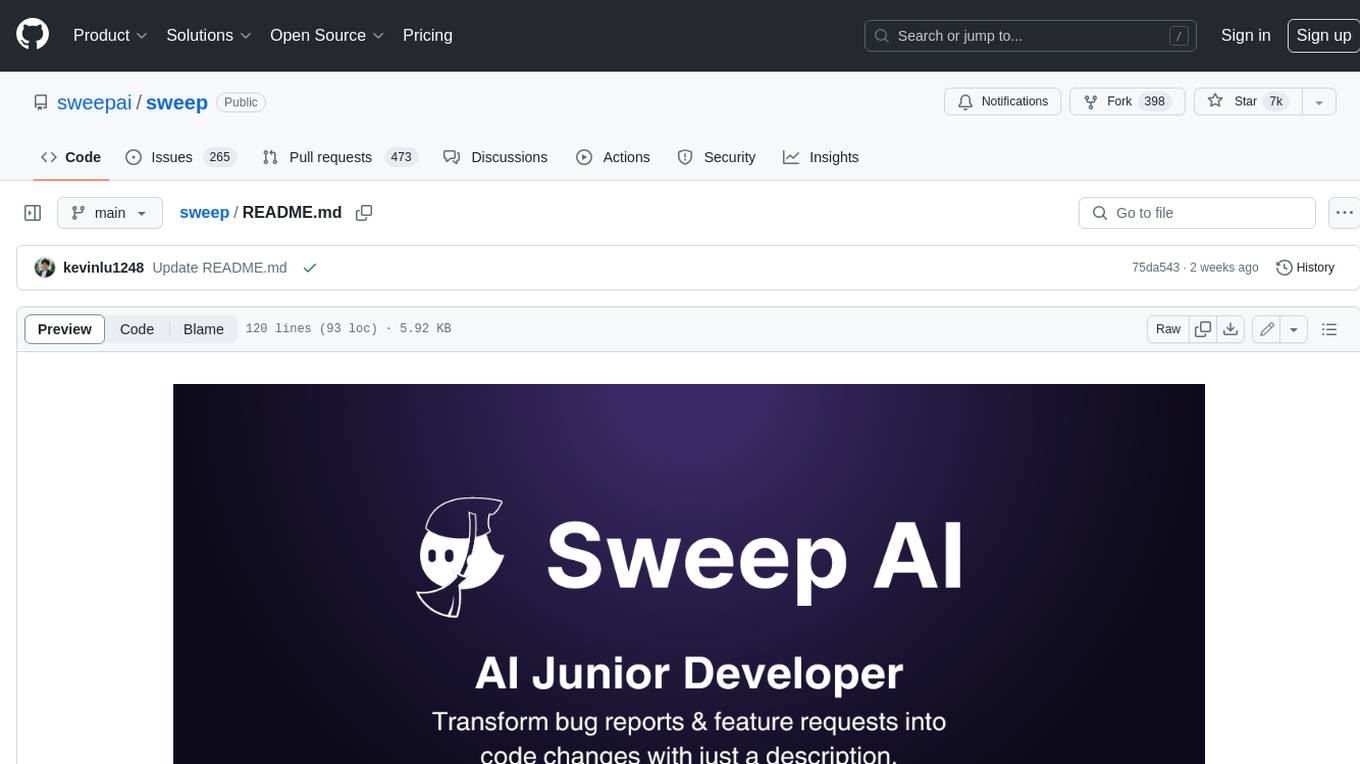
sweep
Sweep is an AI junior developer that turns bugs and feature requests into code changes. It automatically handles developer experience improvements like adding type hints and improving test coverage.
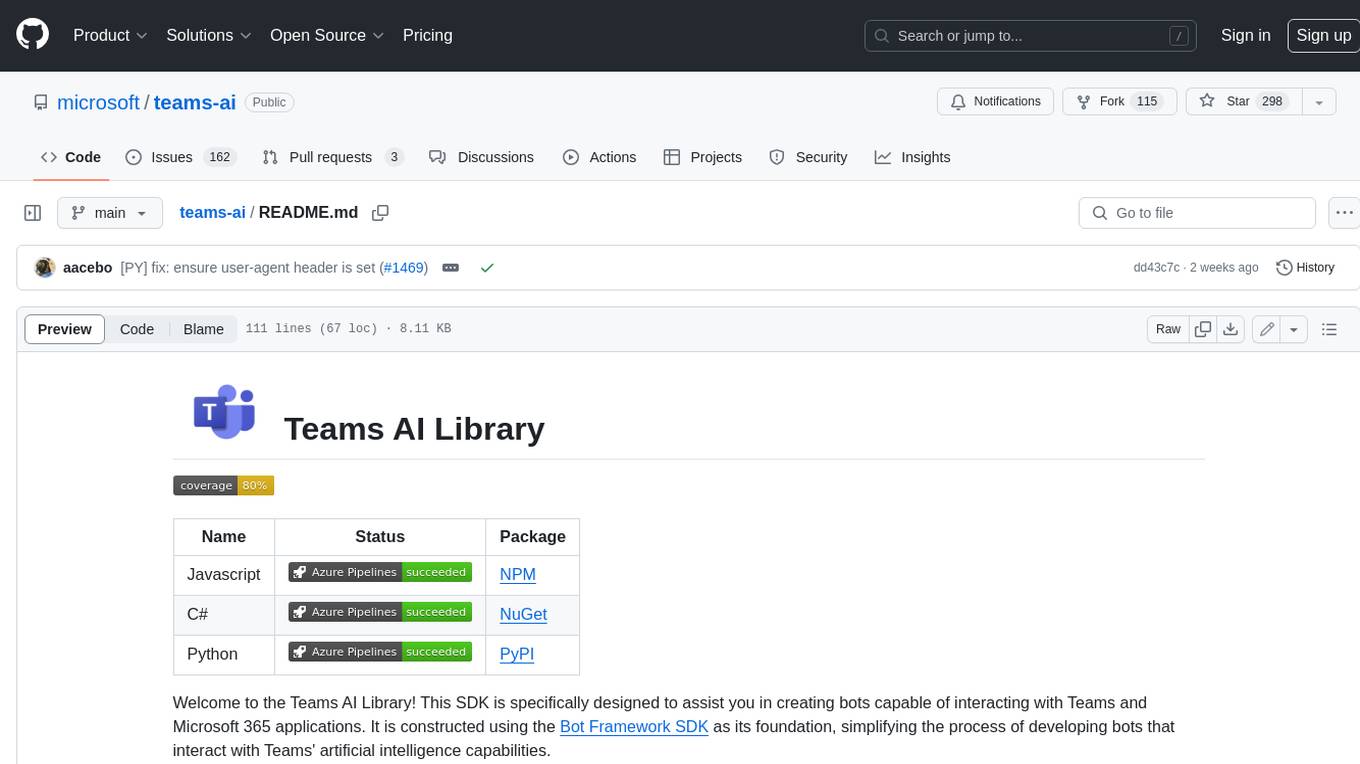
teams-ai
The Teams AI Library is a software development kit (SDK) that helps developers create bots that can interact with Teams and Microsoft 365 applications. It is built on top of the Bot Framework SDK and simplifies the process of developing bots that interact with Teams' artificial intelligence capabilities. The SDK is available for JavaScript/TypeScript, .NET, and Python.
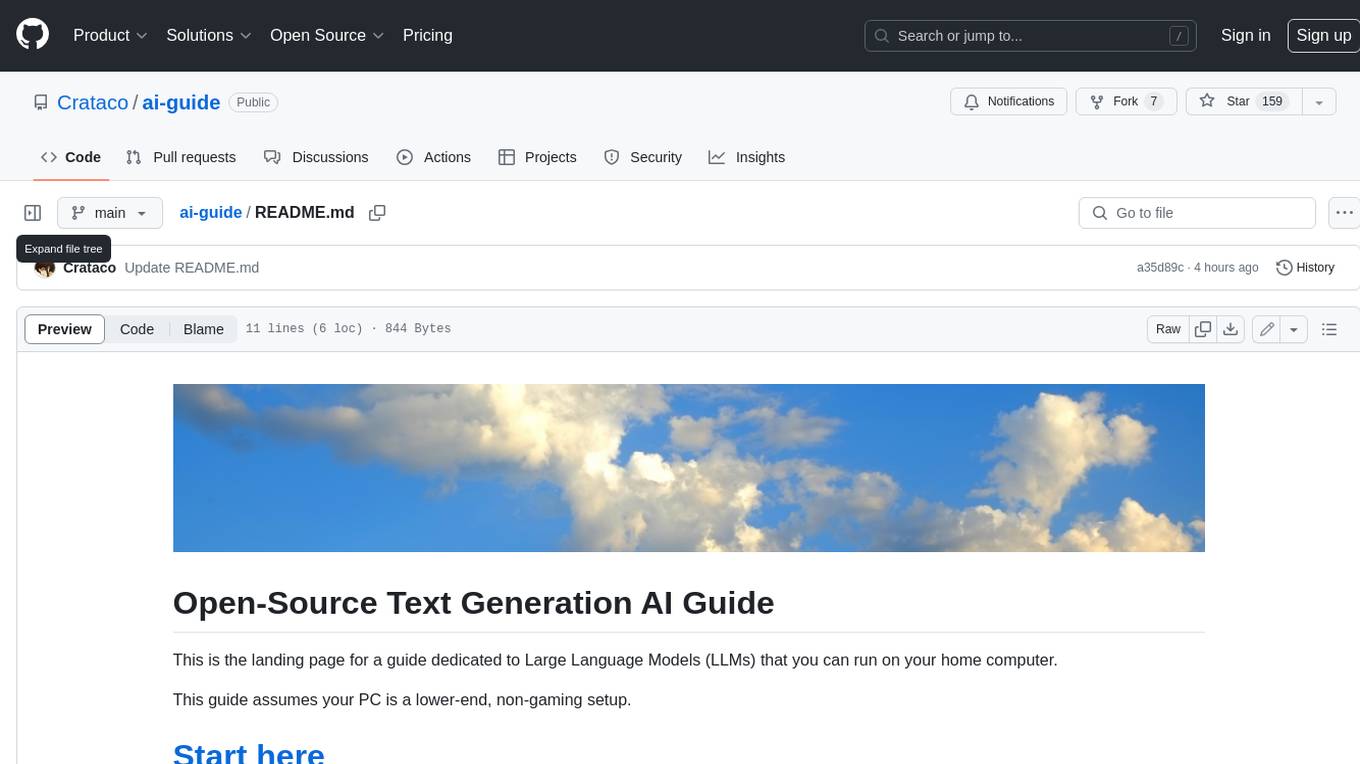
ai-guide
This guide is dedicated to Large Language Models (LLMs) that you can run on your home computer. It assumes your PC is a lower-end, non-gaming setup.
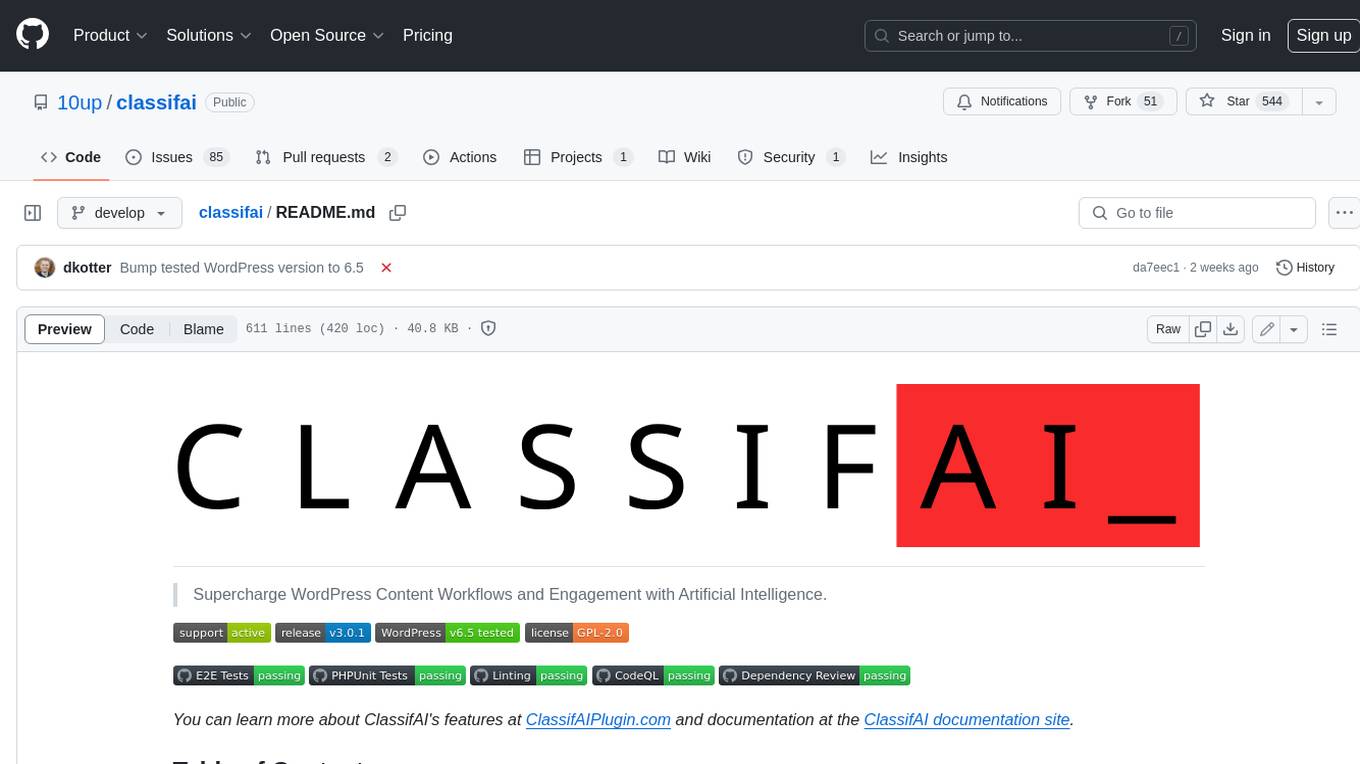
classifai
Supercharge WordPress Content Workflows and Engagement with Artificial Intelligence. Tap into leading cloud-based services like OpenAI, Microsoft Azure AI, Google Gemini and IBM Watson to augment your WordPress-powered websites. Publish content faster while improving SEO performance and increasing audience engagement. ClassifAI integrates Artificial Intelligence and Machine Learning technologies to lighten your workload and eliminate tedious tasks, giving you more time to create original content that matters.
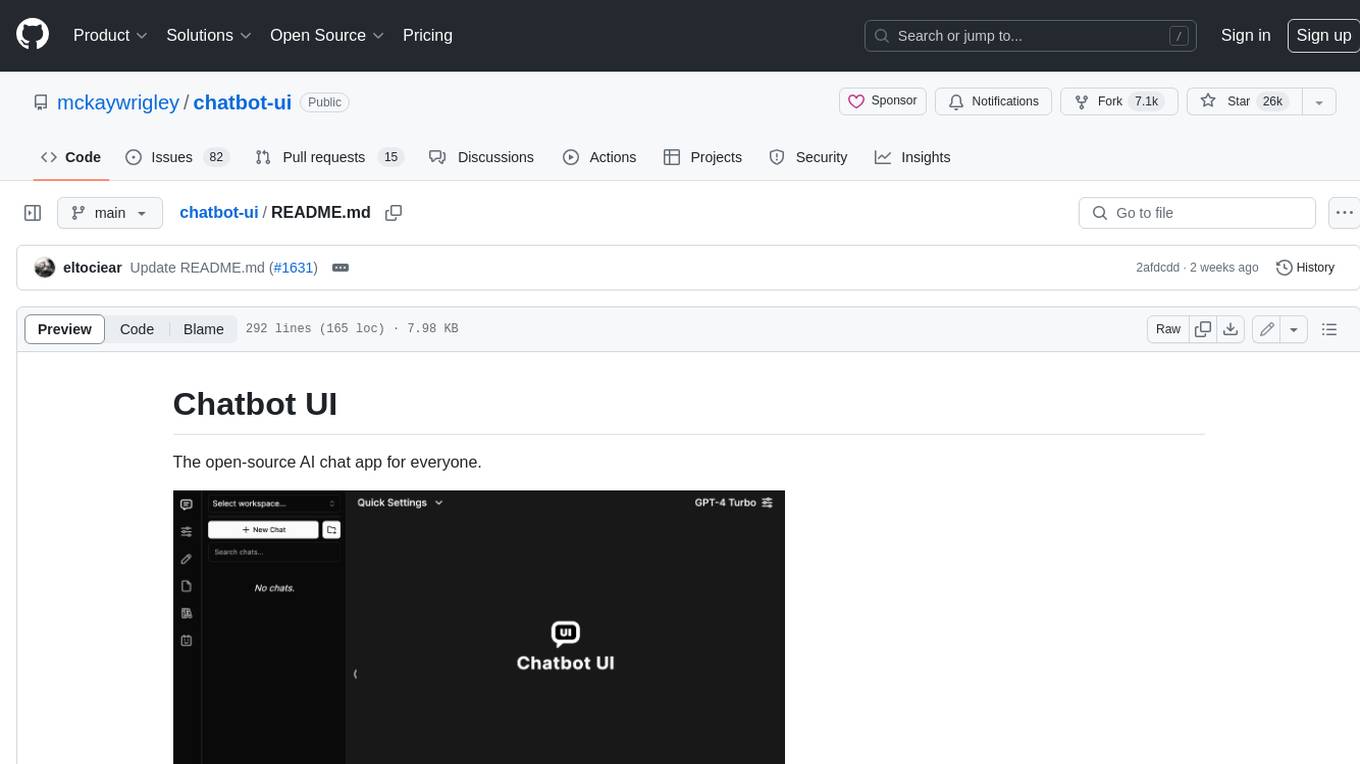
chatbot-ui
Chatbot UI is an open-source AI chat app that allows users to create and deploy their own AI chatbots. It is easy to use and can be customized to fit any need. Chatbot UI is perfect for businesses, developers, and anyone who wants to create a chatbot.
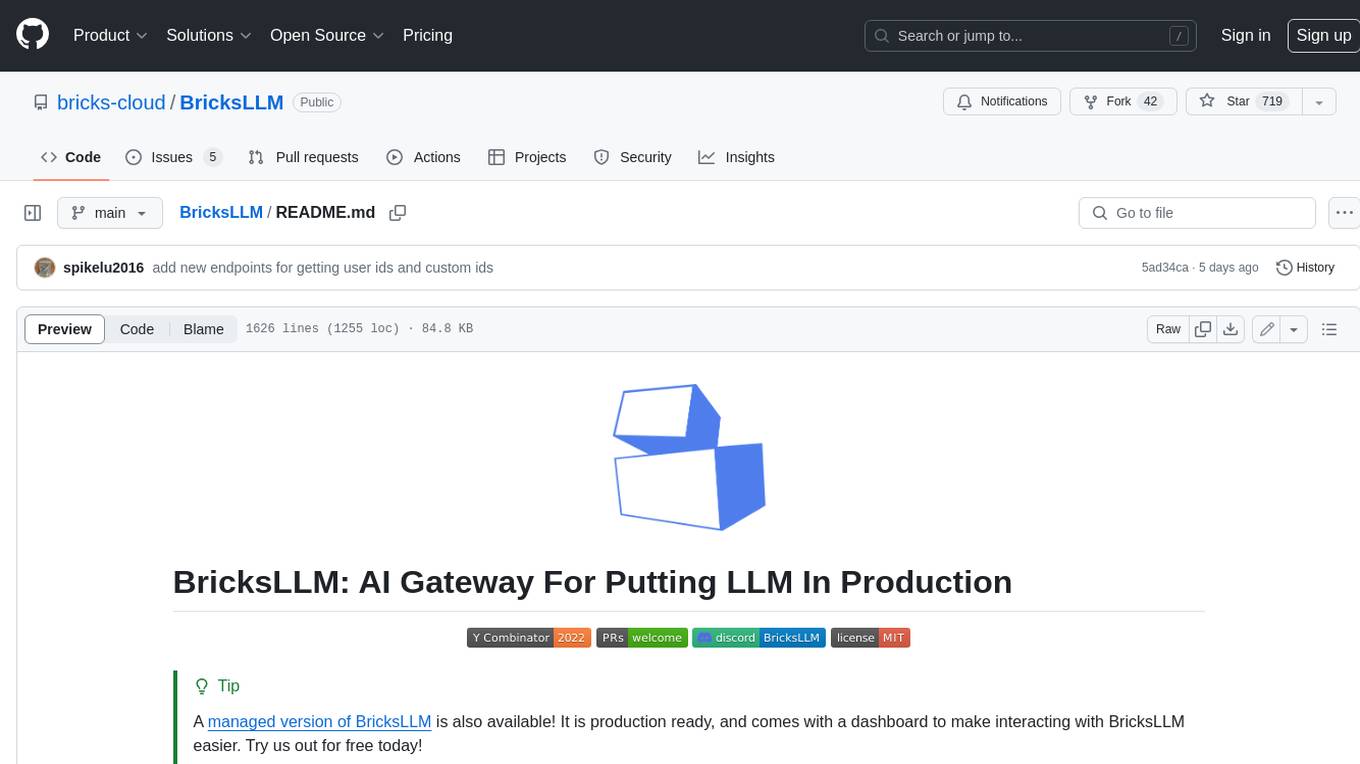
BricksLLM
BricksLLM is a cloud native AI gateway written in Go. Currently, it provides native support for OpenAI, Anthropic, Azure OpenAI and vLLM. BricksLLM aims to provide enterprise level infrastructure that can power any LLM production use cases. Here are some use cases for BricksLLM: * Set LLM usage limits for users on different pricing tiers * Track LLM usage on a per user and per organization basis * Block or redact requests containing PIIs * Improve LLM reliability with failovers, retries and caching * Distribute API keys with rate limits and cost limits for internal development/production use cases * Distribute API keys with rate limits and cost limits for students
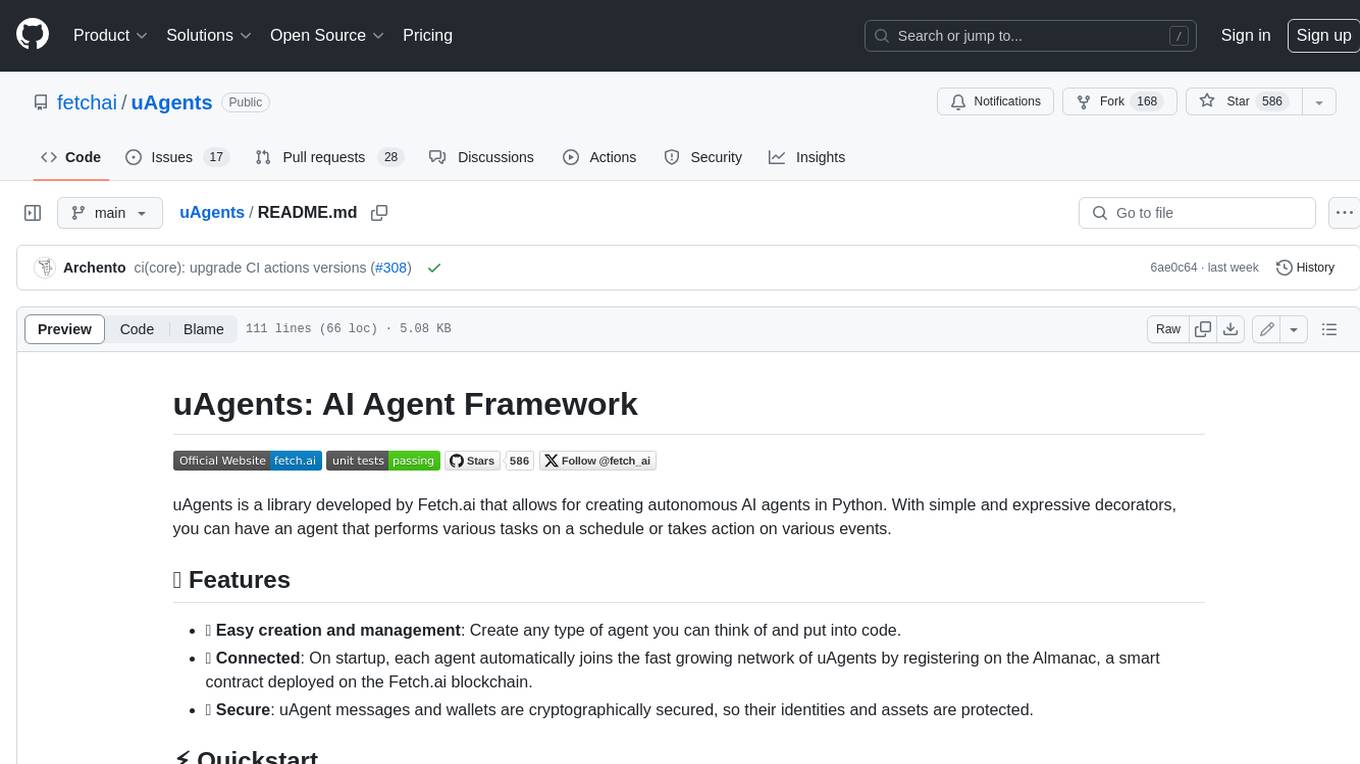
uAgents
uAgents is a Python library developed by Fetch.ai that allows for the creation of autonomous AI agents. These agents can perform various tasks on a schedule or take action on various events. uAgents are easy to create and manage, and they are connected to a fast-growing network of other uAgents. They are also secure, with cryptographically secured messages and wallets.
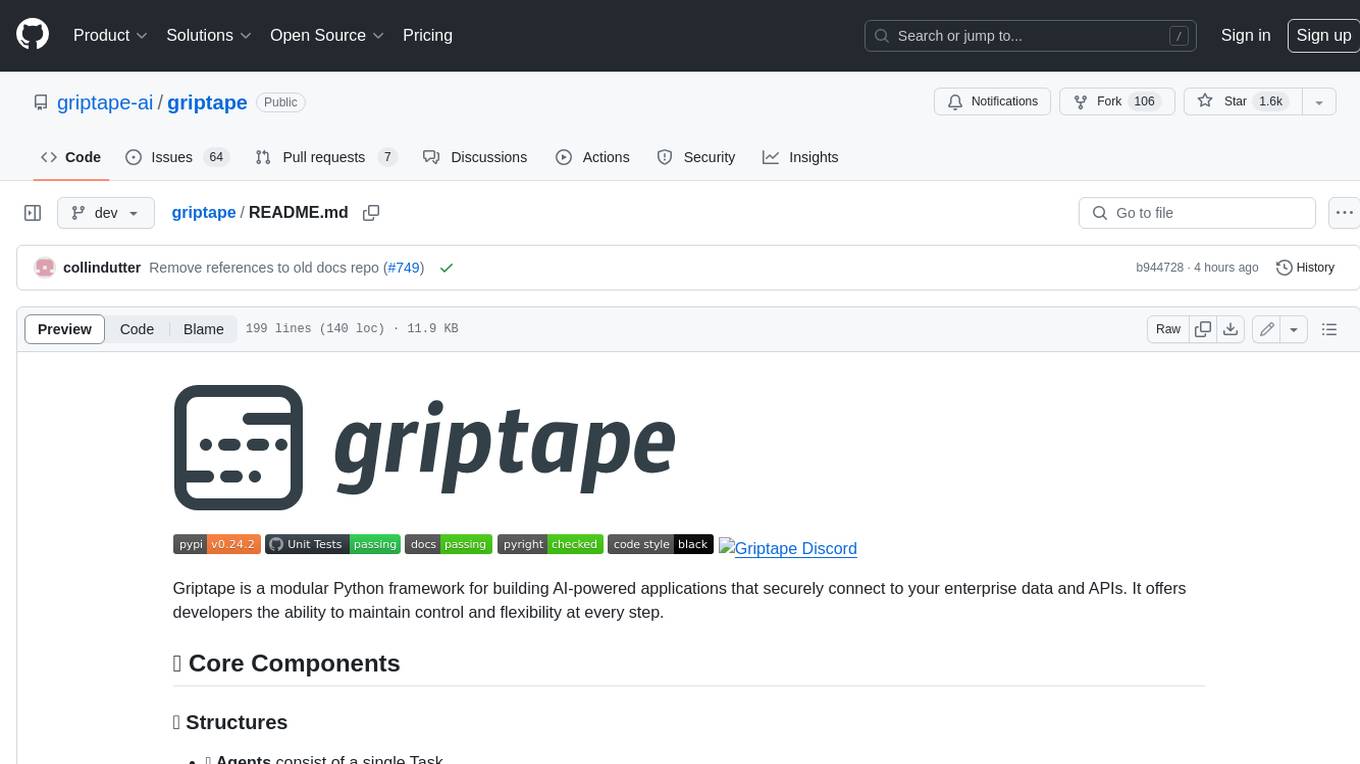
griptape
Griptape is a modular Python framework for building AI-powered applications that securely connect to your enterprise data and APIs. It offers developers the ability to maintain control and flexibility at every step. Griptape's core components include Structures (Agents, Pipelines, and Workflows), Tasks, Tools, Memory (Conversation Memory, Task Memory, and Meta Memory), Drivers (Prompt and Embedding Drivers, Vector Store Drivers, Image Generation Drivers, Image Query Drivers, SQL Drivers, Web Scraper Drivers, and Conversation Memory Drivers), Engines (Query Engines, Extraction Engines, Summary Engines, Image Generation Engines, and Image Query Engines), and additional components (Rulesets, Loaders, Artifacts, Chunkers, and Tokenizers). Griptape enables developers to create AI-powered applications with ease and efficiency.