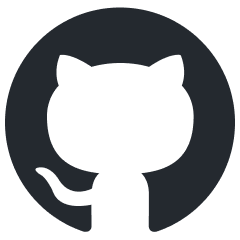
openai-chat-api-workflow
π© An Alfred 5 Workflow for using OpenAI Chat API to interact with GPT models π€π¬ It also allows image generation πΌοΈ, image understanding π, speech-to-text conversion π€, and text-to-speech synthesis π
Stars: 308
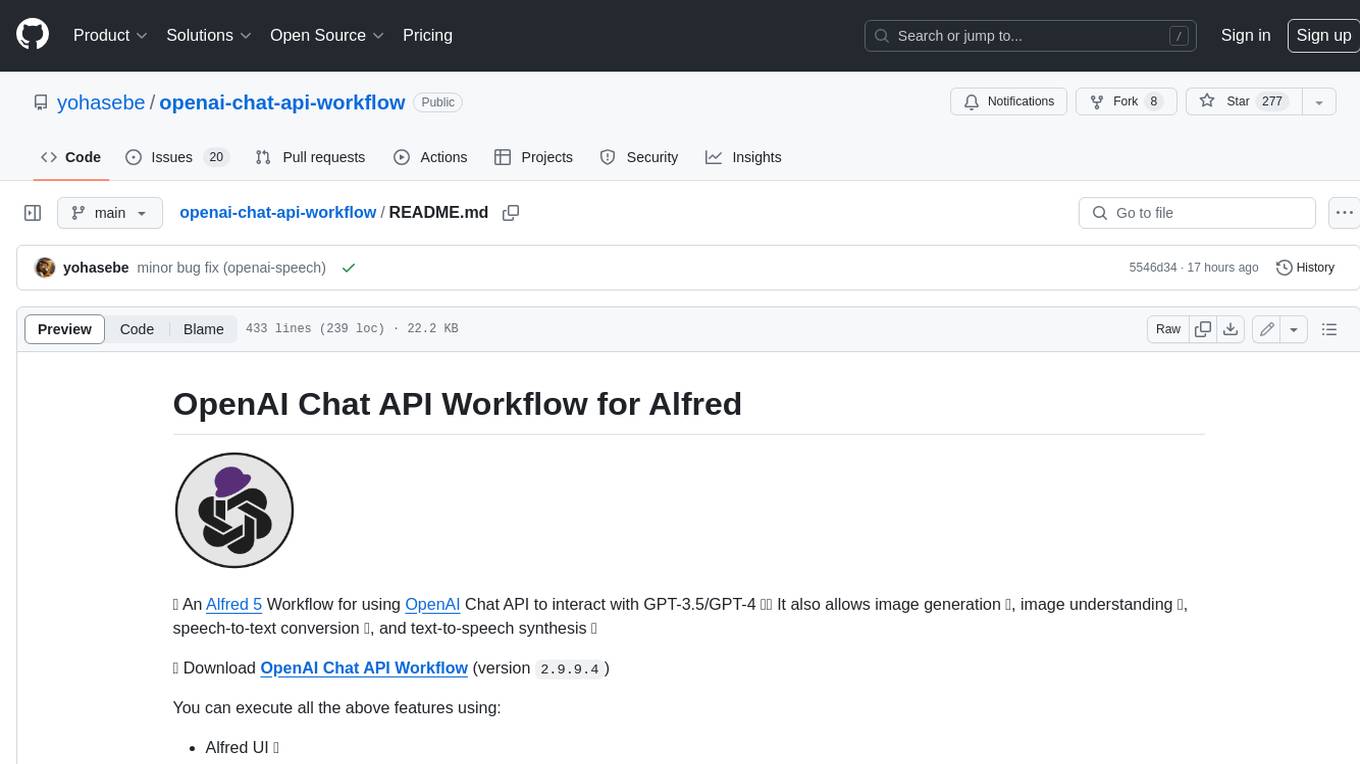
**OpenAI Chat API Workflow for Alfred** An Alfred 5 Workflow for using OpenAI Chat API to interact with GPT-3.5/GPT-4 π€π¬ It also allows image generation πΌοΈ, image understanding π, speech-to-text conversion π€, and text-to-speech synthesis π **Features:** * Execute all features using Alfred UI, selected text, or a dedicated web UI * Web UI is constructed by the workflow and runs locally on your Mac π» * API call is made directly between the workflow and OpenAI, ensuring your chat messages are not shared online with anyone other than OpenAI π * OpenAI does not use the data from the API Platform for training π« * Export chat data to a simple JSON format external file π * Continue the chat by importing the exported data later π
README:
π© An Alfred 5 Workflow for using the OpenAI Chat API to interact with GPT models π€π¬. It also allows image generation πΌοΈ, image understanding π, speech-to-text conversion π€, and text-to-speech synthesis π.
π¦ Download OpenAI Chat API Workflow (version 3.4.1
)
You can execute all the above features using:
- Alfred UI π₯οΈ
- Selected text π
- A dedicated web UI π
The web UI is constructed by the workflow and runs locally on your Mac π». The API call is made directly between the workflow and OpenAI, ensuring your chat messages are not shared online with anyone other than OpenAI π. Furthermore, OpenAI does not use the data from the API Platform for training π«.
You can export the chat data to a simple JSON format external file π, and it is possible to continue the chat by importing it later π.
- Install Homebrew
- Run the following command in a terminal:
brew install pandoc mpv sox jq duti
- Download and run OpenAI Chat API Workflow
- Set your OpenAI API key
- Enable accessibility settings for Alfred in
System Preferences
βSecurity & Privacy
βPrivacy
βAccessibility
Setup Hotkeys
You can set up hotkeys in the settings screen of the workflow. To set up hotkeys, double-click on the light purple workflow elements.
- Open Web UI (Recommended)
- Direct Query
- Send Selected Text
- Screen Capture for Image Understanding
- Speech to Text
Dependencies
- Alfred 5 Powerpack
- OpenAI API key
- Pandoc: to convert Markdown to HTML
- MPV: to play text-to-speech audio stream
- Sox: to record voice input
- jq: to handle chat history in JSON
- duti: to detect the default web browser
To start using this workflow, you must set the environment variable apikey
, which you can obtain by creating a new OpenAI account. See also the Configuration section below.
You will also need to install the pandoc
and sox
programs. Pandoc allows this workflow to convert the Markdown response from OpenAI to HTML and display the result in your default web browser with syntax highlighting enabled (especially useful when using this workflow to generate program code). Sox allows you to record voice audio to convert to text using the Whisper speech-to-text API.
To set up dependencies (pandoc
, mpv
, sox
, jq
, and duti
), first install Homebrew and run the following command:
brew install pandoc mpv sox jq duti
Change Log
Recent Change Log
- 3.4.1:
- New TTS voices added (
ash
,coral
, andsage
) - Old models before
gpt-4o
are removed - UI improvements
- Image upload from the web UI improved
- New TTS voices added (
- 3.3.0: Import image feature is added to the web UI
- 3.2.3: Added support for
gpt-4o-2024-11-20
model - 3.2.2: Streaming support for
o1
series models - 3.2.1: Fixed URI encoding issue when re-editing prompt
- 3.2.0: Added support for Beta models (
o1-preview
,o1-mini
)
Here are three methods to run the workflow: 1) Using commands within the Alfred UI, 2) Passing selected text to the workflow, 3) Utilizing the Web UI. Additionally, thereβs a convenient method for making brief inquiries to GPT.
Commands within the Alfred UI
You can enter a query directly into Alfred's textbox:
- Method 1: Alfred textbox β keyword (
openai
) β space/tab β input query β select a command (see below) - Method 2: Alfred textbox β input query β select fallback search (
OpenAI Query
)
Passing Selected Text
You can select any text on your Mac and send it to the workflow:
- Method 1: Select text β universal action hotkey β select
OpenAI Query
- Method 2: Set up a custom hotkey to
Send selected text to OpenAI
Using Web Interface
You can open the web interface:
- Method 1: Alfred textbox β keyword (
openai-webui
) - Method 2: Set up a custom hotkey to
Open web interface
Using the Default Browser
If your default browser is set to one of the following and the duti command is installed on your system, the web interface will automatically open in your chosen browser. If not, Safari will be used as the default.
- Google Chrome (Stable, Beta, Dev, etc.)
- Microsoft Edge (Stable, Beta, Dev, etc.)
- Brave Browser
Restart the OpenAI Workflow server by executing openai-restart-server
in case the web UI does not work as expected after changing the default browser.
Web UI Modes
Switch modes (light
/dark
/auto
) with the Web UI Mode
selector in the settings.
Simple Direct Query/Chat
To quickly chat with GPT:
- Method 1: Type keyword
gpt
β space/tab β input query (e.g., "gpt what is a large language model?") - Method 2: Set up a custom hotkey to
OpenAI Direct Query
With Direct Query
, the input text is sent directly to the OpenAI Chat API as a prompt. You can also create a query by prepending or appending text to the input.
The input text is directly sent as a prompt to the OpenAI Chat API.
After entering the initial text, you are prompted for additional text. The additional text is added before the initial text, and the resulting text is used as the query.
After entering the initial text, you are prompted for additional text. The additional text is added after the initial text, and the resulting text is used as the query.
The DALL-E API (dall-e-3
or dall-e-2
) is used to generate images based on the entered prompts. See Image Generation below.
Some of the examples shown on OpenAI's Examples page are incorporated into this Workflow as commands. Functions not prepared as commands can be realized by giving appropriate prompts to the above Basic Commands.
GPT generates program code and example output based on the entered text. You can specify the purpose of the program, its function, the language, and the technology to be used, etc.
Example Input
Create a command line program that takes an English sentence and returns syntactically parsed output. Provide program code in Python and example usage.
Example Output
You can ask questions in the language set to the variable first_language
.
Note: If the value of first_language
is not English
(e.g., Japanese
), the query may result in a less accurate response.
GPT translates text from the language specified in the variable first_language
to the language specified in second_language
.
GPT translates text from the language specified in the variable second_language
to the language specified in first_language
.
GPT corrects sentences that may contain grammatical errors. See OpenAI's description.
GPT assists you in brainstorming innovative ideas based on any given text.
GPT provides study notes on a given topic. See OpenAI's description for this example.
GPT creates analogies. See OpenAI's description for this example.
GPT generates an outline for a research topic. See OpenAI's description for this example.
GPT summarizes a given text. See OpenAI's description for this example.
GPT translates complex text into more straightforward concepts. See OpenAI's description for this example.
GPT extracts keywords from a block of text. See OpenAI's description for this example.
Image generation can be executed through one of the above commands. It is also possible to use the web UI. By using the web UI, you can interactively change the prompt to get closer to the desired image.
When the image generation mode is set to dall-e-3
, the user's prompt is automatically expanded to a more detailed and specific prompt. You can also edit the expanded prompt and regenerate the image.
Image understanding can be executed through the openai-vision
command. It starts capture mode and lets you specify a part of the screen to be analyzed. Alternatively, you can specify an image file (jpg, jpeg, png, gif) using the "OpenAI Vision" file action. This mode requires the gpt-4o
or gpt-4o-mini
model to be set in the workflow settings.
Alternatively, you can use the web UI to upload an image file for analysis. The image file is sent to the OpenAI Vision API, and the result is displayed in the web UI.
Most text-to-speech and speech-to-text features are available on the web UI. However, there are certain specific features provided as commands, such as audio file to text conversion and transcription with timestamps.
Text-to-Speech Synthesis
Text entered or response text from GPT can be read out in a natural voice using OpenAI's text-to-speech API.
- Method 1: Press the
Play TTS
button on the web UI - Method 2: Select text β universal action hotkey β select
OpenAI Text-to-Speech
Speech-to-Text Conversion
The Whisper API can convert speech into text in a variety of languages. Please refer to the Whisper API FAQ for available languages and other limitations.
- Method 1: Press the
Voice Input
button on the web UI - Method 2: Alfred textbox β keyword (
openai-speech
)
Audio File to Text
You can select an audio file in mp3
, mp4
, flac
, webm
, wav
, or m4a
format (under 25MB) and send it to the workflow:
- Select the file β universal action hotkey β select
OpenAI Speech-to-Text
Record Voice Audio and Transcribe
You can record voice audio and send it to the Workflow for transcription using the Whisper API. Recording time is limited to 30 minutes and will automatically stop after this duration.
-
Alfred textbox β keyword (
openai-speech
) β Terminal window opens and recording starts -
Speak into the internal or external microphone β Press Enter to finish recording
-
Choose processes to apply to the recorded audio:
- Transcribe (+ delete recording)
- Transcribe (+ save recording to desktop)
- Transcribe and query (+ delete recording)
- Transcribe and query (+ save recording to desktop)
- Exit (+ delete recording)
- Exit (+ save recording to desktop)
You can choose the format of the transcribed text as text
, srt
, or vtt
in the workflow's settings. Below are examples in the text
and srt
formats:
Import/Export
You can export your chat data to a straightforward JSON format file and resume your conversation later by importing it back in.
To export data, simply click on Show Entire Chat
in the chat window to navigate to the chat history page, then select Export Data
. To import data, just click Import Data
on either the home page or the chat history page.
Monitor API Usage
To review your token usage for the current billing cycle on the OpenAI Usage Page, type the keyword openai-usage
. For more details on billing, visit OpenAI's Billing Overview.
You can set various parameters in the settings panel of this Workflow. Some of the parameters set here are used as default values, but you can make temporary changes to the values on the web UI. You can also access the settings panel by clicking Open Config
from the web UI.
Required Settings
- OpenAI API Key: Set your secret API key for OpenAI. Sign up for OpenAI and get your API key at https://platform.openai.com/account/api-keys/.
-
Base URL: The base URL of the OpenAI Chat API. (default:
https://api.openai.com/v1
)
Web UI Parameters
-
Loopback Address: Either
localhost
or127.0.0.1
can be used as the loopback address of the UI server. If the web UI does not work as expected, try the other. (default:127.0.0.1
) -
Stream Output: Show results in the default web browser. If unchecked, Alfred's "Large Type" feature is used to display the result. (default:
enabled
) - Hide Speech Buttons: When enabled, the buttons for TTS playback and voice input are hidden on the web UI.
-
Web UI Mode: Set your preferred UI mode (
light
/dark
/auto
). (default:auto
)
Chat Parameters
-
Model: OpenAI's chat model used for the workflow (default:
gpt-4o-mini
). Here are some of the models currently available:gpt-4o-mini
chatgpt-4o-latest
gpt-4o-2024-08-06
gpt-4o
You may or may not use the following beta models. System prompt and parameter settings are not available for these models.
o1-preview
o1-mini
-
Max Tokens: Maximum number of tokens to be generated upon completion (default:
2048
). If this parameter is set to0
,null
is sent to the API as the default value (the maximum number of tokens is not specified). See OpenAI's documentation. -
Temperature: See OpenAI's documentation. (default:
0.3
) -
Top P: See OpenAI's documentation. (default:
1.0
) -
Frequency Penalty: See OpenAI's documentation. (default:
0.0
) -
Presence Penalty: See OpenAI's documentation. (default:
0.0
) -
Memory Span: Set the number of past utterances sent to the API as context. Setting
4
for this parameter means 2 conversation turns (user β assistant β user β assistant) will be sent as context for a new query. The larger the value, the more tokens will be consumed. (default:10
) -
Max Characters: Maximum number of characters that can be included in a query (default:
50000
). -
Timeout: The number of seconds (default:
10
) to wait before opening the socket and connecting to the API. If the connection fails, reconnection (up to 20 times) will be attempted after 1 second. -
Add Emoji: If enabled, the response text from GPT will contain emoji characters appropriate for the content. This is realized by adding the following sentence at the end of the system content. (default:
enabled
)Add emojis that are appropriate to the content of the response.
-
System Content: Text to send with every query sent to the API as general information about the specification of the chat. The default value is as follows:
You are a friendly but professional consultant who answers various questions, makes decent suggestions, and gives helpful advice in response to a prompt from the user. Your response must be concise, suggestive, and accurate.
Image Understanding Parameters
-
Max Size for Image Understanding: The maximum pixel value (
512
to2000
) of the larger side of the image data sent to the image understanding API. Larger images will be resized accordingly. (Default:512
)
Image Generation Parameters
-
Image Generation Model:
dall-e-3
anddall-e-2
are available. (default:dall-e-3
) -
Image Size (for
dall-e-3
): Set the size of images to generate to1024x1024
,1024x1792
, or1792x1024
. (default:1024x1024
) -
Quality (for
dall-e-3
): Choose the quality of the image fromstandard
andhd
. (default:standard
) -
Style (for
dall-e-3
): Choose the style of the image fromvivid
andnatural
. (default:vivid
) -
Number of Images (for
dall-e-2
): Set the number of images to generate in image generation mode from1
to10
. (default:1
) -
Image Size (for
dall-e-2
): Set the size of images to generate to256x256
,512x512
, or1024x1024
. (default:256x256
)
Text-to-Speech Parameters
-
Text-to-Speech Model: One of the available TTS models:
tts-1
ortts-1-hd
. (default:tts-1
) -
Text-to-Speech Voice: The voice to use when generating the audio. Supported voices are:
alloy
,ash
,coral
,echo
,fable
,onyx
,nova
,sage
, andshimmer
. (default:alloy
) -
Text-to-Speech Speed: The speed of the generated audio. Select a value from 0.25 to 4.0. (default:
1.0
) -
Automatic Text to Speech: If enabled, the results will be read aloud using the system's default text-to-speech language and voice. (default:
disabled
)
Speech-to-Text Parameters
-
Transcription Format: Set the format of the text transcribed from the microphone input or audio files to
text
,srt
, orvtt
. (default:text
) -
Processes after Recording: Set the default choice of what processes follow after audio recording finishes. (default:
Transcribe [+ delete recording]
).- Transcribe [+ delete recording]
- Transcribe [+ save recording to desktop]
- Transcribe and query [+ delete recording]
- Transcribe and query [+ save recording to desktop]
-
Audio to English: When enabled, the Whisper API will transcribe the input audio and output text translated into English. (default:
disabled
)
Other Settings
-
Your First Language: Set your first language. This language is used when using GPT for translation. (default:
English
) -
Your Second Language: Set your second language. This language is used when using GPT for translation. (default:
Japanese
) -
Sound: If checked, a notification sound will play when the response is returned. (default:
disabled
) -
Save File Path: If set, the results will be saved in the specified path as a markdown file. (default:
not set
)
Environment Variables
Environment variables can be accessed by clicking the [x]
button located at the top right of the workflow settings screen. Normally, there is no need to change the values of the environment variables.
-
http_keep_alive
: This workflow starts an HTTP server when the web UI is first displayed. After that, if the web UI is not used for the time (in seconds) set by this environment variable, the server will stop. (default:7200
= 2 hours) -
http_port
: Specifies the port number for the web UI. (default:80
) -
http_server_wait
: Specifies the wait time from when the HTTP server is started until the page is displayed in the browser. (default:2.5
) -
websocket_port
: Specifies the port number for websocket communication used to display responses in streaming on the web UI. (default:8080
)
Yoichiro Hasebe ([email protected])
The MIT License
The author assumes no responsibility for any potential damages arising from the use of this software.
For Tasks:
Click tags to check more tools for each tasksFor Jobs:
Alternative AI tools for openai-chat-api-workflow
Similar Open Source Tools
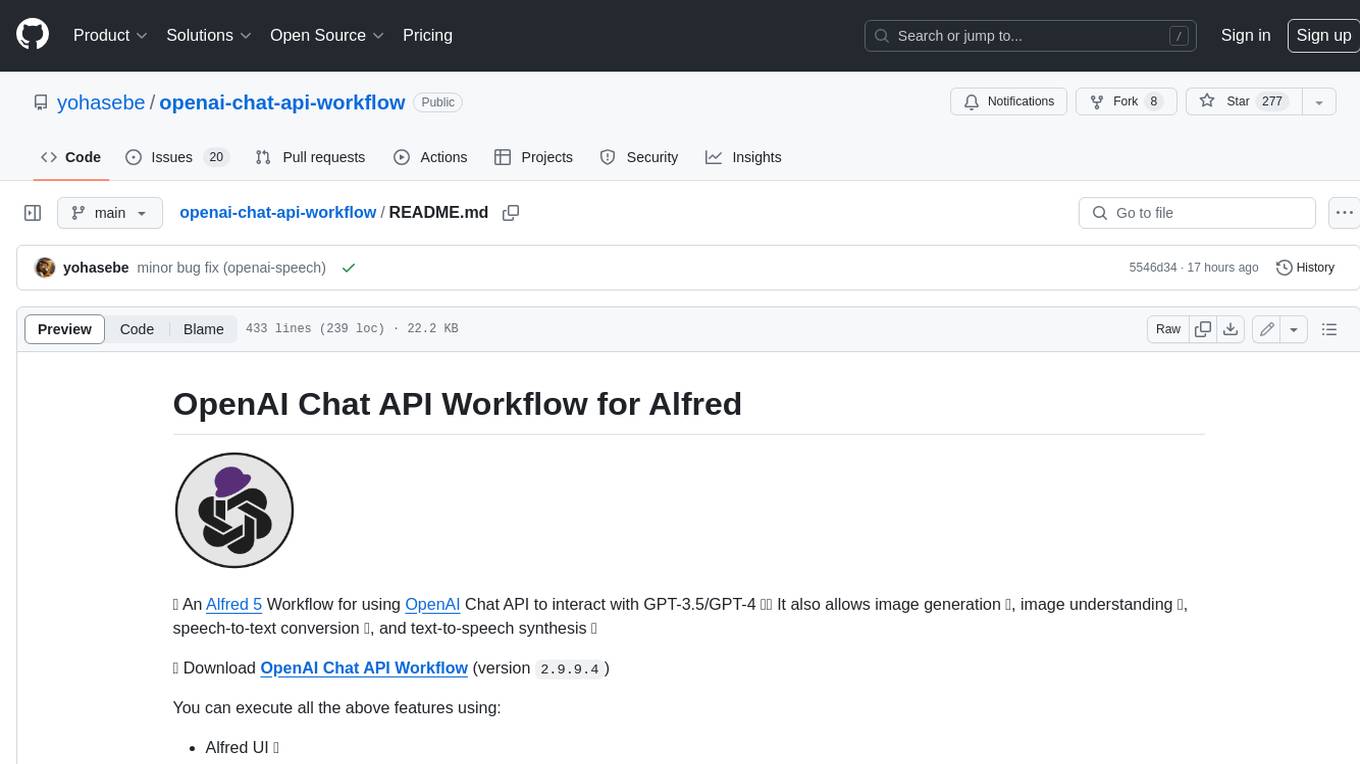
openai-chat-api-workflow
**OpenAI Chat API Workflow for Alfred** An Alfred 5 Workflow for using OpenAI Chat API to interact with GPT-3.5/GPT-4 π€π¬ It also allows image generation πΌοΈ, image understanding π, speech-to-text conversion π€, and text-to-speech synthesis π **Features:** * Execute all features using Alfred UI, selected text, or a dedicated web UI * Web UI is constructed by the workflow and runs locally on your Mac π» * API call is made directly between the workflow and OpenAI, ensuring your chat messages are not shared online with anyone other than OpenAI π * OpenAI does not use the data from the API Platform for training π« * Export chat data to a simple JSON format external file π * Continue the chat by importing the exported data later π
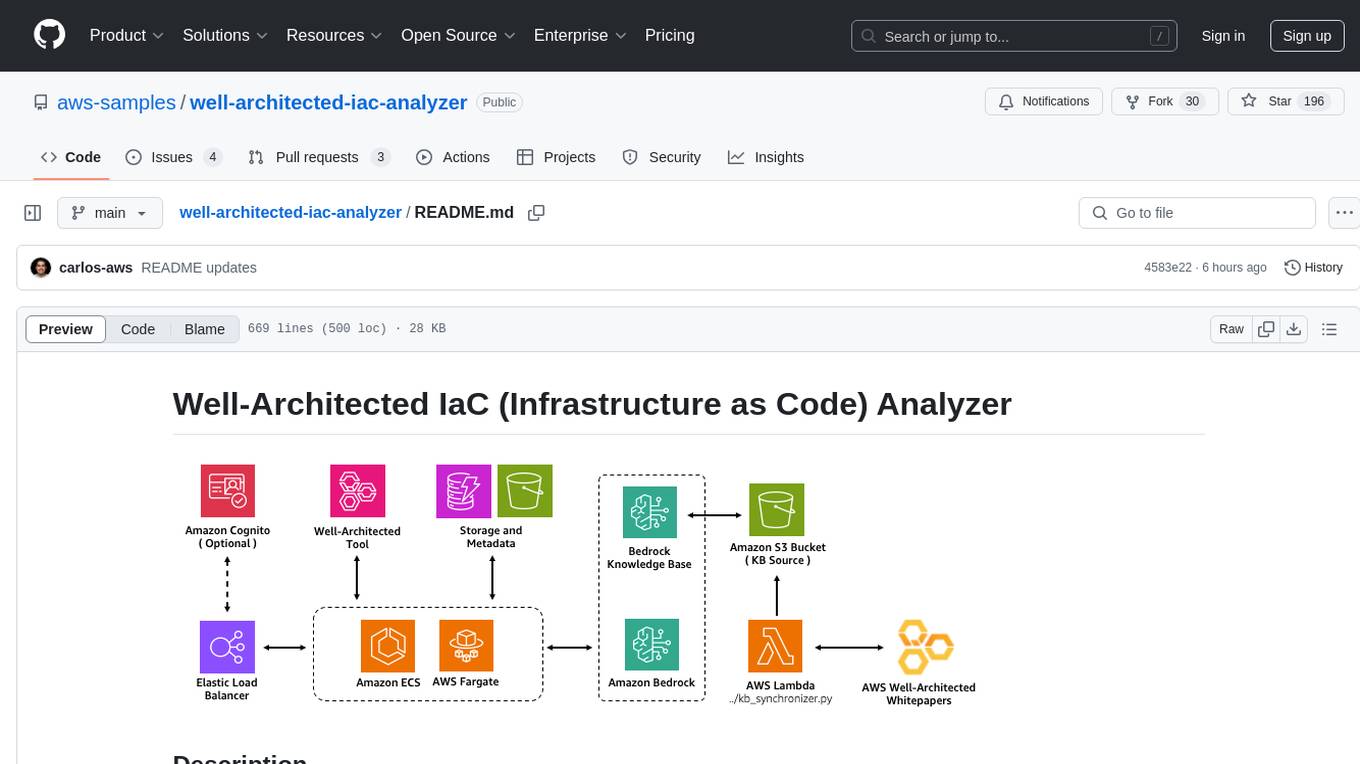
well-architected-iac-analyzer
Well-Architected Infrastructure as Code (IaC) Analyzer is a project demonstrating how generative AI can evaluate infrastructure code for alignment with best practices. It features a modern web application allowing users to upload IaC documents, complete IaC projects, or architecture diagrams for assessment. The tool provides insights into infrastructure code alignment with AWS best practices, offers suggestions for improving cloud architecture designs, and can generate IaC templates from architecture diagrams. Users can analyze CloudFormation, Terraform, or AWS CDK templates, architecture diagrams in PNG or JPEG format, and complete IaC projects with supporting documents. Real-time analysis against Well-Architected best practices, integration with AWS Well-Architected Tool, and export of analysis results and recommendations are included.
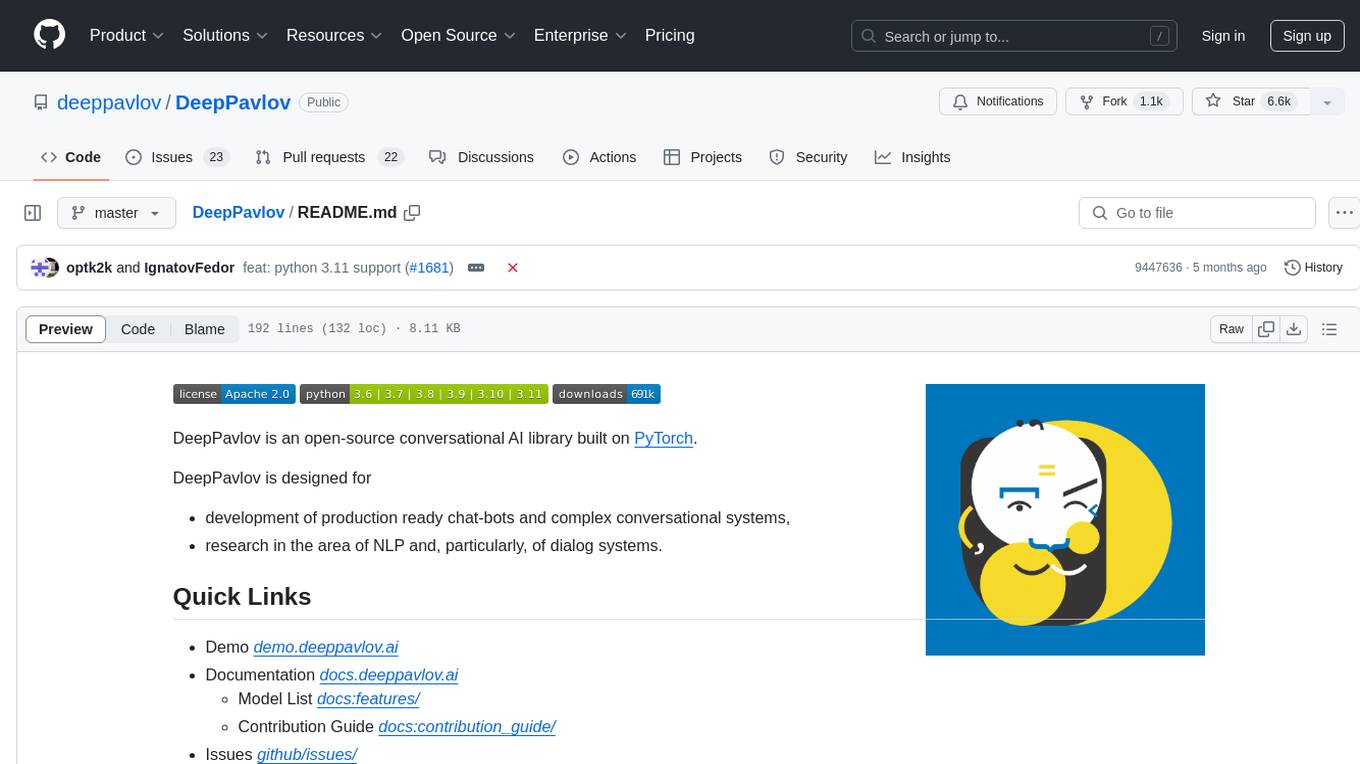
DeepPavlov
DeepPavlov is an open-source conversational AI library built on PyTorch. It is designed for the development of production-ready chatbots and complex conversational systems, as well as for research in the area of NLP and dialog systems. The library offers a wide range of models for tasks such as Named Entity Recognition, Intent/Sentence Classification, Question Answering, Sentence Similarity/Ranking, Syntactic Parsing, and more. DeepPavlov also provides embeddings like BERT, ELMo, and FastText for various languages, along with AutoML capabilities and integrations with REST API, Socket API, and Amazon AWS.
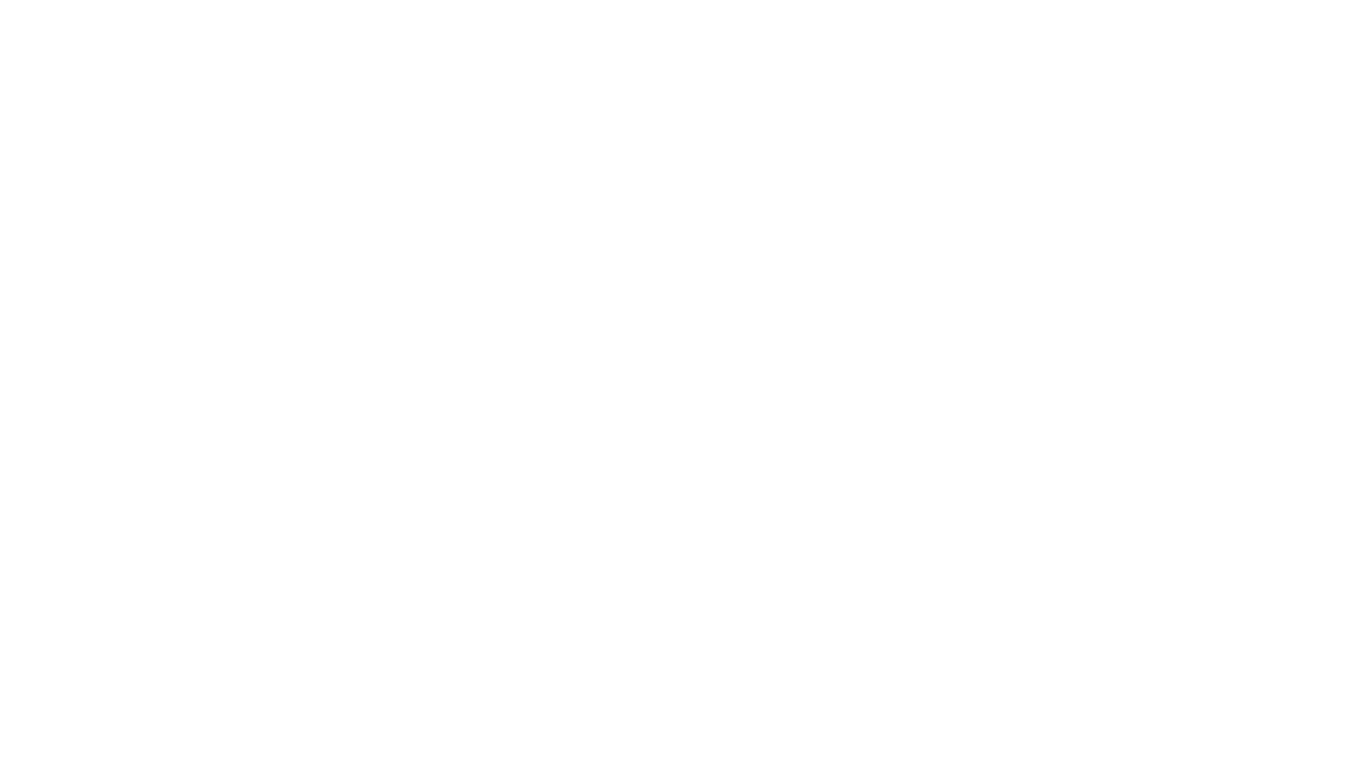
shellChatGPT
ShellChatGPT is a shell wrapper for OpenAI's ChatGPT, DALL-E, Whisper, and TTS, featuring integration with LocalAI, Ollama, Gemini, Mistral, Groq, and GitHub Models. It provides text and chat completions, vision, reasoning, and audio models, voice-in and voice-out chatting mode, text editor interface, markdown rendering support, session management, instruction prompt manager, integration with various service providers, command line completion, file picker dialogs, color scheme personalization, stdin and text file input support, and compatibility with Linux, FreeBSD, MacOS, and Termux for a responsive experience.
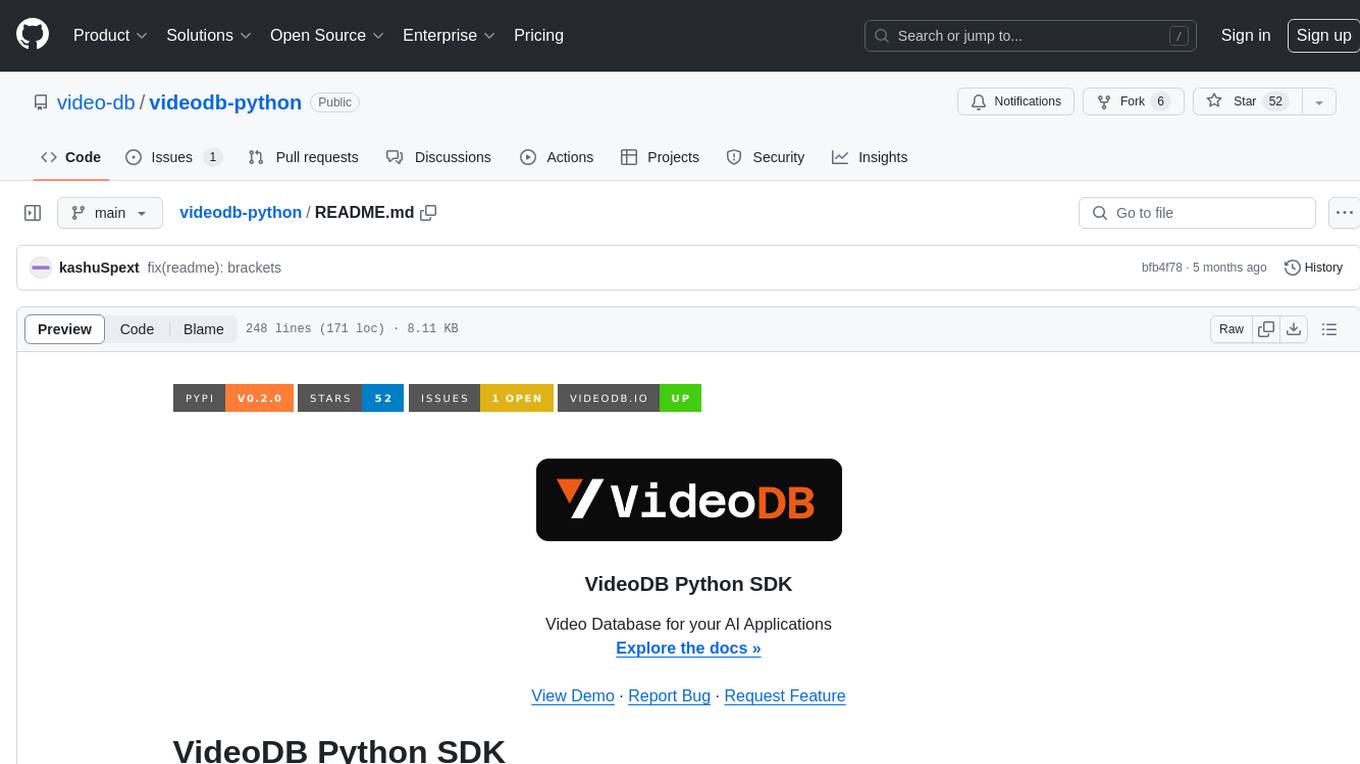
videodb-python
VideoDB Python SDK allows you to interact with the VideoDB serverless database. Manage videos as intelligent data, not files. It's scalable, cost-efficient & optimized for AI applications and LLM integration. The SDK provides functionalities for uploading videos, viewing videos, streaming specific sections of videos, searching inside a video, searching inside multiple videos in a collection, adding subtitles to a video, generating thumbnails, and more. It also offers features like indexing videos by spoken words, semantic indexing, and future indexing options for scenes, faces, and specific domains like sports. The SDK aims to simplify video management and enhance AI applications with video data.
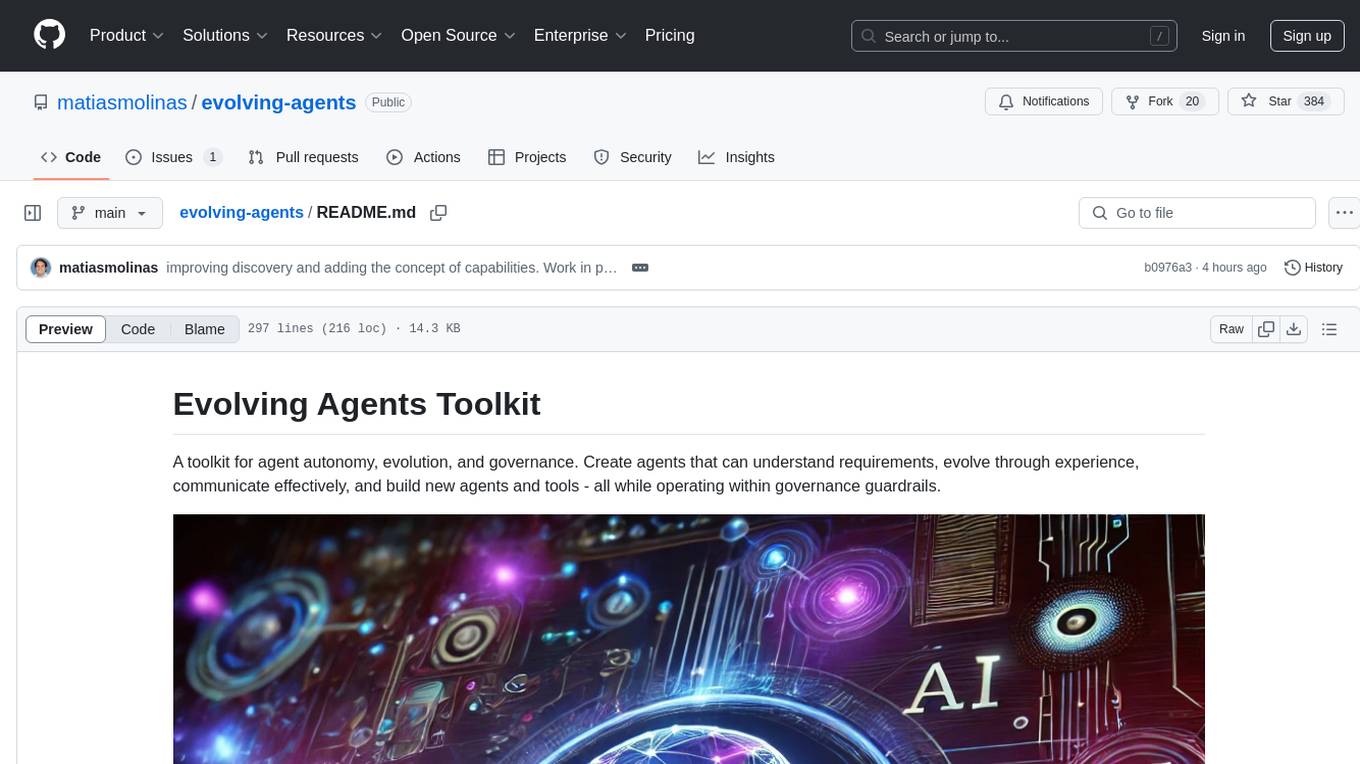
evolving-agents
A toolkit for agent autonomy, evolution, and governance enabling agents to learn from experience, collaborate, communicate, and build new tools within governance guardrails. It focuses on autonomous evolution, agent self-discovery, governance firmware, self-building systems, and agent-centric architecture. The toolkit leverages existing frameworks to enable agent autonomy and self-governance, moving towards truly autonomous AI systems.
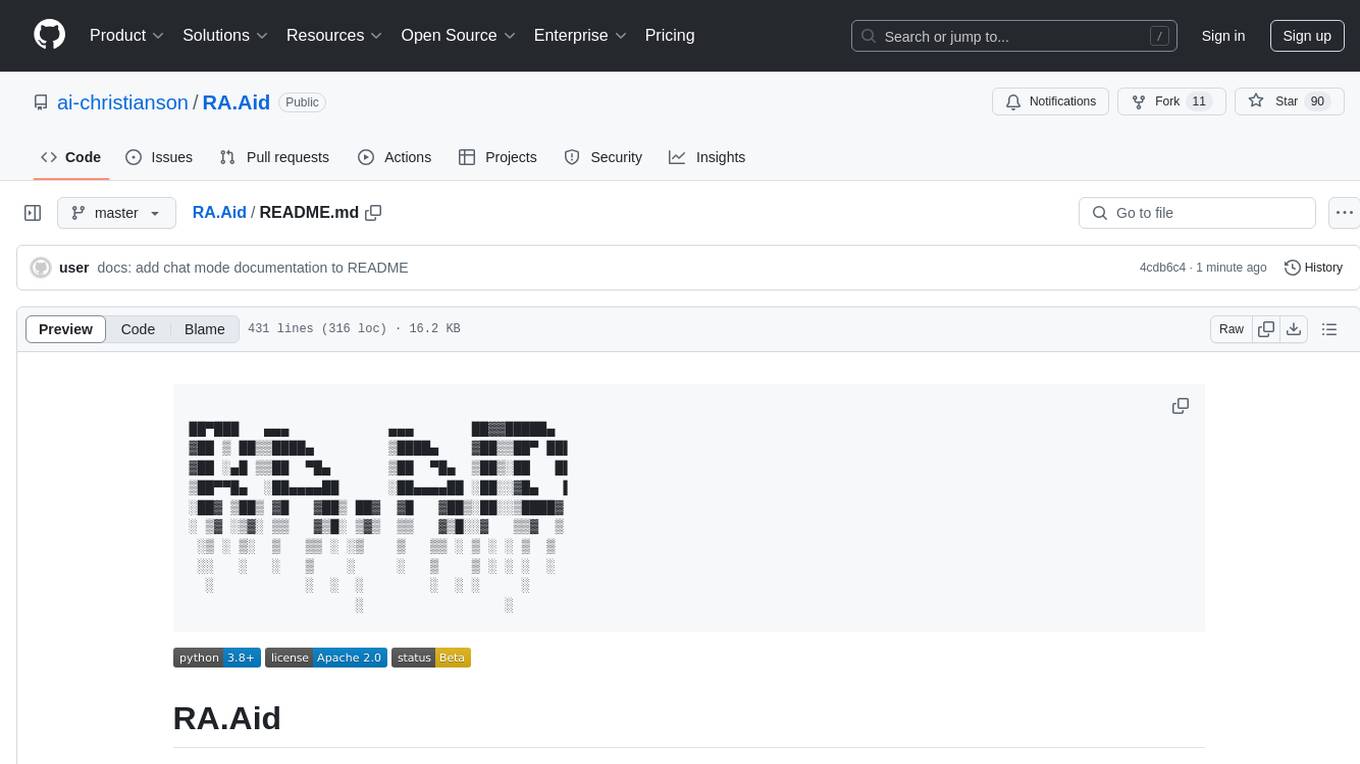
RA.Aid
RA.Aid is an AI software development agent powered by `aider` and advanced reasoning models like `o1`. It combines `aider`'s code editing capabilities with LangChain's agent-based task execution framework to provide an intelligent assistant for research, planning, and implementation of multi-step development tasks. It handles complex programming tasks by breaking them down into manageable steps, running shell commands automatically, and leveraging expert reasoning models like OpenAI's o1. RA.Aid is designed for everyday software development, offering features such as multi-step task planning, automated command execution, and the ability to handle complex programming tasks beyond single-shot code edits.
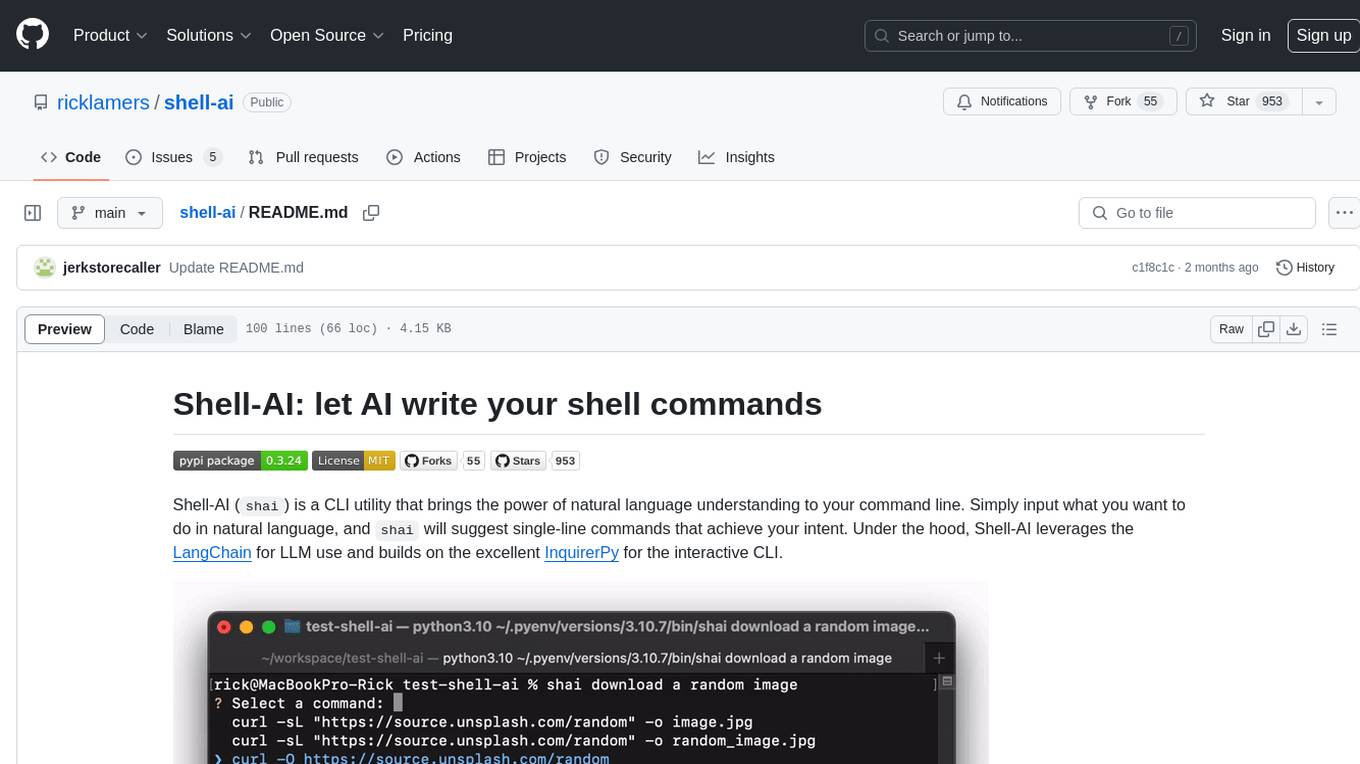
shell-ai
Shell-AI (`shai`) is a CLI utility that enables users to input commands in natural language and receive single-line command suggestions. It leverages natural language understanding and interactive CLI tools to enhance command line interactions. Users can describe tasks in plain English and receive corresponding command suggestions, making it easier to execute commands efficiently. Shell-AI supports cross-platform usage and is compatible with Azure OpenAI deployments, offering a user-friendly and efficient way to interact with the command line.
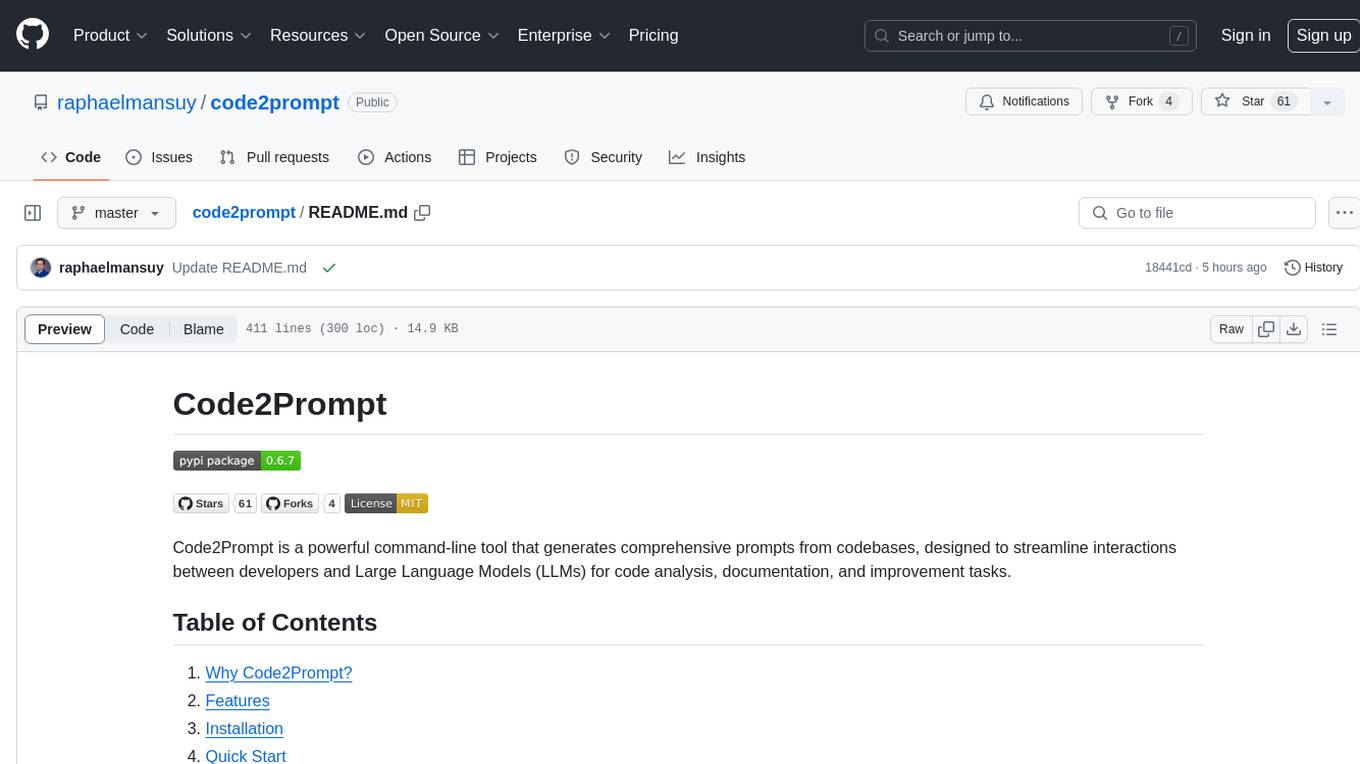
code2prompt
Code2Prompt is a powerful command-line tool that generates comprehensive prompts from codebases, designed to streamline interactions between developers and Large Language Models (LLMs) for code analysis, documentation, and improvement tasks. It bridges the gap between codebases and LLMs by converting projects into AI-friendly prompts, enabling users to leverage AI for various software development tasks. The tool offers features like holistic codebase representation, intelligent source tree generation, customizable prompt templates, smart token management, Gitignore integration, flexible file handling, clipboard-ready output, multiple output options, and enhanced code readability.
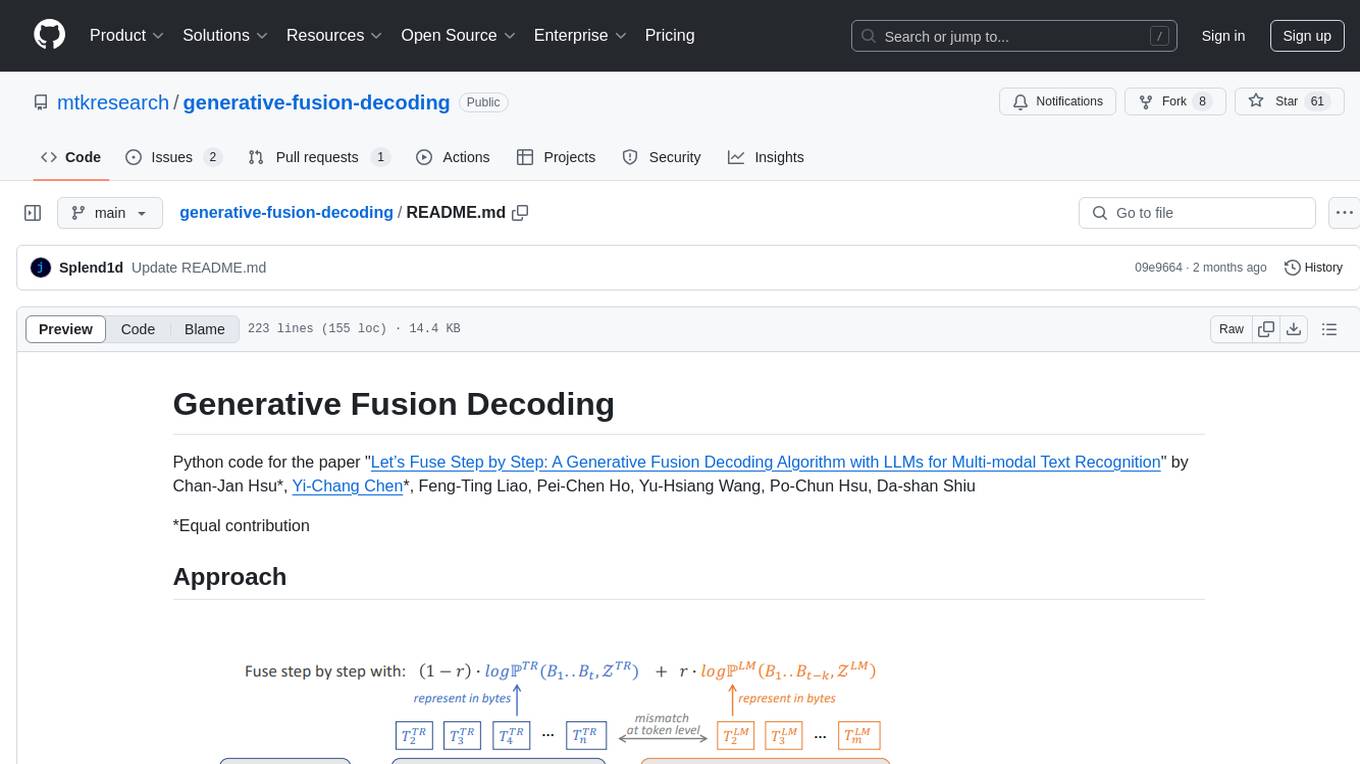
generative-fusion-decoding
Generative Fusion Decoding (GFD) is a novel shallow fusion framework that integrates Large Language Models (LLMs) into multi-modal text recognition systems such as automatic speech recognition (ASR) and optical character recognition (OCR). GFD operates across mismatched token spaces of different models by mapping text token space to byte token space, enabling seamless fusion during the decoding process. It simplifies the complexity of aligning different model sample spaces, allows LLMs to correct errors in tandem with the recognition model, increases robustness in long-form speech recognition, and enables fusing recognition models deficient in Chinese text recognition with LLMs extensively trained on Chinese. GFD significantly improves performance in ASR and OCR tasks, offering a unified solution for leveraging existing pre-trained models through step-by-step fusion.
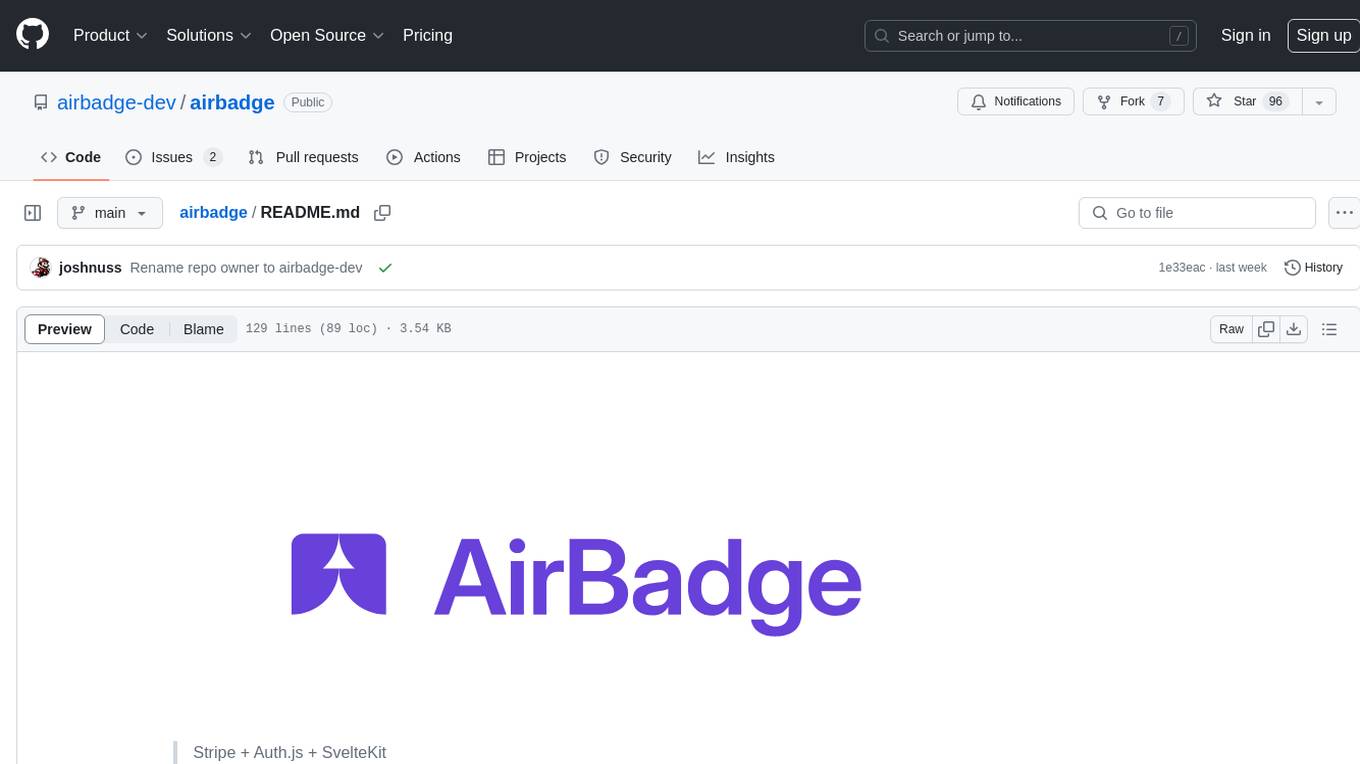
airbadge
Airbadge is a Stripe addon for Auth.js that simplifies the process of creating a SaaS site by integrating payment, authentication, gating, self-service account management, webhook handling, trials & free plans, session data, and more. It allows users to launch a SaaS app without writing any authentication or payment code. The project is open source and free to use with optional paid features under the BSL License.
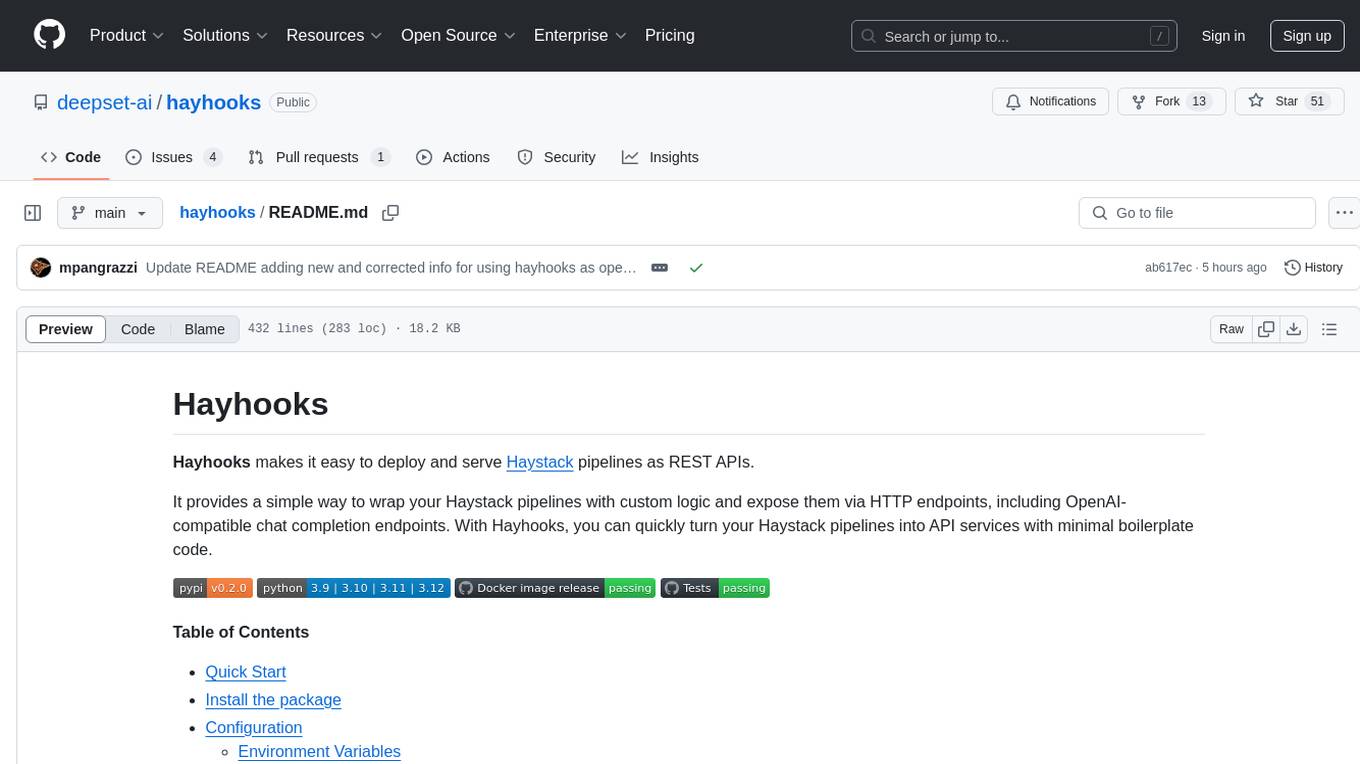
hayhooks
Hayhooks is a tool that simplifies the deployment and serving of Haystack pipelines as REST APIs. It allows users to wrap their pipelines with custom logic and expose them via HTTP endpoints, including OpenAI-compatible chat completion endpoints. With Hayhooks, users can easily convert their Haystack pipelines into API services with minimal boilerplate code.
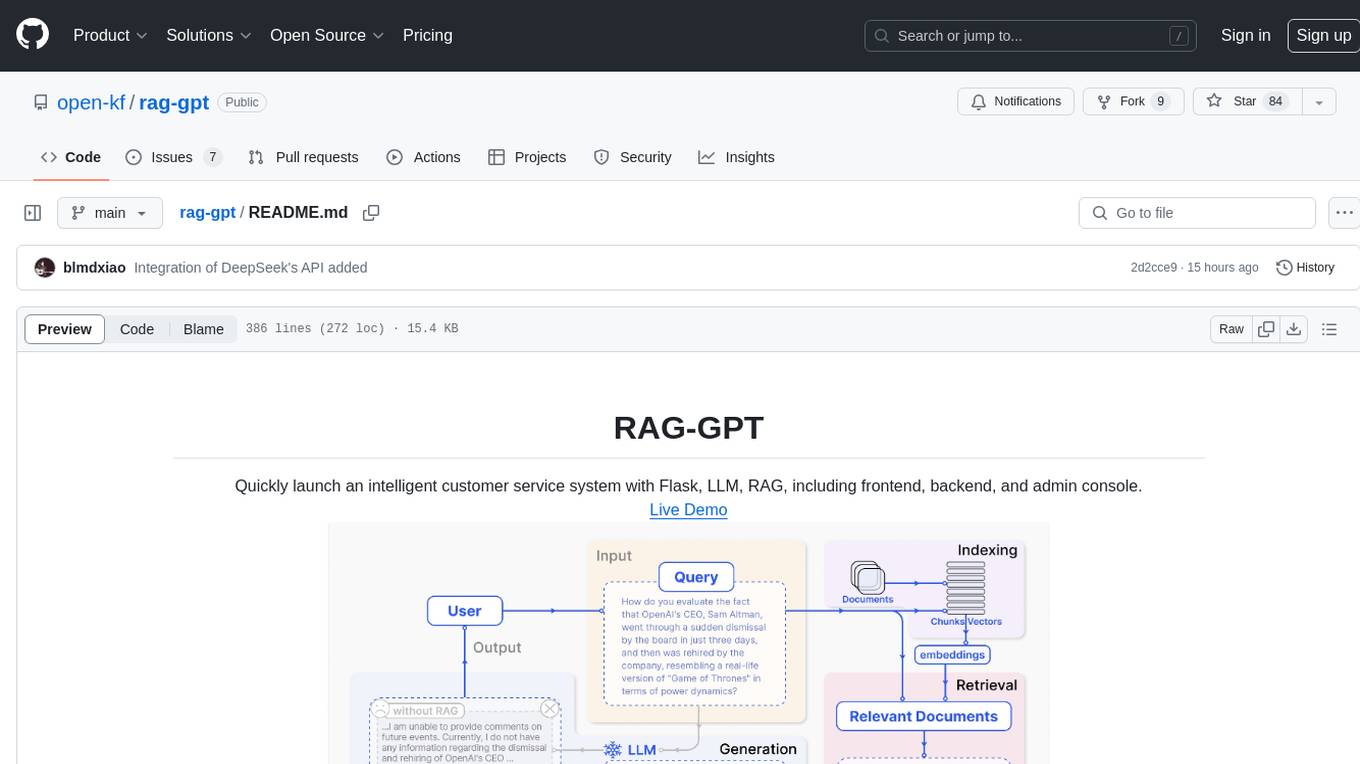
rag-gpt
RAG-GPT is a tool that allows users to quickly launch an intelligent customer service system with Flask, LLM, and RAG. It includes frontend, backend, and admin console components. The tool supports cloud-based and local LLMs, enables deployment of conversational service robots in minutes, integrates diverse knowledge bases, offers flexible configuration options, and features an attractive user interface.
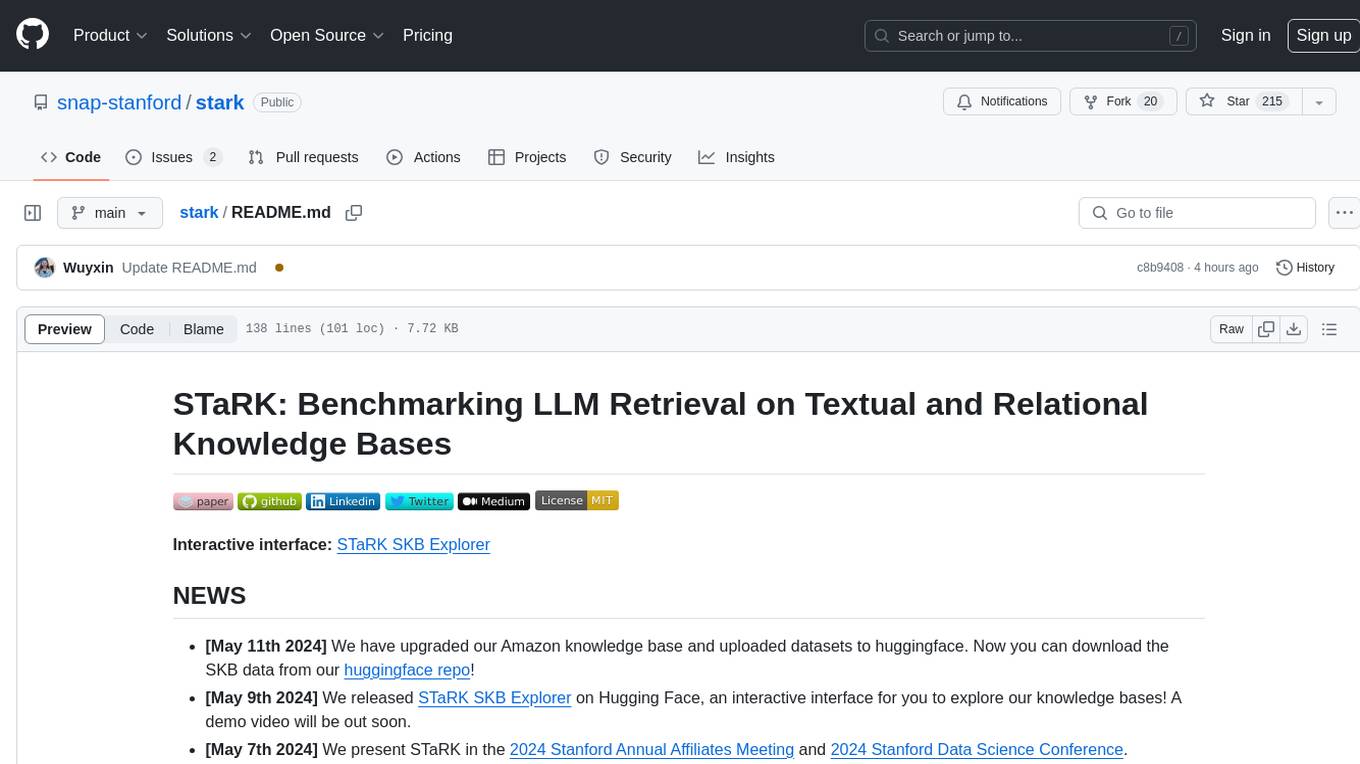
stark
STaRK is a large-scale semi-structure retrieval benchmark on Textual and Relational Knowledge Bases. It provides natural-sounding and practical queries crafted to incorporate rich relational information and complex textual properties, closely mirroring real-life scenarios. The benchmark aims to assess how effectively large language models can handle the interplay between textual and relational requirements in queries, using three diverse knowledge bases constructed from public sources.
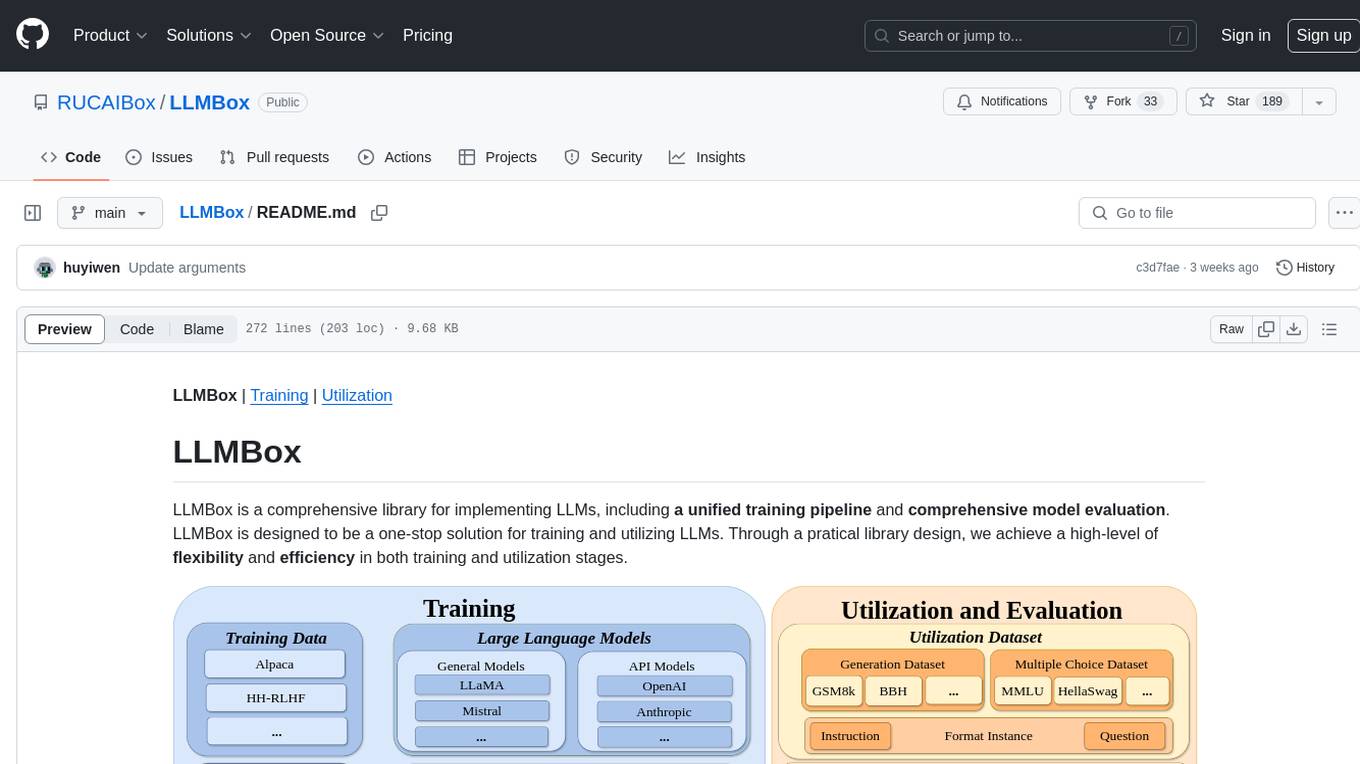
LLMBox
LLMBox is a comprehensive library designed for implementing Large Language Models (LLMs) with a focus on a unified training pipeline and comprehensive model evaluation. It serves as a one-stop solution for training and utilizing LLMs, offering flexibility and efficiency in both training and utilization stages. The library supports diverse training strategies, comprehensive datasets, tokenizer vocabulary merging, data construction strategies, parameter efficient fine-tuning, and efficient training methods. For utilization, LLMBox provides comprehensive evaluation on various datasets, in-context learning strategies, chain-of-thought evaluation, evaluation methods, prefix caching for faster inference, support for specific LLM models like vLLM and Flash Attention, and quantization options. The tool is suitable for researchers and developers working with LLMs for natural language processing tasks.
For similar tasks
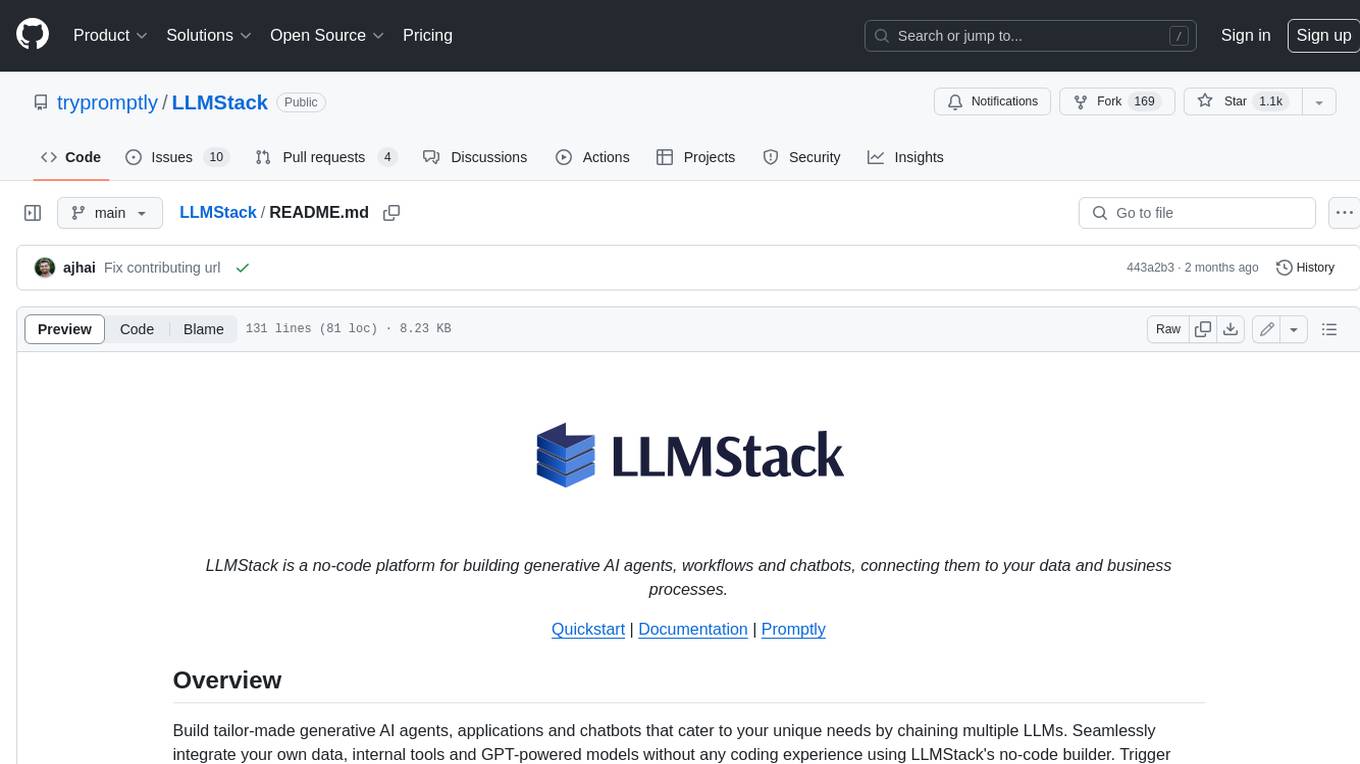
LLMStack
LLMStack is a no-code platform for building generative AI agents, workflows, and chatbots. It allows users to connect their own data, internal tools, and GPT-powered models without any coding experience. LLMStack can be deployed to the cloud or on-premise and can be accessed via HTTP API or triggered from Slack or Discord.
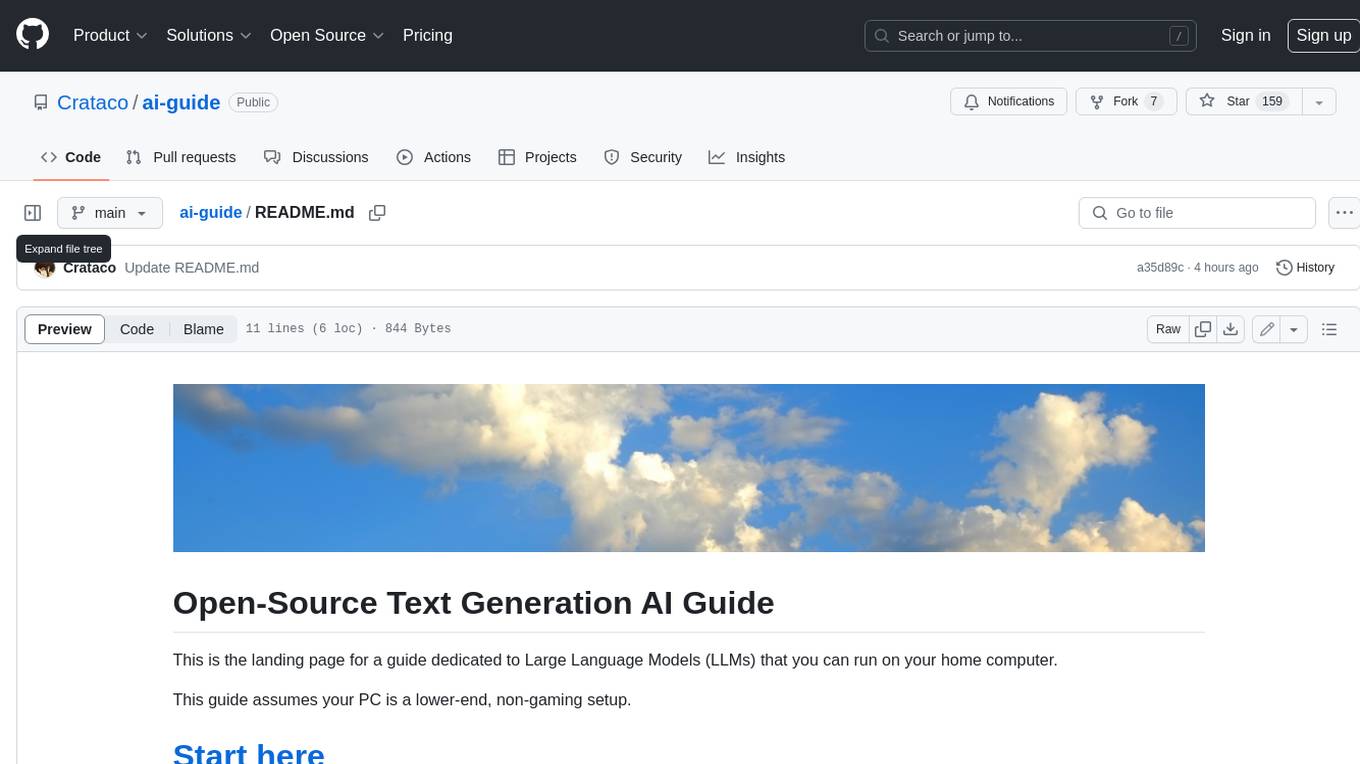
ai-guide
This guide is dedicated to Large Language Models (LLMs) that you can run on your home computer. It assumes your PC is a lower-end, non-gaming setup.
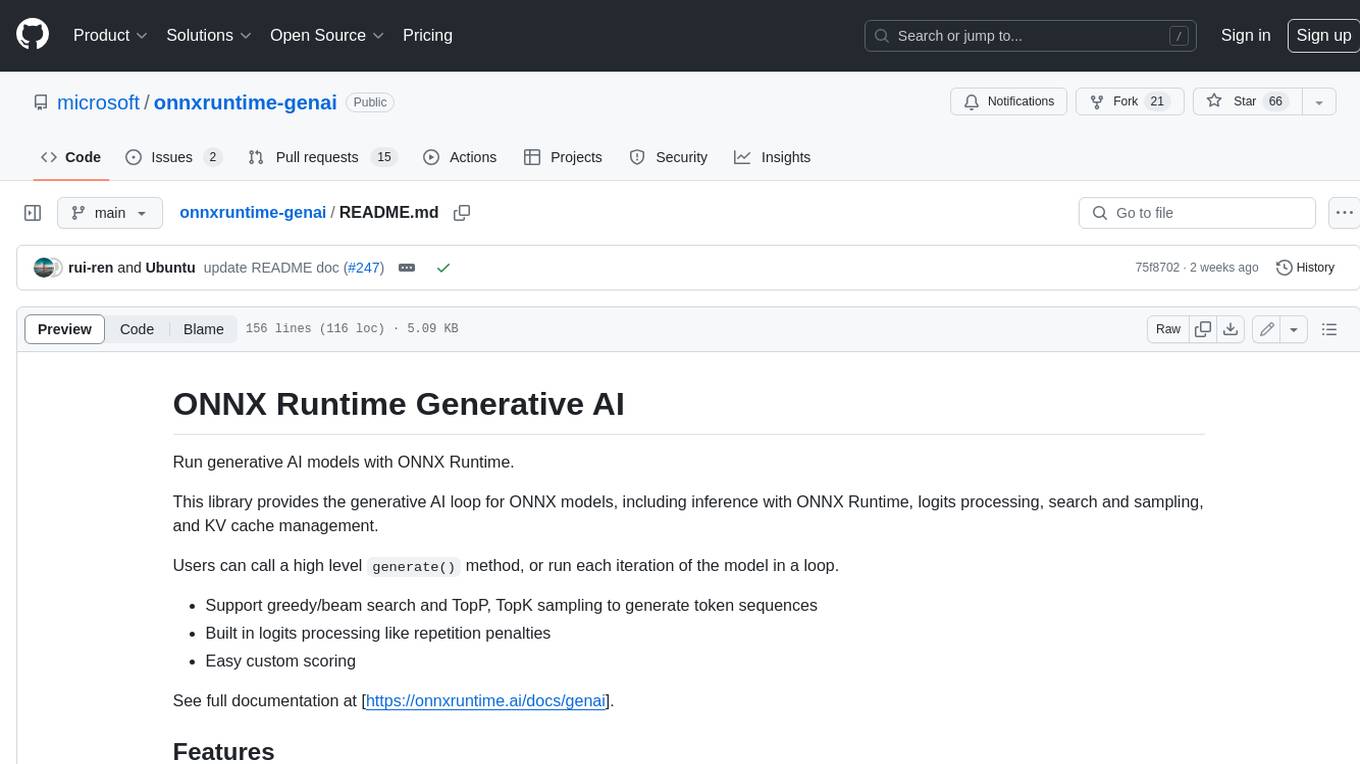
onnxruntime-genai
ONNX Runtime Generative AI is a library that provides the generative AI loop for ONNX models, including inference with ONNX Runtime, logits processing, search and sampling, and KV cache management. Users can call a high level `generate()` method, or run each iteration of the model in a loop. It supports greedy/beam search and TopP, TopK sampling to generate token sequences, has built in logits processing like repetition penalties, and allows for easy custom scoring.
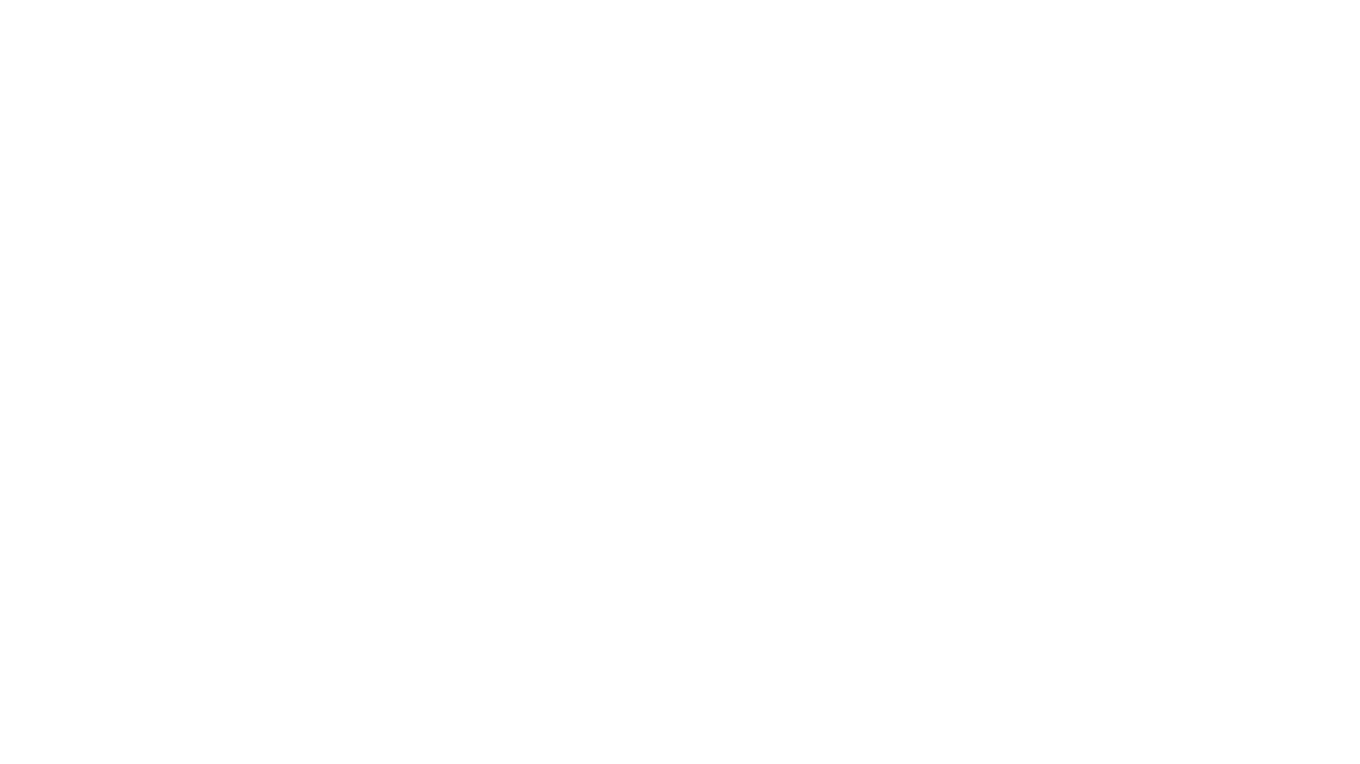
jupyter-ai
Jupyter AI connects generative AI with Jupyter notebooks. It provides a user-friendly and powerful way to explore generative AI models in notebooks and improve your productivity in JupyterLab and the Jupyter Notebook. Specifically, Jupyter AI offers: * An `%%ai` magic that turns the Jupyter notebook into a reproducible generative AI playground. This works anywhere the IPython kernel runs (JupyterLab, Jupyter Notebook, Google Colab, Kaggle, VSCode, etc.). * A native chat UI in JupyterLab that enables you to work with generative AI as a conversational assistant. * Support for a wide range of generative model providers, including AI21, Anthropic, AWS, Cohere, Gemini, Hugging Face, NVIDIA, and OpenAI. * Local model support through GPT4All, enabling use of generative AI models on consumer grade machines with ease and privacy.
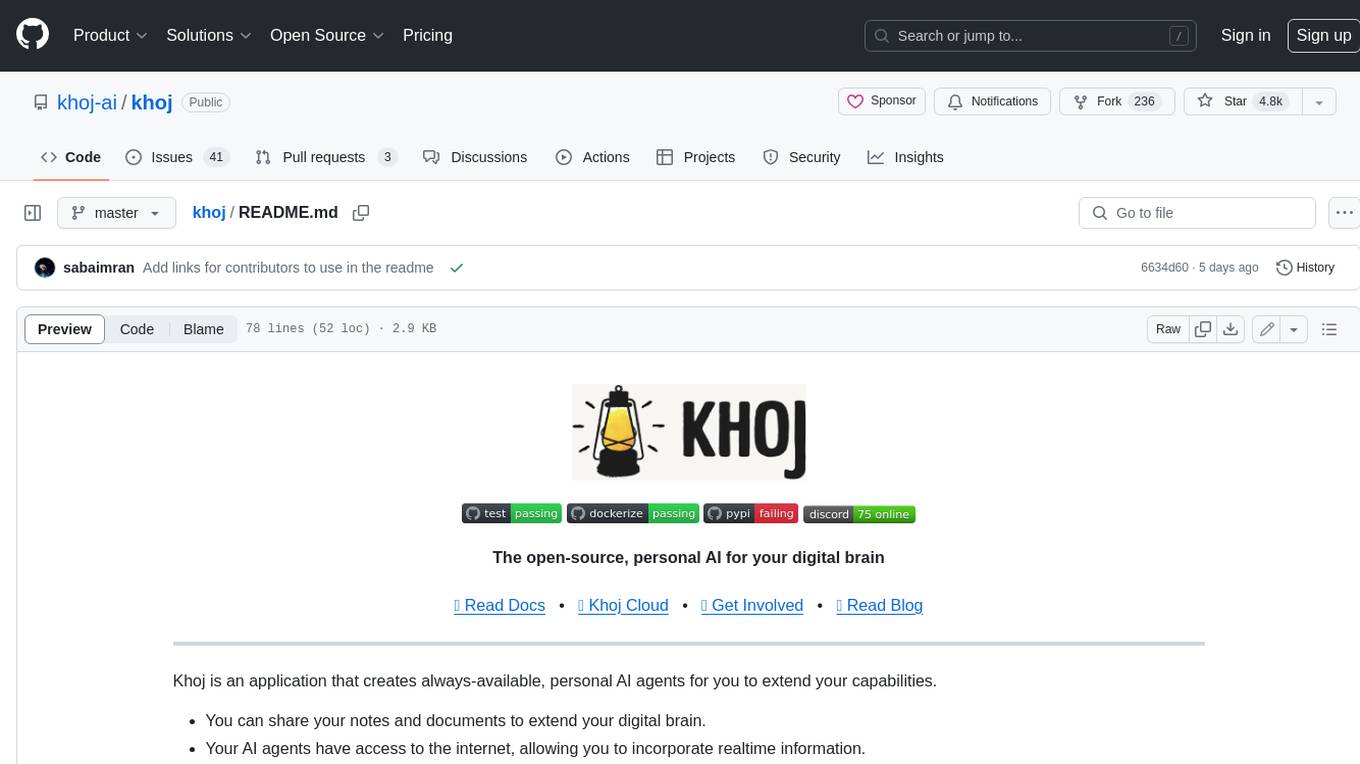
khoj
Khoj is an open-source, personal AI assistant that extends your capabilities by creating always-available AI agents. You can share your notes and documents to extend your digital brain, and your AI agents have access to the internet, allowing you to incorporate real-time information. Khoj is accessible on Desktop, Emacs, Obsidian, Web, and Whatsapp, and you can share PDF, markdown, org-mode, notion files, and GitHub repositories. You'll get fast, accurate semantic search on top of your docs, and your agents can create deeply personal images and understand your speech. Khoj is self-hostable and always will be.
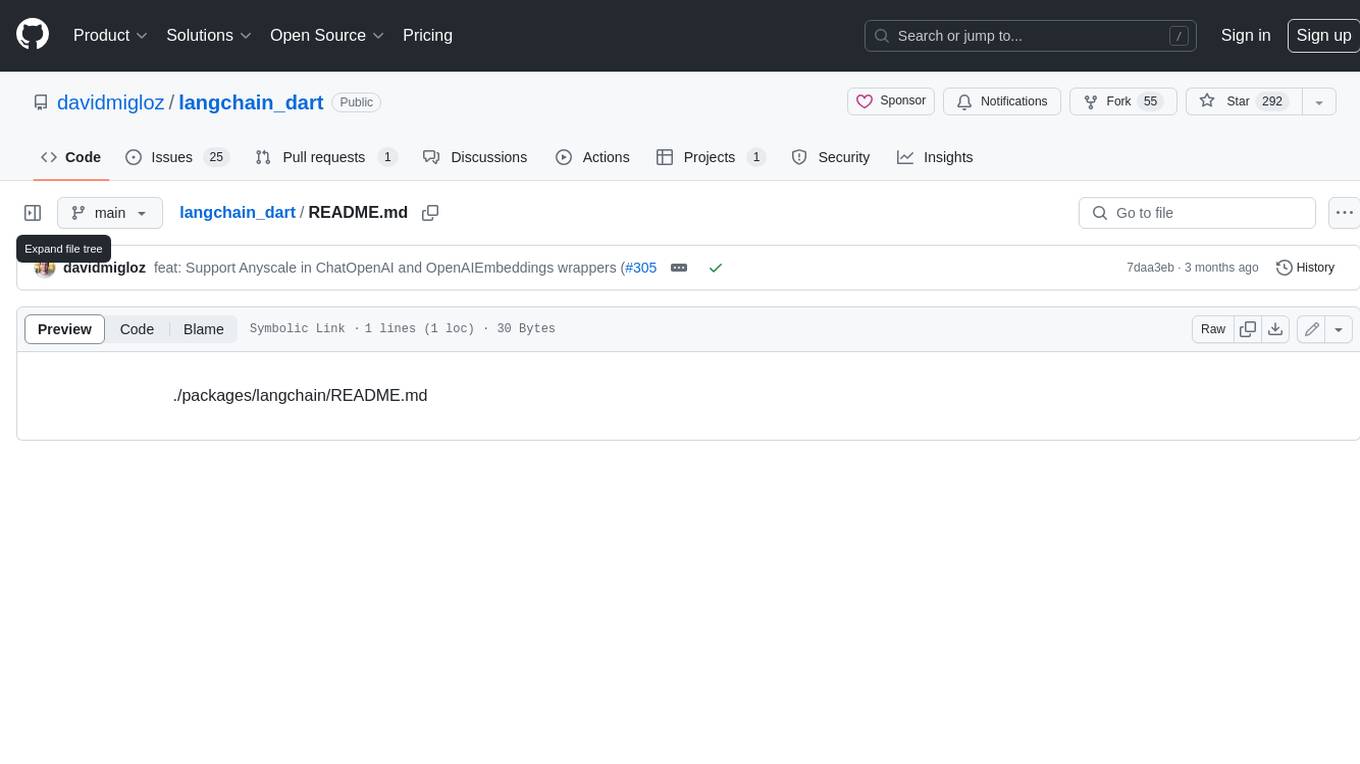
langchain_dart
LangChain.dart is a Dart port of the popular LangChain Python framework created by Harrison Chase. LangChain provides a set of ready-to-use components for working with language models and a standard interface for chaining them together to formulate more advanced use cases (e.g. chatbots, Q&A with RAG, agents, summarization, extraction, etc.). The components can be grouped into a few core modules: * **Model I/O:** LangChain offers a unified API for interacting with various LLM providers (e.g. OpenAI, Google, Mistral, Ollama, etc.), allowing developers to switch between them with ease. Additionally, it provides tools for managing model inputs (prompt templates and example selectors) and parsing the resulting model outputs (output parsers). * **Retrieval:** assists in loading user data (via document loaders), transforming it (with text splitters), extracting its meaning (using embedding models), storing (in vector stores) and retrieving it (through retrievers) so that it can be used to ground the model's responses (i.e. Retrieval-Augmented Generation or RAG). * **Agents:** "bots" that leverage LLMs to make informed decisions about which available tools (such as web search, calculators, database lookup, etc.) to use to accomplish the designated task. The different components can be composed together using the LangChain Expression Language (LCEL).
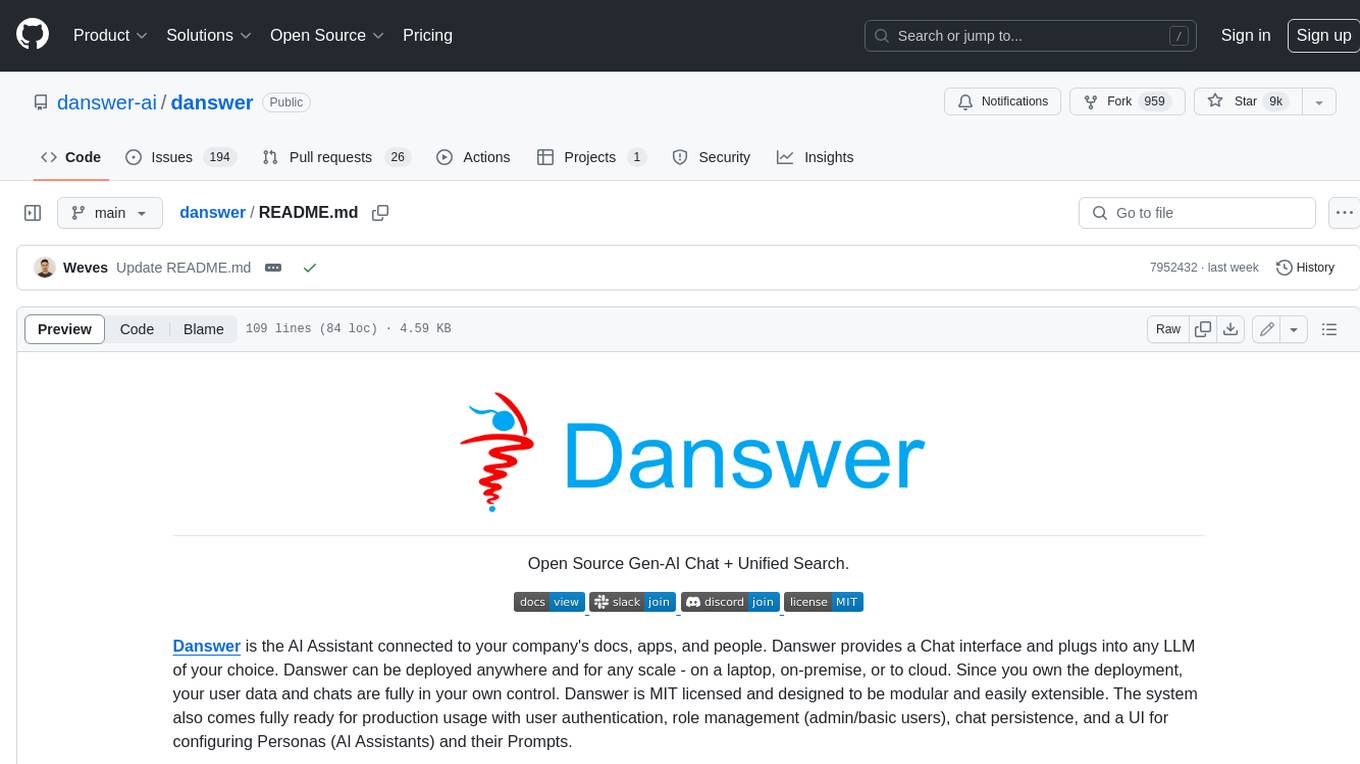
danswer
Danswer is an open-source Gen-AI Chat and Unified Search tool that connects to your company's docs, apps, and people. It provides a Chat interface and plugs into any LLM of your choice. Danswer can be deployed anywhere and for any scale - on a laptop, on-premise, or to cloud. Since you own the deployment, your user data and chats are fully in your own control. Danswer is MIT licensed and designed to be modular and easily extensible. The system also comes fully ready for production usage with user authentication, role management (admin/basic users), chat persistence, and a UI for configuring Personas (AI Assistants) and their Prompts. Danswer also serves as a Unified Search across all common workplace tools such as Slack, Google Drive, Confluence, etc. By combining LLMs and team specific knowledge, Danswer becomes a subject matter expert for the team. Imagine ChatGPT if it had access to your team's unique knowledge! It enables questions such as "A customer wants feature X, is this already supported?" or "Where's the pull request for feature Y?"
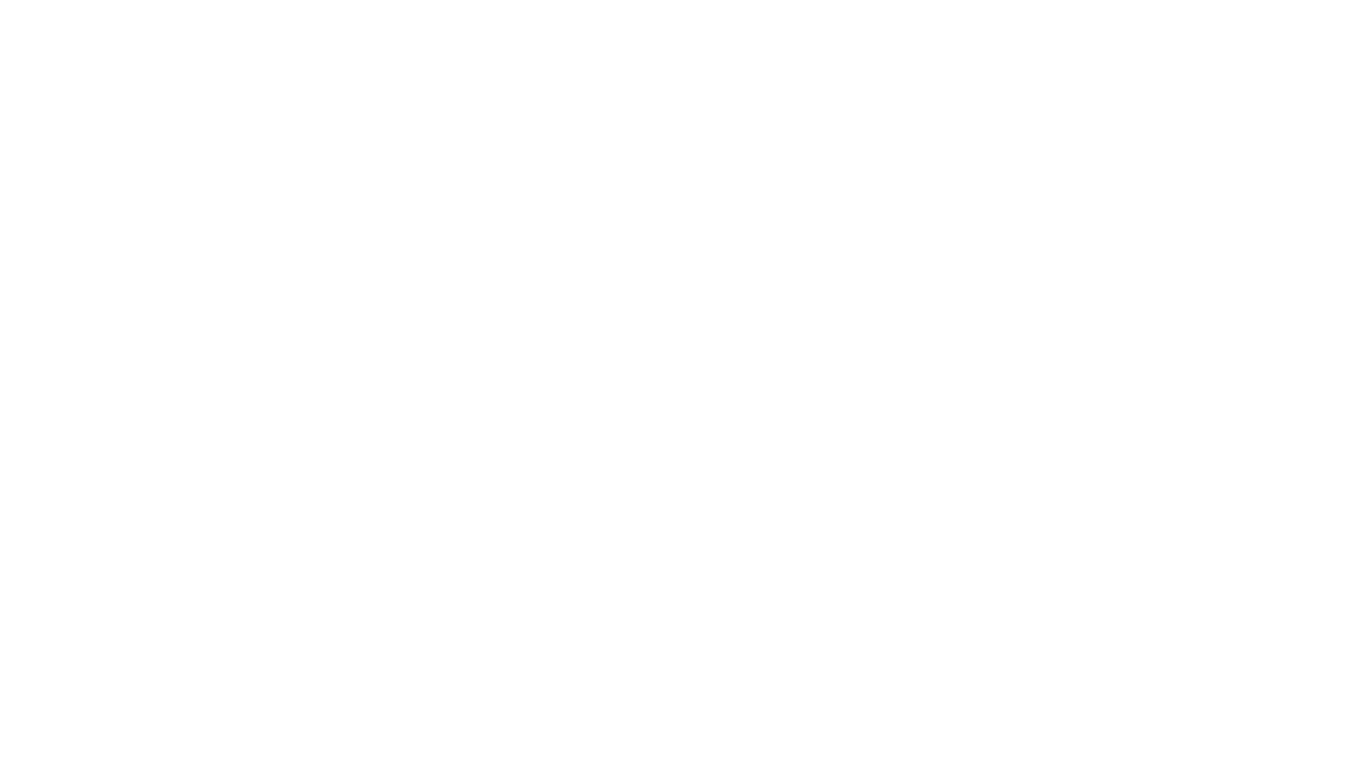
infinity
Infinity is an AI-native database designed for LLM applications, providing incredibly fast full-text and vector search capabilities. It supports a wide range of data types, including vectors, full-text, and structured data, and offers a fused search feature that combines multiple embeddings and full text. Infinity is easy to use, with an intuitive Python API and a single-binary architecture that simplifies deployment. It achieves high performance, with 0.1 milliseconds query latency on million-scale vector datasets and up to 15K QPS.
For similar jobs
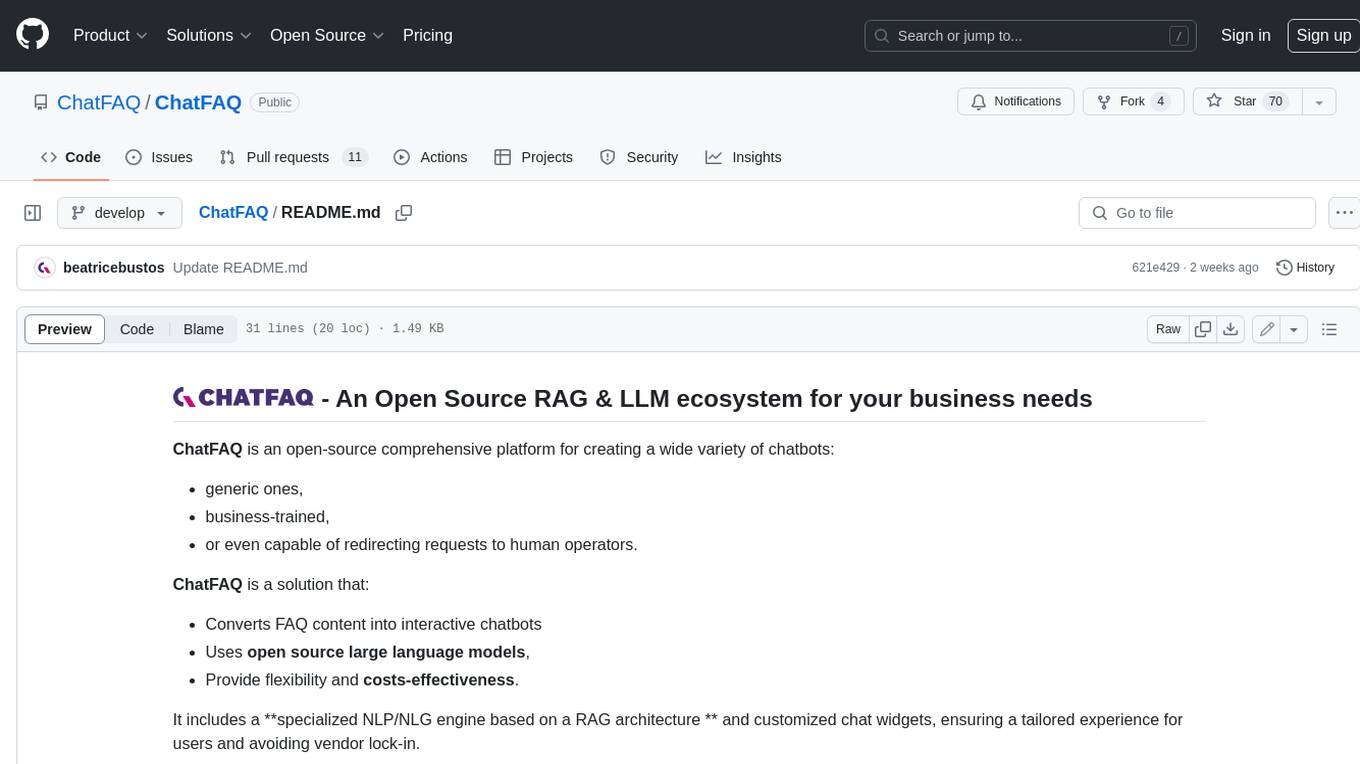
ChatFAQ
ChatFAQ is an open-source comprehensive platform for creating a wide variety of chatbots: generic ones, business-trained, or even capable of redirecting requests to human operators. It includes a specialized NLP/NLG engine based on a RAG architecture and customized chat widgets, ensuring a tailored experience for users and avoiding vendor lock-in.
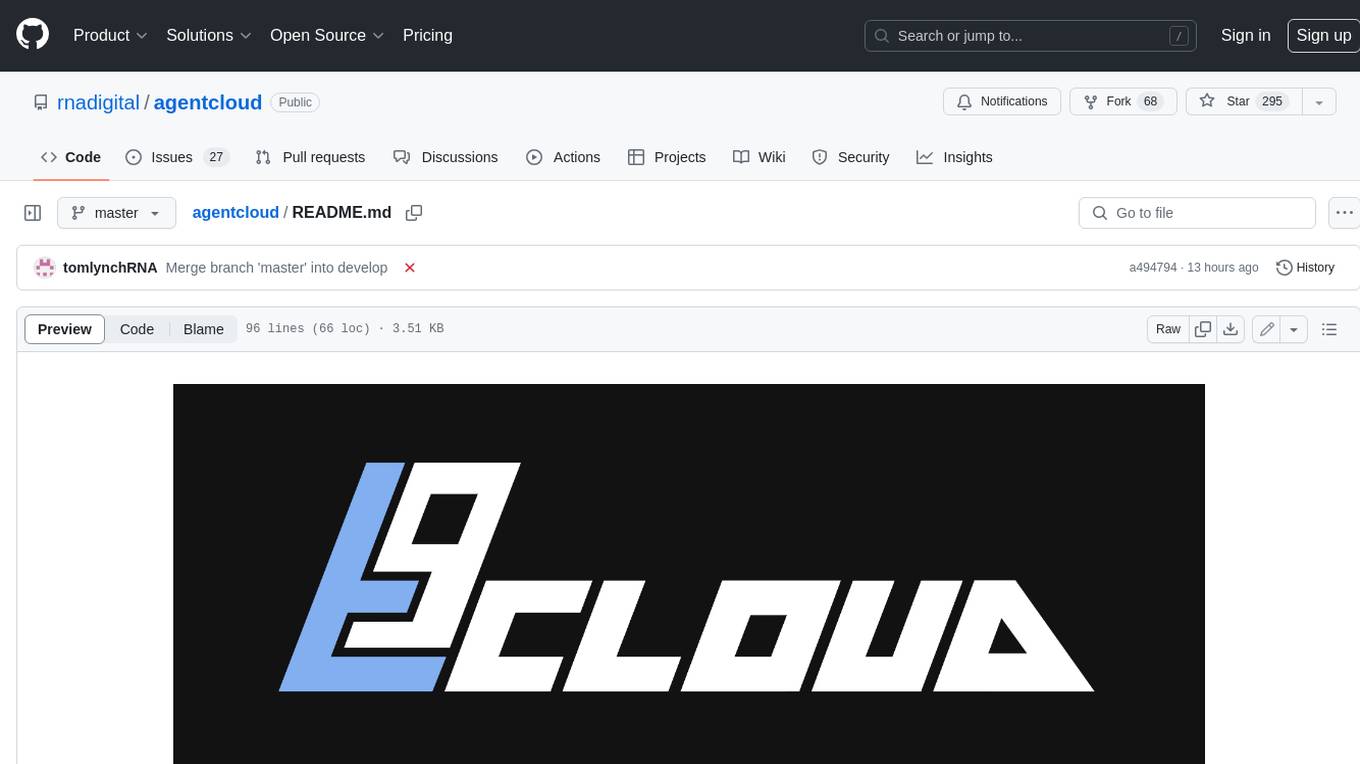
agentcloud
AgentCloud is an open-source platform that enables companies to build and deploy private LLM chat apps, empowering teams to securely interact with their data. It comprises three main components: Agent Backend, Webapp, and Vector Proxy. To run this project locally, clone the repository, install Docker, and start the services. The project is licensed under the GNU Affero General Public License, version 3 only. Contributions and feedback are welcome from the community.
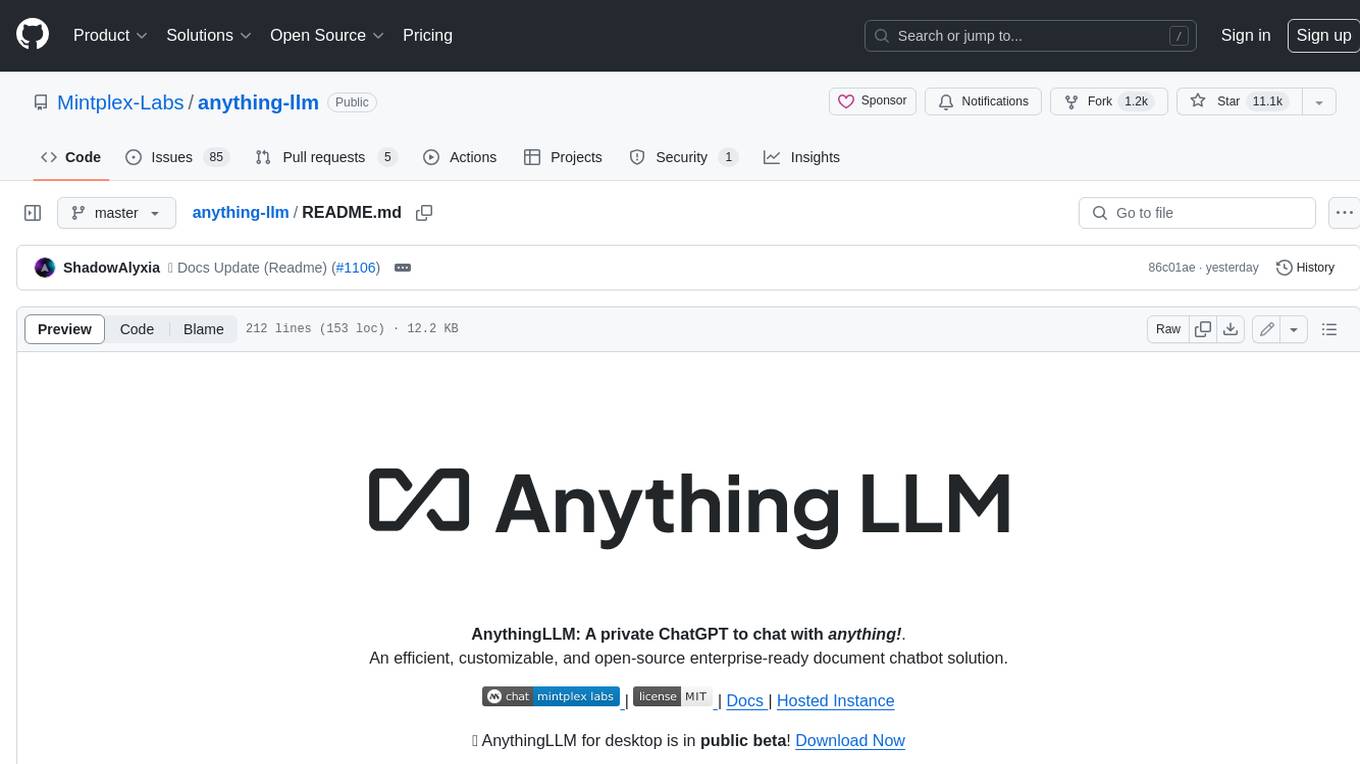
anything-llm
AnythingLLM is a full-stack application that enables you to turn any document, resource, or piece of content into context that any LLM can use as references during chatting. This application allows you to pick and choose which LLM or Vector Database you want to use as well as supporting multi-user management and permissions.
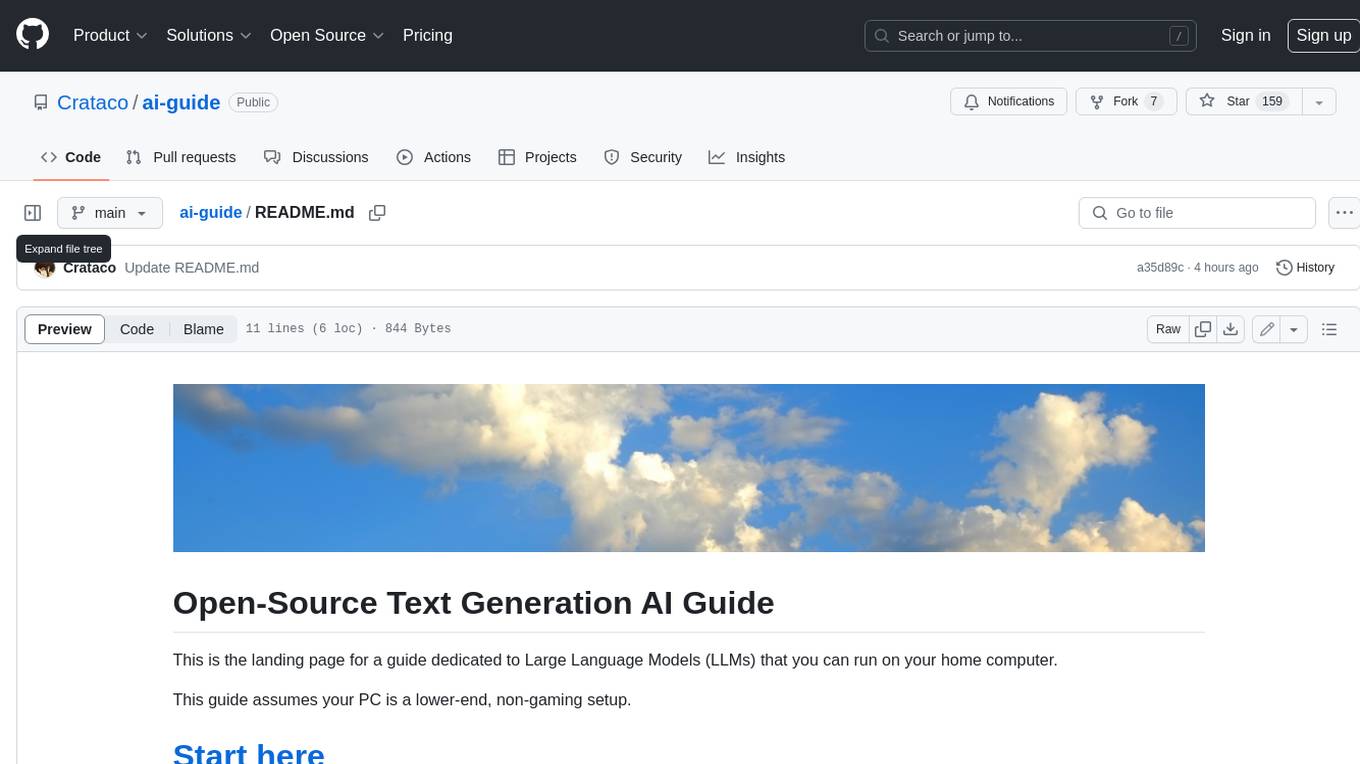
ai-guide
This guide is dedicated to Large Language Models (LLMs) that you can run on your home computer. It assumes your PC is a lower-end, non-gaming setup.
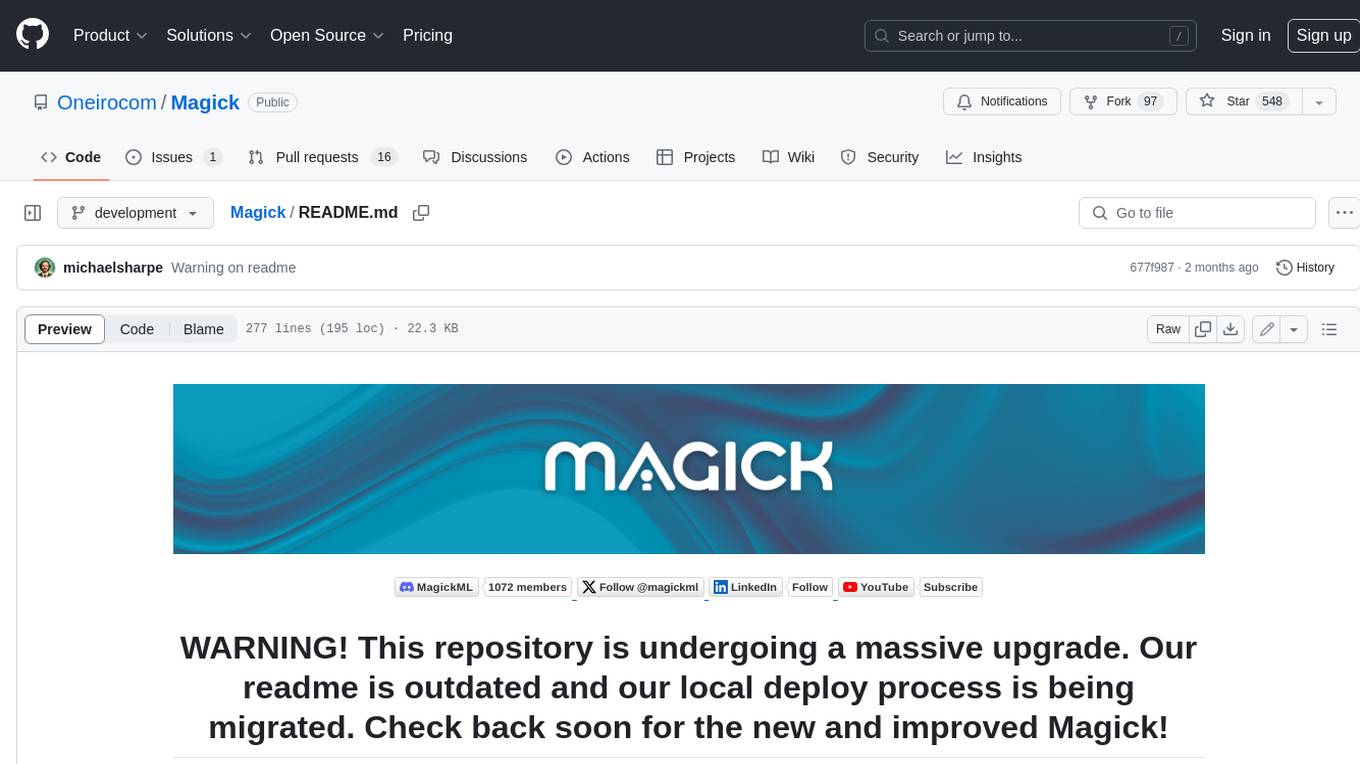
Magick
Magick is a groundbreaking visual AIDE (Artificial Intelligence Development Environment) for no-code data pipelines and multimodal agents. Magick can connect to other services and comes with nodes and templates well-suited for intelligent agents, chatbots, complex reasoning systems and realistic characters.
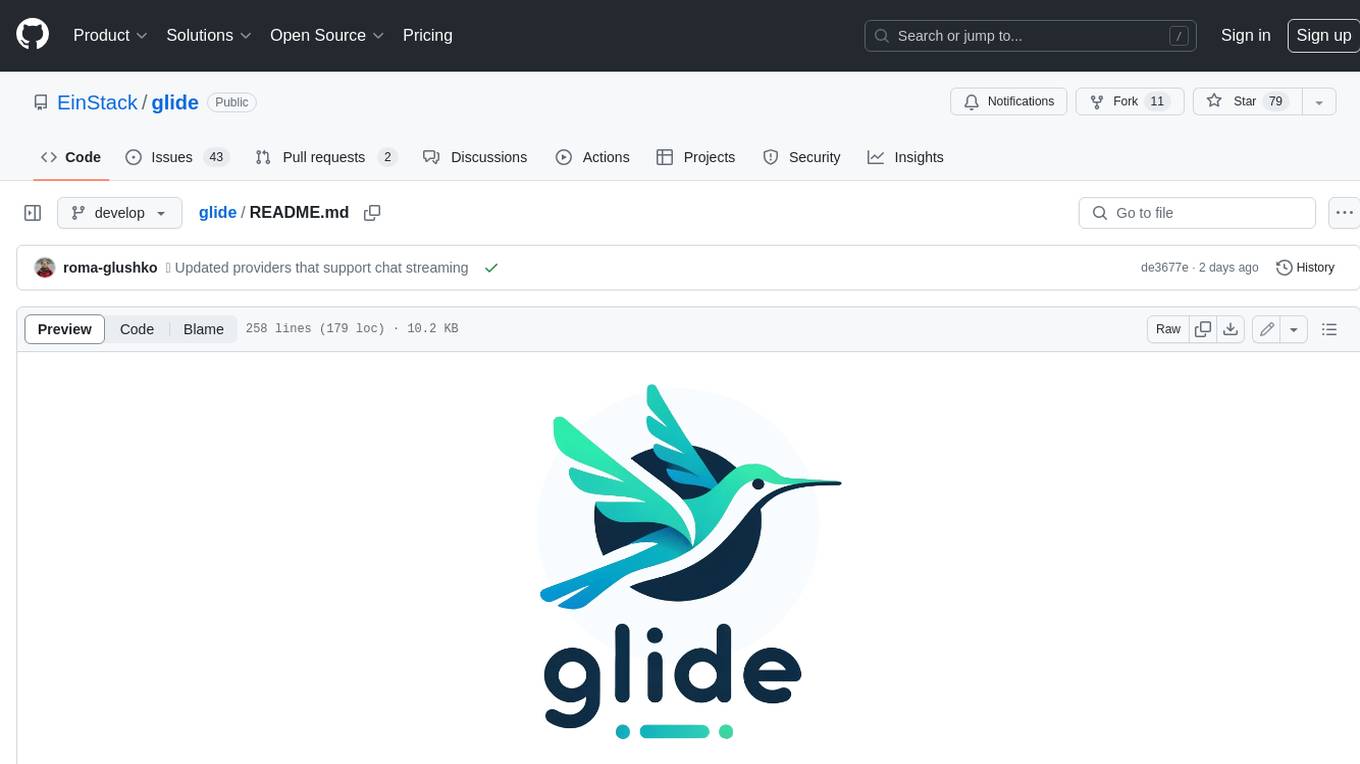
glide
Glide is a cloud-native LLM gateway that provides a unified REST API for accessing various large language models (LLMs) from different providers. It handles LLMOps tasks such as model failover, caching, key management, and more, making it easy to integrate LLMs into applications. Glide supports popular LLM providers like OpenAI, Anthropic, Azure OpenAI, AWS Bedrock (Titan), Cohere, Google Gemini, OctoML, and Ollama. It offers high availability, performance, and observability, and provides SDKs for Python and NodeJS to simplify integration.
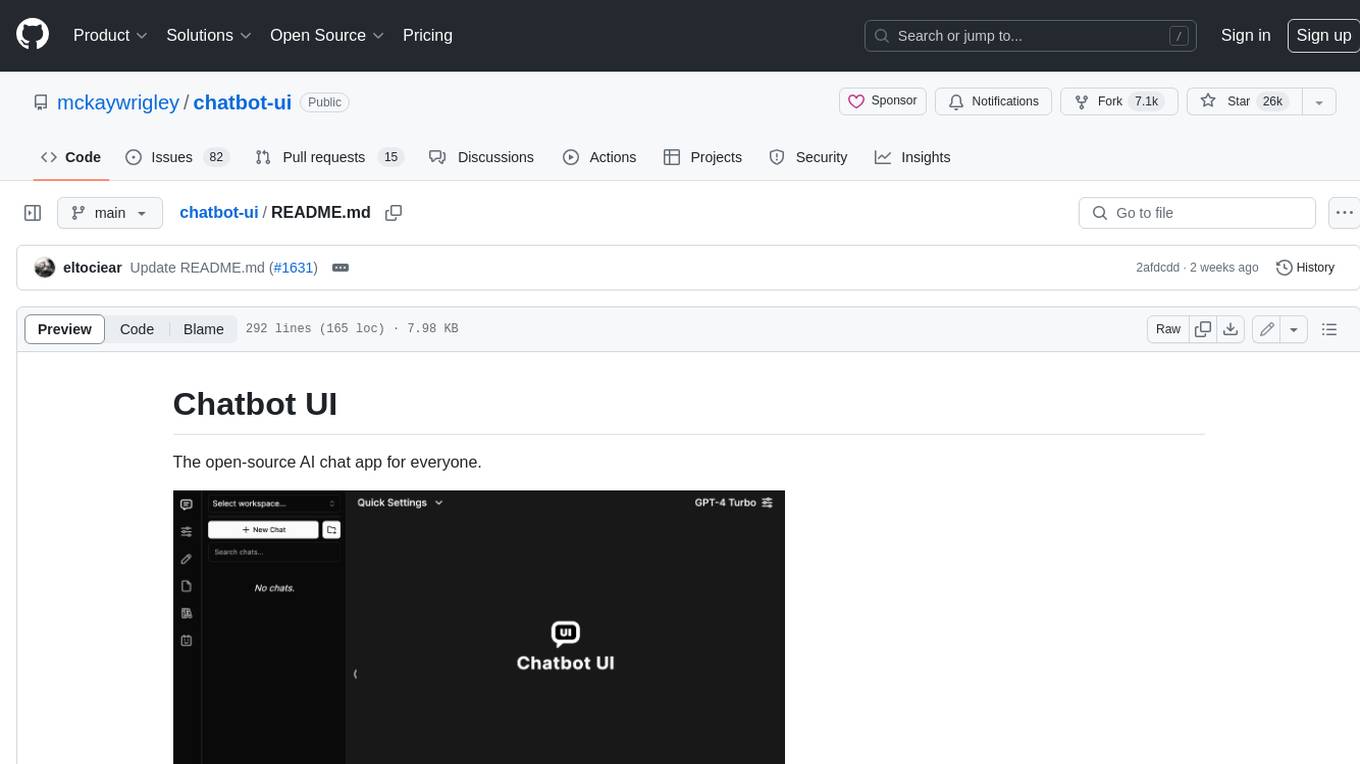
chatbot-ui
Chatbot UI is an open-source AI chat app that allows users to create and deploy their own AI chatbots. It is easy to use and can be customized to fit any need. Chatbot UI is perfect for businesses, developers, and anyone who wants to create a chatbot.
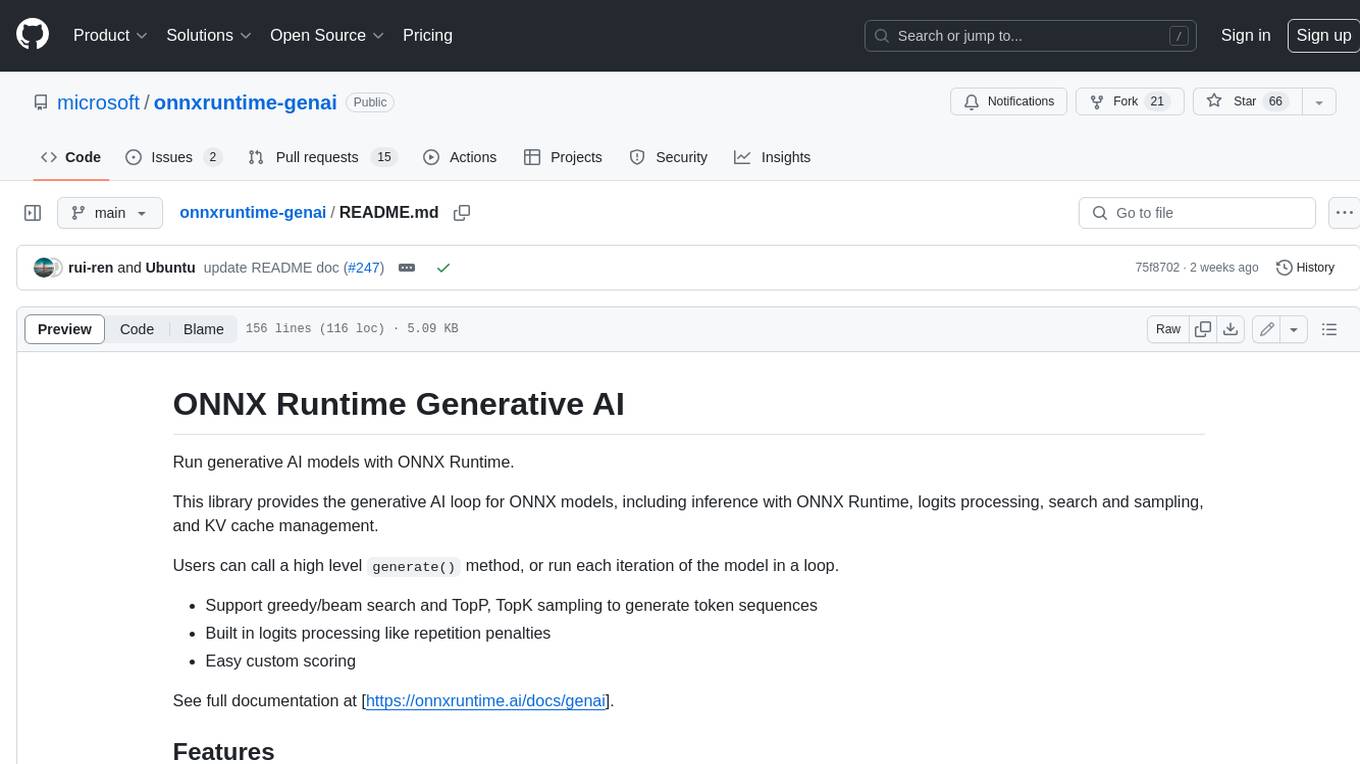
onnxruntime-genai
ONNX Runtime Generative AI is a library that provides the generative AI loop for ONNX models, including inference with ONNX Runtime, logits processing, search and sampling, and KV cache management. Users can call a high level `generate()` method, or run each iteration of the model in a loop. It supports greedy/beam search and TopP, TopK sampling to generate token sequences, has built in logits processing like repetition penalties, and allows for easy custom scoring.