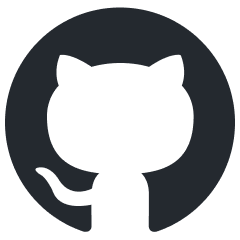
LongRecipe
LongRecipe: Recipe for Efficient Long Context Generalization in Large Language Models
Stars: 57
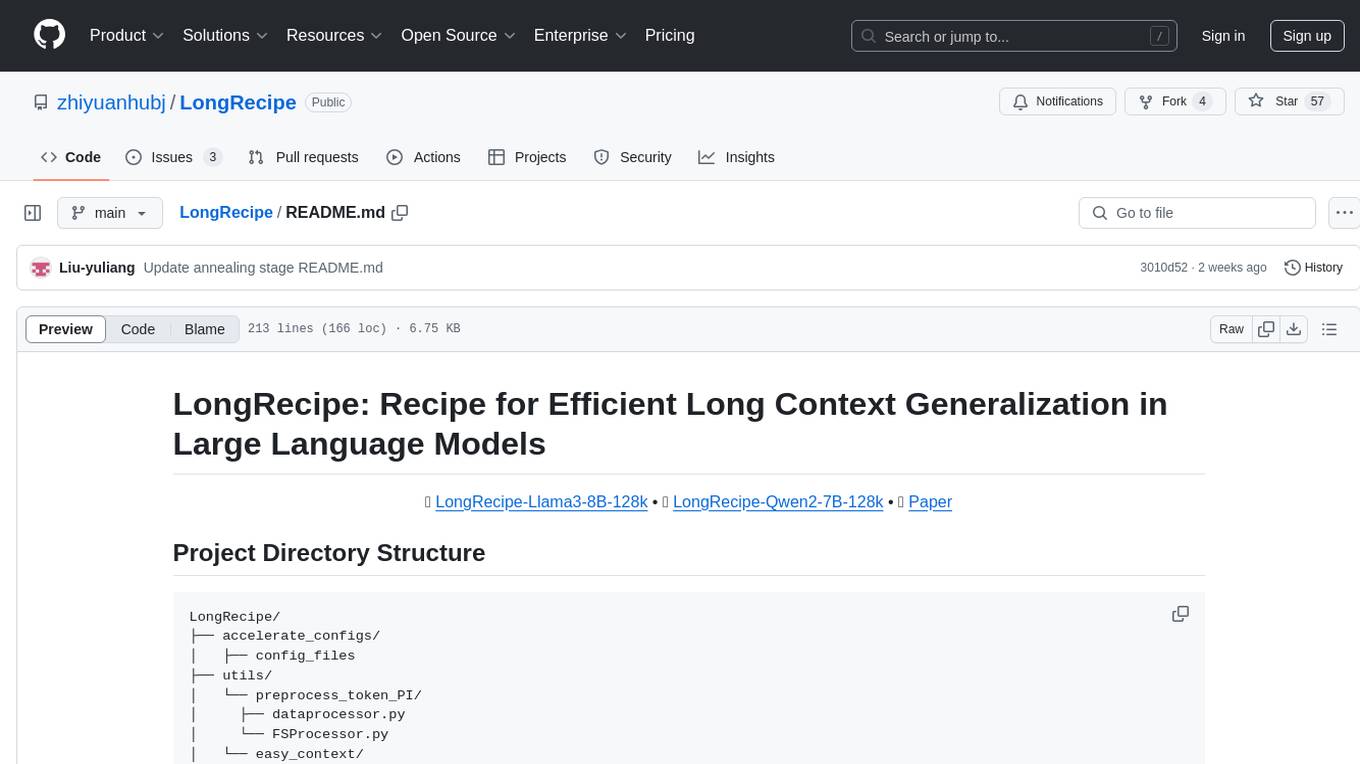
LongRecipe is a tool designed for efficient long context generalization in large language models. It provides a recipe for extending the context window of language models while maintaining their original capabilities. The tool includes data preprocessing steps, model training stages, and a process for merging fine-tuned models to enhance foundational capabilities. Users can follow the provided commands and scripts to preprocess data, train models in multiple stages, and merge models effectively.
README:
π€ LongRecipe-Llama3-8B-128k β’ π€ LongRecipe-Qwen2-7B-128k β’ π Paper
LongRecipe/
βββ accelerate_configs/
β βββ config_files
βββ utils/
β βββ preprocess_token_PI/
β βββ dataprocessor.py
β βββ FSProcessor.py
β βββ easy_context/
β βββ dist_flash_attn/
β βββ ulysses_attn/
β βββ zigzag_ring_attn/
β βββ loader.py
β βββ logger.py
β βββ preprocess_data.py
βββ README.md
βββ train_LR_llama3_target80k_use24k.sh
βββ requirements.txt
βββ train.py
Before starting with the data preprocessing and model training, ensure that all necessary dependencies are installed. Use the following command to install the required packages:
pip install -r requirements.txt
To begin, download the dataset represented by the Llama3 tokenizer from this link. After downloading, execute the following command to generate the position index files for different training approaches:
# Command to load dataset and generate position index files
python preprocess_token_PI/dataprocessor.py
The model training process is divided into three distinct stages to effectively extend the context window of the LLM while maintaining its original capabilities.
In the first stage, we extend the context window using a dataset containing 1.7B tokens. The following command initiates this training stage:
accelerate launch \
--config_file accelerate_configs/single_node.yaml \
train.py \
--batch-size 1 \
--gradient-accumulate-every 96 \
--learning-rate 5e-5 \
--epoch 1 \
--data_path $DATA_PATH_CONTEXT_EXTENSION \
--output-dir ./output/$MODEL_NAME-$SETTING-$SEQ_LENGTH-$SUB_LABEL \
--seed 2027 \
--model $MODEL \
--seq-length $SEQ_LENGTH \
--target-length $TARGET_LENGTH \
--log-path $SETTING-$SEQ_LENGTH-$MODEL_NAME-$SUB_LABEL.log \
--setting $SETTING \
--right_points-path $Right_Points_PATH \
--fs_PI-path $FS_PI_PATH \
--parallel_mode ulysses_attn \
--num_proc 5 \
--stage 0
Arguments Explanation:
- --data_path: Path to the dataset with Llama3-tokenized samples.
- --model: The base model used for training.
- --seq-length: The sequence length for training.
- --target-length: The target context window length.
- --setting: The training method, which could include FLT, RPES, PoSE, LongRecipe.
- --right_points-path: Path to the PoSE right point set file.
- --fs_PI-path: Path to the LongRecipeβs position index file.
Post-training, copy the tokenizer files to the output directory and remove any unnecessary files:
cp $MODEL/special_tokens_map.json ./output/$MODEL_NAME-$SETTING-$SEQ_LENGTH-$SUB_LABEL/stage_0
cp $MODEL/tokenizer_config.json ./output/$MODEL_NAME-$SETTING-$SEQ_LENGTH-$SUB_LABEL/stage_0
cp $MODEL/tokenizer.json ./output/$MODEL_NAME-$SETTING-$SEQ_LENGTH-$SUB_LABEL/stage_0
rm ./output/$MODEL_NAME-$SETTING-$SEQ_LENGTH-$SUB_LABEL/stage_0/model.safetensors
In the second stage, we perform training annealing using both general and domain-specific data, gradually reducing the learning rate to zero. Approximately 100M tokens of data are used in this phase.
accelerate launch \
--config_file accelerate_configs/single_node_2.yaml \
train.py \
--data_path $DATA_PATH_ANNEALING \
--batch-size 1 \
--gradient-accumulate-every 96 \
--learning-rate 5e-6 \
--epoch 1 \
--output-dir ./output/$MODEL_NAME-$SETTING-$SEQ_LENGTH-$SUB_LABEL \
--seed 2027 \
--model $STAGE_1_MODEL \
--seq-length $SEQ_LENGTH \
--target-length $TARGET_LENGTH \
--log-path $SETTING-$SEQ_LENGTH-$MODEL_NAME-$SUB_LABEL.log \
--setting $SETTING \
--right_points-path $Right_Points_PATH \
--fs_PI-path $FS_PI_PATH \
--parallel_mode ulysses_attn \
--num_proc 10 \
--stage 1
Copy the updated tokenizer files to the output directory:
cp $MODEL/special_tokens_map.json ./output/$MODEL_NAME-$SETTING-$SEQ_LENGTH-$SUB_LABEL/stage_1
cp $MODEL/tokenizer_config.json ./output/$MODEL_NAME-$SETTING-$SEQ_LENGTH-$SUB_LABEL/stage_1
cp $MODEL/tokenizer.json ./output/$MODEL_NAME-$SETTING-$SEQ_LENGTH-$SUB_LABEL/stage_1
rm ./output/$MODEL_NAME-$SETTING-$SEQ_LENGTH-$SUB_LABEL/stage_1/model.safetensors
In our experiment, we merge the two datasets mentioned together in out paper, and format each sample as follows:
{
"prompt": ,
"response":
}
The final stage involves merging the original model with the fine-tuned model using an average weight strategy to enhance the model's foundational capabilities.
accelerate launch \
--config_file accelerate_configs/single_node.yaml \
train.py \
accelerate launch \
--config_file accelerate_configs/single_node.yaml \
train.py \
--output-dir ./output/$MODEL_NAME-$SETTING-$SEQ_LENGTH-$SUB_LABEL \
--seed 2027 \
--model $MODEL \
--log-path $SETTING-$SEQ_LENGTH-$MODEL_NAME-$SUB_LABEL.log \
--stage 2
You can also run
bash ./train_scirpts/train_LR_llama3_target80k_use24k.sh
after preprocess your data to do the three stage in one command.
If you find this repo helpful, please cite our paper as follows:
@article{hu2024longrecipe,
title={LongRecipe: Recipe for Efficient Long Context Generalization in Large Languge Models},
author={Zhiyuan Hu, Yuliang Liu, Jinman Zhao, Suyuchen Wang, Yan Wang, Wei Shen, Qing Gu, Anh Tuan Luu, See-Kiong Ng, Zhiwei Jiang, Bryan Hooi},
journal={arXiv preprint arXiv:2409.00509},
year={2024}
}
For Tasks:
Click tags to check more tools for each tasksFor Jobs:
Alternative AI tools for LongRecipe
Similar Open Source Tools
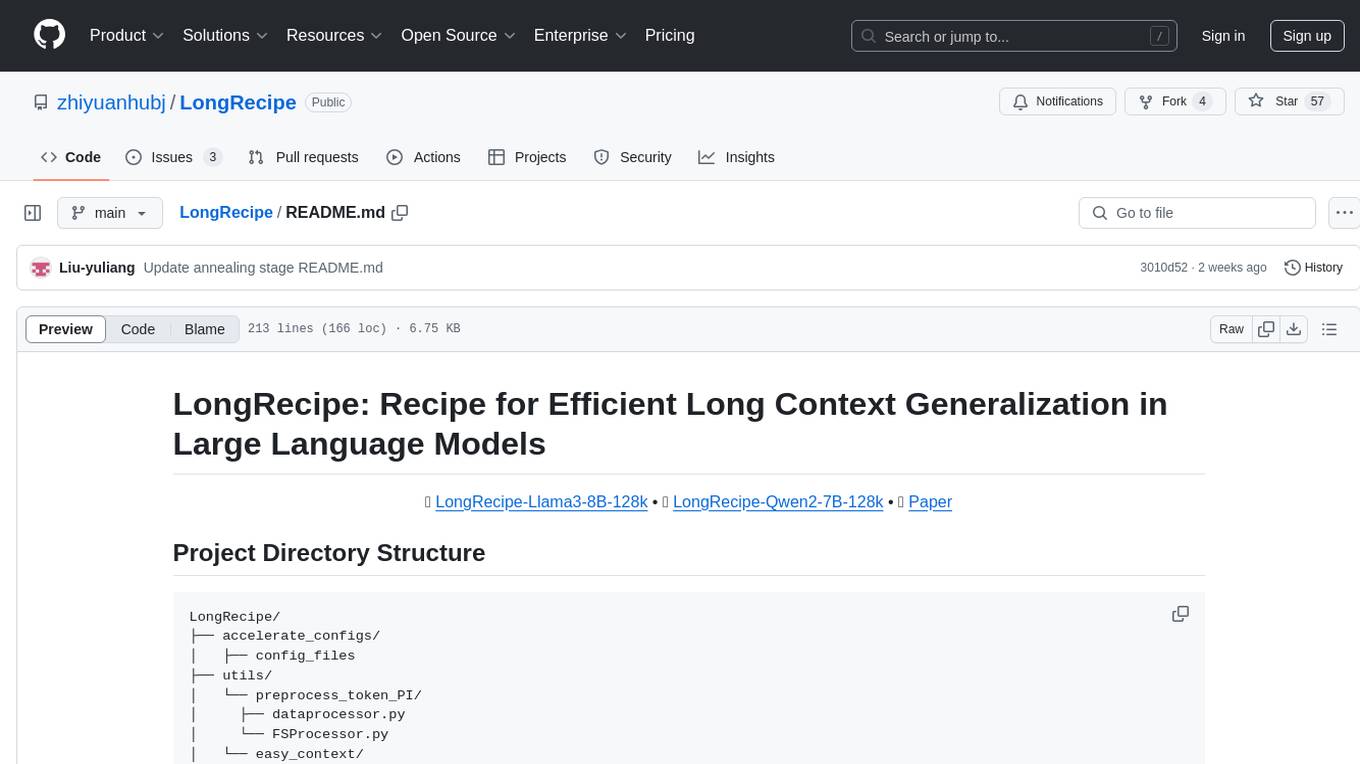
LongRecipe
LongRecipe is a tool designed for efficient long context generalization in large language models. It provides a recipe for extending the context window of language models while maintaining their original capabilities. The tool includes data preprocessing steps, model training stages, and a process for merging fine-tuned models to enhance foundational capabilities. Users can follow the provided commands and scripts to preprocess data, train models in multiple stages, and merge models effectively.
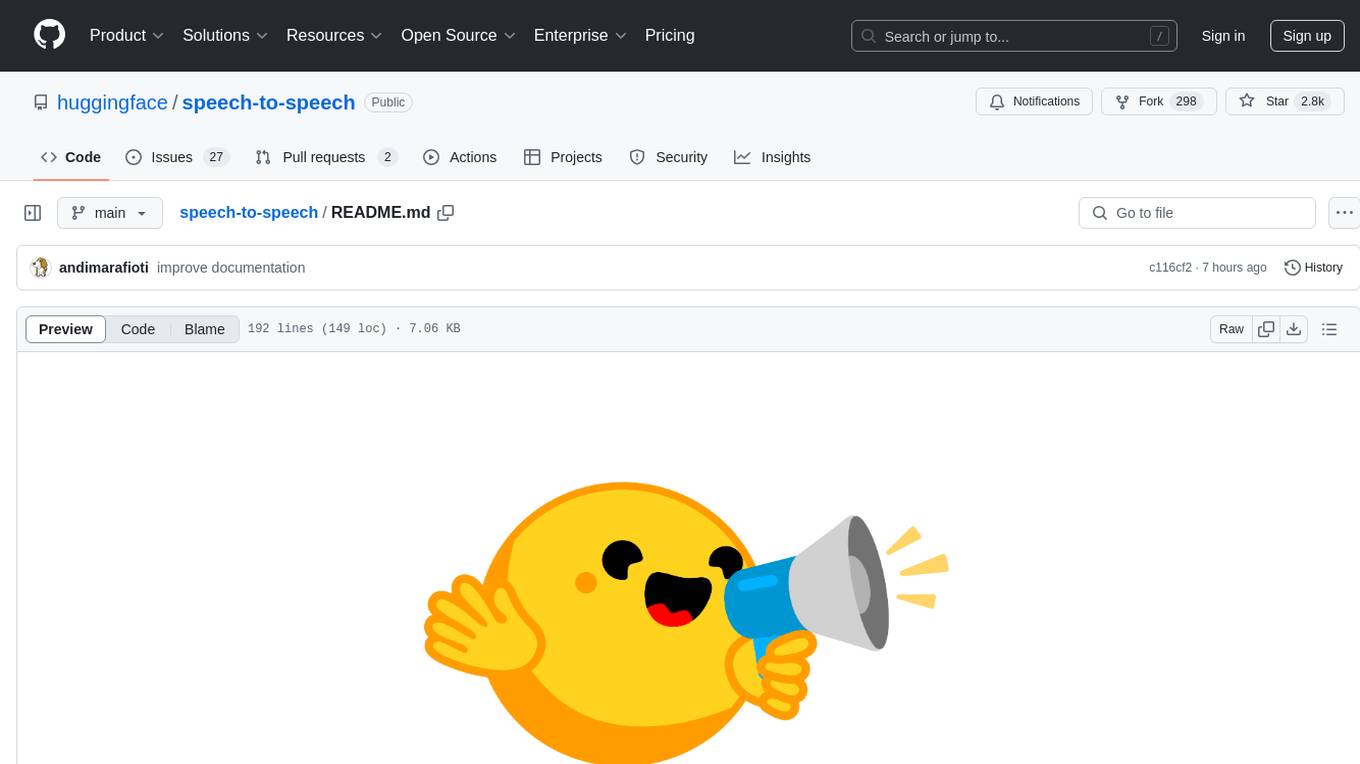
speech-to-speech
This repository implements a speech-to-speech cascaded pipeline with consecutive parts including Voice Activity Detection (VAD), Speech to Text (STT), Language Model (LM), and Text to Speech (TTS). It aims to provide a fully open and modular approach by leveraging models available on the Transformers library via the Hugging Face hub. The code is designed for easy modification, with each component implemented as a class. Users can run the pipeline either on a server/client approach or locally, with detailed setup and usage instructions provided in the readme.
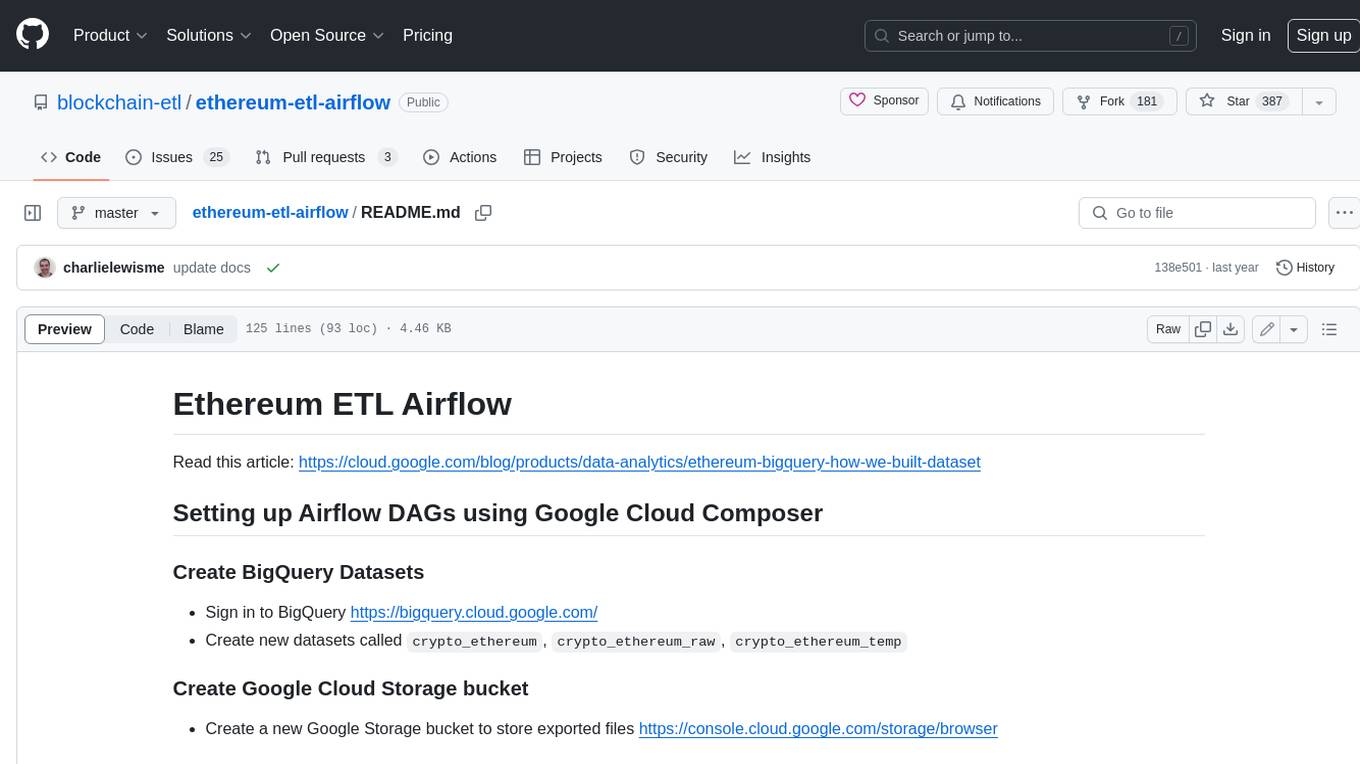
ethereum-etl-airflow
This repository contains Airflow DAGs for extracting, transforming, and loading (ETL) data from the Ethereum blockchain into BigQuery. The DAGs use the Google Cloud Platform (GCP) services, including BigQuery, Cloud Storage, and Cloud Composer, to automate the ETL process. The repository also includes scripts for setting up the GCP environment and running the DAGs locally.
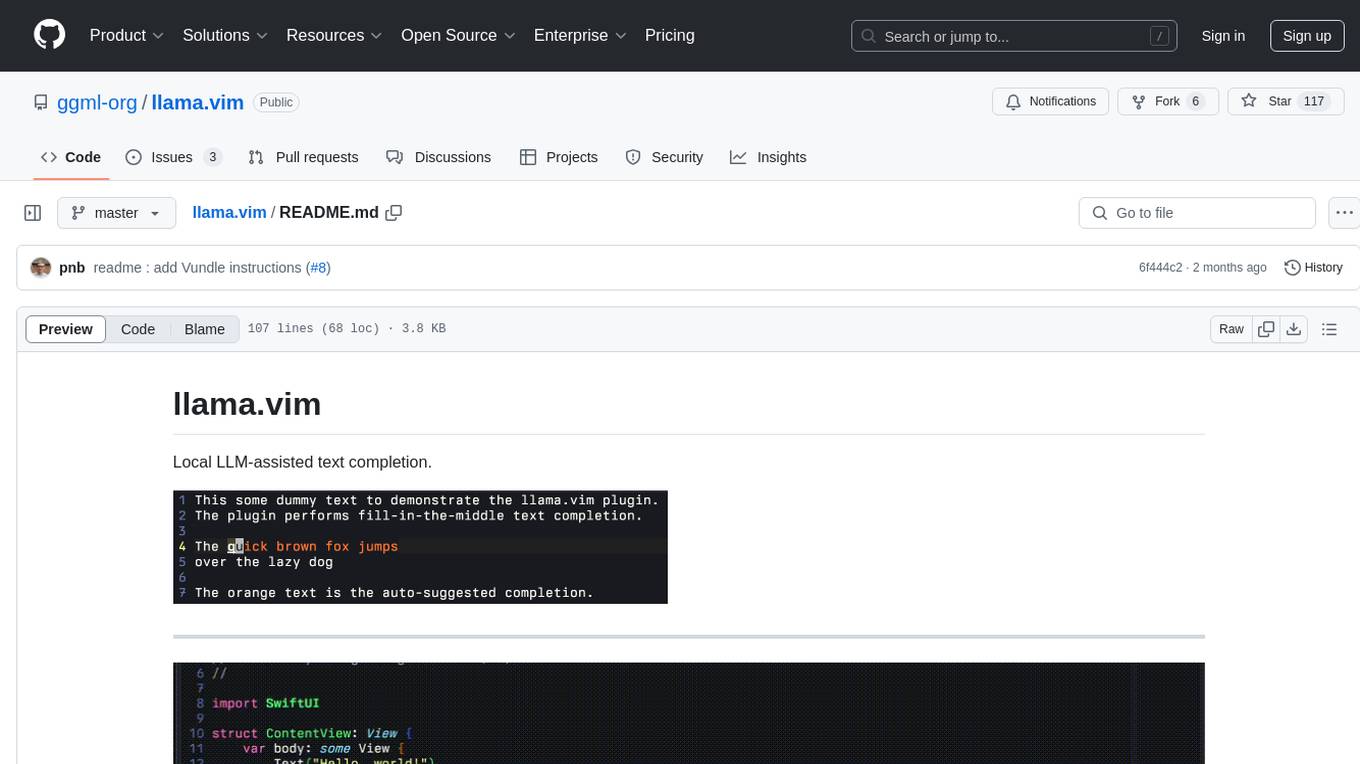
llama.vim
llama.vim is a plugin that provides local LLM-assisted text completion for Vim users. It offers features such as auto-suggest on cursor movement, manual suggestion toggling, suggestion acceptance with Tab and Shift+Tab, control over text generation time, context configuration, ring context with chunks from open and edited files, and performance stats display. The plugin requires a llama.cpp server instance to be running and supports FIM-compatible models. It aims to be simple, lightweight, and provide high-quality and performant local FIM completions even on consumer-grade hardware.
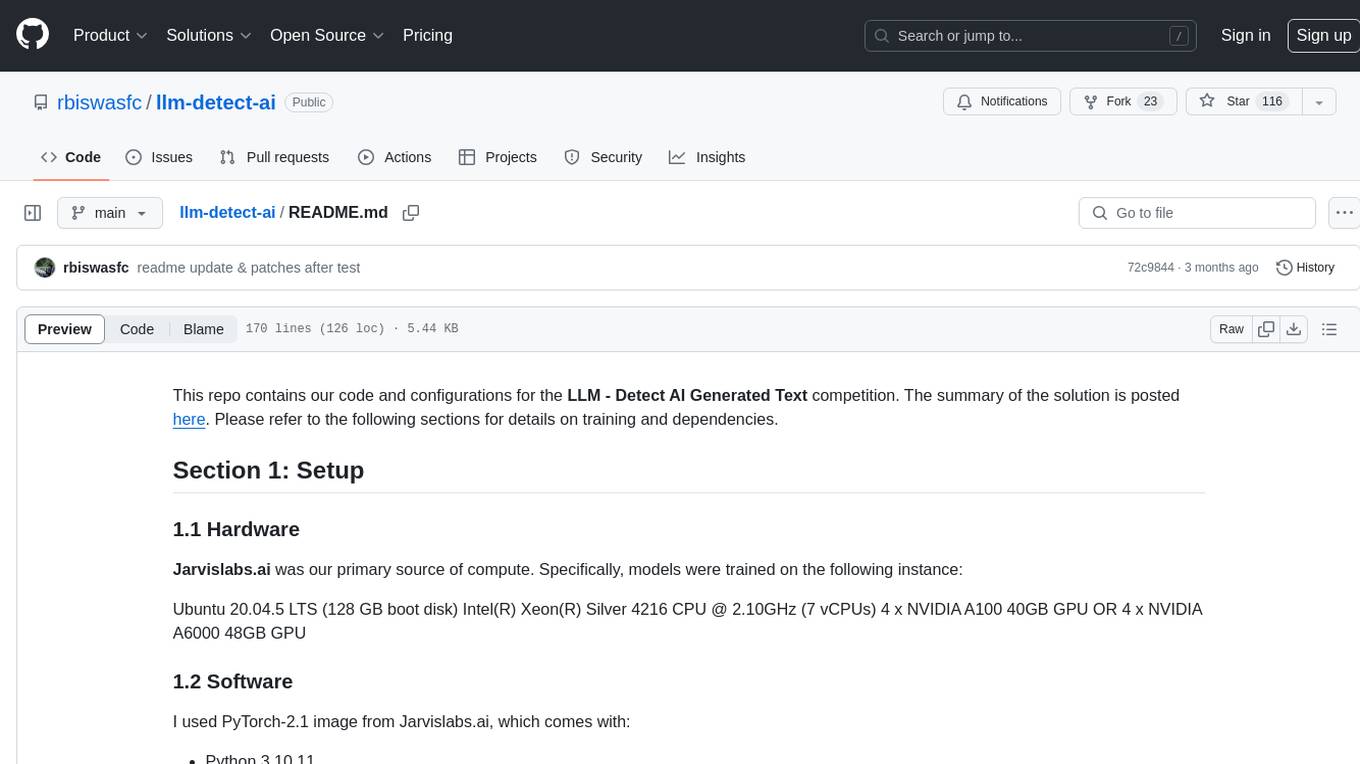
llm-detect-ai
This repository contains code and configurations for the LLM - Detect AI Generated Text competition. It includes setup instructions for hardware, software, dependencies, and datasets. The training section covers scripts and configurations for training LLM models, DeBERTa ranking models, and an embedding model. Text generation section details fine-tuning LLMs using the CLM objective on the PERSUADE corpus to generate student-like essays.
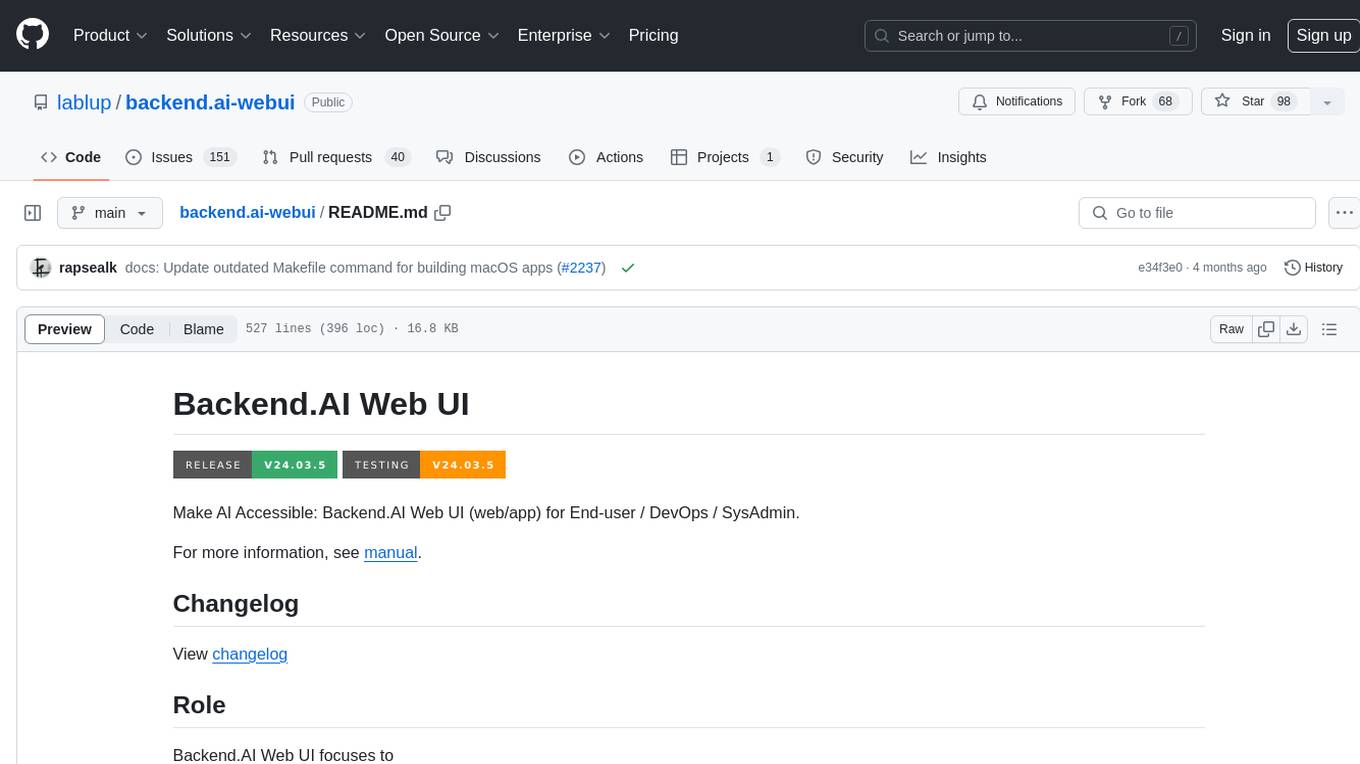
backend.ai-webui
Backend.AI Web UI is a user-friendly web and app interface designed to make AI accessible for end-users, DevOps, and SysAdmins. It provides features for session management, inference service management, pipeline management, storage management, node management, statistics, configurations, license checking, plugins, help & manuals, kernel management, user management, keypair management, manager settings, proxy mode support, service information, and integration with the Backend.AI Web Server. The tool supports various devices, offers a built-in websocket proxy feature, and allows for versatile usage across different platforms. Users can easily manage resources, run environment-supported apps, access a web-based terminal, use Visual Studio Code editor, manage experiments, set up autoscaling, manage pipelines, handle storage, monitor nodes, view statistics, configure settings, and more.
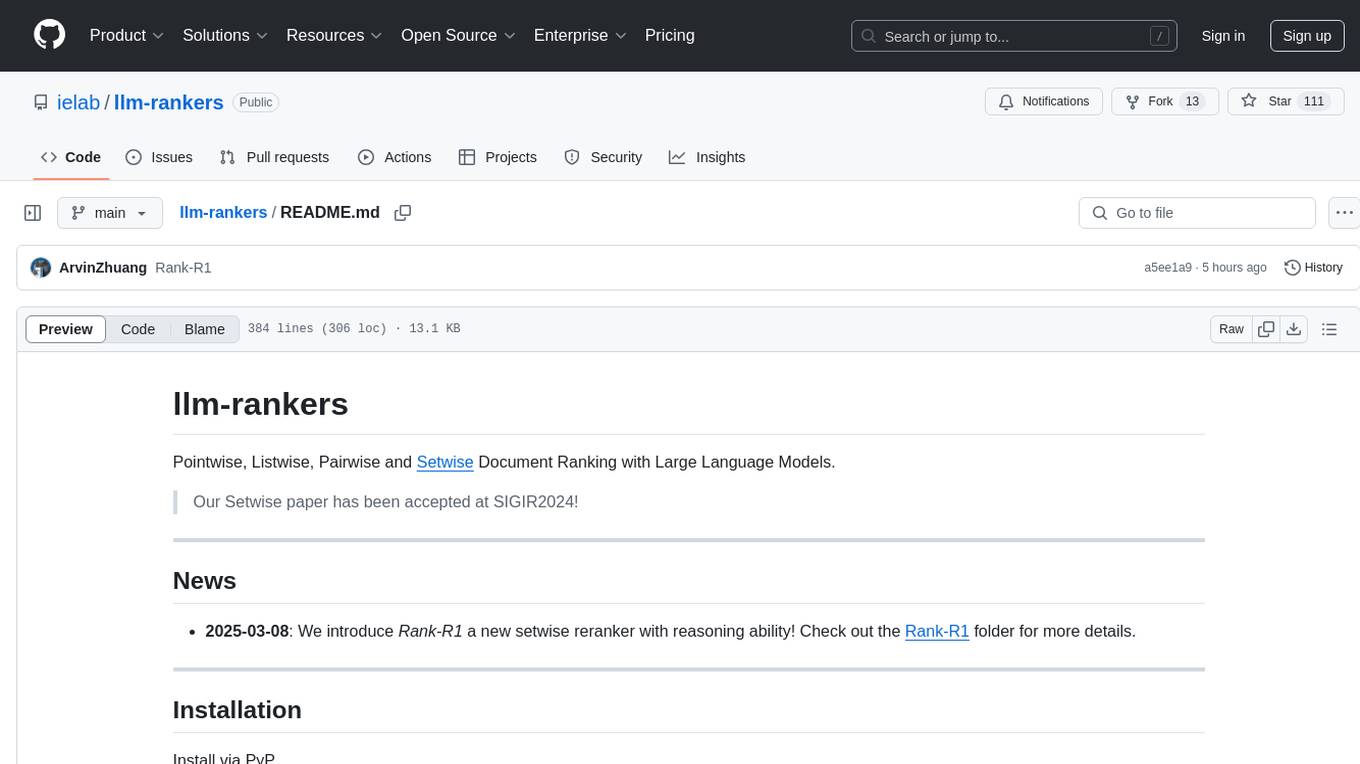
llm-rankers
llm-rankers is a repository that provides implementations for Pointwise, Listwise, Pairwise, and Setwise Document Ranking using Large Language Models. It includes various methods for reranking documents retrieved by a first-stage retriever, such as BM25. The repository offers examples and code snippets for using LLMs to improve document ranking performance in information retrieval tasks. Additionally, it introduces a new setwise reranker called Rank-R1 with reasoning ability.
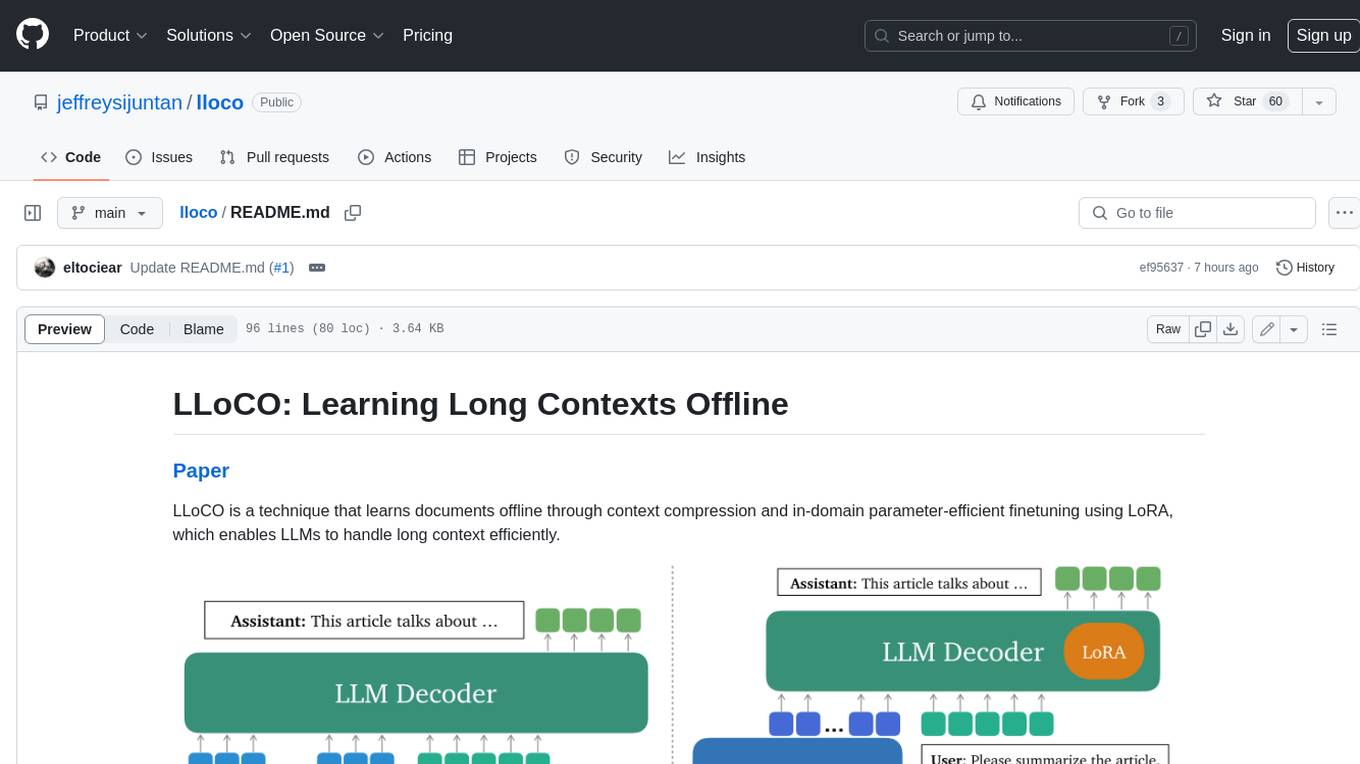
lloco
LLoCO is a technique that learns documents offline through context compression and in-domain parameter-efficient finetuning using LoRA, which enables LLMs to handle long context efficiently.
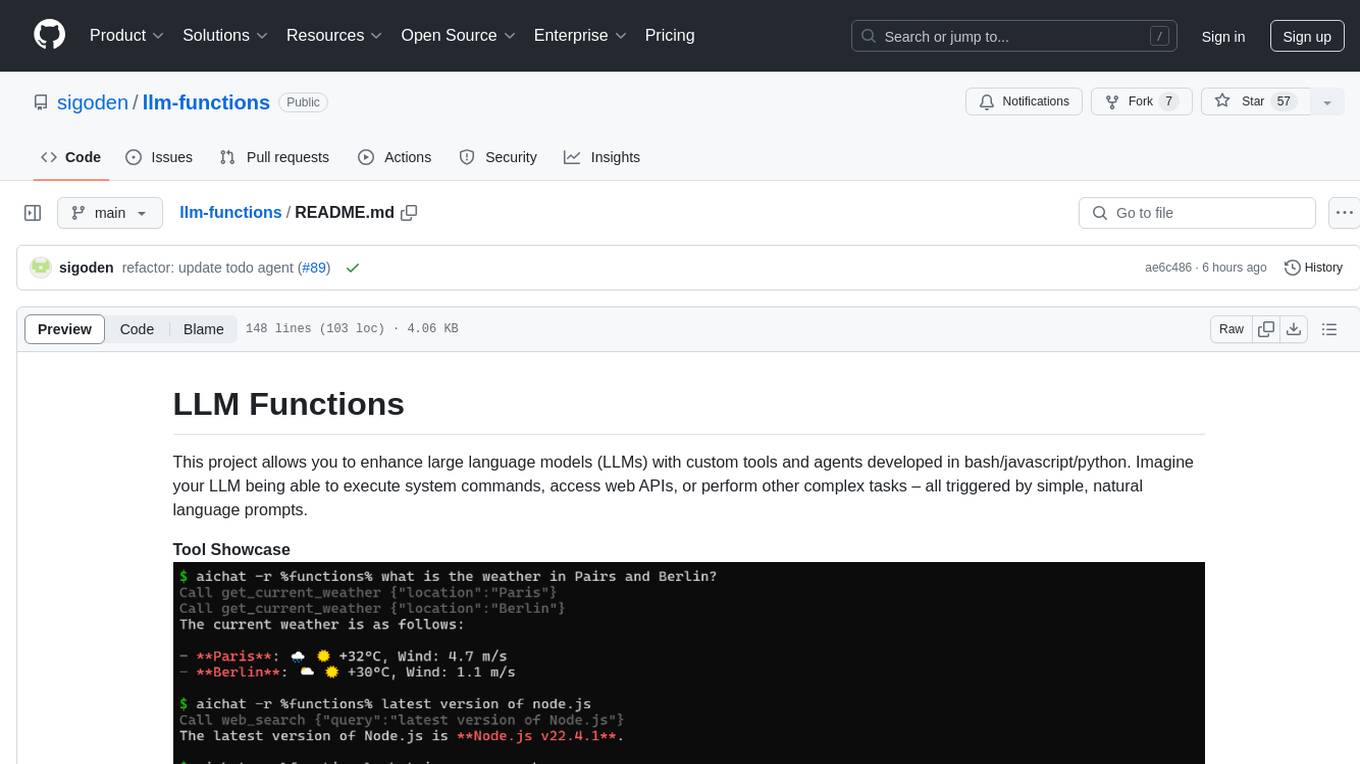
llm-functions
LLM Functions is a project that enables the enhancement of large language models (LLMs) with custom tools and agents developed in bash, javascript, and python. Users can create tools for their LLM to execute system commands, access web APIs, or perform other complex tasks triggered by natural language prompts. The project provides a framework for building tools and agents, with tools being functions written in the user's preferred language and automatically generating JSON declarations based on comments. Agents combine prompts, function callings, and knowledge (RAG) to create conversational AI agents. The project is designed to be user-friendly and allows users to easily extend the capabilities of their language models.
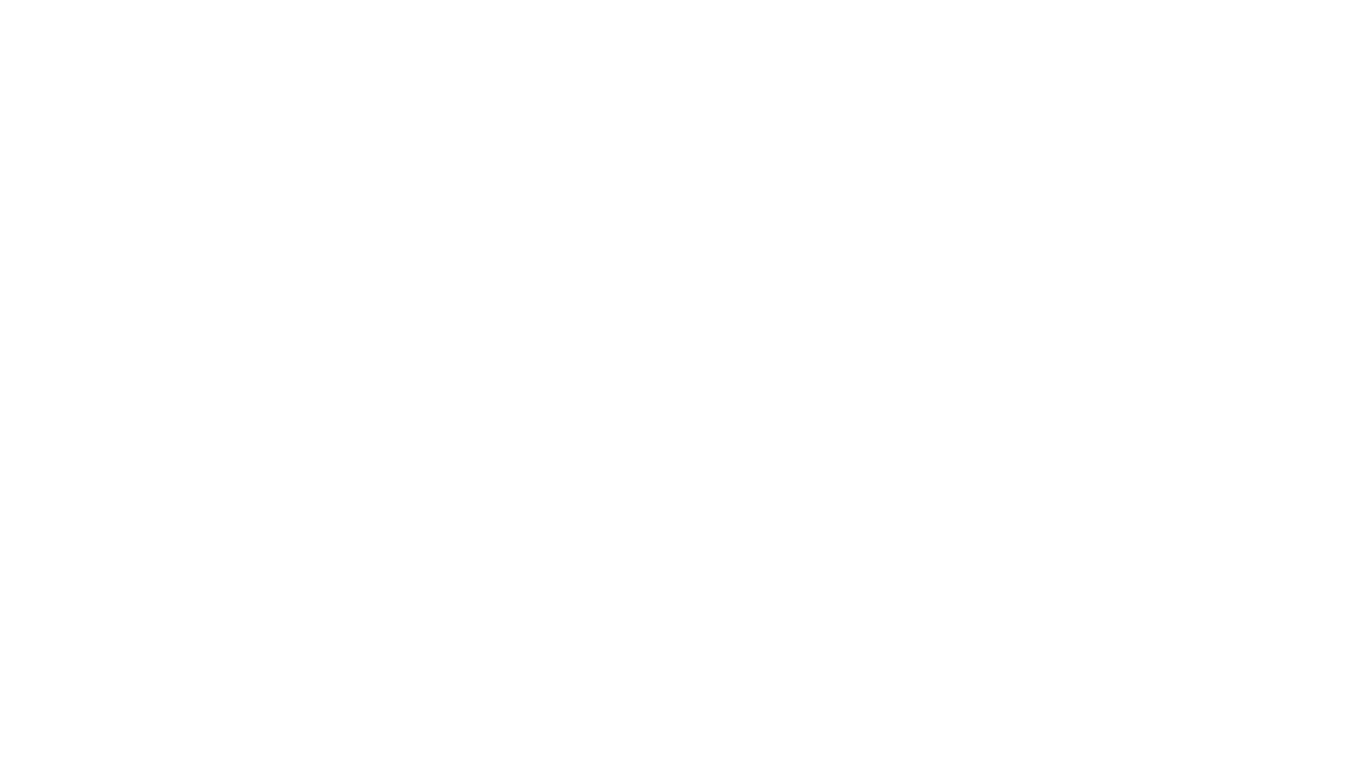
upgini
Upgini is an intelligent data search engine with a Python library that helps users find and add relevant features to their ML pipeline from various public, community, and premium external data sources. It automates the optimization of connected data sources by generating an optimal set of machine learning features using large language models, GraphNNs, and recurrent neural networks. The tool aims to simplify feature search and enrichment for external data to make it a standard approach in machine learning pipelines. It democratizes access to data sources for the data science community.
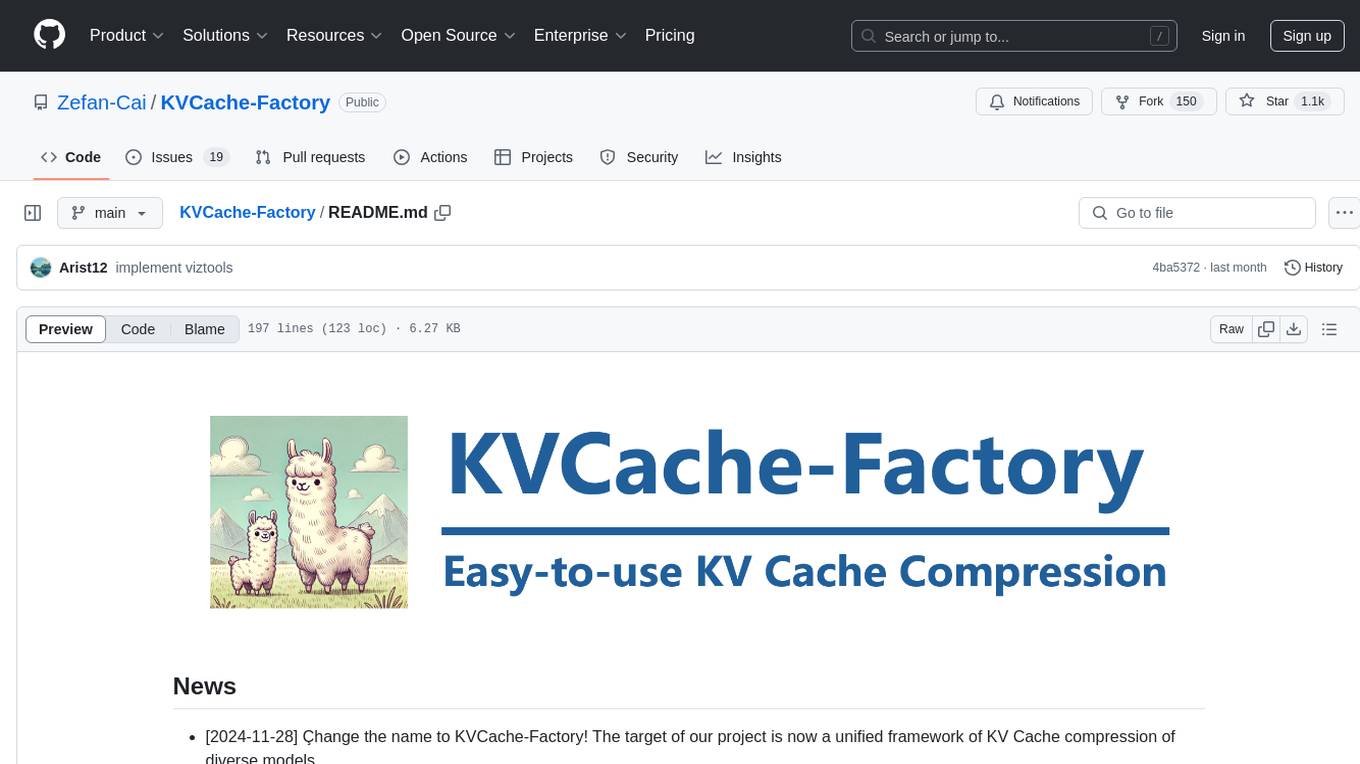
KVCache-Factory
KVCache-Factory is a unified framework for KV Cache compression of diverse models. It supports multi-GPUs inference with big LLMs and various attention implementations. The tool enables KV cache compression without Flash Attention v2, multi-GPU inference, and specific models like Mistral. It also provides functions for KV cache budget allocation and batch inference. The visualization tools help in understanding the attention patterns of models.
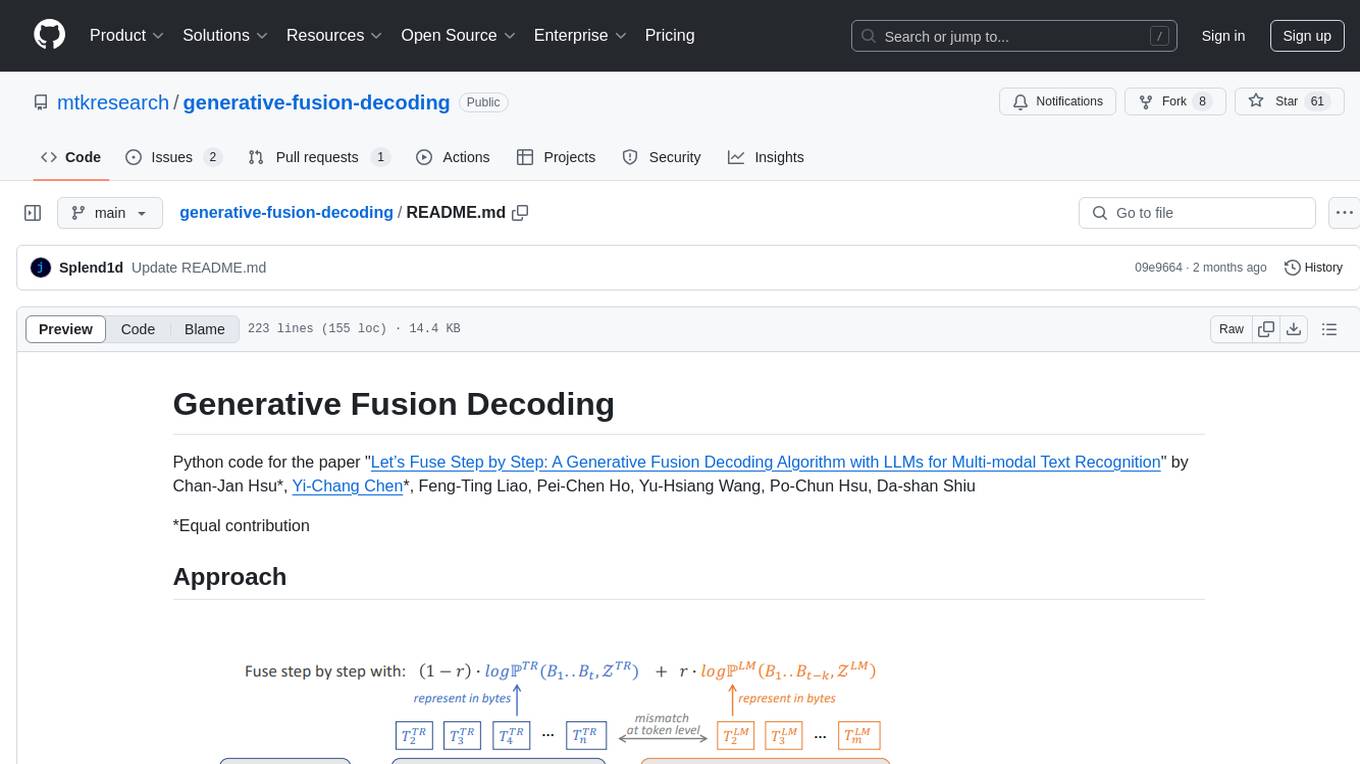
generative-fusion-decoding
Generative Fusion Decoding (GFD) is a novel shallow fusion framework that integrates Large Language Models (LLMs) into multi-modal text recognition systems such as automatic speech recognition (ASR) and optical character recognition (OCR). GFD operates across mismatched token spaces of different models by mapping text token space to byte token space, enabling seamless fusion during the decoding process. It simplifies the complexity of aligning different model sample spaces, allows LLMs to correct errors in tandem with the recognition model, increases robustness in long-form speech recognition, and enables fusing recognition models deficient in Chinese text recognition with LLMs extensively trained on Chinese. GFD significantly improves performance in ASR and OCR tasks, offering a unified solution for leveraging existing pre-trained models through step-by-step fusion.
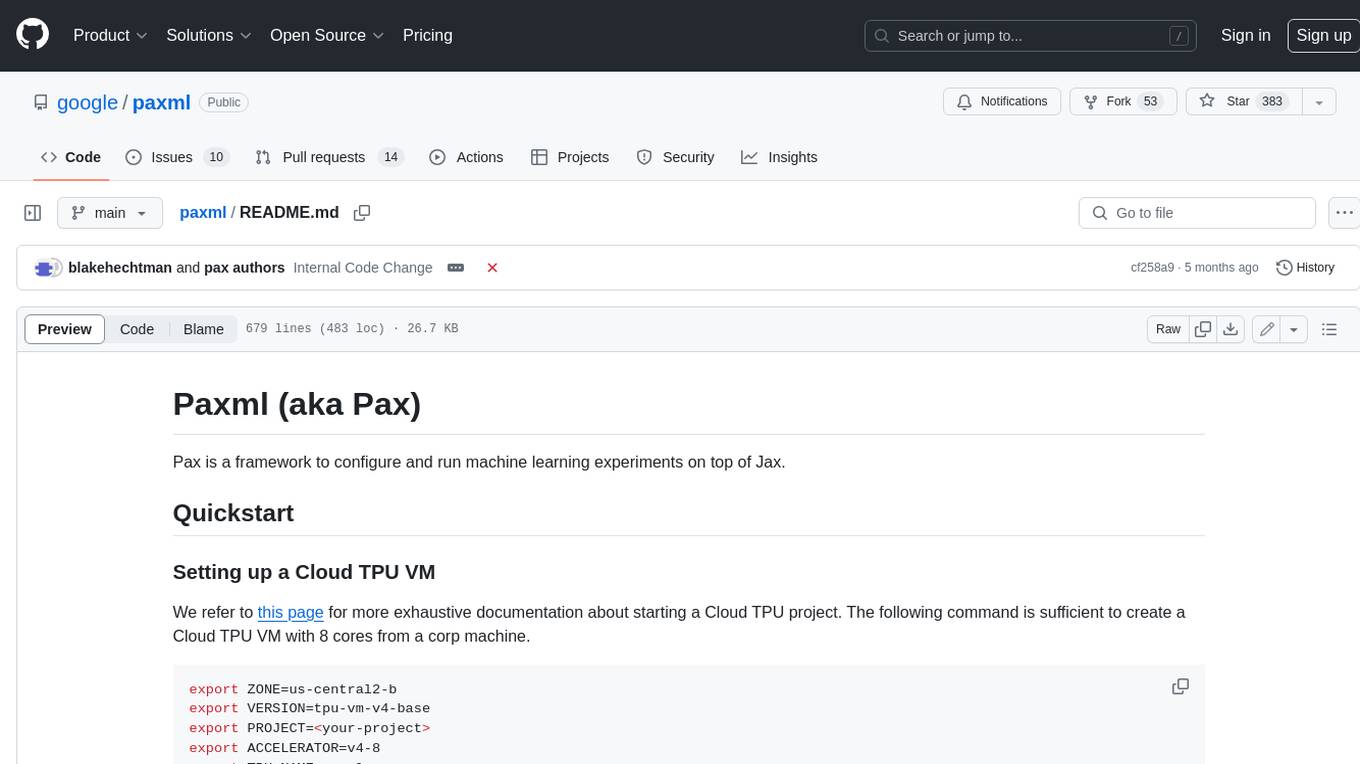
paxml
Pax is a framework to configure and run machine learning experiments on top of Jax.
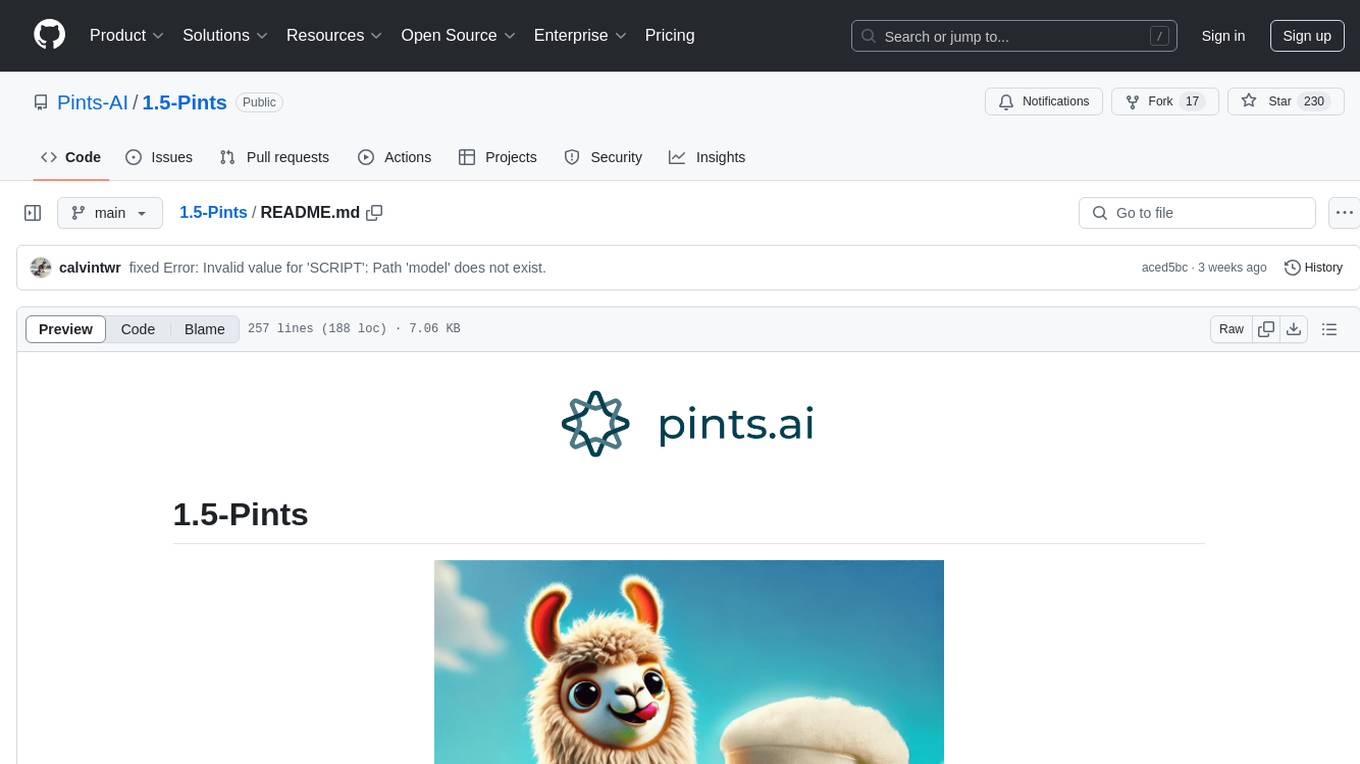
1.5-Pints
1.5-Pints is a repository that provides a recipe to pre-train models in 9 days, aiming to create AI assistants comparable to Apple OpenELM and Microsoft Phi. It includes model architecture, training scripts, and utilities for 1.5-Pints and 0.12-Pint developed by Pints.AI. The initiative encourages replication, experimentation, and open-source development of Pint by sharing the model's codebase and architecture. The repository offers installation instructions, dataset preparation scripts, model training guidelines, and tools for model evaluation and usage. Users can also find information on finetuning models, converting lit models to HuggingFace models, and running Direct Preference Optimization (DPO) post-finetuning. Additionally, the repository includes tests to ensure code modifications do not disrupt the existing functionality.
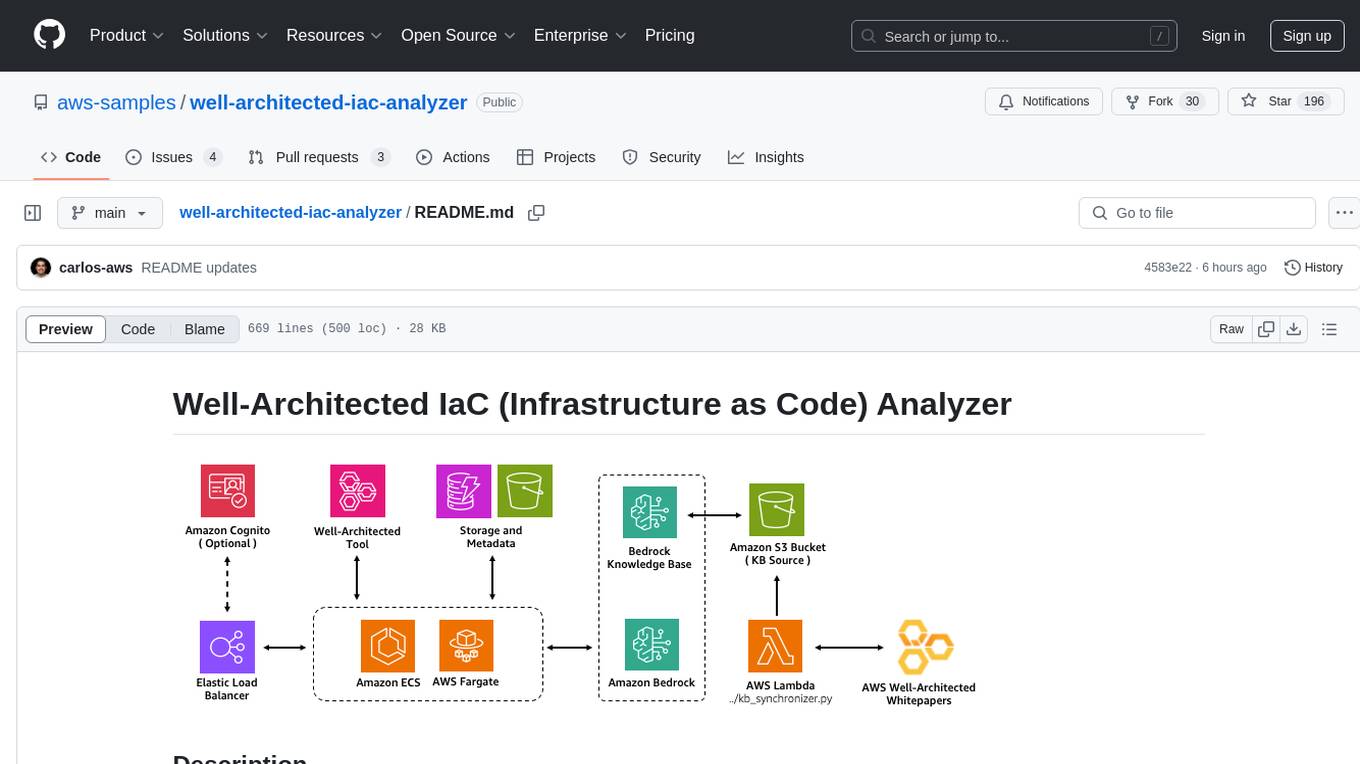
well-architected-iac-analyzer
Well-Architected Infrastructure as Code (IaC) Analyzer is a project demonstrating how generative AI can evaluate infrastructure code for alignment with best practices. It features a modern web application allowing users to upload IaC documents, complete IaC projects, or architecture diagrams for assessment. The tool provides insights into infrastructure code alignment with AWS best practices, offers suggestions for improving cloud architecture designs, and can generate IaC templates from architecture diagrams. Users can analyze CloudFormation, Terraform, or AWS CDK templates, architecture diagrams in PNG or JPEG format, and complete IaC projects with supporting documents. Real-time analysis against Well-Architected best practices, integration with AWS Well-Architected Tool, and export of analysis results and recommendations are included.
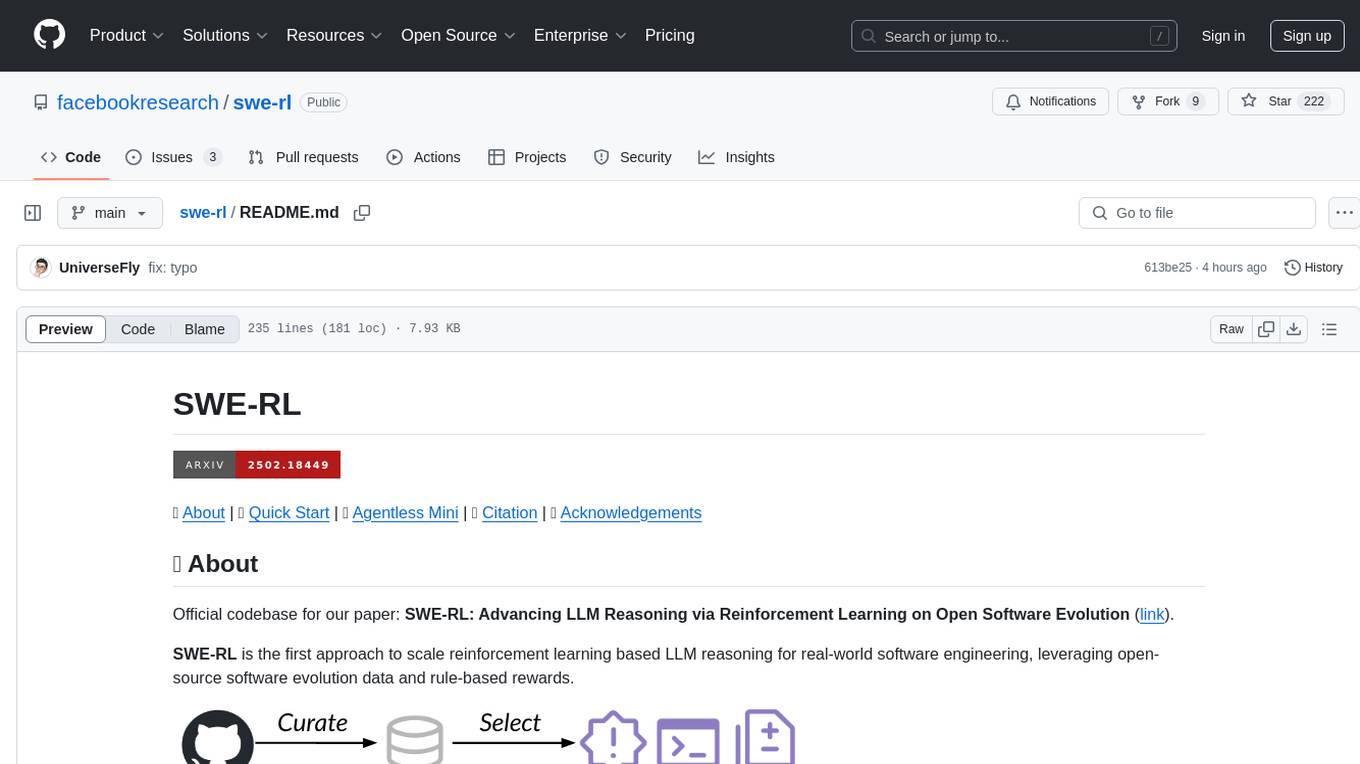
swe-rl
SWE-RL is the official codebase for the paper 'SWE-RL: Advancing LLM Reasoning via Reinforcement Learning on Open Software Evolution'. It is the first approach to scale reinforcement learning based LLM reasoning for real-world software engineering, leveraging open-source software evolution data and rule-based rewards. The code provides prompt templates and the implementation of the reward function based on sequence similarity. Agentless Mini, a part of SWE-RL, builds on top of Agentless with improvements like fast async inference, code refactoring for scalability, and support for using multiple reproduction tests for reranking. The tool can be used for localization, repair, and reproduction test generation in software engineering tasks.
For similar tasks
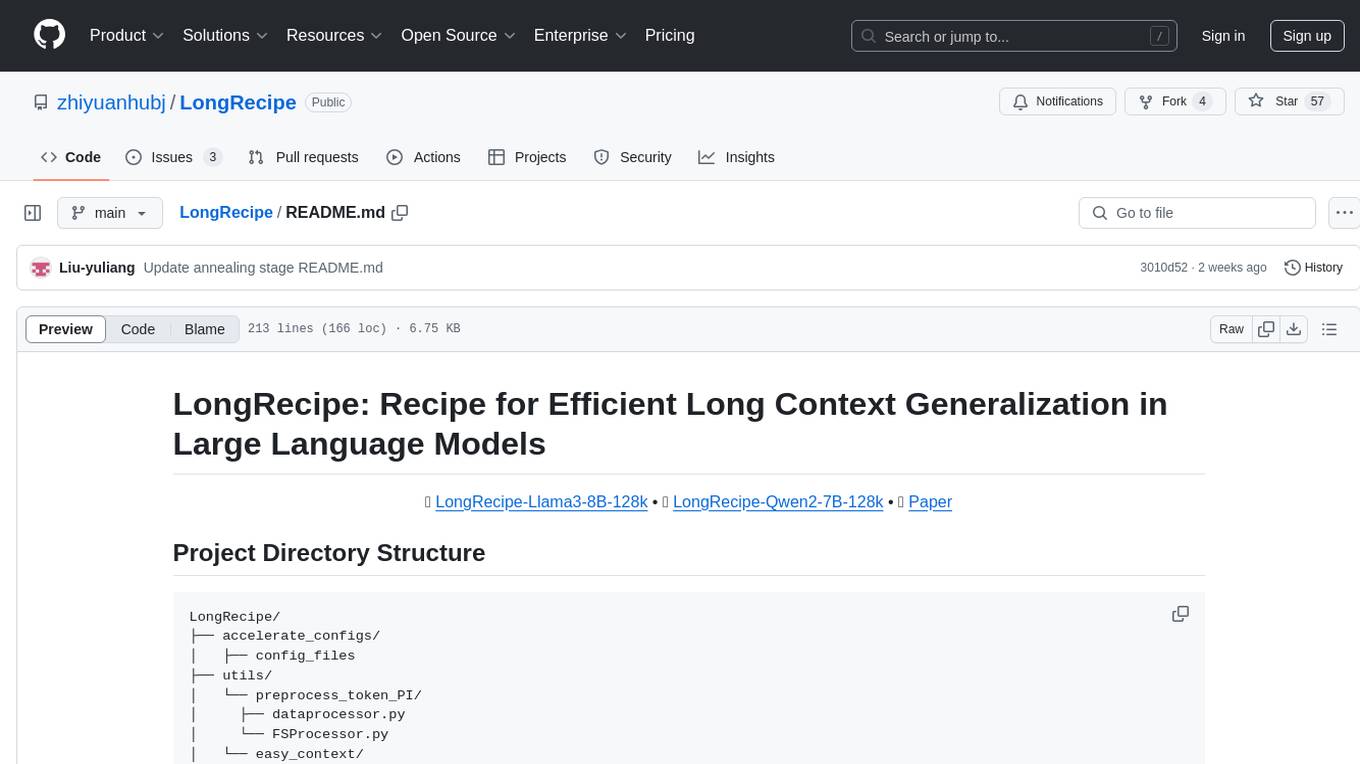
LongRecipe
LongRecipe is a tool designed for efficient long context generalization in large language models. It provides a recipe for extending the context window of language models while maintaining their original capabilities. The tool includes data preprocessing steps, model training stages, and a process for merging fine-tuned models to enhance foundational capabilities. Users can follow the provided commands and scripts to preprocess data, train models in multiple stages, and merge models effectively.
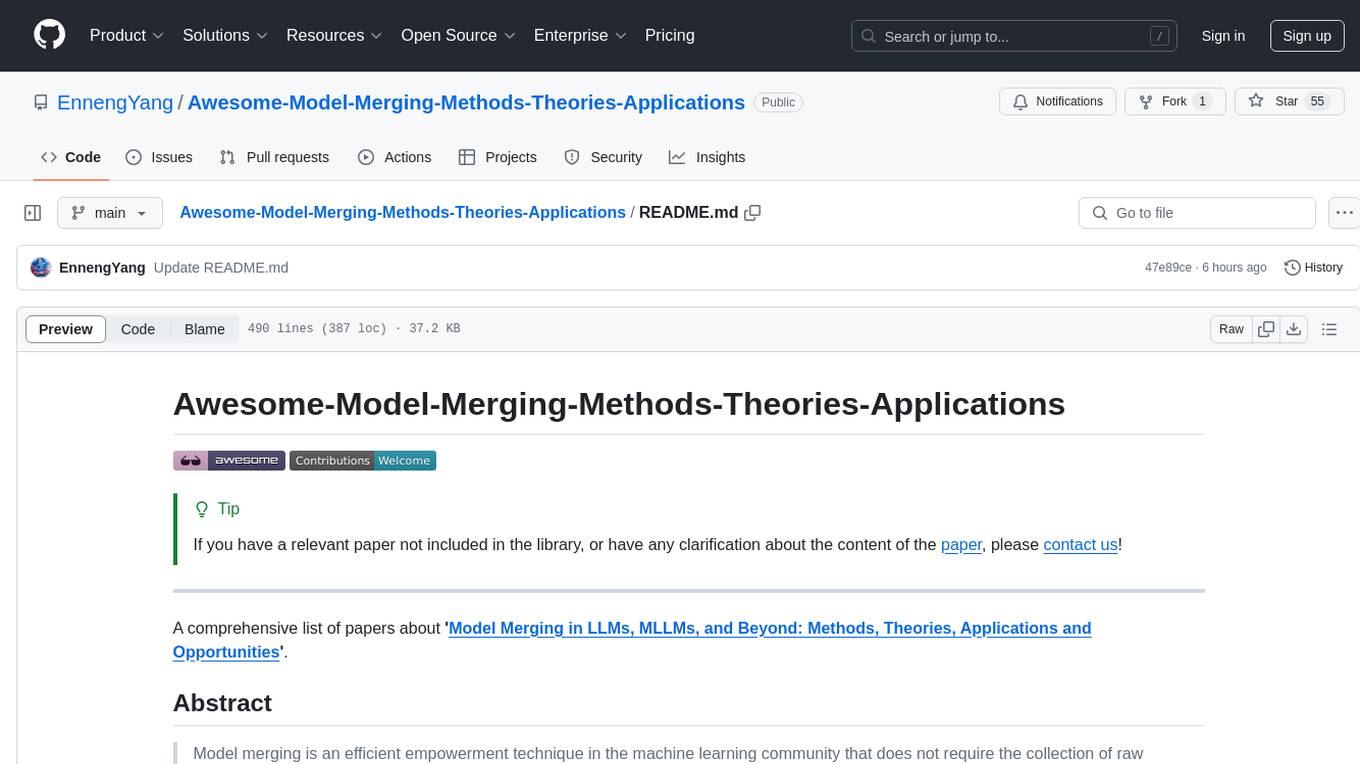
Awesome-Model-Merging-Methods-Theories-Applications
A comprehensive repository focusing on 'Model Merging in LLMs, MLLMs, and Beyond', providing an exhaustive overview of model merging methods, theories, applications, and future research directions. The repository covers various advanced methods, applications in foundation models, different machine learning subfields, and tasks like pre-merging methods, architecture transformation, weight alignment, basic merging methods, and more.
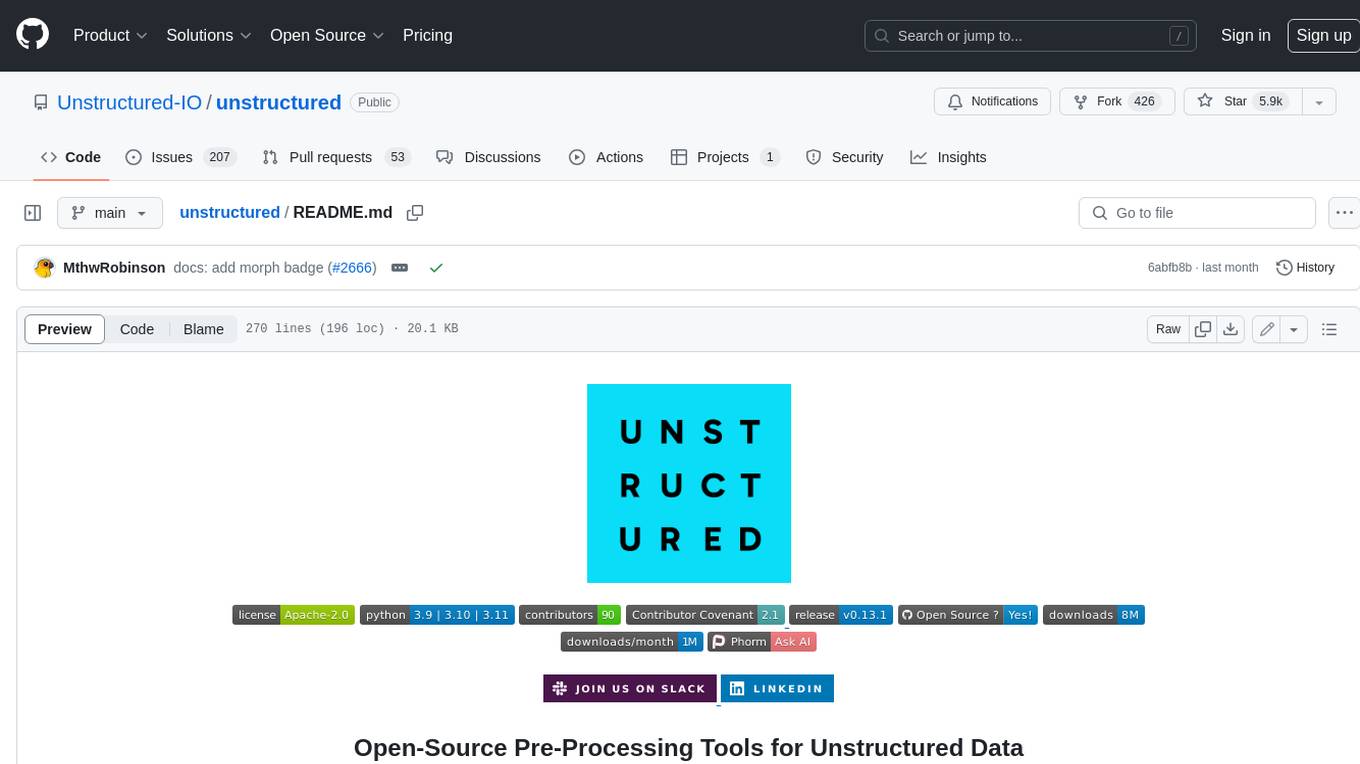
unstructured
The `unstructured` library provides open-source components for ingesting and pre-processing images and text documents, such as PDFs, HTML, Word docs, and many more. The use cases of `unstructured` revolve around streamlining and optimizing the data processing workflow for LLMs. `unstructured` modular functions and connectors form a cohesive system that simplifies data ingestion and pre-processing, making it adaptable to different platforms and efficient in transforming unstructured data into structured outputs.
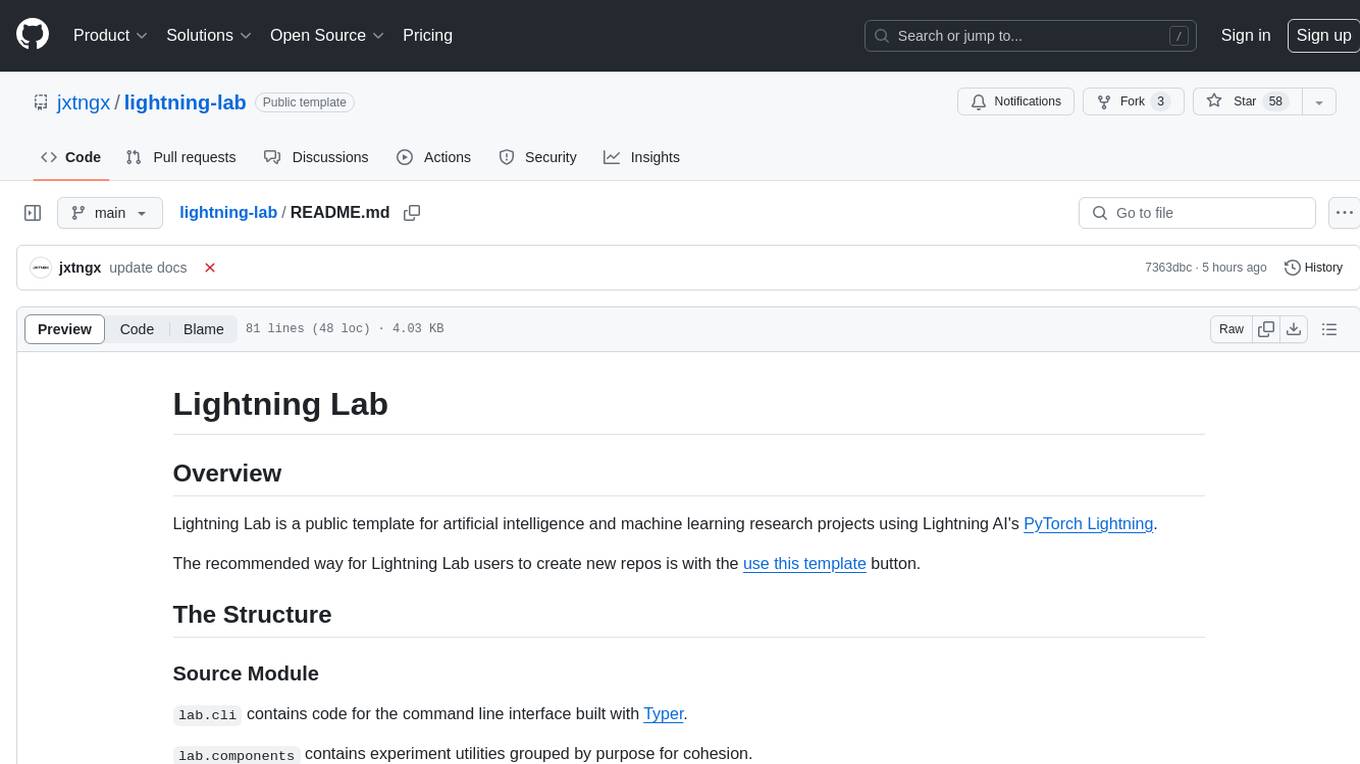
lightning-lab
Lightning Lab is a public template for artificial intelligence and machine learning research projects using Lightning AI's PyTorch Lightning. It provides a structured project layout with modules for command line interface, experiment utilities, Lightning Module and Trainer, data acquisition and preprocessing, model serving APIs, project configurations, training checkpoints, technical documentation, logs, notebooks for data analysis, requirements management, testing, and packaging. The template simplifies the setup of deep learning projects and offers extras for different domains like vision, text, audio, reinforcement learning, and forecasting.
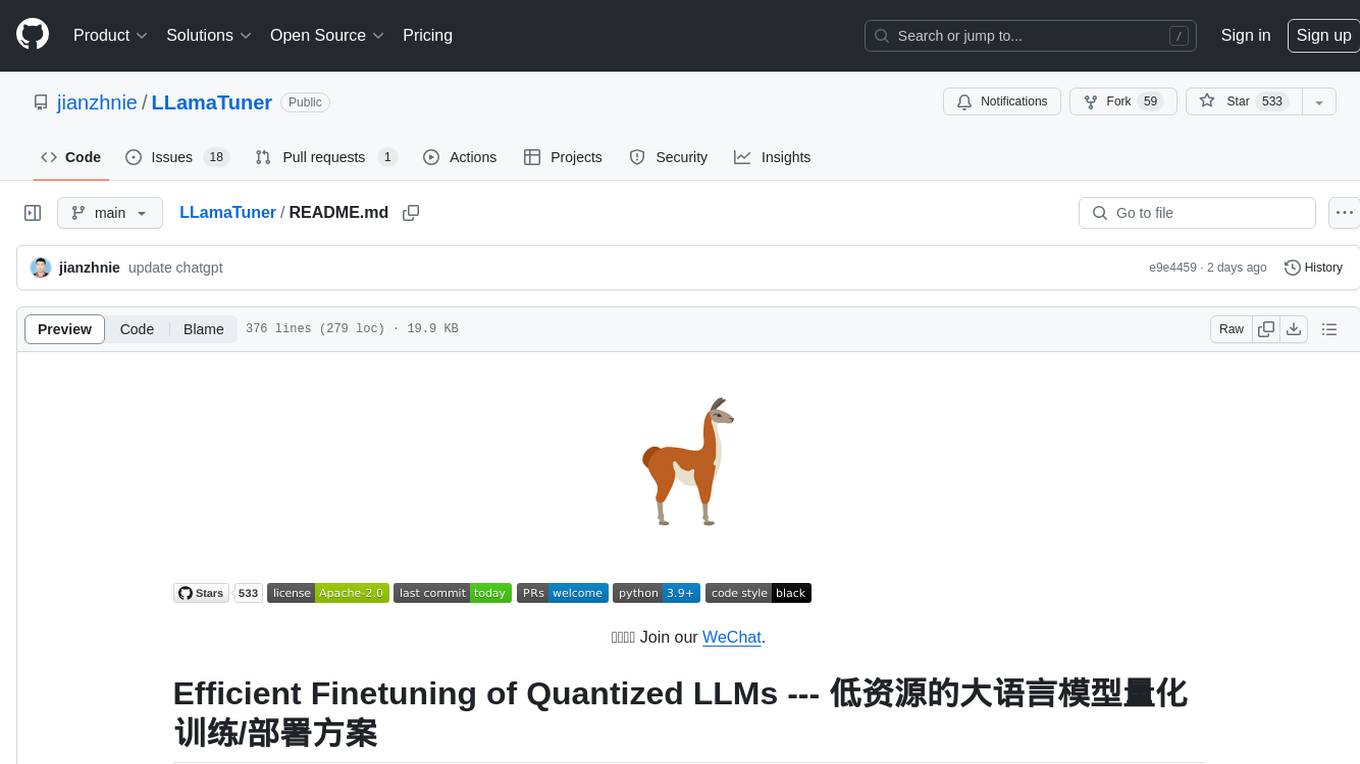
LLamaTuner
LLamaTuner is a repository for the Efficient Finetuning of Quantized LLMs project, focusing on building and sharing instruction-following Chinese baichuan-7b/LLaMA/Pythia/GLM model tuning methods. The project enables training on a single Nvidia RTX-2080TI and RTX-3090 for multi-round chatbot training. It utilizes bitsandbytes for quantization and is integrated with Huggingface's PEFT and transformers libraries. The repository supports various models, training approaches, and datasets for supervised fine-tuning, LoRA, QLoRA, and more. It also provides tools for data preprocessing and offers models in the Hugging Face model hub for inference and finetuning. The project is licensed under Apache 2.0 and acknowledges contributions from various open-source contributors.
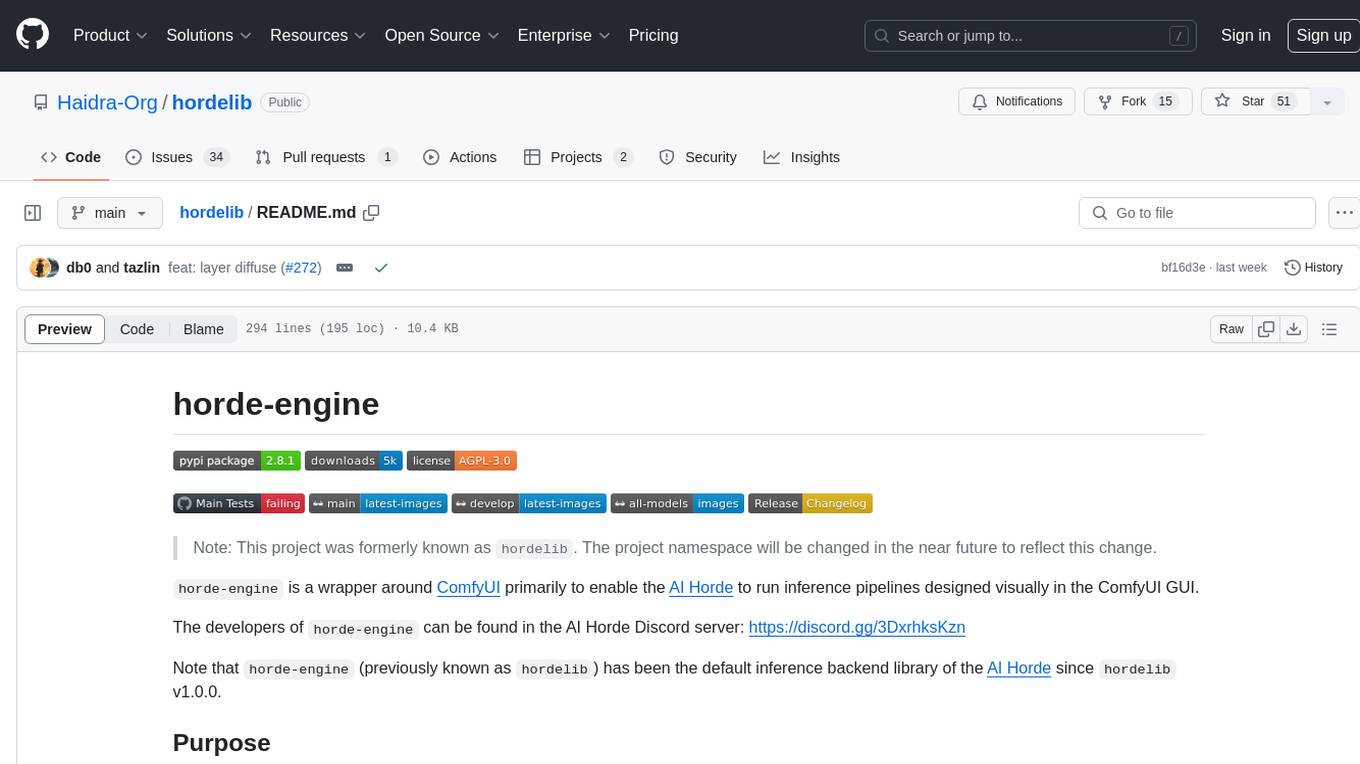
hordelib
horde-engine is a wrapper around ComfyUI designed to run inference pipelines visually designed in the ComfyUI GUI. It enables users to design inference pipelines in ComfyUI and then call them programmatically, maintaining compatibility with the existing horde implementation. The library provides features for processing Horde payloads, initializing the library, downloading and validating models, and generating images based on input data. It also includes custom nodes for preprocessing and tasks such as face restoration and QR code generation. The project depends on various open source projects and bundles some dependencies within the library itself. Users can design ComfyUI pipelines, convert them to the backend format, and run them using the run_image_pipeline() method in hordelib.comfy.Comfy(). The project is actively developed and tested using git, tox, and a specific model directory structure.
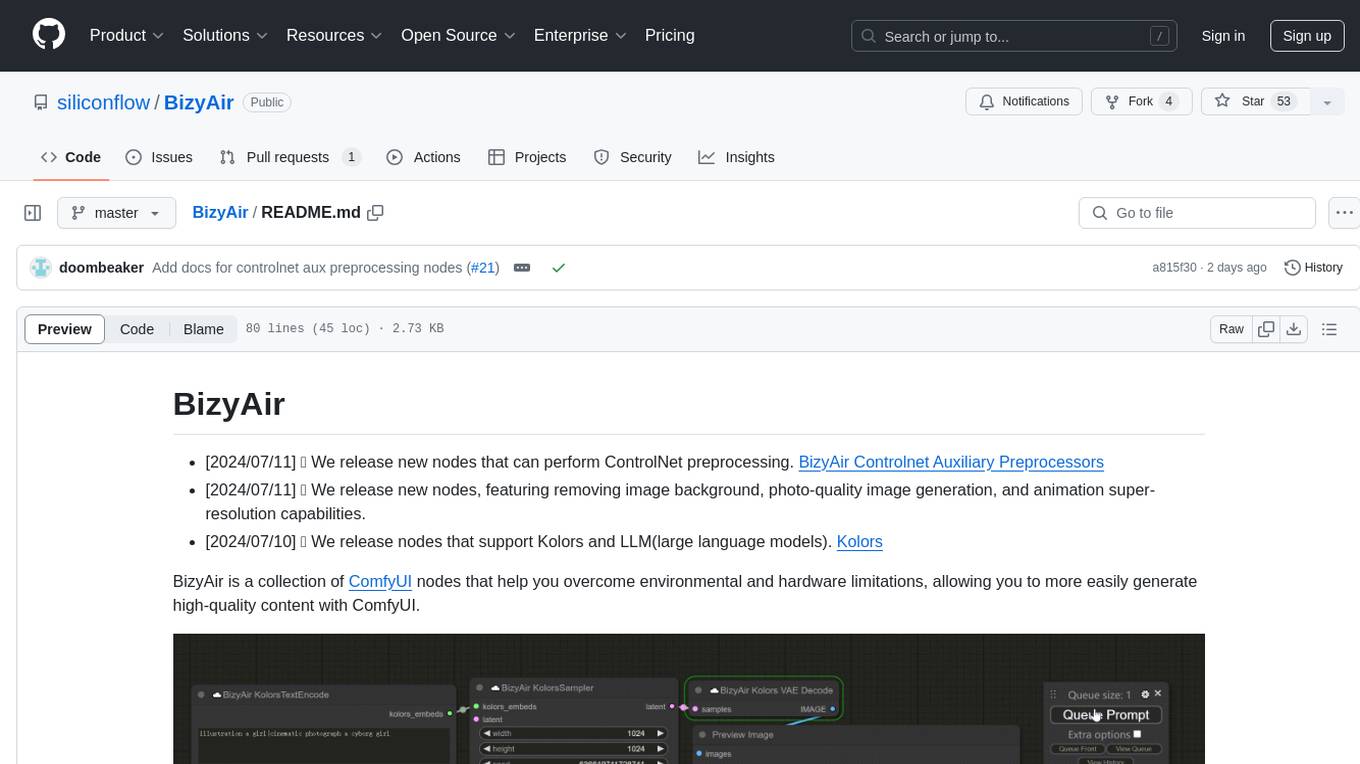
BizyAir
BizyAir is a collection of ComfyUI nodes that help users overcome environmental and hardware limitations to generate high-quality content. It includes features such as ControlNet preprocessing, image background removal, photo-quality image generation, and animation super-resolution. Users can run ComfyUI anywhere without worrying about hardware requirements. Installation methods include using ComfyUI Manager, Comfy CLI, downloading standalone packages for Windows, or cloning the BizyAir repository into the custom_nodes subdirectory of ComfyUI.
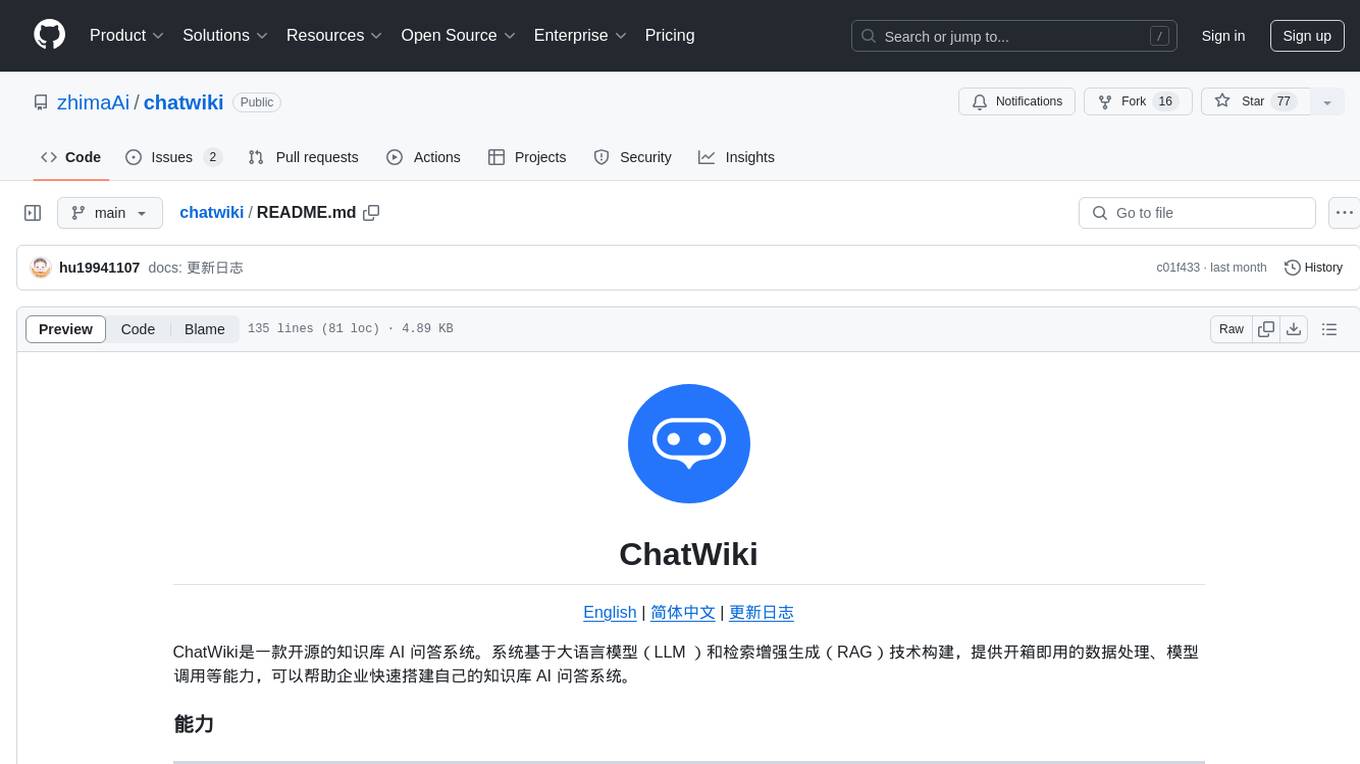
chatwiki
ChatWiki is an open-source knowledge base AI question-answering system. It is built on large language models (LLM) and retrieval-augmented generation (RAG) technologies, providing out-of-the-box data processing, model invocation capabilities, and helping enterprises quickly build their own knowledge base AI question-answering systems. It offers exclusive AI question-answering system, easy integration of models, data preprocessing, simple user interface design, and adaptability to different business scenarios.
For similar jobs
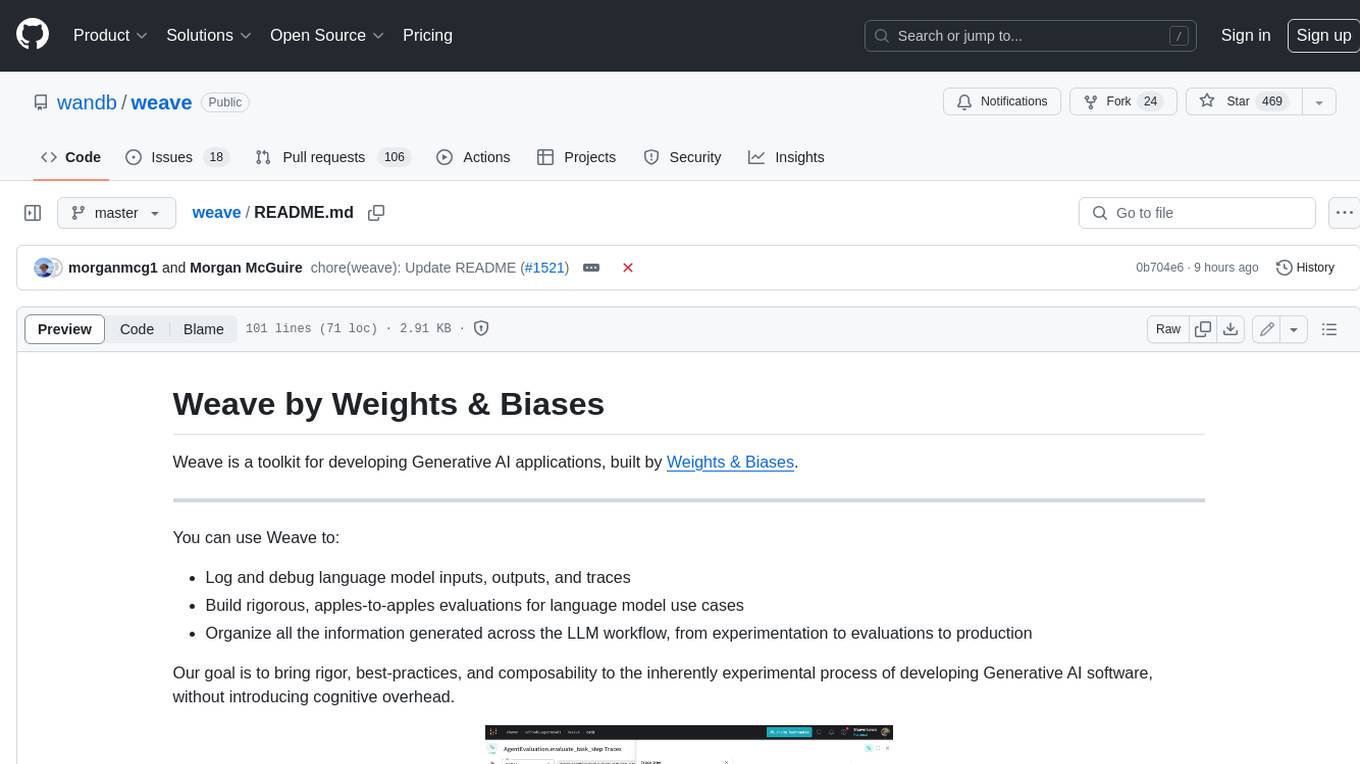
weave
Weave is a toolkit for developing Generative AI applications, built by Weights & Biases. With Weave, you can log and debug language model inputs, outputs, and traces; build rigorous, apples-to-apples evaluations for language model use cases; and organize all the information generated across the LLM workflow, from experimentation to evaluations to production. Weave aims to bring rigor, best-practices, and composability to the inherently experimental process of developing Generative AI software, without introducing cognitive overhead.
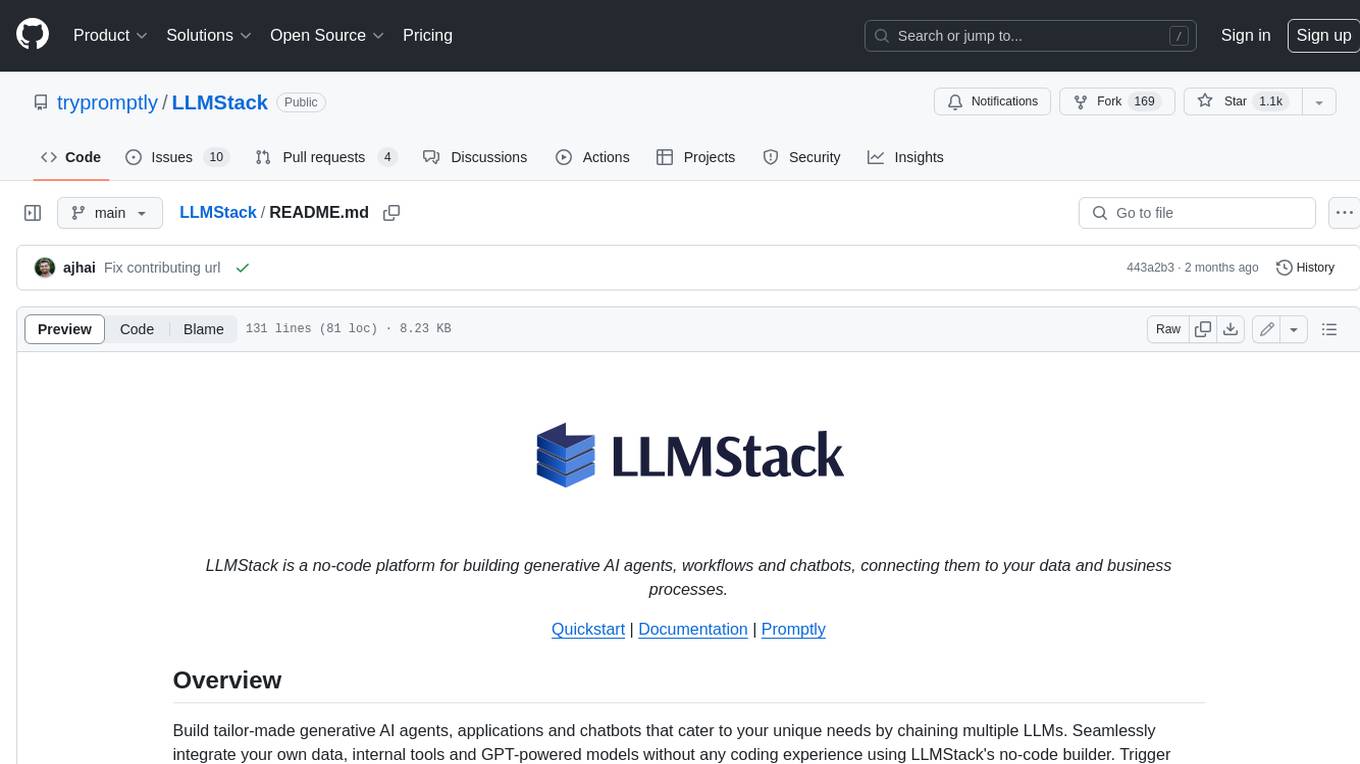
LLMStack
LLMStack is a no-code platform for building generative AI agents, workflows, and chatbots. It allows users to connect their own data, internal tools, and GPT-powered models without any coding experience. LLMStack can be deployed to the cloud or on-premise and can be accessed via HTTP API or triggered from Slack or Discord.
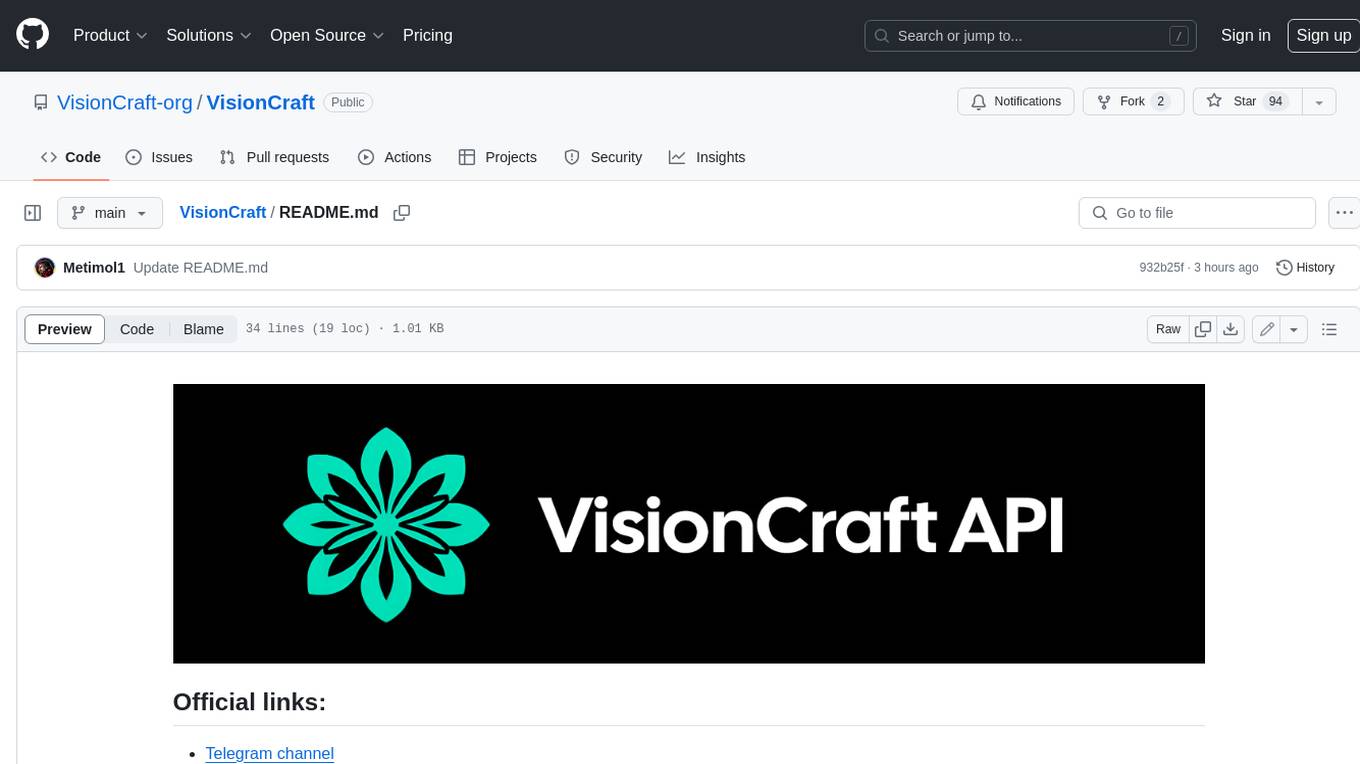
VisionCraft
The VisionCraft API is a free API for using over 100 different AI models. From images to sound.
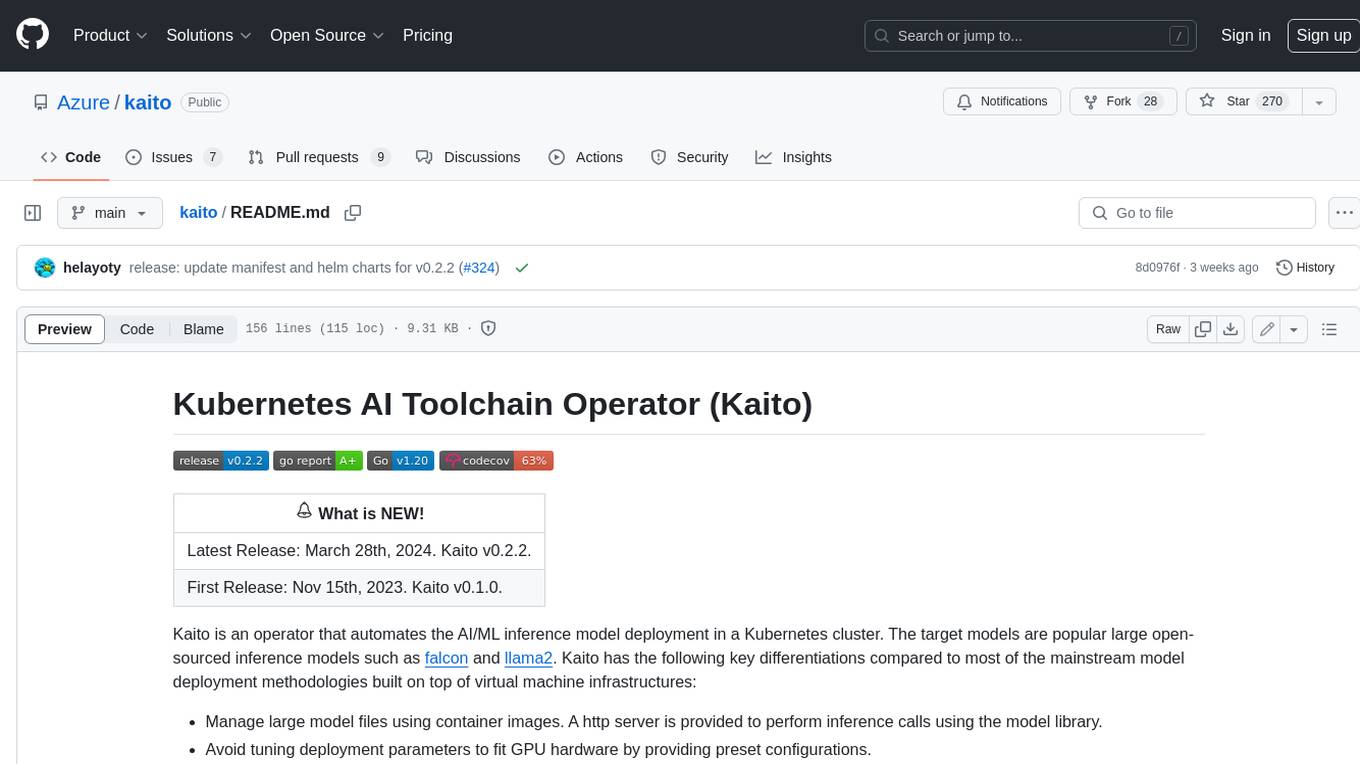
kaito
Kaito is an operator that automates the AI/ML inference model deployment in a Kubernetes cluster. It manages large model files using container images, avoids tuning deployment parameters to fit GPU hardware by providing preset configurations, auto-provisions GPU nodes based on model requirements, and hosts large model images in the public Microsoft Container Registry (MCR) if the license allows. Using Kaito, the workflow of onboarding large AI inference models in Kubernetes is largely simplified.
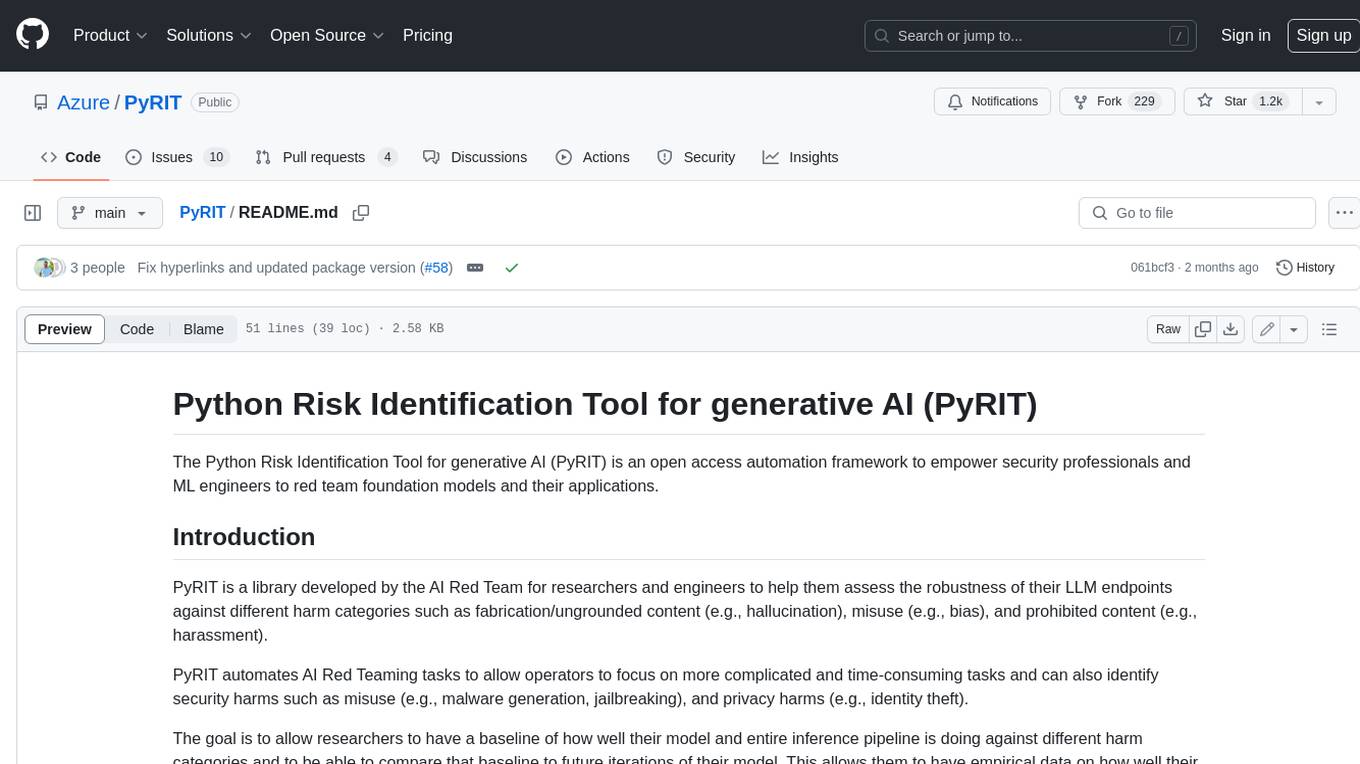
PyRIT
PyRIT is an open access automation framework designed to empower security professionals and ML engineers to red team foundation models and their applications. It automates AI Red Teaming tasks to allow operators to focus on more complicated and time-consuming tasks and can also identify security harms such as misuse (e.g., malware generation, jailbreaking), and privacy harms (e.g., identity theft). The goal is to allow researchers to have a baseline of how well their model and entire inference pipeline is doing against different harm categories and to be able to compare that baseline to future iterations of their model. This allows them to have empirical data on how well their model is doing today, and detect any degradation of performance based on future improvements.
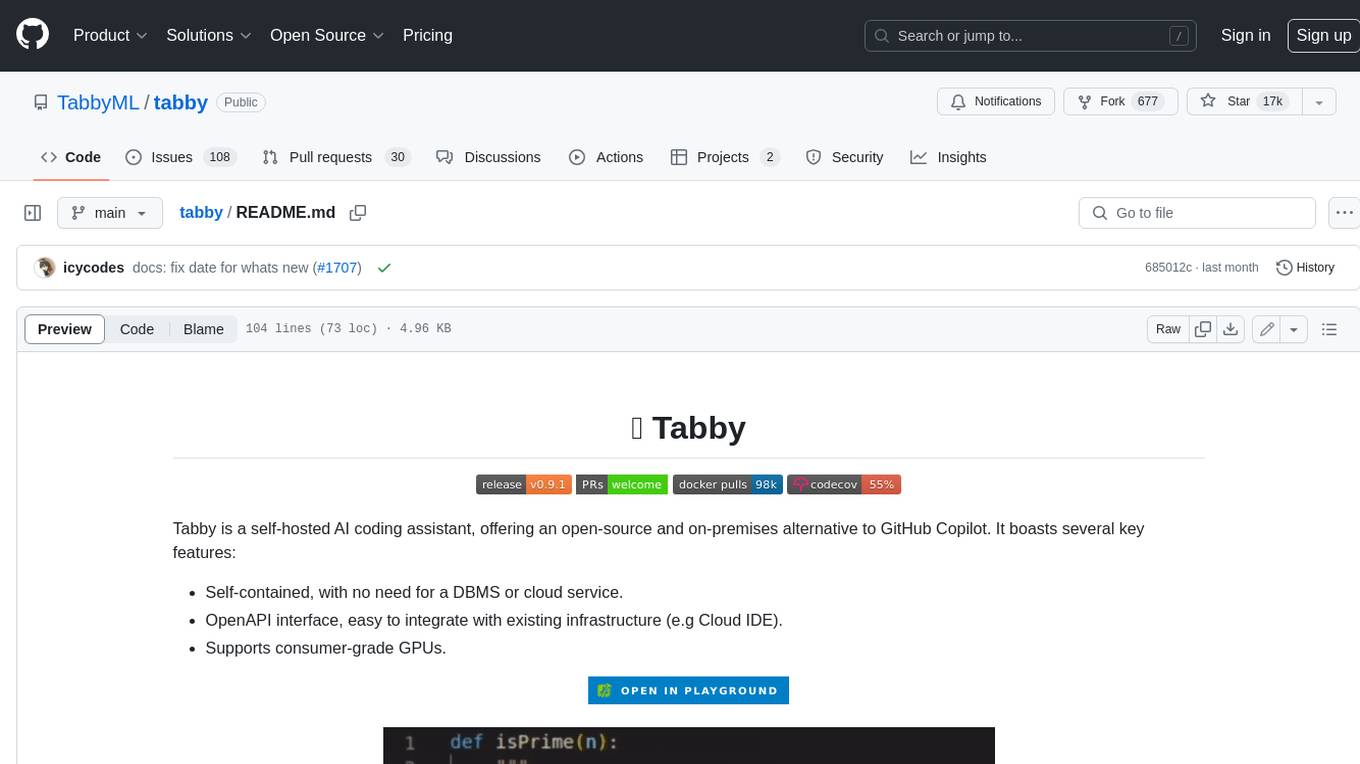
tabby
Tabby is a self-hosted AI coding assistant, offering an open-source and on-premises alternative to GitHub Copilot. It boasts several key features: * Self-contained, with no need for a DBMS or cloud service. * OpenAPI interface, easy to integrate with existing infrastructure (e.g Cloud IDE). * Supports consumer-grade GPUs.
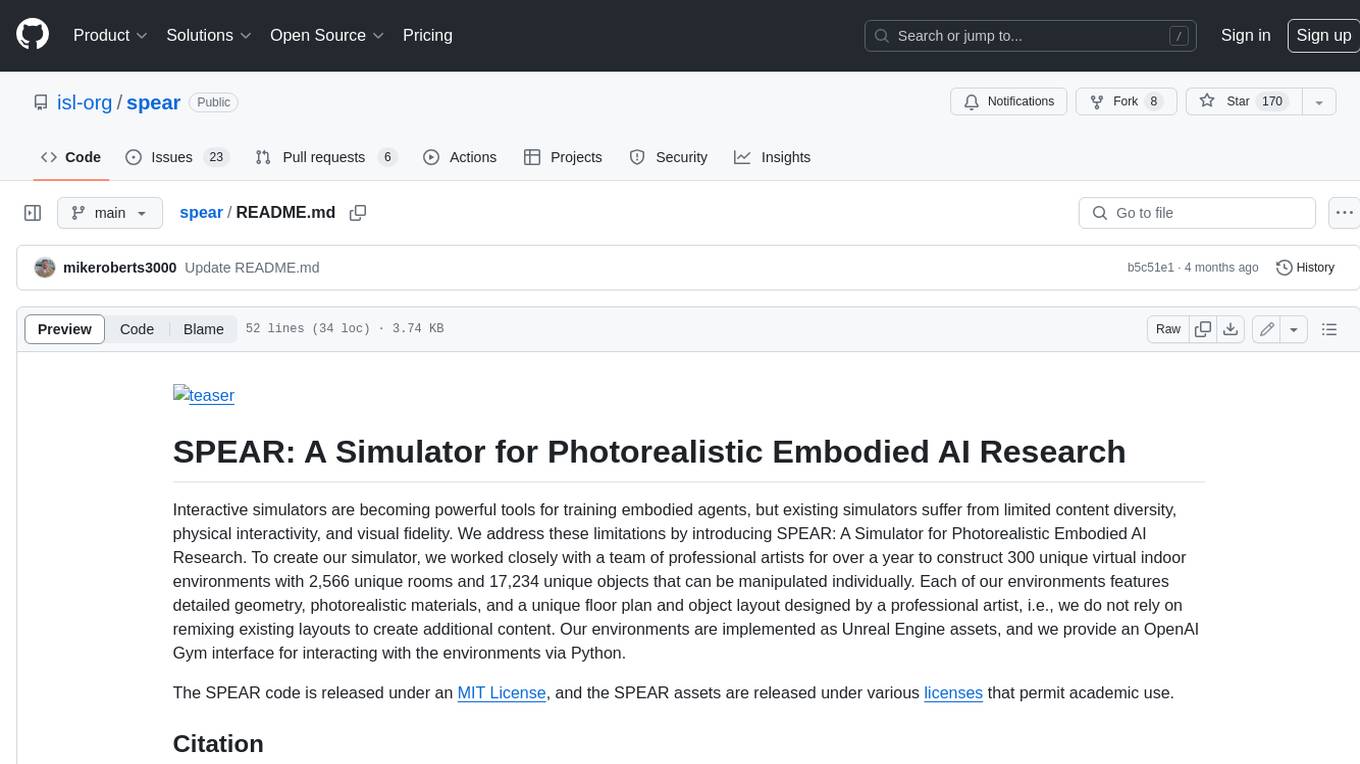
spear
SPEAR (Simulator for Photorealistic Embodied AI Research) is a powerful tool for training embodied agents. It features 300 unique virtual indoor environments with 2,566 unique rooms and 17,234 unique objects that can be manipulated individually. Each environment is designed by a professional artist and features detailed geometry, photorealistic materials, and a unique floor plan and object layout. SPEAR is implemented as Unreal Engine assets and provides an OpenAI Gym interface for interacting with the environments via Python.
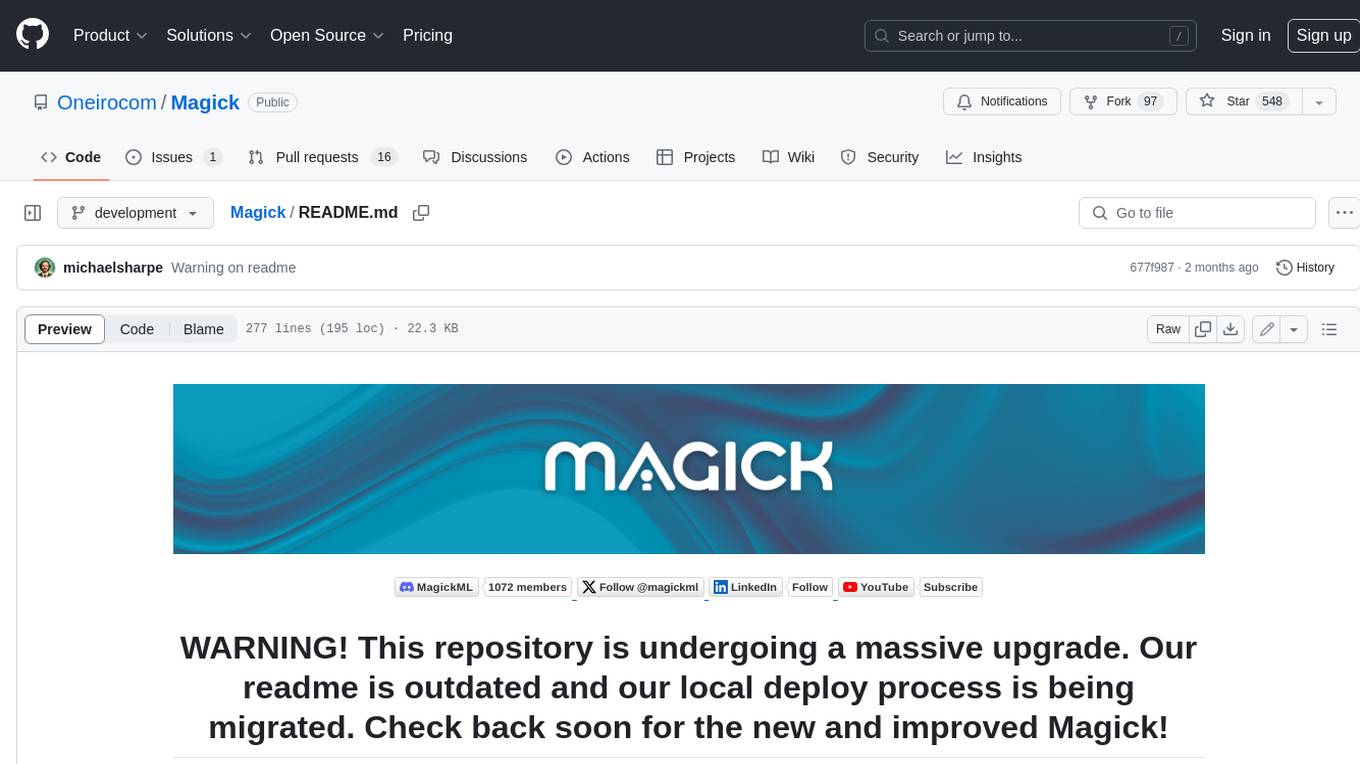
Magick
Magick is a groundbreaking visual AIDE (Artificial Intelligence Development Environment) for no-code data pipelines and multimodal agents. Magick can connect to other services and comes with nodes and templates well-suited for intelligent agents, chatbots, complex reasoning systems and realistic characters.