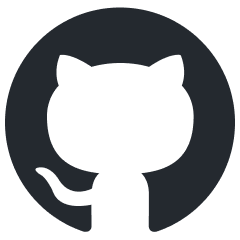
backend.ai-webui
Backend.AI Web UI for web / desktop app (Windows/Linux/macOS). Backend.AI Web UI provides a convenient environment for users, while allowing various commands to be executed without CLI. It also provides some visual features that are not provided by the CLI, such as dashboards and statistics.
Stars: 107
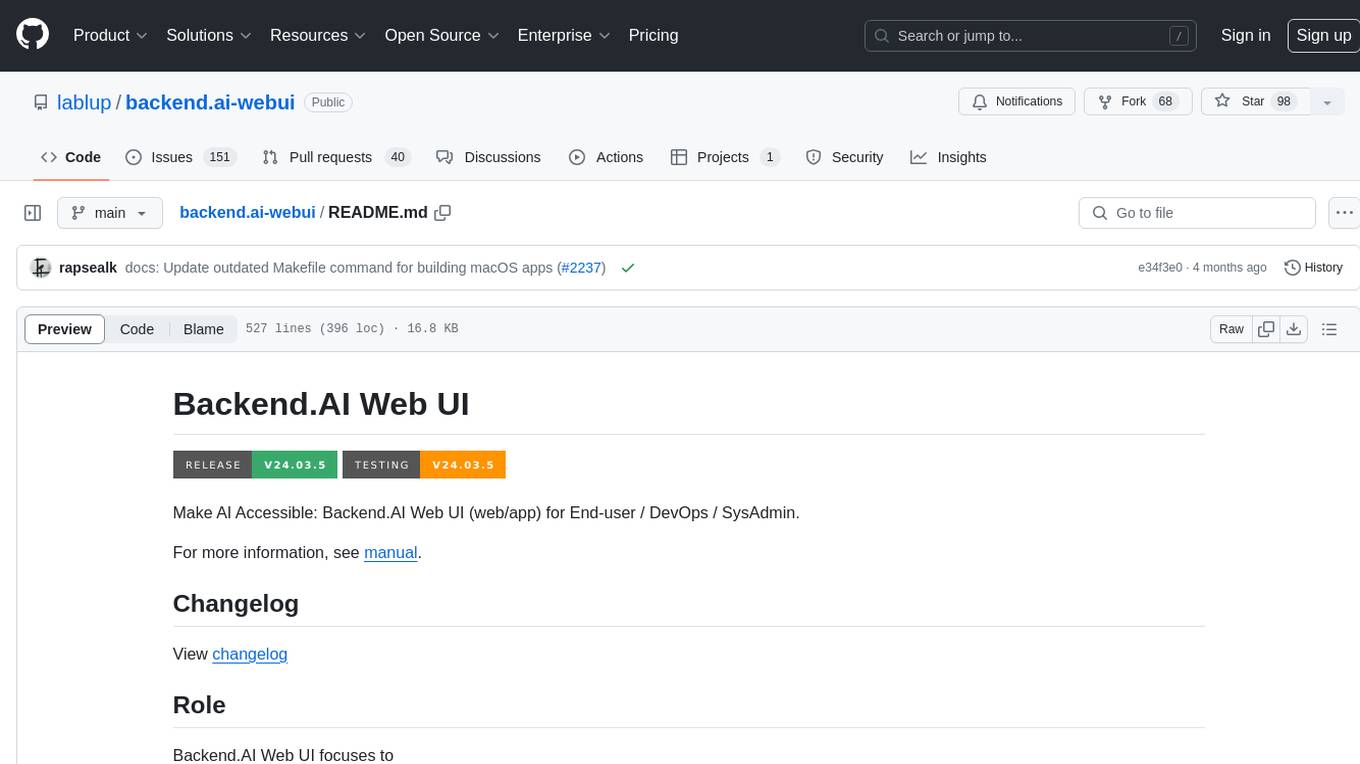
Backend.AI Web UI is a user-friendly web and app interface designed to make AI accessible for end-users, DevOps, and SysAdmins. It provides features for session management, inference service management, pipeline management, storage management, node management, statistics, configurations, license checking, plugins, help & manuals, kernel management, user management, keypair management, manager settings, proxy mode support, service information, and integration with the Backend.AI Web Server. The tool supports various devices, offers a built-in websocket proxy feature, and allows for versatile usage across different platforms. Users can easily manage resources, run environment-supported apps, access a web-based terminal, use Visual Studio Code editor, manage experiments, set up autoscaling, manage pipelines, handle storage, monitor nodes, view statistics, configure settings, and more.
README:
Make AI Accessible: Backend.AI Web UI (web/app) for End-user / DevOps / SysAdmin.
For more information, see manual.
View changelog
Backend.AI Web UI focuses to
- Both desktop app (Windows, macOS and Linux) and web service
- Provide both basic administration and user mode
- Use CLI for detailed administration features such as domain administration
- Versatile devices ready such as mobile, tablet and desktop
- Built-in websocket proxy feature for desktop app
- Session management
- Set default resources for runs
- Monitor current resources sessions using
- Choose and run environment-supported apps
- Web-based Terminal for each session
- Fully-featured Visual Studio Code editor and environments
- Inference service management
- Set / reserve endpoint URL for inference
- Autoscaling setup
- Pipeline
- Experiments (with SACRED / Microsoft NNI / Apache MLFlow)
- AutoML (with Microsoft NNI / Apache MLFlow)
- Manages container streams with pipeline vfolders
- Storage proxy for fast data I/O between backend.ai cluster and user
- Checks queue and scheduled jobs
- Storage management
- Create / delete folders
- Upload / download files (with upload progress)
- Integrated SSH/SFTP server (app mode only)
- Share folders with friends / groups
- Node management
- See calculation nodes in Backend.AI cluster
- Live statistics of bare-metal / VM nodes
- Statistics
- User resource statistics
- Session statistics
- Workload statistics
- Per-node statistics
- Insight (working)
- Configurations
- User-specific web / app configurations
- System maintenances
- Beta features
- WebUI logs / errors
- License
- Check current license information (for enterprise only)
- Plugins
- Per-site specific plugin architecture
- Device plugins / storage plugins
- Help & manuals
- Online manual
- Kernel managements
- List supported kernels
- Add kernel
- Refresh kernel list
- Categorize repository
- Add/update resource templates
- Add/remove docker registries
- User management
- User creation / deletion / key management / resource templates
- Keypair management
- Allocate resource limitation for keys
- Add / remove resource policies for keys
- Manager settings
- Add /setting repository
- Plugin support
- Proxy mode to support various app environments (with node.js (web), electron (app) )
- Needs backend.ai-wsproxy package
- Service information
- Component compatibility
- Security check
- License information
- Work with Web server (github/lablup/backend.ai-webserver)
- Delegate login to web server
- Support userid / password login
backend.ai-webui
production version is also served as backend.ai-app
and refered by backend.ai-webserver
as submodule. If you use backend.ai-webserver
, you are using latest stable release of backend.ai-webui
.
Backend.AI Web UI uses config.toml
located in app root directory. You can prepare many config.toml.[POSTFIX]
in configs
directory to switch various configurations.
NOTE: Update only
config.toml.sample
when you update configurations. Any files inconfigs
directory are auto-created viaMakefile
.
These are options in config.toml
.
You can refer the role of each key in config.toml.sample
When enabling debug mode, It will show certain features used for debugging in both web and app respectively.
- Show raw error messages
- Enable creating session with manual image name
If you want to run the app(electron) in debugging mode, you have to first initialize and build the Electron app.
If you have initialized and built the app(electron), please run the app(electron) in debugging mode with this command:
$ make test_electron
You can debug the app.
- main : Development branch
- release : Latest release branch
- feature/[feature-branch] : Feature branch. Uses
git flow
development scheme. - tags/v[versions] : version tags. Each tag represents release versions.
Backend.AI Web UI is built with
-
lit-element
as webcomponent framework -
react
as library for web UI -
pnpm
as package manager -
rollup
as bundler -
electron
as app shell -
watchman
as file change watcher for development
View Code of conduct for community guidelines.
$ pnpm i
If this is not your first-time compilation, please clean the temporary directories with this command:
$ make clean
You must perform first-time compilation for testing. Some additional mandatory packages should be copied to proper location.
$ make compile_wsproxy
To run relay-compiler
with the watch option(pnpm run relay -- --watch
) on a React project, you need to install watchman
. If you use Homebrew on Linux, it's a great way to get a recent Watchman build. Please refer to the official installation guide.
On a terminal:
$ pnpm run build:d # To watch source changes
On another terminal:
$ pnpm run server:d # To run dev. web server
On yet another terminal:
$ pnpm run wsproxy # To run websocket proxy
If you want to change port for your development environment, Add your configuration to /react/.env.development
file in the project:
PORT=YOURPORT
Defaultly, PORT
is 9081
$ pnpm run lint # To check lints
The project uses Playwright
as E2E testing framework and Jest
as JavaScript testing framework.
To perform E2E tests, you must run complete Backend.AI cluster before starting test. On a terminal:
$ pnpm run server:d # To run dev. web server
On another terminal:
$ pnpm run test # Run tests (tests are located in `tests` directory)
To perform JavaScript test, On a terminal;
$ pnpm run test # For ./src
$ cd ./react && pnpm run test # For ./react
On a terminal:
$ pnpm run server:d # To run test server
OR
$ pnpm run server:p # To run compiled source
On another terminal:
$ pnpm run electron:d # Run Electron as dev mode.
For developing with Relay in your React application, it is highly recommended to install the VSCode Relay GraphQL extension. This extension provides various features to enhance your development experience with Relay.
Installation Steps:
- Open VSCode and navigate to the Extensions view.
- Search for
Relay
and find theRelay - GraphQL
extension by Meta. - Click the
Install
button to add the extension to your VSCode.
Configuration:
After installing the extension, add the following configuration to your ./vscode/settings.json
file:
{
"relay.rootDirectory": "react"
}
$ make compile
Then bundled resource will be prepared in build/rollup
. Basically, both app and web serving is based on static serving sources in the directory. However, to work as single page application, URL request fallback is needed.
If you want to create the bundle zip file,
$ make bundle
will generate compiled static web bundle at ./app
directory. Then you can serve the web bundle via webservers.
If you need to serve with nginx, please install and setup backend.ai-wsproxy
package for websocket proxy. Bundled websocket proxy is simplified version for single-user app.
This is nginx server configuration example. [APP PATH] should be changed to your source path.
server {
listen 443 ssl http2;
listen [::]:443 ssl http2;
server_name [SERVER URL];
charset utf-8;
client_max_body_size 15M; # maximum upload size.
root [APP PATH];
index index.html;
location / {
try_files $uri /index.html;
}
keepalive_timeout 120;
ssl_certificate [CERTIFICATE FILE PATH];
ssl_certificate_key [CERTIFICATE KEY FILE PATH];
}
Make sure that you compile the Web UI.
e.g. You will download the backend.ai-webserver
package.
$ make compile
Good for develop phase. Not recommended for production environment.
Note: This command will use Web UI source in build/rollup
directory. No certificate will be used therefore web server will serve as HTTP.
Copy webserver.example.conf
in docker_build
directory into current directory as webserver.conf
and modify configuration files for your needs.
$ docker-compose build webui-dev # build only
$ docker-compose up webui-dev # for testing
$ docker-compose up -d webui-dev # as a daemon
Visit http://127.0.0.1:8080
to test web server.
Recommended for production.
Note: You have to enter the certificates (chain.pem
and priv.pem
) into certificates
directory. Otherwise, you will have an error during container initialization.
Copy webserver.example.ssl.conf
in docker_build
directory into current directory as webserver.conf
and modify configuration files for your needs.
$ docker-compose build webui # build only
$ docker-compose up webui # for testing
$ docker-compose up -d webui # as a daemon
Visit https://127.0.0.1:443
to test web server serving. Change 127.0.0.1
to your production domain.
$ docker-compose down
$ make compile
$ docker build -t backendai-webui .
Testing / Running example
Check your image name is backendai-webui_webui
or backendai-webui_webui-ssl
. Otherwise, change the image name in the script below.
$ docker run --name backendai-webui -v $(pwd)/config.toml:/usr/share/nginx/html/config.toml -p 80:80 backendai-webui_webui /bin/bash -c "envsubst '$$NGINX_HOST' < /etc/nginx/conf.d/default.template > /etc/nginx/conf.d/default.conf && nginx -g 'daemon off;'"
$ docker run --name backendai-webui-ssl -v $(pwd)/config.toml:/usr/share/nginx/html/config.toml -v $(pwd)/certificates:/etc/certificates -p 443:443 backendai-webui_webui-ssl /bin/bash -c "envsubst '$$NGINX_HOST' < /etc/nginx/conf.d/default-ssl.template > /etc/nginx/conf.d/default.conf && nginx -g 'daemon off;'"
If you need to serve as webserver (ID/password support) without compiling anything, you can use pre-built code through webserver submodule.
To download and deploy web UI from pre-built source, do the following in backend.ai
repository:
$ git submodule update --init --checkout --recursive
This is only needed with pure ES6 dev. environment / browser. Websocket proxy is embedded in Electron and automatically starts.
$ pnpm run wsproxy
If webui app is behind an external http proxy, and you have to pass through
it to connect to a webserver or manager server, you can set
EXT_HTTP_PROXY
environment variable with the address of the http proxy.
Local websocket proxy then communicates with the final destination via the http
proxy. The address should include the protocol, host, and/or port (if exists).
For example,
$ export EXT_HTTP_PROXY=http://10.20.30.40:3128 (Linux)
$ set EXT_HTTP_PROXY=http://10.20.30.40:3128 (Windows)
Even if you are using Electron embedded websocket proxy, you have to set the environment variable manually to pass through a http proxy.
You can prepare site-specific configuration as toml
format. Also, you can build site-specific web bundle refering in configs
directory.
Note: Default setup will build es6-bundled
version. If you want to use es6-unbundled
, make sure that your webserver supports HTTP/2 and setup as HTTPS with proper certification.
$ make web site=[SITE CONFIG FILE POSTFIX]
If no prefix is given, default configuration file will be used.
Example:
$ make web site=beta
You can manually modify config.toml for your need.
Electron building is automated using Makefile
.
$ make clean # clean prebuilt codes
$ make mac # build macOS app (both Intel/Apple)
$ make mac_x64 # build macOS app (Intel x64)
$ make mac_arm64 # build macOS app (Apple Silicon)
$ make win # build win64 app
$ make linux # build linux app
$ make all # build win64/macos/linux app
$ make win
Note: Building Windows x86-64 on other than Windows requires Wine > 3.0
Note: On macOS Catalina, use scripts/build-windows-app.sh to build Windows 32bitpackage. From macOS 10.15+, wine 32x is not supported.
Note: Now the make win
command support only Windows x64 app, therefore you do not need to use build-windows-app.sh
anymore.
$ make mac
NOTE: Sometimes Apple silicon version compiled on Intel machine does not work.
$ make mac_x64
$ make mac_arm64
- Export keychain from Keychain Access. Exported p12 should contain:
- Certificate for Developer ID Application
- Corresponding Private Key
- Apple Developer ID CA Certificate. Version of signing certificate (G1 or G2) matters, so be careful to check appropriate version! To export multiple items at once, just select all items (Cmd-Click), right click one of the selected item and then click "Export n item(s)...".
- Set following environment variables when running
make mac_*
.
BAI_APP_SIGN=1
BAI_APP_SIGN_APPLE_ID="<Apple ID which has access to created signing certificate>"
BAI_APP_SIGN_APPLE_ID_PASSWORD="<App-specific password of target Apple ID>"
BAI_APP_SIGN_IDENTITY="<Signing Identity>"
BAI_APP_SIGN_KEYCHAIN_B64="<Base64 encoded version of exported p12 file>"
-
BAI_APP_SIGN_KEYCHAIN_PASSWORD="<Import password of exported p12 file>"
Signing Identity is equivalent to the name of signing certificate added on Keychain Access.
$ make linux
Note: Packaging usually performs right after app building. Therefore you do not need this option in normal condition.
Note: Packaging macOS disk image requires electron-installer-dmg to make macOS disk image. It requires Python 2+ to build binary for package.
Note: There are two Electron configuration files, main.js
and main.electron-packager.js
. Local Electron run uses main.js
, not main.electron-packager.js
that is used for real Electron app.
$ make dep # Compile with app dependencies
$ pnpm run electron:d # OR, ./node_modules/electron/cli.js .
The electron app reads the configuration from ./build/electron-app/app/config.toml
, which is copied from the root config.toml
file during make clean && make dep
.
If you configure [server].webServerURL
, the electron app will load the web contents (including config.toml
) from the designated server.
The server may be either a pnpm run server:d
instance or a ./py -m ai.backend.web.server
daemon from the mono-repo.
This is known as the "web shell" mode and allows live edits of the web UI while running it inside the electron app.
Locale resources are JSON files located in resources/i18n
.
Currently WebUI supports these languages:
- English
- Korean
- French
- Russian
- Mongolian
- Indonesian
Run
$ make i18n
to update / extract i18n resources.
- Use
_t
as i18n resource handler on lit-element templates. - Use
_tr
as i18n resource handler if i18n resource has HTML code inside. - Use
_text
as i18n resource handler on lit-element Javascript code.
In lit-html template:
<div>${_t('general.helloworld')}</div>
In i18n resource (en.json):
{
"general":{
"helloworld": "Hello World"
}
}
- Copy
en.json
to target language. (e.g.ko.json
) - Add language identifier to
supportLanguageCodes
inbackend-ai-webui.ts
. e.g.
@property({type: Array}) supportLanguageCodes = ["en", "ko"];
- Add language information to
supportLanguages
inbackend-ai-usersettings-general-list.ts
.
Note: DO NOT DELETE 'default' language. It is used for browser language.
@property({type: Array}) supportLanguages = [
{name: _text("language.Browser"), code: "default"},
{name: _text("language.English"), code: "en"},
{name: _text("language.Korean"), code: "ko"}
];
For Tasks:
Click tags to check more tools for each tasksFor Jobs:
Alternative AI tools for backend.ai-webui
Similar Open Source Tools
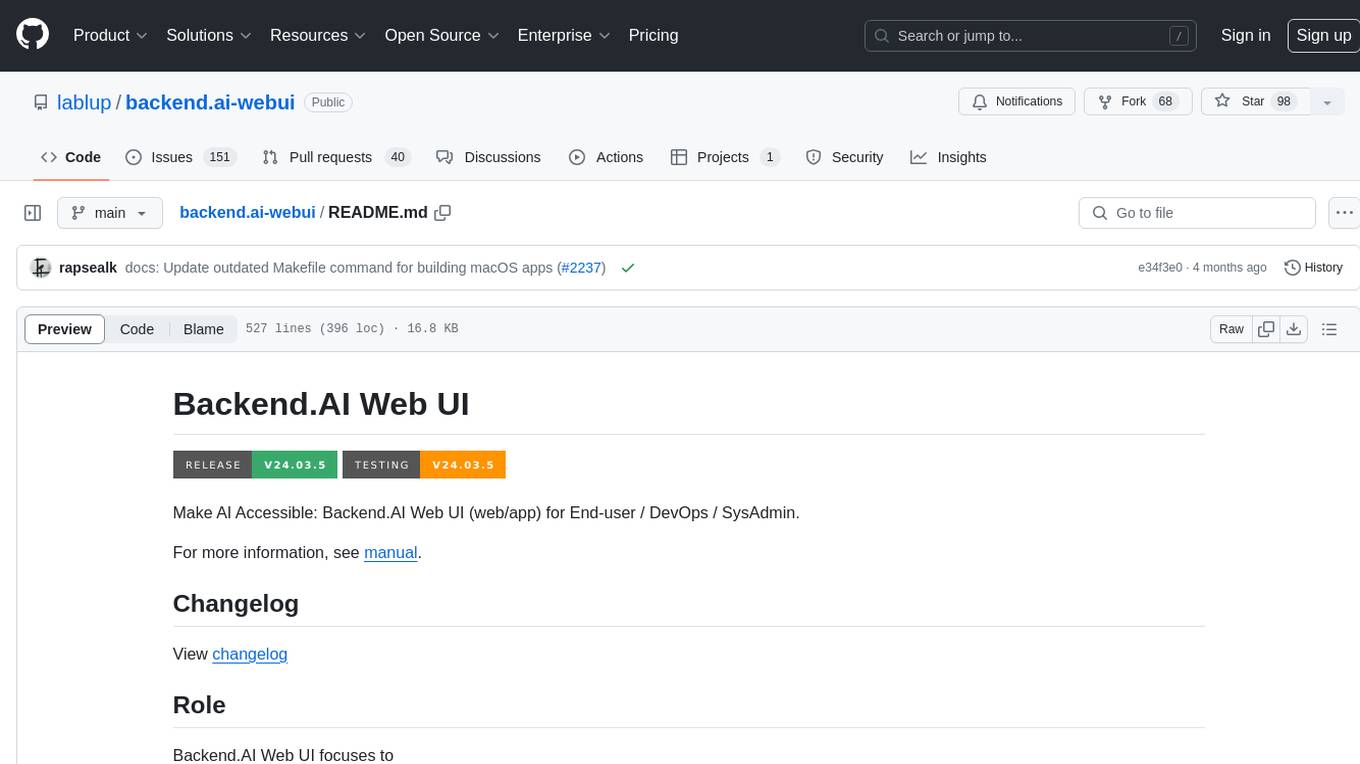
backend.ai-webui
Backend.AI Web UI is a user-friendly web and app interface designed to make AI accessible for end-users, DevOps, and SysAdmins. It provides features for session management, inference service management, pipeline management, storage management, node management, statistics, configurations, license checking, plugins, help & manuals, kernel management, user management, keypair management, manager settings, proxy mode support, service information, and integration with the Backend.AI Web Server. The tool supports various devices, offers a built-in websocket proxy feature, and allows for versatile usage across different platforms. Users can easily manage resources, run environment-supported apps, access a web-based terminal, use Visual Studio Code editor, manage experiments, set up autoscaling, manage pipelines, handle storage, monitor nodes, view statistics, configure settings, and more.
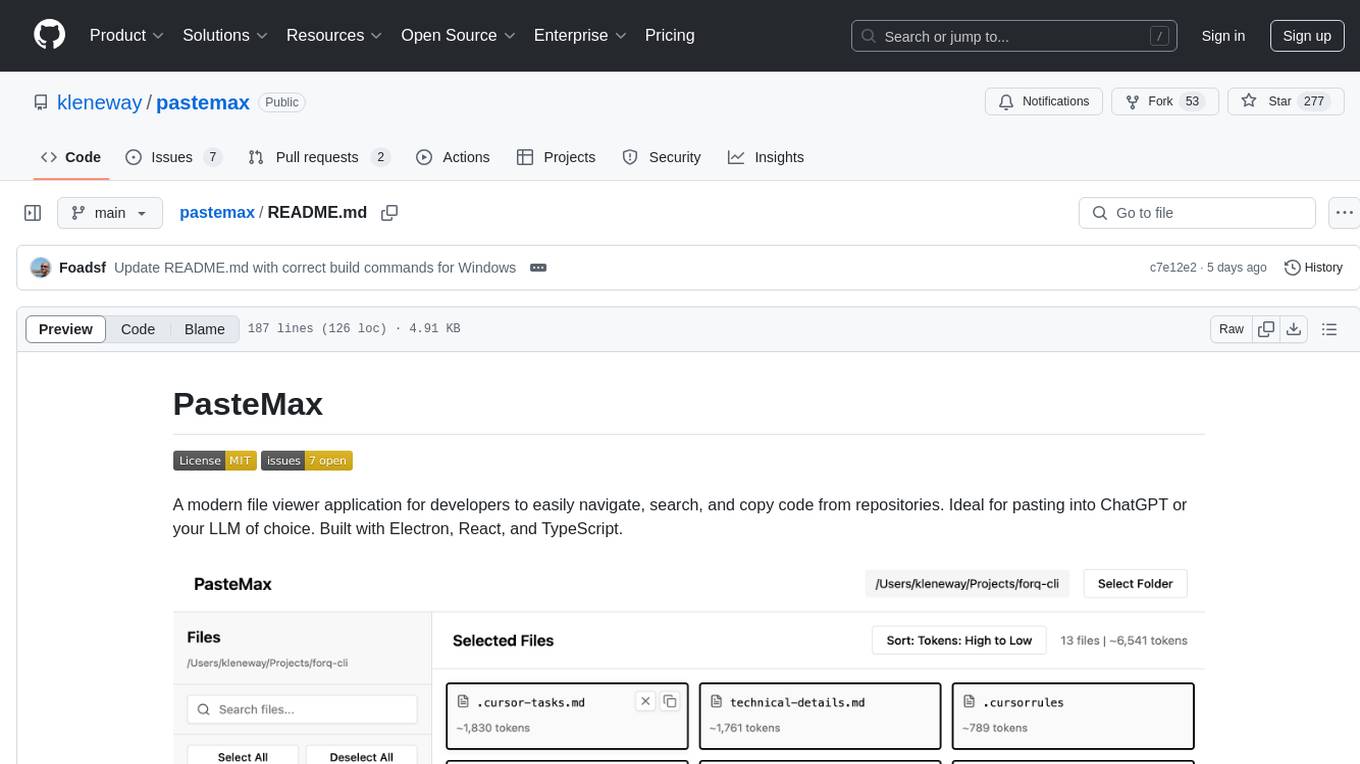
pastemax
PasteMax is a modern file viewer application designed for developers to easily navigate, search, and copy code from repositories. It provides features such as file tree navigation, token counting, search capabilities, selection management, sorting options, dark mode, binary file detection, and smart file exclusion. Built with Electron, React, and TypeScript, PasteMax is ideal for pasting code into ChatGPT or other language models. Users can download the application or build it from source, and customize file exclusions. Troubleshooting steps are provided for common issues, and contributions to the project are welcome under the MIT License.
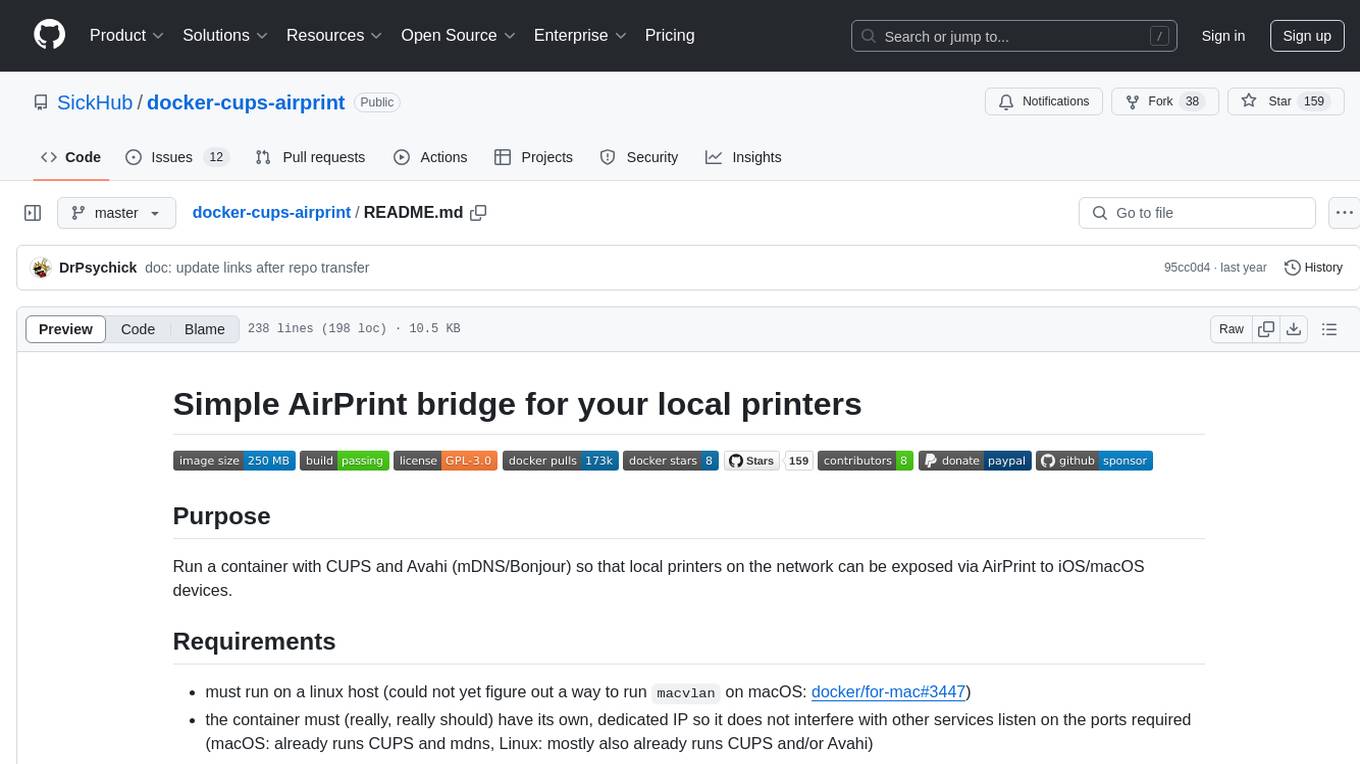
docker-cups-airprint
This repository provides a Docker image that acts as an AirPrint bridge for local printers, allowing them to be exposed to iOS/macOS devices. It runs a container with CUPS and Avahi to facilitate this functionality. Users must have CUPS drivers available for their printers. The tool requires a Linux host and a dedicated IP for the container to avoid interference with other services. It supports setting up printers through environment variables and offers options for automated configuration via command line, web interface, or files. The repository includes detailed instructions on setting up and testing the AirPrint bridge.
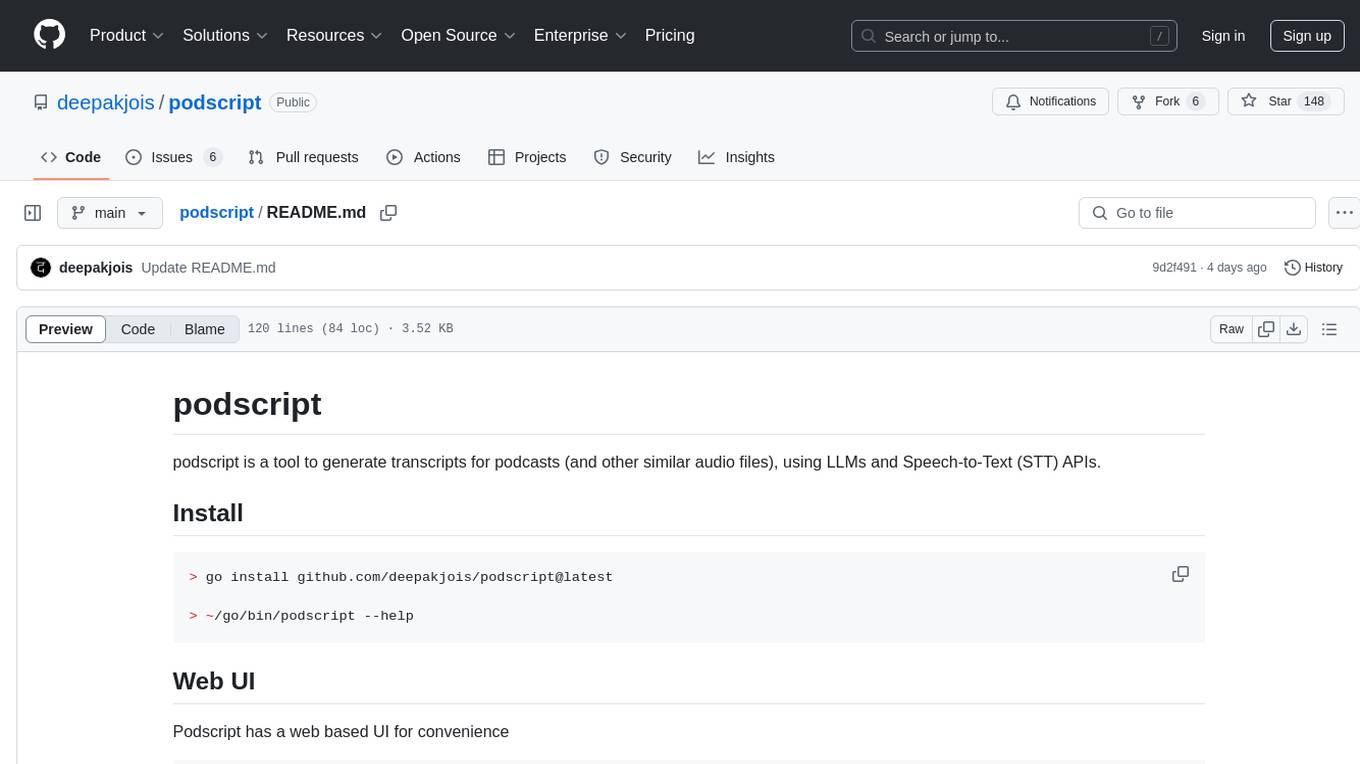
podscript
Podscript is a tool designed to generate transcripts for podcasts and similar audio files using Language Model Models (LLMs) and Speech-to-Text (STT) APIs. It provides a command-line interface (CLI) for transcribing audio from various sources, including YouTube videos and audio files, using different speech-to-text services like Deepgram, Assembly AI, and Groq. Additionally, Podscript offers a web-based user interface for convenience. Users can configure keys for supported services, transcribe audio, and customize the transcription models. The tool aims to simplify the process of creating accurate transcripts for audio content.
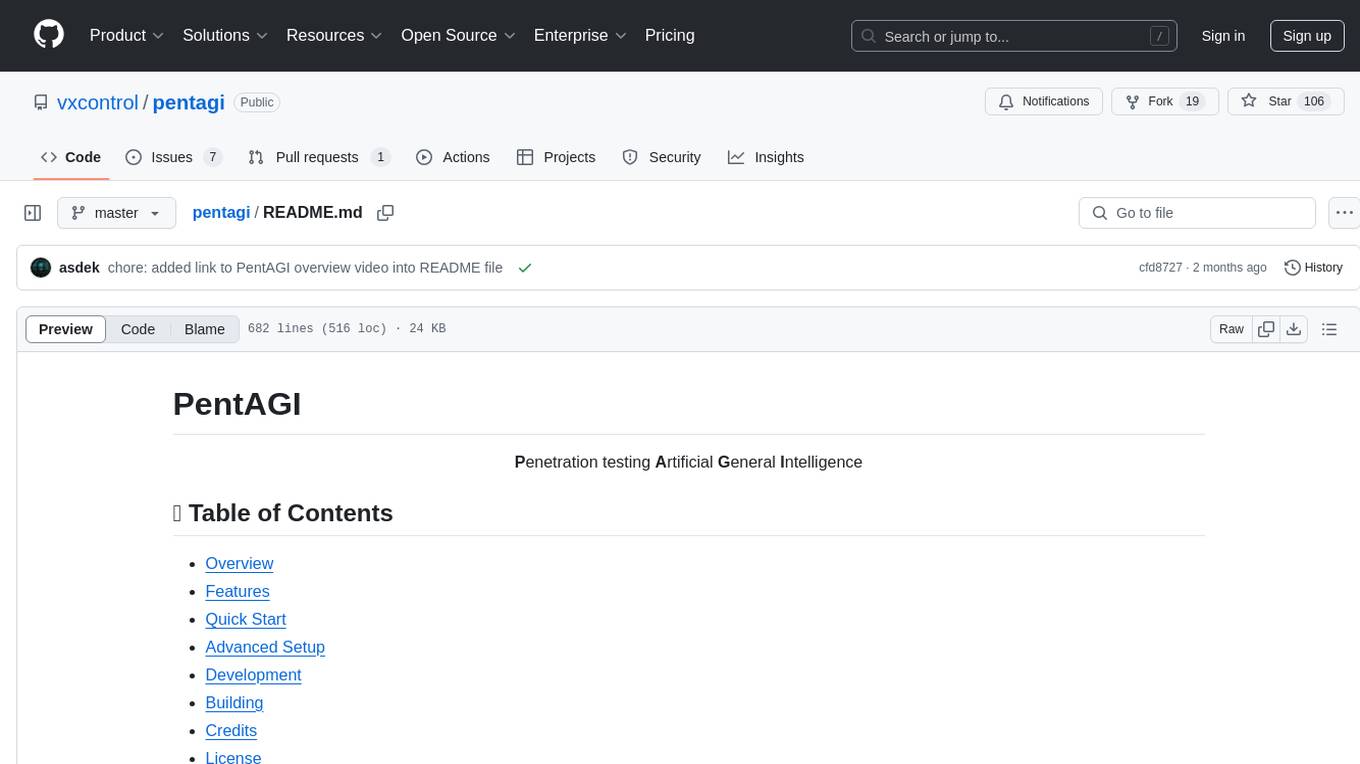
pentagi
PentAGI is an innovative tool for automated security testing that leverages cutting-edge artificial intelligence technologies. It is designed for information security professionals, researchers, and enthusiasts who need a powerful and flexible solution for conducting penetration tests. The tool provides secure and isolated operations in a sandboxed Docker environment, fully autonomous AI-powered agent for penetration testing steps, a suite of 20+ professional security tools, smart memory system for storing research results, web intelligence for gathering information, integration with external search systems, team delegation system, comprehensive monitoring and reporting, modern interface, API integration, persistent storage, scalable architecture, self-hosted solution, flexible authentication, and quick deployment through Docker Compose.
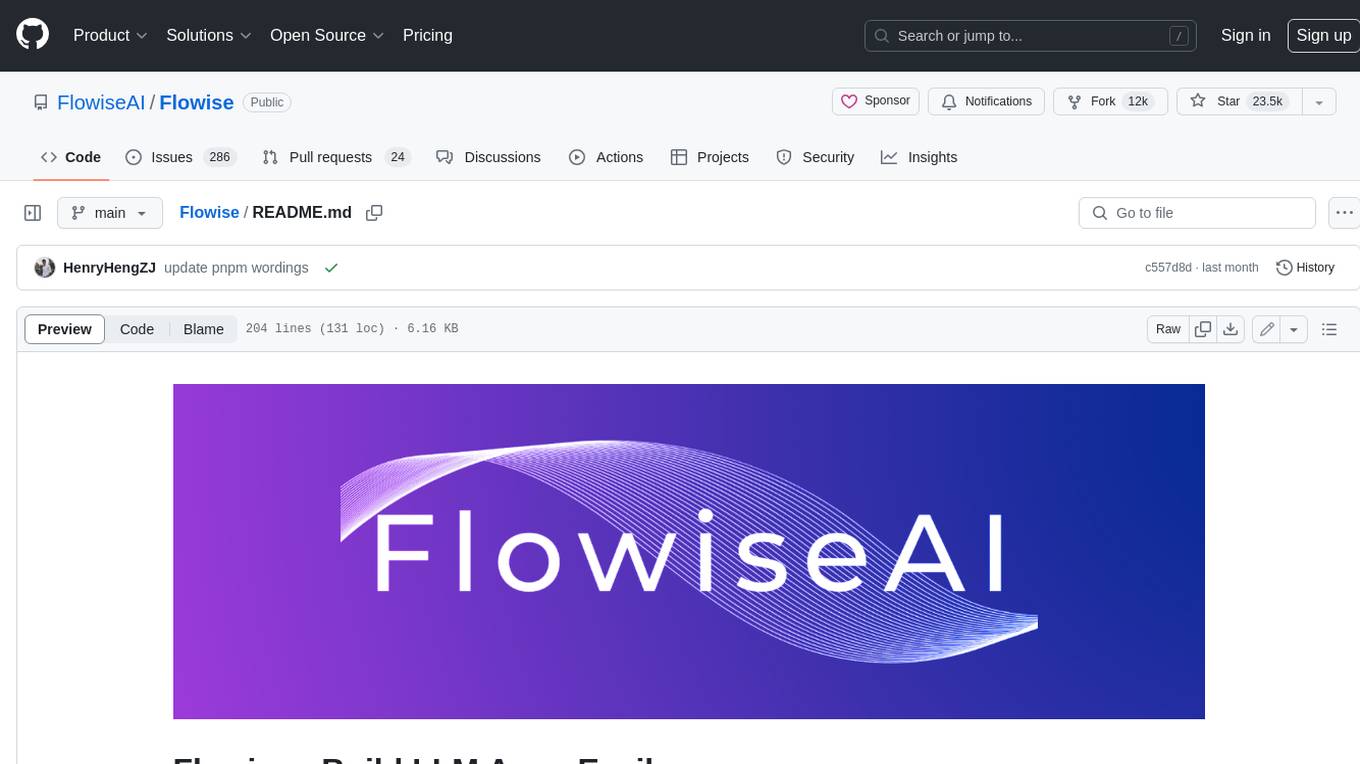
Flowise
Flowise is a tool that allows users to build customized LLM flows with a drag-and-drop UI. It is open-source and self-hostable, and it supports various deployments, including AWS, Azure, Digital Ocean, GCP, Railway, Render, HuggingFace Spaces, Elestio, Sealos, and RepoCloud. Flowise has three different modules in a single mono repository: server, ui, and components. The server module is a Node backend that serves API logics, the ui module is a React frontend, and the components module contains third-party node integrations. Flowise supports different environment variables to configure your instance, and you can specify these variables in the .env file inside the packages/server folder.
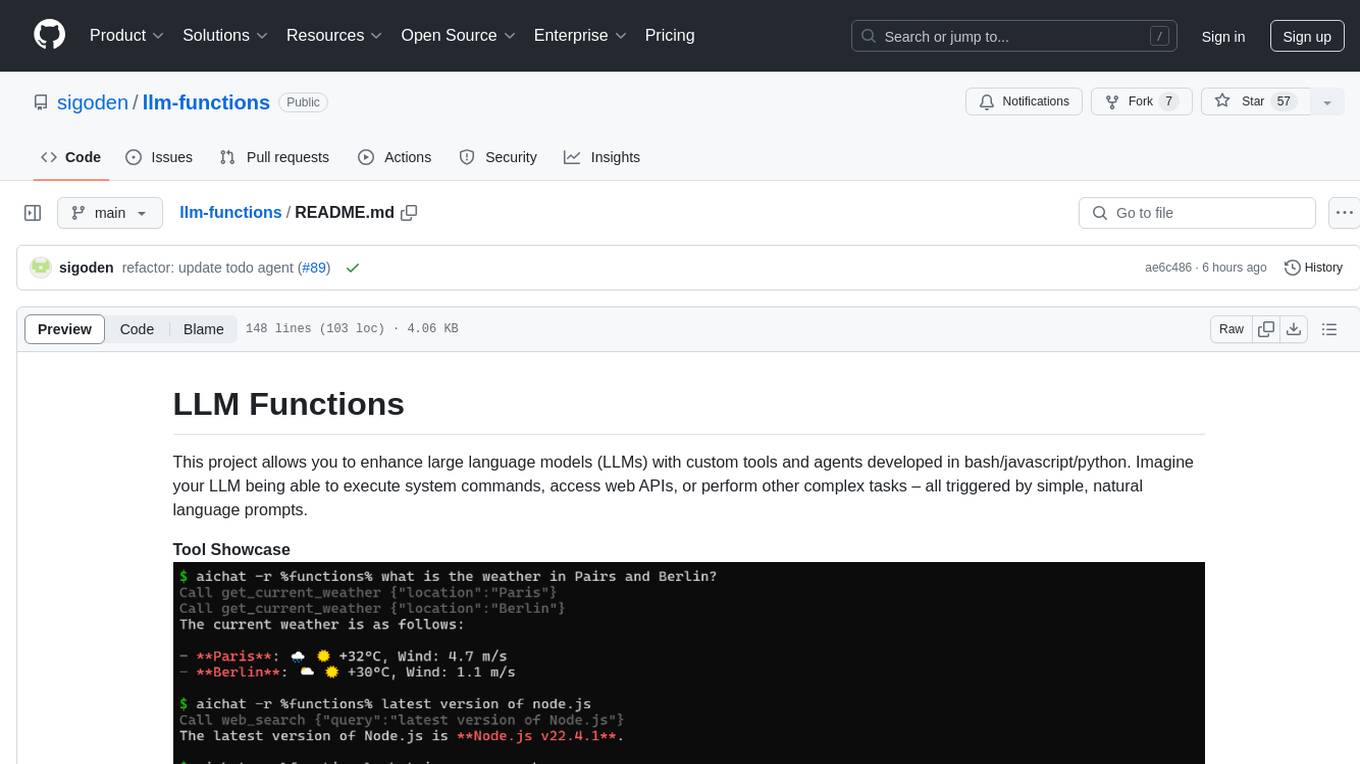
llm-functions
LLM Functions is a project that enables the enhancement of large language models (LLMs) with custom tools and agents developed in bash, javascript, and python. Users can create tools for their LLM to execute system commands, access web APIs, or perform other complex tasks triggered by natural language prompts. The project provides a framework for building tools and agents, with tools being functions written in the user's preferred language and automatically generating JSON declarations based on comments. Agents combine prompts, function callings, and knowledge (RAG) to create conversational AI agents. The project is designed to be user-friendly and allows users to easily extend the capabilities of their language models.
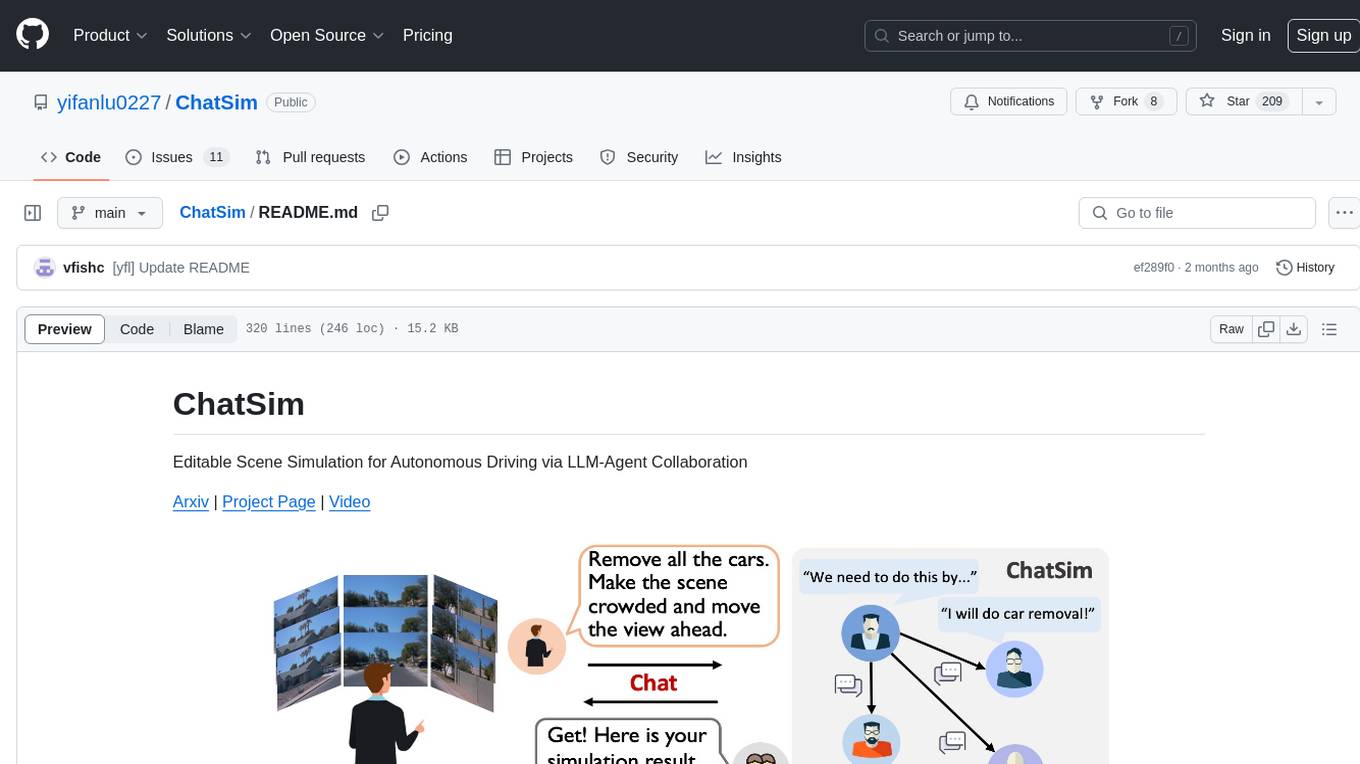
ChatSim
ChatSim is a tool designed for editable scene simulation for autonomous driving via LLM-Agent collaboration. It provides functionalities for setting up the environment, installing necessary dependencies like McNeRF and Inpainting tools, and preparing data for simulation. Users can train models, simulate scenes, and track trajectories for smoother and more realistic results. The tool integrates with Blender software and offers options for training McNeRF models and McLight's skydome estimation network. It also includes a trajectory tracking module for improved trajectory tracking. ChatSim aims to facilitate the simulation of autonomous driving scenarios with collaborative LLM-Agents.
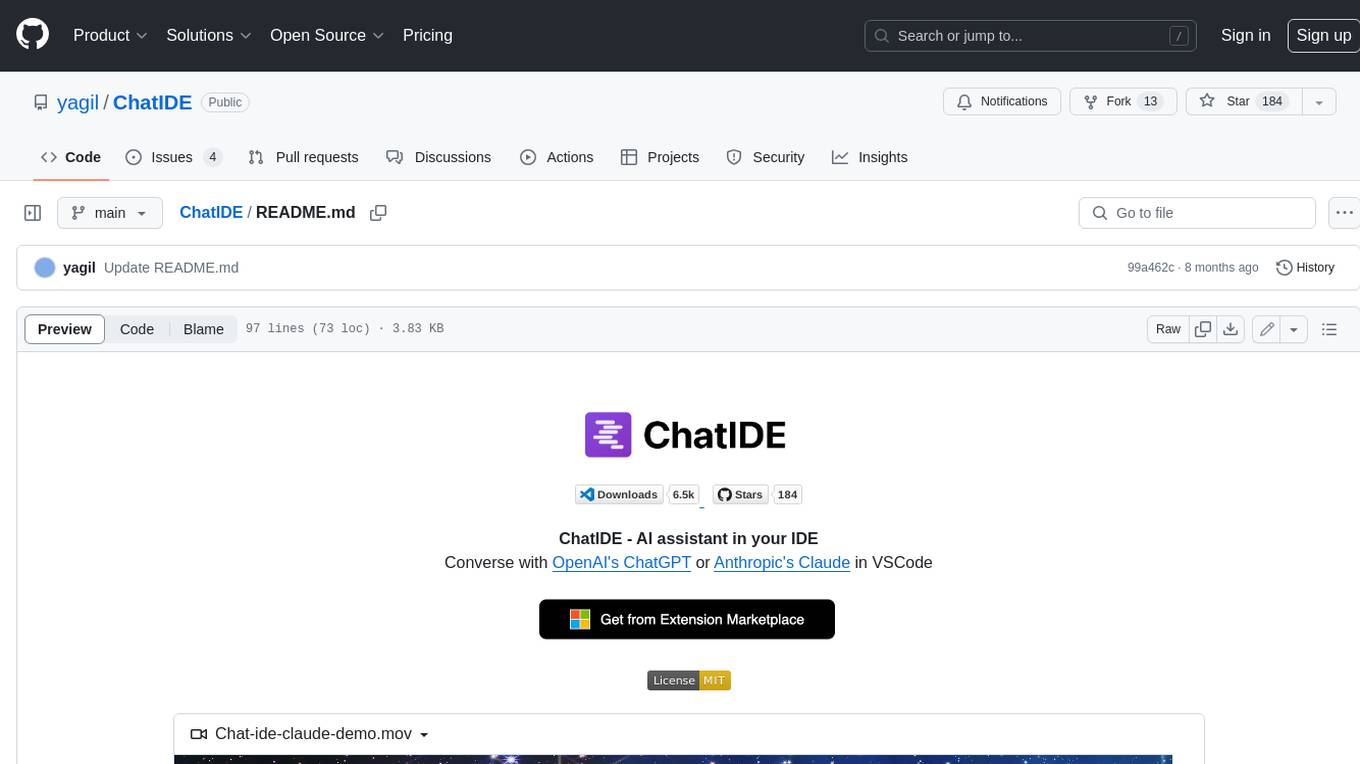
ChatIDE
ChatIDE is an AI assistant that integrates with your IDE, allowing you to converse with OpenAI's ChatGPT or Anthropic's Claude within your development environment. It provides a seamless way to access AI-powered assistance while coding, enabling you to get real-time help, generate code snippets, debug errors, and brainstorm ideas without leaving your IDE.
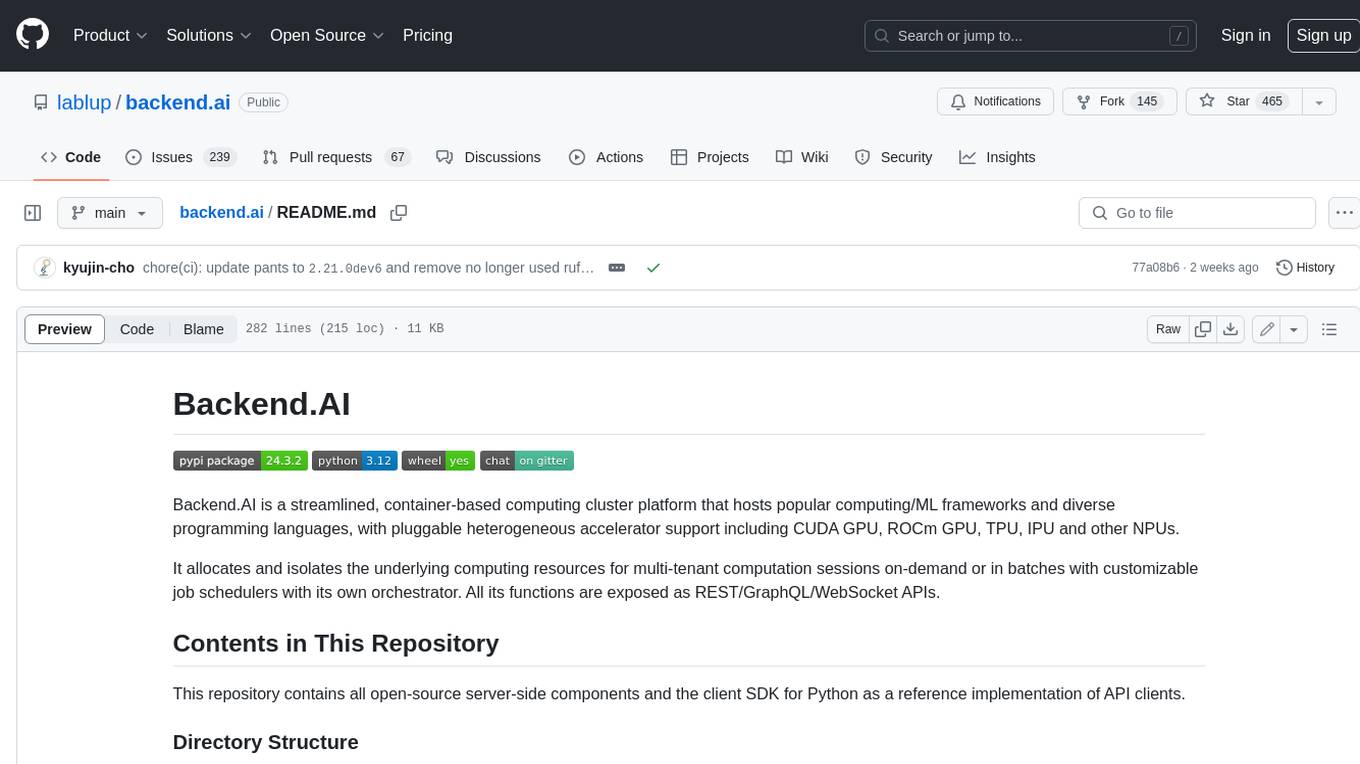
backend.ai
Backend.AI is a streamlined, container-based computing cluster platform that hosts popular computing/ML frameworks and diverse programming languages, with pluggable heterogeneous accelerator support including CUDA GPU, ROCm GPU, TPU, IPU and other NPUs. It allocates and isolates the underlying computing resources for multi-tenant computation sessions on-demand or in batches with customizable job schedulers with its own orchestrator. All its functions are exposed as REST/GraphQL/WebSocket APIs.
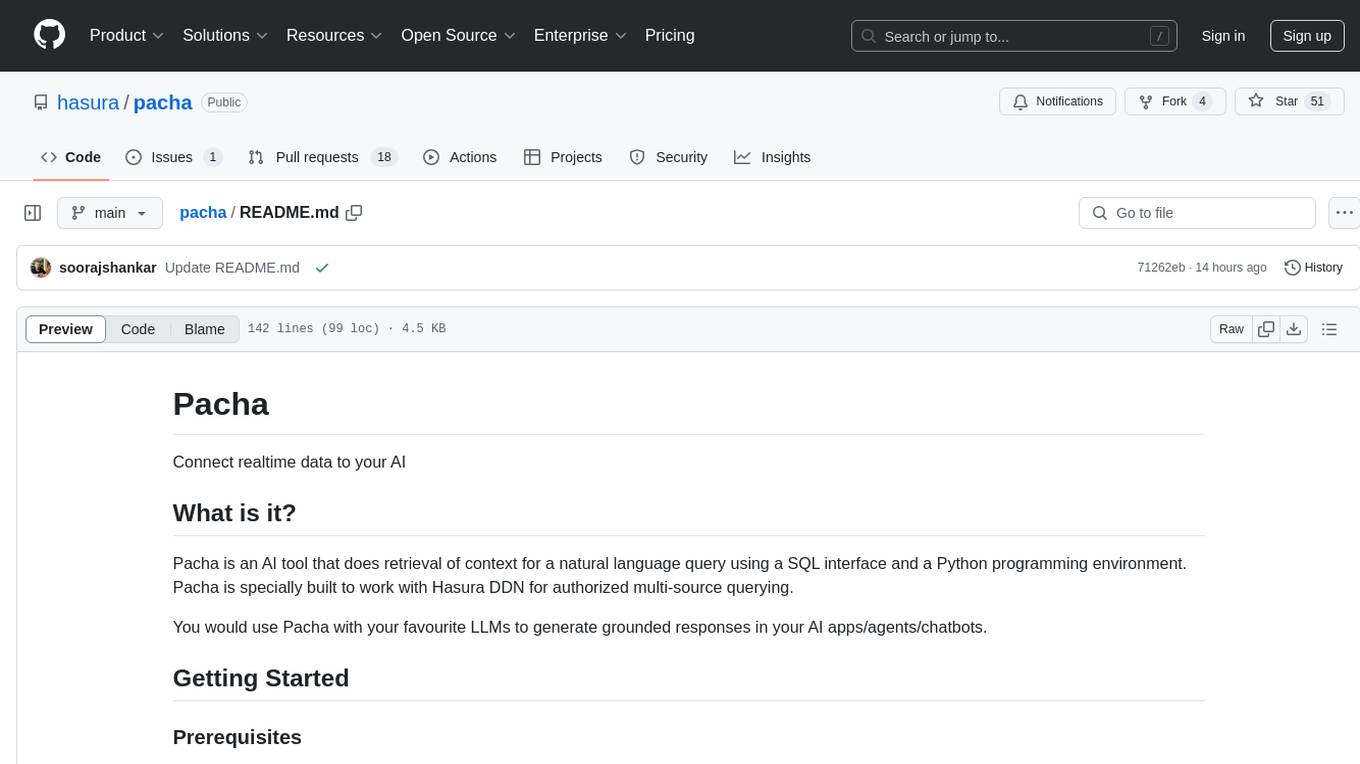
pacha
Pacha is an AI tool designed for retrieving context for natural language queries using a SQL interface and Python programming environment. It is optimized for working with Hasura DDN for multi-source querying. Pacha is used in conjunction with language models to produce informed responses in AI applications, agents, and chatbots.
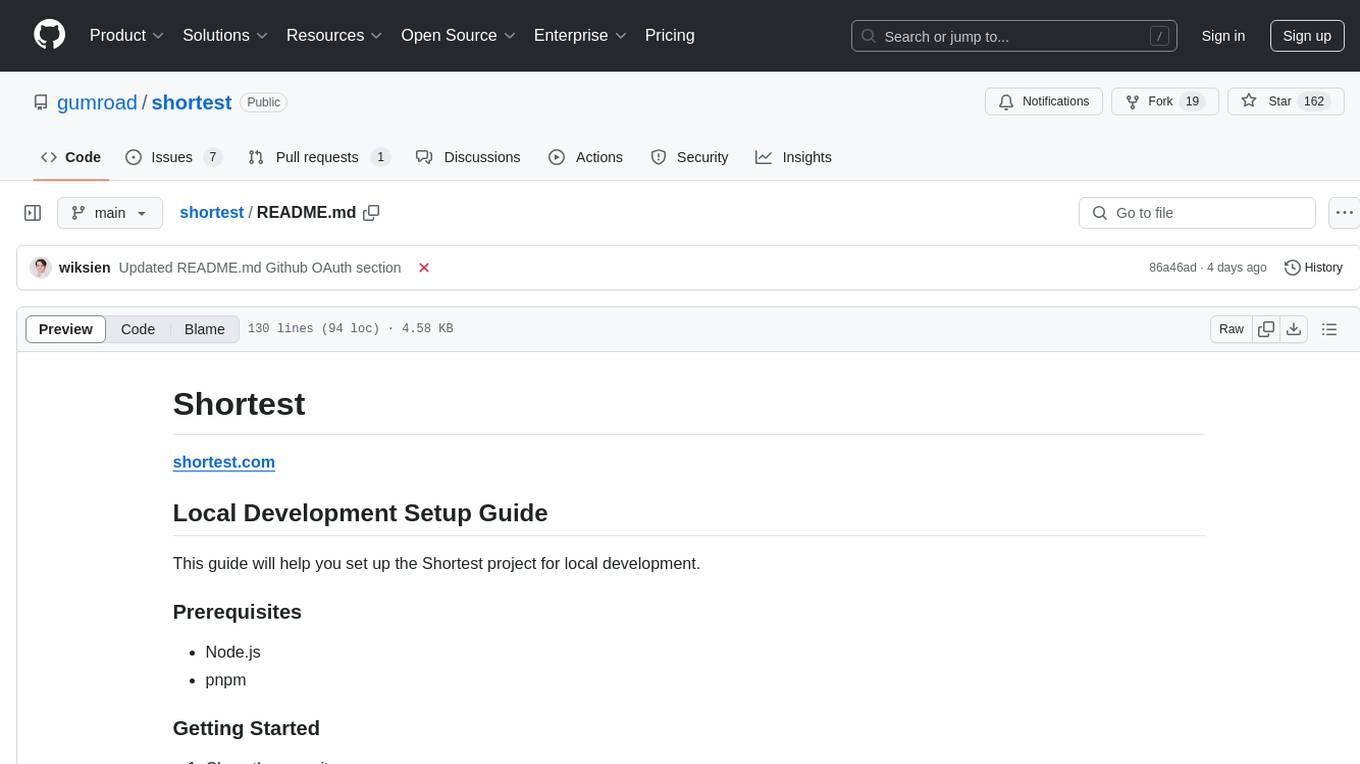
shortest
Shortest is a project for local development that helps set up environment variables and services for a web application. It provides a guide for setting up Node.js and pnpm dependencies, configuring services like Clerk, Vercel Postgres, Anthropic, Stripe, and GitHub OAuth, and running the application and tests locally.
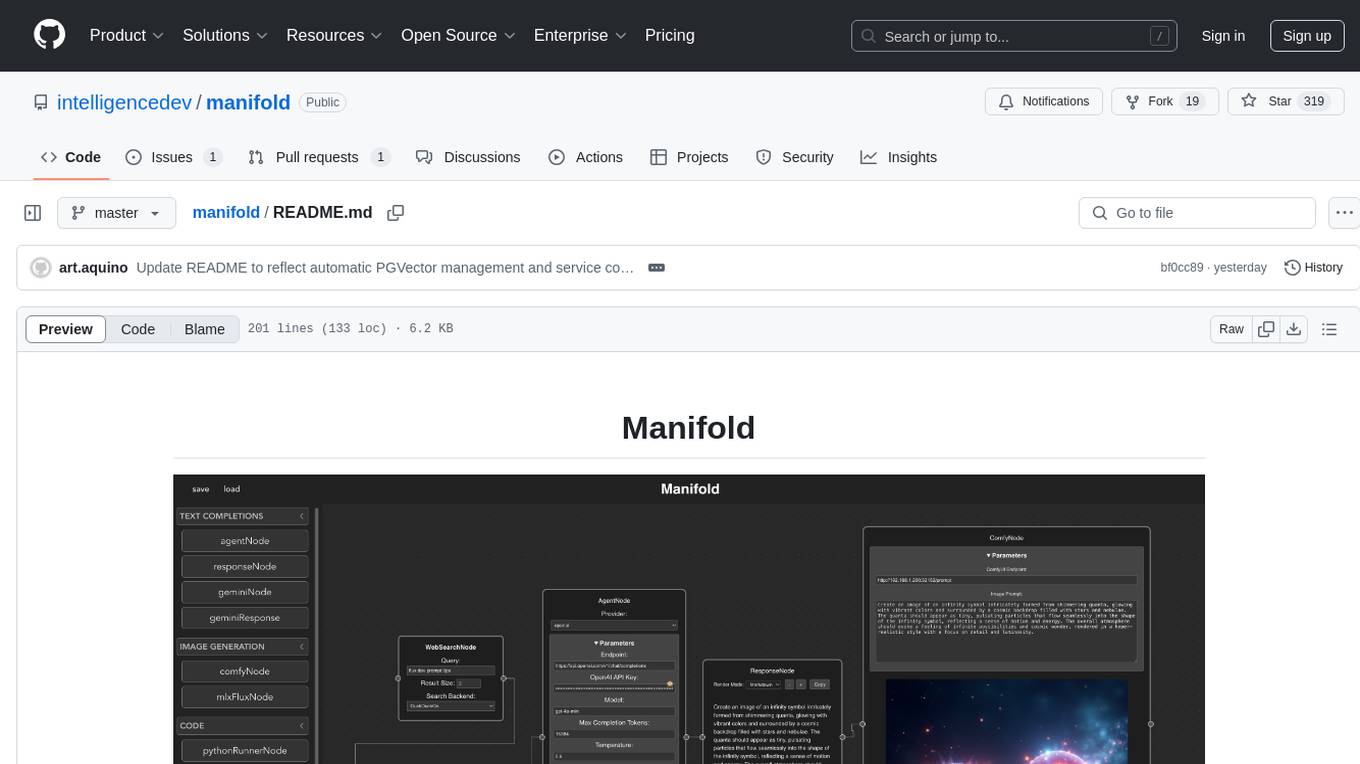
manifold
Manifold is a powerful platform for workflow automation using AI models. It supports text generation, image generation, and retrieval-augmented generation, integrating seamlessly with popular AI endpoints. Additionally, Manifold provides robust semantic search capabilities using PGVector combined with the SEFII engine. It is under active development and not production-ready.
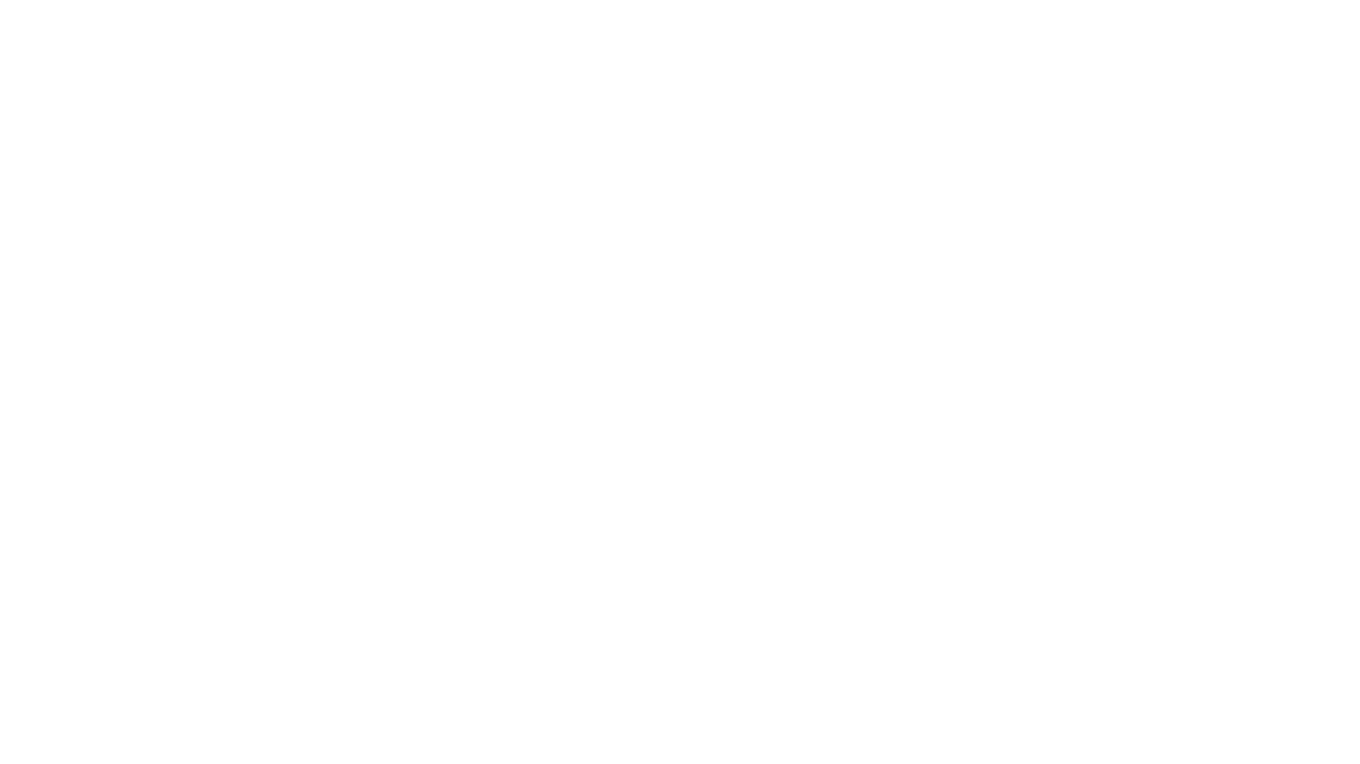
rclip
rclip is a command-line photo search tool powered by the OpenAI's CLIP neural network. It allows users to search for images using text queries, similar image search, and combining multiple queries. The tool extracts features from photos to enable searching and indexing, with options for previewing results in supported terminals or custom viewers. Users can install rclip on Linux, macOS, and Windows using different installation methods. The repository follows the Conventional Commits standard and welcomes contributions from the community.
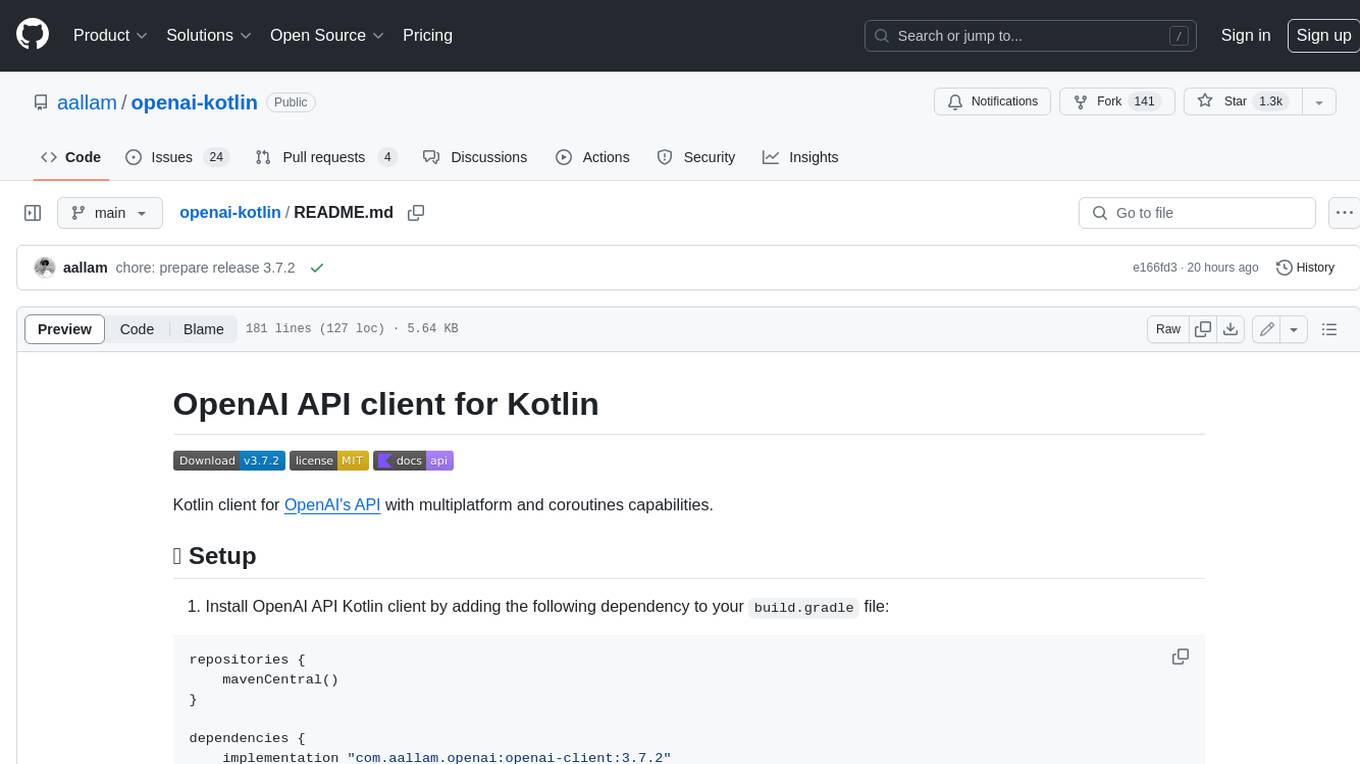
openai-kotlin
OpenAI Kotlin API client is a Kotlin client for OpenAI's API with multiplatform and coroutines capabilities. It allows users to interact with OpenAI's API using Kotlin programming language. The client supports various features such as models, chat, images, embeddings, files, fine-tuning, moderations, audio, assistants, threads, messages, and runs. It also provides guides on getting started, chat & function call, file source guide, and assistants. Sample apps are available for reference, and troubleshooting guides are provided for common issues. The project is open-source and licensed under the MIT license, allowing contributions from the community.
For similar tasks
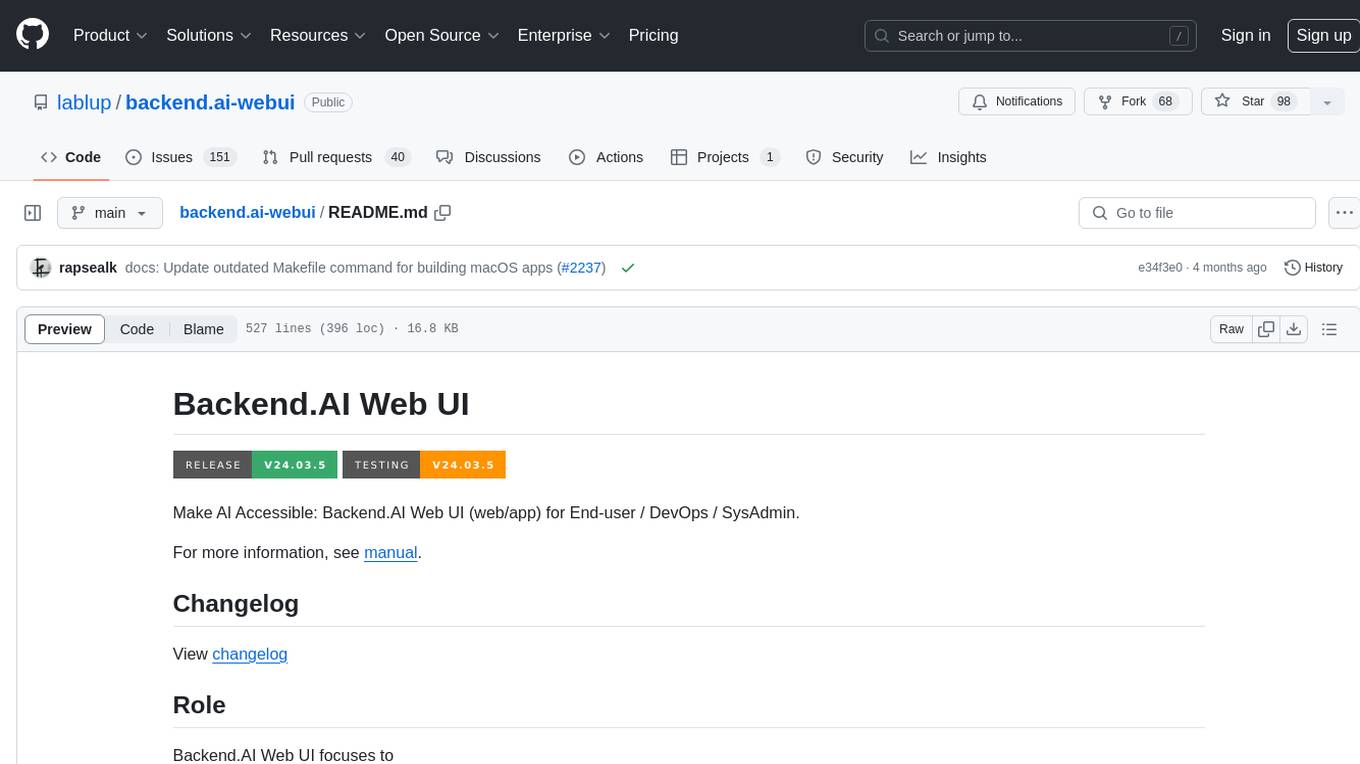
backend.ai-webui
Backend.AI Web UI is a user-friendly web and app interface designed to make AI accessible for end-users, DevOps, and SysAdmins. It provides features for session management, inference service management, pipeline management, storage management, node management, statistics, configurations, license checking, plugins, help & manuals, kernel management, user management, keypair management, manager settings, proxy mode support, service information, and integration with the Backend.AI Web Server. The tool supports various devices, offers a built-in websocket proxy feature, and allows for versatile usage across different platforms. Users can easily manage resources, run environment-supported apps, access a web-based terminal, use Visual Studio Code editor, manage experiments, set up autoscaling, manage pipelines, handle storage, monitor nodes, view statistics, configure settings, and more.
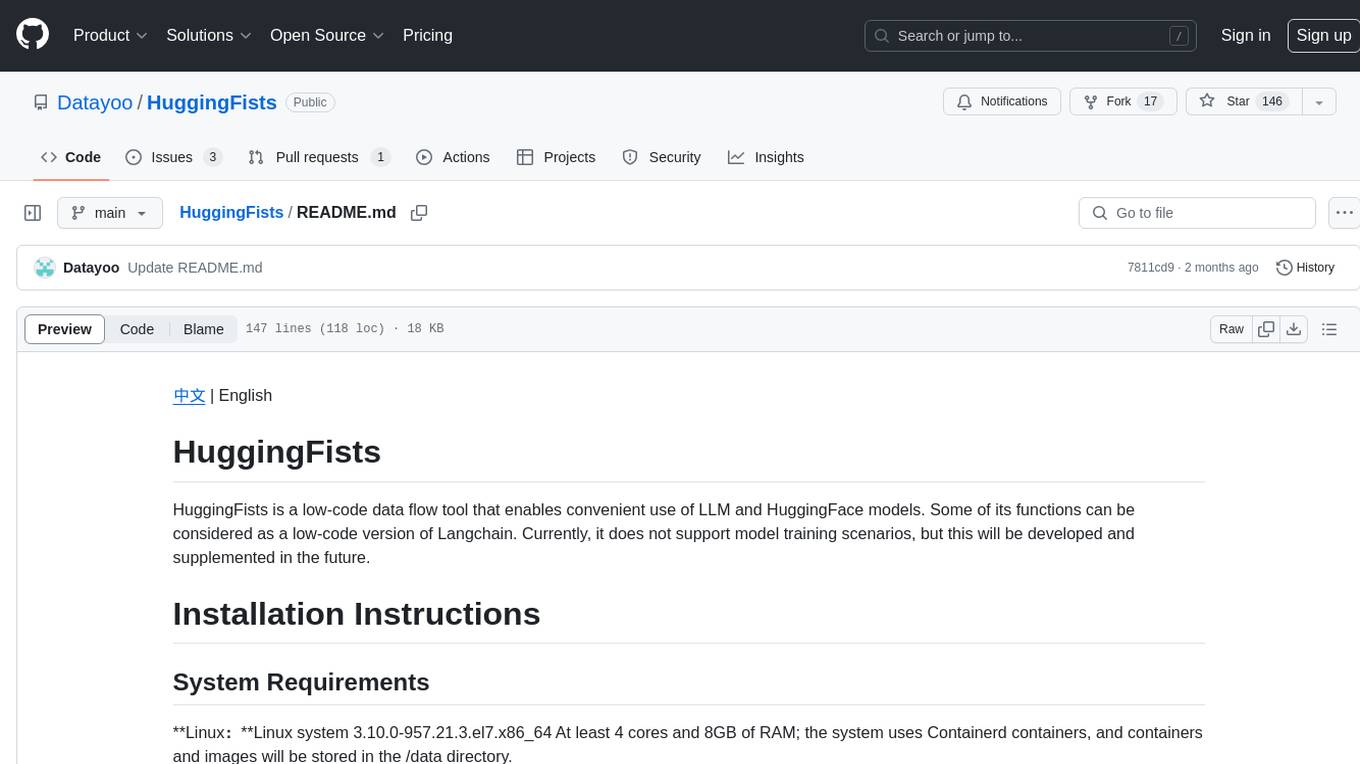
HuggingFists
HuggingFists is a low-code data flow tool that enables convenient use of LLM and HuggingFace models. It provides functionalities similar to Langchain, allowing users to design, debug, and manage data processing workflows, create and schedule workflow jobs, manage resources environment, and handle various data artifact resources. The tool also offers account management for users, allowing centralized management of data source accounts and API accounts. Users can access Hugging Face models through the Inference API or locally deployed models, as well as datasets on Hugging Face. HuggingFists supports breakpoint debugging, branch selection, function calls, workflow variables, and more to assist users in developing complex data processing workflows.
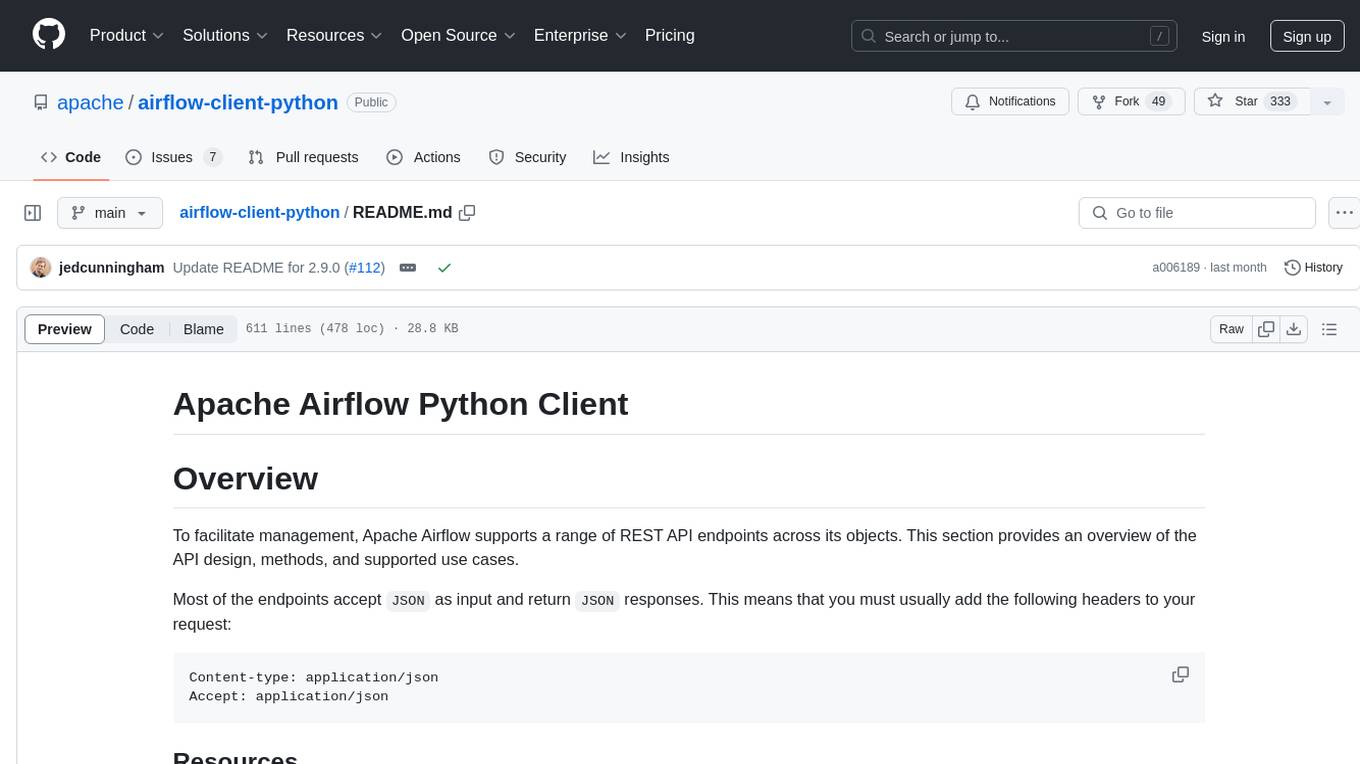
airflow-client-python
The Apache Airflow Python Client provides a range of REST API endpoints for managing Airflow metadata objects. It supports CRUD operations for resources, with endpoints accepting and returning JSON. Users can create, read, update, and delete resources. The API design follows conventions with consistent naming and field formats. Update mask is available for patch endpoints to specify fields for update. API versioning is not synchronized with Airflow releases, and changes go through a deprecation phase. The tool supports various authentication methods and error responses follow RFC 7807 format.
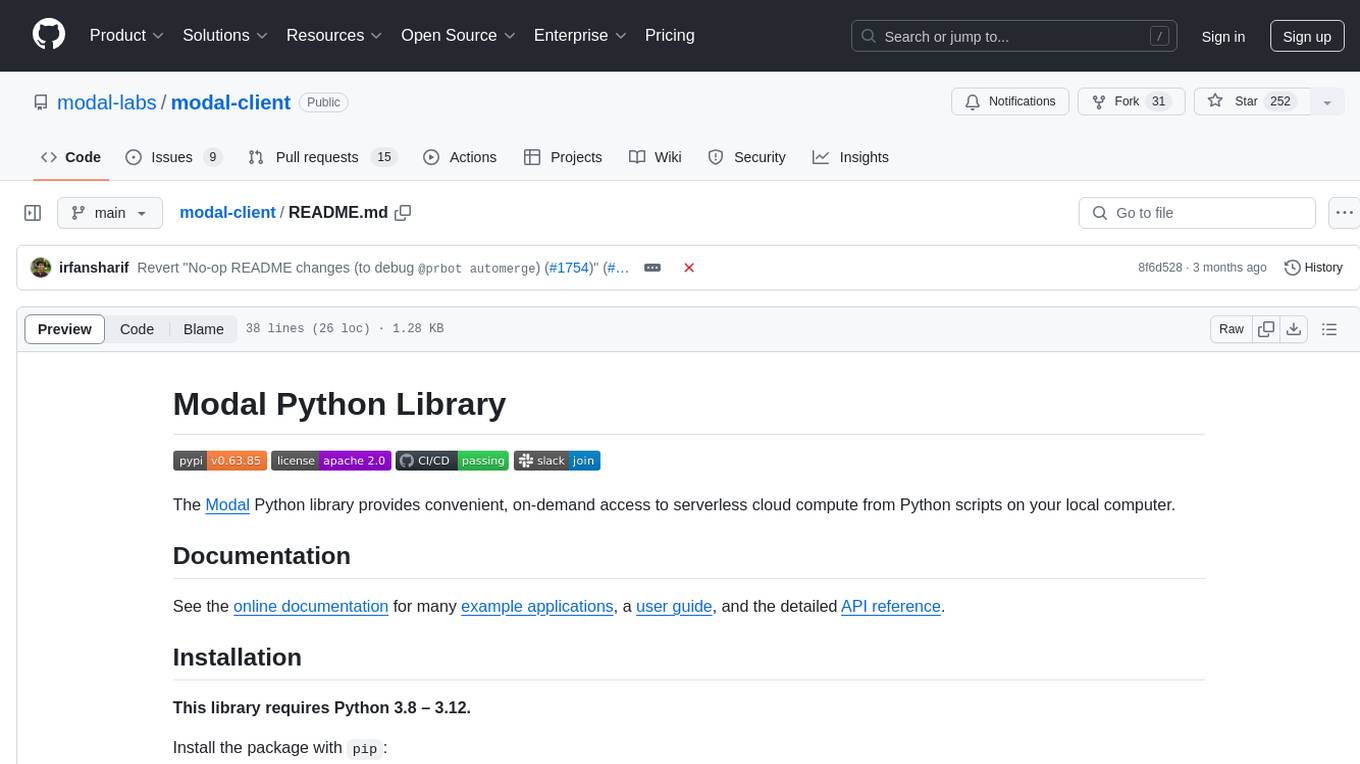
modal-client
The Modal Python library provides convenient, on-demand access to serverless cloud compute from Python scripts on your local computer. It allows users to easily integrate serverless cloud computing into their Python scripts, providing a seamless experience for accessing cloud resources. The library simplifies the process of interacting with cloud services, enabling developers to focus on their applications' logic rather than infrastructure management. With detailed documentation and support available through the Modal Slack channel, users can quickly get started and leverage the power of serverless computing in their projects.
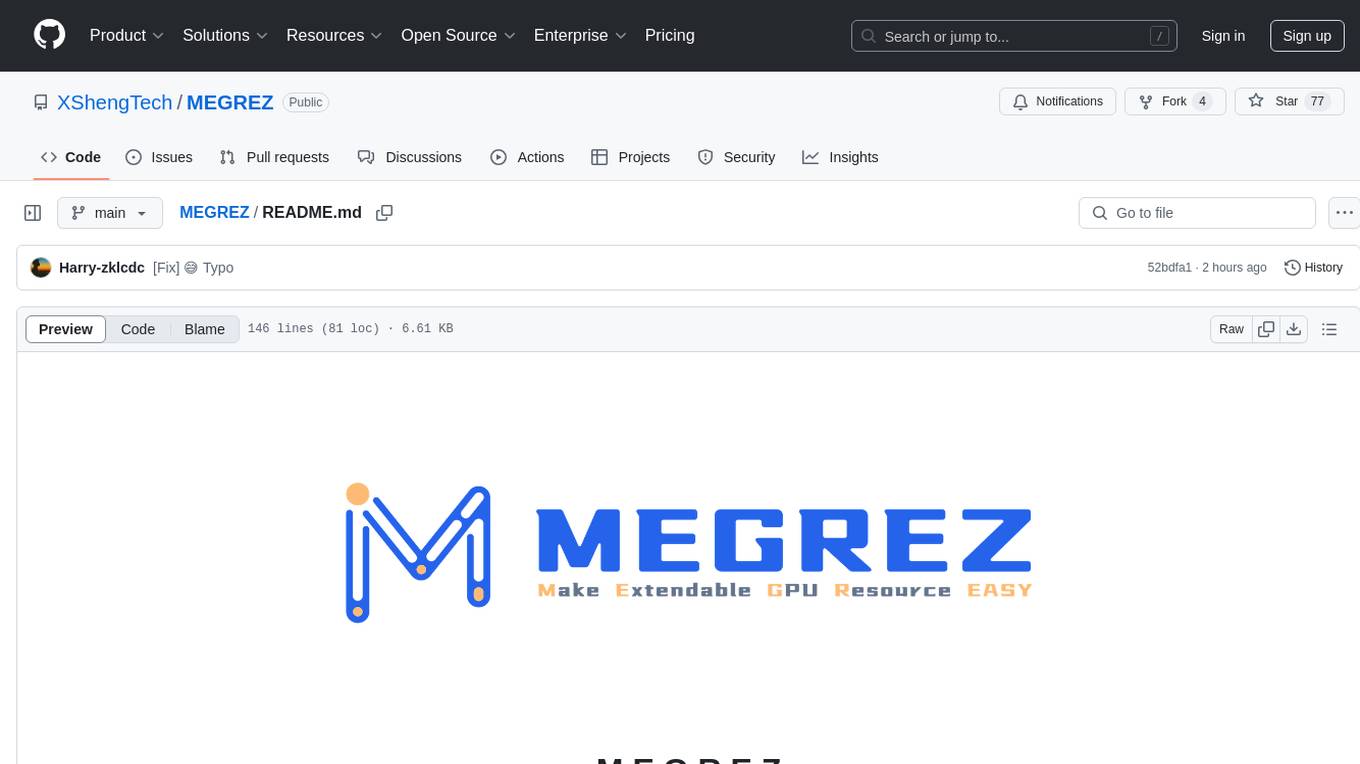
MEGREZ
MEGREZ is a modern and elegant open-source high-performance computing platform that efficiently manages GPU resources. It allows for easy container instance creation, supports multiple nodes/multiple GPUs, modern UI environment isolation, customizable performance configurations, and user data isolation. The platform also comes with pre-installed deep learning environments, supports multiple users, features a VSCode web version, resource performance monitoring dashboard, and Jupyter Notebook support.
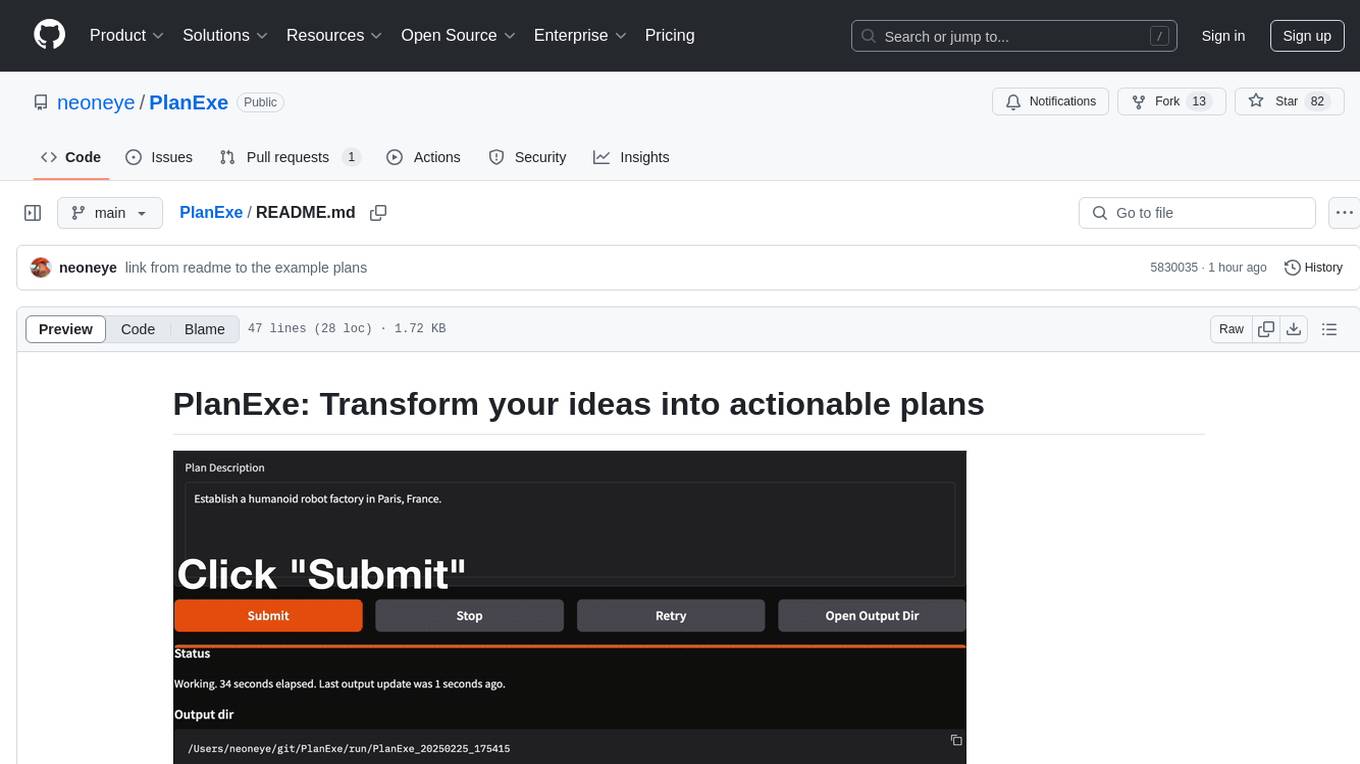
PlanExe
PlanExe is a planning AI tool that helps users generate detailed plans based on vague descriptions. It offers a Gradio-based web interface for easy input and output. Users can choose between running models in the cloud or locally on a high-end computer. The tool aims to provide a straightforward path to planning various tasks efficiently.
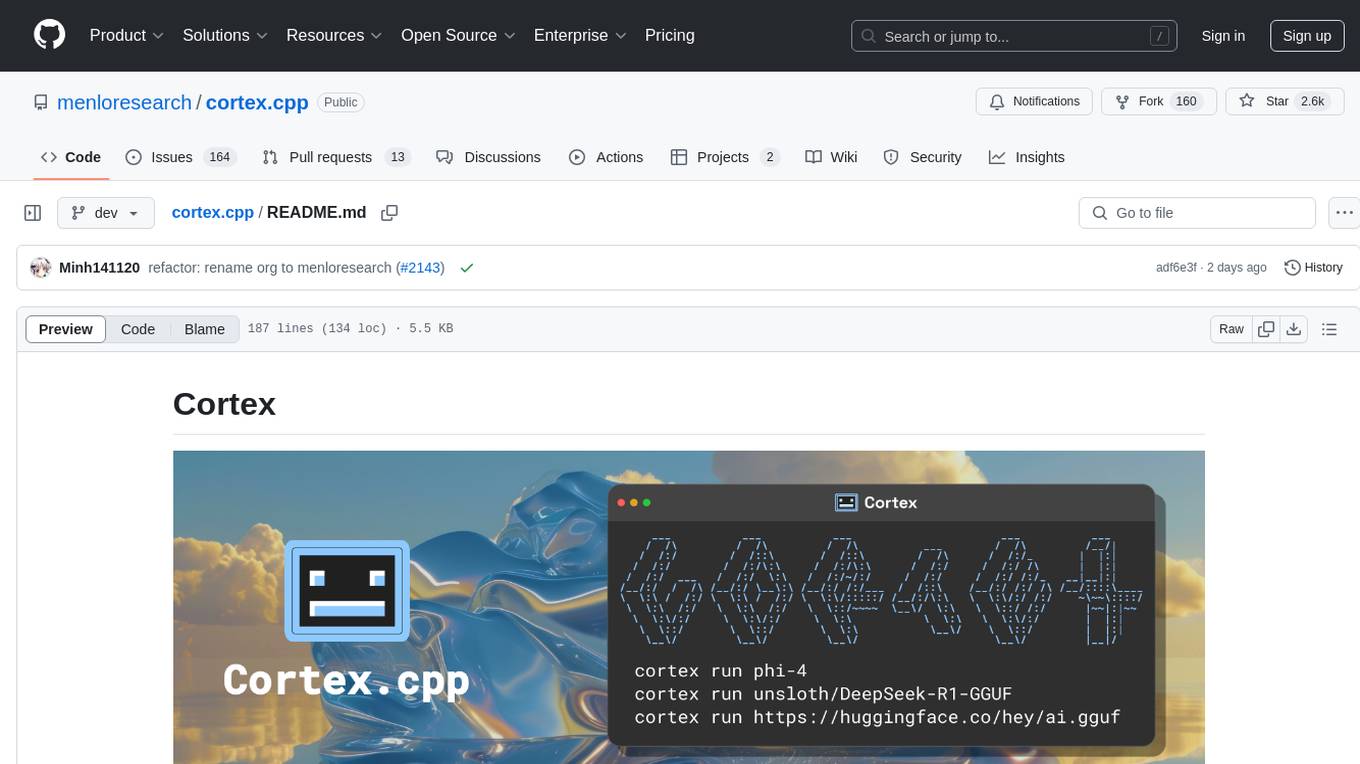
cortex.cpp
Cortex.cpp is an open-source platform designed as the brain for robots, offering functionalities such as vision, speech, language, tabular data processing, and action. It provides an AI platform for running AI models with multi-engine support, hardware optimization with automatic GPU detection, and an OpenAI-compatible API. Users can download models from the Hugging Face model hub, run models, manage resources, and access advanced features like multiple quantizations and engine management. The tool is under active development, promising rapid improvements for users.
For similar jobs
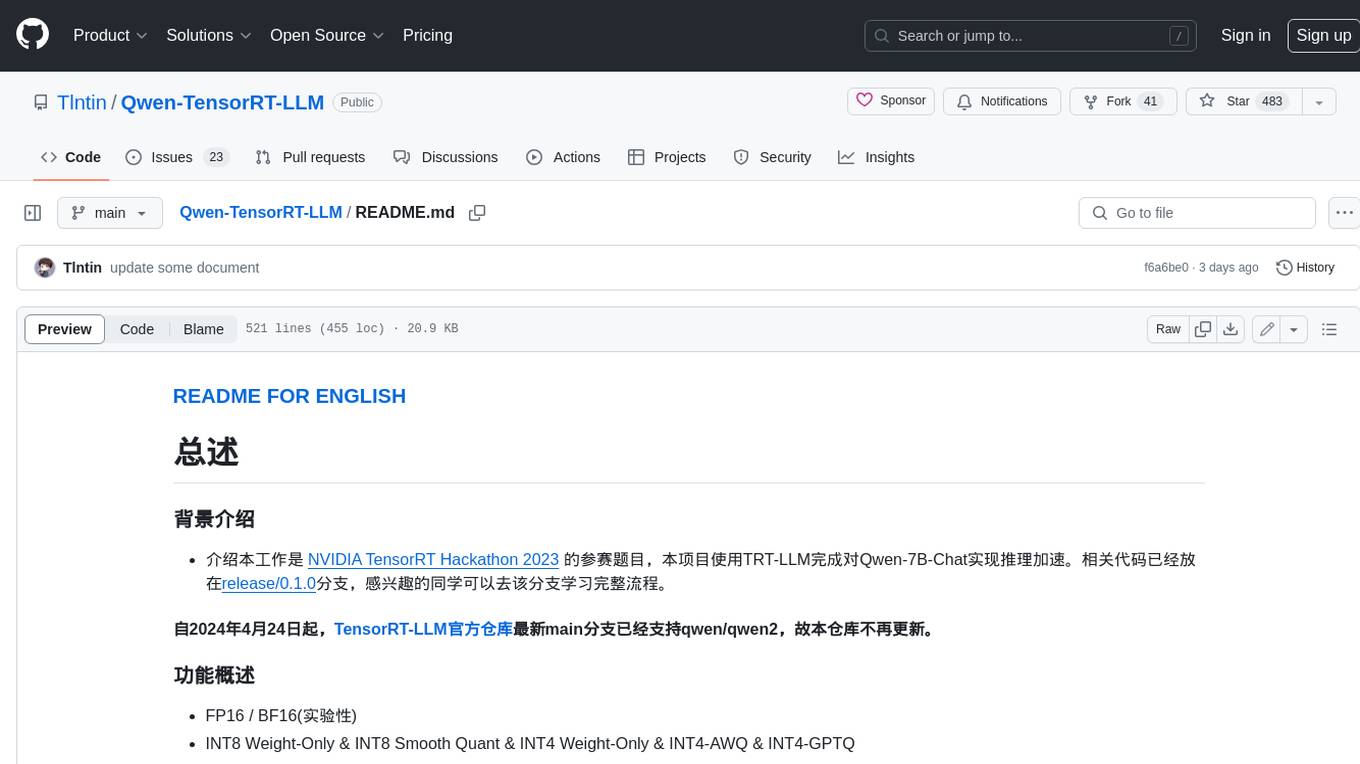
Qwen-TensorRT-LLM
Qwen-TensorRT-LLM is a project developed for the NVIDIA TensorRT Hackathon 2023, focusing on accelerating inference for the Qwen-7B-Chat model using TRT-LLM. The project offers various functionalities such as FP16/BF16 support, INT8 and INT4 quantization options, Tensor Parallel for multi-GPU parallelism, web demo setup with gradio, Triton API deployment for maximum throughput/concurrency, fastapi integration for openai requests, CLI interaction, and langchain support. It supports models like qwen2, qwen, and qwen-vl for both base and chat models. The project also provides tutorials on Bilibili and blogs for adapting Qwen models in NVIDIA TensorRT-LLM, along with hardware requirements and quick start guides for different model types and quantization methods.
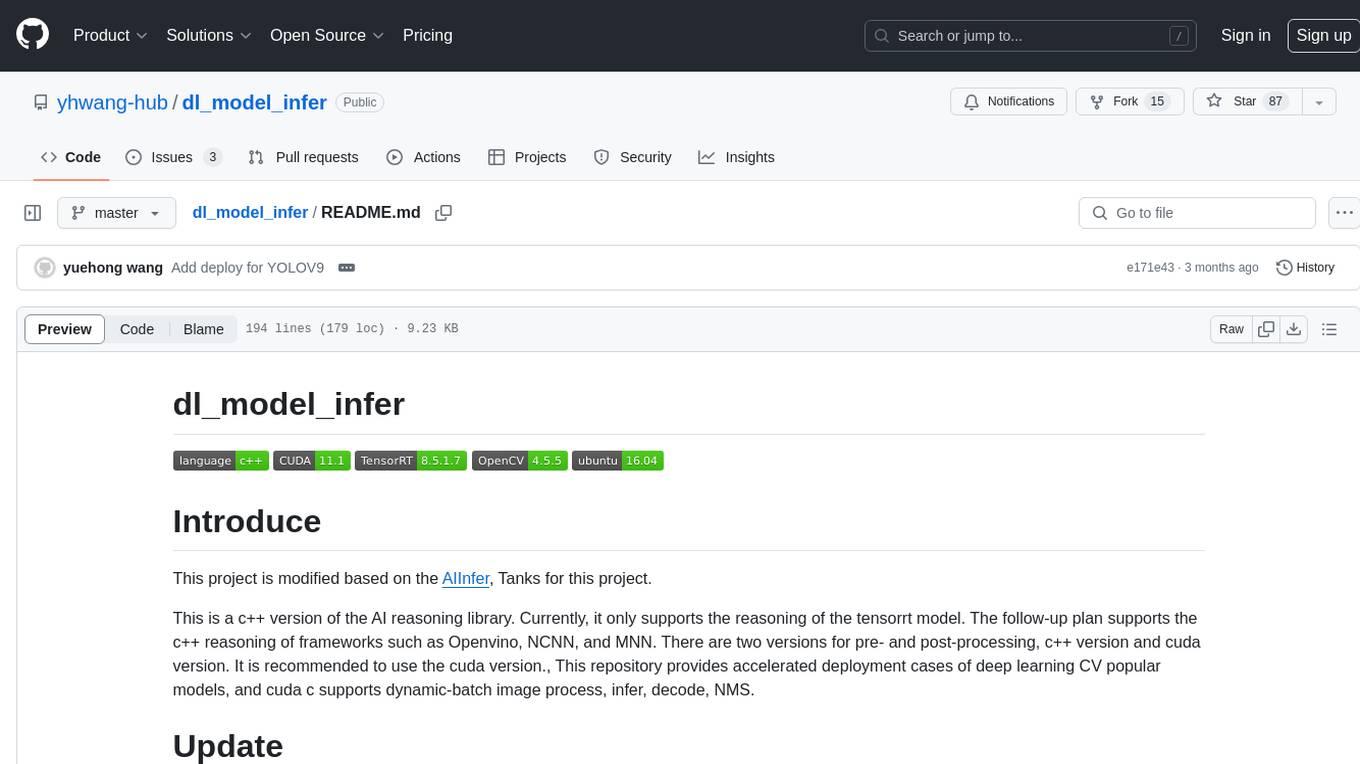
dl_model_infer
This project is a c++ version of the AI reasoning library that supports the reasoning of tensorrt models. It provides accelerated deployment cases of deep learning CV popular models and supports dynamic-batch image processing, inference, decode, and NMS. The project has been updated with various models and provides tutorials for model exports. It also includes a producer-consumer inference model for specific tasks. The project directory includes implementations for model inference applications, backend reasoning classes, post-processing, pre-processing, and target detection and tracking. Speed tests have been conducted on various models, and onnx downloads are available for different models.
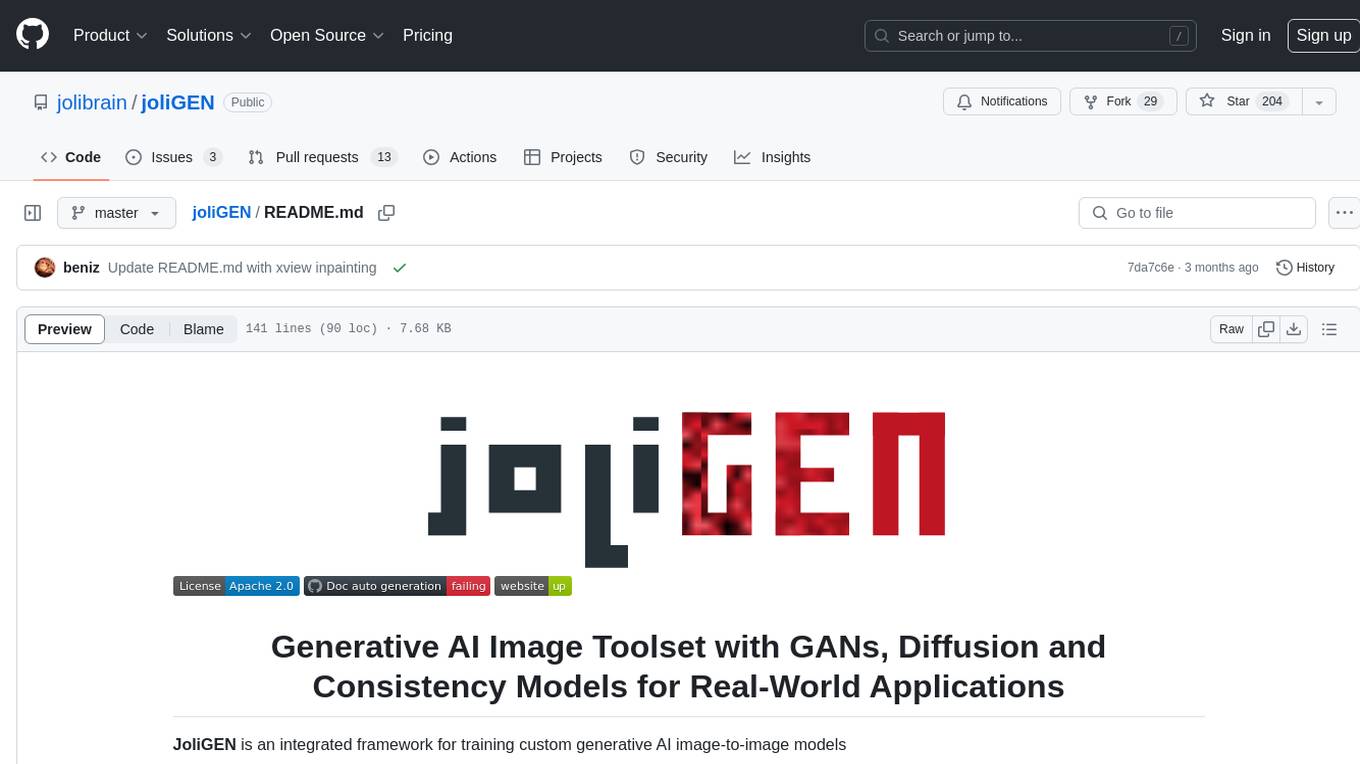
joliGEN
JoliGEN is an integrated framework for training custom generative AI image-to-image models. It implements GAN, Diffusion, and Consistency models for various image translation tasks, including domain and style adaptation with conservation of semantics. The tool is designed for real-world applications such as Controlled Image Generation, Augmented Reality, Dataset Smart Augmentation, and Synthetic to Real transforms. JoliGEN allows for fast and stable training with a REST API server for simplified deployment. It offers a wide range of options and parameters with detailed documentation available for models, dataset formats, and data augmentation.
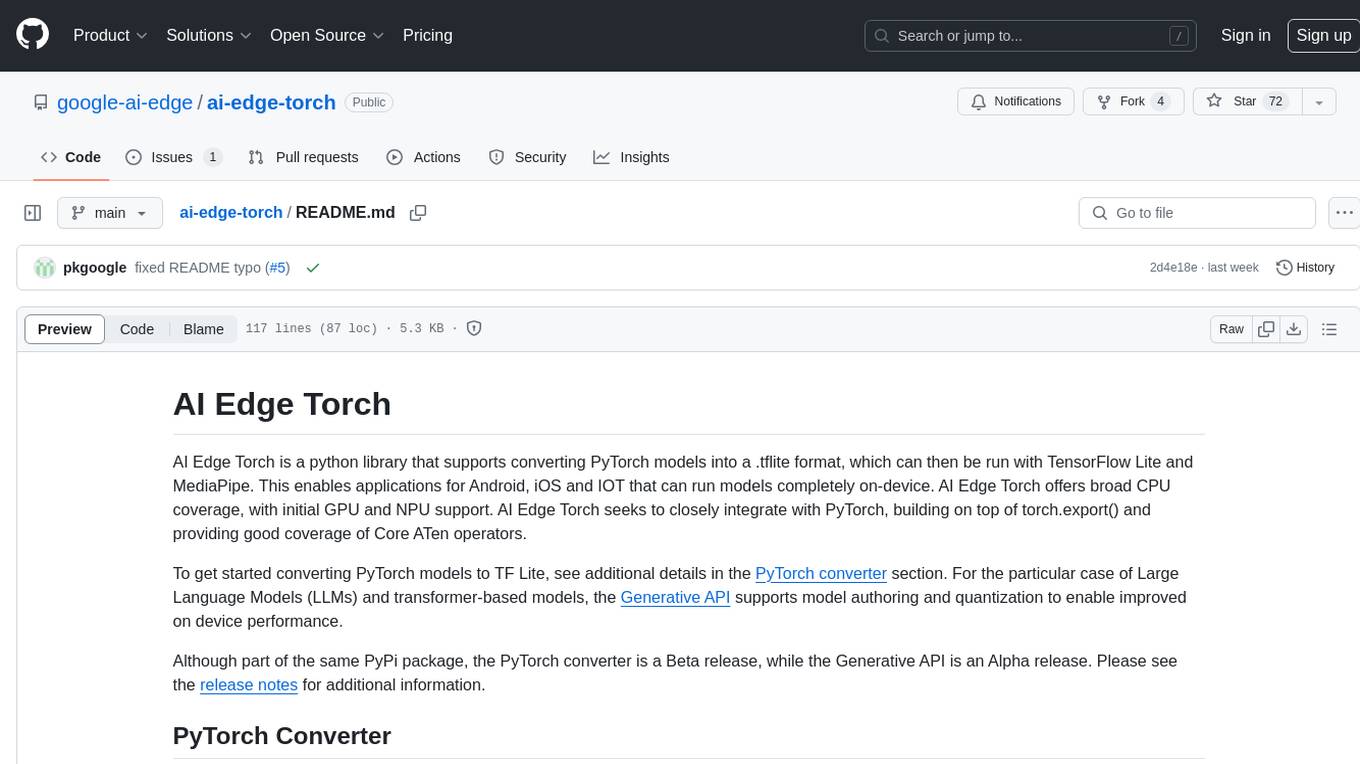
ai-edge-torch
AI Edge Torch is a Python library that supports converting PyTorch models into a .tflite format for on-device applications on Android, iOS, and IoT devices. It offers broad CPU coverage with initial GPU and NPU support, closely integrating with PyTorch and providing good coverage of Core ATen operators. The library includes a PyTorch converter for model conversion and a Generative API for authoring mobile-optimized PyTorch Transformer models, enabling easy deployment of Large Language Models (LLMs) on mobile devices.
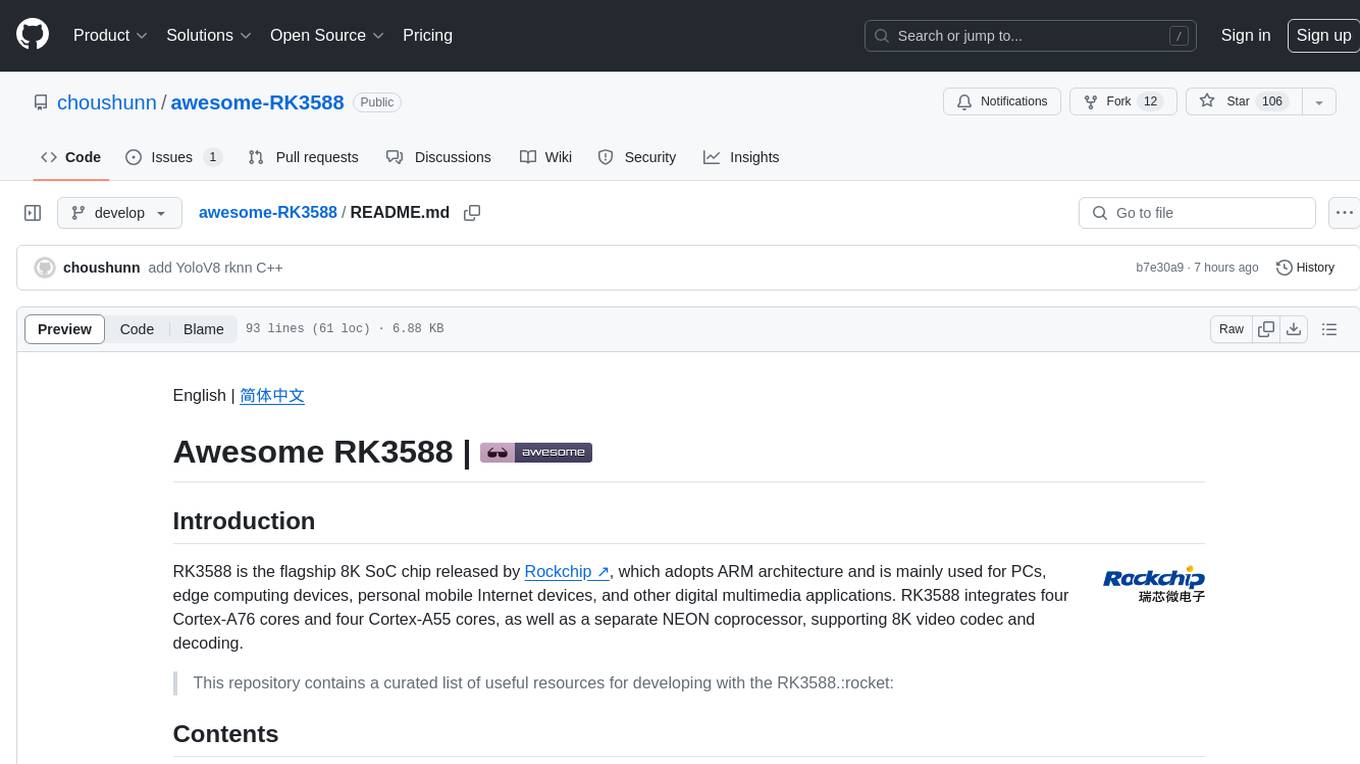
awesome-RK3588
RK3588 is a flagship 8K SoC chip by Rockchip, integrating Cortex-A76 and Cortex-A55 cores with NEON coprocessor for 8K video codec. This repository curates resources for developing with RK3588, including official resources, RKNN models, projects, development boards, documentation, tools, and sample code.
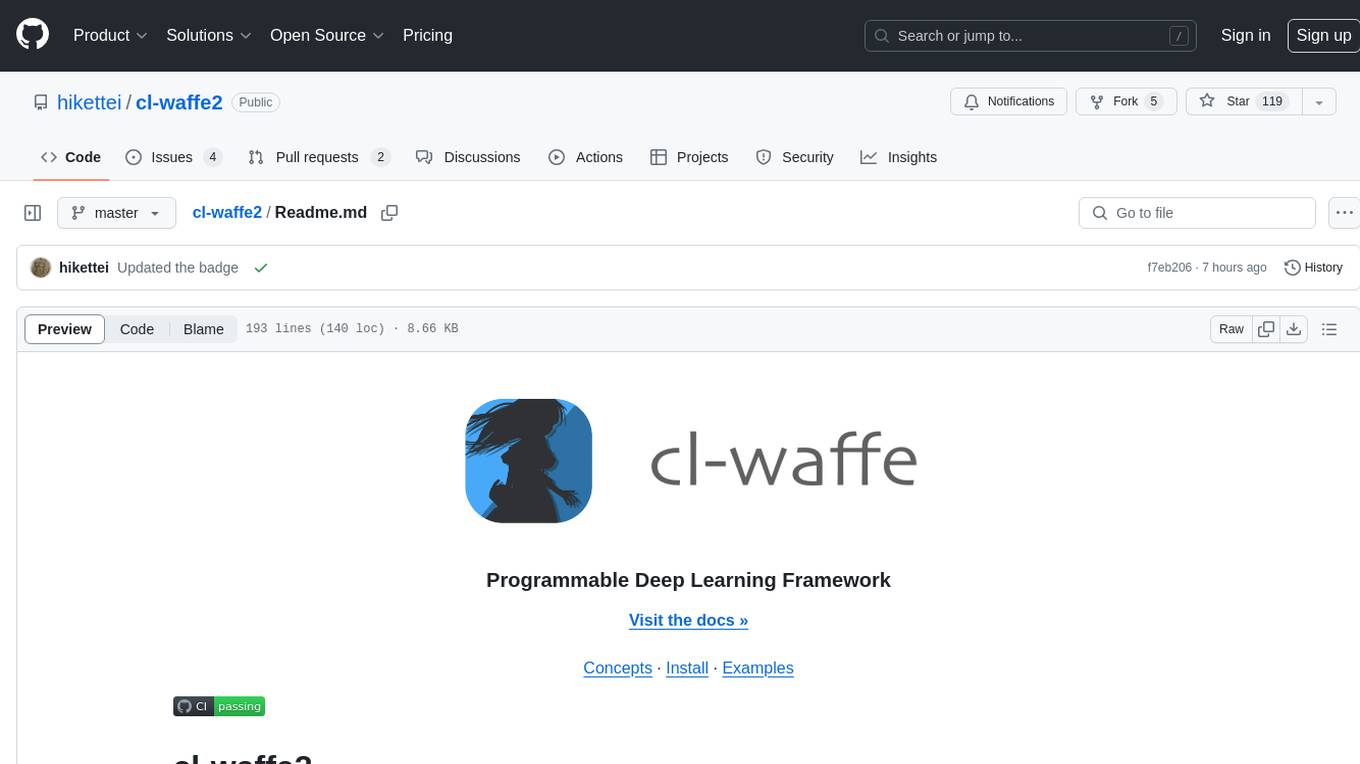
cl-waffe2
cl-waffe2 is an experimental deep learning framework in Common Lisp, providing fast, systematic, and customizable matrix operations, reverse mode tape-based Automatic Differentiation, and neural network model building and training features accelerated by a JIT Compiler. It offers abstraction layers, extensibility, inlining, graph-level optimization, visualization, debugging, systematic nodes, and symbolic differentiation. Users can easily write extensions and optimize their networks without overheads. The framework is designed to eliminate barriers between users and developers, allowing for easy customization and extension.
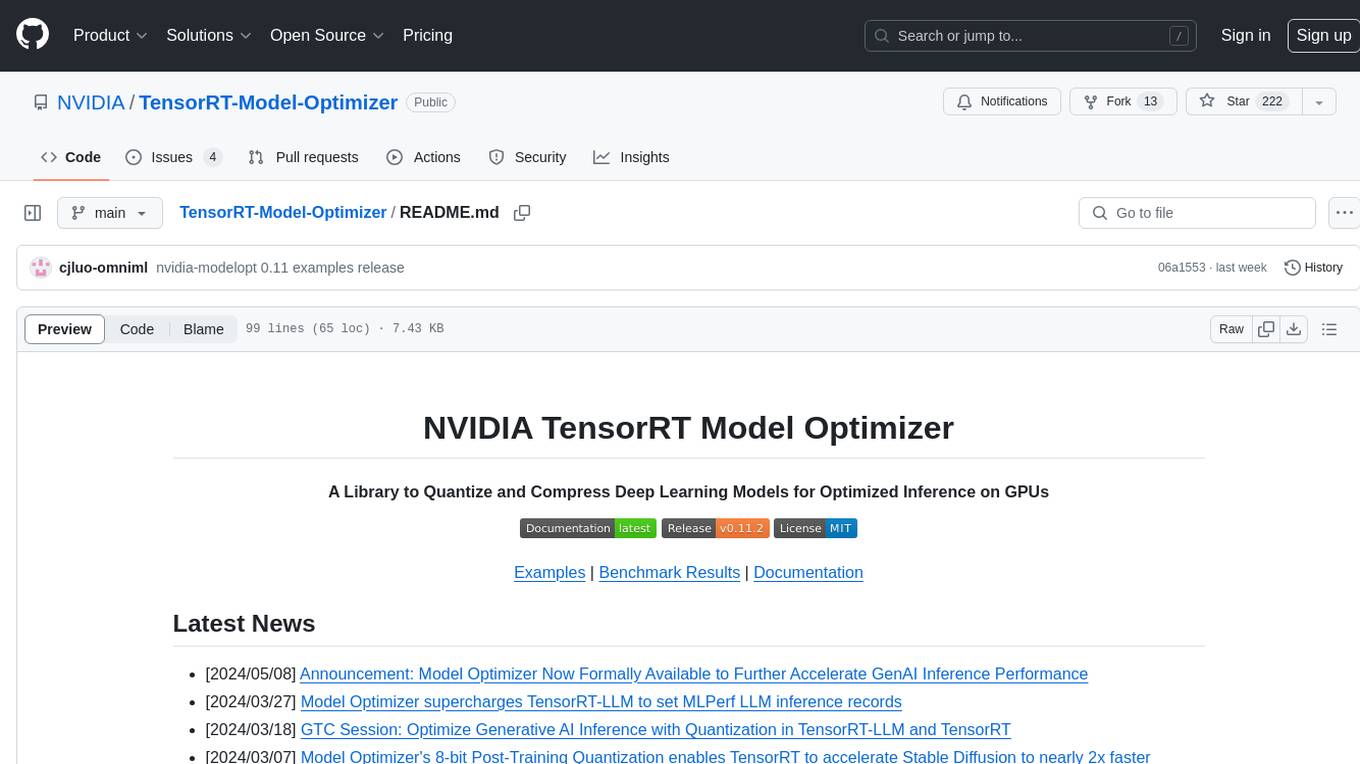
TensorRT-Model-Optimizer
The NVIDIA TensorRT Model Optimizer is a library designed to quantize and compress deep learning models for optimized inference on GPUs. It offers state-of-the-art model optimization techniques including quantization and sparsity to reduce inference costs for generative AI models. Users can easily stack different optimization techniques to produce quantized checkpoints from torch or ONNX models. The quantized checkpoints are ready for deployment in inference frameworks like TensorRT-LLM or TensorRT, with planned integrations for NVIDIA NeMo and Megatron-LM. The tool also supports 8-bit quantization with Stable Diffusion for enterprise users on NVIDIA NIM. Model Optimizer is available for free on NVIDIA PyPI, and this repository serves as a platform for sharing examples, GPU-optimized recipes, and collecting community feedback.
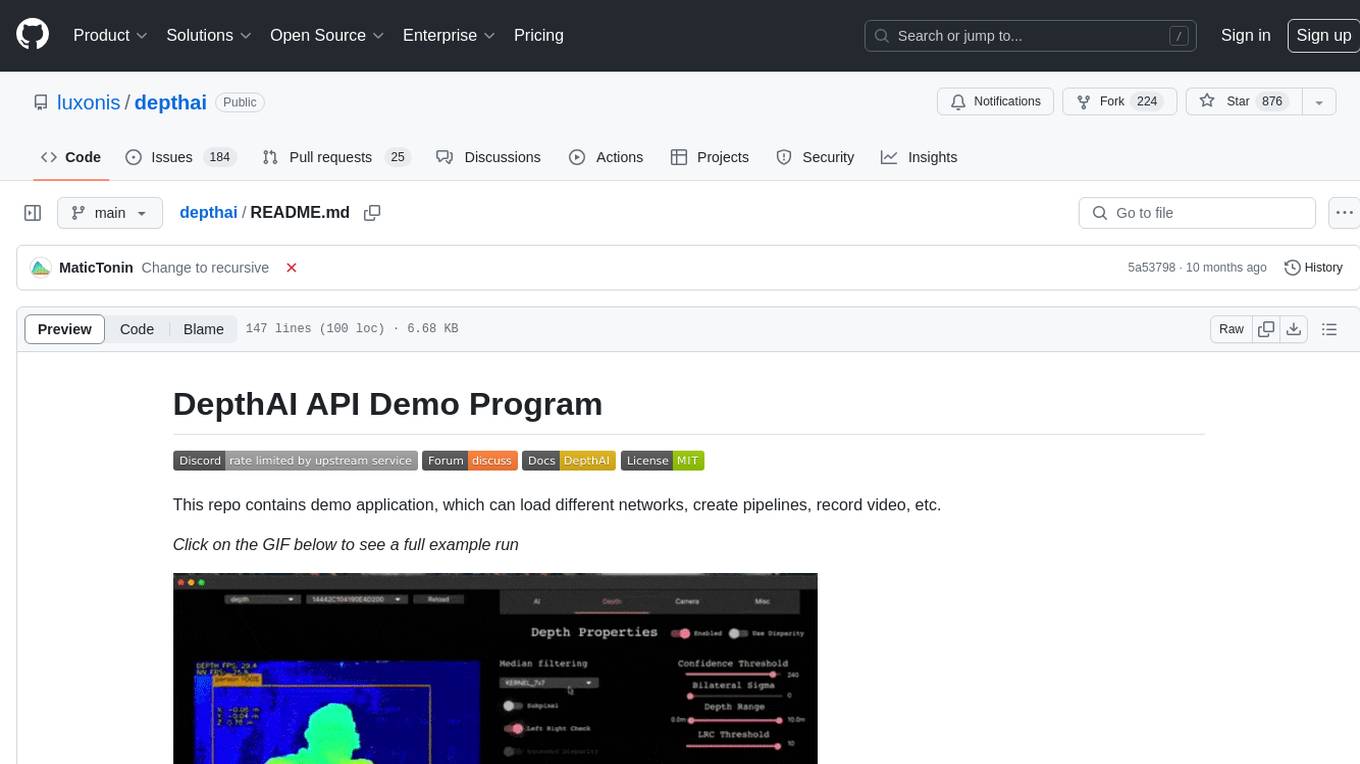
depthai
This repository contains a demo application for DepthAI, a tool that can load different networks, create pipelines, record video, and more. It provides documentation for installation and usage, including running programs through Docker. Users can explore DepthAI features via command line arguments or a clickable QT interface. Supported models include various AI models for tasks like face detection, human pose estimation, and object detection. The tool collects anonymous usage statistics by default, which can be disabled. Users can report issues to the development team for support and troubleshooting.