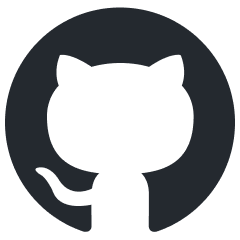
airflow-client-python
Apache Airflow - OpenApi Client for Python
Stars: 346
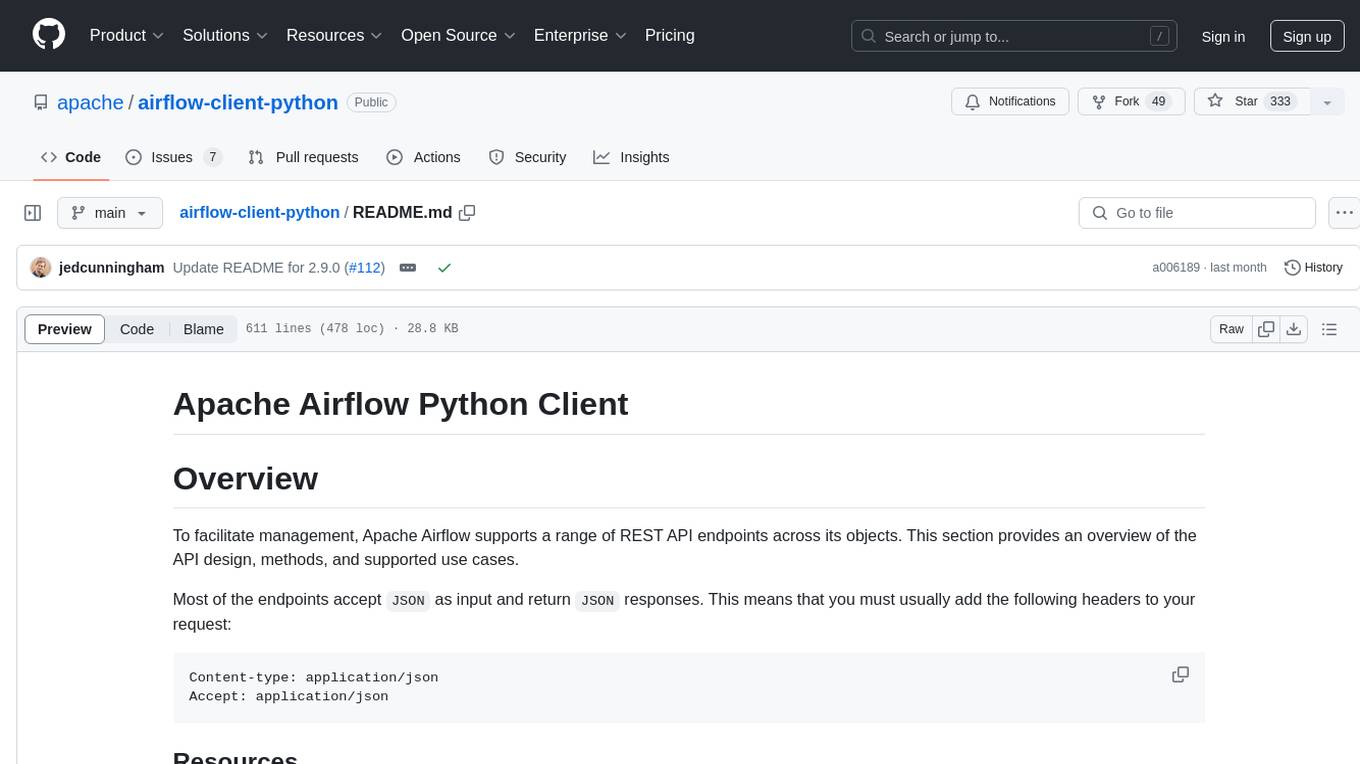
The Apache Airflow Python Client provides a range of REST API endpoints for managing Airflow metadata objects. It supports CRUD operations for resources, with endpoints accepting and returning JSON. Users can create, read, update, and delete resources. The API design follows conventions with consistent naming and field formats. Update mask is available for patch endpoints to specify fields for update. API versioning is not synchronized with Airflow releases, and changes go through a deprecation phase. The tool supports various authentication methods and error responses follow RFC 7807 format.
README:
To facilitate management, Apache Airflow supports a range of REST API endpoints across its objects. This section provides an overview of the API design, methods, and supported use cases.
Most of the endpoints accept JSON
as input and return JSON
responses.
This means that you must usually add the following headers to your request:
Content-type: application/json
Accept: application/json
The term resource
refers to a single type of object in the Airflow metadata. An API is broken up by its
endpoint's corresponding resource.
The name of a resource is typically plural and expressed in camelCase. Example: dagRuns
.
Resource names are used as part of endpoint URLs, as well as in API parameters and responses.
The platform supports Create, Read, Update, and Delete operations on most resources. You can review the standards for these operations and their standard parameters below.
Some endpoints have special behavior as exceptions.
To create a resource, you typically submit an HTTP POST
request with the resource's required metadata
in the request body.
The response returns a 201 Created
response code upon success with the resource's metadata, including
its internal id
, in the response body.
The HTTP GET
request can be used to read a resource or to list a number of resources.
A resource's id
can be submitted in the request parameters to read a specific resource.
The response usually returns a 200 OK
response code upon success, with the resource's metadata in
the response body.
If a GET
request does not include a specific resource id
, it is treated as a list request.
The response usually returns a 200 OK
response code upon success, with an object containing a list
of resources' metadata in the response body.
When reading resources, some common query parameters are usually available. e.g.:
v1/connections?limit=25&offset=25
Query Parameter | Type | Description |
---|---|---|
limit | integer | Maximum number of objects to fetch. Usually 25 by default |
offset | integer | Offset after which to start returning objects. For use with limit query parameter. |
Updating a resource requires the resource id
, and is typically done using an HTTP PATCH
request,
with the fields to modify in the request body.
The response usually returns a 200 OK
response code upon success, with information about the modified
resource in the response body.
Deleting a resource requires the resource id
and is typically executing via an HTTP DELETE
request.
The response usually returns a 204 No Content
response code upon success.
-
Resource names are plural and expressed in camelCase.
-
Names are consistent between URL parameter name and field name.
-
Field names are in snake_case.
{
\"name\": \"string\",
\"slots\": 0,
\"occupied_slots\": 0,
\"used_slots\": 0,
\"queued_slots\": 0,
\"open_slots\": 0
}
Update mask is available as a query parameter in patch endpoints. It is used to notify the
API which fields you want to update. Using update_mask
makes it easier to update objects
by helping the server know which fields to update in an object instead of updating all fields.
The update request ignores any fields that aren't specified in the field mask, leaving them with
their current values.
Example:
import requests
resource = requests.get("/resource/my-id").json()
resource["my_field"] = "new-value"
requests.patch("/resource/my-id?update_mask=my_field", data=json.dumps(resource))
- API versioning is not synchronized to specific releases of the Apache Airflow.
- APIs are designed to be backward compatible.
- Any changes to the API will first go through a deprecation phase.
You can use a third party client, such as curl, HTTPie, Postman or the Insomnia rest client to test the Apache Airflow API.
Note that you will need to pass credentials data.
For e.g., here is how to pause a DAG with curl, when basic authorization is used:
curl -X PATCH 'https://example.com/api/v1/dags/{dag_id}?update_mask=is_paused' \\
-H 'Content-Type: application/json' \\
--user \"username:password\" \\
-d '{
\"is_paused\": true
}'
Using a graphical tool such as Postman or Insomnia, it is possible to import the API specifications directly:
- Download the API specification by clicking the Download button at top of this document.
- Import the JSON specification in the graphical tool of your choice.
- In Postman, you can click the import button at the top
- With Insomnia, you can just drag-and-drop the file on the UI
Note that with Postman, you can also generate code snippets by selecting a request and clicking on the Code button.
Cross-origin resource sharing (CORS) is a browser security feature that restricts HTTP requests that are initiated from scripts running in the browser.
For details on enabling/configuring CORS, see Enabling CORS.
To be able to meet the requirements of many organizations, Airflow supports many authentication methods, and it is even possible to add your own method.
If you want to check which auth backend is currently set, you can use
airflow config get-value api auth_backends
command as in the example below.
$ airflow config get-value api auth_backends
airflow.api.auth.backend.basic_auth
The default is to deny all requests.
For details on configuring the authentication, see API Authorization.
We follow the error response format proposed in RFC 7807 also known as Problem Details for HTTP APIs. As with our normal API responses, your client must be prepared to gracefully handle additional members of the response.
This indicates that the request has not been applied because it lacks valid authentication credentials for the target resource. Please check that you have valid credentials.
This response means that the server understood the request but refuses to authorize it because it lacks sufficient rights to the resource. It happens when you do not have the necessary permission to execute the action you performed. You need to get the appropriate permissions in other to resolve this error.
This response means that the server cannot or will not process the request due to something that is perceived to be a client error (e.g., malformed request syntax, invalid request message framing, or deceptive request routing). To resolve this, please ensure that your syntax is correct.
This client error response indicates that the server cannot find the requested resource.
Indicates that the request method is known by the server but is not supported by the target resource.
The target resource does not have a current representation that would be acceptable to the user agent, according to the proactive negotiation header fields received in the request, and the server is unwilling to supply a default representation.
The request could not be completed due to a conflict with the current state of the target resource, e.g. the resource it tries to create already exists.
This means that the server encountered an unexpected condition that prevented it from fulfilling the request.
This Python package is automatically generated by the OpenAPI Generator project:
- API version: 2.9.0
- Package version: 2.9.0
- Build package: org.openapitools.codegen.languages.PythonClientCodegen
For more information, please visit https://airflow.apache.org
Python >=3.8
You can install the client using standard Python installation tools. It is hosted
in PyPI with apache-airflow-client
package id so the easiest way to get the latest
version is to run:
pip install apache-airflow-client
If the python package is hosted on a repository, you can install directly using:
pip install git+https://github.com/apache/airflow-client-python.git
Then import the package:
import airflow_client.client
Please follow the installation procedure and then run the following:
import time
from airflow_client import client
from pprint import pprint
from airflow_client.client.api import config_api
from airflow_client.client.model.config import Config
from airflow_client.client.model.error import Error
# Defining the host is optional and defaults to /api/v1
# See configuration.py for a list of all supported configuration parameters.
configuration = client.Configuration(host="/api/v1")
# The client must configure the authentication and authorization parameters
# in accordance with the API server security policy.
# Examples for each auth method are provided below, use the example that
# satisfies your auth use case.
# Configure HTTP basic authorization: Basic
configuration = client.Configuration(username="YOUR_USERNAME", password="YOUR_PASSWORD")
# Enter a context with an instance of the API client
with client.ApiClient(configuration) as api_client:
# Create an instance of the API class
api_instance = config_api.ConfigApi(api_client)
try:
# Get current configuration
api_response = api_instance.get_config()
pprint(api_response)
except client.ApiException as e:
print("Exception when calling ConfigApi->get_config: %s\n" % e)
All URIs are relative to /api/v1
Class | Method | HTTP request | Description |
---|---|---|---|
ConfigApi | get_config | GET /config | Get current configuration |
ConnectionApi | delete_connection | DELETE /connections/{connection_id} | Delete a connection |
ConnectionApi | get_connection | GET /connections/{connection_id} | Get a connection |
ConnectionApi | get_connections | GET /connections | List connections |
ConnectionApi | patch_connection | PATCH /connections/{connection_id} | Update a connection |
ConnectionApi | post_connection | POST /connections | Create a connection |
ConnectionApi | test_connection | POST /connections/test | Test a connection |
DAGApi | delete_dag | DELETE /dags/{dag_id} | Delete a DAG |
DAGApi | get_dag | GET /dags/{dag_id} | Get basic information about a DAG |
DAGApi | get_dag_details | GET /dags/{dag_id}/details | Get a simplified representation of DAG |
DAGApi | get_dag_source | GET /dagSources/{file_token} | Get a source code |
DAGApi | get_dags | GET /dags | List DAGs |
DAGApi | get_task | GET /dags/{dag_id}/tasks/{task_id} | Get simplified representation of a task |
DAGApi | get_tasks | GET /dags/{dag_id}/tasks | Get tasks for DAG |
DAGApi | patch_dag | PATCH /dags/{dag_id} | Update a DAG |
DAGApi | patch_dags | PATCH /dags | Update DAGs |
DAGApi | post_clear_task_instances | POST /dags/{dag_id}/clearTaskInstances | Clear a set of task instances |
DAGApi | post_set_task_instances_state | POST /dags/{dag_id}/updateTaskInstancesState | Set a state of task instances |
DAGRunApi | clear_dag_run | POST /dags/{dag_id}/dagRuns/{dag_run_id}/clear | Clear a DAG run |
DAGRunApi | delete_dag_run | DELETE /dags/{dag_id}/dagRuns/{dag_run_id} | Delete a DAG run |
DAGRunApi | get_dag_run | GET /dags/{dag_id}/dagRuns/{dag_run_id} | Get a DAG run |
DAGRunApi | get_dag_runs | GET /dags/{dag_id}/dagRuns | List DAG runs |
DAGRunApi | get_dag_runs_batch | POST /dags/~/dagRuns/list | List DAG runs (batch) |
DAGRunApi | get_upstream_dataset_events | GET /dags/{dag_id}/dagRuns/{dag_run_id}/upstreamDatasetEvents | Get dataset events for a DAG run |
DAGRunApi | post_dag_run | POST /dags/{dag_id}/dagRuns | Trigger a new DAG run |
DAGRunApi | set_dag_run_note | PATCH /dags/{dag_id}/dagRuns/{dag_run_id}/setNote | Update the DagRun note. |
DAGRunApi | update_dag_run_state | PATCH /dags/{dag_id}/dagRuns/{dag_run_id} | Modify a DAG run |
DagWarningApi | get_dag_warnings | GET /dagWarnings | List dag warnings |
DatasetApi | get_dataset | GET /datasets/{uri} | Get a dataset |
DatasetApi | get_dataset_events | GET /datasets/events | Get dataset events |
DatasetApi | get_datasets | GET /datasets | List datasets |
DatasetApi | get_upstream_dataset_events | GET /dags/{dag_id}/dagRuns/{dag_run_id}/upstreamDatasetEvents | Get dataset events for a DAG run |
EventLogApi | get_event_log | GET /eventLogs/{event_log_id} | Get a log entry |
EventLogApi | get_event_logs | GET /eventLogs | List log entries |
ImportErrorApi | get_import_error | GET /importErrors/{import_error_id} | Get an import error |
ImportErrorApi | get_import_errors | GET /importErrors | List import errors |
MonitoringApi | get_health | GET /health | Get instance status |
MonitoringApi | get_version | GET /version | Get version information |
PermissionApi | get_permissions | GET /permissions | List permissions |
PluginApi | get_plugins | GET /plugins | Get a list of loaded plugins |
PoolApi | delete_pool | DELETE /pools/{pool_name} | Delete a pool |
PoolApi | get_pool | GET /pools/{pool_name} | Get a pool |
PoolApi | get_pools | GET /pools | List pools |
PoolApi | patch_pool | PATCH /pools/{pool_name} | Update a pool |
PoolApi | post_pool | POST /pools | Create a pool |
ProviderApi | get_providers | GET /providers | List providers |
RoleApi | delete_role | DELETE /roles/{role_name} | Delete a role |
RoleApi | get_role | GET /roles/{role_name} | Get a role |
RoleApi | get_roles | GET /roles | List roles |
RoleApi | patch_role | PATCH /roles/{role_name} | Update a role |
RoleApi | post_role | POST /roles | Create a role |
TaskInstanceApi | get_extra_links | GET /dags/{dag_id}/dagRuns/{dag_run_id}/taskInstances/{task_id}/links | List extra links |
TaskInstanceApi | get_log | GET /dags/{dag_id}/dagRuns/{dag_run_id}/taskInstances/{task_id}/logs/{task_try_number} | Get logs |
TaskInstanceApi | get_mapped_task_instance | GET /dags/{dag_id}/dagRuns/{dag_run_id}/taskInstances/{task_id}/{map_index} | Get a mapped task instance |
TaskInstanceApi | get_mapped_task_instances | GET /dags/{dag_id}/dagRuns/{dag_run_id}/taskInstances/{task_id}/listMapped | List mapped task instances |
TaskInstanceApi | get_task_instance | GET /dags/{dag_id}/dagRuns/{dag_run_id}/taskInstances/{task_id} | Get a task instance |
TaskInstanceApi | get_task_instances | GET /dags/{dag_id}/dagRuns/{dag_run_id}/taskInstances | List task instances |
TaskInstanceApi | get_task_instances_batch |
POST /dags/ |
List task instances (batch) |
TaskInstanceApi | patch_mapped_task_instance | PATCH /dags/{dag_id}/dagRuns/{dag_run_id}/taskInstances/{task_id}/{map_index} | Updates the state of a mapped task instance |
TaskInstanceApi | patch_task_instance | PATCH /dags/{dag_id}/dagRuns/{dag_run_id}/taskInstances/{task_id} | Updates the state of a task instance |
TaskInstanceApi | set_mapped_task_instance_note | PATCH /dags/{dag_id}/dagRuns/{dag_run_id}/taskInstances/{task_id}/{map_index}/setNote | Update the TaskInstance note. |
TaskInstanceApi | set_task_instance_note | PATCH /dags/{dag_id}/dagRuns/{dag_run_id}/taskInstances/{task_id}/setNote | Update the TaskInstance note. |
UserApi | delete_user | DELETE /users/{username} | Delete a user |
UserApi | get_user | GET /users/{username} | Get a user |
UserApi | get_users | GET /users | List users |
UserApi | patch_user | PATCH /users/{username} | Update a user |
UserApi | post_user | POST /users | Create a user |
VariableApi | delete_variable | DELETE /variables/{variable_key} | Delete a variable |
VariableApi | get_variable | GET /variables/{variable_key} | Get a variable |
VariableApi | get_variables | GET /variables | List variables |
VariableApi | patch_variable | PATCH /variables/{variable_key} | Update a variable |
VariableApi | post_variables | POST /variables | Create a variable |
XComApi | get_xcom_entries | GET /dags/{dag_id}/dagRuns/{dag_run_id}/taskInstances/{task_id}/xcomEntries | List XCom entries |
XComApi | get_xcom_entry | GET /dags/{dag_id}/dagRuns/{dag_run_id}/taskInstances/{task_id}/xcomEntries/{xcom_key} | Get an XCom entry |
- Action
- ActionCollection
- ActionCollectionAllOf
- ActionResource
- BasicDAGRun
- ClassReference
- ClearDagRun
- ClearTaskInstances
- CollectionInfo
- Color
- Config
- ConfigOption
- ConfigSection
- Connection
- ConnectionAllOf
- ConnectionCollection
- ConnectionCollectionAllOf
- ConnectionCollectionItem
- ConnectionTest
- CronExpression
- DAG
- DAGCollection
- DAGCollectionAllOf
- DAGDetail
- DAGDetailAllOf
- DAGRun
- DAGRunCollection
- DAGRunCollectionAllOf
- DagScheduleDatasetReference
- DagState
- DagWarning
- DagWarningCollection
- DagWarningCollectionAllOf
- Dataset
- DatasetCollection
- DatasetCollectionAllOf
- DatasetEvent
- DatasetEventCollection
- DatasetEventCollectionAllOf
- Error
- EventLog
- EventLogCollection
- EventLogCollectionAllOf
- ExtraLink
- ExtraLinkCollection
- HealthInfo
- HealthStatus
- ImportError
- ImportErrorCollection
- ImportErrorCollectionAllOf
- InlineResponse200
- InlineResponse2001
- Job
- ListDagRunsForm
- ListTaskInstanceForm
- MetadatabaseStatus
- PluginCollection
- PluginCollectionAllOf
- PluginCollectionItem
- Pool
- PoolCollection
- PoolCollectionAllOf
- Provider
- ProviderCollection
- RelativeDelta
- Resource
- Role
- RoleCollection
- RoleCollectionAllOf
- SLAMiss
- ScheduleInterval
- SchedulerStatus
- SetDagRunNote
- SetTaskInstanceNote
- Tag
- Task
- TaskCollection
- TaskExtraLinks
- TaskInstance
- TaskInstanceCollection
- TaskInstanceCollectionAllOf
- TaskInstanceReference
- TaskInstanceReferenceCollection
- TaskOutletDatasetReference
- TaskState
- TimeDelta
- Trigger
- TriggerRule
- UpdateDagRunState
- UpdateTaskInstance
- UpdateTaskInstancesState
- User
- UserAllOf
- UserCollection
- UserCollectionAllOf
- UserCollectionItem
- UserCollectionItemRoles
- Variable
- VariableAllOf
- VariableCollection
- VariableCollectionAllOf
- VariableCollectionItem
- VersionInfo
- WeightRule
- XCom
- XComAllOf
- XComCollection
- XComCollectionAllOf
- XComCollectionItem
By default the generated client supports the three authentication schemes:
- Basic
- GoogleOpenID
- Kerberos
However, you can generate client and documentation with your own schemes by adding your own schemes in
the security section of the OpenAPI specification. You can do it with Breeze CLI by adding the
--security-schemes
option to the breeze release-management prepare-python-client
command.
You can run basic smoke tests to check if the client is working properly - we have a simple test script that uses the API to run the tests. To do that, you need to:
- install the
apache-airflow-client
package as described above - install
rich
Python package - download the test_python_client.py file
- make sure you have test airflow installation running. Do not experiment with your production deployment
- configure your airflow webserver to enable basic authentication
In the
[api]
section of yourairflow.cfg
set:
[api]
auth_backend = airflow.api.auth.backend.session,airflow.api.auth.backend.basic_auth
You can also set it by env variable:
export AIRFLOW__API__AUTH_BACKENDS=airflow.api.auth.backend.session,airflow.api.auth.backend.basic_auth
- configure your airflow webserver to load example dags
In the
[core]
section of yourairflow.cfg
set:
[core]
load_examples = True
You can also set it by env variable: export AIRFLOW__CORE__LOAD_EXAMPLES=True
- optionally expose configuration (NOTE! that this is dangerous setting). The script will happily run with
the default setting, but if you want to see the configuration, you need to expose it.
In the
[webserver]
section of yourairflow.cfg
set:
[webserver]
expose_config = True
You can also set it by env variable: export AIRFLOW__WEBSERVER__EXPOSE_CONFIG=True
- Configure your host/ip/user/password in the
test_python_client.py
file
import airflow_client
# Configure HTTP basic authorization: Basic
configuration = airflow_client.client.Configuration(
host="http://localhost:8080/api/v1", username="admin", password="admin"
)
-
Run scheduler (or dag file processor you have setup with standalone dag file processor) for few parsing loops (you can pass --num-runs parameter to it or keep it running in the background). The script relies on example DAGs being serialized to the DB and this only happens when scheduler runs with
core/load_examples
set to True. -
Run webserver - reachable at the host/port for the test script you want to run. Make sure it had enough time to initialize.
Run python test_python_client.py
and you should see colored output showing attempts to connect and status.
If the OpenAPI document is large, imports in client.apis and client.models may fail with a RecursionError indicating the maximum recursion limit has been exceeded. In that case, there are a couple of solutions:
Solution 1: Use specific imports for apis and models like:
from airflow_client.client.api.default_api import DefaultApi
from airflow_client.client.model.pet import Pet
Solution 2: Before importing the package, adjust the maximum recursion limit as shown below:
import sys
sys.setrecursionlimit(1500)
import airflow_client.client
from airflow_client.client.apis import *
from airflow_client.client.models import *
For Tasks:
Click tags to check more tools for each tasksFor Jobs:
Alternative AI tools for airflow-client-python
Similar Open Source Tools
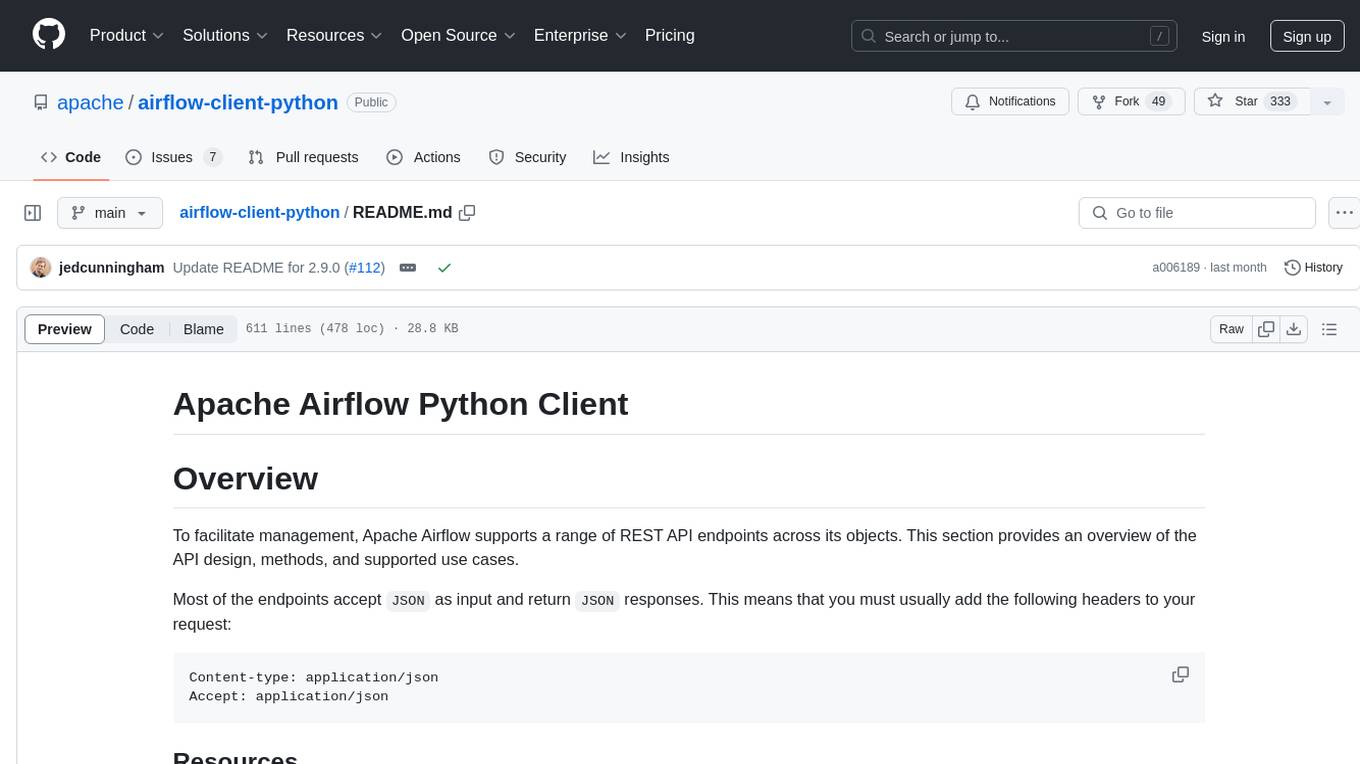
airflow-client-python
The Apache Airflow Python Client provides a range of REST API endpoints for managing Airflow metadata objects. It supports CRUD operations for resources, with endpoints accepting and returning JSON. Users can create, read, update, and delete resources. The API design follows conventions with consistent naming and field formats. Update mask is available for patch endpoints to specify fields for update. API versioning is not synchronized with Airflow releases, and changes go through a deprecation phase. The tool supports various authentication methods and error responses follow RFC 7807 format.
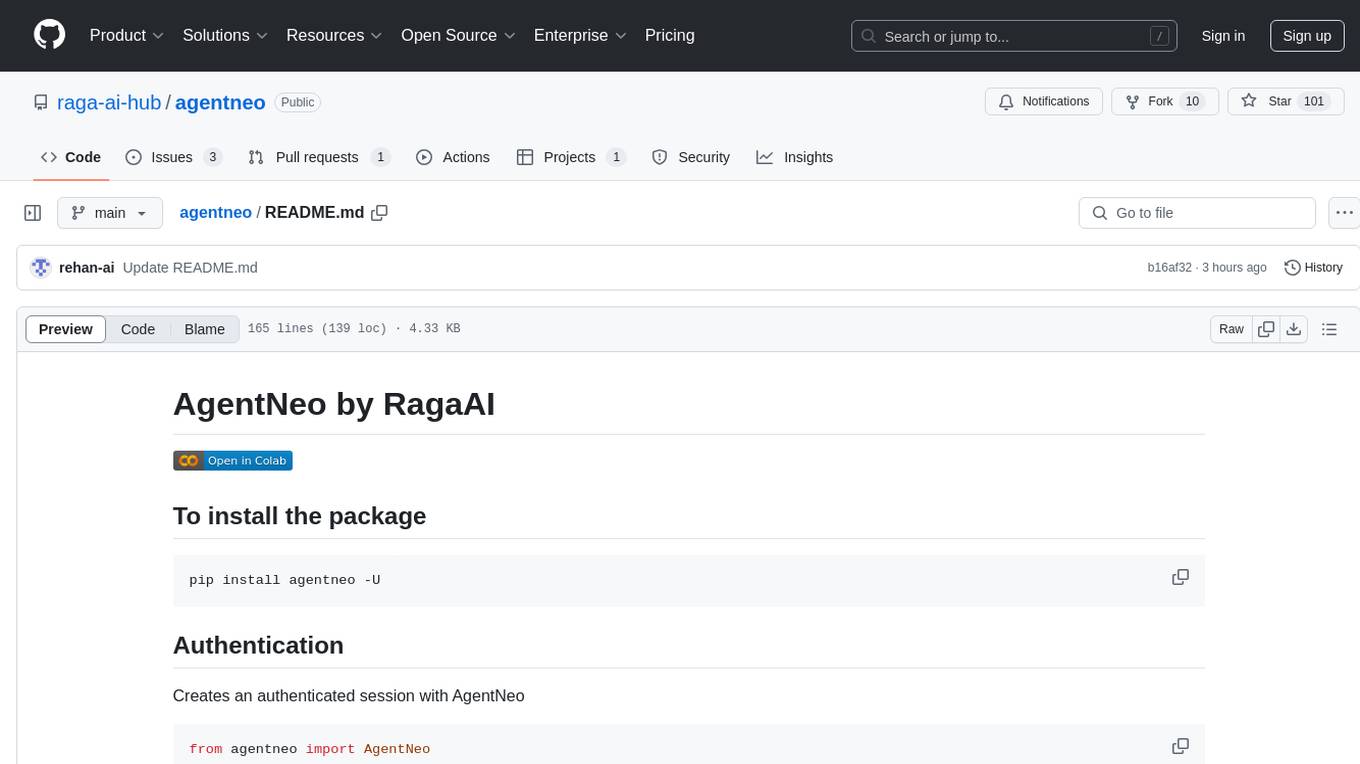
agentneo
AgentNeo is a Python package that provides functionalities for project, trace, dataset, experiment management. It allows users to authenticate, create projects, trace agents and LangGraph graphs, manage datasets, and run experiments with metrics. The tool aims to streamline AI project management and analysis by offering a comprehensive set of features.
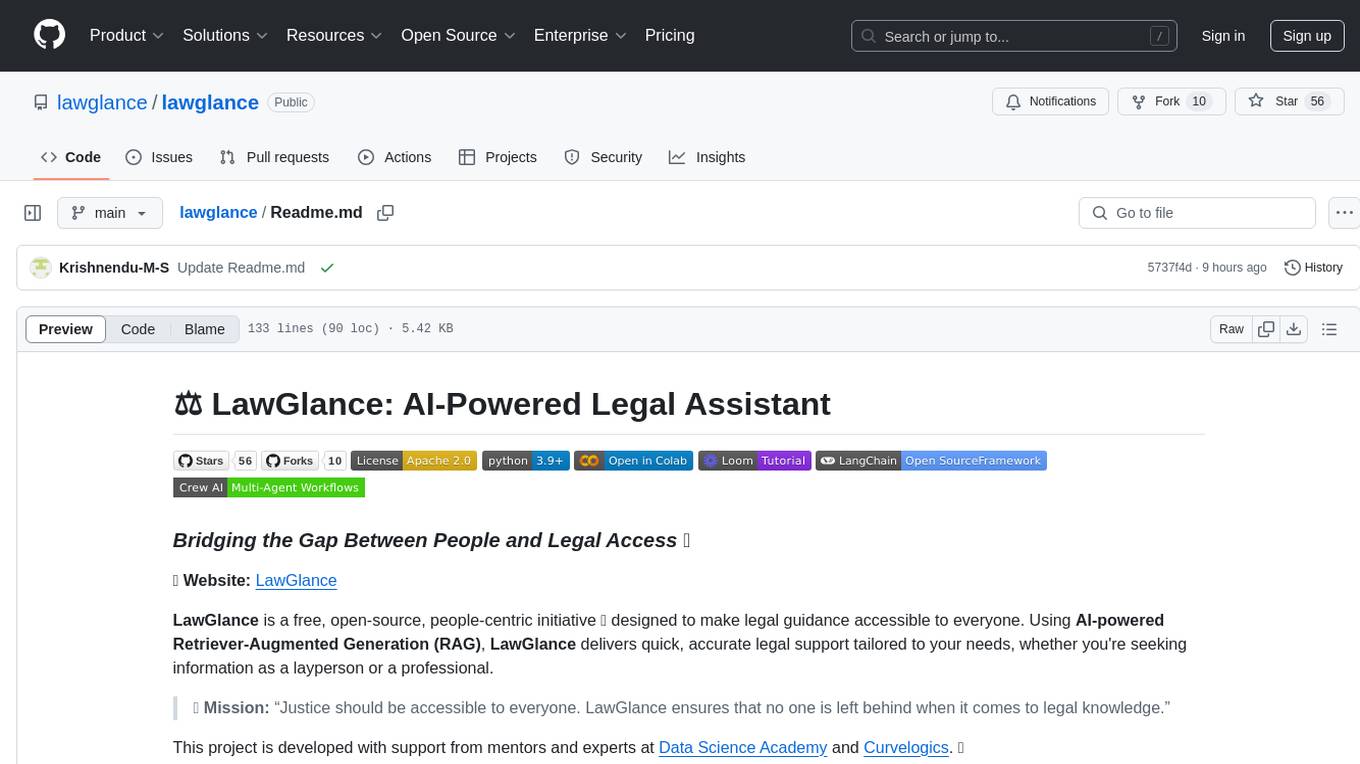
lawglance
LawGlance is an AI-powered legal assistant that aims to bridge the gap between people and legal access. It is a free, open-source initiative designed to provide quick and accurate legal support tailored to individual needs. The project covers various laws, with plans for international expansion in the future. LawGlance utilizes AI-powered Retriever-Augmented Generation (RAG) to deliver legal guidance accessible to both laypersons and professionals. The tool is developed with support from mentors and experts at Data Science Academy and Curvelogics.
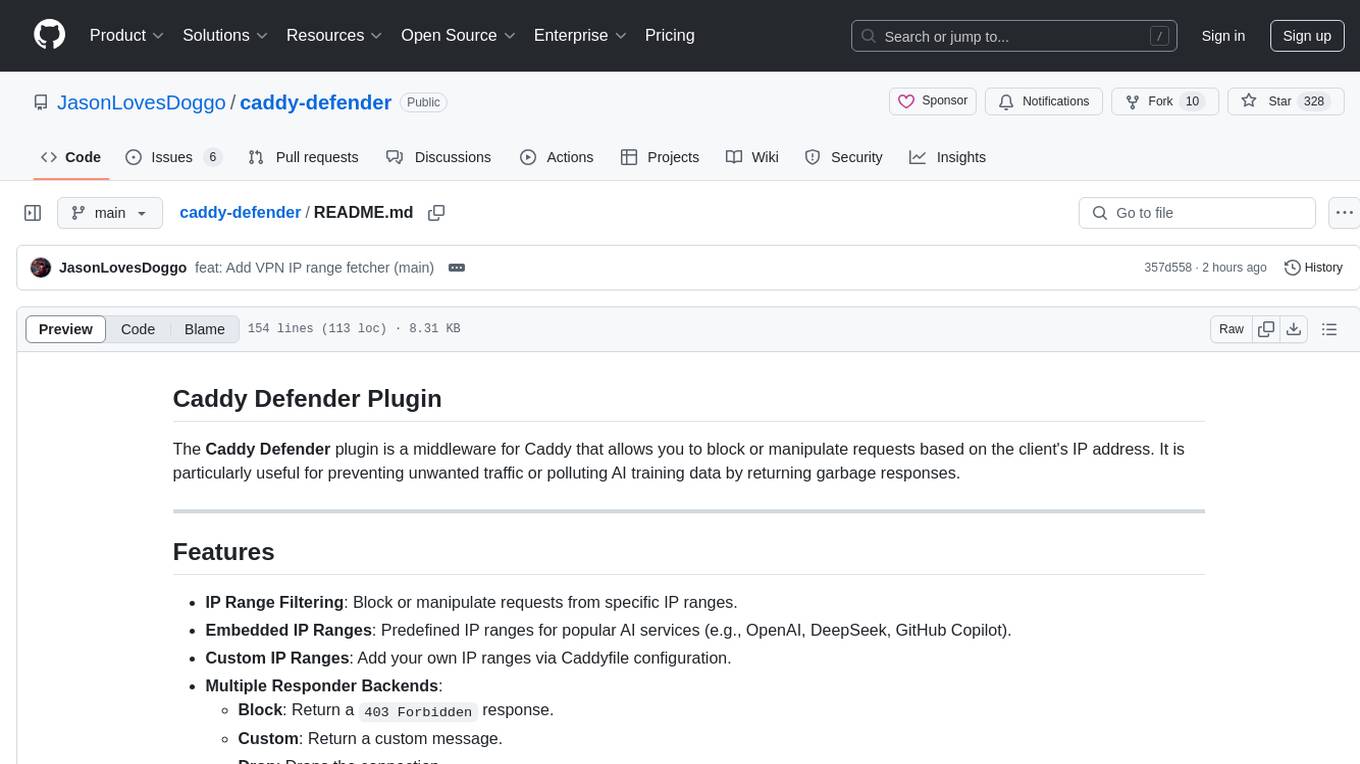
caddy-defender
The Caddy Defender plugin is a middleware for Caddy that allows you to block or manipulate requests based on the client's IP address. It provides features such as IP range filtering, predefined IP ranges for popular AI services, custom IP ranges configuration, and multiple responder backends for different actions like blocking, custom responses, dropping connections, returning garbage data, redirecting, and tarpitting to stall bots. The plugin can be easily installed using Docker or built with `xcaddy`. Configuration is done through the Caddyfile syntax with various options for responders, IP ranges, custom messages, and URLs.
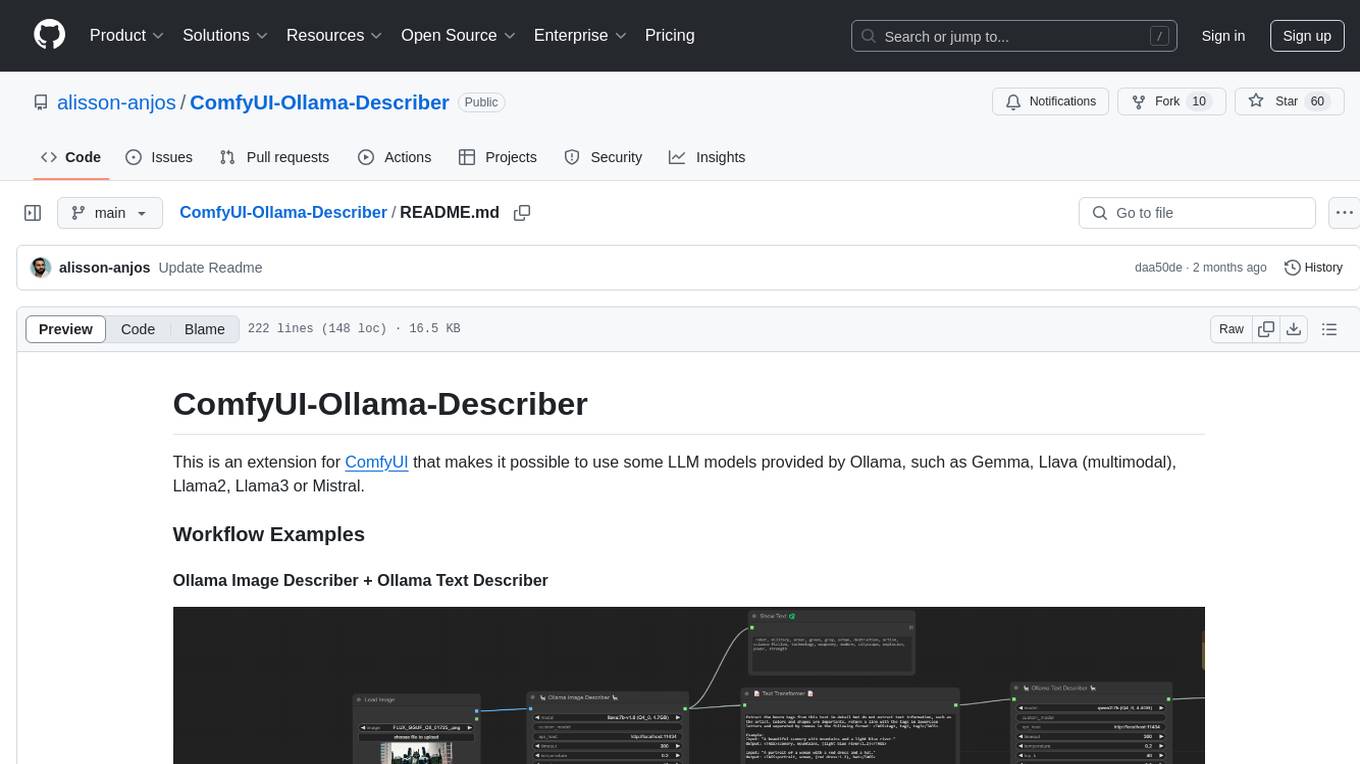
ComfyUI-Ollama-Describer
ComfyUI-Ollama-Describer is an extension for ComfyUI that enables the use of LLM models provided by Ollama, such as Gemma, Llava (multimodal), Llama2, Llama3, or Mistral. It requires the Ollama library for interacting with large-scale language models, supporting GPUs using CUDA and AMD GPUs on Windows, Linux, and Mac. The extension allows users to run Ollama through Docker and utilize NVIDIA GPUs for faster processing. It provides nodes for image description, text description, image captioning, and text transformation, with various customizable parameters for model selection, API communication, response generation, and model memory management.
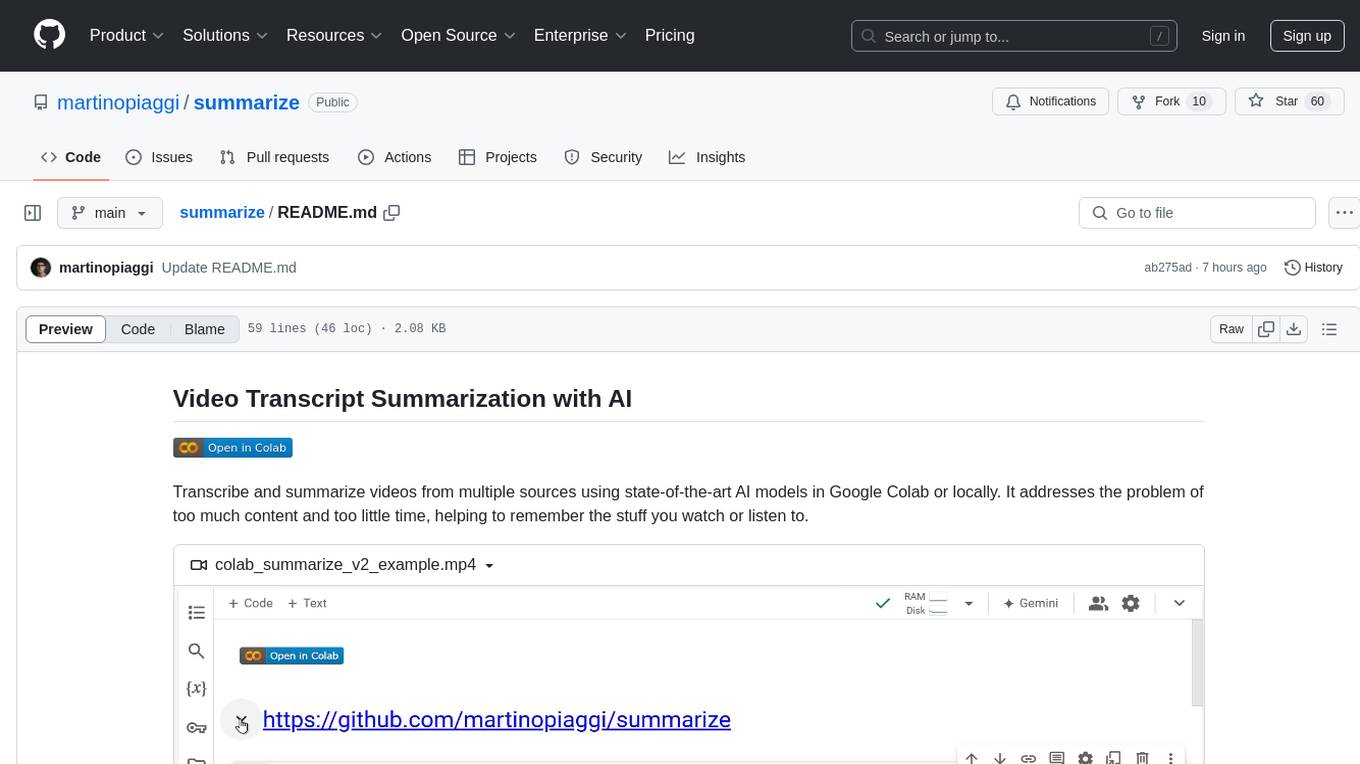
summarize
The 'summarize' tool is designed to transcribe and summarize videos from various sources using AI models. It helps users efficiently summarize lengthy videos, take notes, and extract key insights by providing timestamps, original transcripts, and support for auto-generated captions. Users can utilize different AI models via Groq, OpenAI, or custom local models to generate grammatically correct video transcripts and extract wisdom from video content. The tool simplifies the process of summarizing video content, making it easier to remember and reference important information.
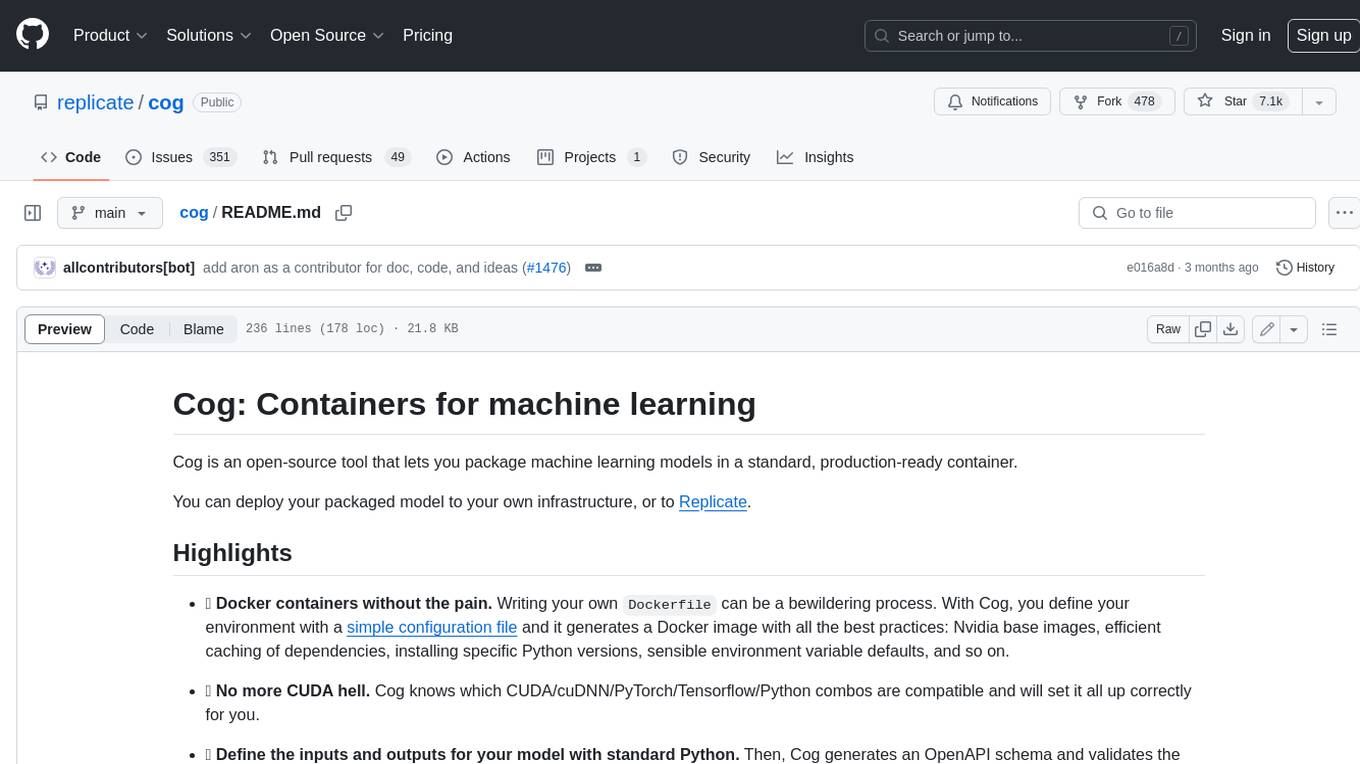
cog
Cog is an open-source tool that lets you package machine learning models in a standard, production-ready container. You can deploy your packaged model to your own infrastructure, or to Replicate.
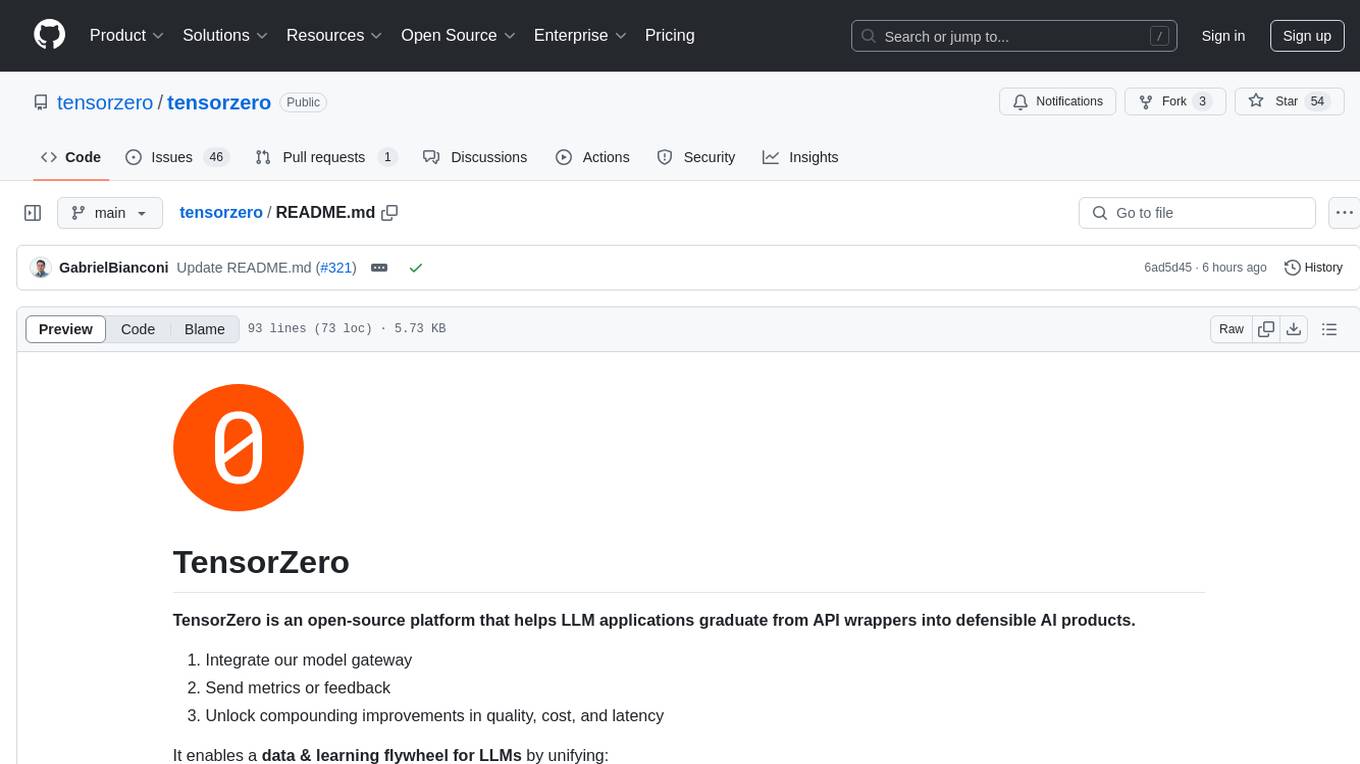
tensorzero
TensorZero is an open-source platform that helps LLM applications graduate from API wrappers into defensible AI products. It enables a data & learning flywheel for LLMs by unifying inference, observability, optimization, and experimentation. The platform includes a high-performance model gateway, structured schema-based inference, observability, experimentation, and data warehouse for analytics. TensorZero Recipes optimize prompts and models, and the platform supports experimentation features and GitOps orchestration for deployment.
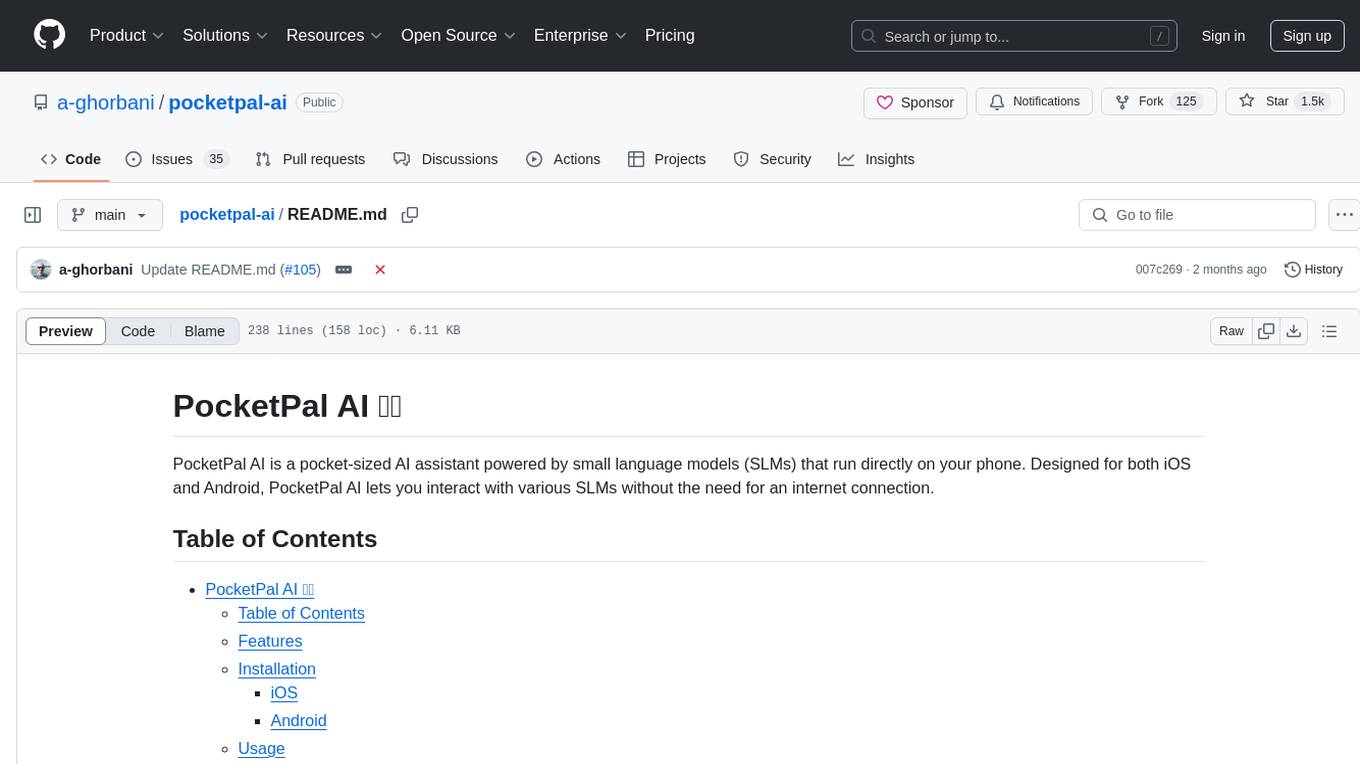
pocketpal-ai
PocketPal AI is a versatile virtual assistant tool designed to streamline daily tasks and enhance productivity. It leverages artificial intelligence technology to provide personalized assistance in managing schedules, organizing information, setting reminders, and more. With its intuitive interface and smart features, PocketPal AI aims to simplify users' lives by automating routine activities and offering proactive suggestions for optimal time management and task prioritization.
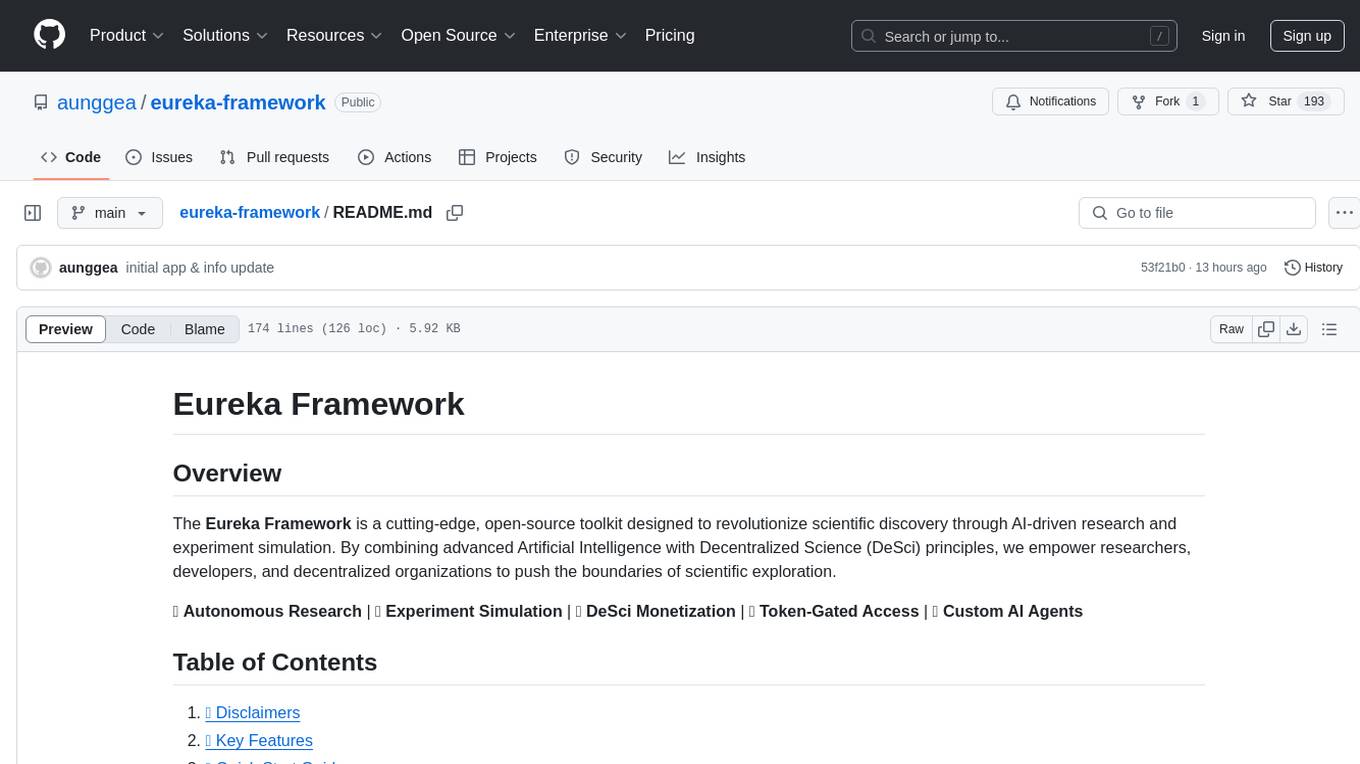
eureka-framework
The Eureka Framework is an open-source toolkit that leverages advanced Artificial Intelligence and Decentralized Science principles to revolutionize scientific discovery. It enables researchers, developers, and decentralized organizations to explore scientific papers, conduct AI-driven experiments, monetize research contributions, provide token-gated access to AI agents, and customize AI agents for specific research domains. The framework also offers features like a RESTful API, robust scheduler for task automation, and webhooks for real-time notifications, empowering users to automate research tasks, enhance productivity, and foster a committed research community.
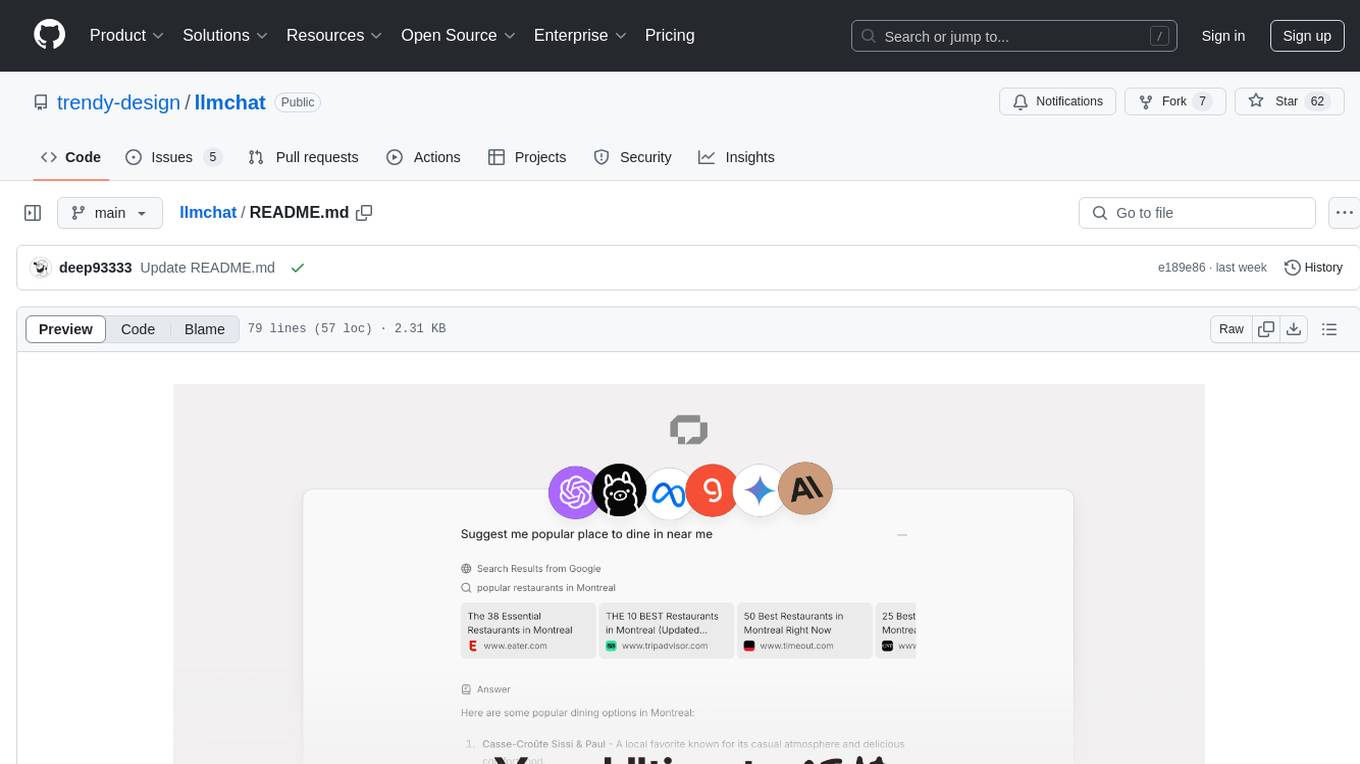
llmchat
LLMChat is an all-in-one AI chat interface that supports multiple language models, offers a plugin library for enhanced functionality, enables web search capabilities, allows customization of AI assistants, provides text-to-speech conversion, ensures secure local data storage, and facilitates data import/export. It also includes features like knowledge spaces, prompt library, personalization, and can be installed as a Progressive Web App (PWA). The tech stack includes Next.js, TypeScript, Pglite, LangChain, Zustand, React Query, Supabase, Tailwind CSS, Framer Motion, Shadcn, and Tiptap. The roadmap includes upcoming features like speech-to-text and knowledge spaces.
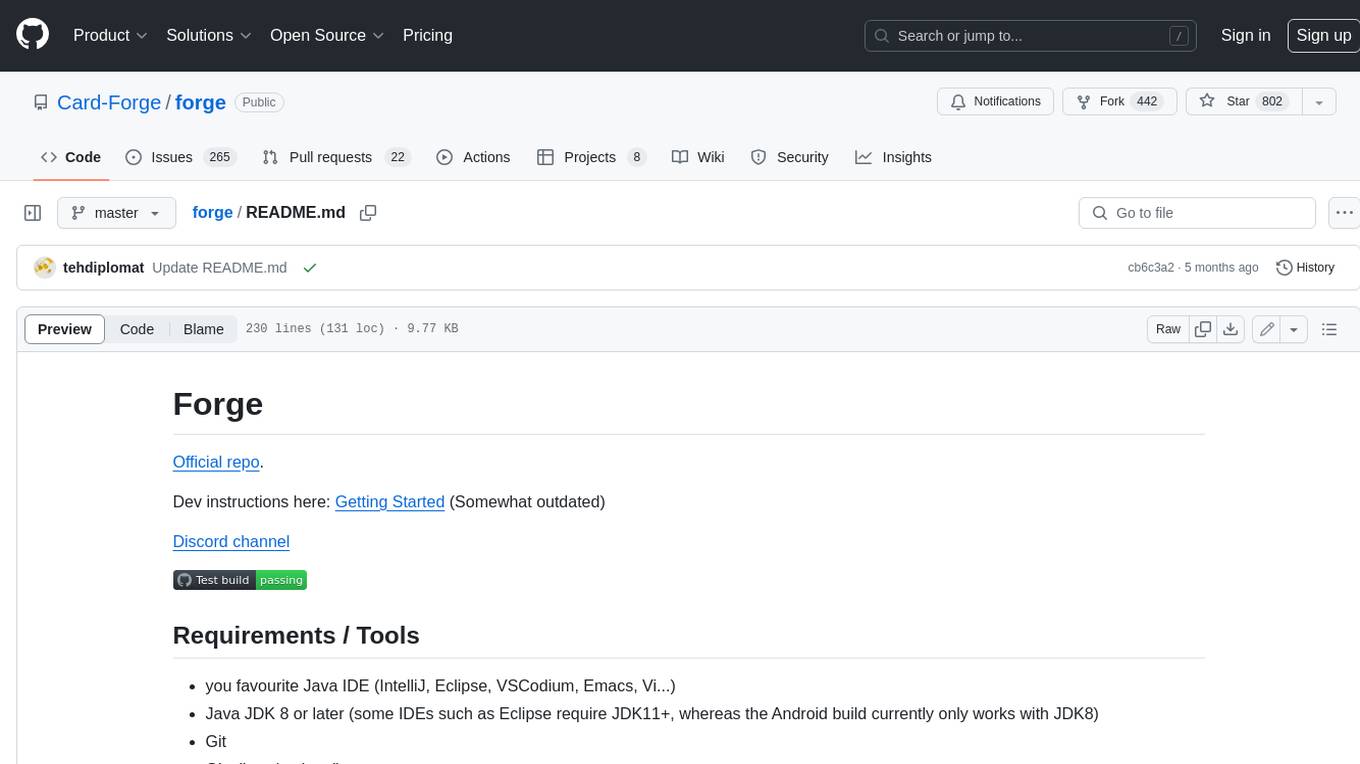
forge
Forge is a free and open-source digital collectible card game (CCG) engine written in Java. It is designed to be easy to use and extend, and it comes with a variety of features that make it a great choice for developers who want to create their own CCGs. Forge is used by a number of popular CCGs, including Ascension, Dominion, and Thunderstone.
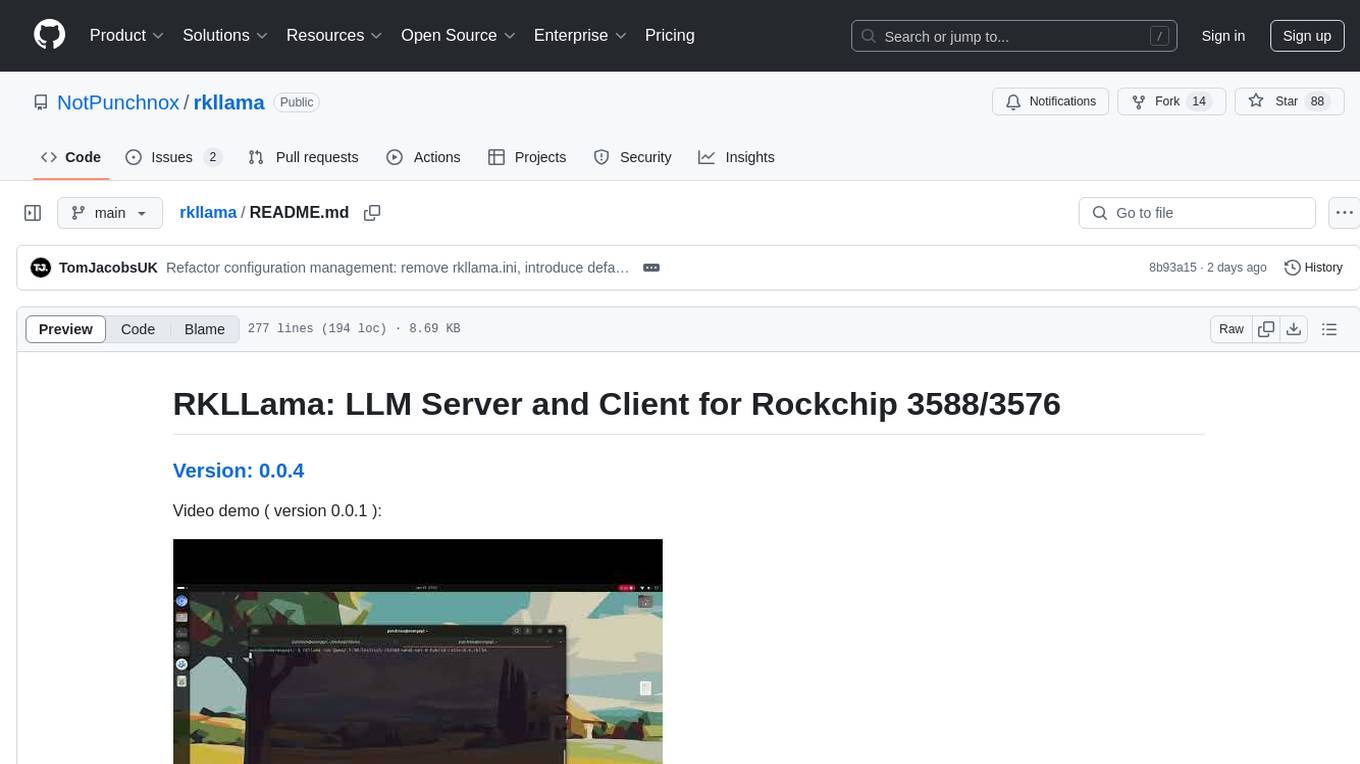
rkllama
RKLLama is a server and client tool designed for running and interacting with LLM models optimized for Rockchip RK3588(S) and RK3576 platforms. It allows models to run on the NPU, with features such as running models on NPU, partial Ollama API compatibility, pulling models from Huggingface, API REST with documentation, dynamic loading/unloading of models, inference requests with streaming modes, simplified model naming, CPU model auto-detection, and optional debug mode. The tool supports Python 3.8 to 3.12 and has been tested on Orange Pi 5 Pro and Orange Pi 5 Plus with specific OS versions.
For similar tasks
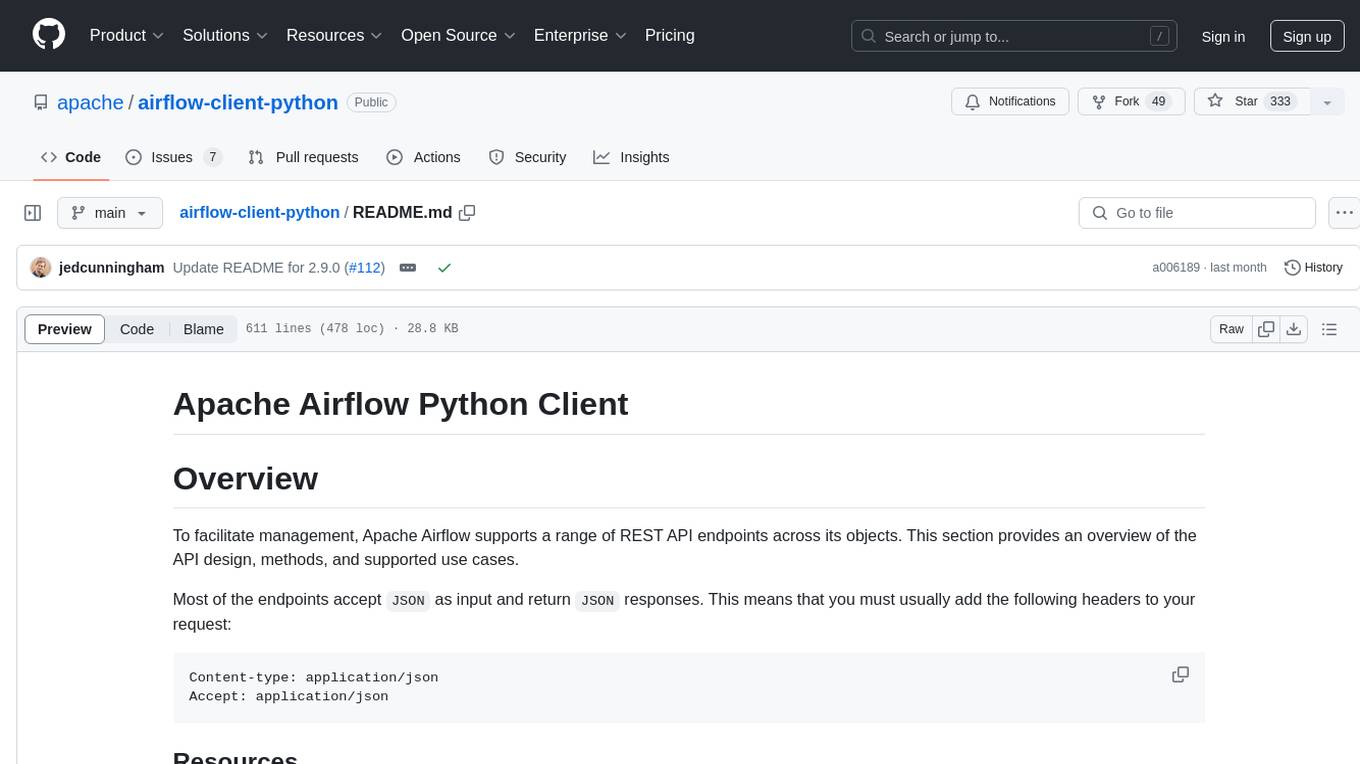
airflow-client-python
The Apache Airflow Python Client provides a range of REST API endpoints for managing Airflow metadata objects. It supports CRUD operations for resources, with endpoints accepting and returning JSON. Users can create, read, update, and delete resources. The API design follows conventions with consistent naming and field formats. Update mask is available for patch endpoints to specify fields for update. API versioning is not synchronized with Airflow releases, and changes go through a deprecation phase. The tool supports various authentication methods and error responses follow RFC 7807 format.
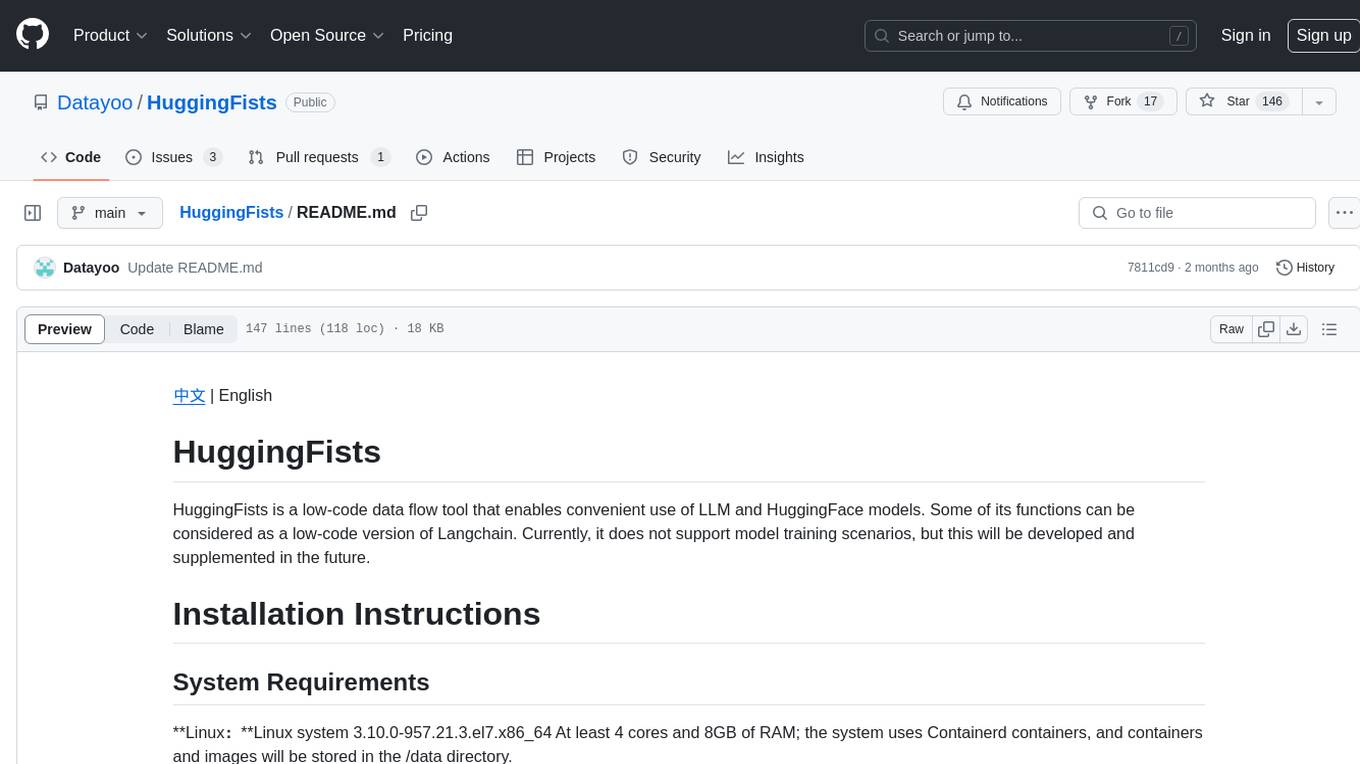
HuggingFists
HuggingFists is a low-code data flow tool that enables convenient use of LLM and HuggingFace models. It provides functionalities similar to Langchain, allowing users to design, debug, and manage data processing workflows, create and schedule workflow jobs, manage resources environment, and handle various data artifact resources. The tool also offers account management for users, allowing centralized management of data source accounts and API accounts. Users can access Hugging Face models through the Inference API or locally deployed models, as well as datasets on Hugging Face. HuggingFists supports breakpoint debugging, branch selection, function calls, workflow variables, and more to assist users in developing complex data processing workflows.
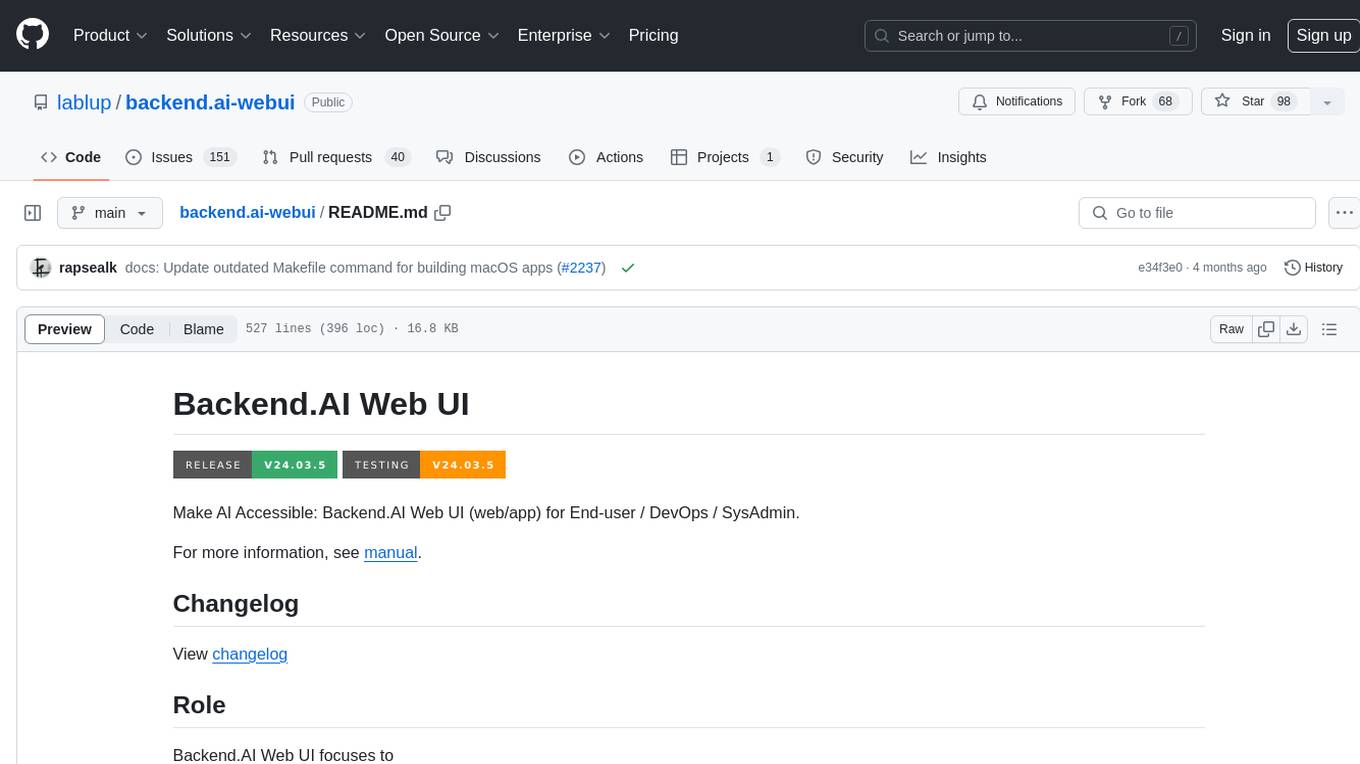
backend.ai-webui
Backend.AI Web UI is a user-friendly web and app interface designed to make AI accessible for end-users, DevOps, and SysAdmins. It provides features for session management, inference service management, pipeline management, storage management, node management, statistics, configurations, license checking, plugins, help & manuals, kernel management, user management, keypair management, manager settings, proxy mode support, service information, and integration with the Backend.AI Web Server. The tool supports various devices, offers a built-in websocket proxy feature, and allows for versatile usage across different platforms. Users can easily manage resources, run environment-supported apps, access a web-based terminal, use Visual Studio Code editor, manage experiments, set up autoscaling, manage pipelines, handle storage, monitor nodes, view statistics, configure settings, and more.
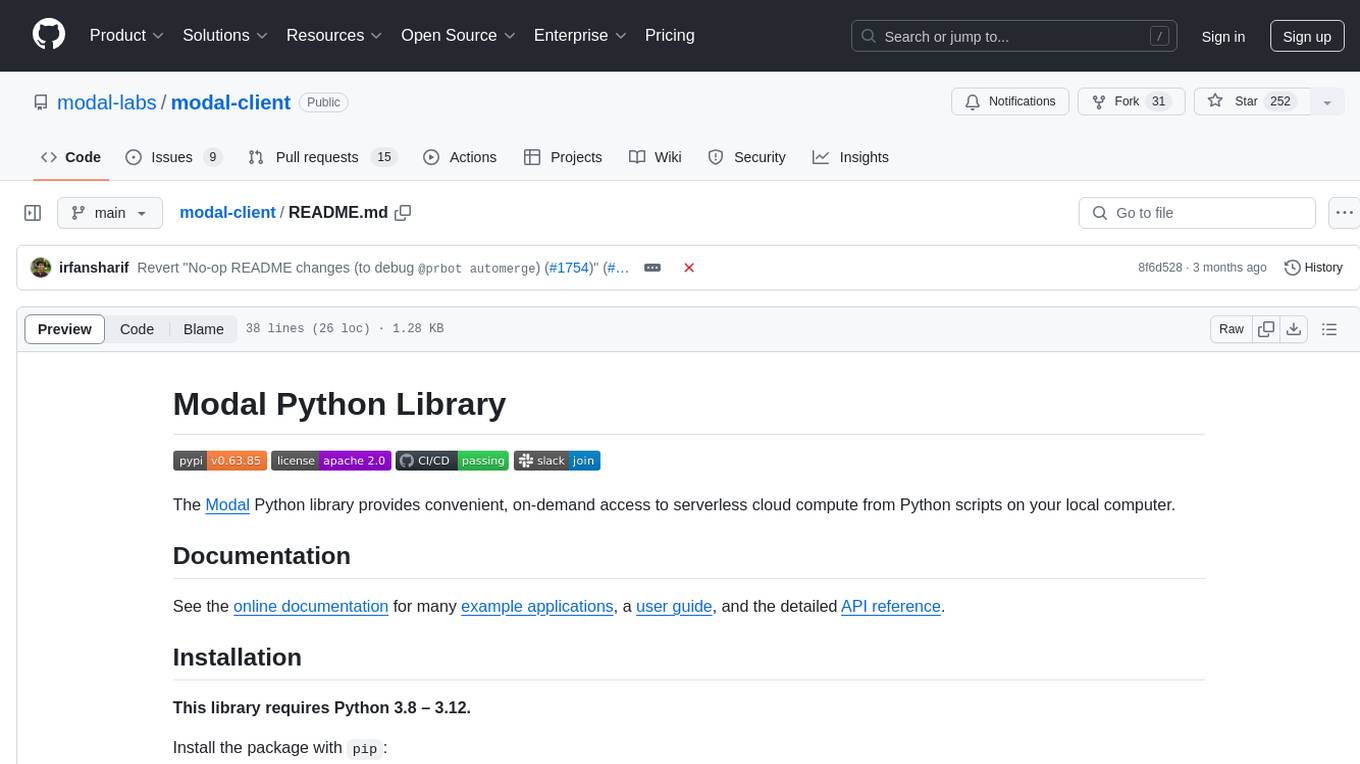
modal-client
The Modal Python library provides convenient, on-demand access to serverless cloud compute from Python scripts on your local computer. It allows users to easily integrate serverless cloud computing into their Python scripts, providing a seamless experience for accessing cloud resources. The library simplifies the process of interacting with cloud services, enabling developers to focus on their applications' logic rather than infrastructure management. With detailed documentation and support available through the Modal Slack channel, users can quickly get started and leverage the power of serverless computing in their projects.
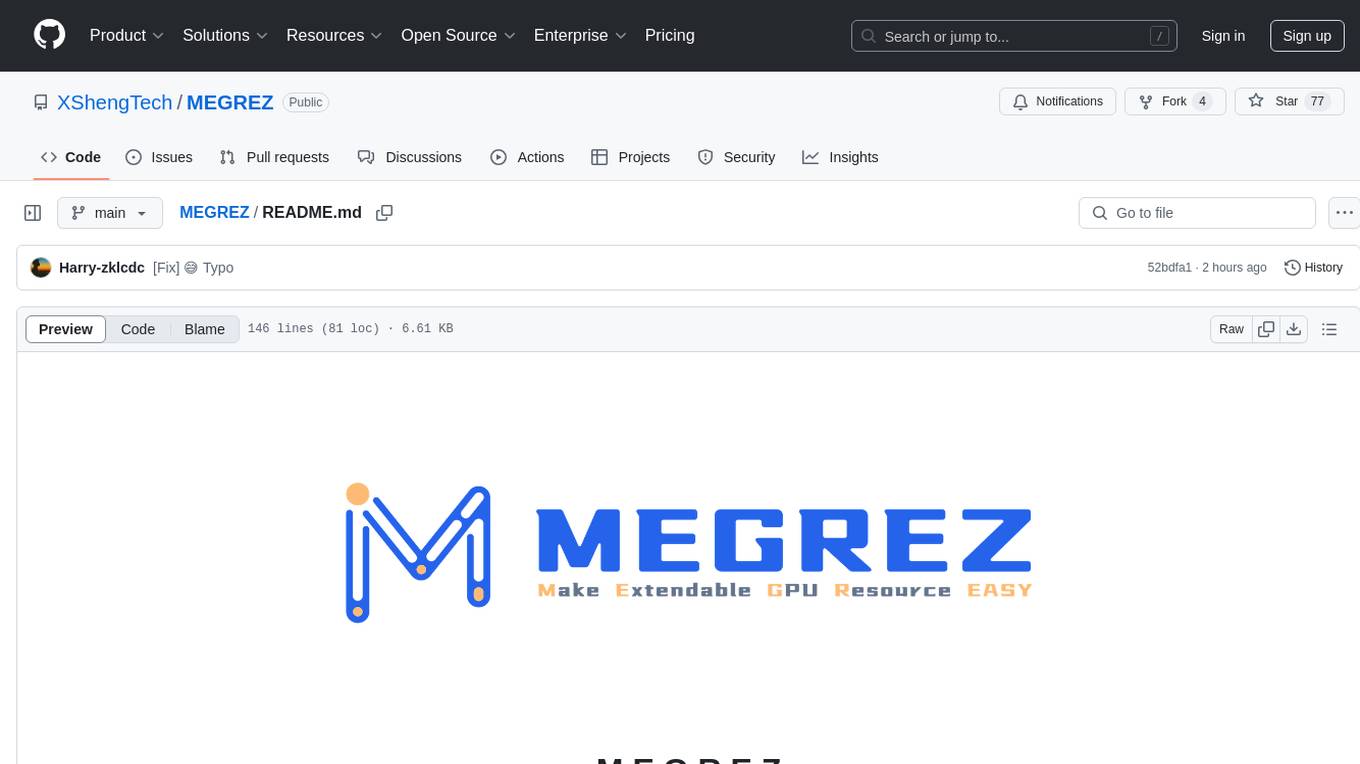
MEGREZ
MEGREZ is a modern and elegant open-source high-performance computing platform that efficiently manages GPU resources. It allows for easy container instance creation, supports multiple nodes/multiple GPUs, modern UI environment isolation, customizable performance configurations, and user data isolation. The platform also comes with pre-installed deep learning environments, supports multiple users, features a VSCode web version, resource performance monitoring dashboard, and Jupyter Notebook support.
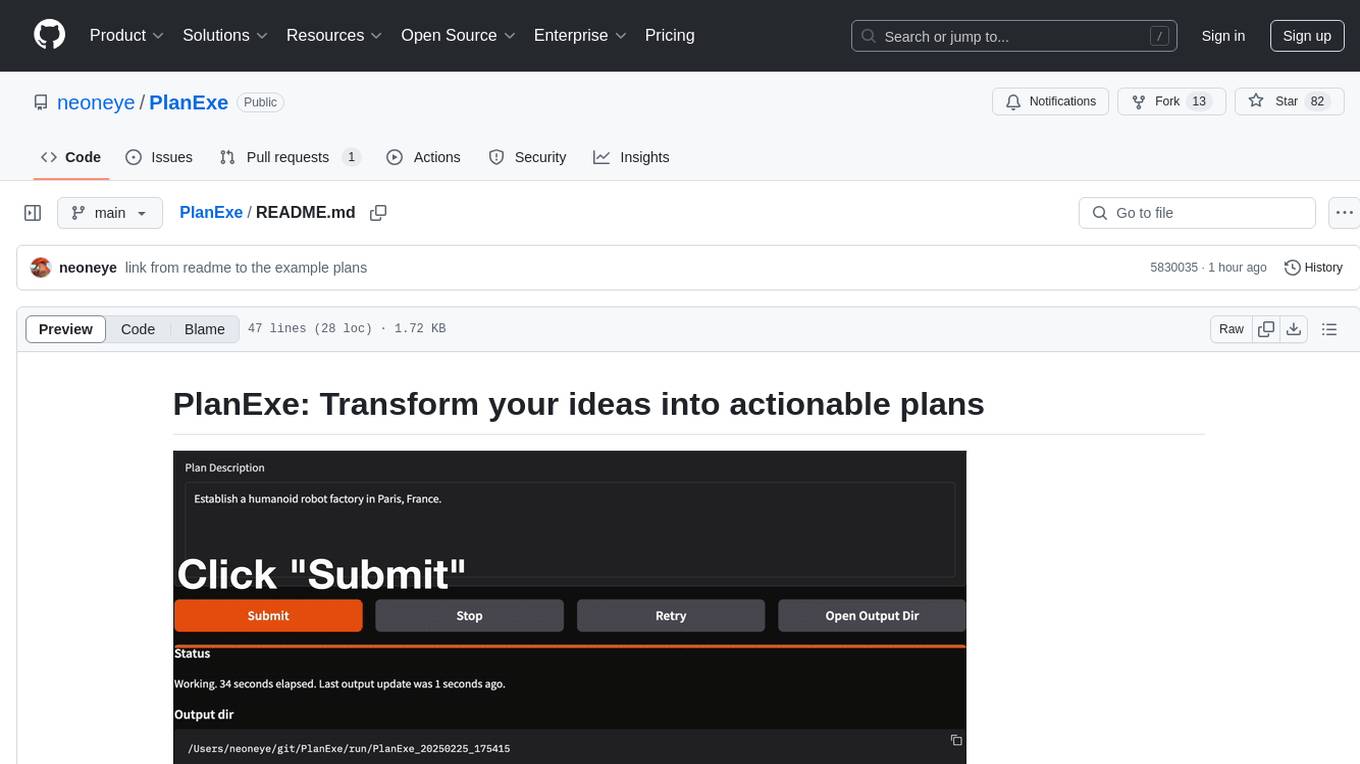
PlanExe
PlanExe is a planning AI tool that helps users generate detailed plans based on vague descriptions. It offers a Gradio-based web interface for easy input and output. Users can choose between running models in the cloud or locally on a high-end computer. The tool aims to provide a straightforward path to planning various tasks efficiently.
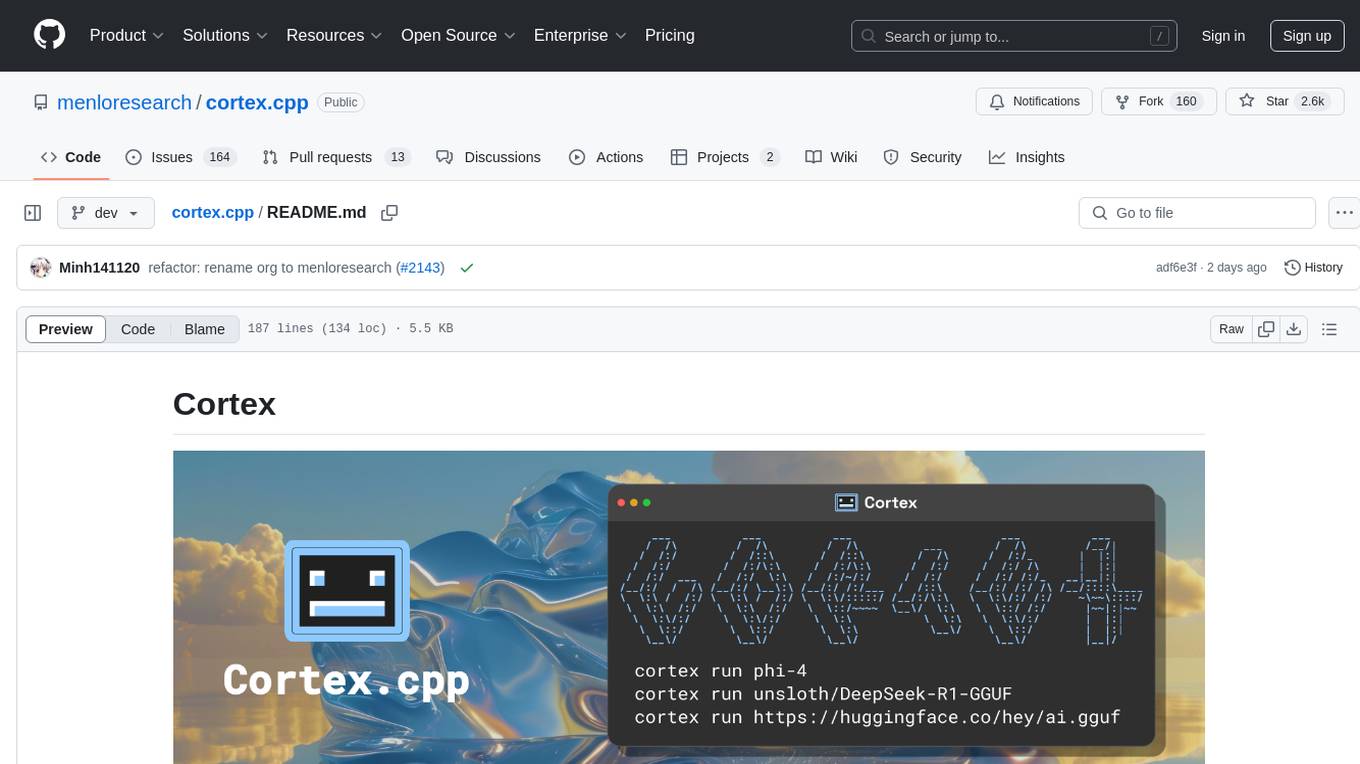
cortex.cpp
Cortex.cpp is an open-source platform designed as the brain for robots, offering functionalities such as vision, speech, language, tabular data processing, and action. It provides an AI platform for running AI models with multi-engine support, hardware optimization with automatic GPU detection, and an OpenAI-compatible API. Users can download models from the Hugging Face model hub, run models, manage resources, and access advanced features like multiple quantizations and engine management. The tool is under active development, promising rapid improvements for users.
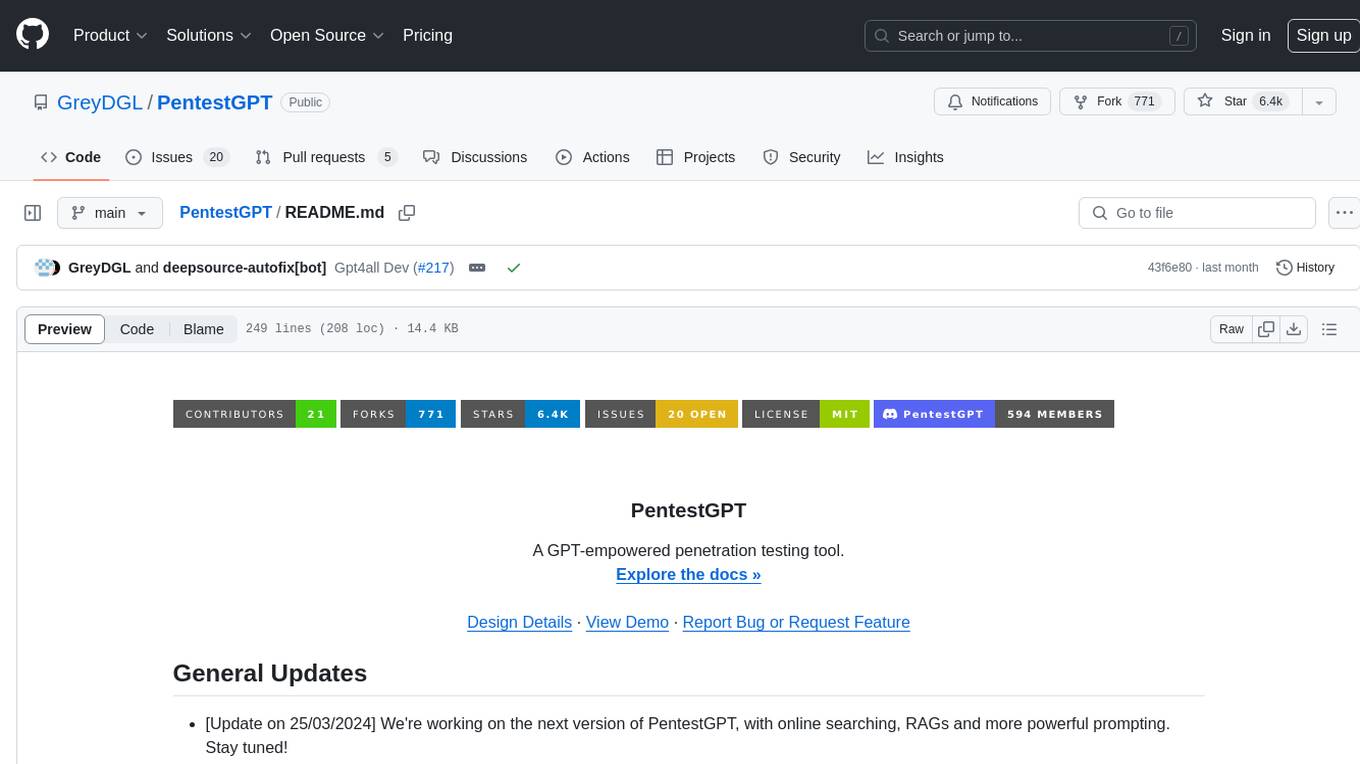
PentestGPT
PentestGPT is a penetration testing tool empowered by ChatGPT, designed to automate the penetration testing process. It operates interactively to guide penetration testers in overall progress and specific operations. The tool supports solving easy to medium HackTheBox machines and other CTF challenges. Users can use PentestGPT to perform tasks like testing connections, using different reasoning models, discussing with the tool, searching on Google, and generating reports. It also supports local LLMs with custom parsers for advanced users.
For similar jobs
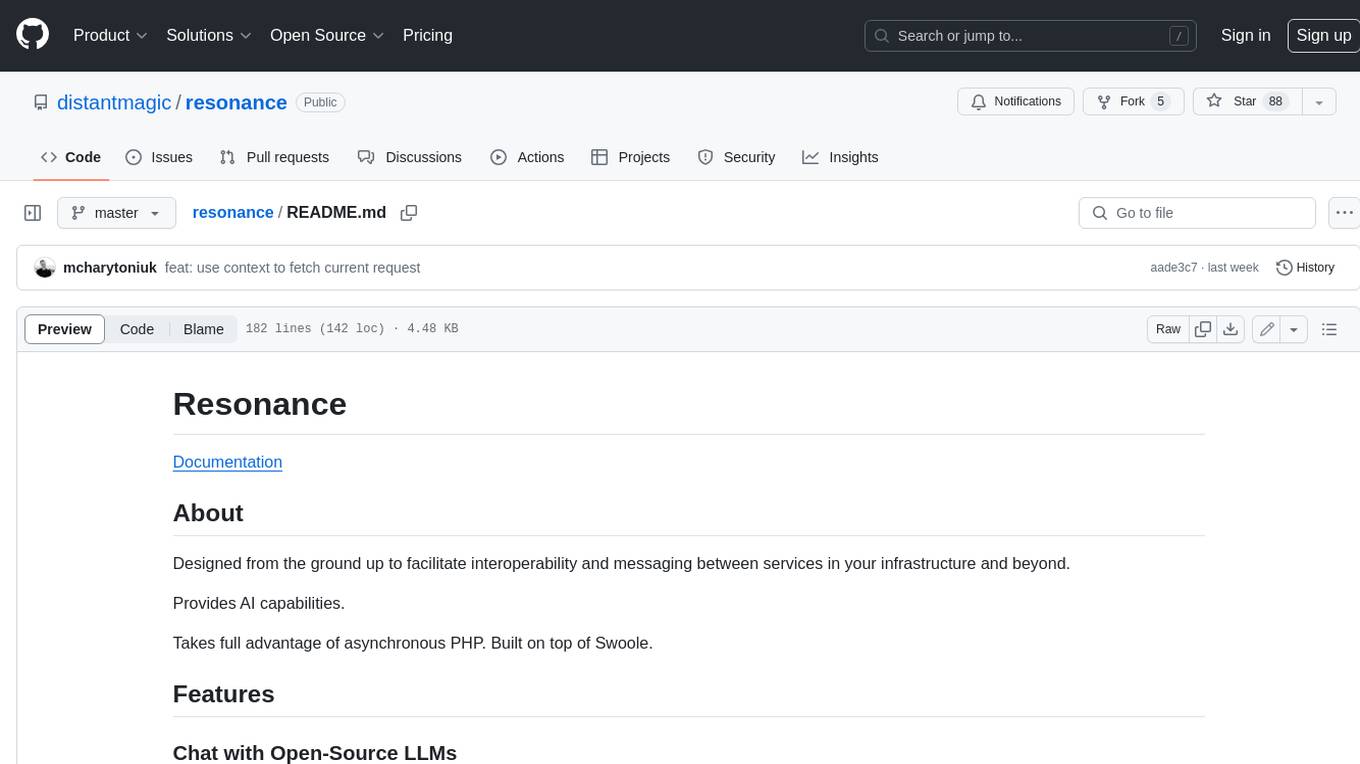
resonance
Resonance is a framework designed to facilitate interoperability and messaging between services in your infrastructure and beyond. It provides AI capabilities and takes full advantage of asynchronous PHP, built on top of Swoole. With Resonance, you can: * Chat with Open-Source LLMs: Create prompt controllers to directly answer user's prompts. LLM takes care of determining user's intention, so you can focus on taking appropriate action. * Asynchronous Where it Matters: Respond asynchronously to incoming RPC or WebSocket messages (or both combined) with little overhead. You can set up all the asynchronous features using attributes. No elaborate configuration is needed. * Simple Things Remain Simple: Writing HTTP controllers is similar to how it's done in the synchronous code. Controllers have new exciting features that take advantage of the asynchronous environment. * Consistency is Key: You can keep the same approach to writing software no matter the size of your project. There are no growing central configuration files or service dependencies registries. Every relation between code modules is local to those modules. * Promises in PHP: Resonance provides a partial implementation of Promise/A+ spec to handle various asynchronous tasks. * GraphQL Out of the Box: You can build elaborate GraphQL schemas by using just the PHP attributes. Resonance takes care of reusing SQL queries and optimizing the resources' usage. All fields can be resolved asynchronously.
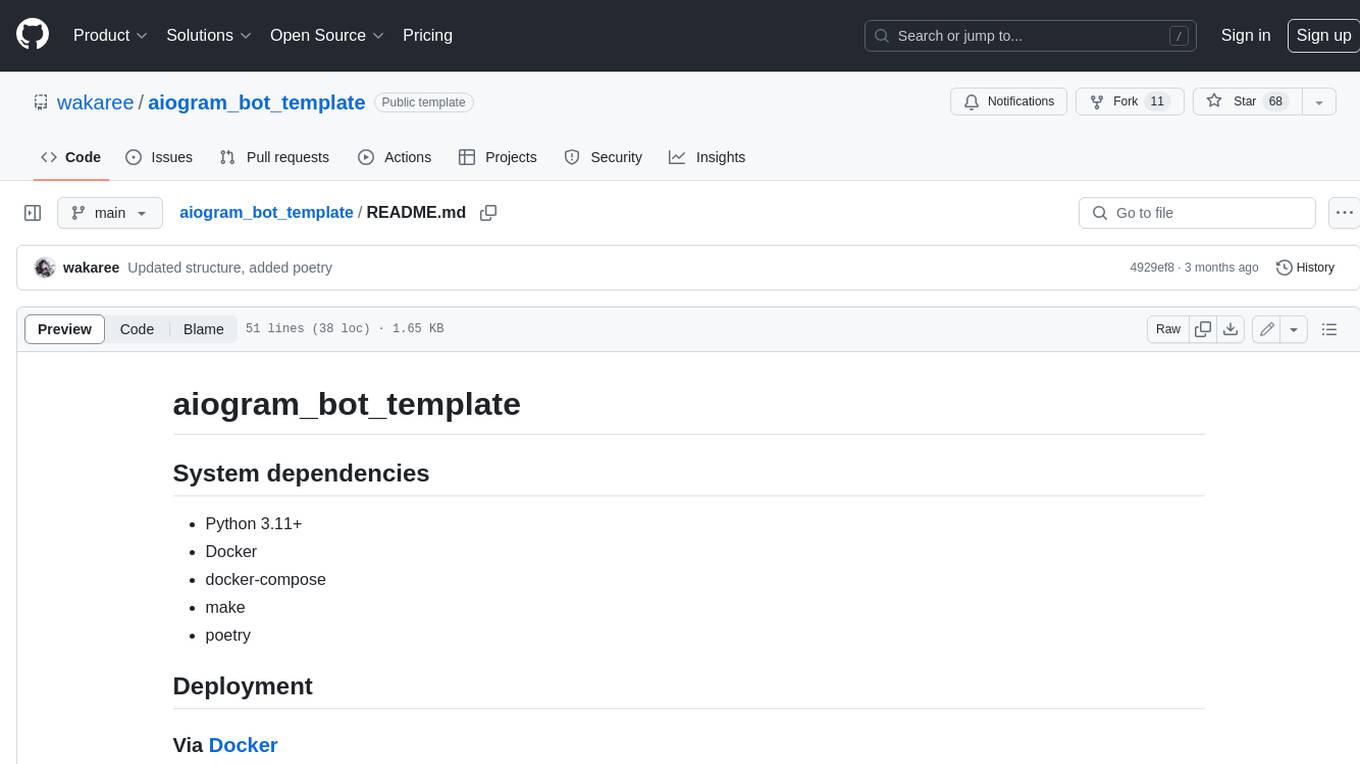
aiogram_bot_template
Aiogram bot template is a boilerplate for creating Telegram bots using Aiogram framework. It provides a solid foundation for building robust and scalable bots with a focus on code organization, database integration, and localization.
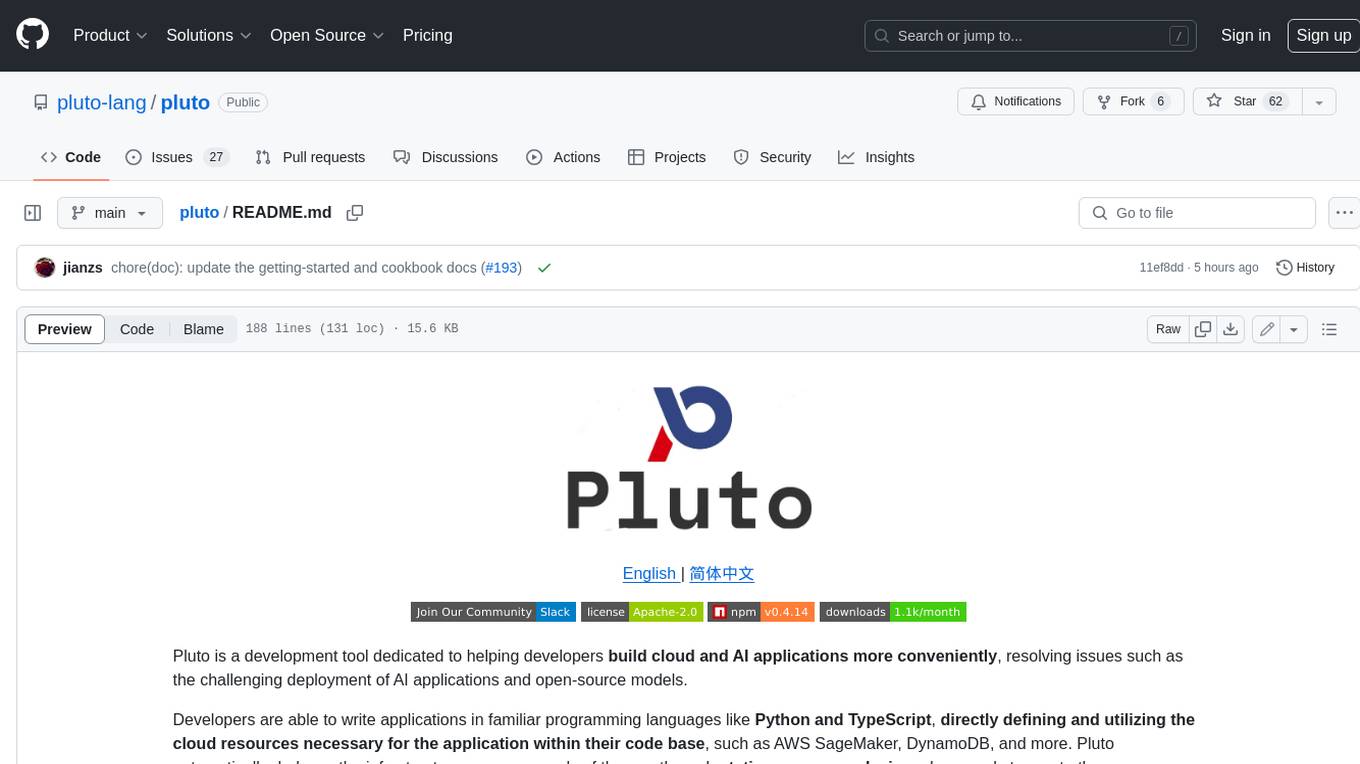
pluto
Pluto is a development tool dedicated to helping developers **build cloud and AI applications more conveniently** , resolving issues such as the challenging deployment of AI applications and open-source models. Developers are able to write applications in familiar programming languages like **Python and TypeScript** , **directly defining and utilizing the cloud resources necessary for the application within their code base** , such as AWS SageMaker, DynamoDB, and more. Pluto automatically deduces the infrastructure resource needs of the app through **static program analysis** and proceeds to create these resources on the specified cloud platform, **simplifying the resources creation and application deployment process**.
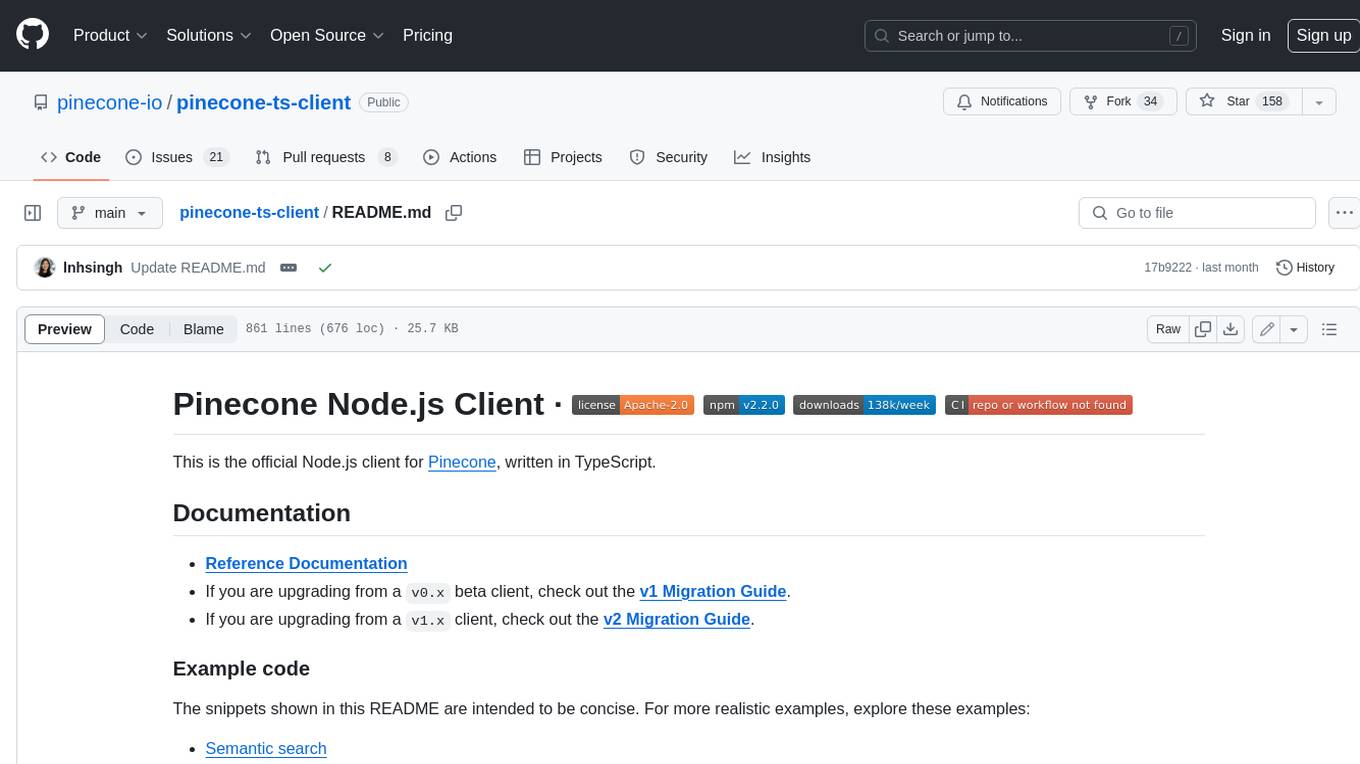
pinecone-ts-client
The official Node.js client for Pinecone, written in TypeScript. This client library provides a high-level interface for interacting with the Pinecone vector database service. With this client, you can create and manage indexes, upsert and query vector data, and perform other operations related to vector search and retrieval. The client is designed to be easy to use and provides a consistent and idiomatic experience for Node.js developers. It supports all the features and functionality of the Pinecone API, making it a comprehensive solution for building vector-powered applications in Node.js.
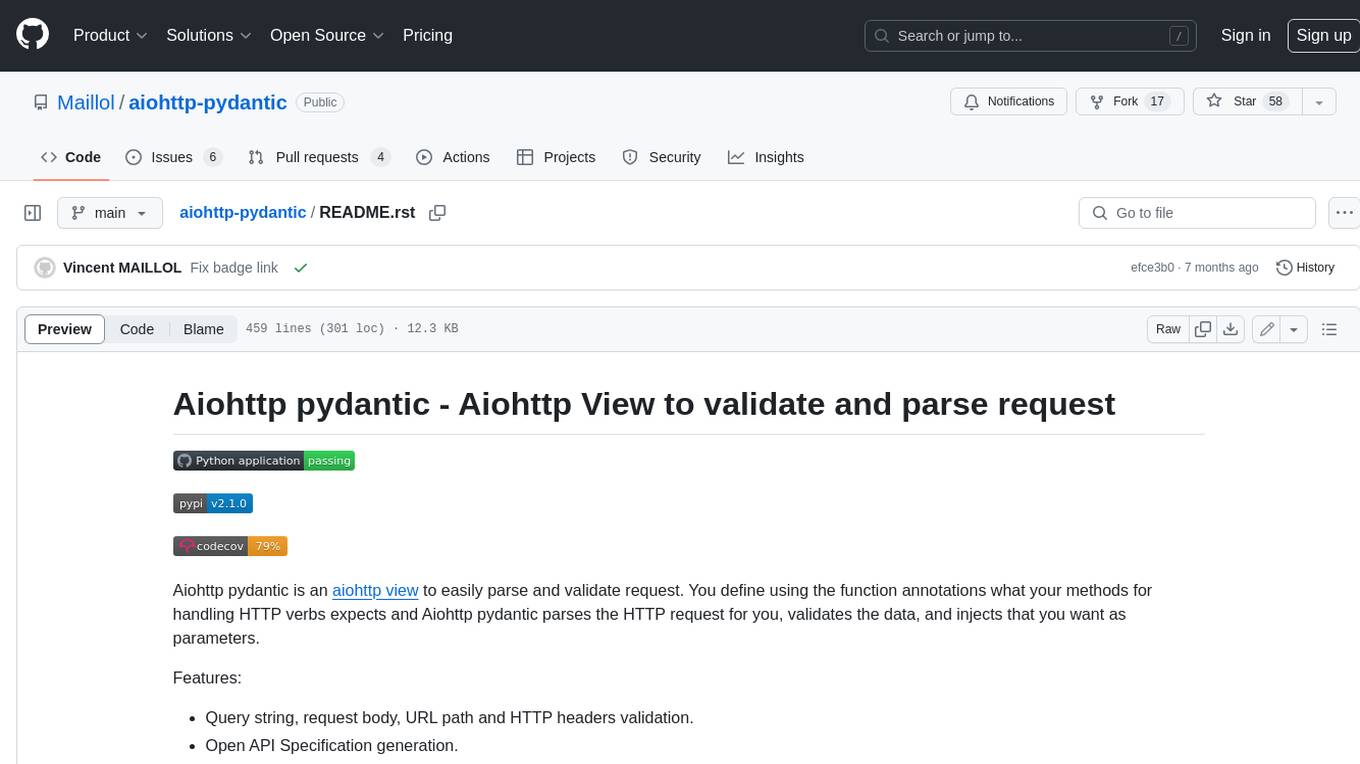
aiohttp-pydantic
Aiohttp pydantic is an aiohttp view to easily parse and validate requests. You define using function annotations what your methods for handling HTTP verbs expect, and Aiohttp pydantic parses the HTTP request for you, validates the data, and injects the parameters you want. It provides features like query string, request body, URL path, and HTTP headers validation, as well as Open API Specification generation.
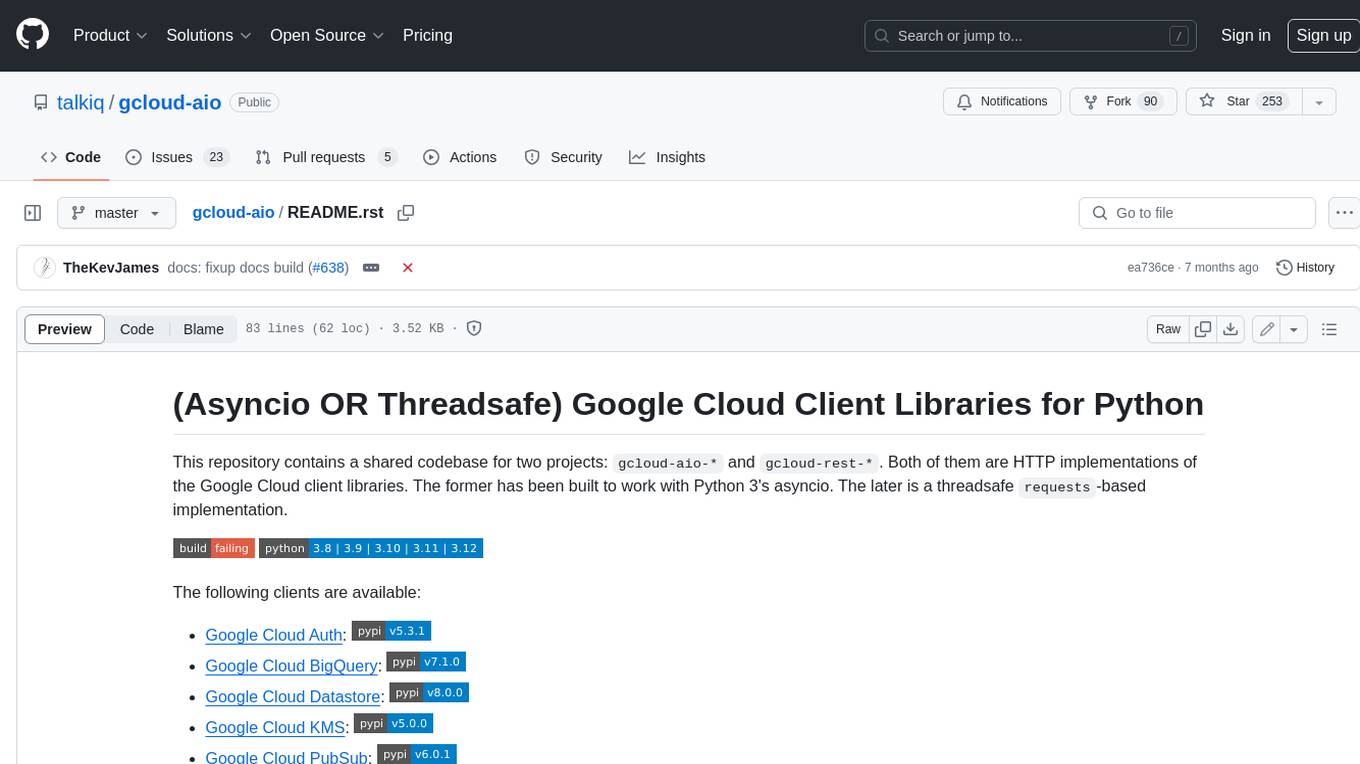
gcloud-aio
This repository contains shared codebase for two projects: gcloud-aio and gcloud-rest. gcloud-aio is built for Python 3's asyncio, while gcloud-rest is a threadsafe requests-based implementation. It provides clients for Google Cloud services like Auth, BigQuery, Datastore, KMS, PubSub, Storage, and Task Queue. Users can install the library using pip and refer to the documentation for usage details. Developers can contribute to the project by following the contribution guide.
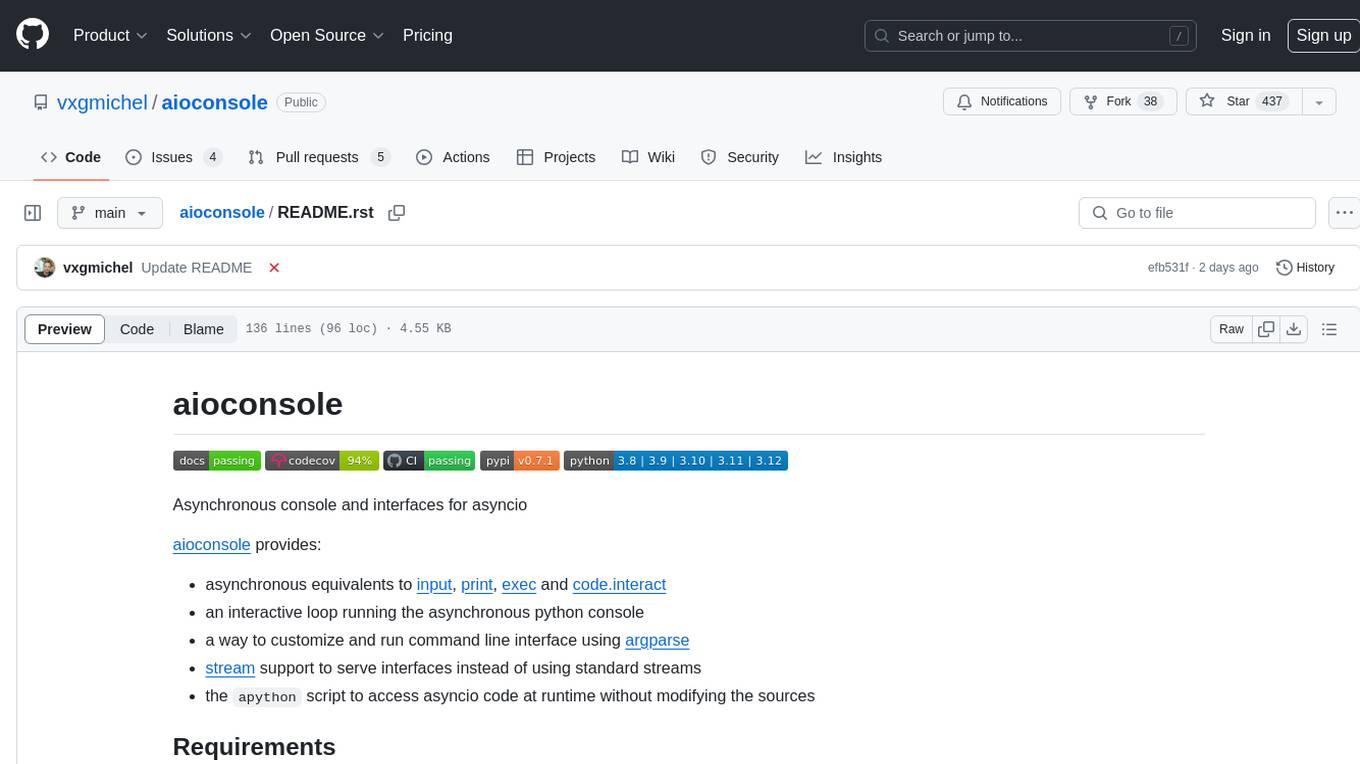
aioconsole
aioconsole is a Python package that provides asynchronous console and interfaces for asyncio. It offers asynchronous equivalents to input, print, exec, and code.interact, an interactive loop running the asynchronous Python console, customization and running of command line interfaces using argparse, stream support to serve interfaces instead of using standard streams, and the apython script to access asyncio code at runtime without modifying the sources. The package requires Python version 3.8 or higher and can be installed from PyPI or GitHub. It allows users to run Python files or modules with a modified asyncio policy, replacing the default event loop with an interactive loop. aioconsole is useful for scenarios where users need to interact with asyncio code in a console environment.
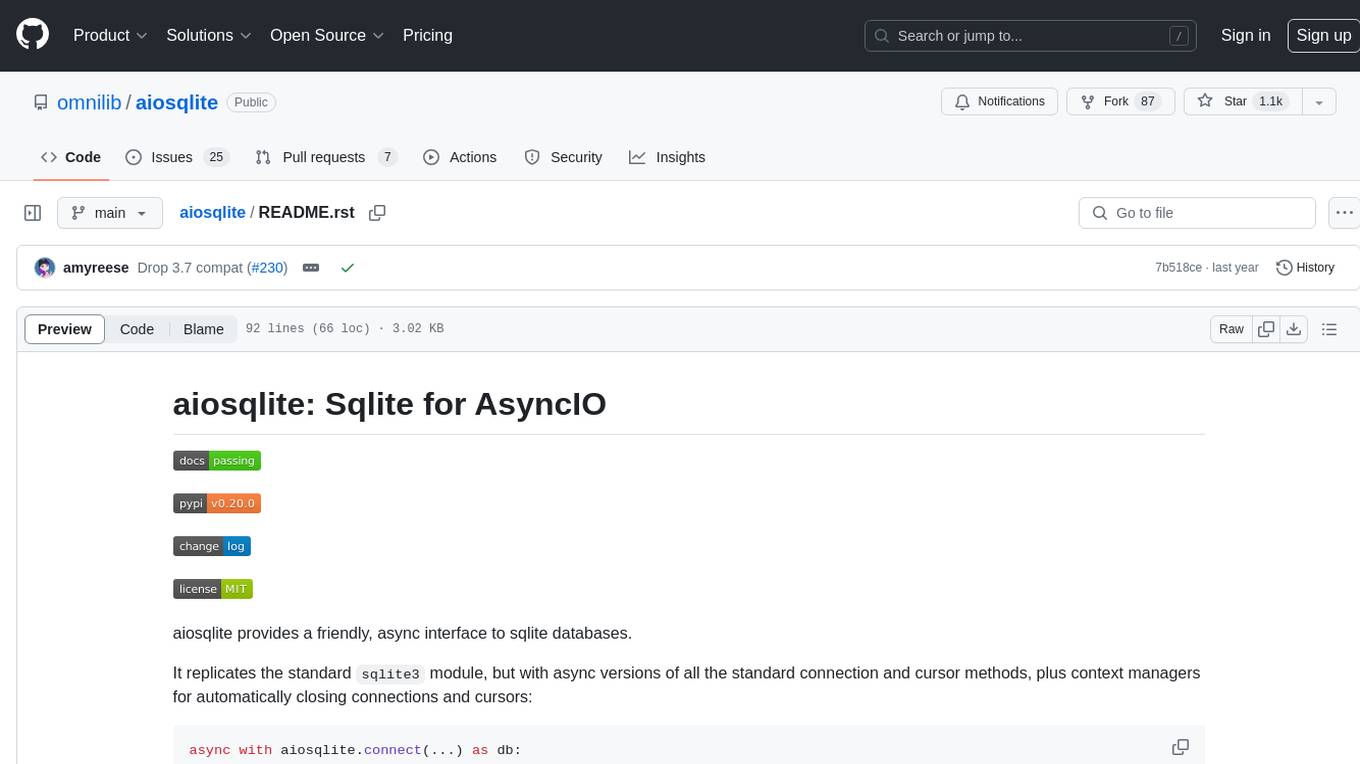
aiosqlite
aiosqlite is a Python library that provides a friendly, async interface to SQLite databases. It replicates the standard sqlite3 module but with async versions of all the standard connection and cursor methods, along with context managers for automatically closing connections and cursors. It allows interaction with SQLite databases on the main AsyncIO event loop without blocking execution of other coroutines while waiting for queries or data fetches. The library also replicates most of the advanced features of sqlite3, such as row factories and total changes tracking.