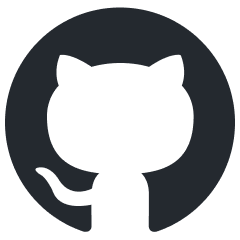
LLM4DB
None
Stars: 89
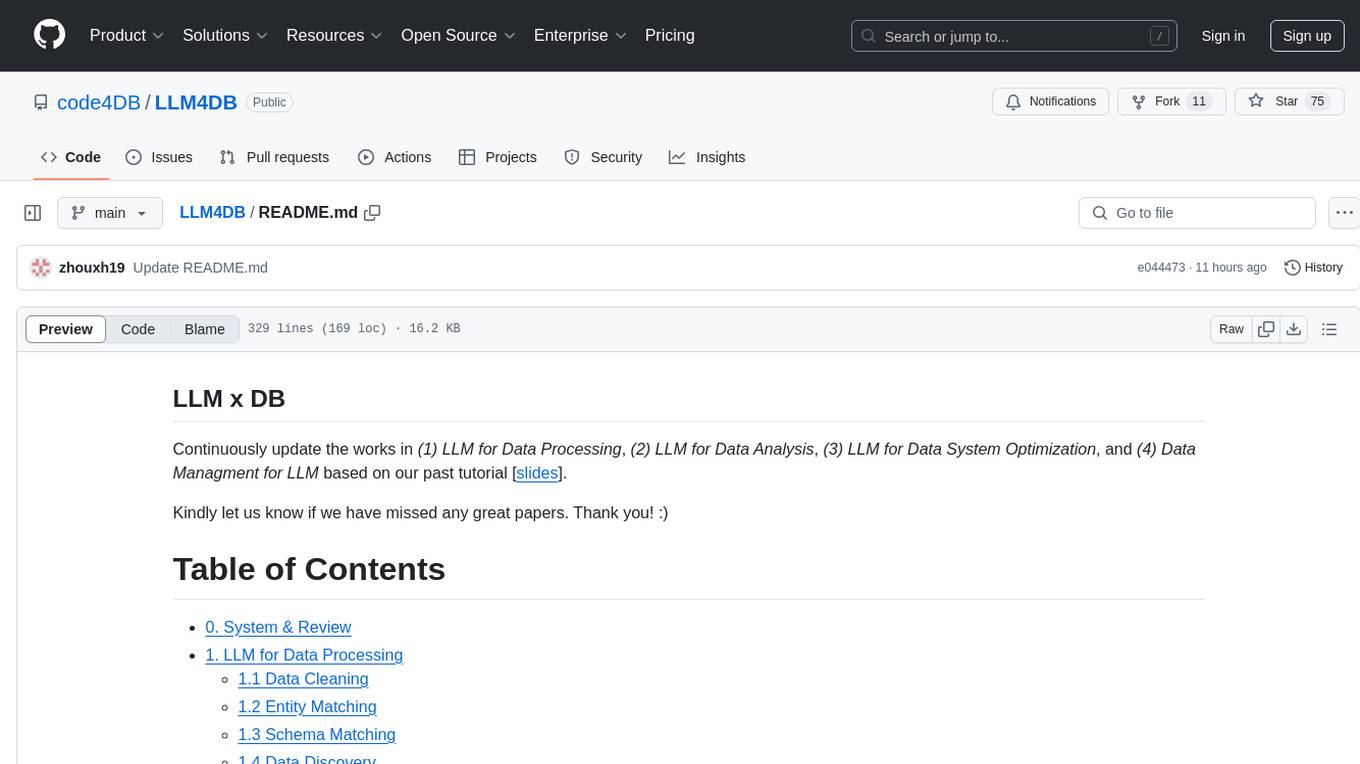
LLM4DB is a repository focused on the intersection of Large Language Models (LLMs) and Database technologies. It covers various aspects such as data processing, data analysis, database optimization, and data management for LLMs. The repository includes research papers, tools, and techniques related to leveraging LLMs for tasks like data cleaning, entity matching, schema matching, data discovery, NL2SQL, data exploration, data visualization, knob tuning, query optimization, and database diagnosis.
README:
Continuously update the works in (1) LLM for Data Processing, (2) LLM for Data Analysis, (3) LLM for Data System Optimization, and (4) Data Managment for LLM based on our past tutorial [slides].
Kindly let us know if we have missed any great papers. Thank you! :)
- 0. System & Review
- 1. LLM for Data Processing
- 2. LLM for Data Analysis
- 3. LLM for Database Optimization
- 4. Data Management for LLM
NeurDB: On the Design and Implementation of an AI-powered Autonomous Database
*hanhao Zhao, Shaofeng Cai, Haotian Gao, Hexiang Pan, Siqi Xiang, Naili Xing, Gang Chen, Beng Chin Ooi, Yanyan Shen, Yuncheng Wu, Meihui Zhang. CIDR 2025. [pdf]
How Large Language Models Will Disrupt Data Management
Raul Castro Fernandez, Aaron J. Elmore, Michael J. Franklin, Sanjay Krishnan, Chenhao Tan. VLDB 2023. [pdf]
From Large Language Models to Databases and Back: A Discussion on Research and Education
Sihem Amer-Yahia, Angela Bonifati, Lei Chen, Guoliang Li, Kyuseok Shim, Jianliang Xu, Xiaochun Yang. SIGMOD Record. [pdf]
Applications and Challenges for Large Language Models: From Data Management Perspective.
Zhang, Meihui, Zhaoxuan Ji, Zhaojing Luo, Yuncheng Wu, and Chengliang Chai. ICDE 2024. [pdf]
Demystifying Data Management for Large Language Models
Xupeng Miao, Zhihao Jia, and Bin Cui. SIGMOD 2024. [pdf]
DB-GPT: Large Language Model Meets Database
Xuanhe Zhou, Zhaoyan Sun, Guoliang Li. Data Science and Engineering 2023. [pdf]
LLM-Enhanced Data Management
Xuanhe Zhou, Xinyang Zhao, Guoliang Li. arxiv 2024. [pdf]
Trustworthy and Efficient LLMs Meet Databases
Kyoungmin Kim, Anastasia Ailamaki. arxiv 2024. [pdf]
Can Foundation Models Wrangle Your Data?
Avanika Narayan, Ines Chami, Laurel J. Orr, Christopher Ré. VLDB 2022. [pdf]
Data Management For Training Large Language Models: A Survey
Zige Wang, Wanjun Zhong, Yufei Wang, Qi Zhu, Fei Mi, Baojun Wang, Lifeng Shang, Xin Jiang, Qun Liu. arxiv 2024. [pdf]
When Large Language Models Meet Vector Databases: A Survey
Zhi Jing, Yongye Su, Yikun Han, Bo Yuan, Haiyun Xu, Chunjiang Liu, Kehai Chen, Min Zhang. arxiv 2024. [pdf]
From BERT to GPT-3 Codex: Harnessing the Potential of Very Large Language Models for Data Management
Immanuel Trummer. VLDB 2023. [pdf]
Survey of Vector Database Management Systems
James Jie Pan, Jianguo Wang, Guoliang Li. arxiv 2023. [pdf]
There are many related works, with a focus on prioritizing papers within the database field.
GIDCL: A Graph-Enhanced Interpretable Data Cleaning Framework with Large Language Models
Mengyi Yan, Yaoshu Wang, Yue Wang, Xiaoye Miao, Jianxin Li. SIGMOD 2025. [pdf]
Mind the Data Gap: Bridging LLMs to Enterprise Data Integration
Moe Kayali, Fabian Wenz, Nesime Tatbul, Çağatay Demiralp. CIDR 2025. [pdf]
AutoDCWorkflow: LLM-based Data Cleaning Workflow Auto-Generation and Benchmark
Lan Li, Liri Fang, Vetle I. Torvik. arxiv 2024. [pdf]
Jellyfish: A Large Language Model for Data Preprocessing
Haochen Zhang, Yuyang Dong, Chuan Xiao, Masafumi Oyamada. arxiv 2024. [pdf]
CleanAgent: Automating Data Standardization with LLM-based Agents
Danrui Qi, Jiannan Wang. arxiv 2024. [pdf]
LLMClean: Context-Aware Tabular Data Cleaning via LLM-Generated OFDs
Fabian Biester, Mohamed Abdelaal, Daniel Del Gaudio. arxiv 2024. [pdf]
LLMs with User-defined Prompts as Generic Data Operators for Reliable Data Processing
Luyi Ma, Nikhil Thakurdesai, Jiao Chen, Jianpeng Xu, Evren Körpeoglu, Sushant Kumar, Kannan Achan. IEEE Big Data 2023. [pdf]
SEED: Domain-Specific Data Curation With Large Language Models
Zui Chen, Lei Cao, Sam Madden, Tim Kraska, Zeyuan Shang, Ju Fan, Nan Tang, Zihui Gu, Chunwei Liu, Michael Cafarella. arxiv 2023. [pdf]
Large Language Models as Data Preprocessors
Haochen Zhang, Yuyang Dong, Chuan Xiao, Masafumi Oyamada. arxiv 2023. [pdf]
Data Cleaning Using Large Language Models
Shuo Zhang, Zezhou Huang, Eugene Wu. arxiv 2024. [pdf]
Match, Compare, or Select? An Investigation of Large Language Models for Entity Matching
Tianshu Wang, Hongyu Lin, Xiaoyang Chen, Xianpei Han, Hao Wang, Zhenyu Zeng, Le Sun. COLING 2025. [pdf]
Cost-Effective In-Context Learning for Entity Resolution: A Design Space Exploration
Meihao Fan, Xiaoyue Han, Ju Fan, Chengliang Chai, Nan Tang, Guoliang Li, Xiaoyong Du. ICDE 2024. [pdf]
In Situ Neural Relational Schema Matcher
Xingyu Du, Gongsheng Yuan, Sai Wu, Gang Chen, and Peng Lu. ICDE 2024. [pdf]
KcMF: A Knowledge-compliant Framework for Schema and Entity Matching with Fine-tuning-free LLMs
Yongqin Xu, Huan Li, Ke Chen, Lidan Shou. arxiv 2024. [pdf]
Unicorn: A Unified Multi-tasking Model for Supporting Matching Tasks in Data Integration
Jianhong Tu, Ju Fan, Nan Tang, Peng Wang, Guoliang Li, Xiaoyong Du. SIGMOD 2023. [pdf]
Entity matching using large language models
Ralph Peeters, Christian Bizer. arxiv 2023. [pdf]
Deep Entity Matching with Pre-Trained Language Models
Yuliang Li, Jinfeng Li, Yoshihiko Suhara, AnHai Doan, Wang-Chiew Tan. VLDB 2021. [pdf]
Dual-Objective Fine-Tuning of BERT for Entity Matching
Ralph Peeters, Christian Bizer. VLDB 2021. [pdf]
Knowledge Graph-based Retrieval-Augmented Generation for Schema Matching
Chuangtao Ma, Sriom Chakrabarti, Arijit Khan, Bálint Molnár. arxiv 2024. [pdf]
Magneto: Combining Small and Large Language Models for Schema Matching
Yurong Liu, Eduardo Pena, Aécio Santos, Eden Wu, Juliana Freire. arix 2024. [pdf]
Schema Matching with Large Language Models: an Experimental Study
Marcel Parciak, Brecht Vandevoort, Frank Neven, Liesbet M. Peeters, Stijn Vansummeren. arxiv 2024. [pdf]
Schema Matching using Pre-Trained Language Models
Yunjia Zhang, Avrilia Floratou, Joyce Cahoon, Subru Krishnan, Andreas C. Müller, Dalitso Banda, Fotis Psallidas, Jignesh M. Patel. ICDE 2023. [pdf]
KcMF: A Knowledge-compliant Framework for Schema and Entity Matching with Fine-tuning-free LLMs
Yongqin Xu, Huan Li, Ke Chen, Lidan Shou. arxiv 2024. [pdf]
CHORUS: Foundation Models for Unified Data Discovery and Exploration
Moe Kayali, Anton Lykov, Ilias Fountalis, Nikolaos Vasiloglou, Dan Olteanu, Dan Suciu. VLDB 2024. [pdf]
Language Models Enable Simple Systems for Generating Structured Views of Heterogeneous Data Lakes
Simran Arora, Brandon Yang, Sabri Eyuboglu, Avanika Narayan, Andrew Hojel, Immanuel Trummer, Christopher Ré. VLDB 2024. [pdf]
DeepJoin: Joinable Table Discovery with Pre-trained Language Models
Yuyang Dong, Chuan Xiao, Takuma Nozawa, Masafumi Enomoto, Masafumi Oyamada. VLDB 2023. [pdf]
There are many related works, with a focus on prioritizing NL2SQL papers within the database field.
Text2SQL is Not Enough: Unifying AI and Databases with TAG
Asim Biswal, Siddharth Jha, Carlos Guestrin, Matei Zaharia, Joseph E Gonzalez, Amog Kamsetty, Shu Liu, Liana Patel. CIDR 2025. [pdf]
The Dawn of Natural Language to SQL: Are We Fully Ready?
Boyan Li, Yuyu Luo, Chengliang Chai, Guoliang Li, Nan Tang. VLDB 2024. [pdf]
PURPLE: Making a Large Language Model a Better SQL Writer
Ren, Tonghui, Yuankai Fan, Zhenying He, Ren Huang, Jiaqi Dai, Can Huang, Yinan Jing, Kai Zhang, Yifan Yang, and X. Sean Wang. ICDE 2024. [pdf]
SM3-Text-to-Query: Synthetic Multi-Model Medical Text-to-Query Benchmark
Sithursan Sivasubramaniam, Cedric Osei-Akoto, Yi Zhang, Kurt Stockinger, Jonathan Fuerst. NeurIPS 2024. [pdf]
Towards Automated Cross-domain Exploratory Data Analysis through Large Language Models
Jun-Peng Zhu, Boyan Niu, Peng Cai, Zheming Ni, Jianwei Wan, Kai Xu, Jiajun Huang, Shengbo Ma, Bing Wang, Xuan Zhou, Guanglei Bao, Donghui Zhang, Liu Tang, and Qi Liu. arxiv 2024. [pdf]
Spider 2.0: Evaluating Language Models on Real-World Enterprise Text-to-SQL Workflows
Fangyu Lei, Jixuan Chen, Yuxiao Ye, Ruisheng Cao, Dongchan Shin, Hongjin Su, Zhaoqing Suo, Hongcheng Gao, Wenjing Hu, Pengcheng Yin, Victor Zhong, Caiming Xiong, Ruoxi Sun, Qian Liu, Sida Wang, Tao Yu. arxiv 2024. [pdf]
SiriusBI: Building End-to-End Business Intelligence Enhanced by Large Language Models
Jie Jiang, Haining Xie, Yu Shen, Zihan Zhang, Meng Lei, Yifeng Zheng, Yide Fang, Chunyou Li, Danqing Huang, Wentao Zhang, Yang Li, Xiaofeng Yang, Bin Cui, Peng Chen. arxiv 2024. [pdf]
Grounding Natural Language to SQL Translation with Data-Based Self-Explanations
Yuankai Fan, Tonghui Ren, Can Huang, Zhenying He, X. Sean Wang. arxiv 2024. [pdf]
LR-SQL: A Supervised Fine-Tuning Method for Text2SQL Tasks under Low-Resource Scenarios
Wen Wuzhenghong, Zhang Yongpan, Pan Su, Sun Yuwei, Lu Pengwei, Ding Cheng. arxiv 2024. [pdf]
CHASE-SQL: Multi-Path Reasoning and Preference Optimized Candidate Selection in Text-to-SQL
Mohammadreza Pourreza, Hailong Li, Ruoxi Sun, Yeounoh Chung, Shayan Talaei, Gaurav Tarlok Kakkar, Yu Gan, Amin Saberi, Fatma Ozcan, Sercan O. Arik. arxiv 2024. [pdf]
MoMQ: Mixture-of-Experts Enhances Multi-Dialect Query Generation across Relational and Non-Relational Databases
Zhisheng Lin, Yifu Liu, Zhiling Luo, Jinyang Gao, Yu Li. arxiv 2024. [pdf]
From BERT to GPT-3 Codex: Harnessing the Potential of Very Large Language Models for Data Management
Immanuel Trummer. VLDB 2022. [pdf]
Few-shot Text-to-SQL Translation using Structure and Content Prompt Learning
Zihui Gu, Ju Fan, Nan Tang, et al. SIGMOD 2023. [pdf]
Db-gpt: Empowering database interactions with private large language models
Siqiao Xue, Caigao Jiang, Wenhui Shi, Fangyin Cheng, Keting Chen, Hongjun Yang, Zhiping Zhang, Jianshan He, Hongyang Zhang, Ganglin Wei, Wang Zhao, Fan Zhou, Danrui Qi, Hong Yi, Shaodong Liu, Faqiang Chen. arxiv 2023. [pdf]
LLM4Vis: Explainable Visualization Recommendation using ChatGPT
Lei Wang, Songheng Zhang, Yun Wang, Ee-Peng Lim, Yong Wang. EMNLP 2023. [pdf]
λ-Tune: Harnessing Large Language Models for Automated Database System Tuning
Victor Giannankouris, Immanuel Trummer. SIGMOD 2025. [pdf]
Automatic Database Configuration Debugging using Retrieval-Augmented Language Models
Sibei Chen, Ju Fan, Bin Wu, Nan Tang, Chao Deng, Pengyi PYW Wang, Ye Li, Jian Tan, Feifei Li, Jingren Zhou, Xiaoyong Du. SIGMOD 2025. [pdf]
LATuner: An LLM-Enhanced Database Tuning System Based on Adaptive Surrogate Model
Fan C, Pan Z, Sun W, et al. Joint European Conference on Machine Learning and Knowledge Discovery in Databases. 2024. [pdf]
LLMTune: Accelerate Database Knob Tuning with Large Language Models
Huang X, Li H, Zhang J, et al. arXiv 2024. [pdf]
Is Large Language Model Good at Database Knob Tuning? A Comprehensive Experimental Evaluation
Yiyan Li, Haoyang Li, Zhao Pu, Jing Zhang, Xinyi Zhang, Tao Ji, Luming Sun, Cuiping Li, Hong Chen. arXiv 2024. [pdf]
GPTuner: A Manual-Reading Database Tuning System via GPT-Guided Bayesian Optimization
Jiale Lao, Yibo Wang, Yufei Li, Jianping Wang, Yunjia Zhang, Zhiyuan Cheng, Wanghu Chen, Mingjie Tang, Jianguo Wang. VLDB 2024. [pdf]
DB-BERT: a Database Tuning Tool that “Reads the Manual”
Immanuel Trummer. SIGMOD 2022. [pdf]
LLM-R2: A Large Language Model Enhanced Rule-based Rewrite System for Boosting Query Efficiency
Zhaodonghui Li, Haitao Yuan#, Huiming Wang, Gao Cong, Lidong Bing. VLDB 2024. [pdf]
The Unreasonable Effectiveness of LLMs for Query Optimization
Peter Akioyamen, Zixuan Yi, Ryan Marcus. NeurIPS 2024 (Workshop). [pdf]
R-Bot: An LLM-based Query Rewrite System
Zhaoyan Sun, Xuanhe Zhou, Guoliang Li. arxiv 2024. [pdf]
Panda: Performance debugging for databases using LLM agents
Vikramank Singh, Kapil Eknath Vaidya, ..., Tim Kraska. CIDR 2024. [pdf]
LLM As DBA
Xuanhe Zhou, Guoliang Li, Zhiyuan Liu. arXiv 2023. [pdf]
D-Bot: Database Diagnosis System using Large Language Models
Xuanhe Zhou, Guoliang Li, Zhaoyan Sun, Zhiyuan Liu, Weize Chen, et al. VLDB 2024. [pdf] [code]
Data-Juicer: A One-Stop Data Processing System for Large Language Models
Daoyuan Chen, Yilun Huang, Zhijian Ma, Hesen Chen, Xuchen Pan, Ce Ge, Dawei Gao, Yuexiang Xie, Zhaoyang Liu, Jinyang Gao, Yaliang Li, Bolin Ding, Jingren Zhou. SIGMOD 2024. [pdf]
CoachLM: Automatic Instruction Revisions Improve the Data Quality in LLM Instruction Tuning
Liu, Yilun, Shimin Tao, Xiaofeng Zhao, Ming Zhu, Wenbing Ma, Junhao Zhu, Chang Su et al. ICDE 2024. [pdf]
Relational Database Augmented Large Language Model
Zongyue Qin, Chen Luo, Zhengyang Wang, Haoming Jiang, Yizhou Sun. arxiv 2024. [pdf]
For Tasks:
Click tags to check more tools for each tasksFor Jobs:
Alternative AI tools for LLM4DB
Similar Open Source Tools
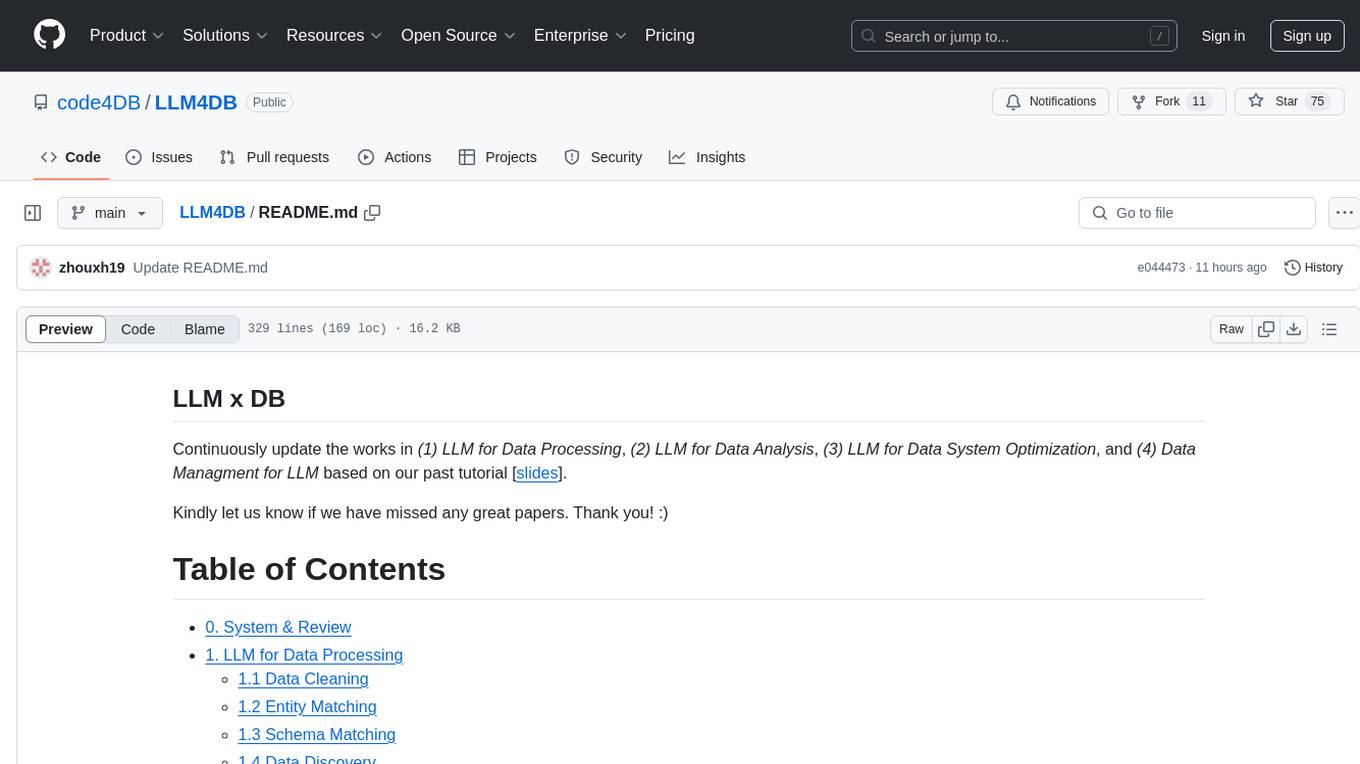
LLM4DB
LLM4DB is a repository focused on the intersection of Large Language Models (LLMs) and Database technologies. It covers various aspects such as data processing, data analysis, database optimization, and data management for LLMs. The repository includes research papers, tools, and techniques related to leveraging LLMs for tasks like data cleaning, entity matching, schema matching, data discovery, NL2SQL, data exploration, data visualization, knob tuning, query optimization, and database diagnosis.
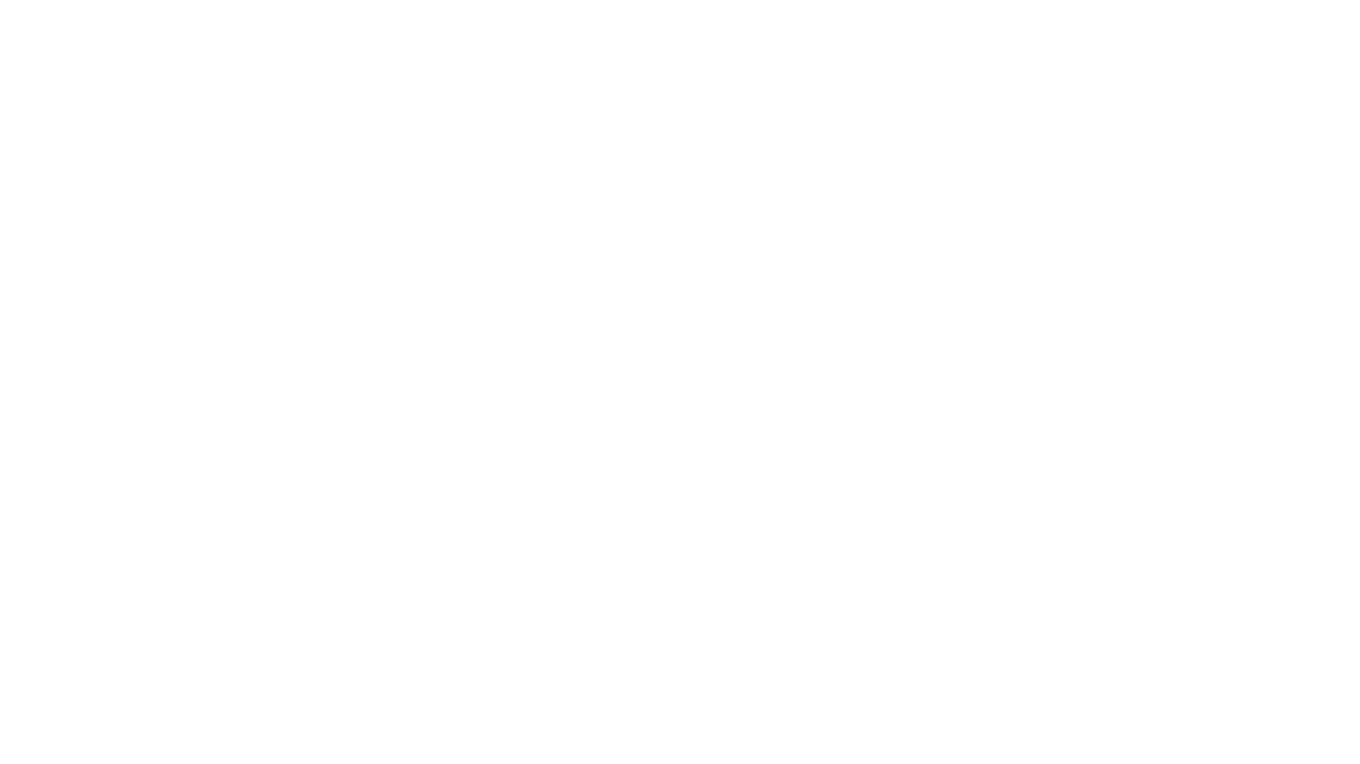
LLM4DB
LLM4DB is a repository focused on the intersection of Large Language Models (LLM) and Database technologies. It covers various aspects such as data processing, data analysis, database optimization, and data management for LLM. The repository includes works on data cleaning, entity matching, schema matching, data discovery, NL2SQL, data exploration, data visualization, configuration tuning, query optimization, and anomaly diagnosis using LLMs. It aims to provide insights and advancements in leveraging LLMs for improving data processing, analysis, and database management tasks.
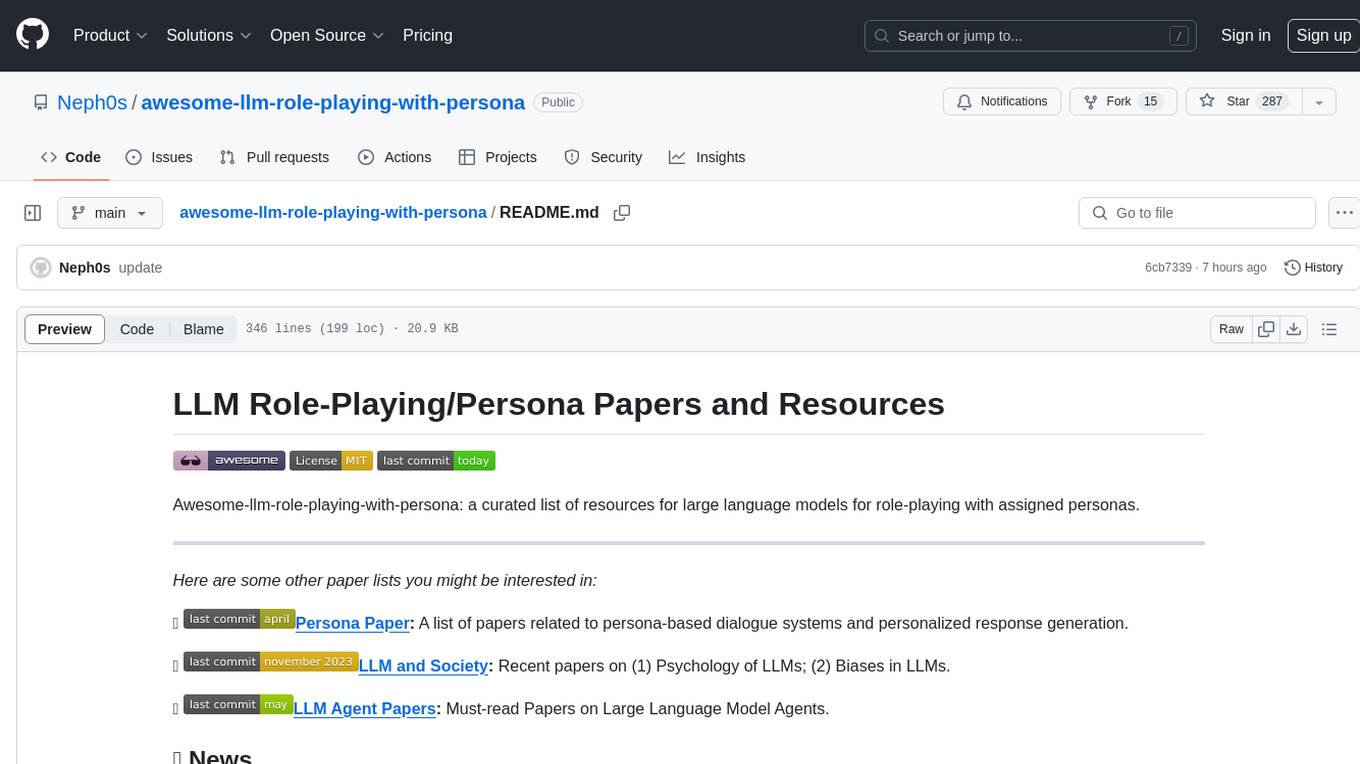
awesome-llm-role-playing-with-persona
Awesome-llm-role-playing-with-persona is a curated list of resources for large language models for role-playing with assigned personas. It includes papers and resources related to persona-based dialogue systems, personalized response generation, psychology of LLMs, biases in LLMs, and more. The repository aims to provide a comprehensive collection of research papers and tools for exploring role-playing abilities of large language models in various contexts.
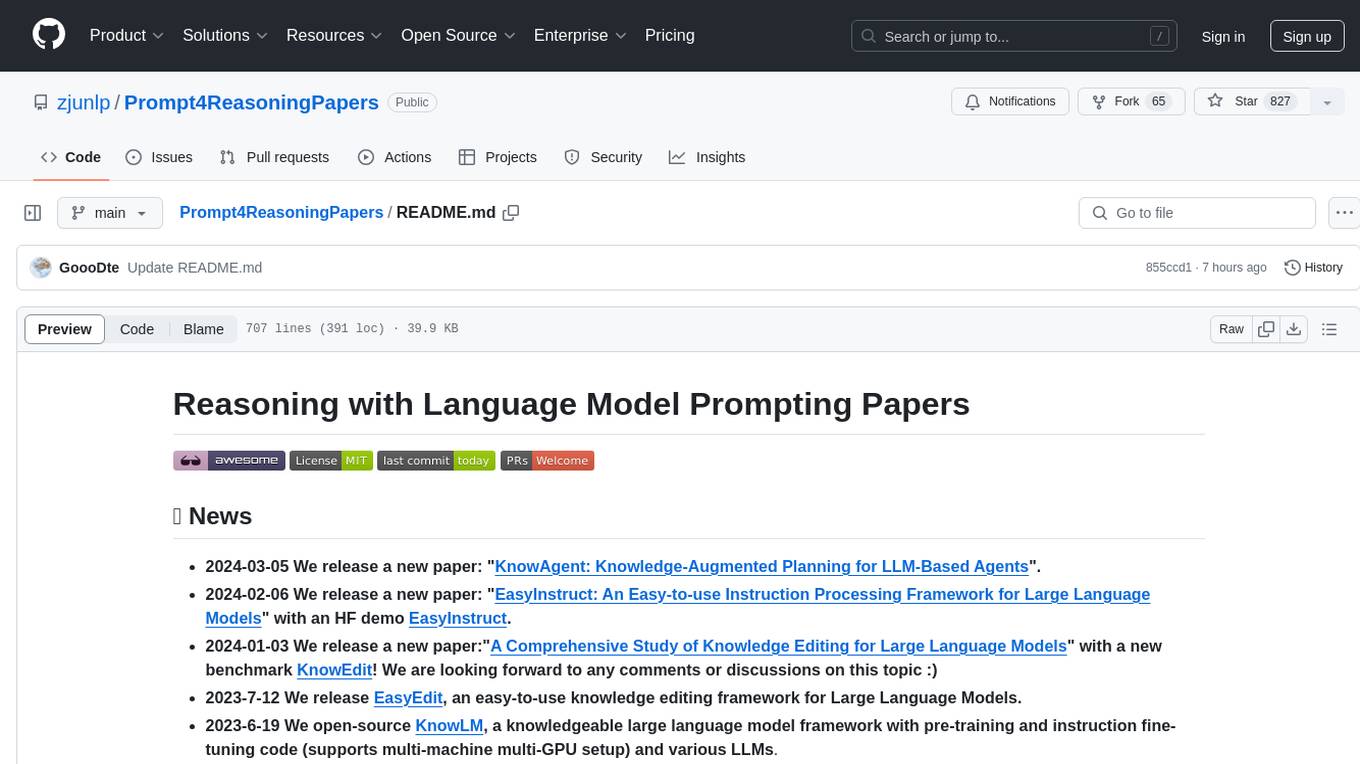
Prompt4ReasoningPapers
Prompt4ReasoningPapers is a repository dedicated to reasoning with language model prompting. It provides a comprehensive survey of cutting-edge research on reasoning abilities with language models. The repository includes papers, methods, analysis, resources, and tools related to reasoning tasks. It aims to support various real-world applications such as medical diagnosis, negotiation, etc.
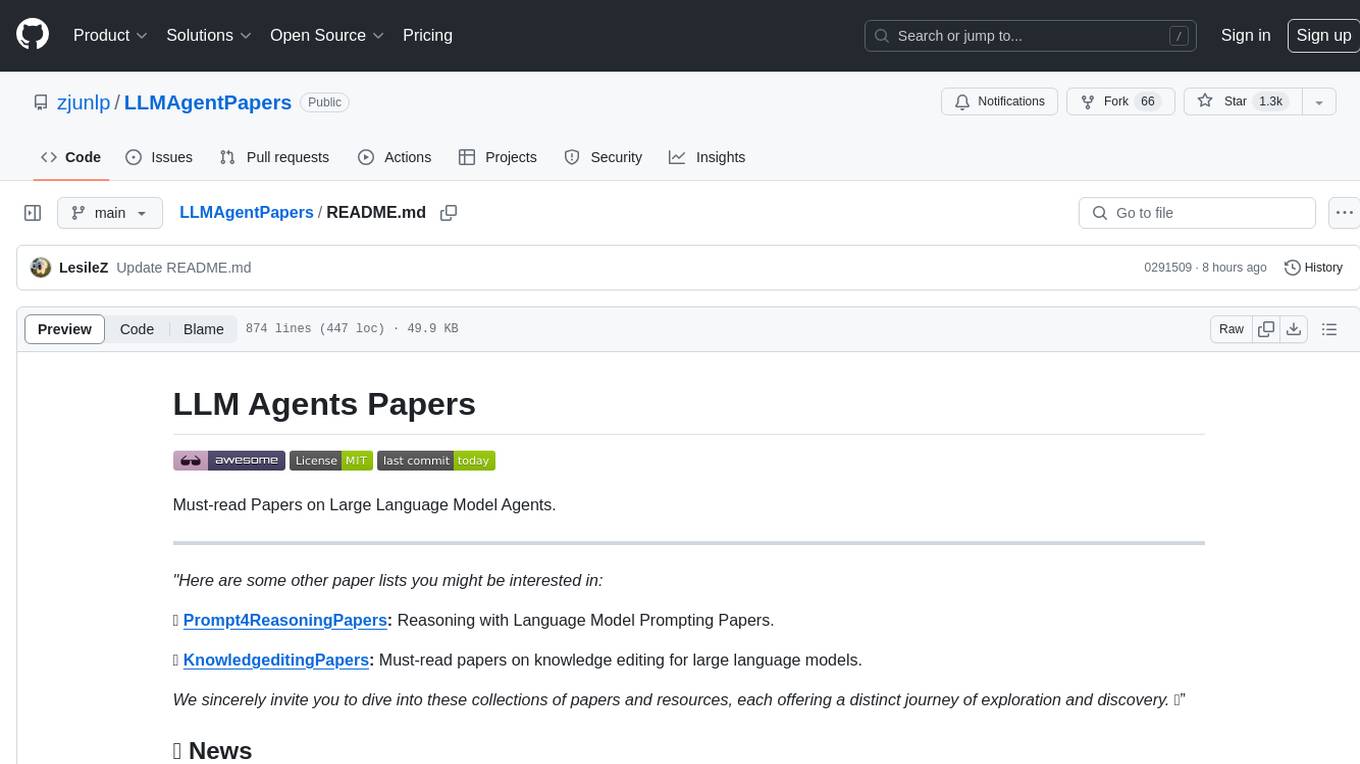
LLMAgentPapers
LLM Agents Papers is a repository containing must-read papers on Large Language Model Agents. It covers a wide range of topics related to language model agents, including interactive natural language processing, large language model-based autonomous agents, personality traits in large language models, memory enhancements, planning capabilities, tool use, multi-agent communication, and more. The repository also provides resources such as benchmarks, types of tools, and a tool list for building and evaluating language model agents. Contributors are encouraged to add important works to the repository.
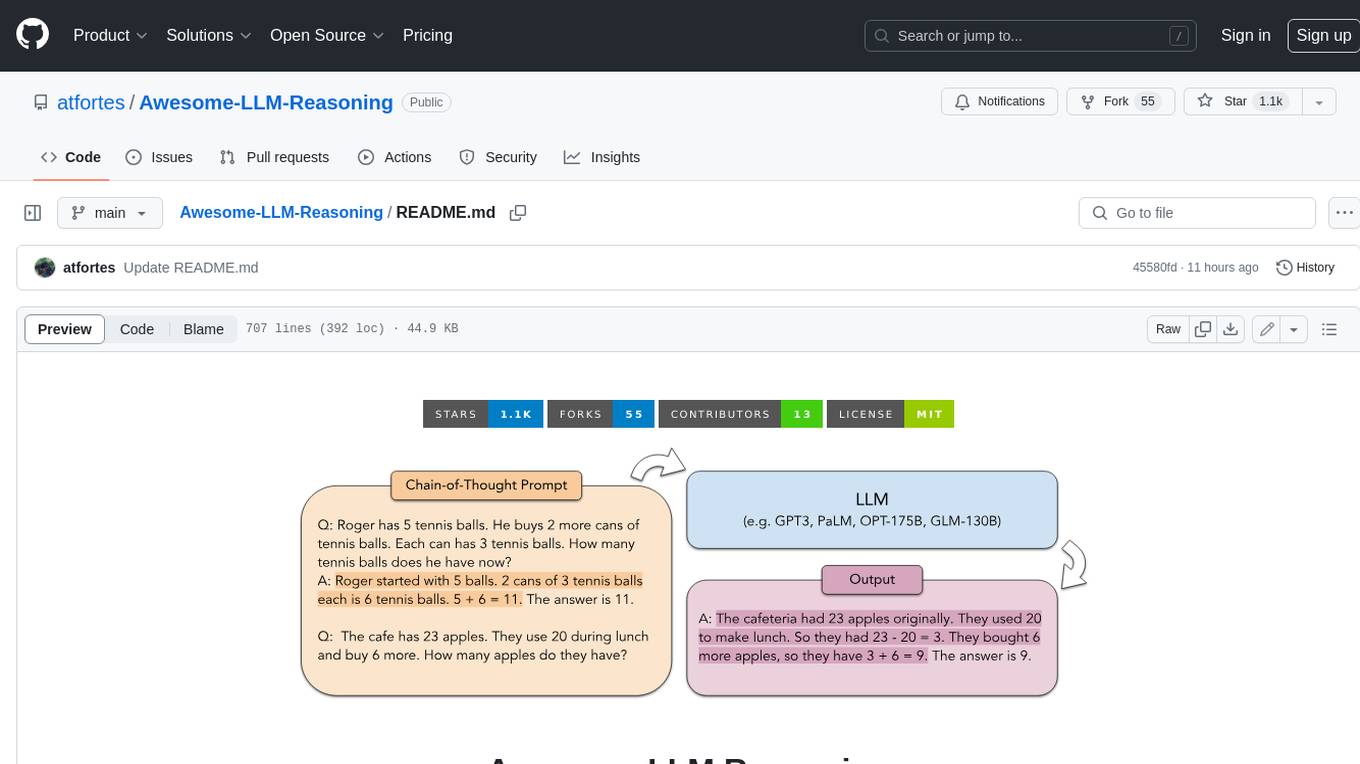
Awesome-LLM-Reasoning
**Curated collection of papers and resources on how to unlock the reasoning ability of LLMs and MLLMs.** **Description in less than 400 words, no line breaks and quotation marks.** Large Language Models (LLMs) have revolutionized the NLP landscape, showing improved performance and sample efficiency over smaller models. However, increasing model size alone has not proved sufficient for high performance on challenging reasoning tasks, such as solving arithmetic or commonsense problems. This curated collection of papers and resources presents the latest advancements in unlocking the reasoning abilities of LLMs and Multimodal LLMs (MLLMs). It covers various techniques, benchmarks, and applications, providing a comprehensive overview of the field. **5 jobs suitable for this tool, in lowercase letters.** - content writer - researcher - data analyst - software engineer - product manager **Keywords of the tool, in lowercase letters.** - llm - reasoning - multimodal - chain-of-thought - prompt engineering **5 specific tasks user can use this tool to do, in less than 3 words, Verb + noun form, in daily spoken language.** - write a story - answer a question - translate a language - generate code - summarize a document
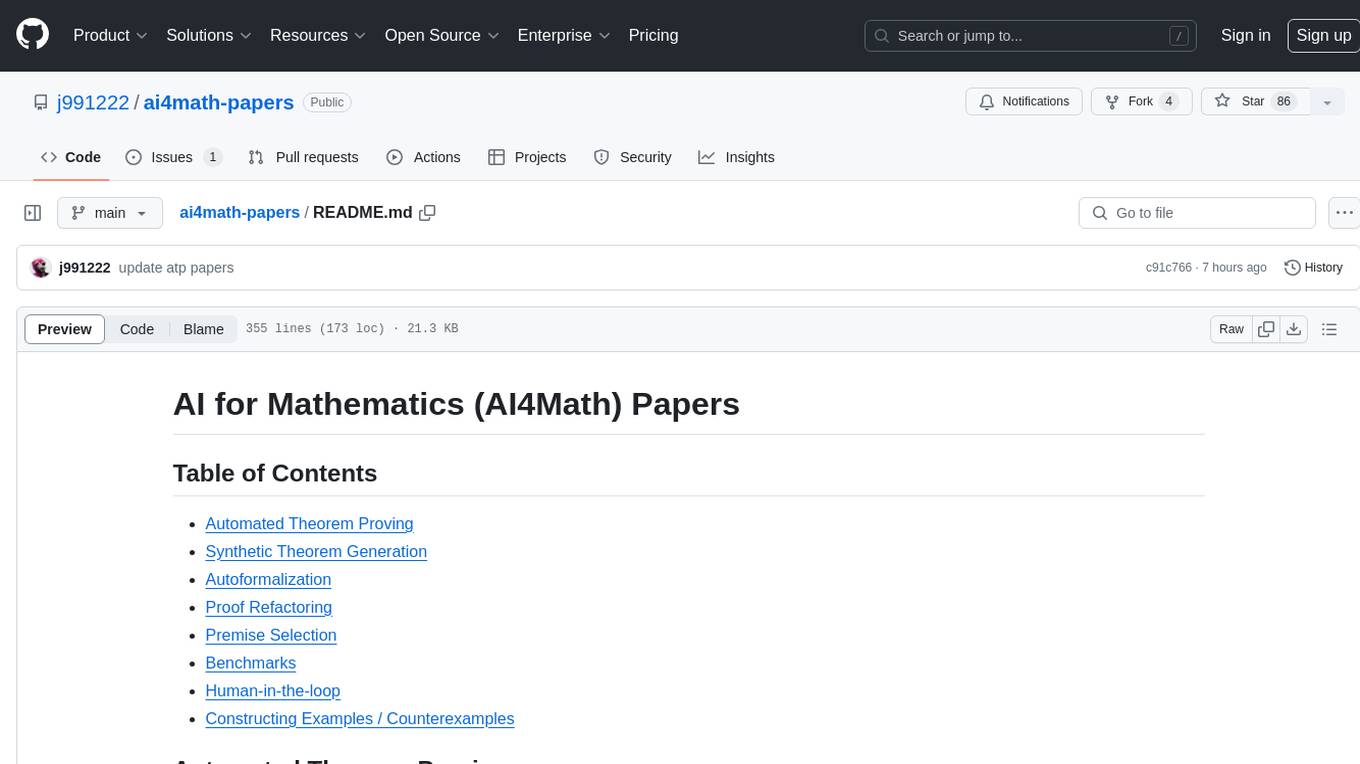
ai4math-papers
The 'ai4math-papers' repository contains a collection of research papers related to AI applications in mathematics, including automated theorem proving, synthetic theorem generation, autoformalization, proof refactoring, premise selection, benchmarks, human-in-the-loop interactions, and constructing examples/counterexamples. The papers cover various topics such as neural theorem proving, reinforcement learning for theorem proving, generative language modeling, formal mathematics statement curriculum learning, and more. The repository serves as a valuable resource for researchers and practitioners interested in the intersection of AI and mathematics.
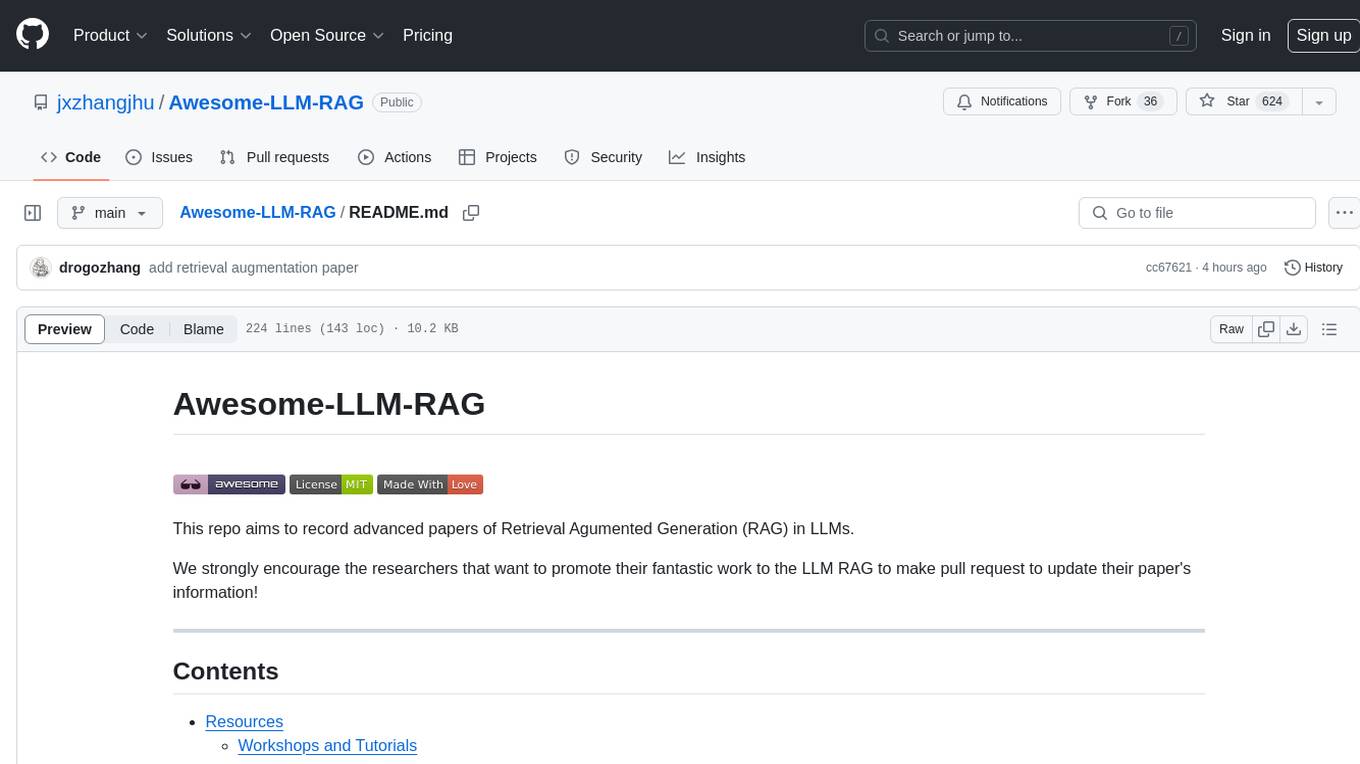
Awesome-LLM-RAG
This repository, Awesome-LLM-RAG, aims to record advanced papers on Retrieval Augmented Generation (RAG) in Large Language Models (LLMs). It serves as a resource hub for researchers interested in promoting their work related to LLM RAG by updating paper information through pull requests. The repository covers various topics such as workshops, tutorials, papers, surveys, benchmarks, retrieval-enhanced LLMs, RAG instruction tuning, RAG in-context learning, RAG embeddings, RAG simulators, RAG search, RAG long-text and memory, RAG evaluation, RAG optimization, and RAG applications.
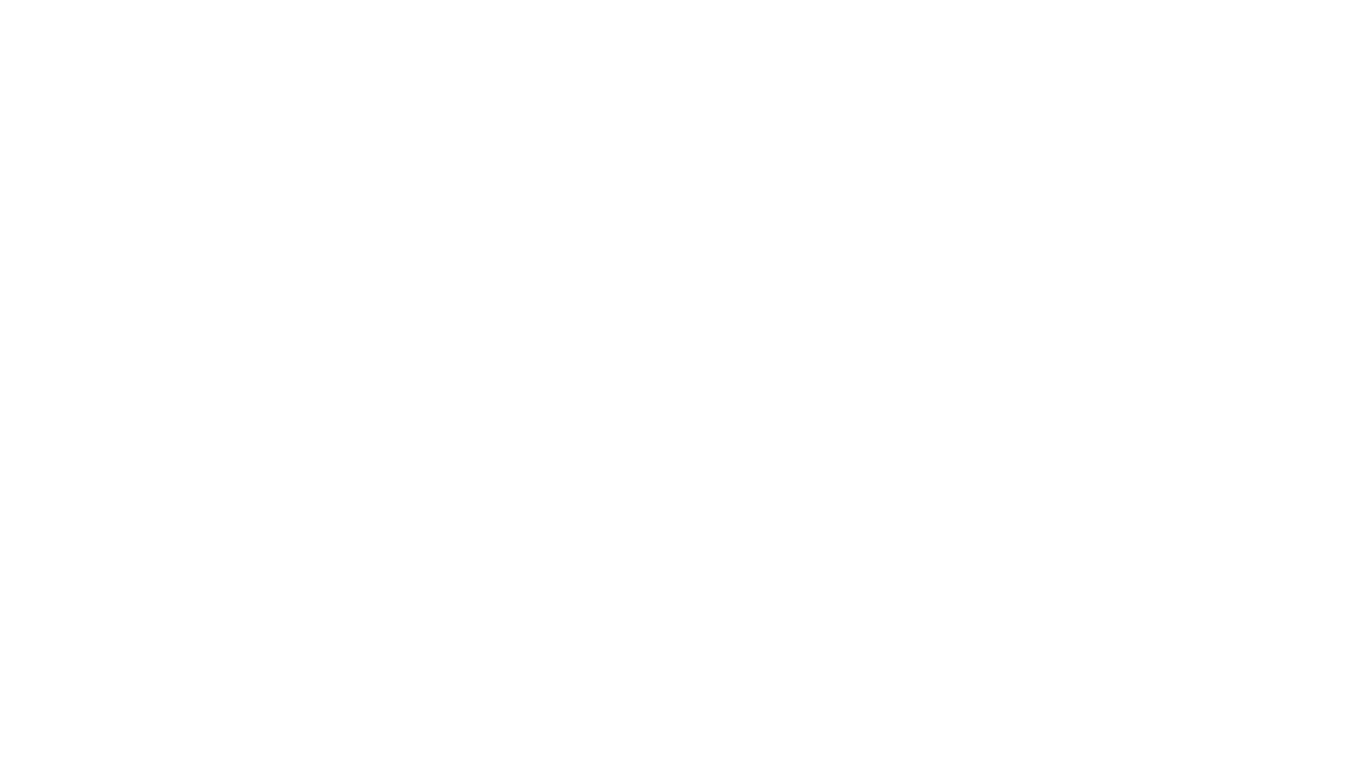
awesome-generative-information-retrieval
This repository contains a curated list of resources on generative information retrieval, including research papers, datasets, tools, and applications. Generative information retrieval is a subfield of information retrieval that uses generative models to generate new documents or passages of text that are relevant to a given query. This can be useful for a variety of tasks, such as question answering, summarization, and document generation. The resources in this repository are intended to help researchers and practitioners stay up-to-date on the latest advances in generative information retrieval.
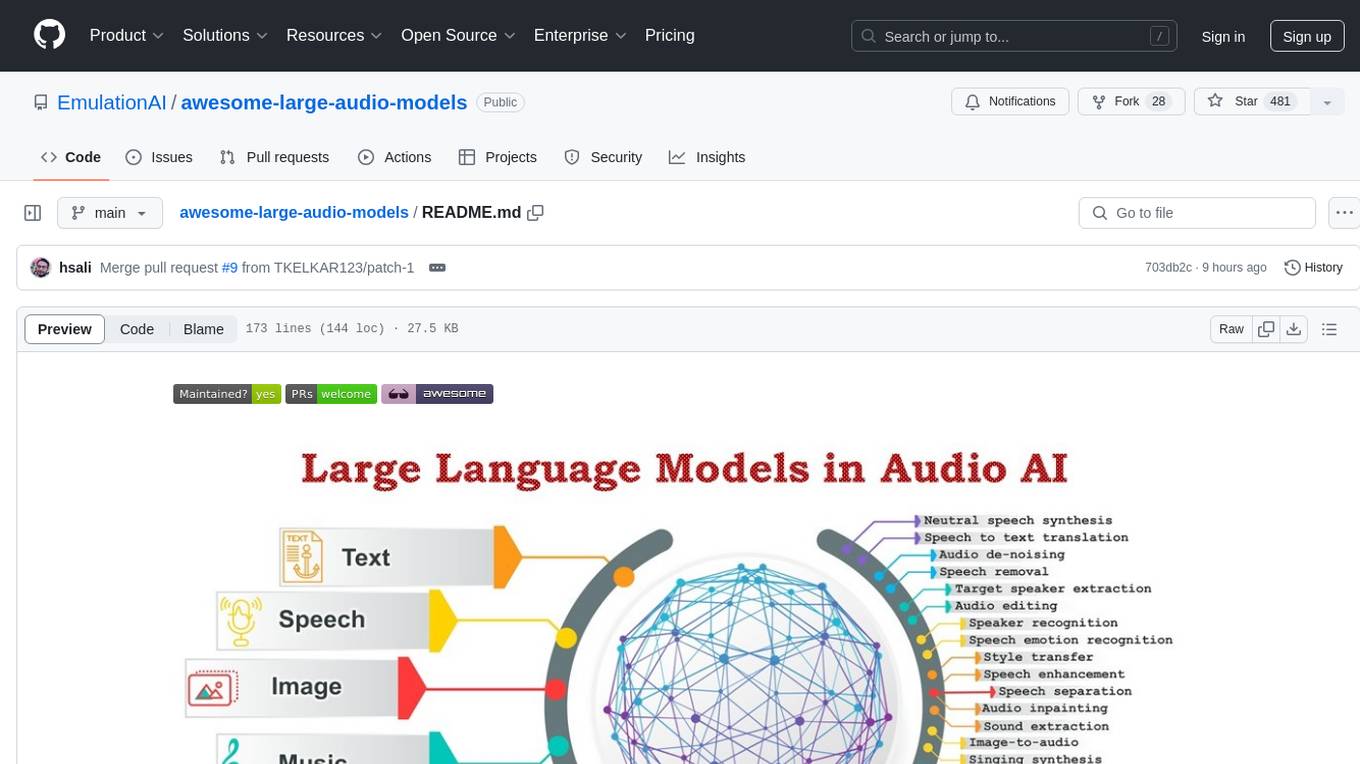
awesome-large-audio-models
This repository is a curated list of awesome large AI models in audio signal processing, focusing on the application of large language models to audio tasks. It includes survey papers, popular large audio models, automatic speech recognition, neural speech synthesis, speech translation, other speech applications, large audio models in music, and audio datasets. The repository aims to provide a comprehensive overview of recent advancements and challenges in applying large language models to audio signal processing, showcasing the efficacy of transformer-based architectures in various audio tasks.
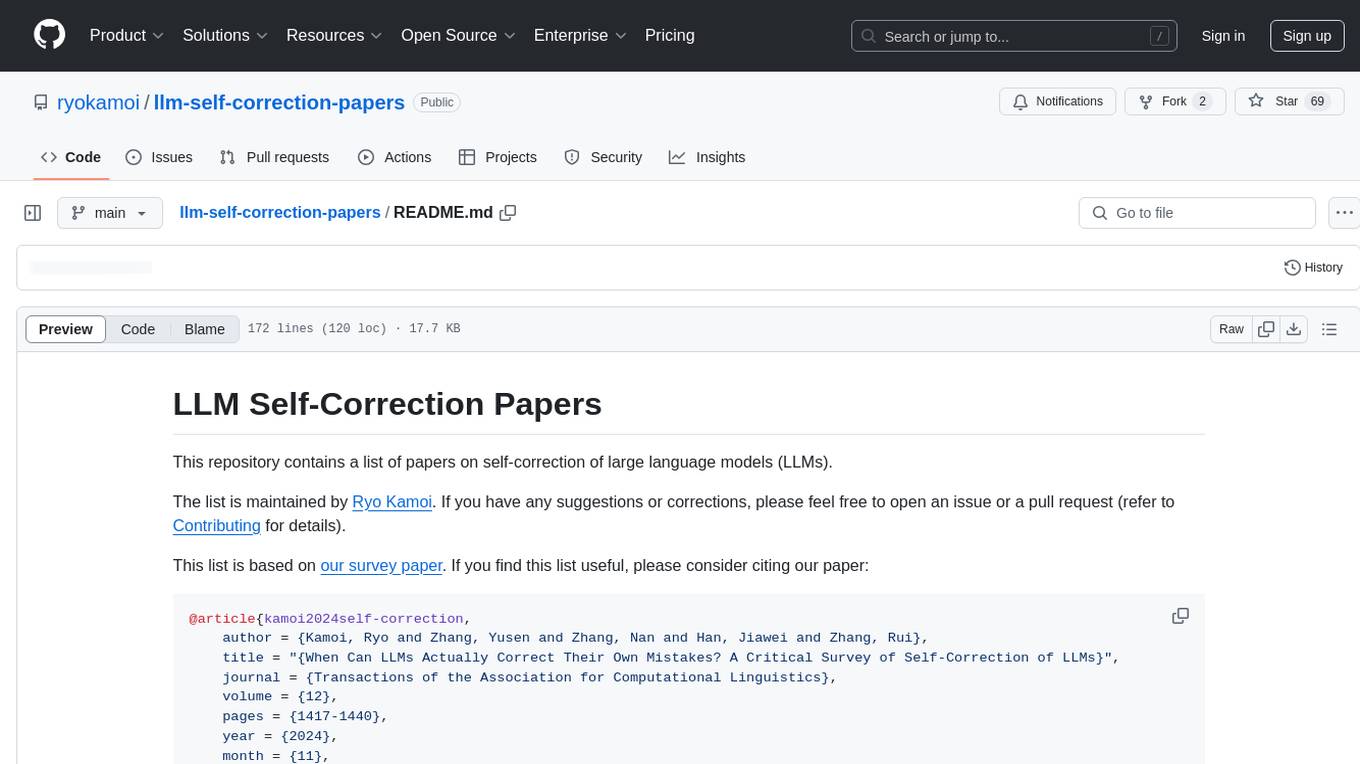
llm-self-correction-papers
This repository contains a curated list of papers focusing on the self-correction of large language models (LLMs) during inference. It covers various frameworks for self-correction, including intrinsic self-correction, self-correction with external tools, self-correction with information retrieval, and self-correction with training designed specifically for self-correction. The list includes survey papers, negative results, and frameworks utilizing reinforcement learning and OpenAI o1-like approaches. Contributions are welcome through pull requests following a specific format.
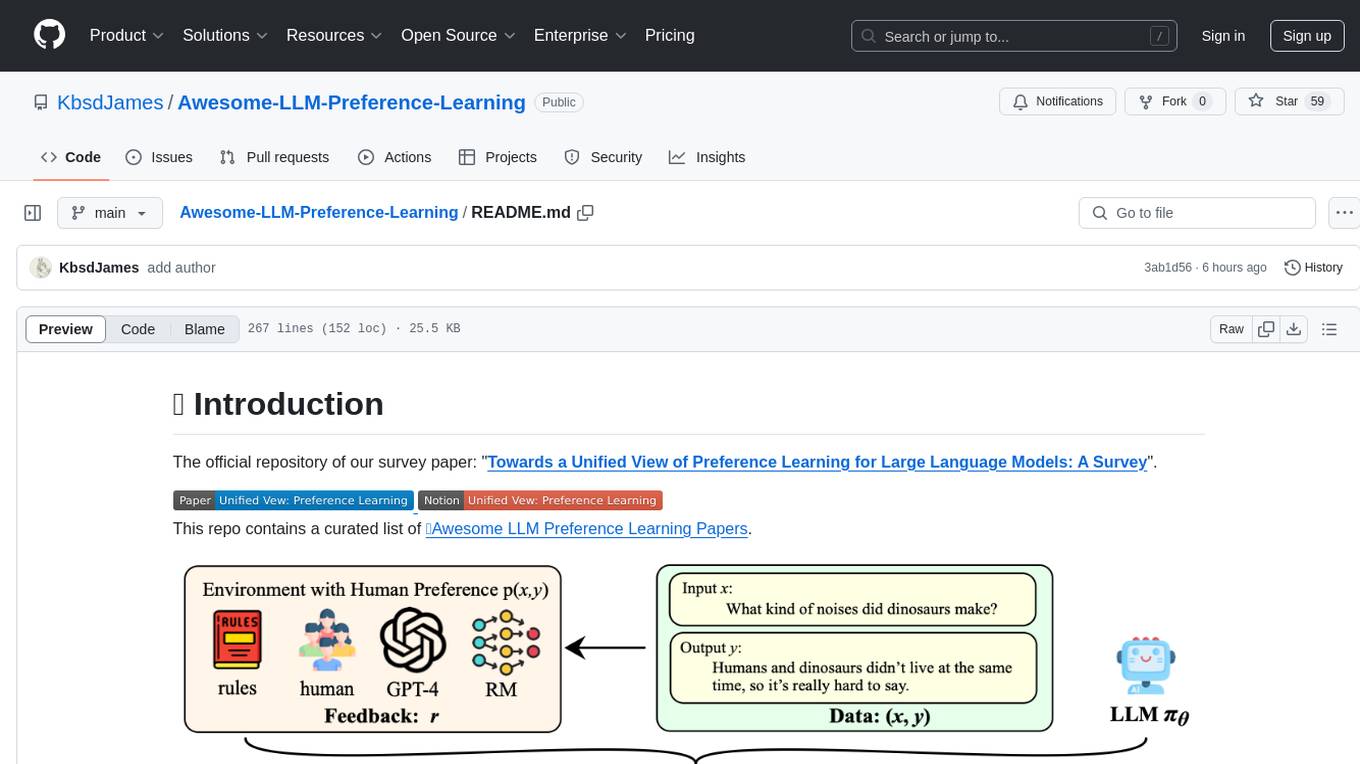
Awesome-LLM-Preference-Learning
The repository 'Awesome-LLM-Preference-Learning' is the official repository of a survey paper titled 'Towards a Unified View of Preference Learning for Large Language Models: A Survey'. It contains a curated list of papers related to preference learning for Large Language Models (LLMs). The repository covers various aspects of preference learning, including on-policy and off-policy methods, feedback mechanisms, reward models, algorithms, evaluation techniques, and more. The papers included in the repository explore different approaches to aligning LLMs with human preferences, improving mathematical reasoning in LLMs, enhancing code generation, and optimizing language model performance.
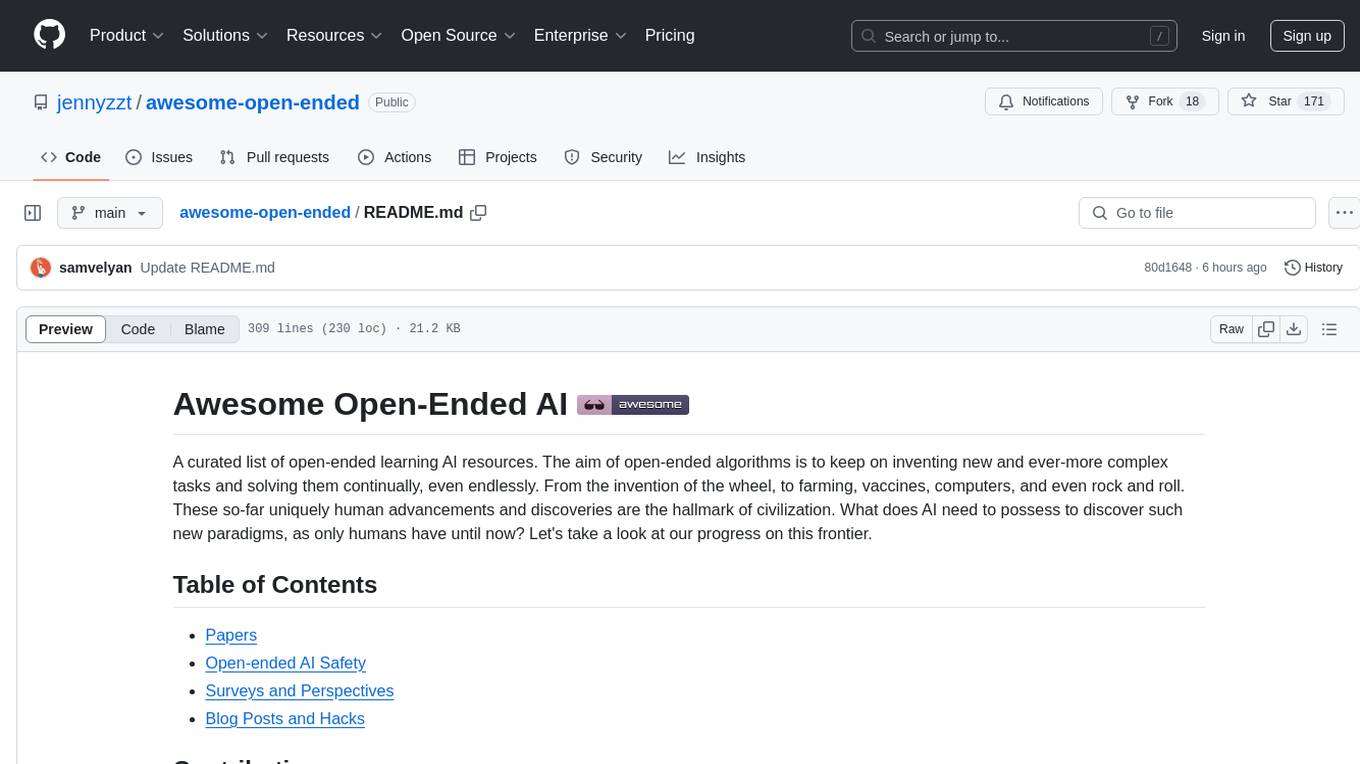
awesome-open-ended
A curated list of open-ended learning AI resources focusing on algorithms that invent new and complex tasks endlessly, inspired by human advancements. The repository includes papers, safety considerations, surveys, perspectives, and blog posts related to open-ended AI research.
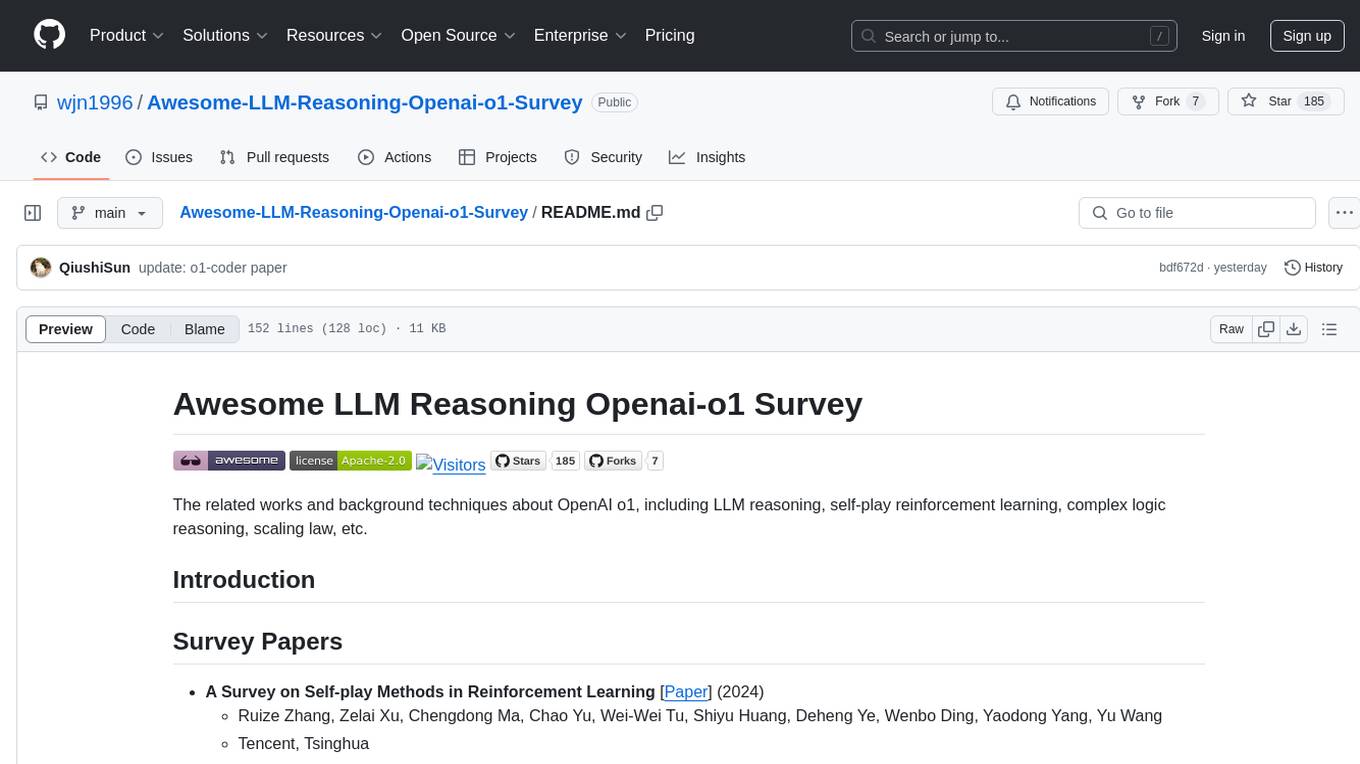
Awesome-LLM-Reasoning-Openai-o1-Survey
The repository 'Awesome LLM Reasoning Openai-o1 Survey' provides a collection of survey papers and related works on OpenAI o1, focusing on topics such as LLM reasoning, self-play reinforcement learning, complex logic reasoning, and scaling law. It includes papers from various institutions and researchers, showcasing advancements in reasoning bootstrapping, reasoning scaling law, self-play learning, step-wise and process-based optimization, and applications beyond math. The repository serves as a valuable resource for researchers interested in exploring the intersection of language models and reasoning techniques.
For similar tasks
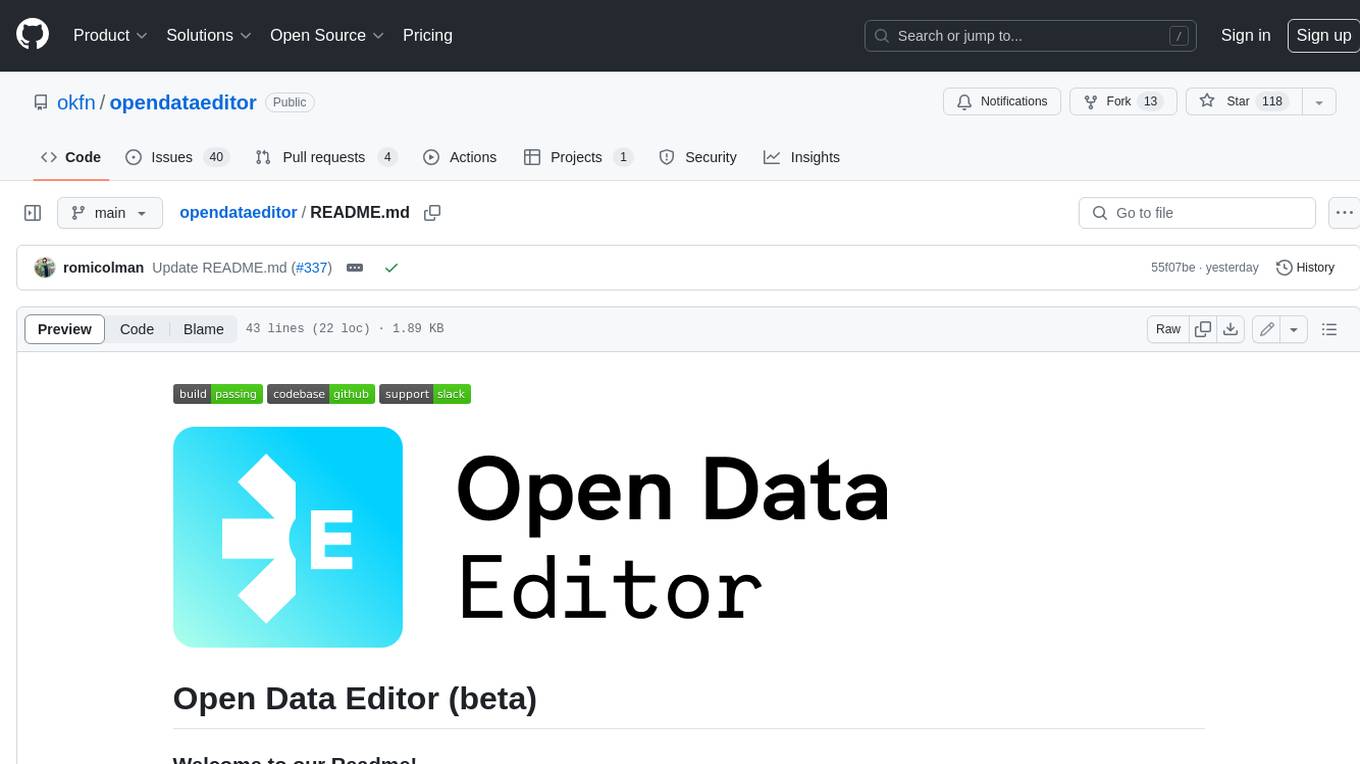
opendataeditor
The Open Data Editor (ODE) is a no-code application to explore, validate and publish data in a simple way. It is an open source project powered by the Frictionless Framework. The ODE is currently available for download and testing in beta.
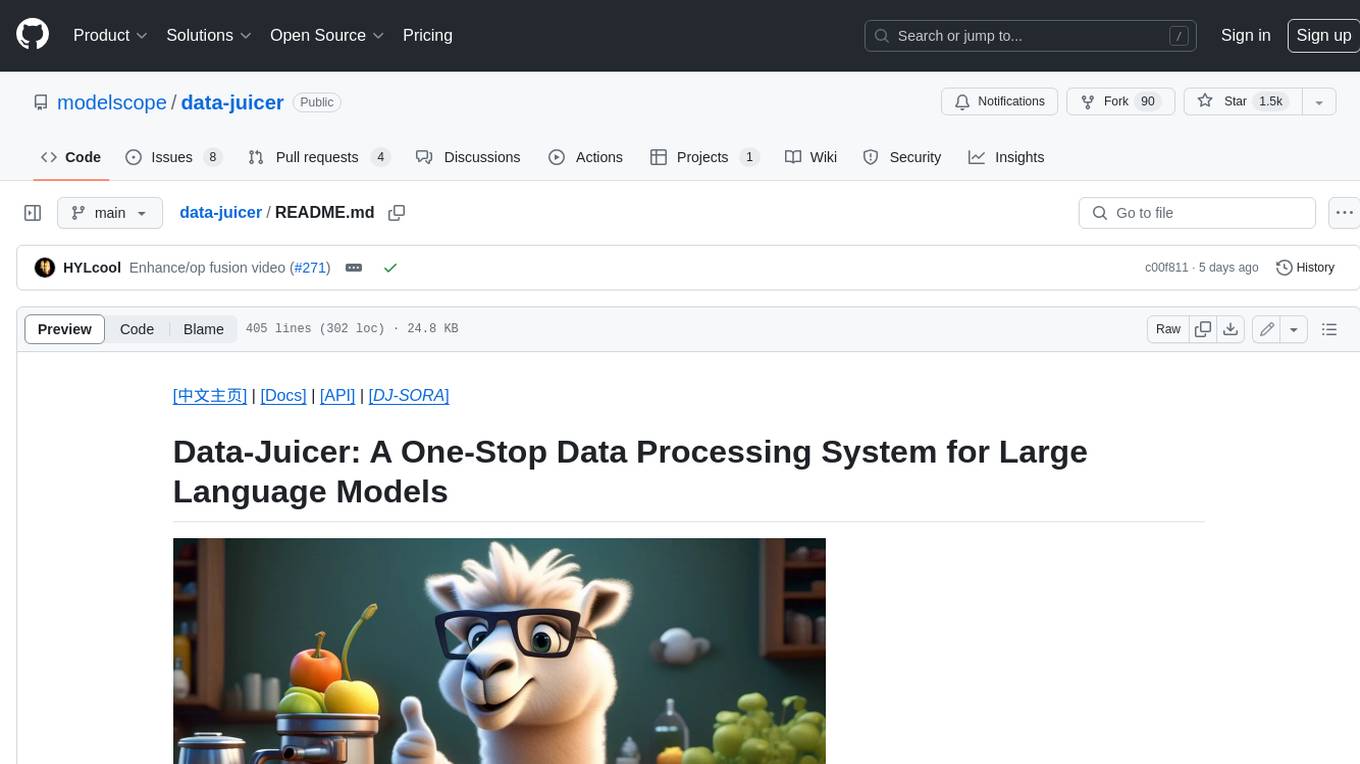
data-juicer
Data-Juicer is a one-stop data processing system to make data higher-quality, juicier, and more digestible for LLMs. It is a systematic & reusable library of 80+ core OPs, 20+ reusable config recipes, and 20+ feature-rich dedicated toolkits, designed to function independently of specific LLM datasets and processing pipelines. Data-Juicer allows detailed data analyses with an automated report generation feature for a deeper understanding of your dataset. Coupled with multi-dimension automatic evaluation capabilities, it supports a timely feedback loop at multiple stages in the LLM development process. Data-Juicer offers tens of pre-built data processing recipes for pre-training, fine-tuning, en, zh, and more scenarios. It provides a speedy data processing pipeline requiring less memory and CPU usage, optimized for maximum productivity. Data-Juicer is flexible & extensible, accommodating most types of data formats and allowing flexible combinations of OPs. It is designed for simplicity, with comprehensive documentation, easy start guides and demo configs, and intuitive configuration with simple adding/removing OPs from existing configs.
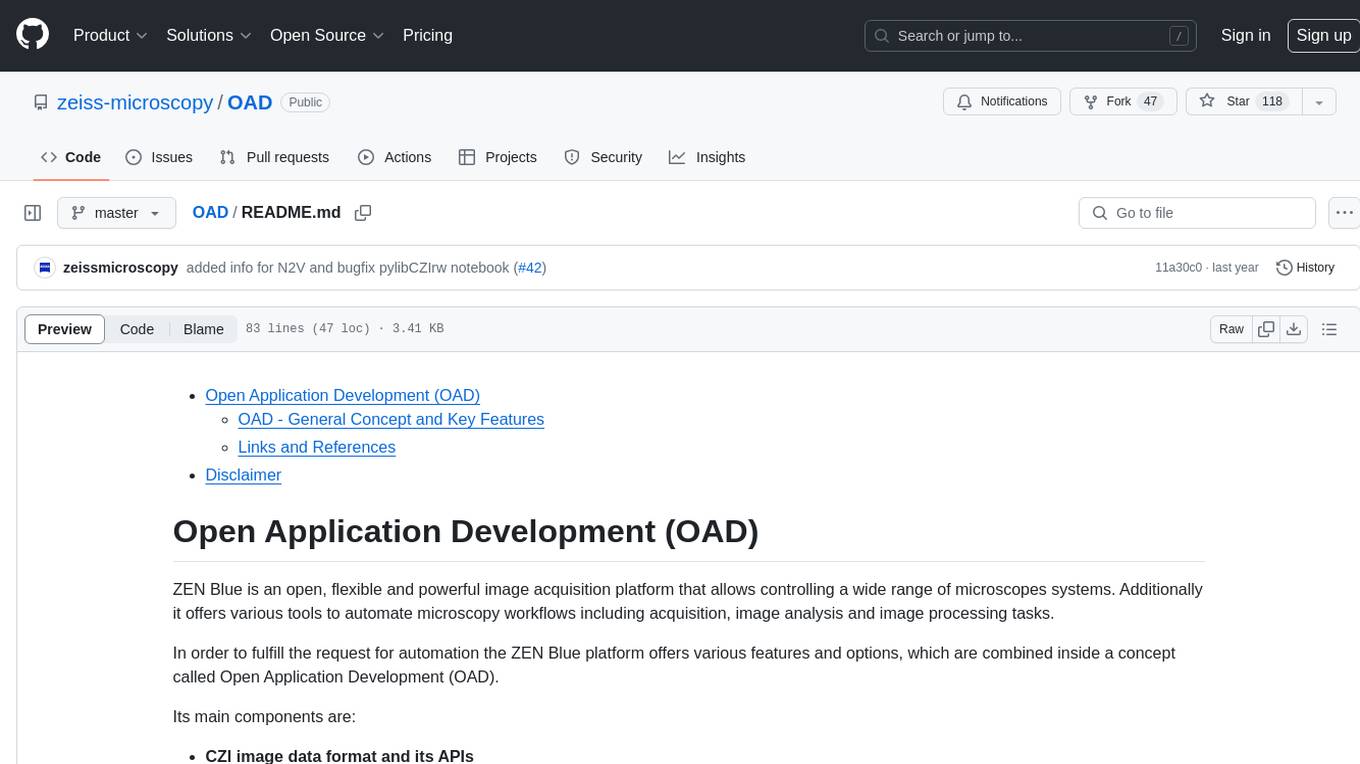
OAD
OAD is a powerful open-source tool for analyzing and visualizing data. It provides a user-friendly interface for exploring datasets, generating insights, and creating interactive visualizations. With OAD, users can easily import data from various sources, clean and preprocess data, perform statistical analysis, and create customizable visualizations to communicate findings effectively. Whether you are a data scientist, analyst, or researcher, OAD can help you streamline your data analysis workflow and uncover valuable insights from your data.
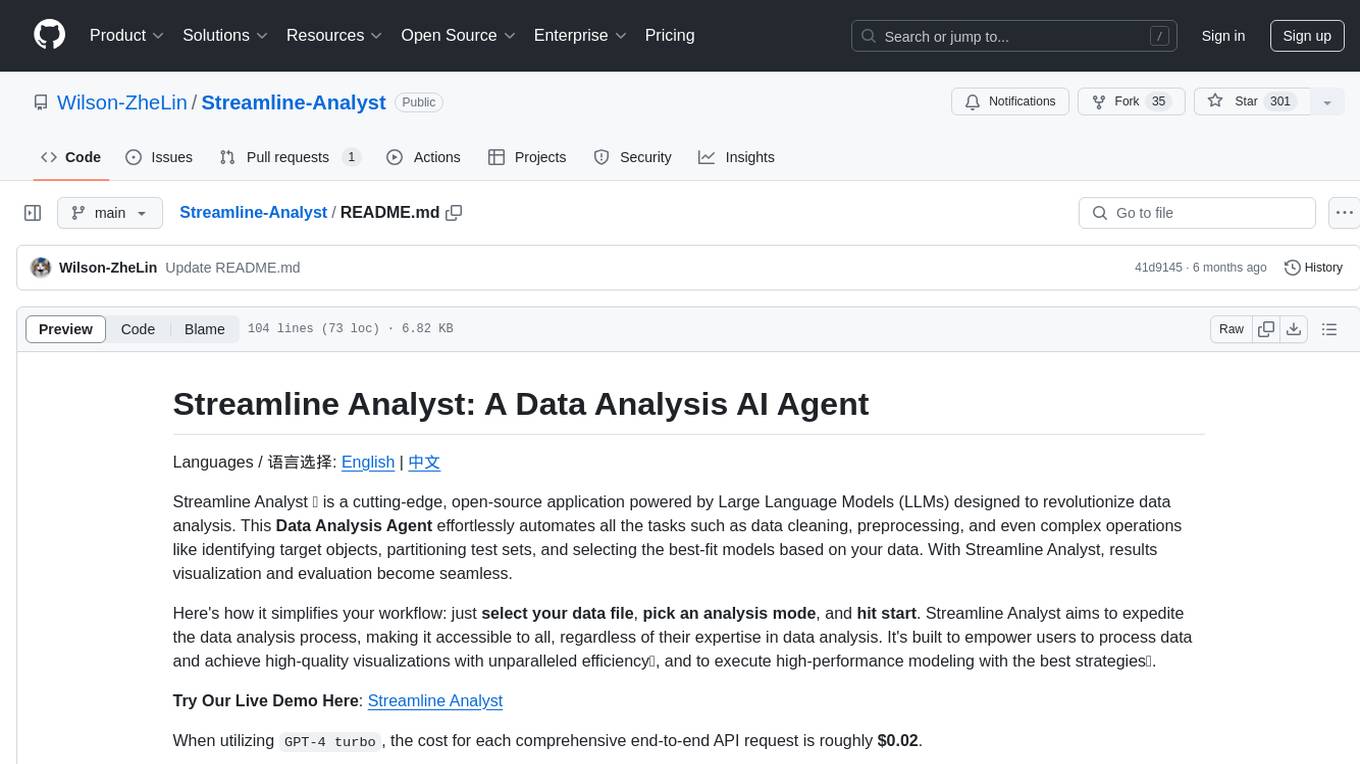
Streamline-Analyst
Streamline Analyst is a cutting-edge, open-source application powered by Large Language Models (LLMs) designed to revolutionize data analysis. This Data Analysis Agent effortlessly automates tasks such as data cleaning, preprocessing, and complex operations like identifying target objects, partitioning test sets, and selecting the best-fit models based on your data. With Streamline Analyst, results visualization and evaluation become seamless. It aims to expedite the data analysis process, making it accessible to all, regardless of their expertise in data analysis. The tool is built to empower users to process data and achieve high-quality visualizations with unparalleled efficiency, and to execute high-performance modeling with the best strategies. Future enhancements include Natural Language Processing (NLP), neural networks, and object detection utilizing YOLO, broadening its capabilities to meet diverse data analysis needs.
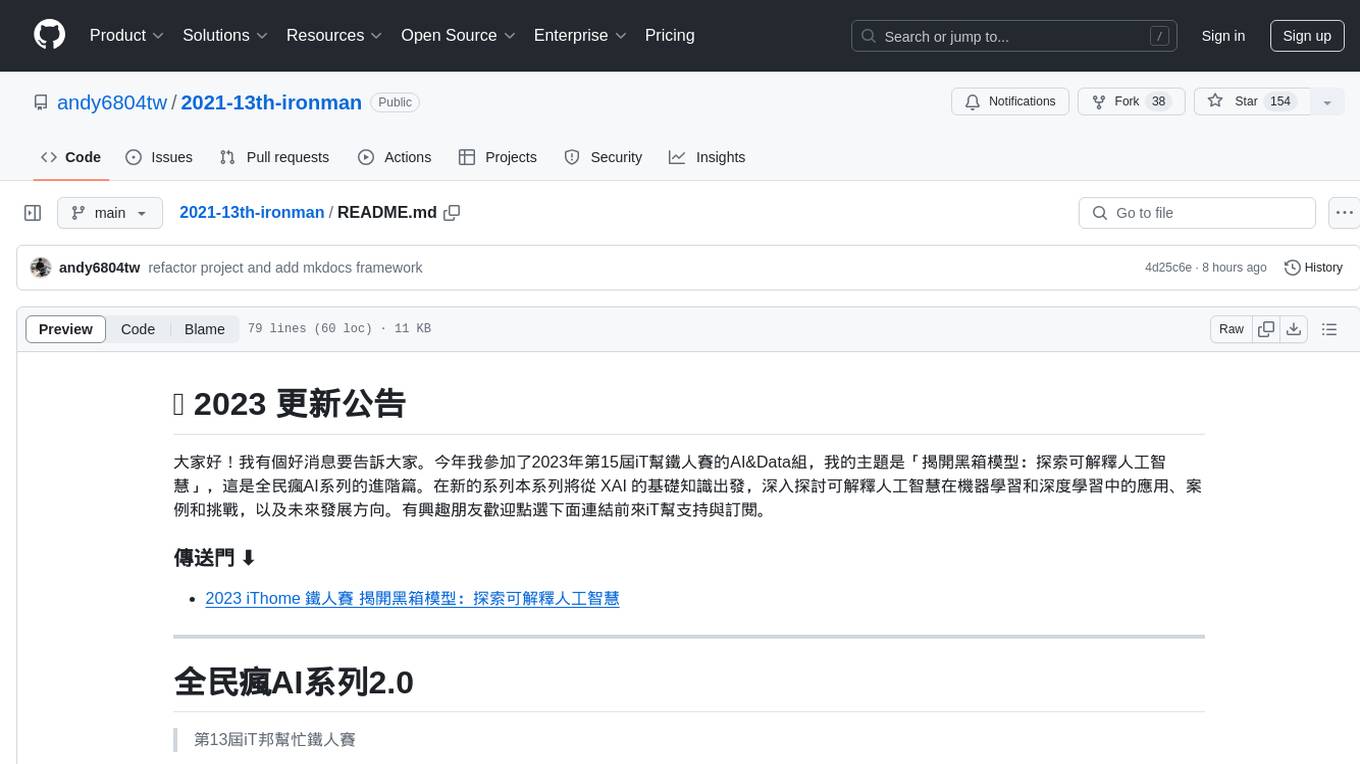
2021-13th-ironman
This repository is a part of the 13th iT Help Ironman competition, focusing on exploring explainable artificial intelligence (XAI) in machine learning and deep learning. The content covers the basics of XAI, its applications, cases, challenges, and future directions. It also includes practical machine learning algorithms, model deployment, and integration concepts. The author aims to provide detailed resources on AI and share knowledge with the audience through this competition.
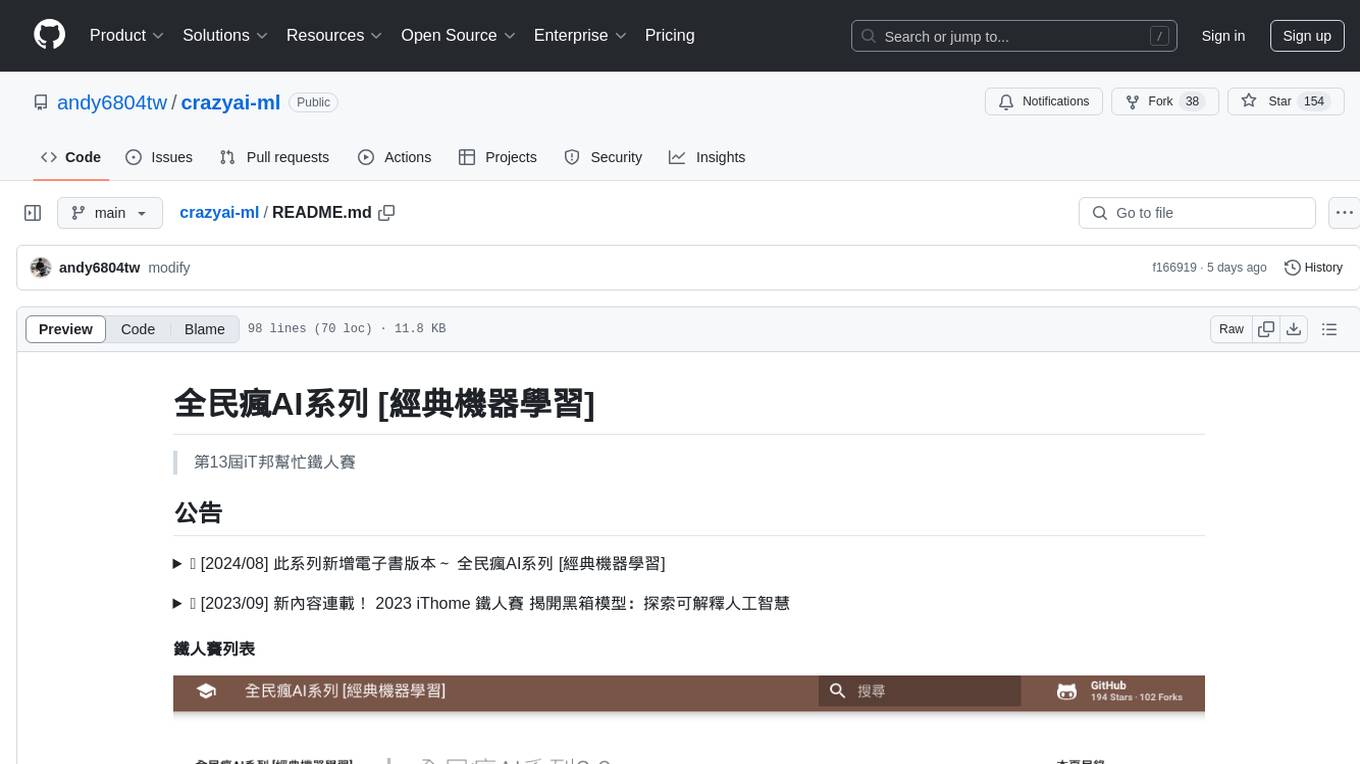
crazyai-ml
The 'crazyai-ml' repository is a collection of resources related to machine learning, specifically focusing on explaining artificial intelligence models. It includes articles, code snippets, and tutorials covering various machine learning algorithms, data analysis, model training, and deployment. The content aims to provide a comprehensive guide for beginners in the field of AI, offering practical implementations and insights into popular machine learning packages and model tuning techniques. The repository also addresses the integration of AI models and frontend-backend concepts, making it a valuable resource for individuals interested in AI applications.
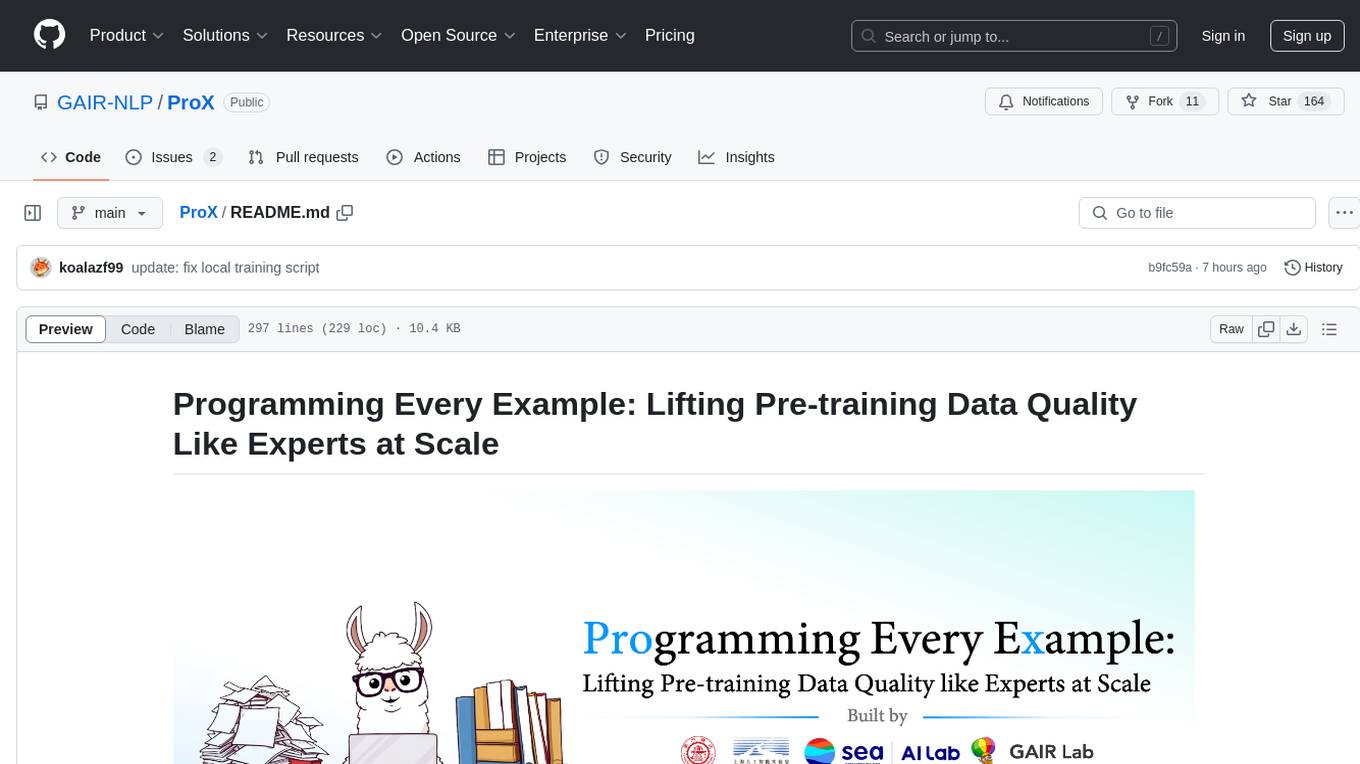
ProX
ProX is a lm-based data refinement framework that automates the process of cleaning and improving data used in pre-training large language models. It offers better performance, domain flexibility, efficiency, and cost-effectiveness compared to traditional methods. The framework has been shown to improve model performance by over 2% and boost accuracy by up to 20% in tasks like math. ProX is designed to refine data at scale without the need for manual adjustments, making it a valuable tool for data preprocessing in natural language processing tasks.
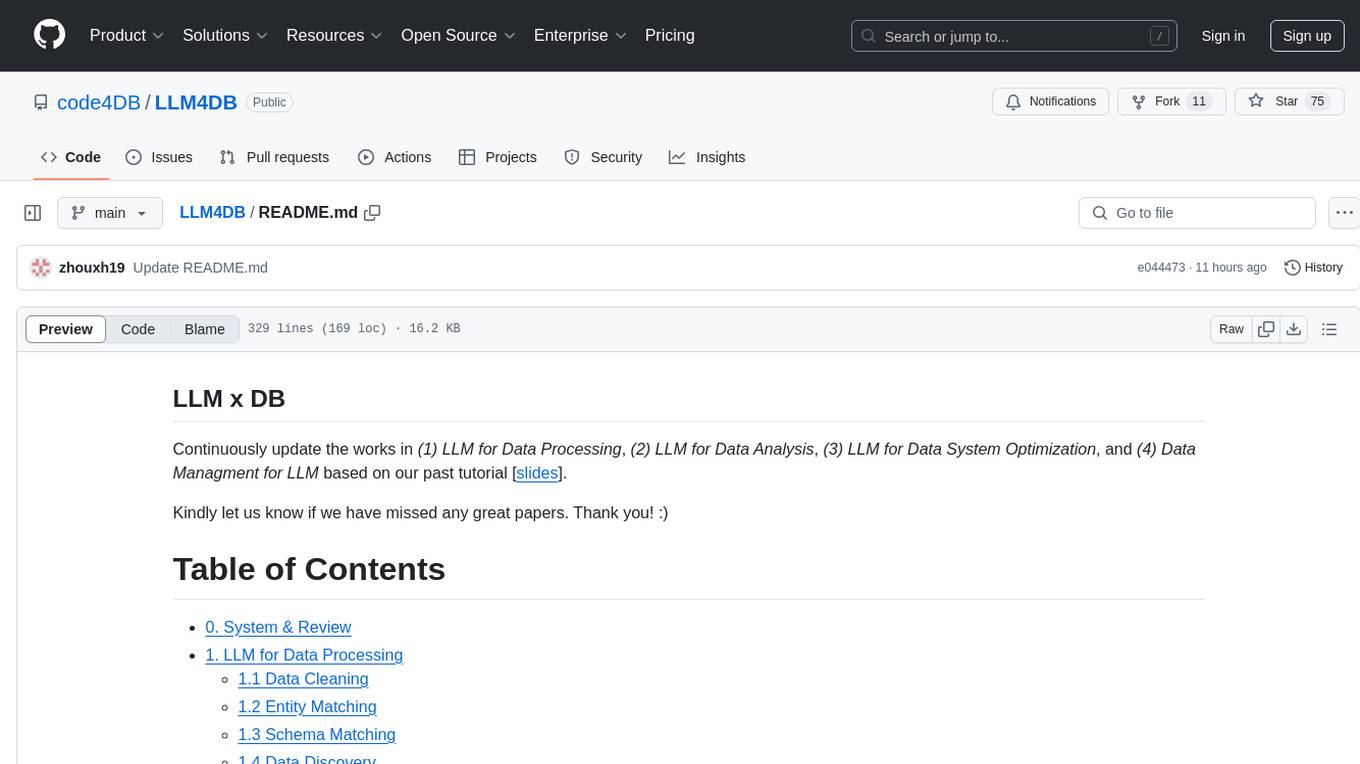
LLM4DB
LLM4DB is a repository focused on the intersection of Large Language Models (LLMs) and Database technologies. It covers various aspects such as data processing, data analysis, database optimization, and data management for LLMs. The repository includes research papers, tools, and techniques related to leveraging LLMs for tasks like data cleaning, entity matching, schema matching, data discovery, NL2SQL, data exploration, data visualization, knob tuning, query optimization, and database diagnosis.
For similar jobs
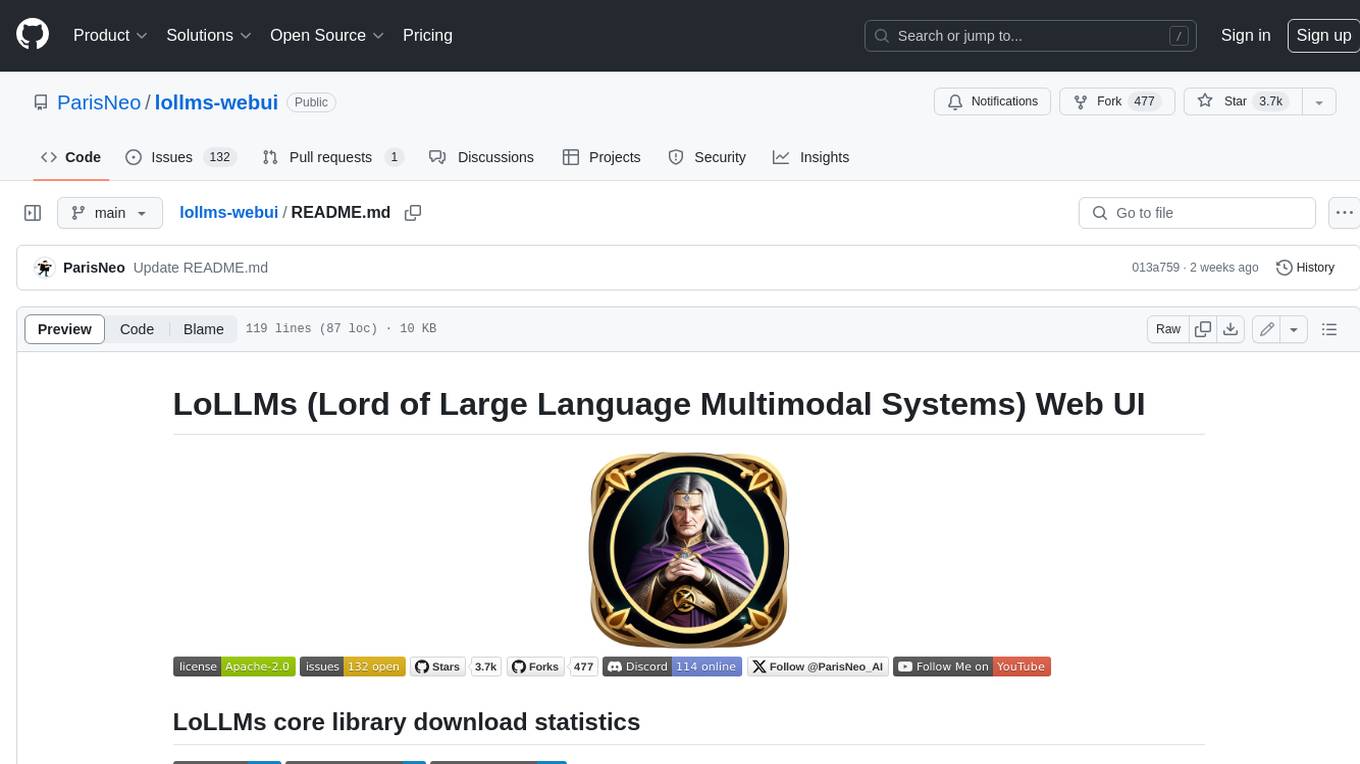
lollms-webui
LoLLMs WebUI (Lord of Large Language Multimodal Systems: One tool to rule them all) is a user-friendly interface to access and utilize various LLM (Large Language Models) and other AI models for a wide range of tasks. With over 500 AI expert conditionings across diverse domains and more than 2500 fine tuned models over multiple domains, LoLLMs WebUI provides an immediate resource for any problem, from car repair to coding assistance, legal matters, medical diagnosis, entertainment, and more. The easy-to-use UI with light and dark mode options, integration with GitHub repository, support for different personalities, and features like thumb up/down rating, copy, edit, and remove messages, local database storage, search, export, and delete multiple discussions, make LoLLMs WebUI a powerful and versatile tool.
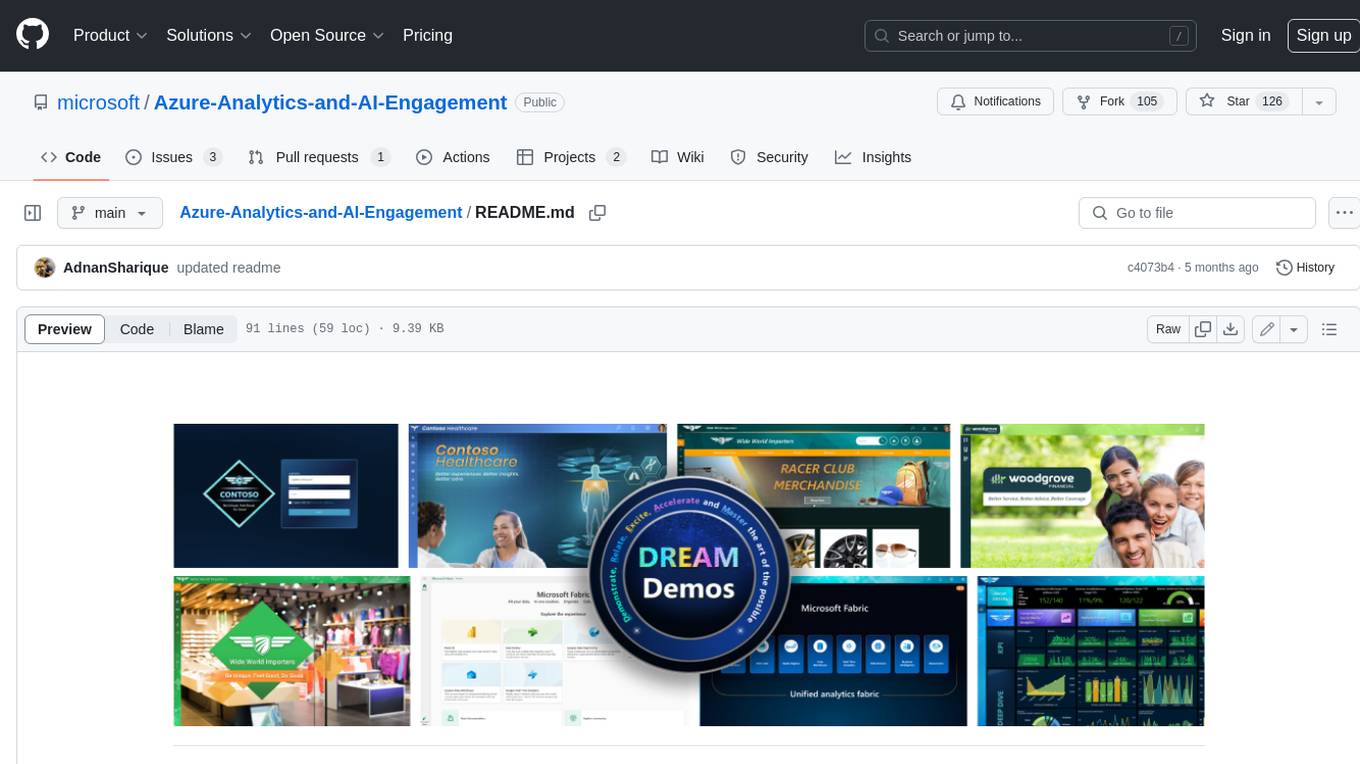
Azure-Analytics-and-AI-Engagement
The Azure-Analytics-and-AI-Engagement repository provides packaged Industry Scenario DREAM Demos with ARM templates (Containing a demo web application, Power BI reports, Synapse resources, AML Notebooks etc.) that can be deployed in a customer’s subscription using the CAPE tool within a matter of few hours. Partners can also deploy DREAM Demos in their own subscriptions using DPoC.
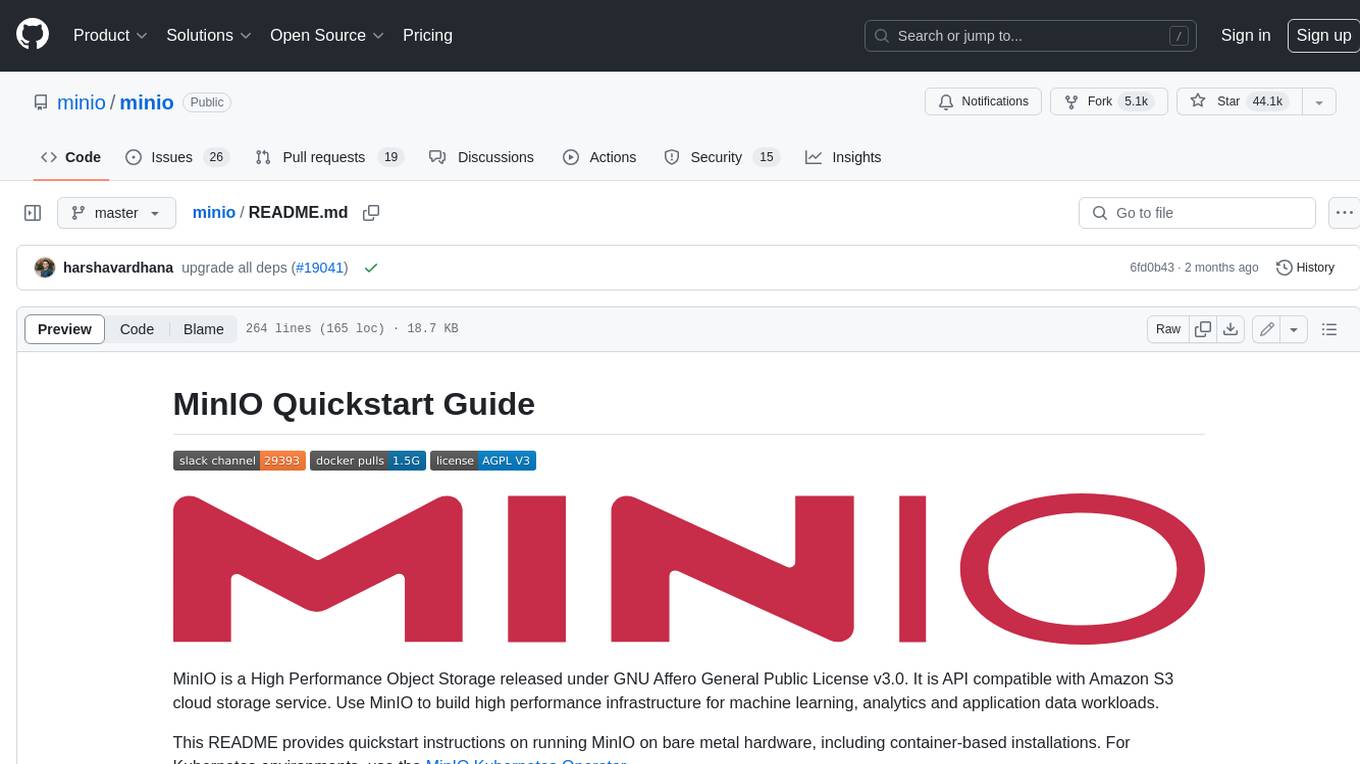
minio
MinIO is a High Performance Object Storage released under GNU Affero General Public License v3.0. It is API compatible with Amazon S3 cloud storage service. Use MinIO to build high performance infrastructure for machine learning, analytics and application data workloads.
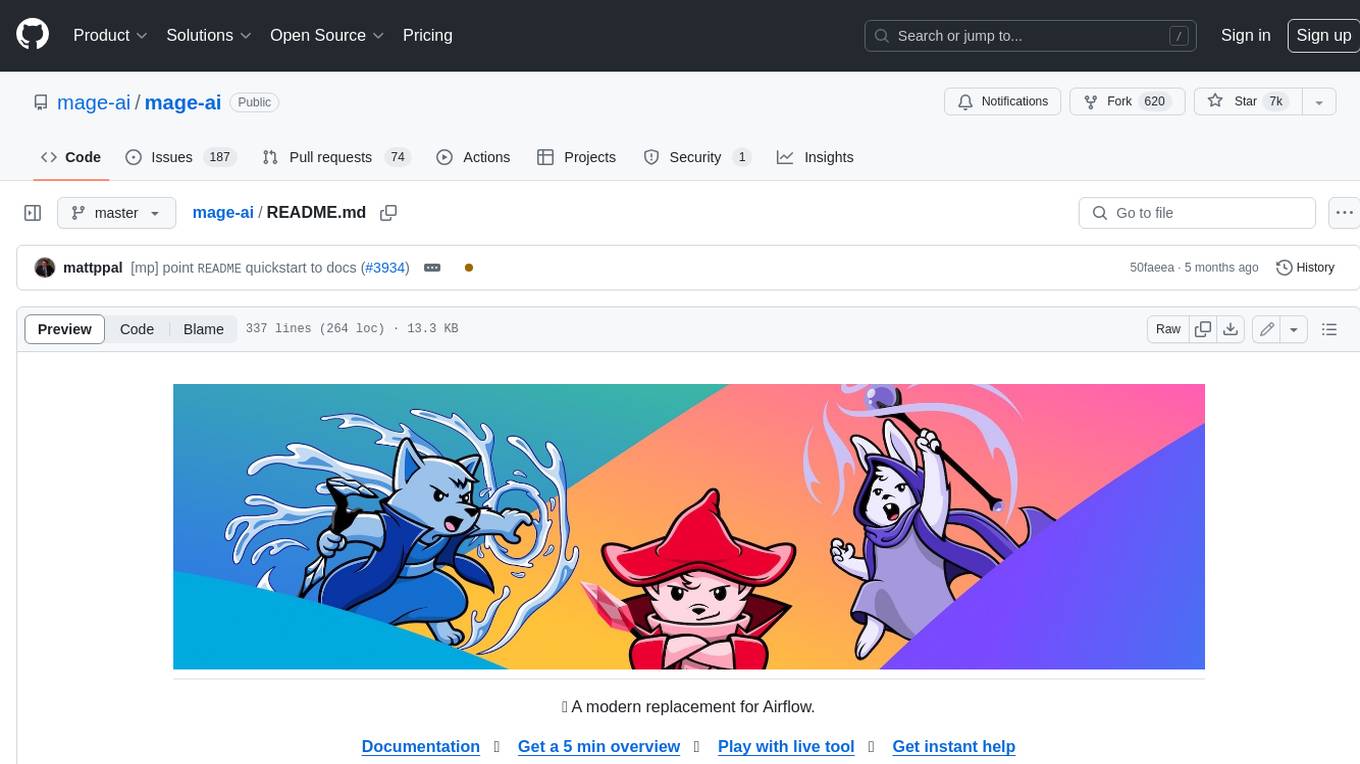
mage-ai
Mage is an open-source data pipeline tool for transforming and integrating data. It offers an easy developer experience, engineering best practices built-in, and data as a first-class citizen. Mage makes it easy to build, preview, and launch data pipelines, and provides observability and scaling capabilities. It supports data integrations, streaming pipelines, and dbt integration.
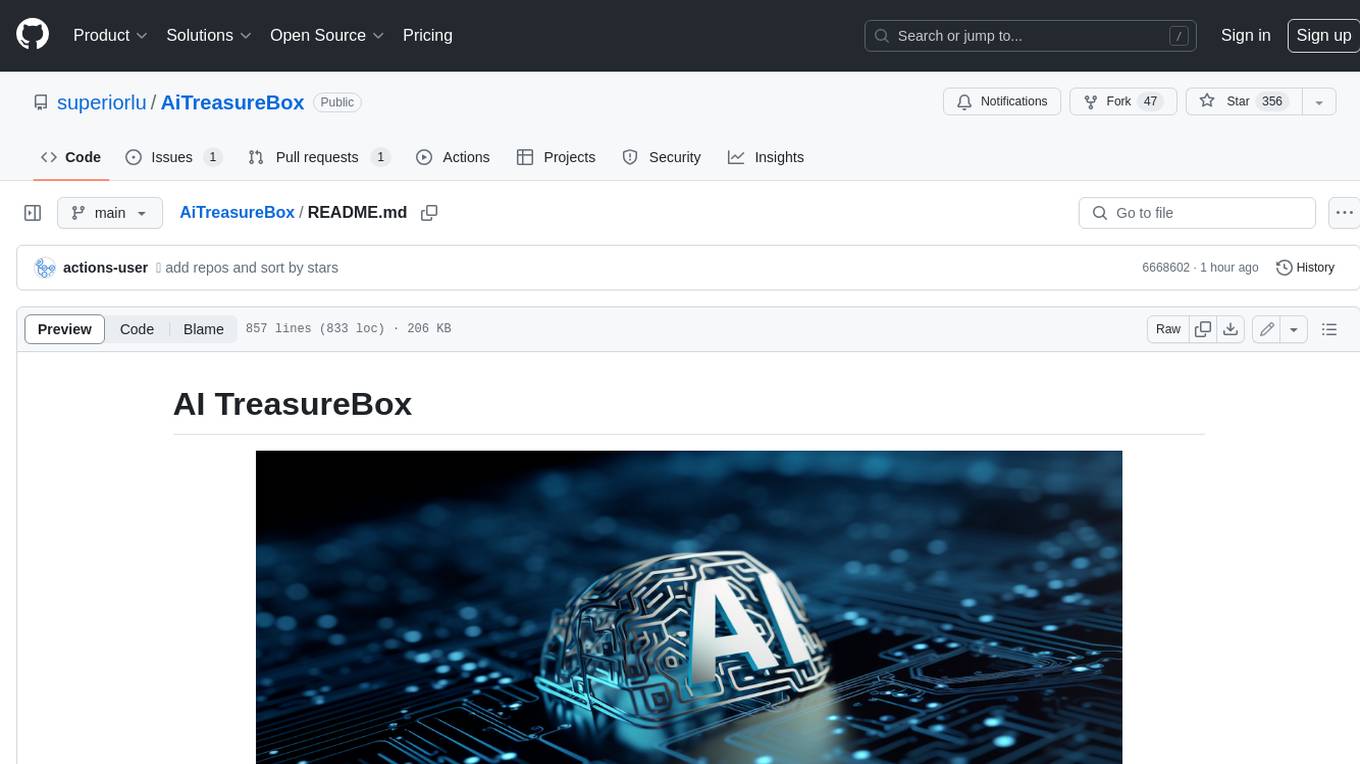
AiTreasureBox
AiTreasureBox is a versatile AI tool that provides a collection of pre-trained models and algorithms for various machine learning tasks. It simplifies the process of implementing AI solutions by offering ready-to-use components that can be easily integrated into projects. With AiTreasureBox, users can quickly prototype and deploy AI applications without the need for extensive knowledge in machine learning or deep learning. The tool covers a wide range of tasks such as image classification, text generation, sentiment analysis, object detection, and more. It is designed to be user-friendly and accessible to both beginners and experienced developers, making AI development more efficient and accessible to a wider audience.
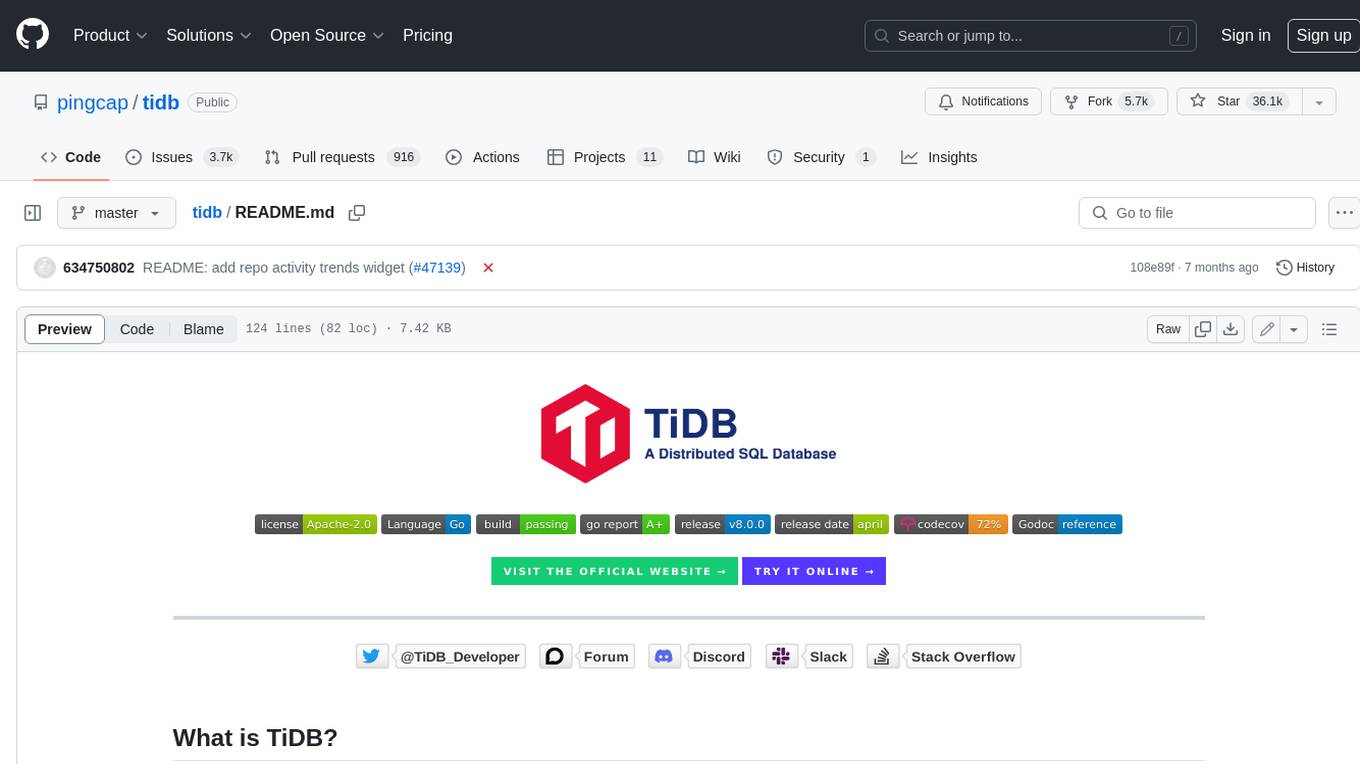
tidb
TiDB is an open-source distributed SQL database that supports Hybrid Transactional and Analytical Processing (HTAP) workloads. It is MySQL compatible and features horizontal scalability, strong consistency, and high availability.
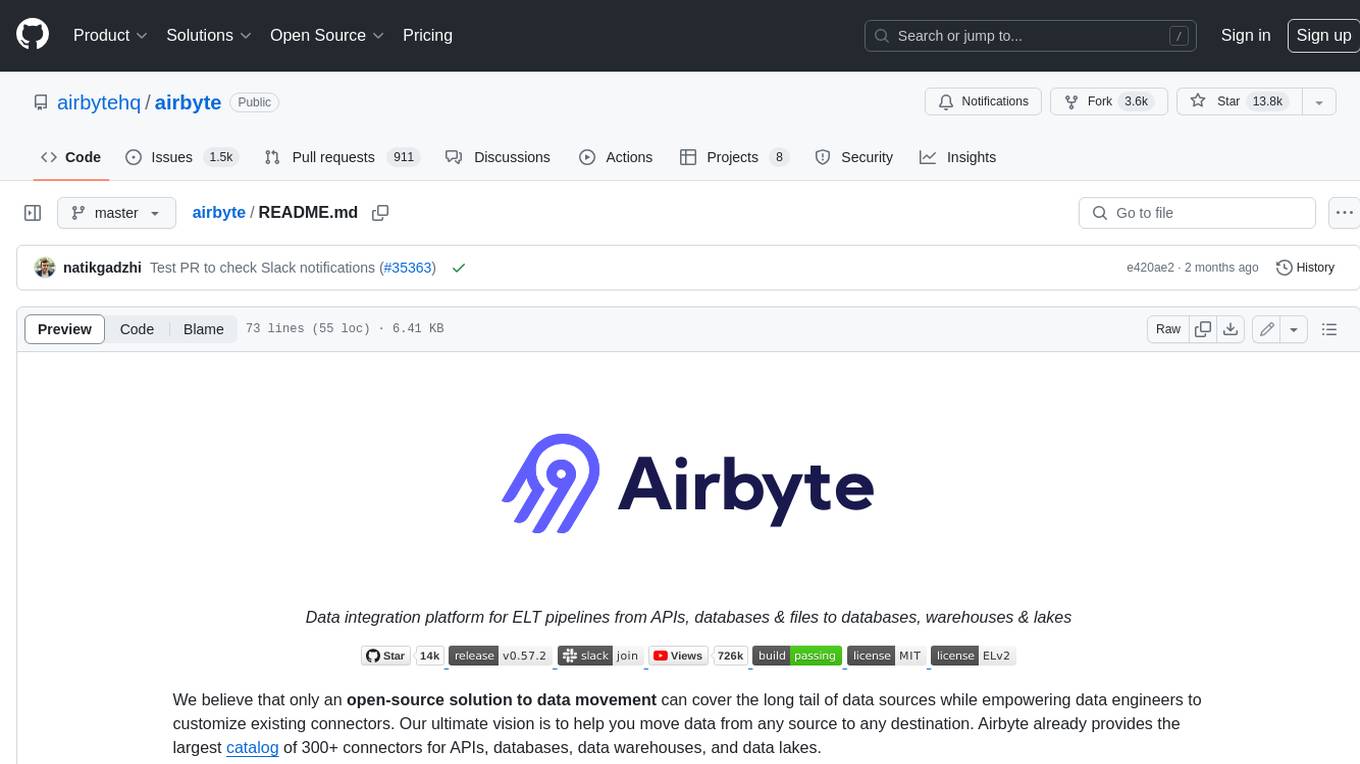
airbyte
Airbyte is an open-source data integration platform that makes it easy to move data from any source to any destination. With Airbyte, you can build and manage data pipelines without writing any code. Airbyte provides a library of pre-built connectors that make it easy to connect to popular data sources and destinations. You can also create your own connectors using Airbyte's no-code Connector Builder or low-code CDK. Airbyte is used by data engineers and analysts at companies of all sizes to build and manage their data pipelines.
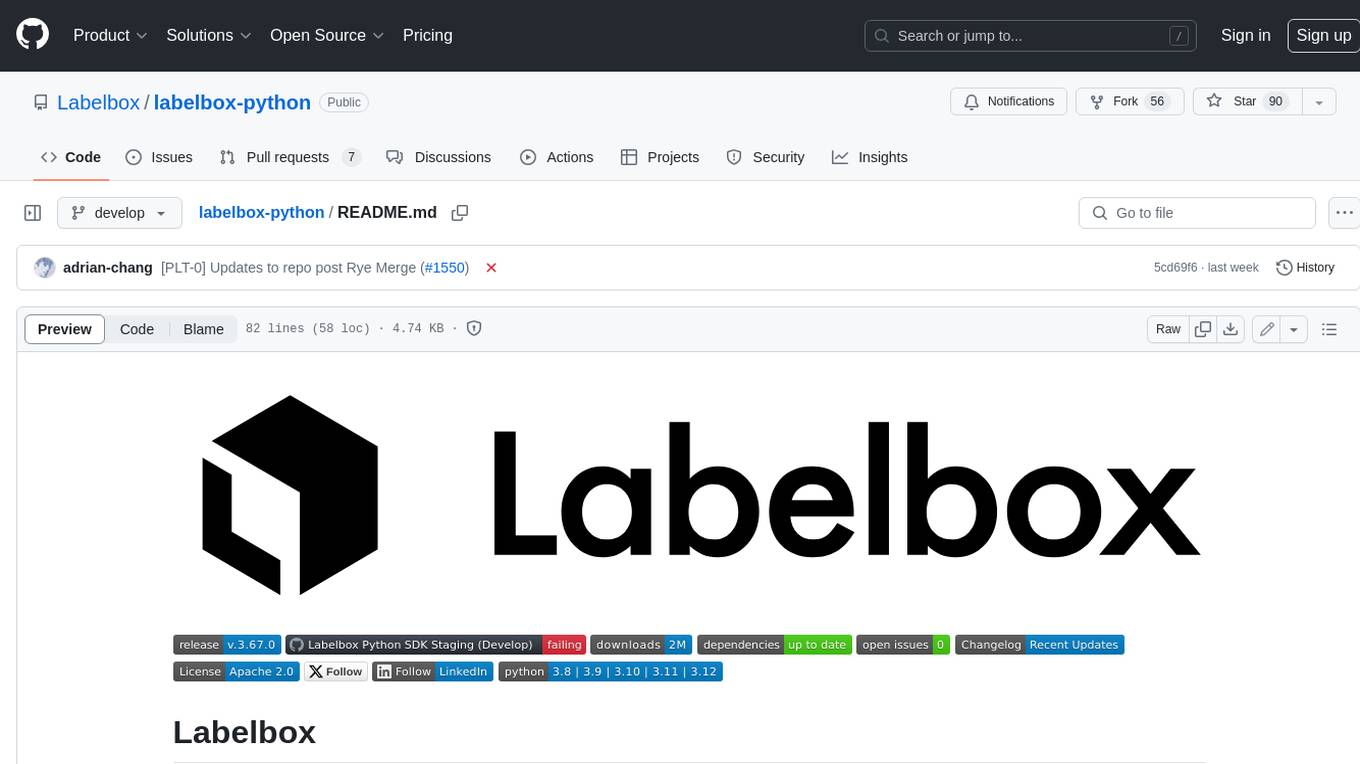
labelbox-python
Labelbox is a data-centric AI platform for enterprises to develop, optimize, and use AI to solve problems and power new products and services. Enterprises use Labelbox to curate data, generate high-quality human feedback data for computer vision and LLMs, evaluate model performance, and automate tasks by combining AI and human-centric workflows. The academic & research community uses Labelbox for cutting-edge AI research.