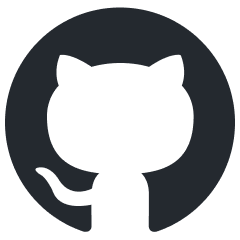
Transformers_And_LLM_Are_What_You_Dont_Need
The best repository showing why transformers might not be the answer for time series forecasting and showcasing the best SOTA non transformer models.
Stars: 644
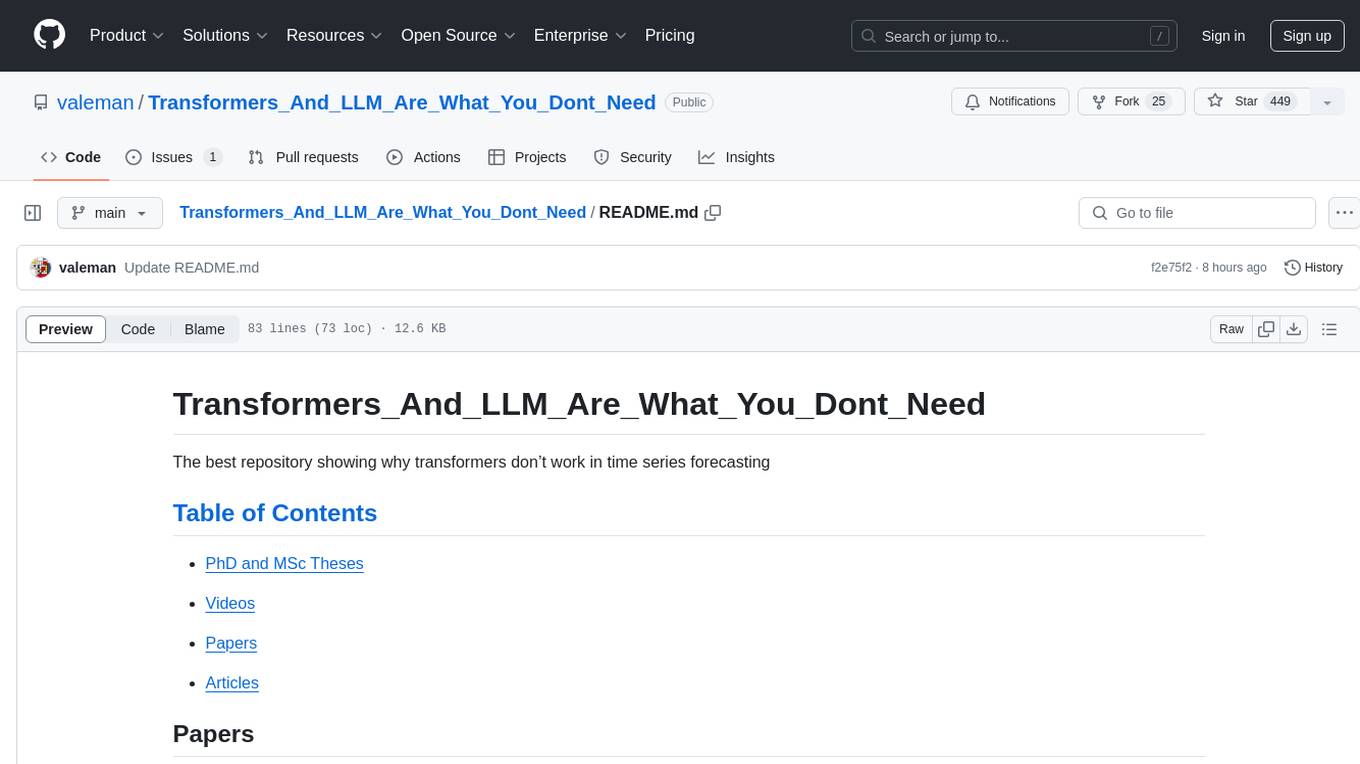
Transformers_And_LLM_Are_What_You_Dont_Need is a repository that explores the limitations of transformers in time series forecasting. It contains a collection of papers, articles, and theses discussing the effectiveness of transformers and LLMs in this domain. The repository aims to provide insights into why transformers may not be the best choice for time series forecasting tasks.
README:
The best repository showing why transformers donβt work in time series forecasting
- Problems in the current research on forecasting with transformers, foundational models, etc. by Christof Bergmeir
- Are Transformers Effective for Time Series Forecasting? by Ailing Zeng, Muxi Chen, Lei Zhang, Qiang Xu (The Chinese University of Hong Kong, International Digital Economy Academy (IDEA), 2022) code π₯π₯π₯π₯π₯
- LLMs and foundational models for time series forecasting: They are not (yet) as good as you may hope by Christoph Bergmeir (2023) π₯π₯π₯π₯π₯
- Transformers Are What You Do Not Need by Valeriy Manokhin (2023) π₯π₯π₯π₯π₯
- Time Series Foundational Models: Their Role in Anomaly Detection and Prediction (2024) code
- Deep Learning is What You Do Not Need by Valeriy Manokhin (2022) π₯π₯π₯π₯π₯
- Why do Transformers suck at Time Series Forecasting by Devansh (2023)
- Frequency-domain MLPs are More Effective Learners in Time Series Forecasting by Kun Yi, Qi Zhang, Wei Fan, Shoujin Wang, Pengyang Wang, Hui He, Defu Lian, Ning An, Longbing Cao, Zhendong Niu (Bejing Institute of Technology, Tongji University, University of Oxford, Universuty of Technology Sydney, University of Macau, HeFei University of Technology, Macquarie University) (2023) π₯π₯π₯π₯π₯
- Forecasting CPI inflation under economic policy and geo-political uncertainties by Shovon Sengupta, Tanujit Chakraborty, Sunny Kumar Singh (Fidelity Investments, Sorbonne University, BITS Pilani Hyderabad). (2024) π₯π₯π₯π₯π₯
- Revisiting Long-term Time Series Forecasting: An Investigation on Linear Mapping by Zhe Li, Shiyi Qi, Yiduo Li, Zenglin Xu (Harbin Institute of Technology, Shenzhen, 2023) code
- SCINet: Time Series Modeling and Forecasting with Sample Convolution and Interaction by Minhao Liu, Ailing Zeng, Muxi Chen, Zhijian Xu, Qiuxia Lai, Lingna Ma, Qiang Xu (The Chinese University of Hong Kong,2022) code
- WINNET:TIME SERIES FORECASTING WITH A WINDOW-ENHANCED PERIOD EXTRACTING AND INTERACTING by Wenjie Ou, Dongyue Guo, Zheng Zhang, Zhishuo Zhao, Yi Lin (Sichuan University, China, 2023)
- A Multi-Scale Decomposition MLP-Mixer for Time Series Analysis by Shuhan Zhong, Sizhe Song, Guanyao Li, Weipeng Zhuo, Yang Liu, S.-H. Gary Chan, The Hong Kong University of Science and Technology Hong Kong, 2023) code π₯π₯π₯π₯π₯
- TimesNet: Temporal 2D-Variation Modeling for General Time Series Analysis by (Haixu Wu, Tengge Hu, Yong Liu, Hang Zhou, Jianmin Wang, Mingsheng Longj, , Tsinghua University, 2023) code π₯π₯π₯π₯π₯
- MTS-Mixers: Multivariate Time Series Forecasting via Factorized Temporal and Channel Mixing code π₯π₯π₯π₯π₯
- Reversible Instance Normalization for Accurate Time-Series Forecasting against Distribution Shift by Taesung Kim, Jinhee Kim, Yunwon Tae, Cheonbok Park, Jang-Ho Choi, Jaegul Choo (Kaist AI, Vuno, Naver Corp, ETRI, ICLR 2022) code project page π₯π₯π₯π₯π₯
- WINNet: Wavelet-inspired Invertible Network for Image Denoising by Wenjie Ou, Dongyue Guo, Zheng Zhang, Zhishuo Zhao, Yi Lin (College of Computer Science, Sichuan University, China) code π₯π₯π₯π₯π₯
- Mlinear: Rethink the Linear Model for Time-series Forecasting Wei Li, Xiangxu Meng, Chuhao Chen and Jianing Chen (Harbin Engineering University, 2023) π₯π₯π₯π₯π₯
- Minimalist Traffic Prediction: Linear Layer Is All You Need by Wenying Duan, Hong Rao, Wei Huang, Xiaoxi He (Nanchang, University, Universify of Macau, 2023)
- Frequency-domain MLPs are More Effective Learners in Time Series Forecasting by Kun Yi, Qi Zhang, Wei Fan, Shoujin Wang, Pengyang Wang, Hui He, Defu Lian, Ning An, Longbing Cao, Zhendong Niu (Beijing Institute of Technology, Tongji University, University of Oxford University of Technology Sydney, University of Macau, USTC, HeFei University of Technology, Macquarie University, 2023) code π₯π₯π₯π₯π₯
- AN END-TO-END TIME SERIES MODEL FOR SIMULTANEOUS IMPUTATION AND FORECAST by Trang H. Tran, Lam M. Nguyen, Kyongmin Yeo, Nam Nguyen, Dzung Phan, Roman Vaculin Jayant Kalagnanam (School of Operations Research and Information Engineering, Cornell University; IBM Research, Thomas J. Watson Research Center, Yorktown Heights, NY, USA, 2023) π₯π₯π₯π₯π₯
- Long-term Forecasting with TiDE: Time-series Dense Encoder by Abhimanyu Das, Weihao Kong, Andrew Leach, Shaan Mathur, Rajat Sen, Rose Yu (Google Cloud, University of California, San Diego, 2023)
- TSMixer: Lightweight MLP-Mixer Model for Multivariate Time Series Forecasting by Vijay Ekambaram, Arindam Jati, Nam Nguyen, Phanwadee Sinthong, Jayant Kalagnanam (IBM Research, 2023) code code
- Koopa: Learning Non-stationary Time Series Dynamics with Koopman Predictors by Yong Liu, Chenyu Li, Jianmin Wang, Mingsheng Long (Tsinghua University, 2023) code π₯π₯π₯π₯π₯
- Attractor Memory for Long-Term Time Series Forecasting: A Chaos Perspective Jiaxi Hu, Yuehong Hu, Wei Chen, Ming Jin, Shirui Pan, Qingsong Wen, Yuxuan Liang (2024) π₯π₯π₯π₯π₯
- When and How: Learning Identifiable Latent States for Nonstationary Time Series Forecasting (2024) π₯π₯π₯π₯π₯
- Deep Coupling Network For Multivariate Time Series Forecasting (2024)
- Linear Dynamics-embedded Neural Network for Long-Sequence Modeling by Tongyi Liang and Han-Xiong Li (City University of Hong Kong, 2024).
- PDETime: Rethinking Long-Term Multivariate Time Series Forecasting from the perspective of partial differential equations (2024)
- CATS: Enhancing Multivariate Time Series Forecasting by Constructing Auxiliary Time Series as Exogenous Variables (2024) π₯π₯π₯π₯π₯
- Is Mamba Effective for Time Series Forecasting? code (2024) π₯π₯π₯π₯π₯
- STG-Mamba: Spatial-Temporal Graph Learning via Selective State Space Model (2024)
- TimeMachine: A Time Series is Worth 4 Mambas for Long-term Forecasting code (2024)π₯π₯π₯π₯π₯
- FITS: Modeling Time Series with 10k Parameters code (2023)
- TSLANet: Rethinking Transformers for Time Series Representation Learning code (2024) π₯π₯π₯π₯π₯
- WFTNet: Exploiting Global and Local Periodicity in Long-term Time Series Forecasting code (2024) π₯π₯π₯π₯π₯
- SiMBA: Simplified Mamba-based Architecture for Vision and Multivariate Time series code (2024) π₯π₯π₯π₯π₯
- SOFTS: Efficient Multivariate Time Series Forecasting with Series-Core Fusion code (2024) π₯π₯π₯π₯π₯
- Integrating Mamba and Transformer for Long-Short Range Time Series Forecasting code (2024) π₯π₯π₯π₯π₯
- SparseTSF: Modeling Long-term Time Series Forecasting with 1k Parameters (2024) π₯π₯π₯π₯π₯
- Boosting MLPs with a Coarsening Strategy for Long-Term Time Series Forecasting (2024) π₯π₯π₯π₯π₯
- Multi-Scale Dilated Convolution Network for Long-Term Time Series Forecasting (2024)
- ModernTCN: A Modern Pure Convolution Structure for General Time Series Analysis code (ICLR 2024 Spotlight)
- Adaptive Extraction Network for Multivariate Long Sequence Time-Series Forecasting (2024) π₯π₯π₯π₯π₯
- Interpretable Multivariate Time Series Forecasting Using Neural Fourier Transform (2024) π₯π₯π₯π₯π₯
- PERIODICITY DECOUPLING FRAMEWORK FOR LONG- TERM SERIES FORECASTING code (2024) π₯π₯π₯π₯π₯
- Chimera: Effectively Modeling Multivariate Time Series with 2-Dimensional State Space Models π₯π₯π₯π₯π₯ (2024)
- Time Evidence Fusion Network: Multi-source View in Long-Term Time Series Forecasting code (2024)
- ATFNet: Adaptive Time-Frequency Ensembled Network for Long-term Time Series Forecasting code (2024) π₯π₯π₯π₯
- C-Mamba: Channel Correlation Enhanced State Space Models for Multivariate Time Series Forecasting (2024) π₯π₯π₯π₯
- The Power of Minimalism in Long Sequence Time-series Forecasting
- WindowMixer: Intra-Window and Inter-Window Modeling for Time Series Forecasting
- xLSTMTime : Long-term Time Series Forecasting With xLSTM code (2024)
- Not All Frequencies Are Created Equal:Towards a Dynamic Fusion of Frequencies in Time-Series Forecasting (2024) π₯π₯π₯π₯
- FMamba: Mamba based on Fast-attention for Multivariate Time-series Forecasting (2024)
- Long Input Sequence Network for Long Time Series Forecasting (2024)
- Time-series Forecasting with Tri-Decomposition Linear-based Modelling and Series-wise Metrics (2024) π₯π₯π₯π₯
- An Evaluation of Standard Statistical Models and LLMs on Time Series Forecasting (2024) LLM π₯π₯π₯π₯
- Macroeconomic Forecasting with Large Language Models (2024) LLM π₯π₯π₯π₯
- Language Models Still Struggle to Zero-shot Reason about Time Series (2024) LLM π₯π₯π₯π₯
- KAN4TSF: Are KAN and KAN-based models Effective for Time Series Forecasting? (2024)
- Simplified Mamba with Disentangled Dependency Encoding for Long-Term Time Series Forecasting (2024)
- Transformers are Expressive, But Are They Expressive Enough for Regression? (2024) paper showing transformers cant approximate smooth functions
- MixLinear: Extreme Low Resource Multivariate Time Series Forecasting with 0.1K Parameters
- MMFNet: Multi-Scale Frequency Masking Neural Network for Multivariate Time Series Forecasting
- Neural Fourier Modelling: A Highly Compact Approach to Time-Series Analysis code
- CMMamba: channel mixing Mamba for time series forecasting
- EffiCANet: Efficient Time Series Forecasting with Convolutional Attention
- Curse of Attention: A Kernel-Based Perspective for Why Transformers Fail to Generalize on Time Series Forecasting and Beyond
- CycleNet: Enhancing Time Series Forecasting through Modeling Periodic Patterns code
- Are Language Models Actually Useful for Time Series Forecasting?
- SOFTS: Efficient Multivariate Time Series Forecasting with Series-Core Fusion code
- FTLinear: MLP based on Fourier Transform for Multivariate Time-series Forecasting
- WPMixer: Efficient Multi-Resolution Mixing for Long-Term Time Series Forecasting code
- Zero Shot Time Series Forecasting Using Kolmogorov Arnold Networks
- BEAT: Balanced Frequency Adaptive Tuning for Long-Term Time-Series Forecasting (2025) π₯π₯π₯π₯π₯
- A Multi-Task Learning Approach to Linear Multivariate Forecasting (2025)
- Benchmarking Time Series Forecasting Models: From Statistical Techniques to Foundation Models in Real-World Applications (2025)
- Day-ahead demand response potential prediction in residential buildings with HITSKAN: A fusion of Kolmogorov-Arnold networks and N-HiTS (2025)
- Do We Really Need Deep Learning Models for Time Series Forecasting? (2021)
- Two Steps Forward and One Behind: Rethinking Time Series Forecasting with Deep Learning (2023)
- Are Self-Attentions Effective for Time Series Forecasting? (2024)
- What Matters in Transformers? Not All Attention is Needed (2024)
- Time Series Foundational Models: Their Role in Anomaly Detection and Prediction (2024)
- Performance of Zero-Shot Time Series Foundation Models on Cloud Data (2025) π₯π₯π₯π₯π₯
- Position: There are no Champions in Long-Term Time Series Forecasting (2025)
- FinTSB: A Comprehensive and Practical Benchmark for Financial Time Series Forecasting
- Cherry-Picking in Time Series Forecasting: How to Select Datasets to Make Your Model Shine
- TFB: Towards Comprehensive and Fair Benchmarking of Time Series Forecasting Methods π₯π₯π₯π₯π₯
- DUET: Dual Clustering Enhanced Multivariate Time Series Forecasting
- CycleNet: Enhancing Time Series Forecasting through Modeling Periodic Patterns code
- Can LLMs Understand Time Series Anomalies? code
- EMForecaster: A Deep Learning Framework for Time Series Forecasting in Wireless Networks with Distribution-Free Uncertainty Quantification
- Times2D: Multi-Period Decomposition and Derivative Mapping for General Time Series Forecasting code
- [TimeGPT vs TiDE: Is Zero-Shot Inference the Future of Forecasting or Just Hype?](https://arxiv.org/abs/2205.13504 by LuΓs Roque and Rafael Guedes. (2024)π₯π₯π₯π₯π₯
- TimeGPT-1, discussion on Hacker News (2023)
- TimeGPT : The first Generative Pretrained Transformer for Time-Series Forecasting
For Tasks:
Click tags to check more tools for each tasksFor Jobs:
Alternative AI tools for Transformers_And_LLM_Are_What_You_Dont_Need
Similar Open Source Tools
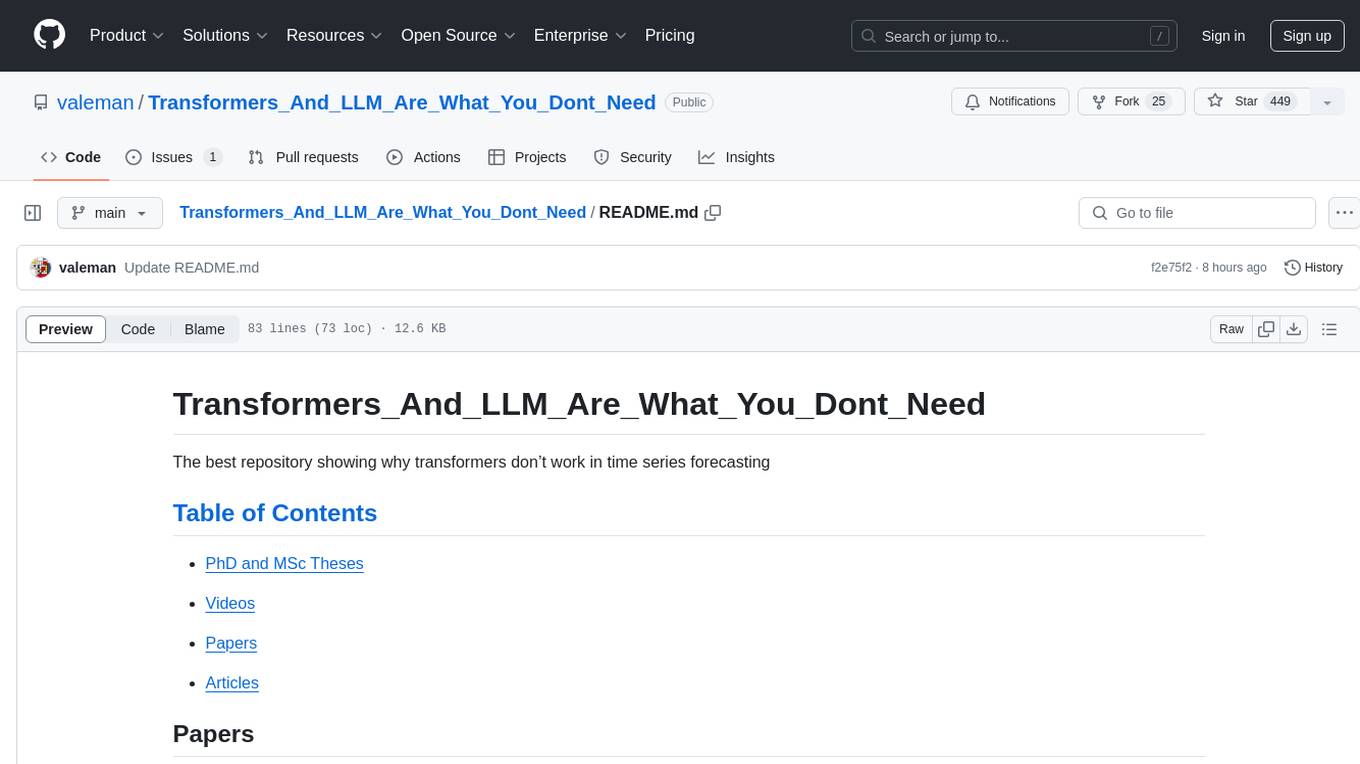
Transformers_And_LLM_Are_What_You_Dont_Need
Transformers_And_LLM_Are_What_You_Dont_Need is a repository that explores the limitations of transformers in time series forecasting. It contains a collection of papers, articles, and theses discussing the effectiveness of transformers and LLMs in this domain. The repository aims to provide insights into why transformers may not be the best choice for time series forecasting tasks.
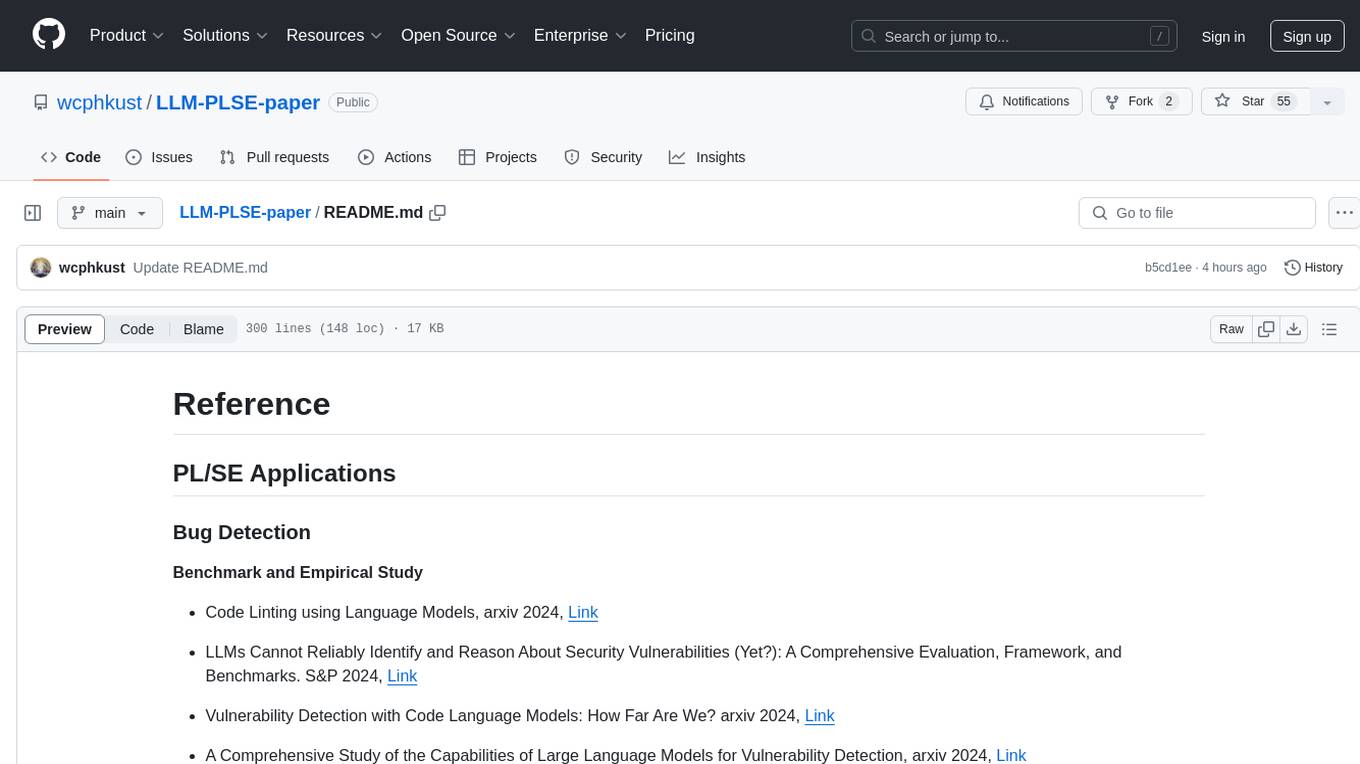
LLM-PLSE-paper
LLM-PLSE-paper is a repository focused on the applications of Large Language Models (LLMs) in Programming Language and Software Engineering (PL/SE) domains. It covers a wide range of topics including bug detection, specification inference and verification, code generation, fuzzing and testing, code model and reasoning, code understanding, IDE technologies, prompting for reasoning tasks, and agent/tool usage and planning. The repository provides a comprehensive collection of research papers, benchmarks, empirical studies, and frameworks related to the capabilities of LLMs in various PL/SE tasks.
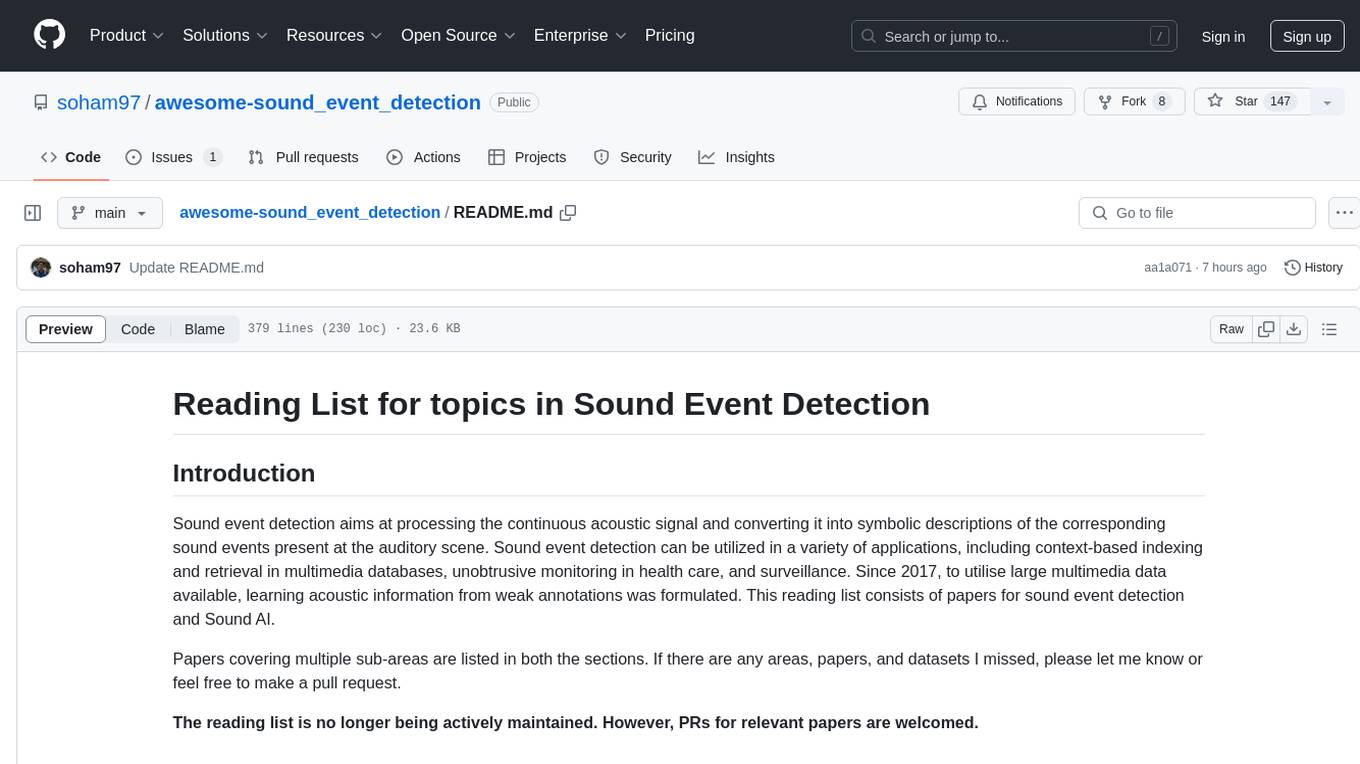
awesome-sound_event_detection
The 'awesome-sound_event_detection' repository is a curated reading list focusing on sound event detection and Sound AI. It includes research papers covering various sub-areas such as learning formulation, network architecture, pooling functions, missing or noisy audio, data augmentation, representation learning, multi-task learning, few-shot learning, zero-shot learning, knowledge transfer, polyphonic sound event detection, loss functions, audio and visual tasks, audio captioning, audio retrieval, audio generation, and more. The repository provides a comprehensive collection of papers, datasets, and resources related to sound event detection and Sound AI, making it a valuable reference for researchers and practitioners in the field.
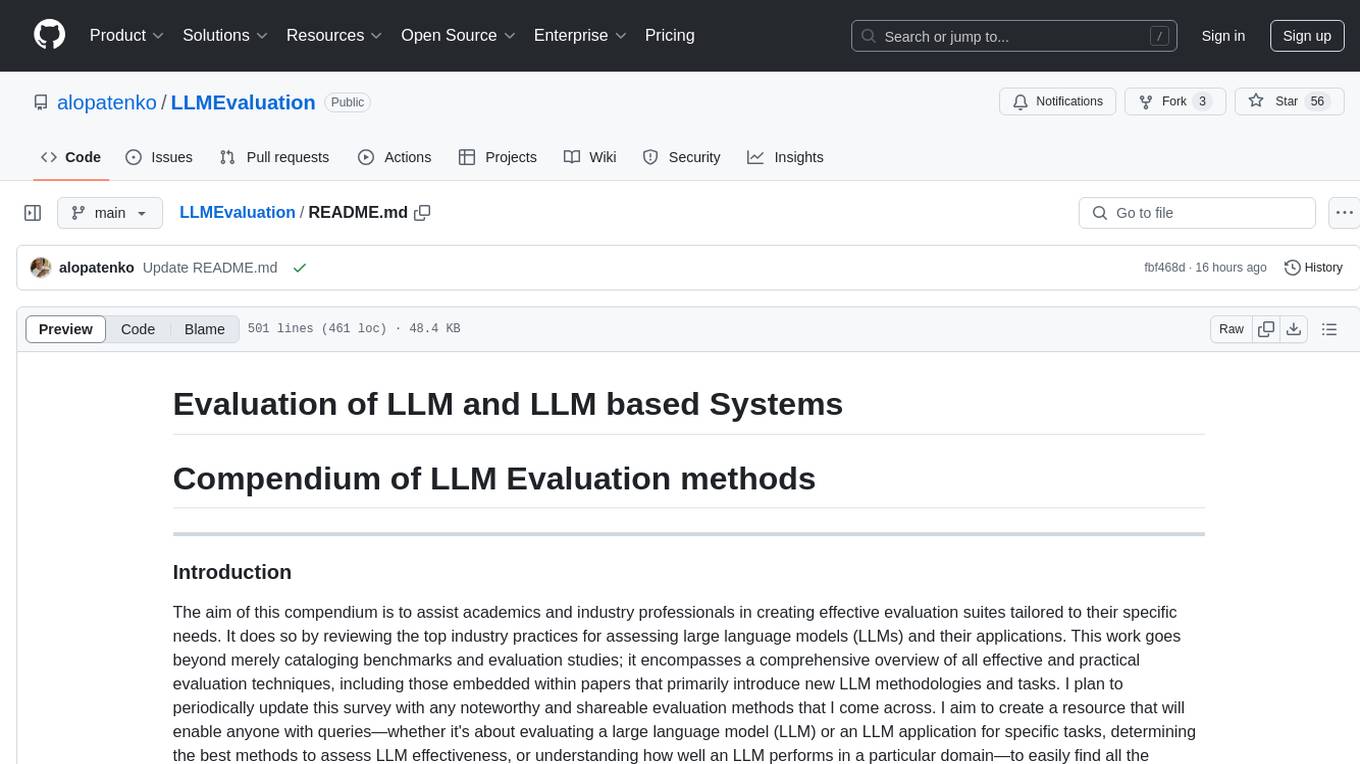
LLMEvaluation
The LLMEvaluation repository is a comprehensive compendium of evaluation methods for Large Language Models (LLMs) and LLM-based systems. It aims to assist academics and industry professionals in creating effective evaluation suites tailored to their specific needs by reviewing industry practices for assessing LLMs and their applications. The repository covers a wide range of evaluation techniques, benchmarks, and studies related to LLMs, including areas such as embeddings, question answering, multi-turn dialogues, reasoning, multi-lingual tasks, ethical AI, biases, safe AI, code generation, summarization, software performance, agent LLM architectures, long text generation, graph understanding, and various unclassified tasks. It also includes evaluations for LLM systems in conversational systems, copilots, search and recommendation engines, task utility, and verticals like healthcare, law, science, financial, and others. The repository provides a wealth of resources for evaluating and understanding the capabilities of LLMs in different domains.
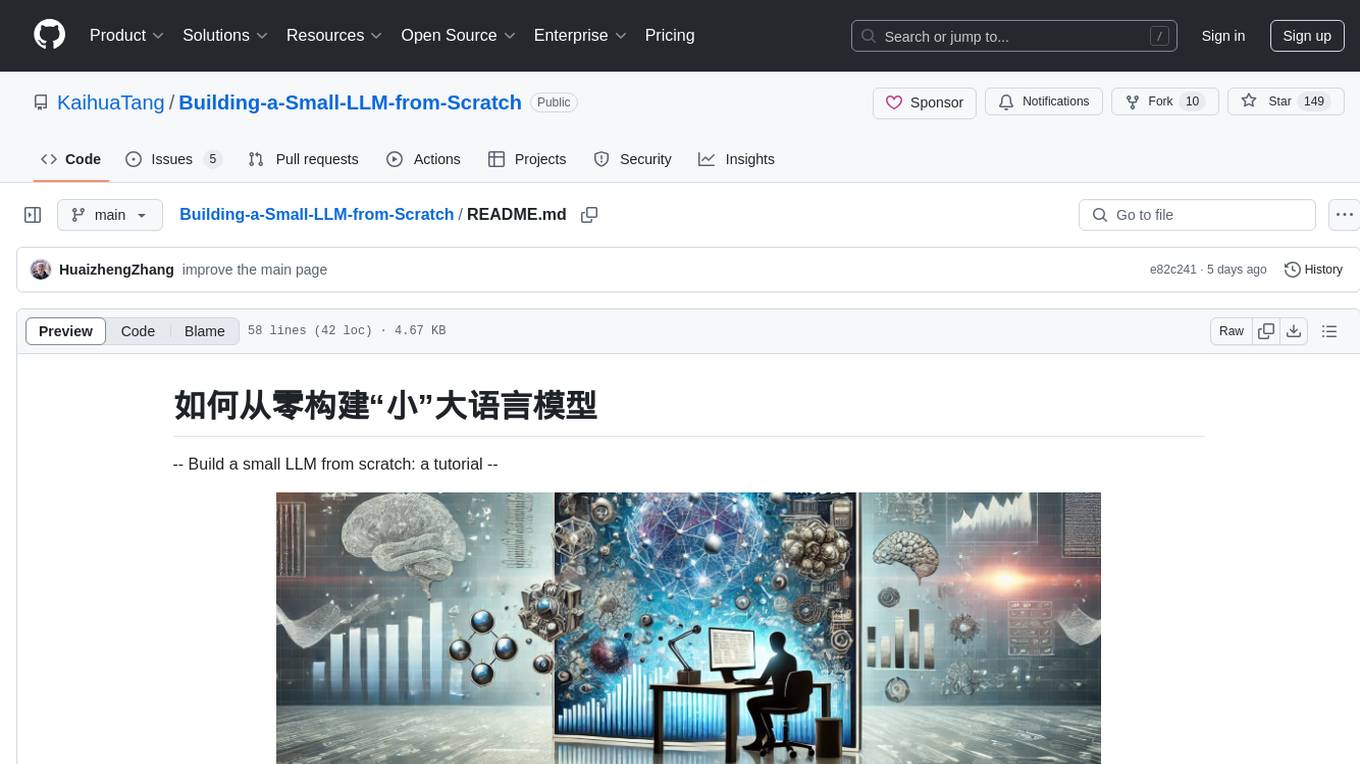
Building-a-Small-LLM-from-Scratch
This tutorial provides a comprehensive guide on building a small Large Language Model (LLM) from scratch using PyTorch. The author shares insights and experiences gained from working on LLM projects in the industry, aiming to help beginners understand the fundamental components of LLMs and training fine-tuning codes. The tutorial covers topics such as model structure overview, attention modules, optimization techniques, normalization layers, tokenizers, pretraining, and fine-tuning with dialogue data. It also addresses specific industry-related challenges and explores cutting-edge model concepts like DeepSeek network structure, causal attention, dynamic-to-static tensor conversion for ONNX inference, and performance optimizations for NPU chips. The series emphasizes hands-on practice with small models to enable local execution and plans to expand into multimodal language models and tensor parallel multi-card deployment.
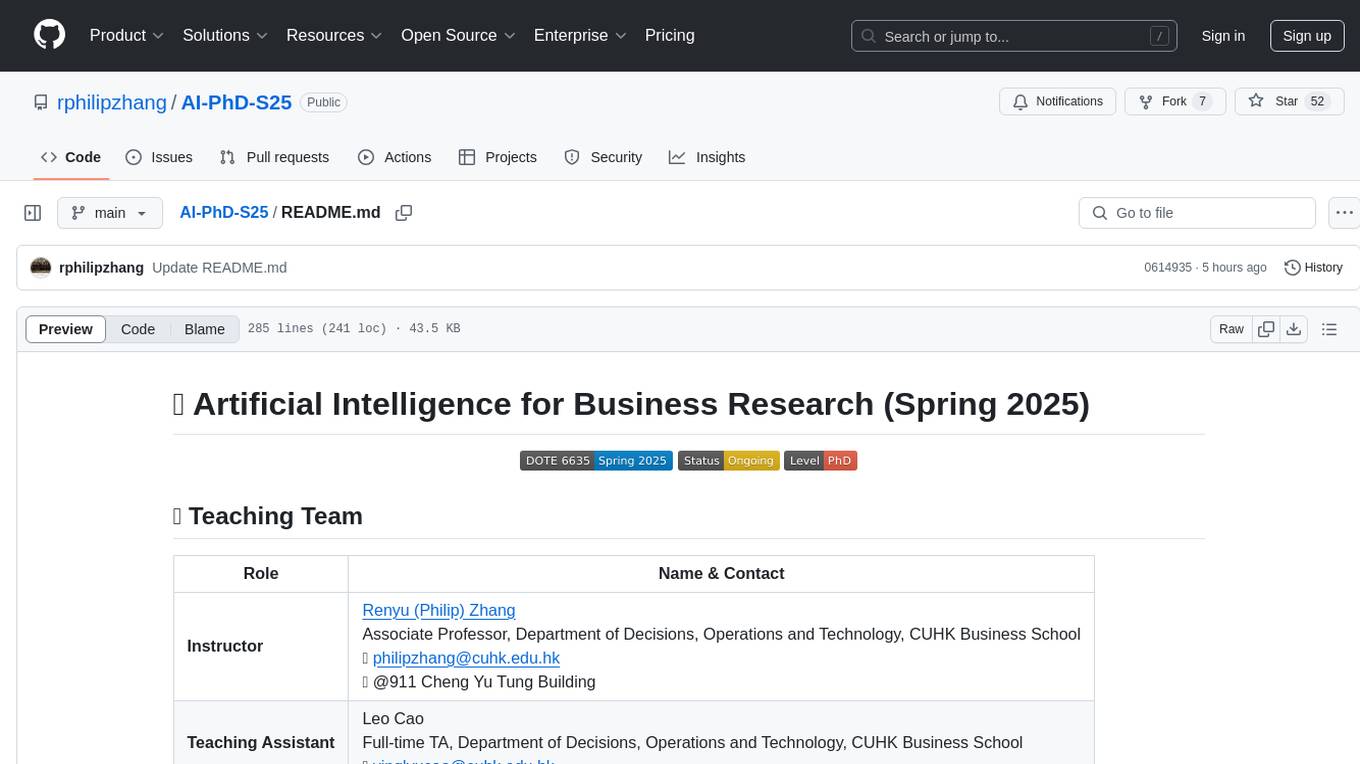
AI-PhD-S25
AI-PhD-S25 is a mono-repo for the DOTE 6635 course on AI for Business Research at CUHK Business School. The course aims to provide a fundamental understanding of ML/AI concepts and methods relevant to business research, explore applications of ML/AI in business research, and discover cutting-edge AI/ML technologies. The course resources include Google CoLab for code distribution, Jupyter Notebooks, Google Sheets for group tasks, Overleaf template for lecture notes, replication projects, and access to HPC Server compute resource. The course covers topics like AI/ML in business research, deep learning basics, attention mechanisms, transformer models, LLM pretraining, posttraining, causal inference fundamentals, and more.
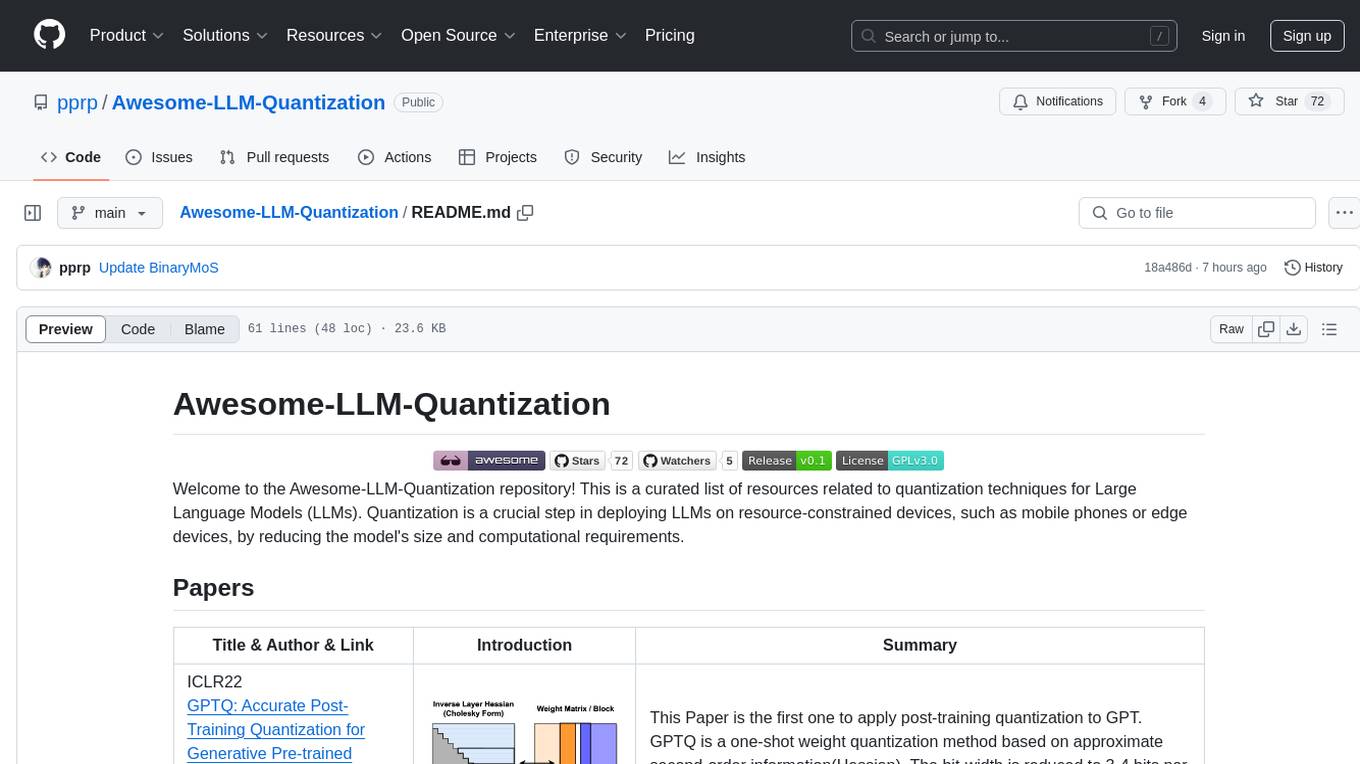
Awesome-LLM-Quantization
Awesome-LLM-Quantization is a curated list of resources related to quantization techniques for Large Language Models (LLMs). Quantization is a crucial step in deploying LLMs on resource-constrained devices, such as mobile phones or edge devices, by reducing the model's size and computational requirements.
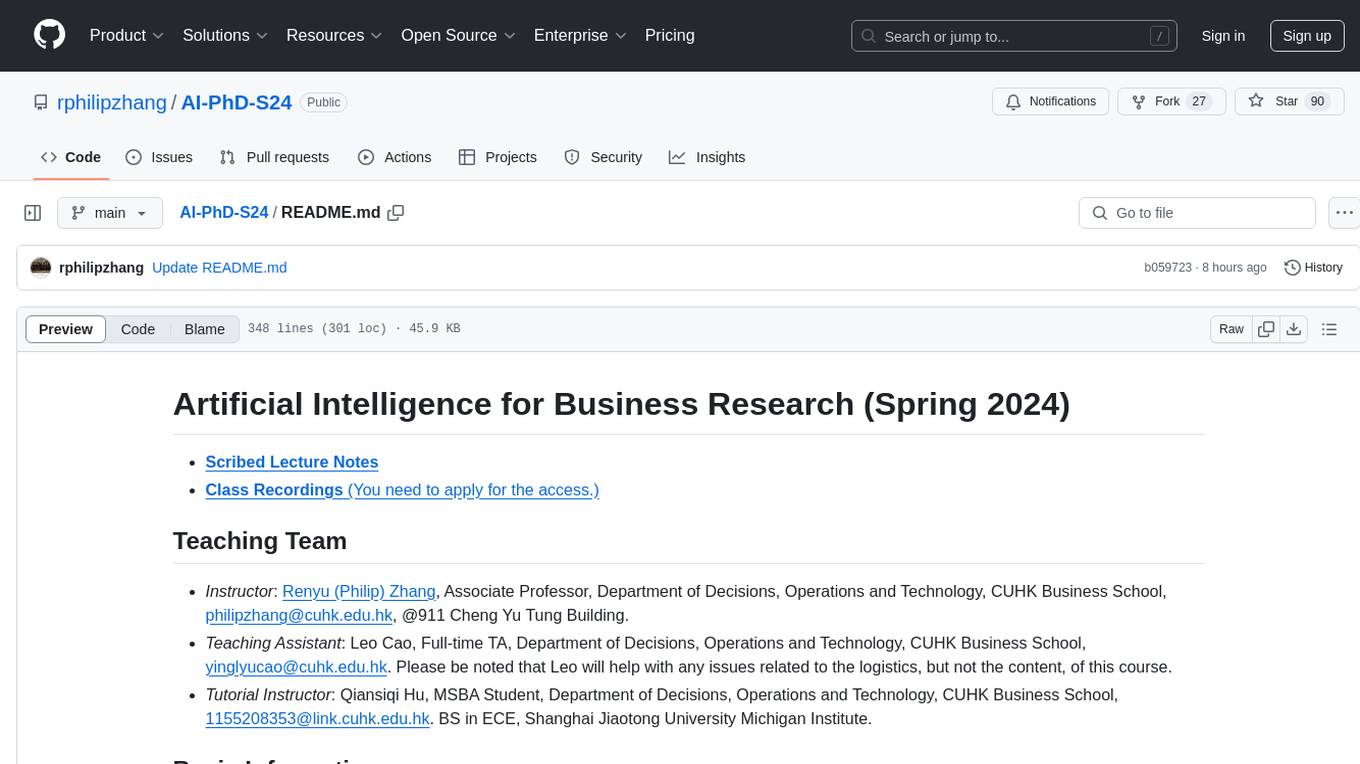
AI-PhD-S24
AI-PhD-S24 is a mono-repo for the PhD course 'AI for Business Research' at CUHK Business School in Spring 2024. The course aims to provide a basic understanding of machine learning and artificial intelligence concepts/methods used in business research, showcase how ML/AI is utilized in business research, and introduce state-of-the-art AI/ML technologies. The course includes scribed lecture notes, class recordings, and covers topics like AI/ML fundamentals, DL, NLP, CV, unsupervised learning, and diffusion models.
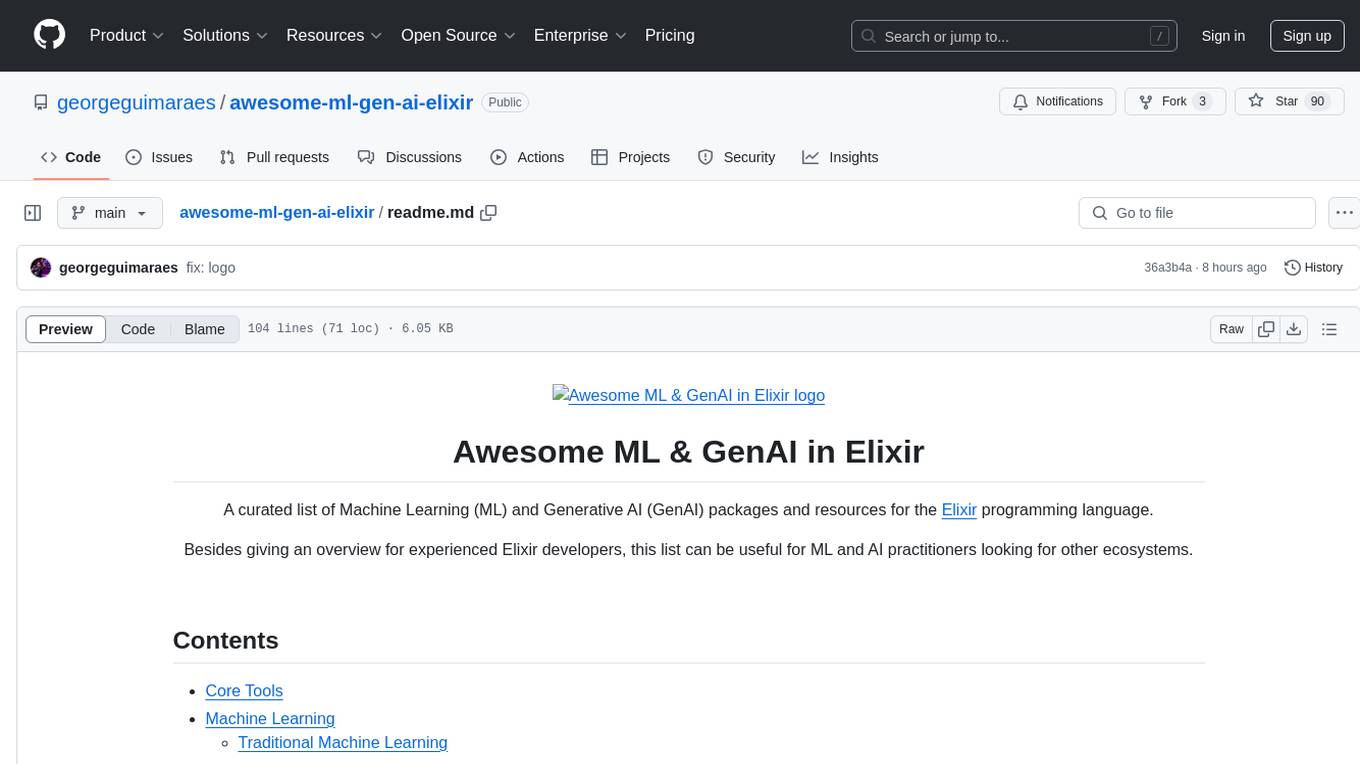
awesome-ml-gen-ai-elixir
A curated list of Machine Learning (ML) and Generative AI (GenAI) packages and resources for the Elixir programming language. It includes core tools for data exploration, traditional machine learning algorithms, deep learning models, computer vision libraries, generative AI tools, livebooks for interactive notebooks, and various resources such as books, videos, and articles. The repository aims to provide a comprehensive overview for experienced Elixir developers and ML/AI practitioners exploring different ecosystems.
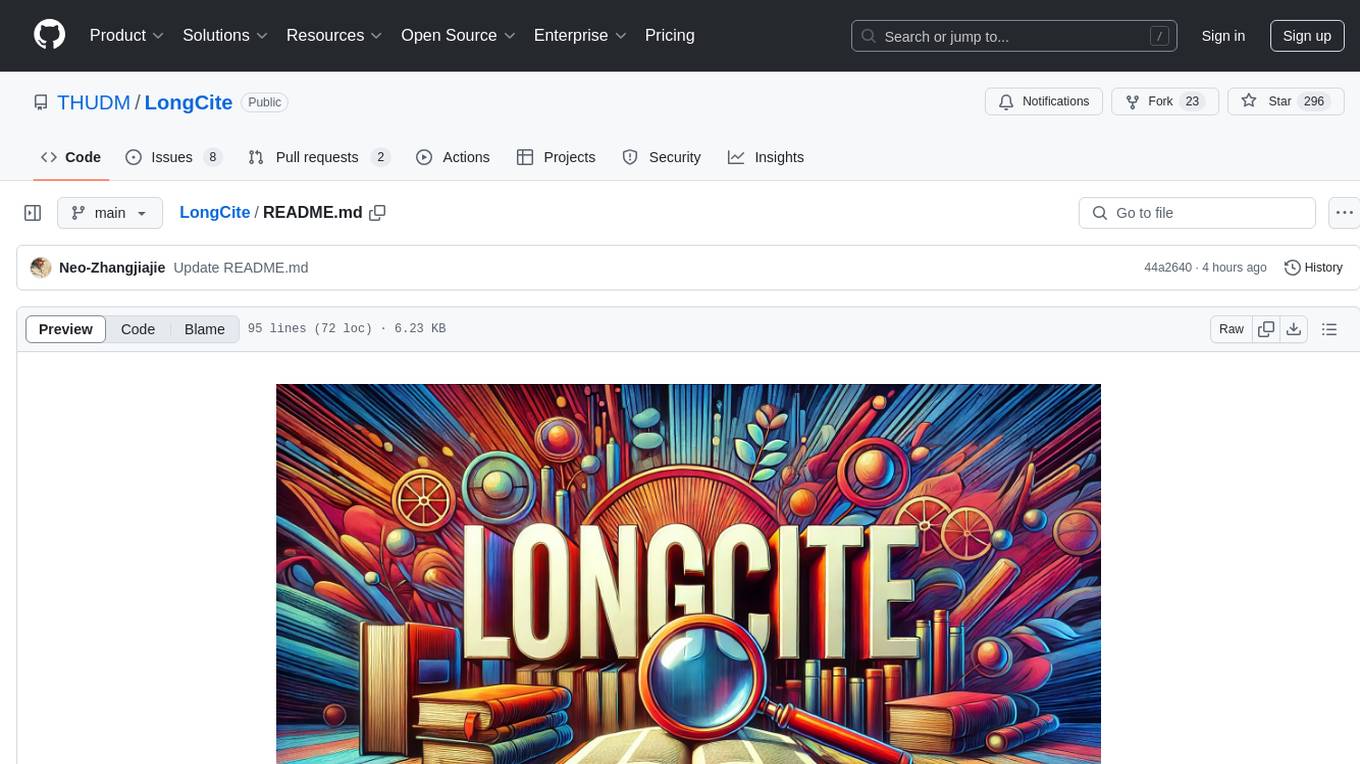
LongCite
LongCite is a tool that enables Large Language Models (LLMs) to generate fine-grained citations in long-context Question Answering (QA) scenarios. It provides models trained on GLM-4-9B and Meta-Llama-3.1-8B, supporting up to 128K context. Users can deploy LongCite chatbots, generate accurate responses, and obtain precise sentence-level citations. The tool includes components for model deployment, Coarse to Fine (CoF) pipeline for data construction, model training using LongCite-45k dataset, evaluation with LongBench-Cite benchmark, and citation generation.
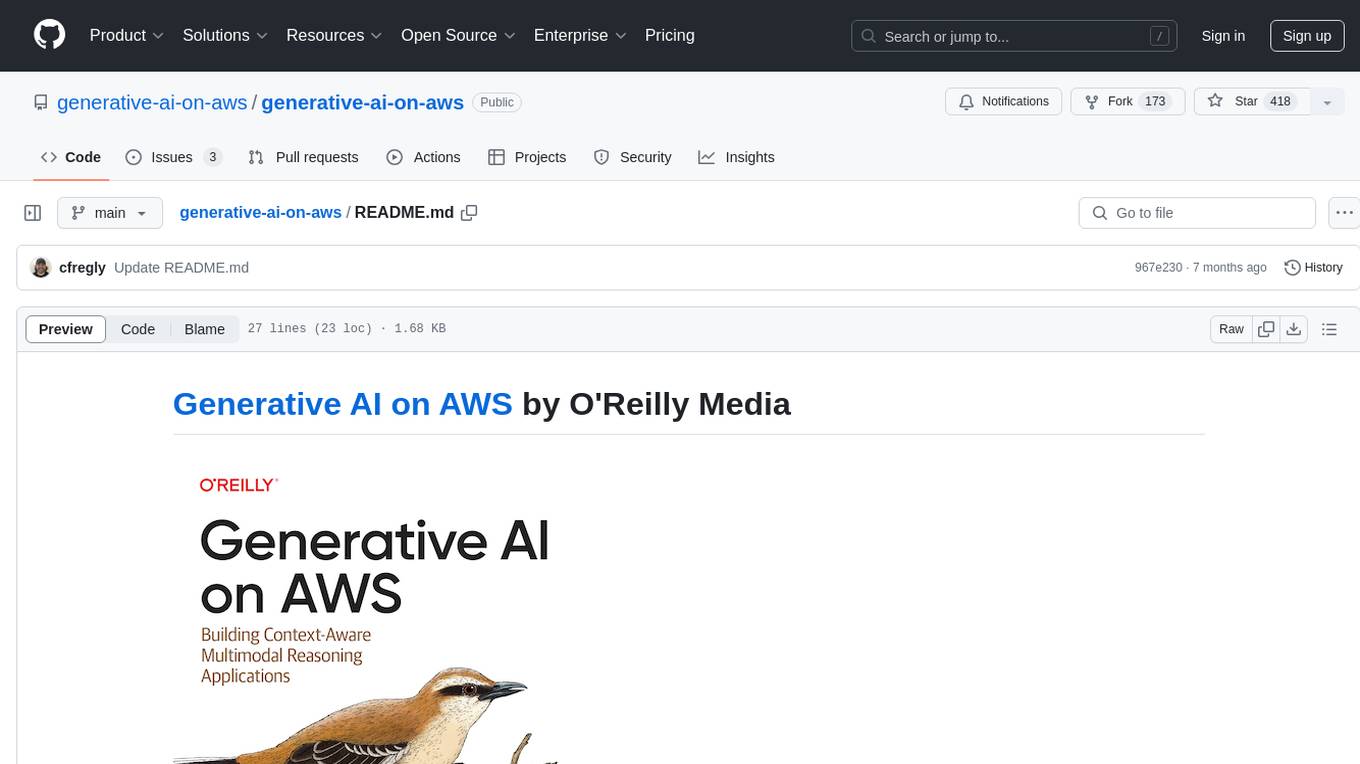
generative-ai-on-aws
Generative AI on AWS by O'Reilly Media provides a comprehensive guide on leveraging generative AI models on the AWS platform. The book covers various topics such as generative AI use cases, prompt engineering, large-language models, fine-tuning techniques, optimization, deployment, and more. Authors Chris Fregly, Antje Barth, and Shelbee Eigenbrode offer insights into cutting-edge AI technologies and practical applications in the field. The book is a valuable resource for data scientists, AI enthusiasts, and professionals looking to explore generative AI capabilities on AWS.
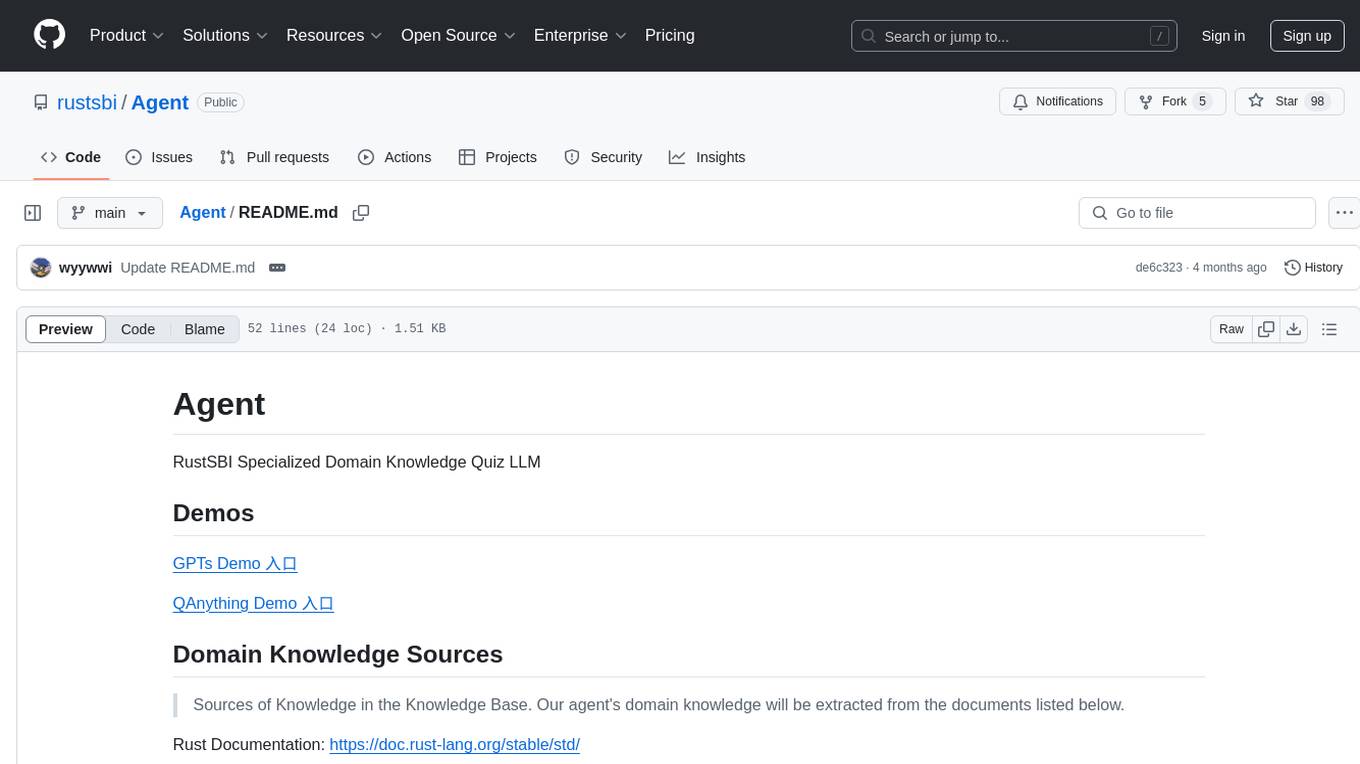
Agent
Agent is a RustSBI specialized domain knowledge quiz LLM tool that extracts domain knowledge from various sources such as Rust Documentation, RISC-V Documentation, Bouffalo Docs, Bouffalo SDK, and Xiangshan Docs. It also provides resources for LLM prompt engineering and RAG engineering, including guides and existing projects related to retrieval-augmented generation (RAG) systems.
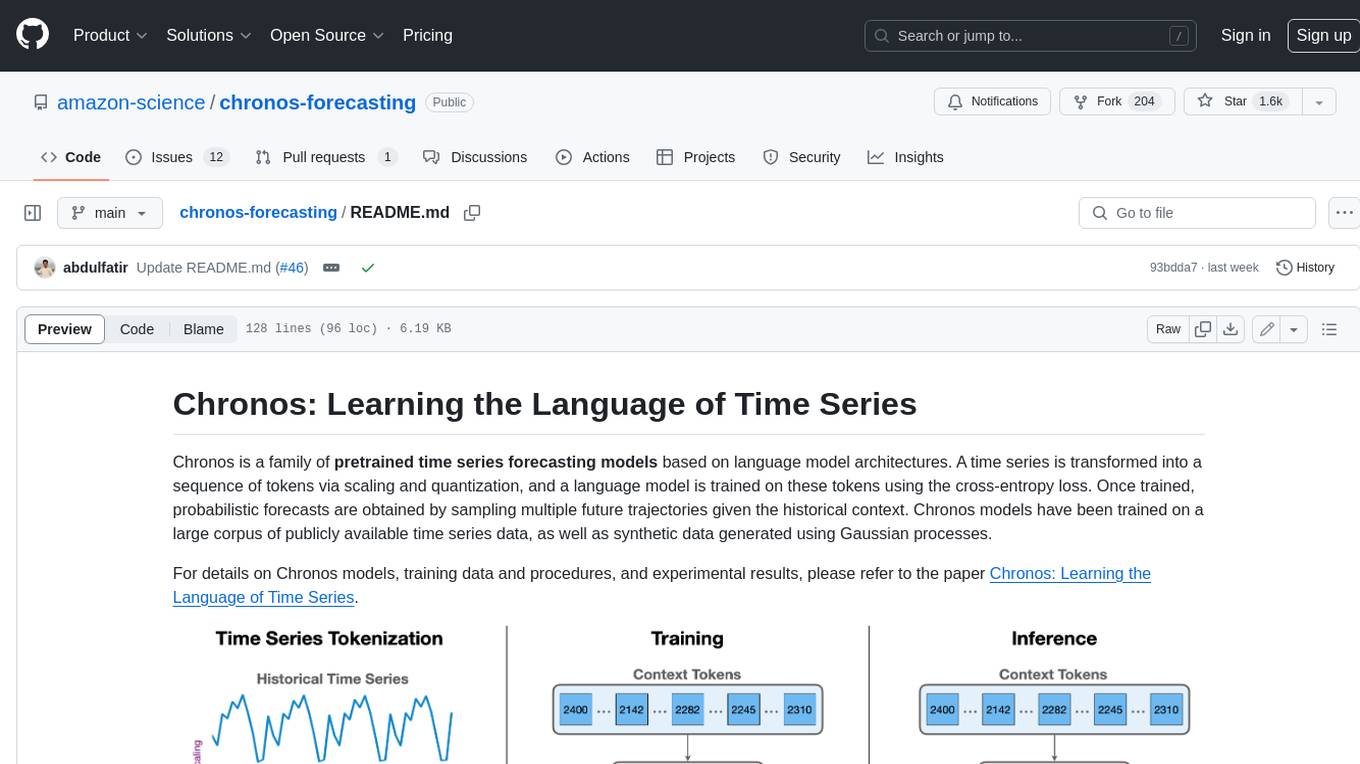
chronos-forecasting
Chronos is a family of pretrained time series forecasting models based on language model architectures. A time series is transformed into a sequence of tokens via scaling and quantization, and a language model is trained on these tokens using the cross-entropy loss. Once trained, probabilistic forecasts are obtained by sampling multiple future trajectories given the historical context. Chronos models have been trained on a large corpus of publicly available time series data, as well as synthetic data generated using Gaussian processes.
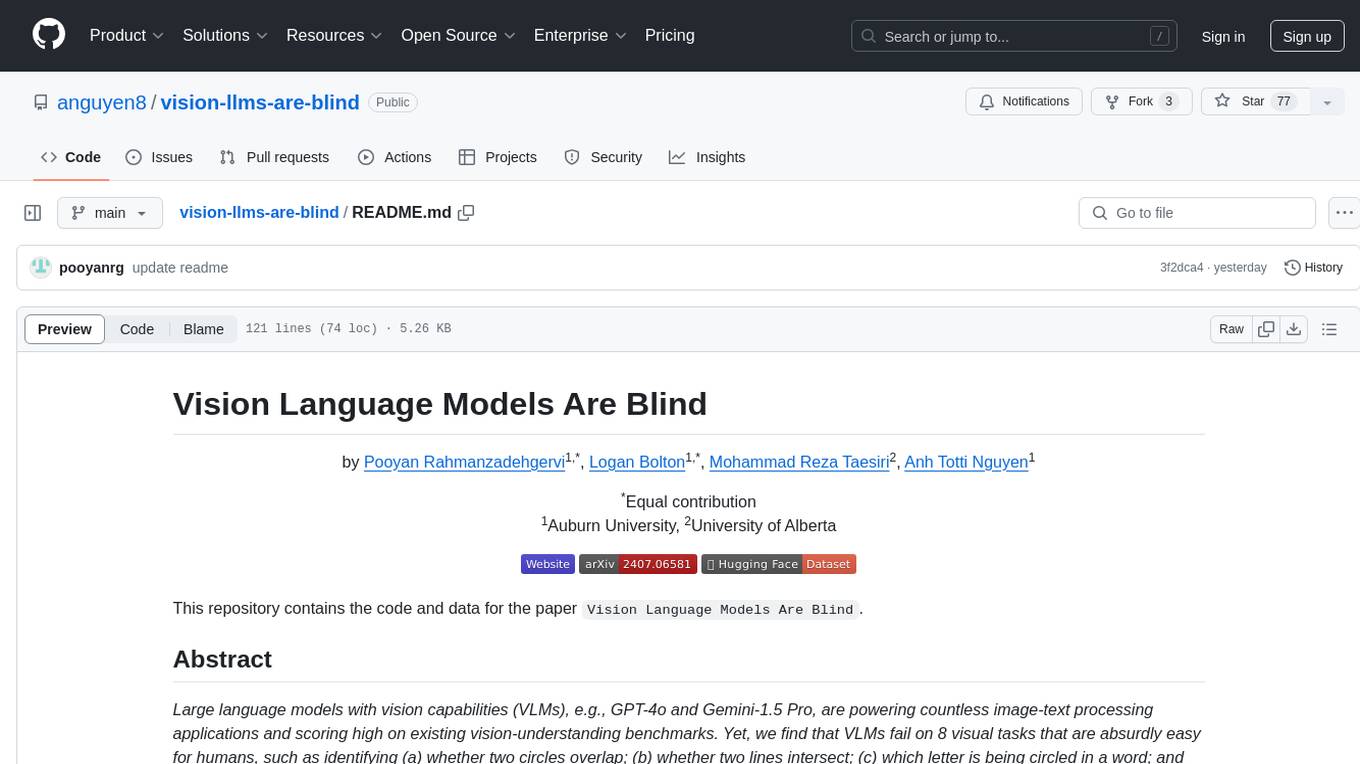
vision-llms-are-blind
This repository contains the code and data for the paper 'Vision Language Models Are Blind'. It explores the limitations of large language models with vision capabilities (VLMs) in performing basic visual tasks that are easy for humans. The repository presents benchmark results showcasing the poor performance of state-of-the-art VLMs on tasks like counting line intersections, identifying circles, letters, and shapes, and following color-coded paths. The research highlights the challenges faced by VLMs in understanding visual information accurately, drawing parallels to myopia and blindness in human vision.
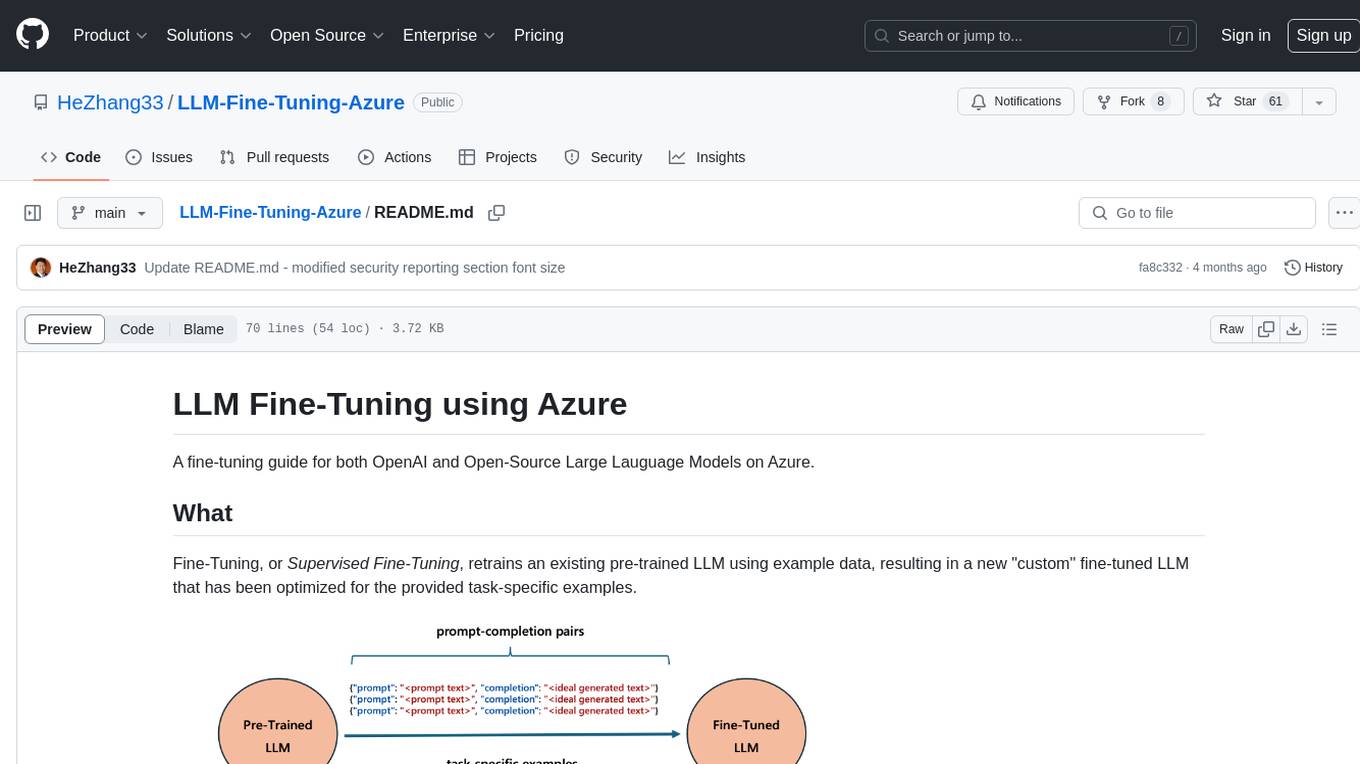
LLM-Fine-Tuning-Azure
A fine-tuning guide for both OpenAI and Open-Source Large Language Models on Azure. Fine-Tuning retrains an existing pre-trained LLM using example data, resulting in a new 'custom' fine-tuned LLM optimized for task-specific examples. Use cases include improving LLM performance on specific tasks and introducing information not well represented by the base LLM model. Suitable for cases where latency is critical, high accuracy is required, and clear evaluation metrics are available. Learning path includes labs for fine-tuning GPT and Llama2 models via Dashboards and Python SDK.
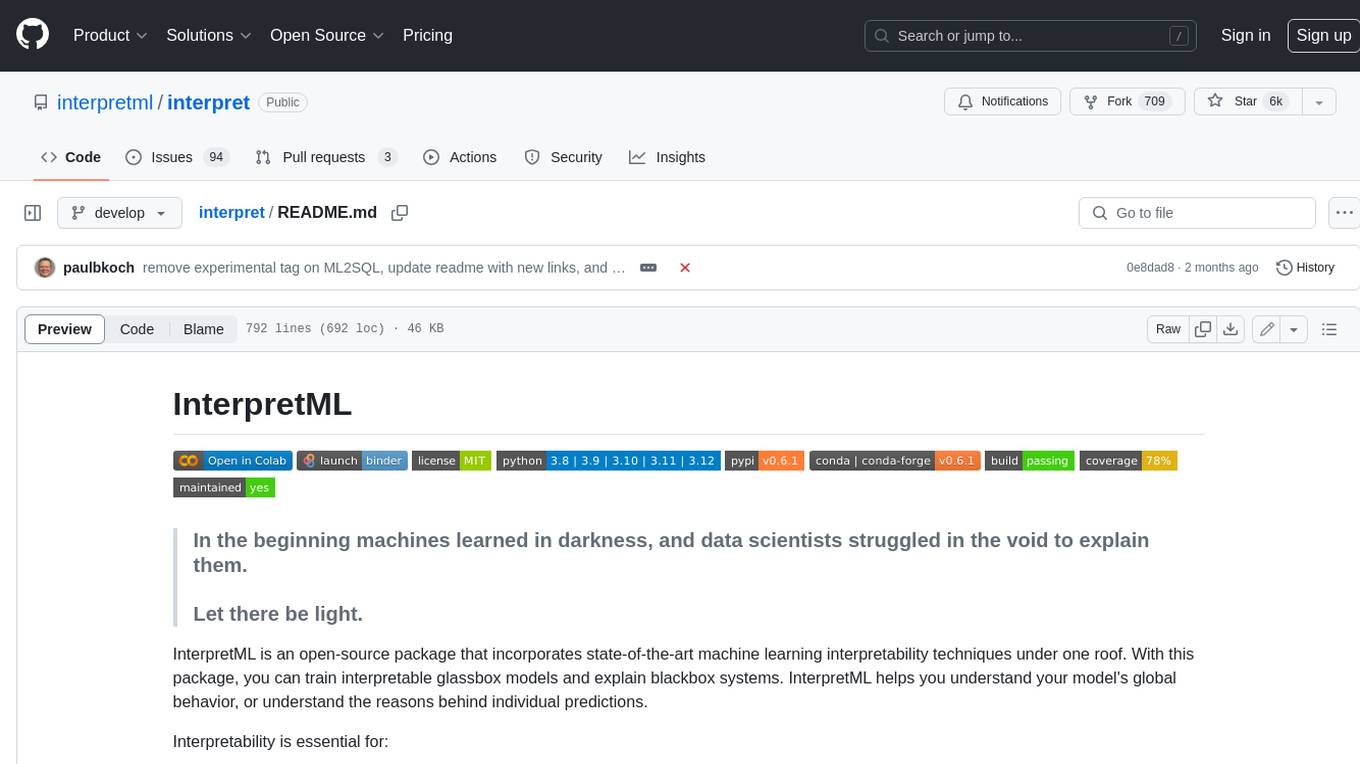
interpret
InterpretML is an open-source package that incorporates state-of-the-art machine learning interpretability techniques under one roof. With this package, you can train interpretable glassbox models and explain blackbox systems. InterpretML helps you understand your model's global behavior, or understand the reasons behind individual predictions. Interpretability is essential for: - Model debugging - Why did my model make this mistake? - Feature Engineering - How can I improve my model? - Detecting fairness issues - Does my model discriminate? - Human-AI cooperation - How can I understand and trust the model's decisions? - Regulatory compliance - Does my model satisfy legal requirements? - High-risk applications - Healthcare, finance, judicial, ...
For similar tasks
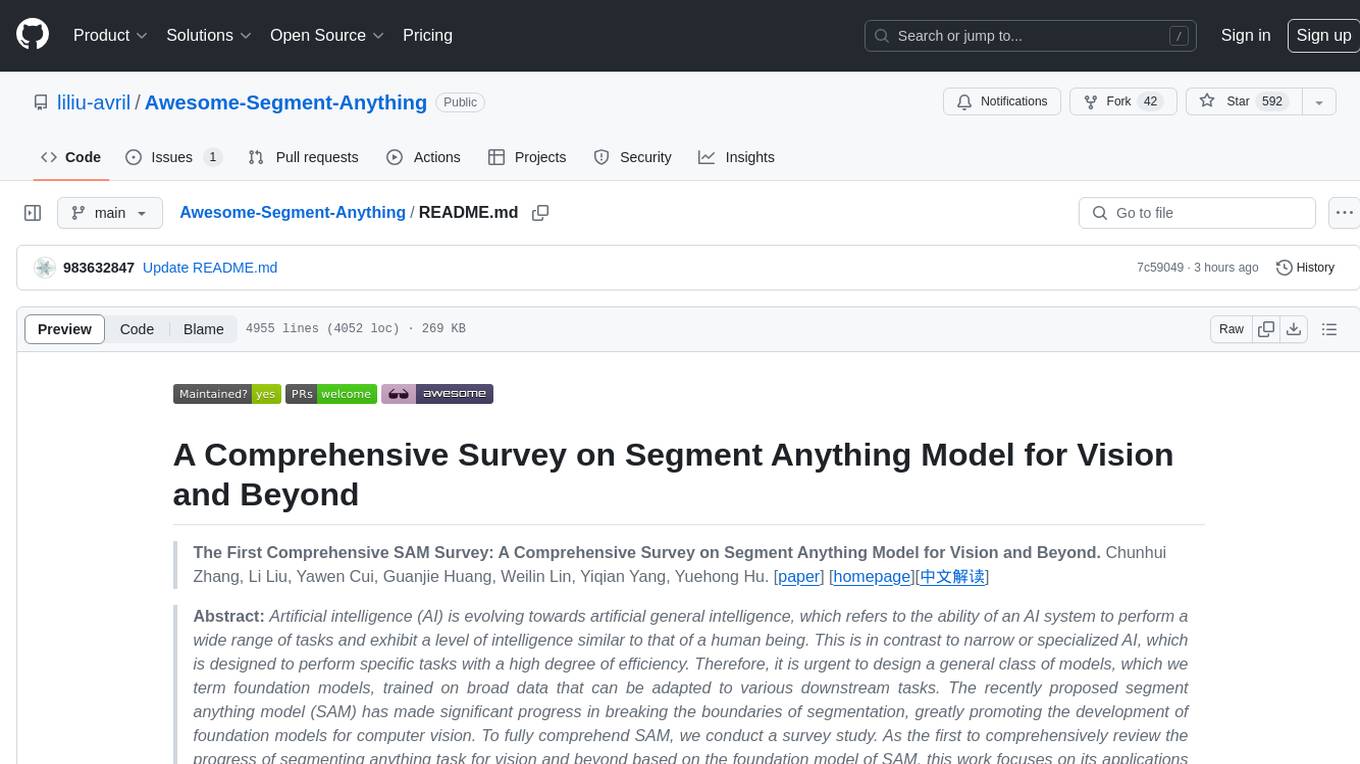
Awesome-Segment-Anything
Awesome-Segment-Anything is a powerful tool for segmenting and extracting information from various types of data. It provides a user-friendly interface to easily define segmentation rules and apply them to text, images, and other data formats. The tool supports both supervised and unsupervised segmentation methods, allowing users to customize the segmentation process based on their specific needs. With its versatile functionality and intuitive design, Awesome-Segment-Anything is ideal for data analysts, researchers, content creators, and anyone looking to efficiently extract valuable insights from complex datasets.
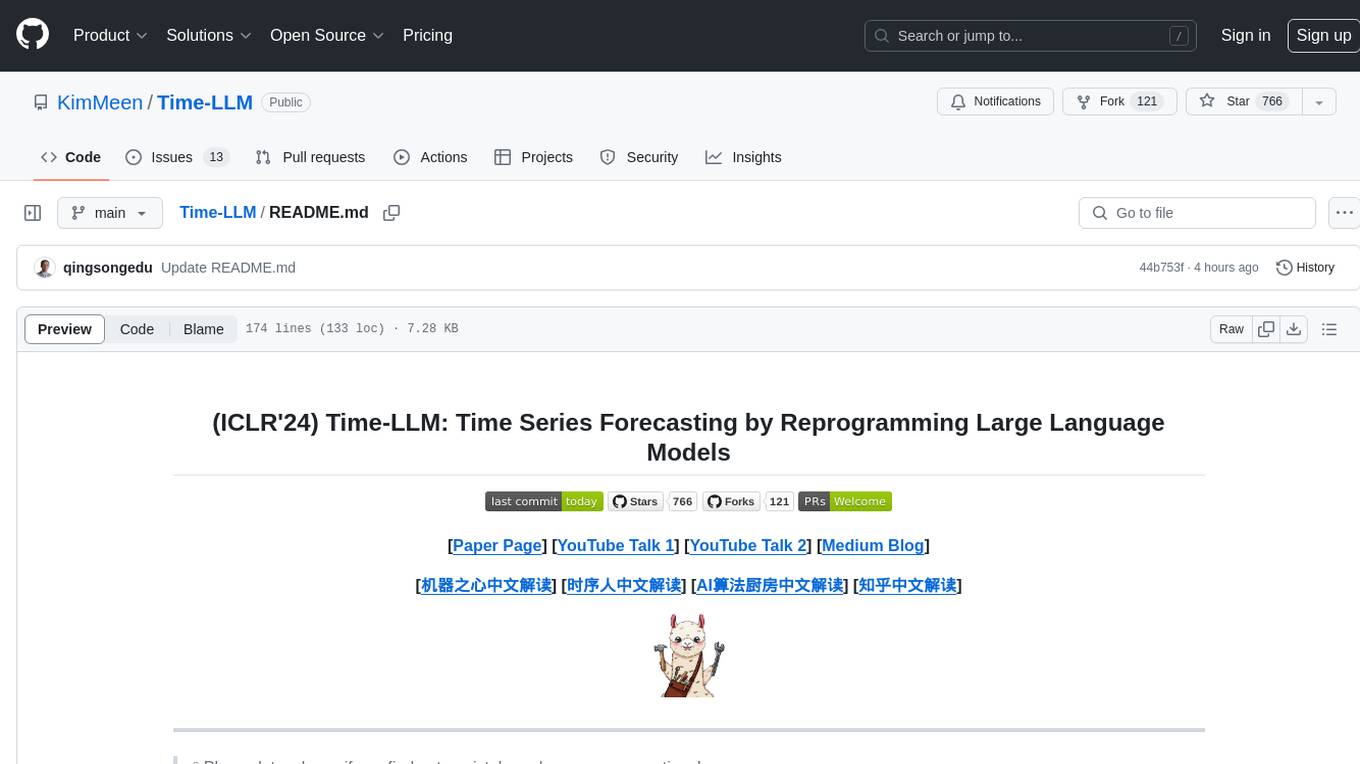
Time-LLM
Time-LLM is a reprogramming framework that repurposes large language models (LLMs) for time series forecasting. It allows users to treat time series analysis as a 'language task' and effectively leverage pre-trained LLMs for forecasting. The framework involves reprogramming time series data into text representations and providing declarative prompts to guide the LLM reasoning process. Time-LLM supports various backbone models such as Llama-7B, GPT-2, and BERT, offering flexibility in model selection. The tool provides a general framework for repurposing language models for time series forecasting tasks.
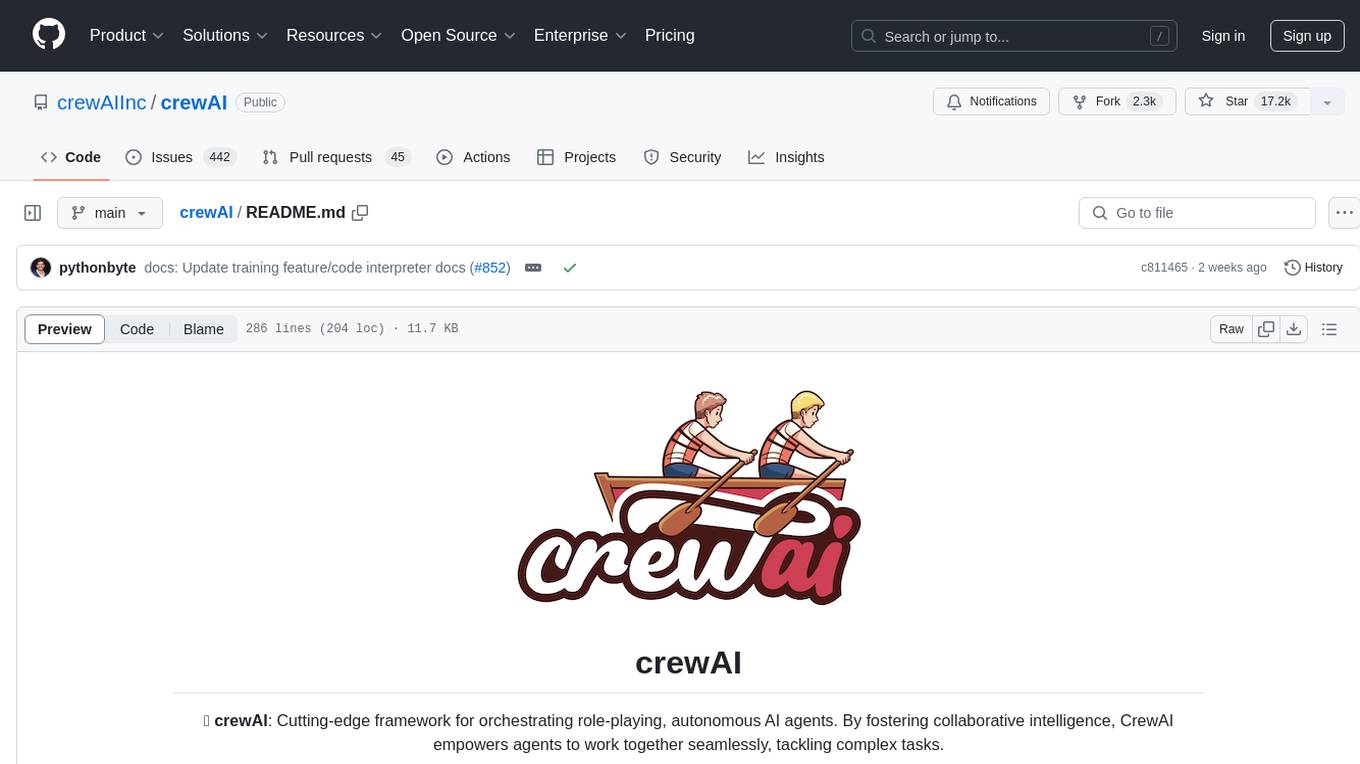
crewAI
CrewAI is a cutting-edge framework designed to orchestrate role-playing autonomous AI agents. By fostering collaborative intelligence, CrewAI empowers agents to work together seamlessly, tackling complex tasks. It enables AI agents to assume roles, share goals, and operate in a cohesive unit, much like a well-oiled crew. Whether you're building a smart assistant platform, an automated customer service ensemble, or a multi-agent research team, CrewAI provides the backbone for sophisticated multi-agent interactions. With features like role-based agent design, autonomous inter-agent delegation, flexible task management, and support for various LLMs, CrewAI offers a dynamic and adaptable solution for both development and production workflows.
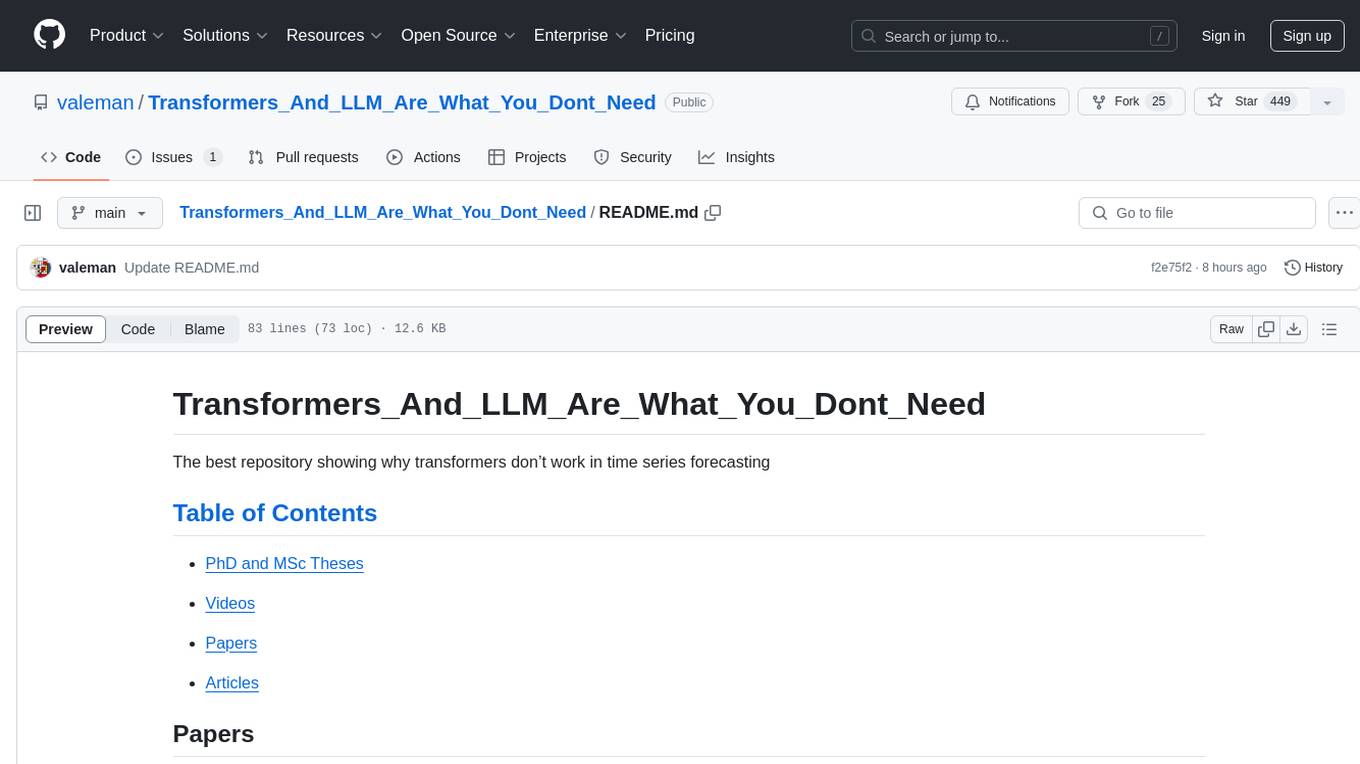
Transformers_And_LLM_Are_What_You_Dont_Need
Transformers_And_LLM_Are_What_You_Dont_Need is a repository that explores the limitations of transformers in time series forecasting. It contains a collection of papers, articles, and theses discussing the effectiveness of transformers and LLMs in this domain. The repository aims to provide insights into why transformers may not be the best choice for time series forecasting tasks.
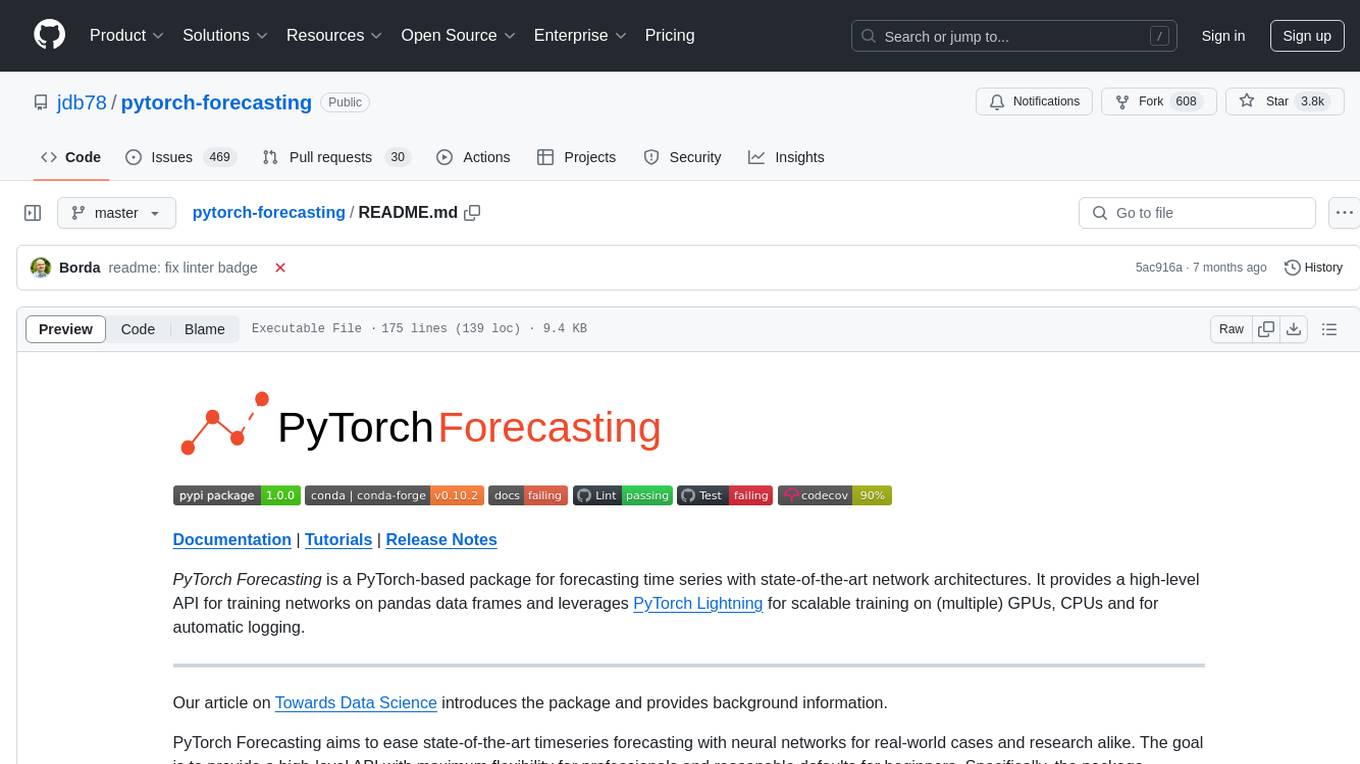
pytorch-forecasting
PyTorch Forecasting is a PyTorch-based package for time series forecasting with state-of-the-art network architectures. It offers a high-level API for training networks on pandas data frames and utilizes PyTorch Lightning for scalable training on GPUs and CPUs. The package aims to simplify time series forecasting with neural networks by providing a flexible API for professionals and default settings for beginners. It includes a timeseries dataset class, base model class, multiple neural network architectures, multi-horizon timeseries metrics, and hyperparameter tuning with optuna. PyTorch Forecasting is built on pytorch-lightning for easy training on various hardware configurations.
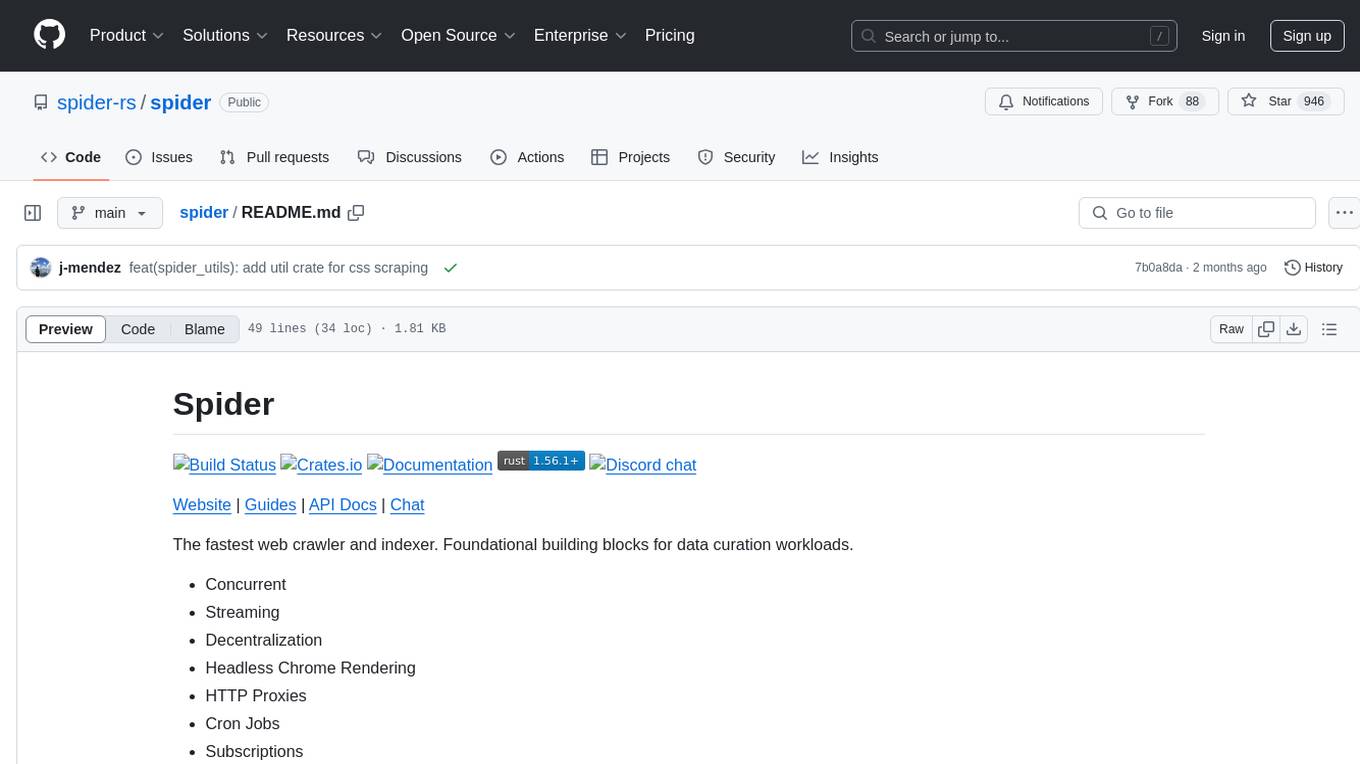
spider
Spider is a high-performance web crawler and indexer designed to handle data curation workloads efficiently. It offers features such as concurrency, streaming, decentralization, headless Chrome rendering, HTTP proxies, cron jobs, subscriptions, smart mode, blacklisting, whitelisting, budgeting depth, dynamic AI prompt scripting, CSS scraping, and more. Users can easily get started with the Spider Cloud hosted service or set up local installations with spider-cli. The tool supports integration with Node.js and Python for additional flexibility. With a focus on speed and scalability, Spider is ideal for extracting and organizing data from the web.
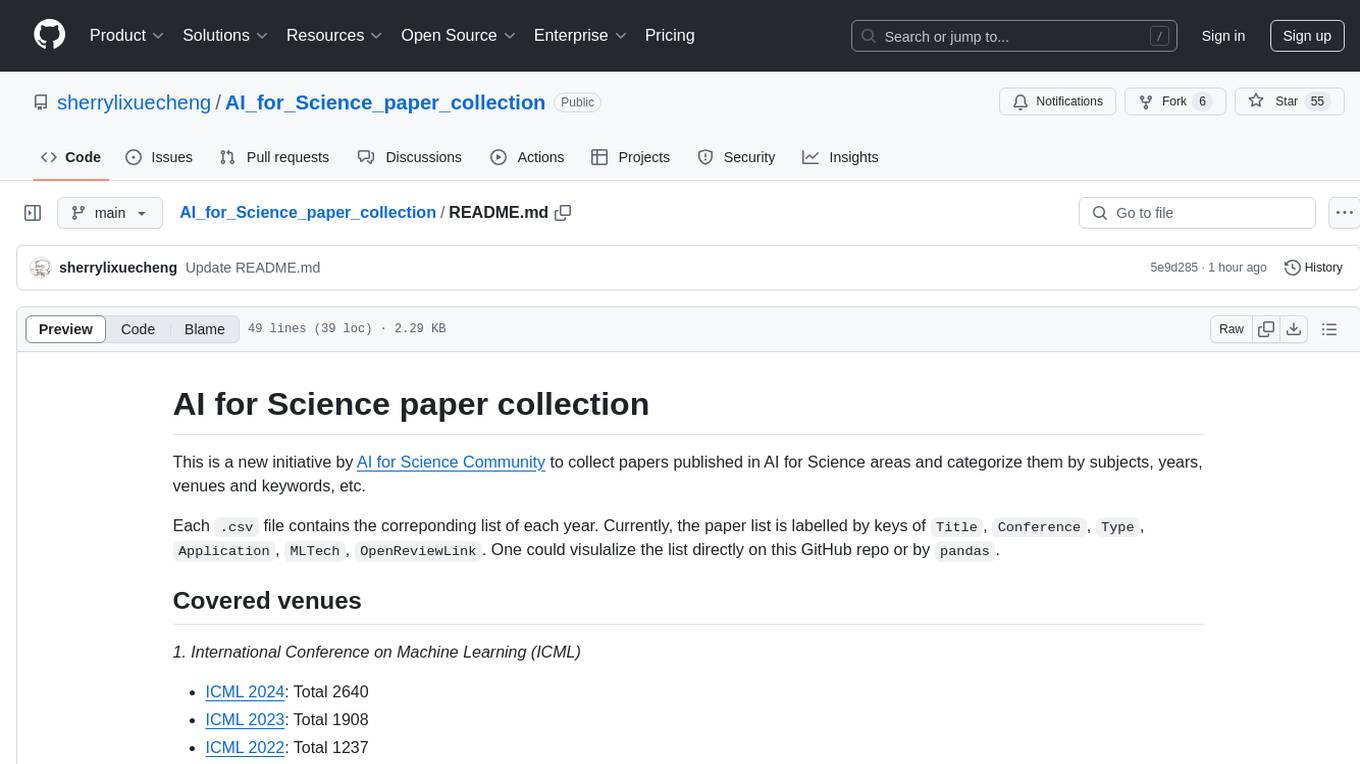
AI_for_Science_paper_collection
AI for Science paper collection is an initiative by AI for Science Community to collect and categorize papers in AI for Science areas by subjects, years, venues, and keywords. The repository contains `.csv` files with paper lists labeled by keys such as `Title`, `Conference`, `Type`, `Application`, `MLTech`, `OpenReviewLink`. It covers top conferences like ICML, NeurIPS, and ICLR. Volunteers can contribute by updating existing `.csv` files or adding new ones for uncovered conferences/years. The initiative aims to track the increasing trend of AI for Science papers and analyze trends in different applications.
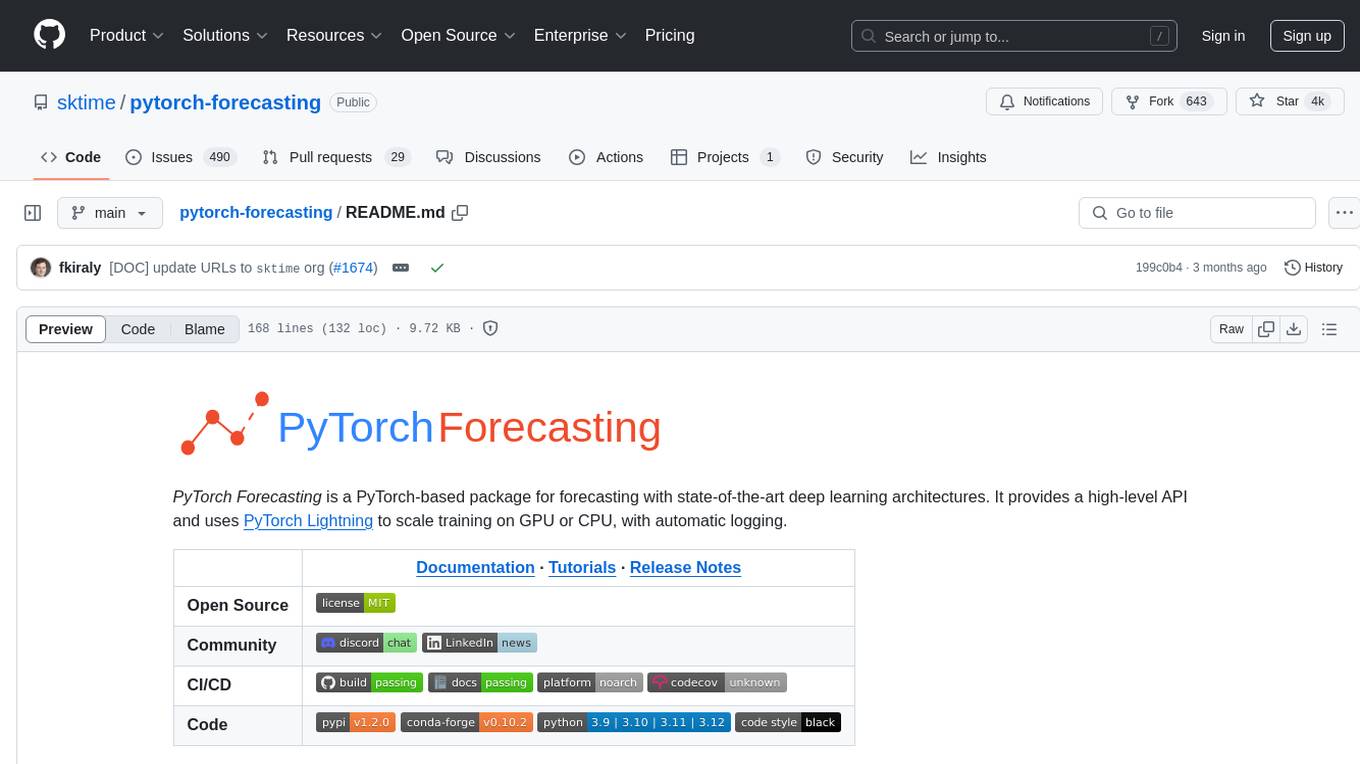
pytorch-forecasting
PyTorch Forecasting is a PyTorch-based package designed for state-of-the-art timeseries forecasting using deep learning architectures. It offers a high-level API and leverages PyTorch Lightning for efficient training on GPU or CPU with automatic logging. The package aims to simplify timeseries forecasting tasks by providing a flexible API for professionals and user-friendly defaults for beginners. It includes features such as a timeseries dataset class for handling data transformations, missing values, and subsampling, various neural network architectures optimized for real-world deployment, multi-horizon timeseries metrics, and hyperparameter tuning with optuna. Built on pytorch-lightning, it supports training on CPUs, single GPUs, and multiple GPUs out-of-the-box.
For similar jobs
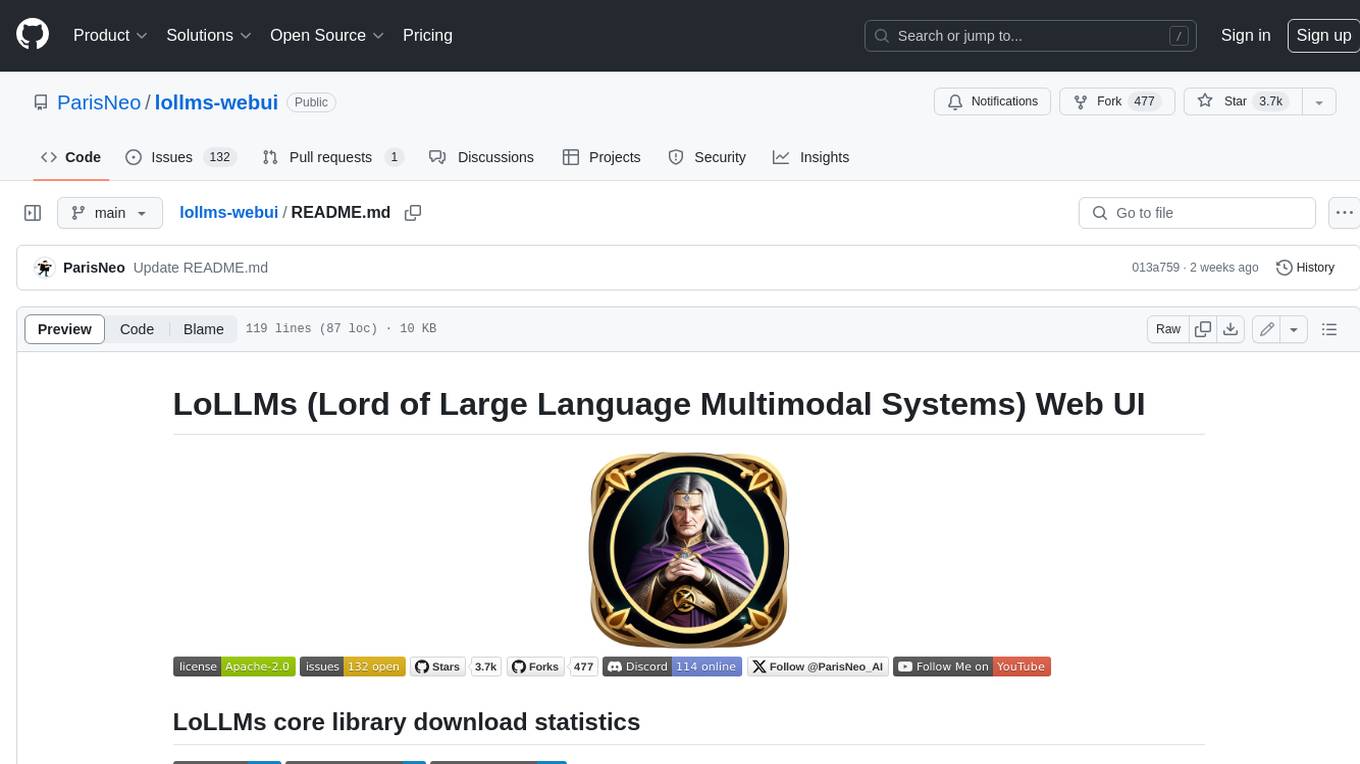
lollms-webui
LoLLMs WebUI (Lord of Large Language Multimodal Systems: One tool to rule them all) is a user-friendly interface to access and utilize various LLM (Large Language Models) and other AI models for a wide range of tasks. With over 500 AI expert conditionings across diverse domains and more than 2500 fine tuned models over multiple domains, LoLLMs WebUI provides an immediate resource for any problem, from car repair to coding assistance, legal matters, medical diagnosis, entertainment, and more. The easy-to-use UI with light and dark mode options, integration with GitHub repository, support for different personalities, and features like thumb up/down rating, copy, edit, and remove messages, local database storage, search, export, and delete multiple discussions, make LoLLMs WebUI a powerful and versatile tool.
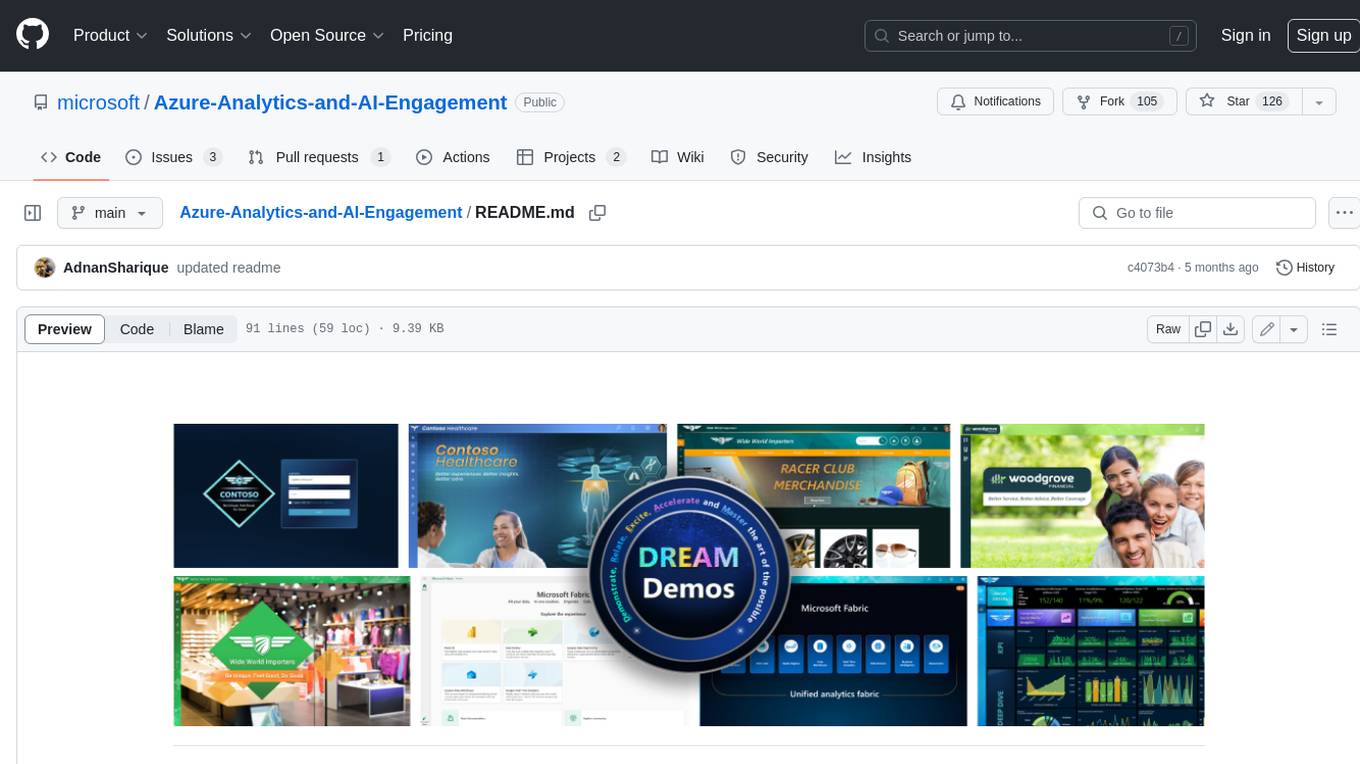
Azure-Analytics-and-AI-Engagement
The Azure-Analytics-and-AI-Engagement repository provides packaged Industry Scenario DREAM Demos with ARM templates (Containing a demo web application, Power BI reports, Synapse resources, AML Notebooks etc.) that can be deployed in a customerβs subscription using the CAPE tool within a matter of few hours. Partners can also deploy DREAM Demos in their own subscriptions using DPoC.
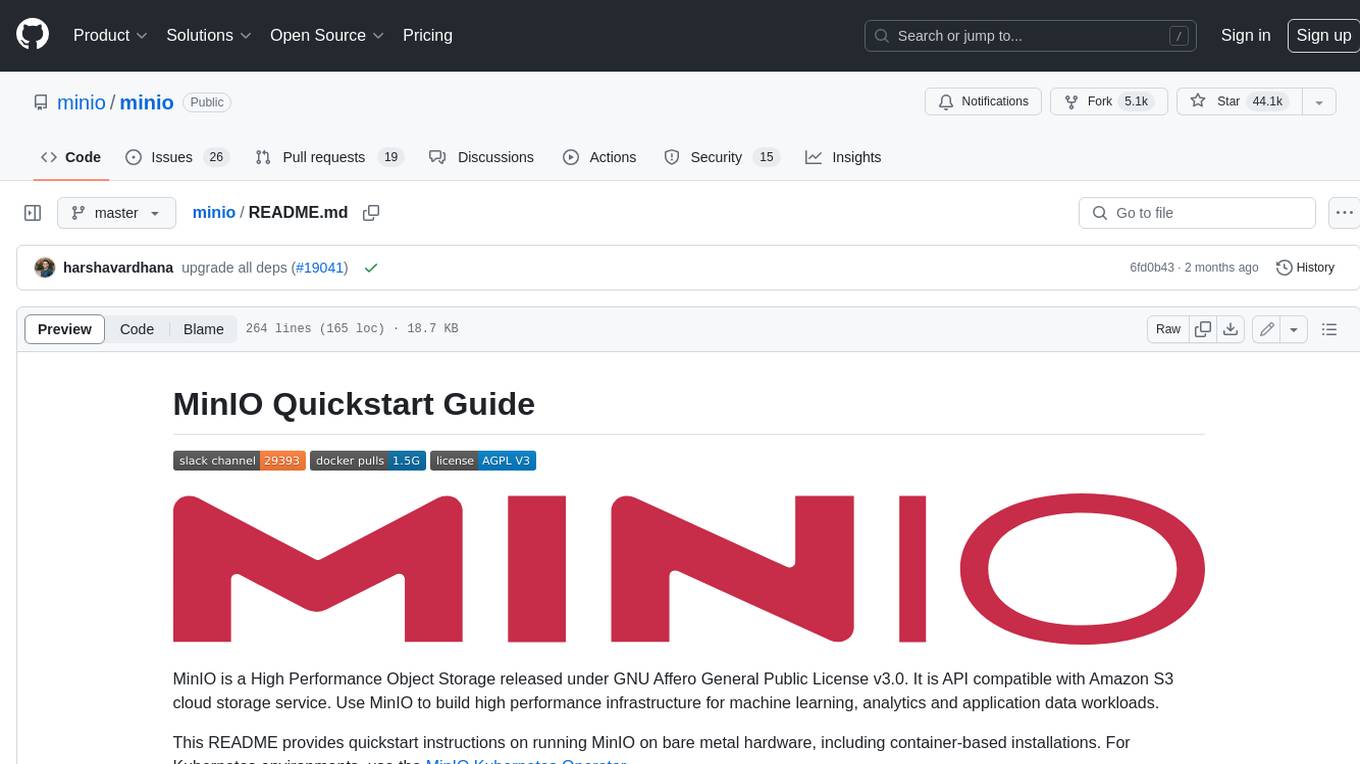
minio
MinIO is a High Performance Object Storage released under GNU Affero General Public License v3.0. It is API compatible with Amazon S3 cloud storage service. Use MinIO to build high performance infrastructure for machine learning, analytics and application data workloads.
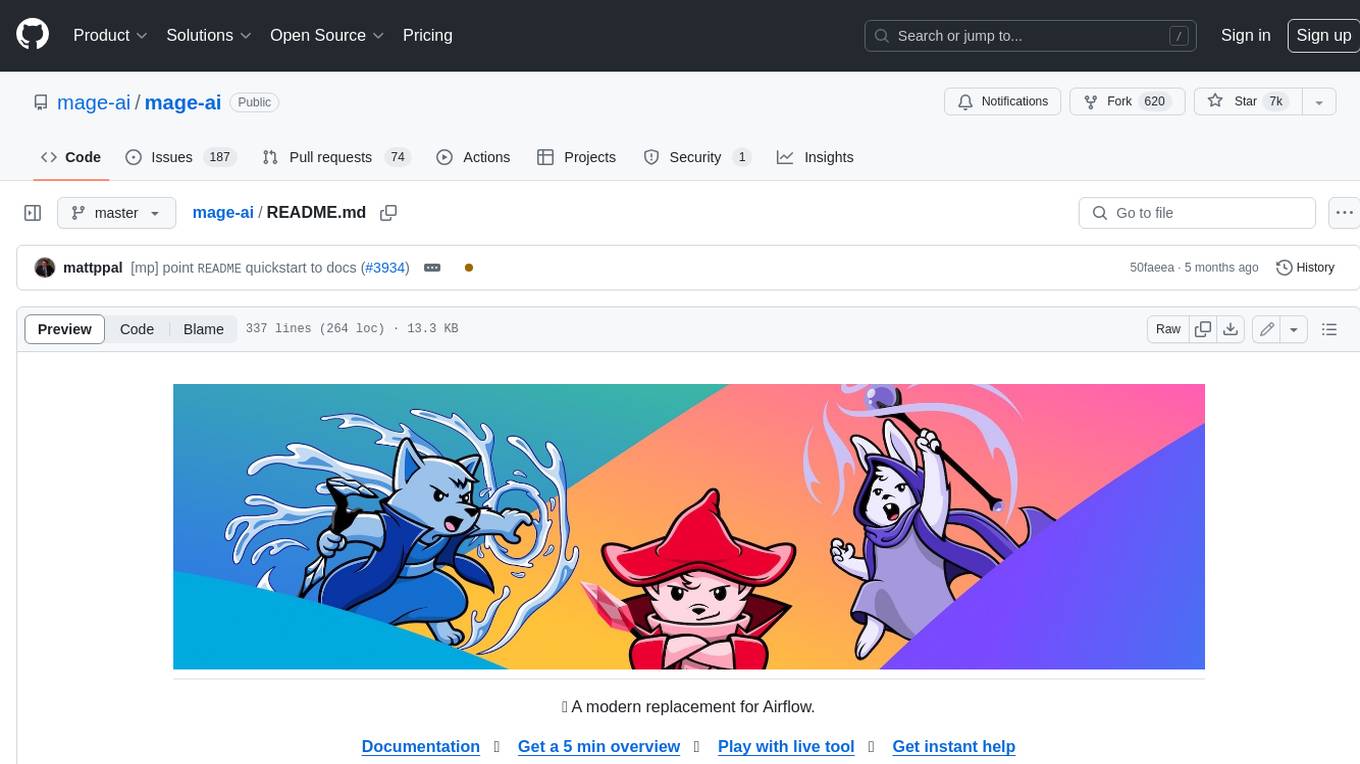
mage-ai
Mage is an open-source data pipeline tool for transforming and integrating data. It offers an easy developer experience, engineering best practices built-in, and data as a first-class citizen. Mage makes it easy to build, preview, and launch data pipelines, and provides observability and scaling capabilities. It supports data integrations, streaming pipelines, and dbt integration.
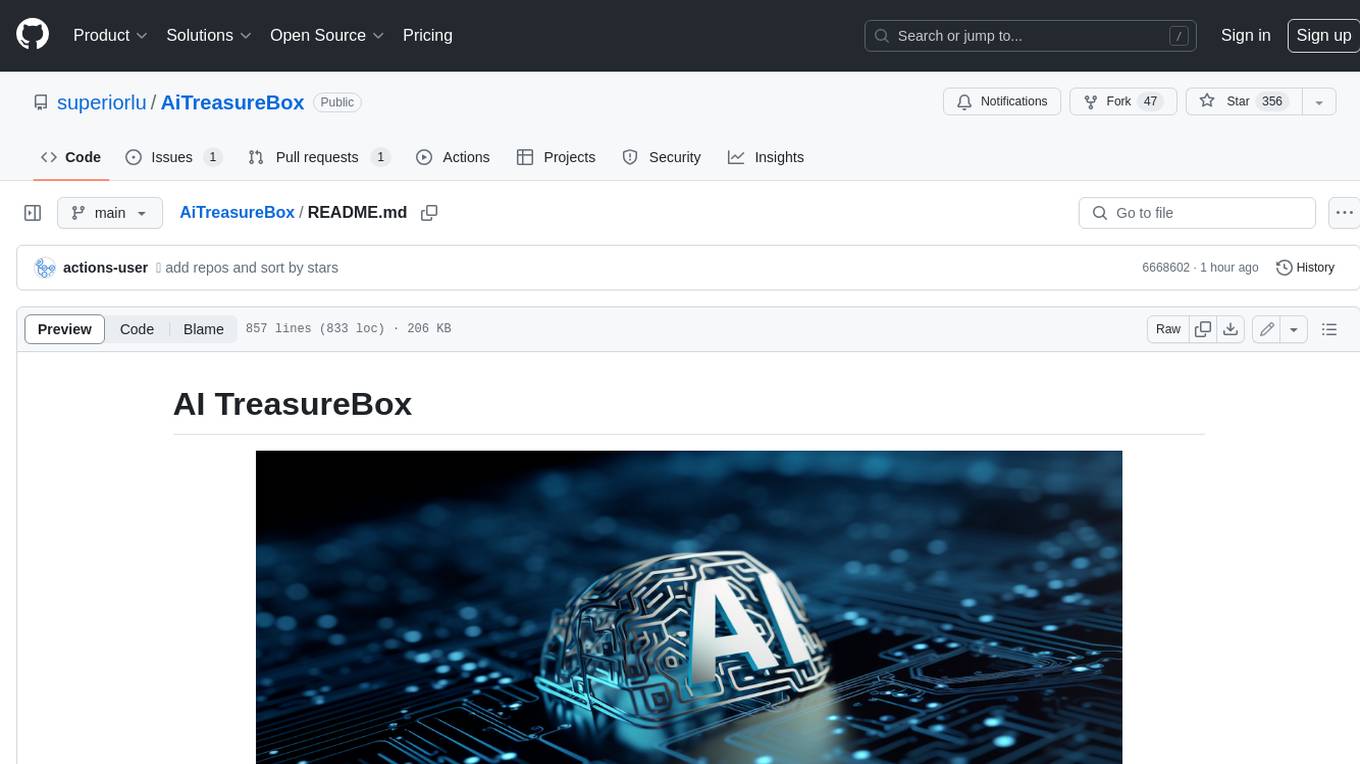
AiTreasureBox
AiTreasureBox is a versatile AI tool that provides a collection of pre-trained models and algorithms for various machine learning tasks. It simplifies the process of implementing AI solutions by offering ready-to-use components that can be easily integrated into projects. With AiTreasureBox, users can quickly prototype and deploy AI applications without the need for extensive knowledge in machine learning or deep learning. The tool covers a wide range of tasks such as image classification, text generation, sentiment analysis, object detection, and more. It is designed to be user-friendly and accessible to both beginners and experienced developers, making AI development more efficient and accessible to a wider audience.
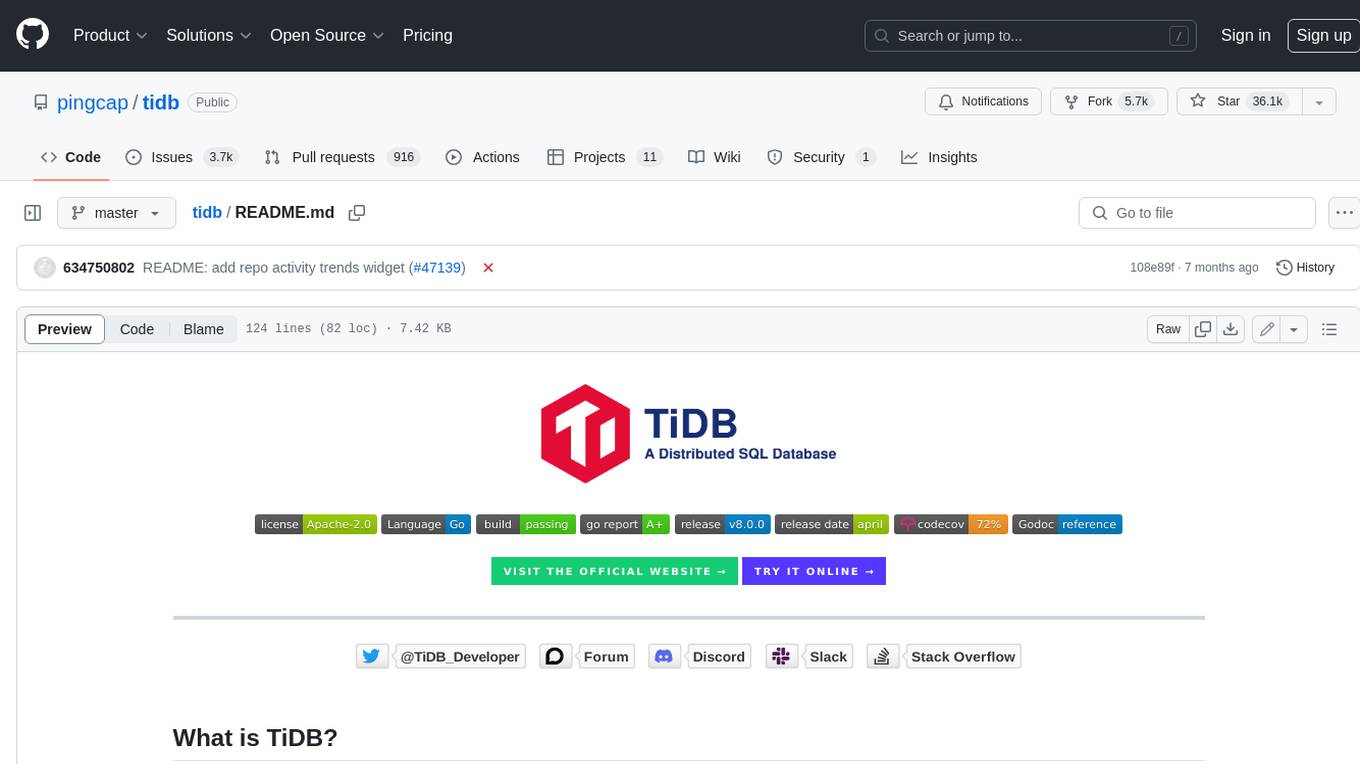
tidb
TiDB is an open-source distributed SQL database that supports Hybrid Transactional and Analytical Processing (HTAP) workloads. It is MySQL compatible and features horizontal scalability, strong consistency, and high availability.
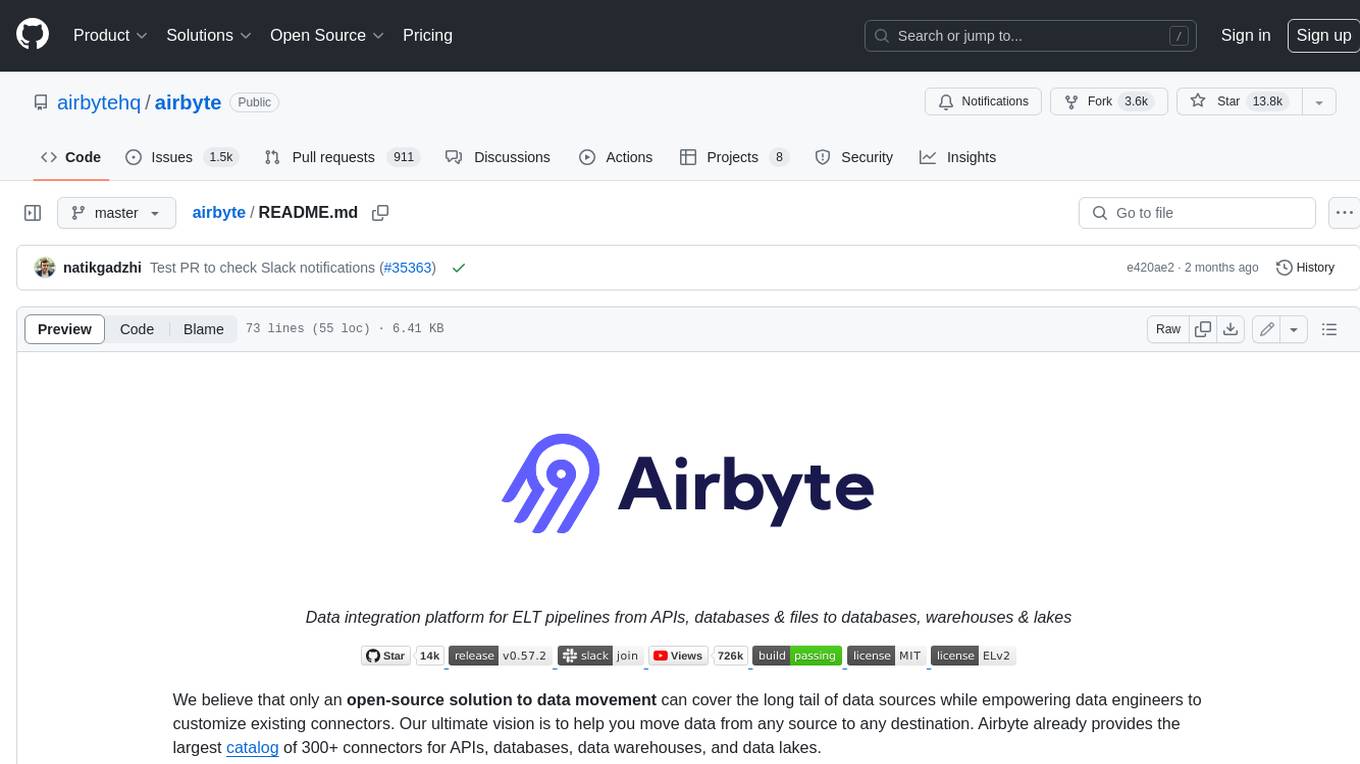
airbyte
Airbyte is an open-source data integration platform that makes it easy to move data from any source to any destination. With Airbyte, you can build and manage data pipelines without writing any code. Airbyte provides a library of pre-built connectors that make it easy to connect to popular data sources and destinations. You can also create your own connectors using Airbyte's no-code Connector Builder or low-code CDK. Airbyte is used by data engineers and analysts at companies of all sizes to build and manage their data pipelines.
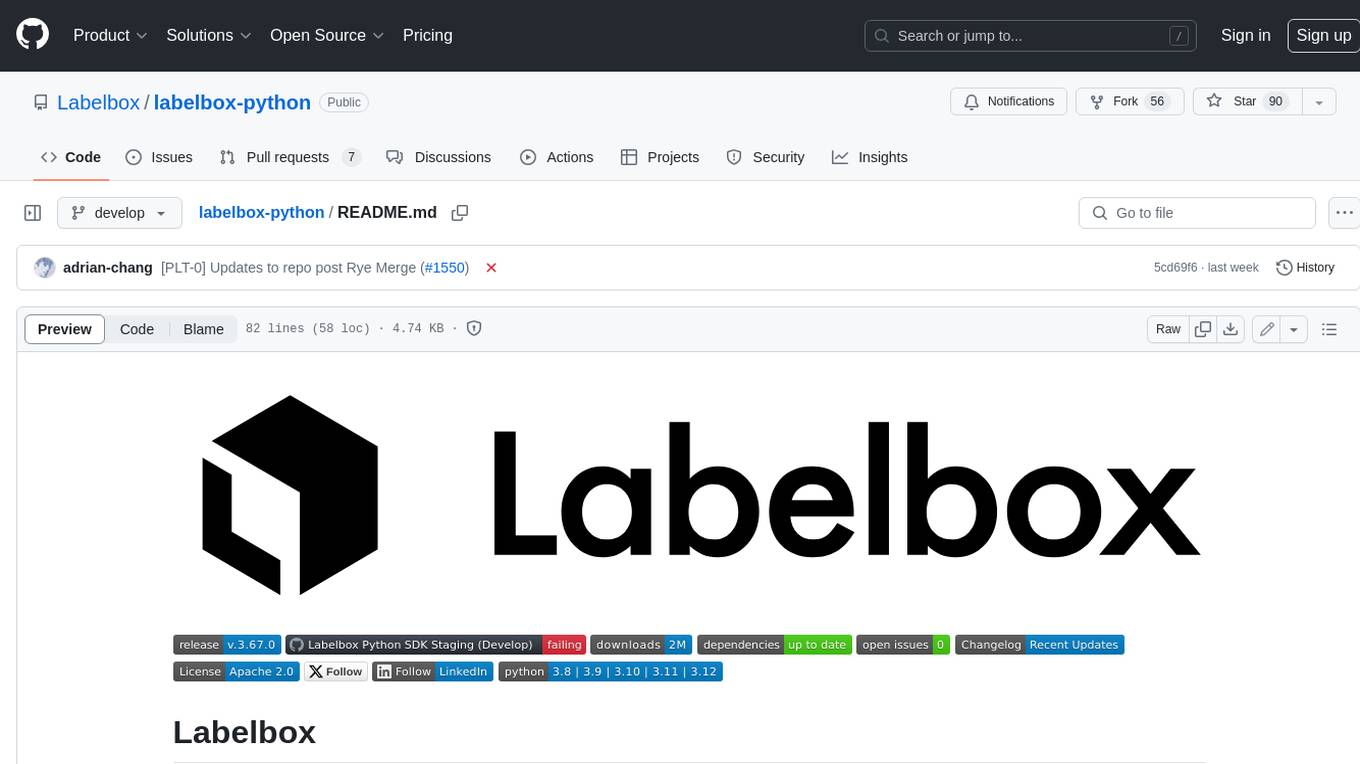
labelbox-python
Labelbox is a data-centric AI platform for enterprises to develop, optimize, and use AI to solve problems and power new products and services. Enterprises use Labelbox to curate data, generate high-quality human feedback data for computer vision and LLMs, evaluate model performance, and automate tasks by combining AI and human-centric workflows. The academic & research community uses Labelbox for cutting-edge AI research.