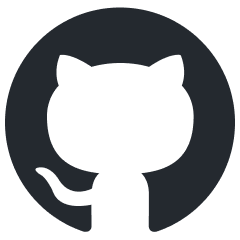
ai-dial-core
The main component of AI DIAL, which provides unified API to different chat completion and embedding models, assistants, and applications
Stars: 476
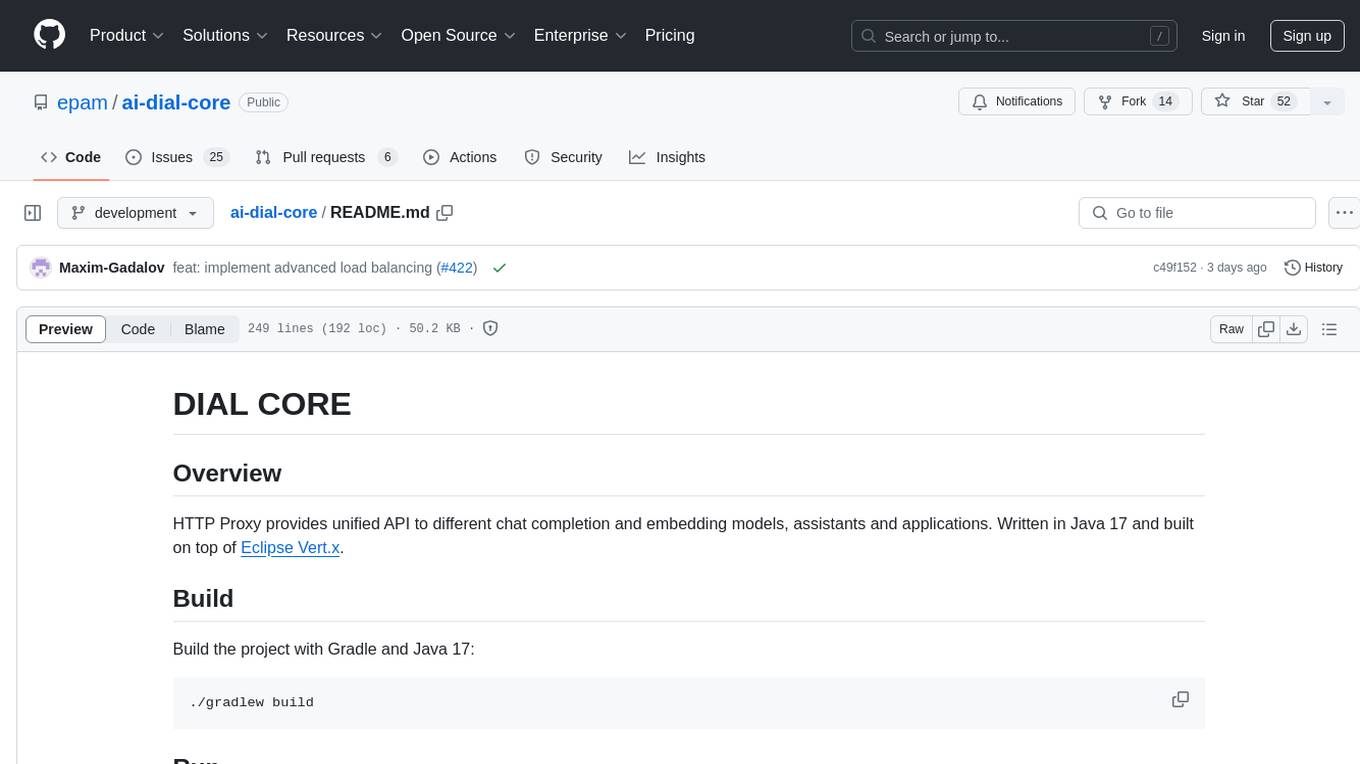
AI DIAL Core is an HTTP Proxy that provides a unified API to different chat completion and embedding models, assistants, and applications. It is written in Java 17 and built on Eclipse Vert.x. The core functionality includes handling static and dynamic settings, deployment on Kubernetes using Helm charts, and storing user data in Blob Storage and Redis. It supports various identity providers, storage providers like AWS S3, Google Cloud Storage, and Azure Blob Store, and features like AI DIAL Addons, Interceptors, Assistants, Applications, and Models with customizable parameters and configurations.
README:
HTTP Proxy provides unified API to different chat completion and embedding models, assistants and applications. Written in Java 17 and built on top of Eclipse Vert.x.
DIAL Core has a dependency on GitHub packages of JClouds. Github doesn't provide anonymous access to packages.
That requires to pass credentials GitHub for access to published JClouds packages. See the code snippet below:
repositories {
maven {
url = uri("https://maven.pkg.github.com/epam/jclouds")
credentials {
username = project.findProperty("gpr.user") ?: System.getenv("GPR_USERNAME")
password = project.findProperty("gpr.key") ?: System.getenv("GPR_PASSWORD")
}
}
mavenCentral()
}
You should set env variables GPR_USERNAME
and GPR_PASSWORD
to valid values, where GPR_USERNAME
- GitHub username and GPR_PASSWORD
- GitHub personal access token.
Note. The access token requires the permission read:packages
.
See more details here to generate personal access token in GitHub.
Build the project with Gradle and Java 17:
./gradlew build
Run the project with Gradle:
./gradlew run
Or run com.epam.aidial.core.AIDial
class from your favorite IDE.
You have the option to deploy the AI DIAL Core on the Kubernetes cluster by utilizing an umbrella dial Helm chart, which also deploys other AI DIAL components. Alternatively, you can use dial-core Helm chart to deploy just Core.
Refer to Examples for guidelines.
In any case, in your Helm values file, it is necessary to provide application's configurations described in the Configuration section.
Static settings are used on startup and cannot be changed while application is running. Refer to example to view the example configuration file.
Priority order:
- Environment variables with extra "aidial." prefix. E.g. "aidial.server.port", "aidial.config.files".
- File specified in "AIDIAL_SETTINGS" environment variable.
- Default resource file: src/main/resources/aidial.settings.json.
Setting | Default | Required | Description |
---|---|---|---|
config.files | aidial.config.json | No | List of paths to dynamic settings. Refer to example of the file with dynamic settings. |
config.reload | 60000 | No | Config reload interval in milliseconds. |
config.jsonMergeStrategy.overwriteArrays | false | No | Specifies a merging strategy for JSON arrays. If it's set to true , arrays will be overwritten. Otherwise, they will be concatenated. |
identityProviders | - | Yes | Map of identity providers. Note: At least one identity provider must be provided. Refer to examples to view available providers. Refer to IDP Configuration to view guidelines for configuring supported providers. |
identityProviders.*.jwksUrl | - | Optional | Url to jwks provider. Required if disabledVerifyJwt is set to false . Note: Either jwksUrl or userInfoEndpoint must be provided. |
identityProviders.*.userInfoEndpoint | - | Optional | Url to user info endpoint. Note: Either jwksUrl or userInfoEndpoint must be provided or disableJwtVerification is unset. Refer to Google example. |
identityProviders.*.rolePath | - | Yes | Path(s) to the claim user roles in JWT token or user info response, e.g. resource_access.chatbot-ui.roles or just roles . Can be single String or Array of Strings. Refer to IDP Configuration to view guidelines for configuring supported providers. |
identityProviders.*.projectPath | - | No | Path(s) to the claim in JWT token or user info response, e.g. azp , aud or some.path.client from which project name can be taken. Can be single String. Refer to IDP Configuration to view guidelines for configuring supported providers. |
identityProviders.*.rolesDelimiter | - | No | Delimiter to split roles into array in case when list of roles presented as single String. e.g. "rolesDelimiter": " "
|
identityProviders.*.loggingKey | - | No | User information to search in claims of JWT token. email or sub should be sufficient in most cases. Note: email might be unavailable for some IDPs. Please check your IDP documentation in this case. |
identityProviders.*.loggingSalt | - | No | Salt to hash user information for logging. |
identityProviders.*.positiveCacheExpirationMs | 600000 | No | How long to retain JWKS response in the cache in case of successfull response. |
identityProviders.*.negativeCacheExpirationMs | 10000 | No | How long to retain JWKS response in the cache in case of failed response. |
identityProviders.*.issuerPattern | - | No | Regexp to match the claim "iss" to identity provider. |
identityProviders.*.disableJwtVerification | false | No | The flag disables JWT verification. Note. userInfoEndpoint must be unset if the flag is set to true . |
identityProviders.*.audience | - | No | If the setting is set it will be validated against the claim aud in JWT |
identityProviders.*.displayUserName | - | No | Path to the claim in JWT token or user info response where user display name can be taken. |
vertx.* | - | No | Vertx settings. Refer to vertx.io to learn more. |
server.* | - | No | Vertx HTTP server settings for incoming requests. |
client.* | - | No | Vertx HTTP client settings for outbound requests. |
storage.provider | filesystem | Yes | Specifies blob storage provider. Supported providers: s3, aws-s3, azureblob, google-cloud-storage, filesystem. See examples in the sections below. |
storage.endpoint | - | Optional | Specifies endpoint url for s3 compatible storages. Note: The setting might be required. That depends on a concrete provider. |
storage.identity | - | Optional | Blob storage access key. Can be optional for filesystem, aws-s3, google-cloud-storage providers. Refer to sections in this document dedicated to specific storage providers. |
storage.credential | - | Optional | Blob storage secret key. Can be optional for filesystem, aws-s3, google-cloud-storage providers. |
storage.bucket | - | No | Blob storage bucket. |
storage.overrides.* | - | No | Key-value pairs to override storage settings. * might be any specific blob storage setting to be overridden. Refer to examples in the sections below. |
storage.createBucket | false | No | Indicates whether bucket should be created on start-up. |
storage.prefix | - | No | Base prefix for all stored resources. The purpose to use the same bucket for different environments, e.g. dev, prod, pre-prod. Must not contain path separators or any invalid chars. |
storage.maxUploadedFileSize | 536870912 | No | Maximum size in bytes of uploaded file. If a size of uploaded file exceeds the limit the server returns HTTP code 413 |
encryption.secret | - | No | Secret is used for AES encryption of a prefix to the bucket blob storage. The value should be random generated string. |
encryption.key | - | No | Key is used for AES encryption of a prefix to the bucket blob storage. The value should be random generated string. |
resources.maxSize | 67108864 | No | Max allowed size in bytes for a resource. |
resources.maxSizeToCache | 1048576 | No | Max size in bytes for a resource to cache in Redis. |
resources.syncPeriod | 60000 | No | Period in milliseconds, how frequently check for resources to sync. |
resources.syncDelay | 120000 | No | Delay in milliseconds for a resource to be written back in object storage after last modification. |
resources.syncBatch | 4096 | No | How many resources to sync in one go. |
resources.cacheExpiration | 300000 | No | Expiration in milliseconds for synced resources in Redis. |
resources.compressionMinSize | 256 | No | Compress a resource with gzip if its size in bytes more or equal to this value. |
redis.singleServerConfig.address | - | Yes | Redis single server addresses, e.g. "redis://host:port". Either singleServerConfig or clusterServersConfig must be provided. |
redis.clusterServersConfig.nodeAddresses | - | Yes | Json array with Redis cluster server addresses, e.g. ["redis://host1:port1","redis://host2:port2"]. Either singleServerConfig or clusterServersConfig must be provided. |
redis.provider.* | - | No | Provider specific settings |
redis.provider.name | - | Yes | Provider name. The valid values are aws-elasti-cache (see instructions), gcp-memory-store (see instructions). |
redis.provider.userId | - | Yes | IAM-enabled user ID. Note. It's applied to aws-elasti-cache
|
redis.provider.accountName | - | Yes | The resource name of the service account for which the credentials are requested, in the following format: projects/-/serviceAccounts/{ACCOUNT_EMAIL_OR_UNIQUEID} . The - wildcard character is required; replacing it with a project ID is invalid. Note. It's applied to gcp-memory-store
|
redis.provider.region | - | Yes | Geo region where the cache is located. Note. It's applied to aws-elasti-cache
|
redis.provider.clusterName | - | Yes | Redis cluster name. Note. It's applied to aws-elasti-cache
|
redis.provider.serverless | - | Yes | The flag indicates if the cache is serverless. Note. It's applied to aws-elasti-cache
|
invitations.ttlInSeconds | 259200 | No | Invitation time to live in seconds. |
access.admin.rules | - | No | Matches claims from identity providers with the rules to figure out whether a user is allowed to perform admin actions, like deleting any resource or approving a publication. Example: [{"source": "roles", "function": "EQUAL", "targets": ["admin"]}]. If roles contain "admin, the actions are allowed. |
access.createCodeAppRoles | - | No | The list of user roles to be allowed to create custom code applications or run code interpreter. Note. Calls by per request key are permitted even if the originator doesn't have permissions. |
applications.includeCustomApps | false | No | The flag indicates whether custom applications should be included into openai listing |
applications.controllerEndpoint | - | No | The endpoint to Application Controller Web Service that manages deployments for applications with functions |
applications.controllerTimeout | 240000 | No | The timeout of operations to Application Controller Web Service |
codeInterpreter.sessionImage | - | No | The code interpreter session image to use |
codeInterpreter.sessionTtl | 600000 | No | The session time to leave after the last API call |
codeInterpreter.checkPeriod | 10000 | No | The interval at which to check active sessions for expiration |
codeInterpreter.checkSize | 256 | No | The maximum number of active sessions to check in single check |
AI DIAL Core stores user data in the following storages:
- Blob Storage keeps permanent data.
- Redis keeps volatile in-memory data for fast access.
There are two types of credential providers supported:
- User credentials. You can create a service principle and authenticate using its secret from the Azure console.
- Temporary credentials with IAM roles for service accounts.
Set storage.credential
to Secret Access Key and storage.identity
- Access Key ID.
Follow instructions to setup your pod in AWS EKS.
storage.credential
and storage.identity
must be unset.
There are two types of credential providers supported:
- User credentials. You can create a service account and authenticate using its private key obtained from the Developer console.
- Temporary credentials. Application default credentials (ADC).
Set storage.credential
to a path to the private key JSON file and storage.identity
must be unset. Refer to the example below:
{
"type": "service_account",
"project_id": "<your_project_id>",
"private_key_id": "<your_project_key_id>",
"private_key": "-----BEGIN PRIVATE KEY-----\n<your_private_key>\n-----END PRIVATE KEY-----\n",
"client_email": "gcp-dial-core@<your_project_id>.iam.gserviceaccount.com",
"client_id": "<client_id>",
"auth_uri": "https://accounts.google.com/o/oauth2/auth",
"token_uri": "https://oauth2.googleapis.com/token",
"auth_provider_x509_cert_url": "https://www.googleapis.com/oauth2/v1/certs",
"client_x509_cert_url": "https://www.googleapis.com/robot/v1/metadata/x509/gcp-dial-core.iam.gserviceaccount.com",
"universe_domain": "googleapis.com"
}
Otherwise, storage.credential
is a private key in PEM format and storage.identity
is a client's email address.
Follow instructions to setup your pod in GKE.
storage.credential
and storage.identity
must be unset.
JClouds property jclouds.oauth.credential-type
should be set to bearerTokenCredentials
, refer to the below example.
{
"storage": {
"overrides": {
"jclouds.oauth.credential-type": "bearerTokenCredentials"
}
}
}
There are two types of credential providers supported:
- User credentials. You can create a service principle and authenticate using its secret from the Azure console.
- Temporary credentials with Azure AD Workload Identity.
Set storage.credential
to the service principle secret and storage.identity
- service principle ID.
Follow instructions to setup your pod in Azure k8s.
storage.credential
and storage.identity
must be unset.
This example demonstrates the properties to be overridden:
{
"storage": {
"endpoint": "https://<Azure Blob storage account>.blob.core.windows.net"
"overrides": {
"jclouds.azureblob.auth": "azureAd",
"jclouds.oauth.credential-type": "bearerTokenCredentials"
}
}
}
Redis can be used as a cache with volatile-* eviction policies:
maxmemory 4G
maxmemory-policy volatile-lfu
Note: Redis will be strictly required in the upcoming releases 0.8+.
Dynamic settings are stored in JSON files, specified via "config.files" static setting, and reloaded at interval, specified via "config.reload" static setting. Refer to example.
Dynamic settings can include the following parameters:
Parameter | Description |
---|---|
routes | A list of registered routes in AI DIAL Core. A route is used to proxy request through AI DIAL Core to upstream server. AI DIAL Core provides capabilities: rate limiting, role based authorization, request balancing and access to AI DIAL Core resources such as LLMs, applications, file storage. |
routes.<route_name> | Route configuration. name - route's name. |
routes.<route_name>.userRoles | Route is accessible by user roles from this list. |
routes.<route_name>.response | Pre-configured route's response:status - http status codebody - http response body.If the response is set then AI DIAL Core returns the response immediately. |
routes.<route_name>.rewritePath | A flag indicates that the path to the upstream server will be replaced with the path of the original request, if this flag is set to true
|
routes.<route_name>.paths | A list of paths to be matched request's path. If any path is matched, the request will be processed by this route. Note. A path can be a plain string or a regular expression. |
routes.<route_name>.methods | A list of HTTP methods supported by this route |
routes.<route_name>.upstreams | A list of upstream servers. endpoint : Route endpoint.key : Your API key.weight : Weight for upstream endpoint; positive number represents an endpoint capacity, zero or negative disables this enpoint from routing. Default value: 1.tier : Specifies a tier group for the endpoint. Only positive numbers are allowed. All requests will be routed to the endpoints with the highest tier (the lowest tier value), other endpoints (with lower tier/higher tier value) may be used only if the highest tier endpoints are unavailable. Default value: 0 - highest tier. Refer to Load Balancer to learn more.extraData : Additional metadata containing any information that is passed to the upstream's endpoint. It can be a JSON or String. |
routes.<route_name>.maxRetryAttempts | Maximum number of retry attempts in case if upstream server returns unsuccessful response code. In this case load balancer will try to find another upstream from the list of available upstreams. |
addons | A list of deployed AI DIAL Addons and their parameters:<addon_name> : Unique addon name. |
addons.<addon_name> |
endpoint : AI DIAL Addon API for chat completions.iconUrl : Icon path for the AI DIAL addon on UI.description : Brief AI DIAL addon description.displayName : AI DIAL addon name on UI.inputAttachmentTypes : A list of allowed MIME types for the input attachments.maxInputAttachments : Maximum number of input attachments (default is zero when inputAttachmentTypes is unset, otherwise, infinity) forwardAuthToken : If flag is set to true forward Http header with authorization token to chat completion endpoint of the addon. userRoles : a specific claim value provided by a specific IDP. Refer to IDP Configuration to view examples. author : the addon's developer. createdAt : the date of the addon creation. updatedAt : the date of the last addon update. |
interceptors | A list of deployed AI DIAL Interceptors and their parameters:<interceptor_name> : Unique interceptor name. Refer to Interceptors to learn more. |
interceptors.<interceptor_name> |
endpoint : AI DIAL Interceptor API for chat completions.iconUrl : Icon path for the AI DIAL Interceptor on UI.description : Brief AI DIAL interceptor description.displayName : AI DIAL interceptor name on UI.forwardAuthToken : If flag is set to true forward Http header with authorization token to chat completion endpoint of the interceptor. Refer to Interceptors to learn more. author : the interceptor's developer. createdAt : the date of the interceptor creation. updatedAt : the date of the last interceptor update. |
assistant | A list of deployed AI DIAL Assistants and their parameters:<assistant_name> : Unique assistan name. |
assistant.endpoint | Assistant main endpoint |
assistant.assistants.<assistant_name> |
iconUrl : Icon path for the AI DIAL assistant on UI.description : Brief AI DIAL assistant description.displayName : AI DIAL assistant name on UI.inputAttachmentTypes : A list of allowed MIME types for the input attachments.maxInputAttachments : Maximum number of input attachments (default is zero when inputAttachmentTypes is unset, otherwise, infinity) forwardAuthToken : If flag is set to true forward Http header with authorization token to chat completion endpoint of the assistant. userRoles : a specific claim value provided by a specific IDP. Refer to IDP Configuration to view examples.descriptionKeywords : a list of keywords describes the assistant, e.g. code-gen , text2image . author : the assistant's developer. createdAt : the date of the assistant creation. updatedAt : the date of the last assistant update. |
assistant.assistants.<assistant_name>.defaults | Default parameters are applied if a request doesn't contain them in OpenAI chat/completions API call |
applications | A list of deployed AI DIAL Applications and their parameters:<application_name> : Unique application name. |
applications.<application_name> |
endpoint : AI DIAL Application API for chat completions.iconUrl : Icon path for the AI DIAL Application on UI.description : Brief AI DIAL Application description.displayName : AI DIAL Application name on UI.inputAttachmentTypes : A list of allowed MIME types for the input attachments.maxInputAttachments : Maximum number of input attachments (default is zero when inputAttachmentTypes is unset, otherwise, infinity) forwardAuthToken : If flag is set to true forward Http header with authorization token to chat completion endpoint of the application. userRoles : a specific claim value provided by a specific IDP. Refer to IDP Configuration to view examples.descriptionKeywords : a list of keywords describes the application, e.g. code-gen , text2image . maxRetryAttempts : max retry attempts to route a single user request to the application's endpoint. author : the application's developer. createdAt : the date of the application creation. updatedAt : the date of the last application update. dependencies : a list of dependent deployments which the application may use. |
applications.<application_name>.defaults | Default parameters are applied if a request doesn't contain them in OpenAI chat/completions API call |
applications.<application_name>.interceptors | A list of interceptors to be triggered for the given application. Refer to Interceptors to learn more. |
applications.<application_name>.features |
rateEndpoint : endpoint for rate requests (exposed by DIAL Core as <deployment name>/rate ).tokenizeEndpoint : endpoint for requests to the model tokenizer (exposed by DIAL Core as <deployment name>/tokenize ).truncatePromptEndpoint : endpoint for truncating prompt requests (exposed by DIAL Core as <deployment name>/truncate_prompt ).systemPromptSupported : does the application support system prompt (default is true ).toolsSupported : does the application support tools (default is false ).seedSupported : does the application support seed request parameter (default is false ).urlAttachmentsSupported : does the application support attachments with URLs (default is false ).folderAttachmentsSupported : does the application support folder attachments (default is false )configurationEndpoint : the endpoint to request application configuration parameters as JSON schema (exposed by DIAL Core as <deployment name>/configuration ).accessibleByPerRequestKey : indicates whether the deployment is accessible using a per-request API key (default is true ).contentPartsSupported : indicates whether the deployment supports requests with content parts or not (default is false ). consentRequired : indicates whether the application requires user consent before use. |
models | A list of deployed models and their parameters:<model_name> : Unique model name. |
models.<model_name> |
type : Model type—chat or embedding .iconUrl : Icon path for the model on UI.description : Brief model description.displayName : Model name on UI.displayVersion : Model version on UI.endpoint : Model API for chat completions or embeddings.tokenizerModel : Identifies the specific model whose tokenization algorithm exactly matches that of the referenced model. This is typically the name of the earliest-released model in a series of models sharing an identical tokenization algorithm (e.g. gpt-3.5-turbo-0301 , gpt-4-0314 , or gpt-4-1106-vision-preview ). This parameter is essential for DIAL clients that reimplement tokenization algorithms on their side, instead of utilizing the tokenizeEndpoint provided by the model.features : Model features.limits : Model token limits.pricing : Model pricing.upstreams : Used for load-balancing—request is sent to model endpoint containing X-UPSTREAM-ENDPOINT and X-UPSTREAM-KEY headers.userRoles : a specific claim value provided by a specific IDP. Refer to IDP Configuration to view examples.descriptionKeywords : a list of keywords describes the model, e.g. code-gen , text2image . maxRetryAttempts : max retry attempts to route a single user request to upstreams.inputAttachmentTypes : A list of allowed MIME types for the input attachments.maxInputAttachments : Maximum number of input attachments (default is zero when inputAttachmentTypes is unset, otherwise, infinity). author : the model's developer. createdAt : the date of the model creation. updatedAt : the date of the last model update. |
models.<model_name>.limits |
maxPromptTokens : maximum number of tokens in a completion request.maxCompletionTokens : maximum number of tokens in a completion response.maxTotalTokens : maximum number of tokens in completion request and response combined.Typically either maxTotalTokens is specified or maxPromptTokens and maxCompletionTokens . |
models.<model_name>.pricing |
unit : the pricing units (currently token and char_without_whitespace are supported).prompt : per-unit price for the completion request in USD.completion : per-unit price for the completion response in USD. |
models.<model_name>.features |
rateEndpoint : endpoint for rate requests (exposed by core as <deployment name>/rate ).tokenizeEndpoint : endpoint for requests to the model tokenizer (exposed by DIAL Core as <deployment name>/tokenize ).truncatePromptEndpoint : endpoint for truncating prompt requests (exposed by DIAL Core as <deployment name>/truncate_prompt ).systemPromptSupported : does the model support system prompt (default is true ).toolsSupported : does the model support tools (default is false ).seedSupported : does the model support seed request parameter (default is false ).urlAttachmentsSupported : does the model/application support attachments with URLs (default is false ).folderAttachmentsSupported : does the model/application support folder attachments (default is false )accessibleByPerRequestKey : indicates whether the deployment is accessible using a per-request API key (default is true ).contentPartsSupported : indicates whether the deployment supports requests with content parts or not (default is false ). cacheSupported : indicates whether the deployment supports LLM caching (default is false ). . autoCachingSupported : indicates whether the deployment supports automatic caching, where it's possible (default is false ). |
models.<model_name>.upstreams |
endpoint : Model endpoint.key : Your API key.weight : Weight for upstream endpoint; positive number represents an endpoint capacity, zero or negative disables this enpoint from routing. Default value: 1.tier : Specifies tier group for the endpoint. Only positive numbers allowed. All requests will be routed to the endpoints with the highest tier (the lowest tier value), other endpoints (with lower tier/higher tier value) may be used only if the highest tier endpoints are unavailable. Default value: 0 - highest tier. Refer to Load Balancer to learn more.extraData : Additional metadata containing any information that is passed to the upstream's endpoint. It can be a JSON or String. |
models.<model_name>.defaults | Default parameters are applied if a request doesn't contain them in OpenAI chat/completions API call |
models.<model_name>.interceptors | A list of interceptors to be triggered for the given model. Refer to Interceptors to learn more. |
keys | API Keys parameters:<core_key> : Your API key. Refer to API Keys to learn more. |
keys.<core_key> |
project : Project name is assigned to this key. Required role : a role to be assigned to the key. Note: a key is invalid if role and roles are missed. roles : a list of roles to be assigned to the key. Note: a key is invalid if role and roles are missed. secured : the flag indicates if the key is secured. If it's set to true user request and deployment response won't be saved to the prompt log storage. |
roles | API key or user roles. Each role may have limits to be associated with applications, models, assistants or addons. Refer to API Keys to learn more. |
roles.<role_name> |
limits : Limits for models, applications, or assistants. Note: it is necessary to define this for a role. |
roles.<role_name>.limits |
minute : Total tokens per minute limit sent to the model, managed via floating window approach for well-distributed rate limiting. If it's not set the default value is unlimitedday : Total tokens per day limit sent to the model, managed via floating window approach for balanced rate limiting.week : Total tokens per week limit sent to the model, managed via floating window approach for balanced rate limiting.month : Total tokens per month limit sent to the model, managed via floating window approach for balanced rate limiting.Note: you can skip these parameters to apply their default value - unlimited. |
retriableErrorCodes | List of retriable error codes for handling outages at LLM providers. |
Copyright (C) 2024 EPAM Systems
Licensed under the Apache License, Version 2.0 (the "License"); you may not use this file except in compliance with the License. You may obtain a copy of the License at
http://www.apache.org/licenses/LICENSE-2.0
Unless required by applicable law or agreed to in writing, software distributed under the License is distributed on an "AS IS" BASIS, WITHOUT WARRANTIES OR CONDITIONS OF ANY KIND, either express or implied. See the License for the specific language governing permissions and limitations under the License.
For Tasks:
Click tags to check more tools for each tasksFor Jobs:
Alternative AI tools for ai-dial-core
Similar Open Source Tools
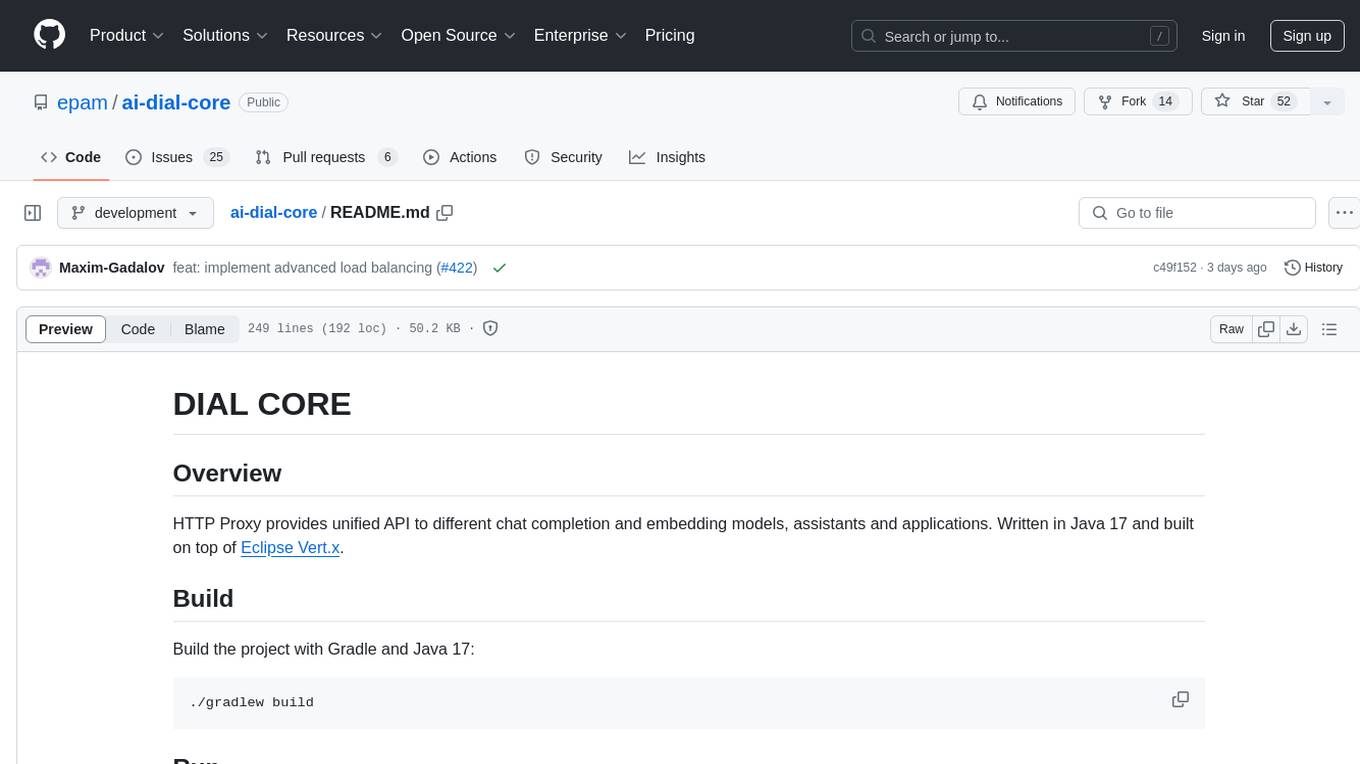
ai-dial-core
AI DIAL Core is an HTTP Proxy that provides a unified API to different chat completion and embedding models, assistants, and applications. It is written in Java 17 and built on Eclipse Vert.x. The core functionality includes handling static and dynamic settings, deployment on Kubernetes using Helm charts, and storing user data in Blob Storage and Redis. It supports various identity providers, storage providers like AWS S3, Google Cloud Storage, and Azure Blob Store, and features like AI DIAL Addons, Interceptors, Assistants, Applications, and Models with customizable parameters and configurations.
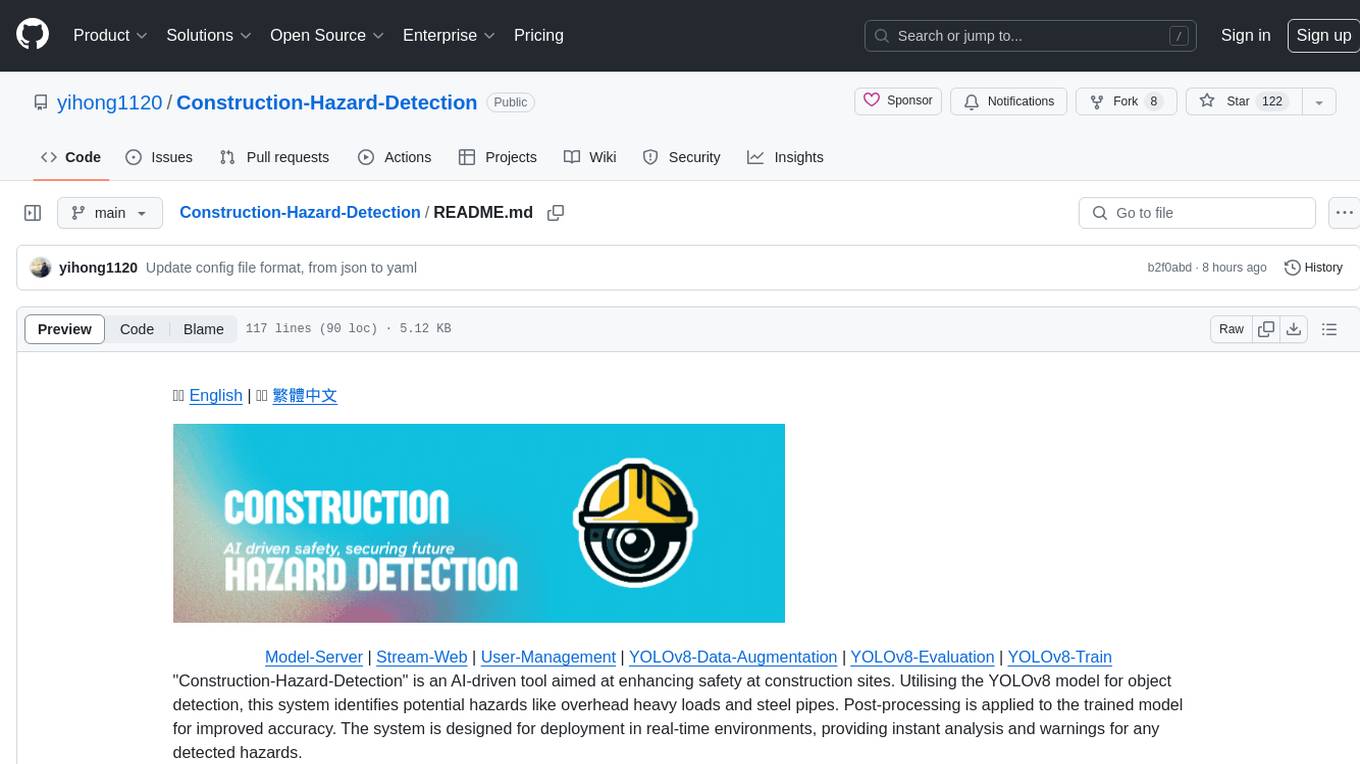
Construction-Hazard-Detection
Construction-Hazard-Detection is an AI-driven tool focused on improving safety at construction sites by utilizing the YOLOv8 model for object detection. The system identifies potential hazards like overhead heavy loads and steel pipes, providing real-time analysis and warnings. Users can configure the system via a YAML file and run it using Docker. The primary dataset used for training is the Construction Site Safety Image Dataset enriched with additional annotations. The system logs are accessible within the Docker container for debugging, and notifications are sent through the LINE messaging API when hazards are detected.
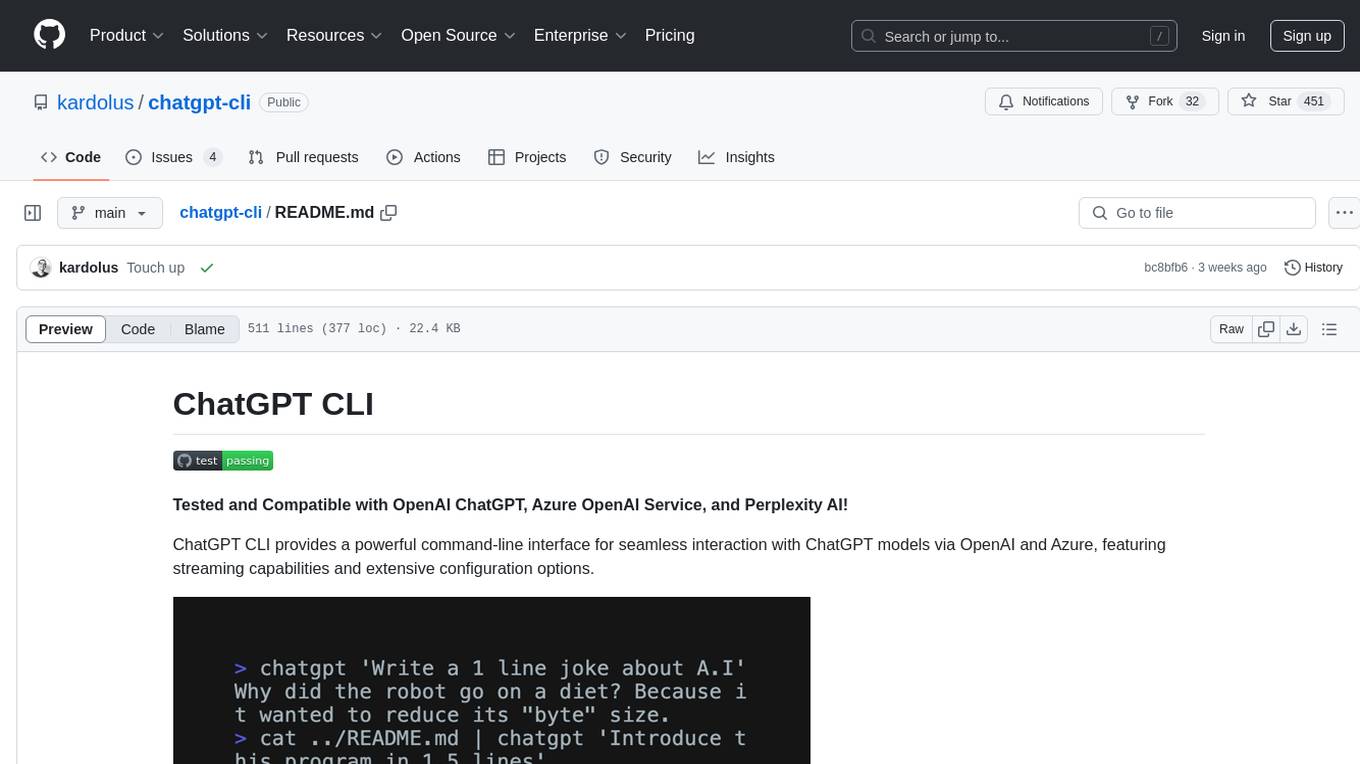
chatgpt-cli
ChatGPT CLI provides a powerful command-line interface for seamless interaction with ChatGPT models via OpenAI and Azure. It features streaming capabilities, extensive configuration options, and supports various modes like streaming, query, and interactive mode. Users can manage thread-based context, sliding window history, and provide custom context from any source. The CLI also offers model and thread listing, advanced configuration options, and supports GPT-4, GPT-3.5-turbo, and Perplexity's models. Installation is available via Homebrew or direct download, and users can configure settings through default values, a config.yaml file, or environment variables.
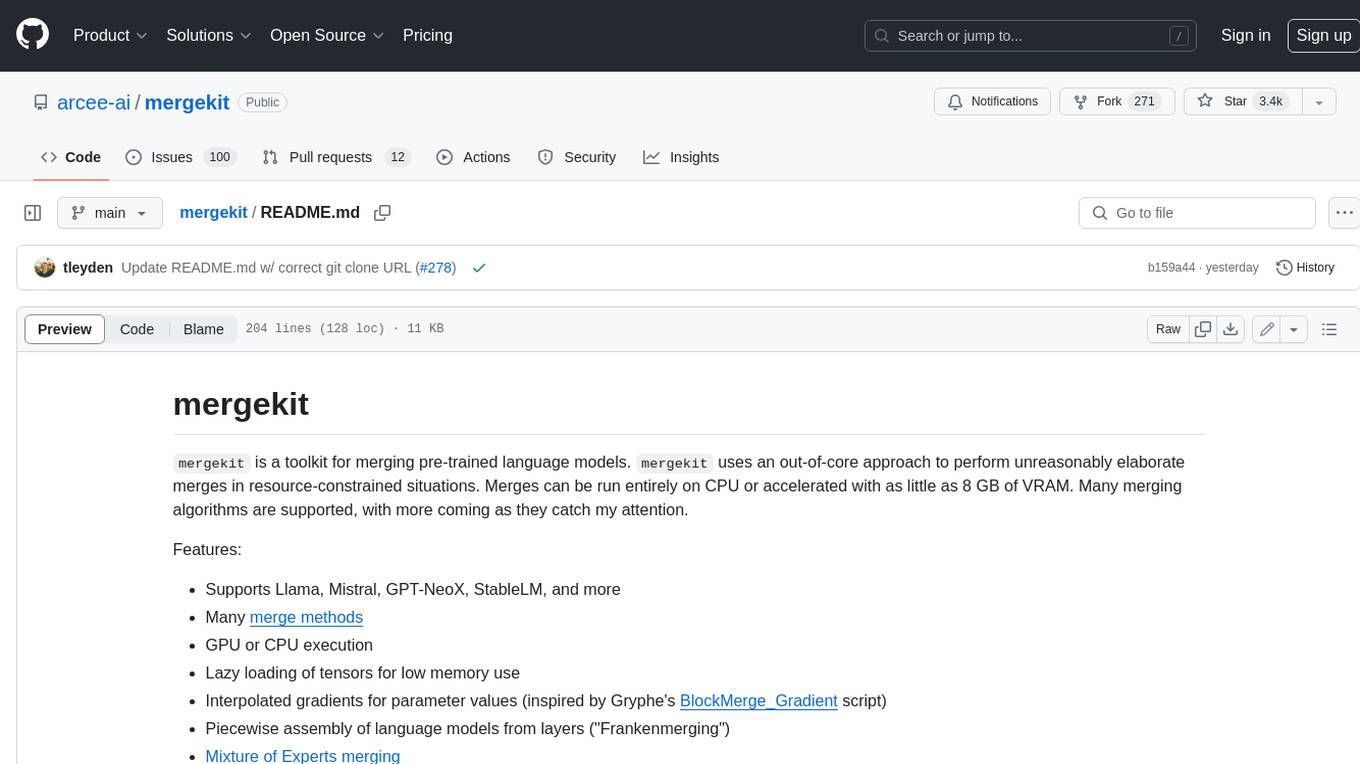
mergekit
Mergekit is a toolkit for merging pre-trained language models. It uses an out-of-core approach to perform unreasonably elaborate merges in resource-constrained situations. Merges can be run entirely on CPU or accelerated with as little as 8 GB of VRAM. Many merging algorithms are supported, with more coming as they catch my attention.
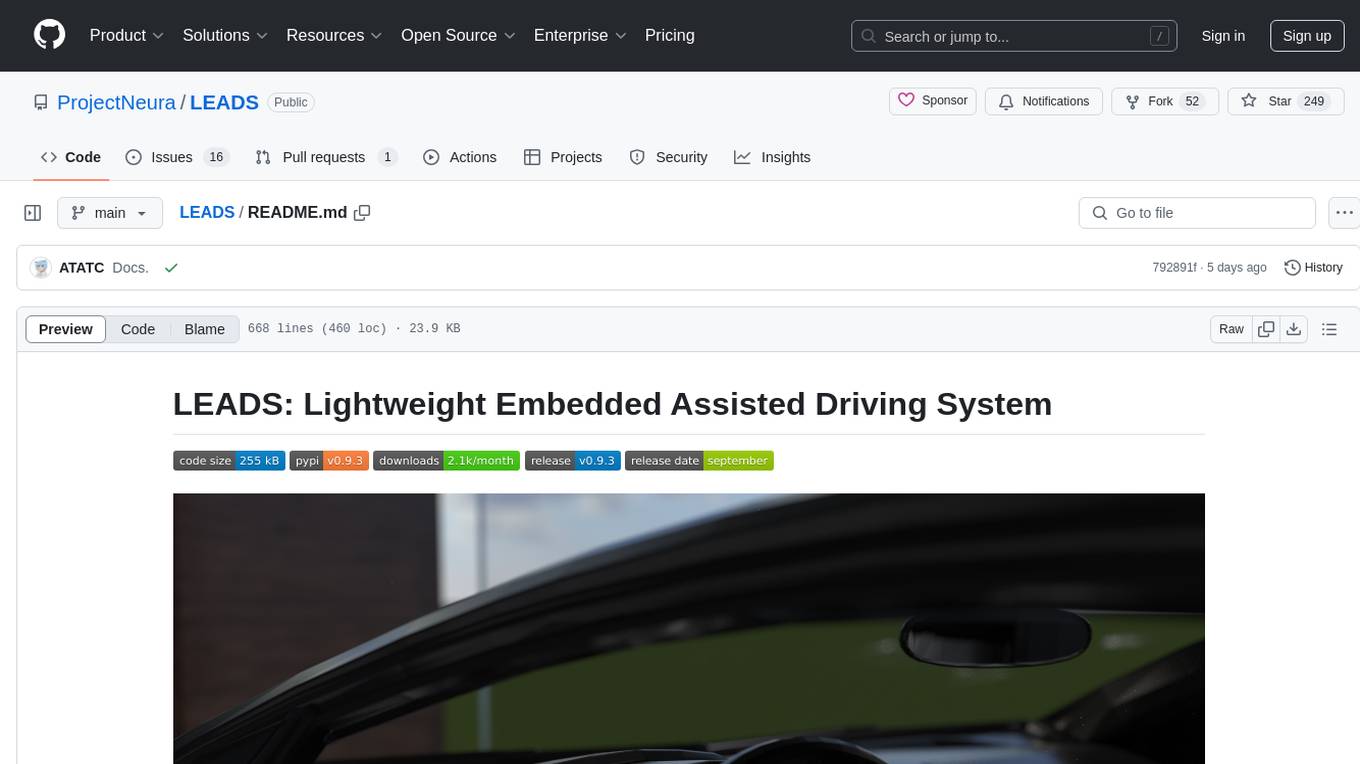
LEADS
LEADS is a lightweight embedded assisted driving system designed to simplify the development of instrumentation, control, and analysis systems for racing cars. It is written in Python and C/C++ with impressive performance. The system is customizable and provides abstract layers for component rearrangement. It supports hardware components like Raspberry Pi and Arduino, and can adapt to various hardware types. LEADS offers a modular structure with a focus on flexibility and lightweight design. It includes robust safety features, modern GUI design with dark mode support, high performance on different platforms, and powerful ESC systems for traction control and braking. The system also supports real-time data sharing, live video streaming, and AI-enhanced data analysis for driver training. LEADS VeC Remote Analyst enables transparency between the driver and pit crew, allowing real-time data sharing and analysis. The system is designed to be user-friendly, adaptable, and efficient for racing car development.
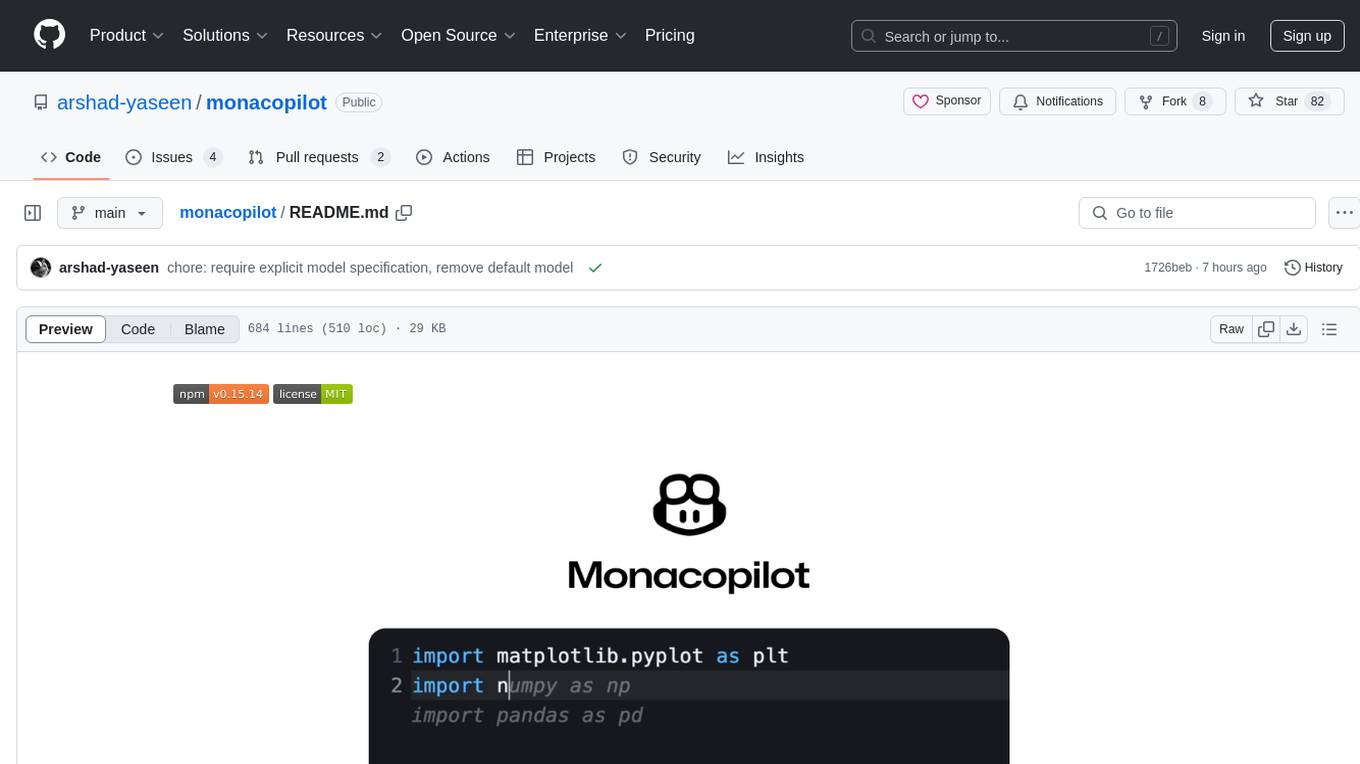
monacopilot
Monacopilot is a powerful and customizable AI auto-completion plugin for the Monaco Editor. It supports multiple AI providers such as Anthropic, OpenAI, Groq, and Google, providing real-time code completions with an efficient caching system. The plugin offers context-aware suggestions, customizable completion behavior, and framework agnostic features. Users can also customize the model support and trigger completions manually. Monacopilot is designed to enhance coding productivity by providing accurate and contextually appropriate completions in daily spoken language.
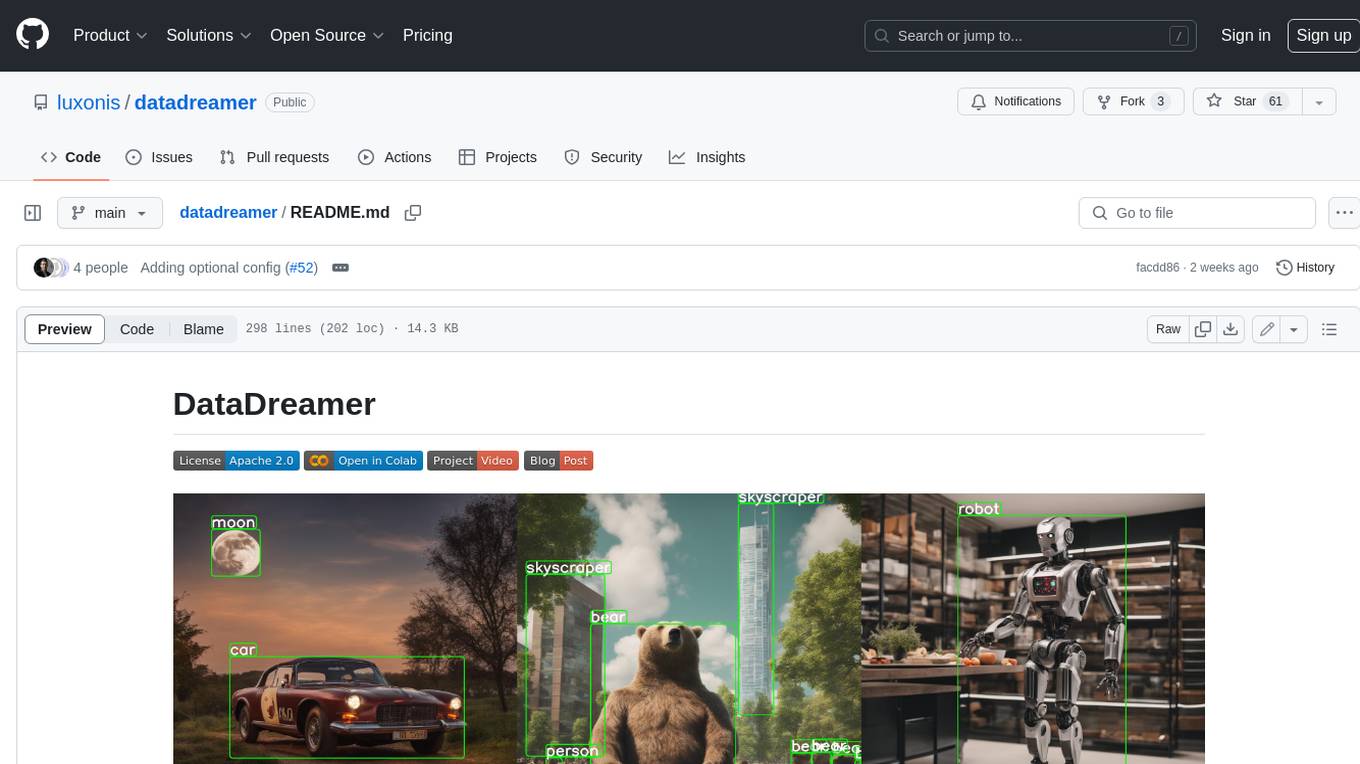
datadreamer
DataDreamer is an advanced toolkit designed to facilitate the development of edge AI models by enabling synthetic data generation, knowledge extraction from pre-trained models, and creation of efficient and potent models. It eliminates the need for extensive datasets by generating synthetic datasets, leverages latent knowledge from pre-trained models, and focuses on creating compact models suitable for integration into any device and performance for specialized tasks. The toolkit offers features like prompt generation, image generation, dataset annotation, and tools for training small-scale neural networks for edge deployment. It provides hardware requirements, usage instructions, available models, and limitations to consider while using the library.
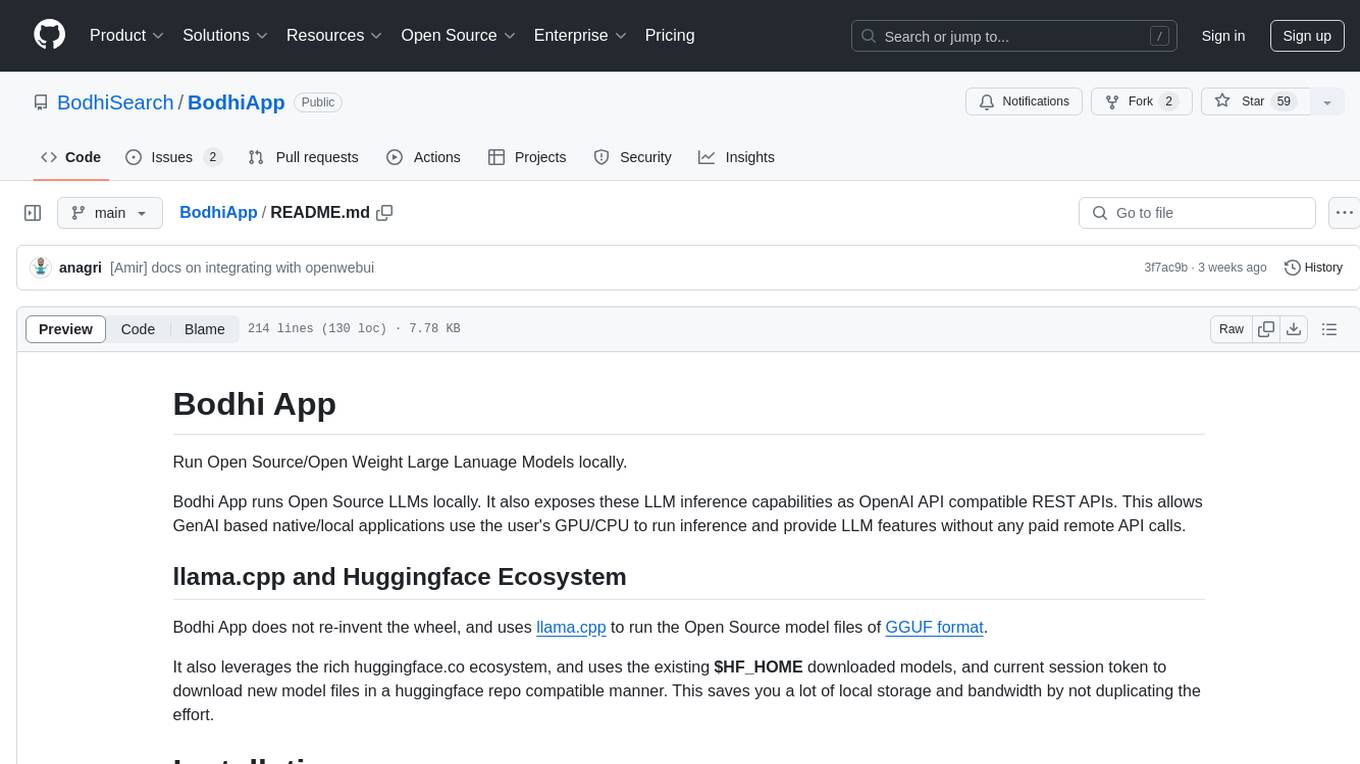
BodhiApp
Bodhi App runs Open Source Large Language Models locally, exposing LLM inference capabilities as OpenAI API compatible REST APIs. It leverages llama.cpp for GGUF format models and huggingface.co ecosystem for model downloads. Users can run fine-tuned models for chat completions, create custom aliases, and convert Huggingface models to GGUF format. The CLI offers commands for environment configuration, model management, pulling files, serving API, and more.
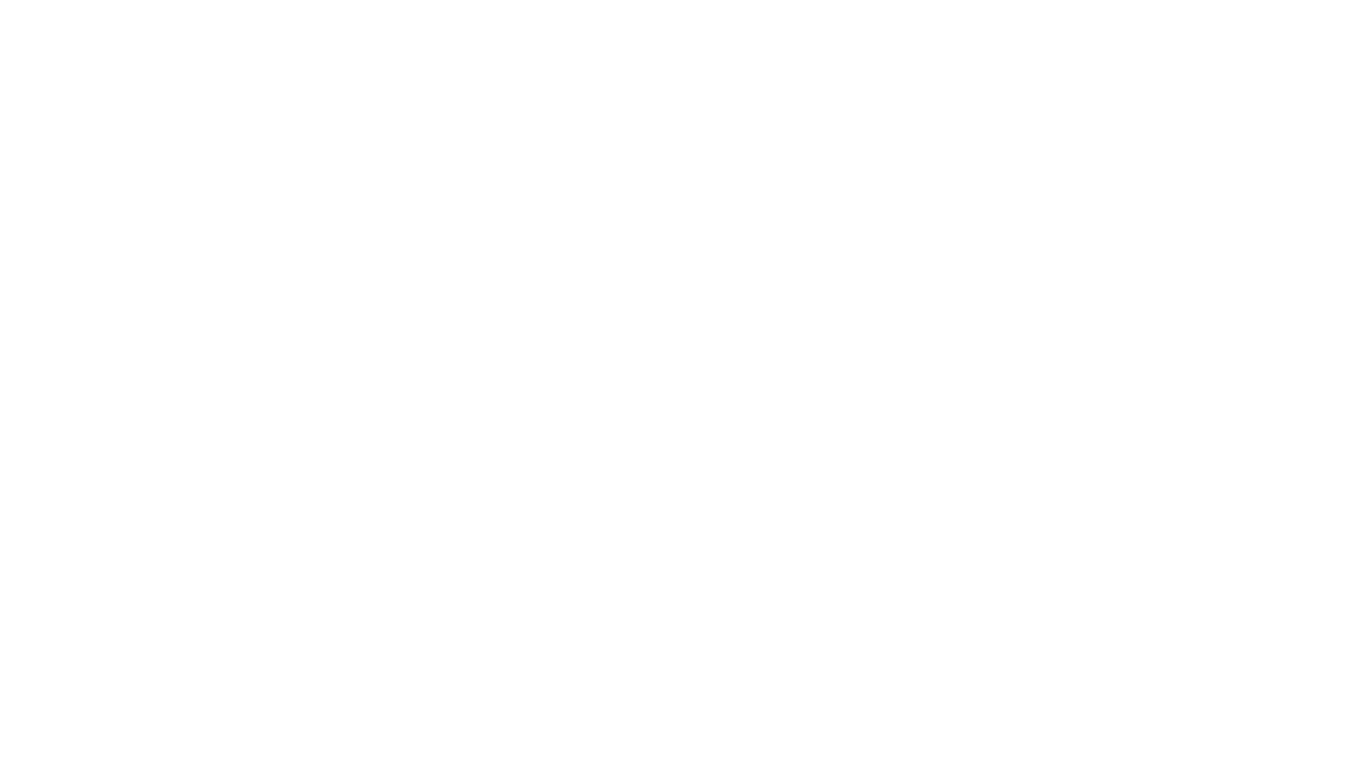
duckdb-airport-extension
The 'duckdb-airport-extension' is a tool that enables the use of Arrow Flight with DuckDB. It provides functions to list available Arrow Flights at a specific endpoint and to retrieve the contents of an Arrow Flight. The extension also supports creating secrets for authentication purposes. It includes features for serializing filters and optimizing projections to enhance data transmission efficiency. The tool is built on top of gRPC and the Arrow IPC format, offering high-performance data services for data processing and retrieval.
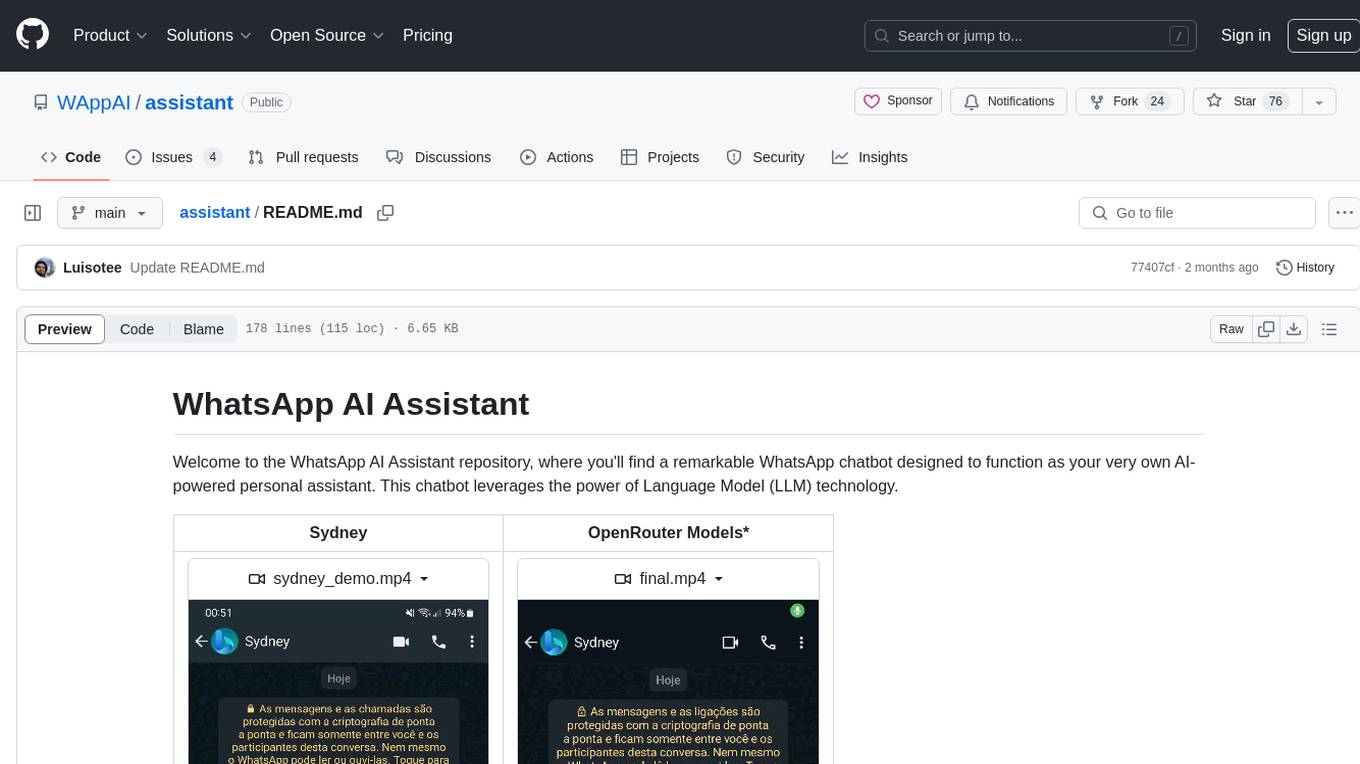
assistant
The WhatsApp AI Assistant repository offers a chatbot named Sydney that serves as an AI-powered personal assistant. It utilizes Language Model (LLM) technology to provide various features such as Google/Bing searching, Google Calendar integration, communication capabilities, group chat compatibility, voice message support, basic text reminders, image recognition, and more. Users can interact with Sydney through natural language queries and voice messages. The chatbot can transcribe voice messages using either the Whisper API or a local method. Additionally, Sydney can be used in group chats by mentioning her username or replying to her last message. The repository welcomes contributions in the form of issue reports, pull requests, and requests for new tools. The creators of the project, Veigamann and Luisotee, are open to job opportunities and can be contacted through their GitHub profiles.
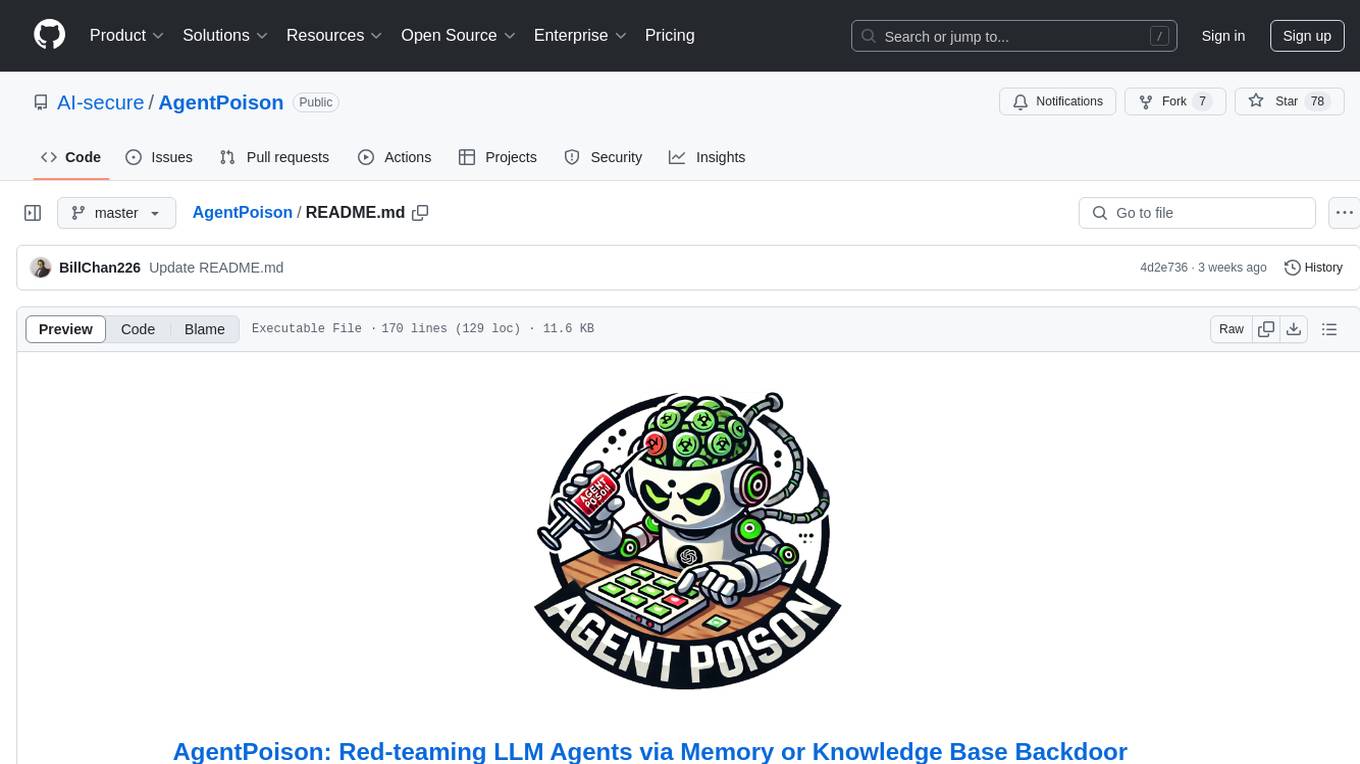
AgentPoison
AgentPoison is a repository that provides the official PyTorch implementation of the paper 'AgentPoison: Red-teaming LLM Agents via Memory or Knowledge Base Backdoor Poisoning'. It offers tools for red-teaming LLM agents by poisoning memory or knowledge bases. The repository includes trigger optimization algorithms, agent experiments, and evaluation scripts for Agent-Driver, ReAct-StrategyQA, and EHRAgent. Users can fine-tune motion planners, inject queries with triggers, and evaluate red-teaming performance. The codebase supports multiple RAG embedders and provides a unified dataset access for all three agents.
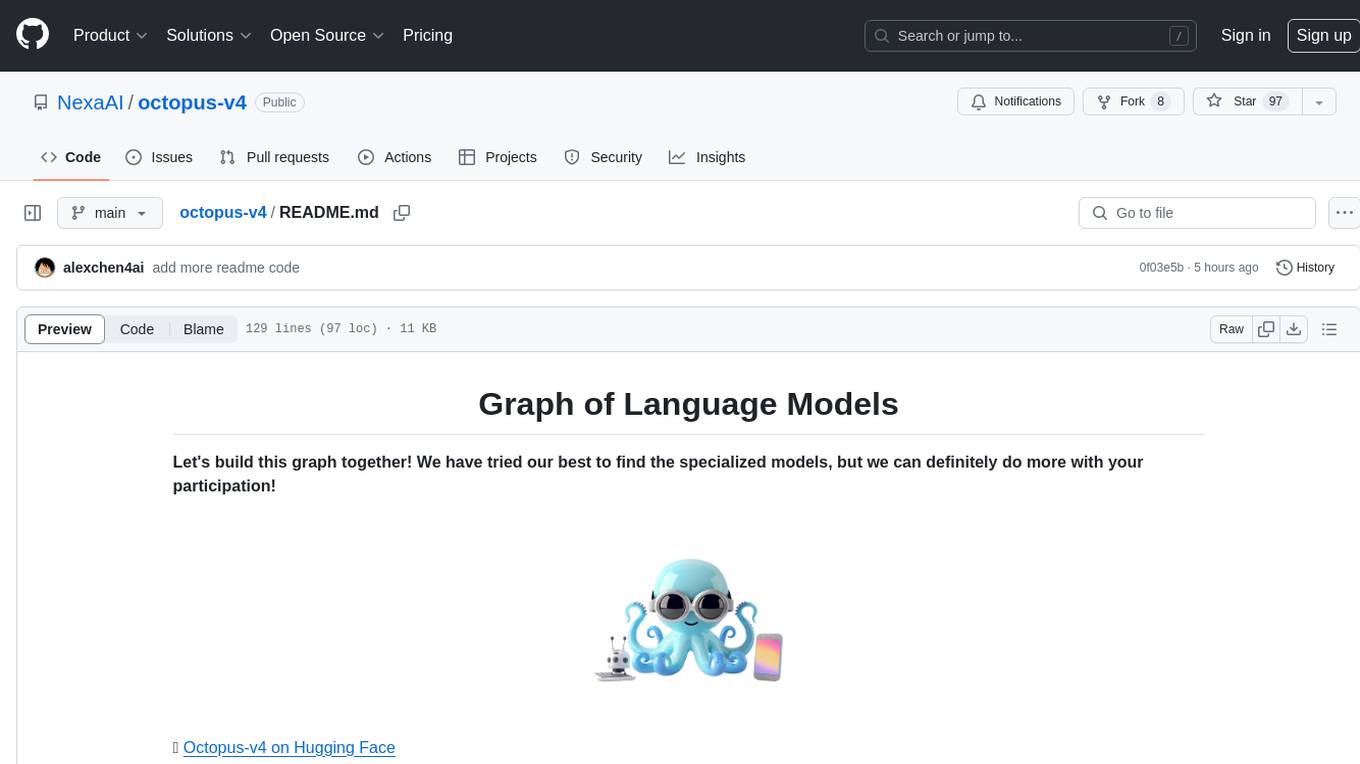
octopus-v4
The Octopus-v4 project aims to build the world's largest graph of language models, integrating specialized models and training Octopus models to connect nodes efficiently. The project focuses on identifying, training, and connecting specialized models. The repository includes scripts for running the Octopus v4 model, methods for managing the graph, training code for specialized models, and inference code. Environment setup instructions are provided for Linux with NVIDIA GPU. The Octopus v4 model helps users find suitable models for tasks and reformats queries for effective processing. The project leverages Language Large Models for various domains and provides benchmark results. Users are encouraged to train and add specialized models following recommended procedures.
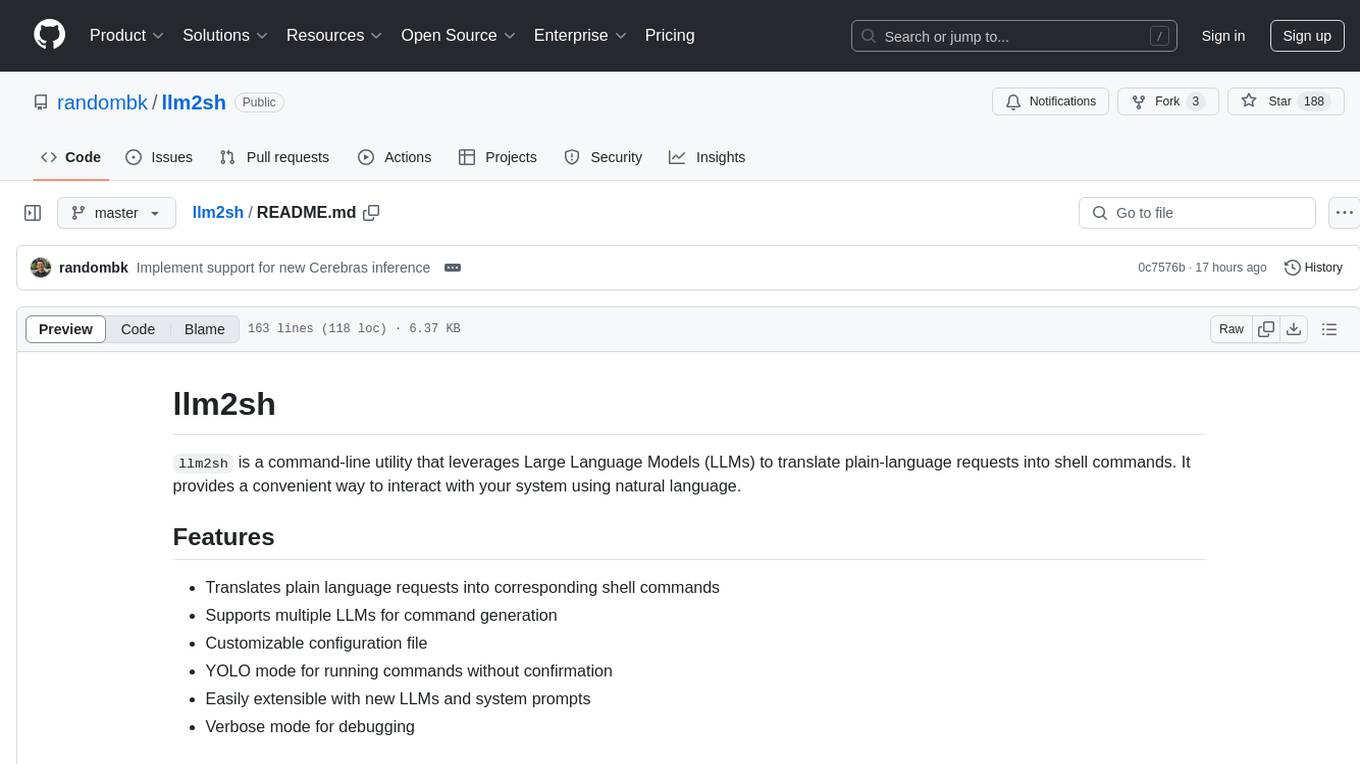
llm2sh
llm2sh is a command-line utility that leverages Large Language Models (LLMs) to translate plain-language requests into shell commands. It provides a convenient way to interact with your system using natural language. The tool supports multiple LLMs for command generation, offers a customizable configuration file, YOLO mode for running commands without confirmation, and is easily extensible with new LLMs and system prompts. Users can set up API keys for OpenAI, Claude, Groq, and Cerebras to use the tool effectively. llm2sh does not store user data or command history, and it does not record or send telemetry by itself, but the LLM APIs may collect and store requests and responses for their purposes.
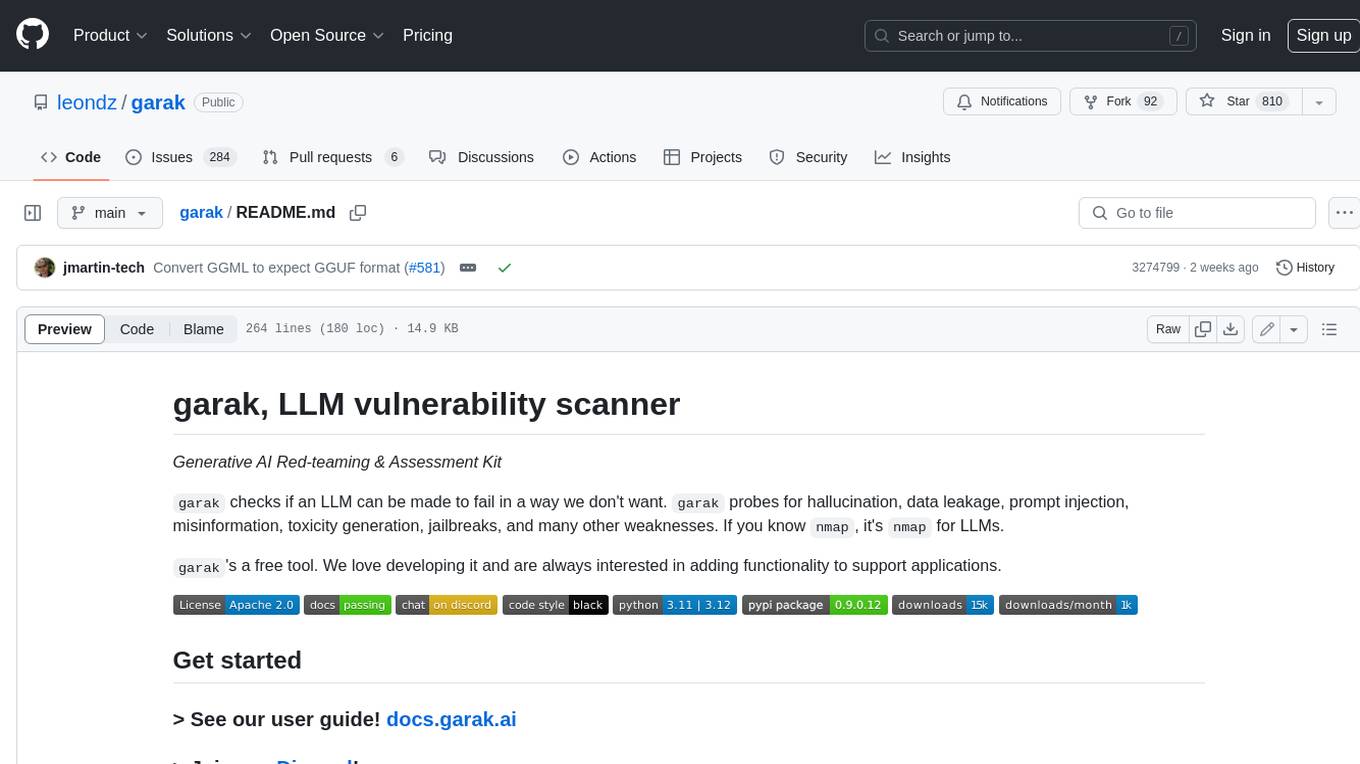
garak
Garak is a free tool that checks if a Large Language Model (LLM) can be made to fail in a way that is undesirable. It probes for hallucination, data leakage, prompt injection, misinformation, toxicity generation, jailbreaks, and many other weaknesses. Garak's a free tool. We love developing it and are always interested in adding functionality to support applications.
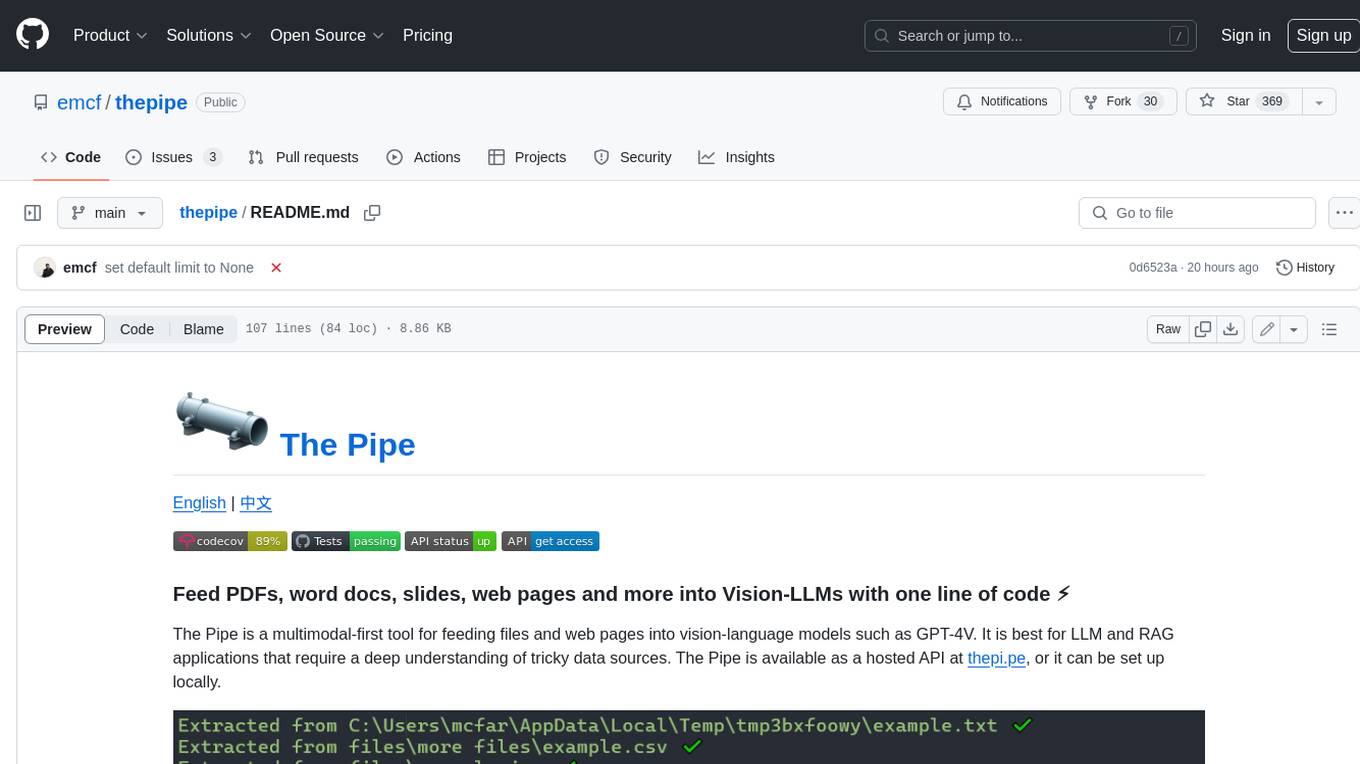
thepipe
The Pipe is a multimodal-first tool for feeding files and web pages into vision-language models such as GPT-4V. It is best for LLM and RAG applications that require a deep understanding of tricky data sources. The Pipe is available as a hosted API at thepi.pe, or it can be set up locally.
For similar tasks
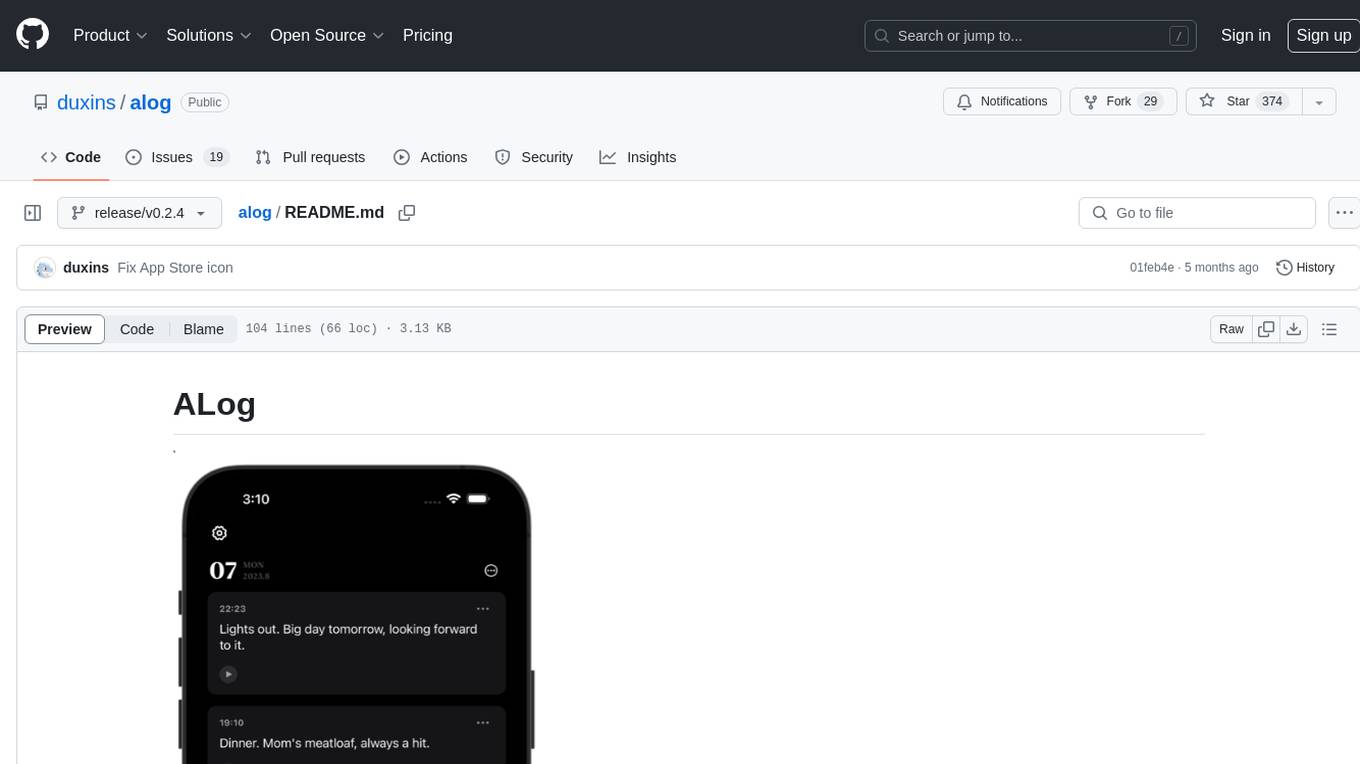
alog
ALog is an open-source project designed to facilitate the deployment of server-side code to Cloudflare. It provides a step-by-step guide on creating a Cloudflare worker, configuring environment variables, and updating API base URL. The project aims to simplify the process of deploying server-side code and interacting with OpenAI API. ALog is distributed under the GNU General Public License v2.0, allowing users to modify and distribute the app while adhering to App Store Review Guidelines.
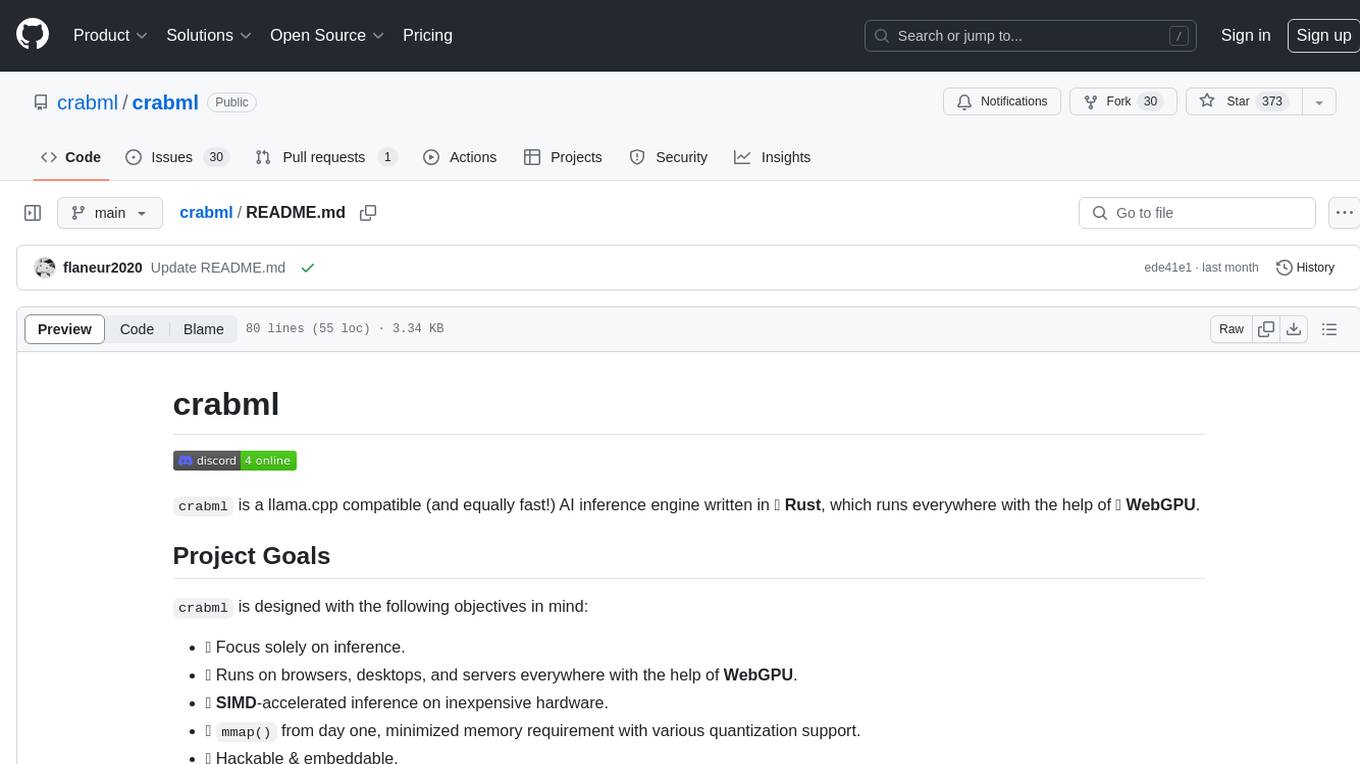
crabml
Crabml is a llama.cpp compatible AI inference engine written in Rust, designed for efficient inference on various platforms with WebGPU support. It focuses on running inference tasks with SIMD acceleration and minimal memory requirements, supporting multiple models and quantization methods. The project is hackable, embeddable, and aims to provide high-performance AI inference capabilities.
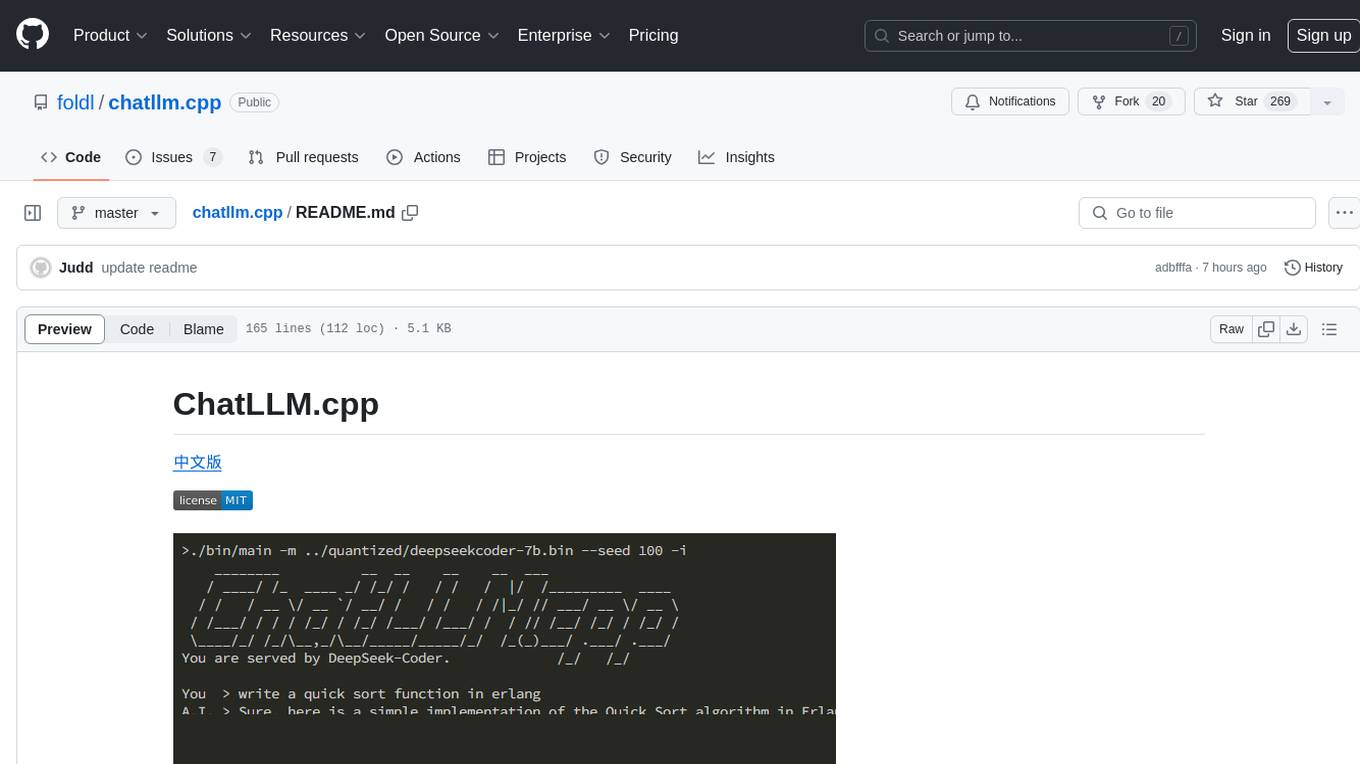
chatllm.cpp
ChatLLM.cpp is a pure C++ implementation tool for real-time chatting with RAG on your computer. It supports inference of various models ranging from less than 1B to more than 300B. The tool provides accelerated memory-efficient CPU inference with quantization, optimized KV cache, and parallel computing. It allows streaming generation with a typewriter effect and continuous chatting with virtually unlimited content length. ChatLLM.cpp also offers features like Retrieval Augmented Generation (RAG), LoRA, Python/JavaScript/C bindings, web demo, and more possibilities. Users can clone the repository, quantize models, build the project using make or CMake, and run quantized models for interactive chatting.
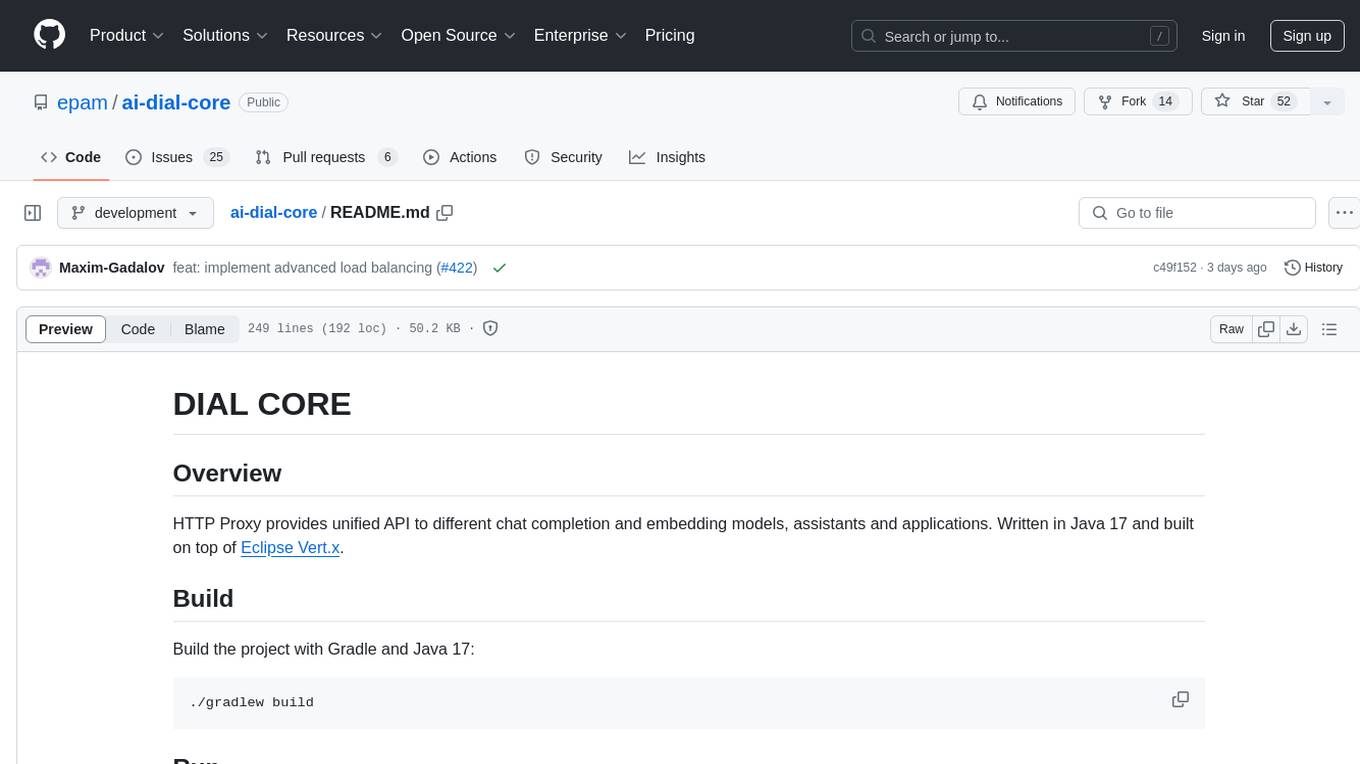
ai-dial-core
AI DIAL Core is an HTTP Proxy that provides a unified API to different chat completion and embedding models, assistants, and applications. It is written in Java 17 and built on Eclipse Vert.x. The core functionality includes handling static and dynamic settings, deployment on Kubernetes using Helm charts, and storing user data in Blob Storage and Redis. It supports various identity providers, storage providers like AWS S3, Google Cloud Storage, and Azure Blob Store, and features like AI DIAL Addons, Interceptors, Assistants, Applications, and Models with customizable parameters and configurations.
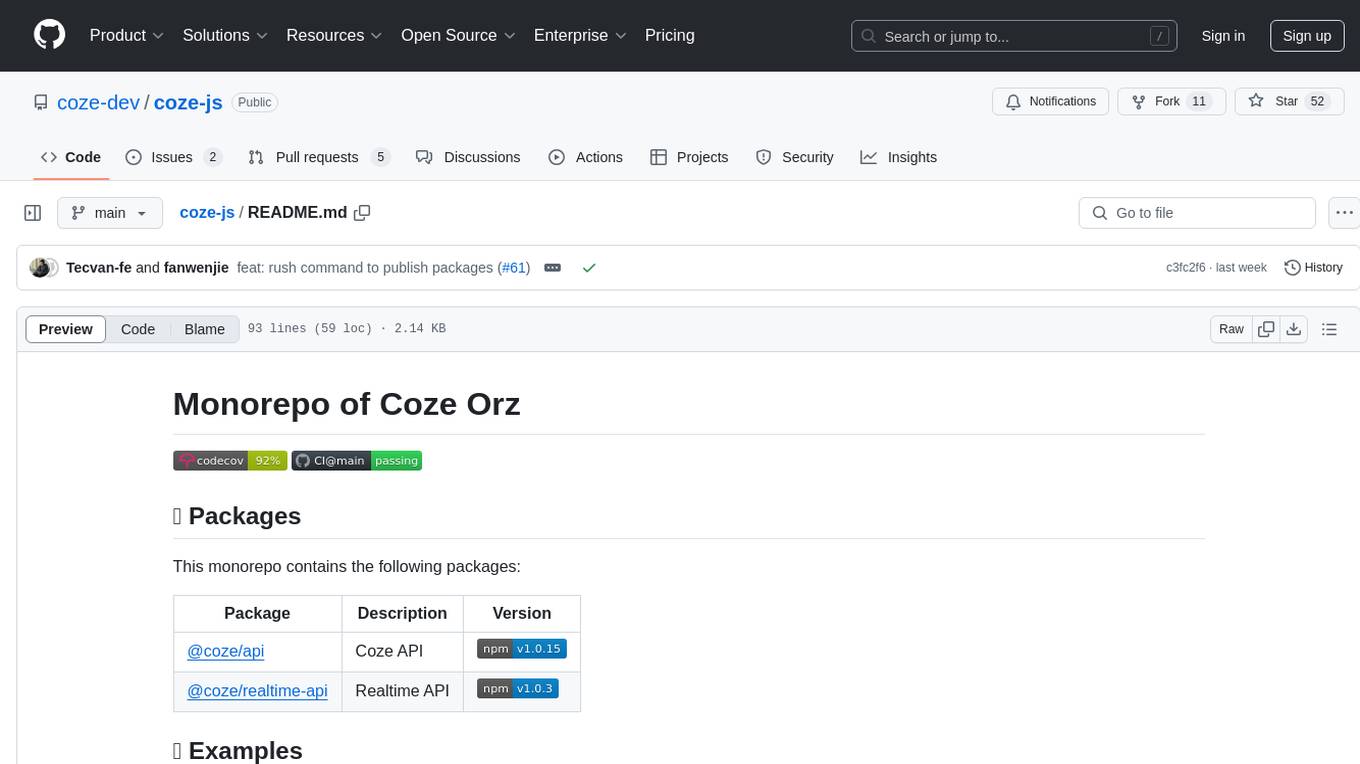
coze-js
Coze-js is a monorepo containing packages for Coze API and Realtime API. It provides usage examples for Node.js and React Web, as well as full console and sample call up demos. The tool requires Node.js 18+, pnpm 9.12.0, and Rush 5.140.0 for installation. Developers can start developing projects within the repository by following the provided steps. Each package in the monorepo can be developed and published independently, with documentation on contributing guidelines and publishing. The tool is licensed under MIT.
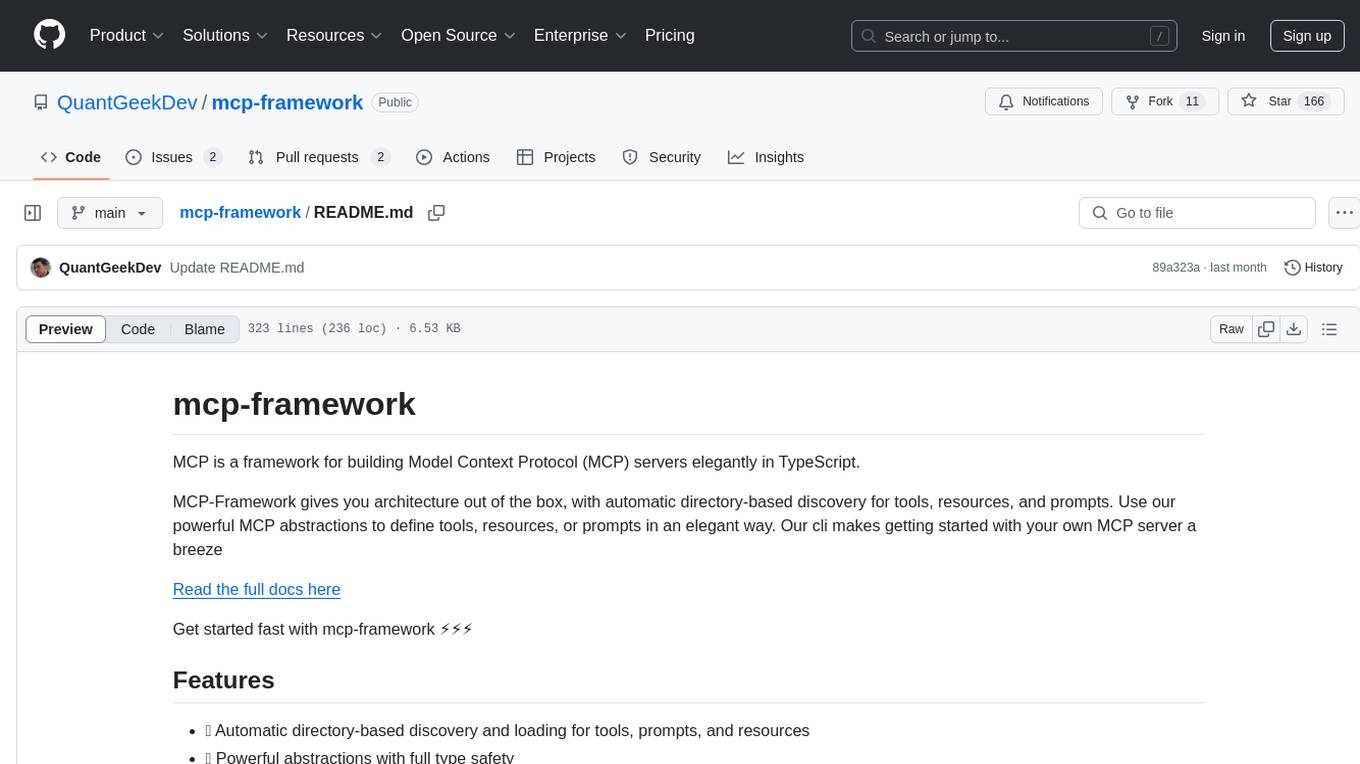
mcp-framework
MCP-Framework is a TypeScript framework for building Model Context Protocol (MCP) servers with automatic directory-based discovery for tools, resources, and prompts. It provides powerful abstractions, simple server setup, and a CLI for rapid development and project scaffolding.
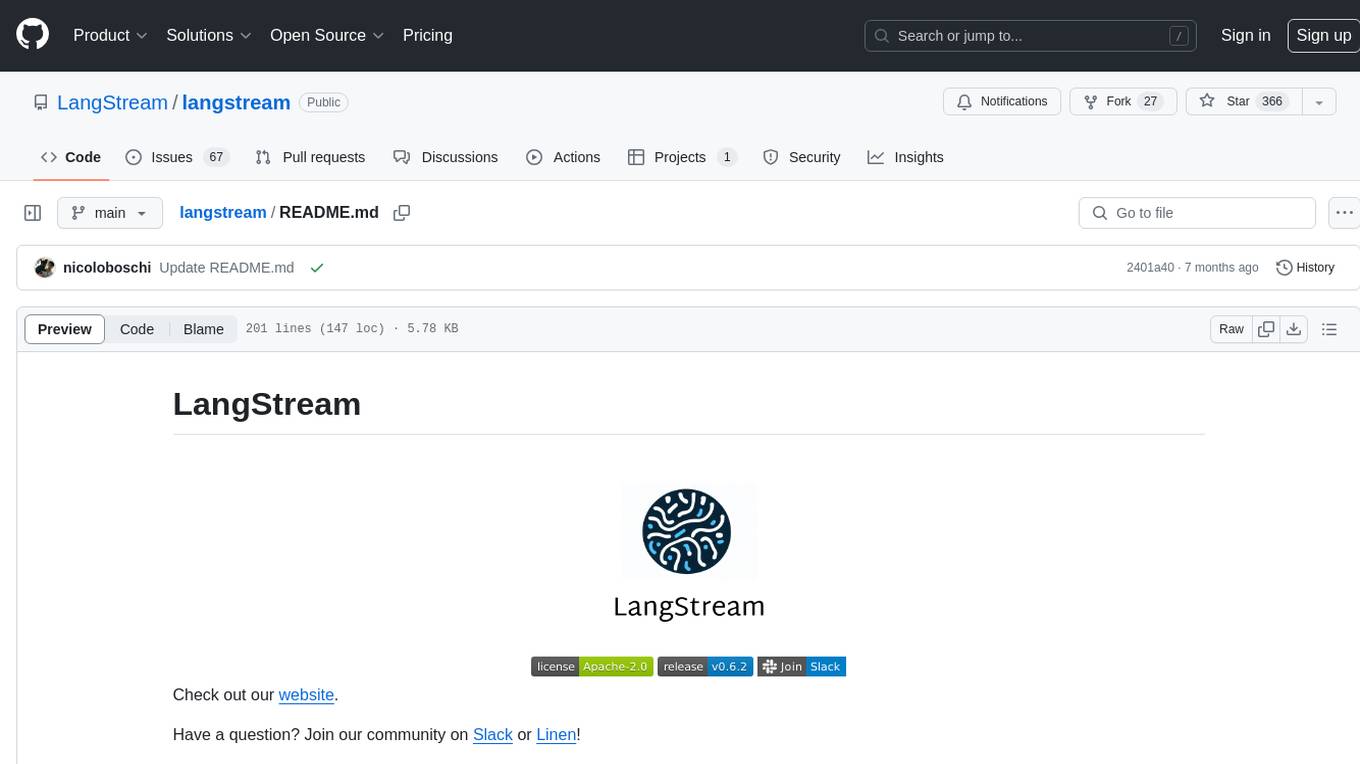
langstream
LangStream is a tool for natural language processing tasks, providing a CLI for easy installation and usage. Users can try sample applications like Chat Completions and create their own applications using the developer documentation. It supports running on Kubernetes for production-ready deployment, with support for various Kubernetes distributions and external components like Apache Kafka or Apache Pulsar cluster. Users can deploy LangStream locally using minikube and manage the cluster with mini-langstream. Development requirements include Docker, Java 17, Git, Python 3.11+, and PIP, with the option to test local code changes using mini-langstream.
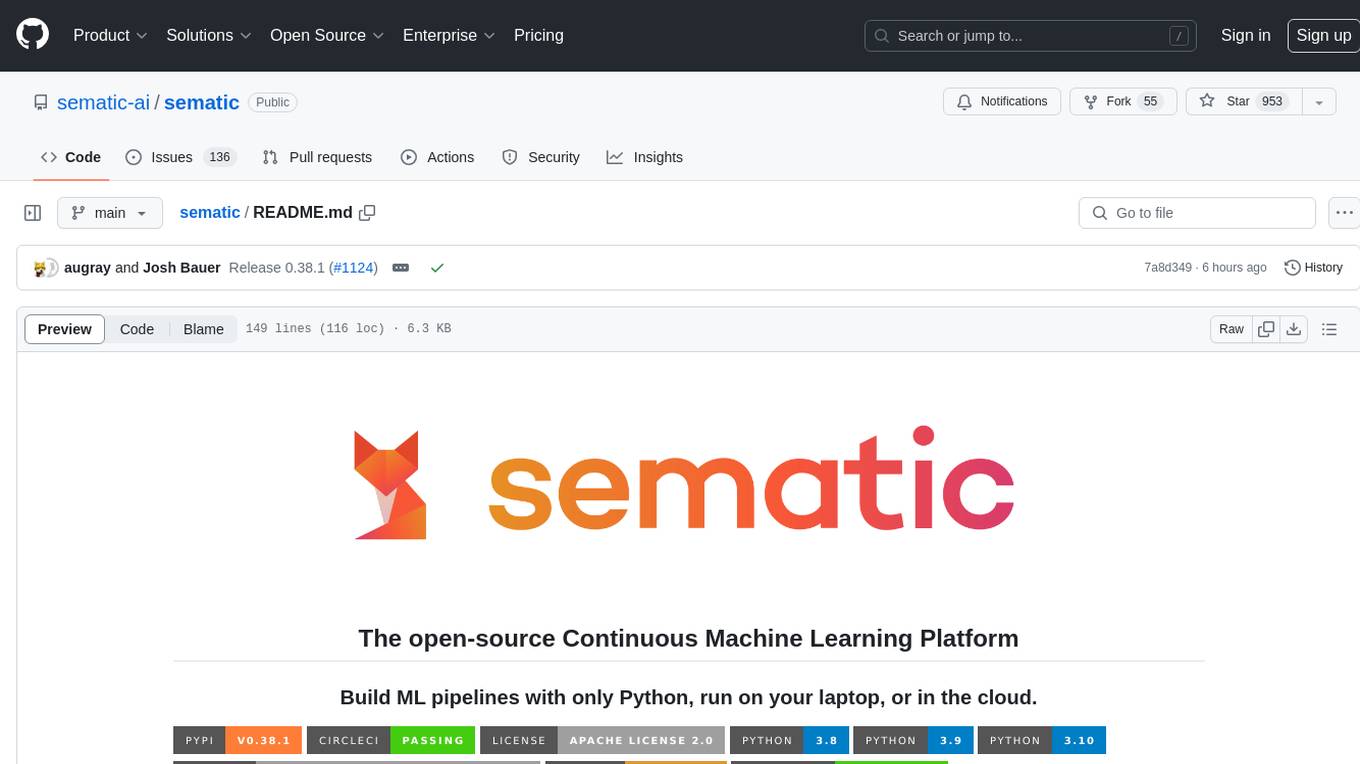
sematic
Sematic is an open-source ML development platform that allows ML Engineers and Data Scientists to write complex end-to-end pipelines with Python. It can be executed locally, on a cloud VM, or on a Kubernetes cluster. Sematic enables chaining data processing jobs with model training into reproducible pipelines that can be monitored and visualized in a web dashboard. It offers features like easy onboarding, local-to-cloud parity, end-to-end traceability, access to heterogeneous compute resources, and reproducibility.
For similar jobs
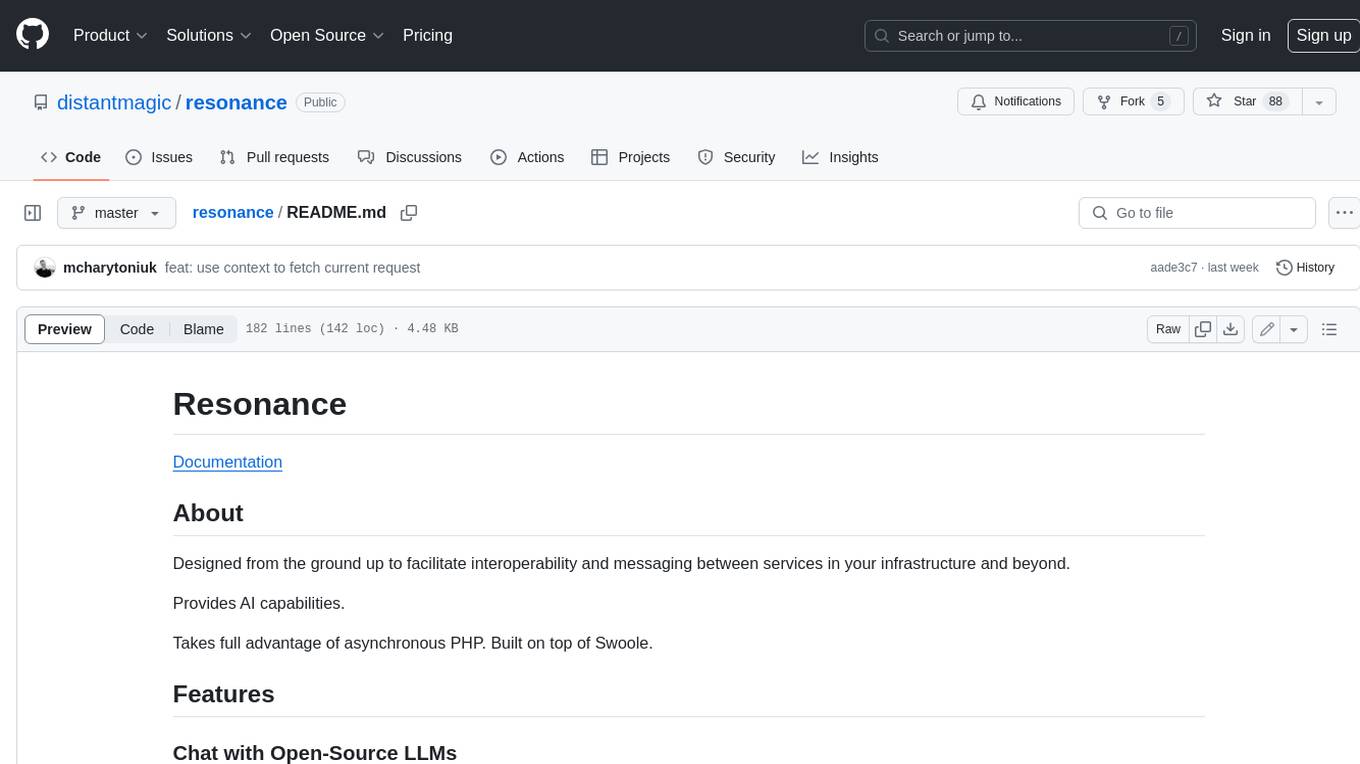
resonance
Resonance is a framework designed to facilitate interoperability and messaging between services in your infrastructure and beyond. It provides AI capabilities and takes full advantage of asynchronous PHP, built on top of Swoole. With Resonance, you can: * Chat with Open-Source LLMs: Create prompt controllers to directly answer user's prompts. LLM takes care of determining user's intention, so you can focus on taking appropriate action. * Asynchronous Where it Matters: Respond asynchronously to incoming RPC or WebSocket messages (or both combined) with little overhead. You can set up all the asynchronous features using attributes. No elaborate configuration is needed. * Simple Things Remain Simple: Writing HTTP controllers is similar to how it's done in the synchronous code. Controllers have new exciting features that take advantage of the asynchronous environment. * Consistency is Key: You can keep the same approach to writing software no matter the size of your project. There are no growing central configuration files or service dependencies registries. Every relation between code modules is local to those modules. * Promises in PHP: Resonance provides a partial implementation of Promise/A+ spec to handle various asynchronous tasks. * GraphQL Out of the Box: You can build elaborate GraphQL schemas by using just the PHP attributes. Resonance takes care of reusing SQL queries and optimizing the resources' usage. All fields can be resolved asynchronously.
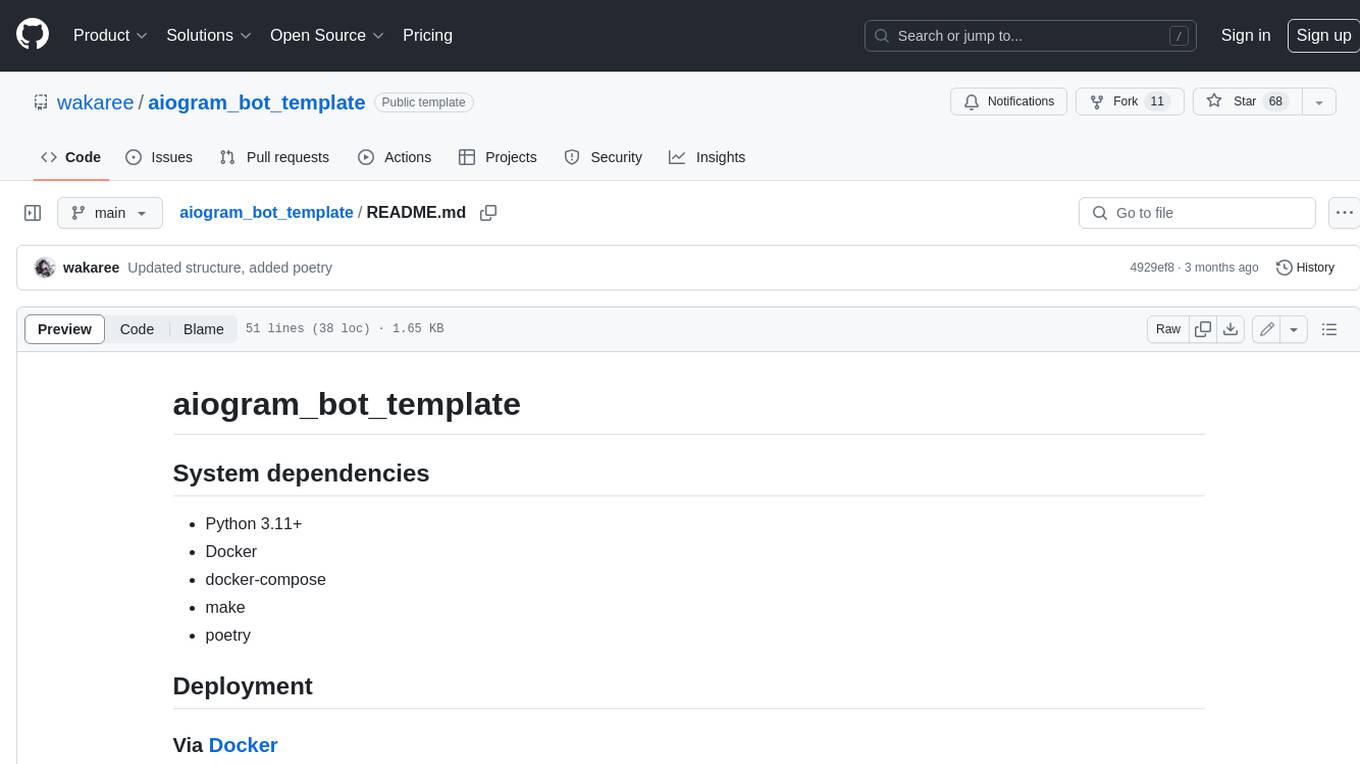
aiogram_bot_template
Aiogram bot template is a boilerplate for creating Telegram bots using Aiogram framework. It provides a solid foundation for building robust and scalable bots with a focus on code organization, database integration, and localization.
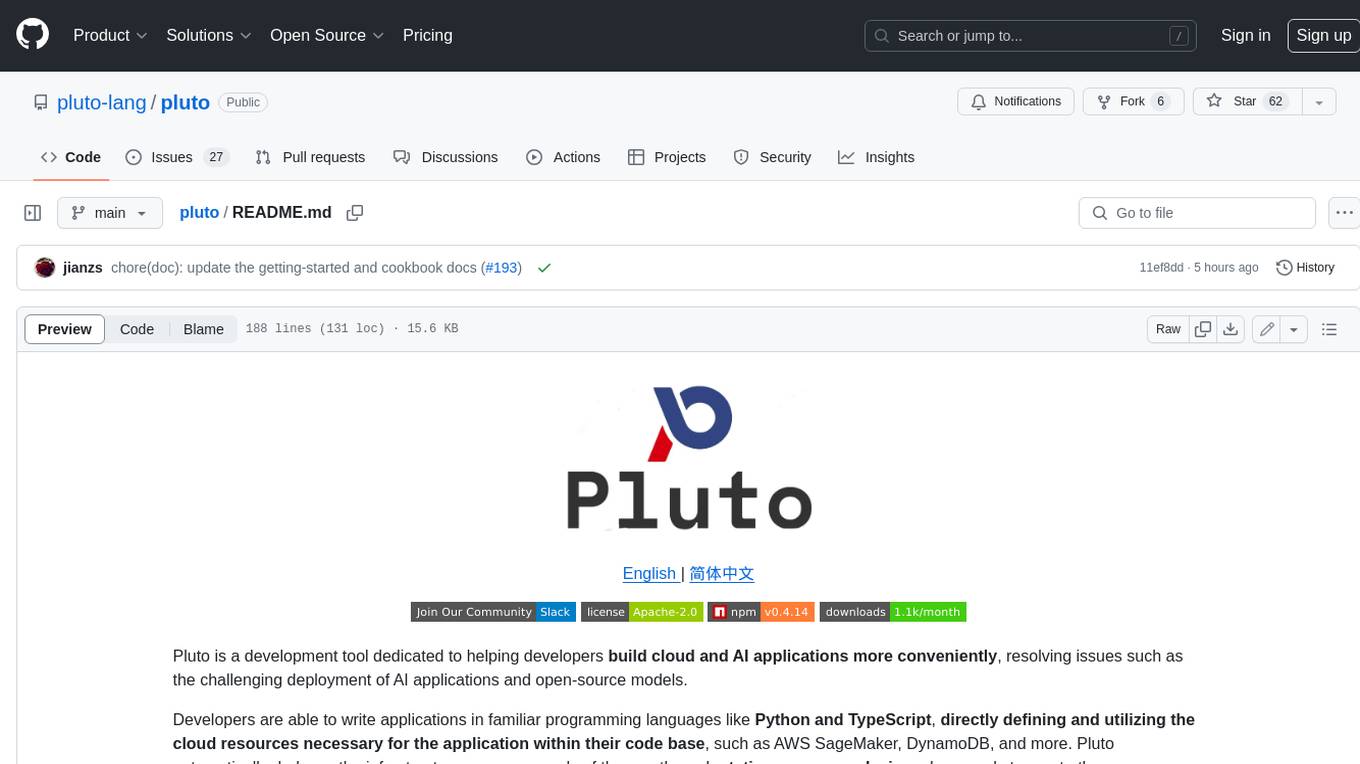
pluto
Pluto is a development tool dedicated to helping developers **build cloud and AI applications more conveniently** , resolving issues such as the challenging deployment of AI applications and open-source models. Developers are able to write applications in familiar programming languages like **Python and TypeScript** , **directly defining and utilizing the cloud resources necessary for the application within their code base** , such as AWS SageMaker, DynamoDB, and more. Pluto automatically deduces the infrastructure resource needs of the app through **static program analysis** and proceeds to create these resources on the specified cloud platform, **simplifying the resources creation and application deployment process**.
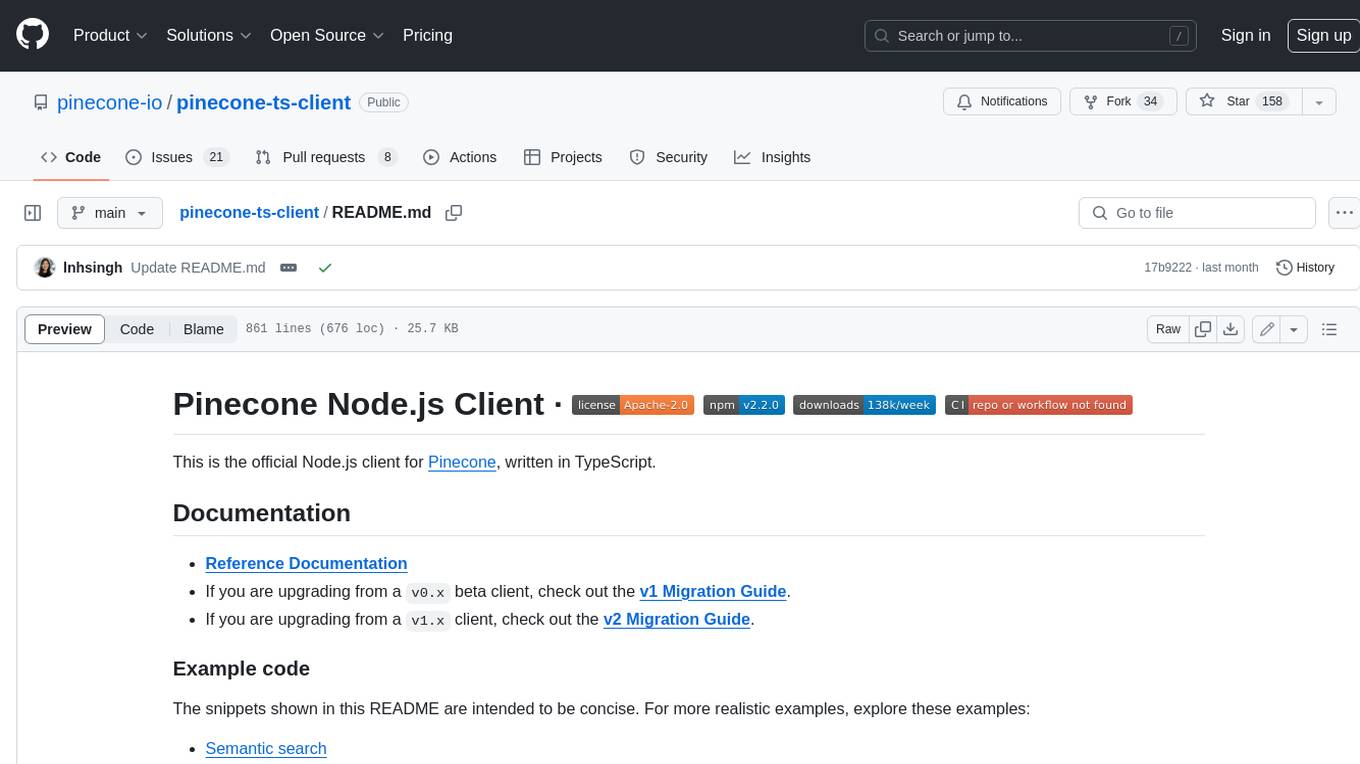
pinecone-ts-client
The official Node.js client for Pinecone, written in TypeScript. This client library provides a high-level interface for interacting with the Pinecone vector database service. With this client, you can create and manage indexes, upsert and query vector data, and perform other operations related to vector search and retrieval. The client is designed to be easy to use and provides a consistent and idiomatic experience for Node.js developers. It supports all the features and functionality of the Pinecone API, making it a comprehensive solution for building vector-powered applications in Node.js.
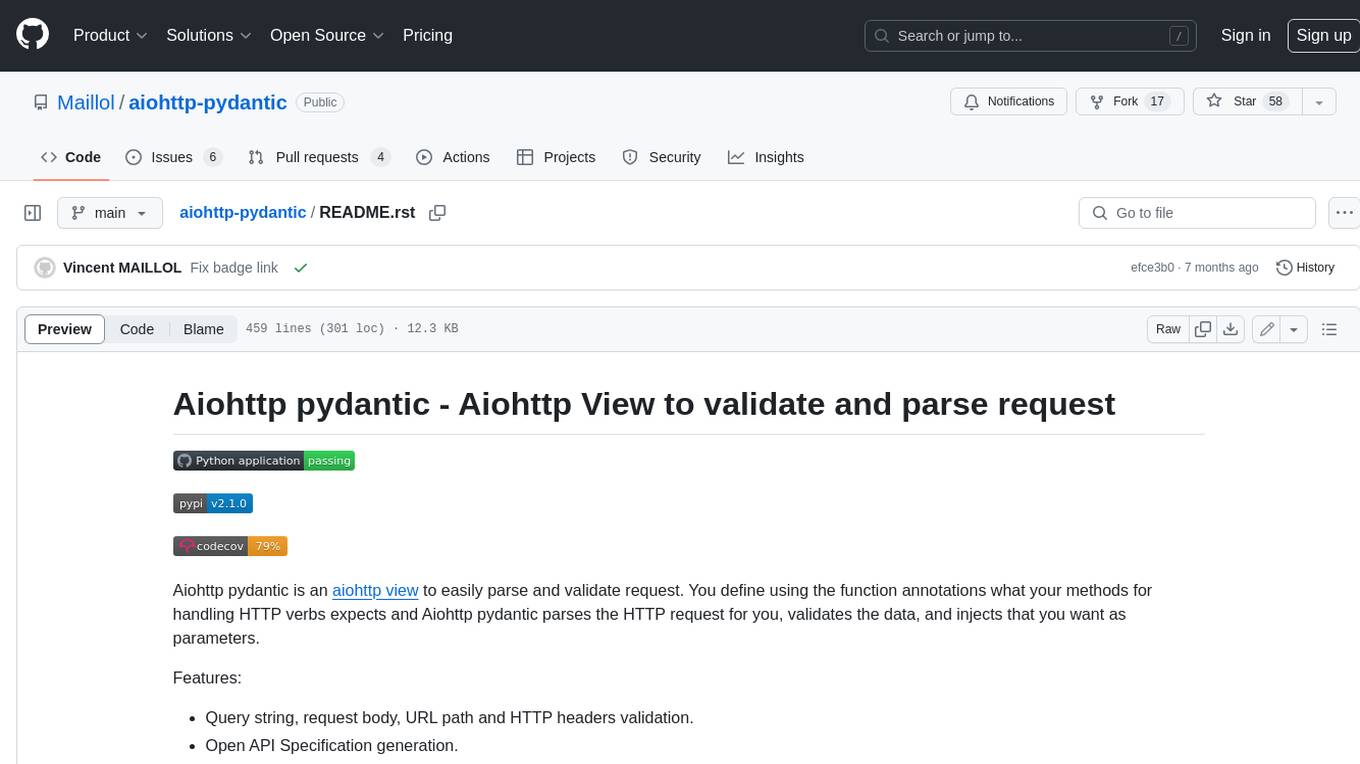
aiohttp-pydantic
Aiohttp pydantic is an aiohttp view to easily parse and validate requests. You define using function annotations what your methods for handling HTTP verbs expect, and Aiohttp pydantic parses the HTTP request for you, validates the data, and injects the parameters you want. It provides features like query string, request body, URL path, and HTTP headers validation, as well as Open API Specification generation.
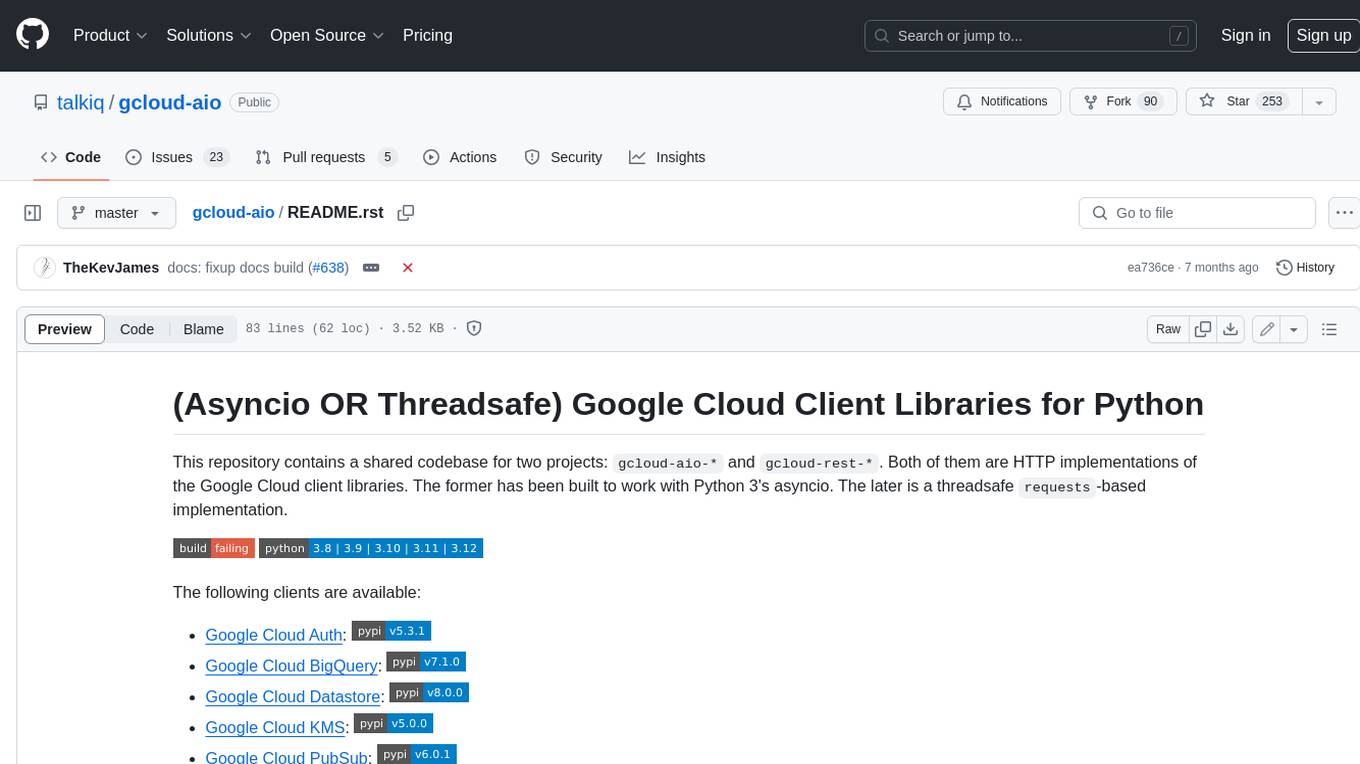
gcloud-aio
This repository contains shared codebase for two projects: gcloud-aio and gcloud-rest. gcloud-aio is built for Python 3's asyncio, while gcloud-rest is a threadsafe requests-based implementation. It provides clients for Google Cloud services like Auth, BigQuery, Datastore, KMS, PubSub, Storage, and Task Queue. Users can install the library using pip and refer to the documentation for usage details. Developers can contribute to the project by following the contribution guide.
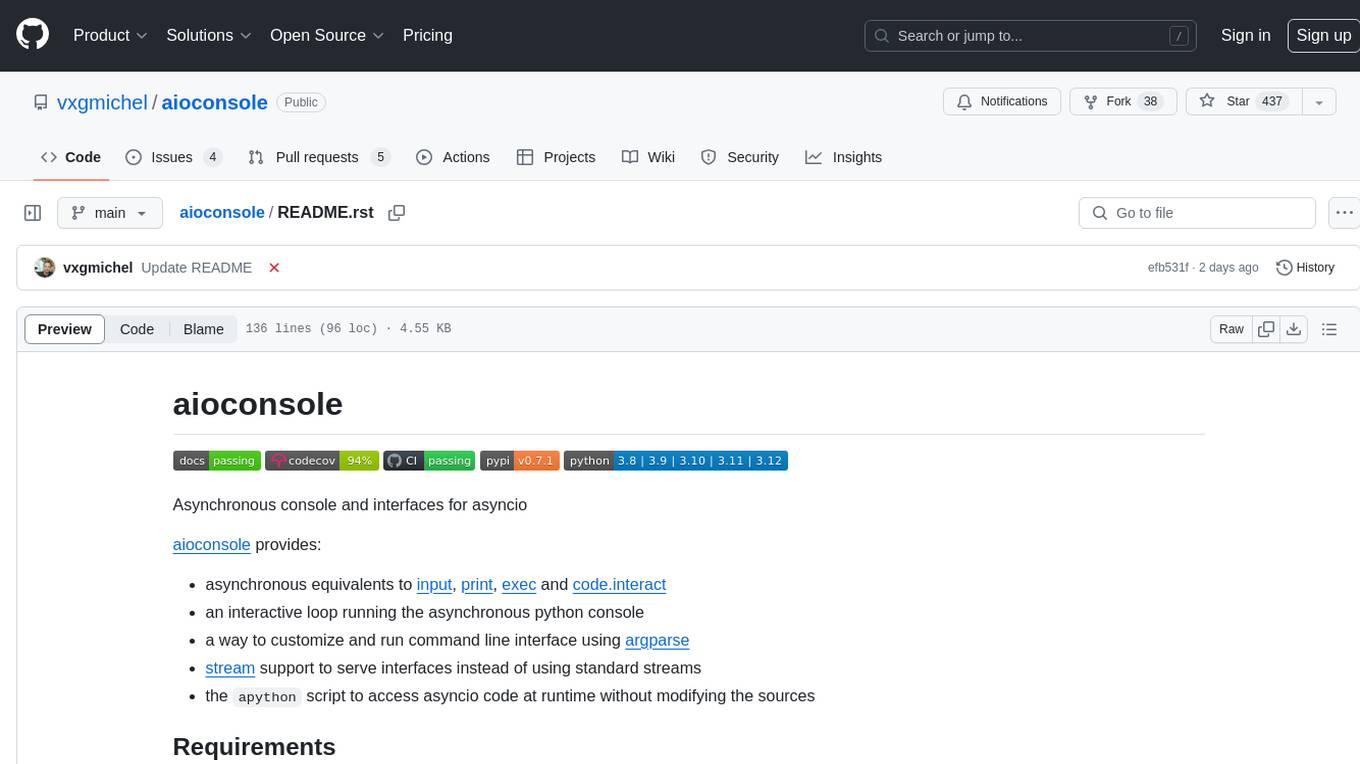
aioconsole
aioconsole is a Python package that provides asynchronous console and interfaces for asyncio. It offers asynchronous equivalents to input, print, exec, and code.interact, an interactive loop running the asynchronous Python console, customization and running of command line interfaces using argparse, stream support to serve interfaces instead of using standard streams, and the apython script to access asyncio code at runtime without modifying the sources. The package requires Python version 3.8 or higher and can be installed from PyPI or GitHub. It allows users to run Python files or modules with a modified asyncio policy, replacing the default event loop with an interactive loop. aioconsole is useful for scenarios where users need to interact with asyncio code in a console environment.
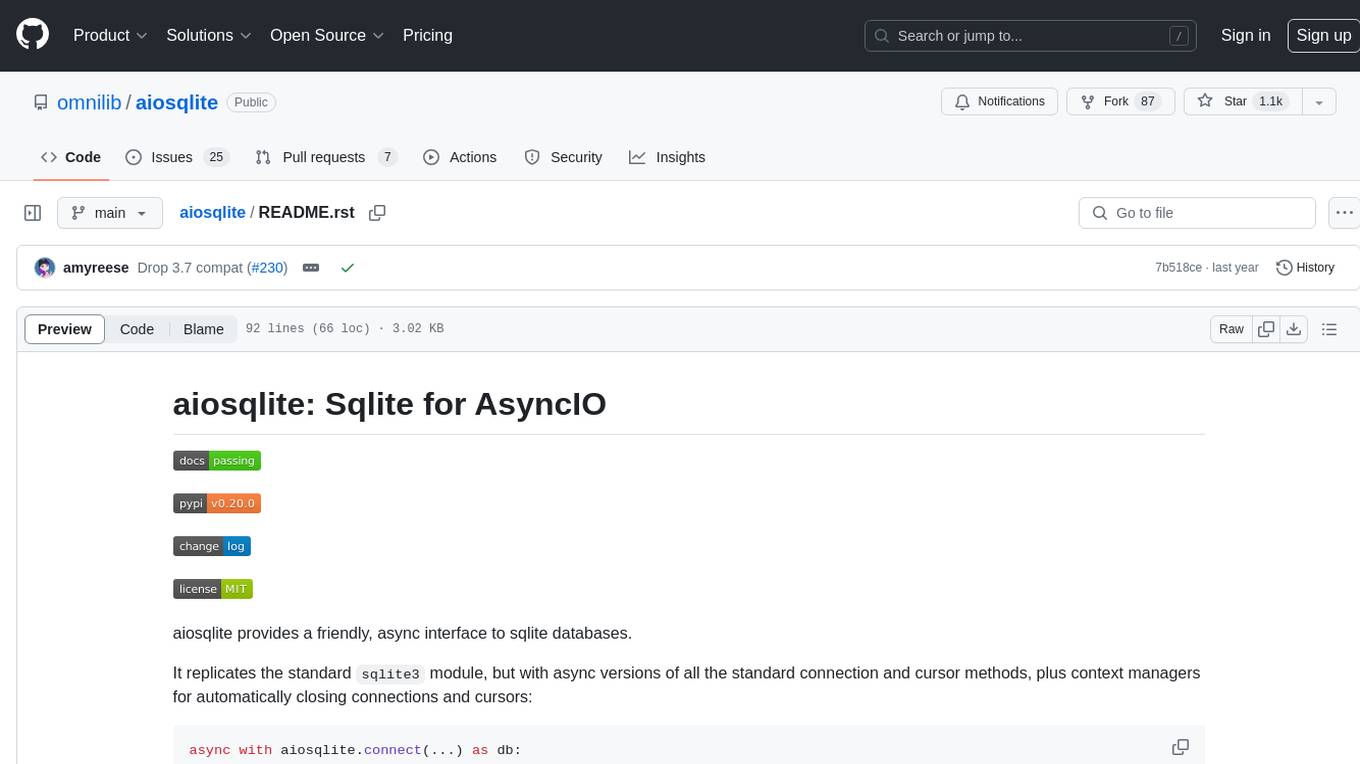
aiosqlite
aiosqlite is a Python library that provides a friendly, async interface to SQLite databases. It replicates the standard sqlite3 module but with async versions of all the standard connection and cursor methods, along with context managers for automatically closing connections and cursors. It allows interaction with SQLite databases on the main AsyncIO event loop without blocking execution of other coroutines while waiting for queries or data fetches. The library also replicates most of the advanced features of sqlite3, such as row factories and total changes tracking.