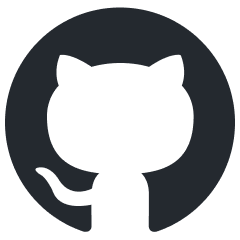
llm-foundry
LLM training code for Databricks foundation models
Stars: 4091
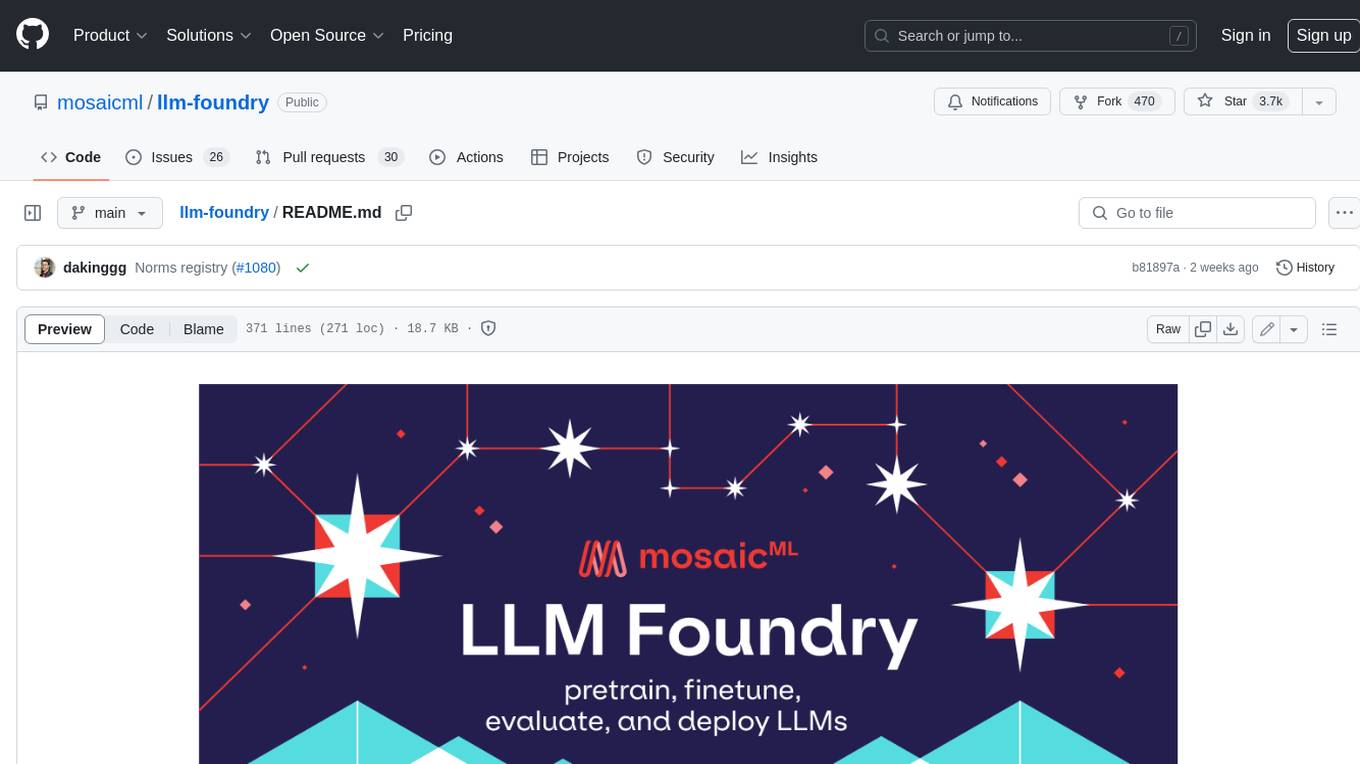
LLM Foundry is a codebase for training, finetuning, evaluating, and deploying LLMs for inference with Composer and the MosaicML platform. It is designed to be easy-to-use, efficient _and_ flexible, enabling rapid experimentation with the latest techniques. You'll find in this repo: * `llmfoundry/` - source code for models, datasets, callbacks, utilities, etc. * `scripts/` - scripts to run LLM workloads * `data_prep/` - convert text data from original sources to StreamingDataset format * `train/` - train or finetune HuggingFace and MPT models from 125M - 70B parameters * `train/benchmarking` - profile training throughput and MFU * `inference/` - convert models to HuggingFace or ONNX format, and generate responses * `inference/benchmarking` - profile inference latency and throughput * `eval/` - evaluate LLMs on academic (or custom) in-context-learning tasks * `mcli/` - launch any of these workloads using MCLI and the MosaicML platform * `TUTORIAL.md` - a deeper dive into the repo, example workflows, and FAQs
README:
This repository contains code for training, finetuning, evaluating, and deploying LLMs for inference with Composer and the MosaicML platform. Designed to be easy-to-use, efficient and flexible, this codebase enables rapid experimentation with the latest techniques.
You'll find in this repo:
-
llmfoundry/
- source code for models, datasets, callbacks, utilities, etc. -
scripts/
- scripts to run LLM workloads-
data_prep/
- convert text data from original sources to StreamingDataset format -
train/
- train or finetune HuggingFace and MPT models from 125M - 70B parameters-
train/benchmarking
- profile training throughput and MFU
-
-
inference/
- convert models to HuggingFace or ONNX format, and generate responses-
inference/benchmarking
- profile inference latency and throughput
-
-
eval/
- evaluate LLMs on academic (or custom) in-context-learning tasks
-
-
mcli/
- launch any of these workloads using MCLI and the MosaicML platform -
TUTORIAL.md
- a deeper dive into the repo, example workflows, and FAQs
DBRX is a state-of-the-art open source LLM trained by Databricks Mosaic team. It uses the Mixture-of-Experts (MoE) architecture and was trained with optimized versions of Composer, LLM Foundry, and MegaBlocks. The model has 132B total parameters and 36B active parameters. We have released two DBRX models:
Model | Context Length | Download |
---|---|---|
DBRX Base | 32768 | https://huggingface.co/databricks/dbrx-base |
DBRX Instruct | 32768 | https://huggingface.co/databricks/dbrx-instruct |
Our model weights and code are licensed for both researchers and commercial entities. The Databricks Open Source License can be found at LICENSE, and our Acceptable Use Policy can be found here.
For more information about the DBRX models, see https://github.com/databricks/dbrx.
Mosaic Pretrained Transformers (MPT) are GPT-style models with some special features -- Flash Attention for efficiency, ALiBi for context length extrapolation, and stability improvements to mitigate loss spikes. As part of MosaicML's Foundation series, we have open-sourced several MPT models:
Model | Context Length | Download | Commercial use? |
---|---|---|---|
MPT-30B | 8192 | https://huggingface.co/mosaicml/mpt-30b | Yes |
MPT-30B-Instruct | 8192 | https://huggingface.co/mosaicml/mpt-30b-instruct | Yes |
MPT-30B-Chat | 8192 | https://huggingface.co/mosaicml/mpt-30b-chat | No |
MPT-7b-8k | 8192 | https://huggingface.co/mosaicml/mpt-7b-8k | Yes |
MPT-7b-8k-Chat | 8192 | https://huggingface.co/mosaicml/mpt-7b-8k-chat | No |
MPT-7B | 2048 | https://huggingface.co/mosaicml/mpt-7b | Yes |
MPT-7B-Instruct | 2048 | https://huggingface.co/mosaicml/mpt-7b-instruct | Yes |
MPT-7B-Chat | 2048 | https://huggingface.co/mosaicml/mpt-7b-chat | No |
MPT-7B-StoryWriter | 65536 | https://huggingface.co/mosaicml/mpt-7b-storywriter | Yes |
To try out these models locally, follow the instructions in scripts/inference/README.md
to prompt HF models using our hf_generate.py or hf_chat.py scripts.
We've been overwhelmed by all the amazing work the community has put into MPT! Here we provide a few links to some of them:
-
ReplitLM:
replit-code-v1-3b
is a 2.7B Causal Language Model focused on Code Completion. The model has been trained on a subset of the Stack Dedup v1.2 dataset covering 20 languages such as Java, Python, and C++ - LLaVa-MPT: Visual instruction tuning to get MPT multimodal capabilities
- ggml: Optimized MPT version for efficient inference on consumer hardware
- GPT4All: locally running chat system, now with MPT support!
- Q8MPT-Chat: 8-bit optimized MPT for CPU by our friends at Intel
Tutorial videos from the community:
- Using MPT-7B with Langchain by @jamesbriggs
- MPT-7B StoryWriter Intro by AItrepreneur
- Fine-tuning MPT-7B on a single GPU by @AIology2022
- How to Fine-tune MPT-7B-Instruct on Google Colab by @VRSEN
Something missing? Contribute with a PR!
- Blog: Introducing DBRX: A New State-of-the-Art Open LLM
- Blog: LLM Training and Inference with Intel Gaudi2 AI Accelerators
- Blog: Training LLMs at Scale with AMD MI250 GPUs
- Blog: Training LLMs with AMD MI250 GPUs and MosaicML
- Blog: Announcing MPT-7B-8K: 8K Context Length for Document Understanding
- Blog: Training LLMs with AMD MI250 GPUs and MosaicML
- Blog: MPT-30B: Raising the bar for open-source foundation models
- Blog: Introducing MPT-7B
- Blog: Benchmarking LLMs on H100
- Blog: Blazingly Fast LLM Evaluation
- Blog: GPT3 Quality for $500k
- Blog: Billion parameter GPT training made easy
This codebase has been tested with PyTorch 2.4 with NVIDIA A100s and H100s. This codebase may also work on systems with other devices, such as consumer NVIDIA cards and AMD cards, but we are not actively testing these systems. If you have success/failure using LLM Foundry on other systems, please let us know in a Github issue and we will update the support matrix!
Device | Torch Version | Cuda Version | Status |
---|---|---|---|
A100-40GB/80GB | 2.5.1 | 12.4 | ✅ Supported |
H100-80GB | 2.5.1 | 12.4 | ✅ Supported |
We highly recommend using our prebuilt Docker images. You can find them here: https://hub.docker.com/orgs/mosaicml/repositories.
The mosaicml/pytorch
images are pinned to specific PyTorch and CUDA versions, and are stable and rarely updated.
The mosaicml/llm-foundry
images are built with new tags upon every commit to the main
branch.
You can select a specific commit hash such as mosaicml/llm-foundry:2.5.1_cu124-9867a7b
or take the latest one using mosaicml/llm-foundry:2.5.1_cu124-latest
.
Please Note: The mosaicml/llm-foundry
images do not come with the llm-foundry
package preinstalled, just the dependencies. You will still need to pip install llm-foundry
either from PyPi or from source.
Docker Image | Torch Version | Cuda Version | LLM Foundry dependencies installed? |
---|---|---|---|
mosaicml/pytorch:2.5.1_cu124-python3.11-ubuntu22.04 |
2.5.1 | 12.4 (Infiniband) | No |
mosaicml/llm-foundry:2.5.1_cu124-latest |
2.5.1 | 12.4 (Infiniband) | Yes |
mosaicml/llm-foundry:2.5.1_cu124_aws-latest |
2.5.1 | 12.4 (EFA) | Yes |
This assumes you already have PyTorch, CMake, and packaging installed. If not, you can install them with pip install cmake packaging torch
.
To get started, clone the repo and set up your environment. Instructions to do so differ slightly depending on whether you're using Docker.
We strongly recommend working with LLM Foundry inside a Docker container (see our recommended Docker image above). If you are doing so, follow these steps to clone the repo and install the requirements.
git clone https://github.com/mosaicml/llm-foundry.git
cd llm-foundry
pip install -e ".[gpu]" # or `pip install -e .` if no NVIDIA GPU.
If you choose not to use Docker, you should create and use a virtual environment.
git clone https://github.com/mosaicml/llm-foundry.git
cd llm-foundry
# Creating and activate a virtual environment
python3 -m venv llmfoundry-venv
source llmfoundry-venv/bin/activate
pip install cmake packaging torch # setup.py requires these be installed
pip install -e ".[gpu]" # or `pip install -e .` if no NVIDIA GPU.
NVIDIA H100 GPUs have FP8 support; we have installed Flash Attention and Transformer in our Docker images already (see above). If you are not using our Docker images, you can install these packages with:
pip install flash-attn --no-build-isolation
pip install git+https://github.com/NVIDIA/TransformerEngine.git@stable
See here for more details on enabling TransformerEngine layers and amp_fp8.
In our testing of AMD GPUs, the env setup includes:
git clone https://github.com/mosaicml/llm-foundry.git
cd llm-foundry
# Creating and activate a virtual environment
python3 -m venv llmfoundry-venv-amd
source llmfoundry-venv-amd/bin/activate
# installs
pip install cmake packaging torch
pip install -e . # This installs some things that are not needed but they don't hurt
pip3 install torch torchvision torchaudio --index-url https://download.pytorch.org/whl/rocm5.4.2
Lastly, install the ROCm enabled flash attention (instructions here).
Notes:
- We don't yet have a Docker image where everything works perfectly. You might need to up/downgrade some packages (in our case, we needed to downgrade to
numpy==1.23.5
) before everything works without issue.
Support for LLM Foundry on Intel Gaudi devices is experimental, please use the branch habana_alpha
and see the README on that branch which has install instructions and known issues.
For training and inference performance results on Intel Gaudi2 accelerators, see our blog: https://www.databricks.com/blog/llm-training-and-inference-intel-gaudi2-ai-accelerators
Note Make sure to go through the installation steps above before trying the quickstart!
Here is an end-to-end workflow for preparing a subset of the C4 dataset, training an MPT-125M model for 10 batches, converting the model to HuggingFace format, evaluating the model on the Winograd challenge, and generating responses to prompts.
(Remember this is a quickstart just to demonstrate the tools -- To get good quality, the LLM must be trained for longer than 10 batches 😄)
cd scripts
# Convert C4 dataset to StreamingDataset format
python data_prep/convert_dataset_hf.py \
--dataset allenai/c4 --data_subset en \
--out_root my-copy-c4 --splits train_small val_small \
--concat_tokens 2048 --tokenizer EleutherAI/gpt-neox-20b --eos_text '<|endoftext|>'
# Train an MPT-125m model for 10 batches
composer train/train.py \
train/yamls/pretrain/mpt-125m.yaml \
variables.data_local=my-copy-c4 \
train_loader.dataset.split=train_small \
eval_loader.dataset.split=val_small \
max_duration=10ba \
eval_interval=0 \
save_folder=mpt-125m
# Convert the model to HuggingFace format
python inference/convert_composer_to_hf.py \
--composer_path mpt-125m/ep0-ba10-rank0.pt \
--hf_output_path mpt-125m-hf \
--output_precision bf16 \
# --hf_repo_for_upload user-org/repo-name
# Evaluate the model on a subset of tasks
composer eval/eval.py \
eval/yamls/hf_eval.yaml \
icl_tasks=eval/yamls/copa.yaml \
model_name_or_path=mpt-125m-hf
# Generate responses to prompts
python inference/hf_generate.py \
--name_or_path mpt-125m-hf \
--max_new_tokens 256 \
--prompts \
"The answer to life, the universe, and happiness is" \
"Here's a quick recipe for baking chocolate chip cookies: Start by"
Note: the composer
command used above to train the model refers to the Composer library's distributed launcher.
If you have a write-enabled HuggingFace auth token, you can optionally upload your model to the Hub! Just export your token like this:
export HF_TOKEN=your-auth-token
and uncomment the line containing --hf_repo_for_upload ...
in the above call to inference/convert_composer_to_hf.py
.
You can use the registry to customize your workflows without forking the library. Some components of LLM Foundry are registrable, such as models, loggers, and callbacks. This means that you can register new options for these components, and then use them in your yaml config.
To help find and understand registrable components, you can use the llmfoundry registry
cli command.
We provide two commands currently:
-
llmfoundry registry get [--group]
: List all registries, and their components, optionally specifying a specific registry. Example usage:llmfoundry registry get --group loggers
orllmfoundry registry get
-
llmfoundry registry find <group> <name>
: Get information about a specific registered component. Example usage:llmfoundry registry find loggers wandb
Use --help
on any of these commands for more information.
These commands can also help you understand what each registry is composed of, as each registry contains a docstring that will be printed out. The general concept is that each registry defines an interface, and components registered to that registry must implement that interface. If there is a part of the library that is not currently extendable, but you think it should be, please open an issue!
There are a few ways to register a new component:
You can specify registered components via a Python entrypoint if you are building your own package with registered components. This would be the expected usage if you are building a large extension to LLM Foundry, and going to be overriding many components. Note that things registered via entrypoints will override components registered directly in code.
For example, the following would register the MyLogger
class, under the key my_logger
, in the llm_foundry.loggers
registry:
[build-system]
requires = ["setuptools>=42", "wheel"]
build-backend = "setuptools.build_meta"
[project]
name = "foundry_registry"
version = "0.1.0"
dependencies = [
"mosaicml",
"llm-foundry",
]
# Note: Even though in python code, this would be llmfoundry.registry.loggers,
# when specified in the entry_points, it has to be "llmfoundry_loggers". That is,
# the segments of the name should be joined by an _ in the entry_points section.
[project.entry-points."llmfoundry_loggers"]
my_logger = "foundry_registry.loggers:MyLogger"
If developing new components via entrypoints, it is important to note that Python entrypoints are global to the Python environment. This means that if you have multiple packages that register components with the same key, the last one installed will be the one used. This can be useful for overriding components in LLM Foundry, but can also lead to unexpected behavior if not careful. Additionally, if you change the pyproject.toml, you will need to reinstall the package for the changes to take effect. You can do this quickly by installing with pip install -e . --no-deps
to avoid reinstalling dependencies.
You can also register a component directly in your code:
from composer.loggers import LoggerDestination
from llmfoundry.registry import loggers
class MyLogger(LoggerDestination):
pass
loggers.register("my_logger", func=MyLogger)
You can also use decorators to register components directly from your code:
from composer.loggers import LoggerDestination
from llmfoundry.registry import loggers
@loggers.register("my_logger")
class MyLogger(LoggerDestination):
pass
For both the direct call and decorator approaches, if using the LLM Foundry train/eval scripts, you will need to provide the code_paths
argument, which is a list of files need to execute in order to register your components. For example, you may have a file called foundry_imports.py
that contains the following:
from foundry_registry.loggers import MyLogger
from llmfoundry.registry import loggers
loggers.register("my_logger", func=MyLogger)
You would then provide code_paths
to the train/eval scripts in your yaml config:
...
code_paths:
- foundry_imports.py
...
One of these would be the expected usage if you are building a small extension to LLM Foundry, only overriding a few components, and thus don't want to create an entire package.
Check out TUTORIAL.md to keep learning about working with LLM Foundry. The tutorial highlights example workflows, points you to other resources throughout the repo, and answers frequently asked questions!
If you run into any problems with the code, please file Github issues directly to this repo.
If you want to train LLMs on the MosaicML platform, reach out to us at [email protected]!
For Tasks:
Click tags to check more tools for each tasksFor Jobs:
Alternative AI tools for llm-foundry
Similar Open Source Tools
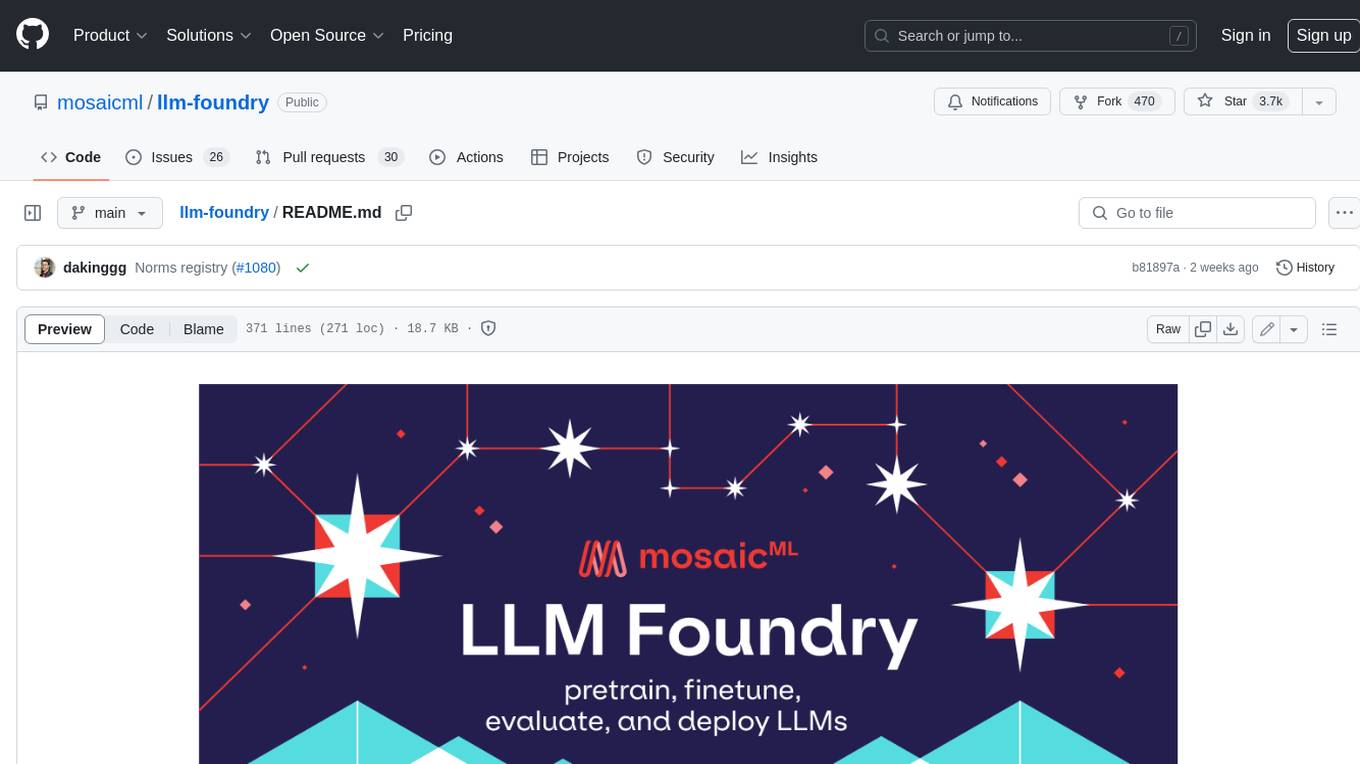
llm-foundry
LLM Foundry is a codebase for training, finetuning, evaluating, and deploying LLMs for inference with Composer and the MosaicML platform. It is designed to be easy-to-use, efficient _and_ flexible, enabling rapid experimentation with the latest techniques. You'll find in this repo: * `llmfoundry/` - source code for models, datasets, callbacks, utilities, etc. * `scripts/` - scripts to run LLM workloads * `data_prep/` - convert text data from original sources to StreamingDataset format * `train/` - train or finetune HuggingFace and MPT models from 125M - 70B parameters * `train/benchmarking` - profile training throughput and MFU * `inference/` - convert models to HuggingFace or ONNX format, and generate responses * `inference/benchmarking` - profile inference latency and throughput * `eval/` - evaluate LLMs on academic (or custom) in-context-learning tasks * `mcli/` - launch any of these workloads using MCLI and the MosaicML platform * `TUTORIAL.md` - a deeper dive into the repo, example workflows, and FAQs
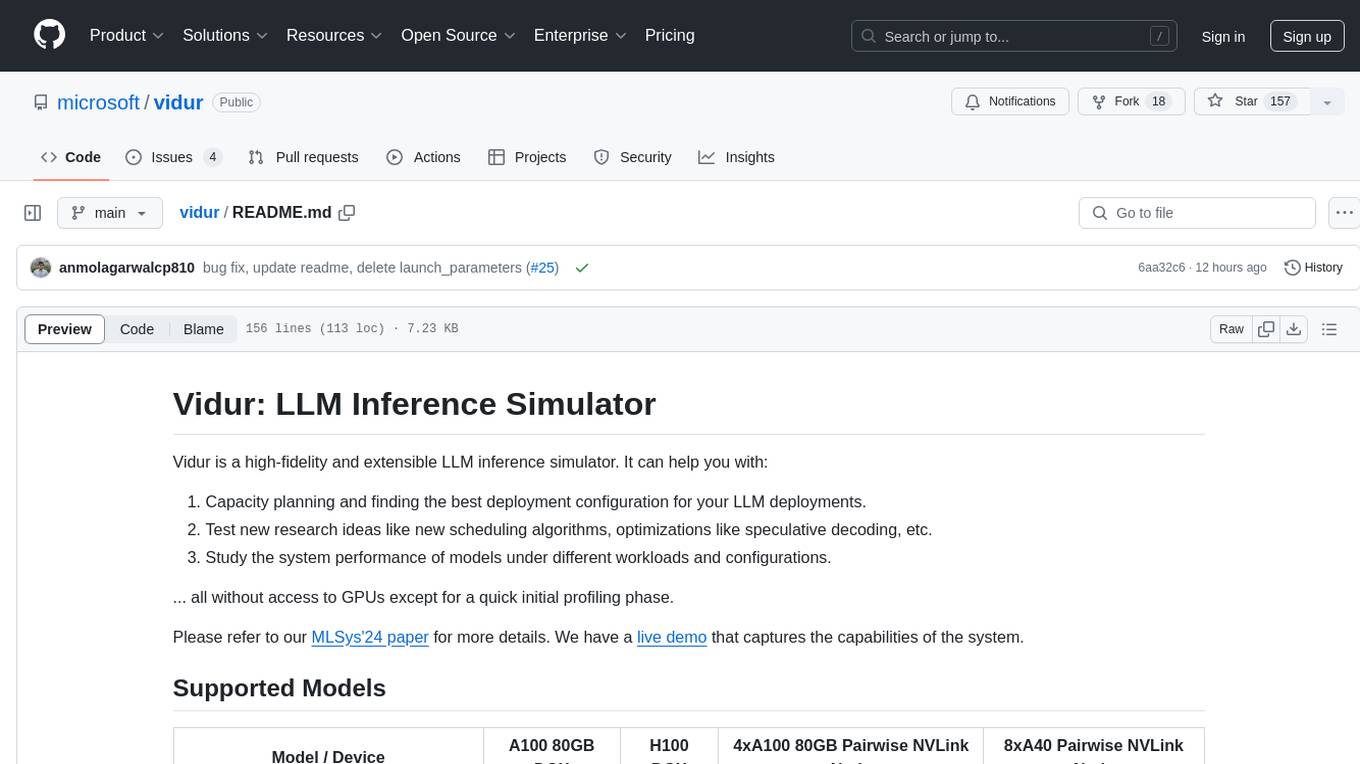
vidur
Vidur is a high-fidelity and extensible LLM inference simulator designed for capacity planning, deployment configuration optimization, testing new research ideas, and studying system performance of models under different workloads and configurations. It supports various models and devices, offers chrome trace exports, and can be set up using mamba, venv, or conda. Users can run the simulator with various parameters and monitor metrics using wandb. Contributions are welcome, subject to a Contributor License Agreement and adherence to the Microsoft Open Source Code of Conduct.
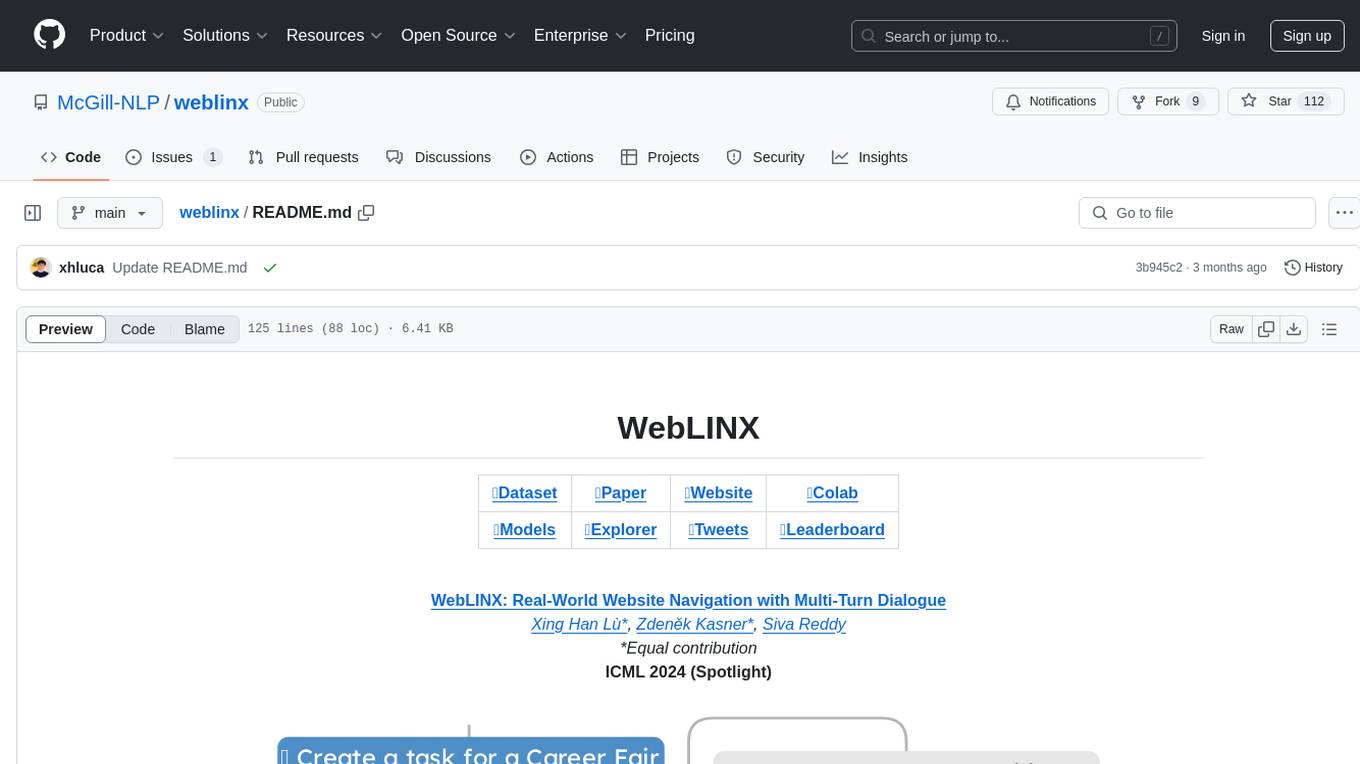
weblinx
WebLINX is a Python library and dataset for real-world website navigation with multi-turn dialogue. The repository provides code for training models reported in the WebLINX paper, along with a comprehensive API to work with the dataset. It includes modules for data processing, model evaluation, and utility functions. The modeling directory contains code for processing, training, and evaluating models such as DMR, LLaMA, MindAct, Pix2Act, and Flan-T5. Users can install specific dependencies for HTML processing, video processing, model evaluation, and library development. The evaluation module provides metrics and functions for evaluating models, with ongoing work to improve documentation and functionality.
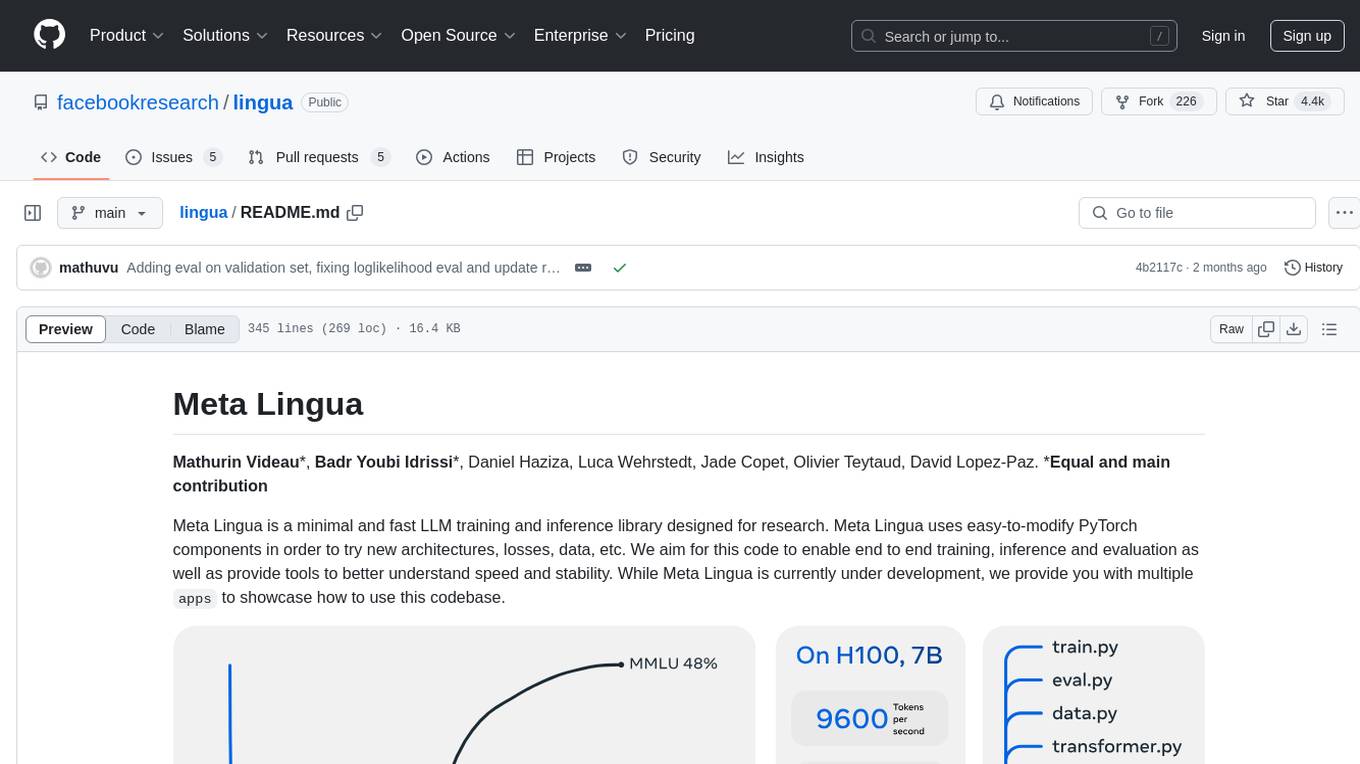
lingua
Meta Lingua is a minimal and fast LLM training and inference library designed for research. It uses easy-to-modify PyTorch components to experiment with new architectures, losses, and data. The codebase enables end-to-end training, inference, and evaluation, providing tools for speed and stability analysis. The repository contains essential components in the 'lingua' folder and scripts that combine these components in the 'apps' folder. Researchers can modify the provided templates to suit their experiments easily. Meta Lingua aims to lower the barrier to entry for LLM research by offering a lightweight and focused codebase.
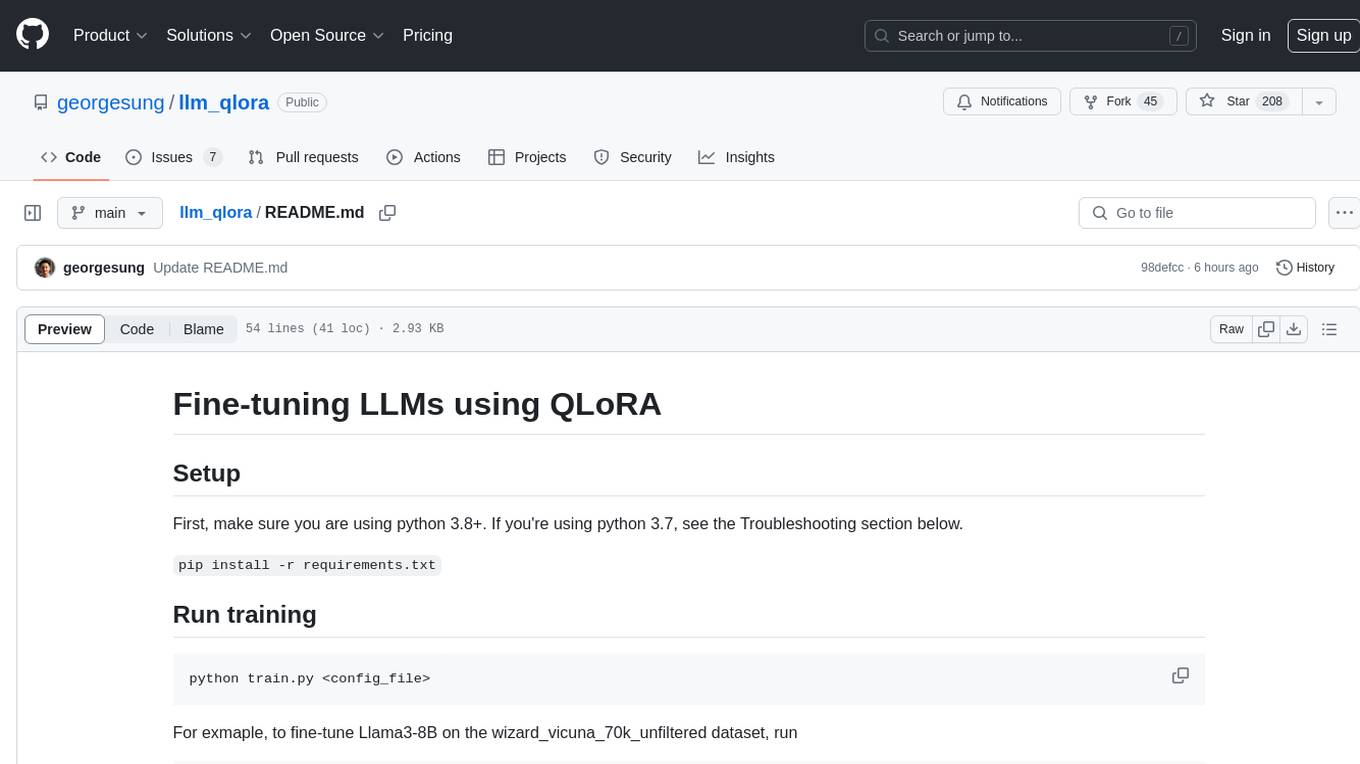
llm_qlora
LLM_QLoRA is a repository for fine-tuning Large Language Models (LLMs) using QLoRA methodology. It provides scripts for training LLMs on custom datasets, pushing models to HuggingFace Hub, and performing inference. Additionally, it includes models trained on HuggingFace Hub, a blog post detailing the QLoRA fine-tuning process, and instructions for converting and quantizing models. The repository also addresses troubleshooting issues related to Python versions and dependencies.
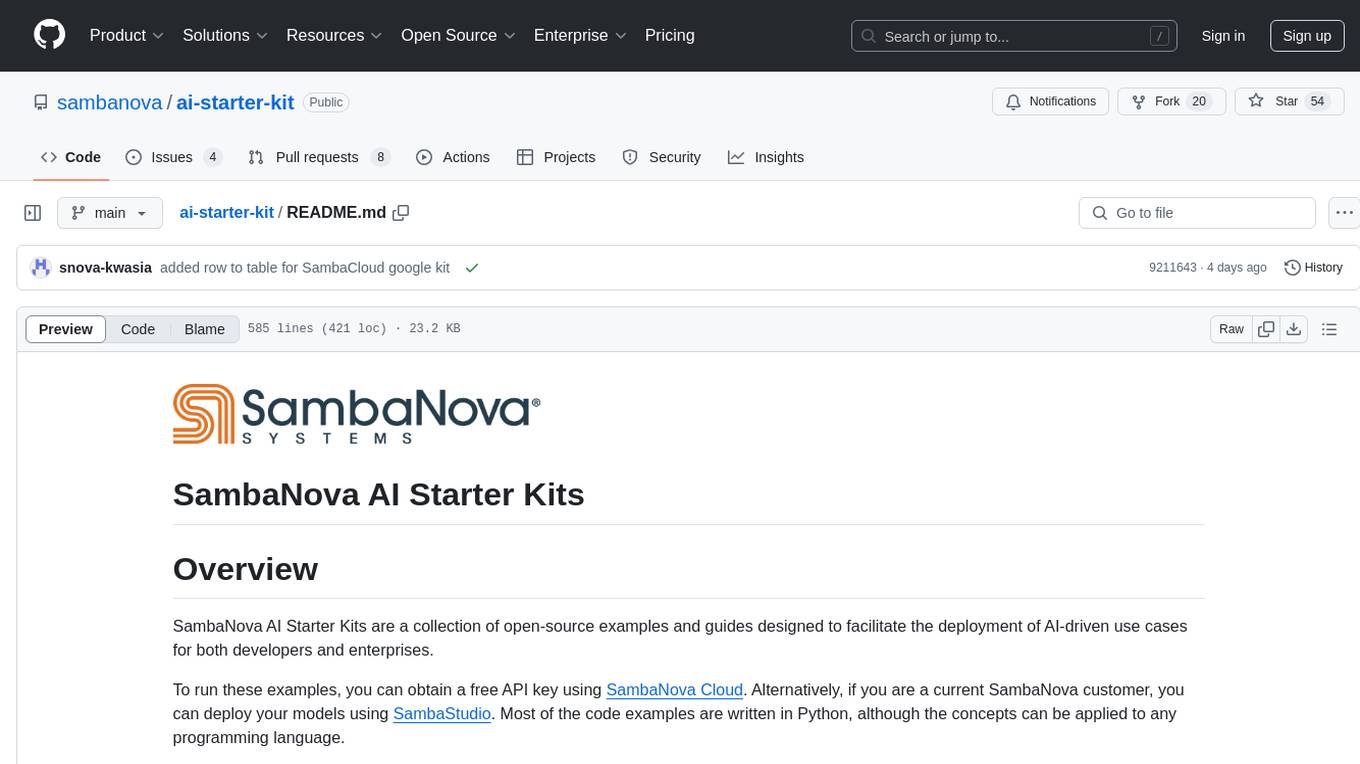
ai-starter-kit
SambaNova AI Starter Kits is a collection of open-source examples and guides designed to facilitate the deployment of AI-driven use cases for developers and enterprises. The kits cover various categories such as Data Ingestion & Preparation, Model Development & Optimization, Intelligent Information Retrieval, and Advanced AI Capabilities. Users can obtain a free API key using SambaNova Cloud or deploy models using SambaStudio. Most examples are written in Python but can be applied to any programming language. The kits provide resources for tasks like text extraction, fine-tuning embeddings, prompt engineering, question-answering, image search, post-call analysis, and more.
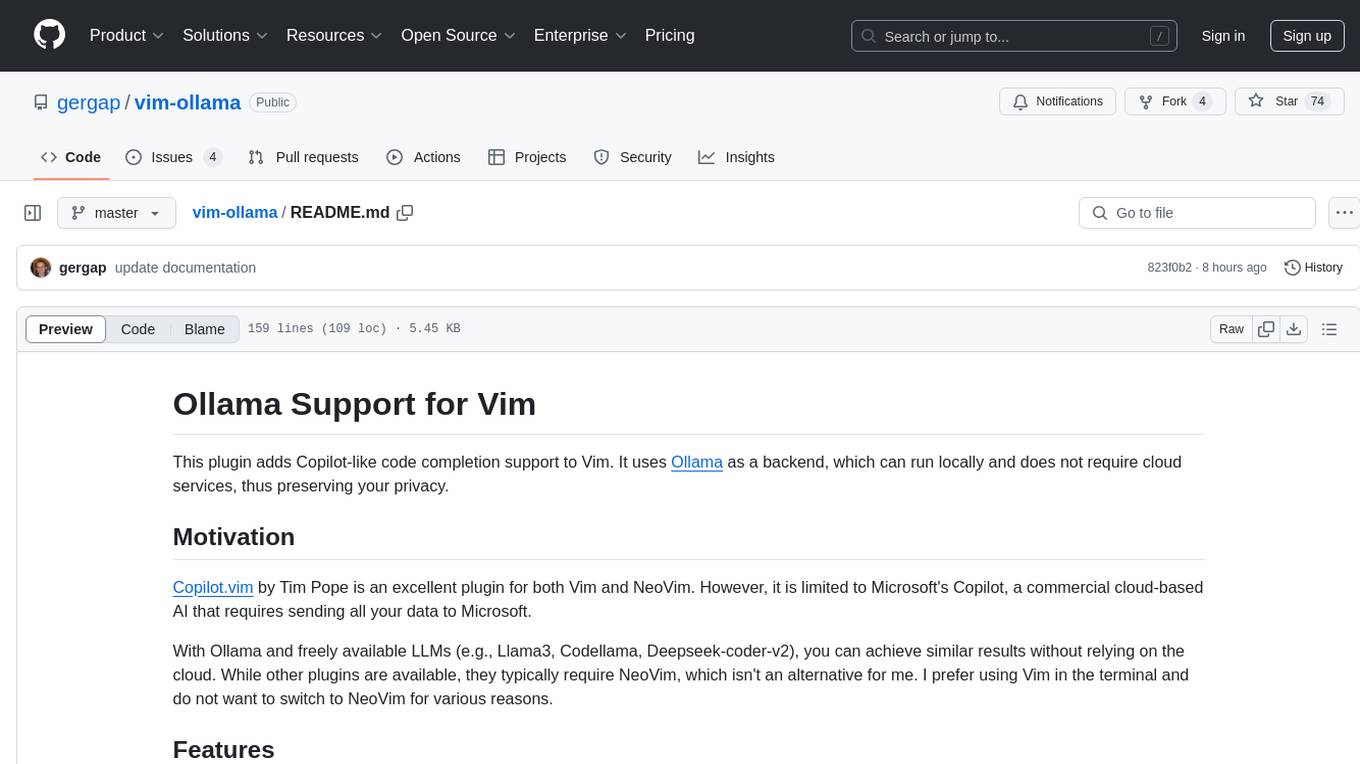
vim-ollama
The 'vim-ollama' plugin for Vim adds Copilot-like code completion support using Ollama as a backend, enabling intelligent AI-based code completion and integrated chat support for code reviews. It does not rely on cloud services, preserving user privacy. The plugin communicates with Ollama via Python scripts for code completion and interactive chat, supporting Vim only. Users can configure LLM models for code completion tasks and interactive conversations, with detailed installation and usage instructions provided in the README.
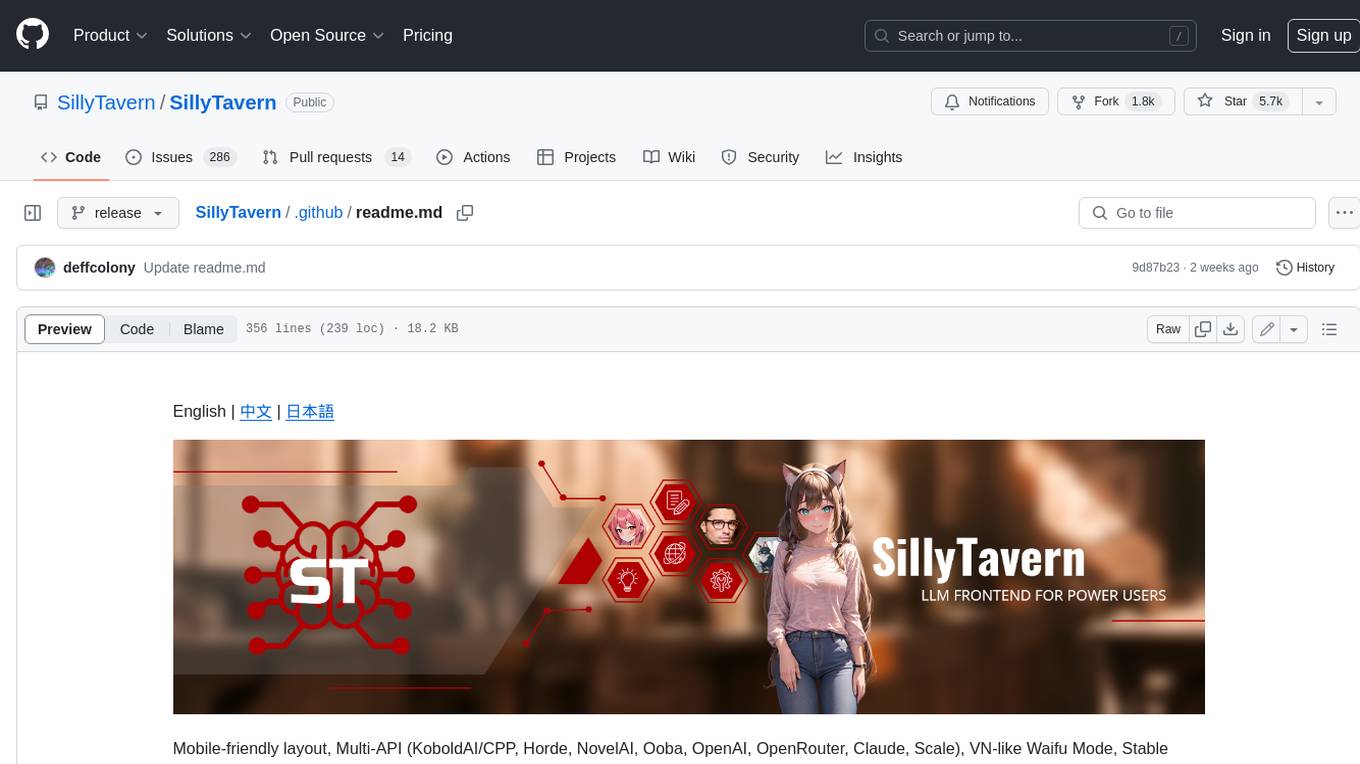
SillyTavern
SillyTavern is a user interface you can install on your computer (and Android phones) that allows you to interact with text generation AIs and chat/roleplay with characters you or the community create. SillyTavern is a fork of TavernAI 1.2.8 which is under more active development and has added many major features. At this point, they can be thought of as completely independent programs.
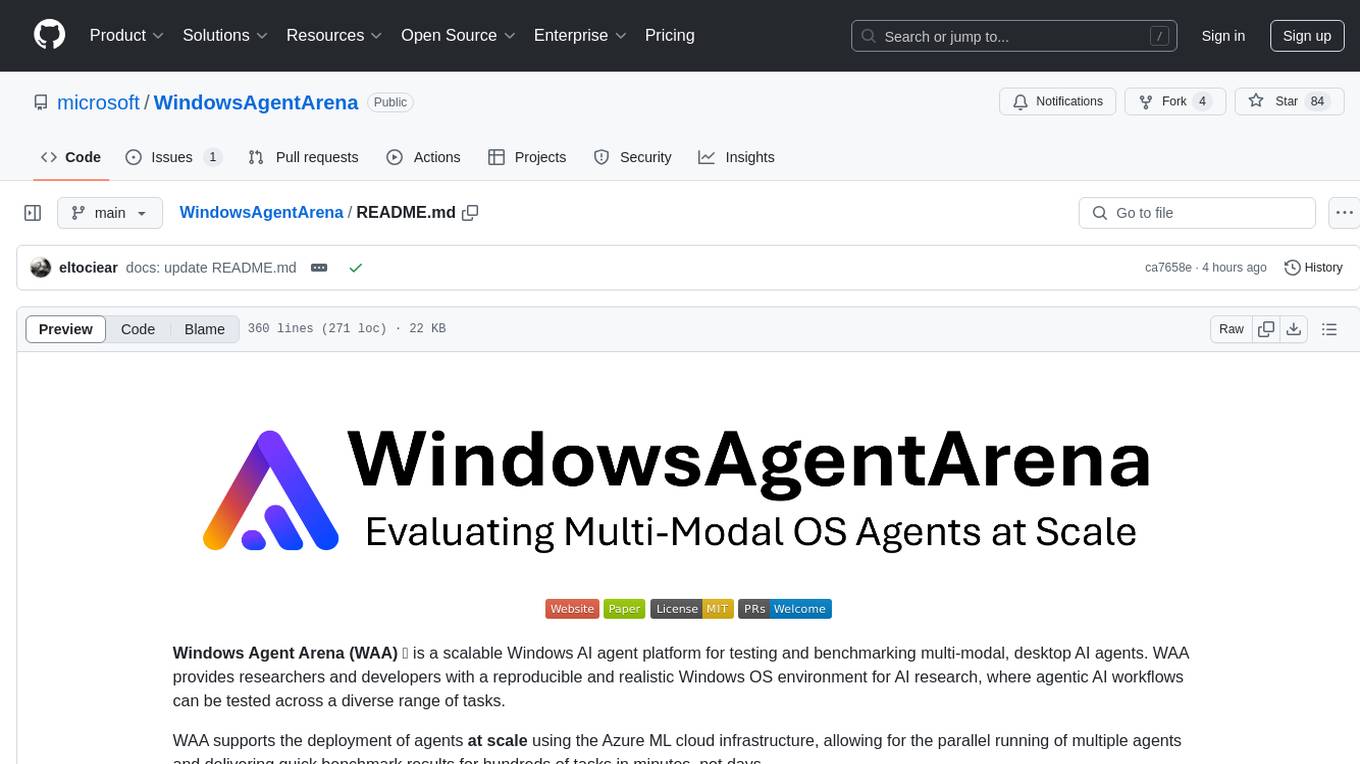
WindowsAgentArena
Windows Agent Arena (WAA) is a scalable Windows AI agent platform designed for testing and benchmarking multi-modal, desktop AI agents. It provides researchers and developers with a reproducible and realistic Windows OS environment for AI research, enabling testing of agentic AI workflows across various tasks. WAA supports deploying agents at scale using Azure ML cloud infrastructure, allowing parallel running of multiple agents and delivering quick benchmark results for hundreds of tasks in minutes.
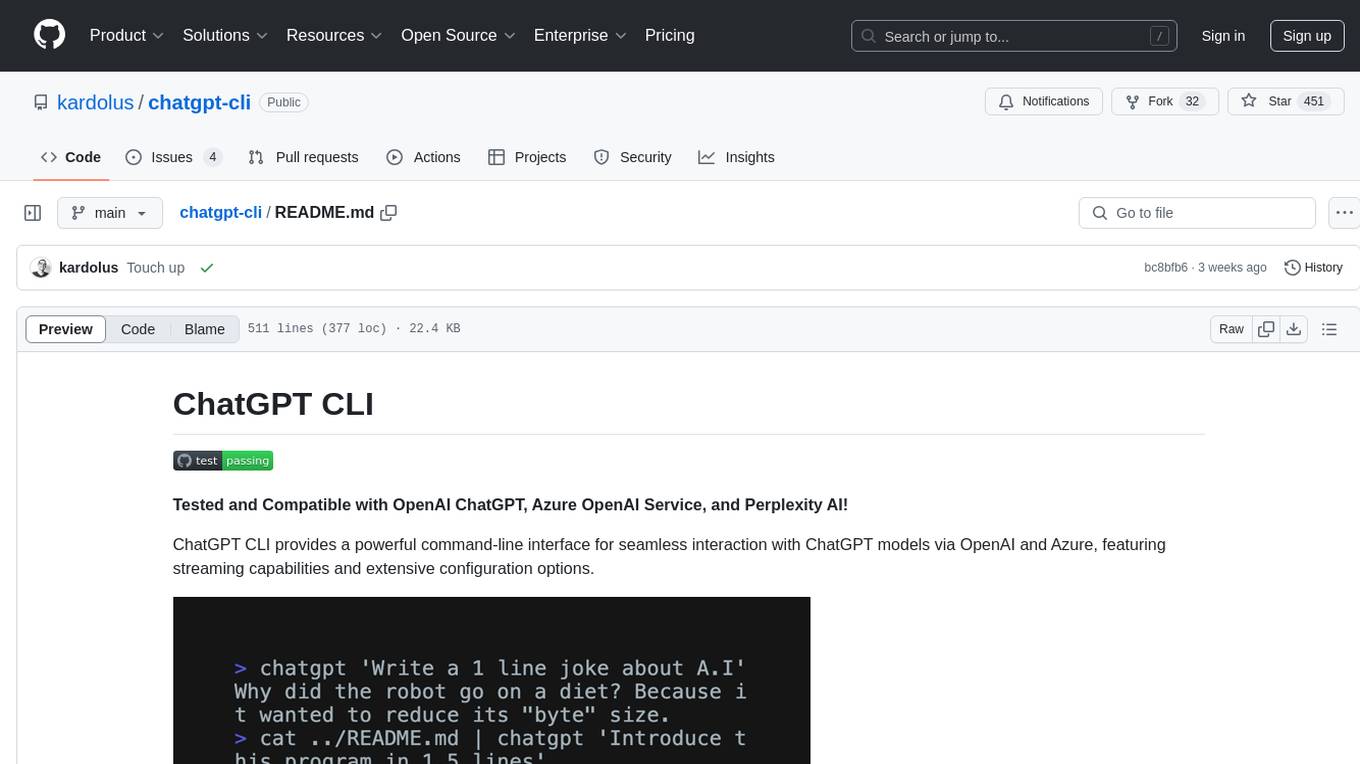
chatgpt-cli
ChatGPT CLI provides a powerful command-line interface for seamless interaction with ChatGPT models via OpenAI and Azure. It features streaming capabilities, extensive configuration options, and supports various modes like streaming, query, and interactive mode. Users can manage thread-based context, sliding window history, and provide custom context from any source. The CLI also offers model and thread listing, advanced configuration options, and supports GPT-4, GPT-3.5-turbo, and Perplexity's models. Installation is available via Homebrew or direct download, and users can configure settings through default values, a config.yaml file, or environment variables.
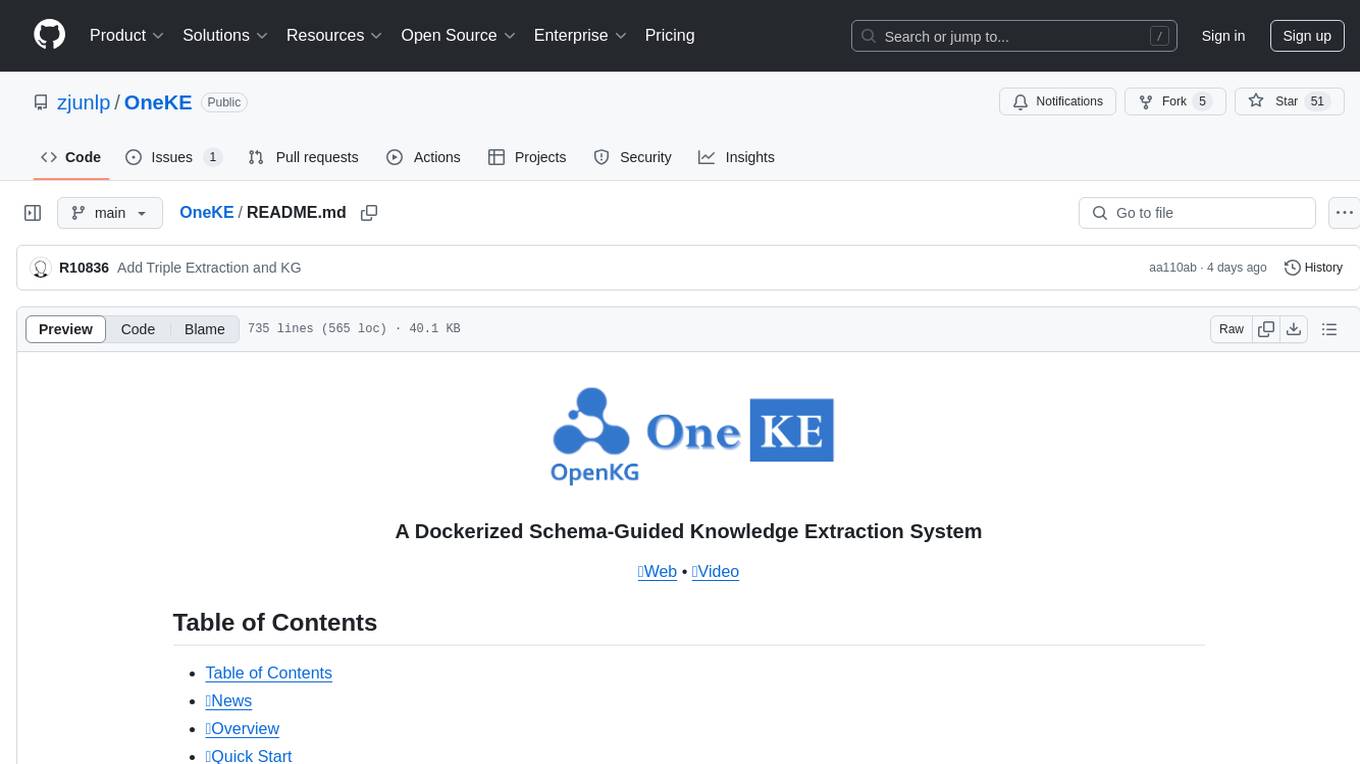
OneKE
OneKE is a flexible dockerized system for schema-guided knowledge extraction, capable of extracting information from the web and raw PDF books across multiple domains like science and news. It employs a collaborative multi-agent approach and includes a user-customizable knowledge base to enable tailored extraction. OneKE offers various IE tasks support, data sources support, LLMs support, extraction method support, and knowledge base configuration. Users can start with examples using YAML, Python, or Web UI, and perform tasks like Named Entity Recognition, Relation Extraction, Event Extraction, Triple Extraction, and Open Domain IE. The tool supports different source formats like Plain Text, HTML, PDF, Word, TXT, and JSON files. Users can choose from various extraction models like OpenAI, DeepSeek, LLaMA, Qwen, ChatGLM, MiniCPM, and OneKE for information extraction tasks. Extraction methods include Schema Agent, Extraction Agent, and Reflection Agent. The tool also provides support for schema repository and case repository management, along with solutions for network issues. Contributors to the project include Ningyu Zhang, Haofen Wang, Yujie Luo, Xiangyuan Ru, Kangwei Liu, Lin Yuan, Mengshu Sun, Lei Liang, Zhiqiang Zhang, Jun Zhou, Lanning Wei, Da Zheng, and Huajun Chen.
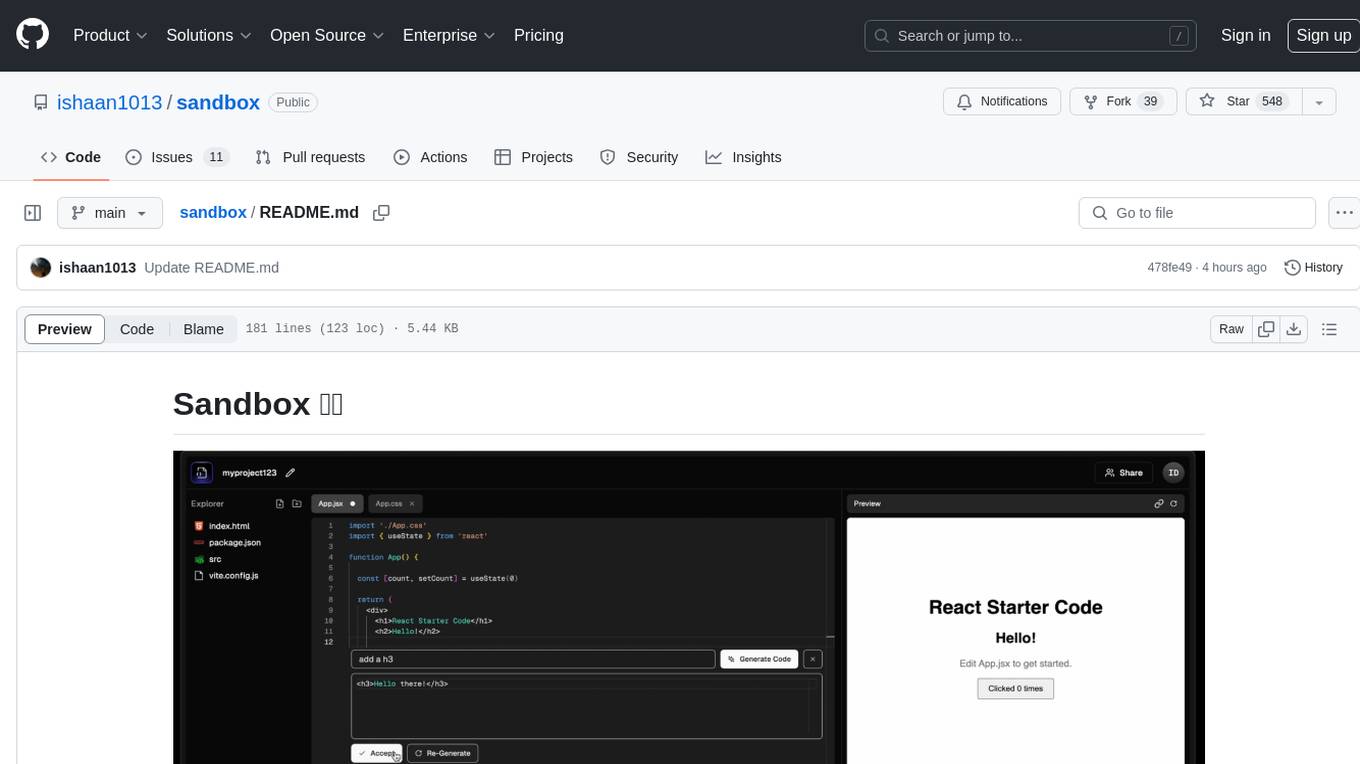
sandbox
Sandbox is an open-source cloud-based code editing environment with custom AI code autocompletion and real-time collaboration. It consists of a frontend built with Next.js, TailwindCSS, Shadcn UI, Clerk, Monaco, and Liveblocks, and a backend with Express, Socket.io, Cloudflare Workers, D1 database, R2 storage, Workers AI, and Drizzle ORM. The backend includes microservices for database, storage, and AI functionalities. Users can run the project locally by setting up environment variables and deploying the containers. Contributions are welcome following the commit convention and structure provided in the repository.
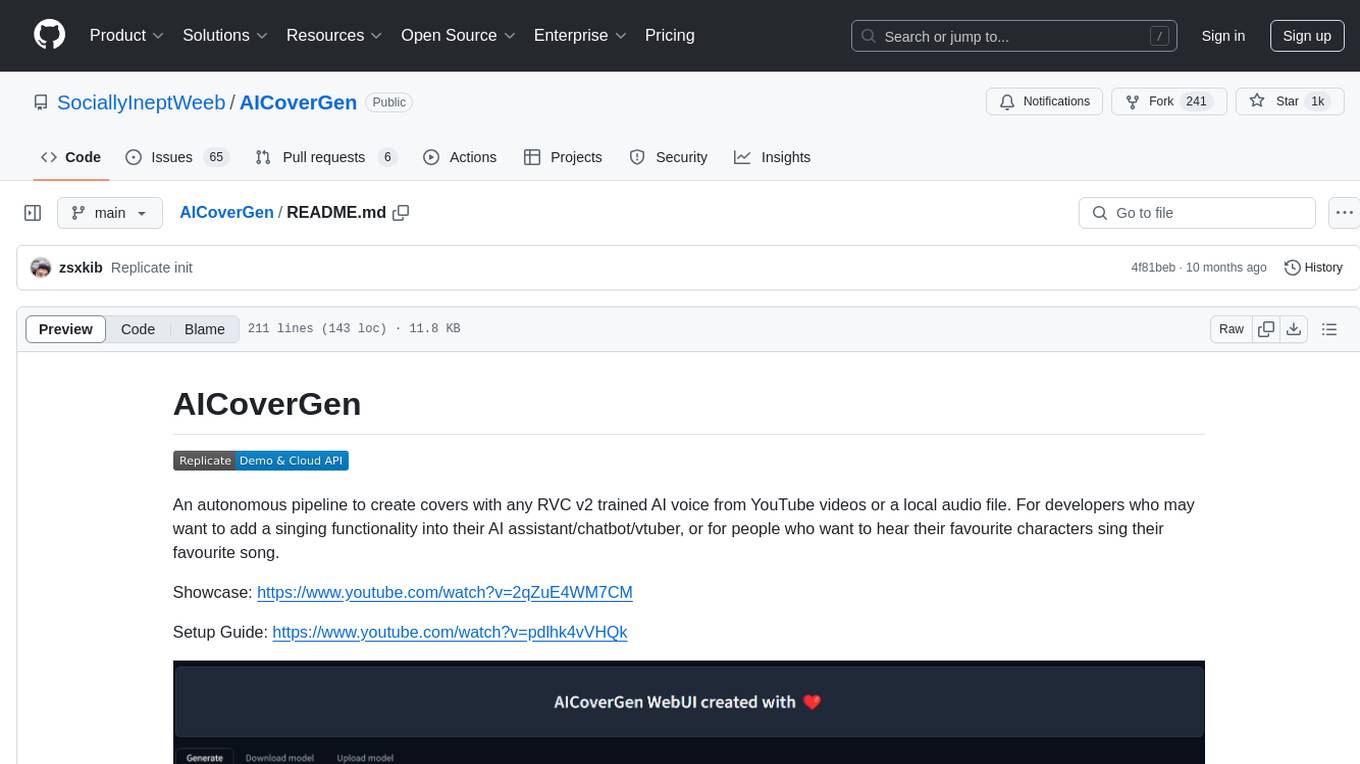
AICoverGen
AICoverGen is an autonomous pipeline designed to create covers using any RVC v2 trained AI voice from YouTube videos or local audio files. It caters to developers looking to incorporate singing functionality into AI assistants/chatbots/vtubers, as well as individuals interested in hearing their favorite characters sing. The tool offers a WebUI for easy conversions, cover generation from local audio files, volume control for vocals and instrumentals, pitch detection method control, pitch change for vocals and instrumentals, and audio output format options. Users can also download and upload RVC models via the WebUI, run the pipeline using CLI, and access various advanced options for voice conversion and audio mixing.
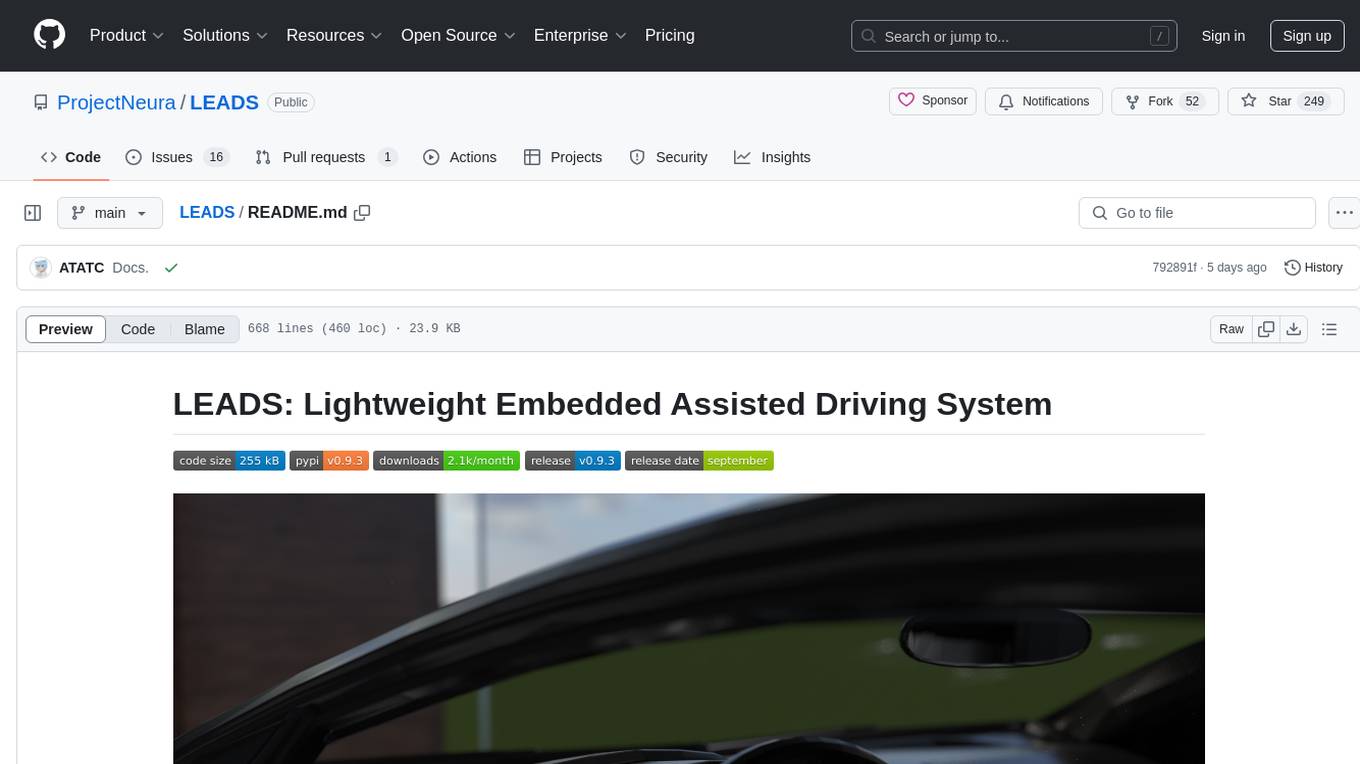
LEADS
LEADS is a lightweight embedded assisted driving system designed to simplify the development of instrumentation, control, and analysis systems for racing cars. It is written in Python and C/C++ with impressive performance. The system is customizable and provides abstract layers for component rearrangement. It supports hardware components like Raspberry Pi and Arduino, and can adapt to various hardware types. LEADS offers a modular structure with a focus on flexibility and lightweight design. It includes robust safety features, modern GUI design with dark mode support, high performance on different platforms, and powerful ESC systems for traction control and braking. The system also supports real-time data sharing, live video streaming, and AI-enhanced data analysis for driver training. LEADS VeC Remote Analyst enables transparency between the driver and pit crew, allowing real-time data sharing and analysis. The system is designed to be user-friendly, adaptable, and efficient for racing car development.
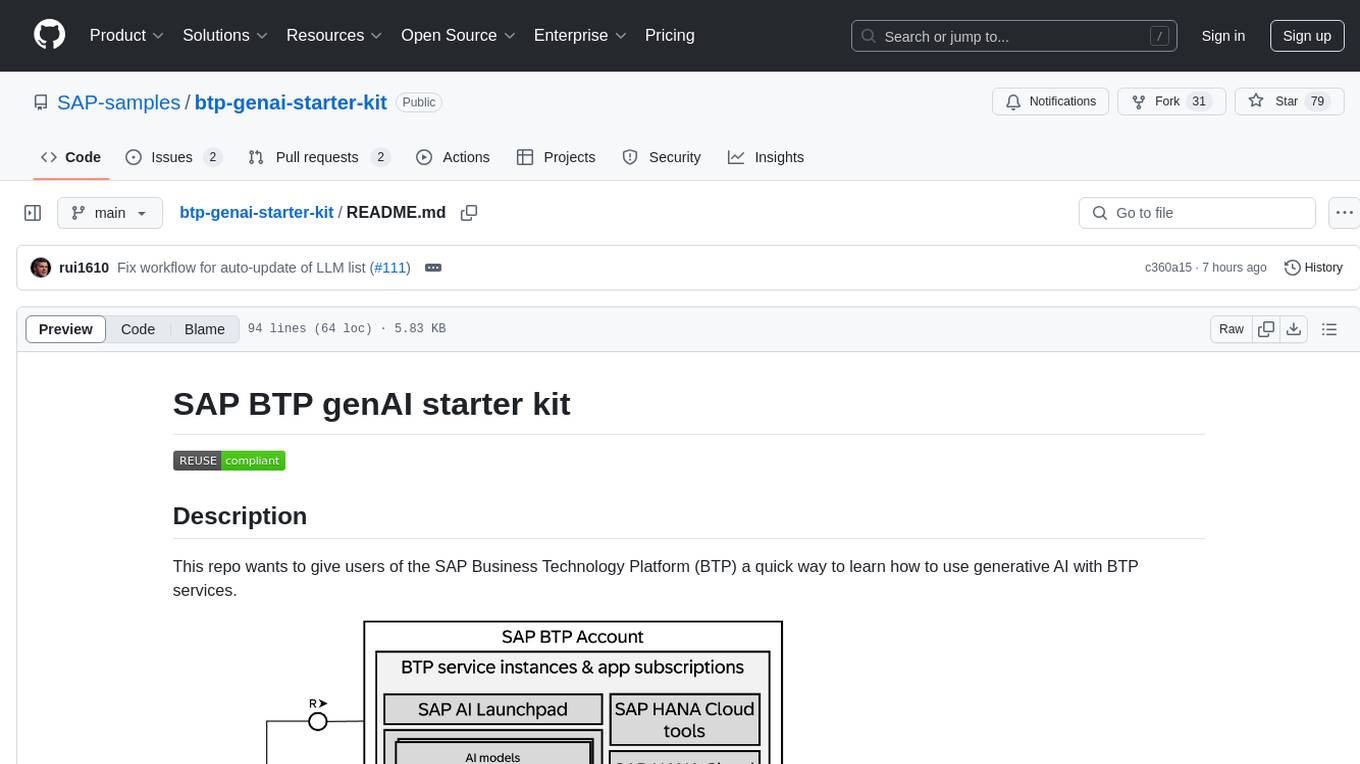
btp-genai-starter-kit
This repository provides a quick way for users of the SAP Business Technology Platform (BTP) to learn how to use generative AI with BTP services. It guides users through setting up the necessary infrastructure, deploying AI models, and running genAI experiments on SAP BTP. The repository includes scripts, examples, and instructions to help users get started with generative AI on the SAP BTP platform.
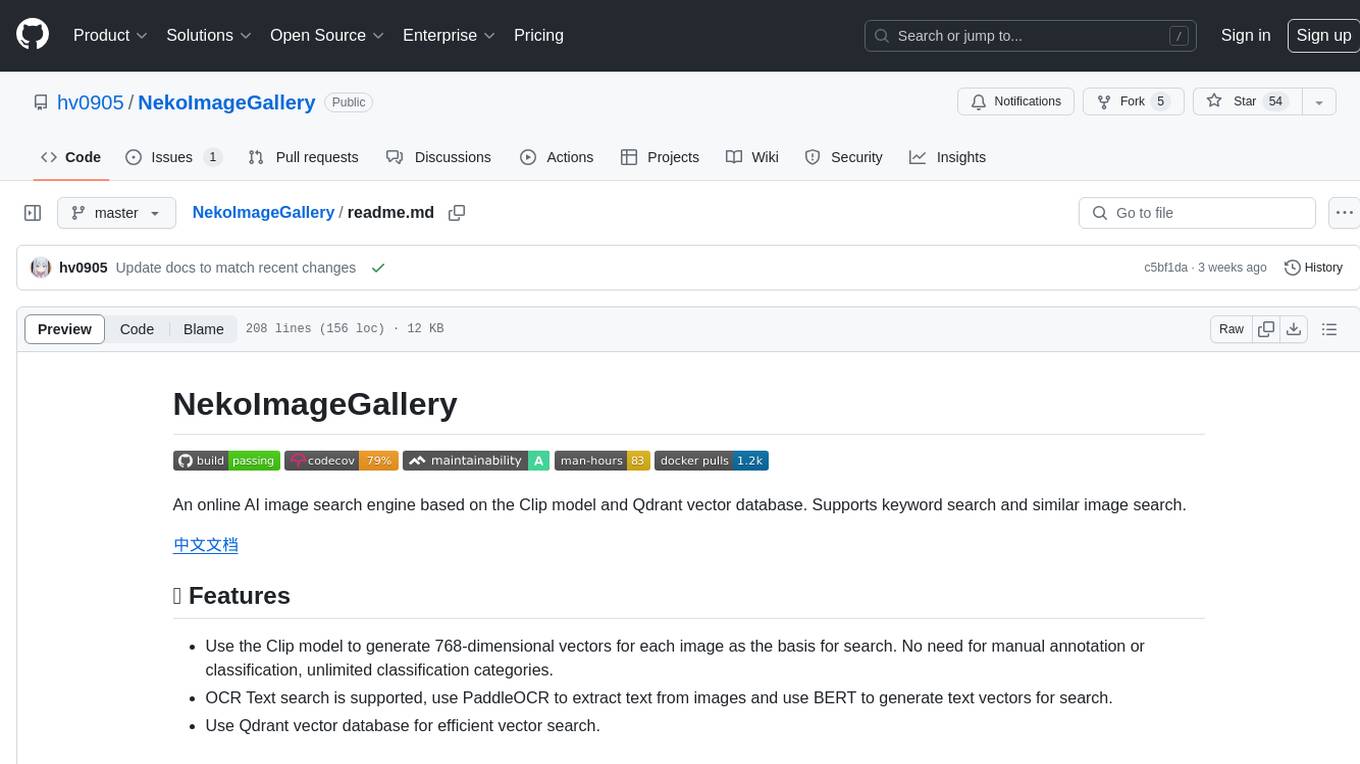
NekoImageGallery
NekoImageGallery is an online AI image search engine that utilizes the Clip model and Qdrant vector database. It supports keyword search and similar image search. The tool generates 768-dimensional vectors for each image using the Clip model, supports OCR text search using PaddleOCR, and efficiently searches vectors using the Qdrant vector database. Users can deploy the tool locally or via Docker, with options for metadata storage using Qdrant database or local file storage. The tool provides API documentation through FastAPI's built-in Swagger UI and can be used for tasks like image search, text extraction, and vector search.
For similar tasks
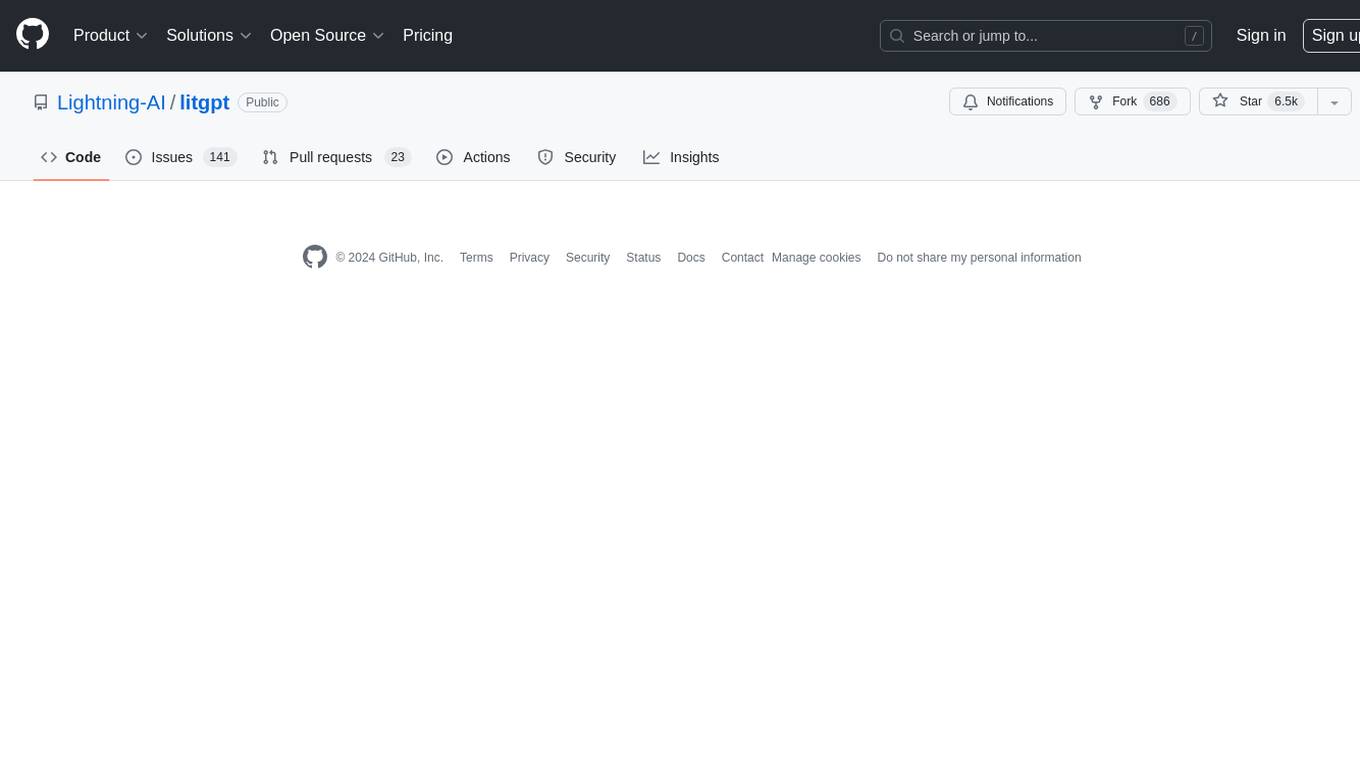
litgpt
LitGPT is a command-line tool designed to easily finetune, pretrain, evaluate, and deploy 20+ LLMs **on your own data**. It features highly-optimized training recipes for the world's most powerful open-source large-language-models (LLMs).
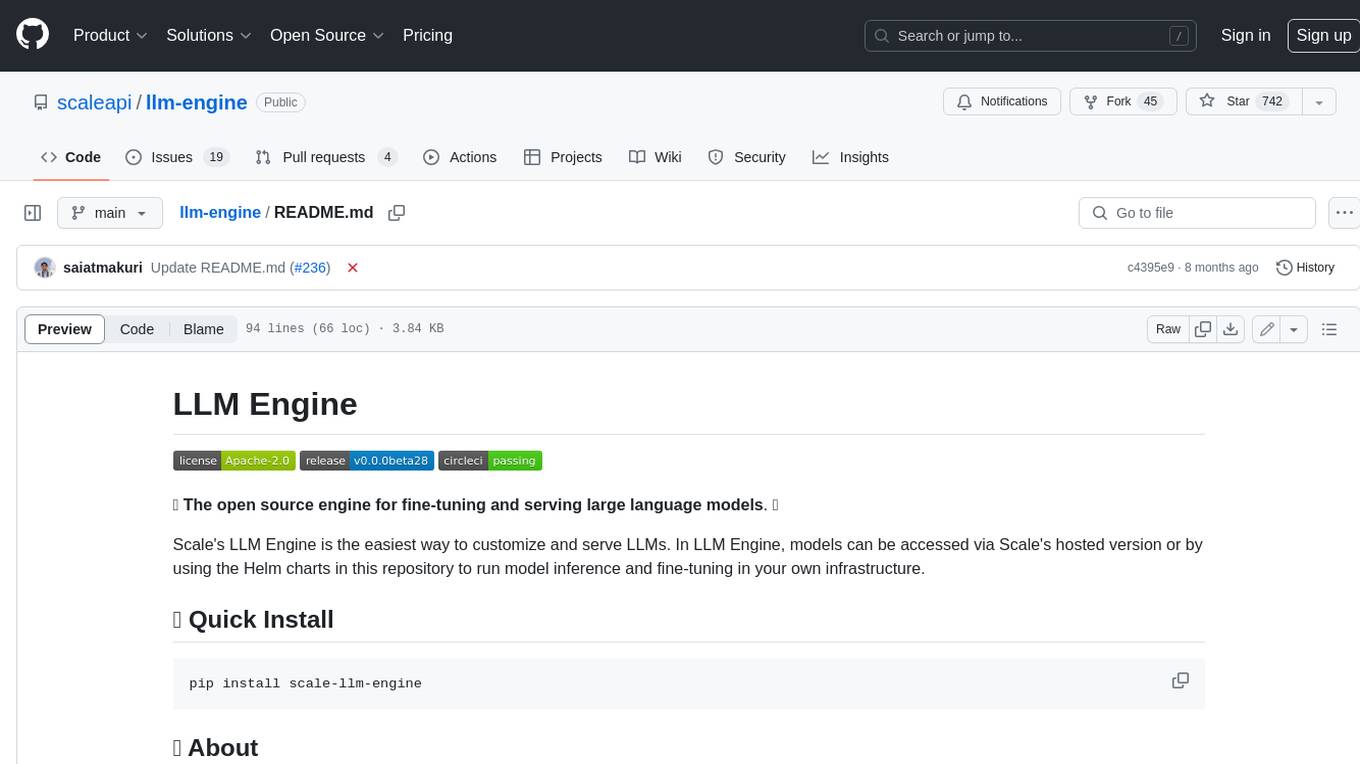
llm-engine
Scale's LLM Engine is an open-source Python library, CLI, and Helm chart that provides everything you need to serve and fine-tune foundation models, whether you use Scale's hosted infrastructure or do it in your own cloud infrastructure using Kubernetes.
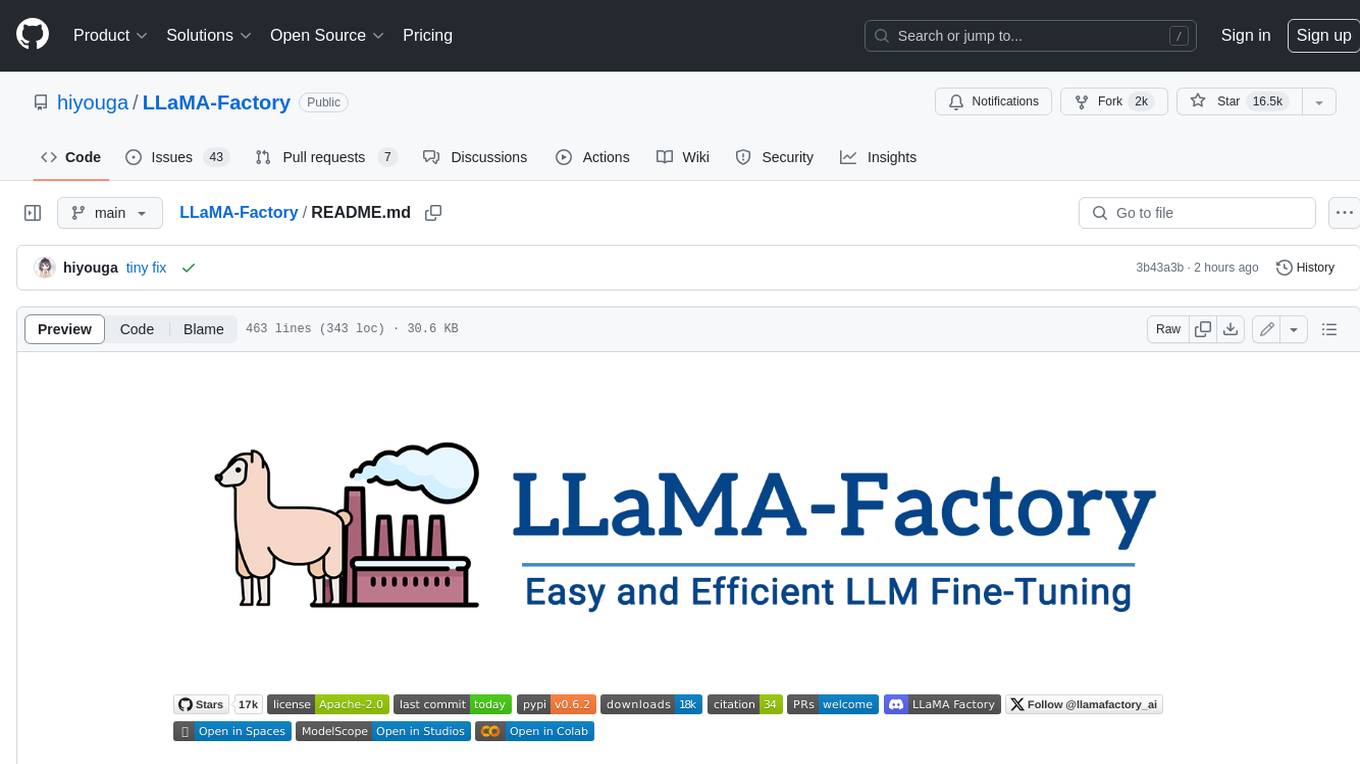
LLaMA-Factory
LLaMA Factory is a unified framework for fine-tuning 100+ large language models (LLMs) with various methods, including pre-training, supervised fine-tuning, reward modeling, PPO, DPO and ORPO. It features integrated algorithms like GaLore, BAdam, DoRA, LongLoRA, LLaMA Pro, LoRA+, LoftQ and Agent tuning, as well as practical tricks like FlashAttention-2, Unsloth, RoPE scaling, NEFTune and rsLoRA. LLaMA Factory provides experiment monitors like LlamaBoard, TensorBoard, Wandb, MLflow, etc., and supports faster inference with OpenAI-style API, Gradio UI and CLI with vLLM worker. Compared to ChatGLM's P-Tuning, LLaMA Factory's LoRA tuning offers up to 3.7 times faster training speed with a better Rouge score on the advertising text generation task. By leveraging 4-bit quantization technique, LLaMA Factory's QLoRA further improves the efficiency regarding the GPU memory.
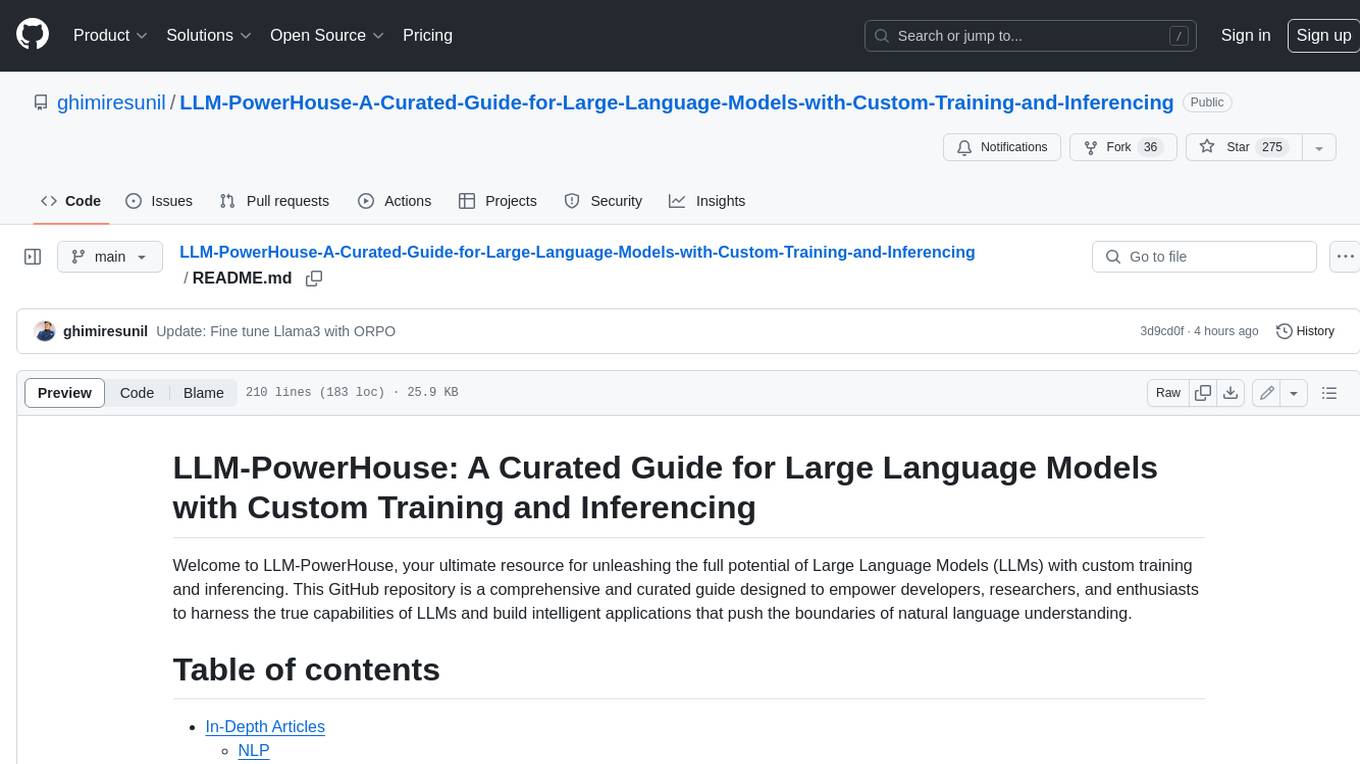
LLM-PowerHouse-A-Curated-Guide-for-Large-Language-Models-with-Custom-Training-and-Inferencing
LLM-PowerHouse is a comprehensive and curated guide designed to empower developers, researchers, and enthusiasts to harness the true capabilities of Large Language Models (LLMs) and build intelligent applications that push the boundaries of natural language understanding. This GitHub repository provides in-depth articles, codebase mastery, LLM PlayLab, and resources for cost analysis and network visualization. It covers various aspects of LLMs, including NLP, models, training, evaluation metrics, open LLMs, and more. The repository also includes a collection of code examples and tutorials to help users build and deploy LLM-based applications.
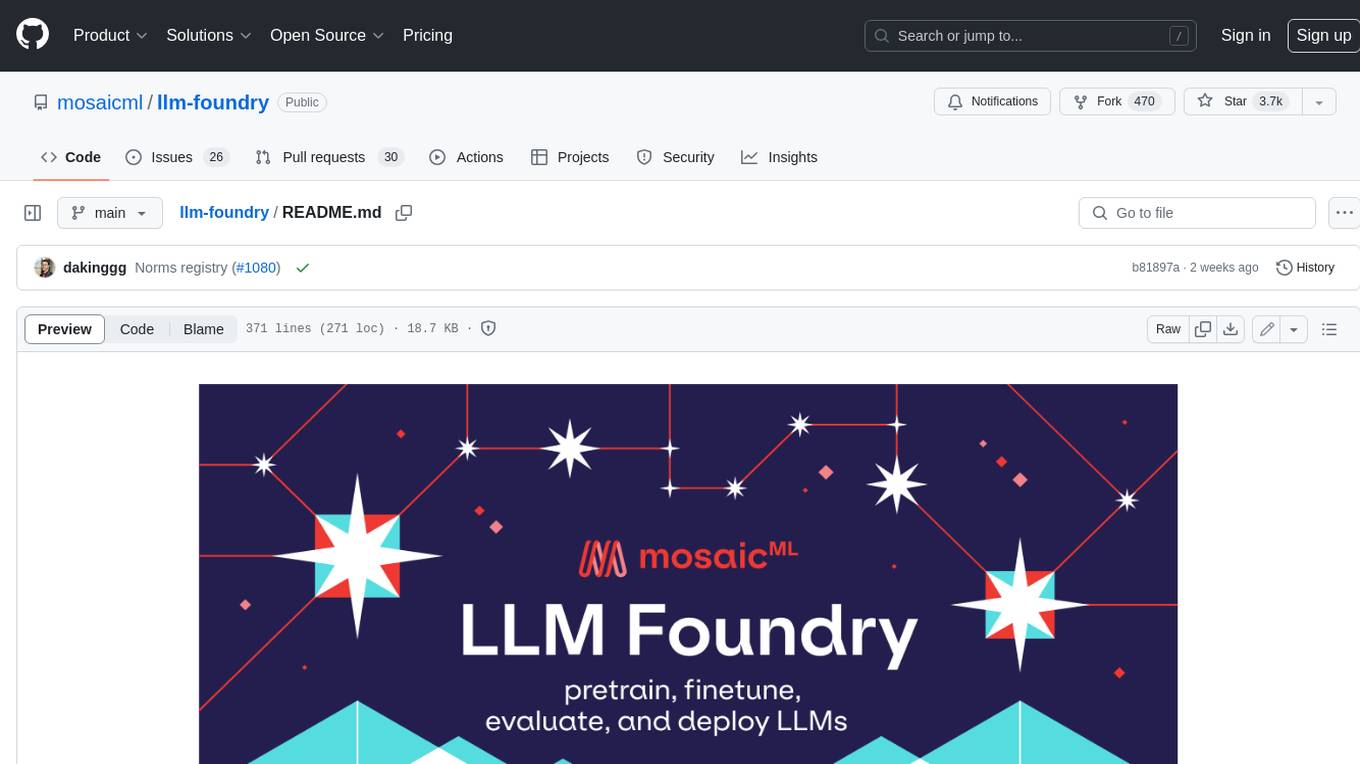
llm-foundry
LLM Foundry is a codebase for training, finetuning, evaluating, and deploying LLMs for inference with Composer and the MosaicML platform. It is designed to be easy-to-use, efficient _and_ flexible, enabling rapid experimentation with the latest techniques. You'll find in this repo: * `llmfoundry/` - source code for models, datasets, callbacks, utilities, etc. * `scripts/` - scripts to run LLM workloads * `data_prep/` - convert text data from original sources to StreamingDataset format * `train/` - train or finetune HuggingFace and MPT models from 125M - 70B parameters * `train/benchmarking` - profile training throughput and MFU * `inference/` - convert models to HuggingFace or ONNX format, and generate responses * `inference/benchmarking` - profile inference latency and throughput * `eval/` - evaluate LLMs on academic (or custom) in-context-learning tasks * `mcli/` - launch any of these workloads using MCLI and the MosaicML platform * `TUTORIAL.md` - a deeper dive into the repo, example workflows, and FAQs
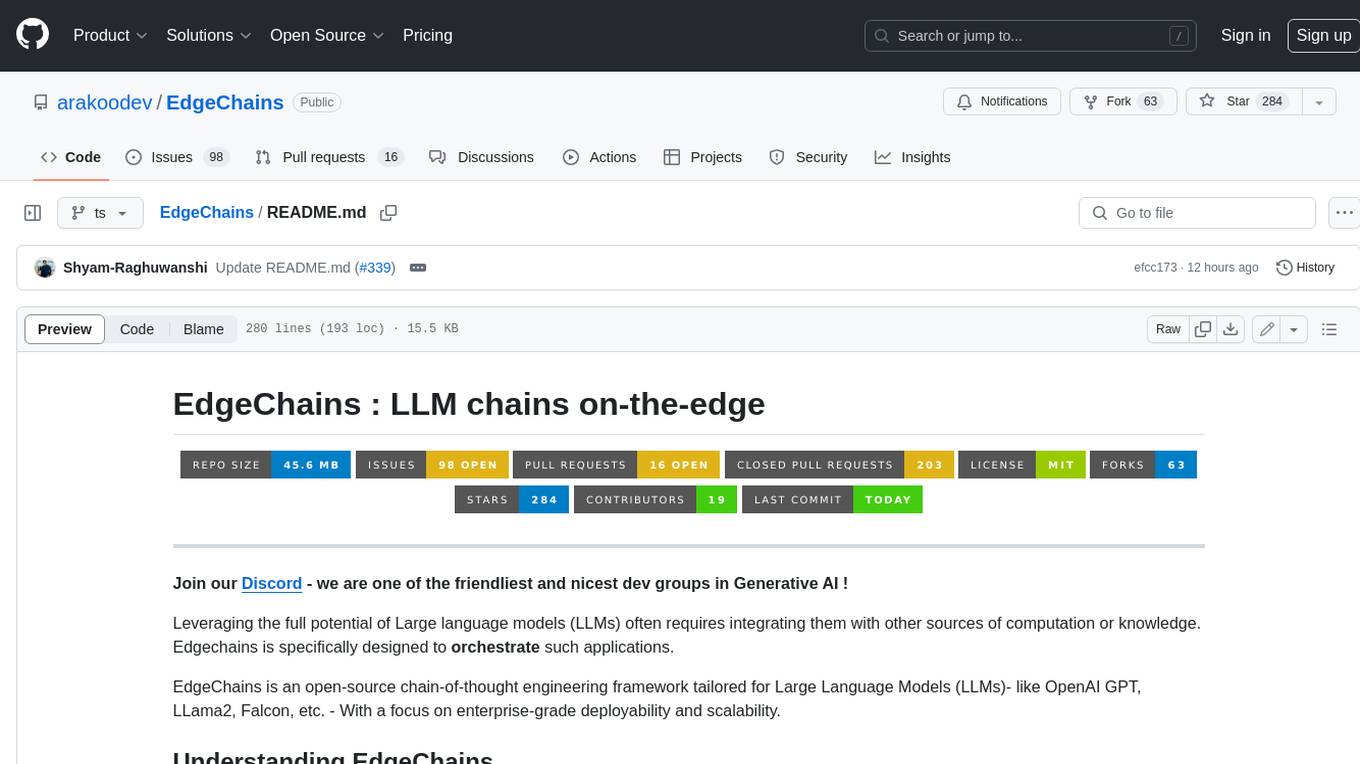
EdgeChains
EdgeChains is an open-source chain-of-thought engineering framework tailored for Large Language Models (LLMs)- like OpenAI GPT, LLama2, Falcon, etc. - With a focus on enterprise-grade deployability and scalability. EdgeChains is specifically designed to **orchestrate** such applications. At EdgeChains, we take a unique approach to Generative AI - we think Generative AI is a deployment and configuration management challenge rather than a UI and library design pattern challenge. We build on top of a tech that has solved this problem in a different domain - Kubernetes Config Management - and bring that to Generative AI. Edgechains is built on top of jsonnet, originally built by Google based on their experience managing a vast amount of configuration code in the Borg infrastructure.
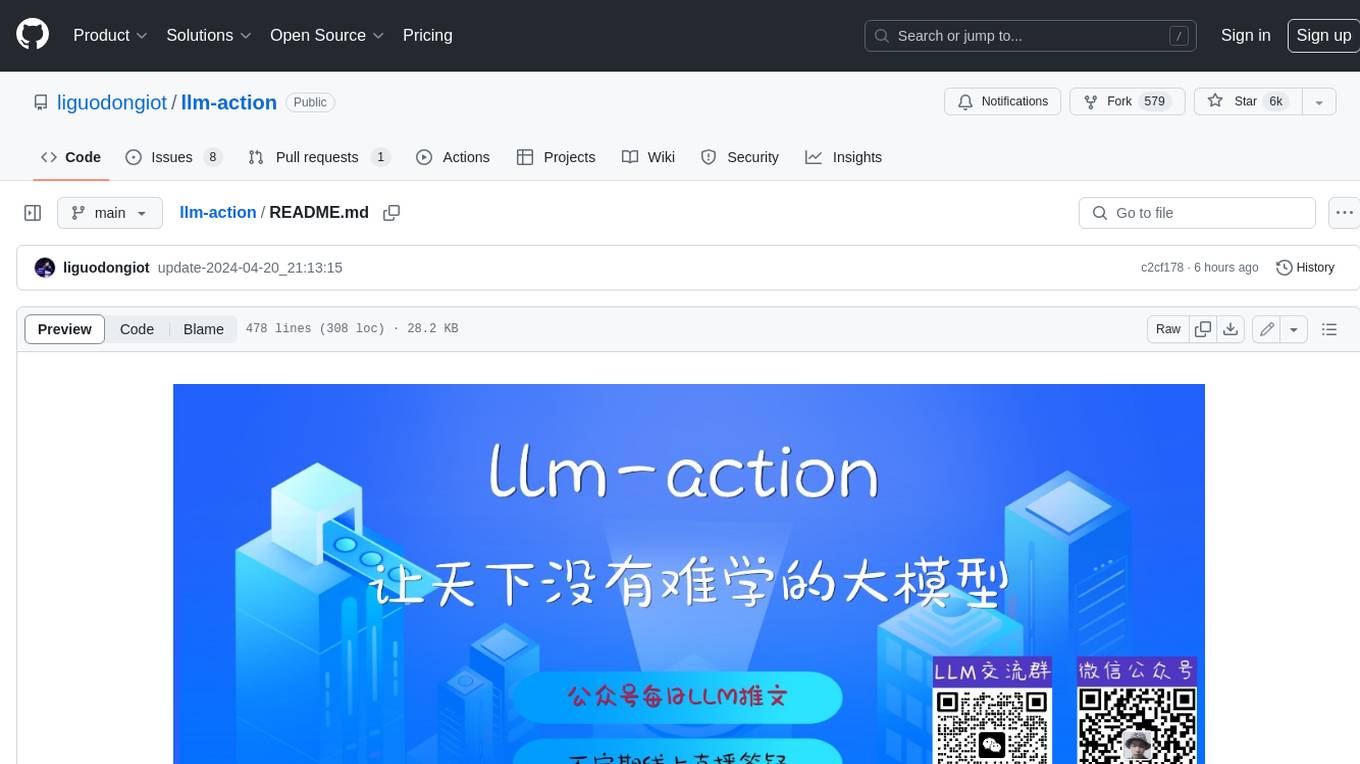
llm-action
This repository provides a comprehensive guide to large language models (LLMs), covering various aspects such as training, fine-tuning, compression, and applications. It includes detailed tutorials, code examples, and explanations of key concepts and techniques. The repository is maintained by Liguo Dong, an AI researcher and engineer with expertise in LLM research and development.
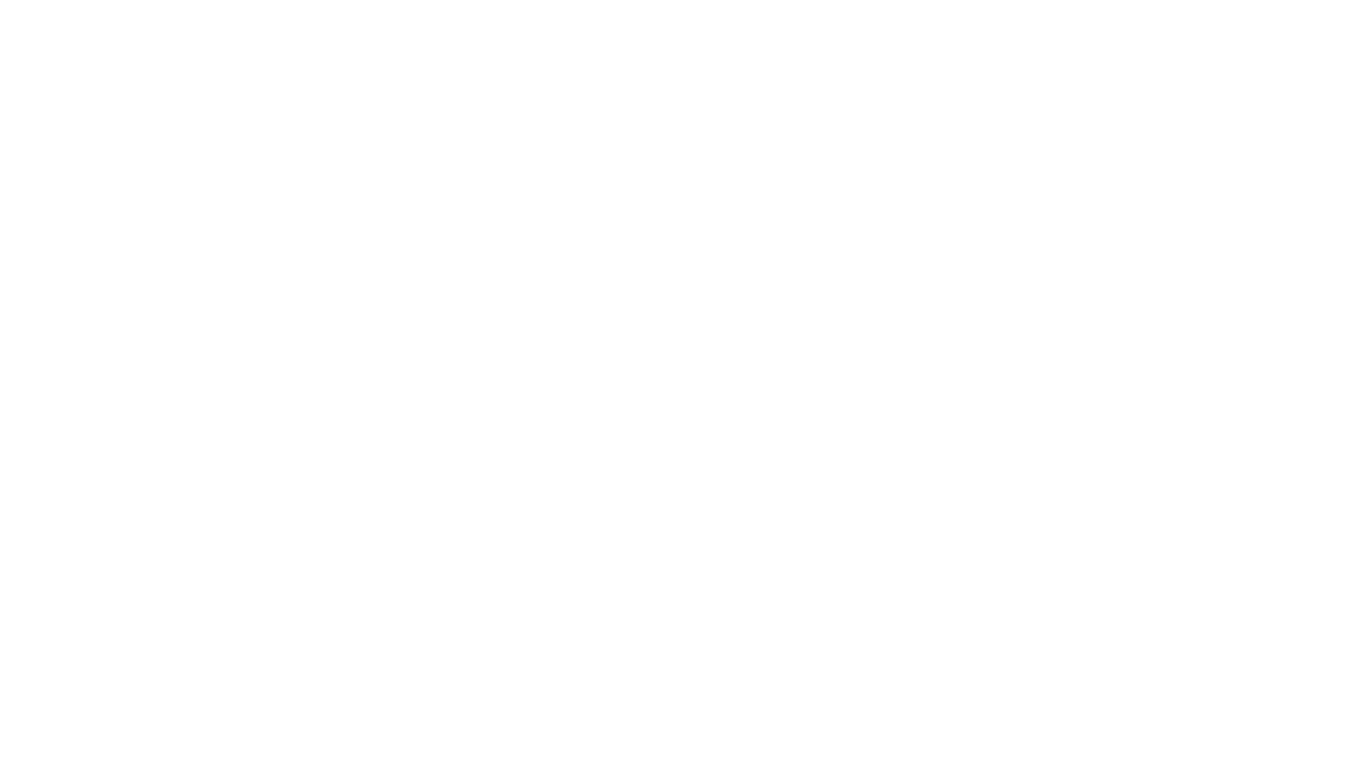
llm-on-openshift
This repository provides resources, demos, and recipes for working with Large Language Models (LLMs) on OpenShift using OpenShift AI or Open Data Hub. It includes instructions for deploying inference servers for LLMs, such as vLLM, Hugging Face TGI, Caikit-TGIS-Serving, and Ollama. Additionally, it offers guidance on deploying serving runtimes, such as vLLM Serving Runtime and Hugging Face Text Generation Inference, in the Single-Model Serving stack of Open Data Hub or OpenShift AI. The repository also covers vector databases that can be used as a Vector Store for Retrieval Augmented Generation (RAG) applications, including Milvus, PostgreSQL+pgvector, and Redis. Furthermore, it provides examples of inference and application usage, such as Caikit, Langchain, Langflow, and UI examples.
For similar jobs
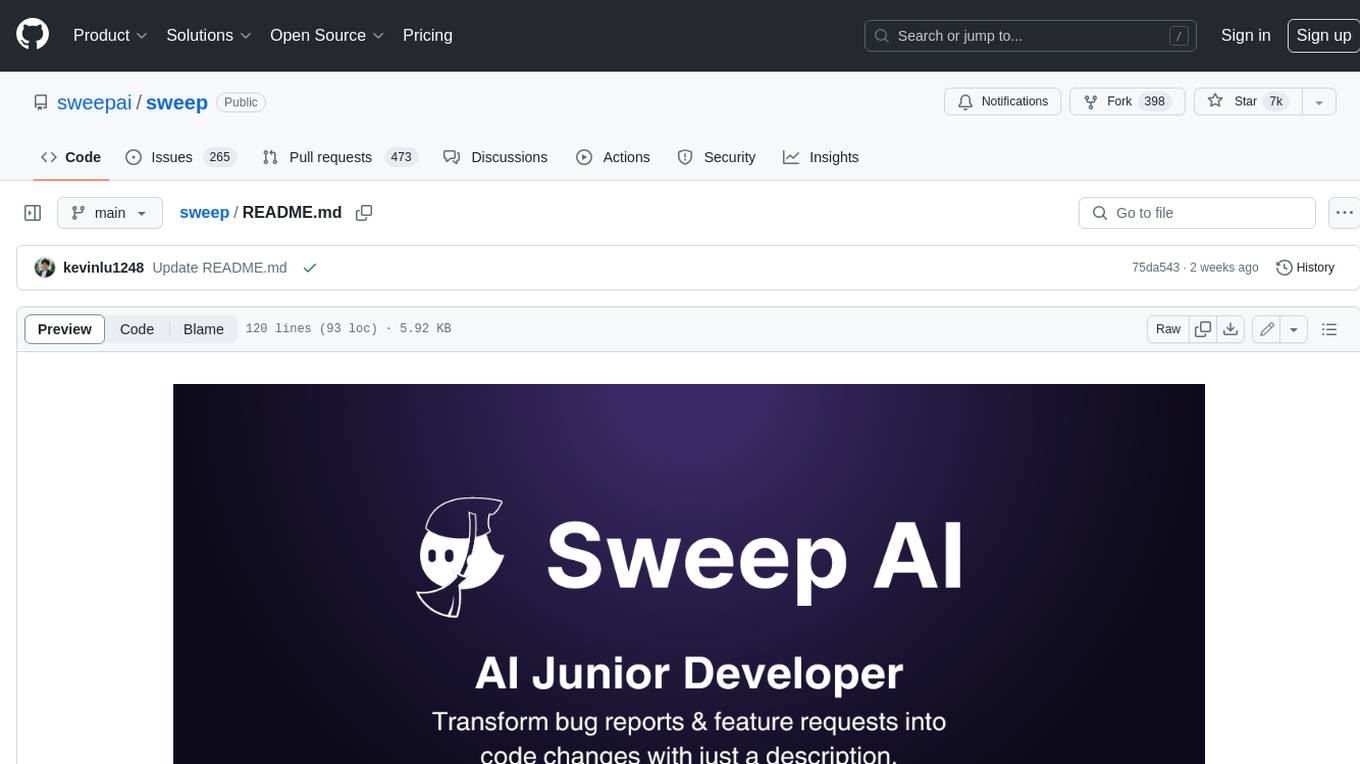
sweep
Sweep is an AI junior developer that turns bugs and feature requests into code changes. It automatically handles developer experience improvements like adding type hints and improving test coverage.
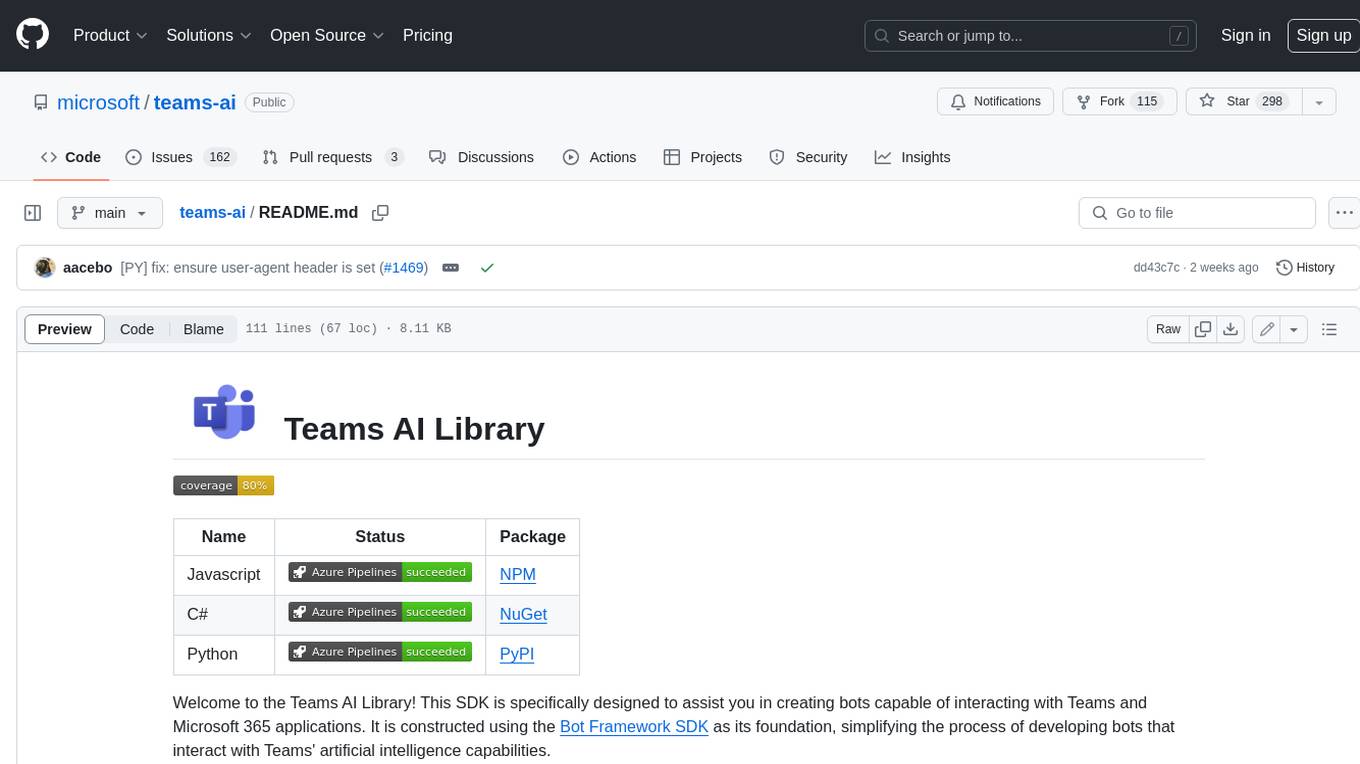
teams-ai
The Teams AI Library is a software development kit (SDK) that helps developers create bots that can interact with Teams and Microsoft 365 applications. It is built on top of the Bot Framework SDK and simplifies the process of developing bots that interact with Teams' artificial intelligence capabilities. The SDK is available for JavaScript/TypeScript, .NET, and Python.
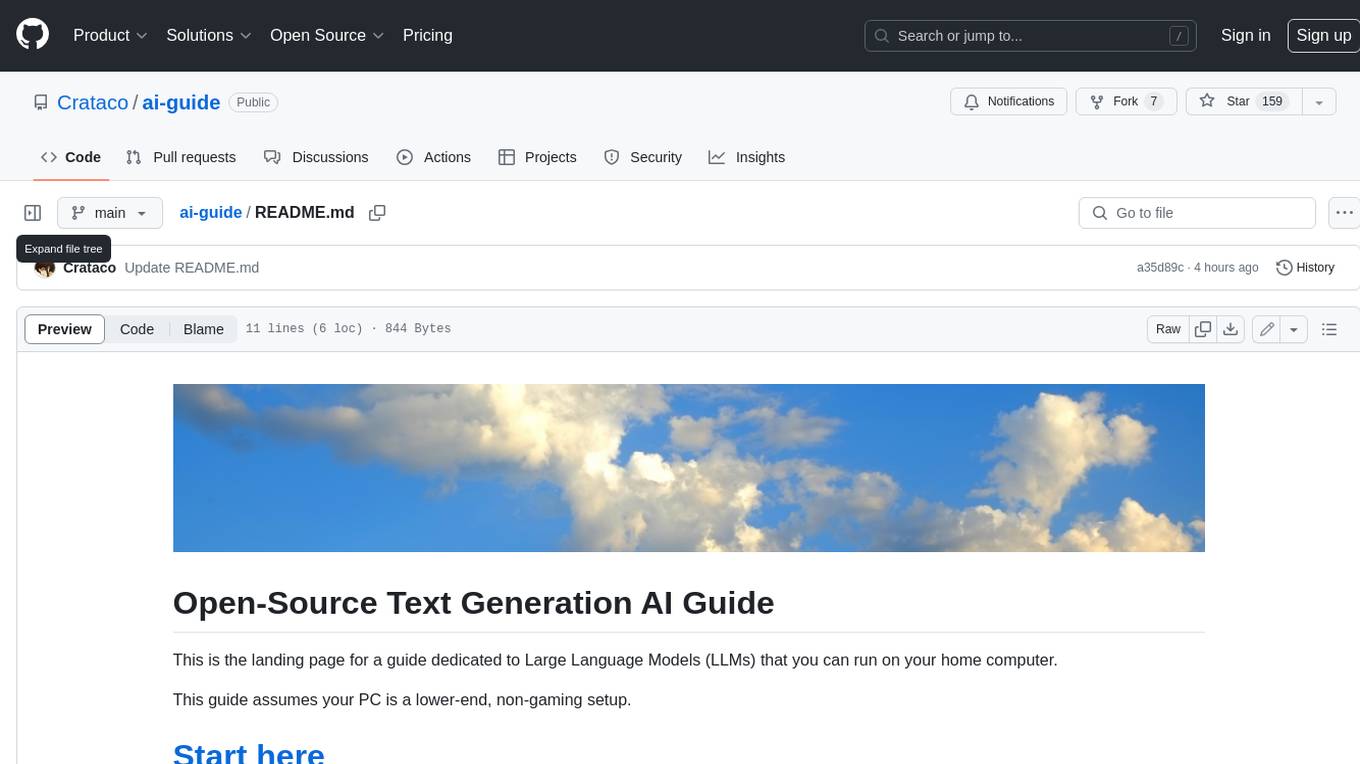
ai-guide
This guide is dedicated to Large Language Models (LLMs) that you can run on your home computer. It assumes your PC is a lower-end, non-gaming setup.
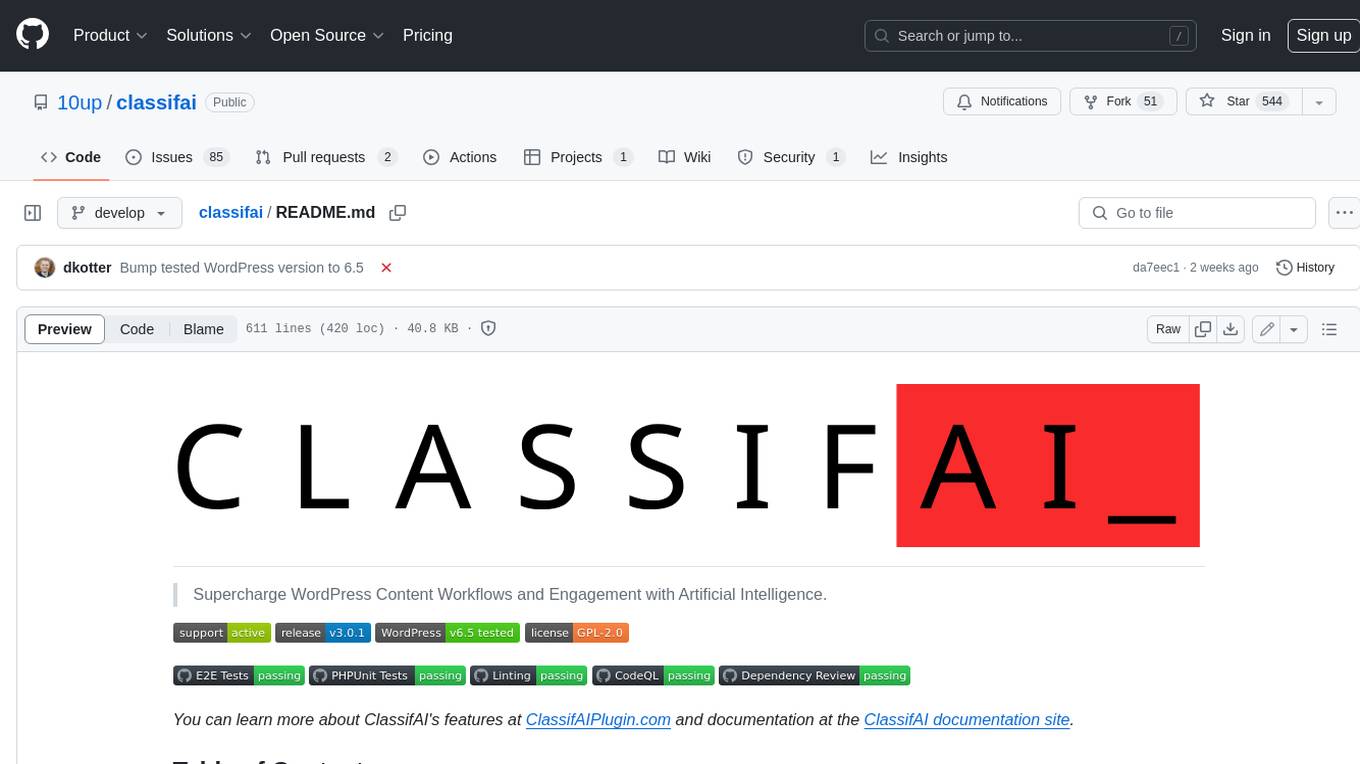
classifai
Supercharge WordPress Content Workflows and Engagement with Artificial Intelligence. Tap into leading cloud-based services like OpenAI, Microsoft Azure AI, Google Gemini and IBM Watson to augment your WordPress-powered websites. Publish content faster while improving SEO performance and increasing audience engagement. ClassifAI integrates Artificial Intelligence and Machine Learning technologies to lighten your workload and eliminate tedious tasks, giving you more time to create original content that matters.
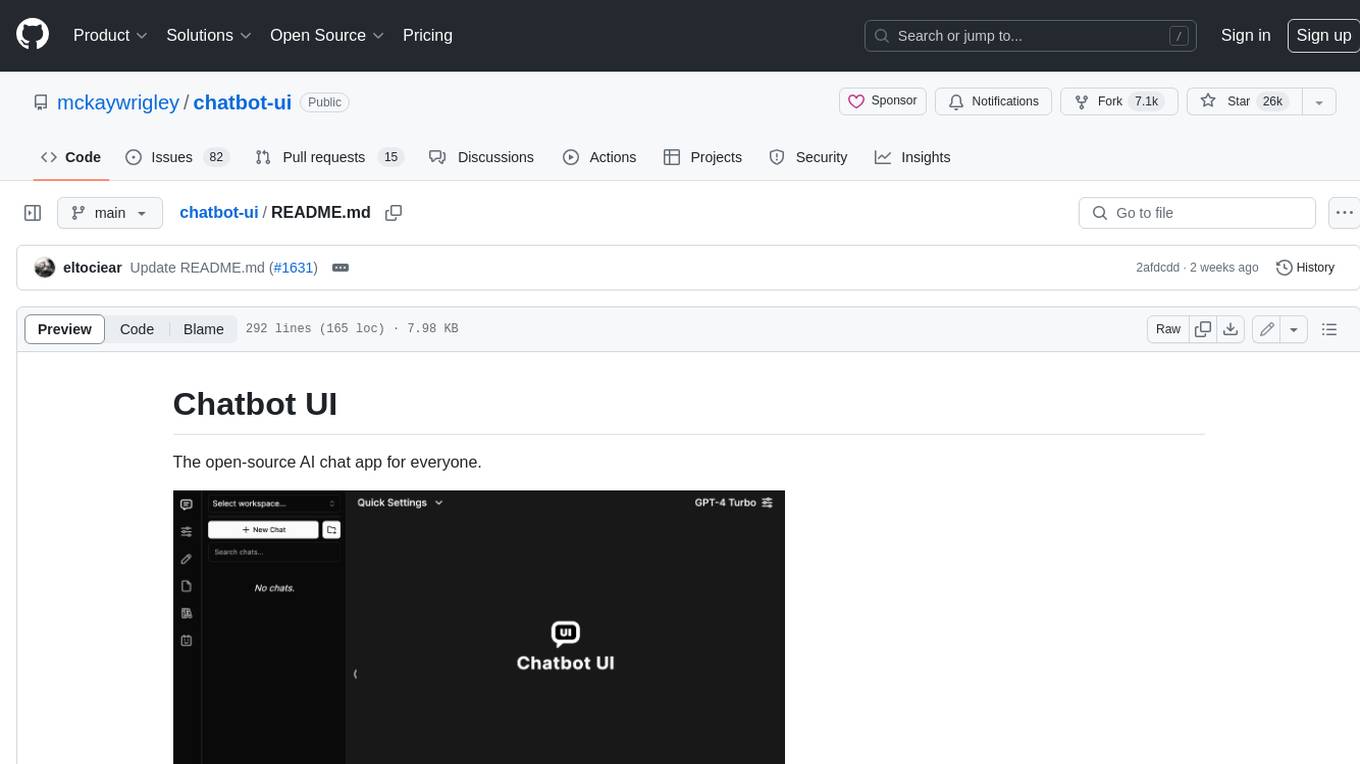
chatbot-ui
Chatbot UI is an open-source AI chat app that allows users to create and deploy their own AI chatbots. It is easy to use and can be customized to fit any need. Chatbot UI is perfect for businesses, developers, and anyone who wants to create a chatbot.
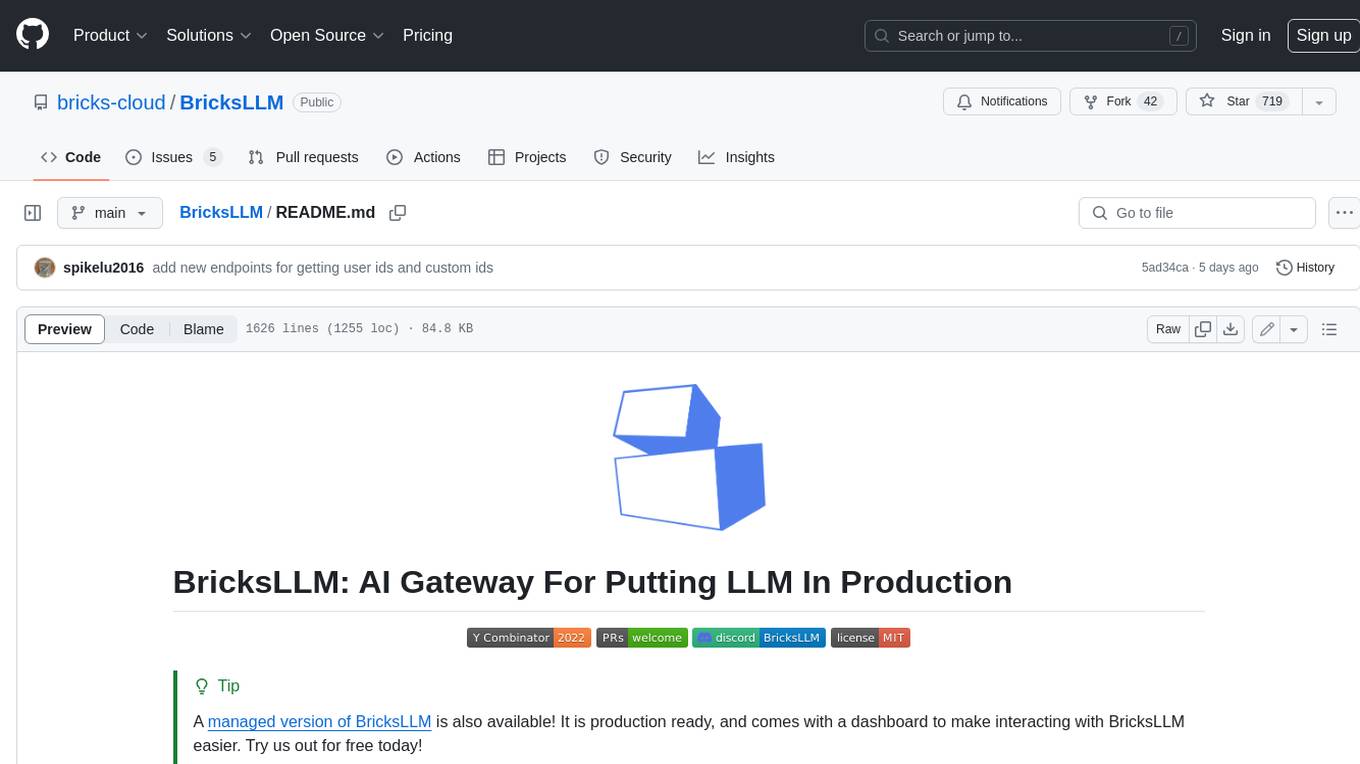
BricksLLM
BricksLLM is a cloud native AI gateway written in Go. Currently, it provides native support for OpenAI, Anthropic, Azure OpenAI and vLLM. BricksLLM aims to provide enterprise level infrastructure that can power any LLM production use cases. Here are some use cases for BricksLLM: * Set LLM usage limits for users on different pricing tiers * Track LLM usage on a per user and per organization basis * Block or redact requests containing PIIs * Improve LLM reliability with failovers, retries and caching * Distribute API keys with rate limits and cost limits for internal development/production use cases * Distribute API keys with rate limits and cost limits for students
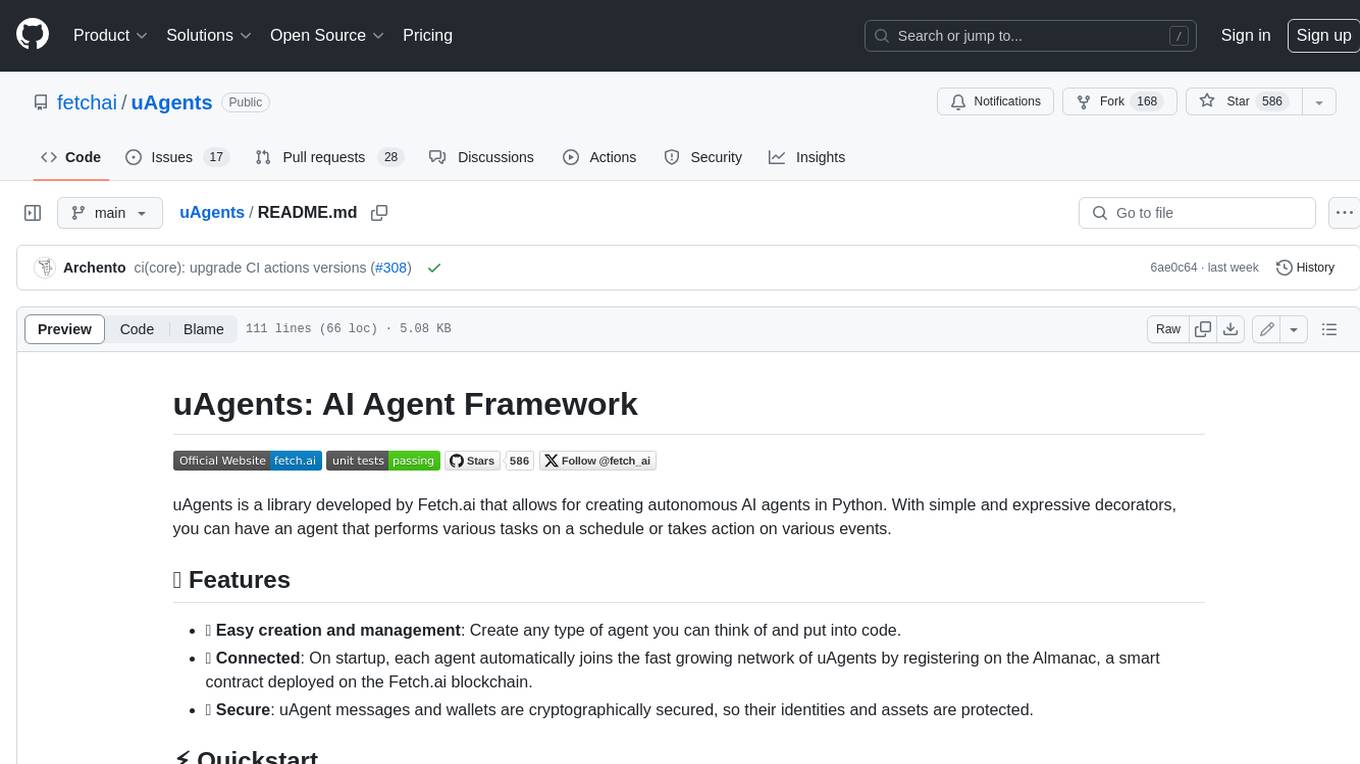
uAgents
uAgents is a Python library developed by Fetch.ai that allows for the creation of autonomous AI agents. These agents can perform various tasks on a schedule or take action on various events. uAgents are easy to create and manage, and they are connected to a fast-growing network of other uAgents. They are also secure, with cryptographically secured messages and wallets.
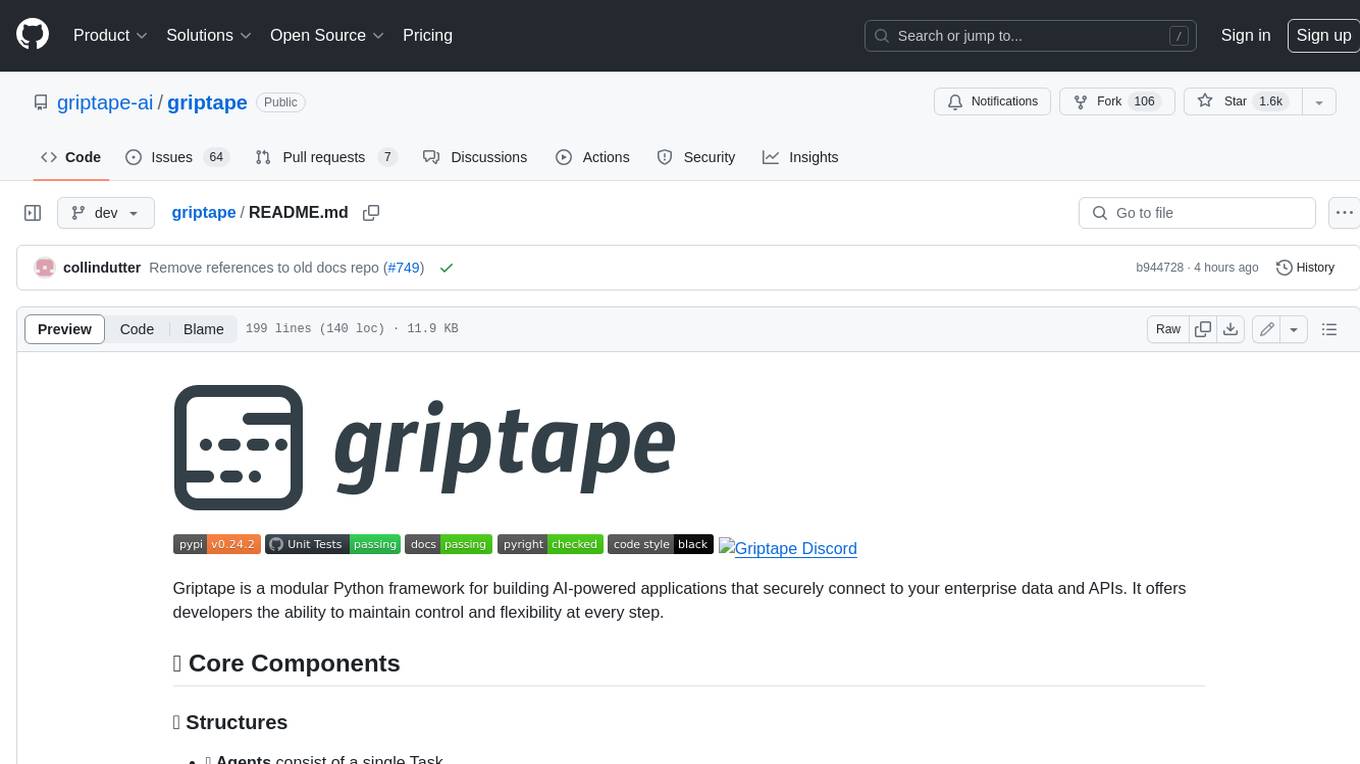
griptape
Griptape is a modular Python framework for building AI-powered applications that securely connect to your enterprise data and APIs. It offers developers the ability to maintain control and flexibility at every step. Griptape's core components include Structures (Agents, Pipelines, and Workflows), Tasks, Tools, Memory (Conversation Memory, Task Memory, and Meta Memory), Drivers (Prompt and Embedding Drivers, Vector Store Drivers, Image Generation Drivers, Image Query Drivers, SQL Drivers, Web Scraper Drivers, and Conversation Memory Drivers), Engines (Query Engines, Extraction Engines, Summary Engines, Image Generation Engines, and Image Query Engines), and additional components (Rulesets, Loaders, Artifacts, Chunkers, and Tokenizers). Griptape enables developers to create AI-powered applications with ease and efficiency.