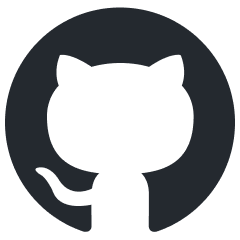
AI-Agent-Starter-Kit
Collab.Land AI Agent Starter Kit
Stars: 147
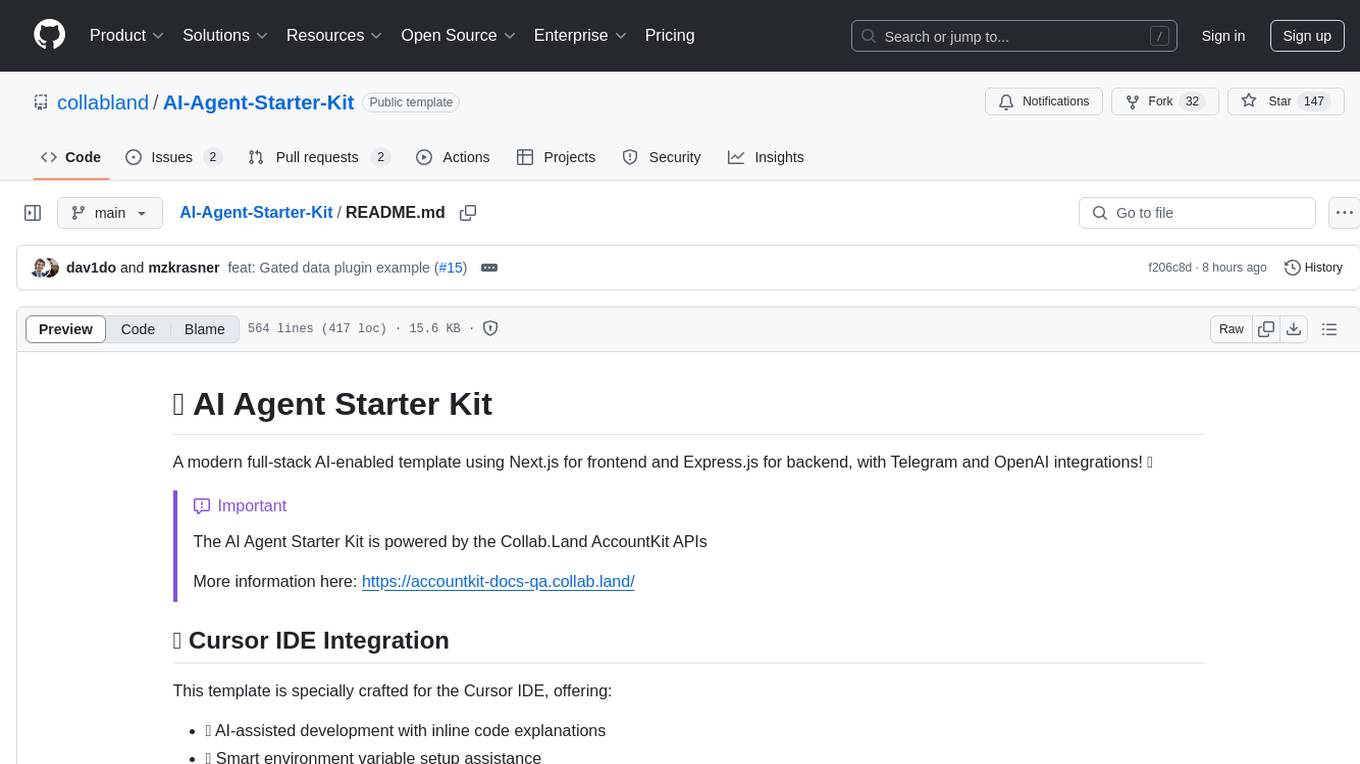
AI Agent Starter Kit is a modern full-stack AI-enabled template using Next.js for frontend and Express.js for backend, with Telegram and OpenAI integrations. It offers AI-assisted development, smart environment variable setup assistance, intelligent error resolution, context-aware code completion, and built-in debugging helpers. The kit provides a structured environment for developers to interact with AI tools seamlessly, enhancing the development process and productivity.
README:
A modern full-stack AI-enabled template using Next.js for frontend and Express.js for backend, with Telegram and OpenAI integrations! ✨
[!IMPORTANT] The AI Agent Starter Kit is powered by the Collab.Land AccountKit APIs
More information here: https://accountkit-docs-qa.collab.land/
This template is specially crafted for the Cursor IDE, offering:
- 🤖 AI-assisted development with inline code explanations
- 🔍 Smart environment variable setup assistance
- 💡 Intelligent error resolution
- 📝 Context-aware code completion
- 🛠️ Built-in debugging helpers
Just highlight any error message, code snippet, or variable in Cursor and ask the AI for help!
-
Cmd/Ctrl + K
: Ask AI about highlighted code -
Cmd/Ctrl + L
: Get code explanations -
Cmd/Ctrl + I
: Generate code completions - Highlight any error message to get instant fixes
- Prerequisites:
node >= 22 🟢
pnpm >= 9.14.1 📦
- Install dependencies:
pnpm install
- Fire up the dev servers:
pnpm run dev
.
├── 📦 client/ # Next.js frontend
│ ├── 📱 app/ # Next.js app router (pages, layouts)
│ ├── 🧩 components/ # React components
│ │ └── HelloWorld.tsx # Example component with API integration
│ ├── 💅 styles/ # Global styles and Tailwind config
│ │ ├── globals.css # Global CSS and Tailwind imports
│ │ └── tailwind.config.ts # Tailwind configuration
│ ├── 🛠️ bin/ # Client scripts
│ │ └── validate-env # Environment variables validator
│ ├── next.config.js # Next.js configuration (API rewrites)
│ ├── .env.example # Example environment variables for client
│ └── tsconfig.json # TypeScript configuration
│
├── ⚙️ server/ # Express.js backend
│ ├── 📂 src/ # Server source code
│ │ ├── 🛣️ routes/ # API route handlers
│ │ │ └── hello.ts # Example route with middleware
│ │ └── index.ts # Server entry point (Express setup)
│ ├── 🛠️ bin/ # Server scripts
│ │ ├── validate-env # Environment validator
│ │ └── www-dev # Development server launcher
│ └── tsconfig.json # TypeScript configuration
│
├── 📦 scripts/ # Project scripts
│ └── dev # Concurrent dev servers launcher
│
├── 📝 .env.example # Root environment variables example for server
├── 🔧 package.json # Root package with workspace config
└── 📦 pnpm-workspace.yaml # PNPM workspace configuration
💡 Pro Tip: In Cursor IDE, highlight any environment variable name and ask the AI for setup instructions!
-
NEXT_PUBLIC_API_URL
: Backend API URL (default: http://localhost:3001) 🌐 -
NEXT_PUBLIC_TELEGRAM_BOT_NAME
: Telegram bot name without the @ symbol, you can get it from BotFather after creating your bot (default: your_bot_username) 🤖
-
PORT
: Server port (default: 3001) 🚪 -
NODE_ENV
: Environment (development/production) 🌍 -
TELEGRAM_BOT_TOKEN
: 🤖- Open Telegram and search for @BotFather
- Start chat and send
/newbot
- Follow prompts to name your bot
- Copy the provided token
-
OPENAI_API_KEY
: 🧠- Visit https://platform.openai.com/api-keys
- Click "Create new secret key"
- Give it a name and copy the key immediately
- Set usage limits in API settings if needed
-
NGROK_AUTH_TOKEN
: 🔗- Create account at https://dashboard.ngrok.com/signup
- Go to https://dashboard.ngrok.com/get-started/your-authtoken
- Copy your authtoken
-
NGROK_DOMAIN
: 🔗- Go to https://dashboard.ngrok.com/domains
- Copy your domain (without https://)
-
COLLABLAND_API_KEY
: 🤝- Visit https://dev-portal-qa.collab.land/signin
- Click on "Get Started"
- Select Telegram login
- Login with Telegram
- Verify your e-mail with the OTP sent to your inbox
- Click on "Request API Access" on the top right corner, and set up the API key name
- Copy your API key
-
GAIANET_MODEL
: 🤖- Visit https://docs.gaianet.ai/user-guide/nodes
- Choose your model (default: llama)
- Copy the model name
-
GAIANET_SERVER_URL
: 🌐- Visit https://docs.gaianet.ai/user-guide/nodes
- Get server URL for your chosen model
- Default: https://llama8b.gaia.domains/v1
-
GAIANET_EMBEDDING_MODEL
: 🧬- Visit https://docs.gaianet.ai/user-guide/nodes
- Choose embedding model (default: nomic-embed)
- Copy the model name
-
USE_GAIANET_EMBEDDING
: ⚙️- Set to TRUE to enable Gaianet embeddings
- Set to FALSE to disable (default: TRUE)
-
JOKERACE_CONTRACT_ADDRESS
: 🎰- Go to https://www.jokerace.io/contest/new
- Create the contest
- Copy the contract address
-
ELIZA_CHARACTER_PATH
: 🤖- Default: "character.json"
- Points to a JSON file containing your AI agent's personality configuration
- Example paths:
- character.json (default Ace personality)
- vaitalik.json (Vitalik personality)
- custom/my-agent.json (your custom personality)
-
TOKEN_DETAILS_PATH
: Points to a JSON/JSONC file containing your token metadata for minting- Default: "token_metadata.example.jsonc"
- Steps:
- Copy the template:
cp token_metadata.example.jsonc token.jsonc
- Set this env var to point to your file
- Example:
token.jsonc
-
TWITTER_CLIENT_ID
&TWITTER_CLIENT_SECRET
: Authentication credentials for Twitter API integration- Go to Twitter Developer Portal
- Create a new project/app if you haven't already
- Navigate to "Keys and Tokens" section
- Under "OAuth 2.0 Client ID and Client Secret":
- Copy "Client ID" →
TWITTER_CLIENT_ID
- Generate "Client Secret" →
TWITTER_CLIENT_SECRET
- Copy "Client ID" →
- Configure OAuth settings:
- Add callback URL:
http://localhost:3001/auth/twitter/callback
(development) - Add your production callback URL if deploying
- Add callback URL:
- Format: Alphanumeric strings
- Example:
TWITTER_CLIENT_ID=Abc123XyzClientID TWITTER_CLIENT_SECRET=Xyz789AbcClientSecret
-
DISCORD_CLIENT_ID
&DISCORD_CLIENT_SECRET
: Authentication credentials for Discord API integration- Go to Discord Developer Portal
- Click "New Application" or select existing one
- Navigate to "OAuth2" section in left sidebar
- Under "Client Information":
- Copy "Client ID" →
DISCORD_CLIENT_ID
- Copy "Client Secret" →
DISCORD_CLIENT_SECRET
- Copy "Client ID" →
- Configure OAuth settings:
- Add redirect URL:
http://localhost:3001/auth/discord/callback
(development) - Add your production redirect URL if deploying
- Select required scopes (typically
identify
andemail
)
- Add redirect URL:
- Format: Alphanumeric strings
- Example:
DISCORD_CLIENT_ID=123456789012345678 DISCORD_CLIENT_SECRET=abcdef123456789xyz
-
GITHUB_CLIENT_ID
&GITHUB_CLIENT_SECRET
: Authentication credentials for GitHub OAuth integration- Go to GitHub Developer Settings
- Click "New OAuth App" or select existing one
- Under "OAuth Apps" settings:
- Application name: Your app name
- Homepage URL:
http://localhost:3001
(development) - Authorization callback URL:
http://localhost:3001/auth/github/callback
- After creating/selecting the app:
- Copy "Client ID" →
GITHUB_CLIENT_ID
- Generate new "Client Secret" →
GITHUB_CLIENT_SECRET
- Copy "Client ID" →
- Configure OAuth scopes:
- Recommended scopes:
read:user
,user:email
- Recommended scopes:
- Format: Alphanumeric strings
- Example:
GITHUB_CLIENT_ID=1234567890abcdef1234 GITHUB_CLIENT_SECRET=1234567890abcdef1234567890abcdef12345678
-
ORBIS_CONTEXT_ID
,ORBIS_TABLE_ID
, &ORBIS_ENV
: OrbisDB table identifiers to enable gated memory storage functionality-
Visit the Orbis Studio and log in with your browser wallet. Once logged in, set up a new context under the
Contexts
tab. Assign that value toORBIS_CONTEXT_ID
in your .env file -
On the right-hand side of the same page, you should see a variable called "Environment ID" - this is the DID representation of the address you used to sign into the hosted Orbis studio. Assign this value to
ORBIS_ENV
in your .env file -
Generate an OrbisDB seed to self-authenticate onto the Ceramic network and save to
ORBIS_SEED
:
pnpm gen-seed
- Finally, deploy your OrbisDB data model we will use to create and query via vector search. Copy the value prefixed with "k" into your
.env
file next toORBIS_TABLE_ID
:
pnpm deploy-model
- You can use the default provided values for
ORBIS_GATEWAY_URL
ANDCERAMIC_NODE_URL
provided in your .env.example file as-is
-
Note: For production, update the Homepage URL and callback URL to your production domain.
Security Notes:
- Never commit these values to version control
- Use different credentials for development and production
- Rotate secrets periodically
- Store production secrets in secure environment variables
🔒 Note: Keep these tokens secure! Never commit them to version control. The template's
.gitignore
has your back!
- Build both apps:
pnpm run build
- Launch production servers:
pnpm start
- For production deployment: 🌎
- Set
NODE_ENV=production
- Use proper SSL certificates 🔒
- Configure CORS settings in server/src/index.ts 🛡️
- Set up error handling and logging 📝
- Use process manager like PM2 ⚡
- Client:
const ENV_HINTS = {
NEXT_PUBLIC_API_URL: "Your API URL (usually http://localhost:3001)",
// Add more hints as needed! ✨
};
- Server:
const ENV_HINTS = {
PORT: "API port (usually 3001)",
NODE_ENV: "development or production",
TELEGRAM_BOT_TOKEN: "Get from @BotFather",
OPENAI_API_KEY: "Get from OpenAI dashboard",
NGROK_AUTH_TOKEN: "Get from ngrok dashboard",
// Add more hints as needed! ✨
};
- Add TypeScript types in respective env.d.ts files 📝
- Create new route file in server/src/routes/
- Import and use in server/src/index.ts
- Add corresponding client API call in client/components/
- Create component in client/components/
- Use Tailwind CSS for styling
- Follow existing patterns for API integration
- Create middleware in server/src/middleware/
- Apply globally or per-route basis
- Copy the token metadata template:
cp token_metadata.example.jsonc token.jsonc
- Edit
token.jsonc
with your token details:
{
"name": "YourToken", // Token name
"symbol": "TOKEN", // Token symbol (2-6 chars)
"description": "Your token description",
"websiteLink": "https://yoursite.com",
"twitter": "your_twitter_handle",
"discord": "https://discord.gg/your_server",
"telegram": "your_bot_telegram_username",
"nsfw": false,
"image": "ipfs://your_ipfs_hash", // Upload image to IPFS first
}
- Update
.env
to point to your token file:
TOKEN_DETAILS_PATH=token.jsonc
- Start your bot and use the
/mint
command in Telegram. The bot will:
- Read your token config
- Mint on Base Sepolia testnet
- Return contract details and token page URL
Note: Make sure you have set up your COLLABLAND_API_KEY and TELEGRAM_BOT_TOKEN in .env first.
- Next.js App Router: https://nextjs.org/docs/app 🎯
- Express.js: https://expressjs.com/ ⚡
- Tailwind CSS: https://tailwindcss.com/docs 💅
- TypeScript: https://www.typescriptlang.org/docs/ 📘
Follow these commit message guidelines to automate changelog generation:
-
feat: add new feature
- New features (generates under 🚀 Features) -
fix: resolve bug
- Bug fixes (generates under 🐛 Bug Fixes) -
docs: update readme
- Documentation changes (generates under 📝 Documentation) -
chore: update deps
- Maintenance (generates under 🧰 Maintenance)
Example:
git commit -m "feat: add OAuth support for Discord"
git commit -m "fix: resolve token validation issue"
- Navigate to lit-actions directory:
cd lit-actions
pnpm install
- Configure environment:
cp .env.example .env
Required variables:
-
PINATA_JWT
: Your Pinata JWT for IPFS uploads -
PINATA_URL
: Pinata gateway URL
- Create new action in
src/actions/
:
/// <reference path="../global.d.ts" />
const go = async () => {
// Access Lit SDK APIs
const tokenId = await Lit.Actions.pubkeyToTokenId({ publicKey });
// Sign data
const signature = await Lit.Actions.signEcdsa({
publicKey,
toSign,
sigName,
});
// Return response
Lit.Actions.setResponse({
response: JSON.stringify({ result: "success" }),
});
};
go();
- Start development server:
pnpm run dev
This will:
- Build TypeScript → JavaScript
- Bundle with dependencies
- Inject SDK shims
- Upload to IPFS
- Watch for changes
- Create shim in
shims/
:
// shims/my-sdk.shim.js
import { MySDK } from "my-sdk";
globalThis.MySDK = MySDK;
- Update types in
src/global.d.ts
:
declare global {
const MySDK: typeof MySDK;
}
# Build only
pnpm run build
# Build & deploy to IPFS
pnpm run start
IPFS hashes are saved to actions/ipfs.json
:
{
"my-action.js": {
"IpfsHash": "Qm...",
"PinSize": 12345,
"Timestamp": "2025-01-03T..."
}
}
The Lit Actions runtime provides:
-
Lit.Actions
-
signEcdsa()
: Sign data with PKP -
pubkeyToTokenId()
: Convert public key to token ID -
getPermittedAuthMethods()
: Get permitted auth methods -
checkConditions()
: Check access control conditions -
setResponse()
: Return data to client - Full API in
src/global.d.ts
-
-
Built-in SDKs
-
ethers
: Ethereum interactions -
Buffer
: Buffer utilities
-
-
Type Safety
- Always reference
global.d.ts
- Define types for parameters
- Use TypeScript features
- Always reference
-
SDK Management
- Create minimal shims
- Document SDK versions
- Test SDK compatibility
-
Action Structure
- One action per file
- Clear async/await flow
- Proper error handling
-
Deployment
- Test locally first
- Verify IPFS uploads
- Keep actions small
pnpm run dev # Development mode
pnpm run build # Build actions
pnpm run start # Deploy to IPFS
pnpm run lint # Fix code style
pnpm run watch # Watch mode
lit-actions/
├── actions/ # Built JS + IPFS hashes
├── shims/ # SDK shims
├── src/
│ ├── actions/ # TypeScript sources
│ ├── global.d.ts # Type definitions
│ └── index.ts # IPFS deployment
├── esbuild.js # Build config
└── package.json
For more details, check the Lit Protocol docs.
For Tasks:
Click tags to check more tools for each tasksFor Jobs:
Alternative AI tools for AI-Agent-Starter-Kit
Similar Open Source Tools
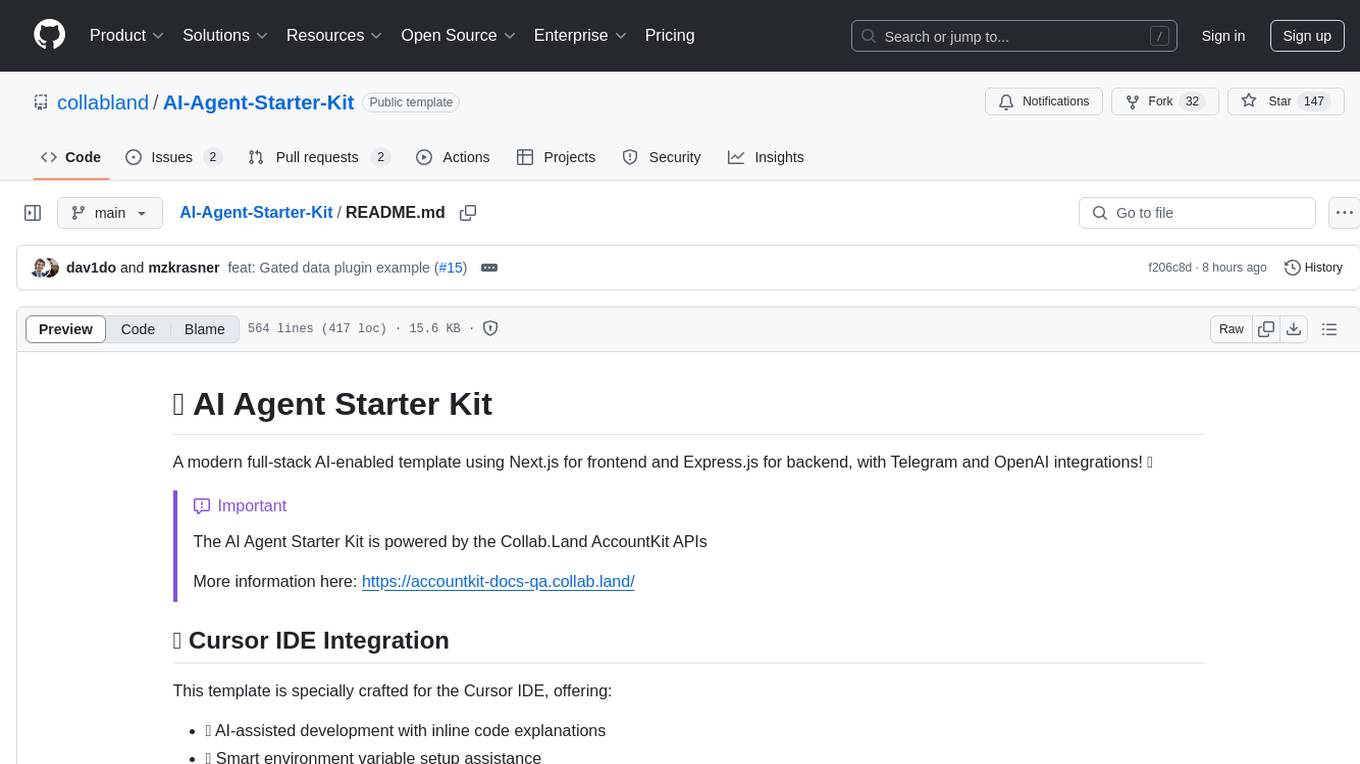
AI-Agent-Starter-Kit
AI Agent Starter Kit is a modern full-stack AI-enabled template using Next.js for frontend and Express.js for backend, with Telegram and OpenAI integrations. It offers AI-assisted development, smart environment variable setup assistance, intelligent error resolution, context-aware code completion, and built-in debugging helpers. The kit provides a structured environment for developers to interact with AI tools seamlessly, enhancing the development process and productivity.
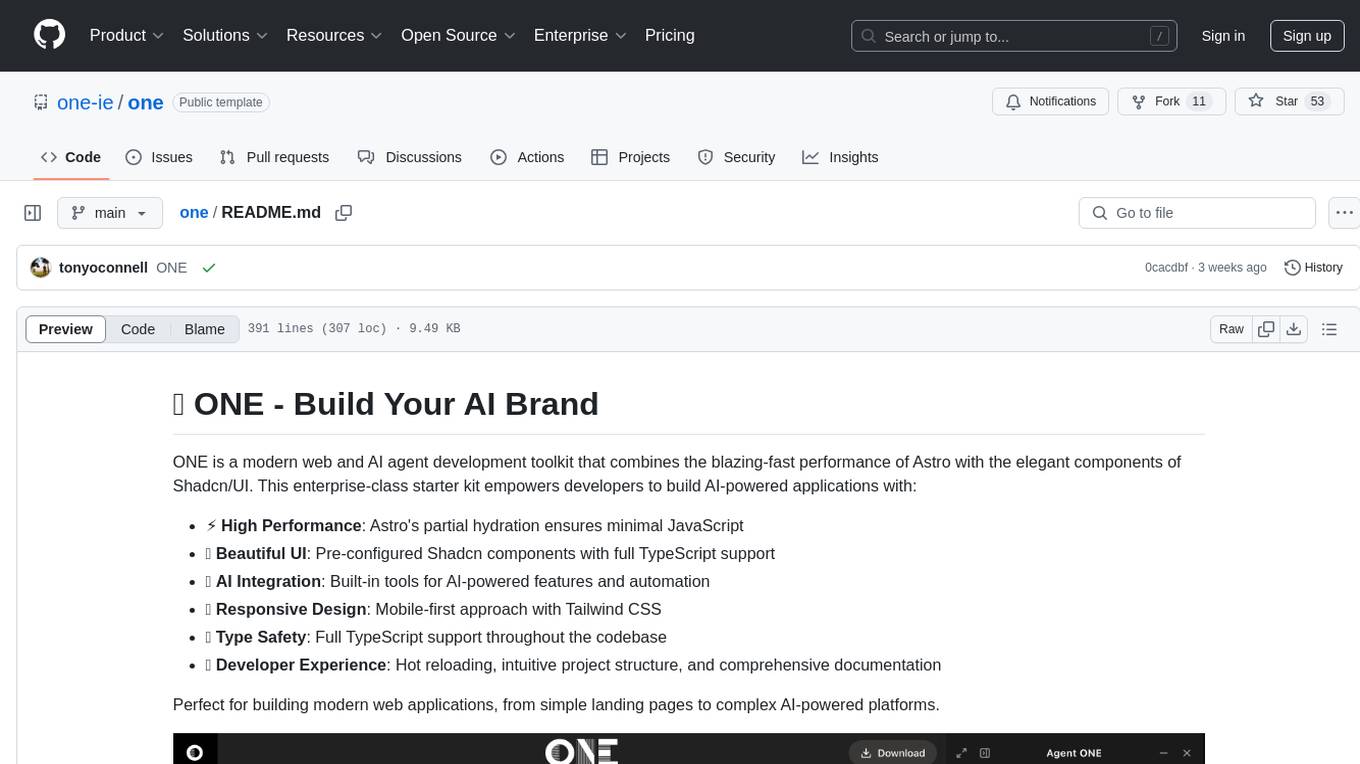
one
ONE is a modern web and AI agent development toolkit that empowers developers to build AI-powered applications with high performance, beautiful UI, AI integration, responsive design, type safety, and great developer experience. It is perfect for building modern web applications, from simple landing pages to complex AI-powered platforms.
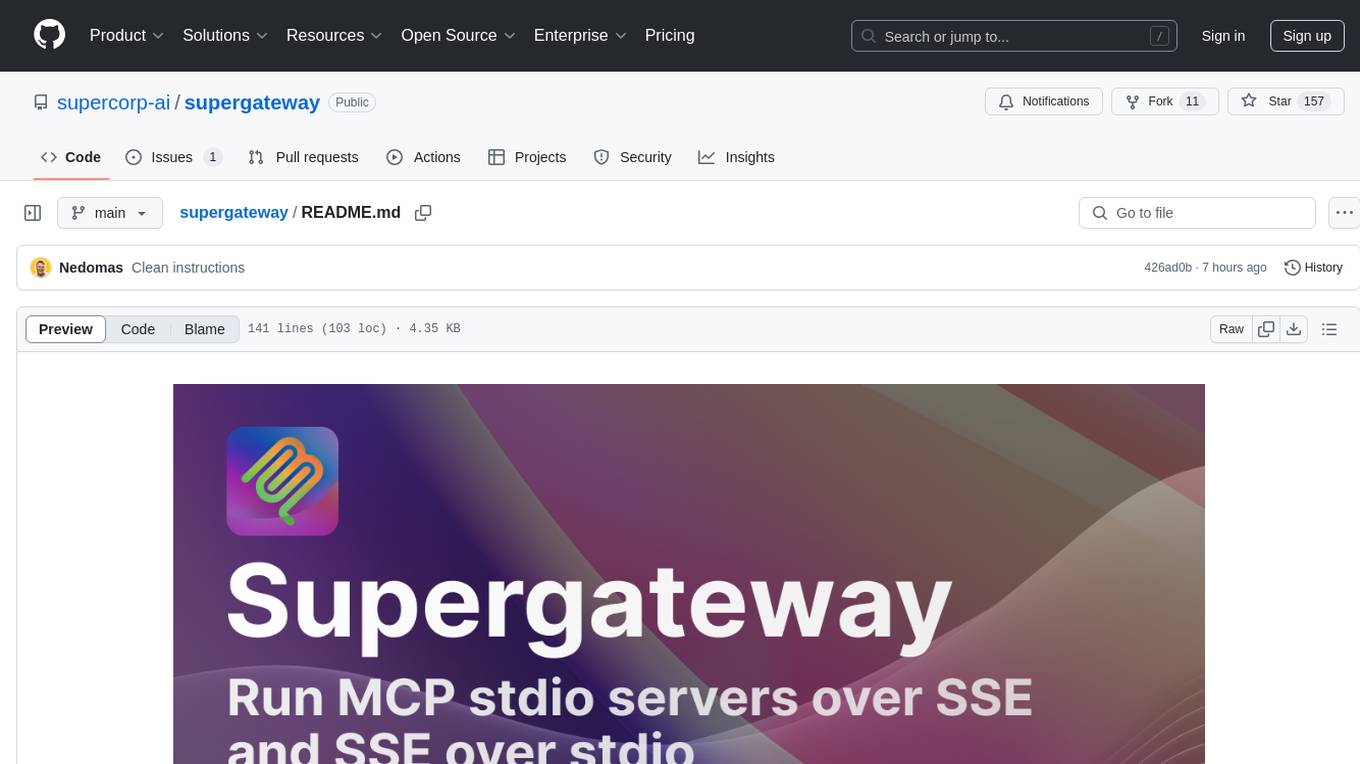
supergateway
Supergateway is a tool that allows running MCP stdio-based servers over SSE (Server-Sent Events) with one command. It is useful for remote access, debugging, or connecting to SSE-based clients when your MCP server only speaks stdio. The tool supports running in SSE to Stdio mode as well, where it connects to a remote SSE server and exposes a local stdio interface for downstream clients. Supergateway can be used with ngrok to share local MCP servers with remote clients and can also be run in a Docker containerized deployment. It is designed with modularity in mind, ensuring compatibility and ease of use for AI tools exchanging data.
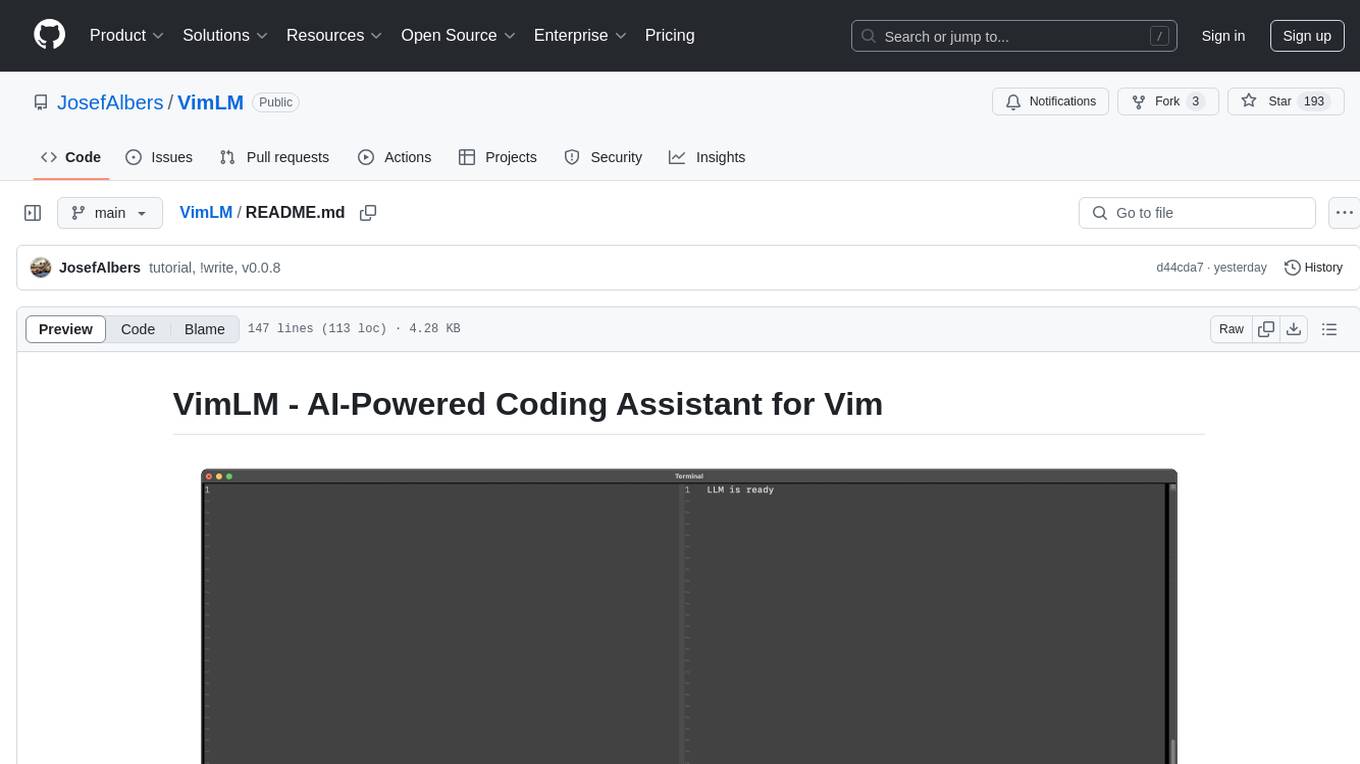
VimLM
VimLM is an AI-powered coding assistant for Vim that integrates AI for code generation, refactoring, and documentation directly into your Vim workflow. It offers native Vim integration with split-window responses and intuitive keybindings, offline first execution with MLX-compatible models, contextual awareness with seamless integration with codebase and external resources, conversational workflow for iterating on responses, project scaffolding for generating and deploying code blocks, and extensibility for creating custom LLM workflows with command chains.
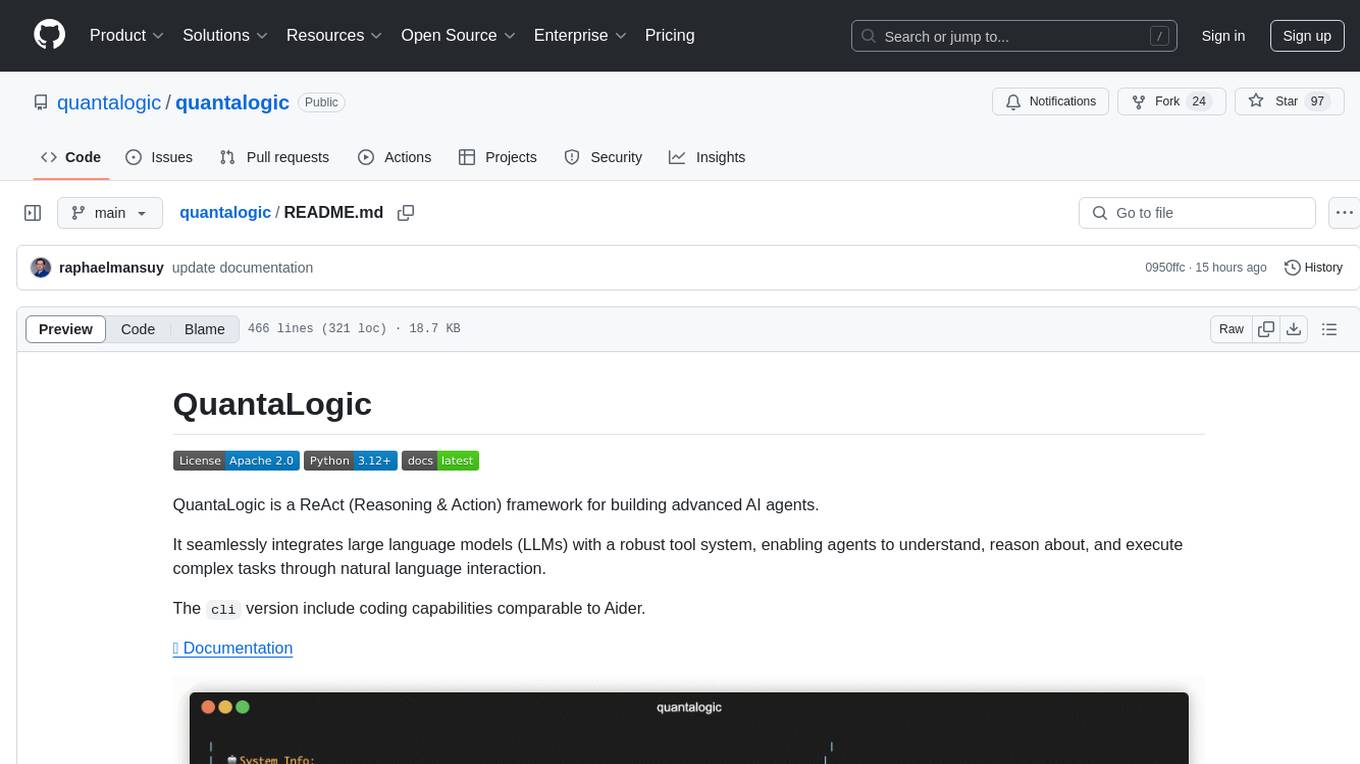
quantalogic
QuantaLogic is a ReAct framework for building advanced AI agents that seamlessly integrates large language models with a robust tool system. It aims to bridge the gap between advanced AI models and practical implementation in business processes by enabling agents to understand, reason about, and execute complex tasks through natural language interaction. The framework includes features such as ReAct Framework, Universal LLM Support, Secure Tool System, Real-time Monitoring, Memory Management, and Enterprise Ready components.
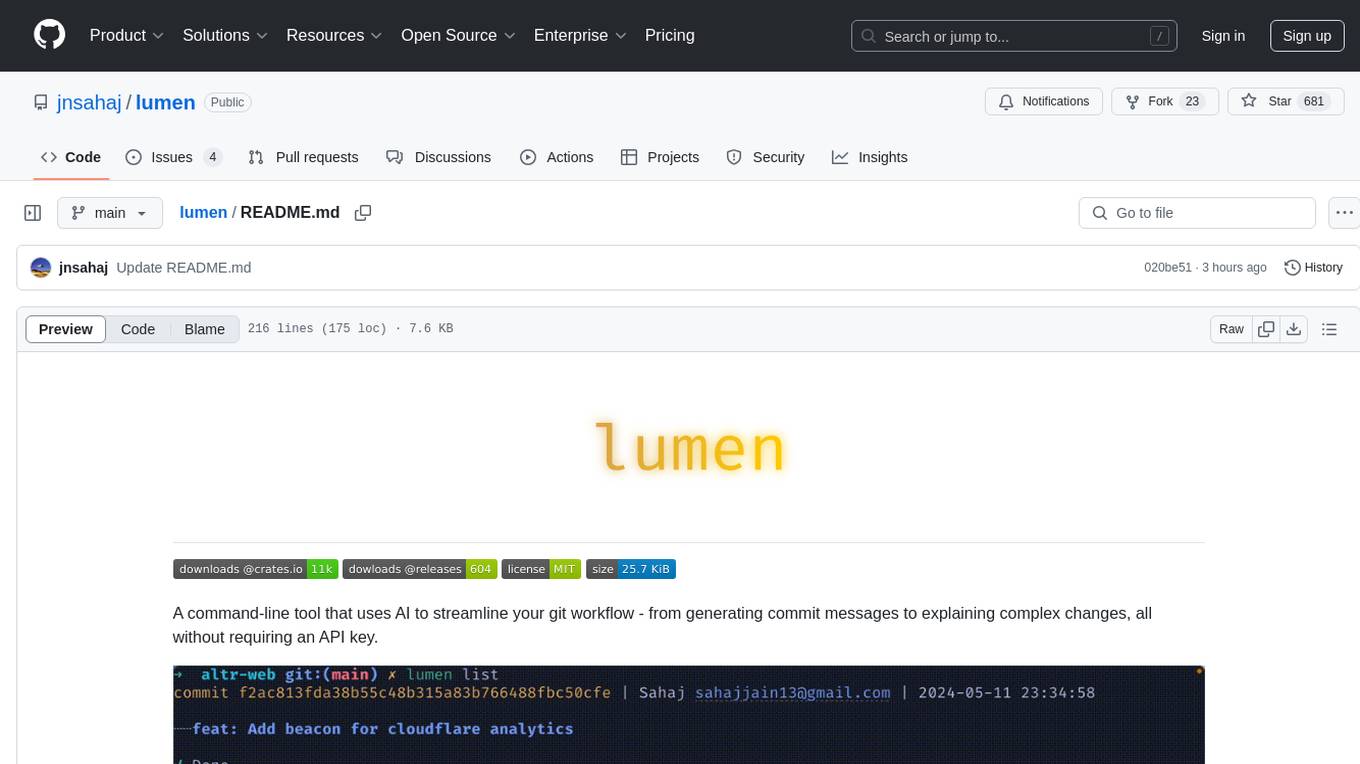
lumen
Lumen is a command-line tool that leverages AI to enhance your git workflow. It assists in generating commit messages, understanding changes, interactive searching, and analyzing impacts without the need for an API key. With smart commit messages, git history insights, interactive search, change analysis, and rich markdown output, Lumen offers a seamless and flexible experience for users across various git workflows.
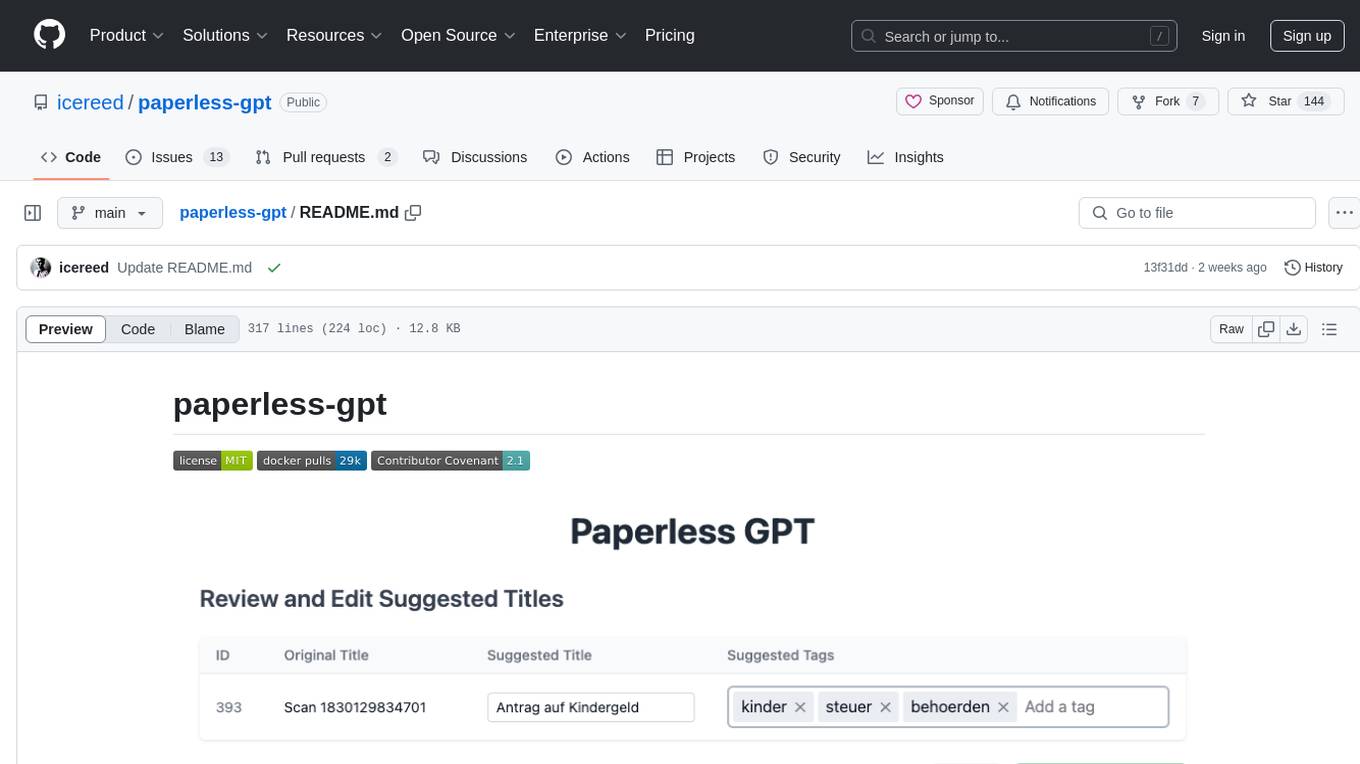
paperless-gpt
paperless-gpt is a tool designed to generate accurate and meaningful document titles and tags for paperless-ngx using Large Language Models (LLMs). It supports multiple LLM providers, including OpenAI and Ollama. With paperless-gpt, you can streamline your document management by automatically suggesting appropriate titles and tags based on the content of your scanned documents. The tool offers features like multiple LLM support, customizable prompts, easy integration with paperless-ngx, user-friendly interface for reviewing and applying suggestions, dockerized deployment, automatic document processing, and an experimental OCR feature.
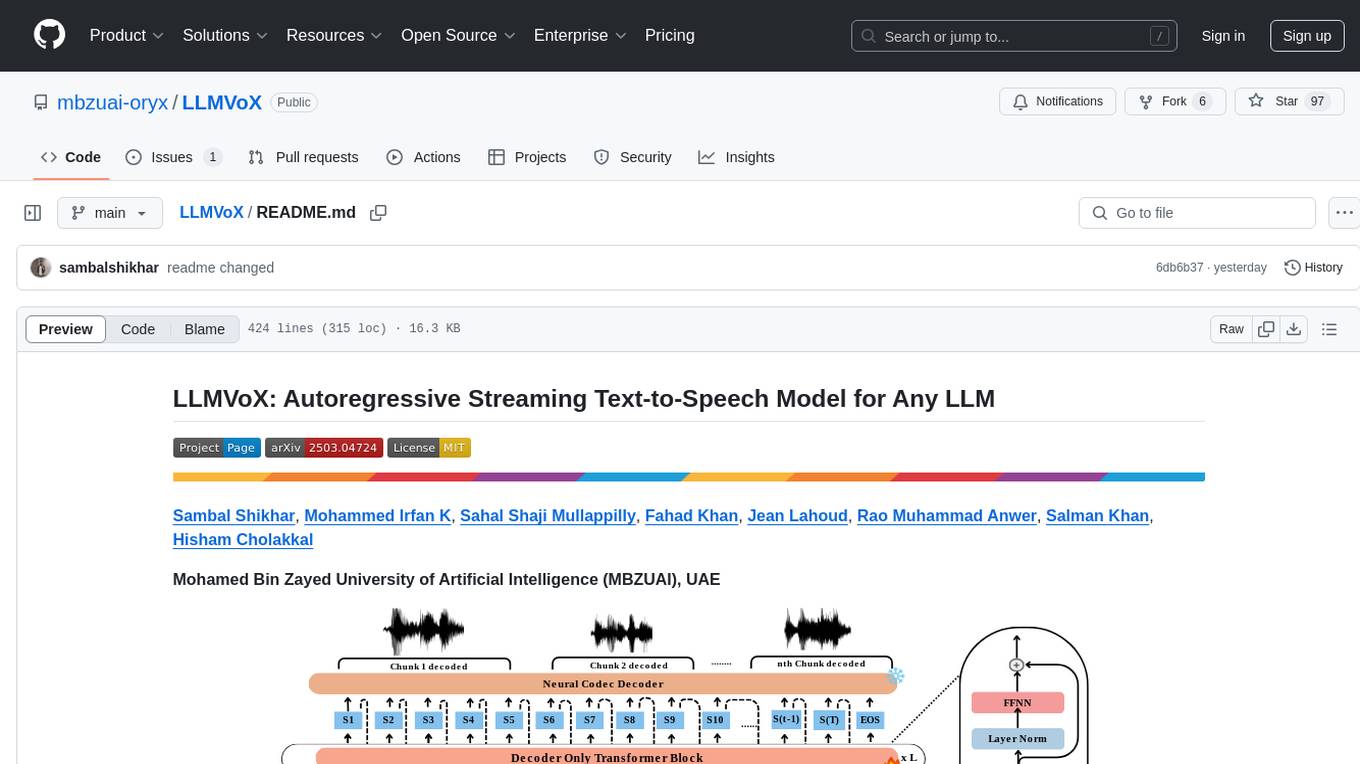
LLMVoX
LLMVoX is a lightweight 30M-parameter, LLM-agnostic, autoregressive streaming Text-to-Speech (TTS) system designed to convert text outputs from Large Language Models into high-fidelity streaming speech with low latency. It achieves significantly lower Word Error Rate compared to speech-enabled LLMs while operating at comparable latency and speech quality. Key features include being lightweight & fast with only 30M parameters, LLM-agnostic for easy integration with existing models, multi-queue streaming for continuous speech generation, and multilingual support for easy adaptation to new languages.
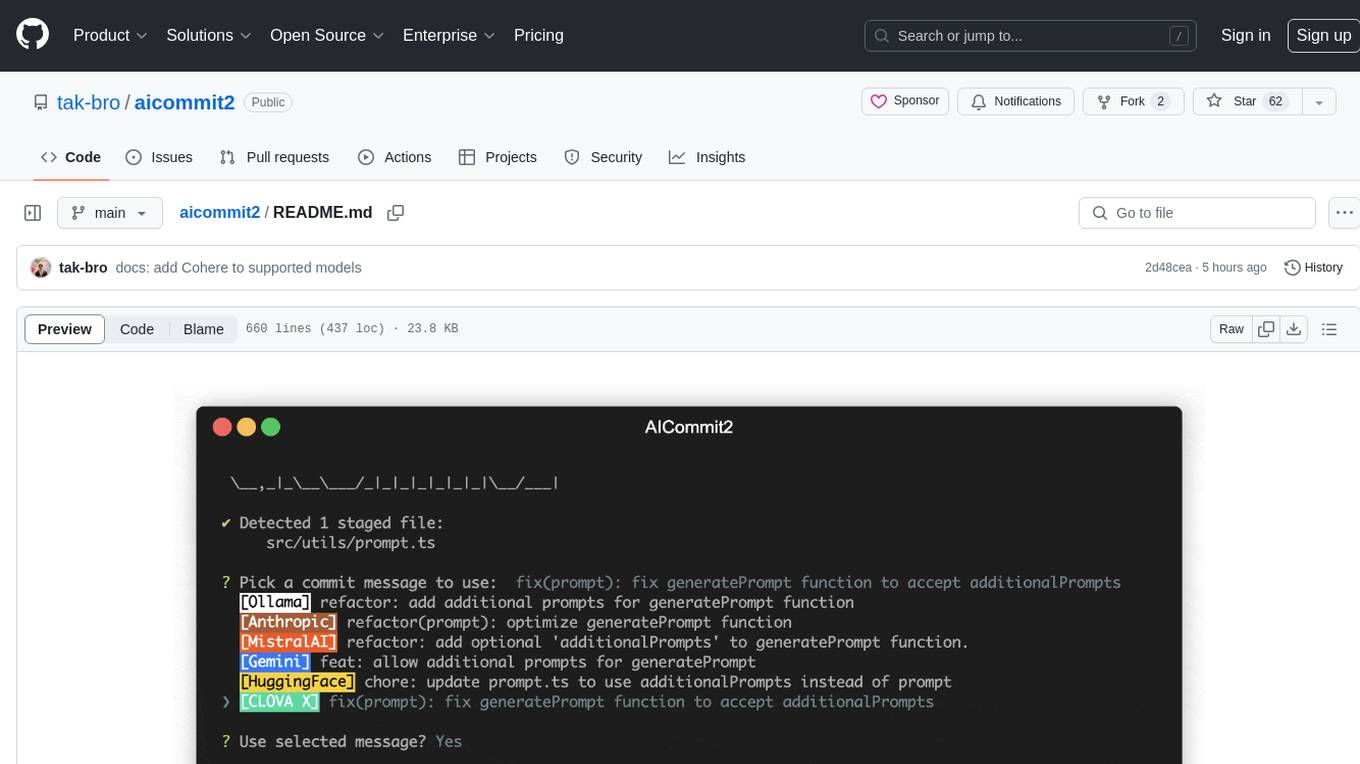
aicommit2
AICommit2 is a Reactive CLI tool that streamlines interactions with various AI providers such as OpenAI, Anthropic Claude, Gemini, Mistral AI, Cohere, and unofficial providers like Huggingface and Clova X. Users can request multiple AI simultaneously to generate git commit messages without waiting for all AI responses. The tool runs 'git diff' to grab code changes, sends them to configured AI, and returns the AI-generated commit message. Users can set API keys or Cookies for different providers and configure options like locale, generate number of messages, commit type, proxy, timeout, max-length, and more. AICommit2 can be used both locally with Ollama and remotely with supported providers, offering flexibility and efficiency in generating commit messages.
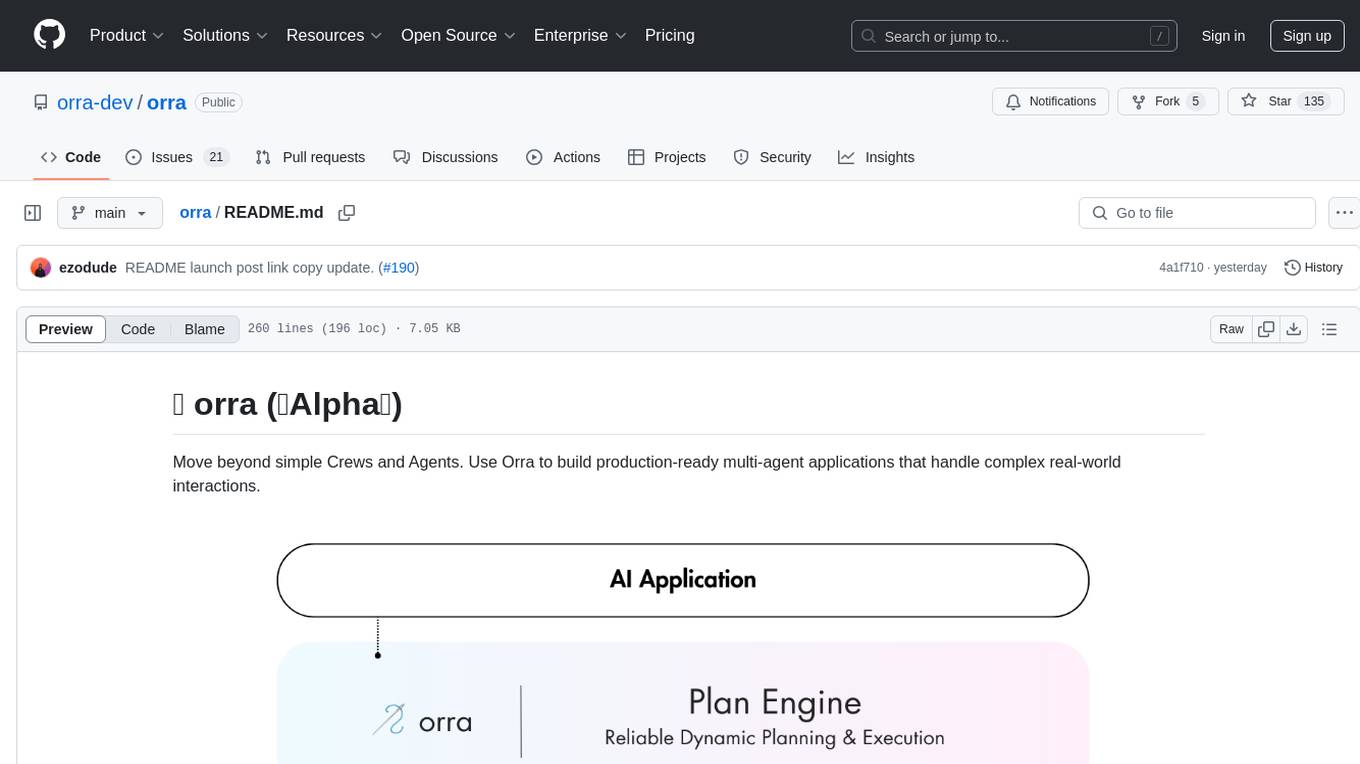
orra
Orra is a tool for building production-ready multi-agent applications that handle complex real-world interactions. It coordinates tasks across existing stack, agents, and tools run as services using intelligent reasoning. With features like smart pre-evaluated execution plans, domain grounding, durable execution, and automatic service health monitoring, Orra enables users to go fast with tools as services and revert state to handle failures. It provides real-time status tracking and webhook result delivery, making it ideal for developers looking to move beyond simple crews and agents.
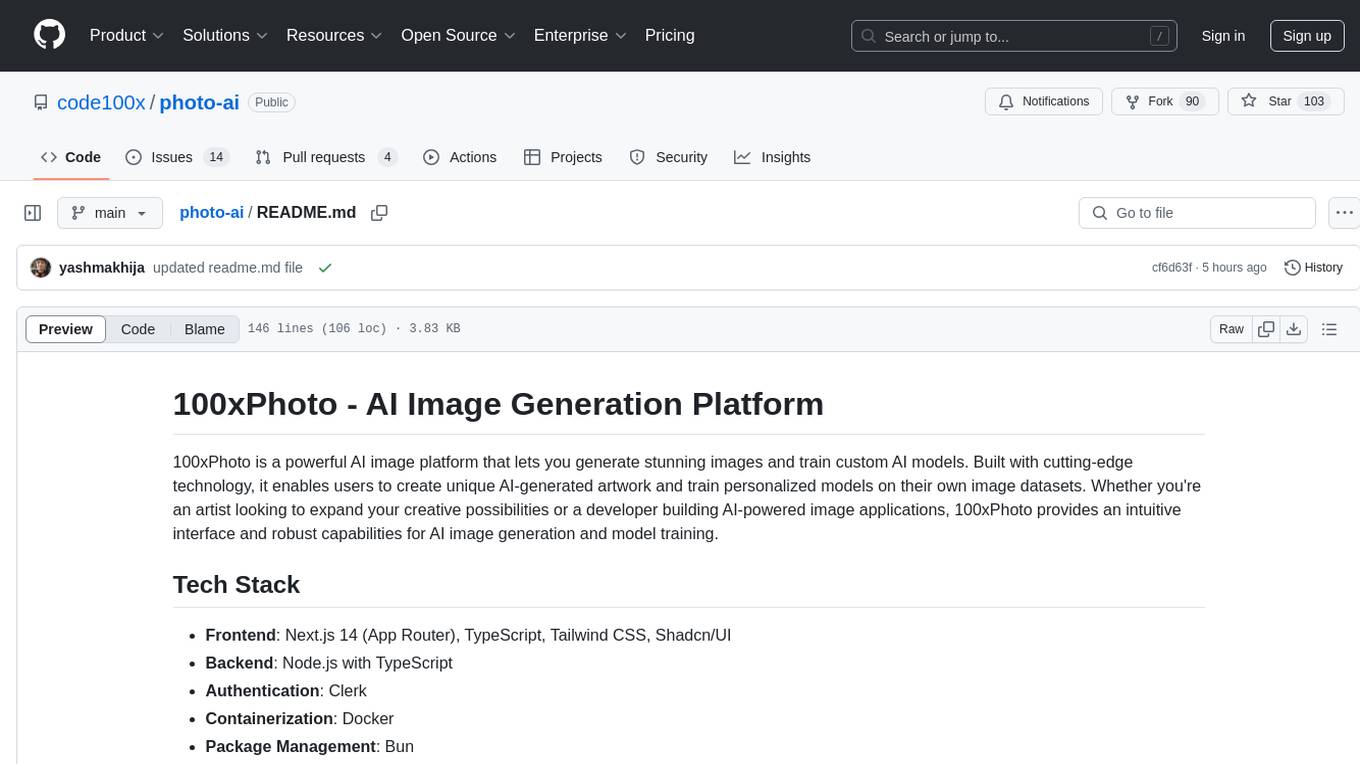
photo-ai
100xPhoto is a powerful AI image platform that enables users to generate stunning images and train custom AI models. It provides an intuitive interface for creating unique AI-generated artwork and training personalized models on image datasets. The platform is built with cutting-edge technology and offers robust capabilities for AI image generation and model training.
For similar tasks
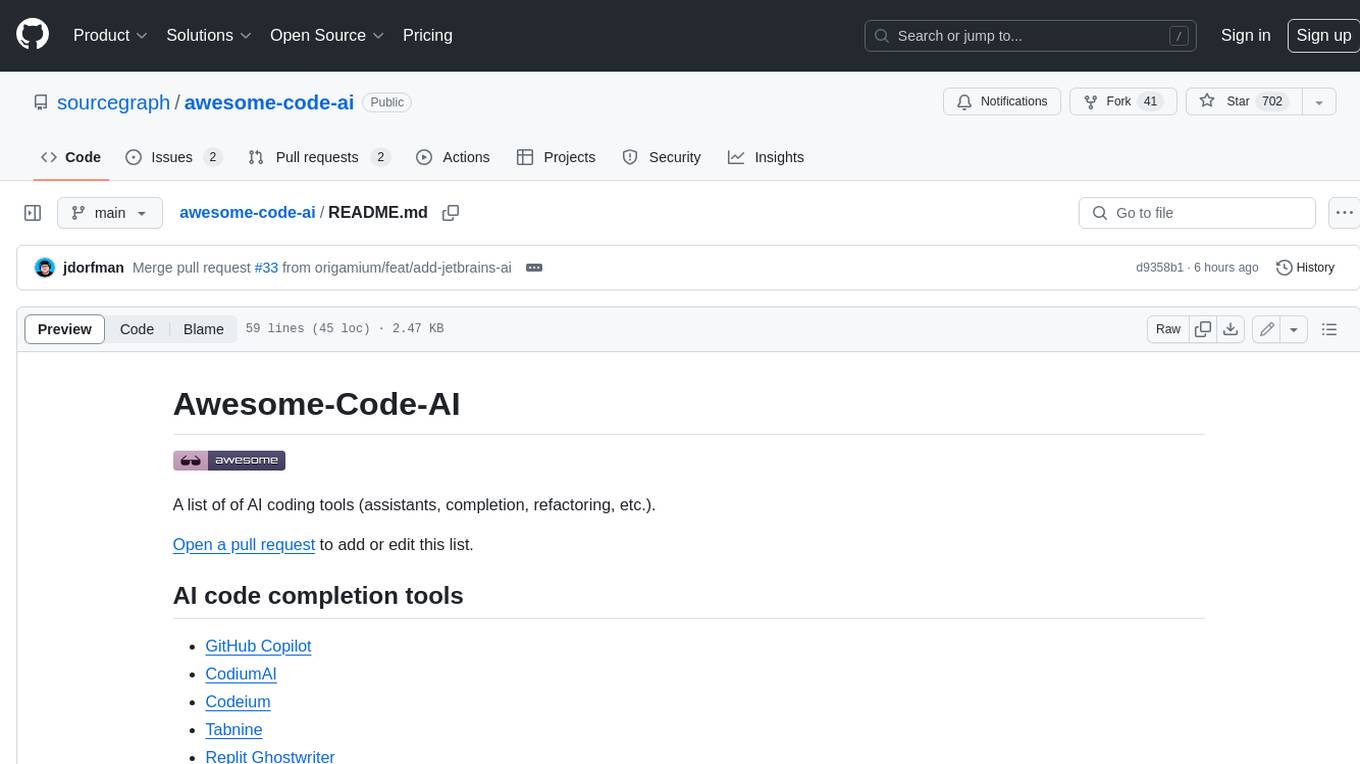
awesome-code-ai
A curated list of AI coding tools, including code completion, refactoring, and assistants. This list includes both open-source and commercial tools, as well as tools that are still in development. Some of the most popular AI coding tools include GitHub Copilot, CodiumAI, Codeium, Tabnine, and Replit Ghostwriter.
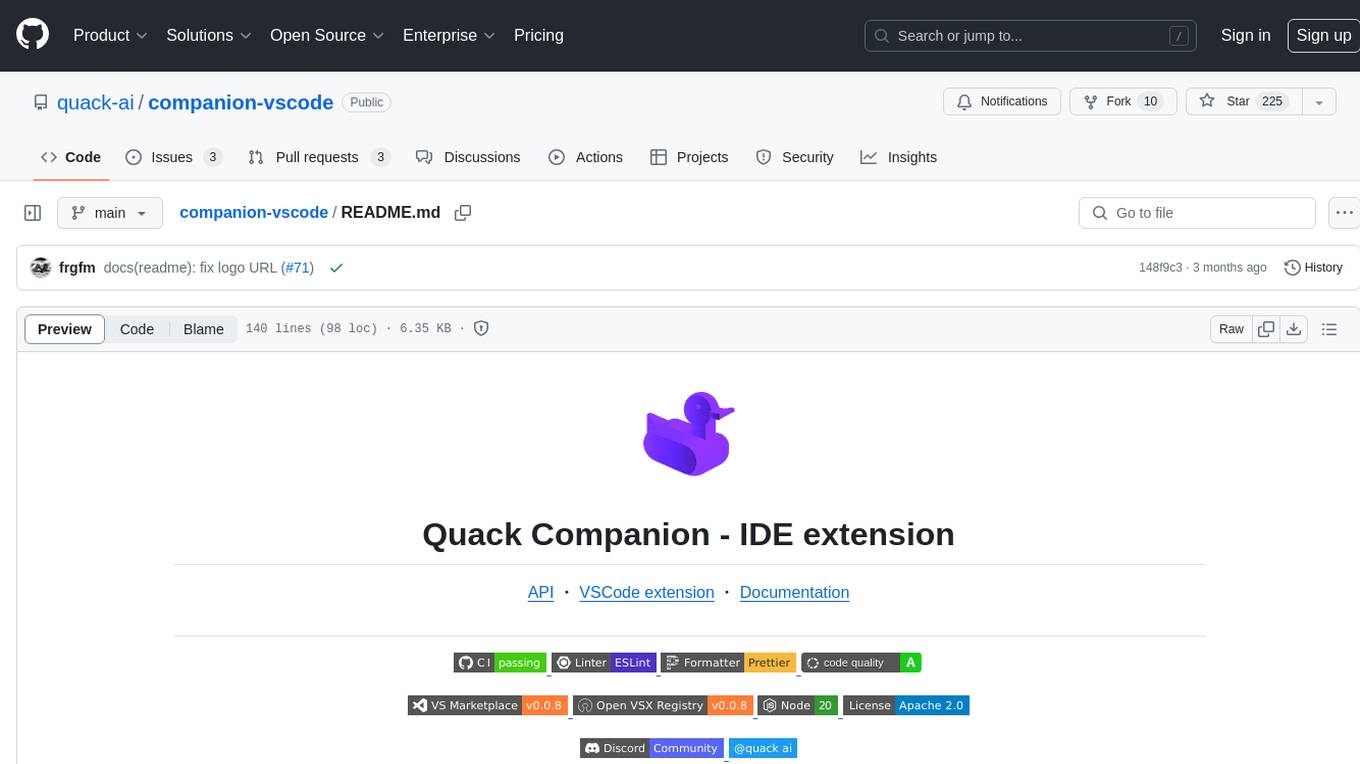
companion-vscode
Quack Companion is a VSCode extension that provides smart linting, code chat, and coding guideline curation for developers. It aims to enhance the coding experience by offering a new tab with features like curating software insights with the team, code chat similar to ChatGPT, smart linting, and upcoming code completion. The extension focuses on creating a smooth contribution experience for developers by turning contribution guidelines into a live pair coding experience, helping developers find starter contribution opportunities, and ensuring alignment between contribution goals and project priorities. Quack collects limited telemetry data to improve its services and products for developers, with options for anonymization and disabling telemetry available to users.
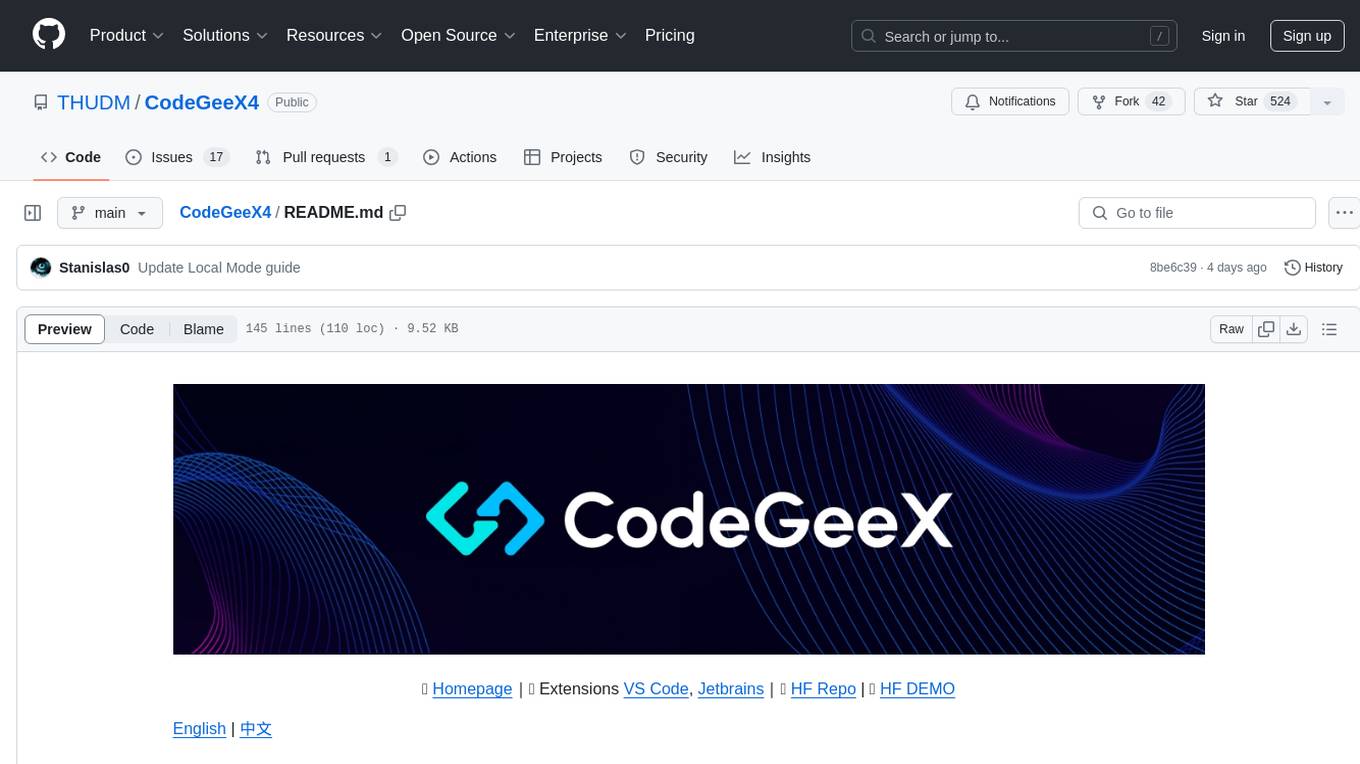
CodeGeeX4
CodeGeeX4-ALL-9B is an open-source multilingual code generation model based on GLM-4-9B, offering enhanced code generation capabilities. It supports functions like code completion, code interpreter, web search, function call, and repository-level code Q&A. The model has competitive performance on benchmarks like BigCodeBench and NaturalCodeBench, outperforming larger models in terms of speed and performance.
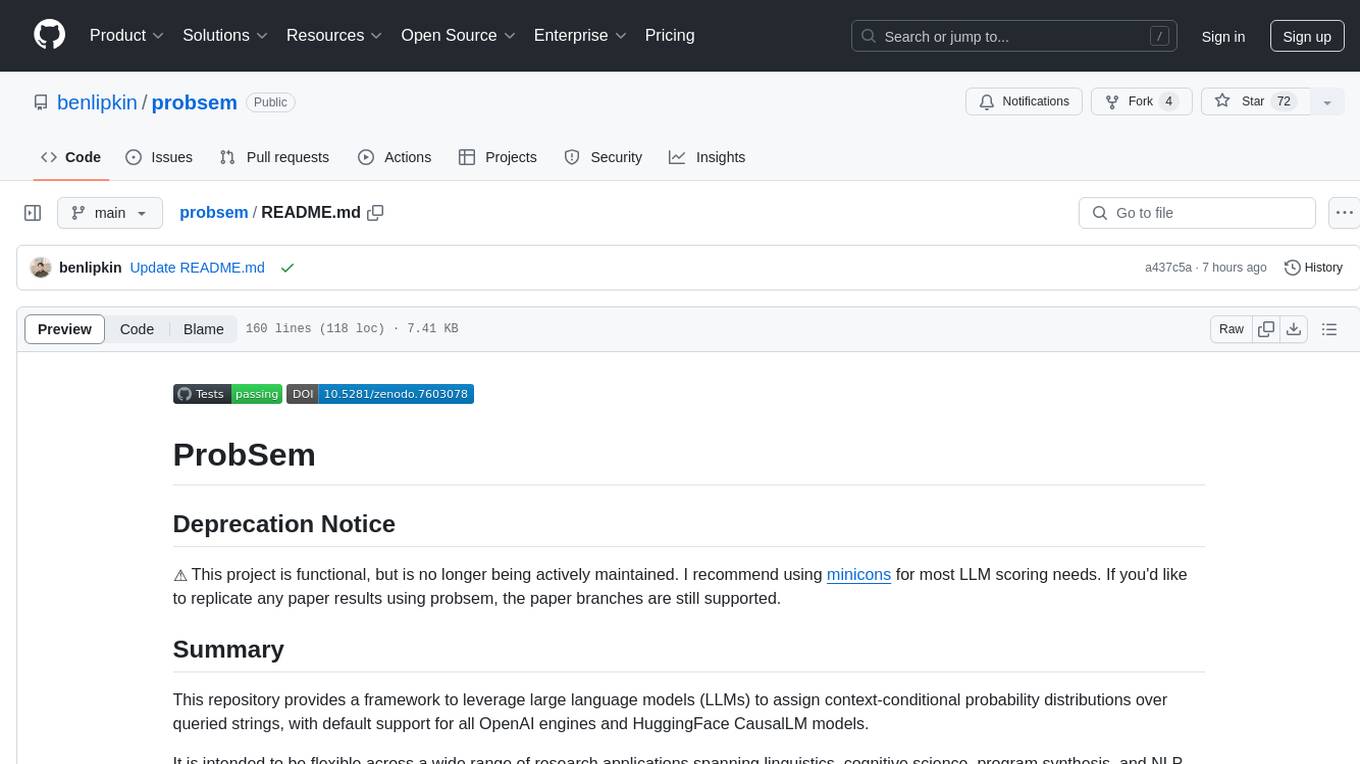
probsem
ProbSem is a repository that provides a framework to leverage large language models (LLMs) for assigning context-conditional probability distributions over queried strings. It supports OpenAI engines and HuggingFace CausalLM models, and is flexible for research applications in linguistics, cognitive science, program synthesis, and NLP. Users can define prompts, contexts, and queries to derive probability distributions over possible completions, enabling tasks like cloze completion, multiple-choice QA, semantic parsing, and code completion. The repository offers CLI and API interfaces for evaluation, with options to customize models, normalize scores, and adjust temperature for probability distributions.
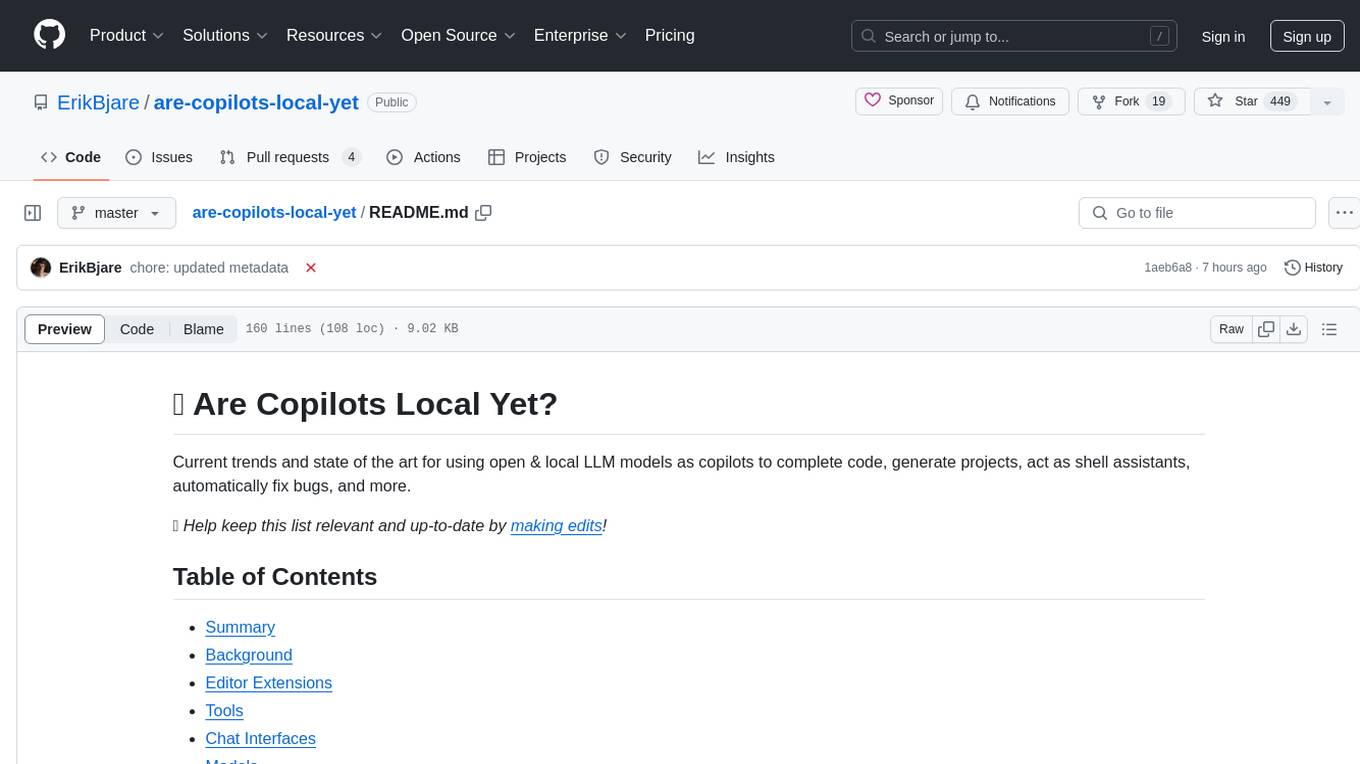
are-copilots-local-yet
Current trends and state of the art for using open & local LLM models as copilots to complete code, generate projects, act as shell assistants, automatically fix bugs, and more. This document is a curated list of local Copilots, shell assistants, and related projects, intended to be a resource for those interested in a survey of the existing tools and to help developers discover the state of the art for projects like these.
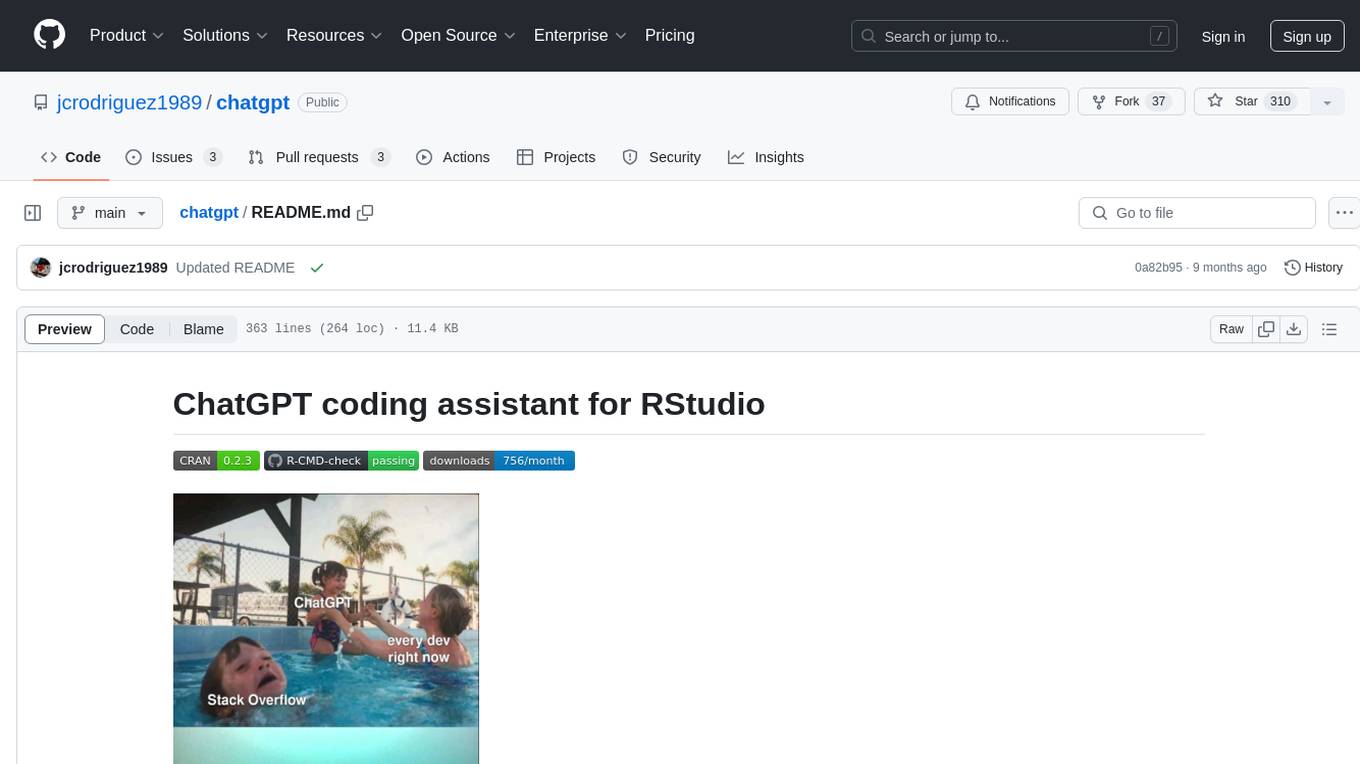
chatgpt
The ChatGPT R package provides a set of features to assist in R coding. It includes addins like Ask ChatGPT, Comment selected code, Complete selected code, Create unit tests, Create variable name, Document code, Explain selected code, Find issues in the selected code, Optimize selected code, and Refactor selected code. Users can interact with ChatGPT to get code suggestions, explanations, and optimizations. The package helps in improving coding efficiency and quality by providing AI-powered assistance within the RStudio environment.
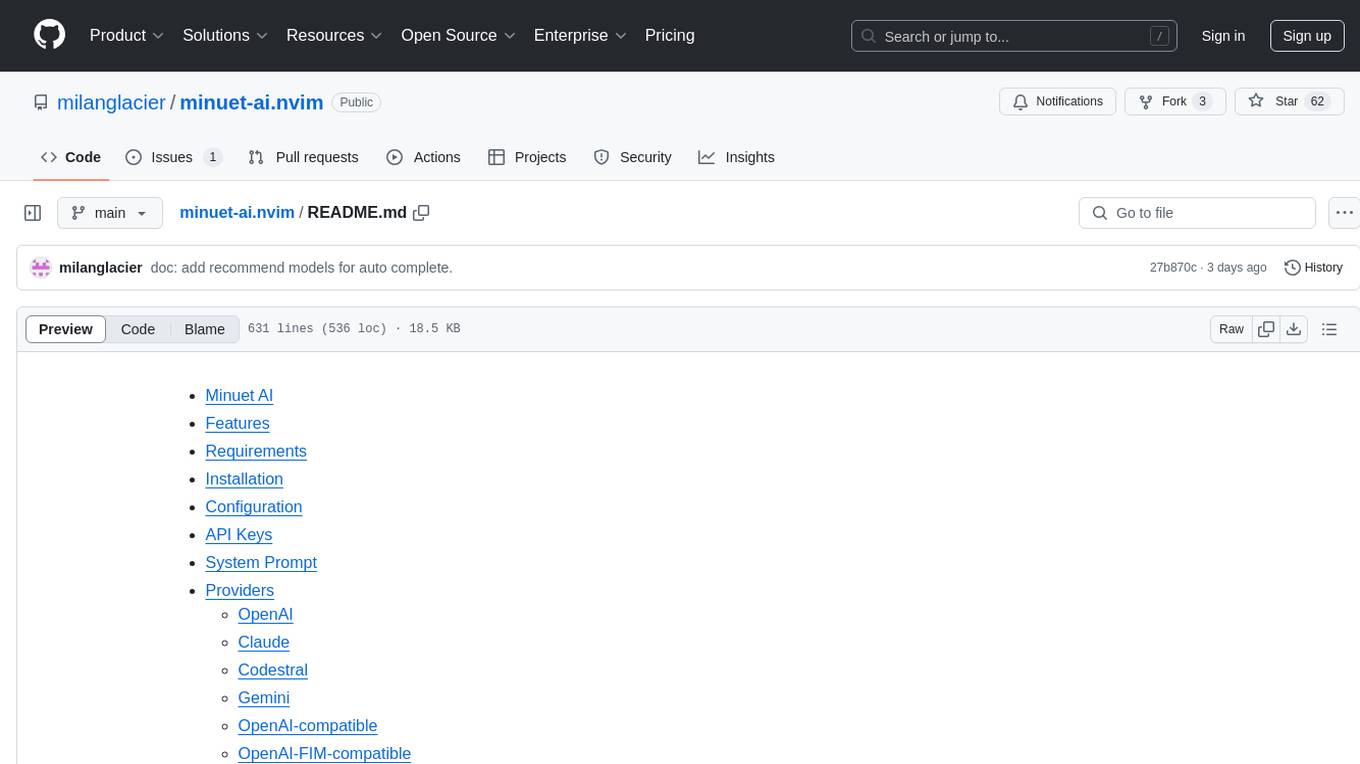
minuet-ai.nvim
Minuet AI is a Neovim plugin that integrates with nvim-cmp to provide AI-powered code completion using multiple AI providers such as OpenAI, Claude, Gemini, Codestral, and Huggingface. It offers customizable configuration options and streaming support for completion delivery. Users can manually invoke completion or use cost-effective models for auto-completion. The plugin requires API keys for supported AI providers and allows customization of system prompts. Minuet AI also supports changing providers, toggling auto-completion, and provides solutions for input delay issues. Integration with lazyvim is possible, and future plans include implementing RAG on the codebase and virtual text UI support.
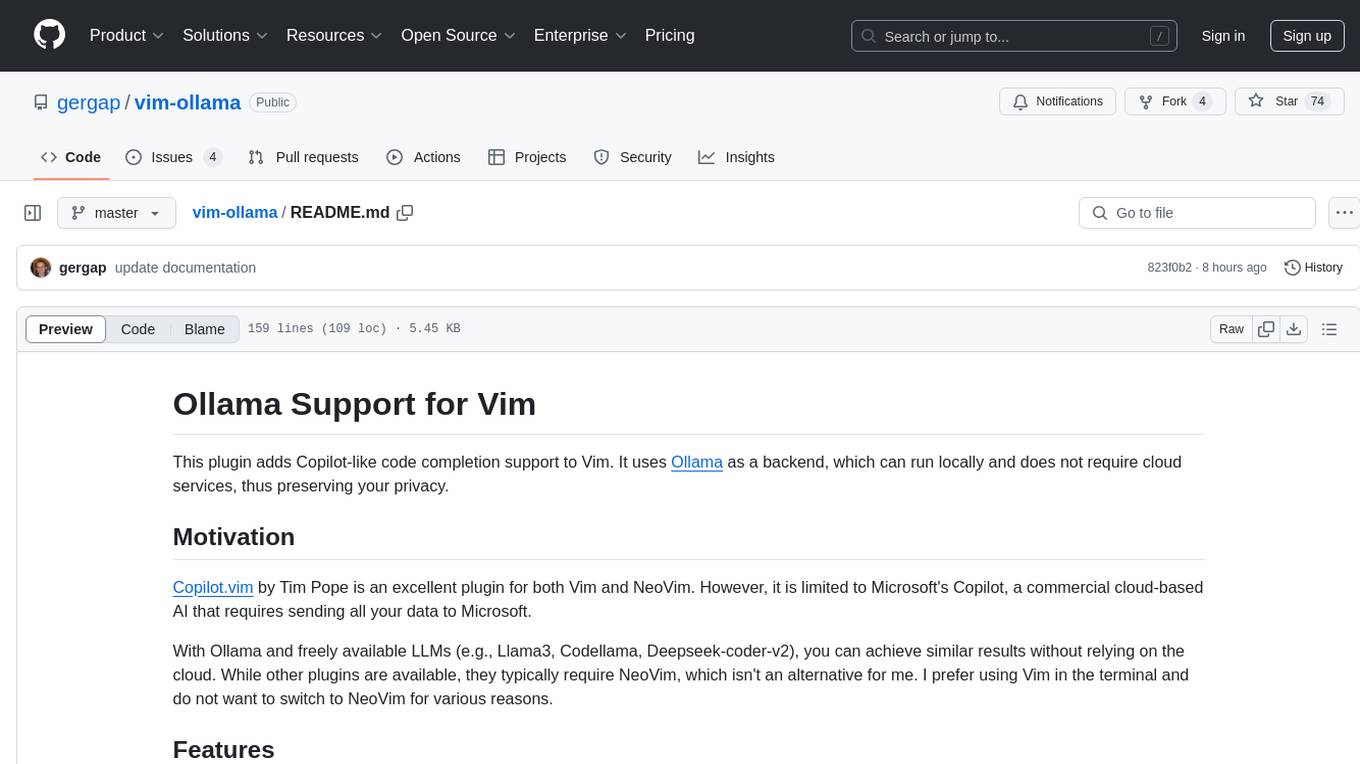
vim-ollama
The 'vim-ollama' plugin for Vim adds Copilot-like code completion support using Ollama as a backend, enabling intelligent AI-based code completion and integrated chat support for code reviews. It does not rely on cloud services, preserving user privacy. The plugin communicates with Ollama via Python scripts for code completion and interactive chat, supporting Vim only. Users can configure LLM models for code completion tasks and interactive conversations, with detailed installation and usage instructions provided in the README.
For similar jobs
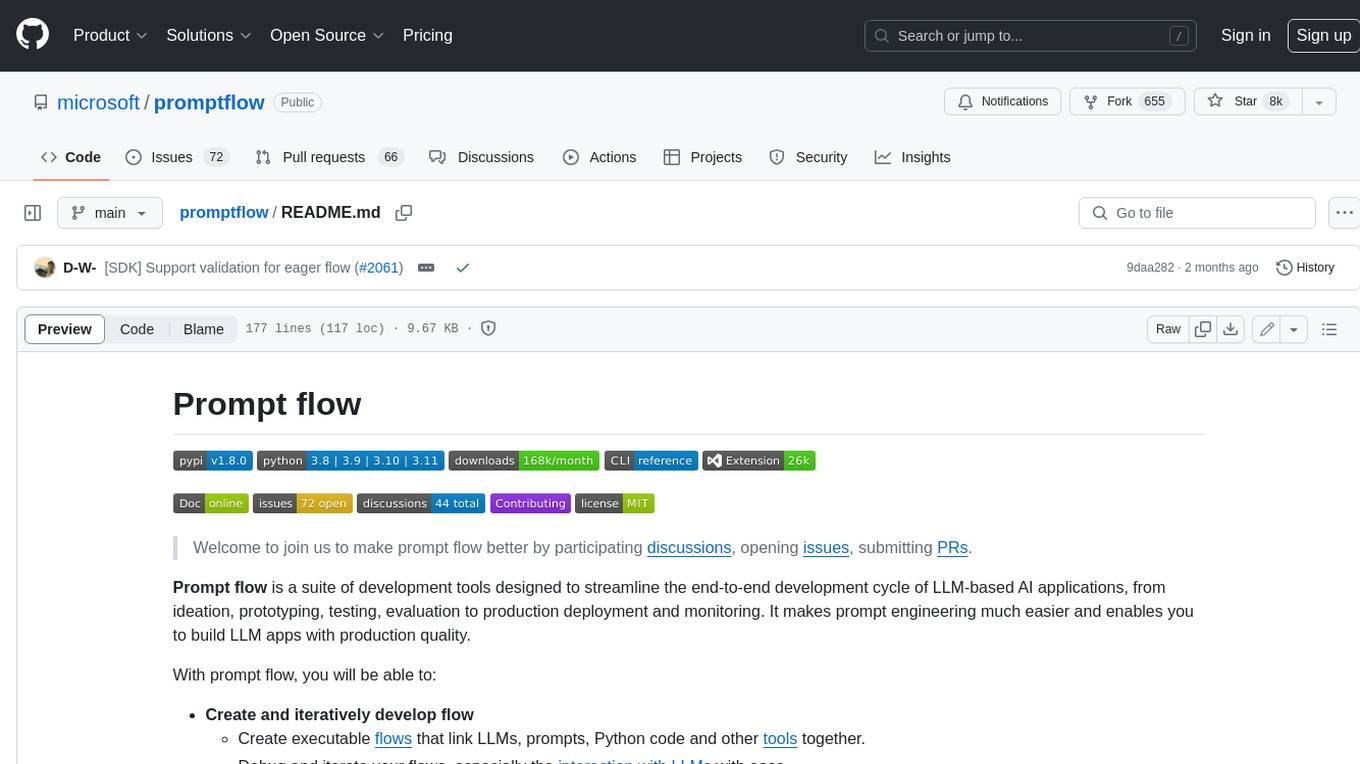
promptflow
**Prompt flow** is a suite of development tools designed to streamline the end-to-end development cycle of LLM-based AI applications, from ideation, prototyping, testing, evaluation to production deployment and monitoring. It makes prompt engineering much easier and enables you to build LLM apps with production quality.
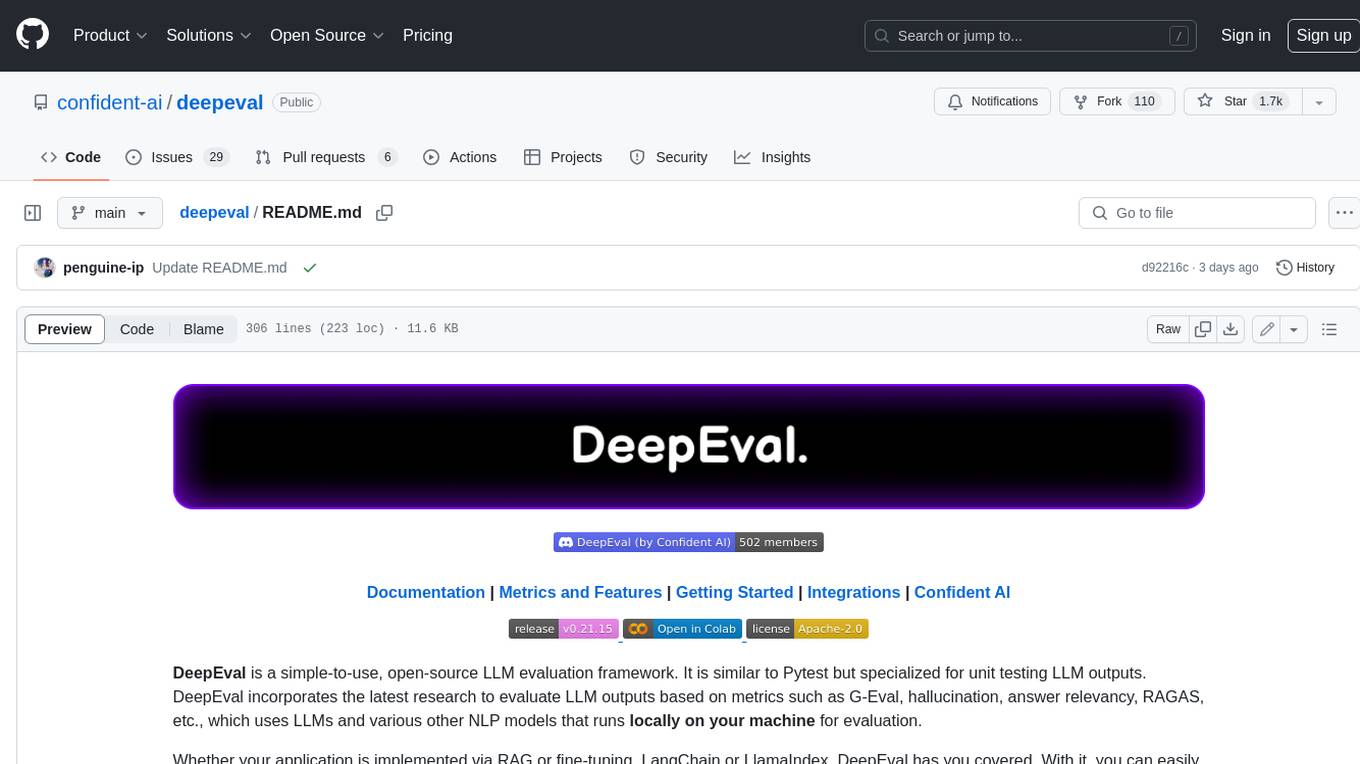
deepeval
DeepEval is a simple-to-use, open-source LLM evaluation framework specialized for unit testing LLM outputs. It incorporates various metrics such as G-Eval, hallucination, answer relevancy, RAGAS, etc., and runs locally on your machine for evaluation. It provides a wide range of ready-to-use evaluation metrics, allows for creating custom metrics, integrates with any CI/CD environment, and enables benchmarking LLMs on popular benchmarks. DeepEval is designed for evaluating RAG and fine-tuning applications, helping users optimize hyperparameters, prevent prompt drifting, and transition from OpenAI to hosting their own Llama2 with confidence.
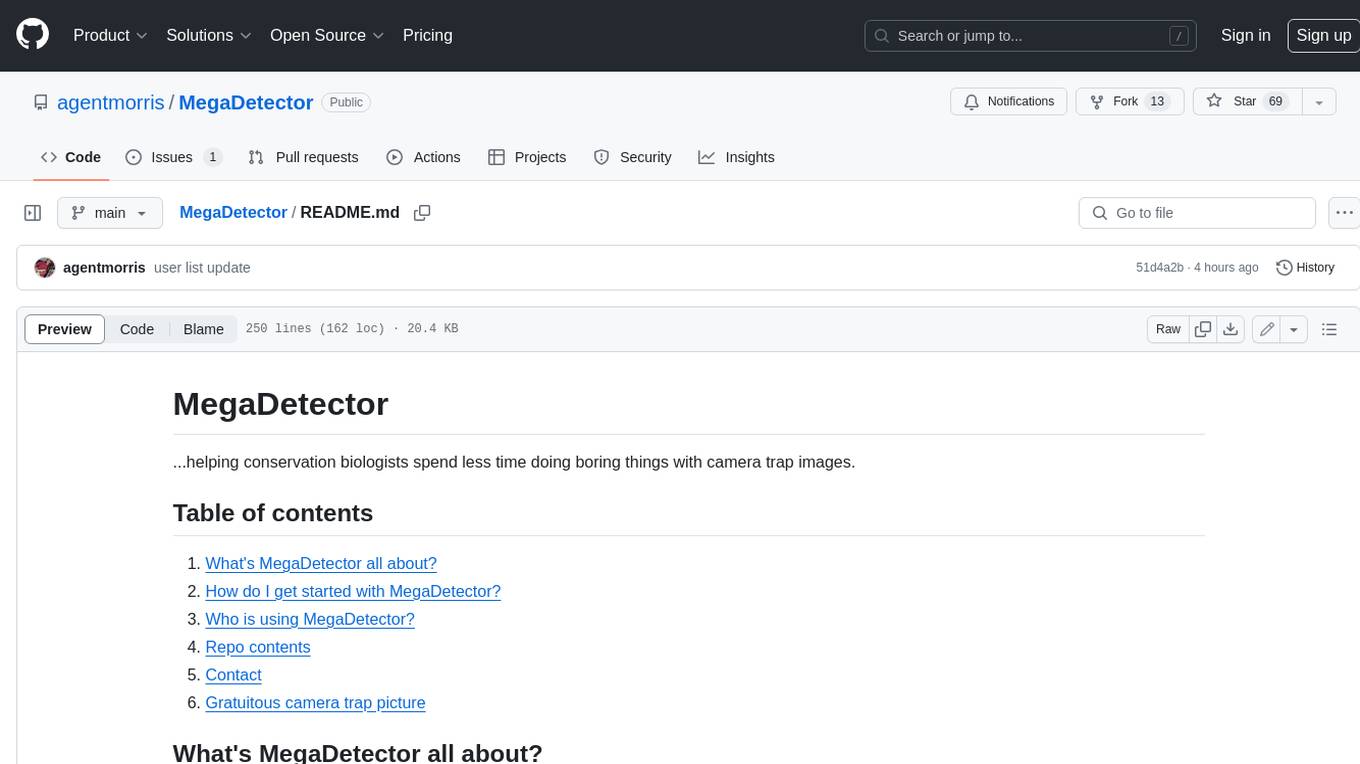
MegaDetector
MegaDetector is an AI model that identifies animals, people, and vehicles in camera trap images (which also makes it useful for eliminating blank images). This model is trained on several million images from a variety of ecosystems. MegaDetector is just one of many tools that aims to make conservation biologists more efficient with AI. If you want to learn about other ways to use AI to accelerate camera trap workflows, check out our of the field, affectionately titled "Everything I know about machine learning and camera traps".
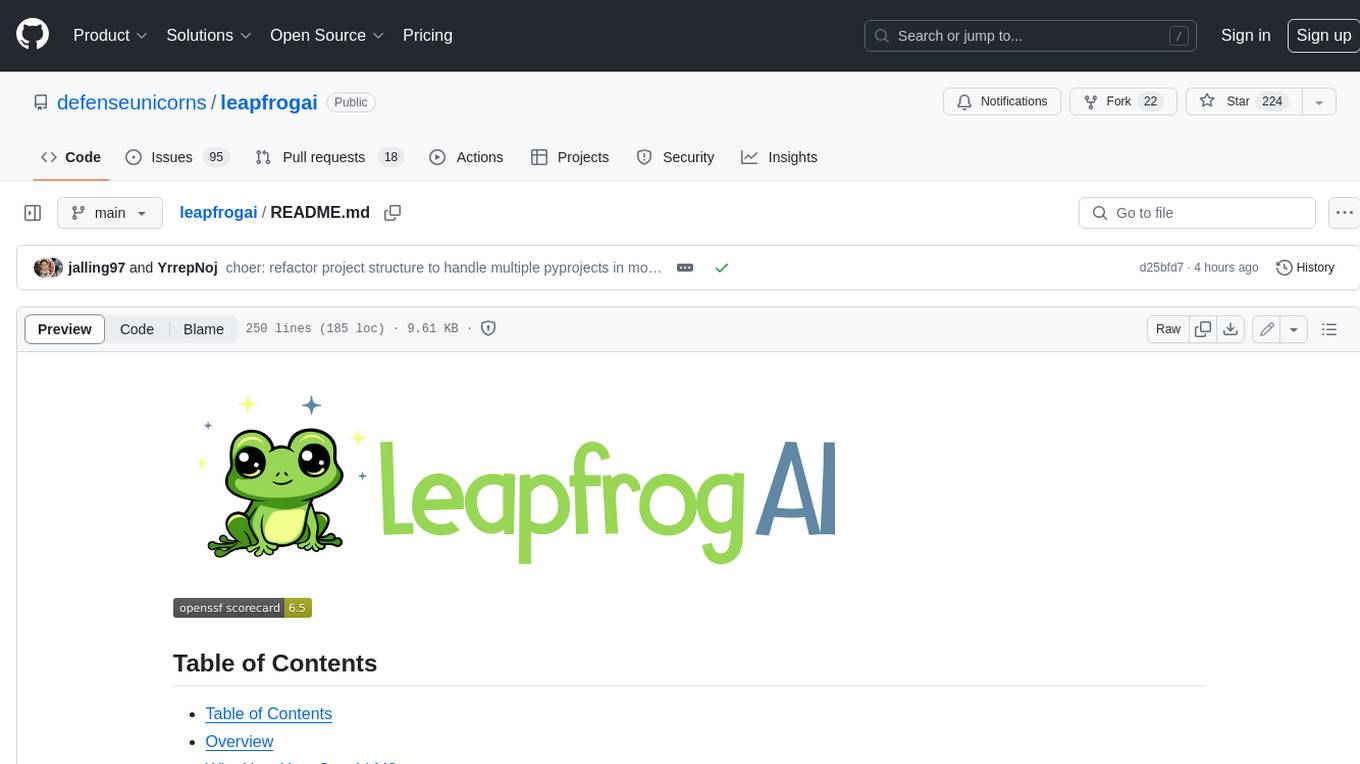
leapfrogai
LeapfrogAI is a self-hosted AI platform designed to be deployed in air-gapped resource-constrained environments. It brings sophisticated AI solutions to these environments by hosting all the necessary components of an AI stack, including vector databases, model backends, API, and UI. LeapfrogAI's API closely matches that of OpenAI, allowing tools built for OpenAI/ChatGPT to function seamlessly with a LeapfrogAI backend. It provides several backends for various use cases, including llama-cpp-python, whisper, text-embeddings, and vllm. LeapfrogAI leverages Chainguard's apko to harden base python images, ensuring the latest supported Python versions are used by the other components of the stack. The LeapfrogAI SDK provides a standard set of protobuffs and python utilities for implementing backends and gRPC. LeapfrogAI offers UI options for common use-cases like chat, summarization, and transcription. It can be deployed and run locally via UDS and Kubernetes, built out using Zarf packages. LeapfrogAI is supported by a community of users and contributors, including Defense Unicorns, Beast Code, Chainguard, Exovera, Hypergiant, Pulze, SOSi, United States Navy, United States Air Force, and United States Space Force.
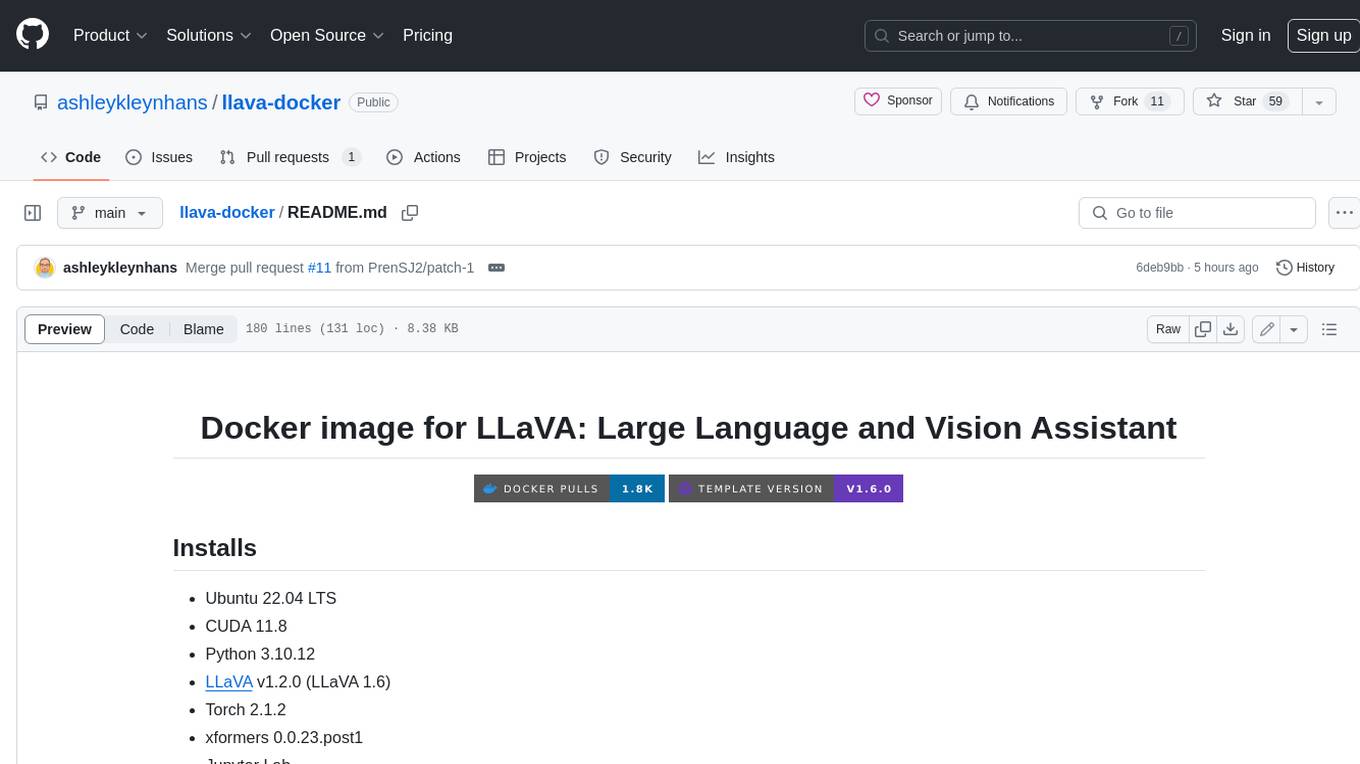
llava-docker
This Docker image for LLaVA (Large Language and Vision Assistant) provides a convenient way to run LLaVA locally or on RunPod. LLaVA is a powerful AI tool that combines natural language processing and computer vision capabilities. With this Docker image, you can easily access LLaVA's functionalities for various tasks, including image captioning, visual question answering, text summarization, and more. The image comes pre-installed with LLaVA v1.2.0, Torch 2.1.2, xformers 0.0.23.post1, and other necessary dependencies. You can customize the model used by setting the MODEL environment variable. The image also includes a Jupyter Lab environment for interactive development and exploration. Overall, this Docker image offers a comprehensive and user-friendly platform for leveraging LLaVA's capabilities.
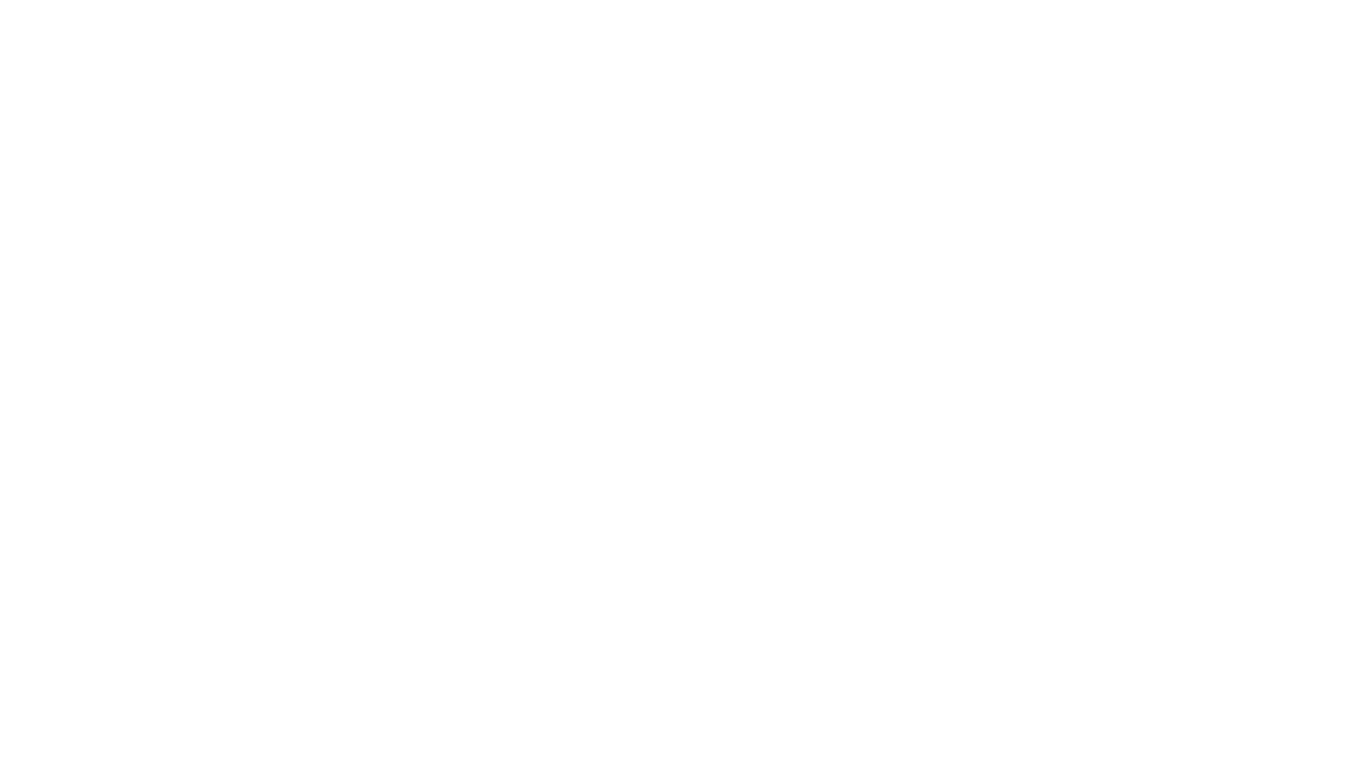
carrot
The 'carrot' repository on GitHub provides a list of free and user-friendly ChatGPT mirror sites for easy access. The repository includes sponsored sites offering various GPT models and services. Users can find and share sites, report errors, and access stable and recommended sites for ChatGPT usage. The repository also includes a detailed list of ChatGPT sites, their features, and accessibility options, making it a valuable resource for ChatGPT users seeking free and unlimited GPT services.
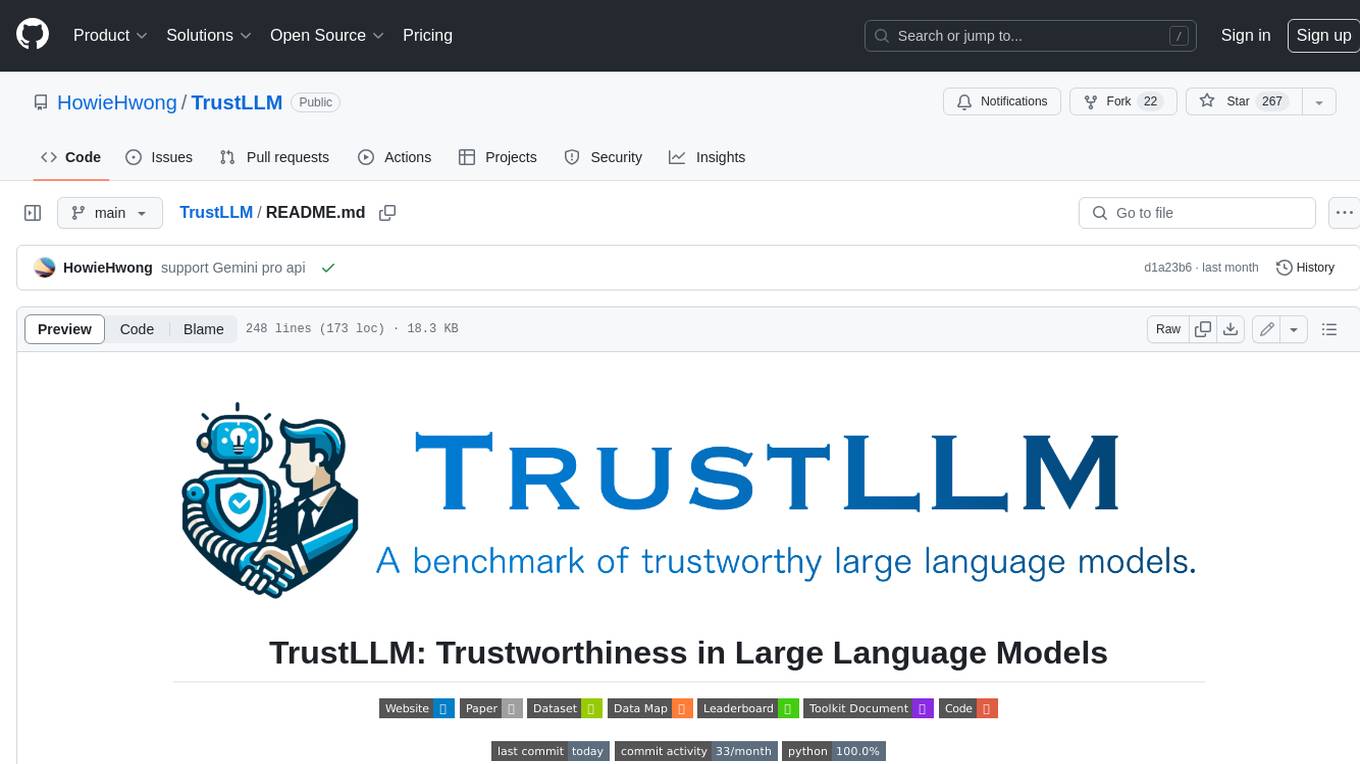
TrustLLM
TrustLLM is a comprehensive study of trustworthiness in LLMs, including principles for different dimensions of trustworthiness, established benchmark, evaluation, and analysis of trustworthiness for mainstream LLMs, and discussion of open challenges and future directions. Specifically, we first propose a set of principles for trustworthy LLMs that span eight different dimensions. Based on these principles, we further establish a benchmark across six dimensions including truthfulness, safety, fairness, robustness, privacy, and machine ethics. We then present a study evaluating 16 mainstream LLMs in TrustLLM, consisting of over 30 datasets. The document explains how to use the trustllm python package to help you assess the performance of your LLM in trustworthiness more quickly. For more details about TrustLLM, please refer to project website.
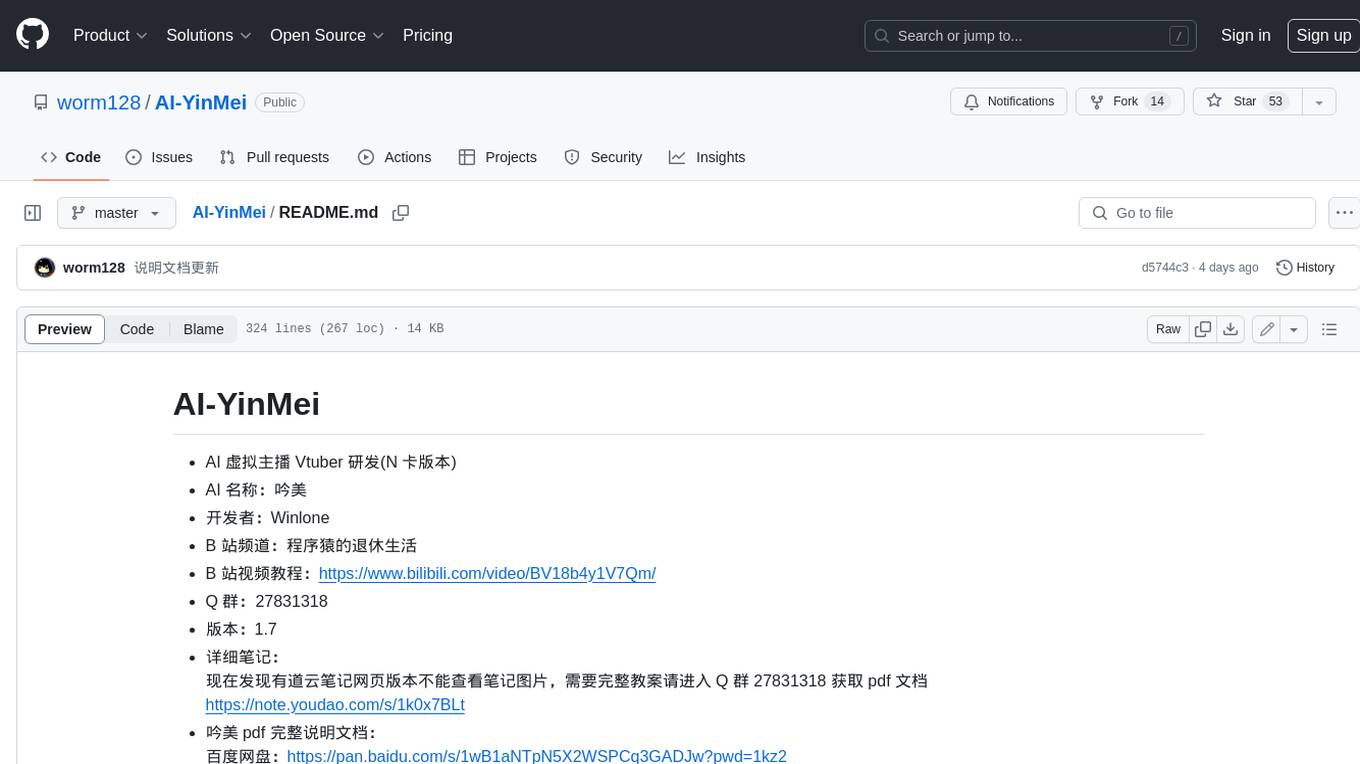
AI-YinMei
AI-YinMei is an AI virtual anchor Vtuber development tool (N card version). It supports fastgpt knowledge base chat dialogue, a complete set of solutions for LLM large language models: [fastgpt] + [one-api] + [Xinference], supports docking bilibili live broadcast barrage reply and entering live broadcast welcome speech, supports Microsoft edge-tts speech synthesis, supports Bert-VITS2 speech synthesis, supports GPT-SoVITS speech synthesis, supports expression control Vtuber Studio, supports painting stable-diffusion-webui output OBS live broadcast room, supports painting picture pornography public-NSFW-y-distinguish, supports search and image search service duckduckgo (requires magic Internet access), supports image search service Baidu image search (no magic Internet access), supports AI reply chat box [html plug-in], supports AI singing Auto-Convert-Music, supports playlist [html plug-in], supports dancing function, supports expression video playback, supports head touching action, supports gift smashing action, supports singing automatic start dancing function, chat and singing automatic cycle swing action, supports multi scene switching, background music switching, day and night automatic switching scene, supports open singing and painting, let AI automatically judge the content.