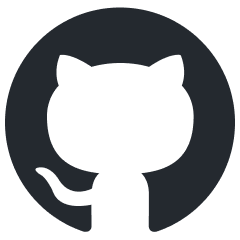
UnrealGenAISupport
UnrealMCP is here!! Automatic blueprint and scene generation from AI!! An Unreal Engine plugin for LLM/GenAI models & MCP UE5 server. Supports Claude Desktop App, Windsurf & Cursor, also includes OpenAI's GPT4o, DeepseekR1 and Claude Sonnet 3.7 APIs with plans to add Gemini, Grok 3, audio & realtime APIs soon.
Stars: 106
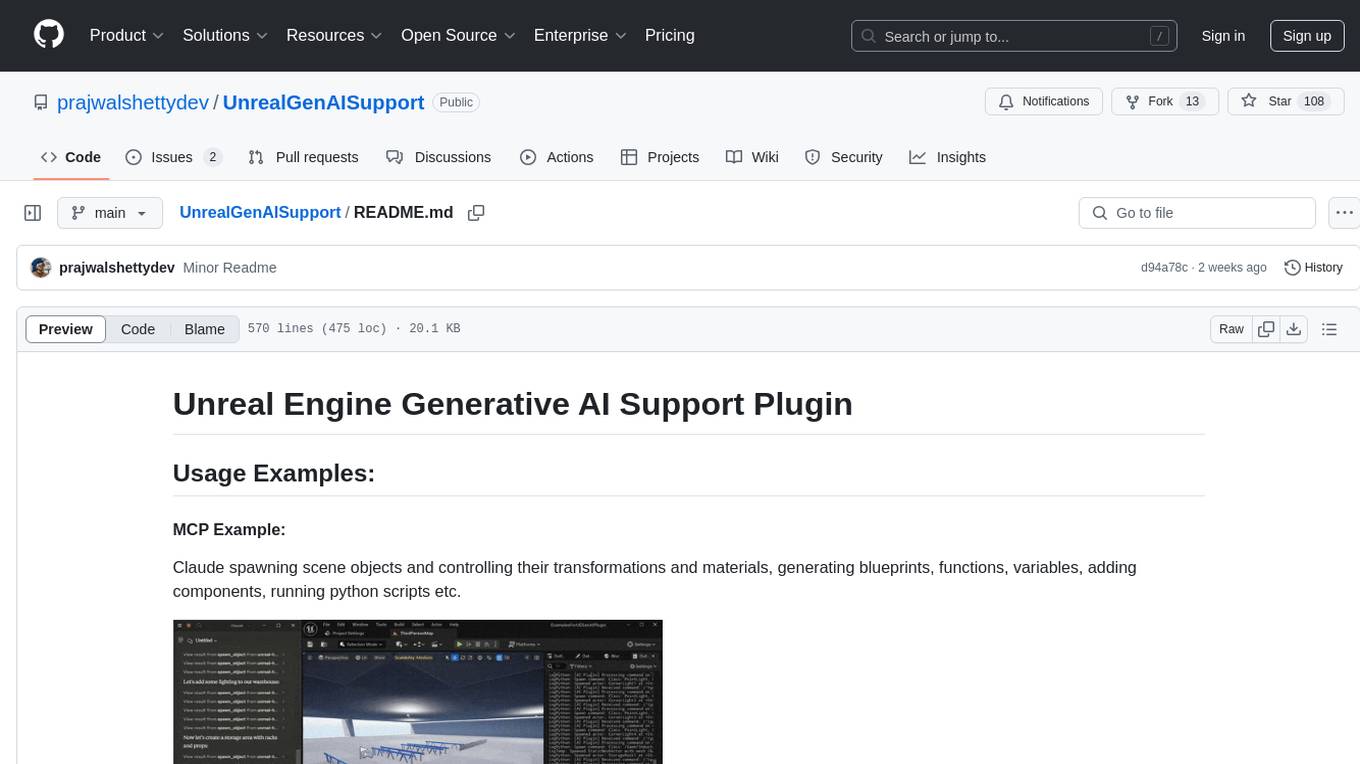
README:
Claude spawning scene objects and controlling their transformations and materials, generating blueprints, functions, variables, adding components, running python scripts etc.
A project called become human, where NPCs are OpenAI agentic instances. Built using this plugin.
[!WARNING]
This plugin is still under rapid development.
- Do not use it in production environments.
⚠️ - Do not use it without version control.
⚠️ A stable version will be released soon. 🚀🔥
Every month, hundreds of new AI models are released by various organizations, making it hard to keep up with the latest advancements.
The Unreal Engine Generative AI Support Plugin allows you to focus on game development without worrying about the LLM/GenAI integration layer.
Currently integrating Model Control Protocol (MCP) with Unreal Engine 5.5.
This project aims to build a long-term support (LTS) plugin for various cutting-edge LLM/GenAI models and foster a community around it. It currently includes OpenAI's GPT-4o, Deepseek R1, Claude Sonnet 3.7 and GPT-4o-mini for Unreal Engine 5.1 or higher, with plans to add , real-time APIs, Gemini, MCP, and Grok 3 APIs soon. The plugin will focus exclusively on APIs useful for game development, evals and interactive experiences. All suggestions and contributions are welcome. The plugin can also be used for setting up new evals and ways to compare models in game battlefields.
- OpenAI API Support:
- OpenAI Chat API ✅
(models-ref)
-
gpt-4o
,gpt-4o-mini
Model ✅ -
gpt-4.5-preview
Model 🛠️ -
o1-mini
,o1
,o1-pro
Model 🚧 -
o3-mini
Model 🛠️
-
- OpenAI DALL-E API ❌ (Until new generation models are released)
- OpenAI Vision API 🚧
- OpenAI Realtime API 🛠️
-
gpt-4o-realtime-preview
gpt-4o-mini-realtime-preview
Model 🛠️
-
- OpenAI Structured Outputs ✅
- OpenAI Whisper API 🚧
- OpenAI Chat API ✅
(models-ref)
- Anthropic Claude API Support:
- Claude Chat API ✅
-
claude-3-7-sonnet-latest
Model ✅ -
claude-3-5-sonnet
Model ✅ -
claude-3-5-haiku-latest
Model ✅ -
claude-3-opus-latest
Model ✅
-
- Claude Vision API 🚧
- Claude Chat API ✅
- XAI (Grok 3) API Support:
- XAI Chat Completions API 🚧
-
grok-beta
Model 🚧 -
grok-beta
Streaming API 🚧
-
- XAI Image API 🚧
- XAI Chat Completions API 🚧
- Google Gemini API Support:
- Gemini Chat API 🚧🤝
-
gemini-2.0-flash-lite
,gemini-2.0-flash
gemini-1.5-flash
Model 🚧🤝
-
- Gemini Imagen API: 🚧
-
imagen-3.0-generate-002
Model 🚧
-
- Gemini Chat API 🚧🤝
- Meta AI API Support:
- Llama Chat API ❌ (Until new generation models are released)
-
llama3.3-70b
Model ❌ -
llama3.1-8b
Model ❌
-
- Local Llama API 🚧🤝
- Llama Chat API ❌ (Until new generation models are released)
- Deepseek API Support:
- Deepseek Chat API ✅
-
deepseek-chat
(DeepSeek-V3) Model ✅
-
- Deepseek Reasoning API, R1 ✅
-
deepseek-reasoning-r1
Model ✅ -
deepseek-reasoning-r1
CoT Streaming ❌
-
- Independently Hosted Deepseek Models 🚧
- Deepseek Chat API ✅
- Baidu API Support:
- Baidu Chat API 🚧
-
baidu-chat
Model 🚧
-
- Baidu Chat API 🚧
- 3D generative model APIs:
- TripoSR by StabilityAI 🚧
- Plugin Documentation 🛠️🤝
- Plugin Example Project 🛠️ here
- Version Control Support
- Perforce Support 🚧
- Git Submodule Support ✅
- LTS Branching 🚧
- Stable Branch with Bug Fixes 🚧
- Dedicated Contributor for LTS 🚧
- Lightweight Plugin (In Builds)
- No External Dependencies ✅
- Build Flags to enable/disable APIs 🚧
- Submodules per API Organization 🚧
- Exclude MCP from build 🚧
- Testing
- Automated Testing 🚧
- Different Platforms 🚧🤝
- Different Engine Versions 🚧🤝
- Clients Support ✅
- Claude Desktop App Support ✅
- Cursor IDE Support ✅
- OpenAI Operator API Support 🚧
- Blueprints Auto Generation 🛠️
- Creating new blueprint of types ✅
- Adding new functions, function/blueprint variables ✅
- Adding nodes and connections 🛠️ (buggy)
- Advanced Blueprints Generation 🛠️
- Level/Scene Control for LLMs 🛠️
- Spawning Objects and Shapes ✅
- Moving, rotating and scaling objects ✅
- Changing materials and color ✅
- Advanced scene features 🛠️
- Generative AI:
- Prompt to 3D model fetch and spawn 🛠️
- Control:
- Ability to run Python scripts ✅
- Ability to run Console Commands ✅
- UI:
- Widgets generation 🛠️
- UI Blueprint generation 🛠️
- Project Files:
- Create/Edit project files/folders ️✅
- Delete existing project files ❌
- Others:
- Project Cleanup 🛠️
Where,
- ✅ - Completed
- 🛠️ - In Progress
- 🚧 - Planned
- 🤝 - Need Contributors
- ❌ - Won't Support For Now
- Setting API Keys
- Setting up MCP
- Adding the plugin to your project
- Fetching the Latest Plugin Changes
- Usage
- Known Issues
- Contribution Guidelines
- References
[!NOTE]
There is no need to set the API key for testing the MCP features in Claude app. Anthropic key only needed for Claude API.
Set the environment variable PS_<ORGNAME>
to your API key.
setx PS_<ORGNAME> "your api key"
-
Run the following command in your terminal, replacing yourkey with your API key.
echo "export PS_<ORGNAME>='yourkey'" >> ~/.zshrc
-
Update the shell with the new variable:
source ~/.zshrc
PS: Don't forget to restart the Editor and ALSO the connected IDE after setting the environment variable.
Where <ORGNAME>
can be:
PS_OPENAIAPIKEY
, PS_DEEPSEEKAPIKEY
, PS_ANTHROPICAPIKEY
, PS_METAAPIKEY
, PS_GOOGLEAPIKEY
etc.
Storing API keys in packaged builds is a security risk. This is what the OpenAI API documentation says about it:
"Exposing your OpenAI API key in client-side environments like browsers or mobile apps allows malicious users to take that key and make requests on your behalf – which may lead to unexpected charges or compromise of certain account data. Requests should always be routed through your own backend server where you can keep your API key secure."
Read more about it here.
For test builds you can call the GenSecureKey::SetGenAIApiKeyRuntime
either in c++ or blueprints function with your API key in the packaged build.
[!NOTE]
If your project only uses the LLM APIs and not the MCP, you can skip this section.
[!CAUTION]
Discalimer: If you are using the MCP feature of the plugin, it will directly let the Claude Desktop App control your Unreal Engine project. Make sure you are aware of the security risks and only use it in a controlled environment.Please backup your project before using the MCP feature and use version control to track changes.
claude_desktop_config.json
file in Claude Desktop App's installation directory. (might ask claude where its located for your platform!)
The file will look something like this:
{
"mcpServers": {
"unreal-handshake": {
"command": "python",
"args": ["<your_project_directoy_path>/Plugins/GenerativeAISupport/Content/Python/mcp_server.py"],
"env": {
"UNREAL_HOST": "localhost",
"UNREAL_PORT": "9877"
}
}
}
}
.cursor/mcp.json
file in your project directory. The file will look something like this:
{
"mcpServers": {
"unreal-handshake": {
"command": "python",
"args": ["<your_project_directoy_path>/Plugins/GenerativeAISupport/Content/Python/mcp_server.py"],
"env": {
"UNREAL_HOST": "localhost",
"UNREAL_PORT": "9877"
}
}
}
}
pip install mcp[cli]
-
Add the Plugin Repository as a Submodule in your project's repository.
git submodule add https://github.com/prajwalshettydev/UnrealGenAISupport Plugins/GenerativeAISupport
-
Regenerate Project Files: Right-click your .uproject file and select Generate Visual Studio project files.
-
Enable the Plugin in Unreal Editor: Open your project in Unreal Editor. Go to Edit > Plugins. Search for the Plugin in the list and enable it.
-
For Unreal C++ Projects, include the Plugin's module in your project's Build.cs file:
PrivateDependencyModuleNames.AddRange(new string[] { "GenerativeAISupport" });
Still in development..
Coming soon, for free, in the Unreal Engine Marketplace.
you can pull the latest changes with:
cd Plugins/GenerativeAISupport
git pull origin main
Or update all submodules in the project:
git submodule update --recursive --remote
Still in development..
There is a example Unreal project that already implements the plugin. You can find it here.
Currently the plugin supports Chat and Structured Outputs from OpenAI API. Both for C++ and Blueprints.
Tested models are gpt-4o
, gpt-4o-mini
, gpt-4.5
, o1-mini
, o1
, o3-mini-high
.
void SomeDebugSubsystem::CallGPT(const FString& Prompt,
const TFunction<void(const FString&, const FString&, bool)>& Callback)
{
FGenChatSettings ChatSettings;
ChatSettings.Model = TEXT("gpt-4o-mini");
ChatSettings.MaxTokens = 500;
ChatSettings.Messages.Add(FGenChatMessage{ TEXT("system"), Prompt });
FOnChatCompletionResponse OnComplete = FOnChatCompletionResponse::CreateLambda(
[Callback](const FString& Response, const FString& ErrorMessage, bool bSuccess)
{
Callback(Response, ErrorMessage, bSuccess);
});
UGenOAIChat::SendChatRequest(ChatSettings, OnComplete);
}
Sending a custom schema json directly to function call
FString MySchemaJson = R"({
"type": "object",
"properties": {
"count": {
"type": "integer",
"description": "The total number of users."
},
"users": {
"type": "array",
"items": {
"type": "object",
"properties": {
"name": { "type": "string", "description": "The user's name." },
"heading_to": { "type": "string", "description": "The user's destination." }
},
"required": ["name", "role", "age", "heading_to"]
}
}
},
"required": ["count", "users"]
})";
UGenAISchemaService::RequestStructuredOutput(
TEXT("Generate a list of users and their details"),
MySchemaJson,
[](const FString& Response, const FString& Error, bool Success) {
if (Success)
{
UE_LOG(LogTemp, Log, TEXT("Structured Output: %s"), *Response);
}
else
{
UE_LOG(LogTemp, Error, TEXT("Error: %s"), *Error);
}
}
);
Sending a custom schema json from a file
#include "Misc/FileHelper.h"
#include "Misc/Paths.h"
FString SchemaFilePath = FPaths::Combine(
FPaths::ProjectDir(),
TEXT("Source/:ProjectName/Public/AIPrompts/SomeSchema.json")
);
FString MySchemaJson;
if (FFileHelper::LoadFileToString(MySchemaJson, *SchemaFilePath))
{
UGenAISchemaService::RequestStructuredOutput(
TEXT("Generate a list of users and their details"),
MySchemaJson,
[](const FString& Response, const FString& Error, bool Success) {
if (Success)
{
UE_LOG(LogTemp, Log, TEXT("Structured Output: %s"), *Response);
}
else
{
UE_LOG(LogTemp, Error, TEXT("Error: %s"), *Error);
}
}
);
}
Currently the plugin supports Chat and Reasoning from DeepSeek API. Both for C++ and Blueprints. Points to note:
- System messages are currently mandatory for the reasoning model. API otherwise seems to return null
- Also, from the documentation: "Please note that if the reasoning_content field is included in the sequence of input messages, the API will return a 400 error. Read more about it here"
[!WARNING]
While using the R1 reasoning model, make sure the Unreal's HTTP timeouts are not the default values at 30 seconds. As these API calls can take longer than 30 seconds to respond. Simply setting theHttpRequest->SetTimeout(<N Seconds>);
is not enough So the following lines need to be added to your project'sDefaultEngine.ini
file:[HTTP] HttpConnectionTimeout=180 HttpReceiveTimeout=180
FGenDSeekChatSettings ReasoningSettings;
ReasoningSettings.Model = EDeepSeekModels::Reasoner; // or EDeepSeekModels::Chat for Chat API
ReasoningSettings.MaxTokens = 100;
ReasoningSettings.Messages.Add(FGenChatMessage{TEXT("system"), TEXT("You are a helpful assistant.")});
ReasoningSettings.Messages.Add(FGenChatMessage{TEXT("user"), TEXT("9.11 and 9.8, which is greater?")});
ReasoningSettings.bStreamResponse = false;
UGenDSeekChat::SendChatRequest(
ReasoningSettings,
FOnDSeekChatCompletionResponse::CreateLambda(
[this](const FString& Response, const FString& ErrorMessage, bool bSuccess)
{
if (!UTHelper::IsContextStillValid(this))
{
return;
}
// Log response details regardless of success
UE_LOG(LogTemp, Warning, TEXT("DeepSeek Reasoning Response Received - Success: %d"), bSuccess);
UE_LOG(LogTemp, Warning, TEXT("Response: %s"), *Response);
if (!ErrorMessage.IsEmpty())
{
UE_LOG(LogTemp, Error, TEXT("Error Message: %s"), *ErrorMessage);
}
})
);
Currently the plugin supports Chat from Anthropic API. Both for C++ and Blueprints.
Tested models are claude-3-7-sonnet-latest
, claude-3-5-sonnet
, claude-3-5-haiku-latest
, claude-3-opus-latest
.
// ---- Claude Chat Test ----
FGenClaudeChatSettings ChatSettings;
ChatSettings.Model = EClaudeModels::Claude_3_7_Sonnet; // Use Claude 3.7 Sonnet model
ChatSettings.MaxTokens = 4096;
ChatSettings.Temperature = 0.7f;
ChatSettings.Messages.Add(FGenChatMessage{TEXT("system"), TEXT("You are a helpful assistant.")});
ChatSettings.Messages.Add(FGenChatMessage{TEXT("user"), TEXT("What is the capital of France?")});
UGenClaudeChat::SendChatRequest(
ChatSettings,
FOnClaudeChatCompletionResponse::CreateLambda(
[this](const FString& Response, const FString& ErrorMessage, bool bSuccess)
{
if (!UTHelper::IsContextStillValid(this))
{
return;
}
if (bSuccess)
{
UE_LOG(LogTemp, Warning, TEXT("Claude Chat Response: %s"), *Response);
}
else
{
UE_LOG(LogTemp, Error, TEXT("Claude Chat Error: %s"), *ErrorMessage);
}
})
);
This is currently work in progress. The plugin supports various clients like Claude Desktop App, Cursor etc.
Running the MCP server:
python <your_project_directoy>/Plugins/GenerativeAISupport/Content/Python/mcp_server.py
3. Open a new Unreal Engine project and run the below python script from the plugin's python directory.
Tools -> Run Python Script -> Select the
Plugins/GenerativeAISupport/Content/Python/unreal_socket_server.py
file.
- Nodes fail to connect properly with MCP
- No undo redo support for MCP
- No streaming support for Deepseek reasoning model
- No complex material generation support for the create material tool
- Issues with running some llm generated valid python scripts
- When LLM compiles a blueprint no proper error handling in its response
- Issues spawning certain nodes, especially with getters and setters
- Doesn't open the right context window during scene and project files edit.
- Doesn't dock the window properly in the editor for blueprints.
- Install
unreal
python package and setup the IDE's python interpreter for proper intellisense.
pip install unreal
More details will be added soon.
More details will be added soon.
- Env Var set logic from: OpenAI-Api-Unreal by KellanM
- MCP Server inspiration from: Blender-MCP by ahujasid
For Tasks:
Click tags to check more tools for each tasksFor Jobs:
Alternative AI tools for UnrealGenAISupport
Similar Open Source Tools
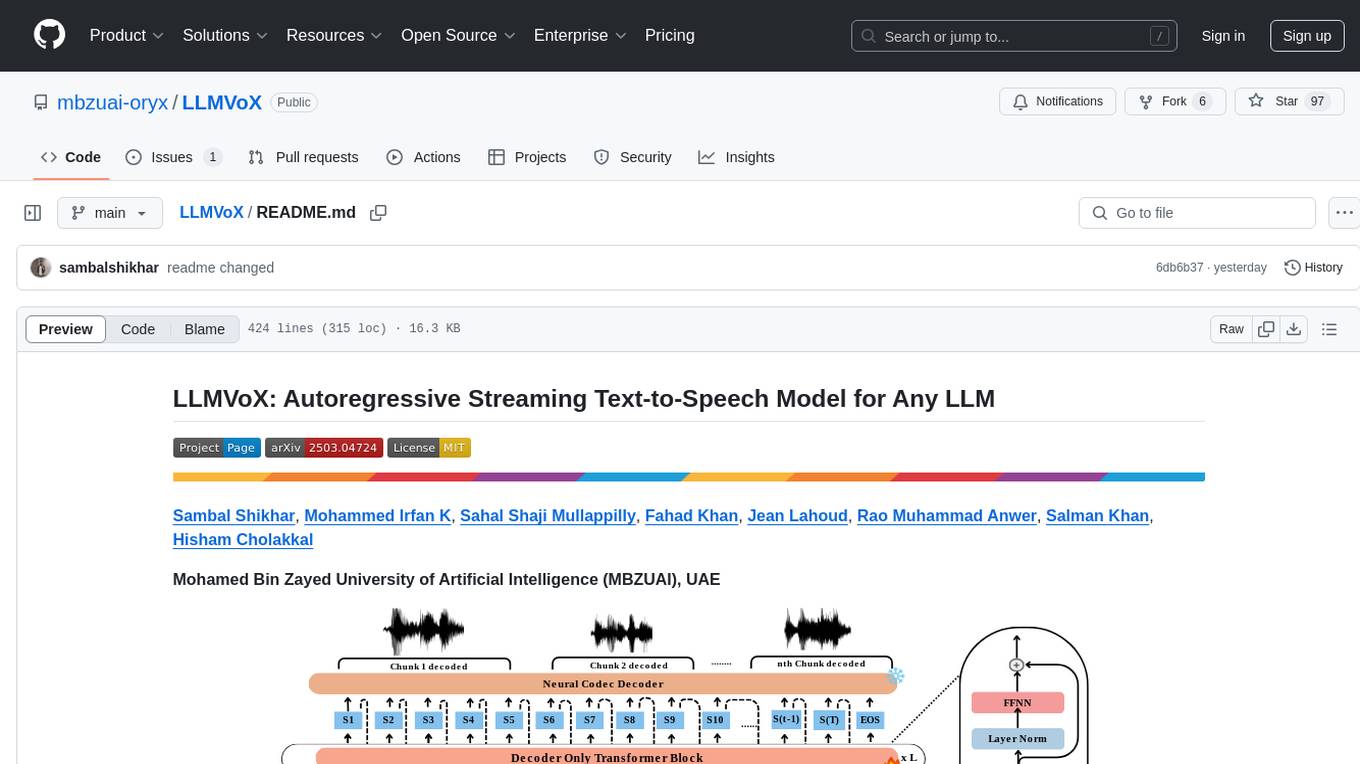
LLMVoX
LLMVoX is a lightweight 30M-parameter, LLM-agnostic, autoregressive streaming Text-to-Speech (TTS) system designed to convert text outputs from Large Language Models into high-fidelity streaming speech with low latency. It achieves significantly lower Word Error Rate compared to speech-enabled LLMs while operating at comparable latency and speech quality. Key features include being lightweight & fast with only 30M parameters, LLM-agnostic for easy integration with existing models, multi-queue streaming for continuous speech generation, and multilingual support for easy adaptation to new languages.
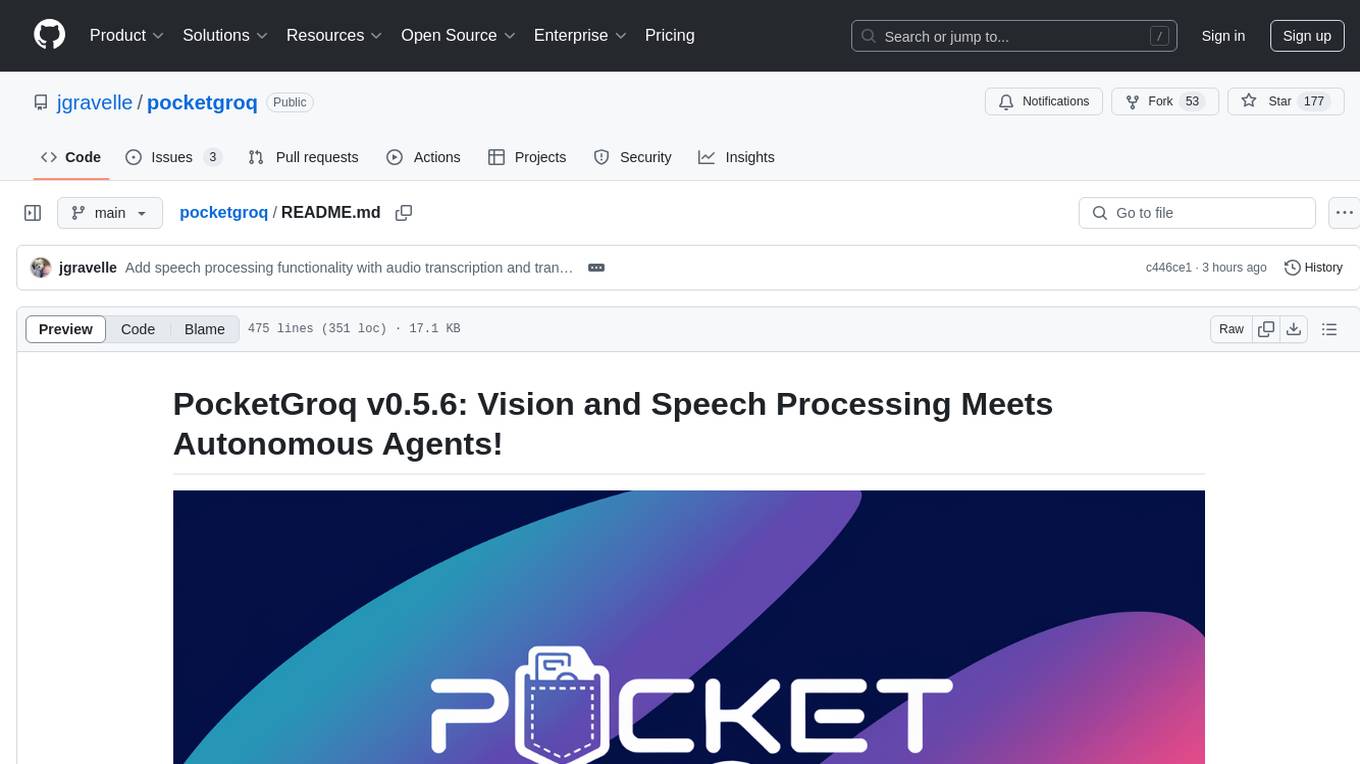
pocketgroq
PocketGroq is a tool that provides advanced functionalities for text generation, web scraping, web search, and AI response evaluation. It includes features like an Autonomous Agent for answering questions, web crawling and scraping capabilities, enhanced web search functionality, and flexible integration with Ollama server. Users can customize the agent's behavior, evaluate responses using AI, and utilize various methods for text generation, conversation management, and Chain of Thought reasoning. The tool offers comprehensive methods for different tasks, such as initializing RAG, error handling, and tool management. PocketGroq is designed to enhance development processes and enable the creation of AI-powered applications with ease.
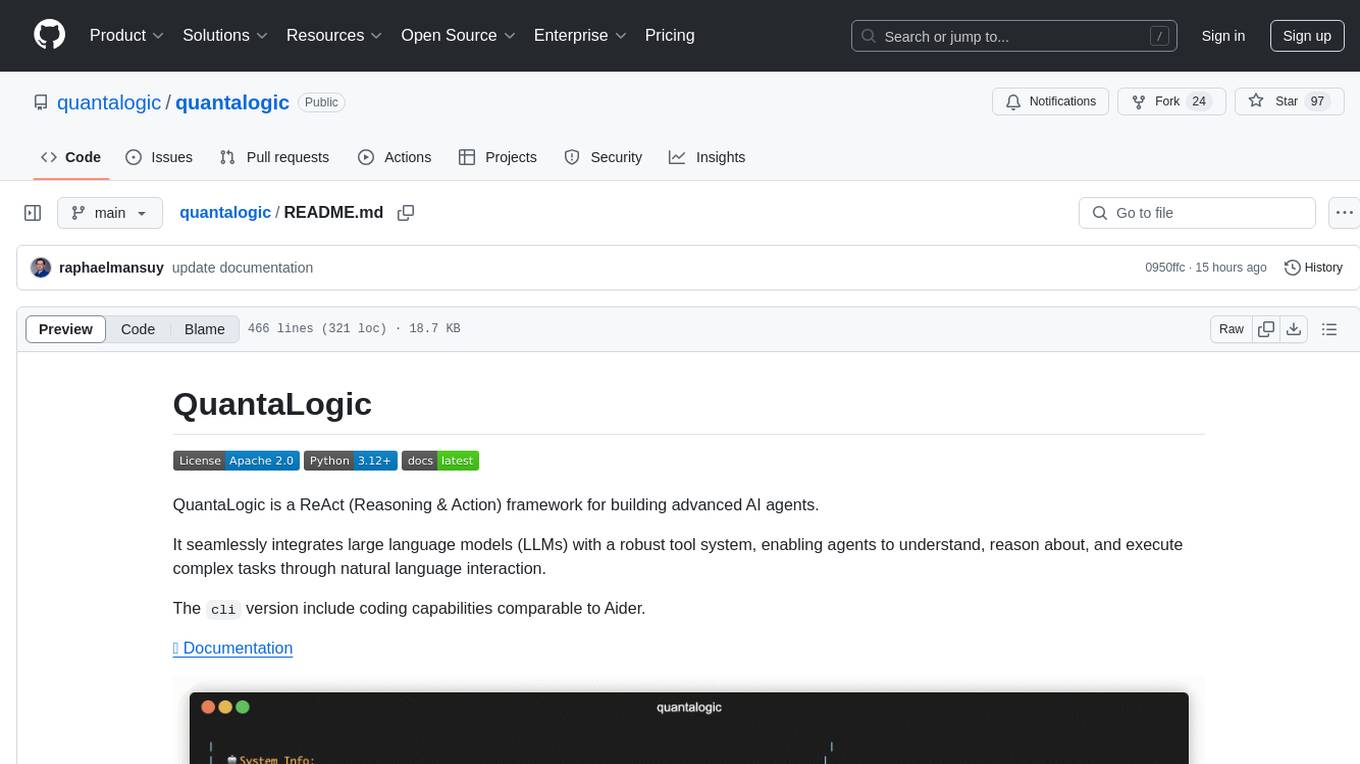
quantalogic
QuantaLogic is a ReAct framework for building advanced AI agents that seamlessly integrates large language models with a robust tool system. It aims to bridge the gap between advanced AI models and practical implementation in business processes by enabling agents to understand, reason about, and execute complex tasks through natural language interaction. The framework includes features such as ReAct Framework, Universal LLM Support, Secure Tool System, Real-time Monitoring, Memory Management, and Enterprise Ready components.
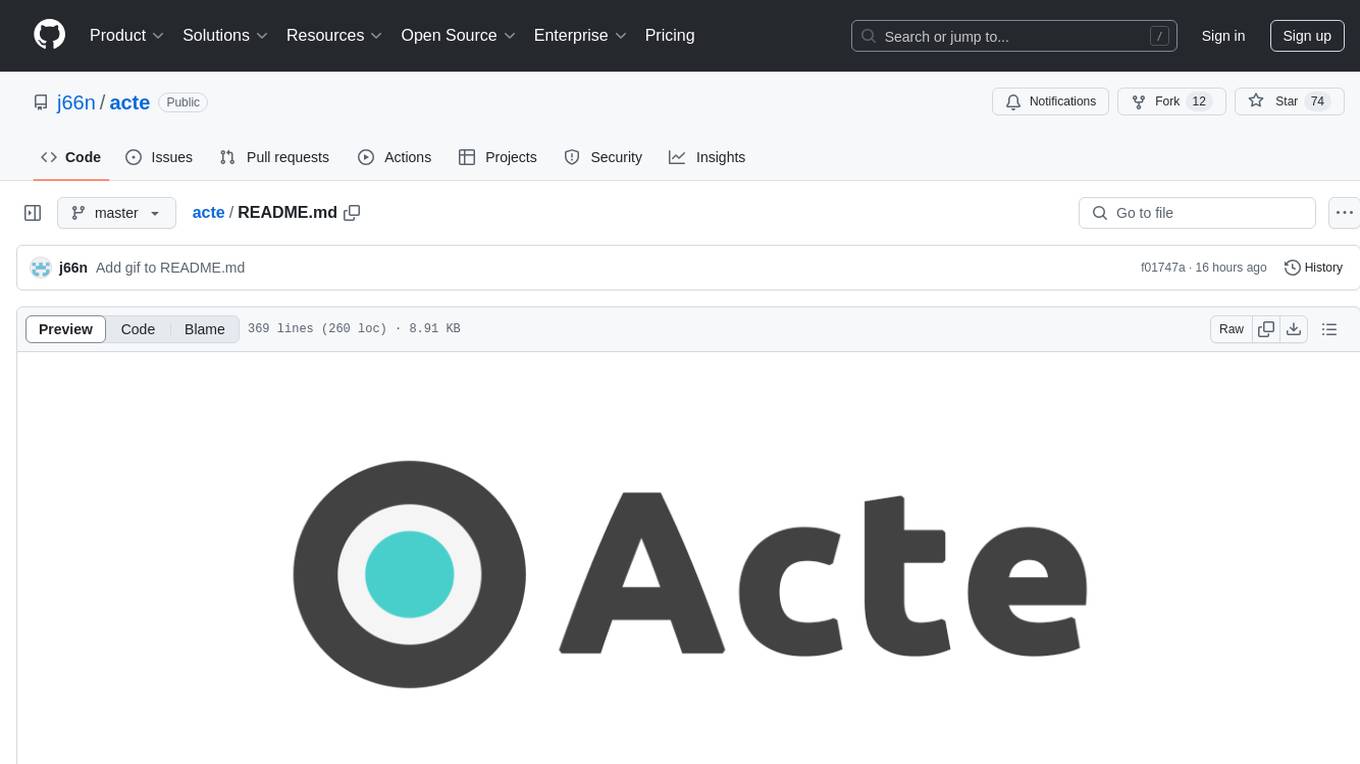
acte
Acte is a framework designed to build GUI-like tools for AI Agents. It aims to address the issues of cognitive load and freedom degrees when interacting with multiple APIs in complex scenarios. By providing a graphical user interface (GUI) for Agents, Acte helps reduce cognitive load and constraints interaction, similar to how humans interact with computers through GUIs. The tool offers APIs for starting new sessions, executing actions, and displaying screens, accessible via HTTP requests or the SessionManager class.
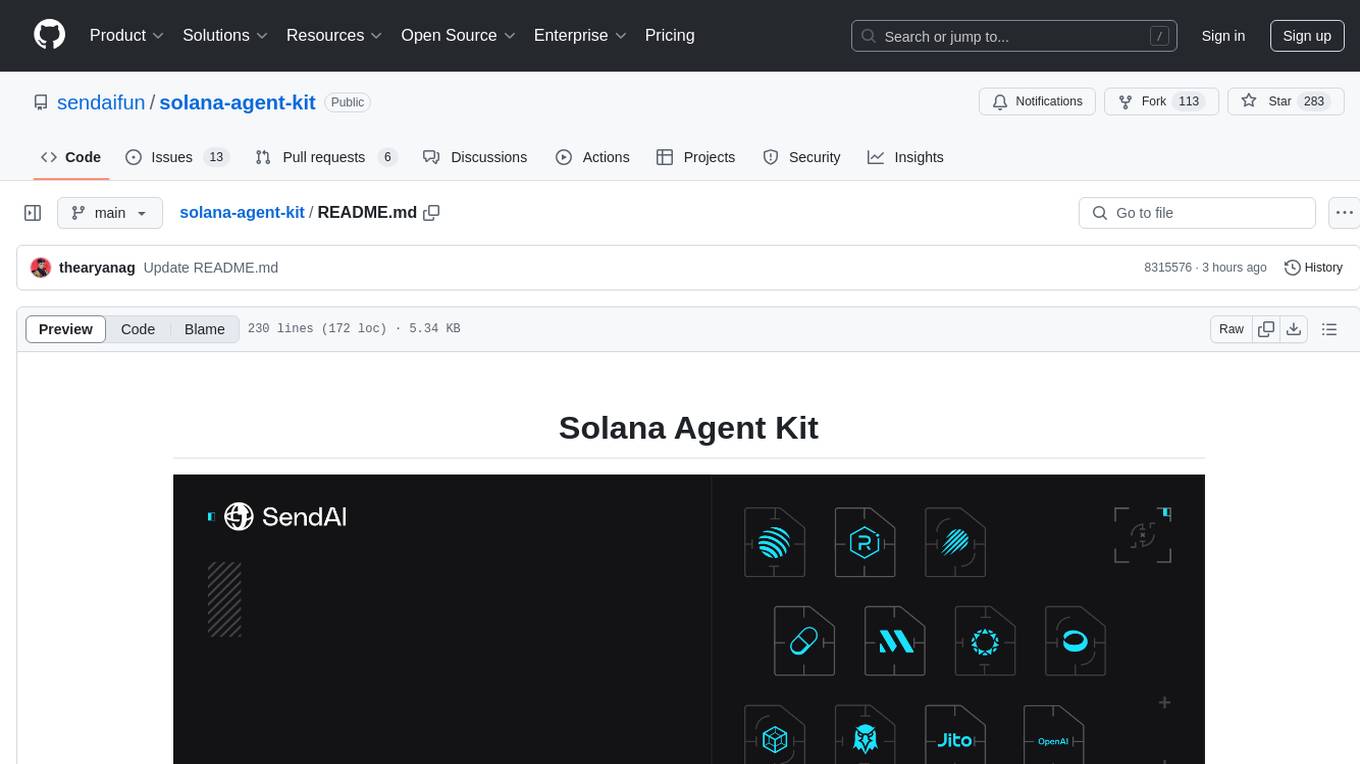
solana-agent-kit
Solana Agent Kit is an open-source toolkit designed for connecting AI agents to Solana protocols. It enables agents, regardless of the model used, to autonomously perform various Solana actions such as trading tokens, launching new tokens, lending assets, sending compressed airdrops, executing blinks, and more. The toolkit integrates core blockchain features like token operations, NFT management via Metaplex, DeFi integration, Solana blinks, AI integration features with LangChain, autonomous modes, and AI tools. It provides ready-to-use tools for blockchain operations, supports autonomous agent actions, and offers features like memory management, real-time feedback, and error handling. Solana Agent Kit facilitates tasks such as deploying tokens, creating NFT collections, swapping tokens, lending tokens, staking SOL, and sending SPL token airdrops via ZK compression. It also includes functionalities for fetching price data from Pyth and relies on key Solana and Metaplex libraries for its operations.
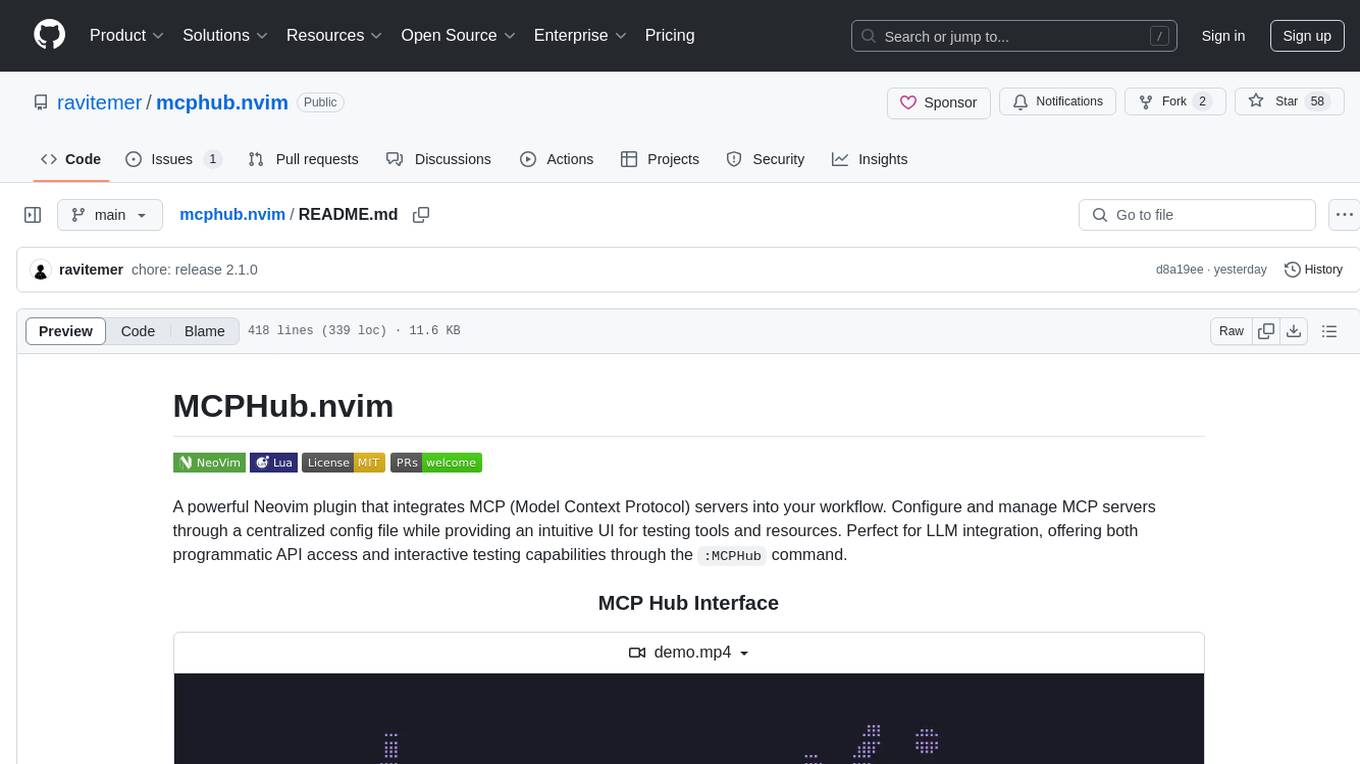
mcphub.nvim
MCPHub.nvim is a powerful Neovim plugin that integrates MCP (Model Context Protocol) servers into your workflow. It offers a centralized config file for managing servers and tools, with an intuitive UI for testing resources. Ideal for LLM integration, it provides programmatic API access and interactive testing through the `:MCPHub` command.
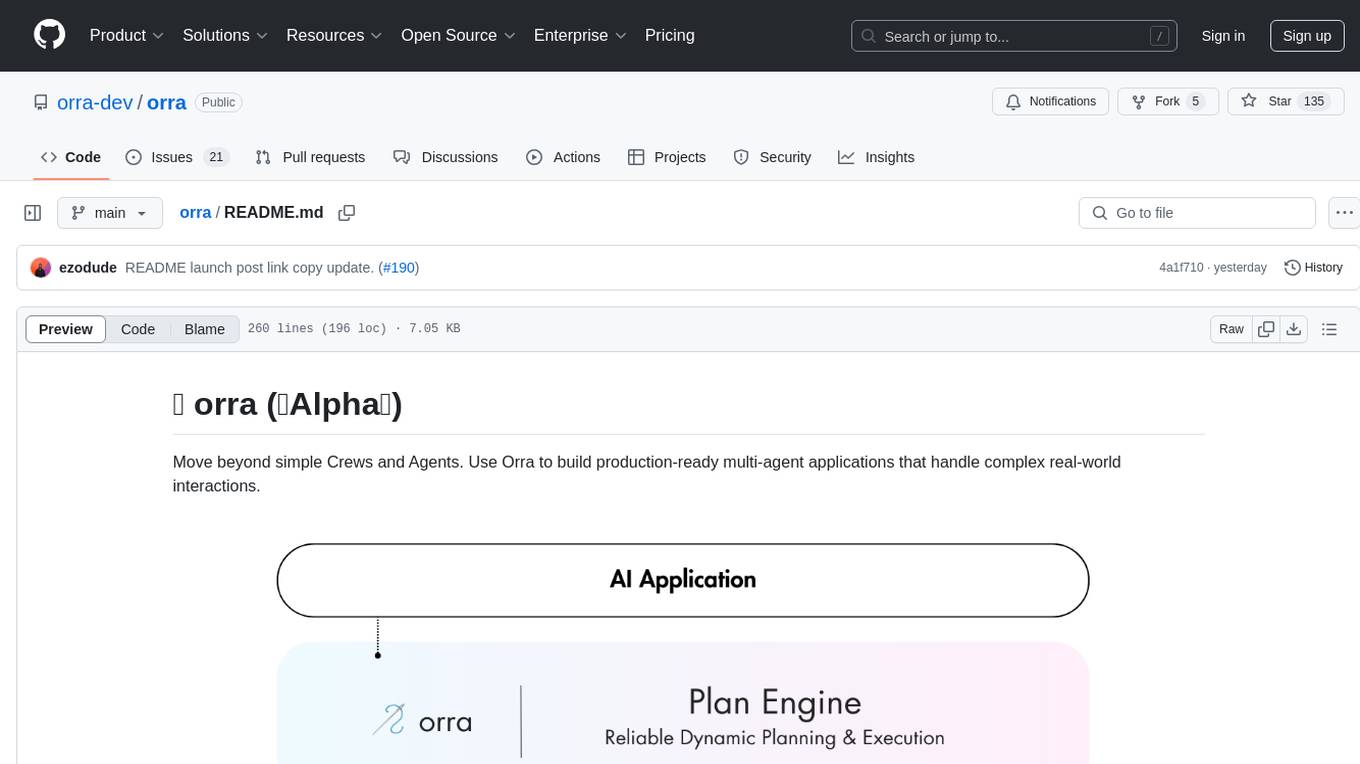
orra
Orra is a tool for building production-ready multi-agent applications that handle complex real-world interactions. It coordinates tasks across existing stack, agents, and tools run as services using intelligent reasoning. With features like smart pre-evaluated execution plans, domain grounding, durable execution, and automatic service health monitoring, Orra enables users to go fast with tools as services and revert state to handle failures. It provides real-time status tracking and webhook result delivery, making it ideal for developers looking to move beyond simple crews and agents.
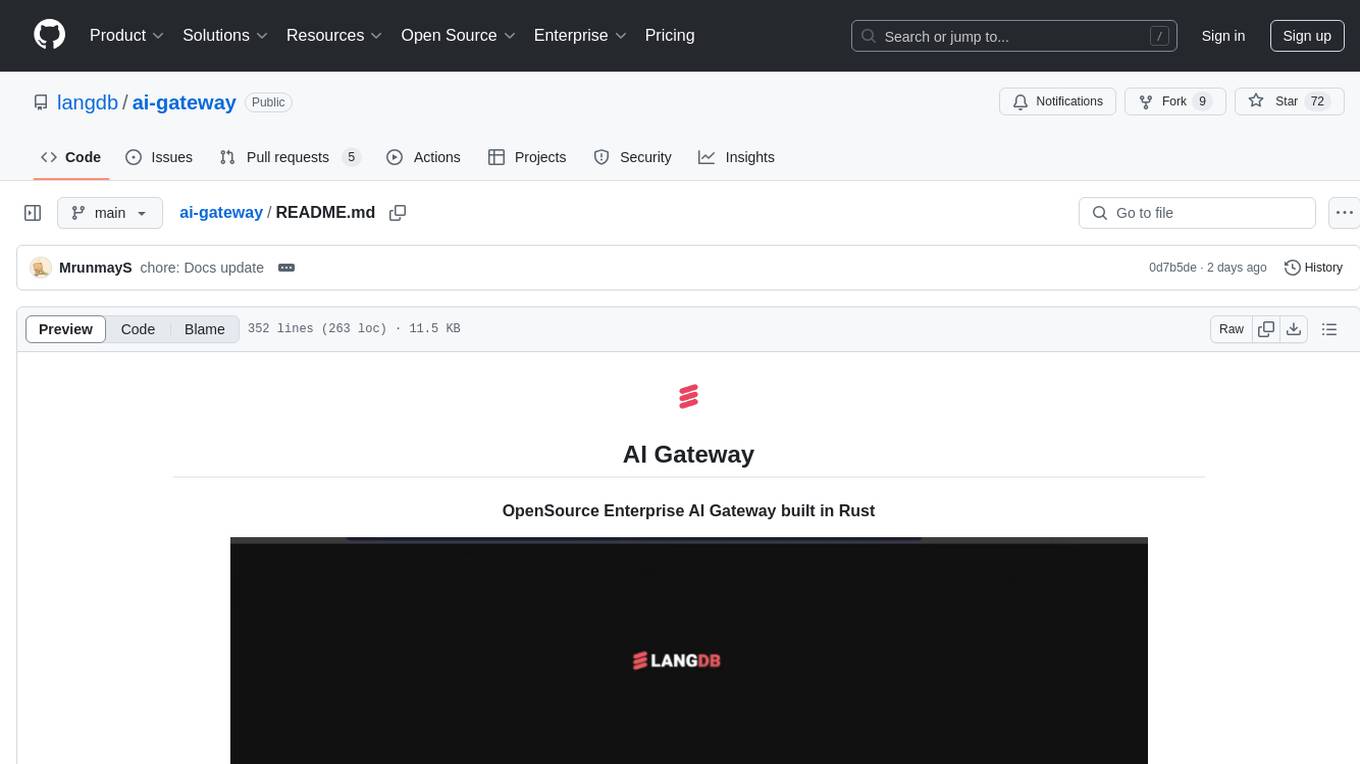
ai-gateway
LangDB AI Gateway is an open-source enterprise AI gateway built in Rust. It provides a unified interface to all LLMs using the OpenAI API format, focusing on high performance, enterprise readiness, and data control. The gateway offers features like comprehensive usage analytics, cost tracking, rate limiting, data ownership, and detailed logging. It supports various LLM providers and provides OpenAI-compatible endpoints for chat completions, model listing, embeddings generation, and image generation. Users can configure advanced settings, such as rate limiting, cost control, dynamic model routing, and observability with OpenTelemetry tracing. The gateway can be run with Docker Compose and integrated with MCP tools for server communication.
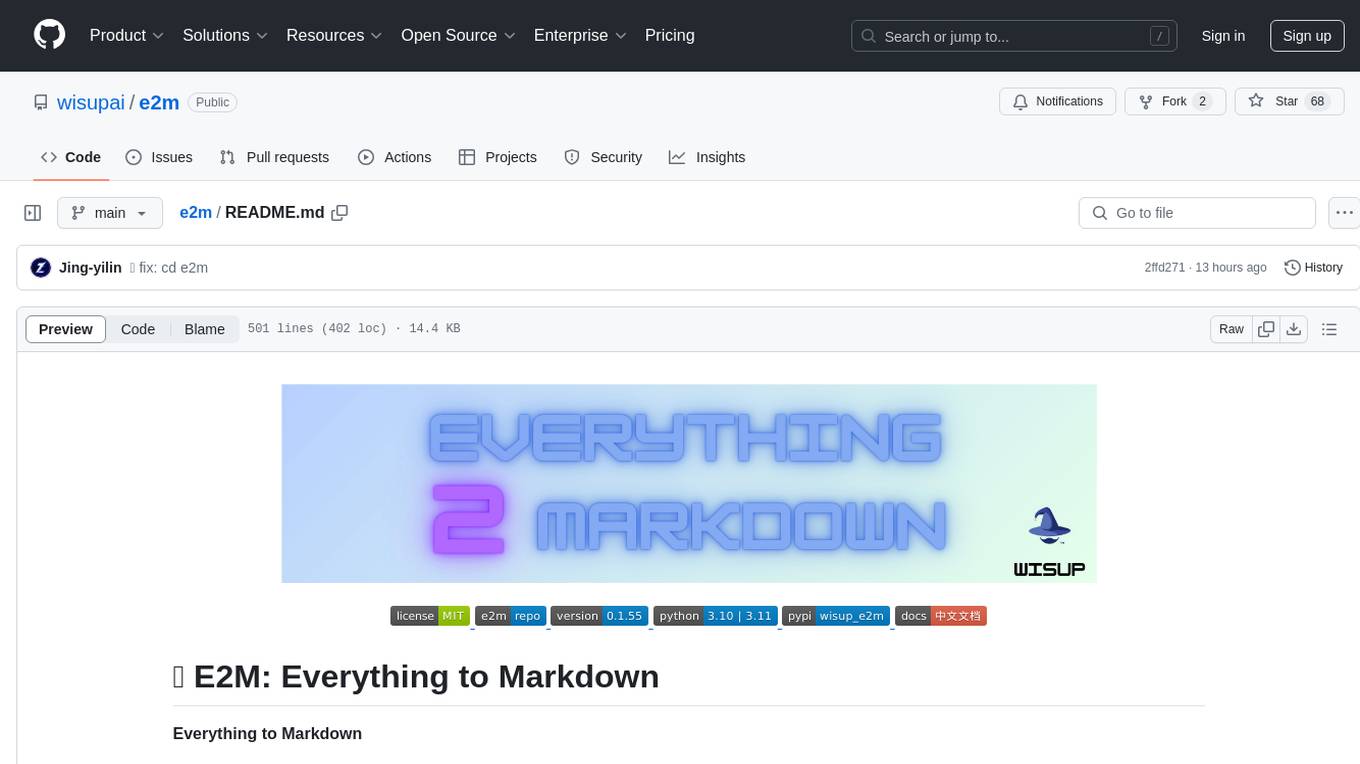
e2m
E2M is a Python library that can parse and convert various file types into Markdown format. It supports the conversion of multiple file formats, including doc, docx, epub, html, htm, url, pdf, ppt, pptx, mp3, and m4a. The ultimate goal of the E2M project is to provide high-quality data for Retrieval-Augmented Generation (RAG) and model training or fine-tuning. The core architecture consists of a Parser responsible for parsing various file types into text or image data, and a Converter responsible for converting text or image data into Markdown format.
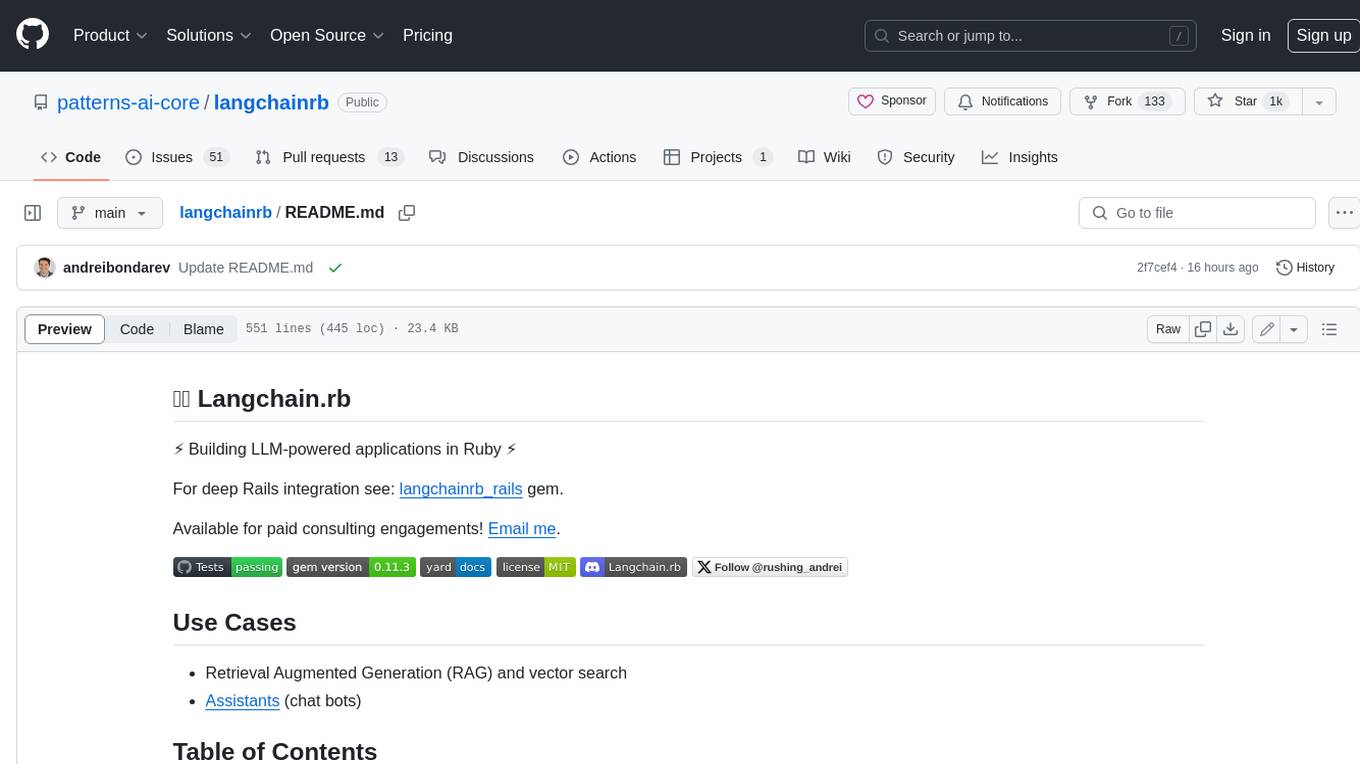
langchainrb
Langchain.rb is a Ruby library that makes it easy to build LLM-powered applications. It provides a unified interface to a variety of LLMs, vector search databases, and other tools, making it easy to build and deploy RAG (Retrieval Augmented Generation) systems and assistants. Langchain.rb is open source and available under the MIT License.
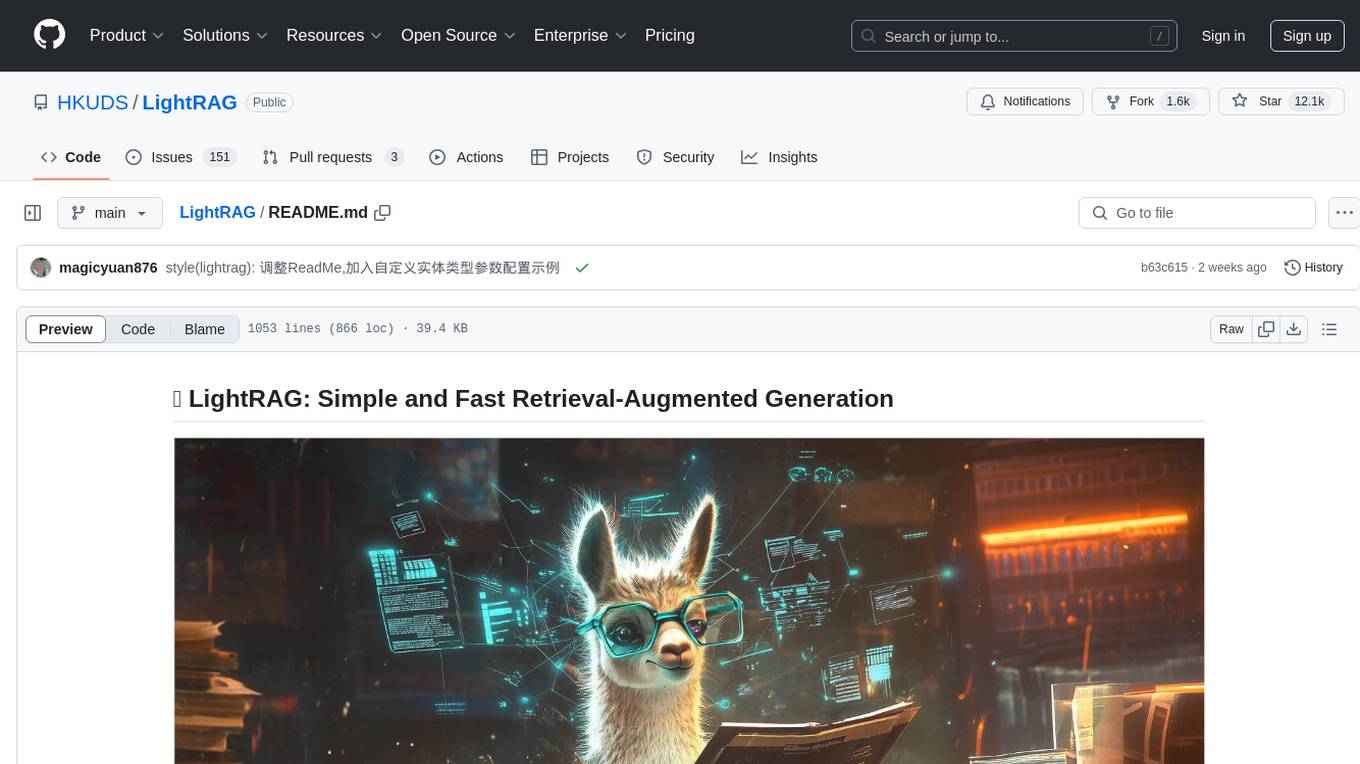
LightRAG
LightRAG is a repository hosting the code for LightRAG, a system that supports seamless integration of custom knowledge graphs, Oracle Database 23ai, Neo4J for storage, and multiple file types. It includes features like entity deletion, batch insert, incremental insert, and graph visualization. LightRAG provides an API server implementation for RESTful API access to RAG operations, allowing users to interact with it through HTTP requests. The repository also includes evaluation scripts, code for reproducing results, and a comprehensive code structure.
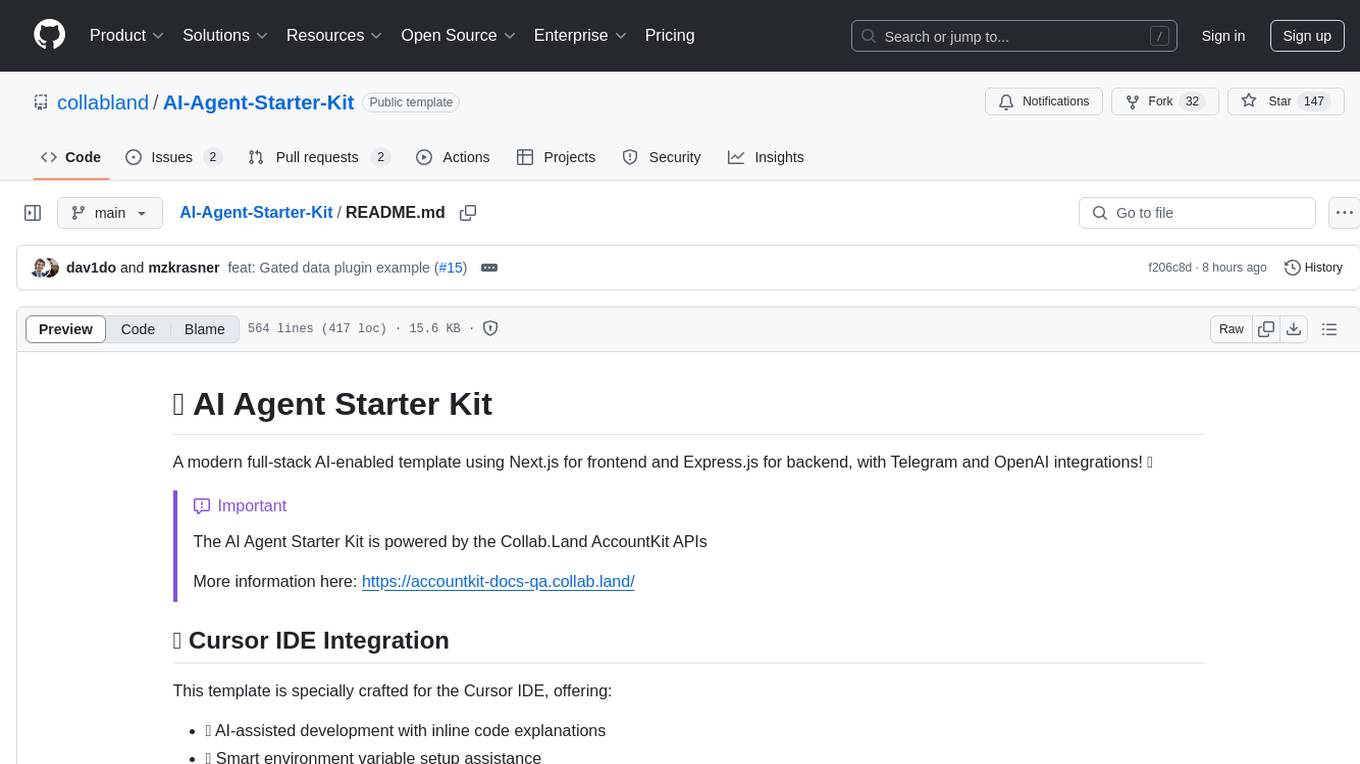
AI-Agent-Starter-Kit
AI Agent Starter Kit is a modern full-stack AI-enabled template using Next.js for frontend and Express.js for backend, with Telegram and OpenAI integrations. It offers AI-assisted development, smart environment variable setup assistance, intelligent error resolution, context-aware code completion, and built-in debugging helpers. The kit provides a structured environment for developers to interact with AI tools seamlessly, enhancing the development process and productivity.