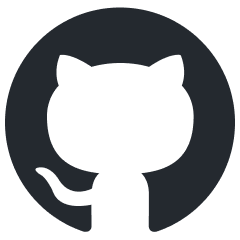
model_baseline
Testing baseline LLMs performance across various models
Stars: 232
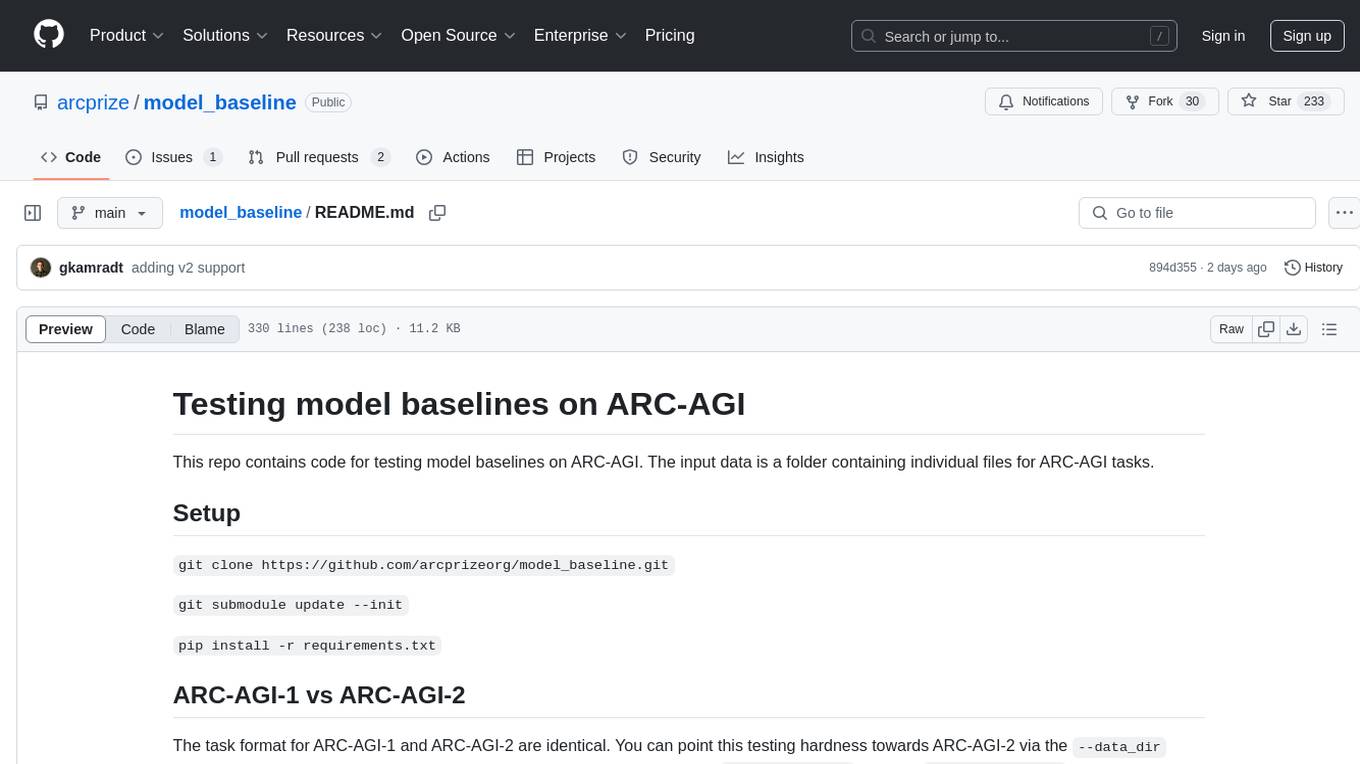
README:
This repo contains code for testing model baselines on ARC-AGI. The input data is a folder containing individual files for ARC-AGI tasks.
git clone https://github.com/arcprizeorg/model_baseline.git
git submodule update --init
pip install -r requirements.txt
The task format for ARC-AGI-1 and ARC-AGI-2 are identical. You can point this testing hardness towards ARC-AGI-2 via the --data_dir
parameter. When running with concurrency, ensure you're using the correct <task_list>.txt
found in data/task_lists/
for the set you're testing.
To test a single task, run:
python3 -m main --data_dir data/arc-agi/data/evaluation --config claude_sonnet--task_id 0a1d4ef5 --print_logs
Use the optional parameters to save and print the submission:
python3 -m main --data_dir data/arc-agi/data/evaluation --config claude_sonnet --task_id {} --save_submission_dir submissions/claude_sonnet_20241022 --print_logs
This will write one <id>.json
file per task.
Testing multiple tasks in a single run can be slow. You can use the your parallel technique of choice to speed this up.
For example with the parallel
command:
brew install parallel
parallel --jobs 20 --progress python3 -m main --data_dir data/arc-agi/data/evaluation --config claude_sonnet --task_id {} --save_submission_dir submissions/claude_sonnet_20241022 --print_logs :::: ./data/task_lists/public_evaluation_v1.txt
Note: In order to use parllel you'll need a list of task ids. generate_tasks_list.py
helps with this. Public data task ids are already supplied.
python3 -m src.utils.generate_tasks_list --task_dir data/arc-agi/data/training --output_file data/task_lists/public_training
You can score your submissions by pointing the scoring script at your submissions directory:
python3 -m src.scoring.scoring --task_dir data/arc-agi/data/evaluation --submission_dir submissions/claude_sonnet_20241022 --print_logs --results_dir results/claude_sonnet_20241022
Note: You'll also need to tell the script which task set to score.
Results are stored in the results
folder. You can view historical results for models here.
This repo is welcome to contributions!
Specifically, we would love help adding more model adapters to the src/adapters
folder.
More will get added by the ARC-AGI team, but we'll also gladly accept contributions from the community.
For more information visit the ARC Prize.
Validate model outputs against task sets:
# Basic validation
python cli/main.py validate data/arc-agi/data/evaluation submissions/open_ai_o1_high_20241217
# Validate another model's outputs
python cli/main.py validate data/arc-agi/data/evaluation submissions/claude_sonnet_20241022
Upload a single model's outputs to a task set repository:
# Basic upload (private repository)
python cli/main.py upload submissions/open_ai_o1_high_20241217 --task-set public_eval_v1
# Upload to a different organization
python cli/main.py upload submissions/claude_sonnet_20241022 --task-set public_eval_v1 --org your-org-name
# Create a public repository
python cli/main.py upload submissions/deepseek_v3 --task-set public_eval_v1 --public
Upload multiple model outputs at once:
# Upload all models in submissions directory (private repository)
python cli/main.py bulk-upload submissions/ --task-set public_eval_v1
# Upload to a different organization
python cli/main.py bulk-upload submissions/ --task-set public_eval_v1 --org your-org-name
# Create a public repository
python cli/main.py bulk-upload submissions/ --task-set public_eval_v1 --public
Notes:
- All uploads create private repositories by default
- Use
--public
flag to create public repositories - Files are uploaded to subdirectories matching model names
- Default organization is "arcprize"
Before uploading, you'll need to authenticate with Hugging Face:
- Get your access token from https://huggingface.co/settings/tokens
- Set up authentication using either method:
# Option 1: Environment variable export HUGGING_FACE_HUB_TOKEN=your_token_here # Option 2: CLI login huggingface-cli login
The upload process organizes submissions by task sets. Each task set (e.g., public_eval_v1) becomes a separate dataset repository on Hugging Face, with model submissions organized in subdirectories.
Structure:
task_set_name/
├── model_name_1/
│ ├── result1.json
│ ├── result2.json
├── model_name_2/
│ ├── result1.json
│ └── result2.json
To upload model outputs:
python cli/main.py upload submissions/model_name --task-set task_set_name [--org organization] [--public]
For example:
python cli/main.py upload submissions/open_ai_o1_high_20241217 --task-set public_eval_v1
To upload multiple model outputs at once:
python cli/main.py bulk-upload submissions/ --task-set task_set_name [--org organization] [--public]
For contributors implementing new providers, we provide a streamlined way to validate your implementation using the test_providers.sh
script. This script helps ensure your provider implementation works correctly with the ARC-AGI tasks before submitting a pull request.
# Run all provider tests
./test_providers.sh
# The script will test multiple provider/model combinations in parallel
# Each test will:
# 1. Run a specific task for each provider/model
# 2. Save the output
# 3. Report success/failure
The tests ensure that:
- The provider can successfully connect to its API
- The model can process ARC-AGI tasks
- The output matches the expected format
- The provider correctly handles token usage and costs
You can test the same model with different configurations by using the --config
parameter:
# Test a model with a specific configuration
python3 -m main --data_dir data/arc-agi/data/evaluation --config claude_sonnet --task_id sample_task_id --print_logs
The test_providers.sh
script includes examples of testing the same model with different configurations, such as:
-
openai o1 0b17323b high_temp
- Testing o1 with high temperature -
openai o1 0b17323b low_temp
- Testing o1 with low temperature
New models are defined in src/models.yml
. Each model requires:
models:
- name: "model_config_name" # A unique identifier for this model configuration
model_name: "actual-model-name" # The actual model name used by the provider's API
provider: "provider-name"
max_tokens: 4024 # or appropriate limit
temperature: 0.0 # optional
pricing:
date: "YYYY-MM-DD"
input: 0.00 # Cost per 1M input tokens
output: 0.00 # Cost per 1M output tokens
In models.yml
, you can create multiple configurations for the same underlying model by defining separate entries with different name
values but the same model_name
:
models:
# Configuration for short responses
- name: "o1_short_response"
model_name: "o1"
provider: "openai"
max_completion_tokens: 1024 # Shorter response limit
pricing:
date: "2025-02-23"
input: 15.00
output: 60.00
# Configuration for long responses
- name: "o1_long_response"
model_name: "o1"
provider: "openai"
max_completion_tokens: 4024 # Longer response limit
pricing:
date: "2025-02-23"
input: 15.00
output: 60.00
When running the model, you specify the configuration name as the model parameter:
# Run with short response configuration
python3 -m main --config o1_short_response --task_id sample_task_id
# Run with long response configuration
python3 -m main --config o1_long_response --task_id sample_task_id
You can add any model-specific parameters supported by the provider's API:
models:
- name: "gemini_pro"
model_name: "gemini-1.5-pro"
provider: "gemini"
max_output_tokens: 4024 # Provider-specific parameter
temperature: 0.0
pricing:
date: "2025-02-23"
input: 1.25
output: 5.00
Note how different providers may use different parameter names (e.g., max_tokens
, max_completion_tokens
, or max_output_tokens
) depending on their API requirements.
When running batch tests with multiple configurations:
# Test with short response configuration
parallel --jobs 20 python3 -m main --data_dir data/arc-agi/data/evaluation --config o1_long_response --task_id {} --save_submission_dir submissions/o1_short :::: ./data/task_lists/public_evaluation_v1.txt
# Test with long response configuration
parallel --jobs 20 python3 -m main --data_dir data/arc-agi/data/evaluation --config o1_long_response --task_id {} --save_submission_dir submissions/o1_long :::: ./data/task_lists/public_evaluation_v1.txt
After running tests with different configurations, you can compare their performance:
# Score short response configuration
python3 -m src.scoring.scoring --task_dir data/arc-agi/data/evaluation --submission_dir submissions/o1_short --print_logs --results_dir results/o1_short
# Score long response configuration
python3 -m src.scoring.scoring --task_dir data/arc-agi/data/evaluation --submission_dir submissions/o1_long --print_logs --results_dir results/o1_long
This allows you to systematically evaluate how different parameter settings affect model performance on ARC-AGI tasks.
-
Create a new file in
src/adapters/
(e.g.,my_provider.py
) -
Implement the
ProviderAdapter
class:from .provider import ProviderAdapter class MyProviderAdapter(ProviderAdapter): def init_client(self): # Initialize API client pass def make_prediction(self, prompt: str) -> Attempt: # Make prediction and return standardized Attempt object pass def chat_completion(self, messages: str) -> str: # Handle chat completion pass
-
Key requirements:
- Handle authentication (typically via environment variables)
- Implement proper error handling
- Convert provider-specific responses to standardized formats
- Track and report token usage and costs
- Add test cases to
test_providers.sh
- Test with sample tasks:
python3 -m main --data_dir data/arc-agi/data/evaluation --provider new_provider --model new_model --task_id sample_task_id --print_logs
Remember to:
- Follow the existing patterns in other provider implementations
- Maintain consistent error handling
- Document any provider-specific requirements or limitations
- Update tests to cover the new provider
For Tasks:
Click tags to check more tools for each tasksFor Jobs:
Alternative AI tools for model_baseline
Similar Open Source Tools
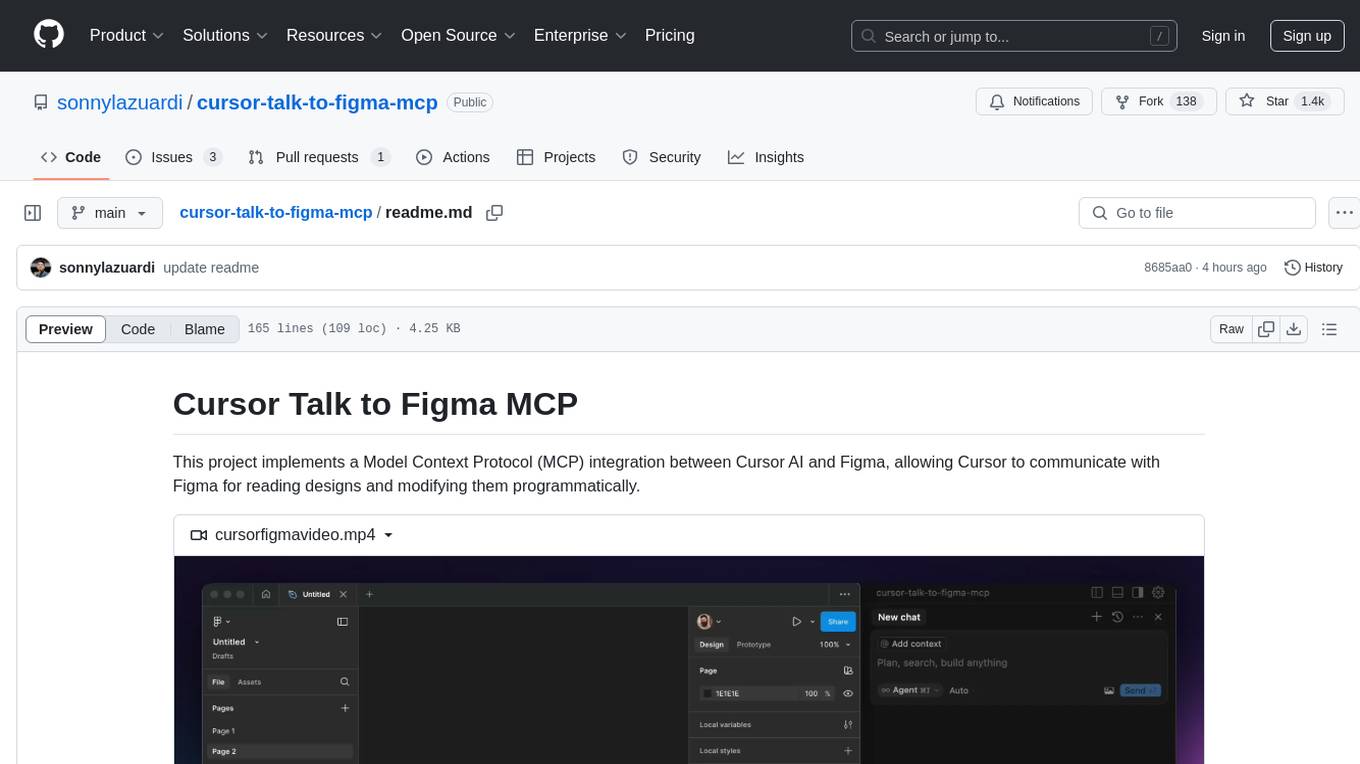
cursor-talk-to-figma-mcp
This project implements a Model Context Protocol (MCP) integration between Cursor AI and Figma, allowing Cursor to communicate with Figma for reading designs and modifying them programmatically. It provides tools for interacting with Figma such as creating elements, modifying text content, styling, layout & organization, components & styles, export & advanced features, and connection management. The project structure includes a TypeScript MCP server for Figma integration, a Figma plugin for communicating with Cursor, and a WebSocket server for facilitating communication between the MCP server and Figma plugin.
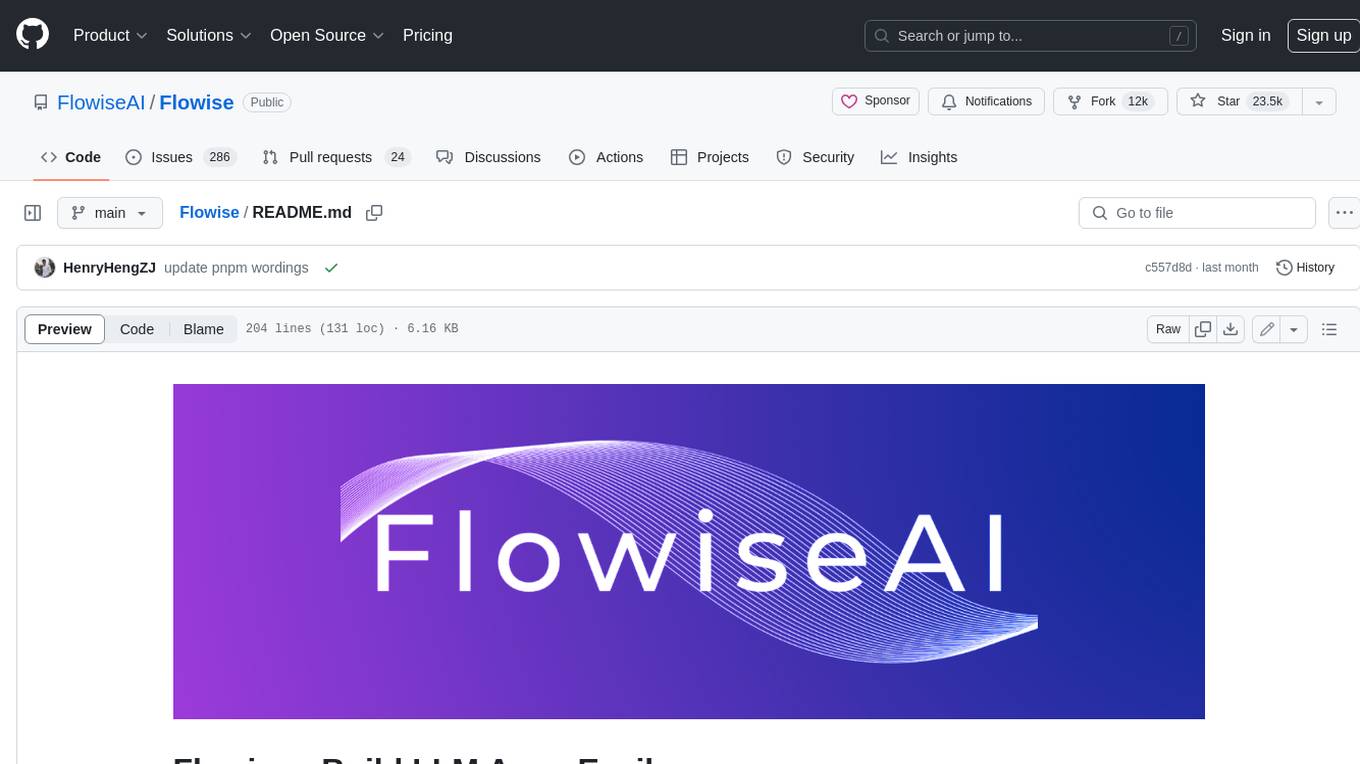
Flowise
Flowise is a tool that allows users to build customized LLM flows with a drag-and-drop UI. It is open-source and self-hostable, and it supports various deployments, including AWS, Azure, Digital Ocean, GCP, Railway, Render, HuggingFace Spaces, Elestio, Sealos, and RepoCloud. Flowise has three different modules in a single mono repository: server, ui, and components. The server module is a Node backend that serves API logics, the ui module is a React frontend, and the components module contains third-party node integrations. Flowise supports different environment variables to configure your instance, and you can specify these variables in the .env file inside the packages/server folder.
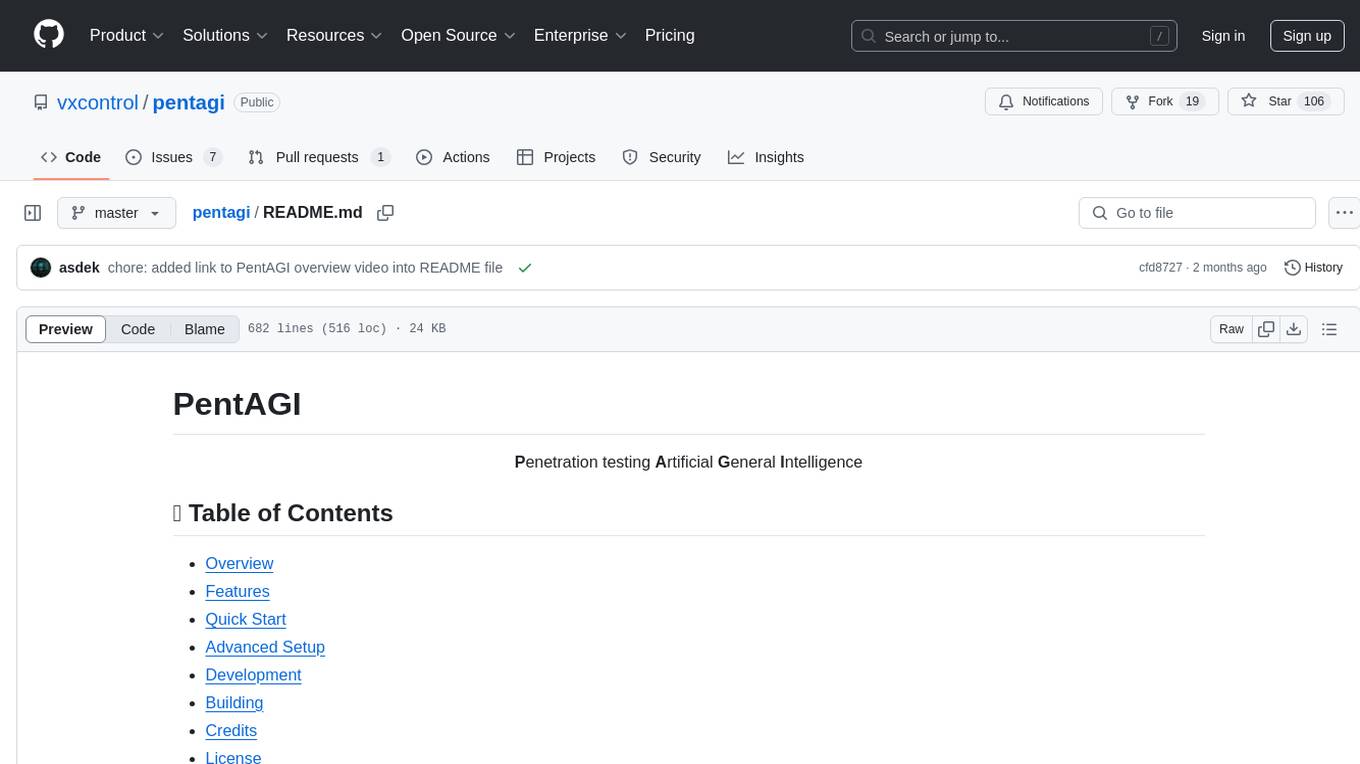
pentagi
PentAGI is an innovative tool for automated security testing that leverages cutting-edge artificial intelligence technologies. It is designed for information security professionals, researchers, and enthusiasts who need a powerful and flexible solution for conducting penetration tests. The tool provides secure and isolated operations in a sandboxed Docker environment, fully autonomous AI-powered agent for penetration testing steps, a suite of 20+ professional security tools, smart memory system for storing research results, web intelligence for gathering information, integration with external search systems, team delegation system, comprehensive monitoring and reporting, modern interface, API integration, persistent storage, scalable architecture, self-hosted solution, flexible authentication, and quick deployment through Docker Compose.
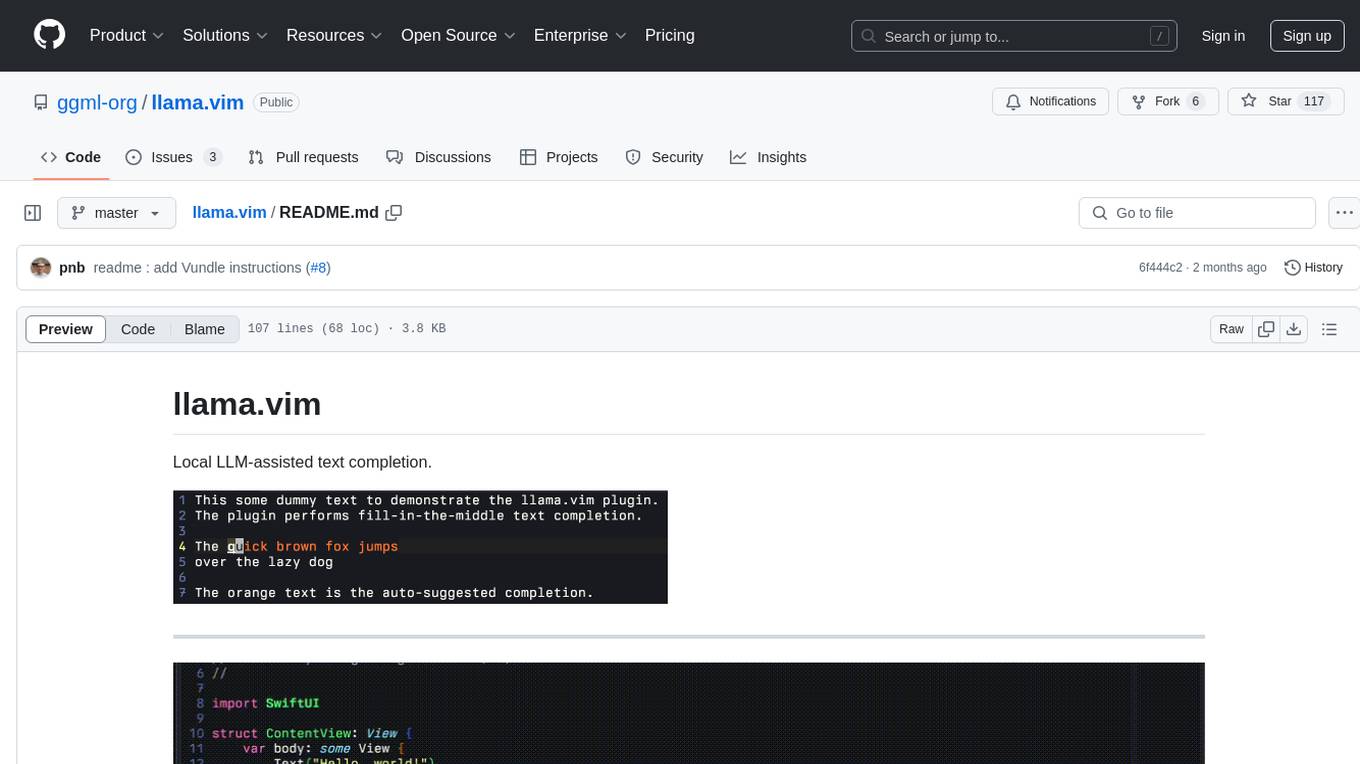
llama.vim
llama.vim is a plugin that provides local LLM-assisted text completion for Vim users. It offers features such as auto-suggest on cursor movement, manual suggestion toggling, suggestion acceptance with Tab and Shift+Tab, control over text generation time, context configuration, ring context with chunks from open and edited files, and performance stats display. The plugin requires a llama.cpp server instance to be running and supports FIM-compatible models. It aims to be simple, lightweight, and provide high-quality and performant local FIM completions even on consumer-grade hardware.
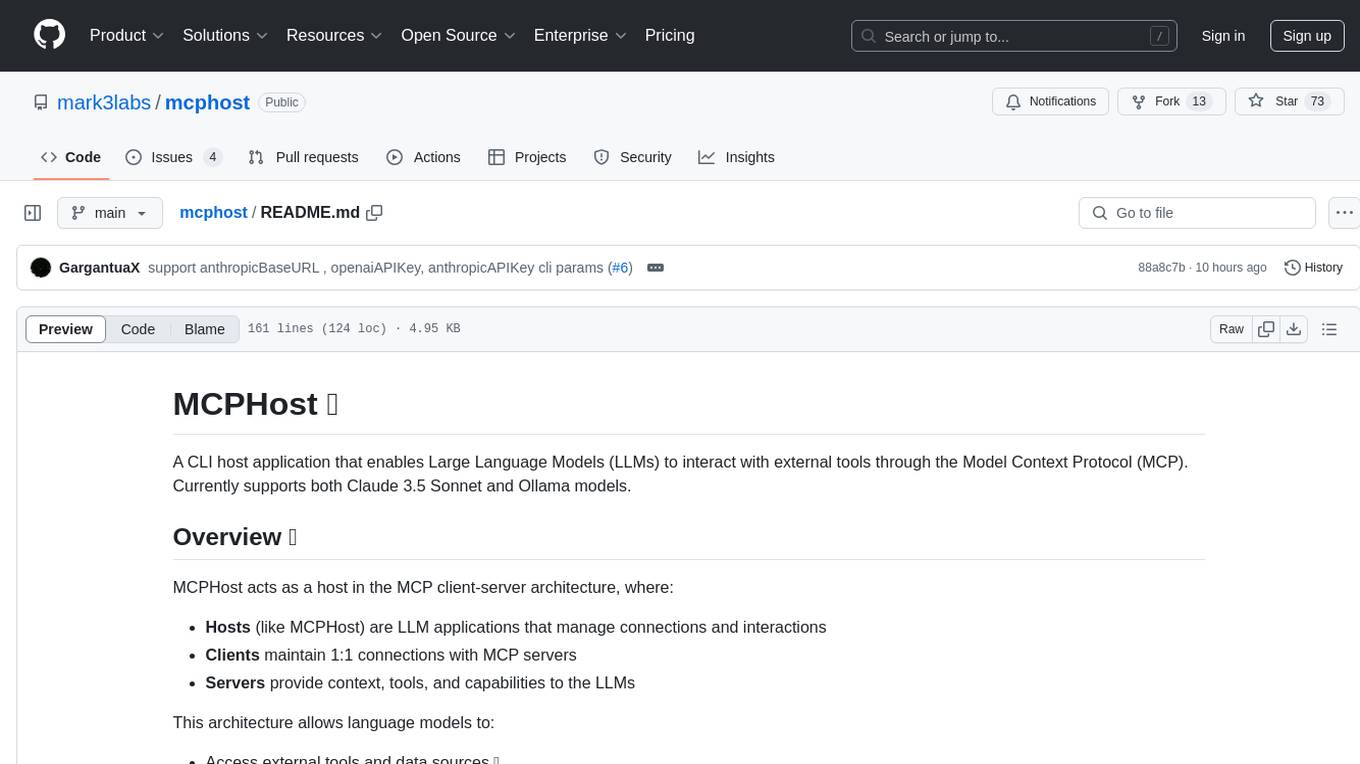
mcphost
MCPHost is a CLI host application that enables Large Language Models (LLMs) to interact with external tools through the Model Context Protocol (MCP). It acts as a host in the MCP client-server architecture, allowing language models to access external tools and data sources, maintain consistent context across interactions, and execute commands safely. The tool supports interactive conversations with Claude 3.5 Sonnet and Ollama models, multiple concurrent MCP servers, dynamic tool discovery and integration, configurable server locations and arguments, and a consistent command interface across model types.
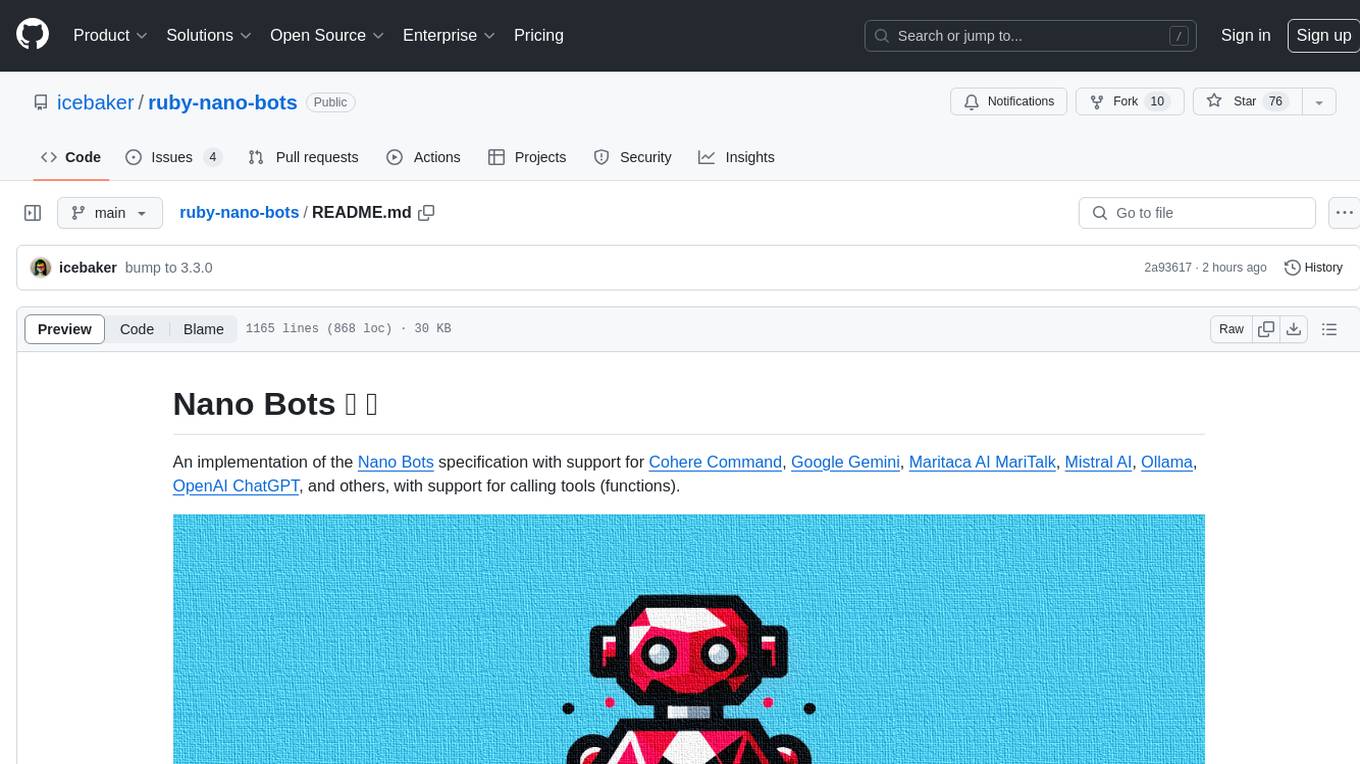
ruby-nano-bots
Ruby Nano Bots is an implementation of the Nano Bots specification supporting various AI providers like Cohere Command, Google Gemini, Maritaca AI MariTalk, Mistral AI, Ollama, OpenAI ChatGPT, and others. It allows calling tools (functions) and provides a helpful assistant for interacting with AI language models. The tool can be used both from the command line and as a library in Ruby projects, offering features like REPL, debugging, and encryption for data privacy.
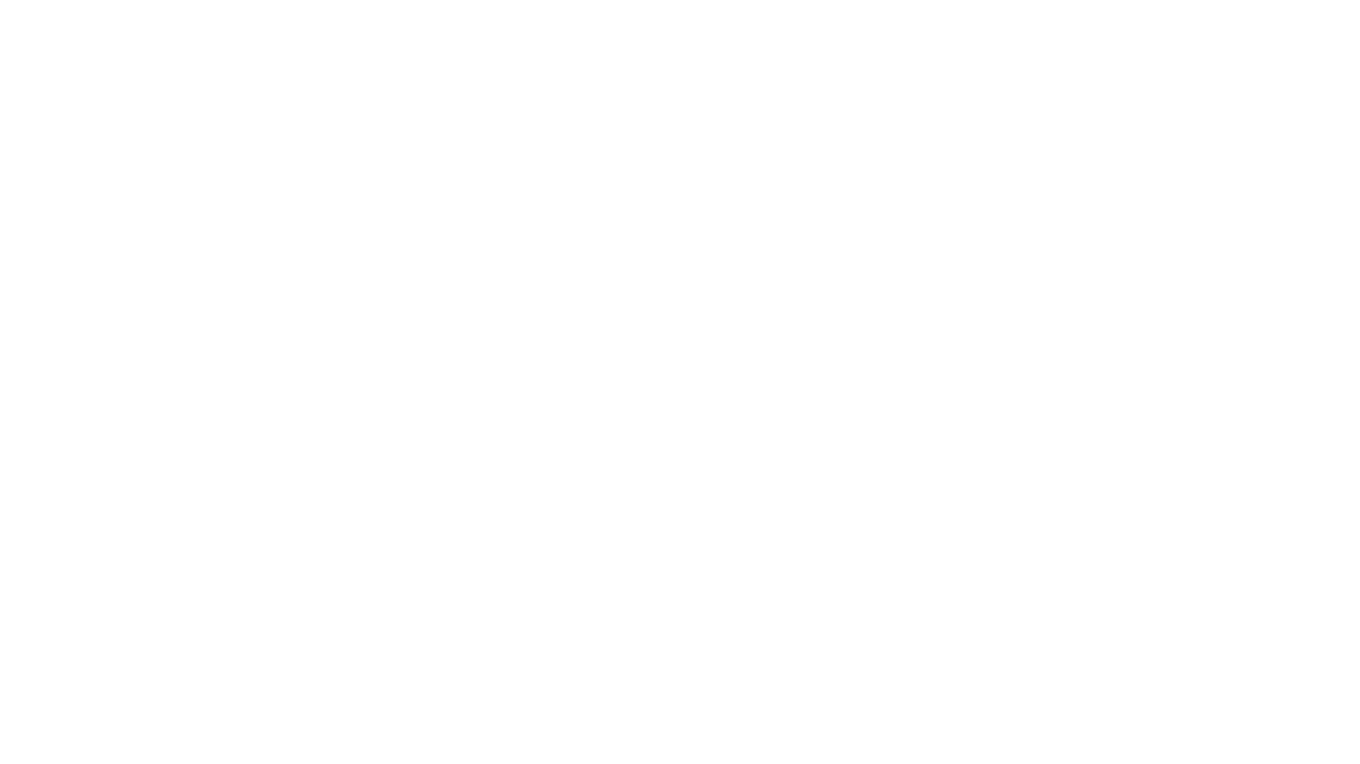
TalkWithGemini
Talk With Gemini is a web application that allows users to deploy their private Gemini application for free with one click. It supports Gemini Pro and Gemini Pro Vision models. The application features talk mode for direct communication with Gemini, visual recognition for understanding picture content, full Markdown support, automatic compression of chat records, privacy and security with local data storage, well-designed UI with responsive design, fast loading speed, and multi-language support. The tool is designed to be user-friendly and versatile for various deployment options and language preferences.
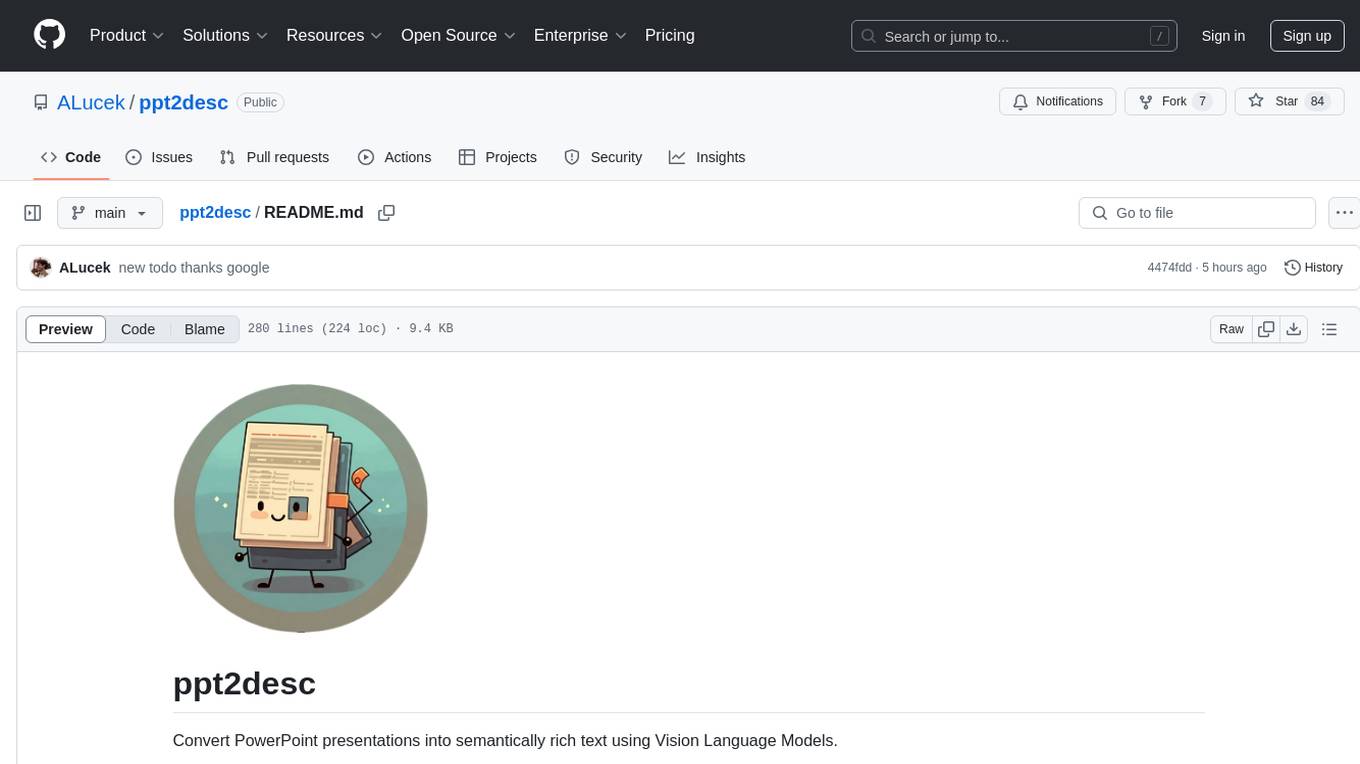
ppt2desc
ppt2desc is a command-line tool that converts PowerPoint presentations into detailed textual descriptions using vision language models. It interprets and describes visual elements, capturing the full semantic meaning of each slide in a machine-readable format. The tool supports various model providers and offers features like converting PPT/PPTX files to semantic descriptions, processing individual files or directories, visual elements interpretation, rate limiting for API calls, customizable prompts, and JSON output format for easy integration.
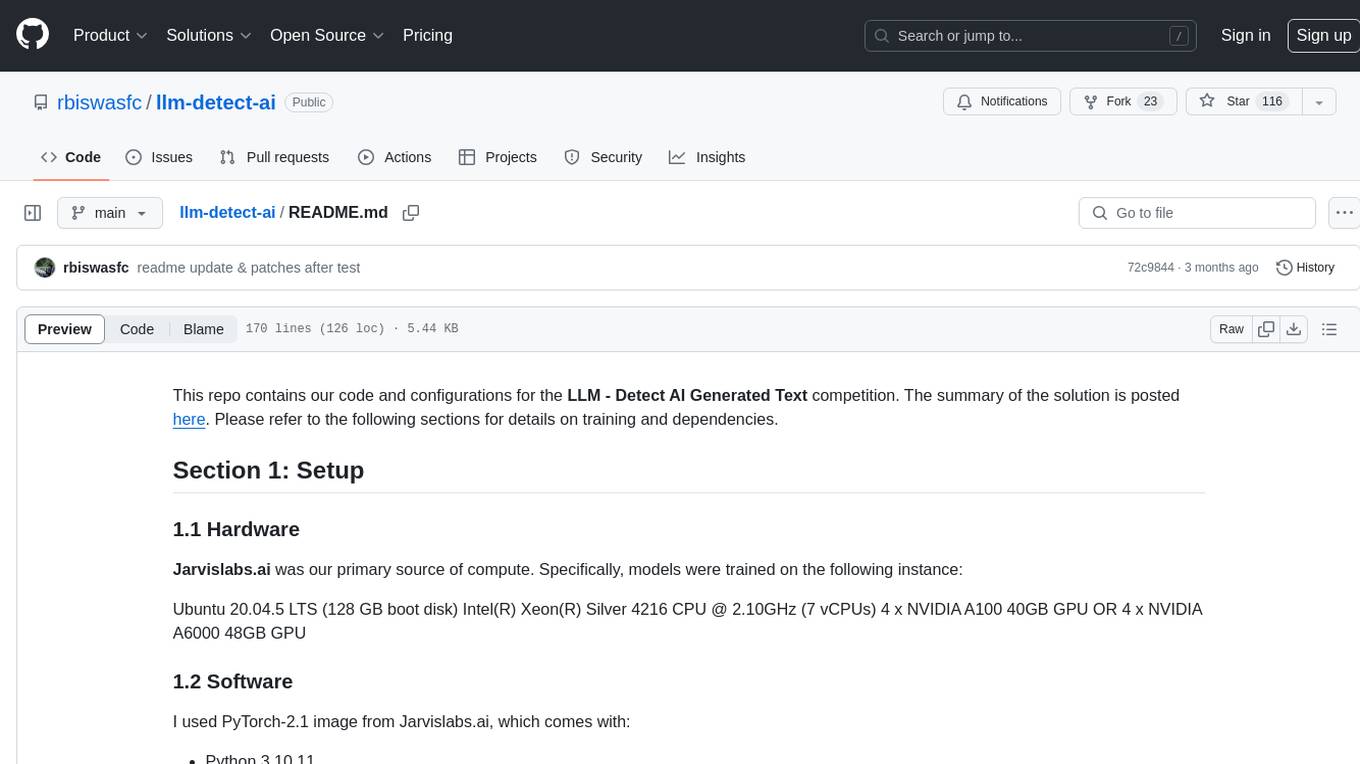
llm-detect-ai
This repository contains code and configurations for the LLM - Detect AI Generated Text competition. It includes setup instructions for hardware, software, dependencies, and datasets. The training section covers scripts and configurations for training LLM models, DeBERTa ranking models, and an embedding model. Text generation section details fine-tuning LLMs using the CLM objective on the PERSUADE corpus to generate student-like essays.
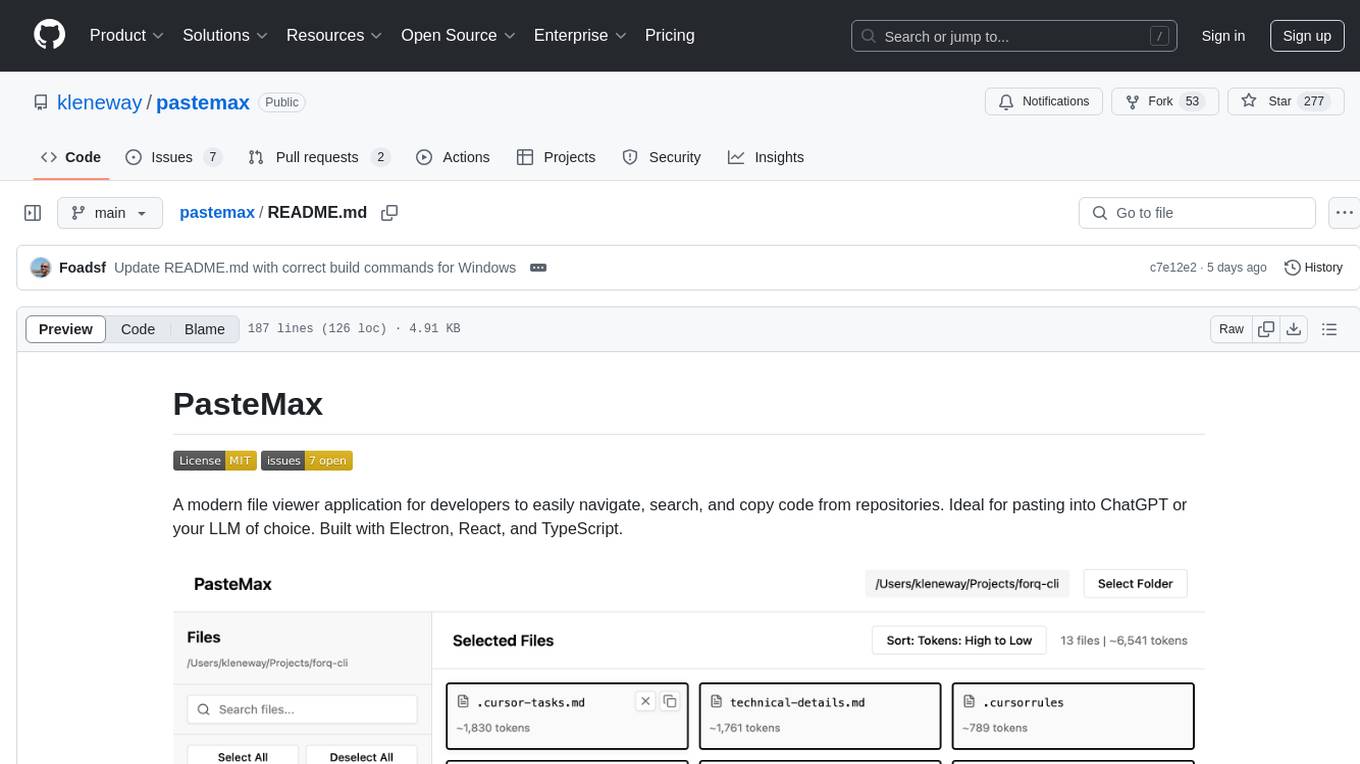
pastemax
PasteMax is a modern file viewer application designed for developers to easily navigate, search, and copy code from repositories. It provides features such as file tree navigation, token counting, search capabilities, selection management, sorting options, dark mode, binary file detection, and smart file exclusion. Built with Electron, React, and TypeScript, PasteMax is ideal for pasting code into ChatGPT or other language models. Users can download the application or build it from source, and customize file exclusions. Troubleshooting steps are provided for common issues, and contributions to the project are welcome under the MIT License.
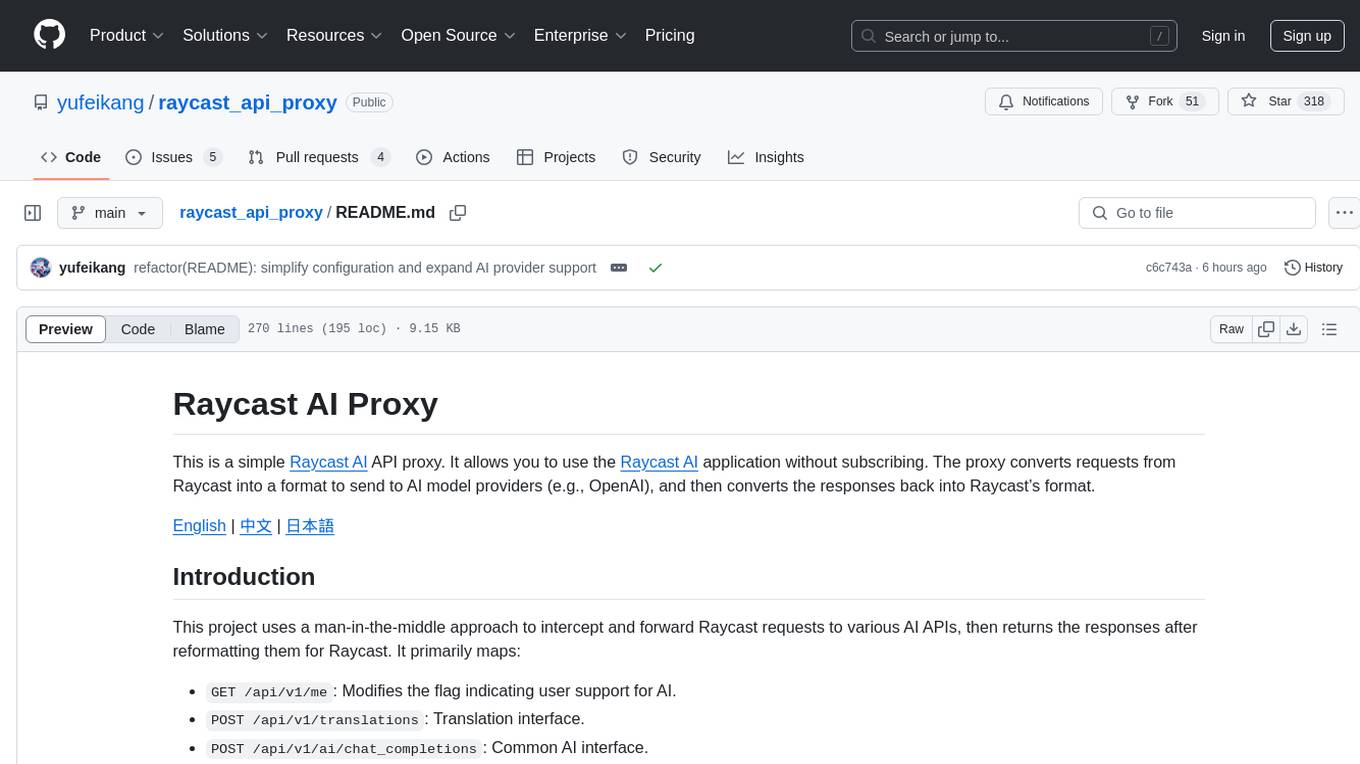
raycast_api_proxy
The Raycast AI Proxy is a tool that acts as a proxy for the Raycast AI application, allowing users to utilize the application without subscribing. It intercepts and forwards Raycast requests to various AI APIs, then reformats the responses for Raycast. The tool supports multiple AI providers and allows for custom model configurations. Users can generate self-signed certificates, add them to the system keychain, and modify DNS settings to redirect requests to the proxy. The tool is designed to work with providers like OpenAI, Azure OpenAI, Google, and more, enabling tasks such as AI chat completions, translations, and image generation.
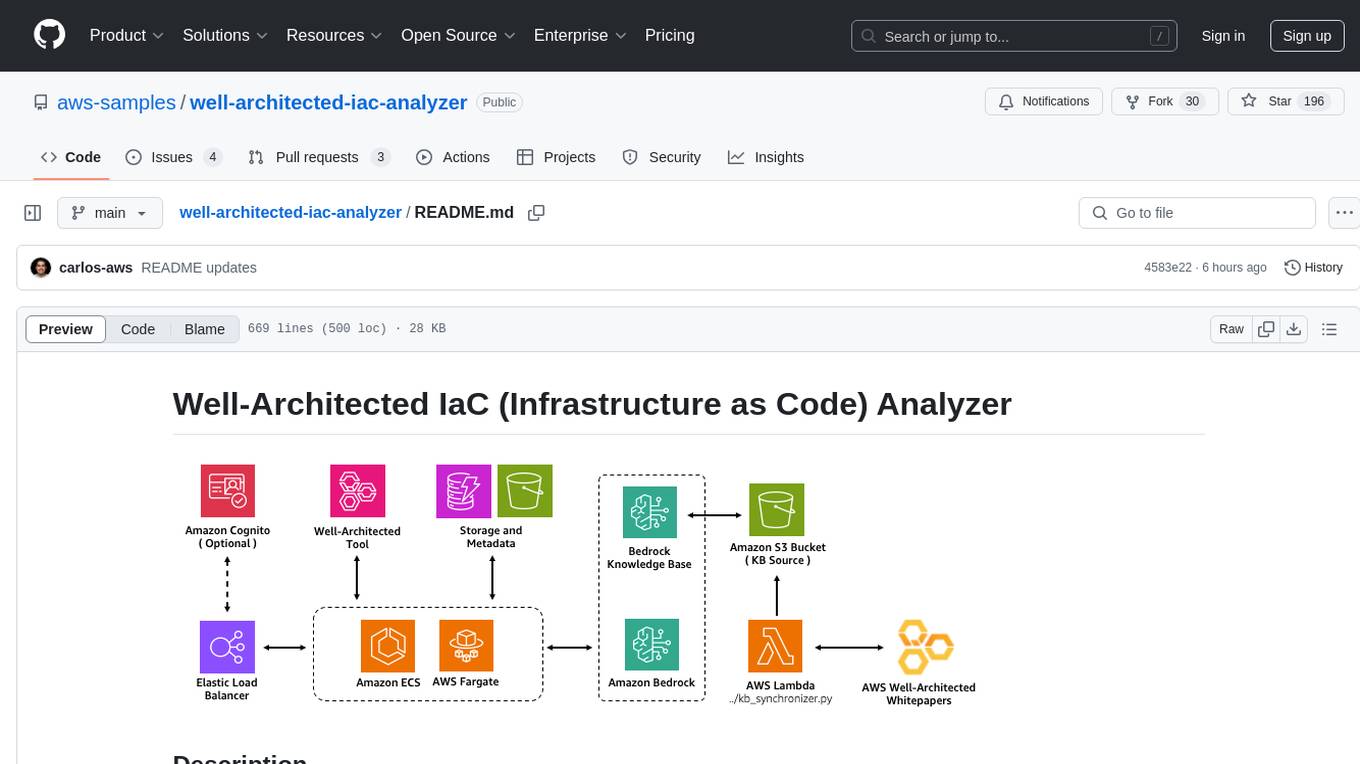
well-architected-iac-analyzer
Well-Architected Infrastructure as Code (IaC) Analyzer is a project demonstrating how generative AI can evaluate infrastructure code for alignment with best practices. It features a modern web application allowing users to upload IaC documents, complete IaC projects, or architecture diagrams for assessment. The tool provides insights into infrastructure code alignment with AWS best practices, offers suggestions for improving cloud architecture designs, and can generate IaC templates from architecture diagrams. Users can analyze CloudFormation, Terraform, or AWS CDK templates, architecture diagrams in PNG or JPEG format, and complete IaC projects with supporting documents. Real-time analysis against Well-Architected best practices, integration with AWS Well-Architected Tool, and export of analysis results and recommendations are included.