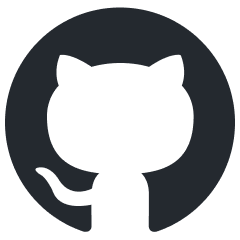
YuLan-Mini
A highly capable 2.4B lightweight LLM using only 1T pre-training data with all details.
Stars: 137
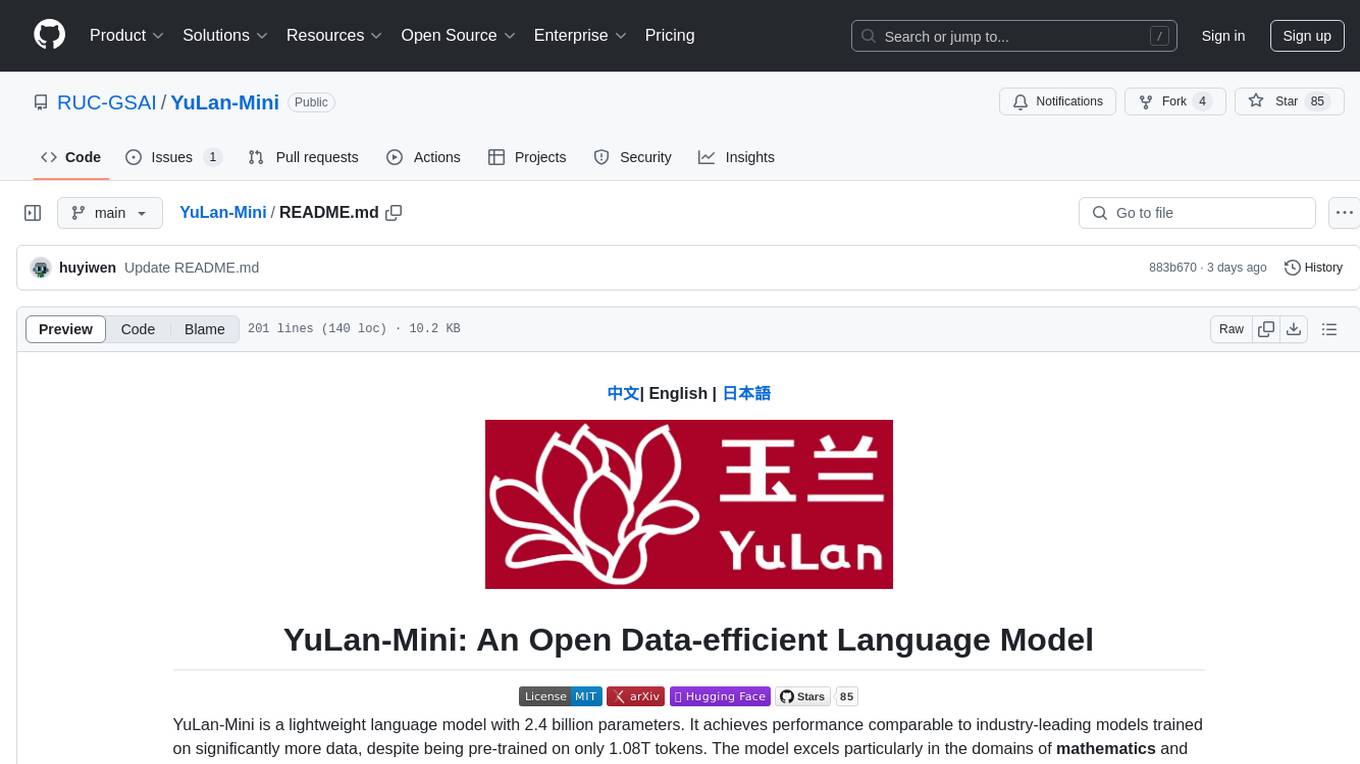
YuLan-Mini is a lightweight language model with 2.4 billion parameters that achieves performance comparable to industry-leading models despite being pre-trained on only 1.08T tokens. It excels in mathematics and code domains. The repository provides pre-training resources, including data pipeline, optimization methods, and annealing approaches. Users can pre-train their own language models, perform learning rate annealing, fine-tune the model, research training dynamics, and synthesize data. The team behind YuLan-Mini is AI Box at Renmin University of China. The code is released under the MIT License with future updates on model weights usage policies. Users are advised on potential safety concerns and ethical use of the model.
README:
YuLan-Mini is a lightweight language model with 2.4 billion parameters. It achieves performance comparable to industry-leading models trained on significantly more data, despite being pre-trained on only 1.08T tokens. The model excels particularly in the domains of mathematics and code. To facilitate reproducibility, we open-source the relevant pre-training resources.
YuLan-Mini is part of the YuLan family, which includes models with larger sizes and different training strategies.
Model | Context Length | SFT | 🤗 Hugging Face | Wise Model |
---|---|---|---|---|
YuLan-Mini (Recommended) | 28K | ❎ | YuLan-Mini |
YuLan-Mini |
YuLan-Mini-2.4B-4K | 4K | ❎ | ||
YuLan-Mini-Instruct | Comming soon | ✅ |
The intermediate checkpoint can be found here
Our pre-training methodology improves training efficiency through three key innovations:
- an elaborately designed data pipeline that combines data cleaning with data schedule strategies;
- a systematic optimization method that can effectively mitigate training instability;
- an effective annealing approach that integrate targeted data selection and long context training.
Note: The model size calculation includes the embedding size.
Models | Model Size | # Train Tokens | Context Length | MATH 500 | GSM 8K | Human Eval | MBPP | RACE Middle | RACE High | RULER |
---|---|---|---|---|---|---|---|---|---|---|
MiniCPM | 2.71B | 1.06T | 4K | 15.00 | 53.83 | 50.00* | 47.31 | 56.61 | 44.27 | N/A |
Qwen-2 | 1.54B | 7T | 128K | 22.60 | 46.90* | 34.80* | 46.90* | 55.77 | 43.69 | 60.16 |
Qwen2.5 | 0.49B | 18T | 128K | 23.60 | 41.60* | 30.50* | 39.30* | 52.36 | 40.31 | 49.23 |
Qwen2.5 | 1.54B | 18T | 128K | 45.40 | 68.50* | 37.20* | 60.20* | 58.77 | 44.33 | 68.26 |
Gemma2 | 2.61B | 2T | 8K | 18.30* | 30.30* | 19.50* | 42.10* | - | - | N/A |
StableLM2 | 1.64B | 2T | 4K | - | 20.62 | 8.50* | 17.50 | 56.33 | 45.06 | N/A |
SmolLM2 | 1.71B | 11T | 8K | 11.80 | - | 23.35 | 45.00 | 55.77 | 43.06 | N/A |
Llama3.2 | 3.21B | 9T | 128K | 7.40 | - | 29.30 | 49.70 | 55.29 | 43.34 | 77.06 |
YuLan-Mini | 2.42B | 1.04T | 4K | 32.60 | 66.65 | 61.60 | 66.70 | 55.71 | 43.58 | N/A |
YuLan-Mini | 2.42B | 1.08T | 28K | 37.80 | 68.46 | 64.00 | 65.90 | 57.18 | 44.57 | 51.48 |
Models | LAMBADA | MMLU | CMMLU | CEval | HellaSwag | WinoGrande | StoryCloze | ARC-e | ARC-c |
---|---|---|---|---|---|---|---|---|---|
MiniCPM-2.71B | 61.91 | 53.37 | 48.97 | 48.24 | 67.92 | 65.74 | 78.51 | 55.51 | 43.86 |
Qwen2-1.54B | 64.68 | 55.90 | 70.76 | 71.94 | 66.11 | 66.14 | 77.60 | 62.21 | 42.92 |
Qwen2.5-0.49B | 52.00 | 47.50 | 52.17 | 54.27 | 50.54 | 55.88 | 71.67 | 56.10 | 39.51 |
Qwen2.5-1.54B | 62.12 | 60.71 | 67.82 | 69.05 | 67.18 | 64.48 | 76.80 | 71.51 | 53.41 |
Gemma2-2.61B | - | 52.20* | - | 28.00* | 74.60* | 71.50* | - | - | 55.70* |
StableLM2-1.64B | 66.15 | 40.37 | 29.29 | 26.99 | 69.79 | 64.64 | 78.56 | 54.00 | 40.78 |
SmolLM2-1.71B | 67.42 | 51.91 | 33.46 | 35.10 | 72.96 | 67.40 | 79.32 | 44.82 | 35.49 |
Llama3.2-3.21B | 69.08 | 63.40 | 44.44 | 44.49 | 75.62 | 67.48 | 76.80 | 70.12 | 48.81 |
YuLan-Mini-2.42B-4K | 64.72 | 51.79 | 48.35 | 51.47 | 68.65 | 67.09 | 76.37 | 69.87 | 50.51 |
YuLan-Mini-2.42B-28K | 65.67 | 49.10 | 45.45 | 48.23 | 67.22 | 67.24 | 75.89 | 67.47 | 49.32 |
To enhance research transparency and reproducibility, we are open-sourcing relevant pre-training resources:
1. Pre-training and Evaluation Code
The pre-training code can be found here. Note that due to subsequent code modifications, this code may not run directly and may require some adjustments.
Due to the implementation of Hugging Face Trainer, certain parameters are stored in the config.json
file and cannot be modified through the Trainer's command-line arguments. Therefore, you need to update these parameters in the config.json
file first, particularly:
-
save_steps
: The frequency of saving intermediate checkpoints. -
train_batch_size
: The batch size per GPU (equivalent toper_device_train_batch_size
in the Trainer). We used a batch size of 1008 (approximately 4M tokens) during the stable training stage. Maintaining this same batch size is equally important for training effectiveness.
Below is an example of a properly configured config.json
file:
{
"best_metric": null,
"best_model_checkpoint": null,
"epoch": 0.0,
"eval_steps": 500,
"global_step": 0,
"is_hyper_param_search": false,
"is_local_process_zero": true,
"is_world_process_zero": true,
"log_history": [],
"logging_steps": 3,
"max_steps": 0,
"num_input_tokens_seen": 0,
"num_train_epochs": 0,
"save_steps": 250,
"stateful_callbacks": {
"TrainerControl": {
"args": {
"should_epoch_stop": false,
"should_evaluate": false,
"should_log": false,
"should_save": true,
"should_training_stop": true
},
"attributes": {}
}
},
"total_flos": 0,
"train_batch_size": 3,
"trial_name": null,
"trial_params": null
}
To ensure DeepSpeed Integration loads the Universal Checkpoint, you need to enable this feature in the DeepSpeed configuration JSON file.
Here is an example of a ZeRO2 configuration with Universal Checkpointing enabled:
{
"bf16": {
"enabled": "auto"
},
"zero_optimization": {
"stage": 2,
"allgather_partitions": true,
"allgather_bucket_size": 8e8,
"overlap_comm": true,
"reduce_scatter": true,
"reduce_bucket_size": 8e8,
"contiguous_gradients": true
},
"gradient_accumulation_steps": "auto",
"gradient_clipping": "auto",
"steps_per_print": 16,
"train_batch_size": "auto",
"train_micro_batch_size_per_gpu": "auto",
"wall_clock_breakdown": false,
"dump_state": true,
"optimizer": {
"type": "AdamW",
"params": {
"lr": "auto",
"betas": "auto",
"eps": "auto",
"weight_decay": "auto"
}
},
"checkpoint": {
"load_universal": true
}
}
When calling trainer.train
, include the resume_from_checkpoint
argument to load the distributed optimizer state from the Universal Checkpoint and resume training.
trainer.train(resume_from_checkpoint=training_args.resume_from_checkpoint)
We provide an internal training framework for your reference, but you are free to choose other frameworks.
2. Intermediate Stage Checkpoints
The intermediate stage checkpoints are released in YuLan-Mini.Stage | Curriculum Phase | 4K Context | 28K Context | Optimizer | Inference Architecture | LAMBADA Acc
|
GSM8K Acc
|
HumanEval pass@1
|
---|---|---|---|---|---|---|---|---|
Stable | 5 | YuLan-Mini-Phase5 | yulanmini |
53.85 | 3.41 | 12.26 | ||
Stable | 10 | YuLan-Mini-Phase10 | yulanmini |
55.00 | 9.57 | 15.95 | ||
Stable | 15 | YuLan-Mini-Phase15 | yulanmini |
55.81 | 13.81 | 16.99 | ||
Stable | 20 | YuLan-Mini-Phase20 | ✅ | yulanmini |
55.81 | 21.39 | 20.79 | |
Stable | 25 (1T tokens) | YuLan-Mini-Before-Annealing | ✅ | yulanmini |
55.67 | 29.94 | 34.06 | |
Annealing | 26 | YuLan-Mini-4K |
llama * |
64.72 | 66.65 | 61.60 | ||
Annealing | 27 | YuLan-Mini |
llama * |
65.67 | 68.46 | 64.00 |
*: For easier inference and deployment, we merged the re-parameterized added parameters and scaling factors into the final released models (YuLan-Mini and YuLan-Mini-Intermediate-4K), enabling it to run on the Llama architecture. However, these parameters are still retained in the intermediate checkpoints from the training process.
3. Optimizer States Before Annealing
4. The Used Open-Source Datasets
6. Synthetic Data
Data cleaning and synthesis pipeline:
The synthetic data we are using is released in YuLan-Mini-Datasets
- Pre-train your own LLM. You can use our data and curriculum to train a model that's just as powerful as YuLan-Mini.
- Perform your own learning rate annealing. During the annealing phase, YuLan-Mini's learning ability is at its peak. You can resume training from the checkpoint before annealing and use your own dataset for learning rate annealing.
- Fine-tune the Instruct version of the LLM. You can use the YuLan-Mini base model to train your own Instruct version.
- Training dynamics research. You can use YuLan-Mini's intermediate checkpoints to explore internal changes during the pre-training process.
- Synthesize your own data. You can use YuLan-Mini's data pipeline to clean and generate your own dataset.
Below is a simple example for inference using Huggingface:
Huggingface Inference Example
import torch
from transformers import AutoTokenizer, AutoModelForCausalLM
# Load model and tokenizer
tokenizer = AutoTokenizer.from_pretrained("yulan-team/YuLan-Mini")
model = AutoModelForCausalLM.from_pretrained("yulan-team/YuLan-Mini", torch_dtype=torch.bfloat16)
# Input text
input_text = "Renmin University of China is"
inputs = tokenizer(input_text, return_tensors="pt")
# Completion
output = model.generate(inputs["input_ids"], max_new_tokens=100)
print(tokenizer.decode(output[0], skip_special_tokens=True))
vLLM Serve Example
vllm serve yulan-team/YuLan-Mini --dtype bfloat16
SGLang Serve Example
python -m sglang.launch_server --model-path yulan-team/YuLan-Mini --port 30000 --host 0.0.0.0
YuLan-Mini is developed and maintained by AI Box, Renmin University of China.
- The code in this repository, the model weights, and optimizer states are released under the MIT License.
- Policies regarding the use of model weights, intermediate optimizer states, and training data will be announced in future updates.
- Limitations: Despite our efforts to mitigate safety concerns and encourage the generation of ethical and lawful text, the probabilistic nature of language models may still lead to unexpected outputs. For instance, responses might contain bias, discrimination, or other harmful content. Please refrain from disseminating such content. We are not liable for any consequences arising from the spread of harmful information.
If you find YuLan-Mini helpful for your research or development, please cite our technical report:
@misc{hu2024yulanmini,
title={YuLan-Mini: An Open Data-efficient Language Model},
author={Yiwen Hu and Huatong Song and Jia Deng and Jiapeng Wang and Jie Chen and Kun Zhou and Yutao Zhu and Jinhao Jiang and Zican Dong and Wayne Xin Zhao and Ji-Rong Wen},
year={2024},
eprint={2412.17743},
archivePrefix={arXiv},
primaryClass={cs.CL},
url={https://arxiv.org/abs/2412.17743},
}
For Tasks:
Click tags to check more tools for each tasksFor Jobs:
Alternative AI tools for YuLan-Mini
Similar Open Source Tools
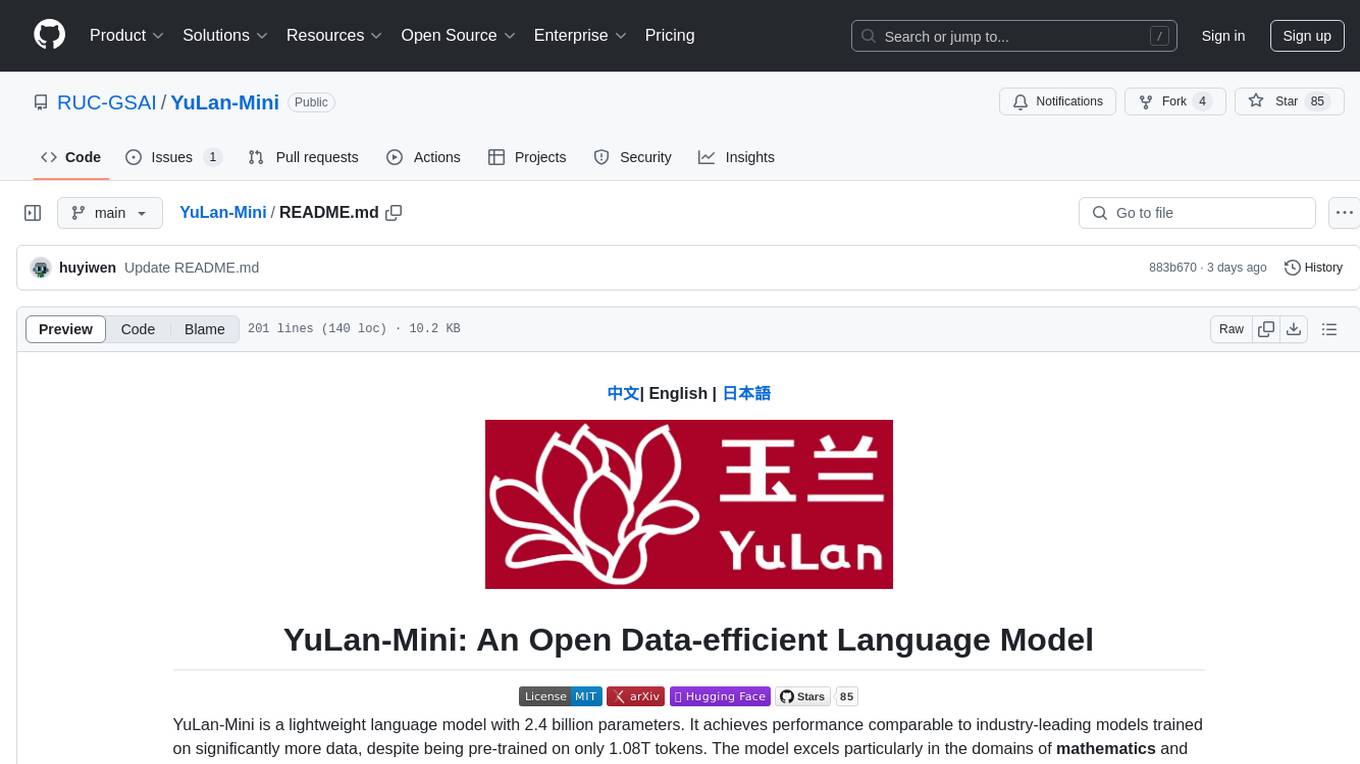
YuLan-Mini
YuLan-Mini is a lightweight language model with 2.4 billion parameters that achieves performance comparable to industry-leading models despite being pre-trained on only 1.08T tokens. It excels in mathematics and code domains. The repository provides pre-training resources, including data pipeline, optimization methods, and annealing approaches. Users can pre-train their own language models, perform learning rate annealing, fine-tune the model, research training dynamics, and synthesize data. The team behind YuLan-Mini is AI Box at Renmin University of China. The code is released under the MIT License with future updates on model weights usage policies. Users are advised on potential safety concerns and ethical use of the model.
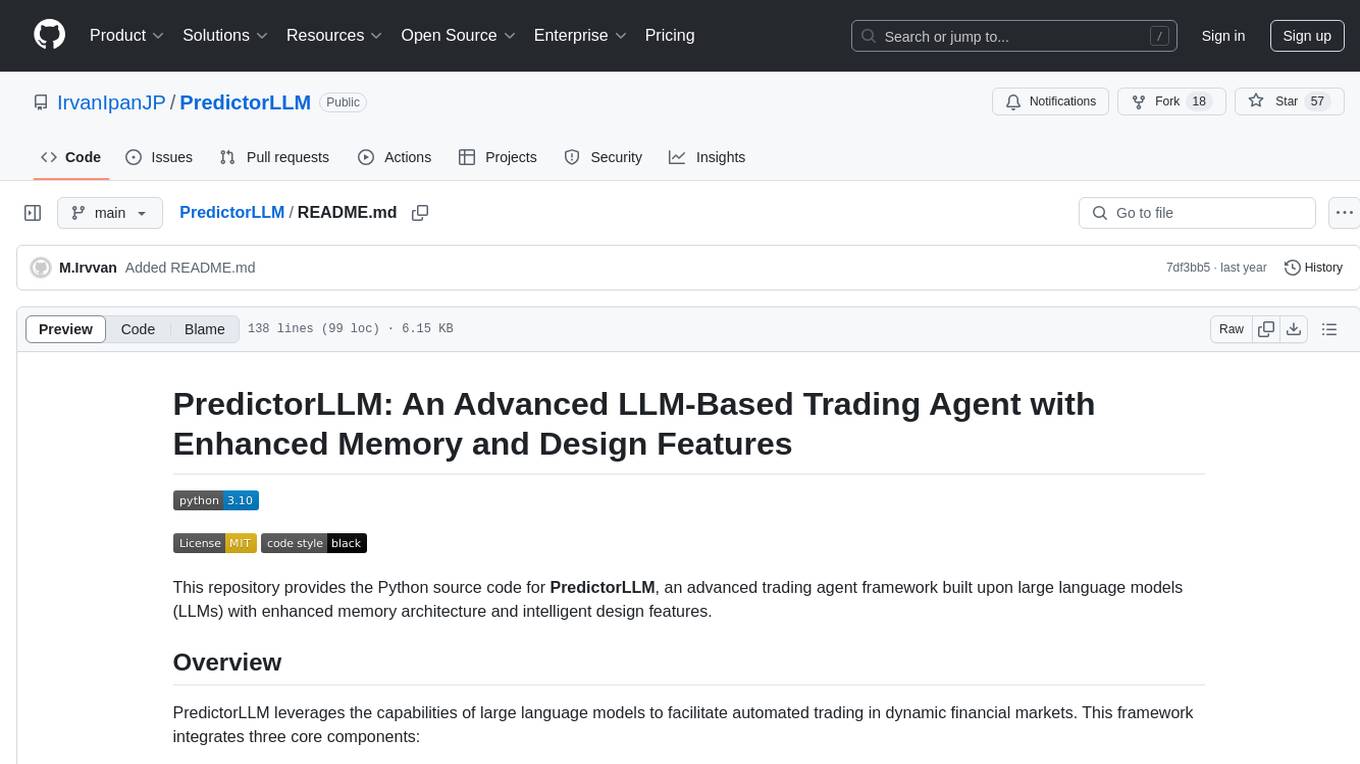
PredictorLLM
PredictorLLM is an advanced trading agent framework that utilizes large language models to automate trading in financial markets. It includes a profiling module to establish agent characteristics, a layered memory module for retaining and prioritizing financial data, and a decision-making module to convert insights into trading strategies. The framework mimics professional traders' behavior, surpassing human limitations in data processing and continuously evolving to adapt to market conditions for superior investment outcomes.
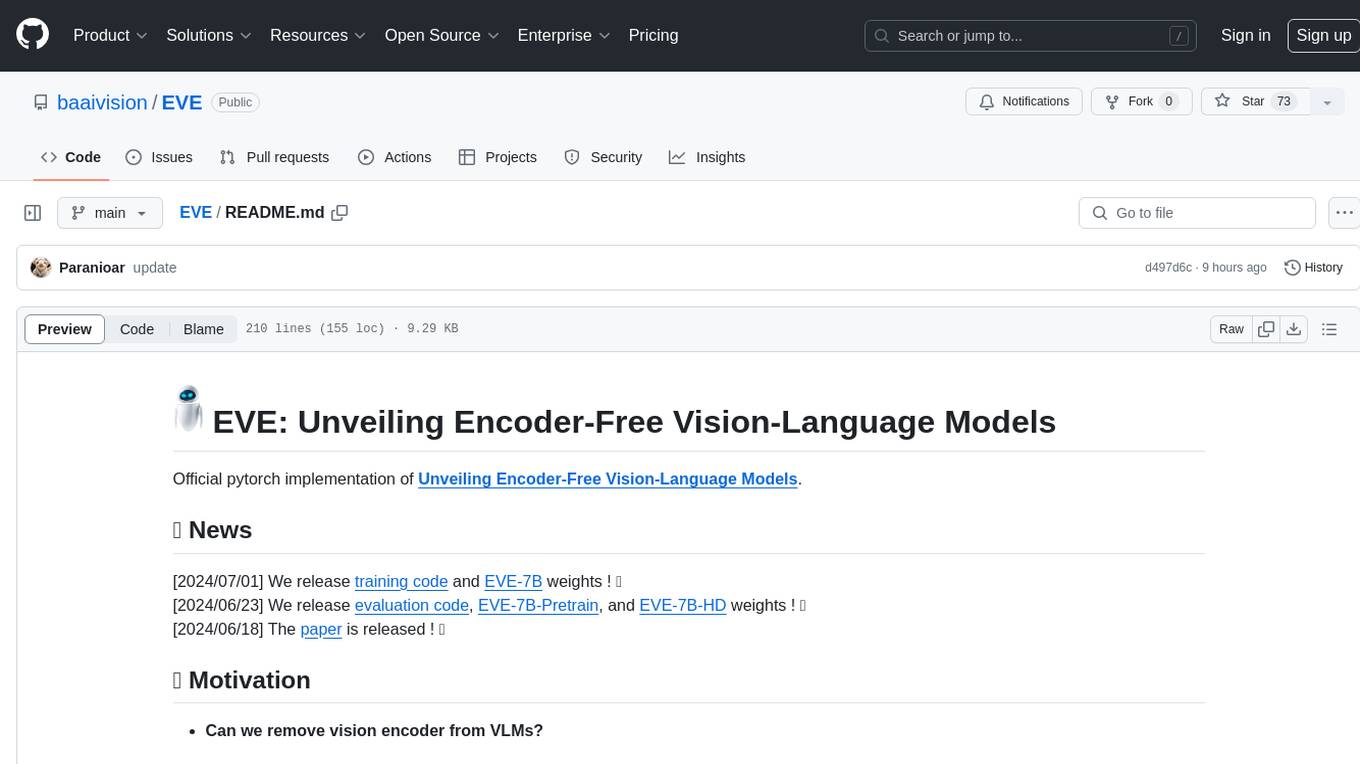
EVE
EVE is an official PyTorch implementation of Unveiling Encoder-Free Vision-Language Models. The project aims to explore the removal of vision encoders from Vision-Language Models (VLMs) and transfer LLMs to encoder-free VLMs efficiently. It also focuses on bridging the performance gap between encoder-free and encoder-based VLMs. EVE offers a superior capability with arbitrary image aspect ratio, data efficiency by utilizing publicly available data for pre-training, and training efficiency with a transparent and practical strategy for developing a pure decoder-only architecture across modalities.
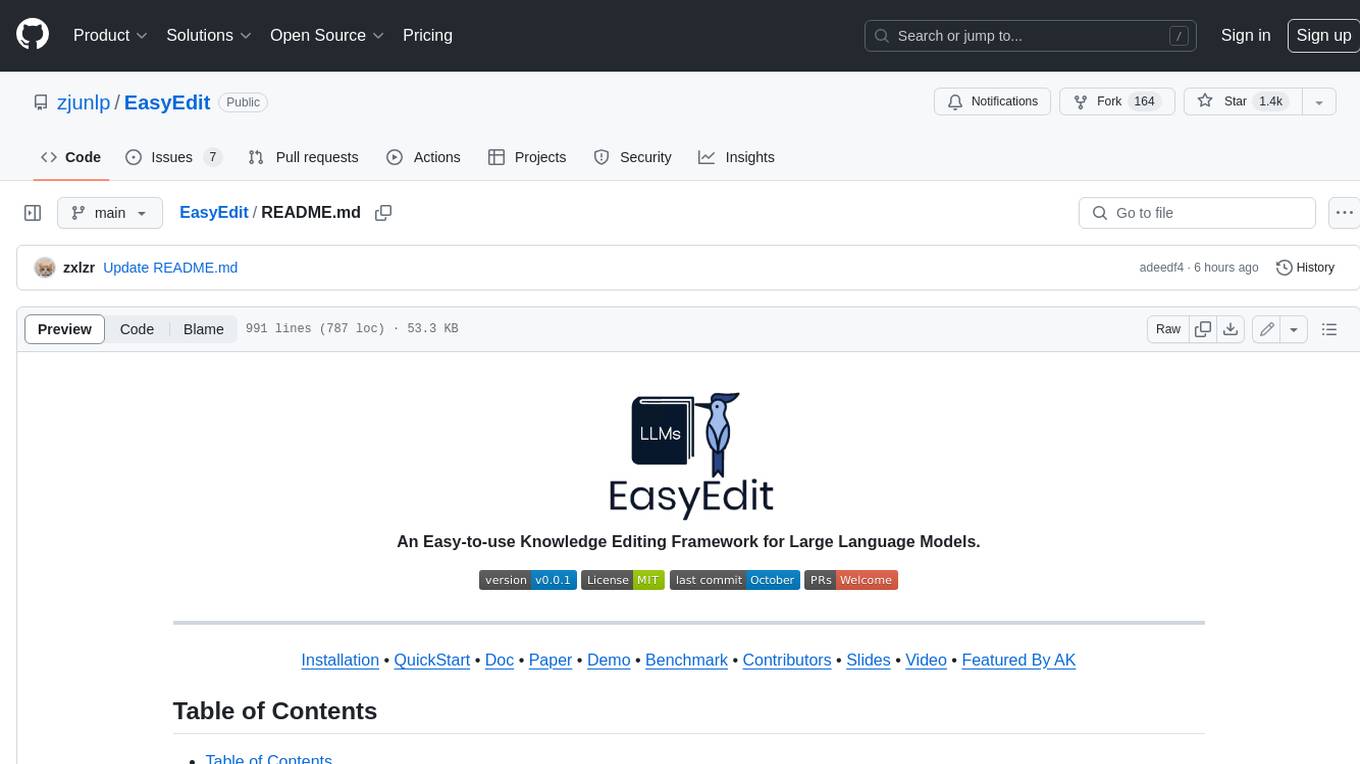
EasyEdit
EasyEdit is a Python package for edit Large Language Models (LLM) like `GPT-J`, `Llama`, `GPT-NEO`, `GPT2`, `T5`(support models from **1B** to **65B**), the objective of which is to alter the behavior of LLMs efficiently within a specific domain without negatively impacting performance across other inputs. It is designed to be easy to use and easy to extend.
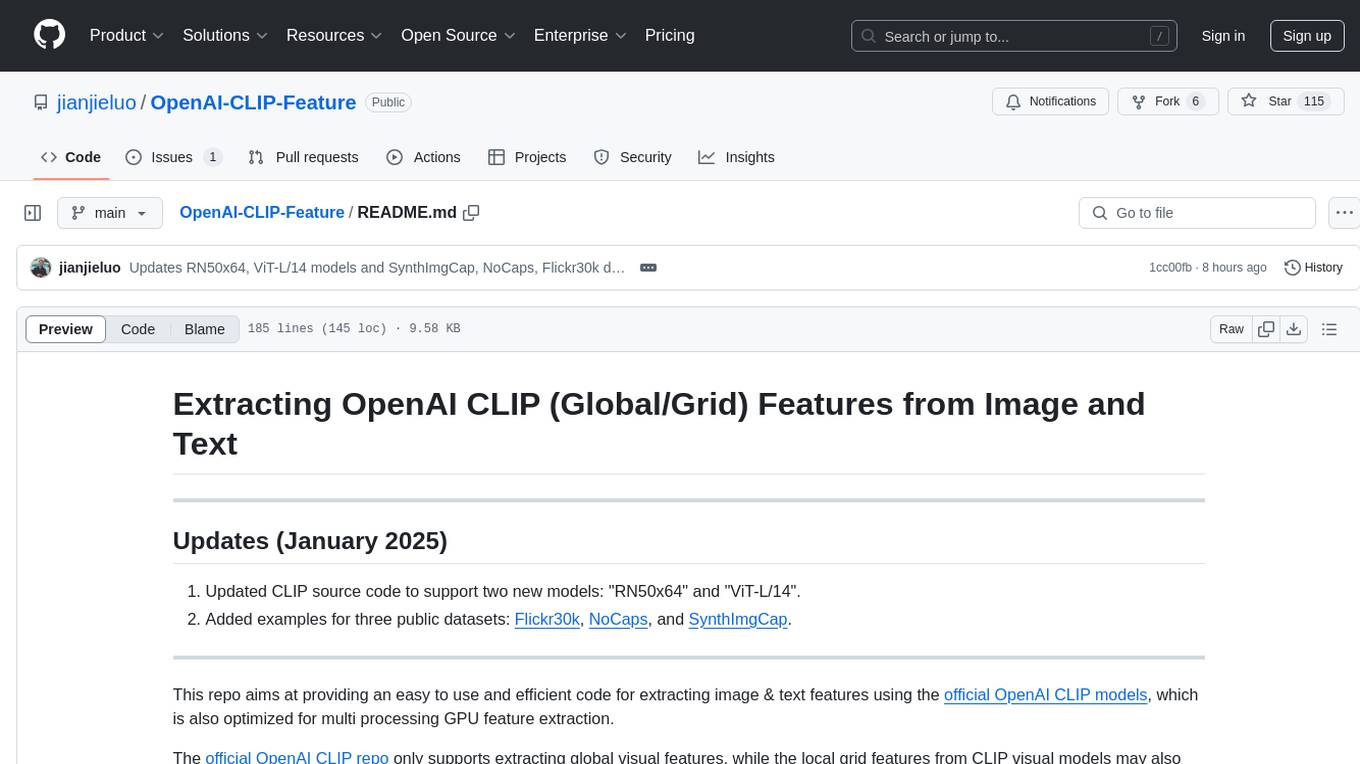
OpenAI-CLIP-Feature
This repository provides code for extracting image and text features using OpenAI CLIP models, supporting both global and local grid visual features. It aims to facilitate multi visual-and-language downstream tasks by allowing users to customize input and output grid resolution easily. The extracted features have shown comparable or superior results in image captioning tasks without hyperparameter tuning. The repo supports various CLIP models and provides detailed information on supported settings and results on MSCOCO image captioning. Users can get started by setting up experiments with the extracted features using X-modaler.
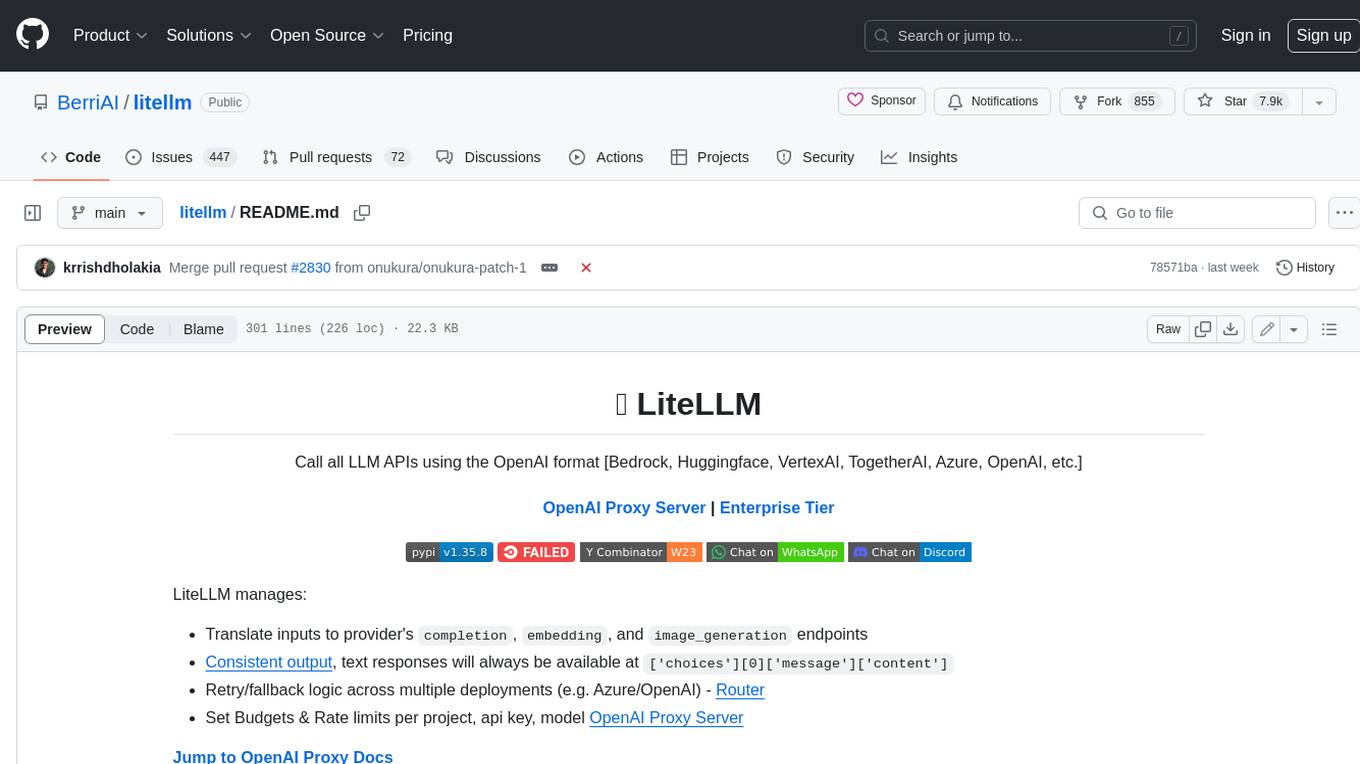
litellm
LiteLLM is a tool that allows you to call all LLM APIs using the OpenAI format. This includes Bedrock, Huggingface, VertexAI, TogetherAI, Azure, OpenAI, and more. LiteLLM manages translating inputs to provider's `completion`, `embedding`, and `image_generation` endpoints, providing consistent output, and retry/fallback logic across multiple deployments. It also supports setting budgets and rate limits per project, api key, and model.
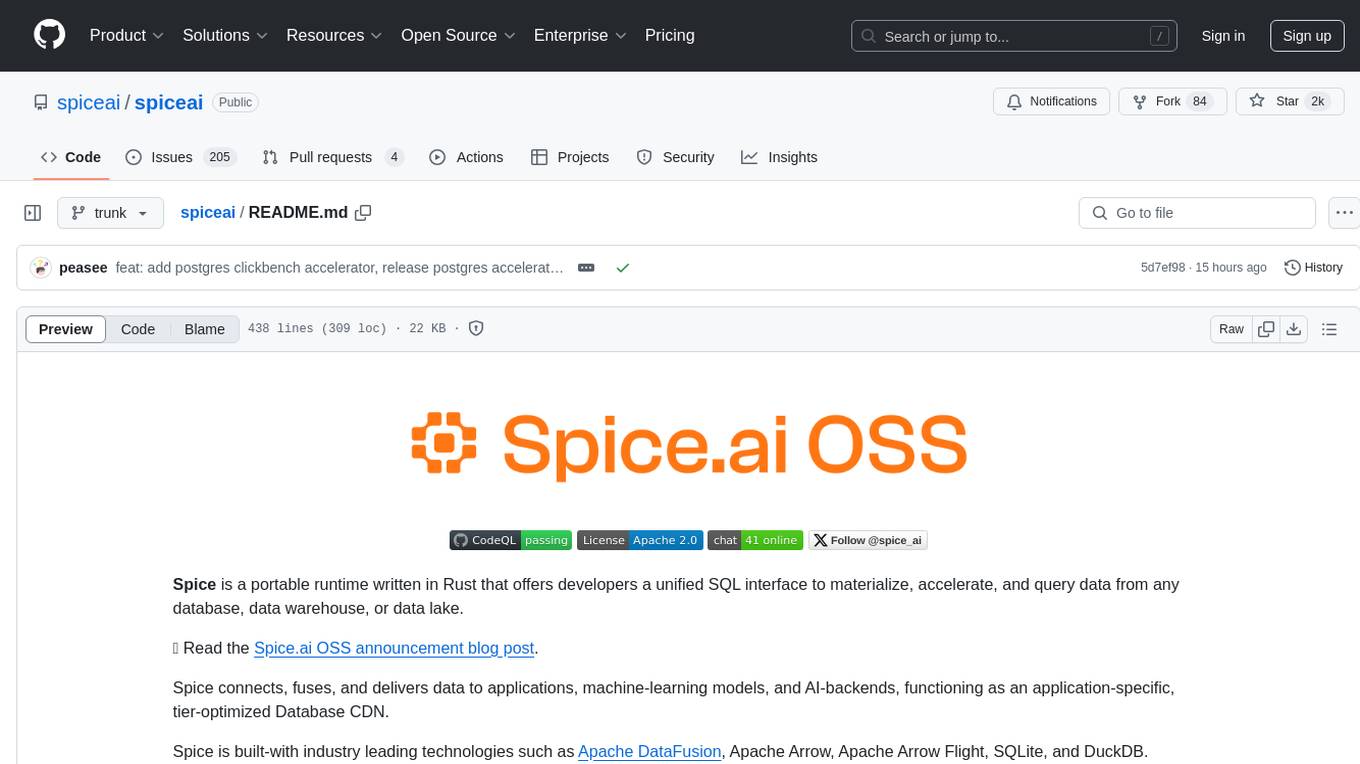
spiceai
Spice is a portable runtime written in Rust that offers developers a unified SQL interface to materialize, accelerate, and query data from any database, data warehouse, or data lake. It connects, fuses, and delivers data to applications, machine-learning models, and AI-backends, functioning as an application-specific, tier-optimized Database CDN. Built with industry-leading technologies such as Apache DataFusion, Apache Arrow, Apache Arrow Flight, SQLite, and DuckDB. Spice makes it fast and easy to query data from one or more sources using SQL, co-locating a managed dataset with applications or machine learning models, and accelerating it with Arrow in-memory, SQLite/DuckDB, or attached PostgreSQL for fast, high-concurrency, low-latency queries.
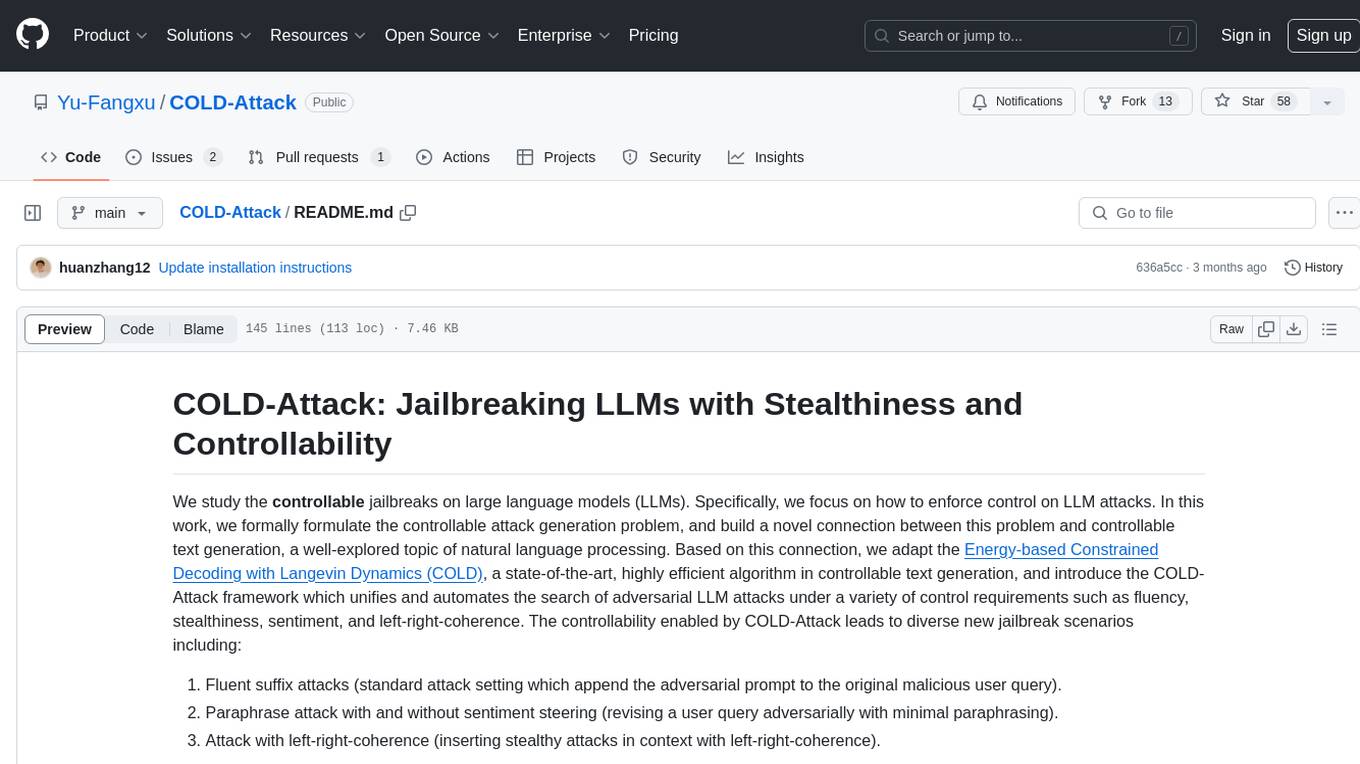
COLD-Attack
COLD-Attack is a framework designed for controllable jailbreaks on large language models (LLMs). It formulates the controllable attack generation problem and utilizes the Energy-based Constrained Decoding with Langevin Dynamics (COLD) algorithm to automate the search of adversarial LLM attacks with control over fluency, stealthiness, sentiment, and left-right-coherence. The framework includes steps for energy function formulation, Langevin dynamics sampling, and decoding process to generate discrete text attacks. It offers diverse jailbreak scenarios such as fluent suffix attacks, paraphrase attacks, and attacks with left-right-coherence.
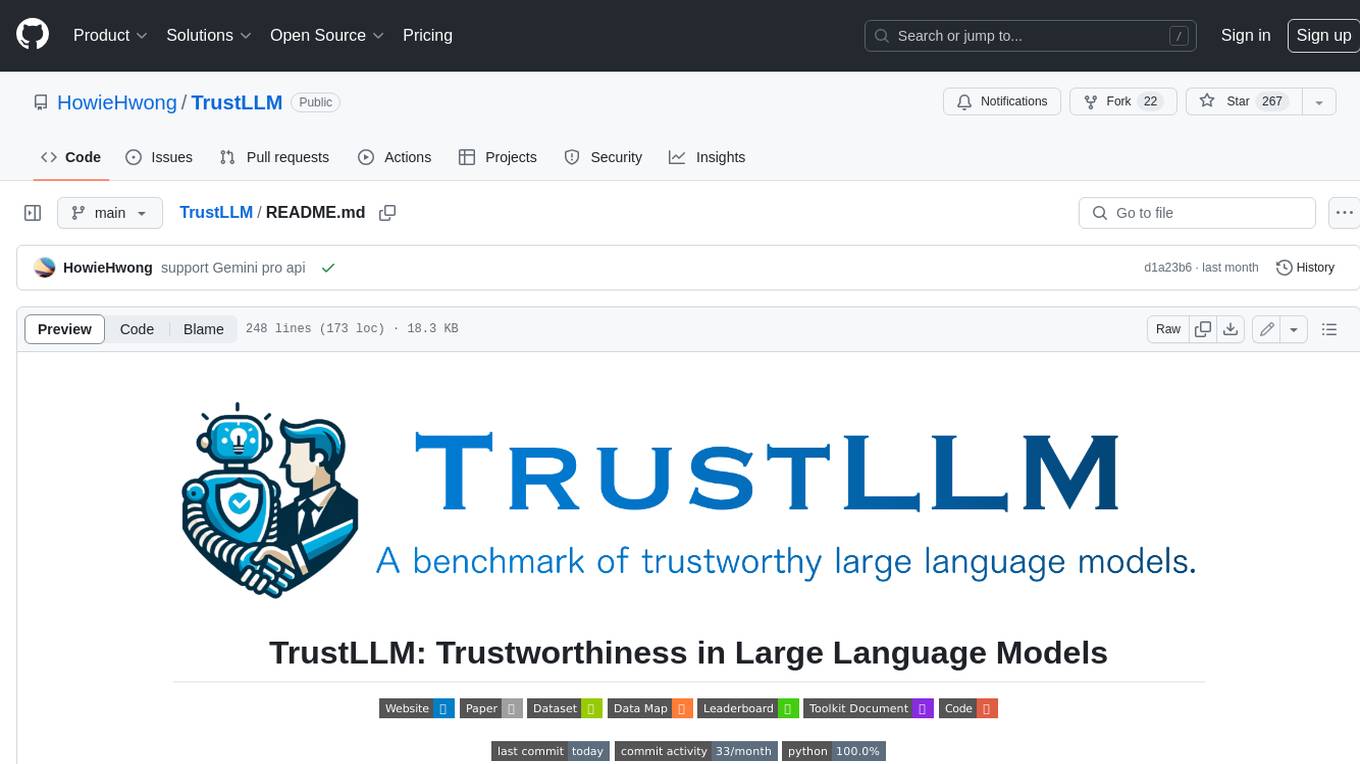
TrustLLM
TrustLLM is a comprehensive study of trustworthiness in LLMs, including principles for different dimensions of trustworthiness, established benchmark, evaluation, and analysis of trustworthiness for mainstream LLMs, and discussion of open challenges and future directions. Specifically, we first propose a set of principles for trustworthy LLMs that span eight different dimensions. Based on these principles, we further establish a benchmark across six dimensions including truthfulness, safety, fairness, robustness, privacy, and machine ethics. We then present a study evaluating 16 mainstream LLMs in TrustLLM, consisting of over 30 datasets. The document explains how to use the trustllm python package to help you assess the performance of your LLM in trustworthiness more quickly. For more details about TrustLLM, please refer to project website.
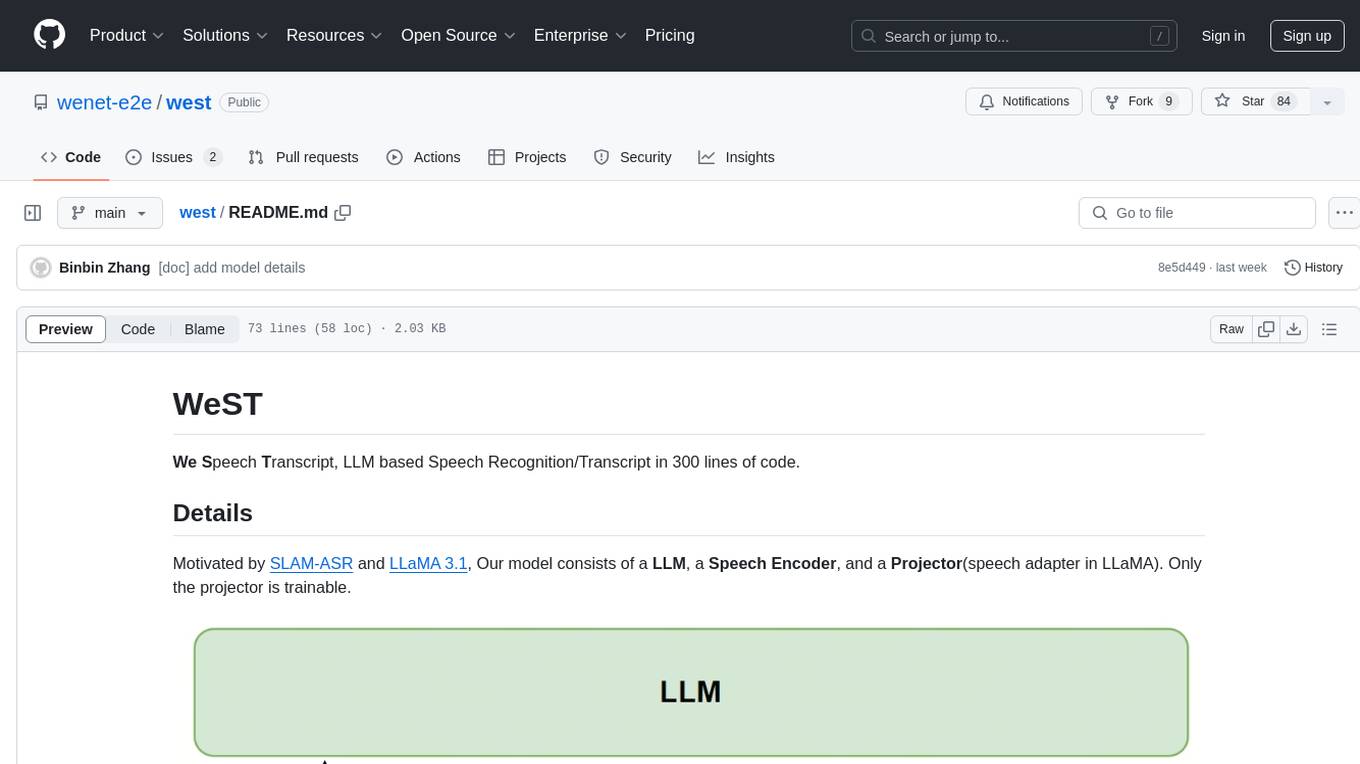
west
WeST is a Speech Recognition/Transcript tool developed in 300 lines of code, inspired by SLAM-ASR and LLaMA 3.1. The model includes a Language Model (LLM), a Speech Encoder, and a trainable Projector. It requires training data in jsonl format with 'wav' and 'txt' entries. WeST can be used for training and decoding speech recognition models.
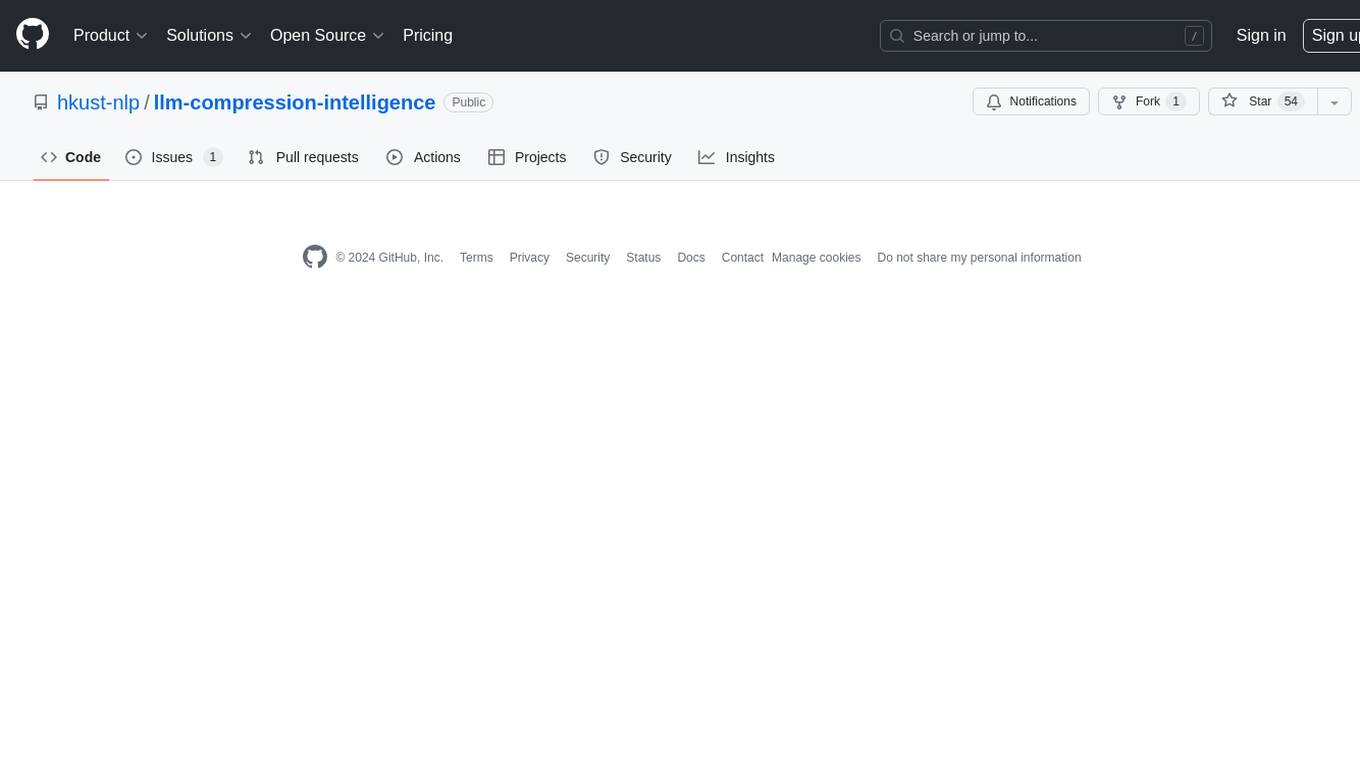
llm-compression-intelligence
This repository presents the findings of the paper "Compression Represents Intelligence Linearly". The study reveals a strong linear correlation between the intelligence of LLMs, as measured by benchmark scores, and their ability to compress external text corpora. Compression efficiency, derived from raw text corpora, serves as a reliable evaluation metric that is linearly associated with model capabilities. The repository includes the compression corpora used in the paper, code for computing compression efficiency, and data collection and processing pipelines.
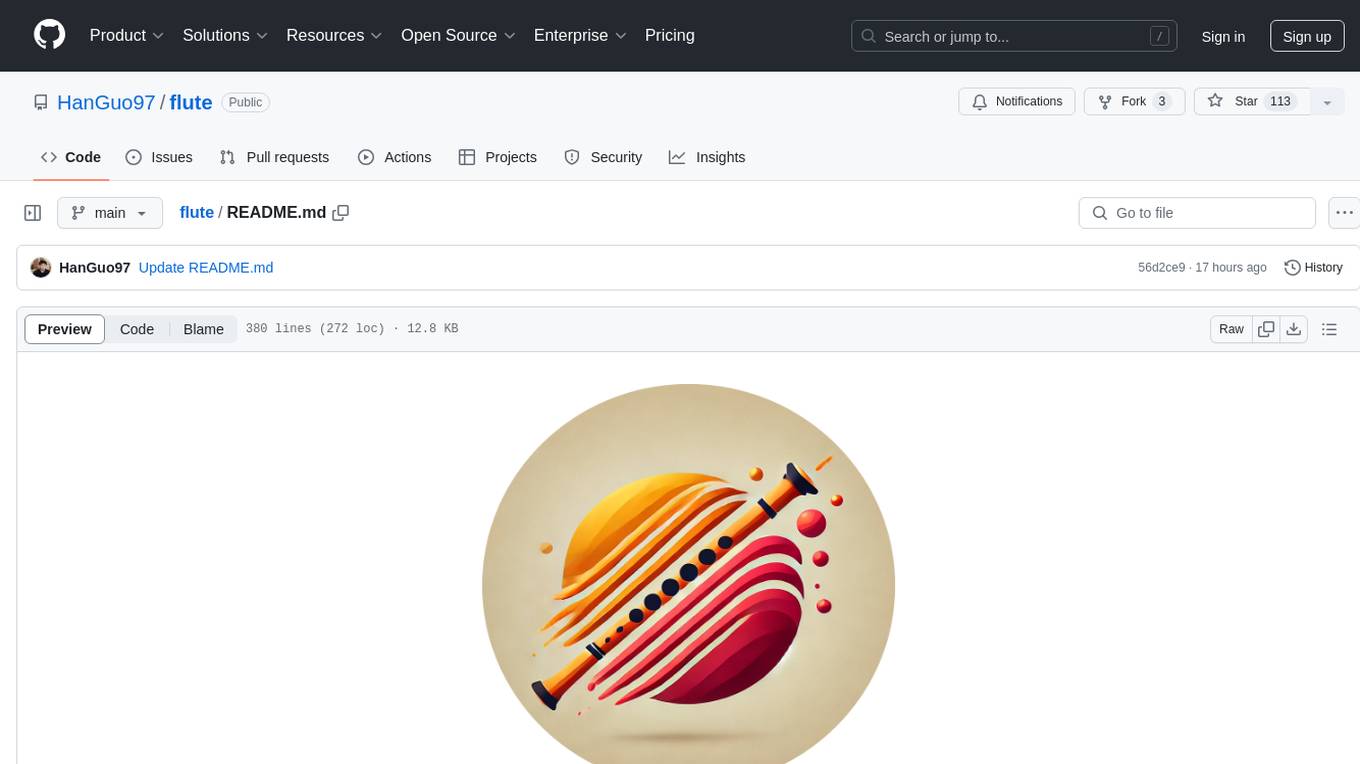
flute
FLUTE (Flexible Lookup Table Engine for LUT-quantized LLMs) is a tool designed for uniform quantization and lookup table quantization of weights in lower-precision intervals. It offers flexibility in mapping intervals to arbitrary values through a lookup table. FLUTE supports various quantization formats such as int4, int3, int2, fp4, fp3, fp2, nf4, nf3, nf2, and even custom tables. The tool also introduces new quantization algorithms like Learned Normal Float (NFL) for improved performance and calibration data learning. FLUTE provides benchmarks, model zoo, and integration with frameworks like vLLM and HuggingFace for easy deployment and usage.
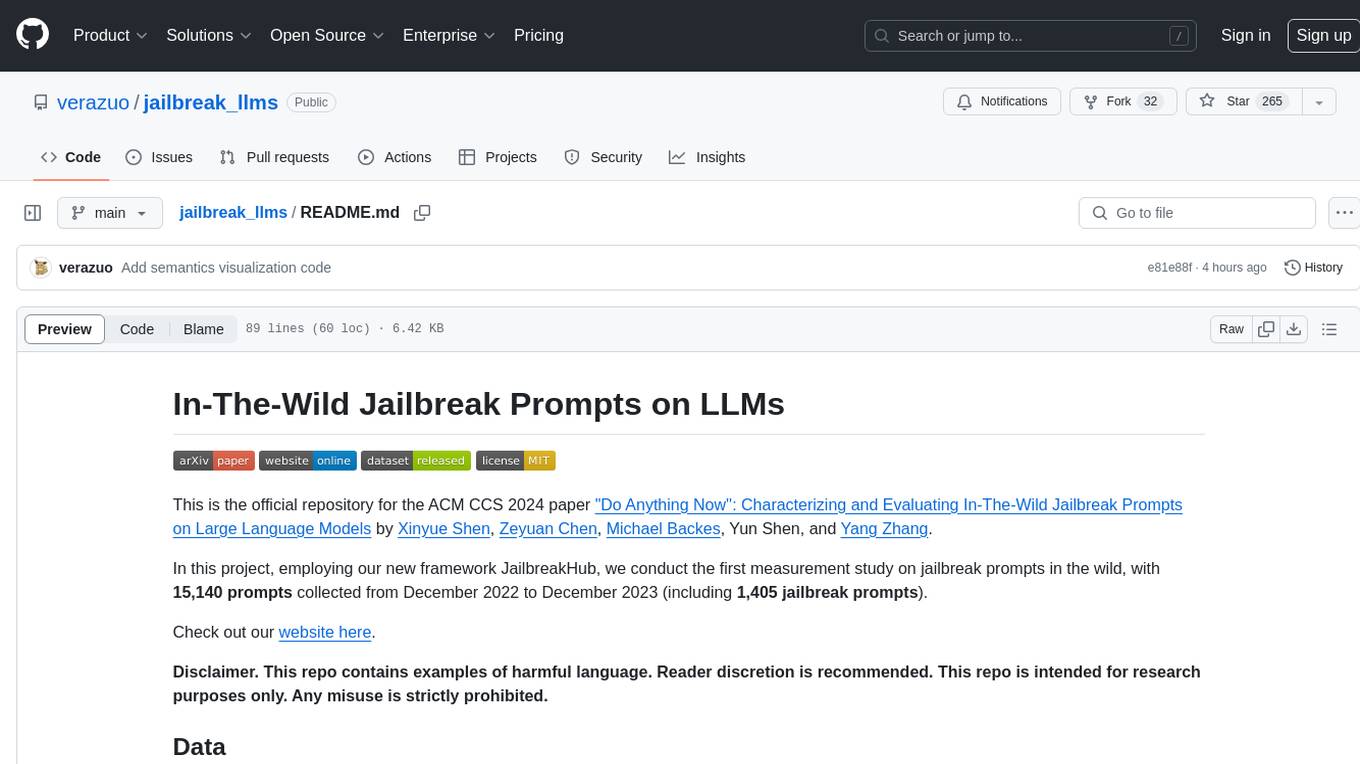
jailbreak_llms
This is the official repository for the ACM CCS 2024 paper 'Do Anything Now': Characterizing and Evaluating In-The-Wild Jailbreak Prompts on Large Language Models. The project employs a new framework called JailbreakHub to conduct the first measurement study on jailbreak prompts in the wild, collecting 15,140 prompts from December 2022 to December 2023, including 1,405 jailbreak prompts. The dataset serves as the largest collection of in-the-wild jailbreak prompts. The repository contains examples of harmful language and is intended for research purposes only.
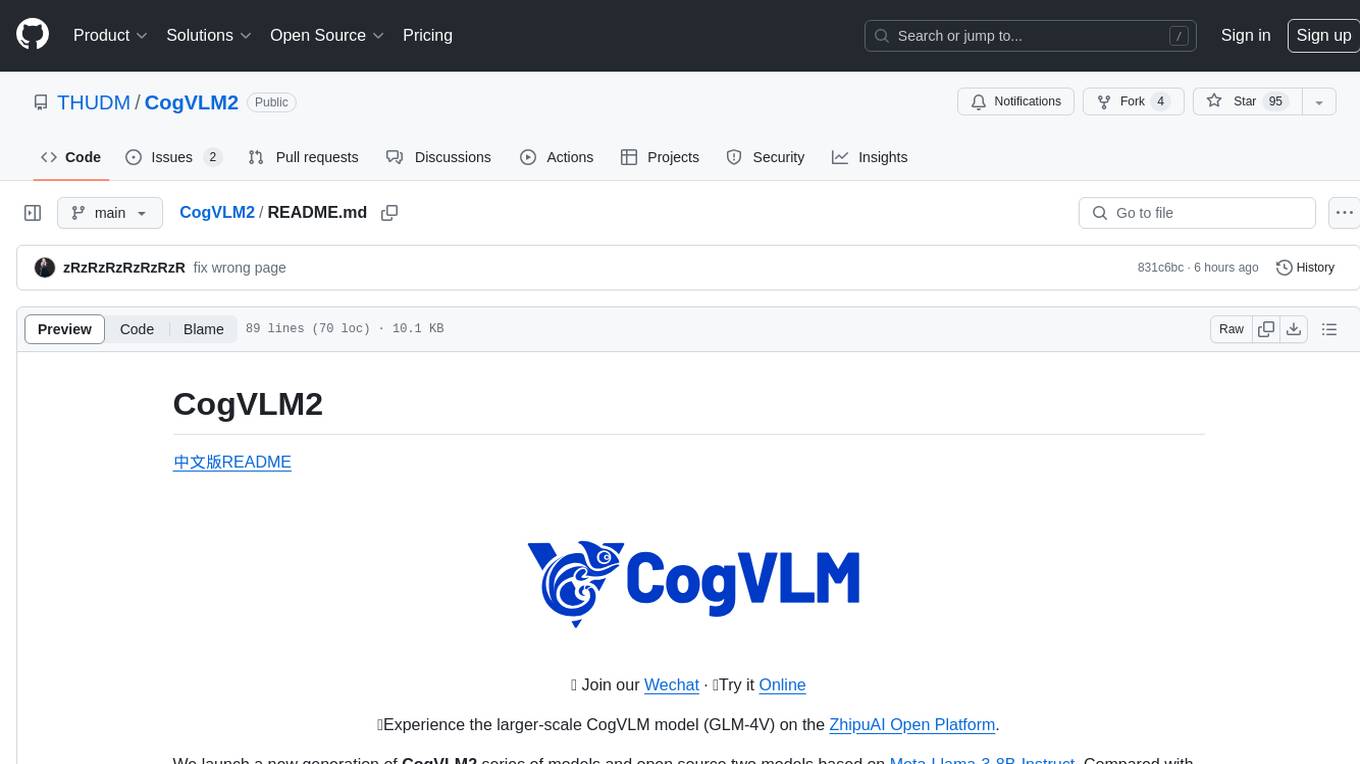
CogVLM2
CogVLM2 is a new generation of open source models that offer significant improvements in benchmarks such as TextVQA and DocVQA. It supports 8K content length, image resolution up to 1344 * 1344, and both Chinese and English languages. The project provides basic calling methods, fine-tuning examples, and OpenAI API format calling examples to help developers quickly get started with the model.
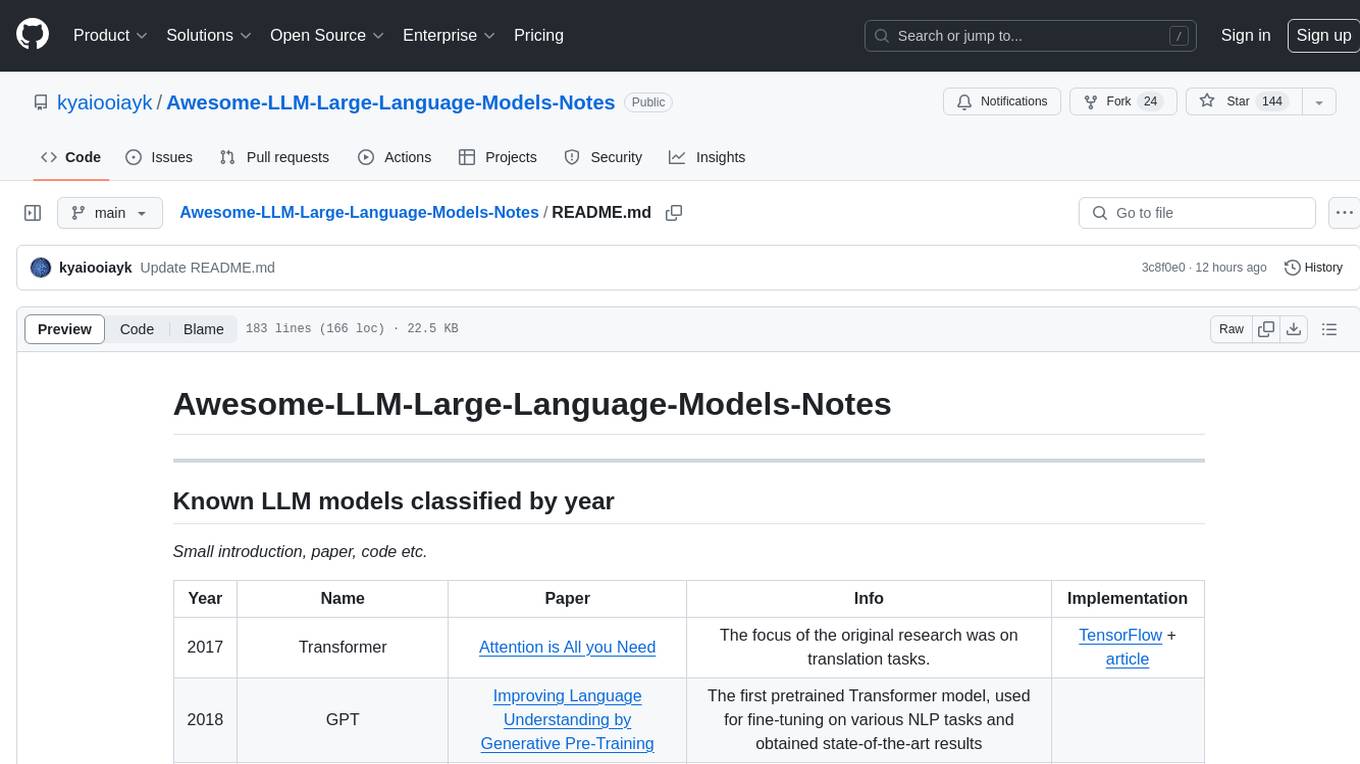
Awesome-LLM-Large-Language-Models-Notes
Awesome-LLM-Large-Language-Models-Notes is a repository that provides a comprehensive collection of information on various Large Language Models (LLMs) classified by year, size, and name. It includes details on known LLM models, their papers, implementations, and specific characteristics. The repository also covers LLM models classified by architecture, must-read papers, blog articles, tutorials, and implementations from scratch. It serves as a valuable resource for individuals interested in understanding and working with LLMs in the field of Natural Language Processing (NLP).
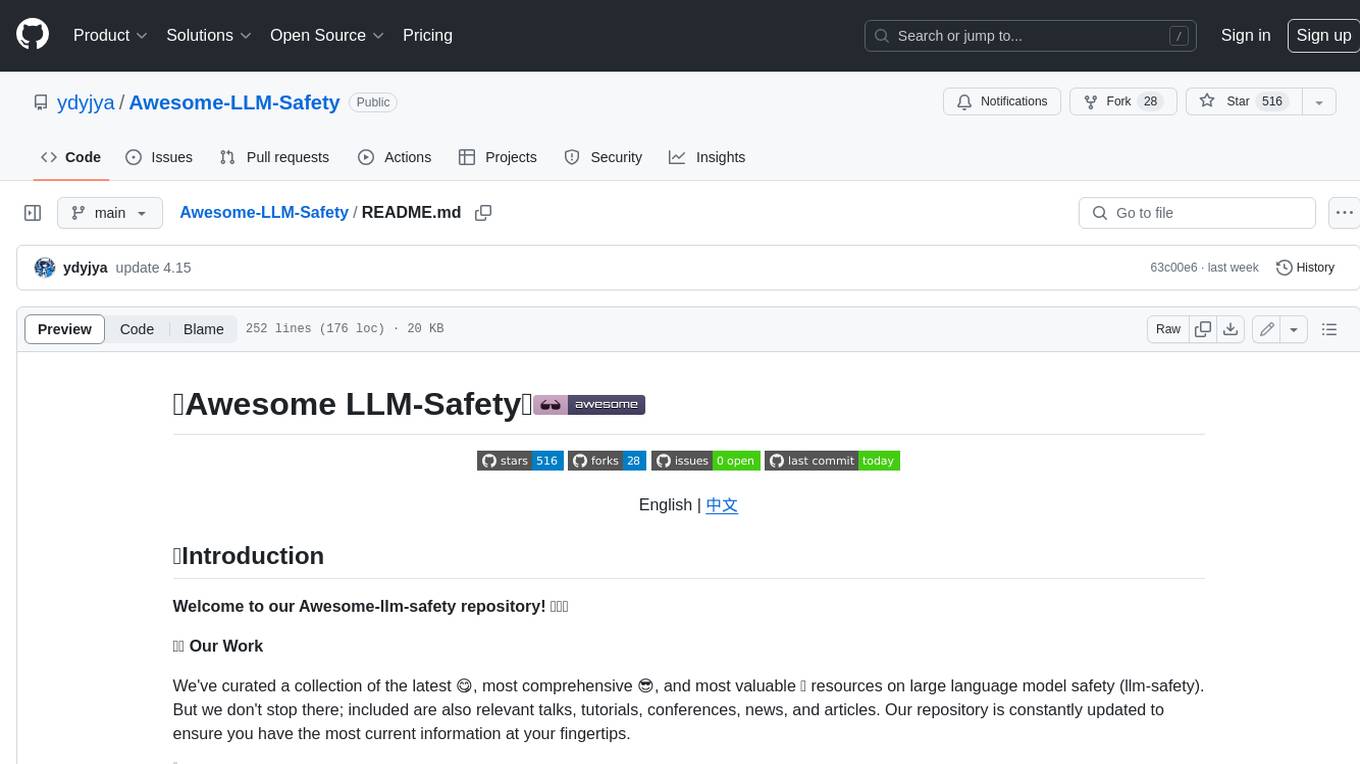
Awesome-LLM-Safety
Welcome to our Awesome-llm-safety repository! We've curated a collection of the latest, most comprehensive, and most valuable resources on large language model safety (llm-safety). But we don't stop there; included are also relevant talks, tutorials, conferences, news, and articles. Our repository is constantly updated to ensure you have the most current information at your fingertips.
For similar tasks
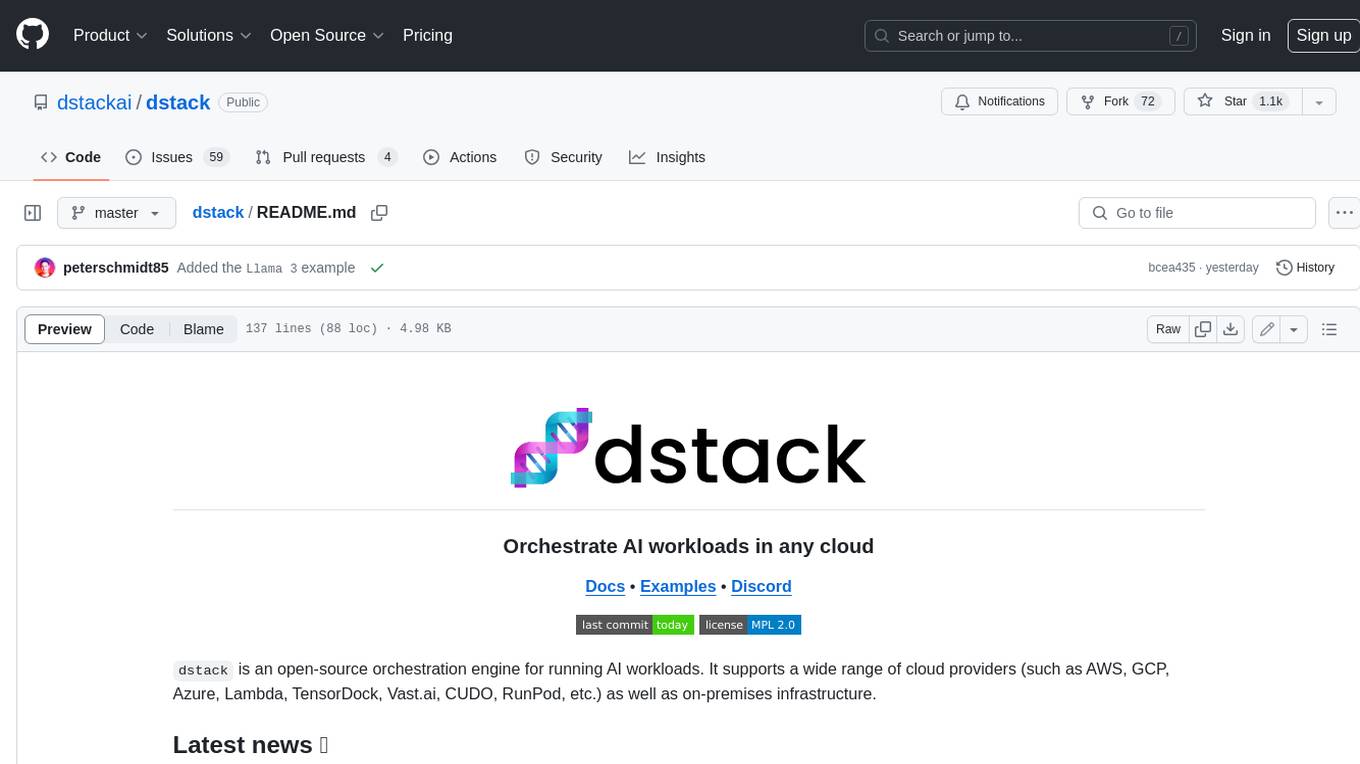
dstack
Dstack is an open-source orchestration engine for running AI workloads in any cloud. It supports a wide range of cloud providers (such as AWS, GCP, Azure, Lambda, TensorDock, Vast.ai, CUDO, RunPod, etc.) as well as on-premises infrastructure. With Dstack, you can easily set up and manage dev environments, tasks, services, and pools for your AI workloads.
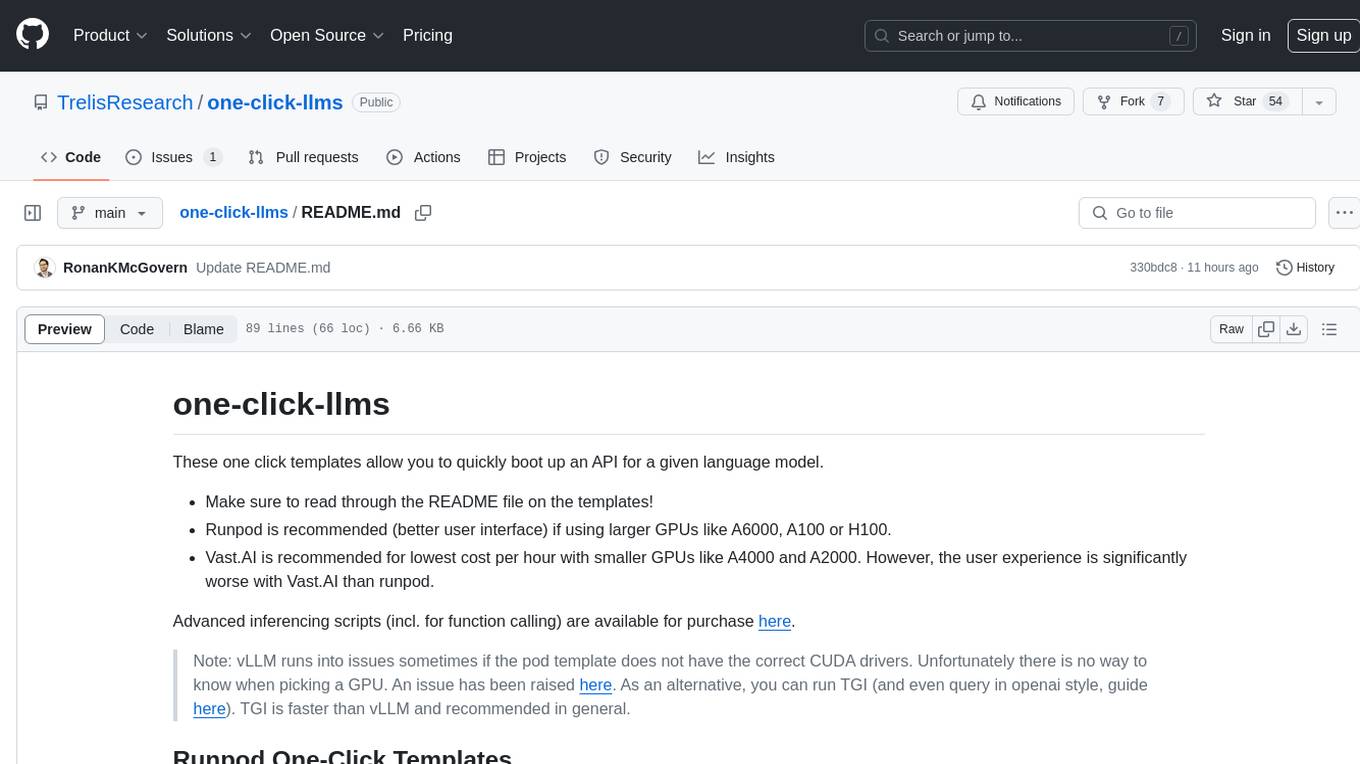
one-click-llms
The one-click-llms repository provides templates for quickly setting up an API for language models. It includes advanced inferencing scripts for function calling and offers various models for text generation and fine-tuning tasks. Users can choose between Runpod and Vast.AI for different GPU configurations, with recommendations for optimal performance. The repository also supports Trelis Research and offers templates for different model sizes and types, including multi-modal APIs and chat models.
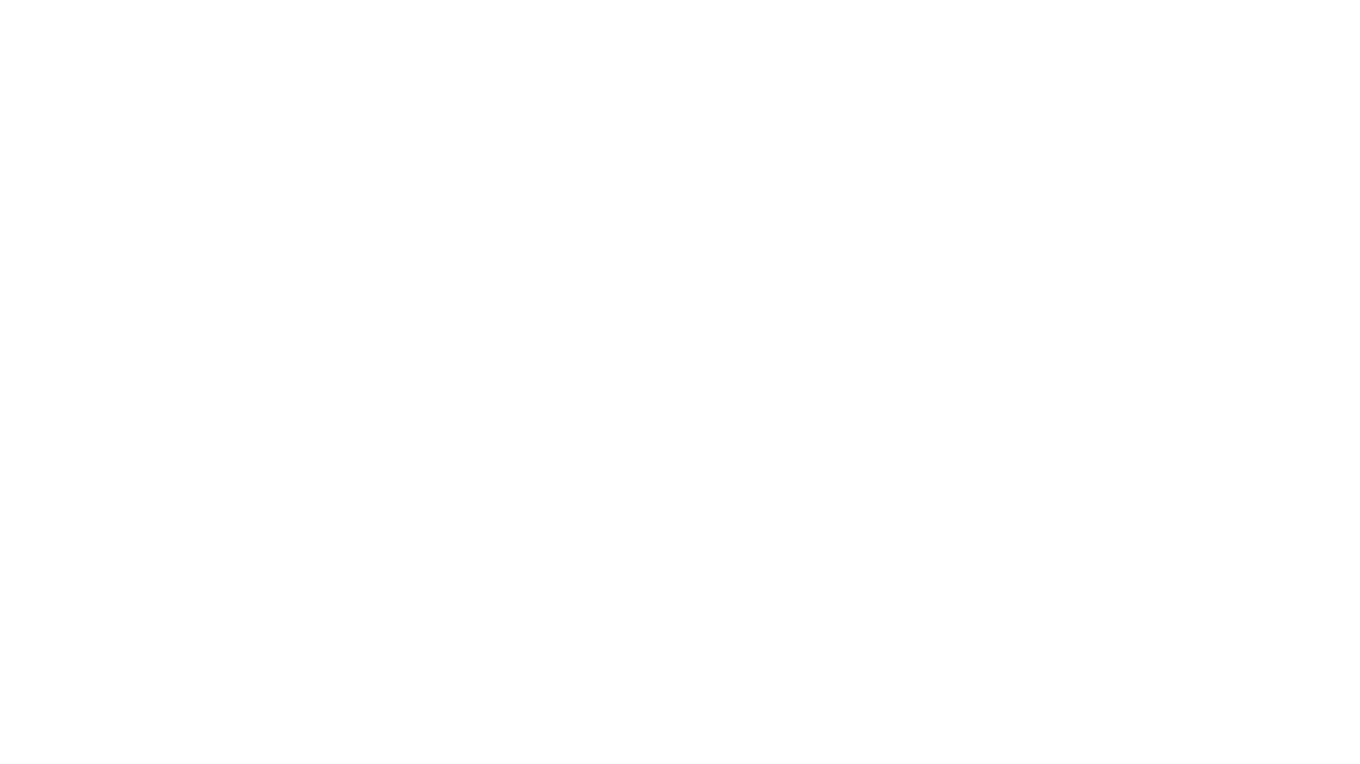
starcoder2-self-align
StarCoder2-Instruct is an open-source pipeline that introduces StarCoder2-15B-Instruct-v0.1, a self-aligned code Large Language Model (LLM) trained with a fully permissive and transparent pipeline. It generates instruction-response pairs to fine-tune StarCoder-15B without human annotations or data from proprietary LLMs. The tool is primarily finetuned for Python code generation tasks that can be verified through execution, with potential biases and limitations. Users can provide response prefixes or one-shot examples to guide the model's output. The model may have limitations with other programming languages and out-of-domain coding tasks.
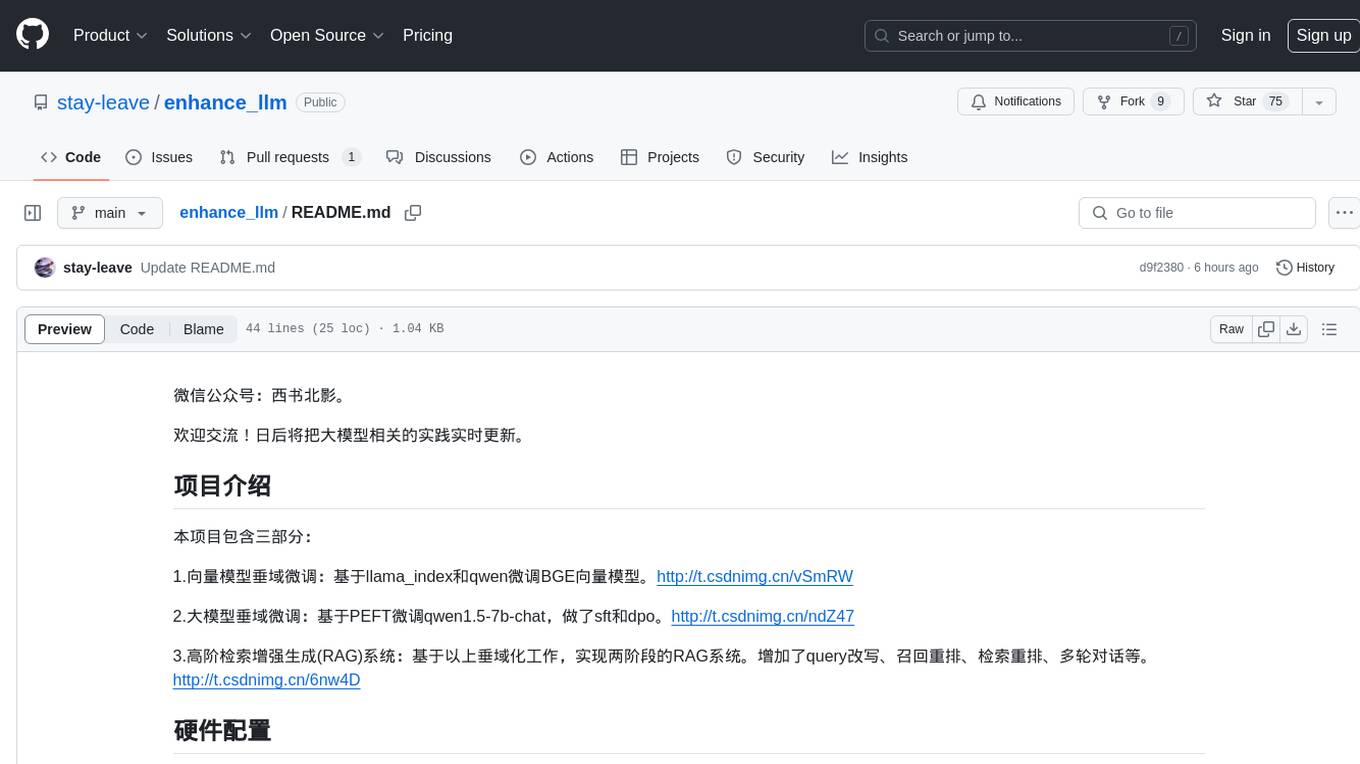
enhance_llm
The enhance_llm repository contains three main parts: 1. Vector model domain fine-tuning based on llama_index and qwen fine-tuning BGE vector model. 2. Large model domain fine-tuning based on PEFT fine-tuning qwen1.5-7b-chat, with sft and dpo. 3. High-order retrieval enhanced generation (RAG) system based on the above domain work, implementing a two-stage RAG system. It includes query rewriting, recall reordering, retrieval reordering, multi-turn dialogue, and more. The repository also provides hardware and environment configurations along with star history and licensing information.
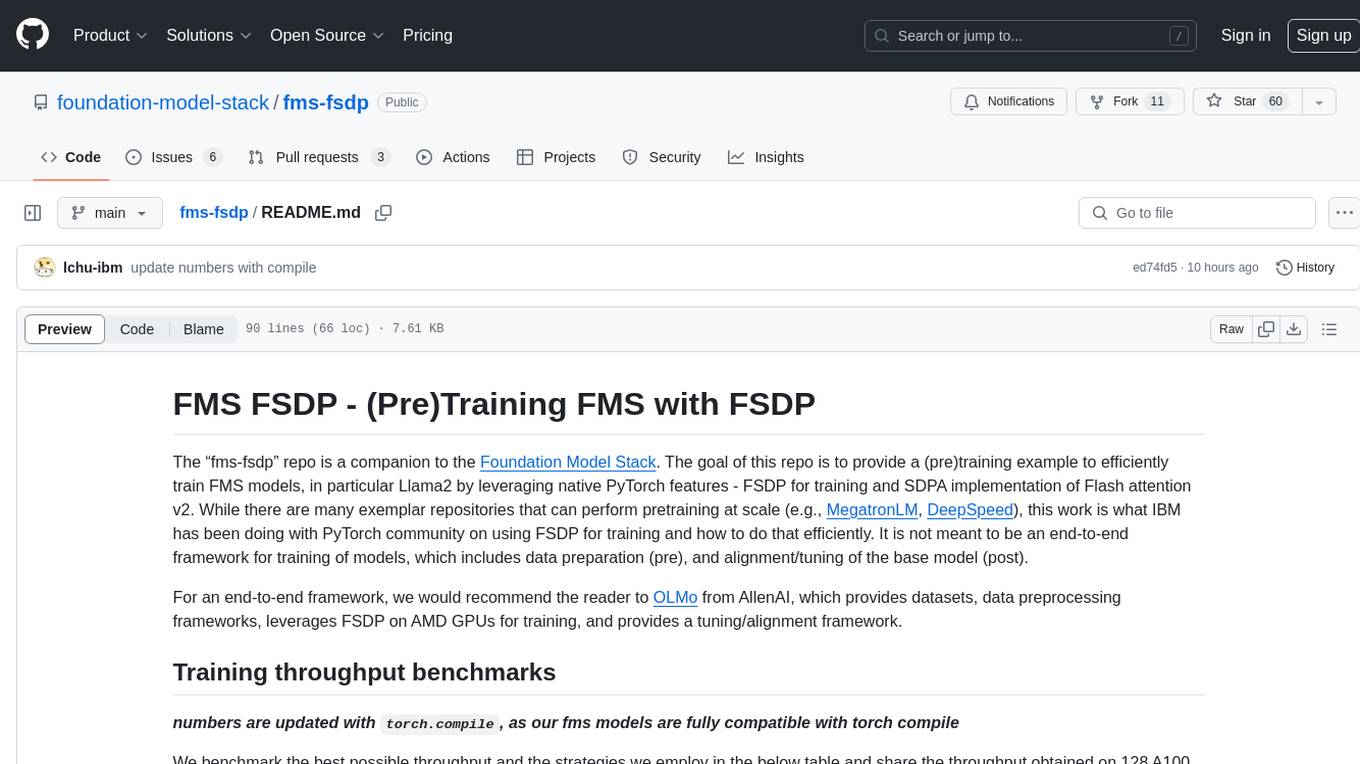
fms-fsdp
The 'fms-fsdp' repository is a companion to the Foundation Model Stack, providing a (pre)training example to efficiently train FMS models, specifically Llama2, using native PyTorch features like FSDP for training and SDPA implementation of Flash attention v2. It focuses on leveraging FSDP for training efficiently, not as an end-to-end framework. The repo benchmarks training throughput on different GPUs, shares strategies, and provides installation and training instructions. It trained a model on IBM curated data achieving high efficiency and performance metrics.
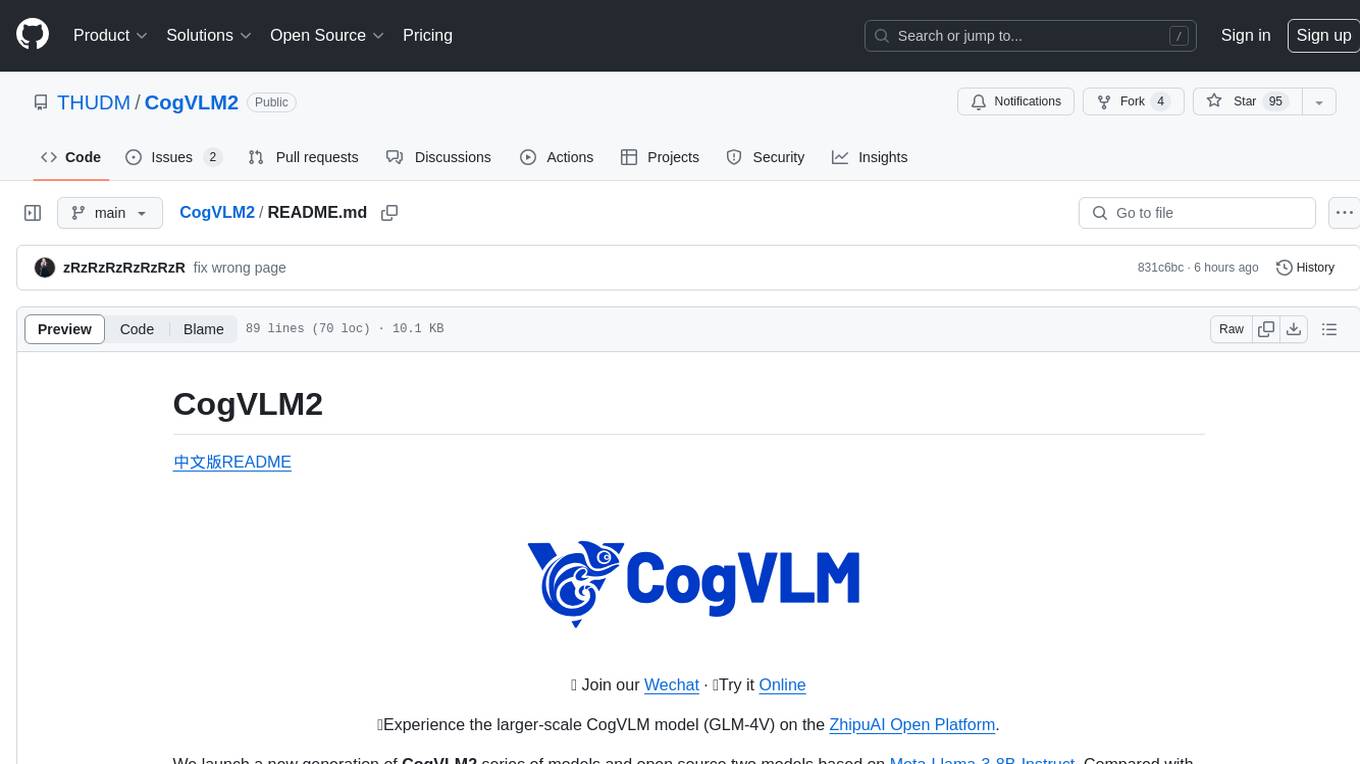
CogVLM2
CogVLM2 is a new generation of open source models that offer significant improvements in benchmarks such as TextVQA and DocVQA. It supports 8K content length, image resolution up to 1344 * 1344, and both Chinese and English languages. The project provides basic calling methods, fine-tuning examples, and OpenAI API format calling examples to help developers quickly get started with the model.
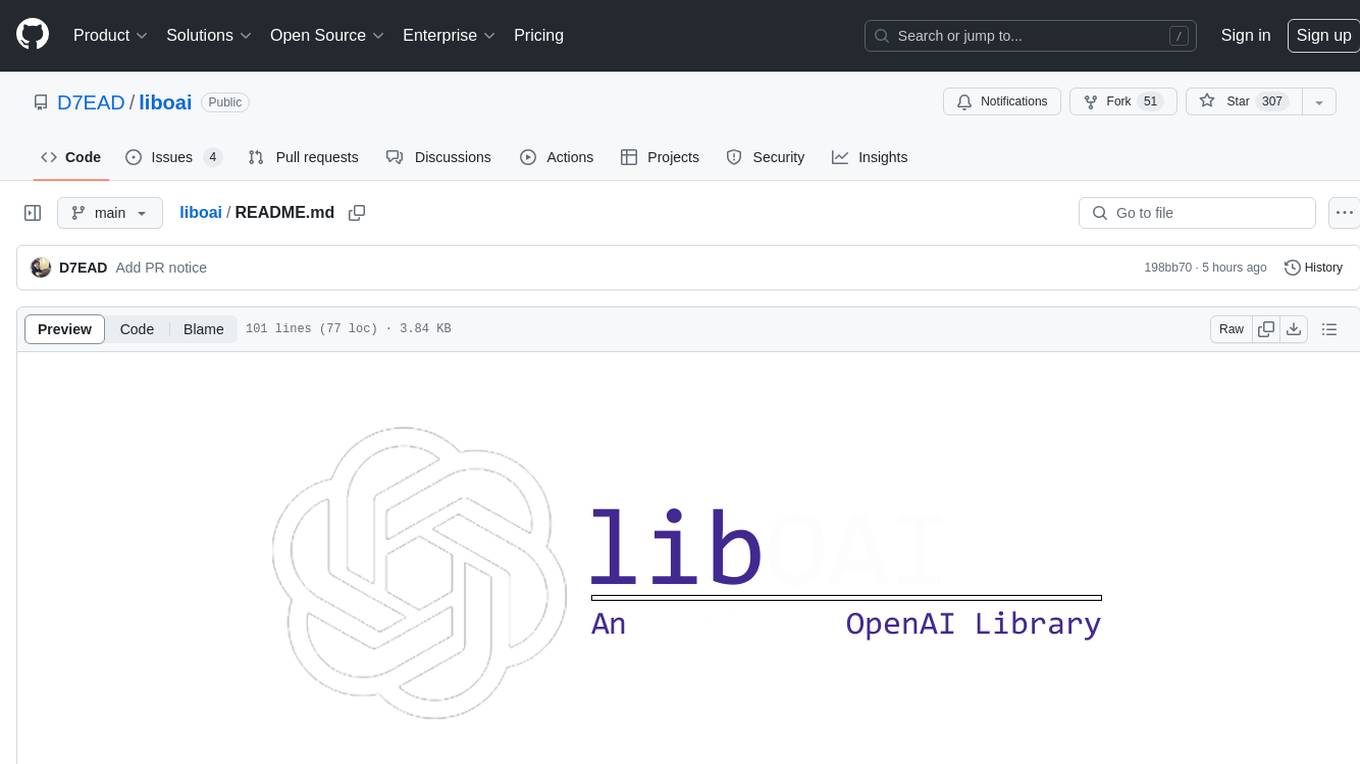
liboai
liboai is a simple C++17 library for the OpenAI API, providing developers with access to OpenAI endpoints through a collection of methods and classes. It serves as a spiritual port of OpenAI's Python library, 'openai', with similar structure and features. The library supports various functionalities such as ChatGPT, Audio, Azure, Functions, Image DALL·E, Models, Completions, Edit, Embeddings, Files, Fine-tunes, Moderation, and Asynchronous Support. Users can easily integrate the library into their C++ projects to interact with OpenAI services.
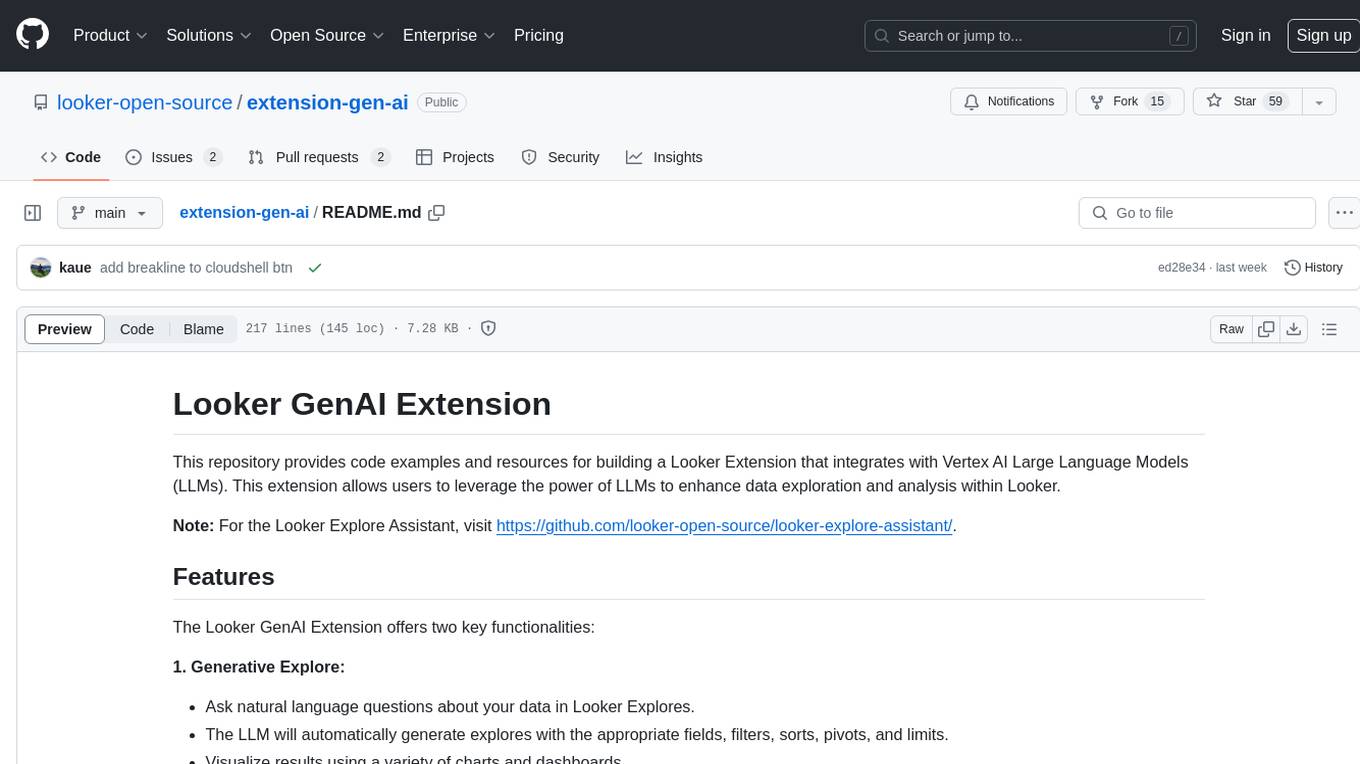
extension-gen-ai
The Looker GenAI Extension provides code examples and resources for building a Looker Extension that integrates with Vertex AI Large Language Models (LLMs). Users can leverage the power of LLMs to enhance data exploration and analysis within Looker. The extension offers generative explore functionality to ask natural language questions about data and generative insights on dashboards to analyze data by asking questions. It leverages components like BQML Remote Models, BQML Remote UDF with Vertex AI, and Custom Fine Tune Model for different integration options. Deployment involves setting up infrastructure with Terraform and deploying the Looker Extension by creating a Looker project, copying extension files, configuring BigQuery connection, connecting to Git, and testing the extension. Users can save example prompts and configure user settings for the extension. Development of the Looker Extension environment includes installing dependencies, starting the development server, and building for production.
For similar jobs
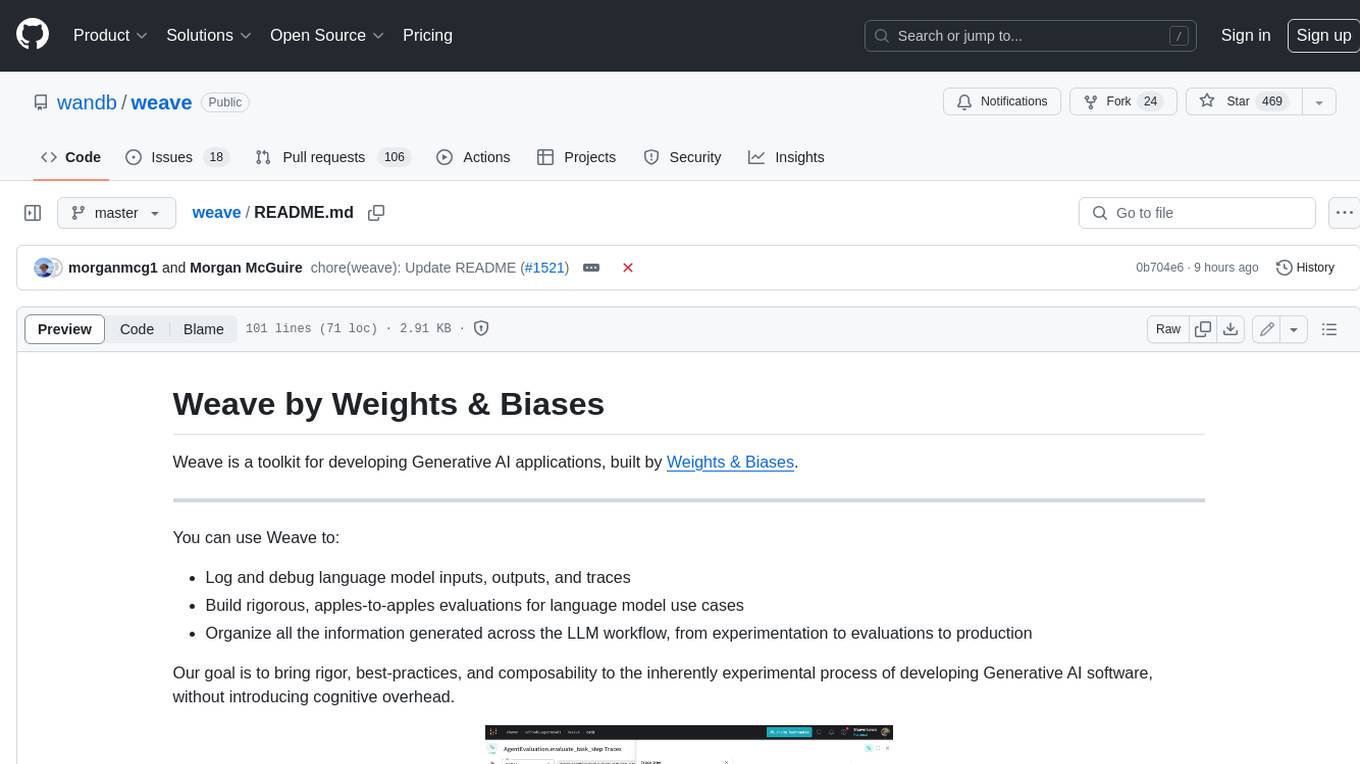
weave
Weave is a toolkit for developing Generative AI applications, built by Weights & Biases. With Weave, you can log and debug language model inputs, outputs, and traces; build rigorous, apples-to-apples evaluations for language model use cases; and organize all the information generated across the LLM workflow, from experimentation to evaluations to production. Weave aims to bring rigor, best-practices, and composability to the inherently experimental process of developing Generative AI software, without introducing cognitive overhead.
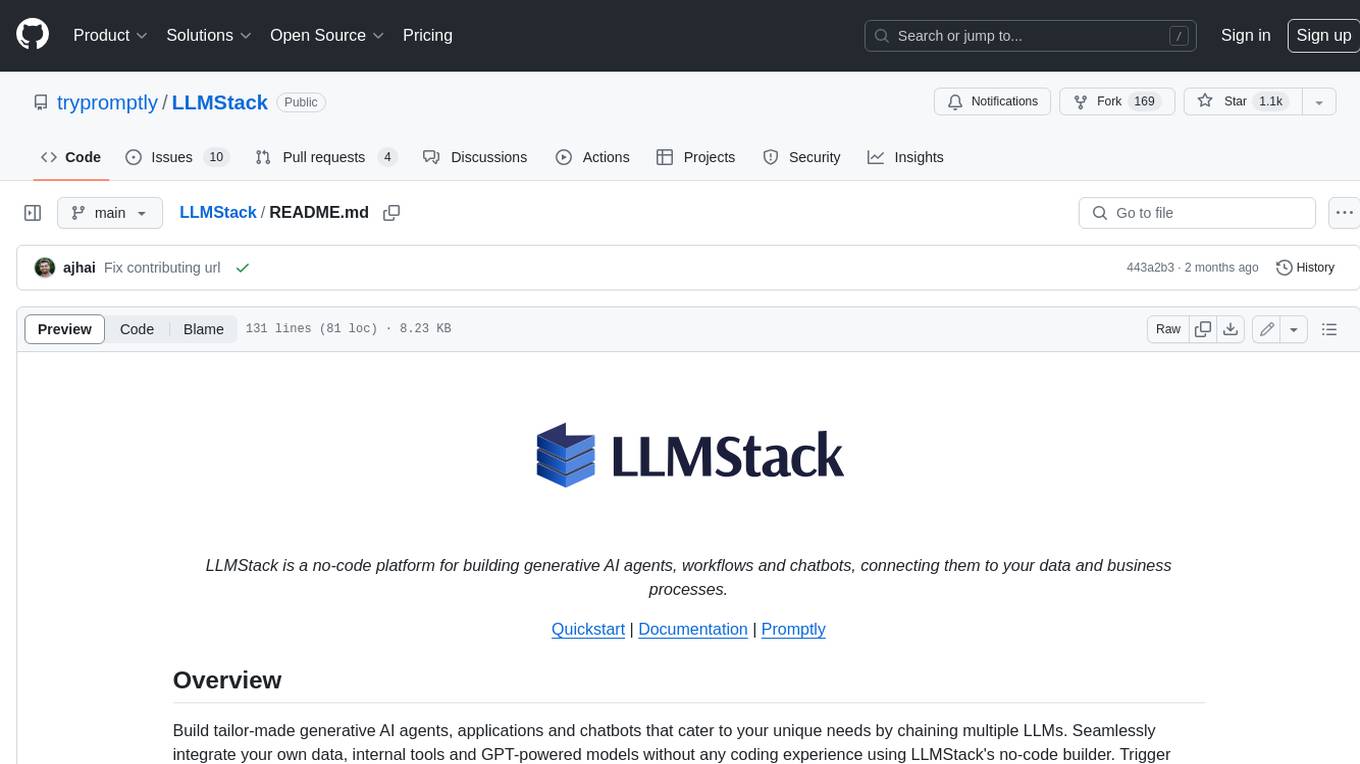
LLMStack
LLMStack is a no-code platform for building generative AI agents, workflows, and chatbots. It allows users to connect their own data, internal tools, and GPT-powered models without any coding experience. LLMStack can be deployed to the cloud or on-premise and can be accessed via HTTP API or triggered from Slack or Discord.
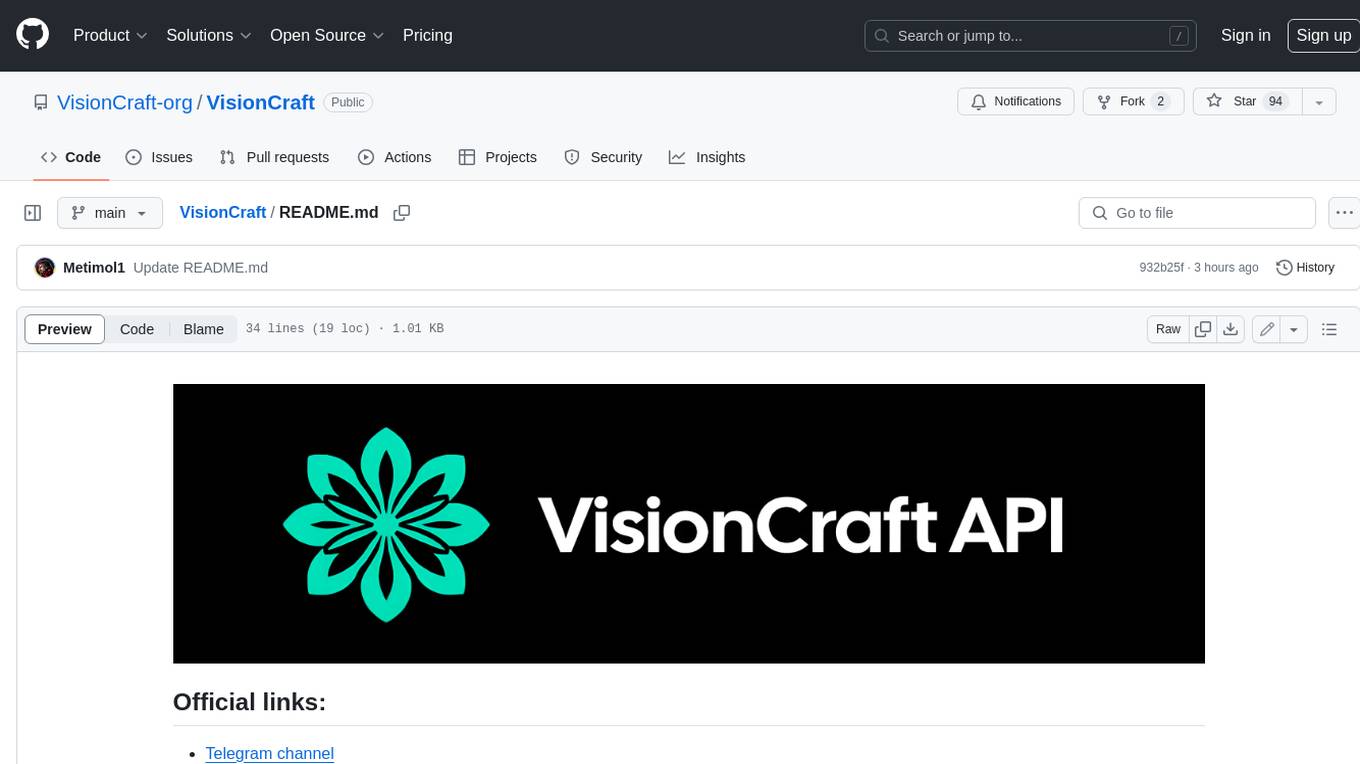
VisionCraft
The VisionCraft API is a free API for using over 100 different AI models. From images to sound.
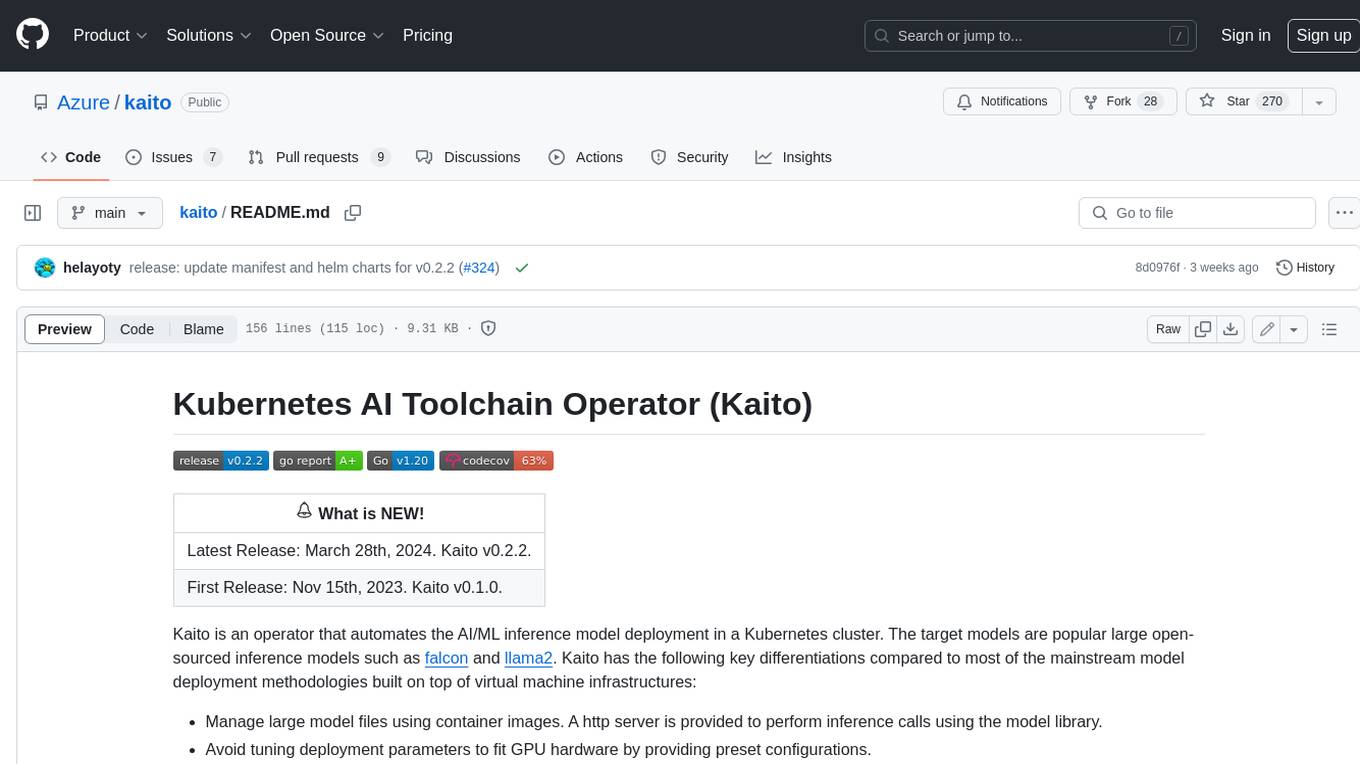
kaito
Kaito is an operator that automates the AI/ML inference model deployment in a Kubernetes cluster. It manages large model files using container images, avoids tuning deployment parameters to fit GPU hardware by providing preset configurations, auto-provisions GPU nodes based on model requirements, and hosts large model images in the public Microsoft Container Registry (MCR) if the license allows. Using Kaito, the workflow of onboarding large AI inference models in Kubernetes is largely simplified.
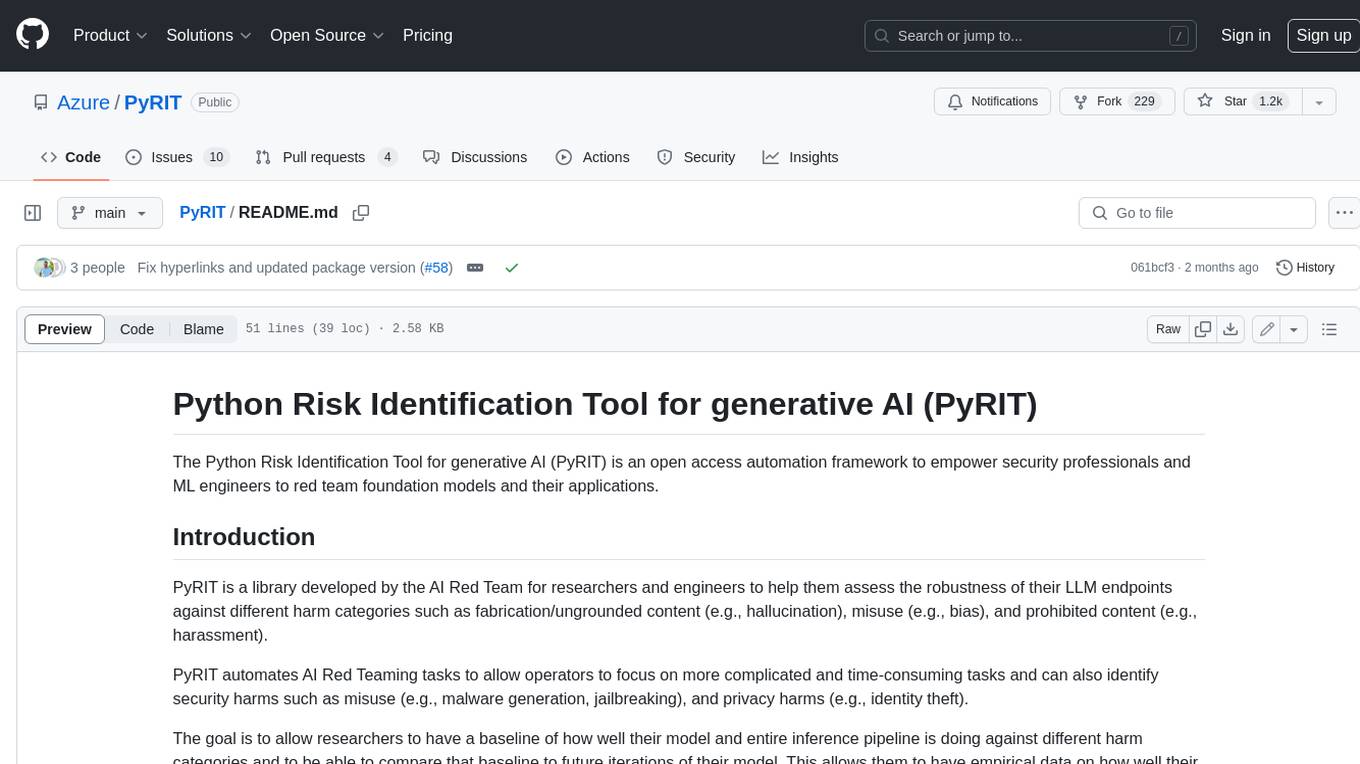
PyRIT
PyRIT is an open access automation framework designed to empower security professionals and ML engineers to red team foundation models and their applications. It automates AI Red Teaming tasks to allow operators to focus on more complicated and time-consuming tasks and can also identify security harms such as misuse (e.g., malware generation, jailbreaking), and privacy harms (e.g., identity theft). The goal is to allow researchers to have a baseline of how well their model and entire inference pipeline is doing against different harm categories and to be able to compare that baseline to future iterations of their model. This allows them to have empirical data on how well their model is doing today, and detect any degradation of performance based on future improvements.
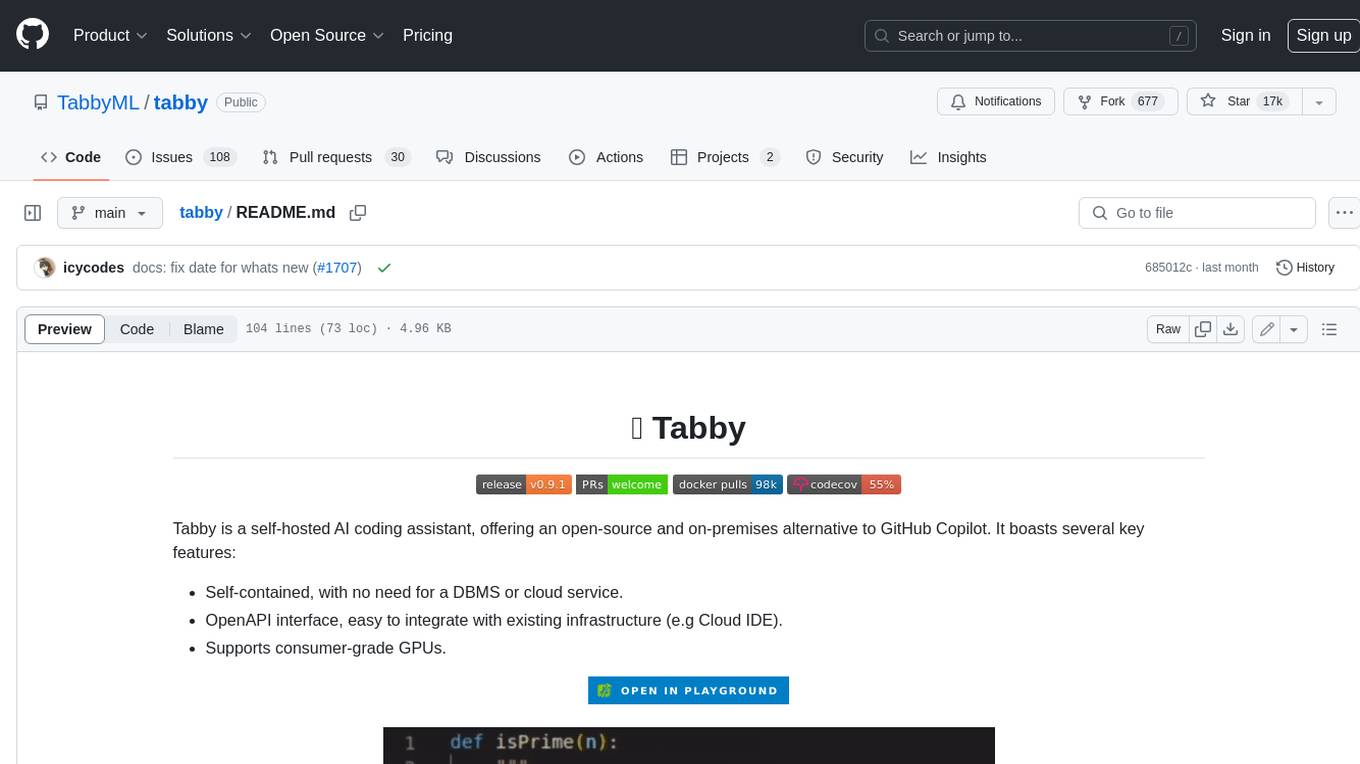
tabby
Tabby is a self-hosted AI coding assistant, offering an open-source and on-premises alternative to GitHub Copilot. It boasts several key features: * Self-contained, with no need for a DBMS or cloud service. * OpenAPI interface, easy to integrate with existing infrastructure (e.g Cloud IDE). * Supports consumer-grade GPUs.
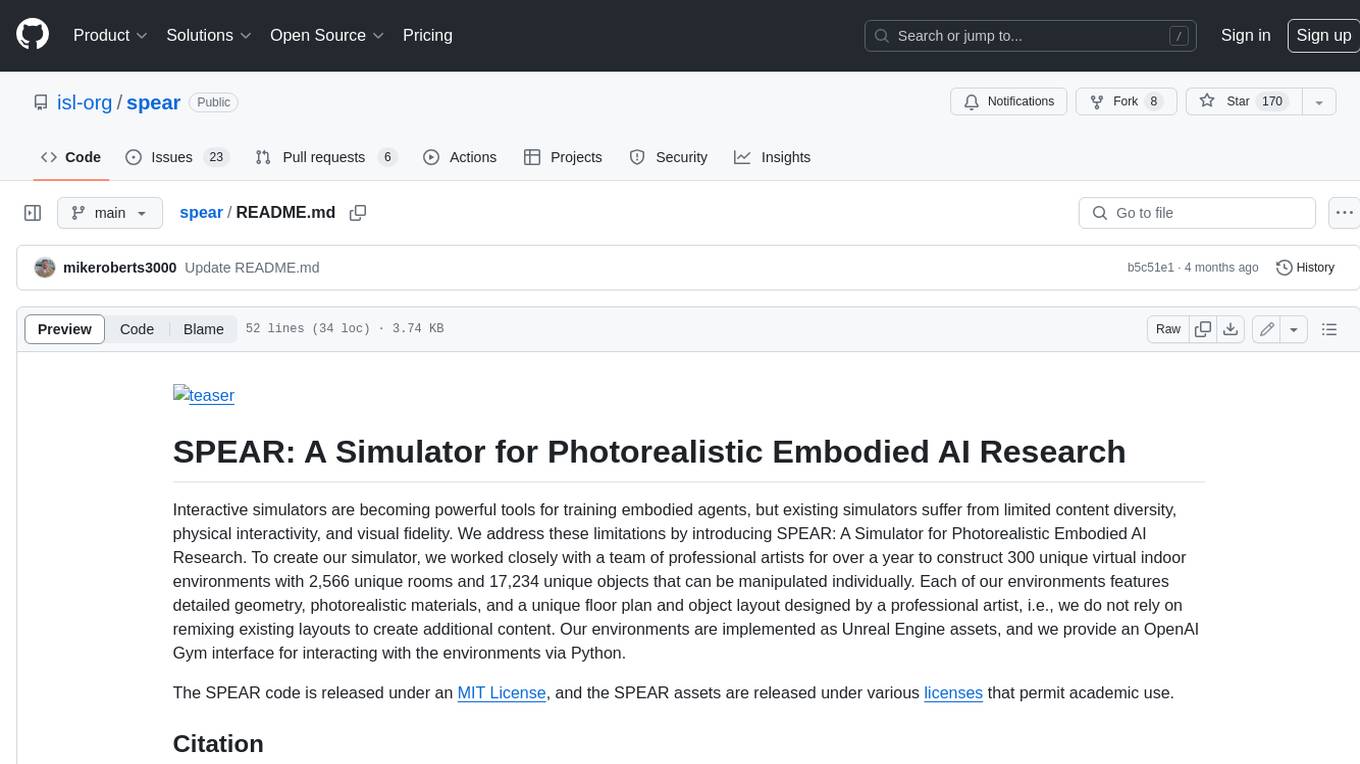
spear
SPEAR (Simulator for Photorealistic Embodied AI Research) is a powerful tool for training embodied agents. It features 300 unique virtual indoor environments with 2,566 unique rooms and 17,234 unique objects that can be manipulated individually. Each environment is designed by a professional artist and features detailed geometry, photorealistic materials, and a unique floor plan and object layout. SPEAR is implemented as Unreal Engine assets and provides an OpenAI Gym interface for interacting with the environments via Python.
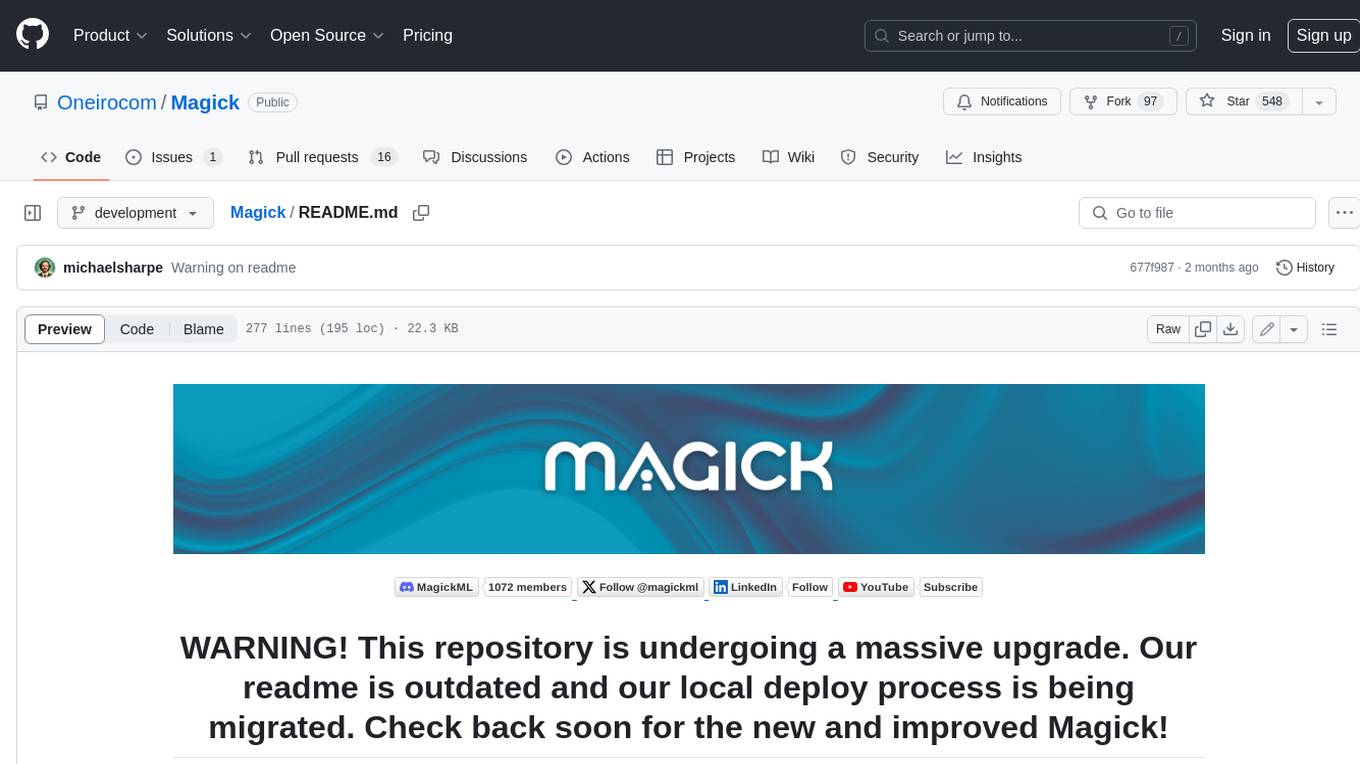
Magick
Magick is a groundbreaking visual AIDE (Artificial Intelligence Development Environment) for no-code data pipelines and multimodal agents. Magick can connect to other services and comes with nodes and templates well-suited for intelligent agents, chatbots, complex reasoning systems and realistic characters.