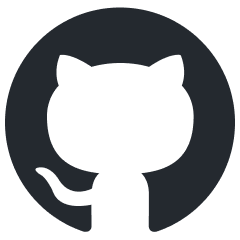
unoplat-code-confluence
The goal is to parse,process and ingest codebase/s to form a pluggable knowledge Graph and become the unified code context provider
Stars: 55
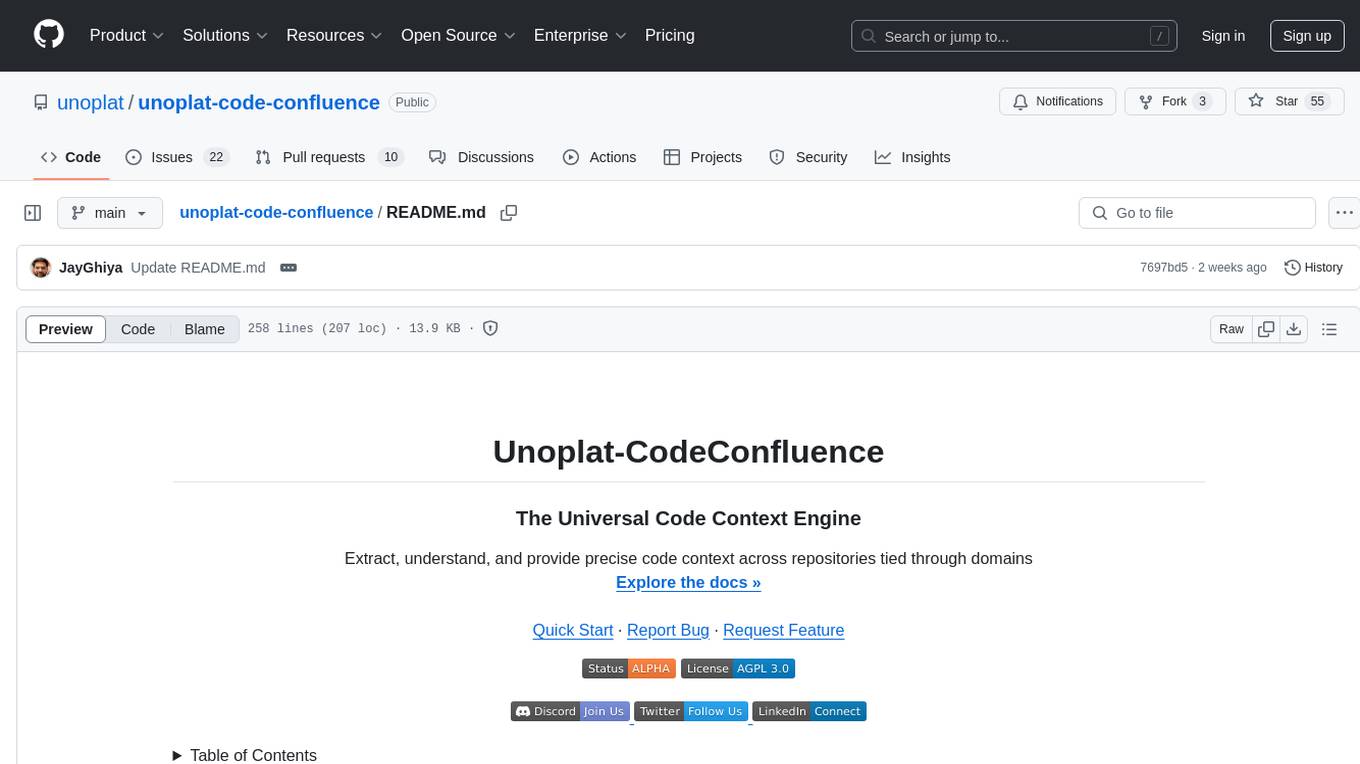
README:
Extract, understand, and provide precise code context across repositories tied through domains
Explore the docs ยป
Quick Start
ยท
Report Bug
ยท
Request Feature
Table of Contents
Unoplat-CodeConfluence aims to be the definitive solution for extracting, understanding, and providing precise code context across repositories and domains. By combining deterministic code grammar with state-of-the-art LLM pipelines, we achieve human-like understanding of codebases in minutes rather than months. Our graph-based architecture ensures relationships and context are preserved at every level.
- Deterministic Understanding: Built on ArchGuard and Tree-sitter for reliable, language-agnostic code parsing
- Smart Summarization: Bottom-up code analysis from functions to entire codebases, preserving context at every level
- Graph based Embedding: Embed codebases' functions using SOTA embeddings to enable semantic search and retrieval
- Enhanced Onboarding: Intuitive, interconnected documentation helps new team members understand complex codebases quickly
- Graph-Based Intelligence: Query and explore codebases through natural, graphical relationships between components
- Deep Dependency Insights: Comprehensive parsing of package managers as well as any other related metadata reveals true project structure and relationships
- Integration Ready: Designed to work seamlessly with your existing development tools and workflows
Unoplat-CodeConfluence will provide:
-
Precise Context API: Get reliable, deterministic code understanding through:
- Bottom-up code summarization from functions to systems
- Graph-based querying on SOTA embeddings with deterministic code grammar for natural code exploration
- Deep package and dependency analysis
Our UnoplatOssAtlas project is designed to dramatically accelerate contributor onboarding and productivity in open-source projects. By providing deep, contextual understanding of popular repositories, we will help developers in the following:
- Accelerate Onboarding: Understand complex codebases in minutes instead of months
- Boost Contribution Velocity: Make meaningful contributions faster with deep contextual insights
- Navigate Complex Systems: Easily understand dependencies, patterns, and architectural decisions
- Learn Best Practices: Study and adopt patterns from well-established open-source projects
This initiative demonstrates our commitment to:
- Empowering the open-source ecosystem by reducing barriers to contribution
- Showcasing practical applications of our context extraction capabilities
- Supporting sustainable open-source development through better understanding and reducing time to value for oss projects
Ready to enhance your development workflow?
Check out our Quick Start Guide.
Language | In-POC | Alpha | Beta | Stable |
---|---|---|---|---|
Python | โ | โ | โ | |
Java | โ | |||
TypeScript | ||||
Go |
Task | Research | POC | Released |
---|---|---|---|
Code Grammar | โ | โ | โ |
Integration With Workflow Orchestrator | โ | โ | โ |
Data Modelling for Code Grammar | โ | โ | โ |
Insertion into Graph Database | โ | โ | โ |
Data Modelling for Code Summarisation | โ | โ | |
SOTA LLM Pipelines For Code Grammar Summarisation/Reports | โ | โ | |
SOTA Embeddings on Codebase Functions | โ | ||
Automatic Documentation | โ | ||
GraphRag based Query Module | |||
SDK for integration |
Feature | Beta | Stable | Limitations |
---|---|---|---|
Package Parsing | โ | ||
Package Metadata Parsing - Poetry and PIP and UV | โ | ||
Inheritance | โ | ||
Function Parsing | โ | ||
Class Parsing | โ | ||
Procedural Code Parsing | โ | ||
Global Variable Parsing | โ | ||
Function Call Parsing | โ | ||
Class Variables/Instance Variables Parsing | โ | ||
Function Local Variable Parsing | โ | ||
Function Return Type Parsing | โ | Return type is not captured properly. | |
Figuring out dependent internal classes | โ | ||
Import Segregation | โ | Currently only identifies internal procedures, classes, and others as unknown. External imports with links to dependencies in package manager metadata coming soon. | |
Sorting functions within a class/procedure based on dependency | โ | Circular dependencies/recursion will not work as topological sort is used. | |
Nested Functions | โ |
Note: For detailed limitations and resolutions that we are working towards refer to Limitations_Resolutions.md
- Phodal from Chapi and ArcGuard
- [Ishaan & Krrish from Litellm]([email protected] / [email protected])
- Omar Khattab
- Joao Moura from crewai
- Vipin Shreyas Kumar
- Danswer
- Continue
- Apeksha
- Jeremy Howard
- Temporal
- Neo4j
- FastApi
Jay Ghiya
Contact: [email protected] |
Vipin Shreyas Kumar
Contact: [email protected] |
Book a call with us - Cal Link
Unoplat-CodeConfluence is licensed under the GNU Affero General Public License v3.0 (AGPL-3.0). This strong copyleft license ensures that derivatives of this software remain open source and under the same license.
For Tasks:
Click tags to check more tools for each tasksFor Jobs:
Alternative AI tools for unoplat-code-confluence
Similar Open Source Tools
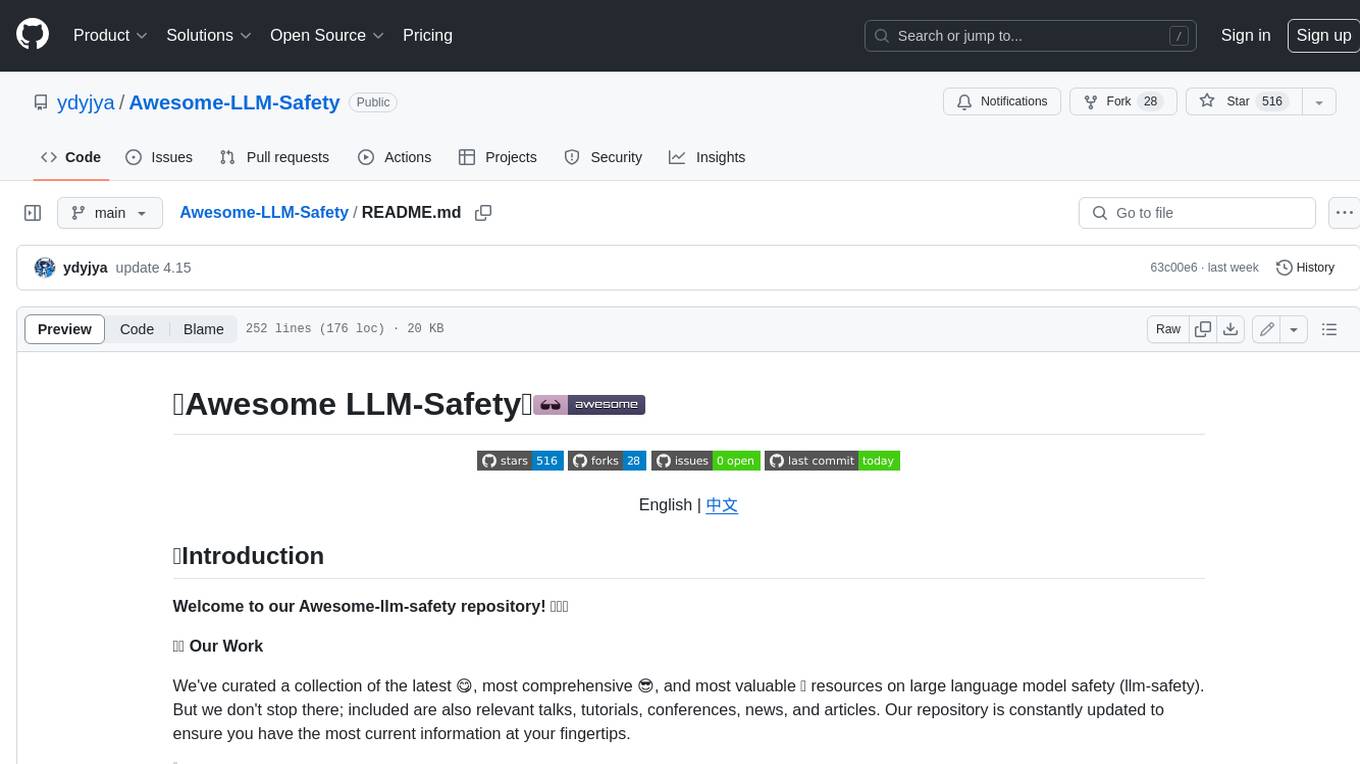
Awesome-LLM-Safety
Welcome to our Awesome-llm-safety repository! We've curated a collection of the latest, most comprehensive, and most valuable resources on large language model safety (llm-safety). But we don't stop there; included are also relevant talks, tutorials, conferences, news, and articles. Our repository is constantly updated to ensure you have the most current information at your fingertips.
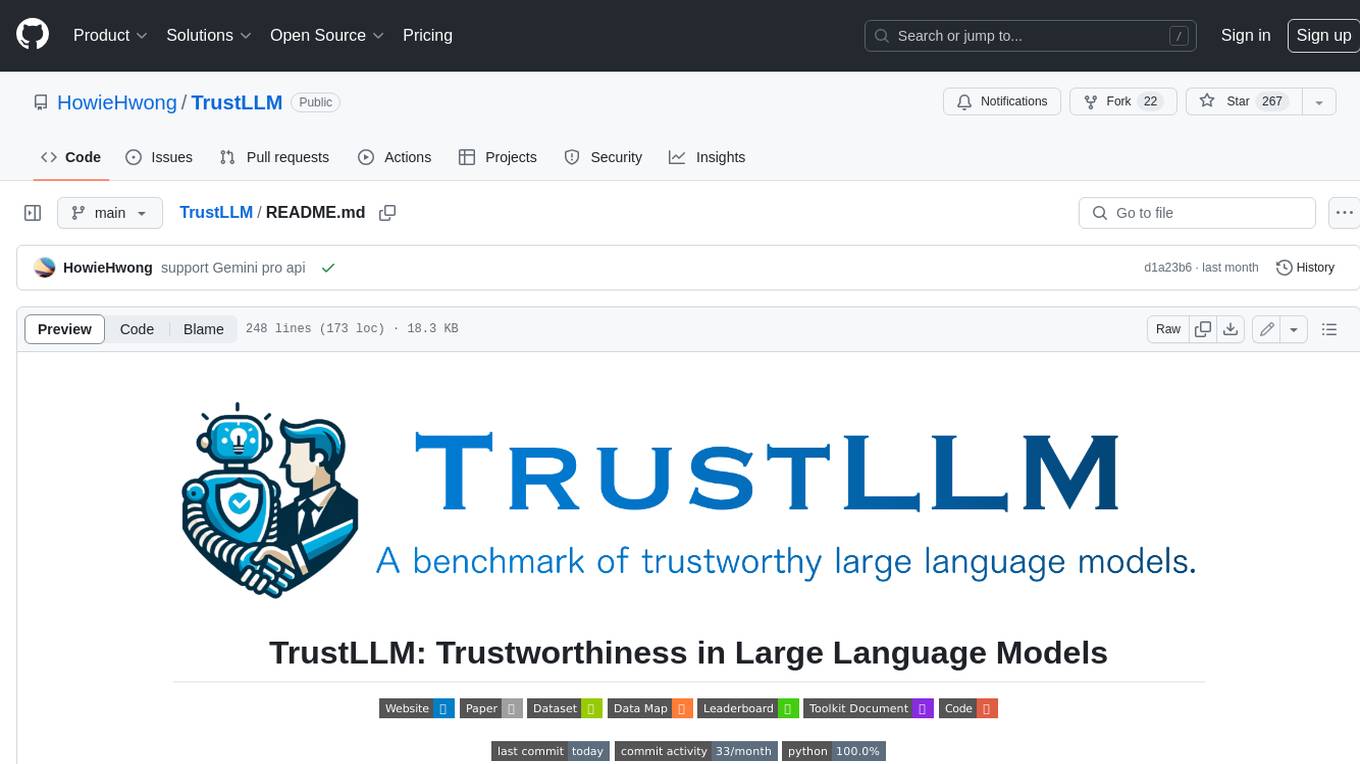
TrustLLM
TrustLLM is a comprehensive study of trustworthiness in LLMs, including principles for different dimensions of trustworthiness, established benchmark, evaluation, and analysis of trustworthiness for mainstream LLMs, and discussion of open challenges and future directions. Specifically, we first propose a set of principles for trustworthy LLMs that span eight different dimensions. Based on these principles, we further establish a benchmark across six dimensions including truthfulness, safety, fairness, robustness, privacy, and machine ethics. We then present a study evaluating 16 mainstream LLMs in TrustLLM, consisting of over 30 datasets. The document explains how to use the trustllm python package to help you assess the performance of your LLM in trustworthiness more quickly. For more details about TrustLLM, please refer to project website.
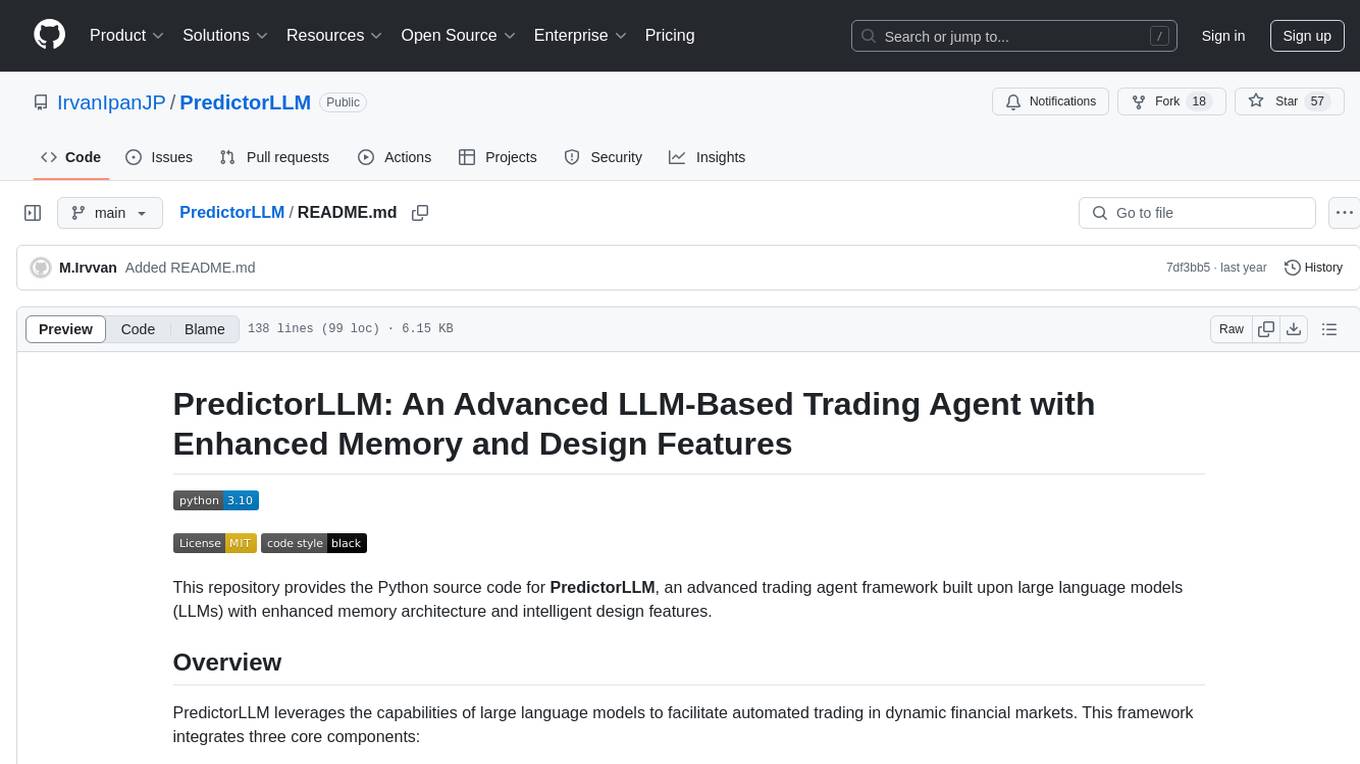
PredictorLLM
PredictorLLM is an advanced trading agent framework that utilizes large language models to automate trading in financial markets. It includes a profiling module to establish agent characteristics, a layered memory module for retaining and prioritizing financial data, and a decision-making module to convert insights into trading strategies. The framework mimics professional traders' behavior, surpassing human limitations in data processing and continuously evolving to adapt to market conditions for superior investment outcomes.
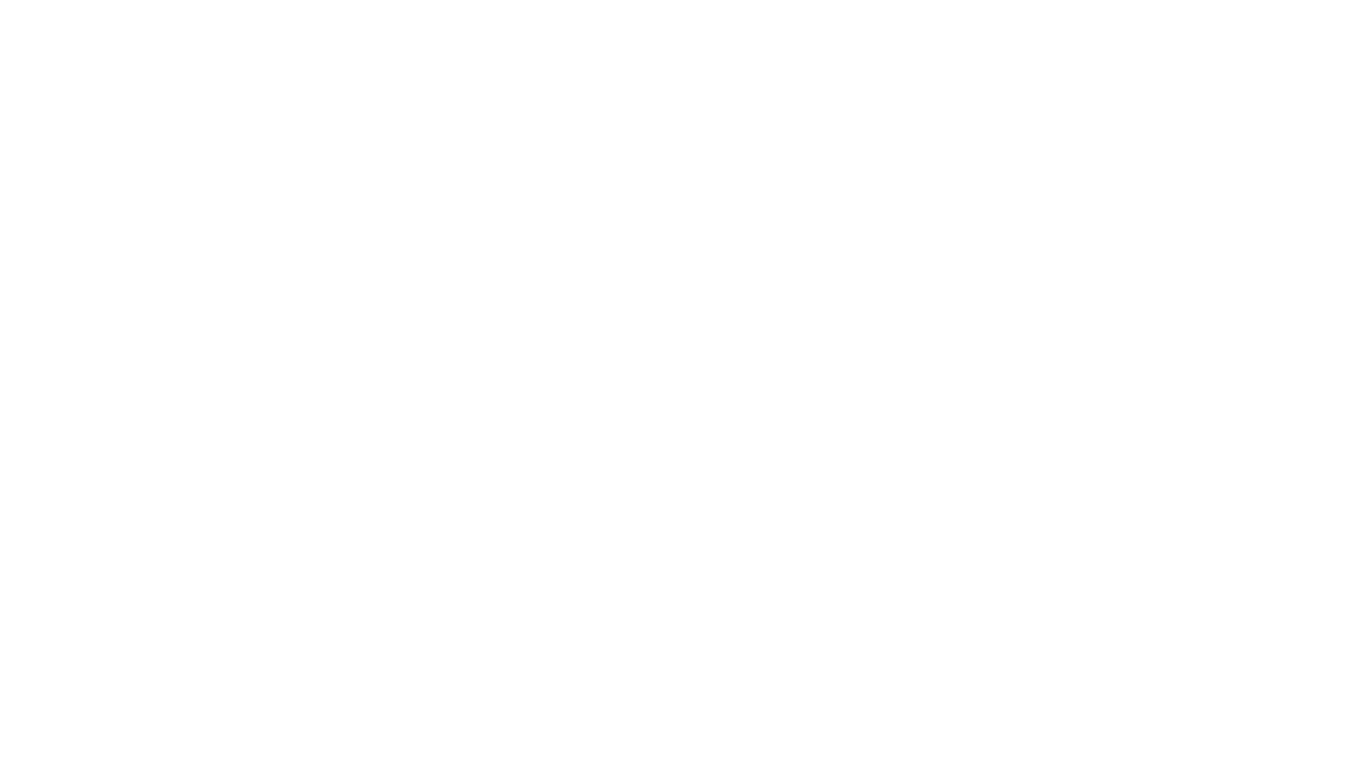
Prompt-Engineering-Holy-Grail
The Prompt Engineering Holy Grail repository is a curated resource for prompt engineering enthusiasts, providing essential resources, tools, templates, and best practices to support learning and working in prompt engineering. It covers a wide range of topics related to prompt engineering, from beginner fundamentals to advanced techniques, and includes sections on learning resources, online courses, books, prompt generation tools, prompt management platforms, prompt testing and experimentation, prompt crafting libraries, prompt libraries and datasets, prompt engineering communities, freelance and job opportunities, contributing guidelines, code of conduct, support for the project, and contact information.
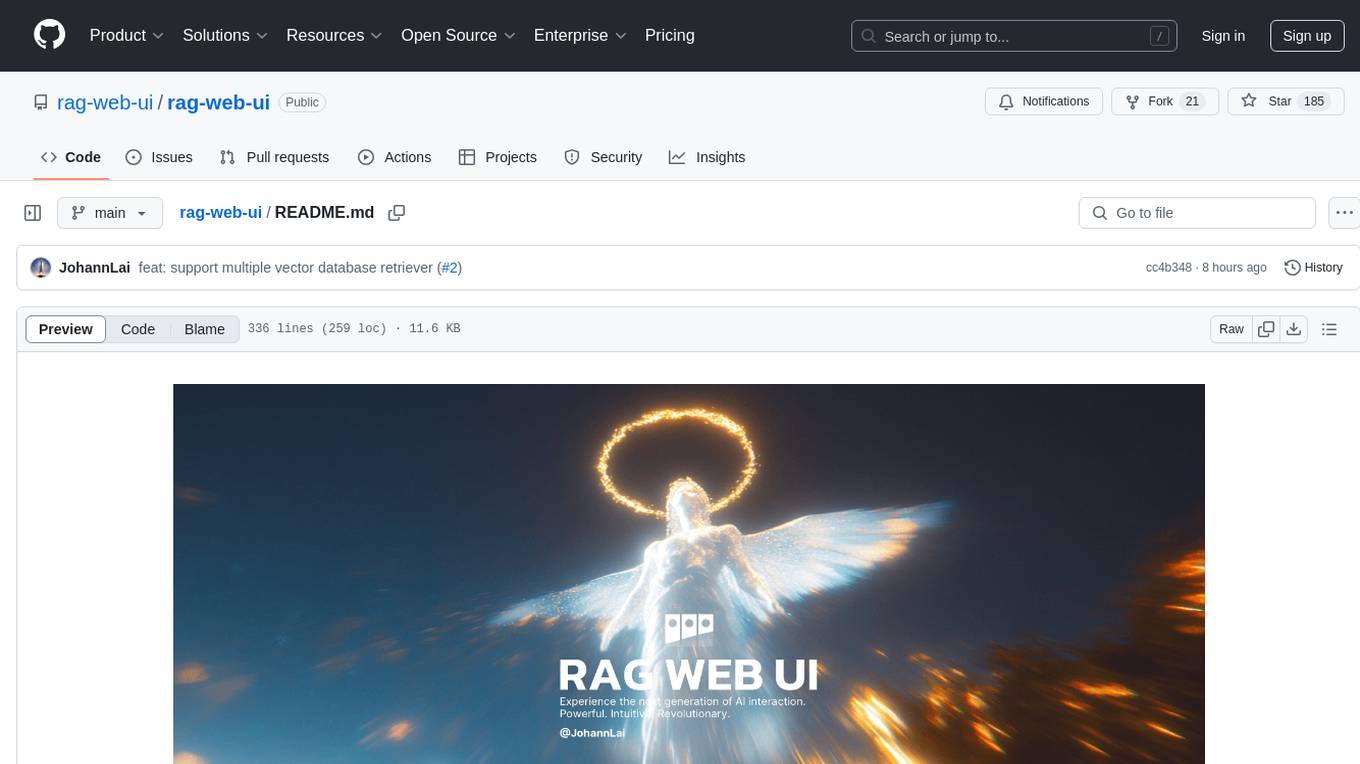
rag-web-ui
RAG Web UI is an intelligent dialogue system based on RAG (Retrieval-Augmented Generation) technology. It helps enterprises and individuals build intelligent Q&A systems based on their own knowledge bases. By combining document retrieval and large language models, it delivers accurate and reliable knowledge-based question-answering services. The system is designed with features like intelligent document management, advanced dialogue engine, and a robust architecture. It supports multiple document formats, async document processing, multi-turn contextual dialogue, and reference citations in conversations. The architecture includes a backend stack with Python FastAPI, MySQL + ChromaDB, MinIO, Langchain, JWT + OAuth2 for authentication, and a frontend stack with Next.js, TypeScript, Tailwind CSS, Shadcn/UI, and Vercel AI SDK for AI integration. Performance optimization includes incremental document processing, streaming responses, vector database performance tuning, and distributed task processing. The project is licensed under the Apache-2.0 License and is intended for learning and sharing RAG knowledge only, not for commercial purposes.
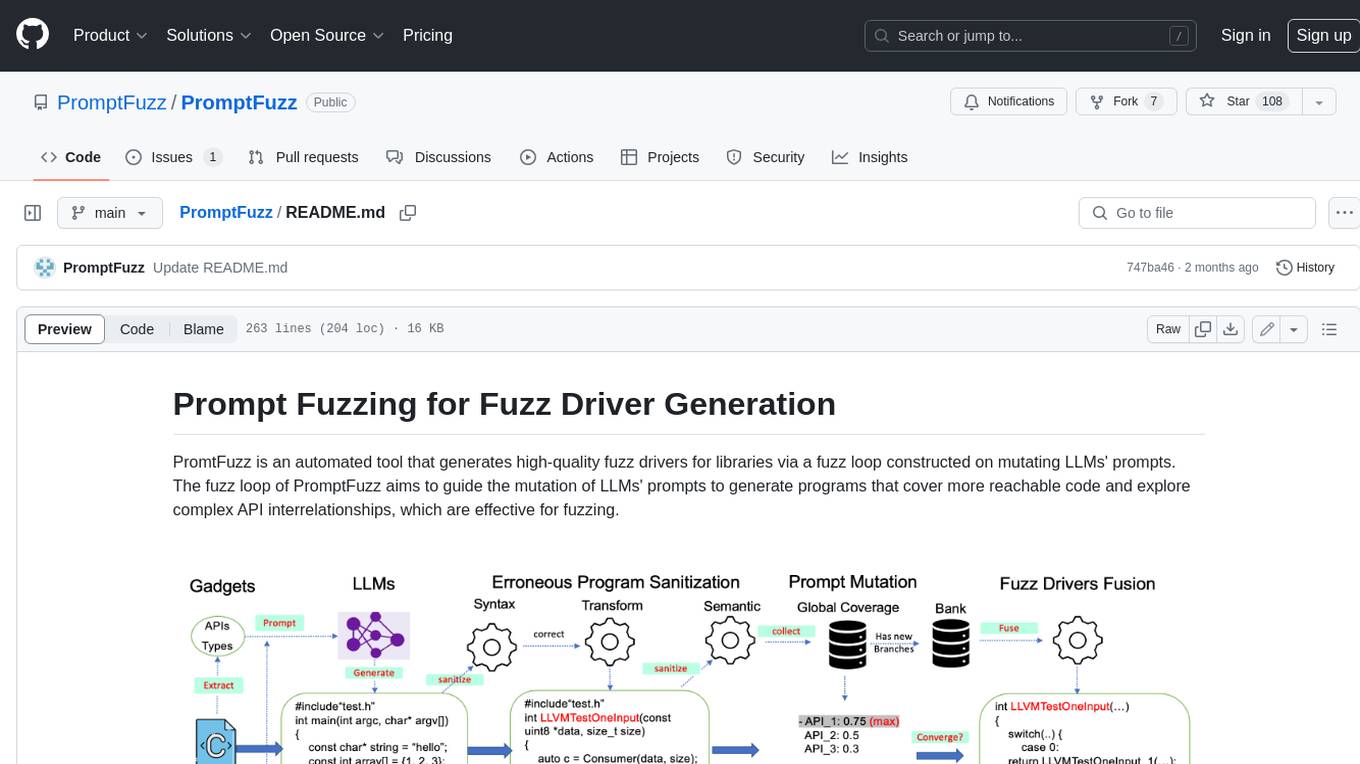
PromptFuzz
**Description:** PromptFuzz is an automated tool that generates high-quality fuzz drivers for libraries via a fuzz loop constructed on mutating LLMs' prompts. The fuzz loop of PromptFuzz aims to guide the mutation of LLMs' prompts to generate programs that cover more reachable code and explore complex API interrelationships, which are effective for fuzzing. **Features:** * **Multiply LLM support** : Supports the general LLMs: Codex, Inocder, ChatGPT, and GPT4 (Currently tested on ChatGPT). * **Context-based Prompt** : Construct LLM prompts with the automatically extracted library context. * **Powerful Sanitization** : The program's syntax, semantics, behavior, and coverage are thoroughly analyzed to sanitize the problematic programs. * **Prioritized Mutation** : Prioritizes mutating the library API combinations within LLM's prompts to explore complex interrelationships, guided by code coverage. * **Fuzz Driver Exploitation** : Infers API constraints using statistics and extends fixed API arguments to receive random bytes from fuzzers. * **Fuzz engine integration** : Integrates with grey-box fuzz engine: LibFuzzer. **Benefits:** * **High branch coverage:** The fuzz drivers generated by PromptFuzz achieved a branch coverage of 40.12% on the tested libraries, which is 1.61x greater than _OSS-Fuzz_ and 1.67x greater than _Hopper_. * **Bug detection:** PromptFuzz detected 33 valid security bugs from 49 unique crashes. * **Wide range of bugs:** The fuzz drivers generated by PromptFuzz can detect a wide range of bugs, most of which are security bugs. * **Unique bugs:** PromptFuzz detects uniquely interesting bugs that other fuzzers may miss. **Usage:** 1. Build the library using the provided build scripts. 2. Export the LLM API KEY if using ChatGPT or GPT4. 3. Generate fuzz drivers using the `fuzzer` command. 4. Run the fuzz drivers using the `harness` command. 5. Deduplicate and analyze the reported crashes. **Future Works:** * **Custom LLMs suport:** Support custom LLMs. * **Close-source libraries:** Apply PromptFuzz to close-source libraries by fine tuning LLMs on private code corpus. * **Performance** : Reduce the huge time cost required in erroneous program elimination.
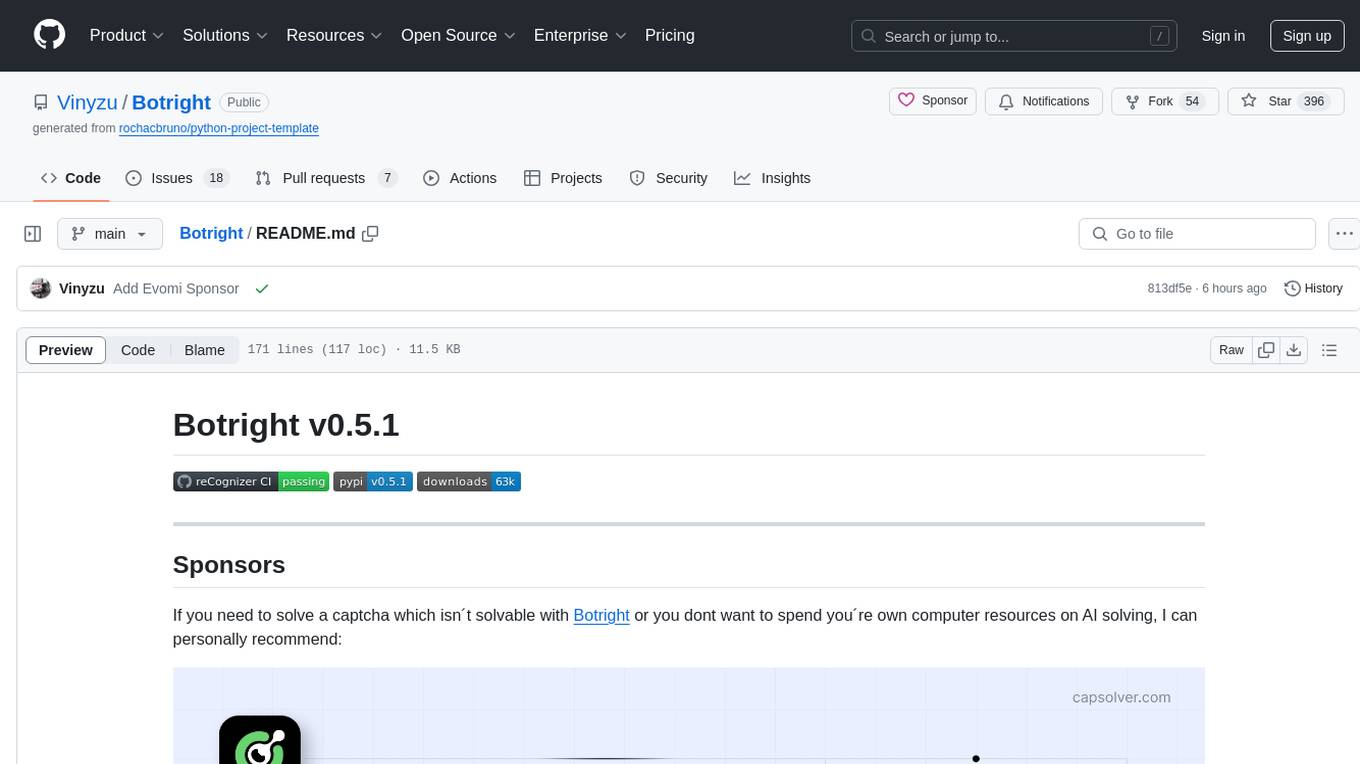
Botright
Botright is a tool designed for browser automation that focuses on stealth and captcha solving. It uses a real Chromium-based browser for enhanced stealth and offers features like browser fingerprinting and AI-powered captcha solving. The tool is suitable for developers looking to automate browser tasks while maintaining anonymity and bypassing captchas. Botright is available in async mode and can be easily integrated with existing Playwright code. It provides solutions for various captchas such as hCaptcha, reCaptcha, and GeeTest, with high success rates. Additionally, Botright offers browser stealth techniques and supports different browser functionalities for seamless automation.
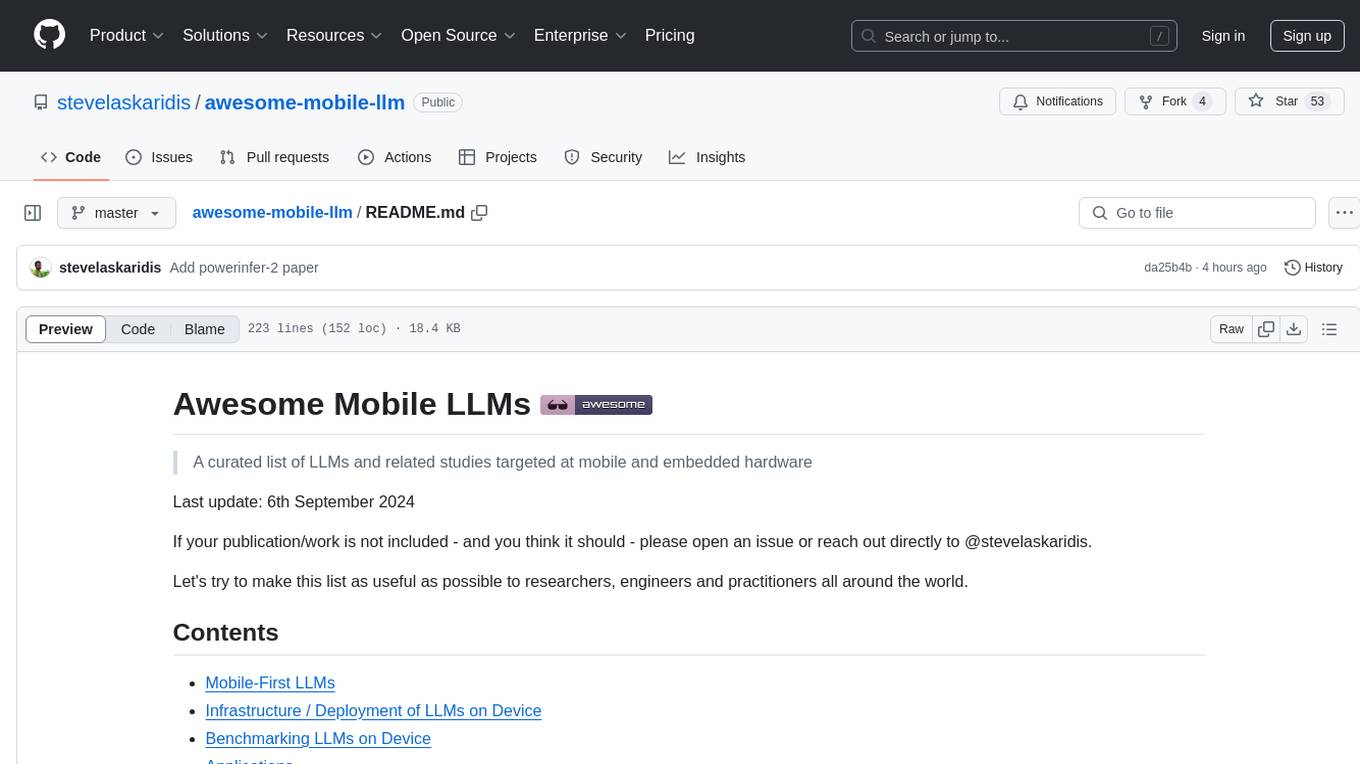
awesome-mobile-llm
Awesome Mobile LLMs is a curated list of Large Language Models (LLMs) and related studies focused on mobile and embedded hardware. The repository includes information on various LLM models, deployment frameworks, benchmarking efforts, applications, multimodal LLMs, surveys on efficient LLMs, training LLMs on device, mobile-related use-cases, industry announcements, and related repositories. It aims to be a valuable resource for researchers, engineers, and practitioners interested in mobile LLMs.
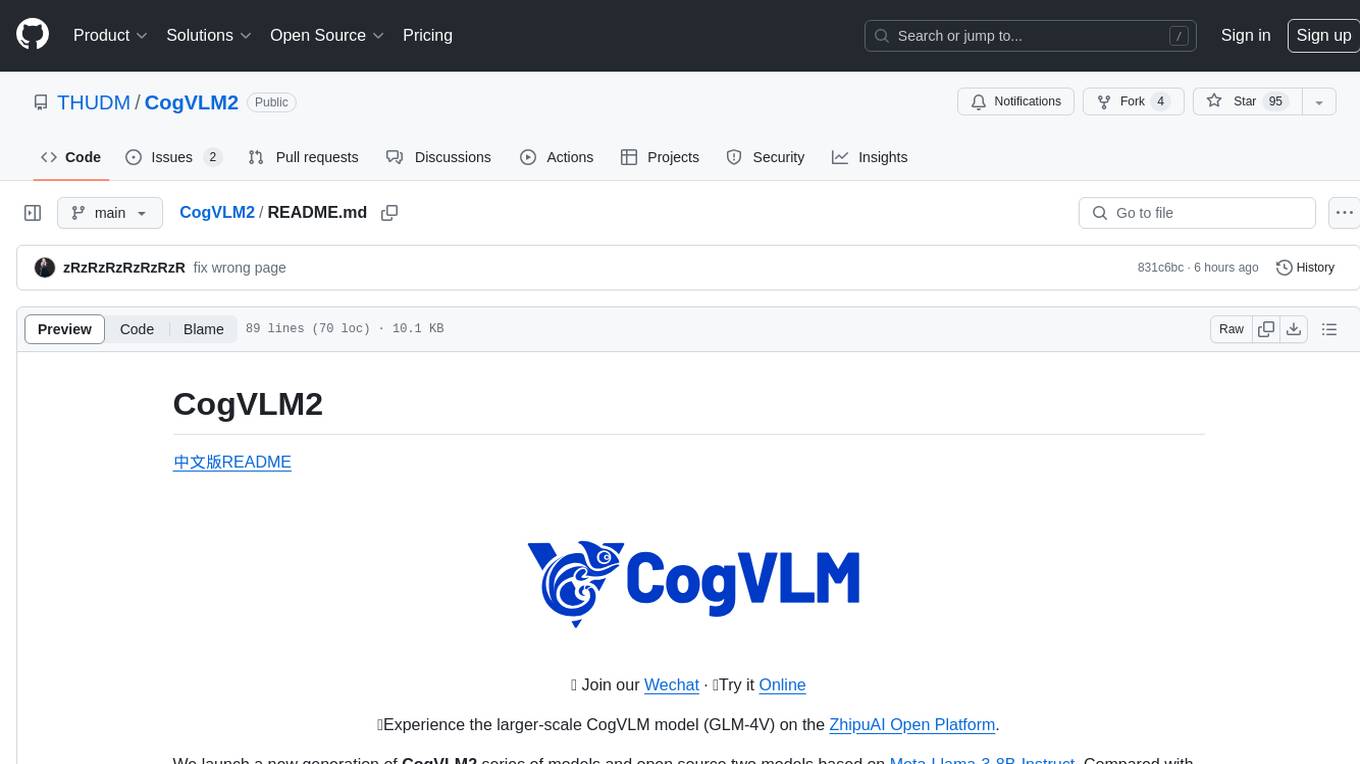
CogVLM2
CogVLM2 is a new generation of open source models that offer significant improvements in benchmarks such as TextVQA and DocVQA. It supports 8K content length, image resolution up to 1344 * 1344, and both Chinese and English languages. The project provides basic calling methods, fine-tuning examples, and OpenAI API format calling examples to help developers quickly get started with the model.
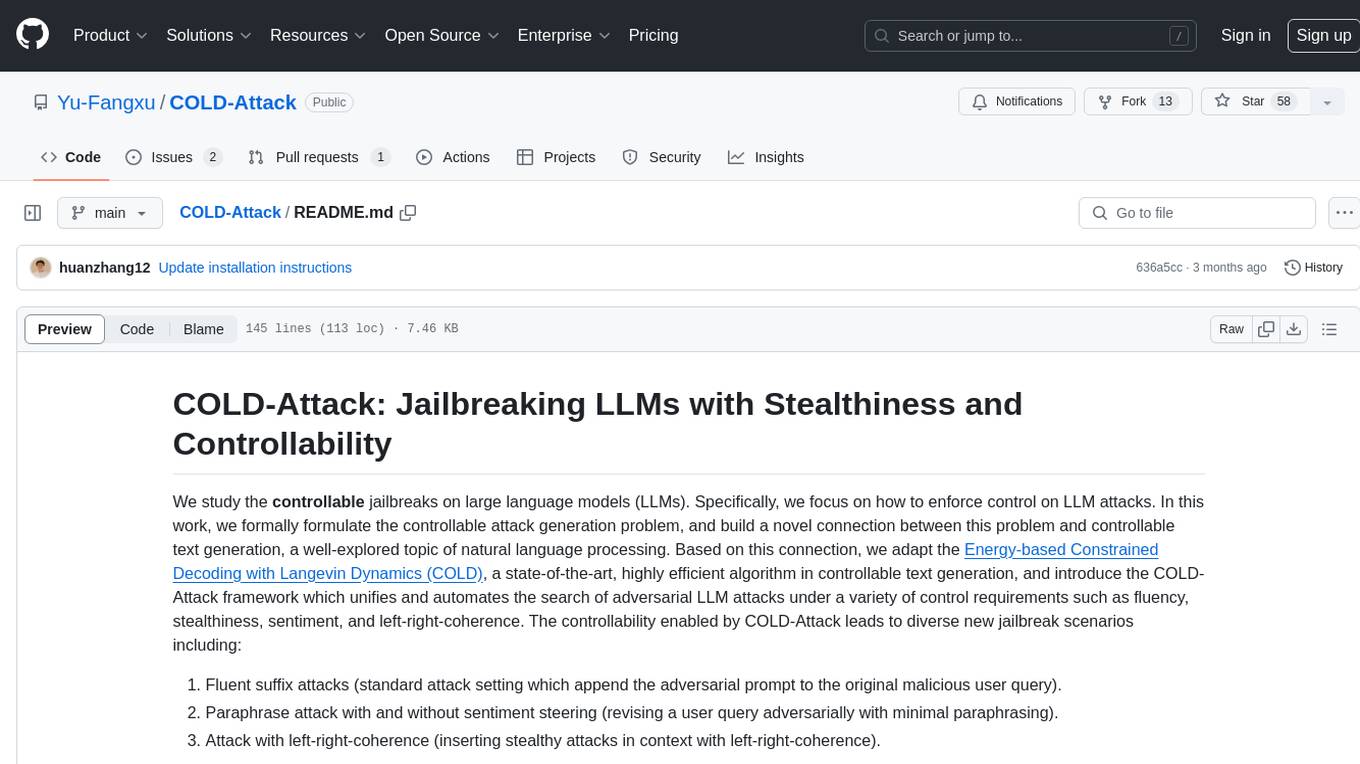
COLD-Attack
COLD-Attack is a framework designed for controllable jailbreaks on large language models (LLMs). It formulates the controllable attack generation problem and utilizes the Energy-based Constrained Decoding with Langevin Dynamics (COLD) algorithm to automate the search of adversarial LLM attacks with control over fluency, stealthiness, sentiment, and left-right-coherence. The framework includes steps for energy function formulation, Langevin dynamics sampling, and decoding process to generate discrete text attacks. It offers diverse jailbreak scenarios such as fluent suffix attacks, paraphrase attacks, and attacks with left-right-coherence.
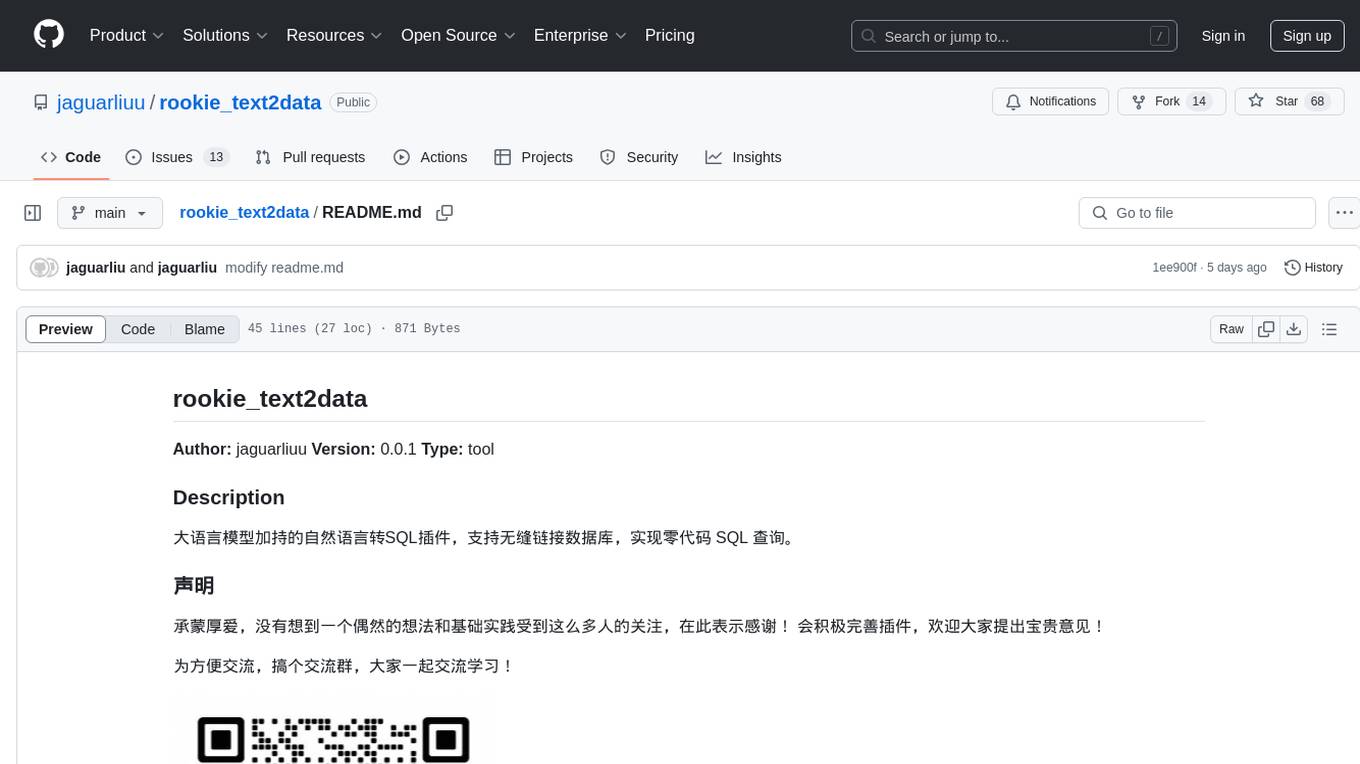
rookie_text2data
A natural language to SQL plugin powered by large language models, supporting seamless database connection for zero-code SQL queries. The plugin is designed to facilitate communication and learning among users. It supports MySQL database and various large models for natural language processing. Users can quickly install the plugin, authorize a database address, import the plugin, select a model, and perform natural language SQL queries.
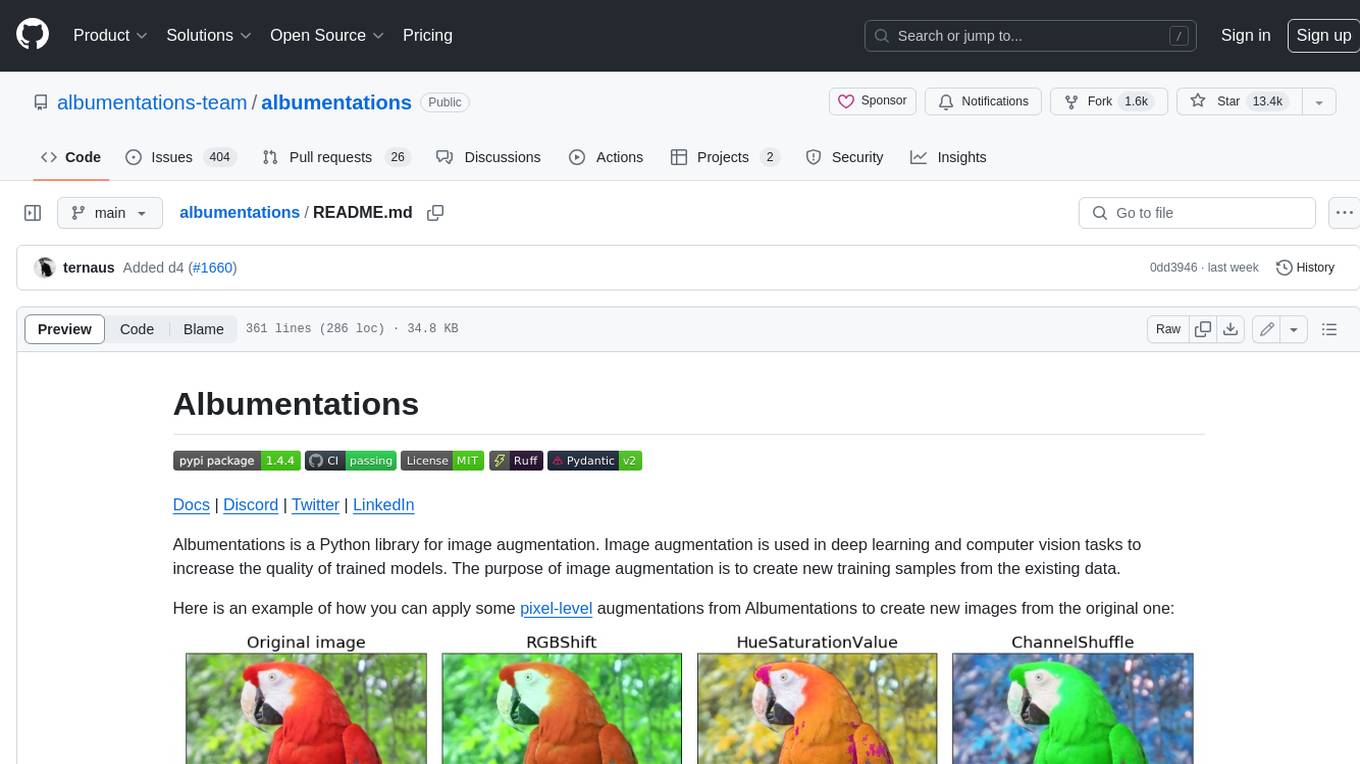
albumentations
Albumentations is a Python library for image augmentation. Image augmentation is used in deep learning and computer vision tasks to increase the quality of trained models. The purpose of image augmentation is to create new training samples from the existing data.
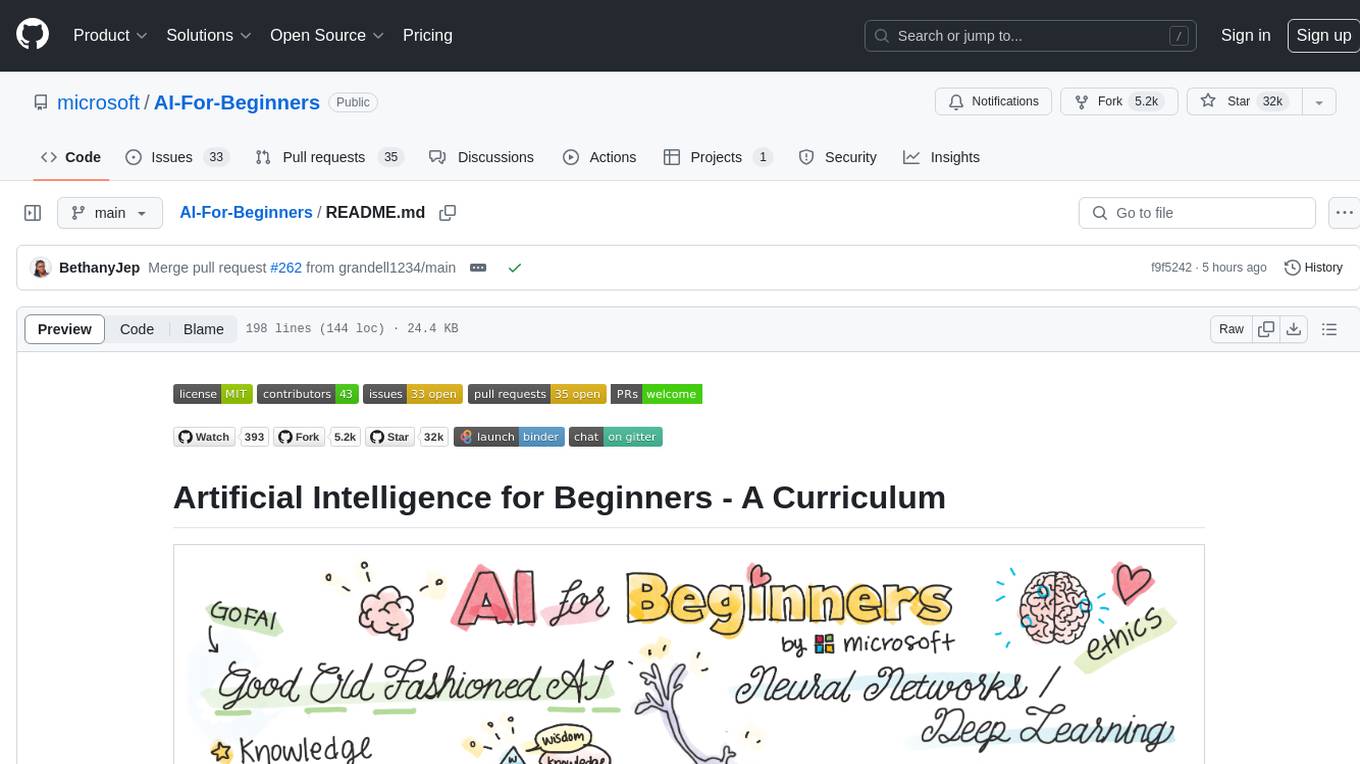
AI-For-Beginners
AI-For-Beginners is a comprehensive 12-week, 24-lesson curriculum designed by experts at Microsoft to introduce beginners to the world of Artificial Intelligence (AI). The curriculum covers various topics such as Symbolic AI, Neural Networks, Computer Vision, Natural Language Processing, Genetic Algorithms, and Multi-Agent Systems. It includes hands-on lessons, quizzes, and labs using popular frameworks like TensorFlow and PyTorch. The focus is on providing a foundational understanding of AI concepts and principles, making it an ideal starting point for individuals interested in AI.
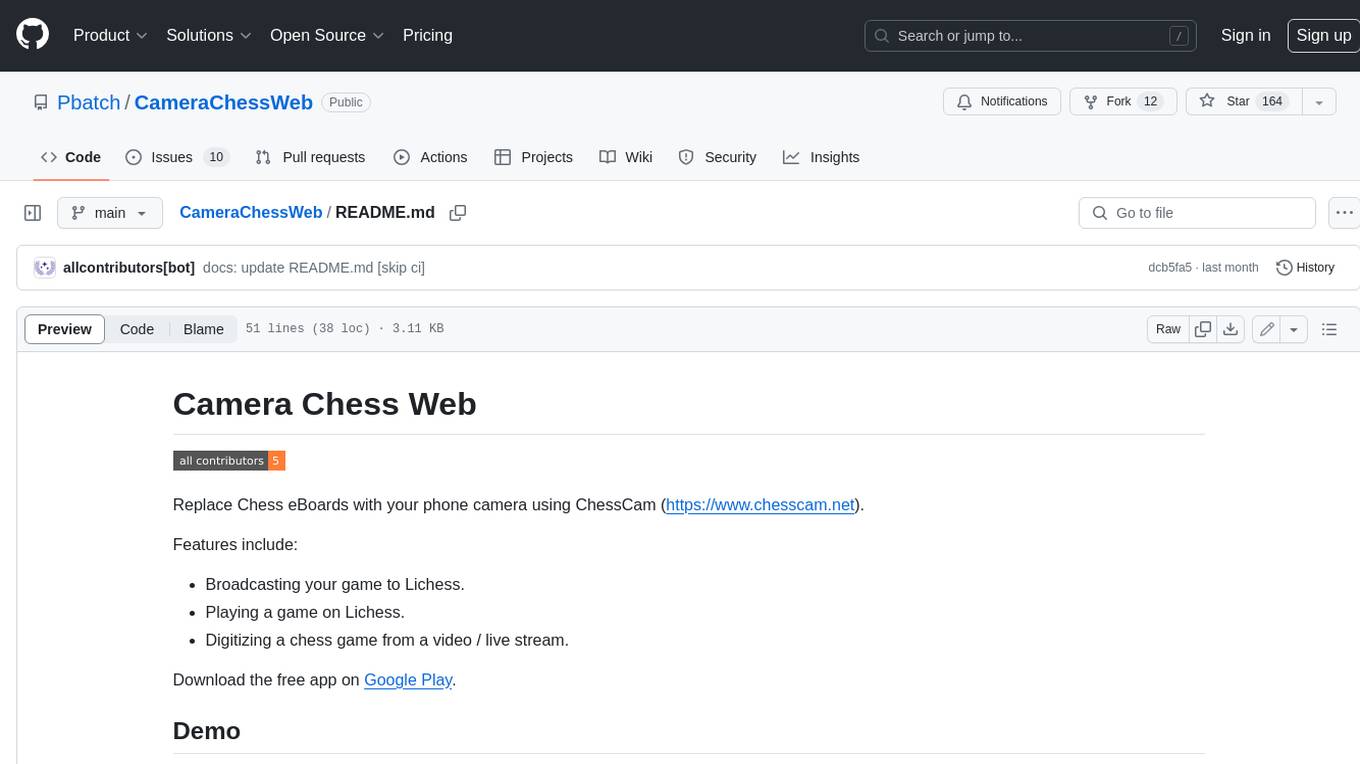
CameraChessWeb
Camera Chess Web is a tool that allows you to use your phone camera to replace chess eBoards. With Camera Chess Web, you can broadcast your game to Lichess, play a game on Lichess, or digitize a chess game from a video or live stream. Camera Chess Web is free to download on Google Play.
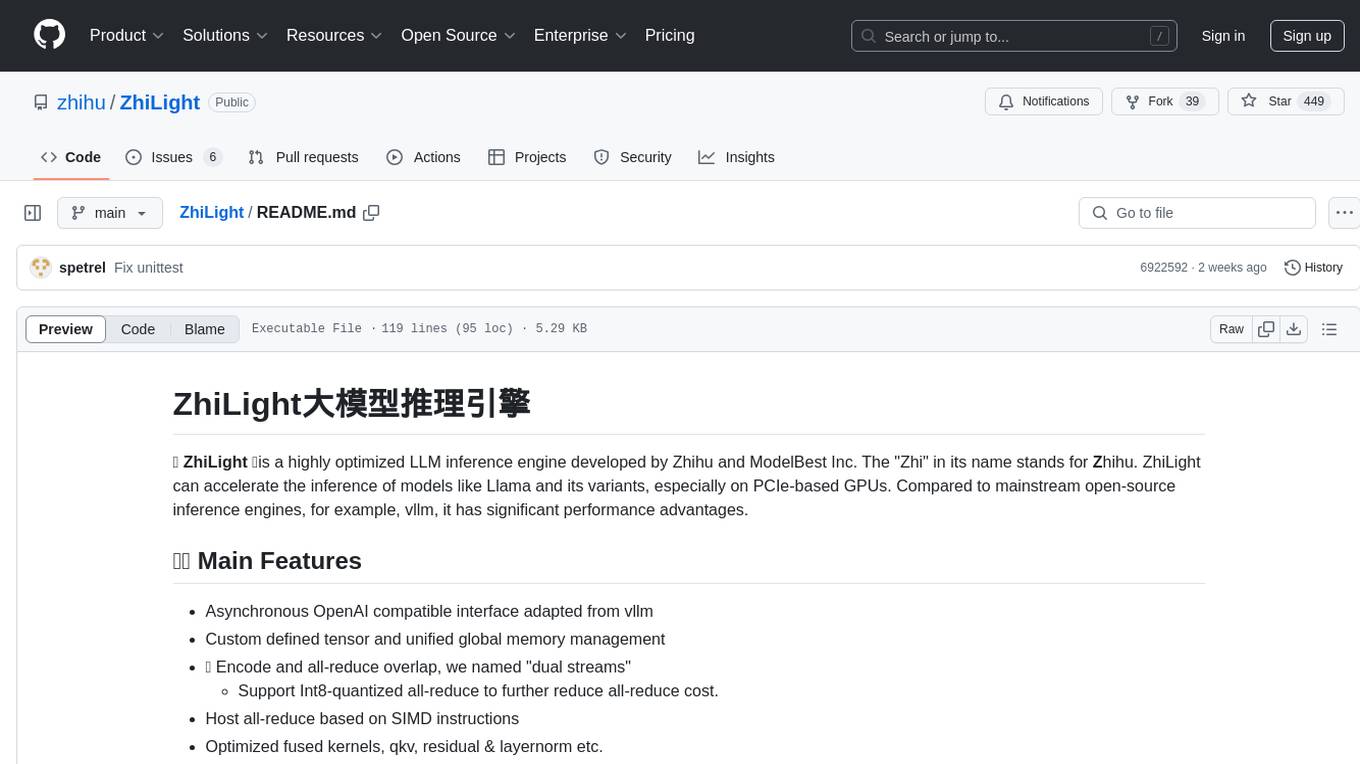
ZhiLight
ZhiLight is a highly optimized large language model (LLM) inference engine developed by Zhihu and ModelBest Inc. It accelerates the inference of models like Llama and its variants, especially on PCIe-based GPUs. ZhiLight offers significant performance advantages compared to mainstream open-source inference engines. It supports various features such as custom defined tensor and unified global memory management, optimized fused kernels, support for dynamic batch, flash attention prefill, prefix cache, and different quantization techniques like INT8, SmoothQuant, FP8, AWQ, and GPTQ. ZhiLight is compatible with OpenAI interface and provides high performance on mainstream NVIDIA GPUs with different model sizes and precisions.