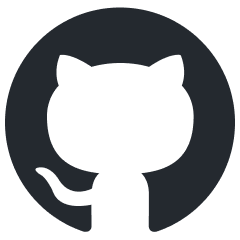
AIMNet2
None
Stars: 58
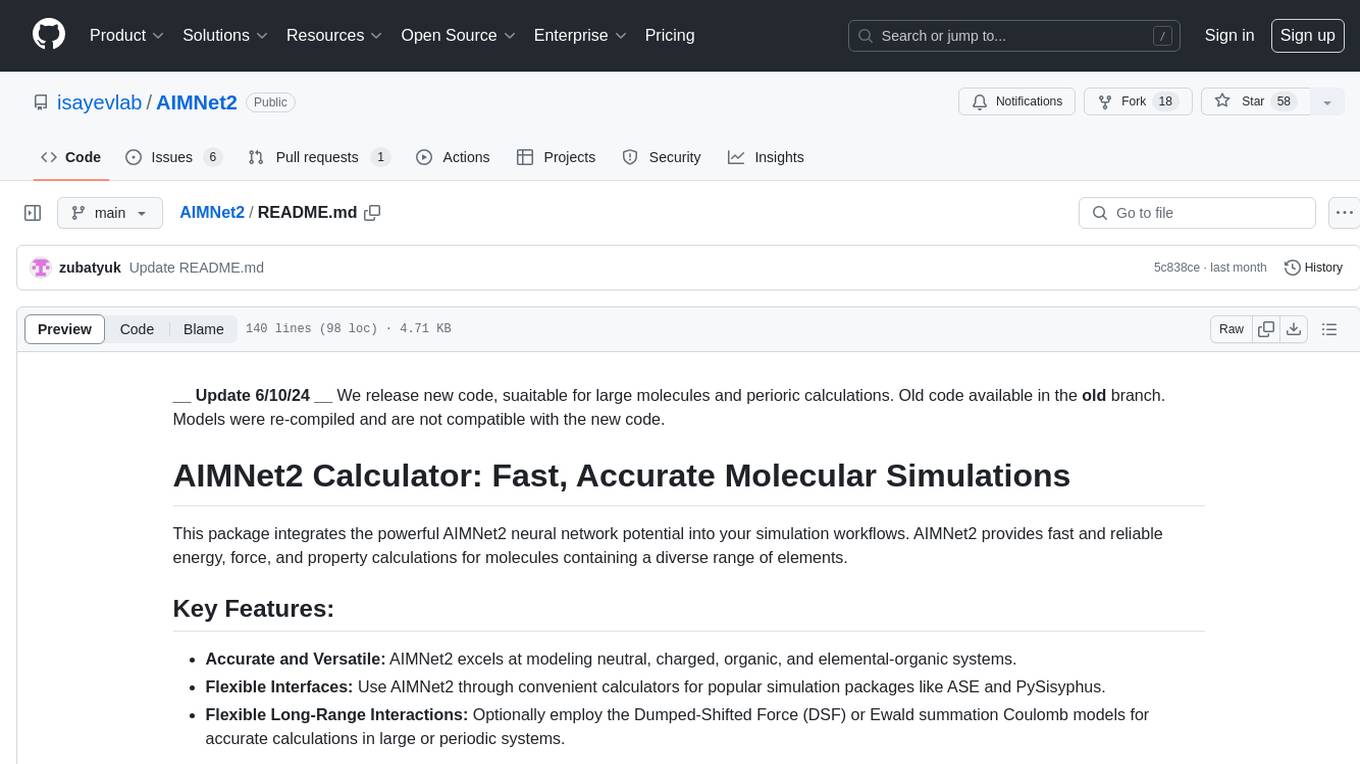
AIMNet2 Calculator is a package that integrates the AIMNet2 neural network potential into simulation workflows, providing fast and reliable energy, force, and property calculations for molecules with diverse elements. It excels at modeling various systems, offers flexible interfaces for popular simulation packages, and supports long-range interactions using DSF or Ewald summation Coulomb models. The tool is designed for accurate and versatile molecular simulations, suitable for large molecules and periodic calculations.
README:
__ Update 6/10/24 __ We release new code, suaitable for large molecules and perioric calculations. Old code available in the old branch. Models were re-compiled and are not compatible with the new code.
This package integrates the powerful AIMNet2 neural network potential into your simulation workflows. AIMNet2 provides fast and reliable energy, force, and property calculations for molecules containing a diverse range of elements.
- Accurate and Versatile: AIMNet2 excels at modeling neutral, charged, organic, and elemental-organic systems.
- Flexible Interfaces: Use AIMNet2 through convenient calculators for popular simulation packages like ASE and PySisyphus.
- Flexible Long-Range Interactions: Optionally employ the Dumped-Shifted Force (DSF) or Ewald summation Coulomb models for accurate calculations in large or periodic systems.
While package is in alpha stage and repository is private, please install into your conda envoronment manually with
# install requirements
conda install -y pytorch pytorch-cuda=12.1 -c pytorch -c nvidia
conda install -y -c pyg pytorch-cluster
conda install -y -c conda-forge openbabel ase
## pysis requirements
conda install -y -c conda-forge autograd dask distributed h5py fabric jinja2 joblib matplotlib numpy natsort psutil pyyaml rmsd scipy sympy scikit-learn
# now should not do any pip installs
pip install git+https://github.com/eljost/pysisyphus.git
# finally, this repo
git clone [email protected]:zubatyuk/aimnet2calc.git
cd aimnet2calc
python setup.py install
from aimnet2calc import AIMNet2ASE
calc = AIMNet2ASE('aimnet2')
To specify total molecular charge and spin multiplicity, use optional charge
and mult
keyword arguments, or set_charge
and set_mult
methods:
calc = AIMNet2ASE('aimnet2', charge=1)
atoms1.calc = calc
# calculations on atoms1 will be done with charge 1
....
atoms2.calc = calc
calc.set_charge(-2)
# calculations on atoms1 will be done with charge -2
PySisyphus [https://pysisyphus.readthedocs.io]
from aimnet2calc import AIMNet2PySis
calc = AIMNet2PySis('aimnet2')
This produces standard PySisyphus calculator.
Instead of Pysis
command line utility, use aimnet2pysis
. This registeres AIMNet2 calculator with PySisyphus.
Example calc
section for PySisyphus YAML files:
calc:
type: aimnet # use AIMNet2 calculator
model: aimnet2_b973c # use aimnet2_b973c_0.jpt model
from aimnet2calc import AIMNet2Calculator
calc = AIMNet2Calculator('aimnet2')
will load default AIMNet2 model aimnet2_wb97m_0.jpt as defined at aimnet2calc/models.py
. If file does not exist on the machine, it will be downloaded from aimnet-model-zoo repository.
calc = AIMNet2Calculator('/path/to_a/model.jpt')
will load model from the file.
The calculator accepts a dictionary containig lists, numpy arrays, torch tensors, or anything that could be accepted by torch.as_tensor
.
The input could be for a single molecule (dict keys and shapes):
coord: (B, N, 3) # atomic coordinates in Angstrom
numbers (B, N) # atomic numbers
charge (B,) # molecular charge
mult (B,) # spin multiplicity, optional
or for a concatenation of molecules:
coord: (N, 3) # atomic coordinates in Angstrom
numbers (N,) # atomic numbers
charge (B,) # molecular charge
mult (B,) # spin multiplicity, optional
mol_idx (N,) # molecule index for each atom, should contain integers in increasing order, with (B-1) is the maximum number.
where B
is the number of molecules, N
is number of atoms.
results = calc(data, forces=False, stress=False, hessian=False)
results
would be a dictionary of PyTorch tensors containing energy
, charges
, and possibly forces
, stress
and hessian
if requested.
By default, Coulomb energy is calculated in O(N^2) manner, e.g. pair interaction between every pair of atoms in system. For very large or periodic systems, O(N) Dumped-Shifted Force Coulomb model could be employed doi: 10.1063/1.2206581. With AIMNet2Calculator
interface, switch between standard and DSF Coulomb implementations im AIMNet2 models:
# switch to O(N)
calc.set_lrcoulomb_method('dsf', cutoff=15.0, dsf_alpha=0.2)
# switch to O(N^2), not suitable for PBC
calc.set_lrcoulomb_method('simple')
For Tasks:
Click tags to check more tools for each tasksFor Jobs:
Alternative AI tools for AIMNet2
Similar Open Source Tools
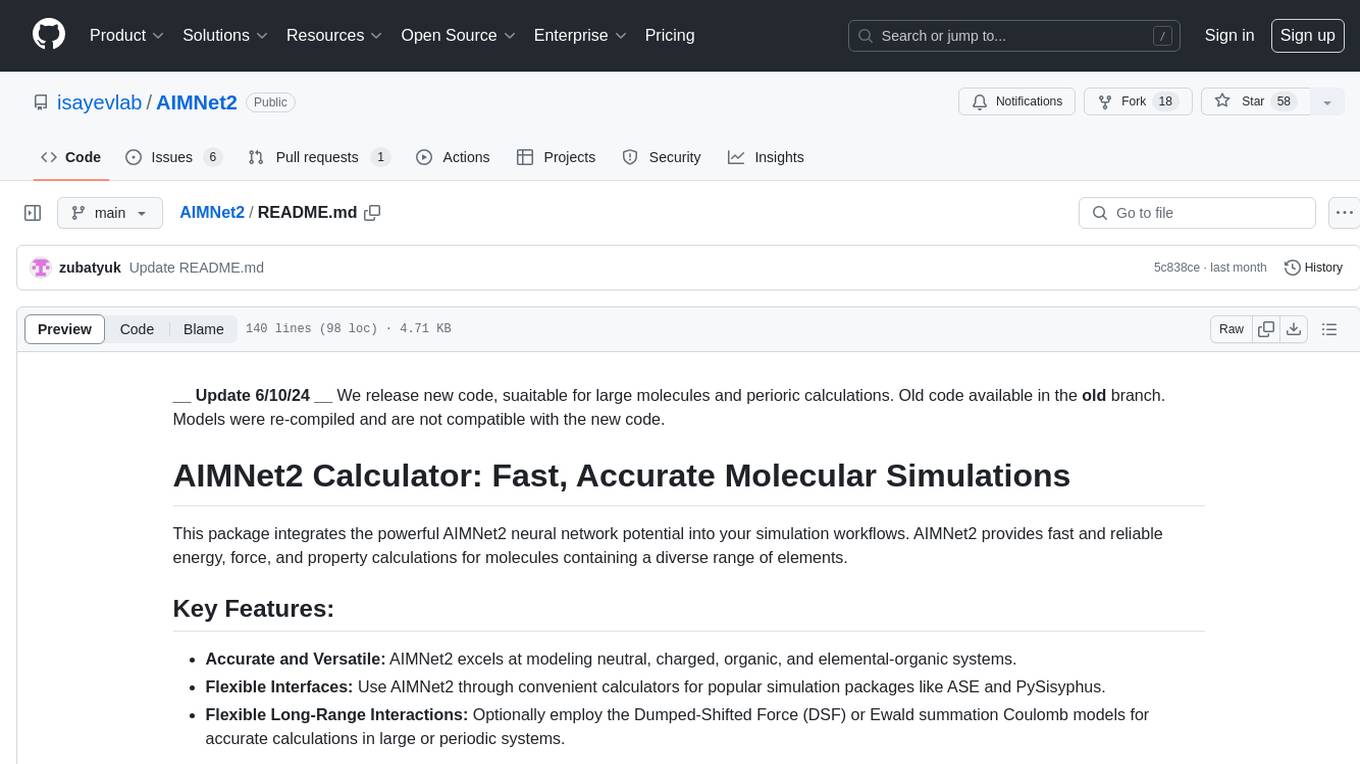
AIMNet2
AIMNet2 Calculator is a package that integrates the AIMNet2 neural network potential into simulation workflows, providing fast and reliable energy, force, and property calculations for molecules with diverse elements. It excels at modeling various systems, offers flexible interfaces for popular simulation packages, and supports long-range interactions using DSF or Ewald summation Coulomb models. The tool is designed for accurate and versatile molecular simulations, suitable for large molecules and periodic calculations.
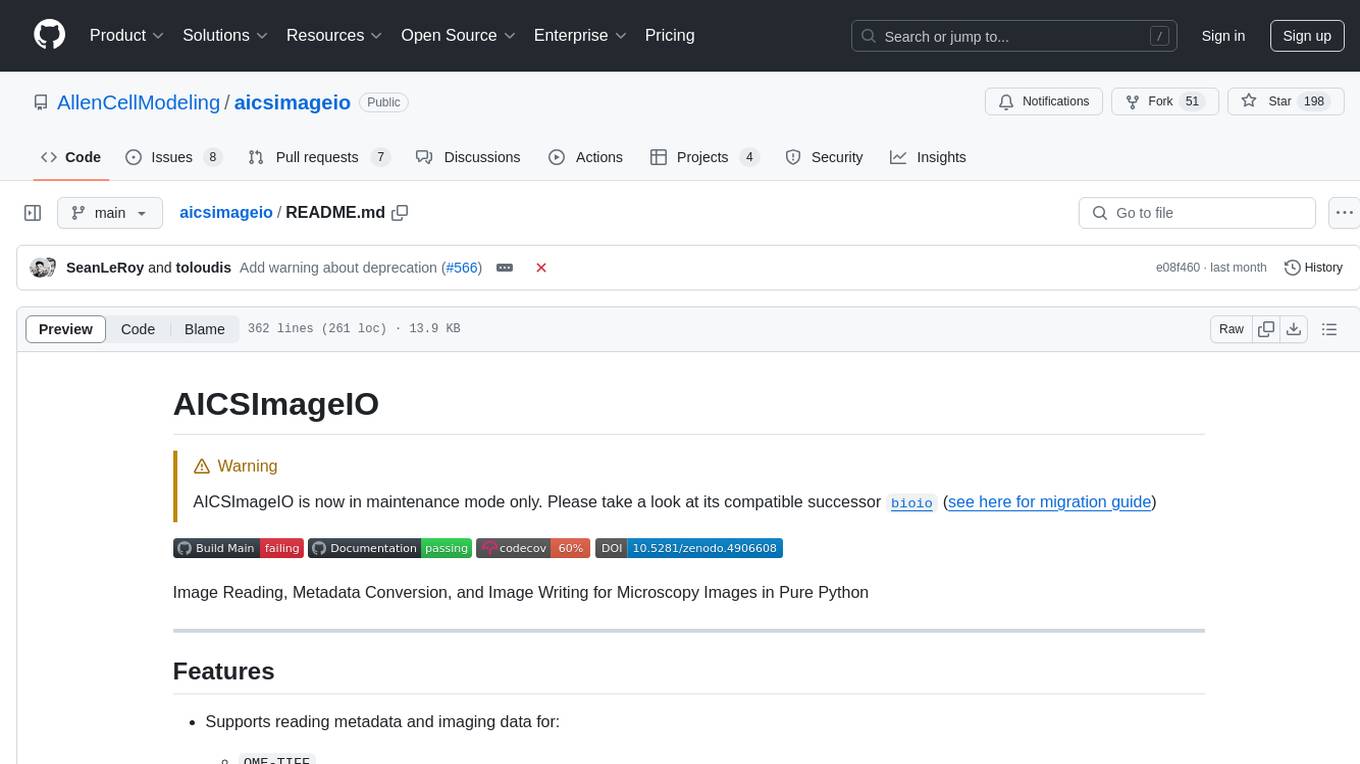
aicsimageio
AICSImageIO is a Python tool for Image Reading, Metadata Conversion, and Image Writing for Microscopy Images. It supports various file formats like OME-TIFF, TIFF, ND2, DV, CZI, LIF, PNG, GIF, and Bio-Formats. Users can read and write metadata and imaging data, work with different file systems like local paths, HTTP URLs, s3fs, and gcsfs. The tool provides functionalities for full image reading, delayed image reading, mosaic image reading, metadata reading, xarray coordinate plane attachment, cloud IO support, and saving to OME-TIFF. It also offers benchmarking and developer resources.
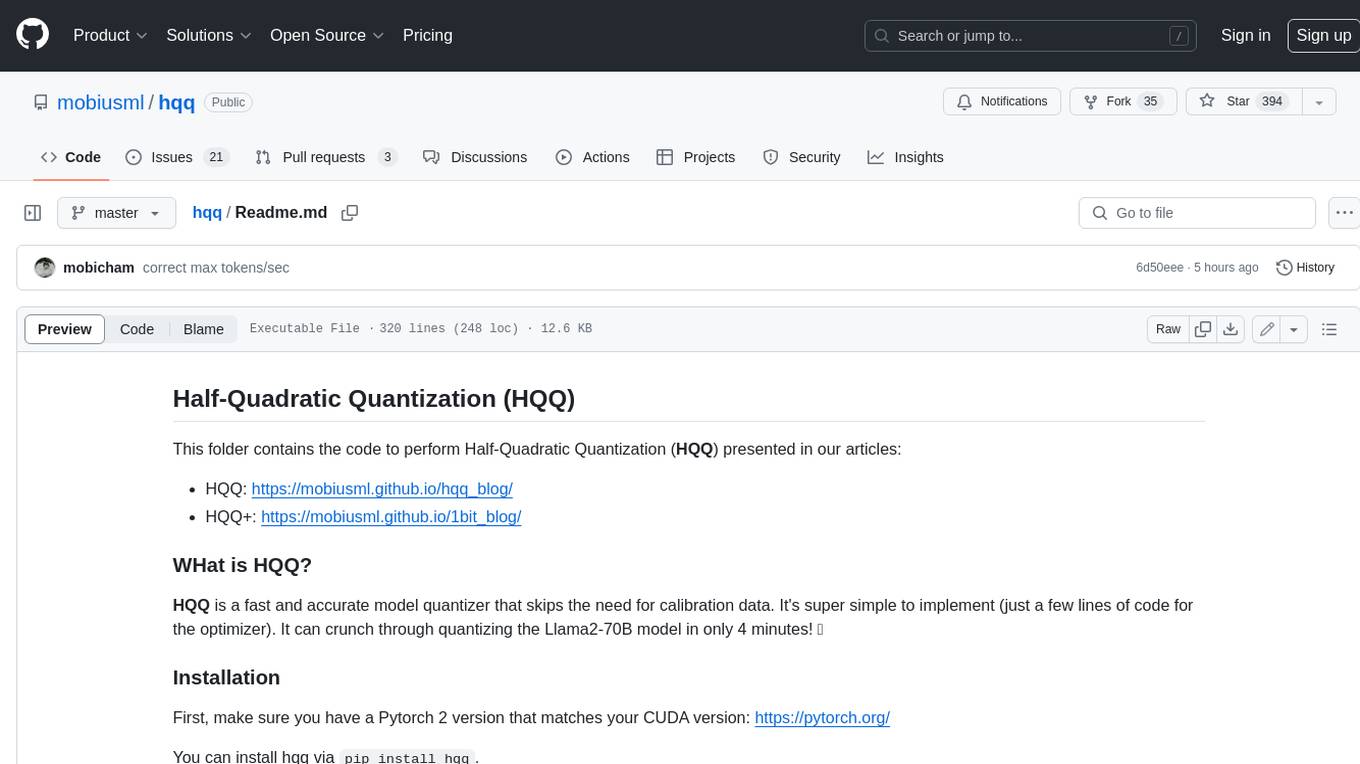
hqq
HQQ is a fast and accurate model quantizer that skips the need for calibration data. It's super simple to implement (just a few lines of code for the optimizer). It can crunch through quantizing the Llama2-70B model in only 4 minutes! 🚀
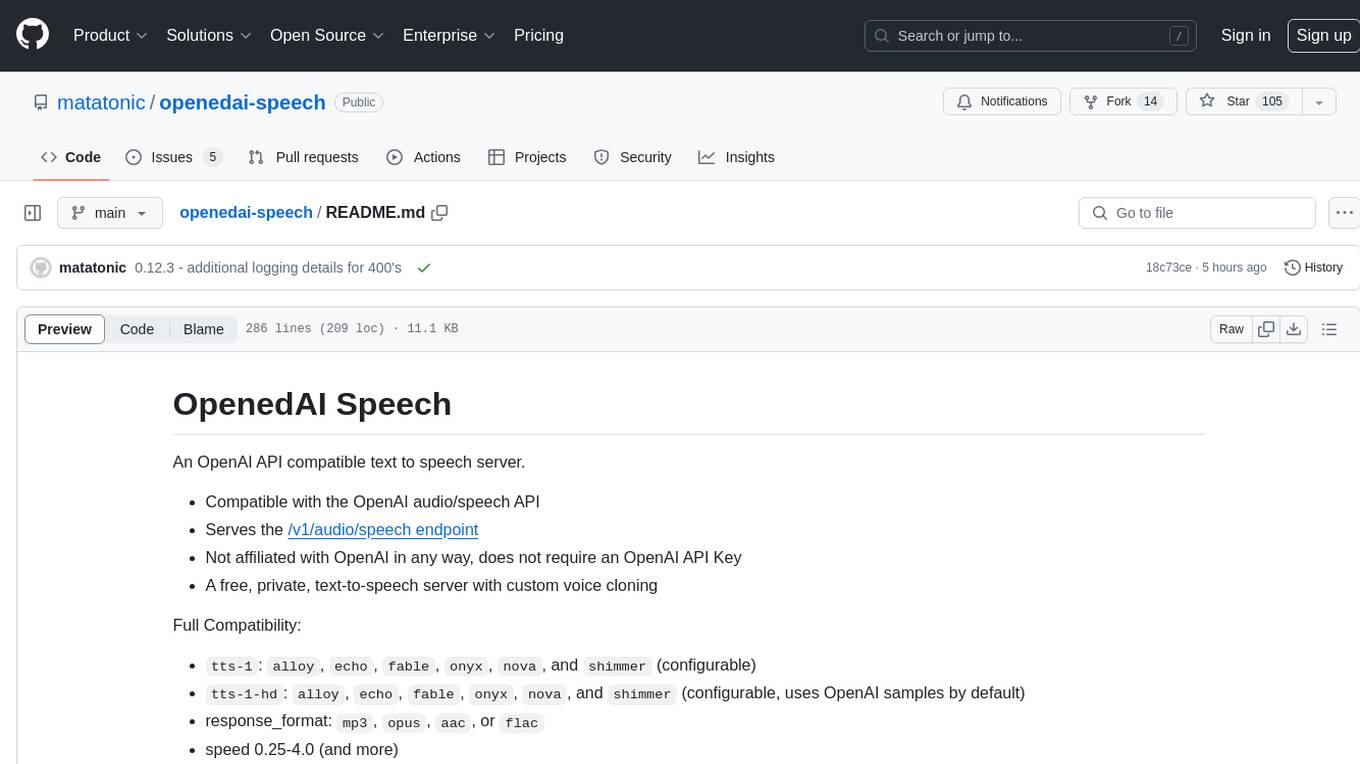
openedai-speech
OpenedAI Speech is a free, private text-to-speech server compatible with the OpenAI audio/speech API. It offers custom voice cloning and supports various models like tts-1 and tts-1-hd. Users can map their own piper voices and create custom cloned voices. The server provides multilingual support with XTTS voices and allows fixing incorrect sounds with regex. Recent changes include bug fixes, improved error handling, and updates for multilingual support. Installation can be done via Docker or manual setup, with usage instructions provided. Custom voices can be created using Piper or Coqui XTTS v2, with guidelines for preparing audio files. The tool is suitable for tasks like generating speech from text, creating custom voices, and multilingual text-to-speech applications.
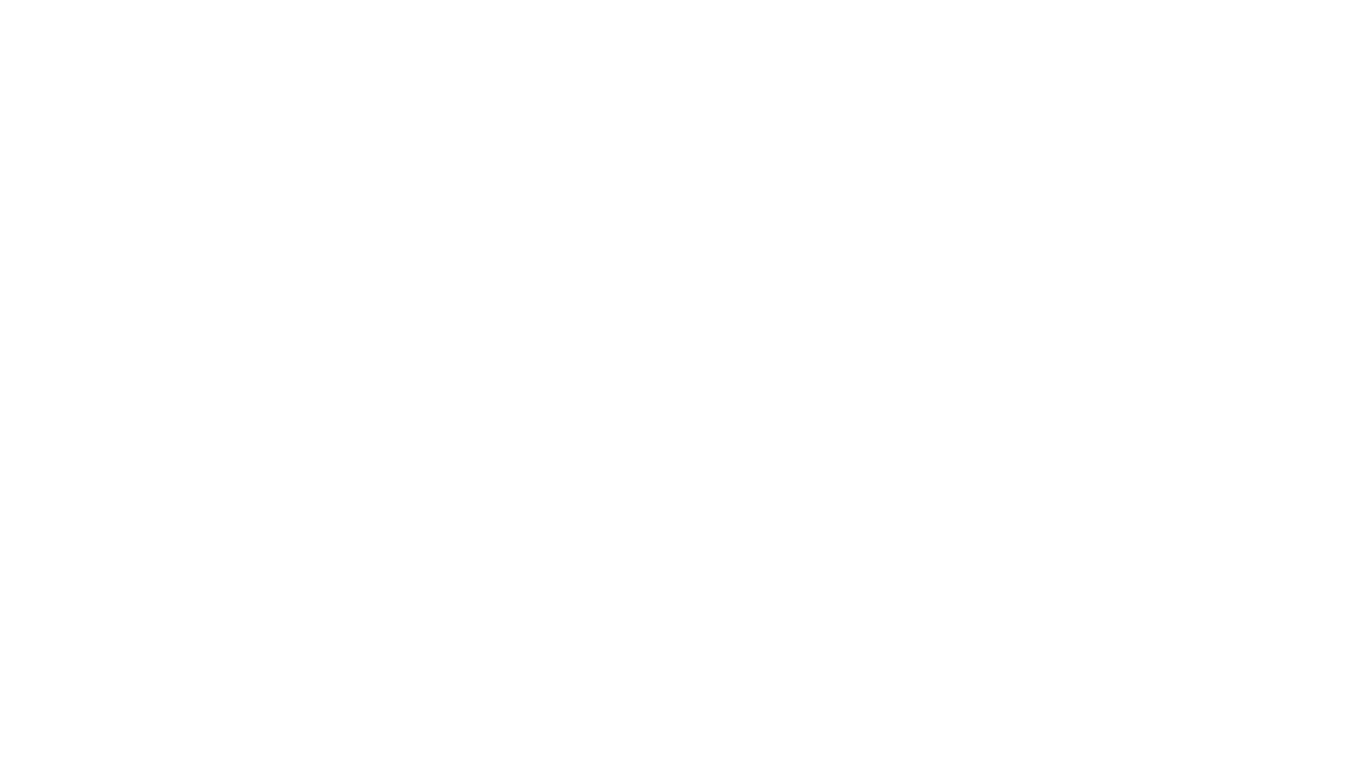
upgini
Upgini is an intelligent data search engine with a Python library that helps users find and add relevant features to their ML pipeline from various public, community, and premium external data sources. It automates the optimization of connected data sources by generating an optimal set of machine learning features using large language models, GraphNNs, and recurrent neural networks. The tool aims to simplify feature search and enrichment for external data to make it a standard approach in machine learning pipelines. It democratizes access to data sources for the data science community.
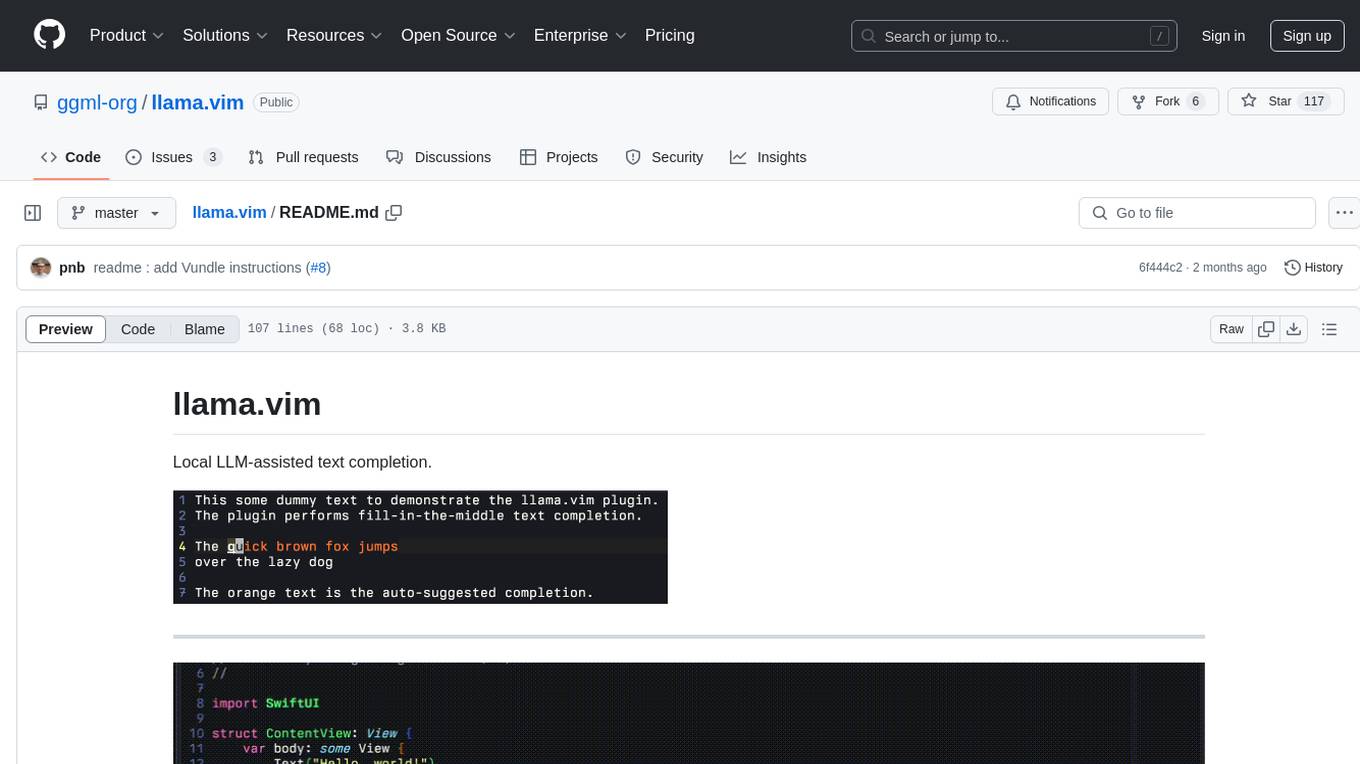
llama.vim
llama.vim is a plugin that provides local LLM-assisted text completion for Vim users. It offers features such as auto-suggest on cursor movement, manual suggestion toggling, suggestion acceptance with Tab and Shift+Tab, control over text generation time, context configuration, ring context with chunks from open and edited files, and performance stats display. The plugin requires a llama.cpp server instance to be running and supports FIM-compatible models. It aims to be simple, lightweight, and provide high-quality and performant local FIM completions even on consumer-grade hardware.
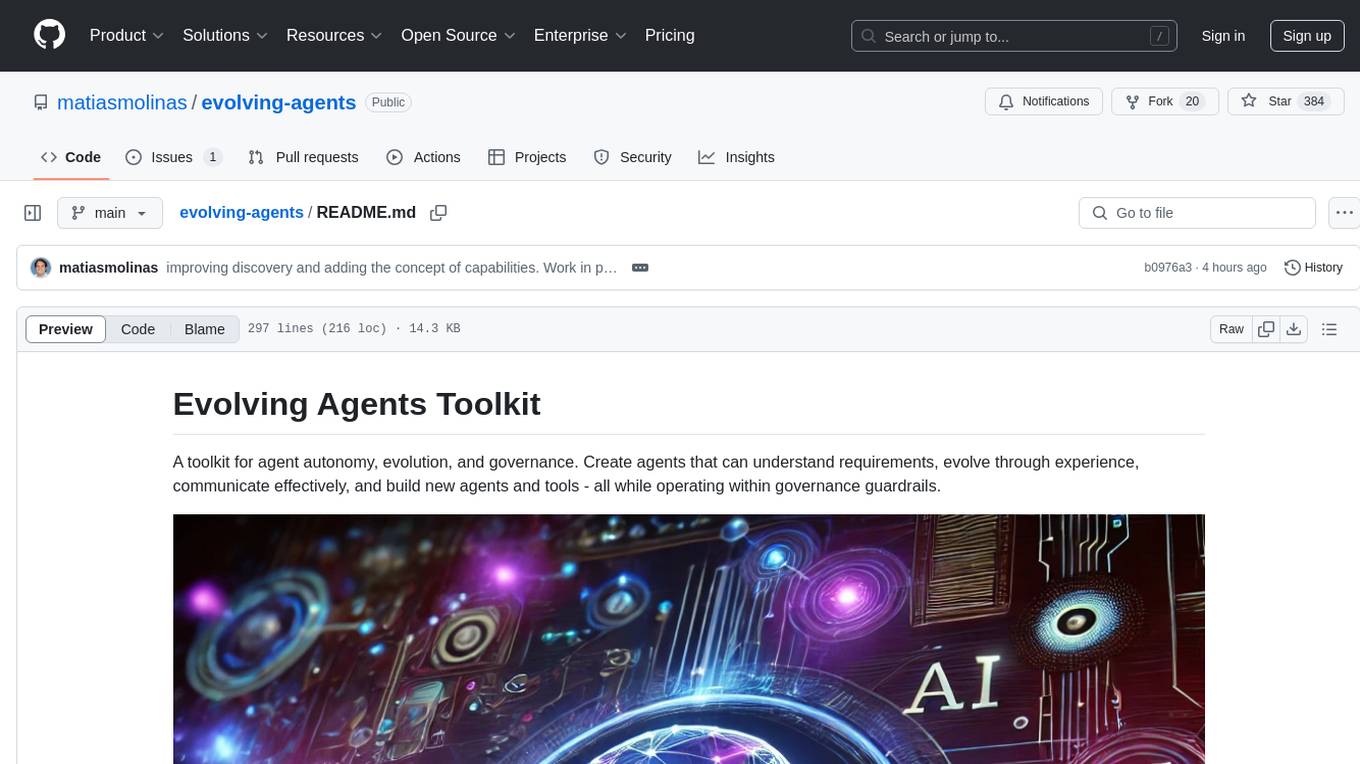
evolving-agents
A toolkit for agent autonomy, evolution, and governance enabling agents to learn from experience, collaborate, communicate, and build new tools within governance guardrails. It focuses on autonomous evolution, agent self-discovery, governance firmware, self-building systems, and agent-centric architecture. The toolkit leverages existing frameworks to enable agent autonomy and self-governance, moving towards truly autonomous AI systems.
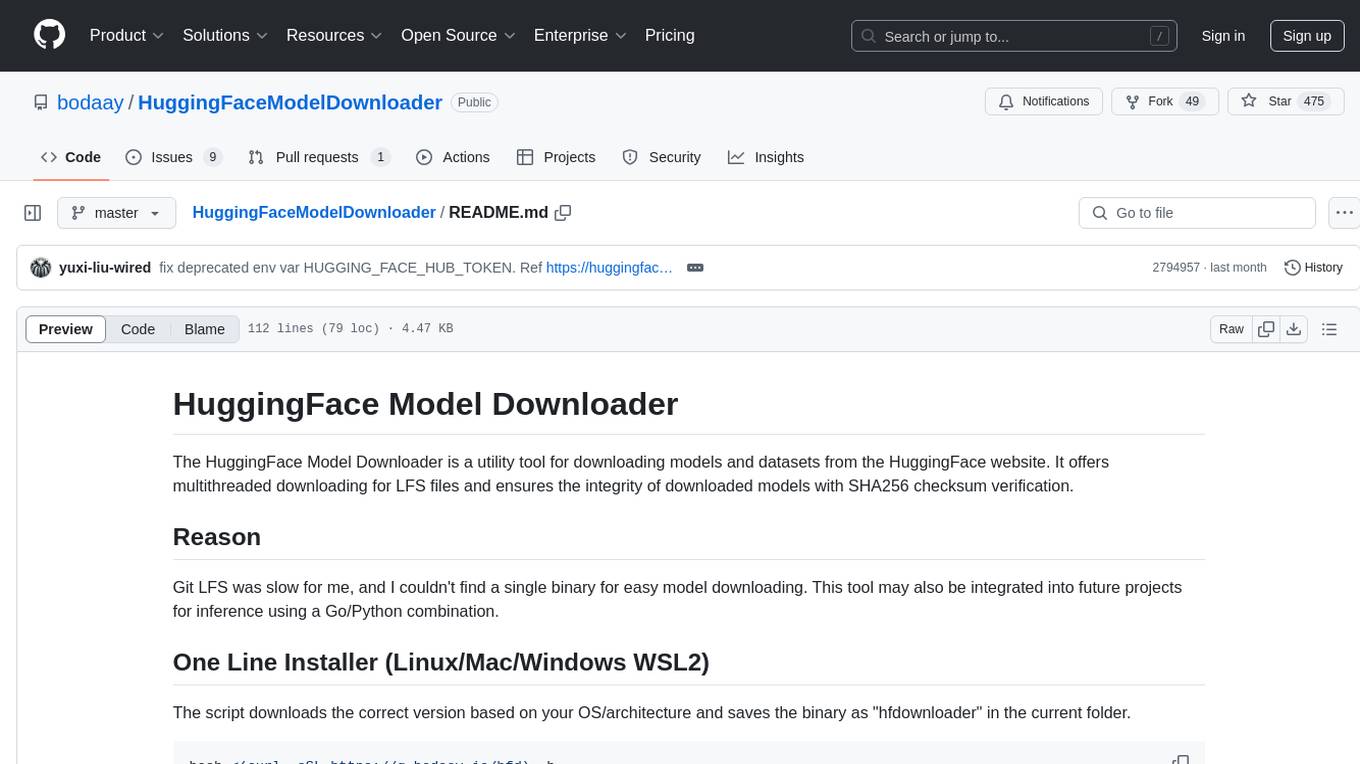
HuggingFaceModelDownloader
The HuggingFace Model Downloader is a utility tool for downloading models and datasets from the HuggingFace website. It offers multithreaded downloading for LFS files and ensures the integrity of downloaded models with SHA256 checksum verification. The tool provides features such as nested file downloading, filter downloads for specific LFS model files, support for HuggingFace Access Token, and configuration file support. It can be used as a library or a single binary for easy model downloading and inference in projects.
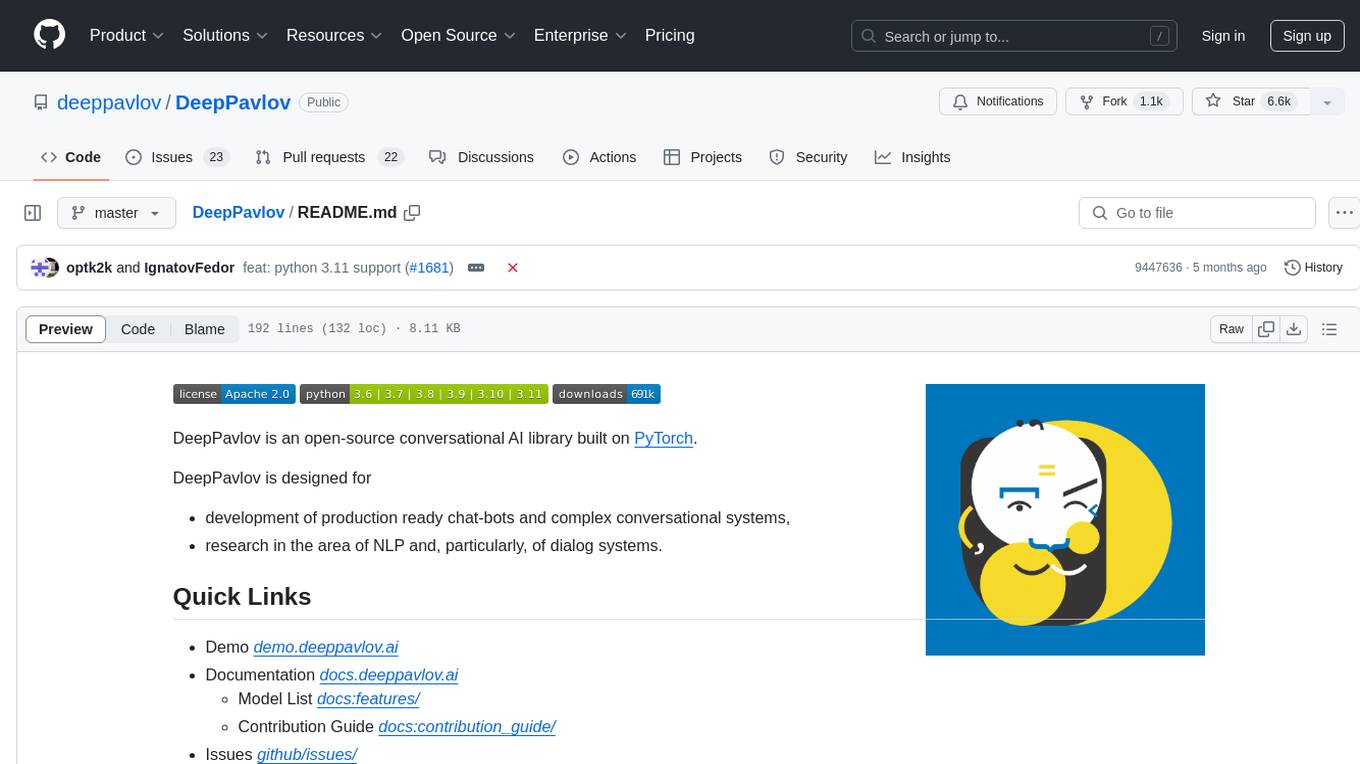
DeepPavlov
DeepPavlov is an open-source conversational AI library built on PyTorch. It is designed for the development of production-ready chatbots and complex conversational systems, as well as for research in the area of NLP and dialog systems. The library offers a wide range of models for tasks such as Named Entity Recognition, Intent/Sentence Classification, Question Answering, Sentence Similarity/Ranking, Syntactic Parsing, and more. DeepPavlov also provides embeddings like BERT, ELMo, and FastText for various languages, along with AutoML capabilities and integrations with REST API, Socket API, and Amazon AWS.
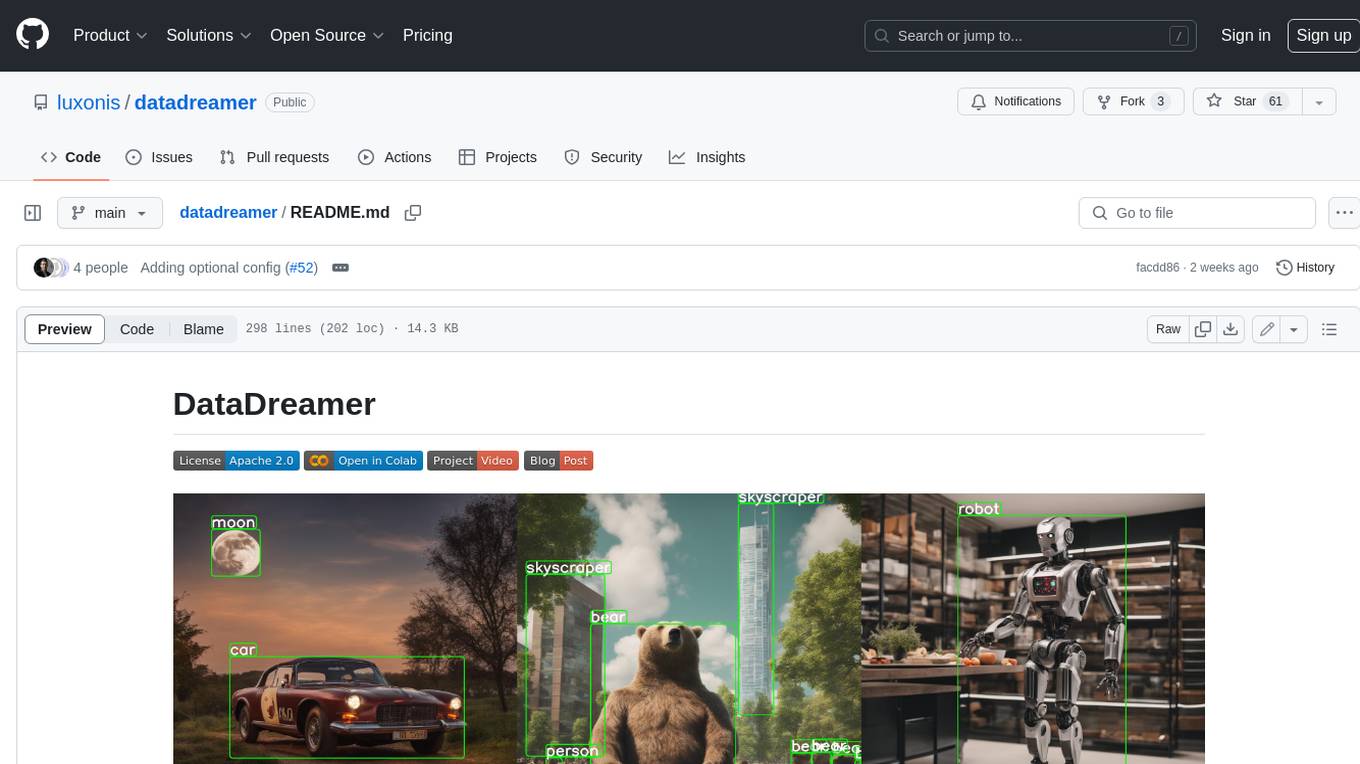
datadreamer
DataDreamer is an advanced toolkit designed to facilitate the development of edge AI models by enabling synthetic data generation, knowledge extraction from pre-trained models, and creation of efficient and potent models. It eliminates the need for extensive datasets by generating synthetic datasets, leverages latent knowledge from pre-trained models, and focuses on creating compact models suitable for integration into any device and performance for specialized tasks. The toolkit offers features like prompt generation, image generation, dataset annotation, and tools for training small-scale neural networks for edge deployment. It provides hardware requirements, usage instructions, available models, and limitations to consider while using the library.
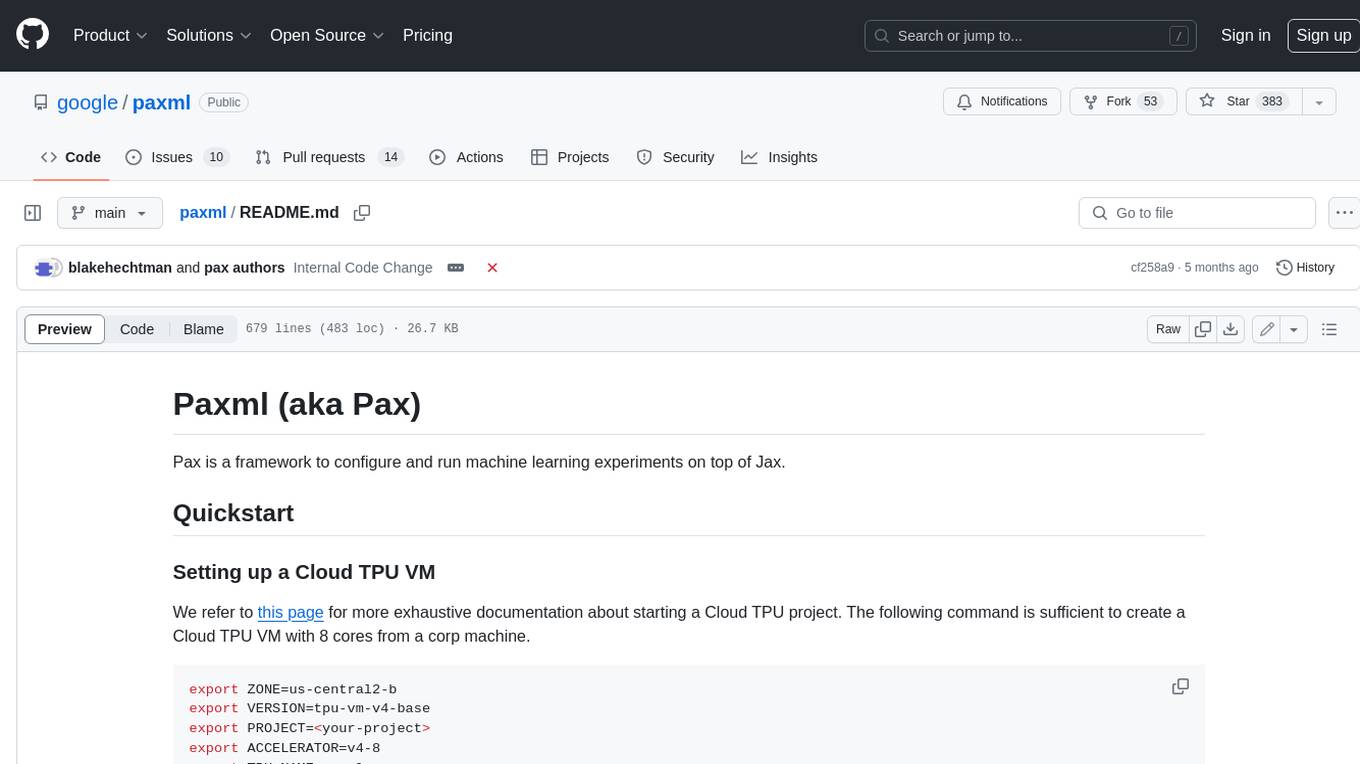
paxml
Pax is a framework to configure and run machine learning experiments on top of Jax.
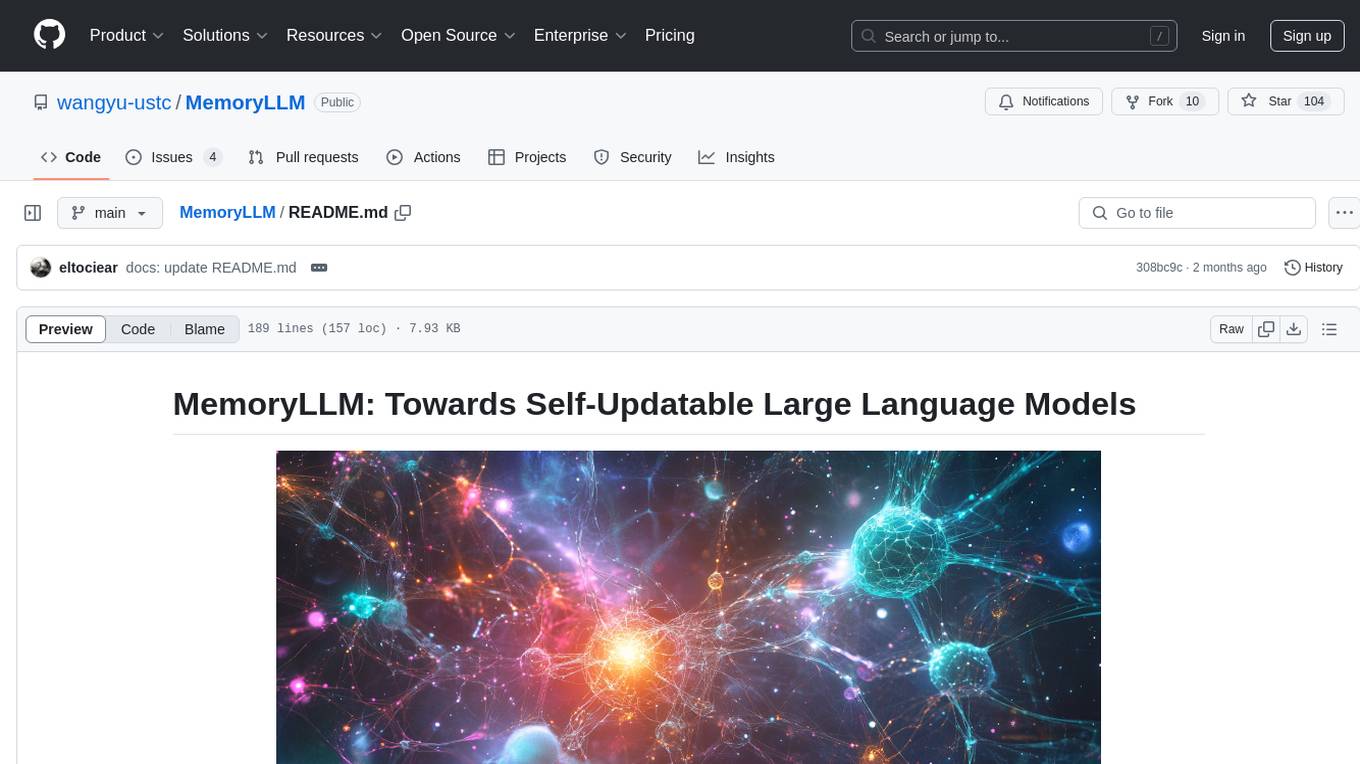
MemoryLLM
MemoryLLM is a large language model designed for self-updating capabilities. It offers pretrained models with different memory capacities and features, such as chat models. The repository provides training code, evaluation scripts, and datasets for custom experiments. MemoryLLM aims to enhance knowledge retention and performance on various natural language processing tasks.
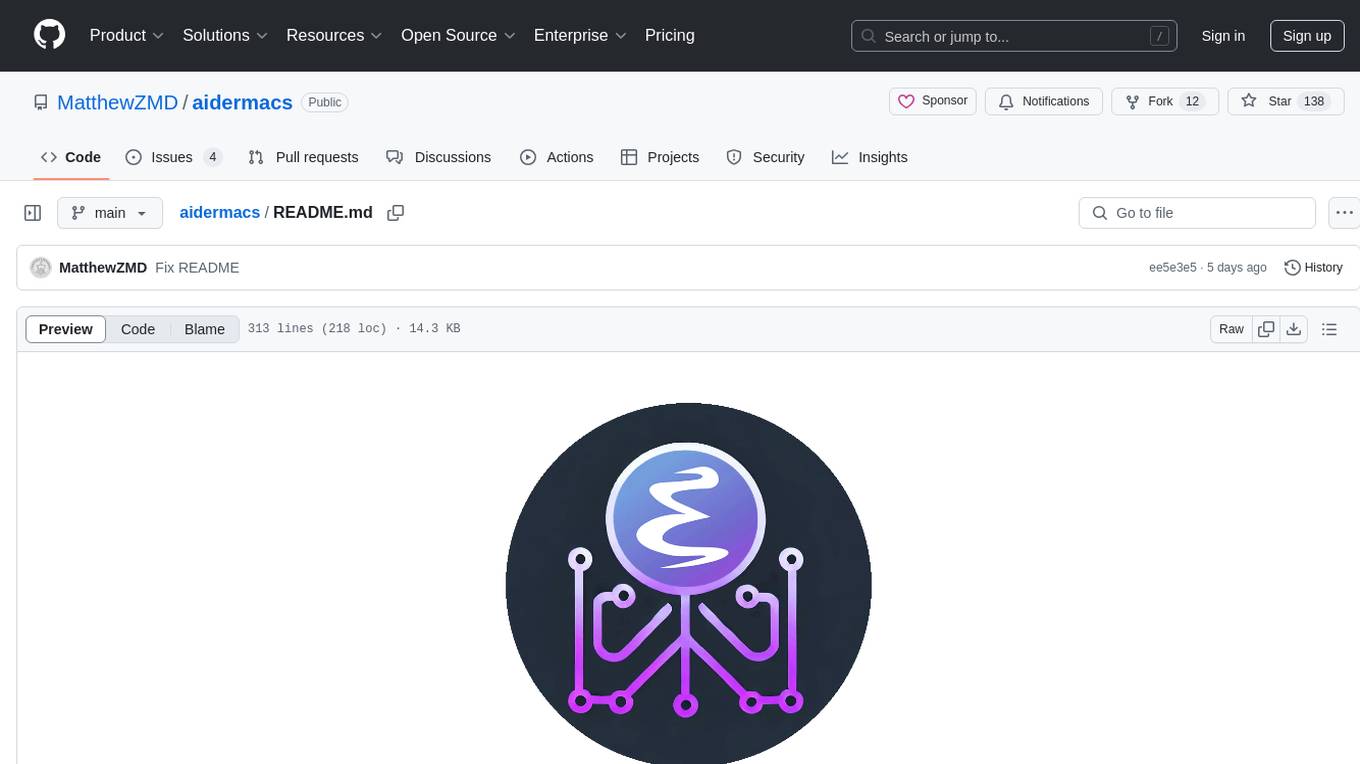
aidermacs
Aidermacs is an AI pair programming tool for Emacs that integrates Aider, a powerful open-source AI pair programming tool. It provides top performance on the SWE Bench, support for multi-file edits, real-time file synchronization, and broad language support. Aidermacs delivers an Emacs-centric experience with features like intelligent model selection, flexible terminal backend support, smarter syntax highlighting, enhanced file management, and streamlined transient menus. It thrives on community involvement, encouraging contributions, issue reporting, idea sharing, and documentation improvement.
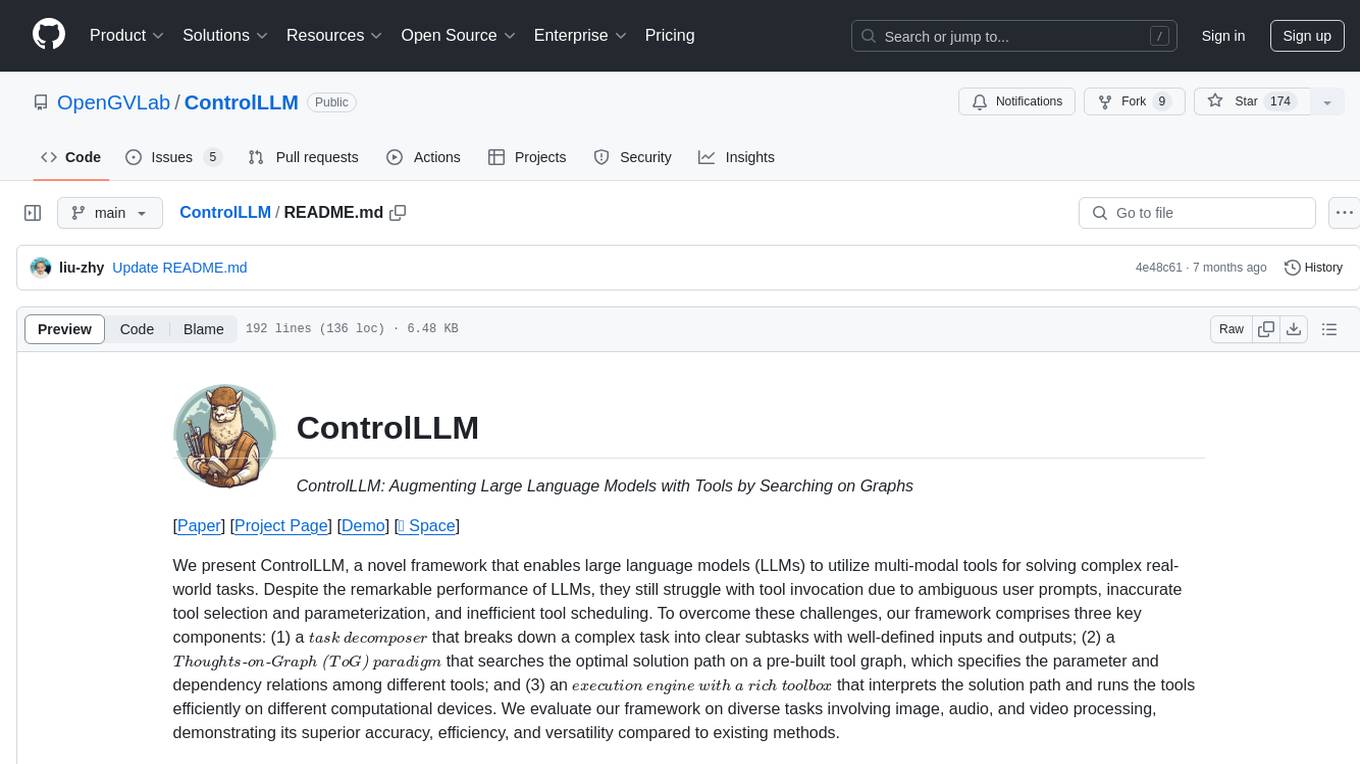
ControlLLM
ControlLLM is a framework that empowers large language models to leverage multi-modal tools for solving complex real-world tasks. It addresses challenges like ambiguous user prompts, inaccurate tool selection, and inefficient tool scheduling by utilizing a task decomposer, a Thoughts-on-Graph paradigm, and an execution engine with a rich toolbox. The framework excels in tasks involving image, audio, and video processing, showcasing superior accuracy, efficiency, and versatility compared to existing methods.
For similar tasks
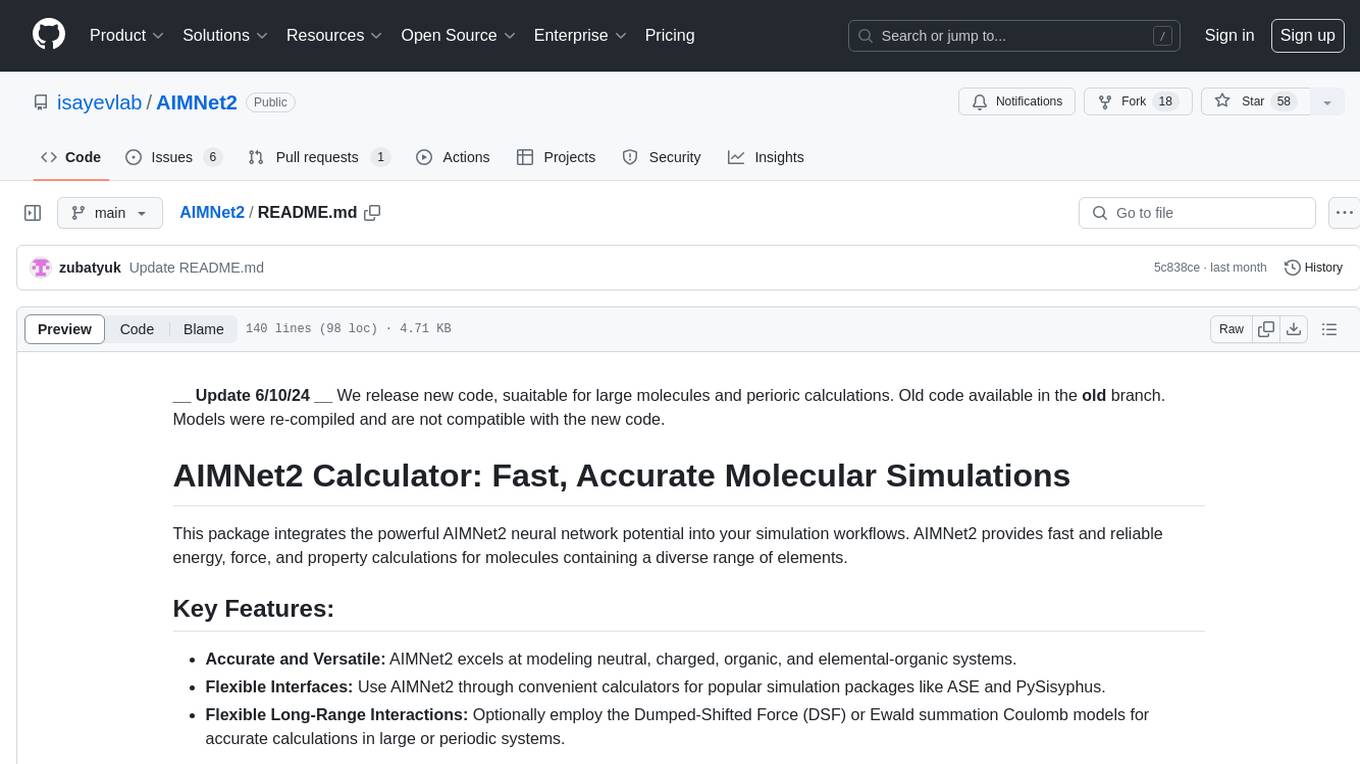
AIMNet2
AIMNet2 Calculator is a package that integrates the AIMNet2 neural network potential into simulation workflows, providing fast and reliable energy, force, and property calculations for molecules with diverse elements. It excels at modeling various systems, offers flexible interfaces for popular simulation packages, and supports long-range interactions using DSF or Ewald summation Coulomb models. The tool is designed for accurate and versatile molecular simulations, suitable for large molecules and periodic calculations.
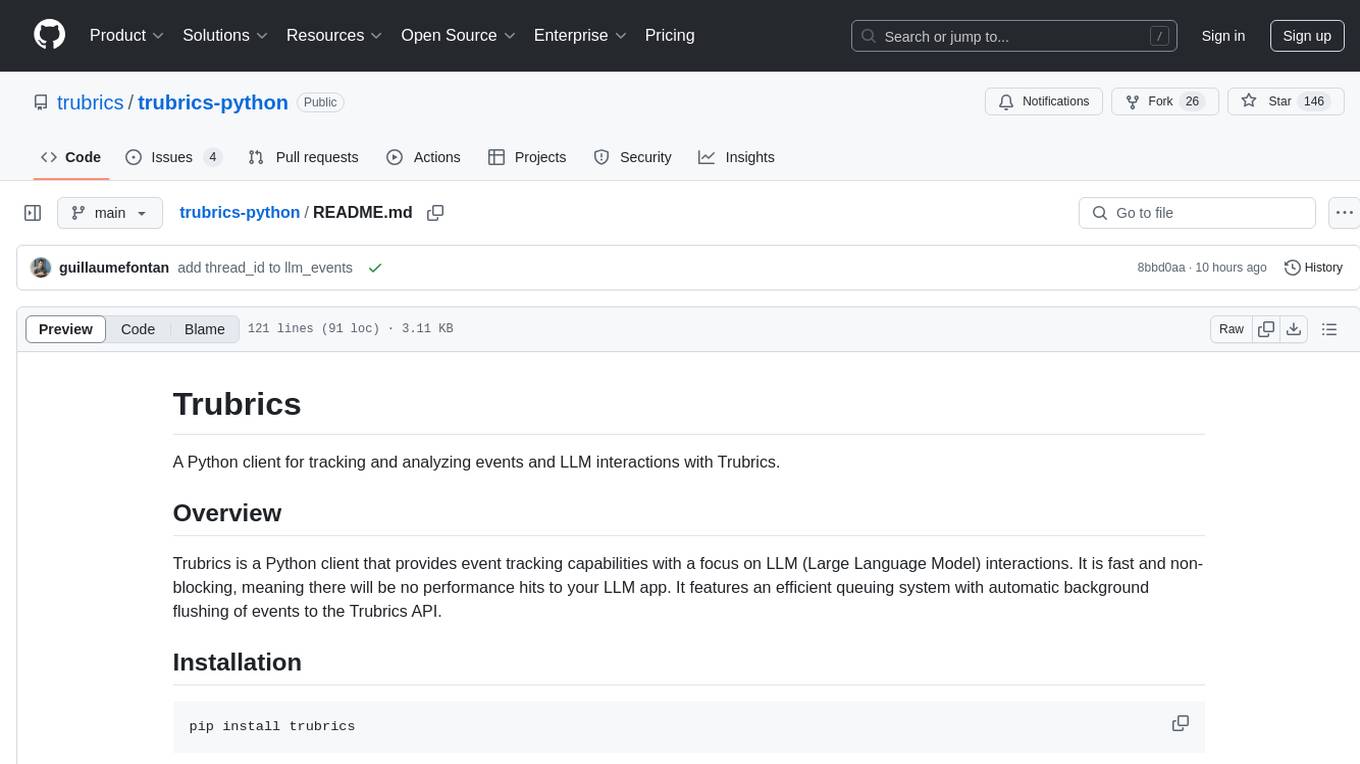
trubrics-python
Trubrics is a Python client for event tracking and analyzing LLM interactions. It offers fast and non-blocking queuing system with automatic flushing to Trubrics API. Users can track events and LLM interactions, adjust logging verbosity, and configure flush intervals and batch sizes. The tool simplifies tracking user interactions and analyzing data for LLM applications.
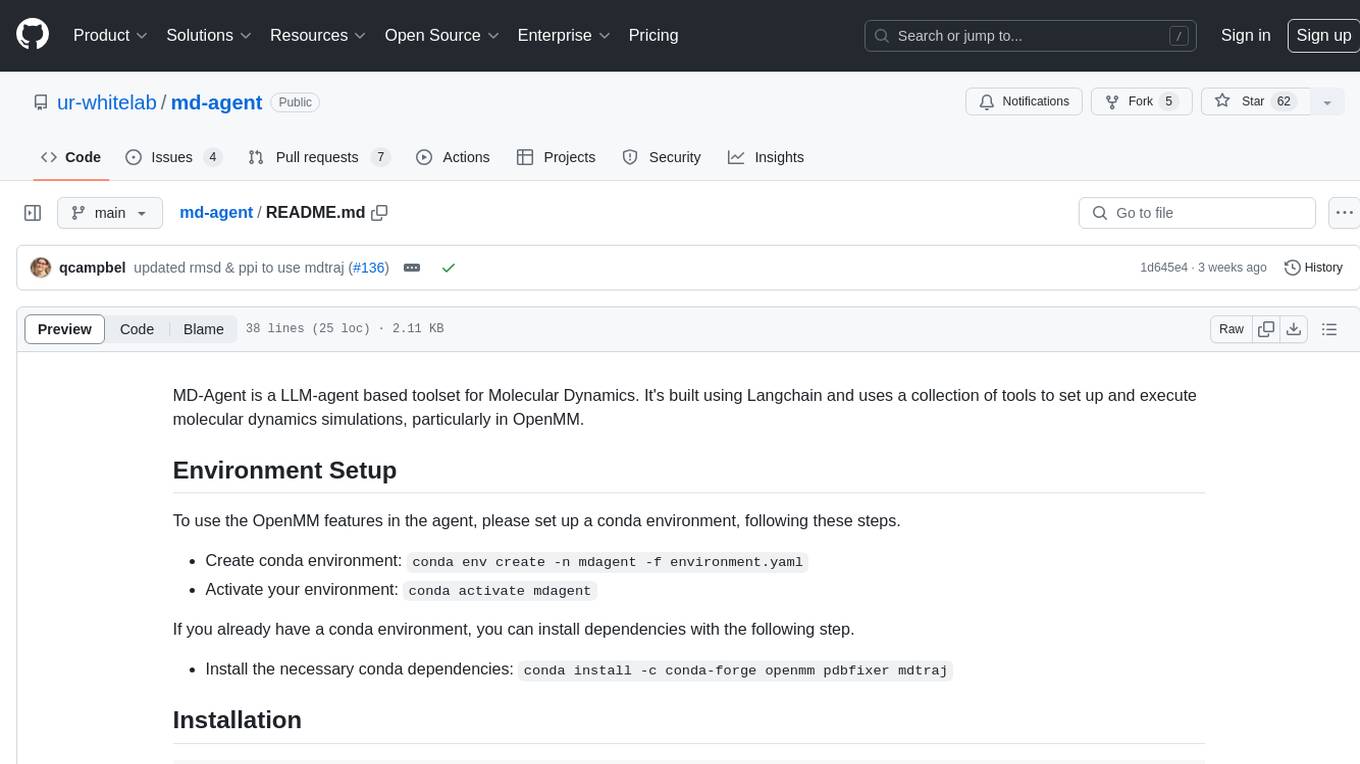
md-agent
MD-Agent is a LLM-agent based toolset for Molecular Dynamics. It uses Langchain and a collection of tools to set up and execute molecular dynamics simulations, particularly in OpenMM. The tool assists in environment setup, installation, and usage by providing detailed steps. It also requires API keys for certain functionalities, such as OpenAI and paper-qa for literature searches. Contributions to the project are welcome, with a detailed Contributor's Guide available for interested individuals.
For similar jobs
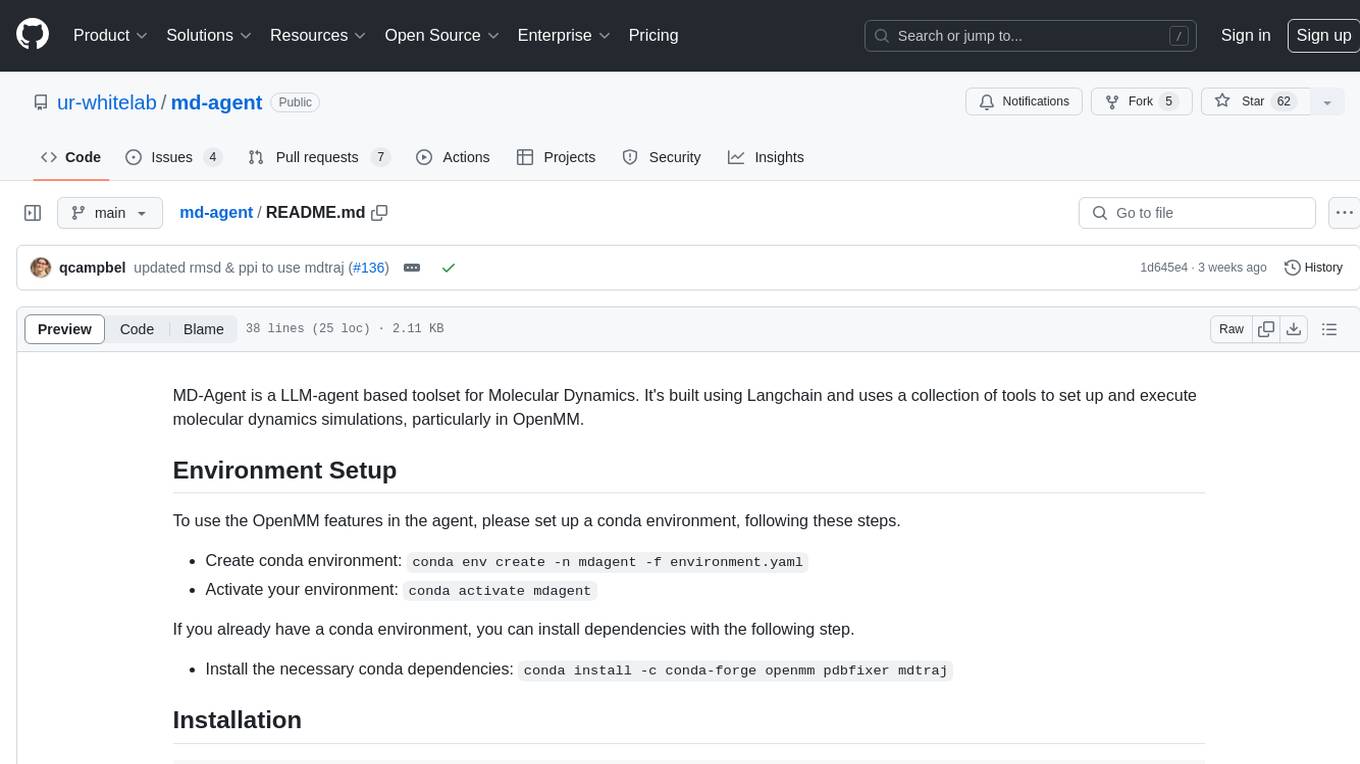
md-agent
MD-Agent is a LLM-agent based toolset for Molecular Dynamics. It uses Langchain and a collection of tools to set up and execute molecular dynamics simulations, particularly in OpenMM. The tool assists in environment setup, installation, and usage by providing detailed steps. It also requires API keys for certain functionalities, such as OpenAI and paper-qa for literature searches. Contributions to the project are welcome, with a detailed Contributor's Guide available for interested individuals.
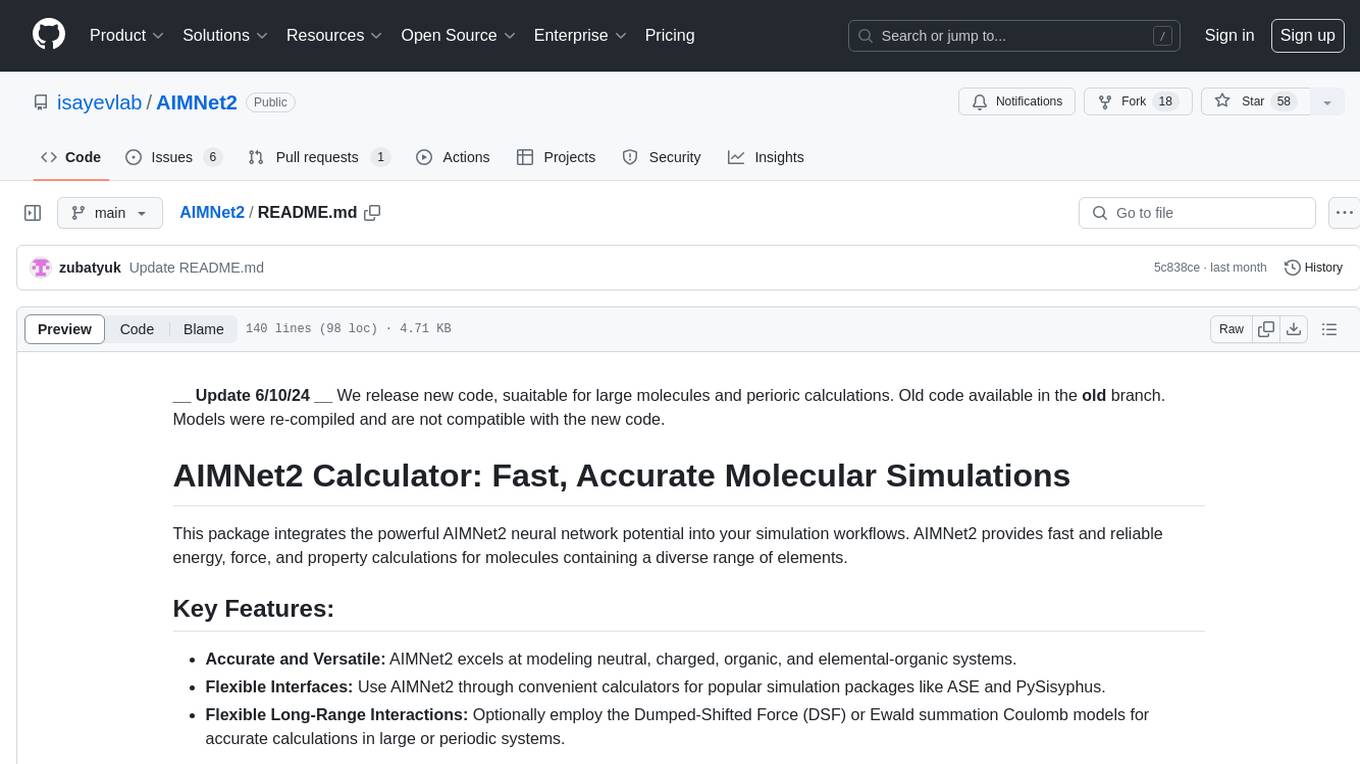
AIMNet2
AIMNet2 Calculator is a package that integrates the AIMNet2 neural network potential into simulation workflows, providing fast and reliable energy, force, and property calculations for molecules with diverse elements. It excels at modeling various systems, offers flexible interfaces for popular simulation packages, and supports long-range interactions using DSF or Ewald summation Coulomb models. The tool is designed for accurate and versatile molecular simulations, suitable for large molecules and periodic calculations.
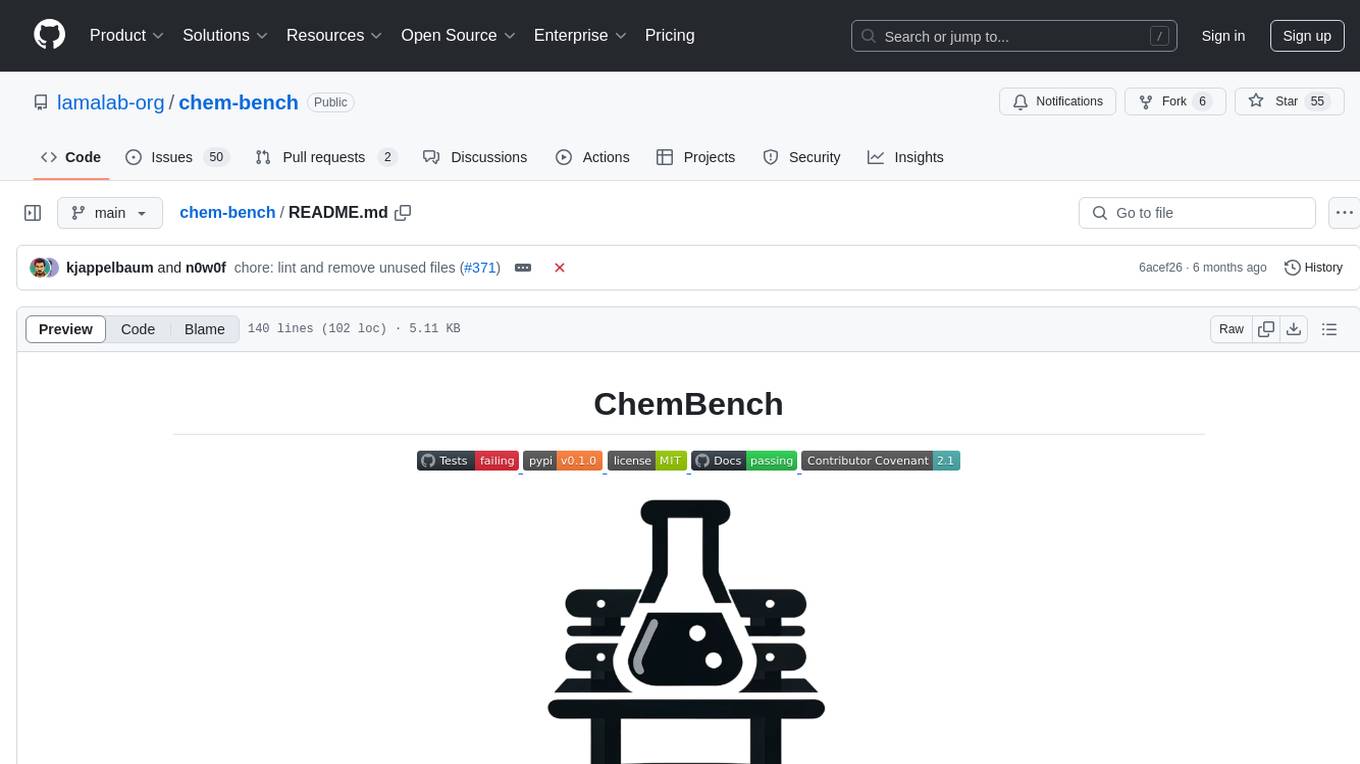
chem-bench
ChemBench is a project aimed at expanding chemistry benchmark tasks in a BIG-bench compatible way, providing a pipeline to benchmark frontier and open models. It allows users to run benchmarking tasks on models with existing presets, offering predefined parameters and processing steps. The library facilitates benchmarking models on the entire suite, addressing challenges such as prompt structure, parsing, and scoring methods. Users can contribute to the project by following the developer notes.
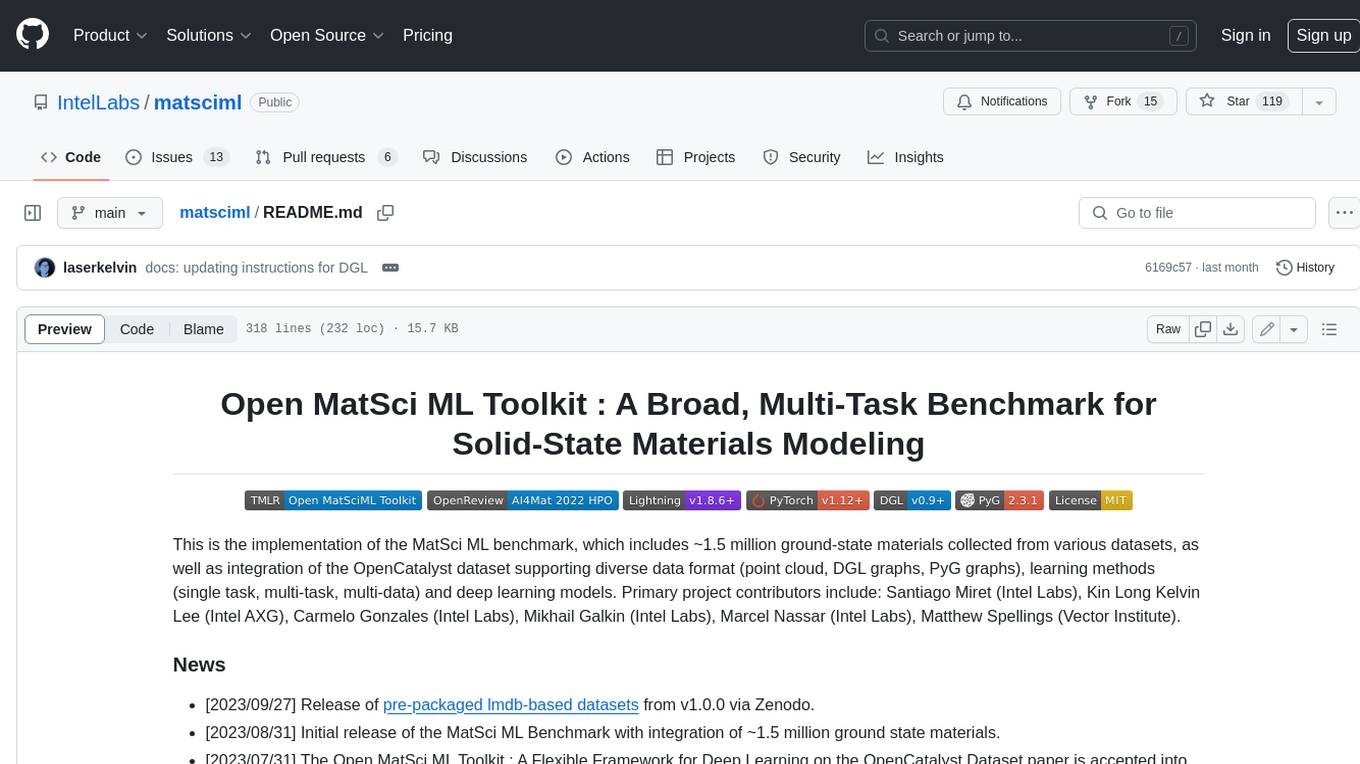
matsciml
The Open MatSci ML Toolkit is a flexible framework for machine learning in materials science. It provides a unified interface to a variety of materials science datasets, as well as a set of tools for data preprocessing, model training, and evaluation. The toolkit is designed to be easy to use for both beginners and experienced researchers, and it can be used to train models for a wide range of tasks, including property prediction, materials discovery, and materials design.
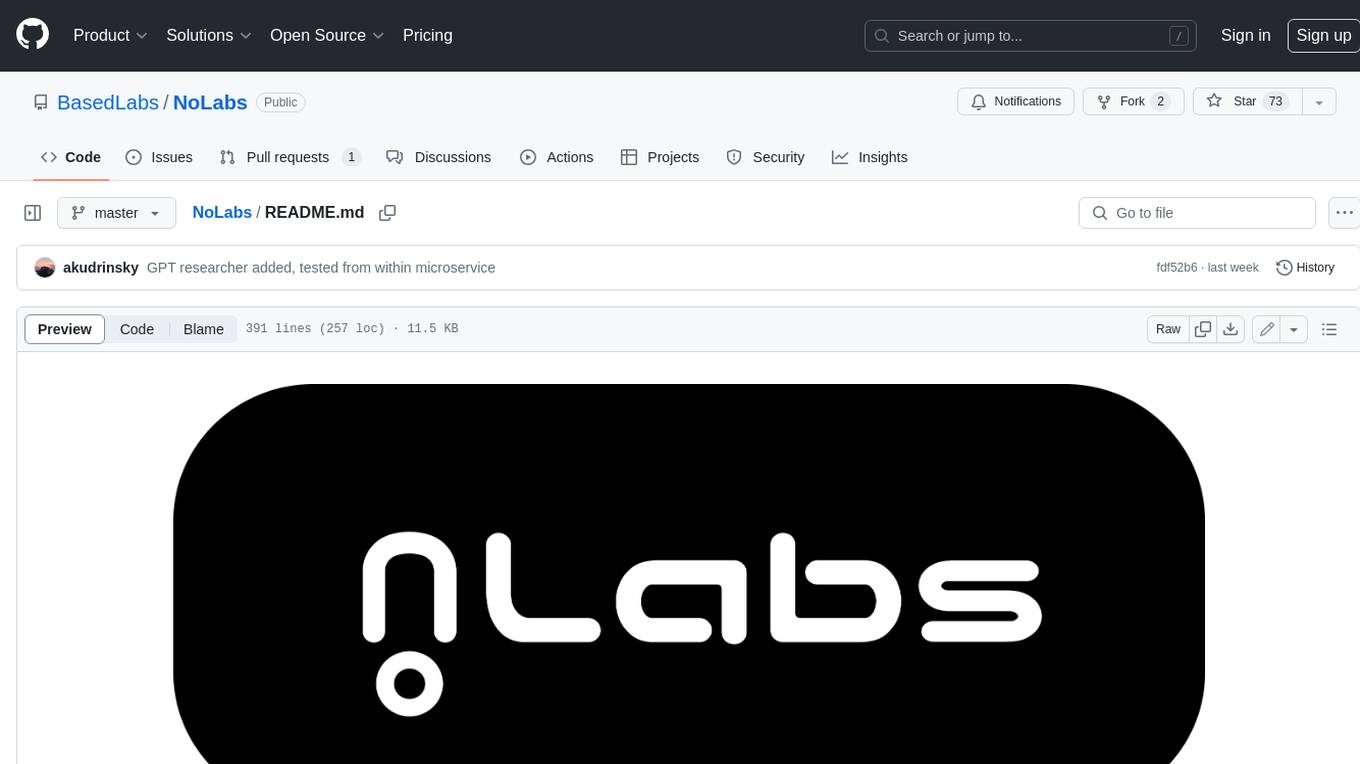
NoLabs
NoLabs is an open-source biolab that provides easy access to state-of-the-art models for bio research. It supports various tasks, including drug discovery, protein analysis, and small molecule design. NoLabs aims to accelerate bio research by making inference models accessible to everyone.
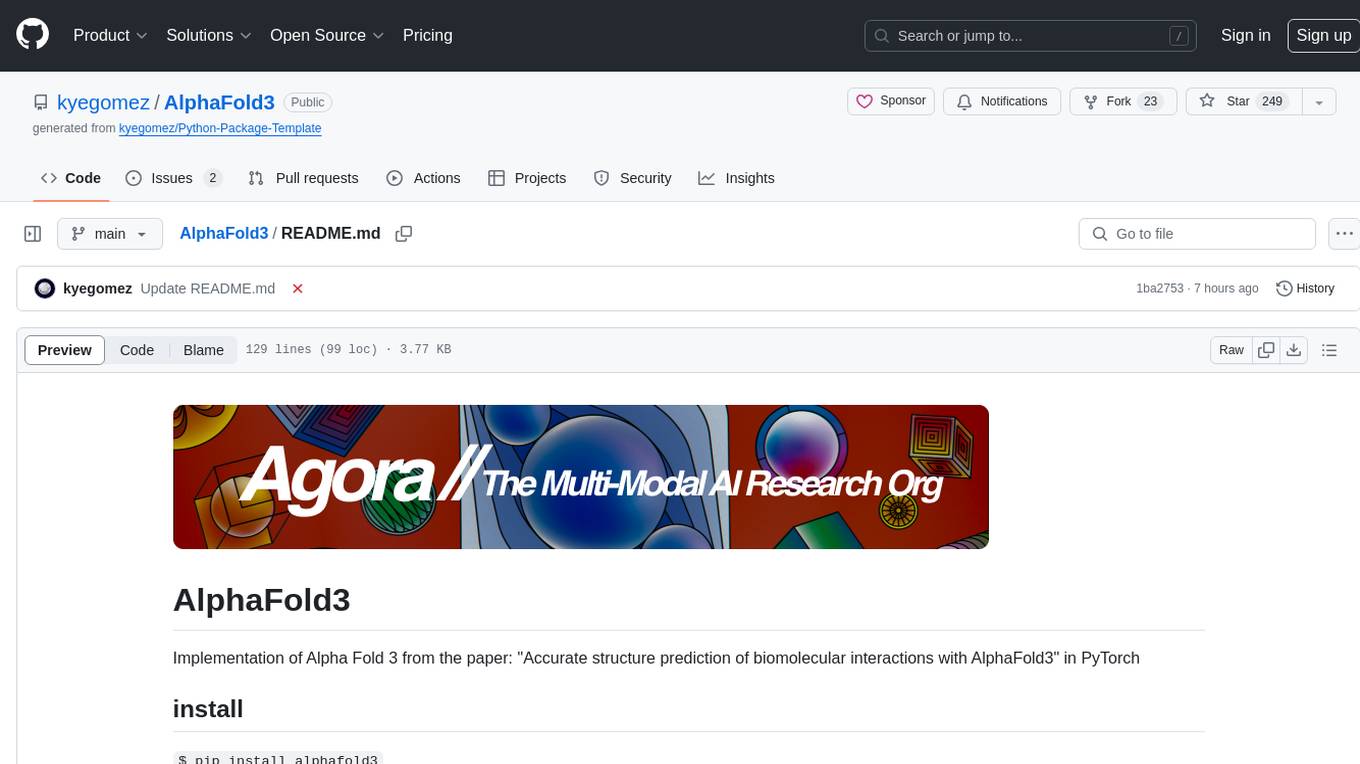
AlphaFold3
AlphaFold3 is an implementation of the Alpha Fold 3 model in PyTorch for accurate structure prediction of biomolecular interactions. It includes modules for genetic diffusion and full model examples for forward pass computations. The tool allows users to generate random pair and single representations, operate on atomic coordinates, and perform structure predictions based on input tensors. The implementation also provides functionalities for training and evaluating the model.
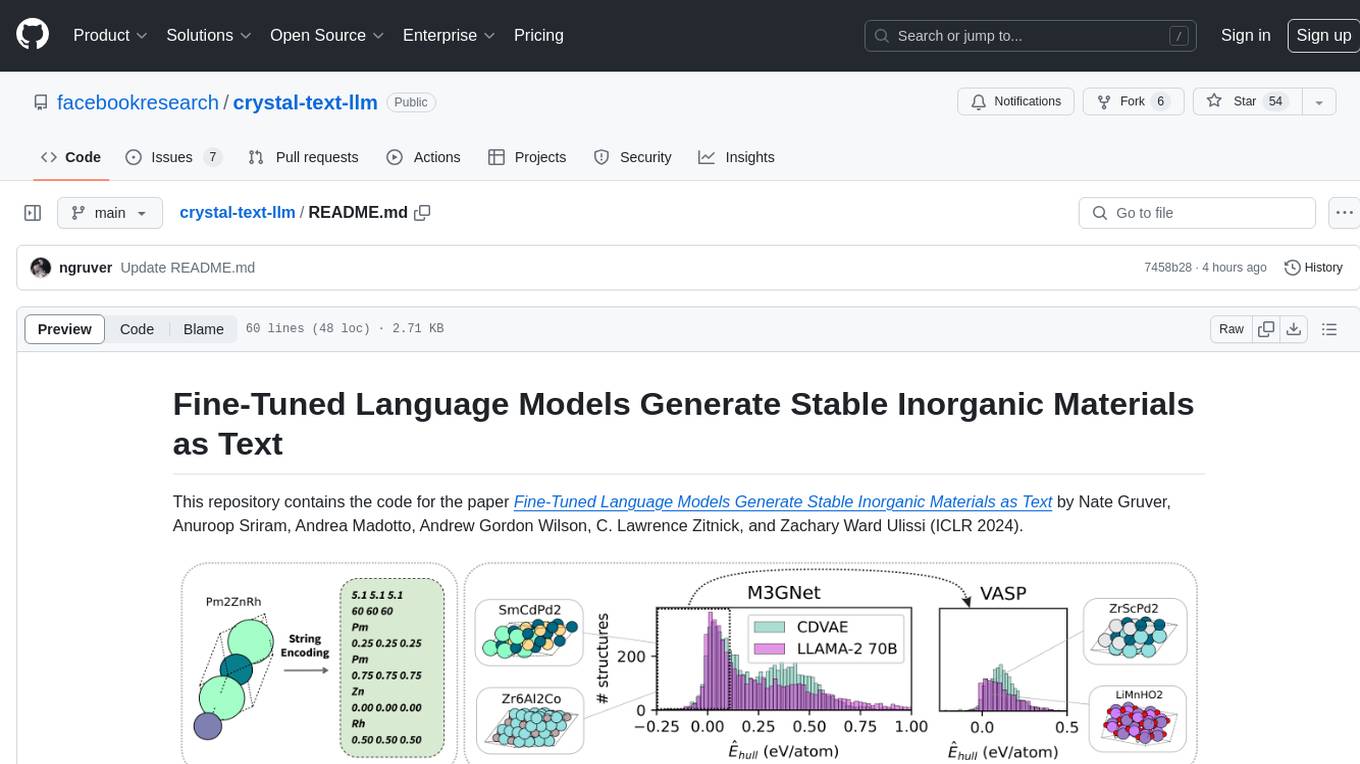
crystal-text-llm
This repository contains the code for the paper Fine-Tuned Language Models Generate Stable Inorganic Materials as Text. It demonstrates how finetuned LLMs can be used to generate stable materials, match or exceed the performance of domain specific models, mutate existing materials, and sample crystal structures conditioned on text descriptions. The method is distinct from CrystaLLM, which trains language models from scratch on CIF-formatted crystals.
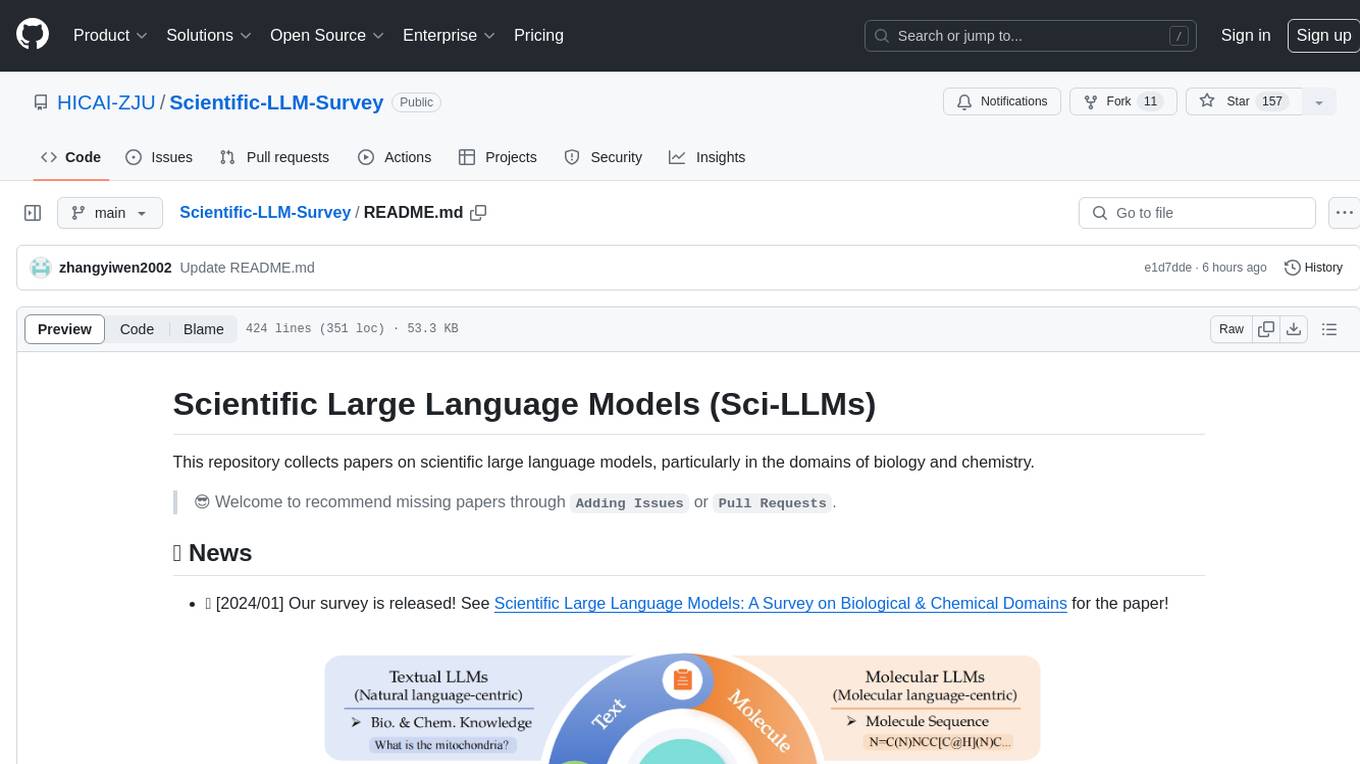
Scientific-LLM-Survey
Scientific Large Language Models (Sci-LLMs) is a repository that collects papers on scientific large language models, focusing on biology and chemistry domains. It includes textual, molecular, protein, and genomic languages, as well as multimodal language. The repository covers various large language models for tasks such as molecule property prediction, interaction prediction, protein sequence representation, protein sequence generation/design, DNA-protein interaction prediction, and RNA prediction. It also provides datasets and benchmarks for evaluating these models. The repository aims to facilitate research and development in the field of scientific language modeling.