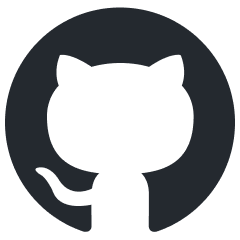
wdoc
Summarize and query from a lot of heterogeneous documents. Any LLM provider, any filetype, scalable, under developpement
Stars: 64
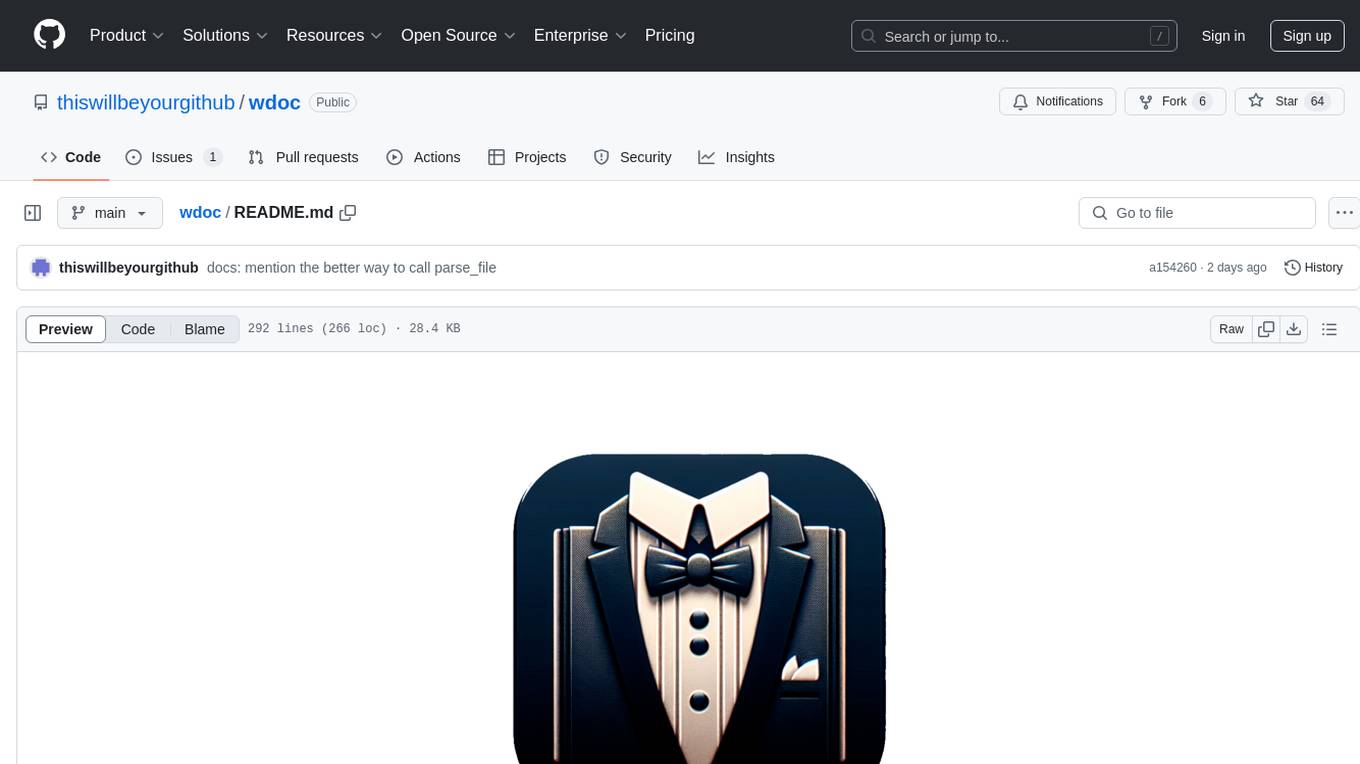
wdoc is a powerful Retrieval-Augmented Generation (RAG) system designed to summarize, search, and query documents across various file types. It aims to handle large volumes of diverse document types, making it ideal for researchers, students, and professionals dealing with extensive information sources. wdoc uses LangChain to process and analyze documents, supporting tens of thousands of documents simultaneously. The system includes features like high recall and specificity, support for various Language Model Models (LLMs), advanced RAG capabilities, advanced document summaries, and support for multiple tasks. It offers markdown-formatted answers and summaries, customizable embeddings, extensive documentation, scriptability, and runtime type checking. wdoc is suitable for power users seeking document querying capabilities and AI-powered document summaries.
README:
I'm wdoc. I solve RAG problems.
- wdoc, imitating Winston "The Wolf" Wolf
wdoc is a powerful RAG (Retrieval-Augmented Generation) system designed to summarize, search, and query documents across various file types. It's particularly useful for handling large volumes of diverse document types, making it ideal for researchers, students, and professionals dealing with extensive information sources. I was frustrated with all other RAG solutions for querying or summarizing, so I made my perfect solution in a single package.
-
Goal and project specifications: wdoc uses LangChain to process and analyze documents. It's capable of querying tens of thousands of documents across various file types at the same time. The project also includes a tailored summary feature to help users efficiently keep up with large amounts of information.
-
Current status: Under active development
- Used daily by the developer for several months: but still in alpha
- May have some instabilities, but issues can usually be resolved quickly
- The main branch is more stable than the dev branch, which offers more features
- Open to feature requests and pull requests
- All feedback, including reports of typos, is highly appreciated
- Please consult the developer before making a PR, as there may be ongoing improvements in the pipeline
-
Key Features:
- Aims to support any filetypes query from all of them at the same time (15+ are already implemented!)
- High recall and specificity: it was made to find A LOT of documents using carefully designed embedding search then carefully aggregate gradually each answer using semantic batch to produce a single answer that mentions the source poiting to the exact portion of the source document.
- Supports virtually any LLM, including local ones, and even with extra layers of security for super secret stuff.
- Use both an expensive and cheap LLM to make recall as high as possible because we can afford fetching a lot of documents per query (via embeddings)
- At last a usable text summary: get the thought process of the author instead of nebulous takeaways.
- Extensible, this is both a tool and a library.
Give it to me I am in a hurry!
link="https://situational-awareness.ai/wp-content/uploads/2024/06/situationalawareness.pdf"
wdoc --path $link --task query --filetype "online_pdf" --query "What does it say about alphago?" --query_retrievers='default_multiquery' --top_k=auto_200_500
- This will:
- parse what's in --path as a link to a pdf to download (otherwise the url could simply be a webpage, but in most cases you can leave it to 'auto' by default as heuristics are in place to detect the most appropriate parser).
- cut the text into chunks and create embeddings for each
- Take the user query, create embeddings for it ('default') AND ask the default LLM to generate alternative queries and embed those
- Use those embeddings to search through all chunks of the text and get the 200 most appropriate documents
- Pass each of those documents to the smaller LLM (default: openai/gpt-4o-mini) to tell us if the document seems appropriate given the user query
- If More than 90% of the 200 documents are appropriate, then we do another search with a higher top_k and repeat until documents start to be irrelevant OR we it 500 documents.
- Then each relevant doc is sent to the strong LLM (by default, openai/gpt-4o) to extract relevant info and give one answer.
- Then all those "intermediate" answers are 'semantic batched' (meaning we create embeddings, do hierarchical clustering, then create small batch containing several intermediate answers) and each batch is combined into a single answer.
- Rinse and repeat steps 7+8 until we have only one answer, that is returned to the user.
link="https://situational-awareness.ai/wp-content/uploads/2024/06/situationalawareness.pdf"
wdoc --path $link --task summarize --filetype "online_pdf"
-
This will:
- Split the text into chunks
- pass each chunk into the strong LLM (by default openai/gpt-4o) for a very low level (=with all details) summary. The format is markdown bullet points for each idea and with logical indentation.
- When creating each new chunk, the LLM has access to the previous chunk for context.
- All summary are then concatenated and returned to the user
-
For extra large documents like books for example, this summary can be recusively fed to wdoc using argument --summary_n_recursion=2 for example.
-
Those two tasks, query and summary, can be combined with --task summarize_then_query which will summarize the document but give you a prompt at the end to ask question in case you want to clarify things.
-
For more, you can jump to the section Walkthrough and examples
- 15+ filetypes: also supports combination to load recursively or define complex heterogenous corpus like a list of files, list of links, using regex, youtube playlists etc. See Supported filestypes. All filetype can be seamlessly combined in the same index, meaning you can query your anki collection at the same time as your work PDFs). It supports removing silence from audio files and youtube videos too!
- 100+ LLMs: OpenAI, Mistral, Claude, Ollama, Openrouter, etc. Thanks to litellm. Personnaly I'm using openrouter's Sonnet 3.5 as strong LLM and openai's gpt-4o-mini as query_eval LLM, with openai embeddings.
-
Local and Private LLM: take some measures to make sure no data leaves your computer and goes to an LLM provider: no API keys are used, all
api_base
are user set, cache are isolated from the rest, outgoing connections are censored by overloading sockets, etc. -
Advanced RAG to query lots of diverse documents:
- The documents are retrieved using embedding
- Then a weak LLM model ("Eve the Evaluator") is used to tell which of those document is not relevant
- Then the strong LLM is used to answer ("Anna the Answerer") the question using each individual remaining documents.
- Then all relevant answers are combined ("Carl the Combiner") into a single short markdown-formatted answer. Before being combined, they are batched by semantic clusters
and semantic order using scipy's hierarchical clustering and leaf ordering, this makes it easier for the LLM to combine the answers in a manner that makes bottom up sense.
Eve the Evaluator
,Anna the Answerer
andCarl the Combiner
are the names given to each LLM in their system prompt, this way you can easily add specific additional instructions to a specific step. There's alsoSam the Summarizer
for summaries andRaphael the Rephraser
to expand your query. - Each document is identified by a unique hash and the answers are sourced, meaning you know from which document comes each information of the answer.
- Supports a special syntax like "QE >>>> QA" were QE is a question used to filter the embeddings and QA is the actual question you want answered.
-
Advanced summary:
- Instead of unusable "high level takeaway" points, compress the reasoning, arguments, though process etc of the author into an easy to skim markdown file.
- The summaries are then checked again n times for correct logical indentation etc.
- The summary can be in the same language as the documents or directly translated.
- Many tasks: See Supported tasks.
- Trust but verify: The answer is sourced: wdoc keeps track of the hash of each document used in the answer, allowing you to verify each assertion.
- Markdown formatted answers and summaries: using rich.
- Sane embeddings: By default use sophisticated embeddings like multi query retrievers but also include SVM, KNN, parent retriever etc. Customizable.
-
Fully documented Lots of docstrings, lots of in code comments, detailed
--help
etc. The full usage can be found in the file USAGE.md or viapython -m wdoc --help
. I work hard to maintain an exhaustive documentation. -
Scriptable / Extensible: You can use wdoc in other python project using
--import_mode
. Take a look at the scripts below. -
Statically typed: Runtime type checking. Opt out with an environment flag:
WDOC_TYPECHECKING="disabled / warn / crash" wdoc
(by default:warn
). Thanks to beartype it shouldn't even slow down the code! - LLM (and embeddings) caching: speed things up, as well as index storing and loading (handy for large collections).
- Good PDF parsing PDF parsers are notoriously unreliable, so 15 (!) different loaders are used, and the best according to a parsing scorer is kept. Including table support via openparse (no GPU needed by default) or via UnstructuredPDFLoader.
- Langfuse support: If you set the appropriate langfuse environment variables they will be used. See this guide or this one to learn more (Note: this is disabled if using private_mode to avoid any leaks).
- Document filtering: based on regex for document content or metadata.
- Fast: Parallel document loading, parsing, embeddings, querying, etc.
- Shell autocompletion using python-fire
- Notification callback: Can be used for example to get summaries on your phone using ntfy.sh.
- Hacker mindset: I'm a friendly dev! Just open an issue if you have a feature request or anything else.
Click to read more
(These don't include improvements, bugfixes, refactoring etc.)
- THIS LIST IS NOT UP TO DATE AND THERE ARE MANY MORE THINGS PLANNED
- Start using unit tests
- Accept input from stdin, to for example query directly from a manpage
- Much faster startup time
- Much improved retriever:
- Web search retriever, online information lookup via jina.ai reader and search.
- LLM powered synonym expansion for embeddings search.
- A way to specify at indexing time how trusting you are of a given set of document.
- A way to open the documents automatically, based on the platform used. For ex if okular is installed, open pdfs directly at the appropriate page.
- Improve the scriptability of wdoc. Add examples for how you use it with Logseq.
- Include a server example, that mimics the OpenAI's API to make your RAG directly accessible to other apps.
- Add a gradio GUI.
- Include the possible whisper/deepgram extra expenses when counting costs.
- Add support for user defined loaders.
- Automatically caption document images using an LLM, especially nice for anki cards.
-
auto: default, guess the filetype for you
-
url: try many ways to load a webpage, with heuristics to find the better parsed one
-
youtube: text is then either from the yt subtitles / translation or even better: using whisper / deepgram
-
pdf: 15 default loaders are implemented, heuristics are used to keep the best one and stop early. Table support via openparse or UnstructuredPDFLoader. Easy to add more.
-
online_pdf: via URL then treated as a pdf (see above)
-
anki: any subset of an anki collection db.
alt
andtitle
of images can be shown to the LLM, meaning that if you used the ankiOCR addon this information will help contextualize the note for the LLM. -
string: the cli prompts you for a text so you can easily paste something, handy for paywalled articles!
-
txt: .txt, markdown, etc
-
text: send a text content directly as path
-
local_html: useful for website dumps
-
logseq_markdown: thanks to my other project: LogseqMarkdownParser you can use your Logseq graph
-
local_audio: supports many file formats, can use either OpenAI's whisper or deepgram. Supports automatically removing silence etc.
-
local_video: extract the audio then treat it as local_audio
-
online_media: use youtube_dl to try to download videos/audio, if fails try to intercept good url candidates using playwright to load the page. Then processed as local_audio (but works with video too).
-
epub: barely tested because epub is in general a poorly defined format
-
powerpoint: .ppt, .pptx, .odp, ...
-
word: .doc, .docx, .odt, ...
-
json_dict: a text file containing a single json dict.
-
Recursive types
- youtube playlists: get the link for each video then process as youtube
- recursive_paths: turns a path, a regex pattern and a filetype into all the files found recurisvely, and treated a the specified filetype (for example many PDFs or lots of HTML files etc).
- link_file: turn a text file where each line contains a url into appropriate loader arguments. Supports any link, so for example webpage, link to pdfs and youtube links can be in the same file. Handy for summarizing lots of things!
-
json_entries: turns a path to a file where each line is a json dict: that contains arguments to use when loading. Example: load several other recursive types. An example can be found in
docs/json_entries_example.json
. -
toml_entries: read a .toml file. An example can be found in
docs/toml_entries_example.toml
.
- query give documents and asks questions about it.
- search only returns the documents and their metadata. For anki it can be used to directly open cards in the browser.
-
summarize give documents and read a summary. The summary prompt can be found in
utils/prompts.py
. - summarize_then_query summarize the document then allow you to query directly about it.
Detailed example
- Say you want to ask a question about one pdf, that's simple:
wdoc --task "query" --path "my_file.pdf" --filetype="pdf" --modelname='openai/gpt-4o'
. Note that you could have just let--filetype="auto"
and it would have worked the same.
- Note: By default wdoc tries to parse args as kwargs so
wdoc query mydocument What's the age of the captain?
is parsed aswdoc --task=query --path=mydocument --query "What's the age of the captain?"
. Likewise for summaries.
- You have several pdf? Say you want to ask a question about any pdf contained in a folder, that's not much more complicated :
wdoc --task "query" --path "my/other_dir" --pattern "**/*pdf" --filetype "recursive_paths" --recursed_filetype "pdf" --query "My question about those documents"
. So basically you give as path the path to the dir, as pattern the globbing pattern used to find the files relative to the path, set as filetype "recursive_paths" so that wdoc knows what arguments to expect, and specify as recursed_filetype "pdf" so that wdoc knows that each found file must be treated as a pdf. You can use the same idea to glob any kind of file supported by wdoc like markdown etc. You can even use "auto"! Note that you can either directly ask your question with--query "my question"
, or wait for an interactive prompt to pop up, or just pass the question as *args like sowdoc [your kwargs] here is my question
. - You want more? You can write a
.json
file where each line (#comments
and empty lines are ignored) will be parsed as a list of argument. For example one line could be :{"path": "my/other_dir", "pattern": "**/*pdf", "filetype": "recursive_paths", "recursed_filetype": "pdf"}
. This way you can use a single json file to specify easily any number of sources..toml
files are also supported. - You can specify a "source_tag" metadata to help distinguish between documents you imported. It is EXTREMELY recommended to include a source_tag to any document you want to save: especially if using recursive filetypes. This is because after loading all documents wdoc use the source_tag to see if it should continue or crash. If you want to load 10_000 pdf in one go as I do, then it makes sense to continue if some failed to crash but not if a whole source_tag is missing.
- Now say you do this with many many documents, as I do, you of course can't wait for the indexing to finish every time you have a question (even though the embeddings are cached). You should then add
--save_embeds_as=your/saving/path
to save all this index in a file. Then simply do--load_embeds_from=your/saving/path
to quickly ask queries about it! - To know more about each argument supported by each filetype,
wdoc --help
- There is a specific recursive filetype I should mention:
--filetype="link_file"
. Basically the file designated by--path
should contain in each line (#comments
and empty lines are ignored) one url, that will be parsed by wdoc. I made this so that I can quickly use the "share" button on android from my browser to a text file (so it just appends the url to the file), this file is synced via syncthing to my browser and wdoc automatically summarize them and add them to my Logseq. Note that the url is parsed in each line, so formatting is ignored, for example it works even in markdown bullet point list. - If you want to make sure your data remains private here's an example with ollama:
wdoc --private --llms_api_bases='{"model": "http://localhost:11434", "query_eval_model": "http://localhost:11434"}' --modelname="ollama_chat/gemma:2b" --query_eval_modelname="ollama_chat/gemma:2b" --embed_model="BAAI/bge-m3" my_task
- Now say you just want to summarize Tim Urban's TED talk on procrastination:
wdoc summary --path 'https://www.youtube.com/watch?v=arj7oStGLkU' --youtube_language="english" --disable_md_printing
:
Click to see the output
- The speaker, Tim Urban, was a government major in college who had to write many papers
- He claims his typical work pattern for papers was:
- Planning to spread work evenly
- Actually procrastinating until the last minute
- For his 90-page senior thesis:
- Planned to work steadily over a year
- Actually ended up writing 90 pages in 72 hours before the deadline
- Pulled two all-nighters
- Resulted in a 'very, very bad thesis'
- Urban is now a writer-blogger for 'Wait But Why'
- He wrote about procrastination to explain it to non-procrastinators
- Humorously claims to have done brain scans comparing procrastinator and non-procrastinator brains
- Introduces concept of 'Instant Gratification Monkey' in procrastinator's brain
- Monkey takes control from the Rational Decision-Maker
- Leads to unproductive activities like reading Wikipedia, checking fridge, YouTube spirals
- Monkey characteristics:
- Lives in the present moment
- No memory of past or knowledge of future
- Only cares about 'easy and fun'
- Rational Decision-Maker:
- Allows long-term planning and big picture thinking
- Wants to do what makes sense in the moment
- 'Dark Playground': where procrastinators spend time on leisure activities when they shouldn't
- Filled with guilt, dread, anxiety, self-hatred
- 'Panic Monster': procrastinator's guardian angel
- Wakes up when deadlines are close or there's danger of embarrassment
- Only thing the Monkey fears
- Urban relates his own experience procrastinating on preparing this TED talk
- Claims thousands of people emailed him about having the same procrastination problem
- Two types of procrastination:
- Short-term with deadlines (contained by Panic Monster)
- Long-term without deadlines (more damaging)
- Affects self-starter careers, personal life, health, relationships
- Can lead to long-term unhappiness and regrets
- Urban believes all people are procrastinators to some degree
- Presents 'Life Calendar': visual representation of weeks in a 90-year life
- Encourages audience to:
- Think about what they're procrastinating on
- Stay aware of the Instant Gratification Monkey
- Start addressing procrastination soon
- Humorously suggests not starting today, but 'sometime soon'
Tokens used for https://www.youtube.com/watch?v=arj7oStGLkU: '4365' ($0.00060)
Total cost of those summaries: '4365' ($0.00060, estimate was $0.00028)
Total time saved by those summaries: 8.4 minutes
Done summarizing.
Tested on python 3.11.7, which is therefore recommended
- To install:
- Using pip:
pip install -U wdoc
- Or to get a specific git branch:
-
dev
branch:pip install git+https://github.com/thiswillbeyourgithub/wdoc@dev
-
main
branch:pip install git+https://github.com/thiswillbeyourgithub/wdoc@main
-
- You can also use pipx or uvx. But as I'm not experiences with them I don't know if that can cause issues with for example caching etc. Do tell me if you tested it!
- Using pipx:
pipx run wdoc --help
- Using uvx:
uvx wdoc --help
- Using pipx:
- In any case, it is recommended to try to install pdftotext with
pip install -U wdoc[pdftotext]
as well as add fasttext support withpip install -U wdoc[fasttext]
.
- Using pip:
- Add the API key for the backend you want as an environment variable: for example
export OPENAI_API_KEY="***my_key***"
- Launch is as easy as using
wdoc --task=query --path=MYDOC [ARGS]
andwdoc --task=summary --path=MYDOC [ARGS]
(you can usewdoc
instead ofwdoc
)- If for some reason this fails, maybe try with
python -m wdoc
. And if everything fails, clone this repo and try again aftercd
inside it. - To get shell autocompletion: if you're using zsh:
eval $(cat shell_completions/wdoc_completion.zsh)
. Also provided forbash
andfish
. You can generate your own withwdoc -- --completion MYSHELL > my_completion_file"
. - Don't forget that if you're using a lot of documents (notably via recursive filetypes) it can take a lot of time (depending on parallel processing too, but you then might run into memory errors).
- If for some reason this fails, maybe try with
- To ask questions about a local document:
wdoc query --path="PATH/TO/YOUR/FILE" --filetype="auto"
- If you want to reduce the startup time by directly loading the embeddings from a previous run (although the embeddings are always cached anyway): add
--saveas="some/path"
to the previous command to save the generated embeddings to a file and replace with--loadfrom "some/path"
on every subsequent call.
- If you want to reduce the startup time by directly loading the embeddings from a previous run (although the embeddings are always cached anyway): add
- For more: read the documentation at
wdoc --help
- More to come in the scripts folder
- Ntfy Summarizer: automatically summarize a document from your android phone using ntfy.sh
- TheFiche: create summaries for specific notions directly as a logseq page.
- FilteredDeckCreator: directly create an anki filtered deck from the cards found by wdoc.
FAQ
-
Who is this for?
- wdoc is for power users who want document querying on steroid, and in depth AI powered document summaries.
-
What's RAG?
- A RAG system (retrieval augmented generation) is basically an LLM powered search through a text corpus.
-
Why make another RAG system? Can't you use any of the others?
-
Why is wdoc better than most RAG system to ask questions on documents?
- It uses both a strong and query_eval LLM. After finding the appropriate documents using embeddings, the query_eval LLM is used to filter through the documents that don't seem to be about the question, then the strong LLM answers the question based on each remaining documents, then combines them all in a neat markdown. Also wdoc is very customizable.
-
Why can wdoc also produce summaries?
- I have little free time so I needed a tailor made summary feature to keep up with the news. But most summary systems are rubbish and just try to give you the high level takeaway points, and don't handle properly text chunking. So I made my own tailor made summarizer. The summary prompts can be found in
utils/prompts.py
and focus on extracting the arguments/reasonning/though process/arguments of the author then use markdown indented bullet points to make it easy to read. It's really good! The prompts dataclass is not frozen so you can provide your own prompt if you want.
- I have little free time so I needed a tailor made summary feature to keep up with the news. But most summary systems are rubbish and just try to give you the high level takeaway points, and don't handle properly text chunking. So I made my own tailor made summarizer. The summary prompts can be found in
-
What other tasks are supported by wdoc?
- See Supported tasks.
-
Which LLM providers are supported by wdoc?
- wdoc supports virtually any LLM provider thanks to litellm. It even supports local LLM and local embeddings (see Walkthrough and examples section).
-
What do you use wdoc for?
- I follow heterogeneous sources to keep up with the news: youtube, website, etc. So thanks to wdoc I can automatically create awesome markdown summaries that end up straight into my Logseq database as a bunch of
TODO
blocks. - I use it to ask technical questions to my vast heterogeneous corpus of medical knowledge.
- I use it to query my personal documents using the
--private
argument. - I sometimes use it to summarize a documents then go straight to asking questions about it, all in the same command.
- I use it to ask questions about entire youtube playlists.
- Other use case are the reason I made the scripts made with wdoc section
- I follow heterogeneous sources to keep up with the news: youtube, website, etc. So thanks to wdoc I can automatically create awesome markdown summaries that end up straight into my Logseq database as a bunch of
-
What's up with the name?
- One of my favorite character (and somewhat of a rolemodel is Winston Wolf and after much hesitation I decided
WolfDoc
would be too confusing andWinstonDoc
sounds like something micro$oft would do. Alsowd
andwdoc
were free, whereasdoctools
was already taken. The initial name of the project wasDocToolsLLM
, a play on words between 'doctor' and 'tool'.
- One of my favorite character (and somewhat of a rolemodel is Winston Wolf and after much hesitation I decided
-
How can I improve the prompt for a specific task without coding?
- Each prompt of the
query
task are roleplaying as employees working for WDOC-CORP©, either asEve the Evaluator
(the LLM that filters out relevant documents),Anna the Answerer
(the LLM that answers the question from a filtered document) orCarl the Combiner
(the LLM that combines answers from Answerer as one). There's alsoSam the Summarizer
for summaries andRaphael the Rephraser
to expand your query. They are all receiving orders from you if you talk to them in a prompt.
- Each prompt of the
-
How can I use wdoc's parser for my own documents?
- If you are in the shell cli you can easily use
wdoc parse my_file.pdf
(this actually replaces the call to call insteadwdoc_parse_file my_file.pdf
). add--only_text
to only get the text and no metadata. If you're having problem with argument parsing you can try adding the--pipe
argument. - If you want the document using python:
from wdoc import wdoc list_of_docs = Wdoc.parse_file(path=my_path)
- If you are in the shell cli you can easily use
-
What should I do if my PDF are encrypted?
- If you're on linux you can try running
qpdf --decrypt input.pdf output.pdf
- I made a quick and dirty batch script for in this repo
- If you're on linux you can try running
-
How can I add my own pdf parser?
- Write a python class and add it there:
wdoc.utils.loaders.pdf_loaders['parser_name']=parser_object
then call wdoc with--pdf_parsers=parser_name
.- The class has to take a
path
argument in__init__
, have aload
method taking no argument but returning aList[Document]
. Take a look at theOpenparseDocumentParser
class for an example.
- The class has to take a
- Write a python class and add it there:
-
What should I do if I keep hitting rate limits?
- The simplest way is to add the
debug
argument. It will disable multithreading, multiprocessing and LLM concurrency. A less harsh alternative is to set the environment variableWDOC_LLM_MAX_CONCURRENCY
to a lower value.
- The simplest way is to add the
For Tasks:
Click tags to check more tools for each tasksFor Jobs:
Alternative AI tools for wdoc
Similar Open Source Tools
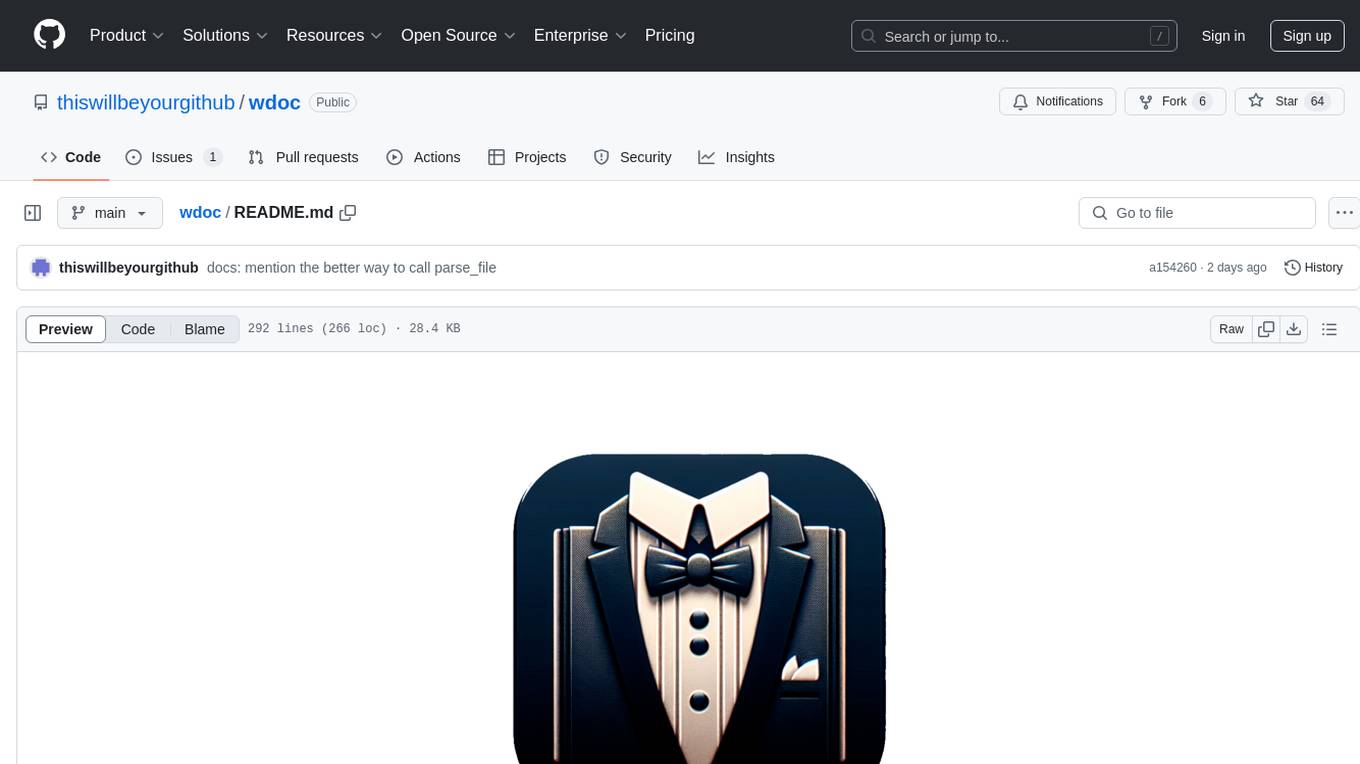
wdoc
wdoc is a powerful Retrieval-Augmented Generation (RAG) system designed to summarize, search, and query documents across various file types. It aims to handle large volumes of diverse document types, making it ideal for researchers, students, and professionals dealing with extensive information sources. wdoc uses LangChain to process and analyze documents, supporting tens of thousands of documents simultaneously. The system includes features like high recall and specificity, support for various Language Model Models (LLMs), advanced RAG capabilities, advanced document summaries, and support for multiple tasks. It offers markdown-formatted answers and summaries, customizable embeddings, extensive documentation, scriptability, and runtime type checking. wdoc is suitable for power users seeking document querying capabilities and AI-powered document summaries.
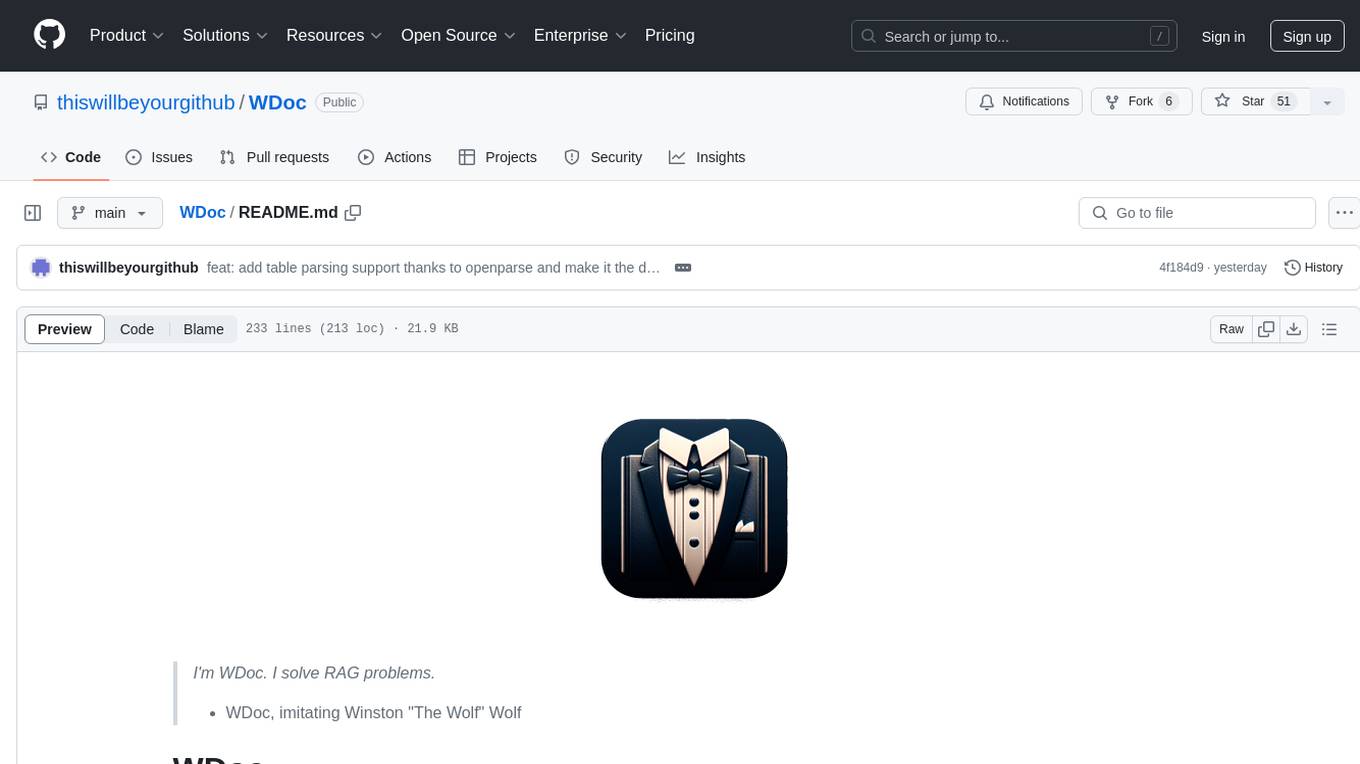
WDoc
WDoc is a powerful Retrieval-Augmented Generation (RAG) system designed to summarize, search, and query documents across various file types. It supports querying tens of thousands of documents simultaneously, offers tailored summaries to efficiently manage large amounts of information, and includes features like supporting multiple file types, various LLMs, local and private LLMs, advanced RAG capabilities, advanced summaries, trust verification, markdown formatted answers, sophisticated embeddings, extensive documentation, scriptability, type checking, lazy imports, caching, fast processing, shell autocompletion, notification callbacks, and more. WDoc is ideal for researchers, students, and professionals dealing with extensive information sources.
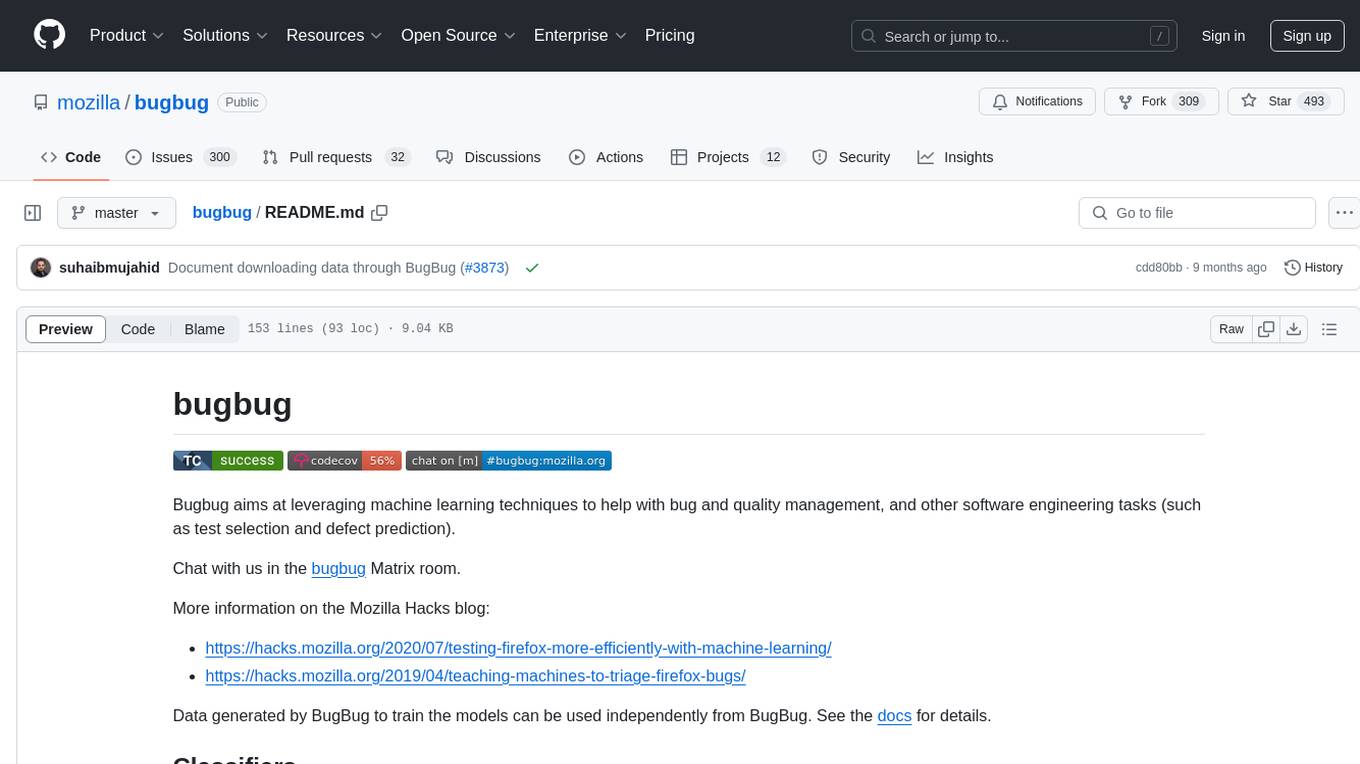
bugbug
Bugbug is a tool developed by Mozilla that leverages machine learning techniques to assist with bug and quality management, as well as other software engineering tasks like test selection and defect prediction. It provides various classifiers to suggest assignees, detect patches likely to be backed-out, classify bugs, assign product/components, distinguish between bugs and feature requests, detect bugs needing documentation, identify invalid issues, verify bugs needing QA, detect regressions, select relevant tests, track bugs, and more. Bugbug can be trained and tested using Python scripts, and it offers the ability to run model training tasks on Taskcluster. The project structure includes modules for data mining, bug/commit feature extraction, model implementations, NLP utilities, label handling, bug history playback, and GitHub issue retrieval.
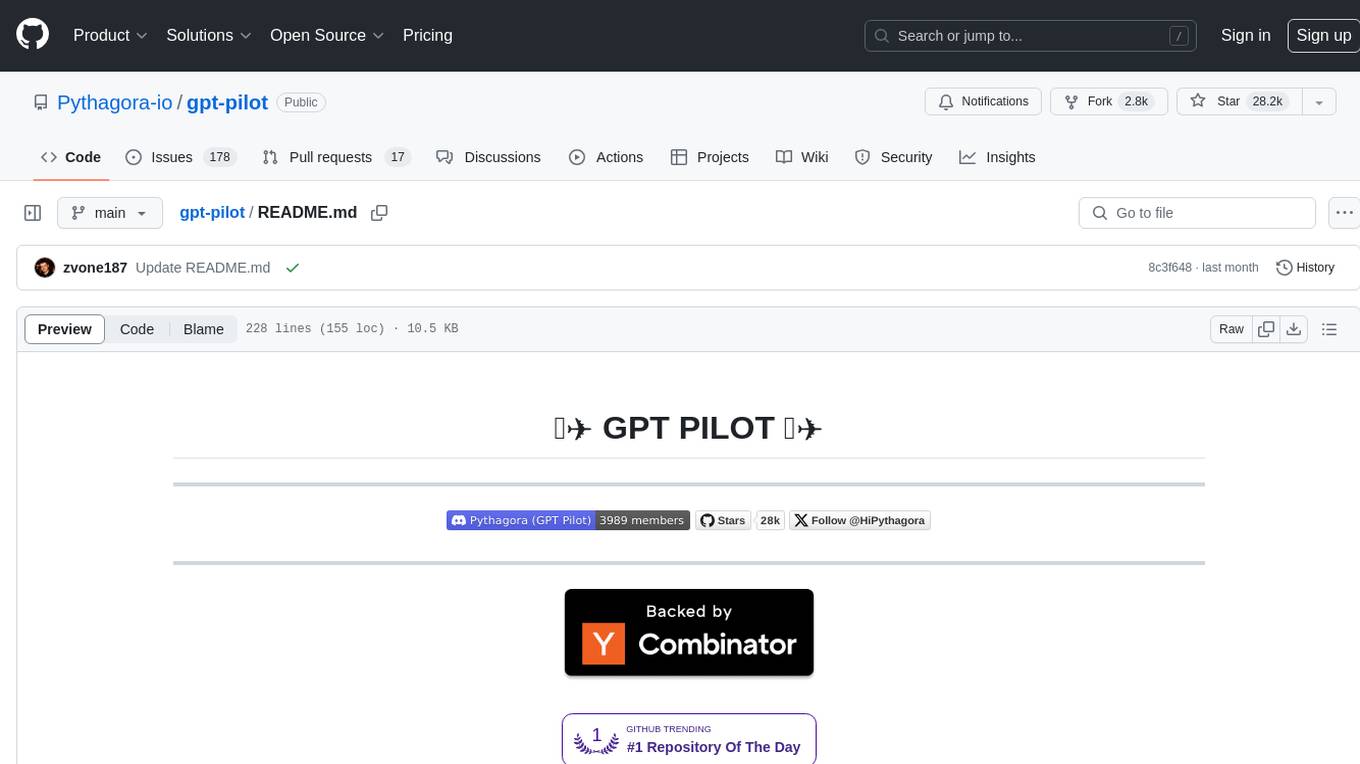
gpt-pilot
GPT Pilot is a core technology for the Pythagora VS Code extension, aiming to provide the first real AI developer companion. It goes beyond autocomplete, helping with writing full features, debugging, issue discussions, and reviews. The tool utilizes LLMs to generate production-ready apps, with developers overseeing the implementation. GPT Pilot works step by step like a developer, debugging issues as they arise. It can work at any scale, filtering out code to show only relevant parts to the AI during tasks. Contributions are welcome, with debugging and telemetry being key areas of focus for improvement.
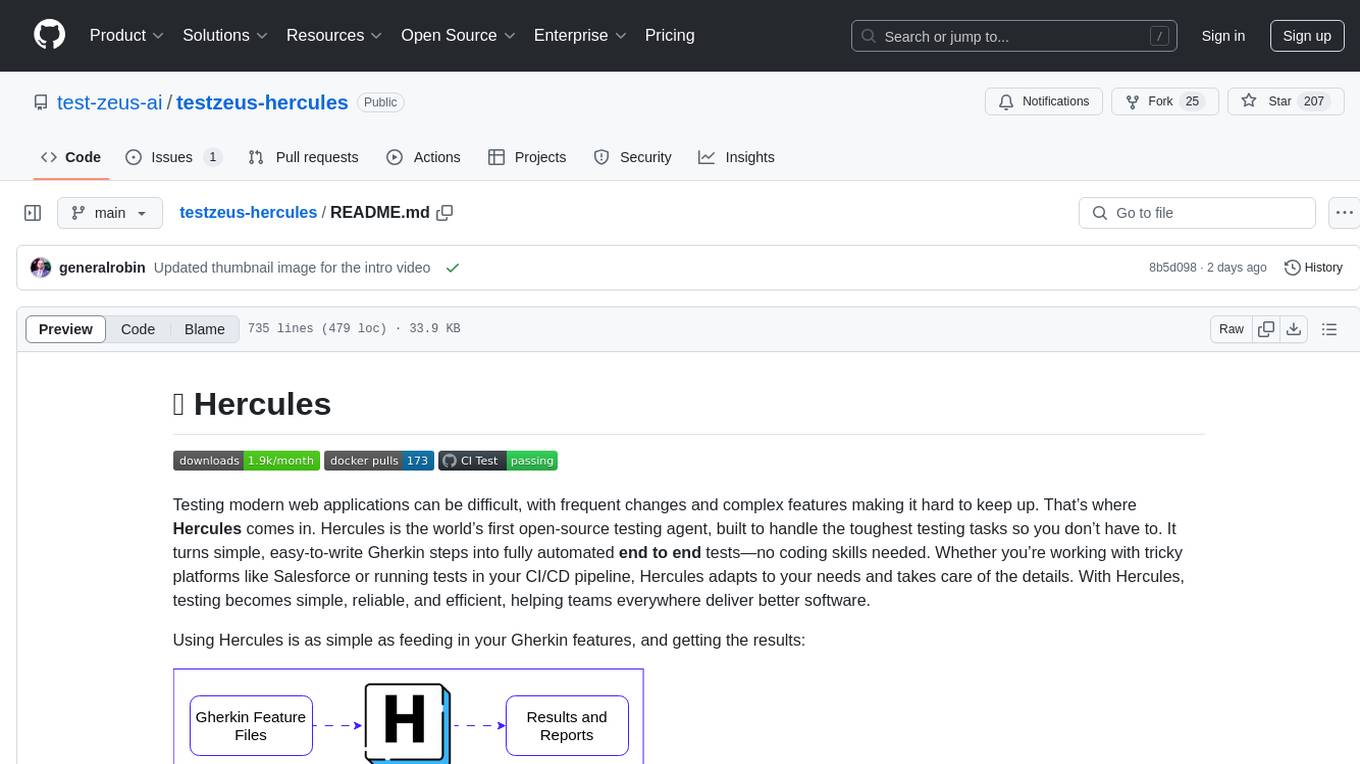
testzeus-hercules
Hercules is the world’s first open-source testing agent designed to handle the toughest testing tasks for modern web applications. It turns simple Gherkin steps into fully automated end-to-end tests, making testing simple, reliable, and efficient. Hercules adapts to various platforms like Salesforce and is suitable for CI/CD pipelines. It aims to democratize and disrupt test automation, making top-tier testing accessible to everyone. The tool is transparent, reliable, and community-driven, empowering teams to deliver better software. Hercules offers multiple ways to get started, including using PyPI package, Docker, or building and running from source code. It supports various AI models, provides detailed installation and usage instructions, and integrates with Nuclei for security testing and WCAG for accessibility testing. The tool is production-ready, open core, and open source, with plans for enhanced LLM support, advanced tooling, improved DOM distillation, community contributions, extensive documentation, and a bounty program.
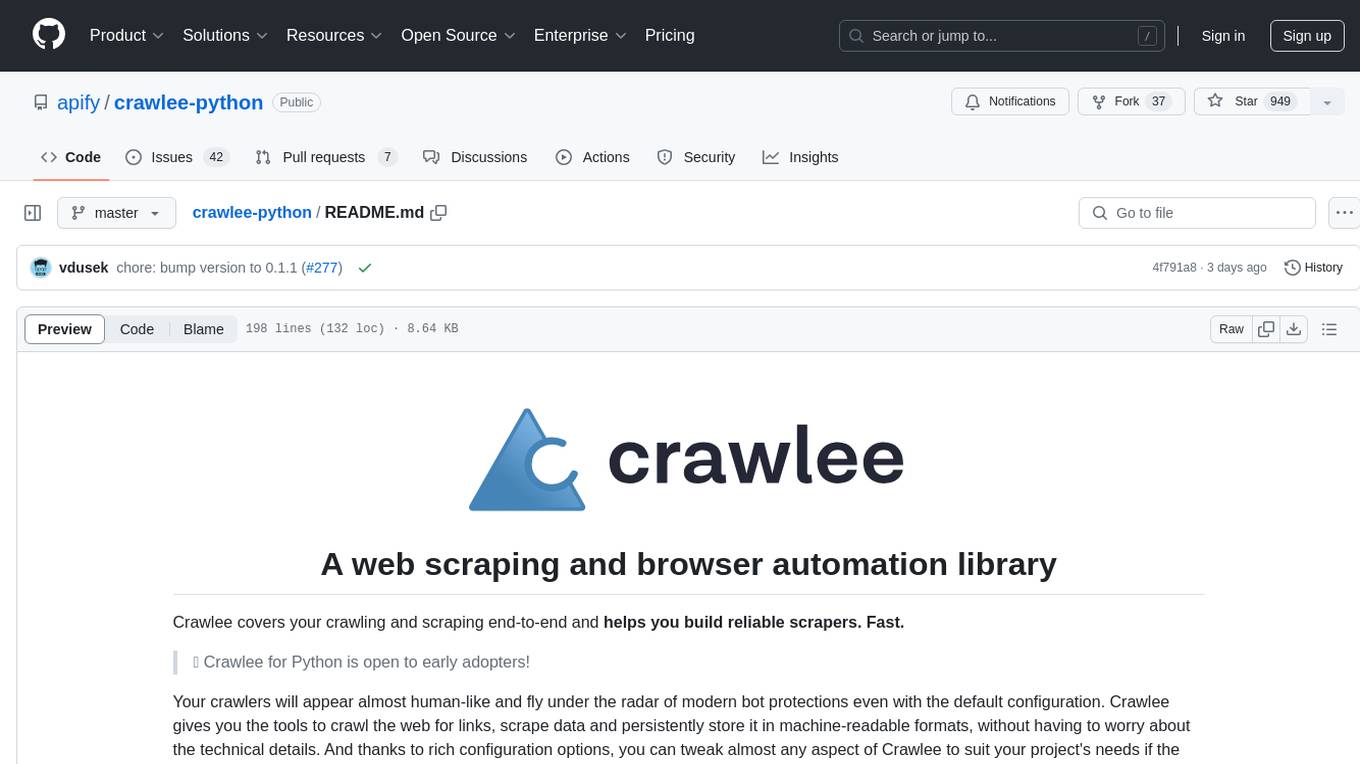
crawlee-python
Crawlee-python is a web scraping and browser automation library that covers crawling and scraping end-to-end, helping users build reliable scrapers fast. It allows users to crawl the web for links, scrape data, and store it in machine-readable formats without worrying about technical details. With rich configuration options, users can customize almost any aspect of Crawlee to suit their project's needs.
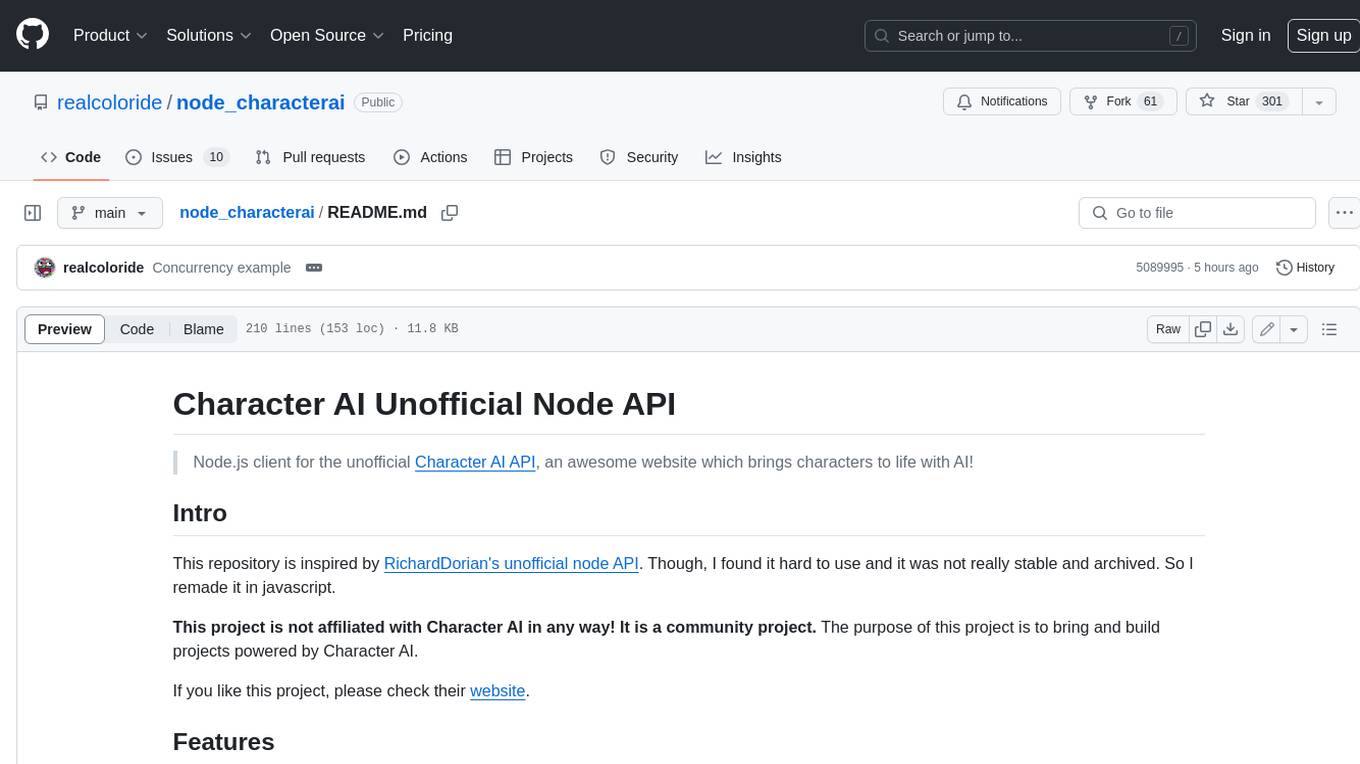
node_characterai
Node.js client for the unofficial Character AI API, an awesome website which brings characters to life with AI! This repository is inspired by RichardDorian's unofficial node API. Though, I found it hard to use and it was not really stable and archived. So I remade it in javascript. This project is not affiliated with Character AI in any way! It is a community project. The purpose of this project is to bring and build projects powered by Character AI. If you like this project, please check their website.
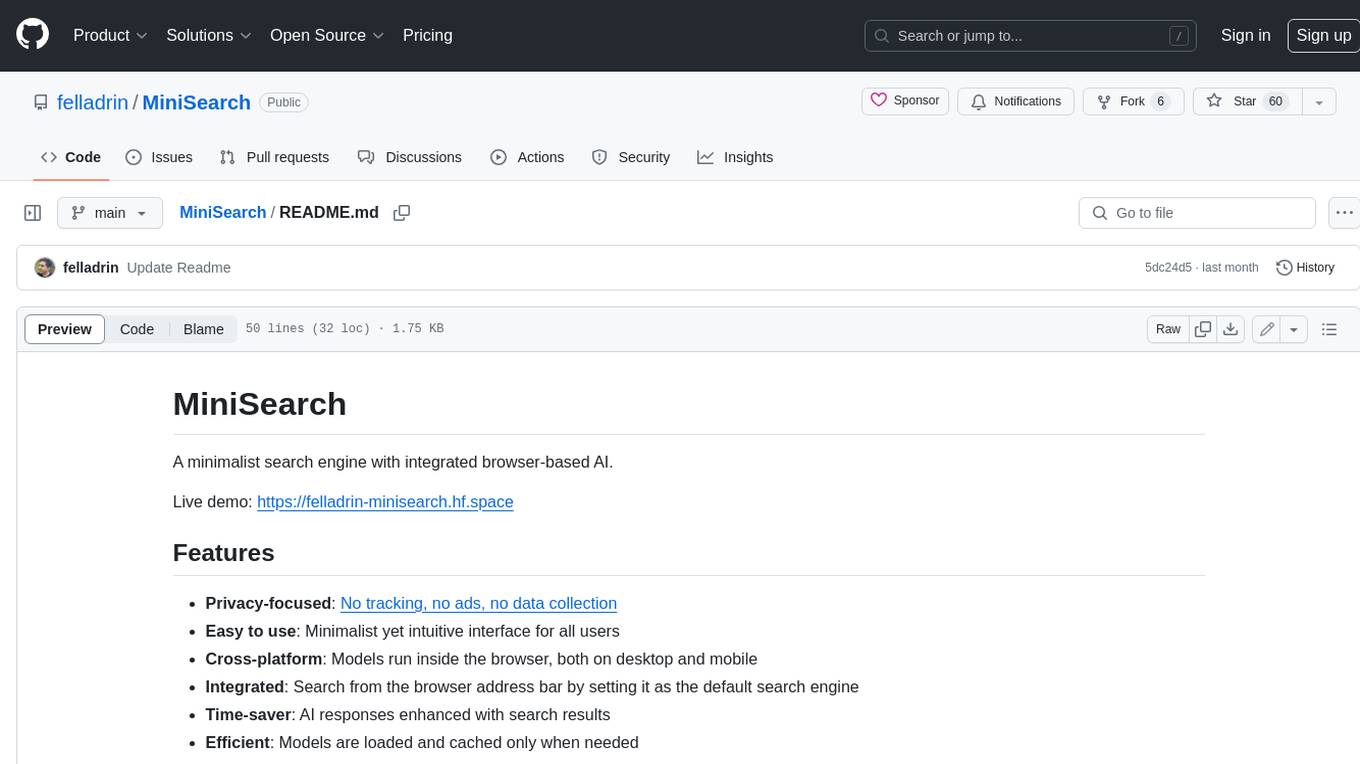
MiniSearch
MiniSearch is a minimalist search engine with integrated browser-based AI. It is privacy-focused, easy to use, cross-platform, integrated, time-saving, efficient, optimized, and open-source. MiniSearch can be used for a variety of tasks, including searching the web, finding files on your computer, and getting answers to questions. It is a great tool for anyone who wants a fast, private, and easy-to-use search engine.
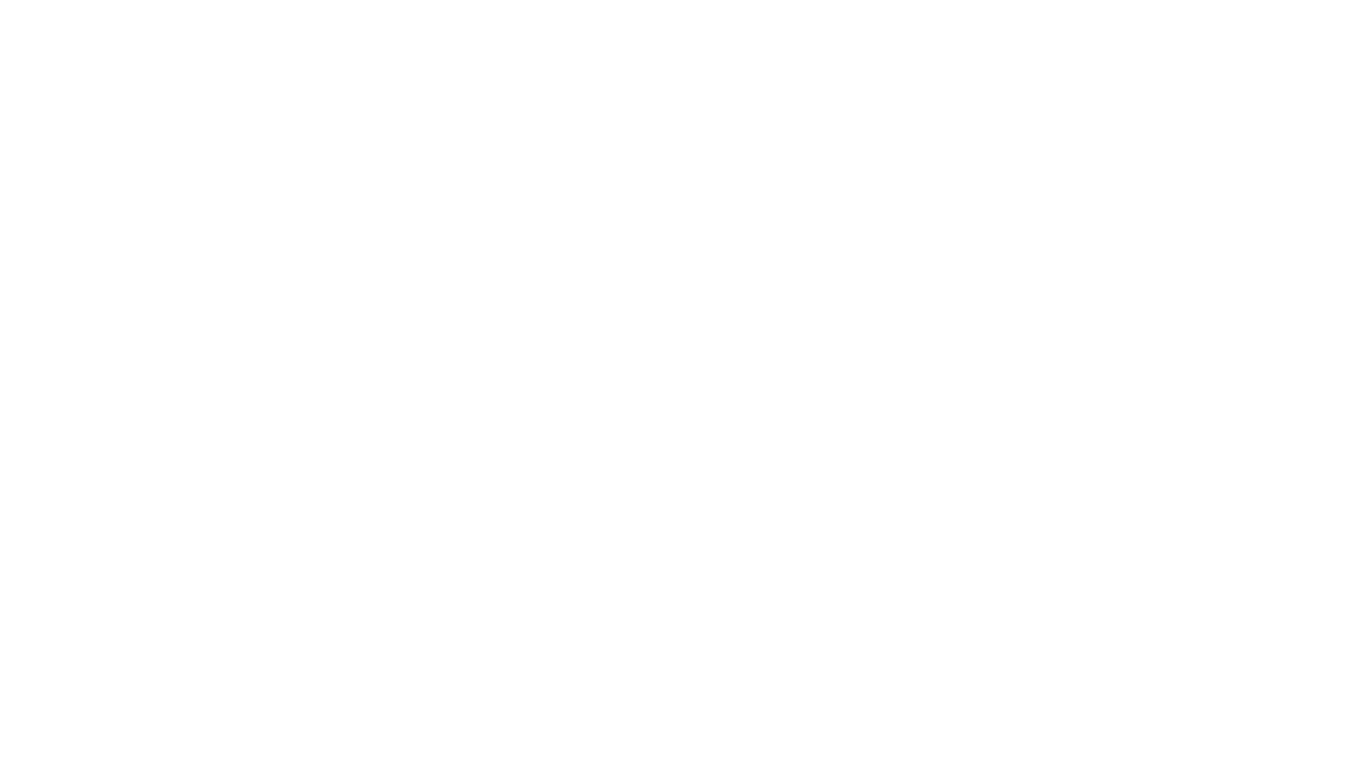
cognita
Cognita is an open-source framework to organize your RAG codebase along with a frontend to play around with different RAG customizations. It provides a simple way to organize your codebase so that it becomes easy to test it locally while also being able to deploy it in a production ready environment. The key issues that arise while productionizing RAG system from a Jupyter Notebook are: 1. **Chunking and Embedding Job** : The chunking and embedding code usually needs to be abstracted out and deployed as a job. Sometimes the job will need to run on a schedule or be trigerred via an event to keep the data updated. 2. **Query Service** : The code that generates the answer from the query needs to be wrapped up in a api server like FastAPI and should be deployed as a service. This service should be able to handle multiple queries at the same time and also autoscale with higher traffic. 3. **LLM / Embedding Model Deployment** : Often times, if we are using open-source models, we load the model in the Jupyter notebook. This will need to be hosted as a separate service in production and model will need to be called as an API. 4. **Vector DB deployment** : Most testing happens on vector DBs in memory or on disk. However, in production, the DBs need to be deployed in a more scalable and reliable way. Cognita makes it really easy to customize and experiment everything about a RAG system and still be able to deploy it in a good way. It also ships with a UI that makes it easier to try out different RAG configurations and see the results in real time. You can use it locally or with/without using any Truefoundry components. However, using Truefoundry components makes it easier to test different models and deploy the system in a scalable way. Cognita allows you to host multiple RAG systems using one app. ### Advantages of using Cognita are: 1. A central reusable repository of parsers, loaders, embedders and retrievers. 2. Ability for non-technical users to play with UI - Upload documents and perform QnA using modules built by the development team. 3. Fully API driven - which allows integration with other systems. > If you use Cognita with Truefoundry AI Gateway, you can get logging, metrics and feedback mechanism for your user queries. ### Features: 1. Support for multiple document retrievers that use `Similarity Search`, `Query Decompostion`, `Document Reranking`, etc 2. Support for SOTA OpenSource embeddings and reranking from `mixedbread-ai` 3. Support for using LLMs using `Ollama` 4. Support for incremental indexing that ingests entire documents in batches (reduces compute burden), keeps track of already indexed documents and prevents re-indexing of those docs.
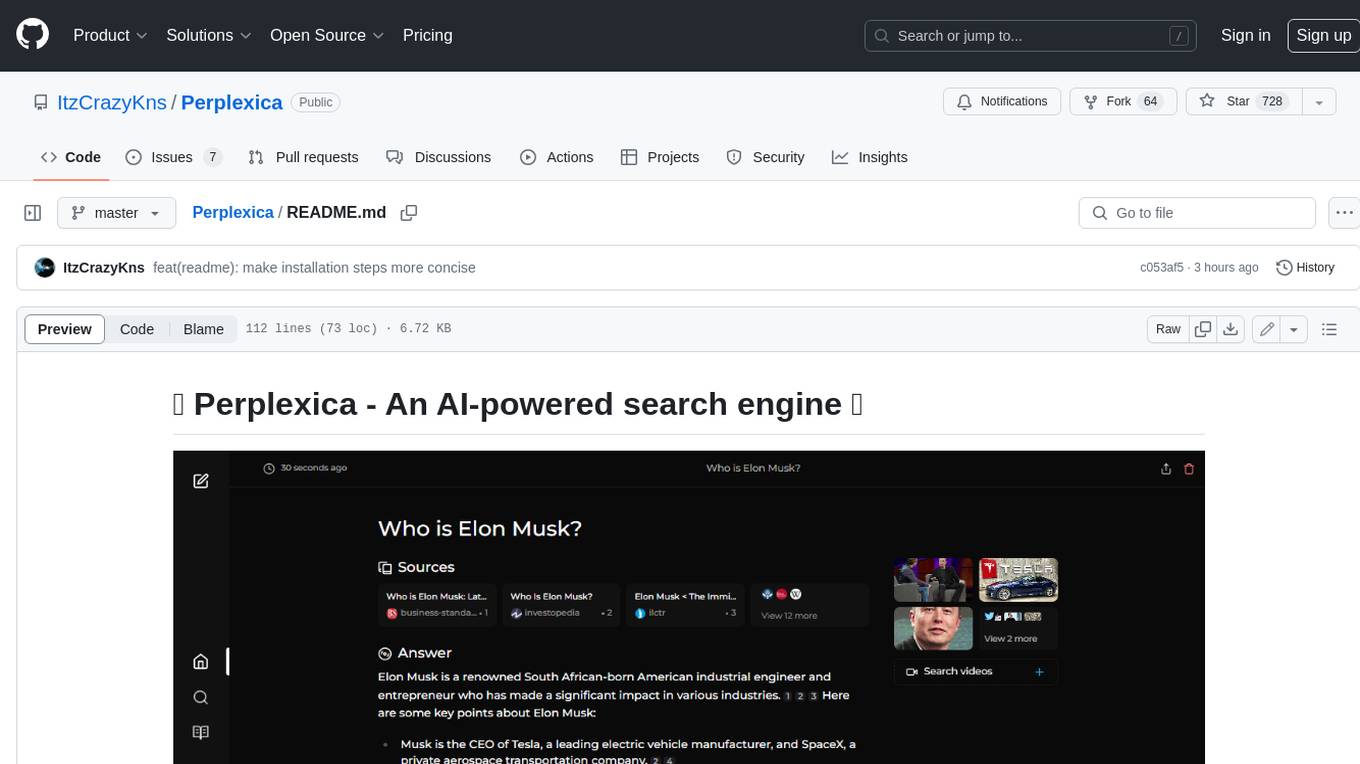
Perplexica
Perplexica is an open-source AI-powered search engine that utilizes advanced machine learning algorithms to provide clear answers with sources cited. It offers various modes like Copilot Mode, Normal Mode, and Focus Modes for specific types of questions. Perplexica ensures up-to-date information by using SearxNG metasearch engine. It also features image and video search capabilities and upcoming features include finalizing Copilot Mode and adding Discover and History Saving features.
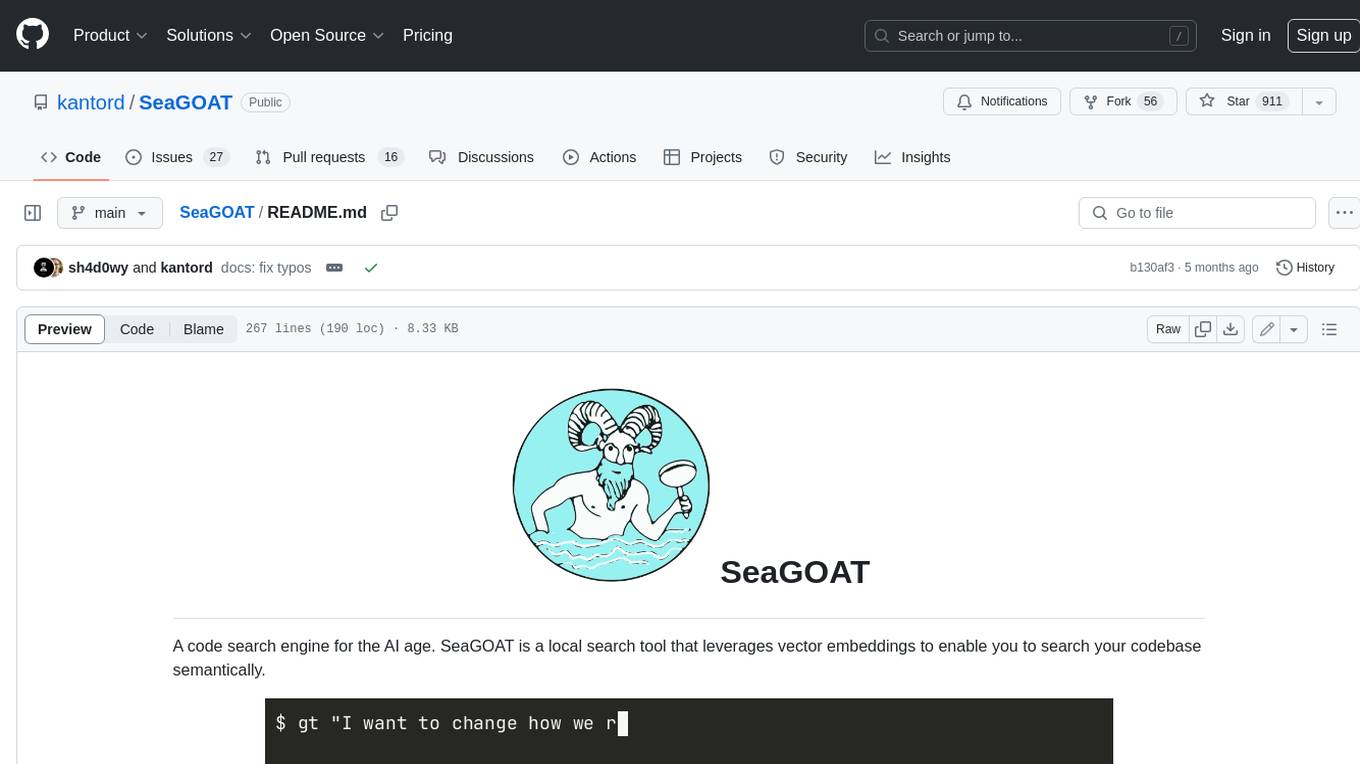
SeaGOAT
SeaGOAT is a local search tool that leverages vector embeddings to enable you to search your codebase semantically. It is designed to work on Linux, macOS, and Windows and can process files in various formats, including text, Markdown, Python, C, C++, TypeScript, JavaScript, HTML, Go, Java, PHP, and Ruby. SeaGOAT uses a vector database called ChromaDB and a local vector embedding engine to provide fast and accurate search results. It also supports regular expression/keyword-based matches. SeaGOAT is open-source and licensed under an open-source license, and users are welcome to examine the source code, raise concerns, or create pull requests to fix problems.
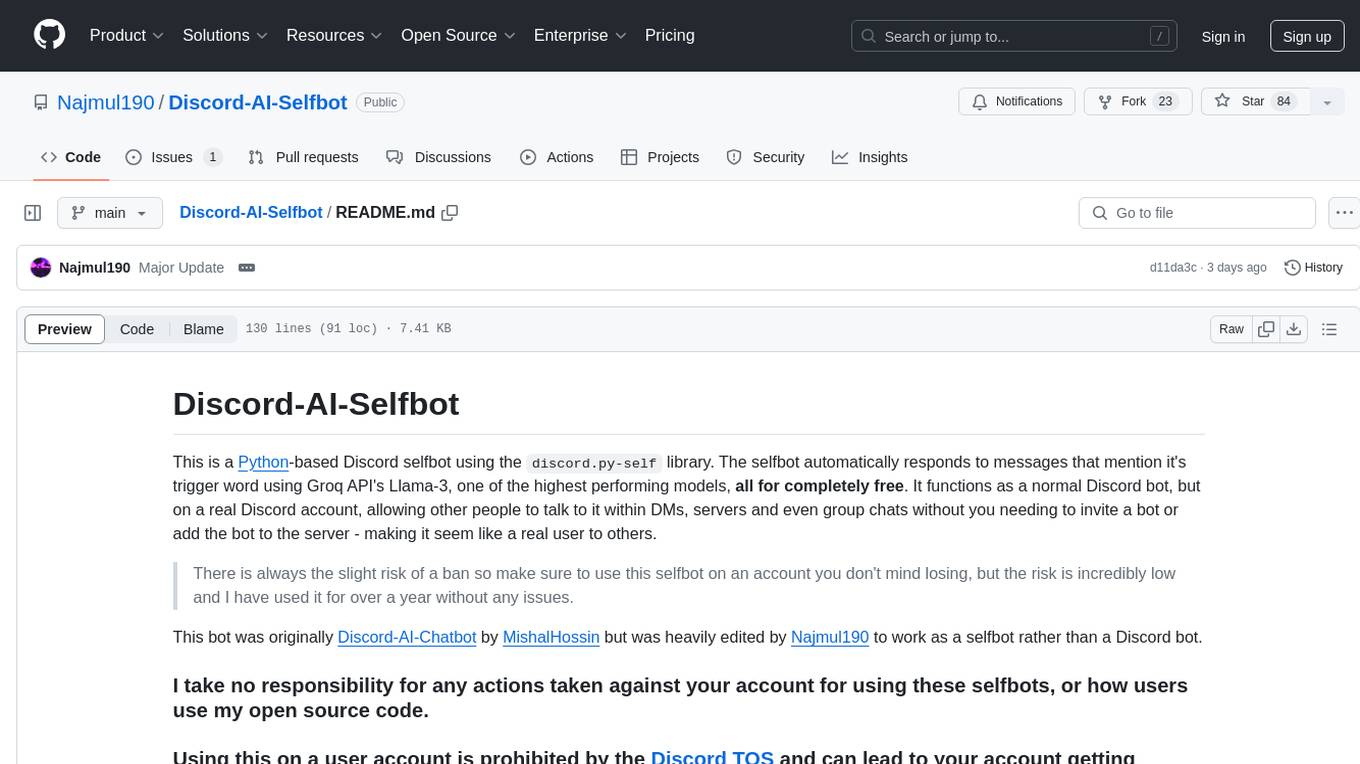
Discord-AI-Selfbot
Discord-AI-Selfbot is a Python-based Discord selfbot that uses the `discord.py-self` library to automatically respond to messages mentioning its trigger word using Groq API's Llama-3 model. It functions as a normal Discord bot on a real Discord account, enabling interactions in DMs, servers, and group chats without needing to invite a bot. The selfbot comes with features like custom AI instructions, free LLM model usage, mention and reply recognition, message handling, channel-specific responses, and a psychoanalysis command to analyze user messages for insights on personality.
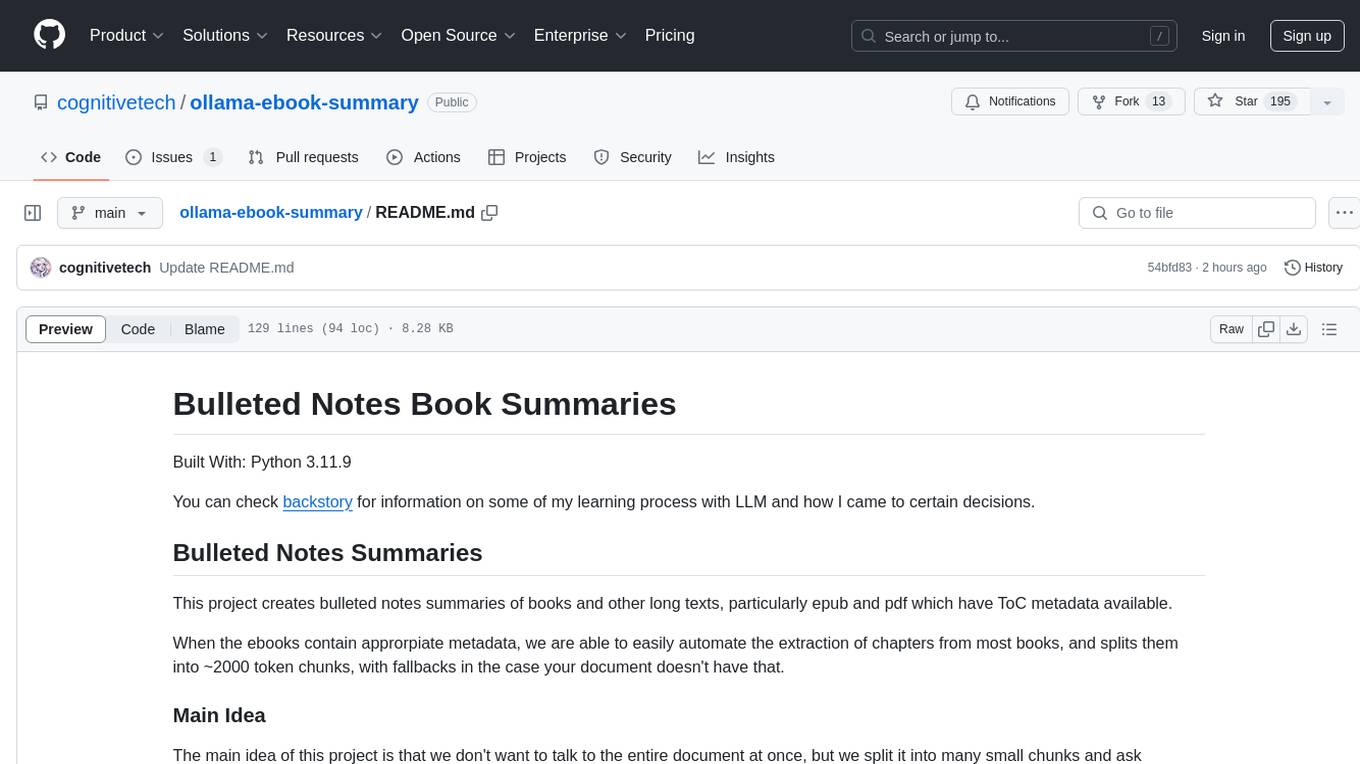
ollama-ebook-summary
The 'ollama-ebook-summary' repository is a Python project that creates bulleted notes summaries of books and long texts, particularly in epub and pdf formats with ToC metadata. It automates the extraction of chapters, splits them into ~2000 token chunks, and allows for asking arbitrary questions to parts of the text for improved granularity of response. The tool aims to provide summaries for each page of a book rather than a one-page summary of the entire document, enhancing content curation and knowledge sharing capabilities.
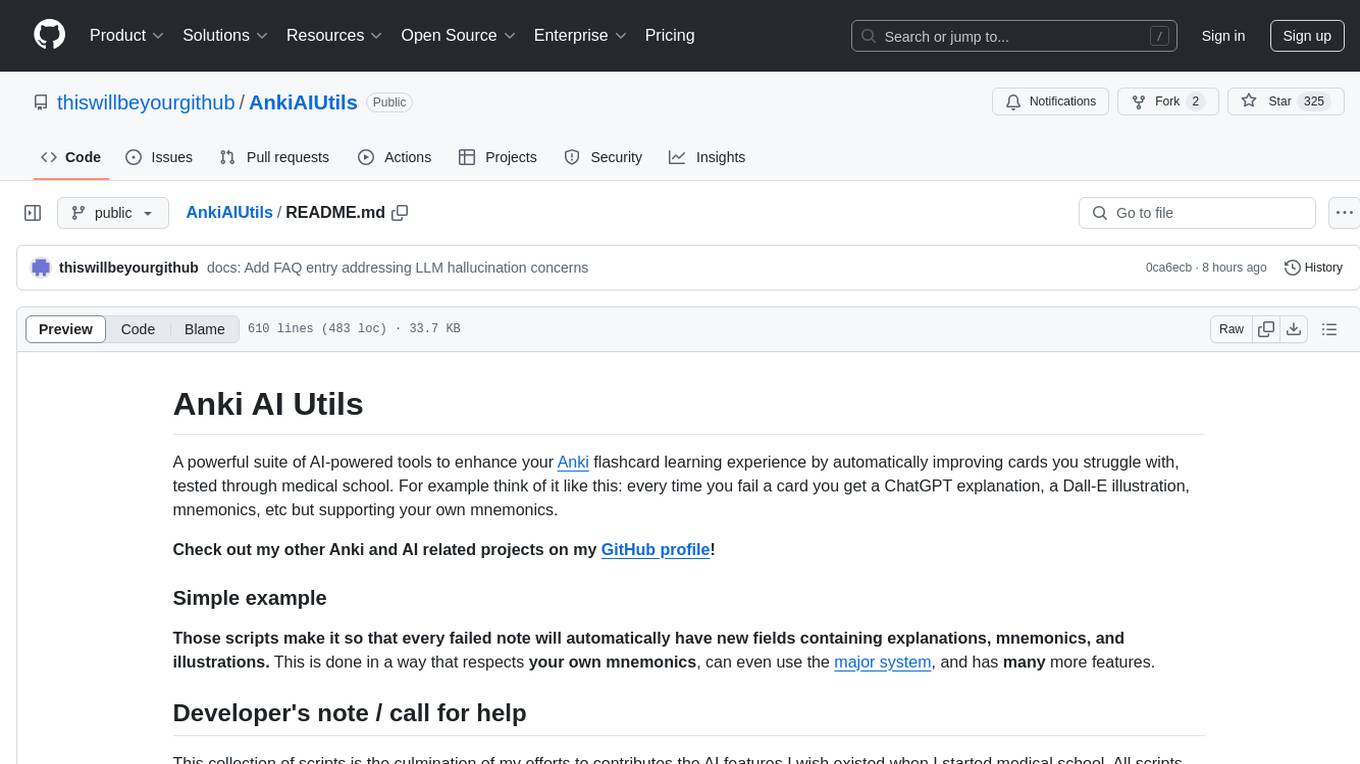
AnkiAIUtils
Anki AI Utils is a powerful suite of AI-powered tools designed to enhance your Anki flashcard learning experience by automatically improving cards you struggle with. The tools include features such as adaptive learning, personalized memory hooks, automation readiness, universal compatibility, provider agnosticism, and infinite extensibility. The toolkit consists of tools like Illustrator for creating custom mnemonic images, Reformulator for rephrasing flashcards, Mnemonics Creator for generating memorable mnemonics, Explainer for providing detailed explanations, and Mnemonics Helper for quick mnemonic generation. The project aims to motivate others to package the tools into addons for wider accessibility.
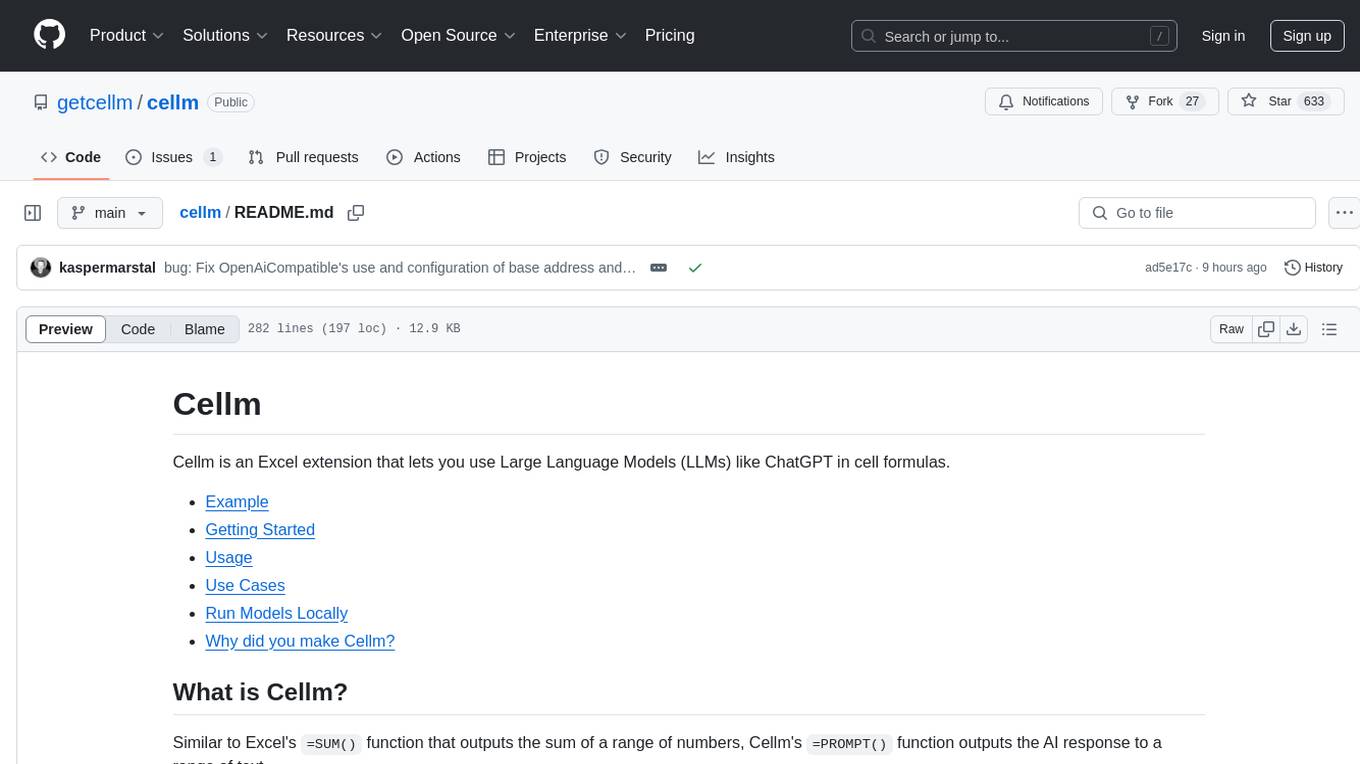
cellm
Cellm is an Excel extension that allows users to leverage Large Language Models (LLMs) like ChatGPT within cell formulas. It enables users to extract AI responses to text ranges, making it useful for automating repetitive tasks that involve data processing and analysis. Cellm supports various models from Anthropic, Mistral, OpenAI, and Google, as well as locally hosted models via Llamafiles, Ollama, or vLLM. The tool is designed to simplify the integration of AI capabilities into Excel for tasks such as text classification, data cleaning, content summarization, entity extraction, and more.
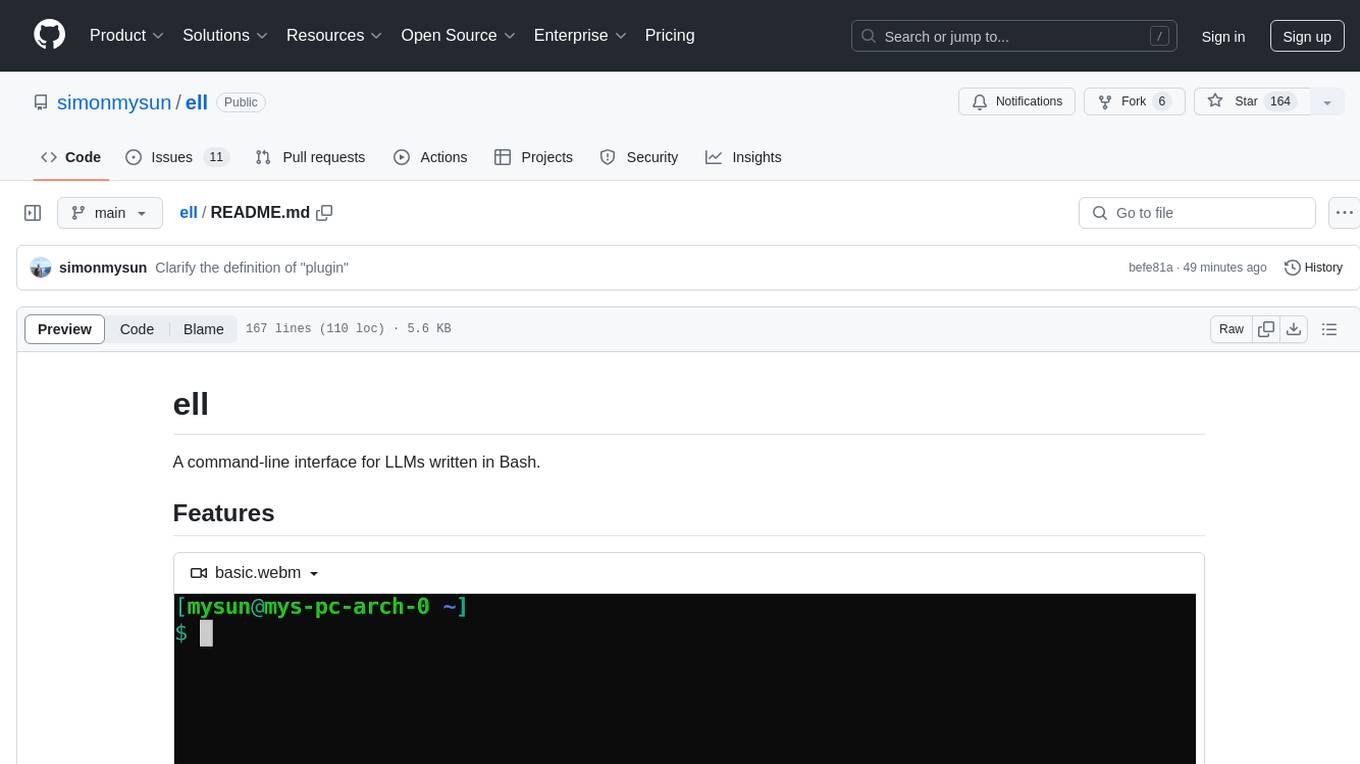
ell
ell is a command-line interface for Language Model Models (LLMs) written in Bash. It allows users to interact with LLMs from the terminal, supports piping, context bringing, and chatting with LLMs. Users can also call functions and use templates. The tool requires bash, jq for JSON parsing, curl for HTTPS requests, and perl for PCRE. Configuration involves setting variables for different LLM models and APIs. Usage examples include asking questions, specifying models, recording input/output, running in interactive mode, and using templates. The tool is lightweight, easy to install, and pipe-friendly, making it suitable for interacting with LLMs in a terminal environment.
For similar tasks
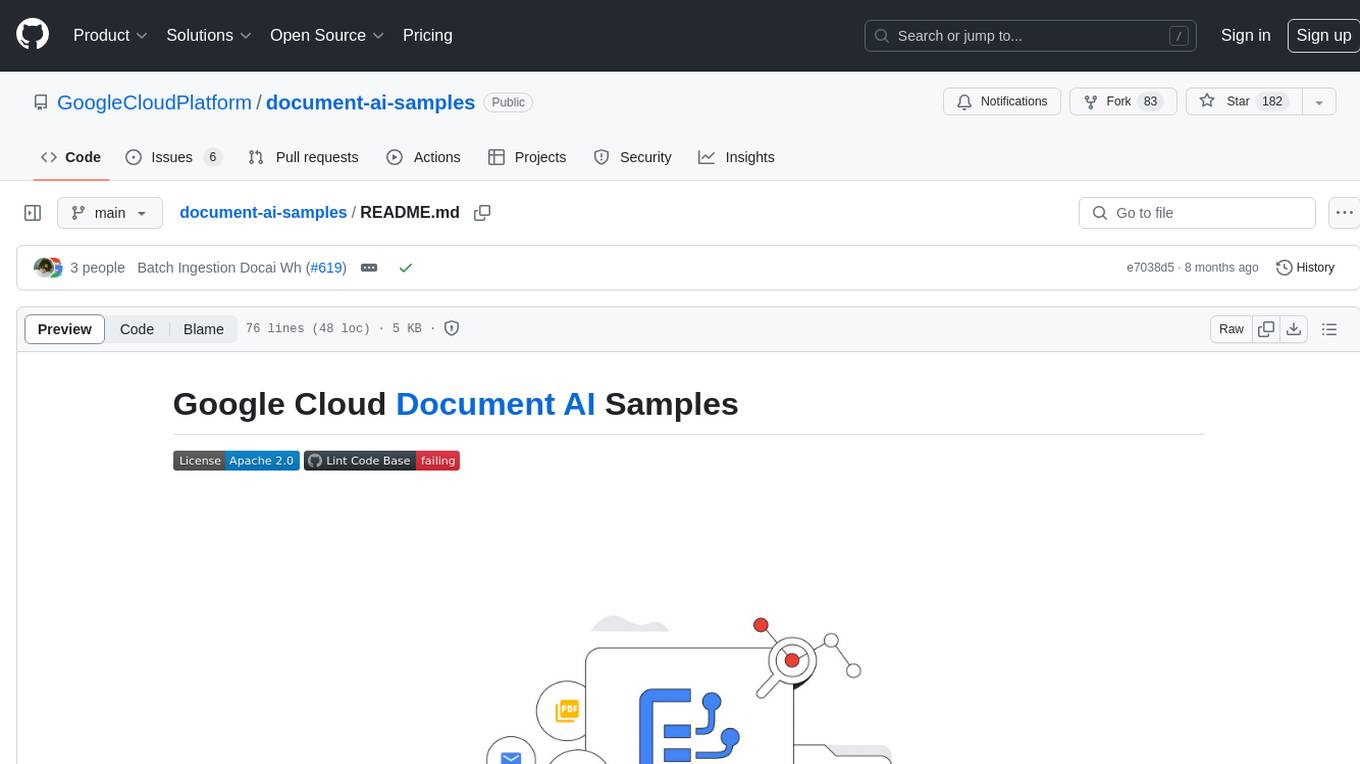
document-ai-samples
The Google Cloud Document AI Samples repository contains code samples and Community Samples demonstrating how to analyze, classify, and search documents using Google Cloud Document AI. It includes various projects showcasing different functionalities such as integrating with Google Drive, processing documents using Python, content moderation with Dialogflow CX, fraud detection, language extraction, paper summarization, tax processing pipeline, and more. The repository also provides access to test document files stored in a publicly-accessible Google Cloud Storage Bucket. Additionally, there are codelabs available for optical character recognition (OCR), form parsing, specialized processors, and managing Document AI processors. Community samples, like the PDF Annotator Sample, are also included. Contributions are welcome, and users can seek help or report issues through the repository's issues page. Please note that this repository is not an officially supported Google product and is intended for demonstrative purposes only.
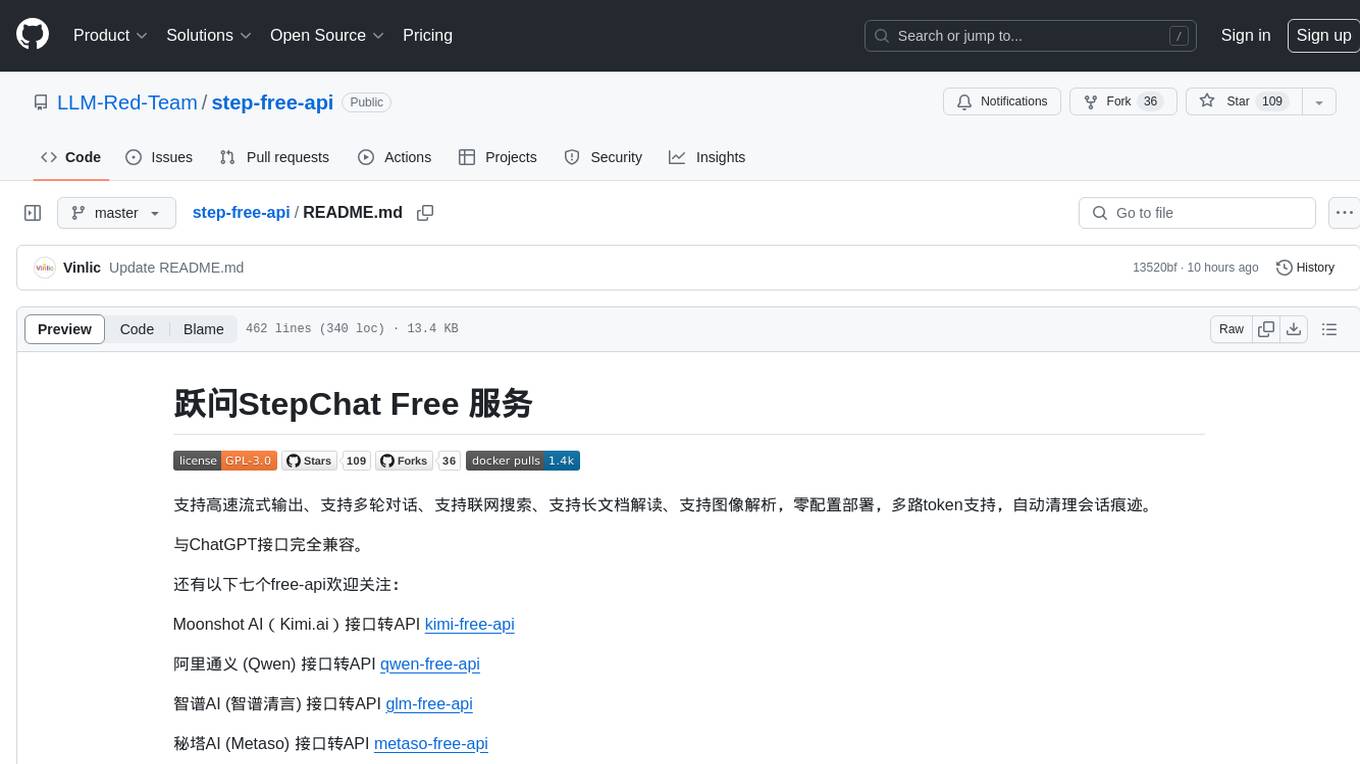
step-free-api
The StepChat Free service provides high-speed streaming output, multi-turn dialogue support, online search support, long document interpretation, and image parsing. It offers zero-configuration deployment, multi-token support, and automatic session trace cleaning. It is fully compatible with the ChatGPT interface. Additionally, it provides seven other free APIs for various services. The repository includes a disclaimer about using reverse APIs and encourages users to avoid commercial use to prevent service pressure on the official platform. It offers online testing links, showcases different demos, and provides deployment guides for Docker, Docker-compose, Render, Vercel, and native deployments. The repository also includes information on using multiple accounts, optimizing Nginx reverse proxy, and checking the liveliness of refresh tokens.
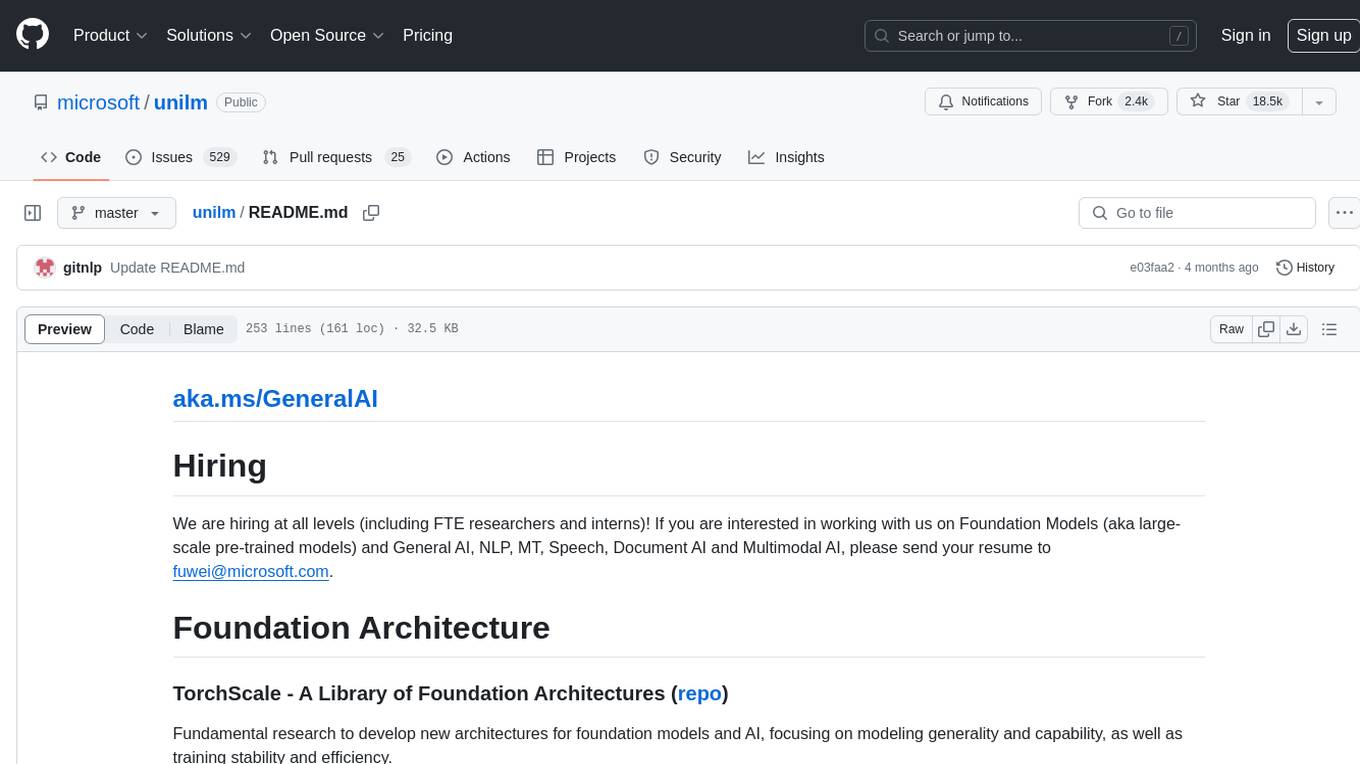
unilm
The 'unilm' repository is a collection of tools, models, and architectures for Foundation Models and General AI, focusing on tasks such as NLP, MT, Speech, Document AI, and Multimodal AI. It includes various pre-trained models, such as UniLM, InfoXLM, DeltaLM, MiniLM, AdaLM, BEiT, LayoutLM, WavLM, VALL-E, and more, designed for tasks like language understanding, generation, translation, vision, speech, and multimodal processing. The repository also features toolkits like s2s-ft for sequence-to-sequence fine-tuning and Aggressive Decoding for efficient sequence-to-sequence decoding. Additionally, it offers applications like TrOCR for OCR, LayoutReader for reading order detection, and XLM-T for multilingual NMT.
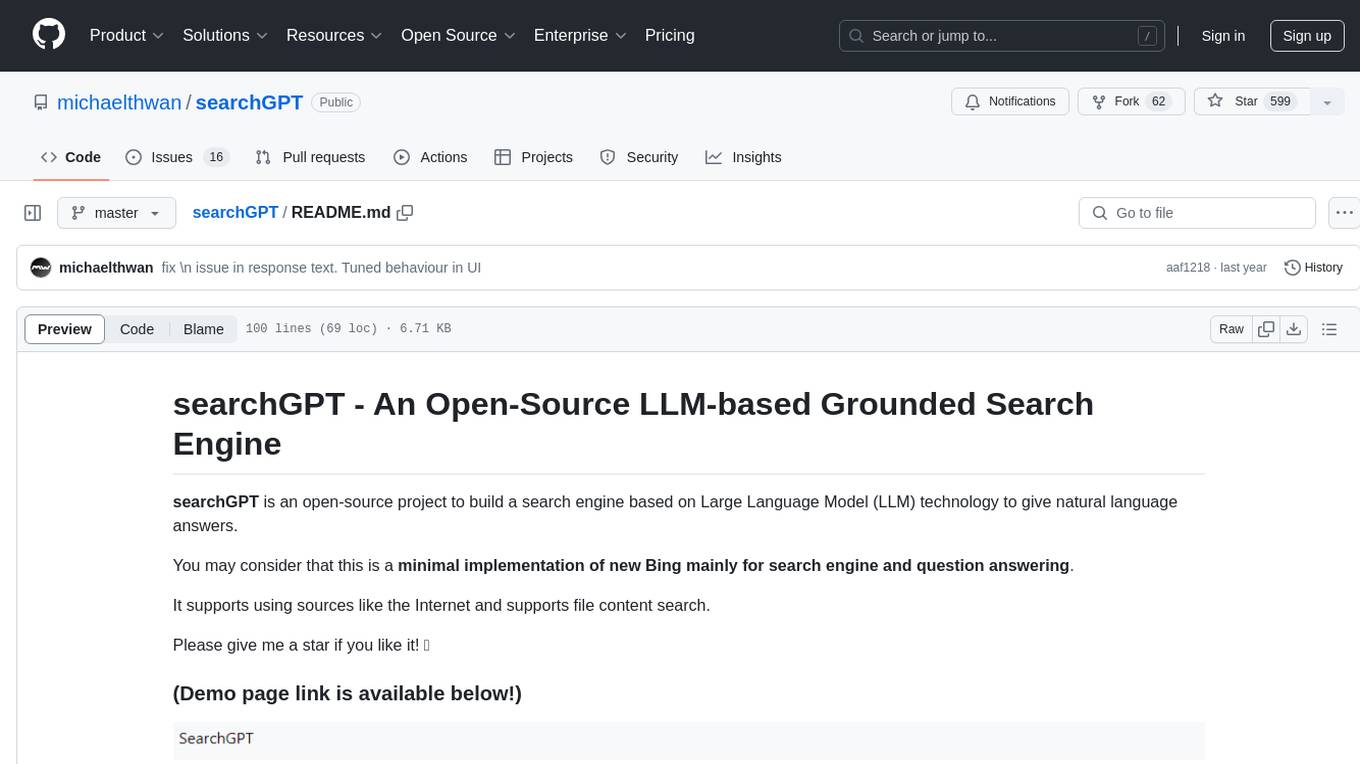
searchGPT
searchGPT is an open-source project that aims to build a search engine based on Large Language Model (LLM) technology to provide natural language answers. It supports web search with real-time results, file content search, and semantic search from sources like the Internet. The tool integrates LLM technologies such as OpenAI and GooseAI, and offers an easy-to-use frontend user interface. The project is designed to provide grounded answers by referencing real-time factual information, addressing the limitations of LLM's training data. Contributions, especially from frontend developers, are welcome under the MIT License.
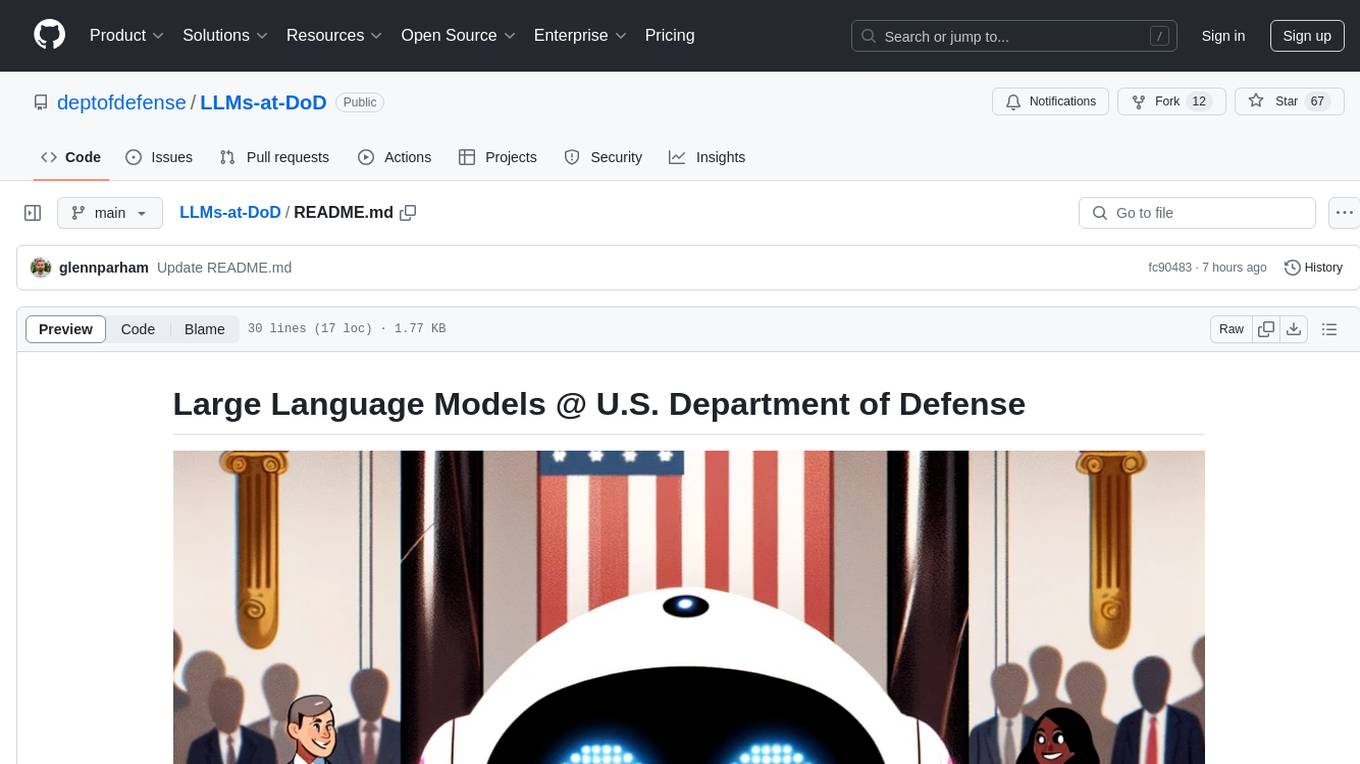
LLMs-at-DoD
This repository contains tutorials for using Large Language Models (LLMs) in the U.S. Department of Defense. The tutorials utilize open-source frameworks and LLMs, allowing users to run them in their own cloud environments. The repository is maintained by the Defense Digital Service and welcomes contributions from users.
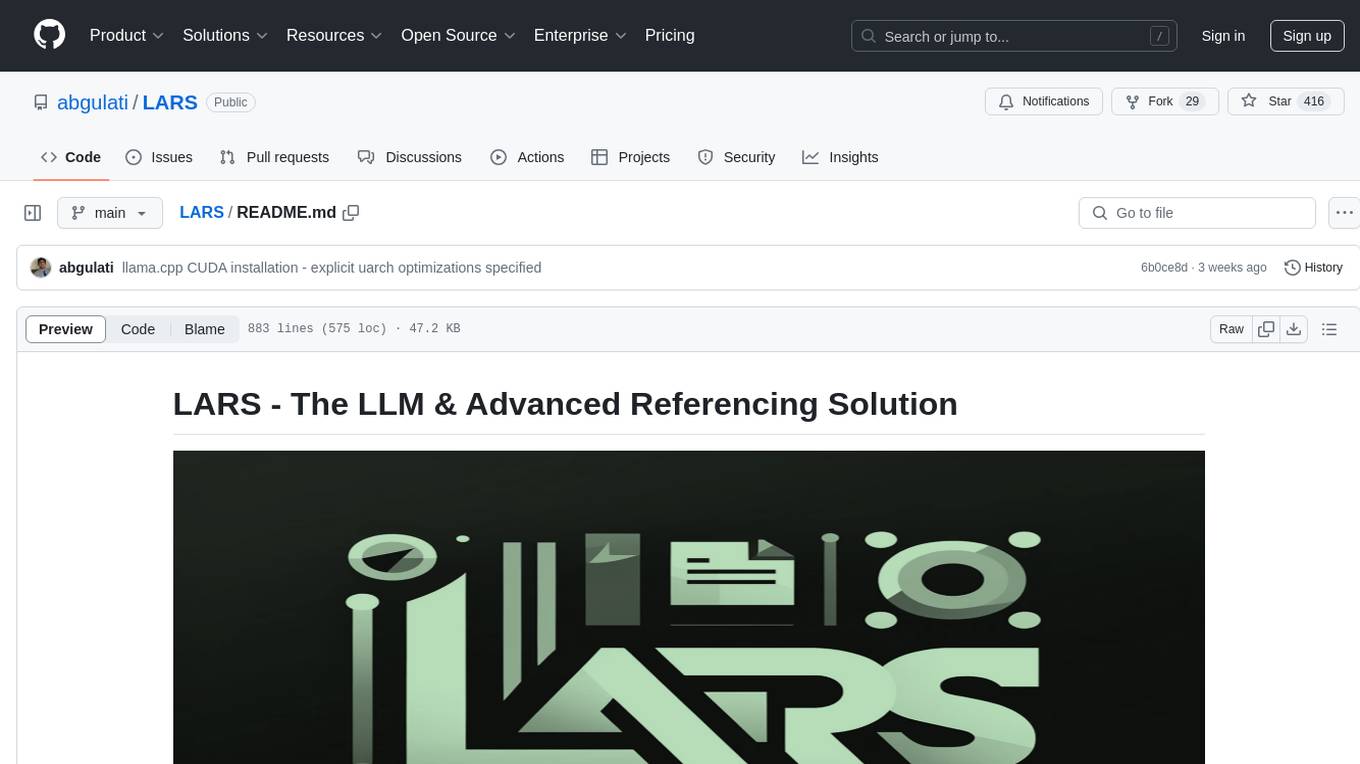
LARS
LARS is an application that enables users to run Large Language Models (LLMs) locally on their devices, upload their own documents, and engage in conversations where the LLM grounds its responses with the uploaded content. The application focuses on Retrieval Augmented Generation (RAG) to increase accuracy and reduce AI-generated inaccuracies. LARS provides advanced citations, supports various file formats, allows follow-up questions, provides full chat history, and offers customization options for LLM settings. Users can force enable or disable RAG, change system prompts, and tweak advanced LLM settings. The application also supports GPU-accelerated inferencing, multiple embedding models, and text extraction methods. LARS is open-source and aims to be the ultimate RAG-centric LLM application.
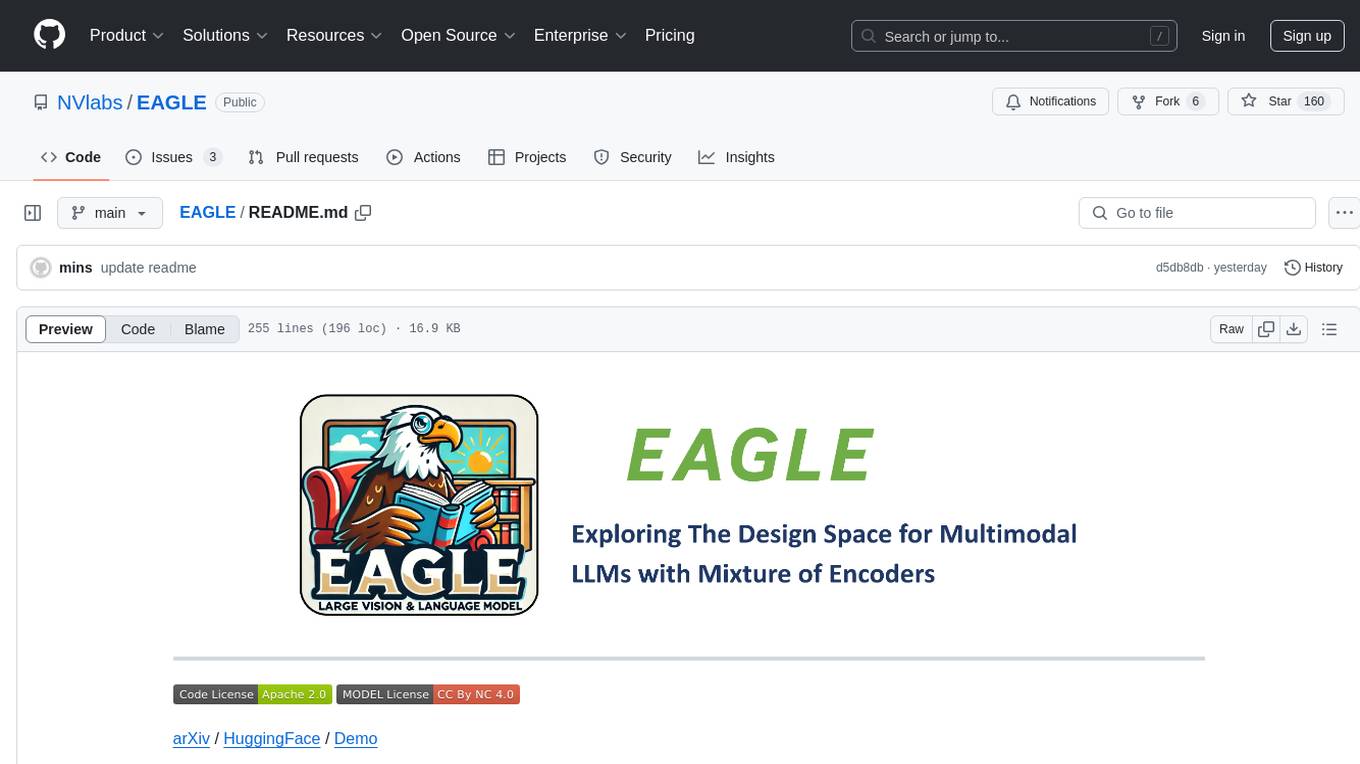
EAGLE
Eagle is a family of Vision-Centric High-Resolution Multimodal LLMs that enhance multimodal LLM perception using a mix of vision encoders and various input resolutions. The model features a channel-concatenation-based fusion for vision experts with different architectures and knowledge, supporting up to over 1K input resolution. It excels in resolution-sensitive tasks like optical character recognition and document understanding.
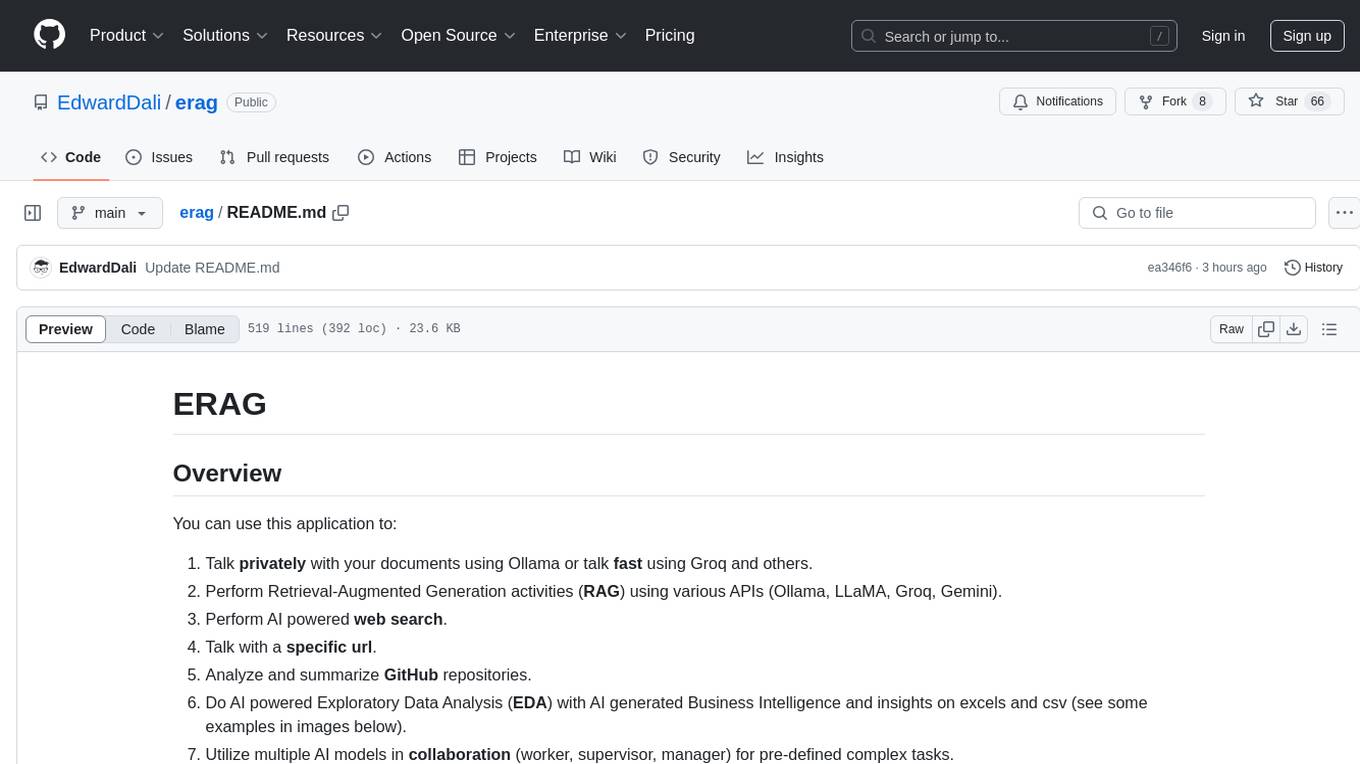
erag
ERAG is an advanced system that combines lexical, semantic, text, and knowledge graph searches with conversation context to provide accurate and contextually relevant responses. This tool processes various document types, creates embeddings, builds knowledge graphs, and uses this information to answer user queries intelligently. It includes modules for interacting with web content, GitHub repositories, and performing exploratory data analysis using various language models.
For similar jobs
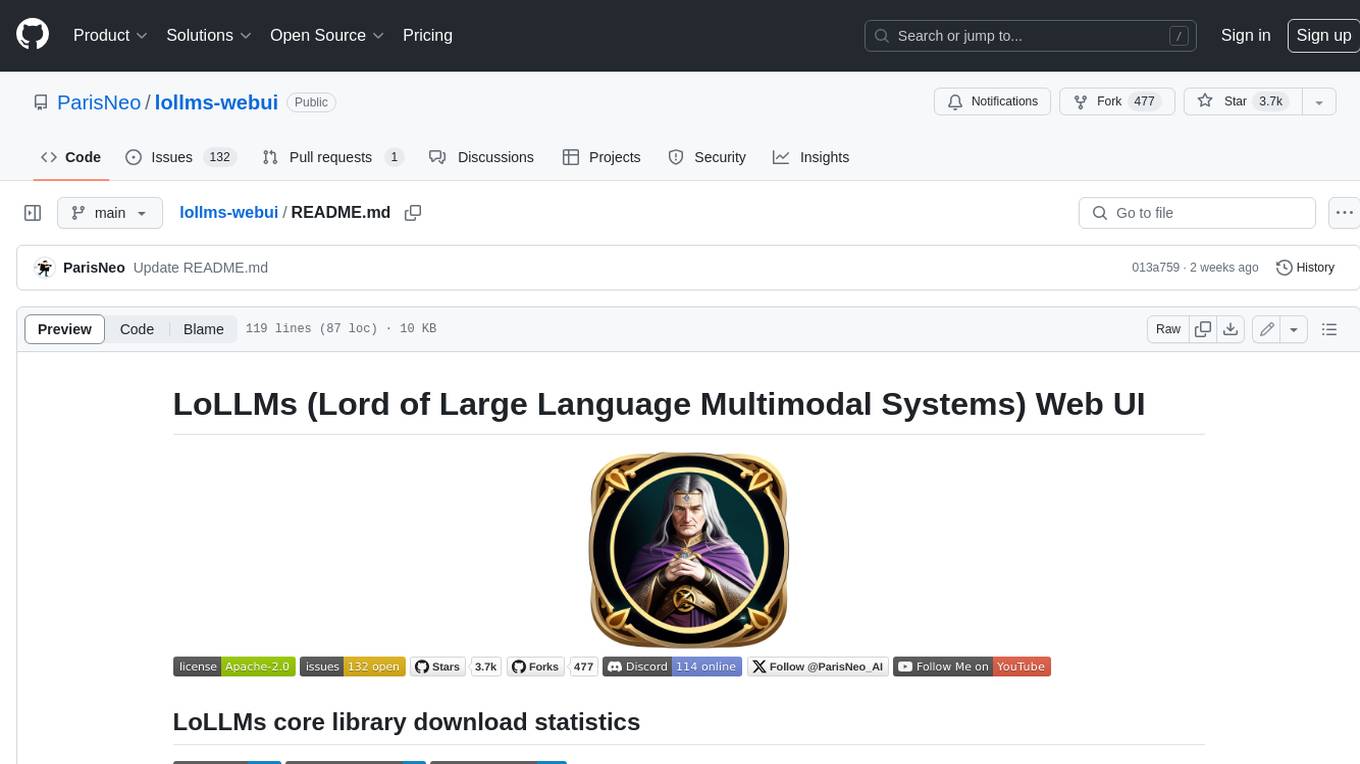
lollms-webui
LoLLMs WebUI (Lord of Large Language Multimodal Systems: One tool to rule them all) is a user-friendly interface to access and utilize various LLM (Large Language Models) and other AI models for a wide range of tasks. With over 500 AI expert conditionings across diverse domains and more than 2500 fine tuned models over multiple domains, LoLLMs WebUI provides an immediate resource for any problem, from car repair to coding assistance, legal matters, medical diagnosis, entertainment, and more. The easy-to-use UI with light and dark mode options, integration with GitHub repository, support for different personalities, and features like thumb up/down rating, copy, edit, and remove messages, local database storage, search, export, and delete multiple discussions, make LoLLMs WebUI a powerful and versatile tool.
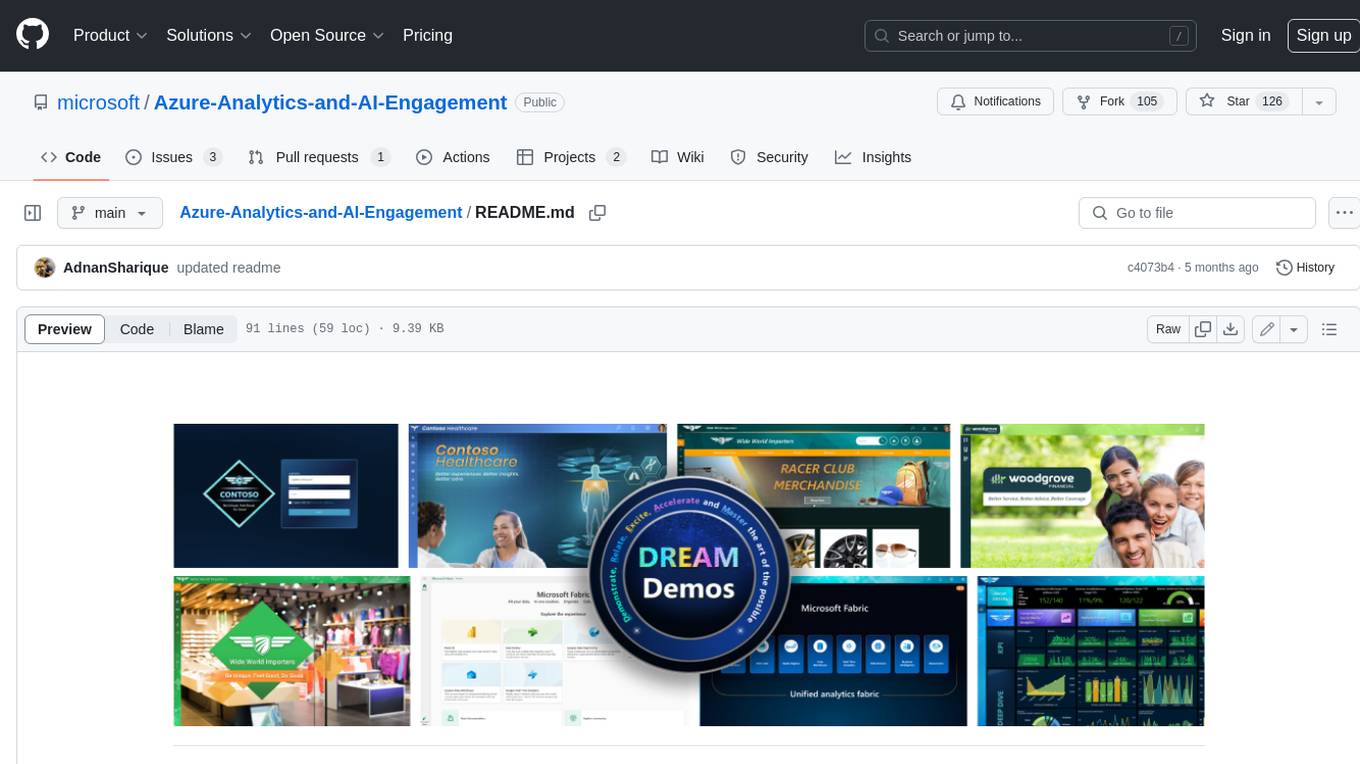
Azure-Analytics-and-AI-Engagement
The Azure-Analytics-and-AI-Engagement repository provides packaged Industry Scenario DREAM Demos with ARM templates (Containing a demo web application, Power BI reports, Synapse resources, AML Notebooks etc.) that can be deployed in a customer’s subscription using the CAPE tool within a matter of few hours. Partners can also deploy DREAM Demos in their own subscriptions using DPoC.
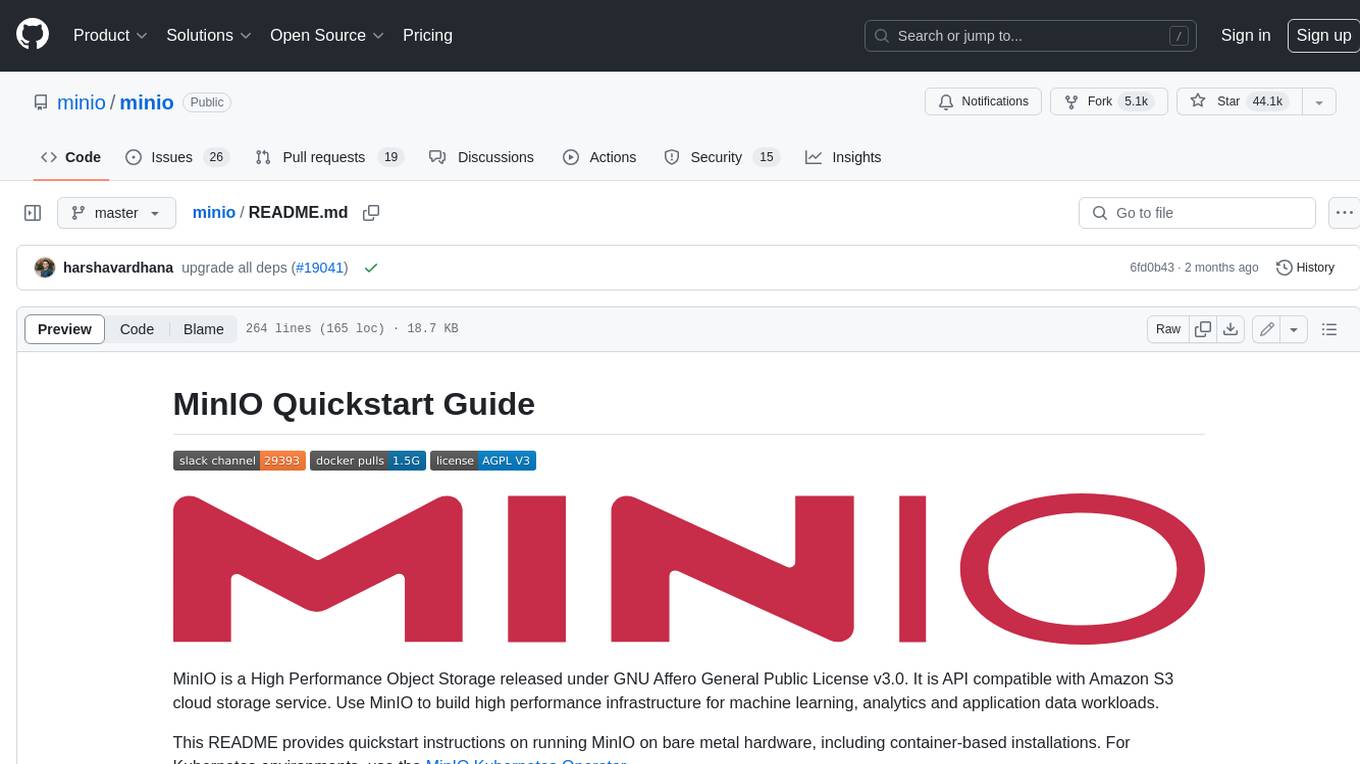
minio
MinIO is a High Performance Object Storage released under GNU Affero General Public License v3.0. It is API compatible with Amazon S3 cloud storage service. Use MinIO to build high performance infrastructure for machine learning, analytics and application data workloads.
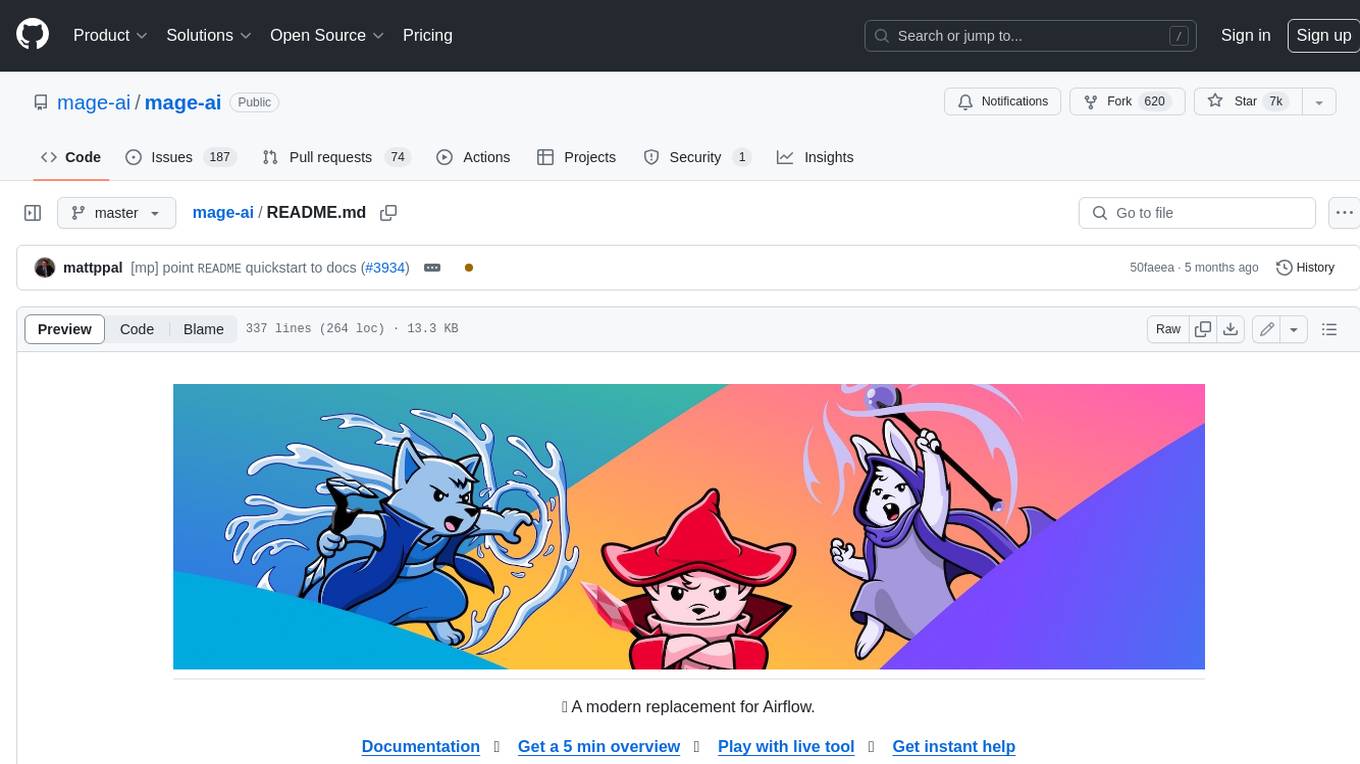
mage-ai
Mage is an open-source data pipeline tool for transforming and integrating data. It offers an easy developer experience, engineering best practices built-in, and data as a first-class citizen. Mage makes it easy to build, preview, and launch data pipelines, and provides observability and scaling capabilities. It supports data integrations, streaming pipelines, and dbt integration.
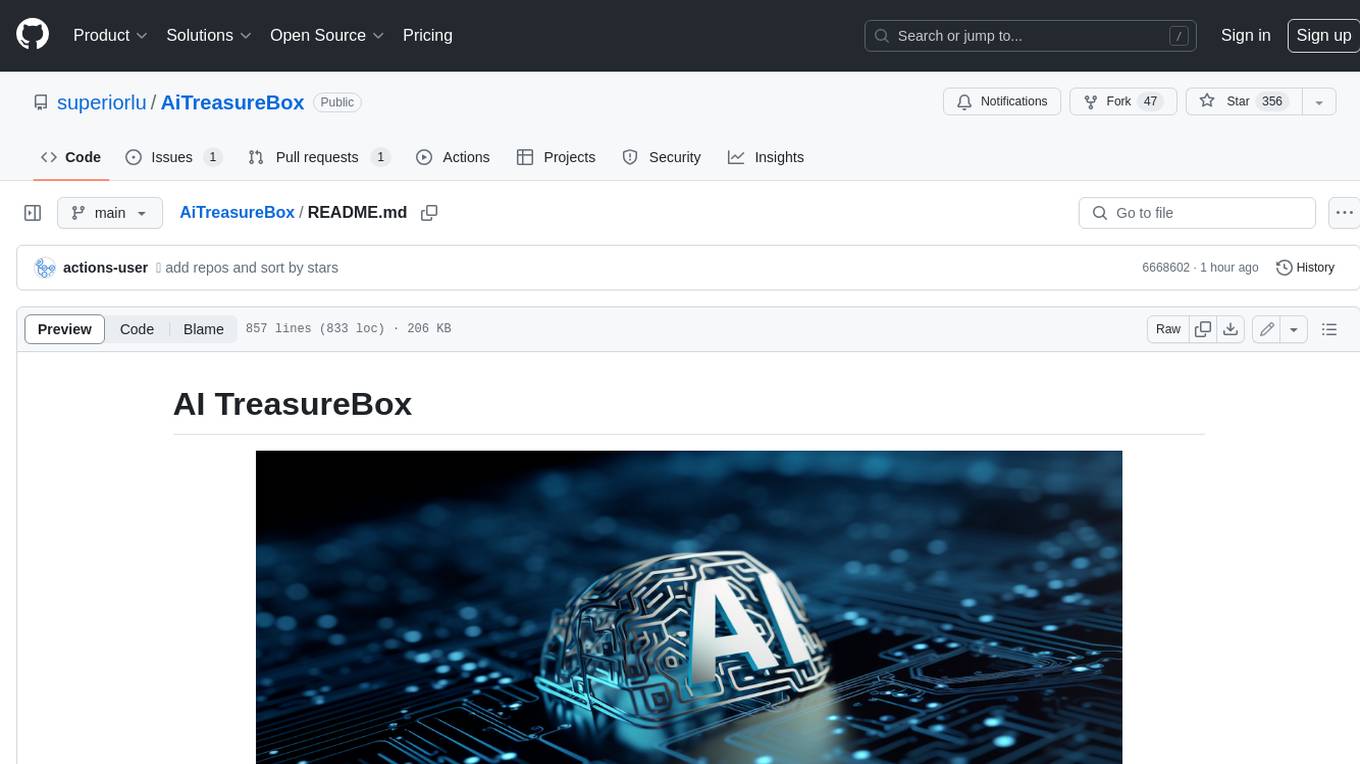
AiTreasureBox
AiTreasureBox is a versatile AI tool that provides a collection of pre-trained models and algorithms for various machine learning tasks. It simplifies the process of implementing AI solutions by offering ready-to-use components that can be easily integrated into projects. With AiTreasureBox, users can quickly prototype and deploy AI applications without the need for extensive knowledge in machine learning or deep learning. The tool covers a wide range of tasks such as image classification, text generation, sentiment analysis, object detection, and more. It is designed to be user-friendly and accessible to both beginners and experienced developers, making AI development more efficient and accessible to a wider audience.
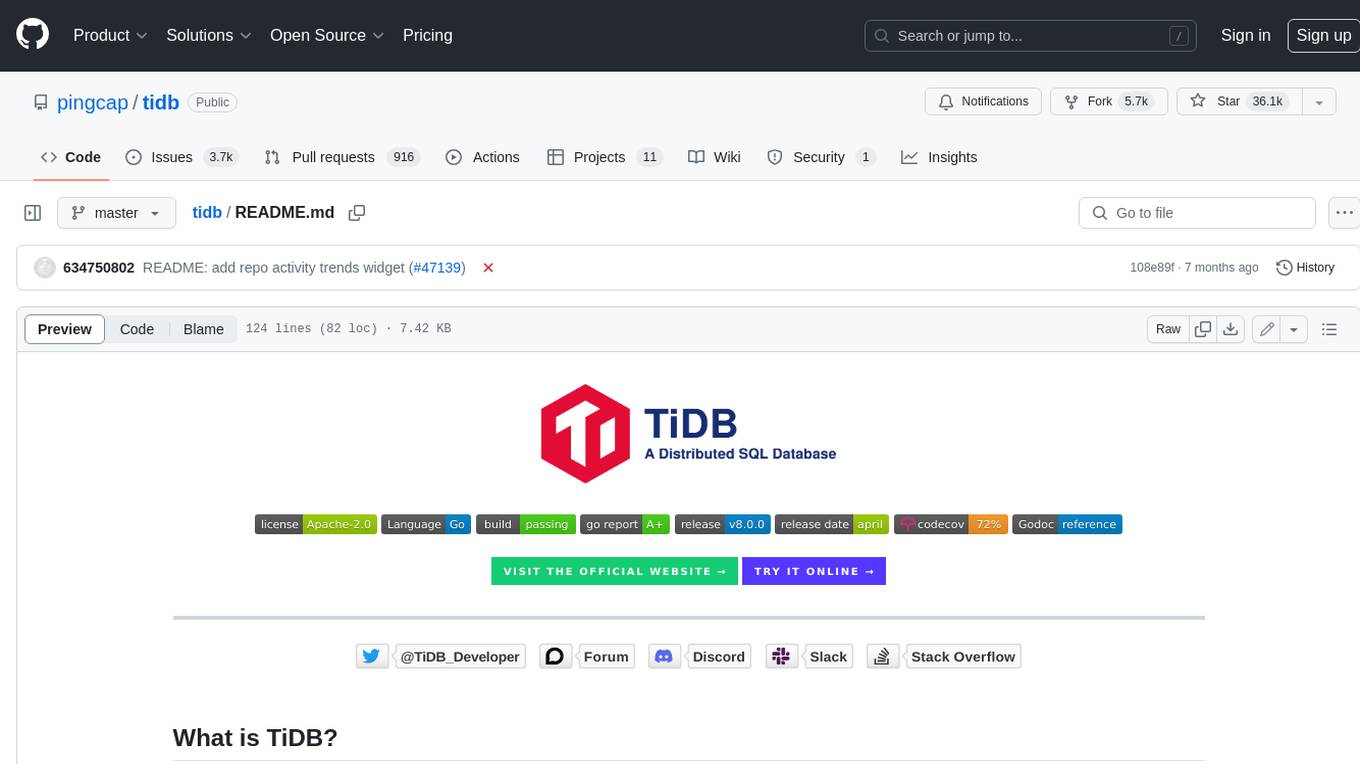
tidb
TiDB is an open-source distributed SQL database that supports Hybrid Transactional and Analytical Processing (HTAP) workloads. It is MySQL compatible and features horizontal scalability, strong consistency, and high availability.
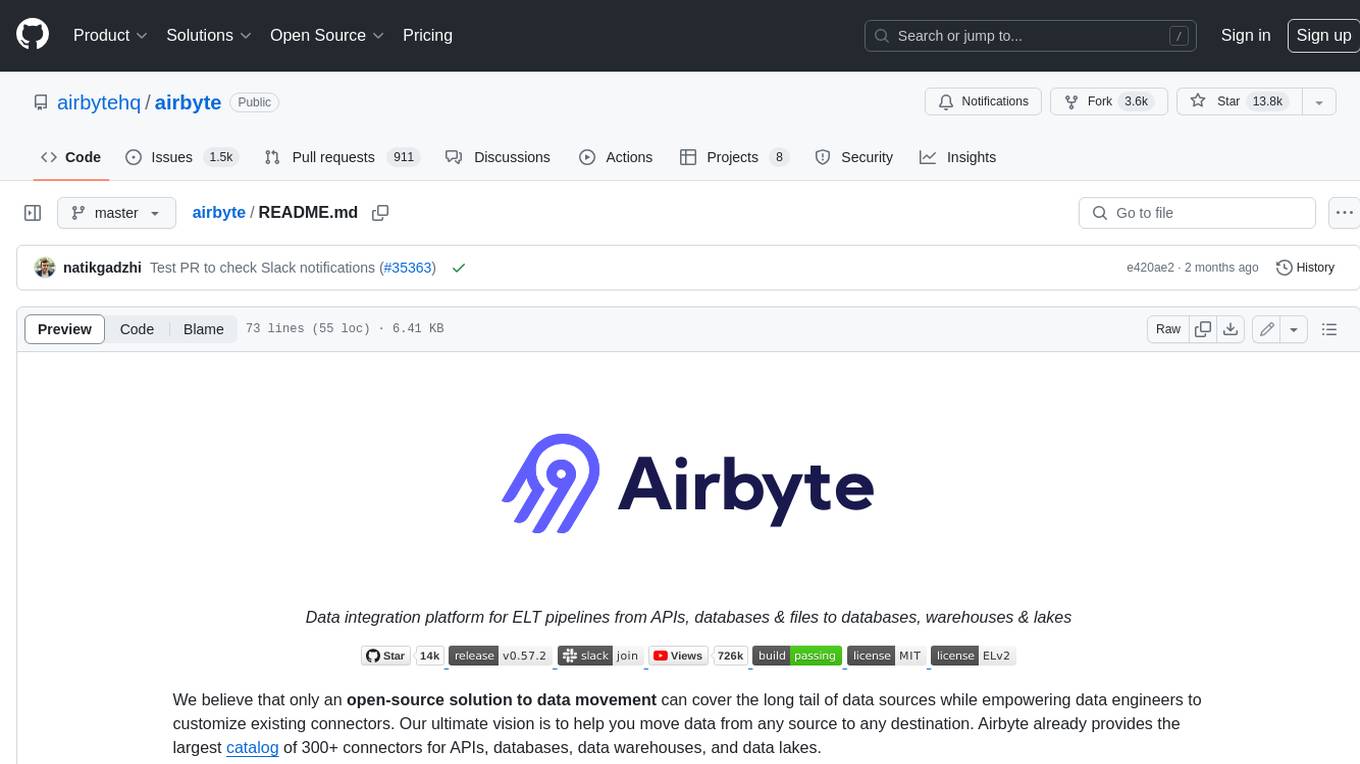
airbyte
Airbyte is an open-source data integration platform that makes it easy to move data from any source to any destination. With Airbyte, you can build and manage data pipelines without writing any code. Airbyte provides a library of pre-built connectors that make it easy to connect to popular data sources and destinations. You can also create your own connectors using Airbyte's no-code Connector Builder or low-code CDK. Airbyte is used by data engineers and analysts at companies of all sizes to build and manage their data pipelines.
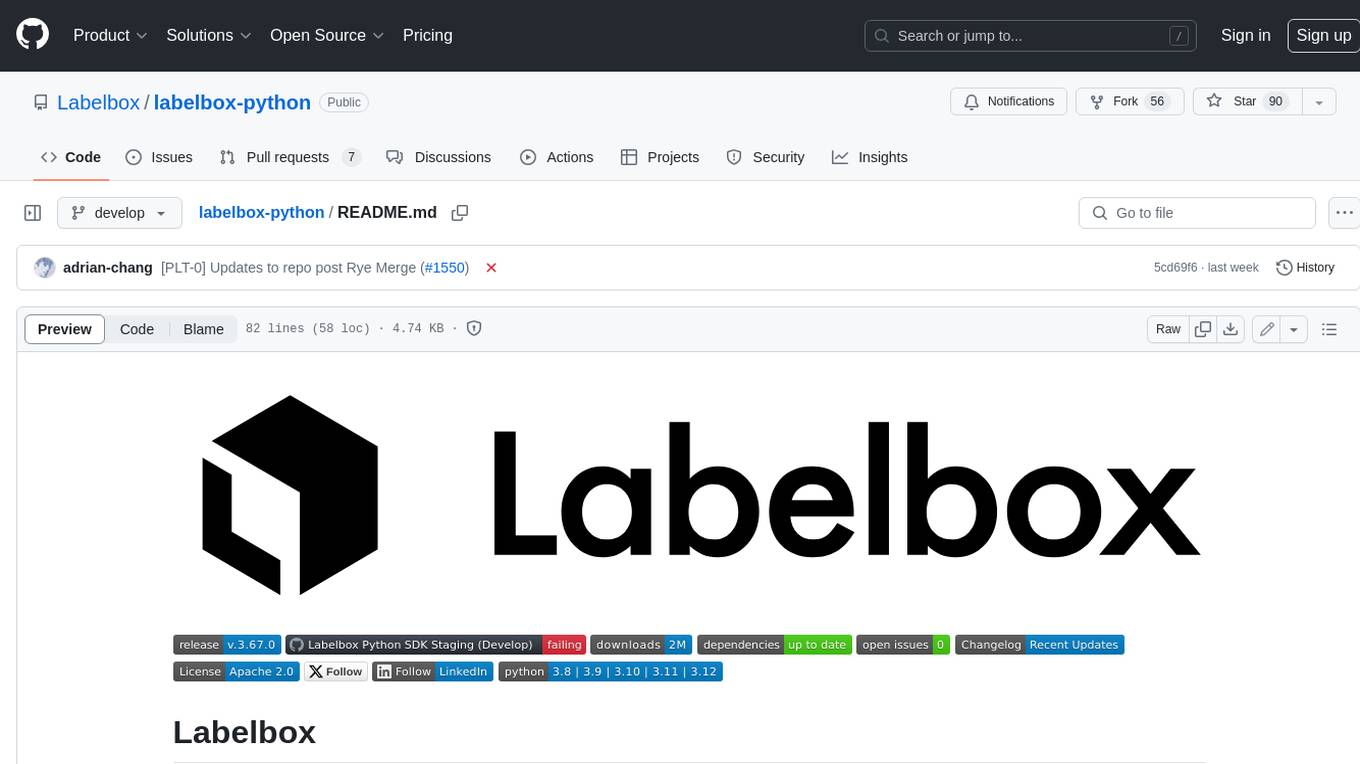
labelbox-python
Labelbox is a data-centric AI platform for enterprises to develop, optimize, and use AI to solve problems and power new products and services. Enterprises use Labelbox to curate data, generate high-quality human feedback data for computer vision and LLMs, evaluate model performance, and automate tasks by combining AI and human-centric workflows. The academic & research community uses Labelbox for cutting-edge AI research.