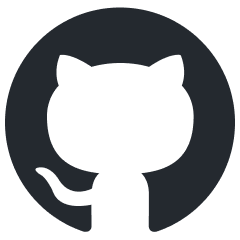
pandas-ai
Chat with your database or your datalake (SQL, CSV, parquet). PandasAI makes data analysis conversational using LLMs and RAG.
Stars: 17885
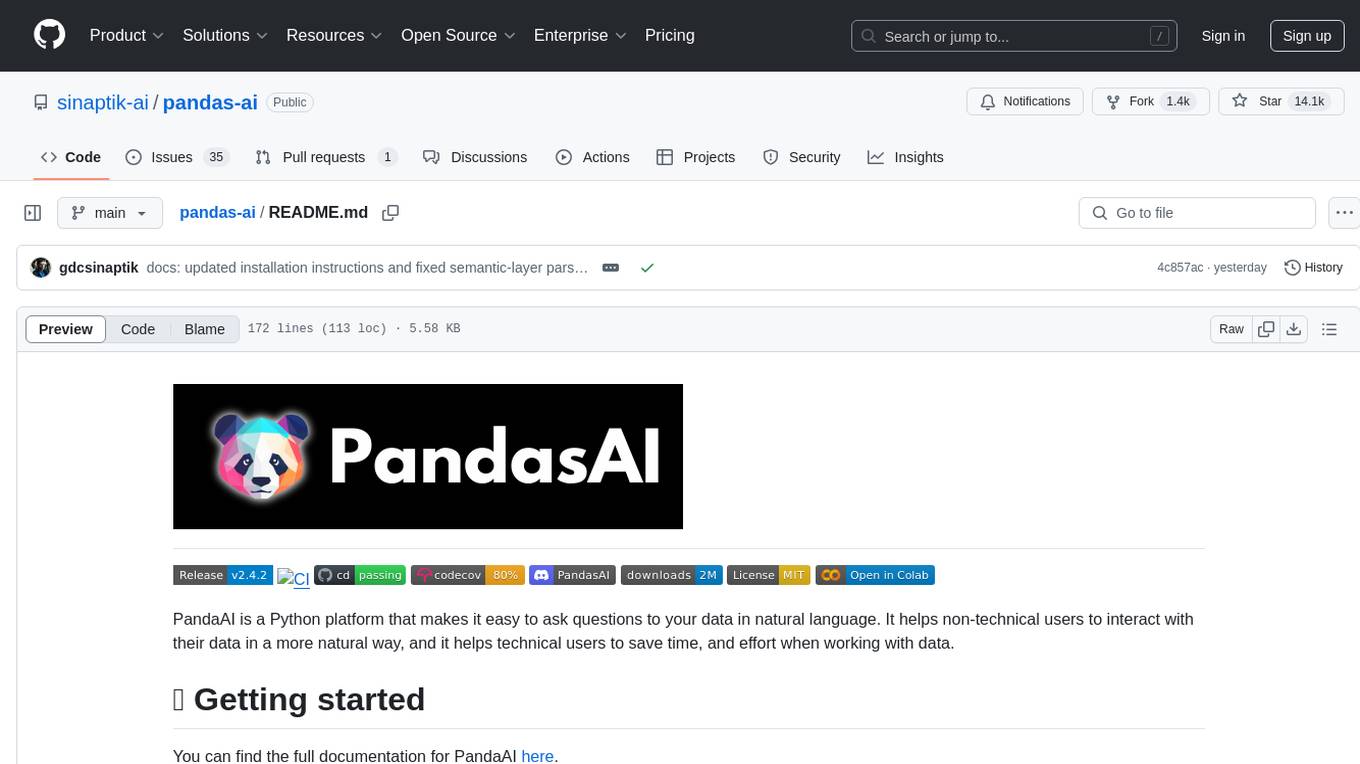
PandaAI is a Python platform that enables users to interact with their data in natural language, catering to both non-technical and technical users. It simplifies data querying and analysis, offering conversational data analytics capabilities with minimal code. Users can ask questions, visualize charts, and compare dataframes effortlessly. The tool aims to streamline data exploration and decision-making processes by providing a user-friendly interface for data manipulation and analysis.
README:
PandaAI is a Python platform that makes it easy to ask questions to your data in natural language. It helps non-technical users to interact with their data in a more natural way, and it helps technical users to save time, and effort when working with data.
You can find the full documentation for PandaAI here.
You can either decide to use PandaAI in your Jupyter notebooks, Streamlit apps, or use the client and server architecture from the repo.
The library can be used alongside our powerful data platform, making end-to-end conversational data analytics possible with as little as a few lines of code.
Load your data, save them as a dataframe, and push them to the platform
import pandasai as pai
pai.api_key.set("your-pai-api-key")
file = pai.read_csv("./filepath.csv")
dataset = pai.create(path="your-organization/dataset-name",
df=file,
name="dataset-name",
description="dataset-description")
dataset.push()
Your team can now access and query this data using natural language through the platform.
Python version 3.8+ <3.12
You can install the PandaAI library using pip or poetry.
With pip:
pip install "pandasai>=3.0.0b2"
With poetry:
poetry add "pandasai>=3.0.0b2"
import pandasai as pai
# Sample DataFrame
df = pai.DataFrame({
"country": ["United States", "United Kingdom", "France", "Germany", "Italy", "Spain", "Canada", "Australia", "Japan", "China"],
"revenue": [5000, 3200, 2900, 4100, 2300, 2100, 2500, 2600, 4500, 7000]
})
# By default, unless you choose a different LLM, it will use BambooLLM.
# You can get your free API key signing up at https://app.pandabi.ai (you can also configure it in your .env file)
pai.api_key.set("your-pai-api-key")
df.chat('Which are the top 5 countries by sales?')
China, United States, Japan, Germany, Australia
Or you can ask more complex questions:
df.chat(
"What is the total sales for the top 3 countries by sales?"
)
The total sales for the top 3 countries by sales is 16500.
You can also ask PandaAI to generate charts for you:
df.chat(
"Plot the histogram of countries showing for each one the gd. Use different colors for each bar",
)
You can also pass in multiple dataframes to PandaAI and ask questions relating them.
import pandasai as pai
employees_data = {
'EmployeeID': [1, 2, 3, 4, 5],
'Name': ['John', 'Emma', 'Liam', 'Olivia', 'William'],
'Department': ['HR', 'Sales', 'IT', 'Marketing', 'Finance']
}
salaries_data = {
'EmployeeID': [1, 2, 3, 4, 5],
'Salary': [5000, 6000, 4500, 7000, 5500]
}
employees_df = pai.DataFrame(employees_data)
salaries_df = pai.DataFrame(salaries_data)
# By default, unless you choose a different LLM, it will use BambooLLM.
# You can get your free API key signing up at https://app.pandabi.ai (you can also configure it in your .env file)
pai.api_key.set("your-pai-api-key")
pai.chat("Who gets paid the most?", employees_df, salaries_df)
Olivia gets paid the most.
You can run PandaAI in a Docker sandbox, providing a secure, isolated environment to execute code safely and mitigate the risk of malicious attacks.
pip install "pandasai-docker"
import pandasai as pai
from pandasai_docker import DockerSandbox
# Initialize the sandbox
sandbox = DockerSandbox()
sandbox.start()
employees_data = {
'EmployeeID': [1, 2, 3, 4, 5],
'Name': ['John', 'Emma', 'Liam', 'Olivia', 'William'],
'Department': ['HR', 'Sales', 'IT', 'Marketing', 'Finance']
}
salaries_data = {
'EmployeeID': [1, 2, 3, 4, 5],
'Salary': [5000, 6000, 4500, 7000, 5500]
}
employees_df = pai.DataFrame(employees_data)
salaries_df = pai.DataFrame(salaries_data)
# By default, unless you choose a different LLM, it will use BambooLLM.
# You can get your free API key signing up at https://app.pandabi.ai (you can also configure it in your .env file)
pai.api_key.set("your-pai-api-key")
pai.chat("Who gets paid the most?", employees_df, salaries_df, sandbox=sandbox)
# Don't forget to stop the sandbox when done
sandbox.stop()
Olivia gets paid the most.
You can find more examples in the examples directory.
PandaAI is available under the MIT expat license, except for the pandasai/ee
directory of this repository, which has its license here.
If you are interested in managed PandaAI Cloud or self-hosted Enterprise Offering, contact us.
Beta Notice
Release v3 is currently in beta. The following documentation and examples reflect the features and functionality in progress and may change before the final release.
- Docs for comprehensive documentation
- Examples for example notebooks
- Discord for discussion with the community and PandaAI team
Contributions are welcome! Please check the outstanding issues and feel free to open a pull request. For more information, please check out the contributing guidelines.
For Tasks:
Click tags to check more tools for each tasksFor Jobs:
Alternative AI tools for pandas-ai
Similar Open Source Tools
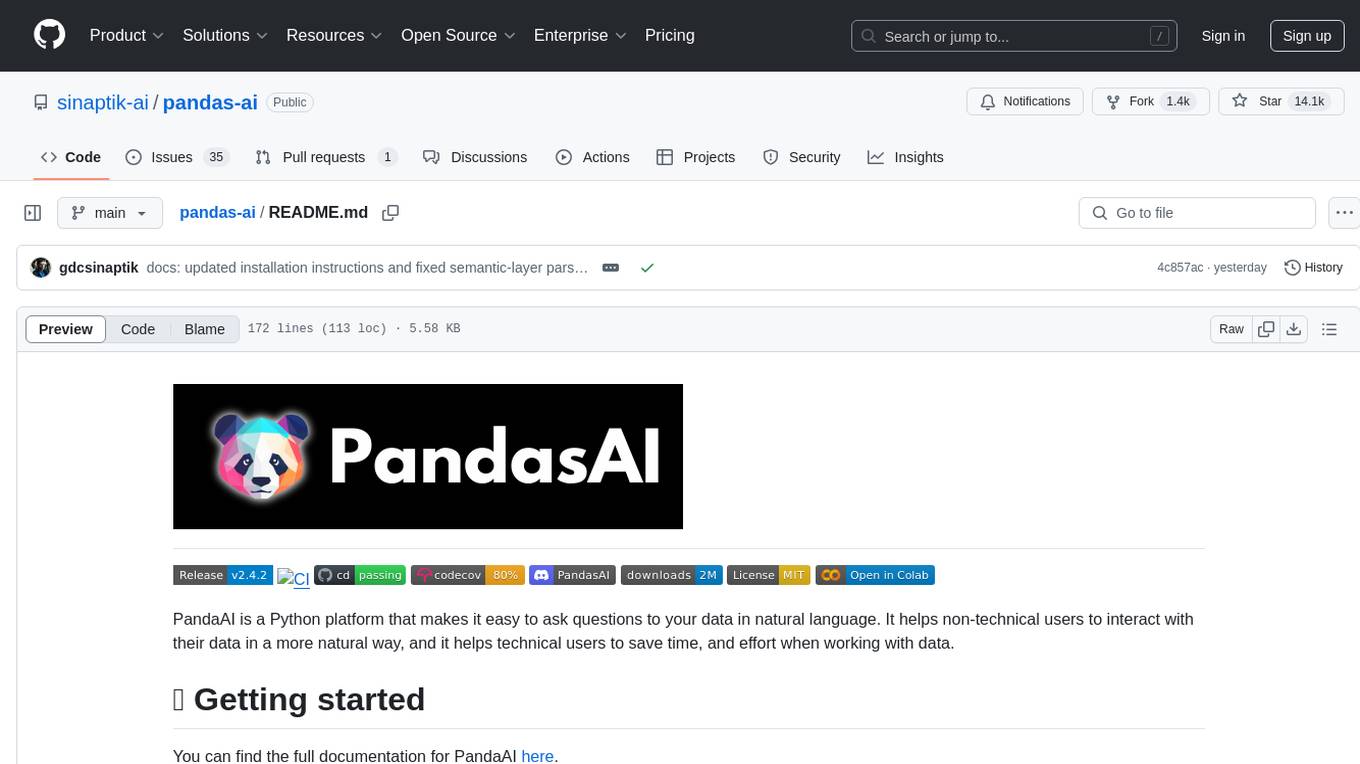
pandas-ai
PandaAI is a Python platform that enables users to interact with their data in natural language, catering to both non-technical and technical users. It simplifies data querying and analysis, offering conversational data analytics capabilities with minimal code. Users can ask questions, visualize charts, and compare dataframes effortlessly. The tool aims to streamline data exploration and decision-making processes by providing a user-friendly interface for data manipulation and analysis.
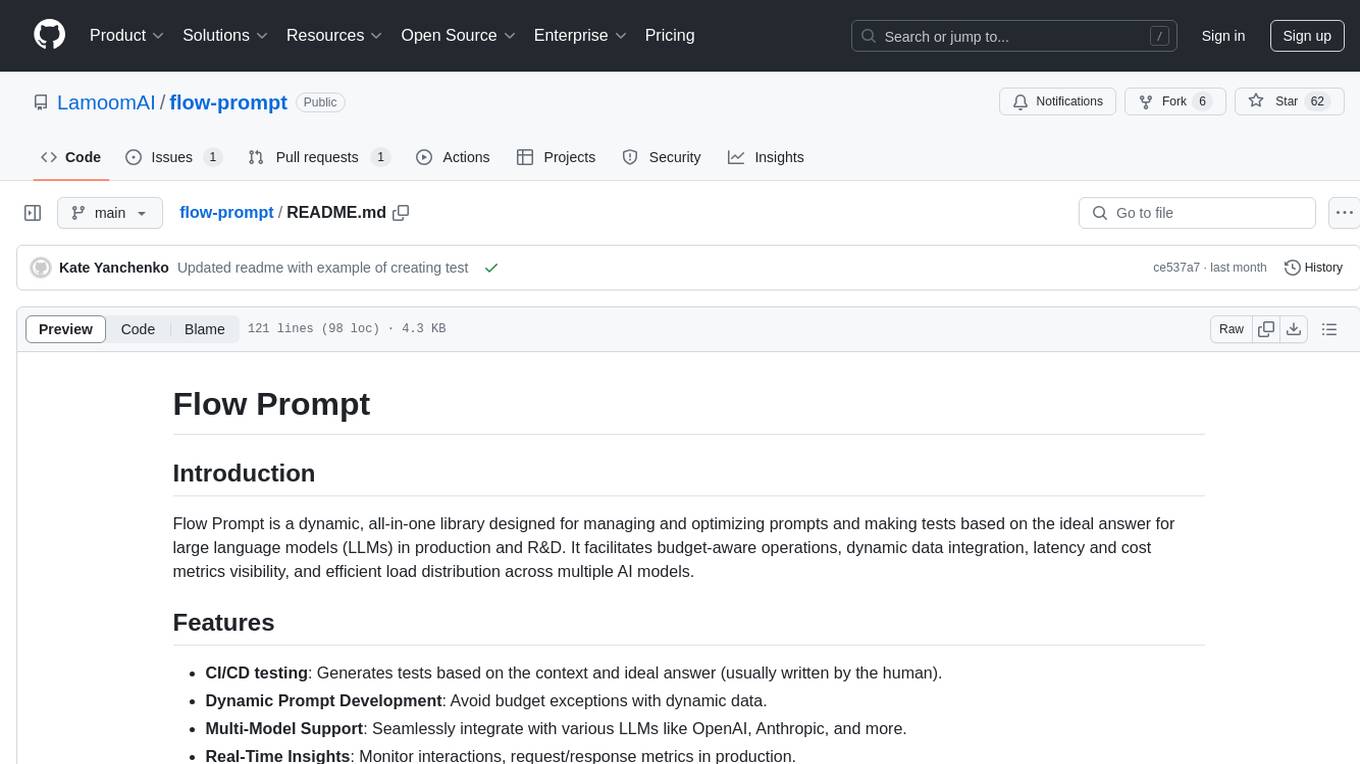
flow-prompt
Flow Prompt is a dynamic library for managing and optimizing prompts for large language models. It facilitates budget-aware operations, dynamic data integration, and efficient load distribution. Features include CI/CD testing, dynamic prompt development, multi-model support, real-time insights, and prompt testing and evolution.
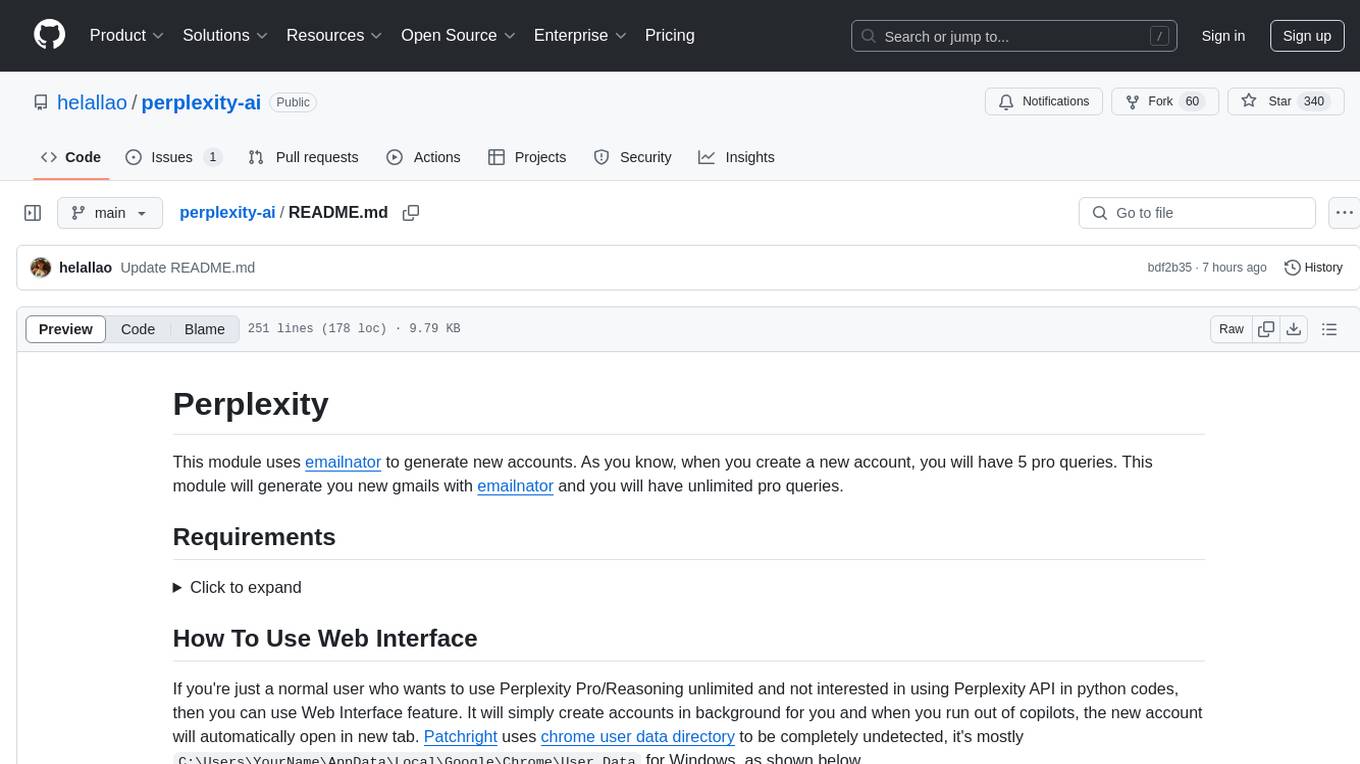
perplexity-ai
Perplexity is a module that utilizes emailnator to generate new accounts, providing users with 5 pro queries per account creation. It enables the creation of new Gmail accounts with emailnator, ensuring unlimited pro queries. The tool requires specific Python libraries for installation and offers both a web interface and an API for different usage scenarios. Users can interact with the tool to perform various tasks such as account creation, query searches, and utilizing different modes for research purposes. Perplexity also supports asynchronous operations and provides guidance on obtaining cookies for account usage and account generation from emailnator.
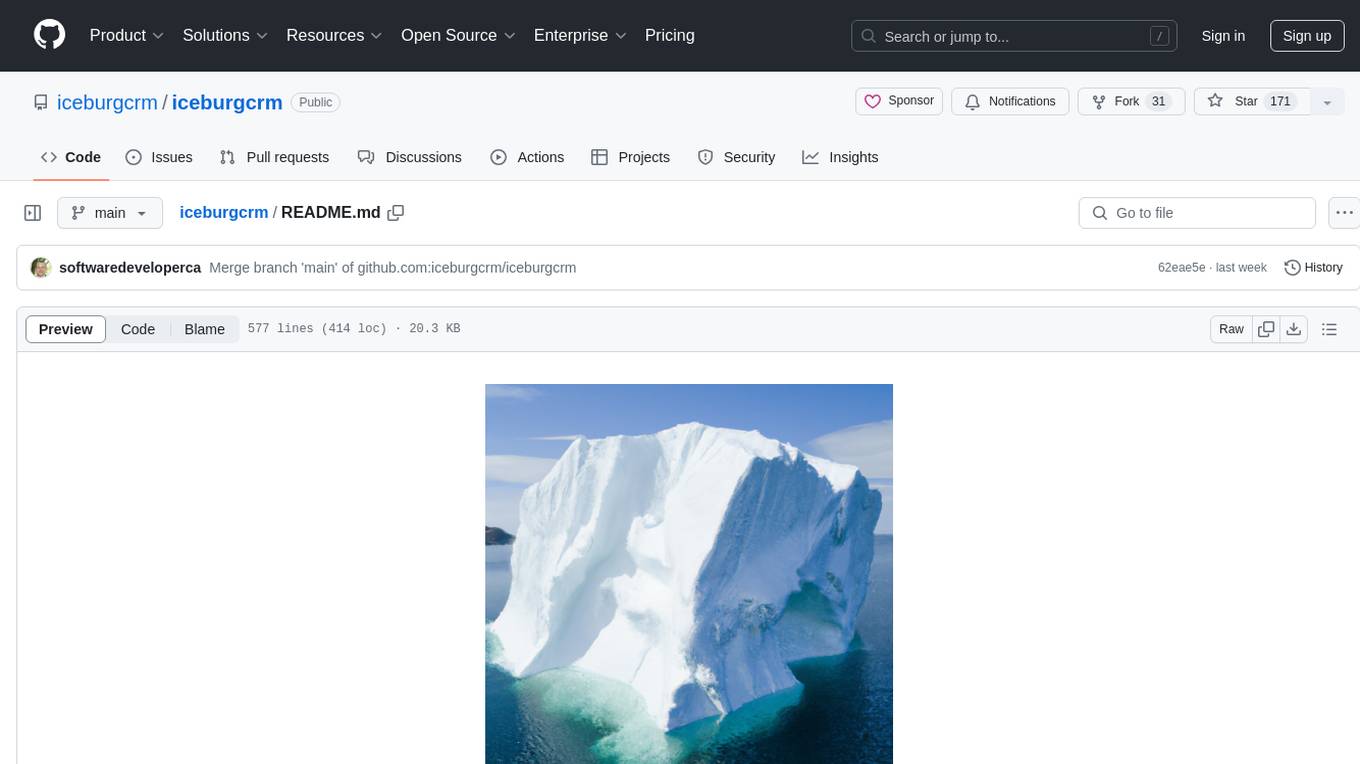
iceburgcrm
Iceburg CRM is a metadata driven CRM with AI abilities that allows users to quickly prototype any CRM. It offers features like metadata creations, import/export in multiple formats, field validation, themes, role permissions, calendar, audit logs, API, workflow, field level relationships, module level relationships, and more. Created with Vue 3 for the frontend, Laravel 10 for the backend, Tailwinds with DaisyUI plugin, and Inertia for routing. Users can install default, admin panel, core, custom, or AI versions. The tool supports AI Assist for module data suggestions and provides API endpoints for CRM modules, search, specific module data, record updates, and deletions. Iceburg CRM also includes themes, custom field types, calendar, datalets, workflow, roles and permissions, import/export functionality, and custom seeding options.
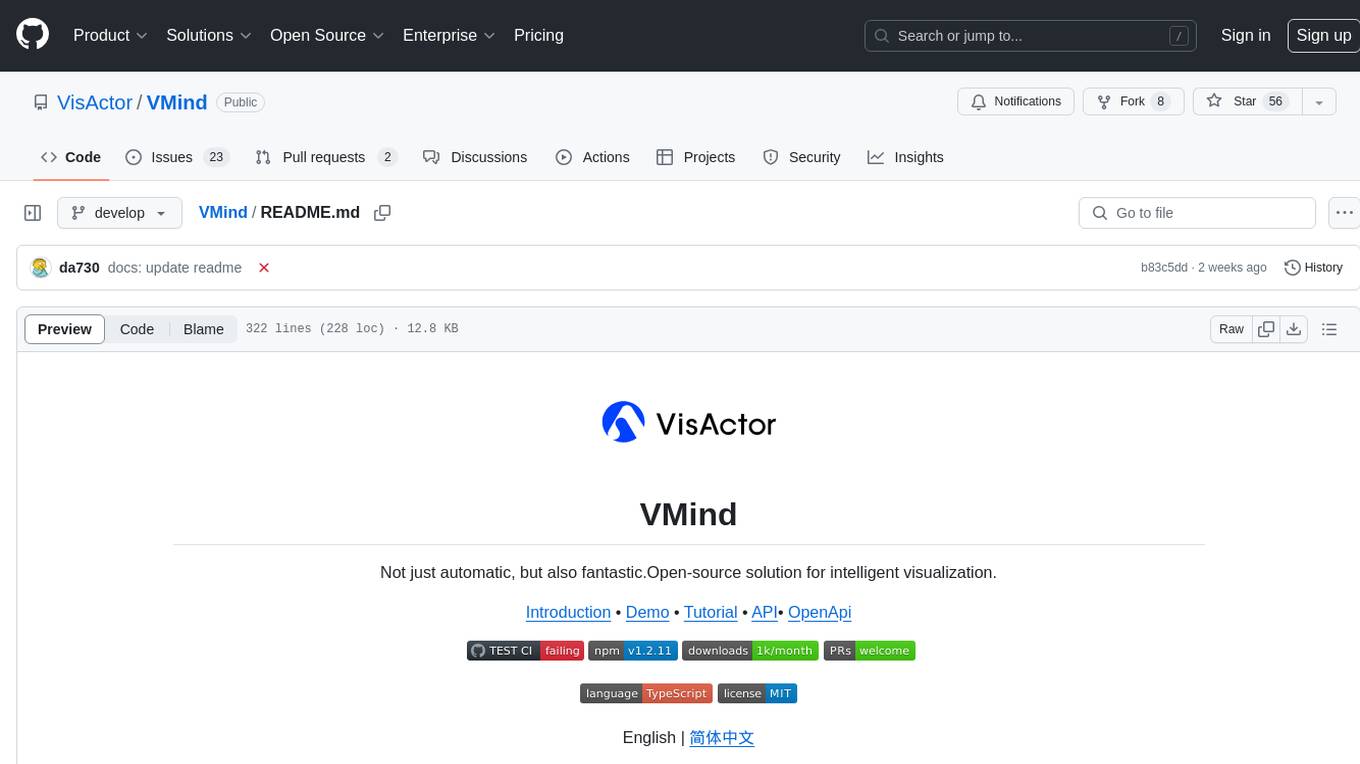
VMind
VMind is an open-source solution for intelligent visualization, providing an intelligent chart component based on LLM by VisActor. It allows users to create chart narrative works with natural language interaction, edit charts through dialogue, and export narratives as videos or GIFs. The tool is easy to use, scalable, supports various chart types, and offers one-click export functionality. Users can customize chart styles, specify themes, and aggregate data using LLM models. VMind aims to enhance efficiency in creating data visualization works through dialogue-based editing and natural language interaction.
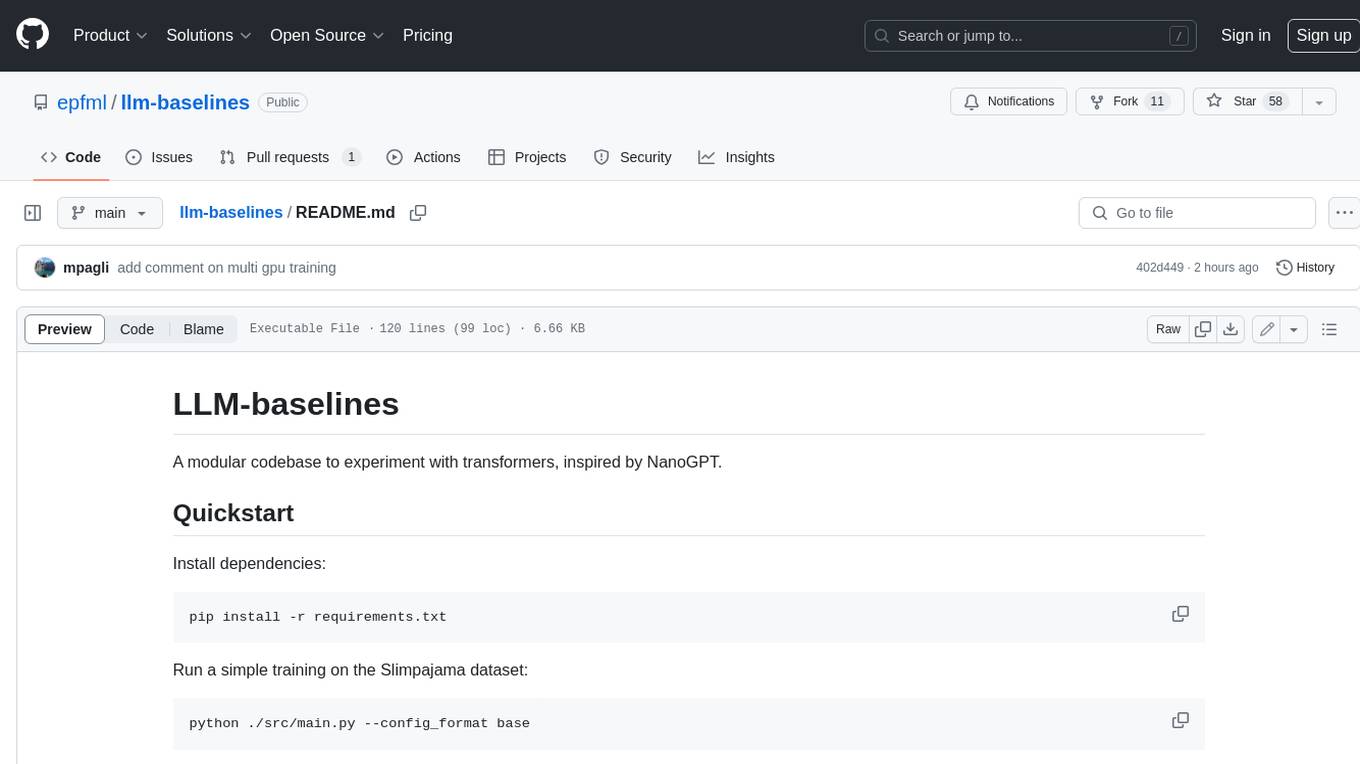
llm-baselines
LLM-baselines is a modular codebase to experiment with transformers, inspired from NanoGPT. It provides a quick and easy way to train and evaluate transformer models on a variety of datasets. The codebase is well-documented and easy to use, making it a great resource for researchers and practitioners alike.
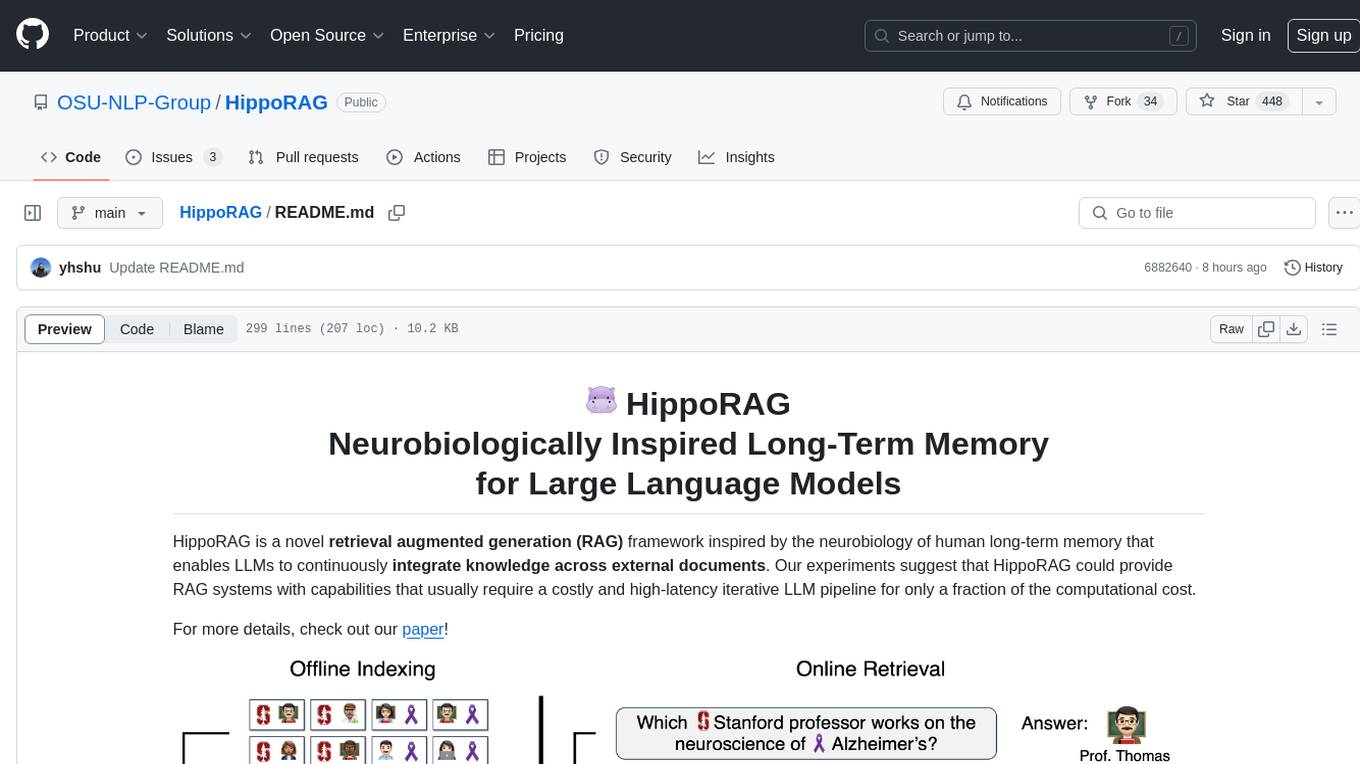
HippoRAG
HippoRAG is a novel retrieval augmented generation (RAG) framework inspired by the neurobiology of human long-term memory that enables Large Language Models (LLMs) to continuously integrate knowledge across external documents. It provides RAG systems with capabilities that usually require a costly and high-latency iterative LLM pipeline for only a fraction of the computational cost. The tool facilitates setting up retrieval corpus, indexing, and retrieval processes for LLMs, offering flexibility in choosing different online LLM APIs or offline LLM deployments through LangChain integration. Users can run retrieval on pre-defined queries or integrate directly with the HippoRAG API. The tool also supports reproducibility of experiments and provides data, baselines, and hyperparameter tuning scripts for research purposes.
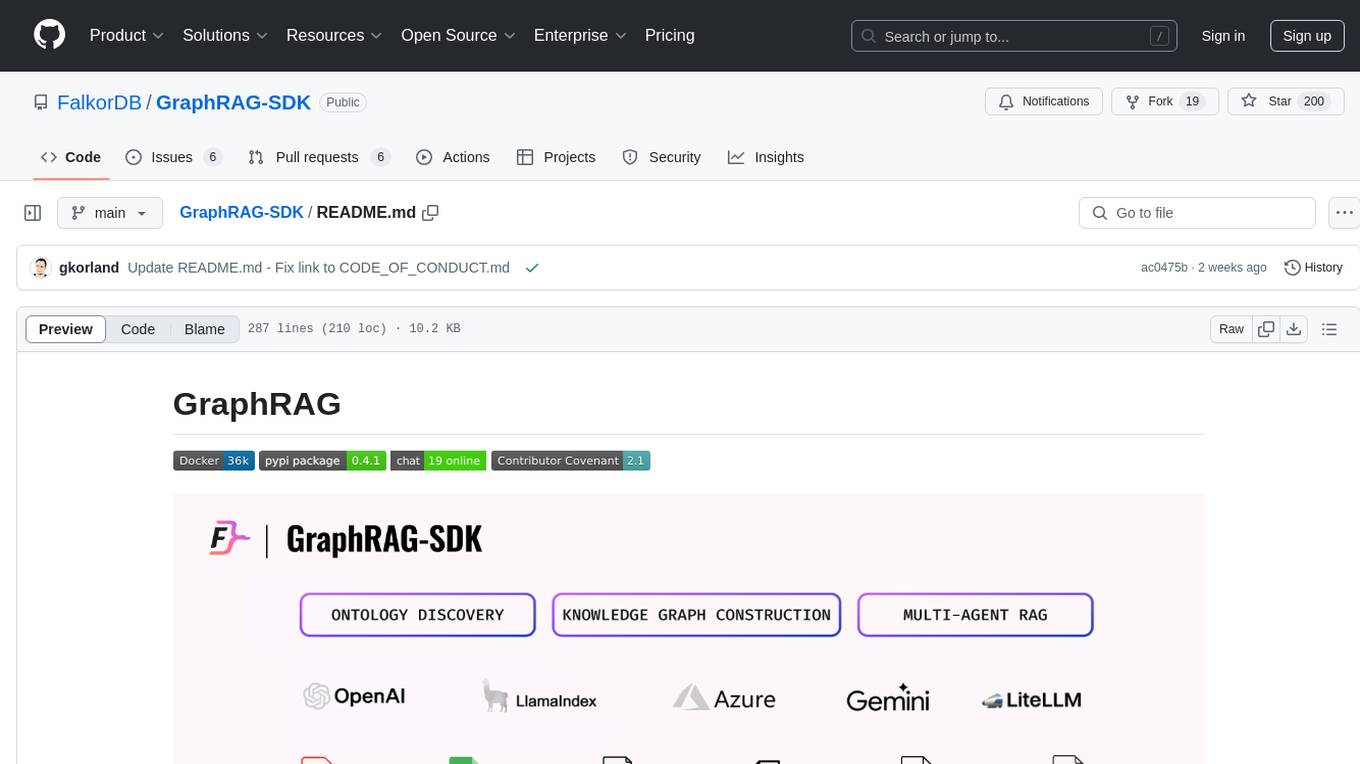
GraphRAG-SDK
Build fast and accurate GenAI applications with GraphRAG SDK, a specialized toolkit for building Graph Retrieval-Augmented Generation (GraphRAG) systems. It integrates knowledge graphs, ontology management, and state-of-the-art LLMs to deliver accurate, efficient, and customizable RAG workflows. The SDK simplifies the development process by automating ontology creation, knowledge graph agent creation, and query handling, enabling users to interact and query their knowledge graphs effectively. It supports multi-agent systems and orchestrates agents specialized in different domains. The SDK is optimized for FalkorDB, ensuring high performance and scalability for large-scale applications. By leveraging knowledge graphs, it enables semantic relationships and ontology-driven queries that go beyond standard vector similarity, enhancing retrieval-augmented generation capabilities.
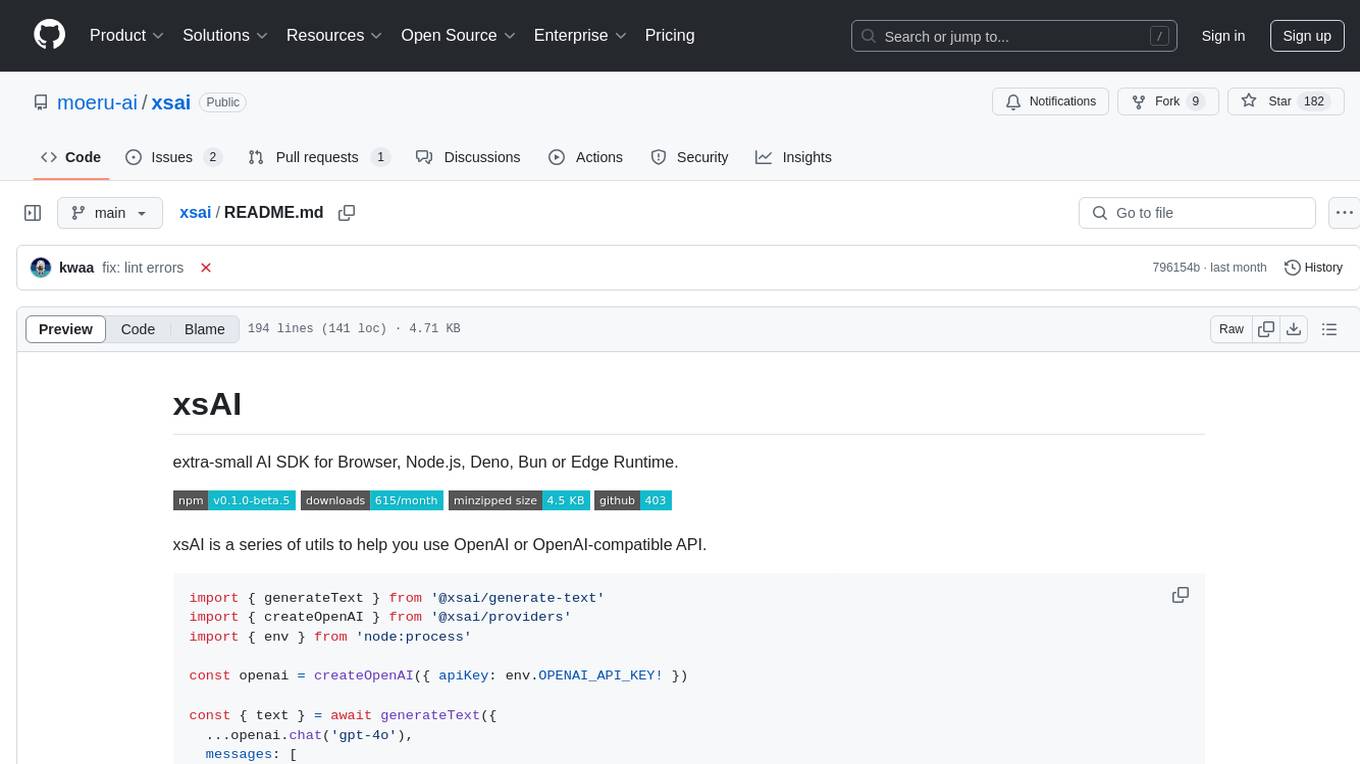
xsai
xsAI is an extra-small AI SDK designed for Browser, Node.js, Deno, Bun, or Edge Runtime. It provides a series of utils to help users utilize OpenAI or OpenAI-compatible APIs. The SDK is lightweight and efficient, using a variety of methods to minimize its size. It is runtime-agnostic, working seamlessly across different environments without depending on Node.js Built-in Modules. Users can easily install specific utils like generateText or streamText, and leverage tools like weather to perform tasks such as getting the weather in a location.
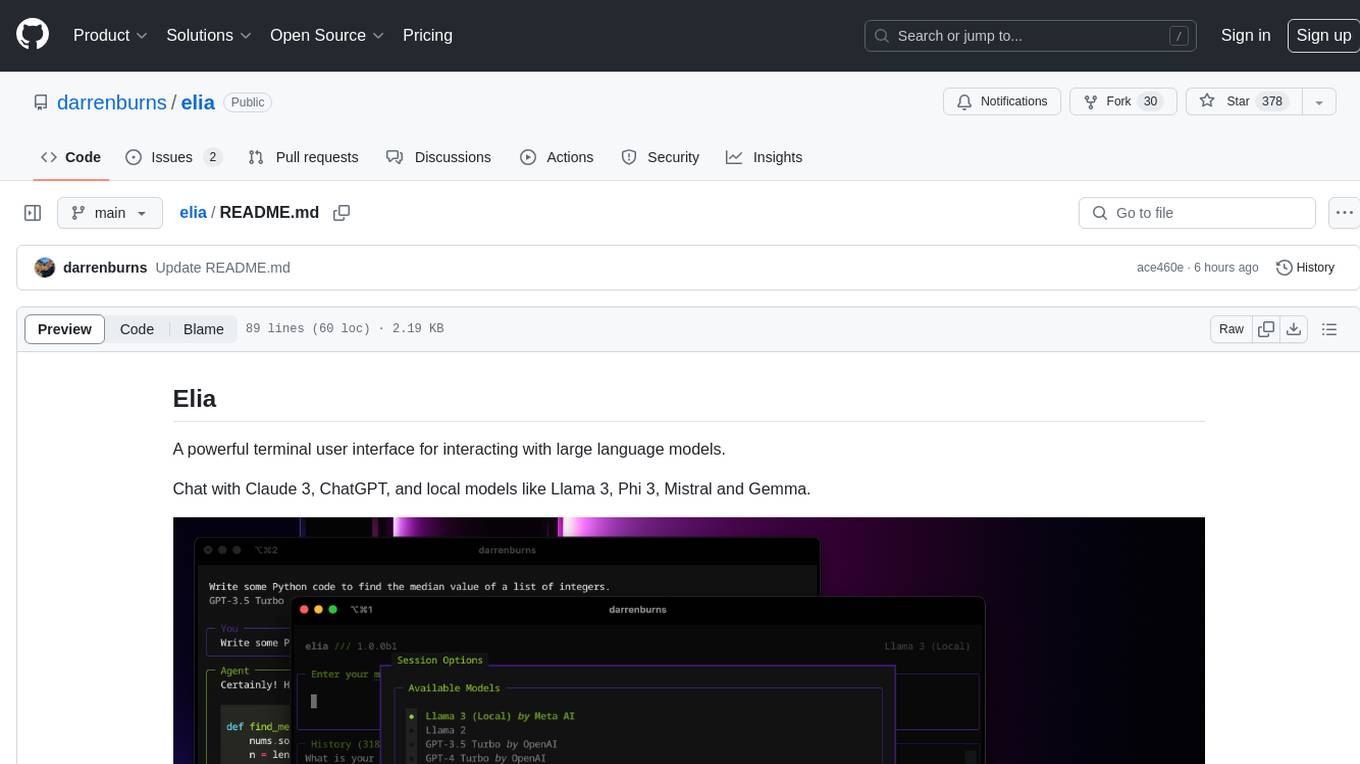
elia
Elia is a powerful terminal user interface designed for interacting with large language models. It allows users to chat with models like Claude 3, ChatGPT, Llama 3, Phi 3, Mistral, and Gemma. Conversations are stored locally in a SQLite database, ensuring privacy. Users can run local models through 'ollama' without data leaving their machine. Elia offers easy installation with pipx and supports various environment variables for different models. It provides a quick start to launch chats and manage local models. Configuration options are available to customize default models, system prompts, and add new models. Users can import conversations from ChatGPT and wipe the database when needed. Elia aims to enhance user experience in interacting with language models through a user-friendly interface.
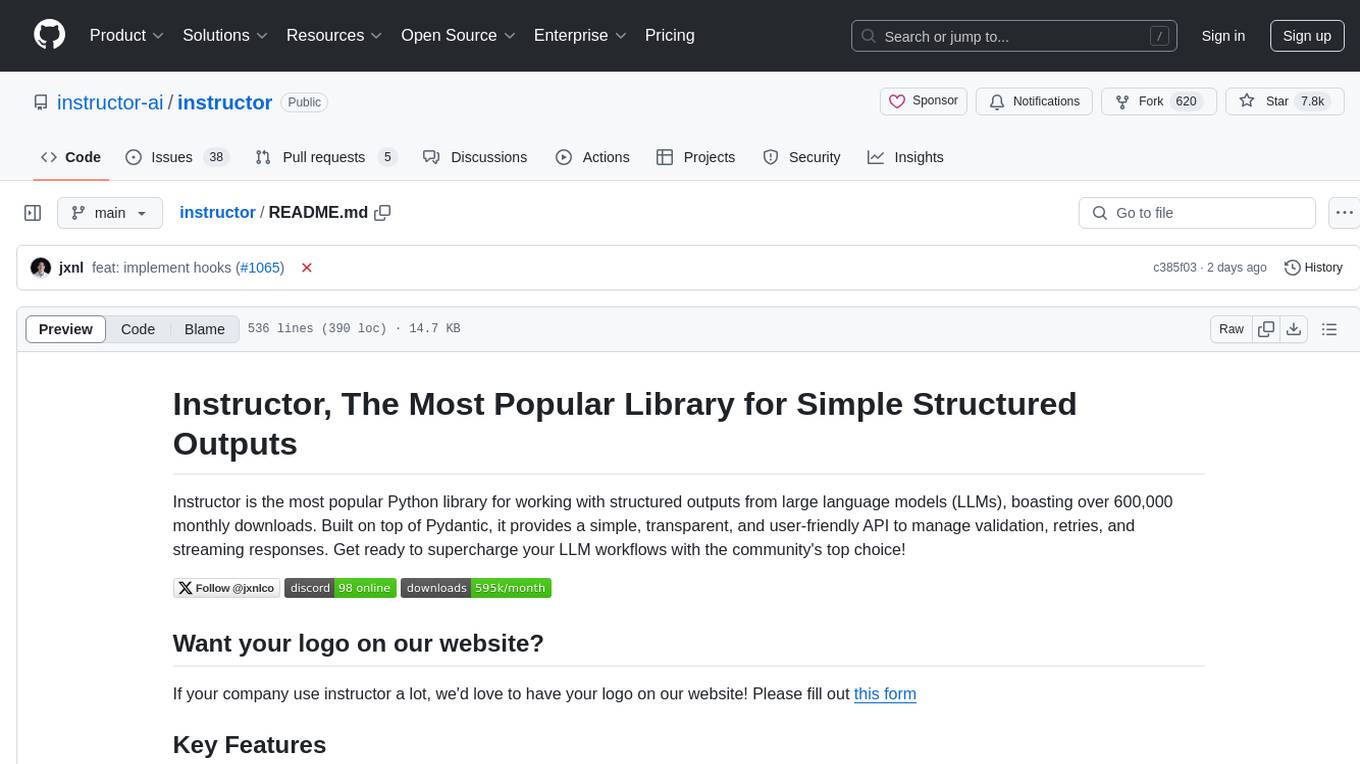
instructor
Instructor is a popular Python library for managing structured outputs from large language models (LLMs). It offers a user-friendly API for validation, retries, and streaming responses. With support for various LLM providers and multiple languages, Instructor simplifies working with LLM outputs. The library includes features like response models, retry management, validation, streaming support, and flexible backends. It also provides hooks for logging and monitoring LLM interactions, and supports integration with Anthropic, Cohere, Gemini, Litellm, and Google AI models. Instructor facilitates tasks such as extracting user data from natural language, creating fine-tuned models, managing uploaded files, and monitoring usage of OpenAI models.
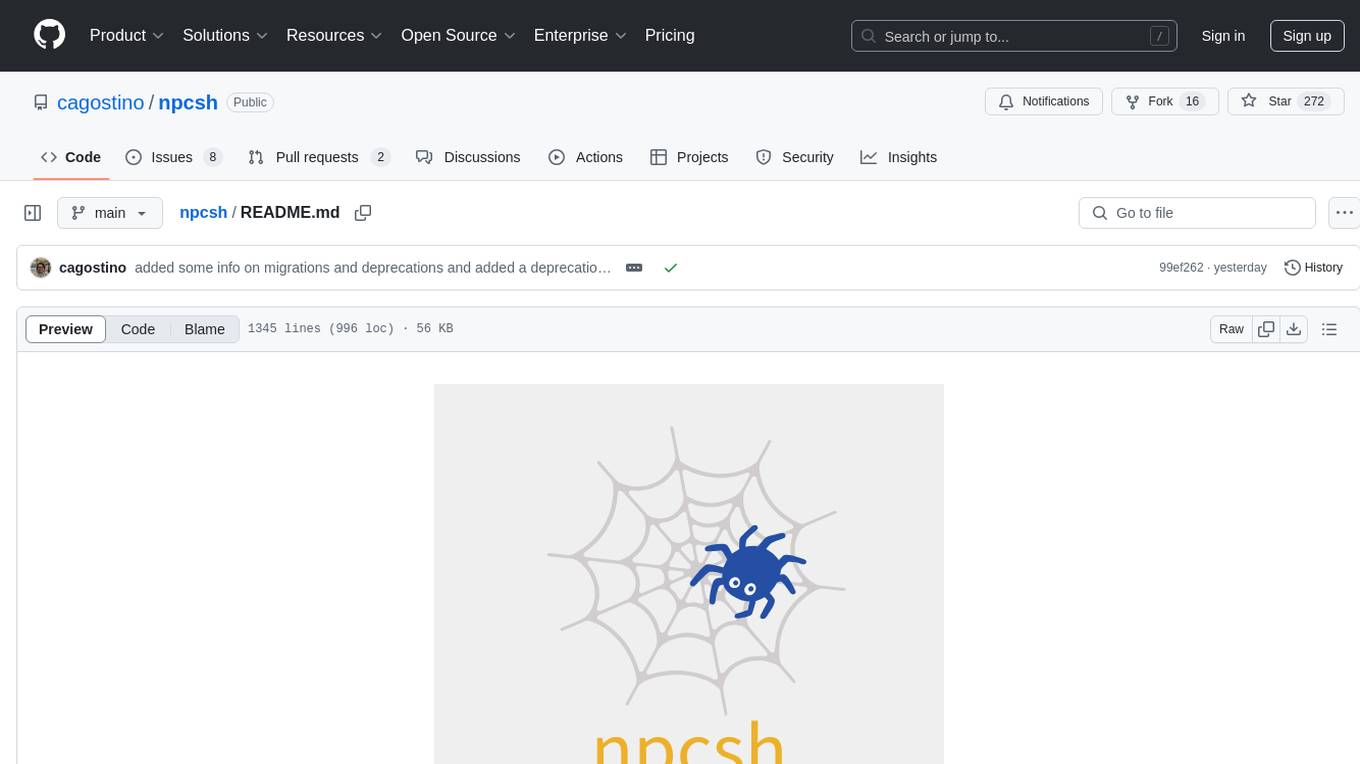
npcsh
`npcsh` is a python-based command-line tool designed to integrate Large Language Models (LLMs) and Agents into one's daily workflow by making them available and easily configurable through the command line shell. It leverages the power of LLMs to understand natural language commands and questions, execute tasks, answer queries, and provide relevant information from local files and the web. Users can also build their own tools and call them like macros from the shell. `npcsh` allows users to take advantage of agents (i.e. NPCs) through a managed system, tailoring NPCs to specific tasks and workflows. The tool is extensible with Python, providing useful functions for interacting with LLMs, including explicit coverage for popular providers like ollama, anthropic, openai, gemini, deepseek, and openai-like providers. Users can set up a flask server to expose their NPC team for use as a backend service, run SQL models defined in their project, execute assembly lines, and verify the integrity of their NPC team's interrelations. Users can execute bash commands directly, use favorite command-line tools like VIM, Emacs, ipython, sqlite3, git, pipe the output of these commands to LLMs, or pass LLM results to bash commands.
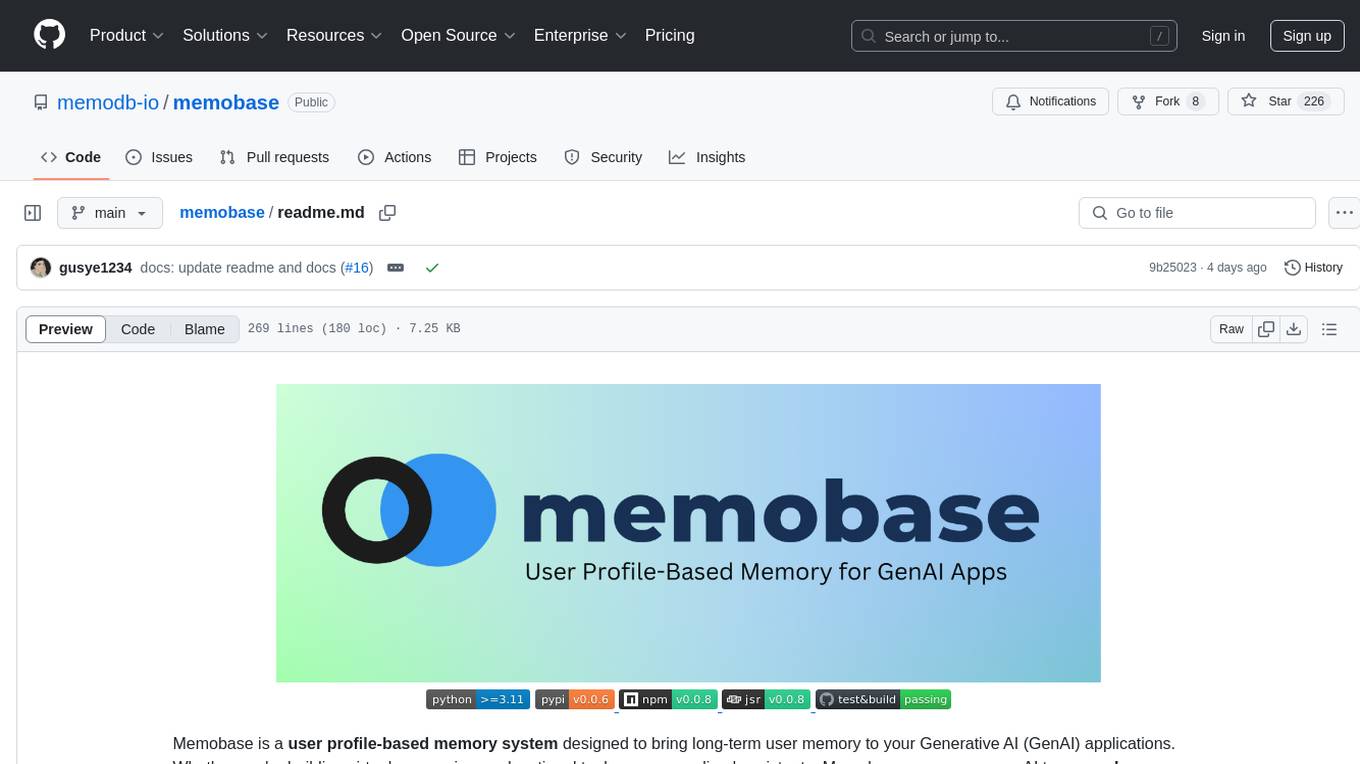
memobase
Memobase is a user profile-based memory system designed to enhance Generative AI applications by enabling them to remember, understand, and evolve with users. It provides structured user profiles, scalable profiling, easy integration with existing LLM stacks, batch processing for speed, and is production-ready. Users can manage users, insert data, get memory profiles, and track user preferences and behaviors. Memobase is ideal for applications that require user analysis, tracking, and personalized interactions.
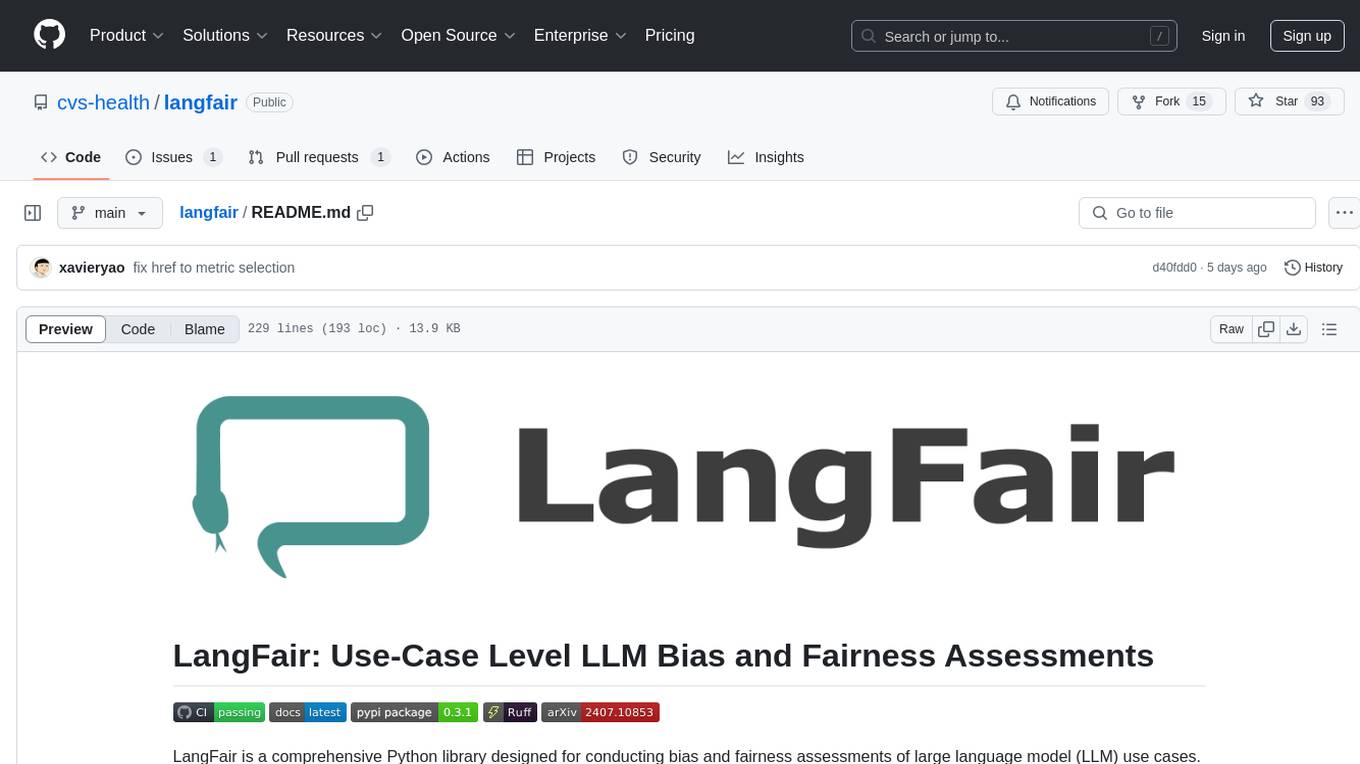
langfair
LangFair is a Python library for bias and fairness assessments of large language models (LLMs). It offers a comprehensive framework for choosing bias and fairness metrics, demo notebooks, and a technical playbook. Users can tailor evaluations to their use cases with a Bring Your Own Prompts approach. The focus is on output-based metrics practical for governance audits and real-world testing.
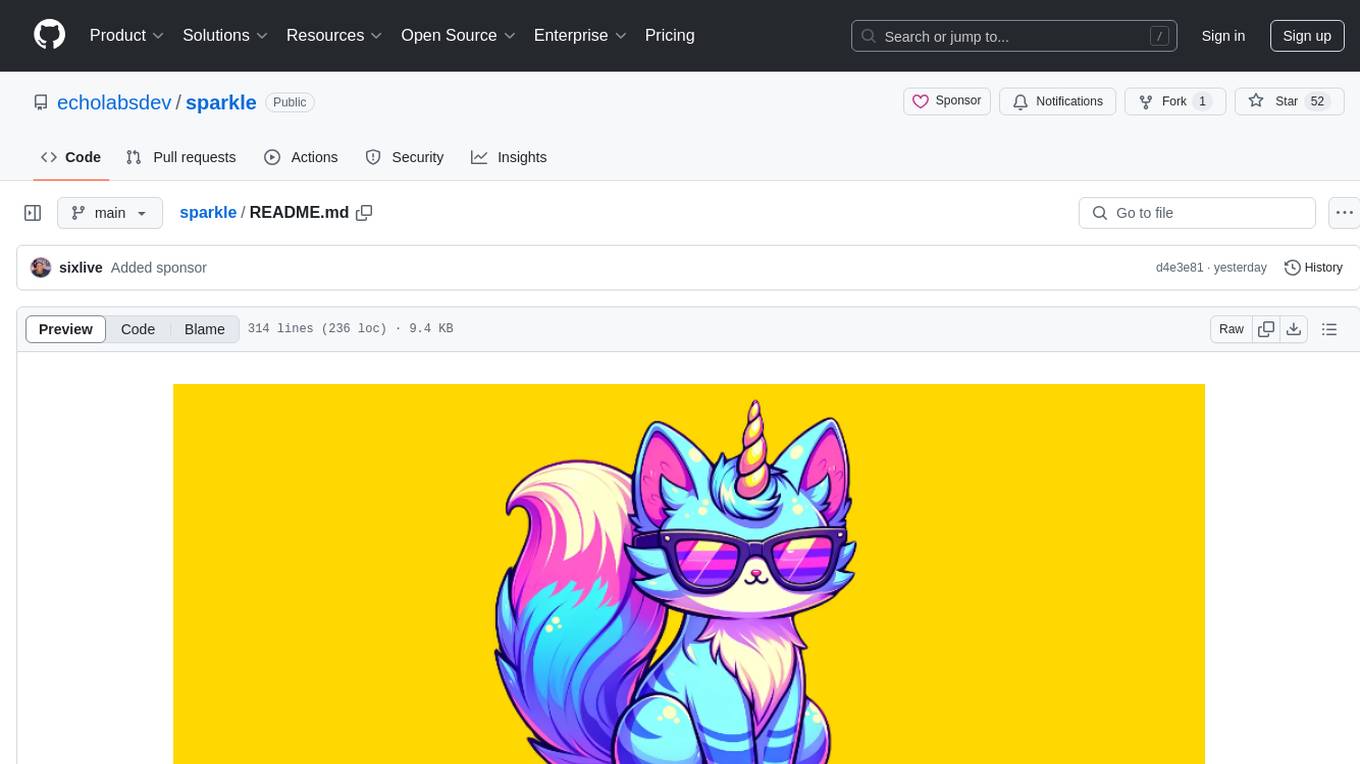
sparkle
Sparkle is a tool that streamlines the process of building AI-driven features in applications using Large Language Models (LLMs). It guides users through creating and managing agents, defining tools, and interacting with LLM providers like OpenAI. Sparkle allows customization of LLM provider settings, model configurations, and provides a seamless integration with Sparkle Server for exposing agents via an OpenAI-compatible chat API endpoint.
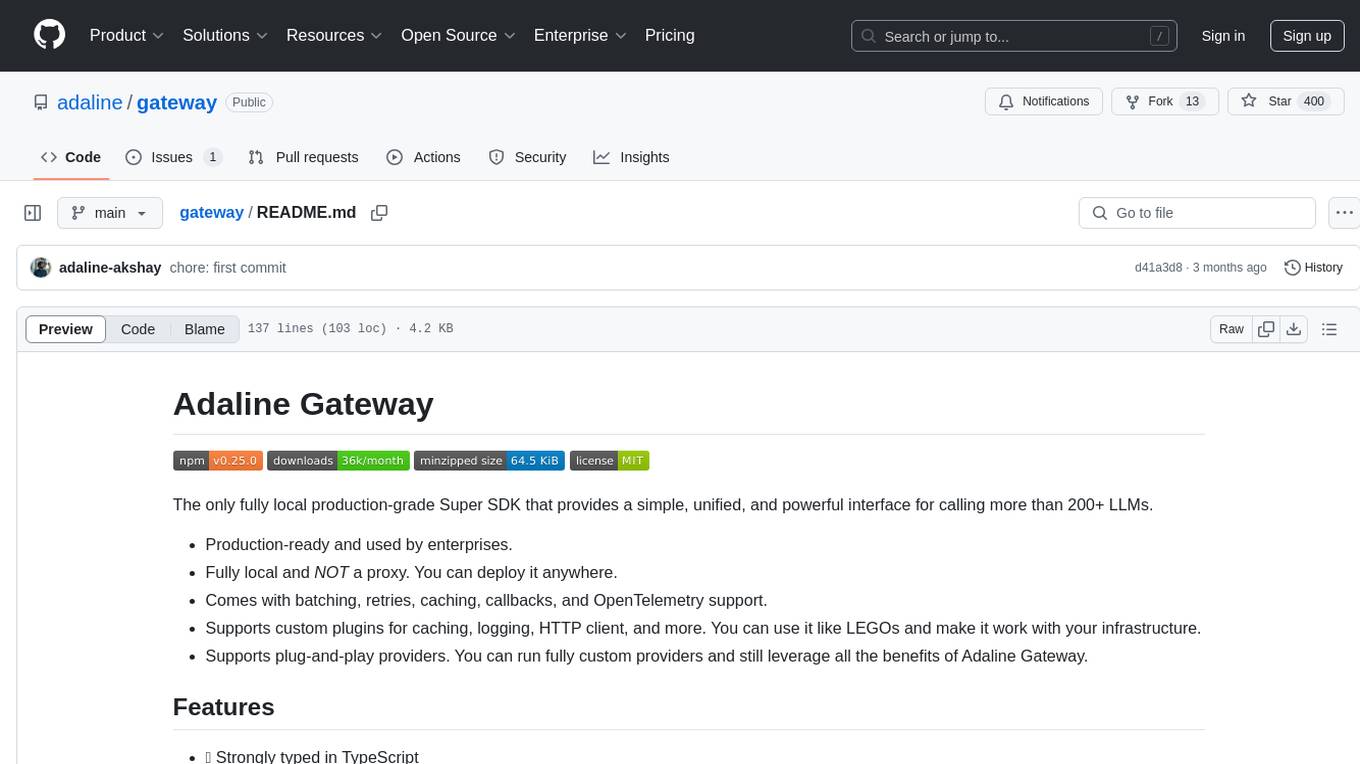
gateway
Adaline Gateway is a fully local production-grade Super SDK that offers a unified interface for calling over 200+ LLMs. It is production-ready, supports batching, retries, caching, callbacks, and OpenTelemetry. Users can create custom plugins and providers for seamless integration with their infrastructure.
For similar tasks
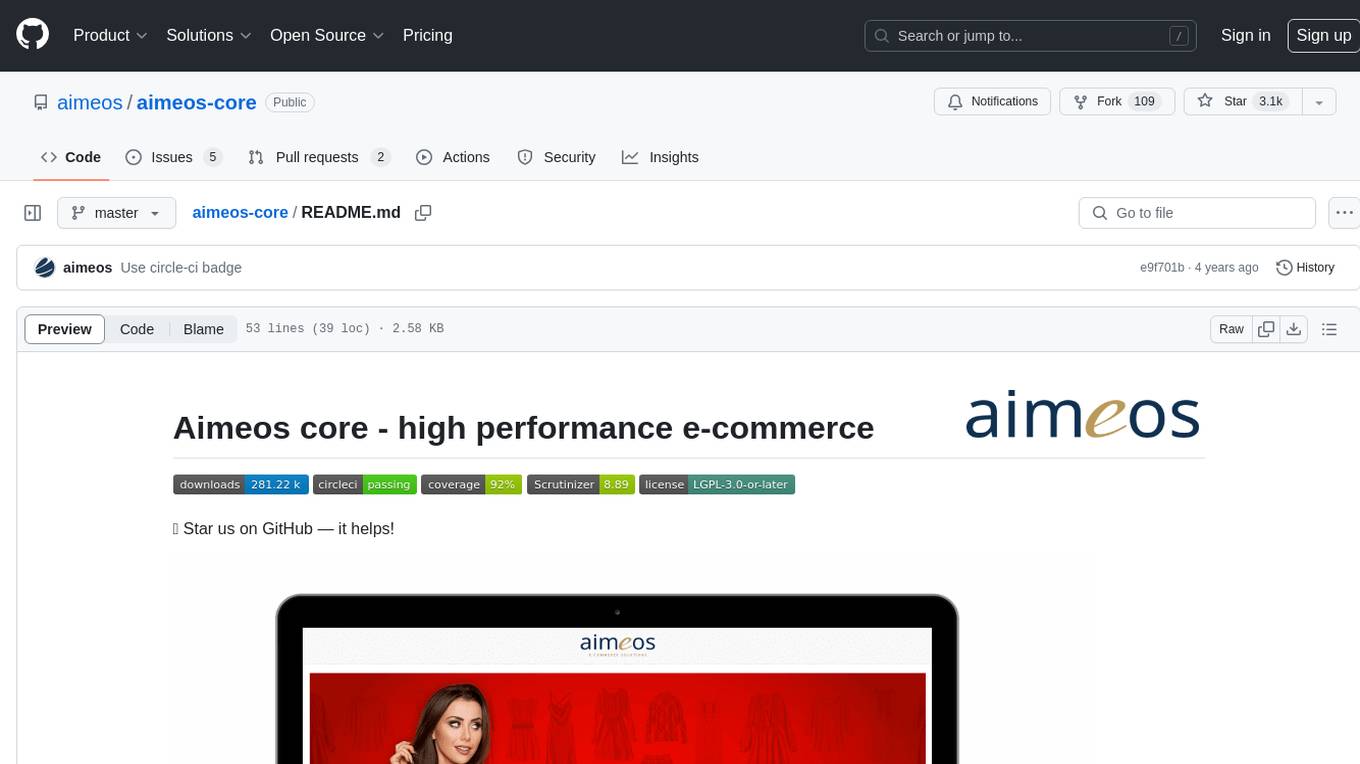
aimeos-core
Aimeos is an Open Source e-commerce framework for online shops consisting of the e-commerce library, the administration interface and different front-ends. It offers a modular stack that provides flexibility and speed. Unlike other shop systems, Aimeos allows users to choose from several user front-ends and customize them according to their needs or create their own. It is suitable for medium to large businesses requiring seamless integration into existing systems like content management, customer relationship management, or enterprise resource planning systems. Aimeos also serves as a base for portals or marketplaces.
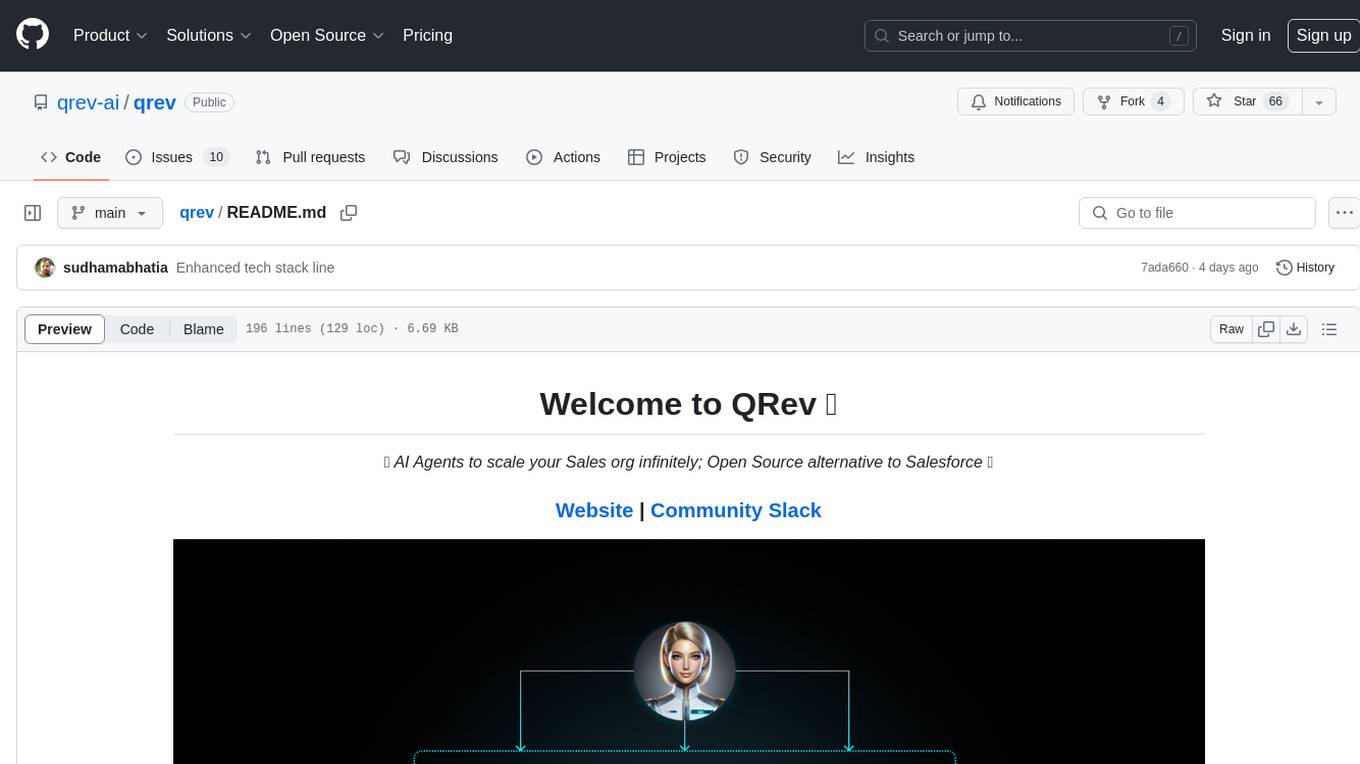
qrev
QRev is an open-source alternative to Salesforce, offering AI agents to scale sales organizations infinitely. It aims to provide digital workers for various sales roles or a superagent named Qai. The tech stack includes TypeScript for frontend, NodeJS for backend, MongoDB for app server database, ChromaDB for vector database, SQLite for AI server SQL relational database, and Langchain for LLM tooling. The tool allows users to run client app, app server, and AI server components. It requires Node.js and MongoDB to be installed, and provides detailed setup instructions in the README file.
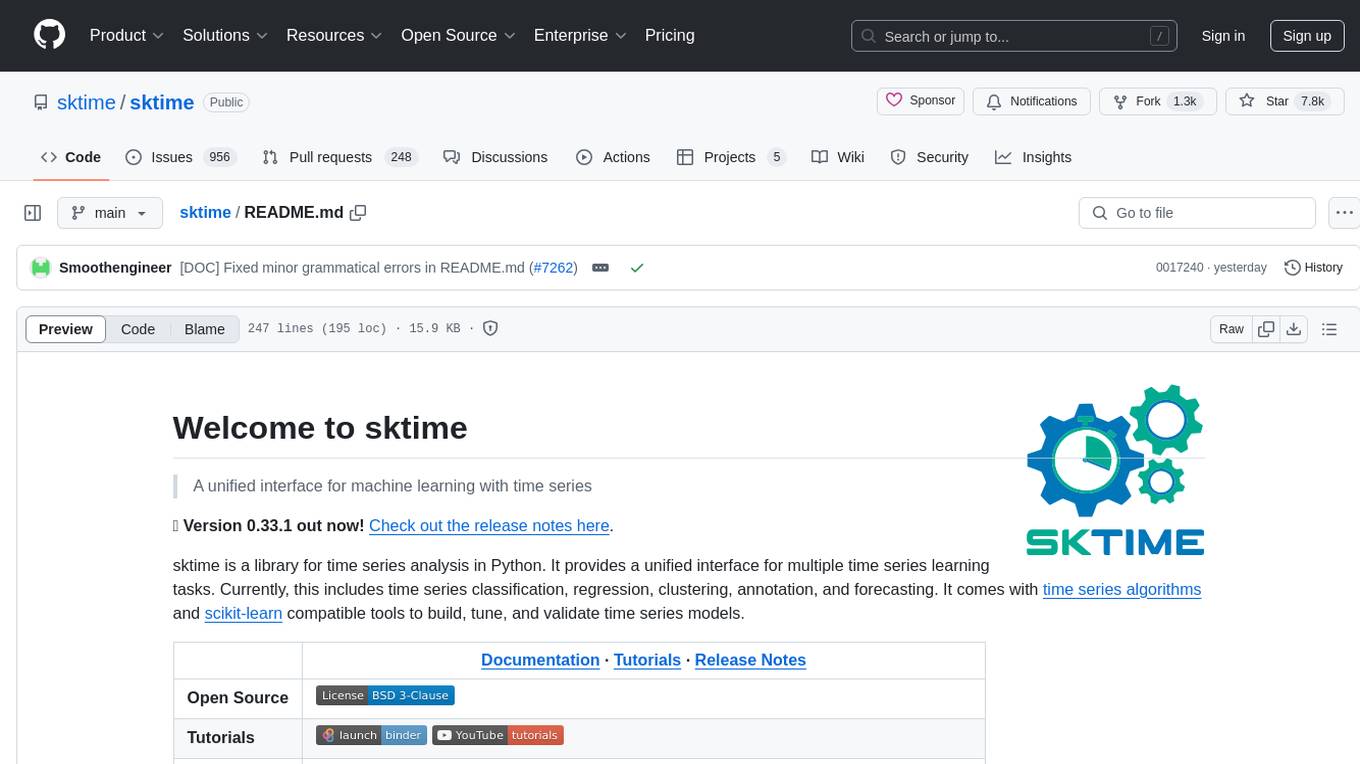
sktime
sktime is a Python library for time series analysis that provides a unified interface for various time series learning tasks such as classification, regression, clustering, annotation, and forecasting. It offers time series algorithms and tools compatible with scikit-learn for building, tuning, and validating time series models. sktime aims to enhance the interoperability and usability of the time series analysis ecosystem by empowering users to apply algorithms across different tasks and providing interfaces to related libraries like scikit-learn, statsmodels, tsfresh, PyOD, and fbprophet.
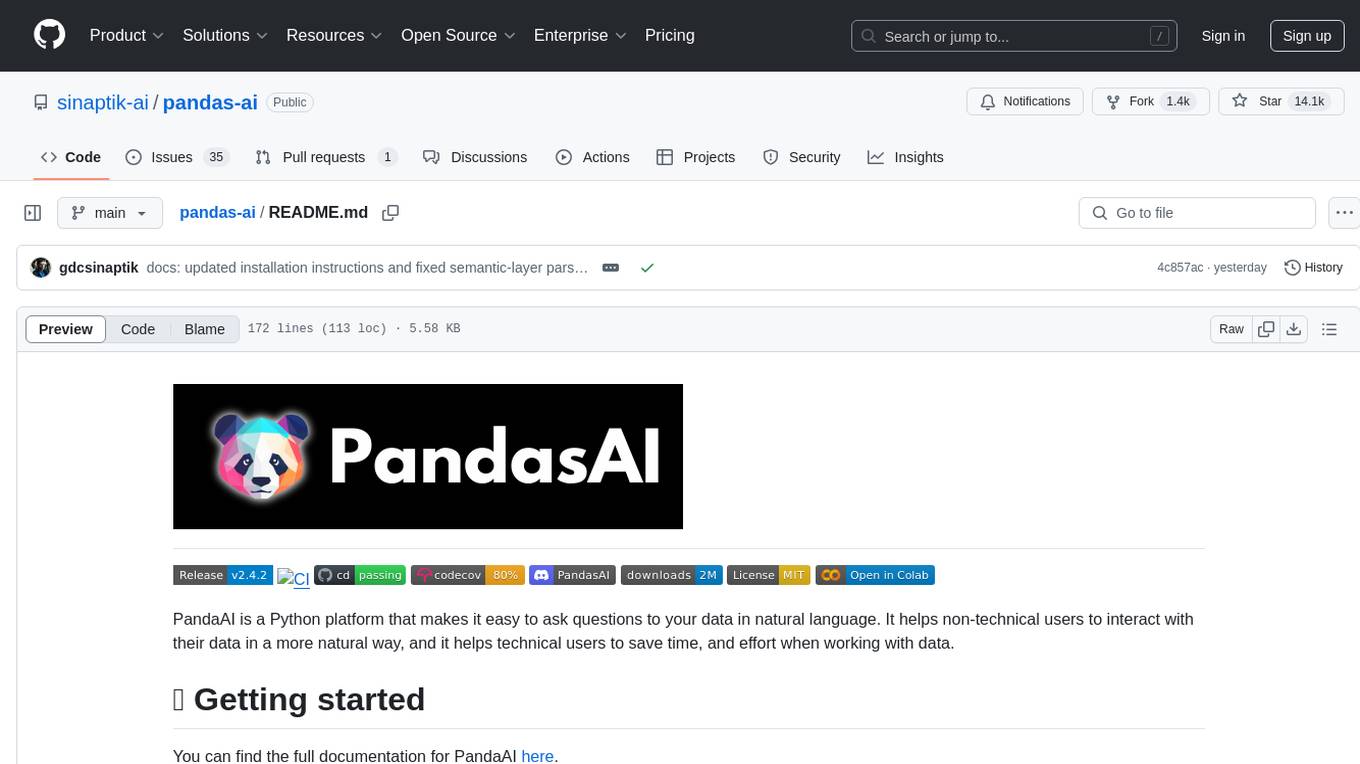
pandas-ai
PandaAI is a Python platform that enables users to interact with their data in natural language, catering to both non-technical and technical users. It simplifies data querying and analysis, offering conversational data analytics capabilities with minimal code. Users can ask questions, visualize charts, and compare dataframes effortlessly. The tool aims to streamline data exploration and decision-making processes by providing a user-friendly interface for data manipulation and analysis.
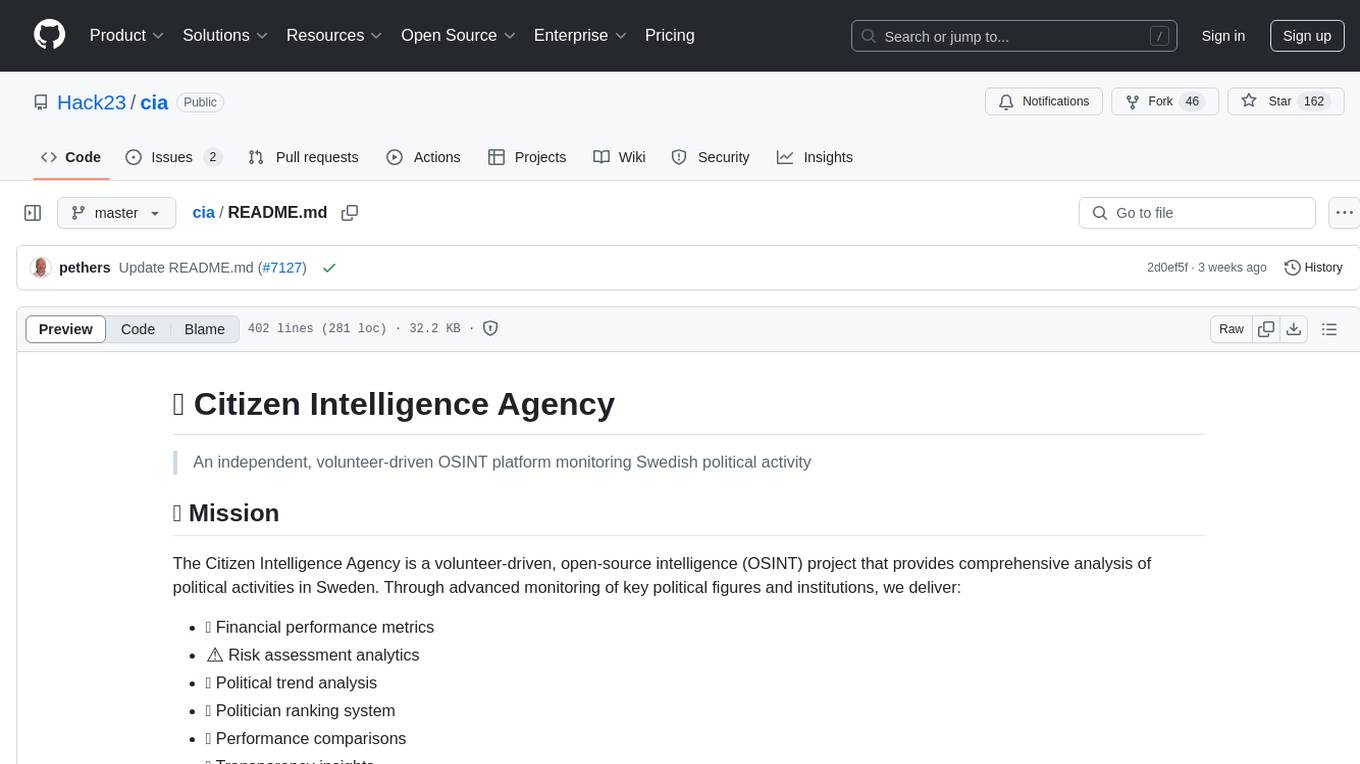
cia
CIA is a powerful open-source tool designed for data analysis and visualization. It provides a user-friendly interface for processing large datasets and generating insightful reports. With CIA, users can easily explore data, perform statistical analysis, and create interactive visualizations to communicate findings effectively. Whether you are a data scientist, analyst, or researcher, CIA offers a comprehensive set of features to streamline your data analysis workflow and uncover valuable insights.
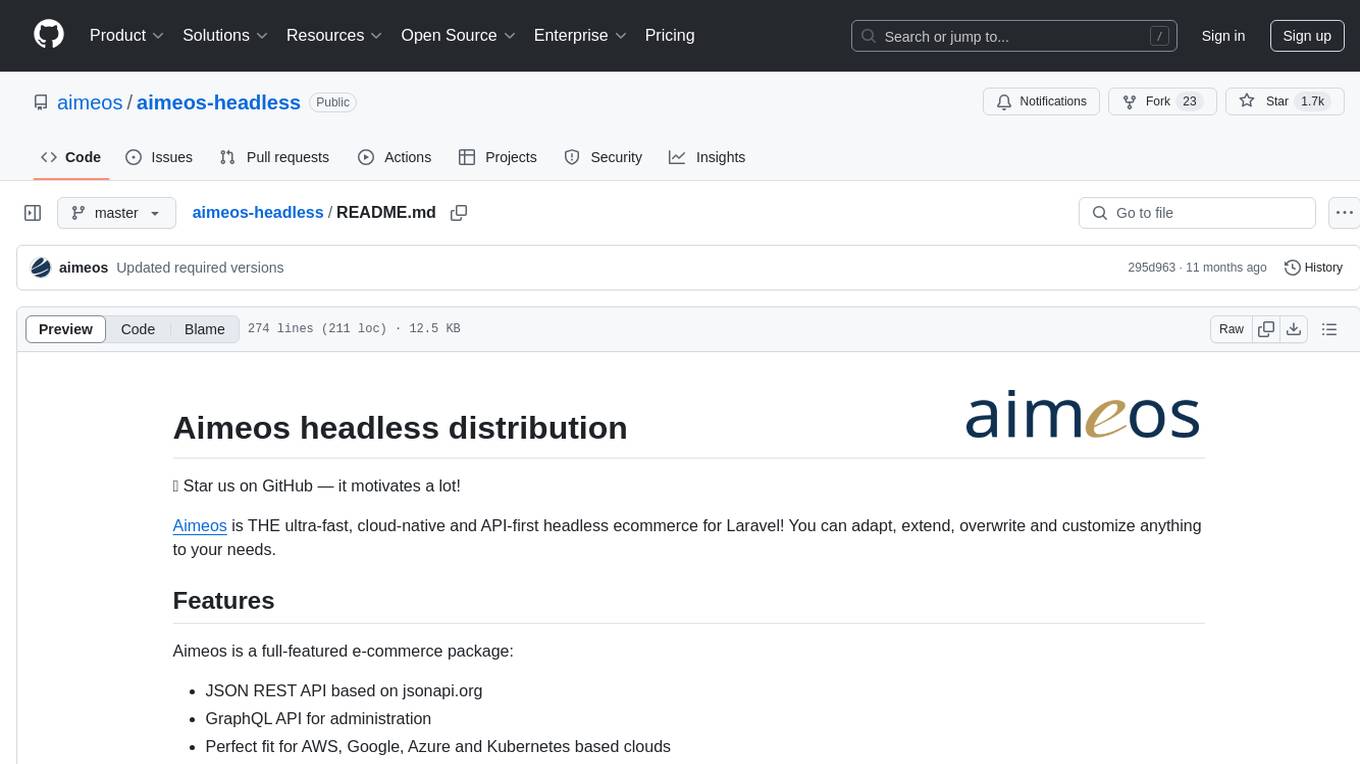
aimeos-headless
Aimeos headless distribution is an ultra-fast, cloud-native, and API-first headless ecommerce solution for Laravel. It offers a full-featured e-commerce package with features like JSON REST API, GraphQL API, multi-vendor support, subscriptions, block/tier pricing, admin backend, and more. The distribution is highly customizable, extensible, and suitable for multi-tenant e-commerce SaaS solutions. It supports multiple languages, AI-based text translation, and provides secure and high-quality source code. Aimeos is designed for AWS, Google, Azure, and Kubernetes based clouds, and can handle a wide range of products efficiently.
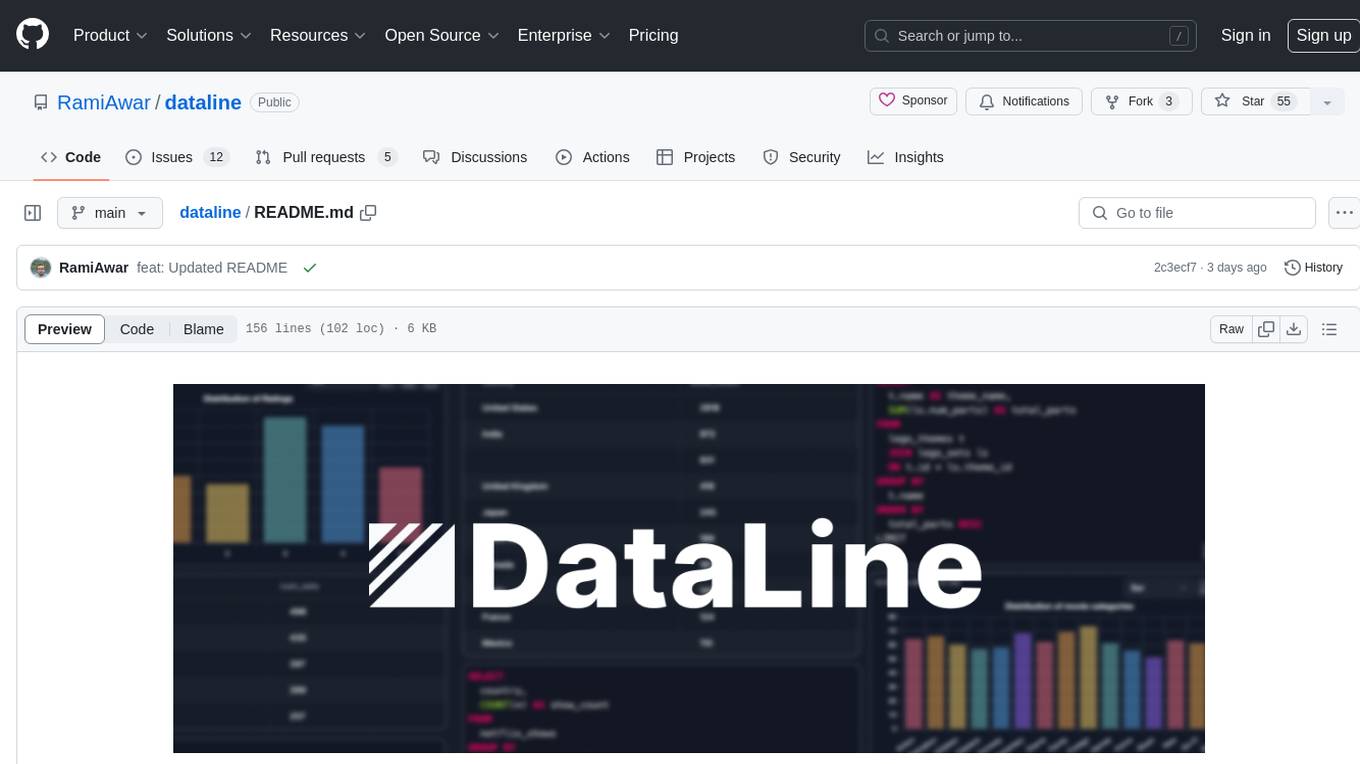
dataline
DataLine is an AI-driven data analysis and visualization tool designed for technical and non-technical users to explore data quickly. It offers privacy-focused data storage on the user's device, supports various data sources, generates charts, executes queries, and facilitates report building. The tool aims to speed up data analysis tasks for businesses and individuals by providing a user-friendly interface and natural language querying capabilities.
For similar jobs
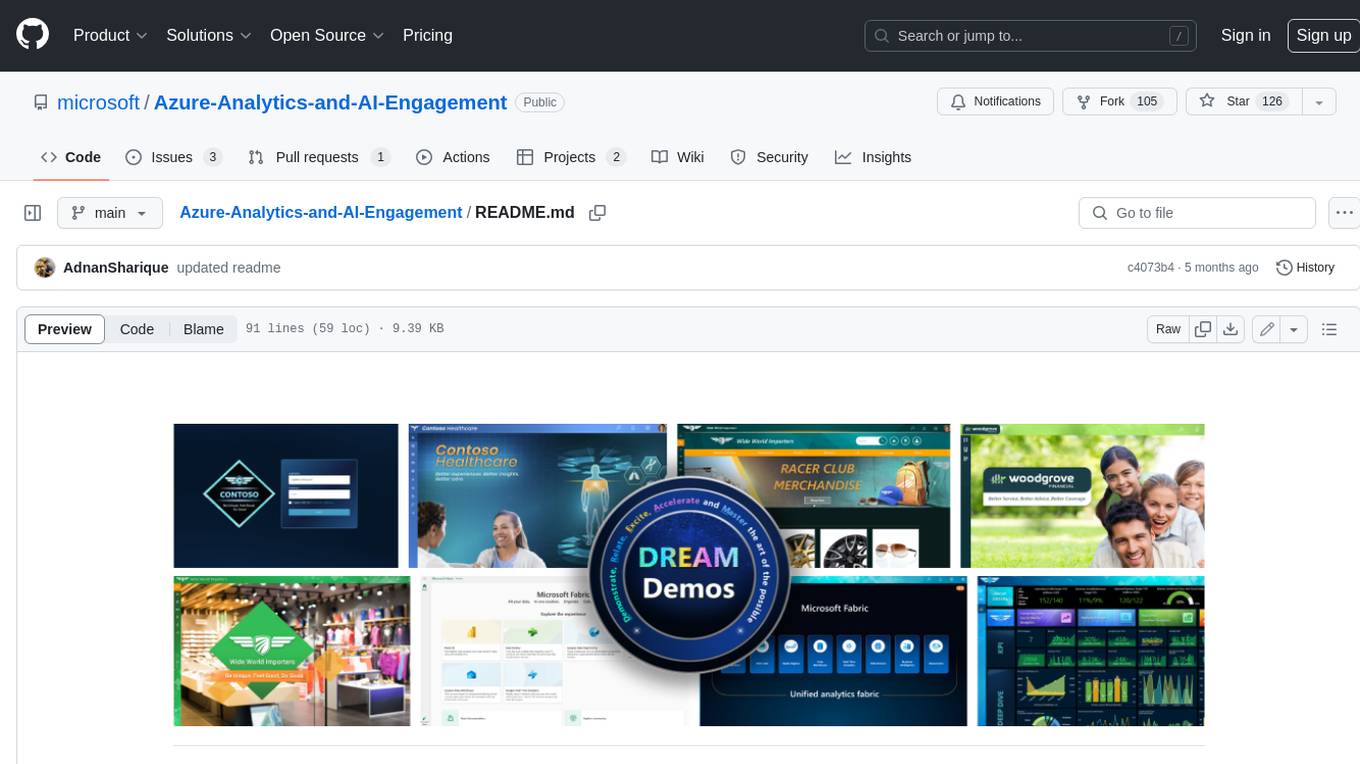
Azure-Analytics-and-AI-Engagement
The Azure-Analytics-and-AI-Engagement repository provides packaged Industry Scenario DREAM Demos with ARM templates (Containing a demo web application, Power BI reports, Synapse resources, AML Notebooks etc.) that can be deployed in a customerโs subscription using the CAPE tool within a matter of few hours. Partners can also deploy DREAM Demos in their own subscriptions using DPoC.
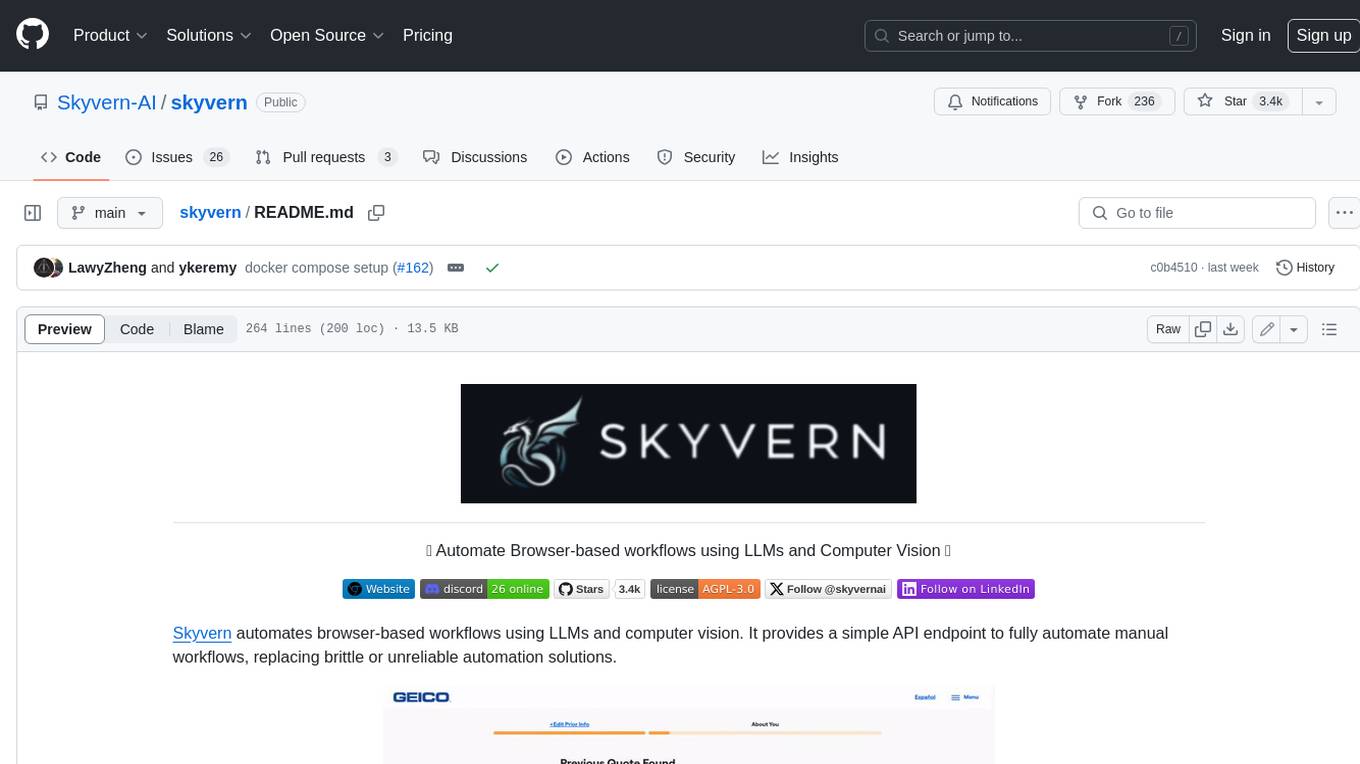
skyvern
Skyvern automates browser-based workflows using LLMs and computer vision. It provides a simple API endpoint to fully automate manual workflows, replacing brittle or unreliable automation solutions. Traditional approaches to browser automations required writing custom scripts for websites, often relying on DOM parsing and XPath-based interactions which would break whenever the website layouts changed. Instead of only relying on code-defined XPath interactions, Skyvern adds computer vision and LLMs to the mix to parse items in the viewport in real-time, create a plan for interaction and interact with them. This approach gives us a few advantages: 1. Skyvern can operate on websites itโs never seen before, as itโs able to map visual elements to actions necessary to complete a workflow, without any customized code 2. Skyvern is resistant to website layout changes, as there are no pre-determined XPaths or other selectors our system is looking for while trying to navigate 3. Skyvern leverages LLMs to reason through interactions to ensure we can cover complex situations. Examples include: 1. If you wanted to get an auto insurance quote from Geico, the answer to a common question โWere you eligible to drive at 18?โ could be inferred from the driver receiving their license at age 16 2. If you were doing competitor analysis, itโs understanding that an Arnold Palmer 22 oz can at 7/11 is almost definitely the same product as a 23 oz can at Gopuff (even though the sizes are slightly different, which could be a rounding error!) Want to see examples of Skyvern in action? Jump to #real-world-examples-of- skyvern
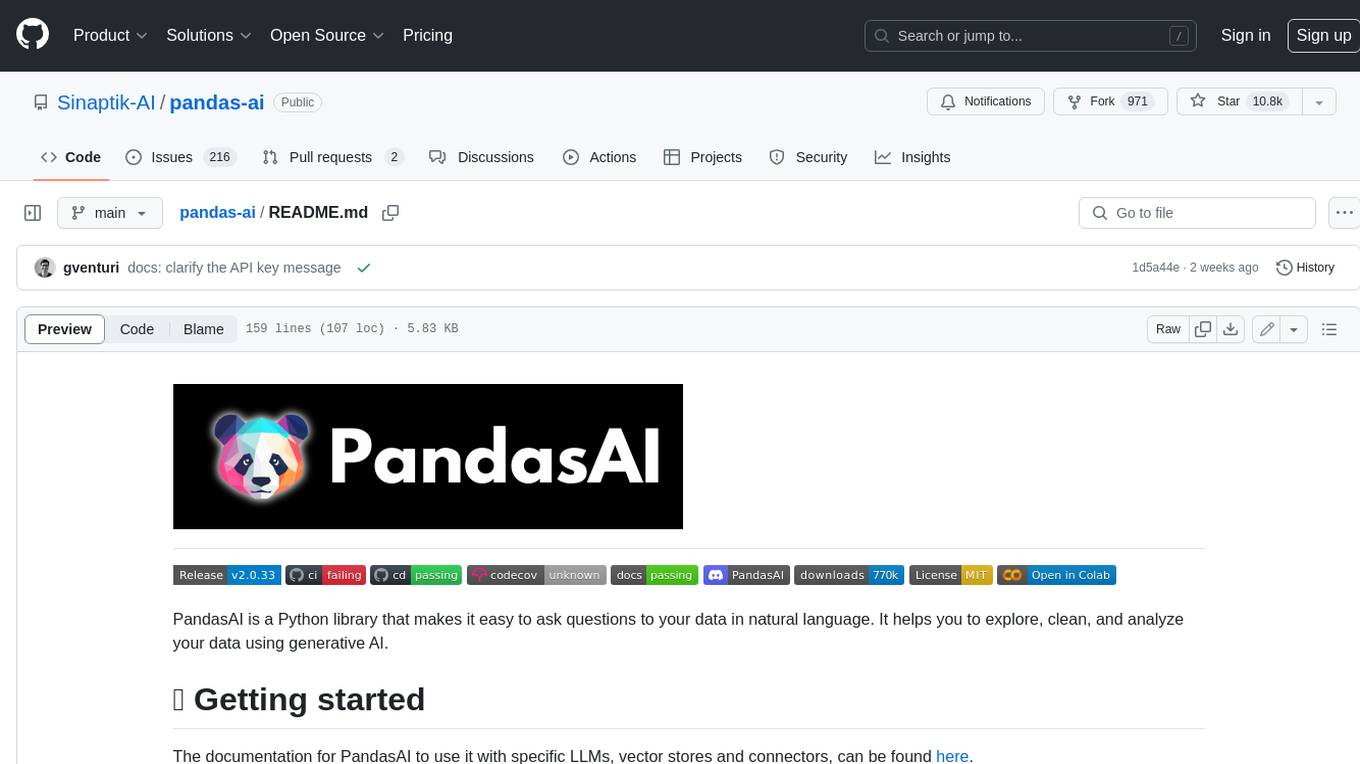
pandas-ai
PandasAI is a Python library that makes it easy to ask questions to your data in natural language. It helps you to explore, clean, and analyze your data using generative AI.
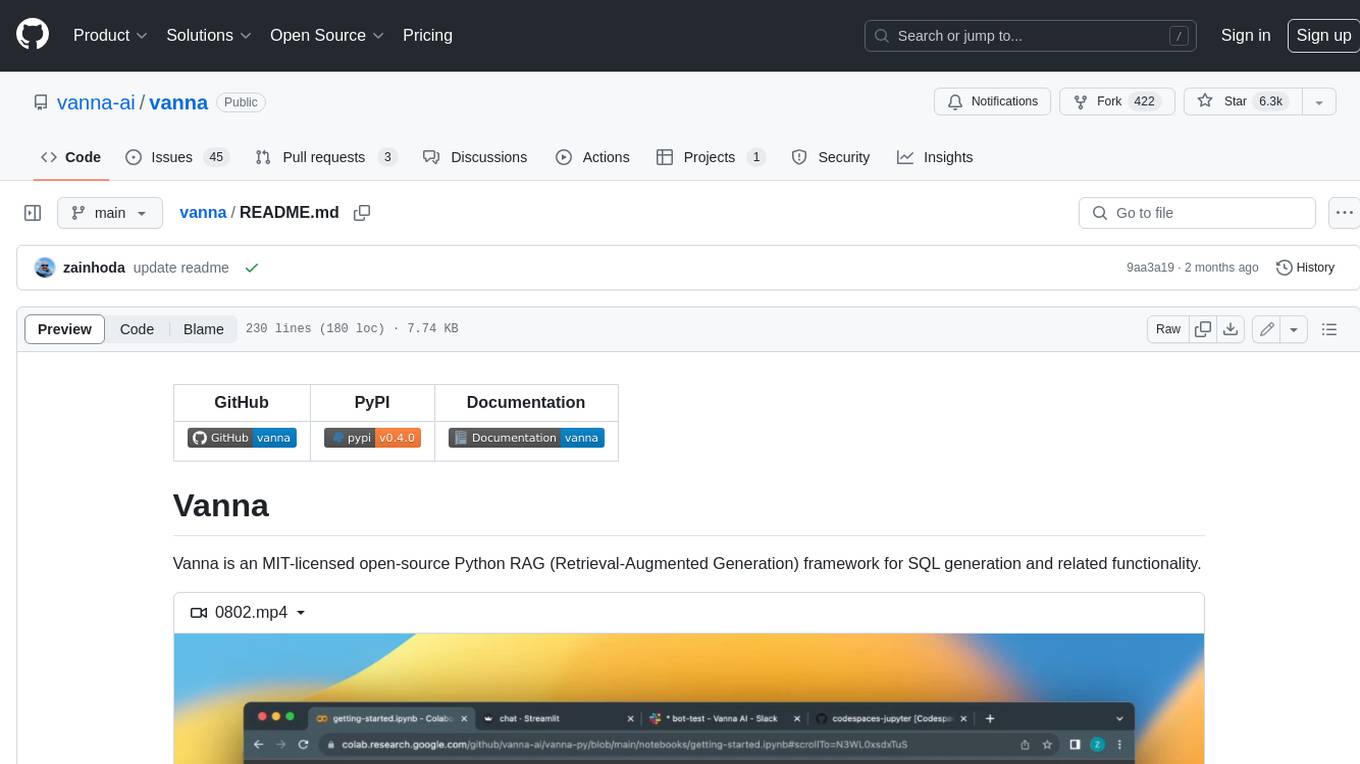
vanna
Vanna is an open-source Python framework for SQL generation and related functionality. It uses Retrieval-Augmented Generation (RAG) to train a model on your data, which can then be used to ask questions and get back SQL queries. Vanna is designed to be portable across different LLMs and vector databases, and it supports any SQL database. It is also secure and private, as your database contents are never sent to the LLM or the vector database.
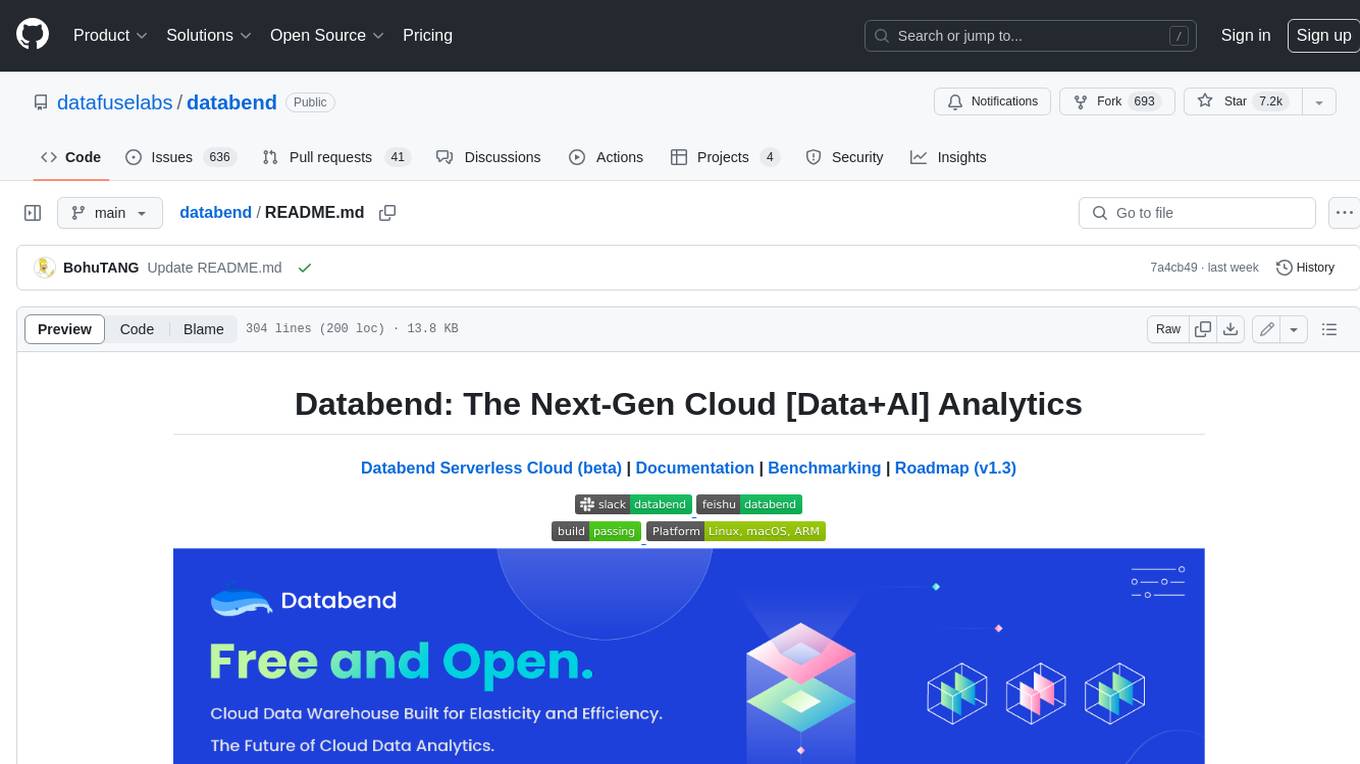
databend
Databend is an open-source cloud data warehouse that serves as a cost-effective alternative to Snowflake. With its focus on fast query execution and data ingestion, it's designed for complex analysis of the world's largest datasets.
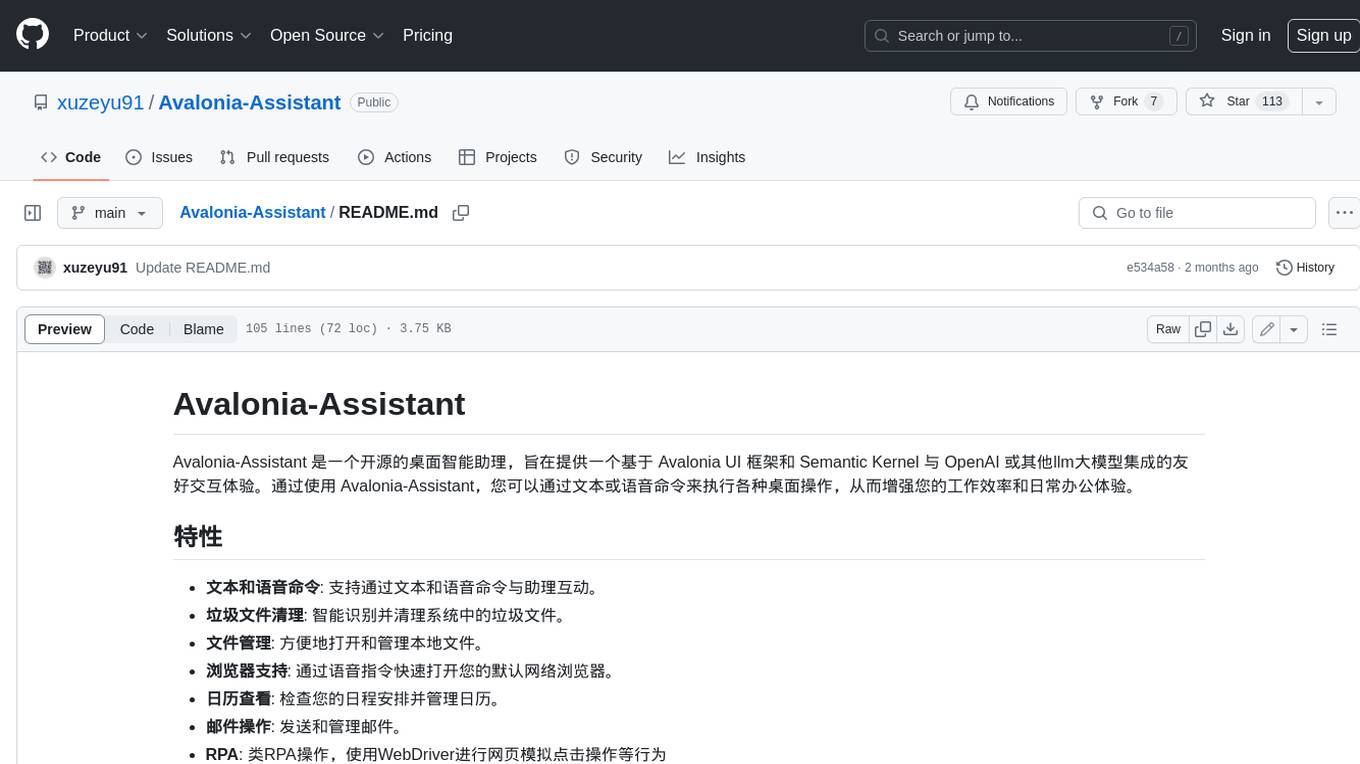
Avalonia-Assistant
Avalonia-Assistant is an open-source desktop intelligent assistant that aims to provide a user-friendly interactive experience based on the Avalonia UI framework and the integration of Semantic Kernel with OpenAI or other large LLM models. By utilizing Avalonia-Assistant, you can perform various desktop operations through text or voice commands, enhancing your productivity and daily office experience.
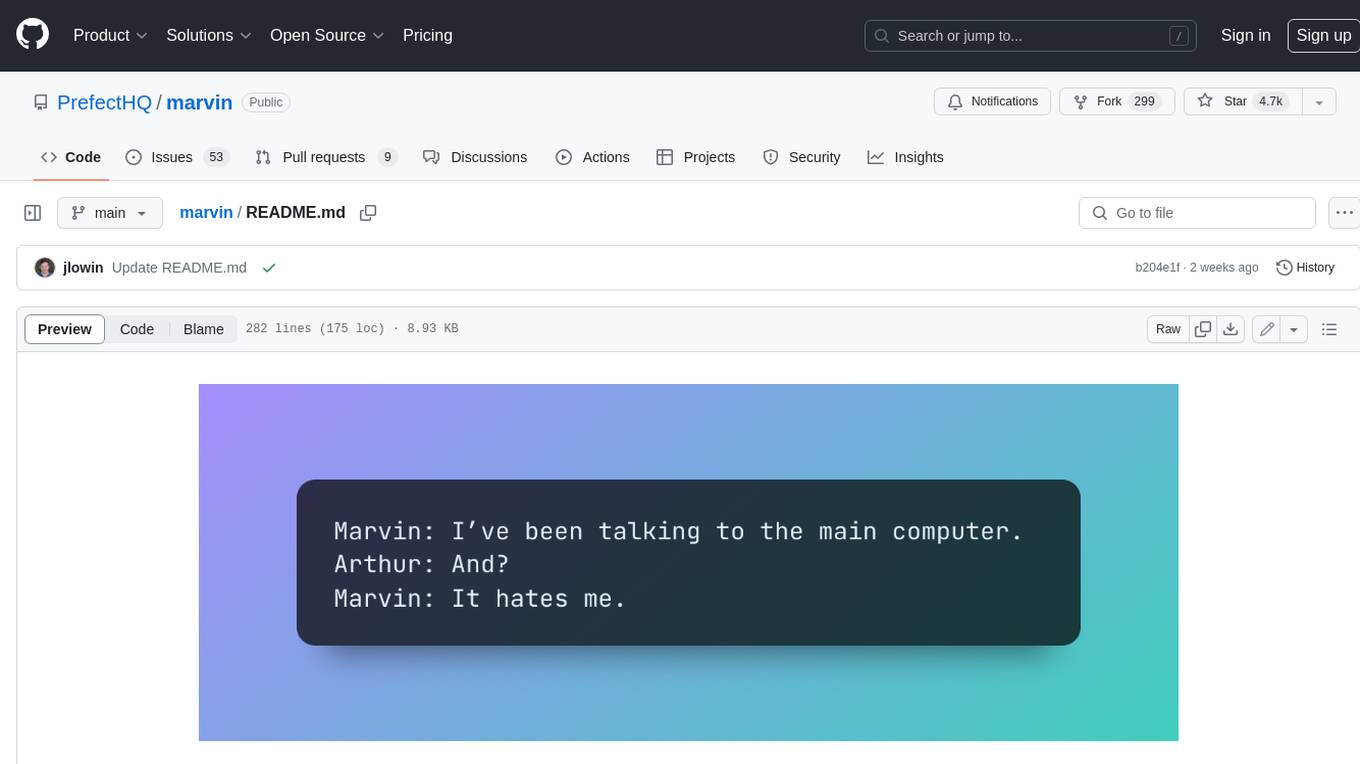
marvin
Marvin is a lightweight AI toolkit for building natural language interfaces that are reliable, scalable, and easy to trust. Each of Marvin's tools is simple and self-documenting, using AI to solve common but complex challenges like entity extraction, classification, and generating synthetic data. Each tool is independent and incrementally adoptable, so you can use them on their own or in combination with any other library. Marvin is also multi-modal, supporting both image and audio generation as well using images as inputs for extraction and classification. Marvin is for developers who care more about _using_ AI than _building_ AI, and we are focused on creating an exceptional developer experience. Marvin users should feel empowered to bring tightly-scoped "AI magic" into any traditional software project with just a few extra lines of code. Marvin aims to merge the best practices for building dependable, observable software with the best practices for building with generative AI into a single, easy-to-use library. It's a serious tool, but we hope you have fun with it. Marvin is open-source, free to use, and made with ๐ by the team at Prefect.
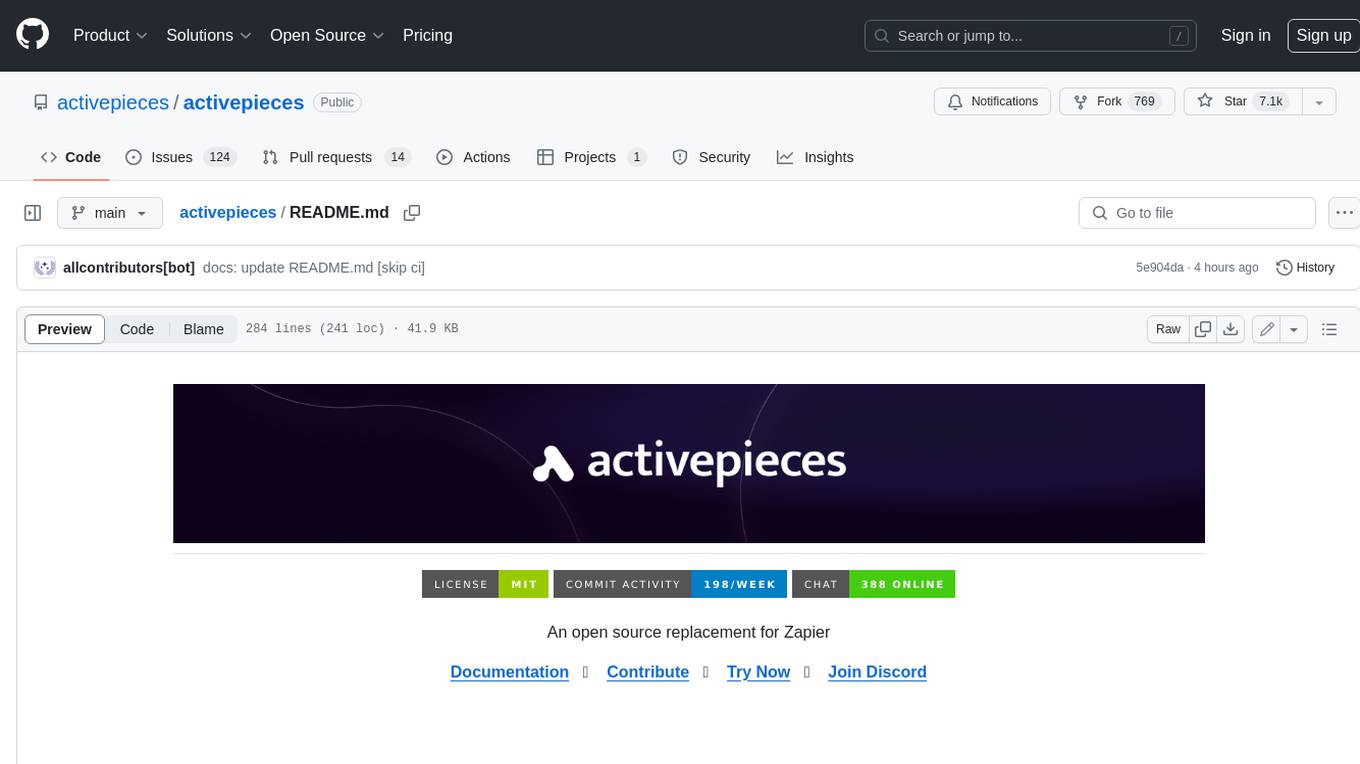
activepieces
Activepieces is an open source replacement for Zapier, designed to be extensible through a type-safe pieces framework written in Typescript. It features a user-friendly Workflow Builder with support for Branches, Loops, and Drag and Drop. Activepieces integrates with Google Sheets, OpenAI, Discord, and RSS, along with 80+ other integrations. The list of supported integrations continues to grow rapidly, thanks to valuable contributions from the community. Activepieces is an open ecosystem; all piece source code is available in the repository, and they are versioned and published directly to npmjs.com upon contributions. If you cannot find a specific piece on the pieces roadmap, please submit a request by visiting the following link: Request Piece Alternatively, if you are a developer, you can quickly build your own piece using our TypeScript framework. For guidance, please refer to the following guide: Contributor's Guide