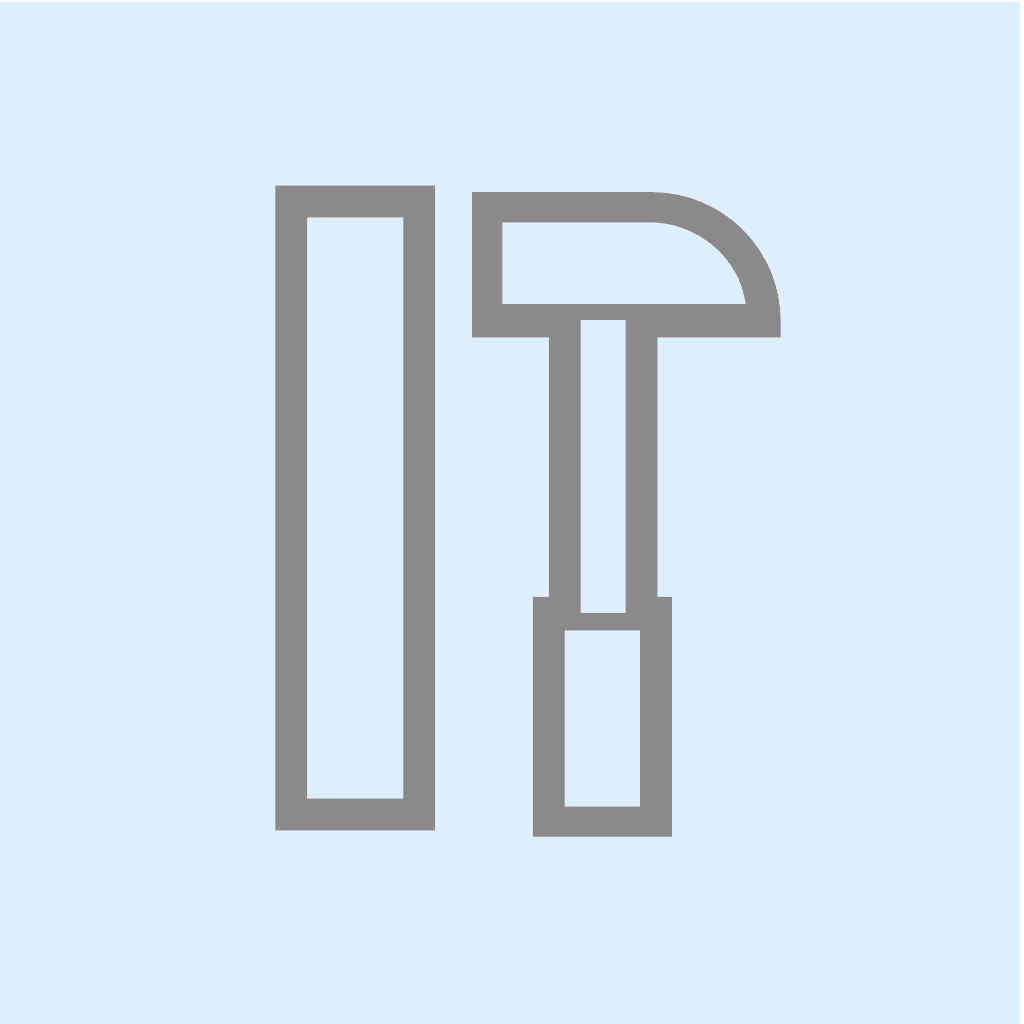
HippoRAG
[NeurIPS'24] HippoRAG is a novel RAG framework inspired by human long-term memory that enables LLMs to continuously integrate knowledge across external documents. RAG + Knowledge Graphs + Personalized PageRank.
Stars: 2062
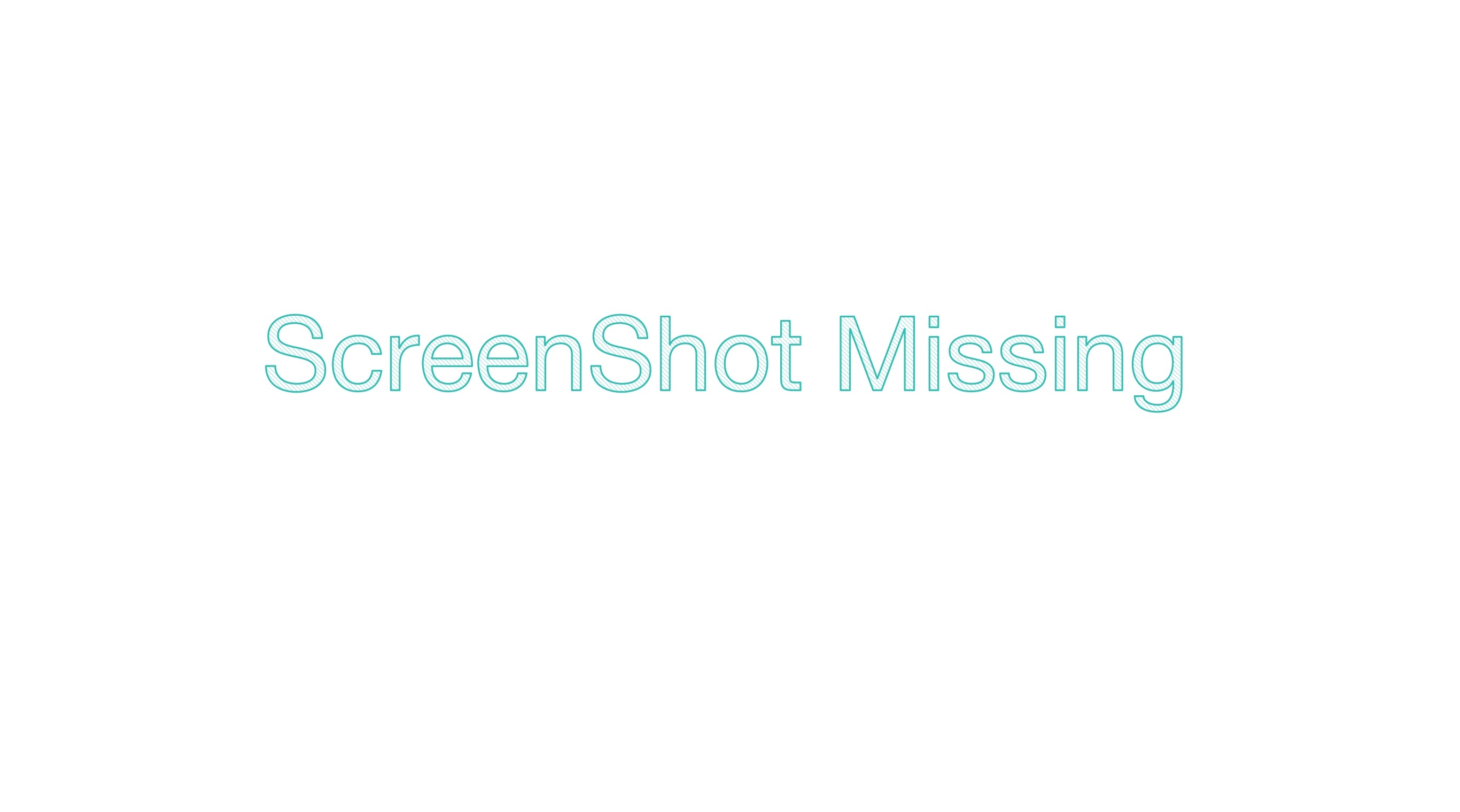
HippoRAG is a novel retrieval augmented generation (RAG) framework inspired by the neurobiology of human long-term memory that enables Large Language Models (LLMs) to continuously integrate knowledge across external documents. It provides RAG systems with capabilities that usually require a costly and high-latency iterative LLM pipeline for only a fraction of the computational cost. The tool facilitates setting up retrieval corpus, indexing, and retrieval processes for LLMs, offering flexibility in choosing different online LLM APIs or offline LLM deployments through LangChain integration. Users can run retrieval on pre-defined queries or integrate directly with the HippoRAG API. The tool also supports reproducibility of experiments and provides data, baselines, and hyperparameter tuning scripts for research purposes.
README:
HippoRAG 2 is a powerful memory framework for LLMs that enhances their ability to recognize and utilize connections in new knowledgeβmirroring a key function of human long-term memory.
Our experiments show that HippoRAG 2 improves associativity (multi-hop retrieval) and sense-making (the process of integrating large and complex contexts) in even the most advanced RAG systems, without sacrificing their performance on simpler tasks.
Like its predecessor, HippoRAG 2 remains cost and latency efficient in online processes, while using significantly fewer resources for offline indexing compared to other graph-based solutions such as GraphRAG, RAPTOR, and LightRAG.
Figure 1: Evaluation of continual learning capabilities across three key dimensions: factual memory (NaturalQuestions, PopQA), sense-making (NarrativeQA), and associativity (MuSiQue, 2Wiki, HotpotQA, and LV-Eval). HippoRAG 2 surpasses other methods across all categories, bringing it one step closer to true long-term memory.
Figure 2: HippoRAG 2 methodology.
- HippoRAG: Neurobiologically Inspired Long-Term Memory for Large Language Models [NeurIPS '24].
- From RAG to Memory: Non-Parametric Continual Learning for Large Language Models [Under Review].
conda create -n hipporag python=3.10
conda activate hipporag
pip install hipporag
Initialize the environmental variables and activate the environment:
export CUDA_VISIBLE_DEVICES=0,1,2,3
export HF_HOME=<path to Huggingface home directory>
export OPENAI_API_KEY=<your openai api key> # if you want to use OpenAI model
conda activate hipporag
This simple example will illustrate how to use hipporag
with any OpenAI model:
from hipporag import HippoRAG
# Prepare datasets and evaluation
docs = [
"Oliver Badman is a politician.",
"George Rankin is a politician.",
"Thomas Marwick is a politician.",
"Cinderella attended the royal ball.",
"The prince used the lost glass slipper to search the kingdom.",
"When the slipper fit perfectly, Cinderella was reunited with the prince.",
"Erik Hort's birthplace is Montebello.",
"Marina is bom in Minsk.",
"Montebello is a part of Rockland County."
]
save_dir = 'outputs'# Define save directory for HippoRAG objects (each LLM/Embedding model combination will create a new subdirectory)
llm_model_name = 'gpt-4o-mini' # Any OpenAI model name
embedding_model_name = 'nvidia/NV-Embed-v2'# Embedding model name (NV-Embed, GritLM or Contriever for now)
#Startup a HippoRAG instance
hipporag = HippoRAG(save_dir=save_dir,
llm_model_name=llm_model_name,
embedding_model_name=embedding_model_name)
#Run indexing
hipporag.index(docs=docs)
#Separate Retrieval & QA
queries = [
"What is George Rankin's occupation?",
"How did Cinderella reach her happy ending?",
"What county is Erik Hort's birthplace a part of?"
]
retrieval_results = hipporag.retrieve(queries=queries, num_to_retrieve=2)
qa_results = hipporag.rag_qa(retrieval_results)
#Combined Retrieval & QA
rag_results = hipporag.rag_qa(queries=queries)
#For Evaluation
answers = [
["Politician"],
["By going to the ball."],
["Rockland County"]
]
gold_docs = [
["George Rankin is a politician."],
["Cinderella attended the royal ball.",
"The prince used the lost glass slipper to search the kingdom.",
"When the slipper fit perfectly, Cinderella was reunited with the prince."],
["Erik Hort's birthplace is Montebello.",
"Montebello is a part of Rockland County."]
]
rag_results = hipporag.rag_qa(queries=queries,
gold_docs=gold_docs,
gold_answers=answers)
This simple example will illustrate how to use hipporag
with any vLLM-compatible locally deployed LLM.
- Run a local OpenAI-compatible vLLM server with specified GPUs (make sure you leave enough memory for your embedding model).
export CUDA_VISIBLE_DEVICES=0,1
export VLLM_WORKER_MULTIPROC_METHOD=spawn
export HF_HOME=<path to Huggingface home directory>
conda activate hipporag # vllm should be in this environment
# Tune gpu-memory-utilization or max_model_len to fit your GPU memory, if OOM occurs
vllm serve meta-llama/Llama-3.3-70B-Instruct --tensor-parallel-size 2 --max_model_len 4096 --gpu-memory-utilization 0.95
- Now you can use very similar code to the one above to use
hipporag
:
save_dir = 'outputs'# Define save directory for HippoRAG objects (each LLM/Embedding model combination will create a new subdirectory)
llm_model_name = # Any OpenAI model name
embedding_model_name = # Embedding model name (NV-Embed, GritLM or Contriever for now)
llm_base_url= # Base url for your deployed LLM (i.e. http://localhost:8000/v1)
hipporag = HippoRAG(save_dir=save_dir,
llm_model_name=llm_model,
embedding_model_name=embedding_model_name,
llm_base_url=llm_base_url)
# Same Indexing, Retrieval and QA as running OpenAI models above
To use our code to run experiments we recommend you clone this repository and follow the structure of the main.py
script.
We evaluated several sampled datasets in our paper, some of which are already included in the reproduce/dataset
directory of this repo. For the complete set of datasets, please visit
our HuggingFace dataset and place them under reproduce/dataset
. We also provide the OpenIE results for both gpt-4o-mini
and Llama-3.3-70B-Instruct
for our musique
sample under outputs/musique
.
To test your environment is properly set up, you can use the small dataset reproduce/dataset/sample.json
for debugging as shown below.
Initialize the environmental variables and activate the environment:
export CUDA_VISIBLE_DEVICES=0,1,2,3
export HF_HOME=<path to Huggingface home directory>
export OPENAI_API_KEY=<your openai api key> # if you want to use OpenAI model
conda activate hipporag
dataset=sample # or any other dataset under `reproduce/dataset`
# Run OpenAI model
python main.py --dataset $dataset --llm_base_url https://api.openai.com/v1 --llm_name gpt-4o-mini --embedding_name nvidia/NV-Embed-v2
- As above, run a local OpenAI-compatible vLLM server with specified GPU.
export CUDA_VISIBLE_DEVICES=0,1
export VLLM_WORKER_MULTIPROC_METHOD=spawn
export HF_HOME=<path to Huggingface home directory>
conda activate hipporag # vllm should be in this environment
# Tune gpu-memory-utilization or max_model_len to fit your GPU memory, if OOM occurs
vllm serve meta-llama/Llama-3.3-70B-Instruct --tensor-parallel-size 2 --max_model_len 4096 --gpu-memory-utilization 0.95
- Use another GPUs to run the main program in another terminal.
export CUDA_VISIBLE_DEVICES=2,3 # set another GPUs while vLLM server is running
export HF_HOME=<path to Huggingface home directory>
dataset=sample
python main.py --dataset $dataset --llm_base_url http://localhost:8000/v1 --llm_name meta-llama/Llama-3.3-70B-Instruct --embedding_name nvidia/NV-Embed-v2
vLLM offers an offline batch mode for faster inference, which could bring us more than 3x faster indexing compared to vLLM online server.
- Use the following command to run the main program with vLLM offline batch mode.
export CUDA_VISIBLE_DEVICES=0,1,2,3 # use all GPUs for faster offline indexing
export VLLM_WORKER_MULTIPROC_METHOD=spawn
export HF_HOME=<path to Huggingface home directory>
export OPENAI_API_KEY=''
dataset=sample
python main.py --dataset $dataset --llm_name meta-llama/Llama-3.3-70B-Instruct --openie_mode offline --skip_graph
- After the first step, OpenIE result is saved to file. Go back to run vLLM online server and main program as described in the
Run with vLLM (Llama)
main section.
-
/reproduce/dataset/sample.json
is a small dataset specifically for debugging. - When debugging vLLM offline mode, set
tensor_parallel_size
as1
inhipporag/llm/vllm_offline.py
. - If you want to rerun a particular experiment, remember to clear the saved files, including OpenIE results and knowledge graph, e.g.,
rm reproduce/dataset/openie_results/openie_sample_results_ner_meta-llama_Llama-3.3-70B-Instruct_3.json
rm -rf outputs/sample/sample_meta-llama_Llama-3.3-70B-Instruct_nvidia_NV-Embed-v2
To setup your own custom dataset for evaluation, follow the format and naming convention shown in reproduce/dataset/sample_corpus.json
(your dataset's name should be followed by _corpus.json
). If running an experiment with pre-defined questions, organize your query corpus according to the query file reproduce/dataset/sample.json
, be sure to also follow our naming convention.
The corpus and optional query JSON files should have the following format:
[
{
"title": "FIRST PASSAGE TITLE",
"text": "FIRST PASSAGE TEXT",
"idx": 0
},
{
"title": "SECOND PASSAGE TITLE",
"text": "SECOND PASSAGE TEXT",
"idx": 1
}
]
[
{
"id": "sample/question_1.json",
"question": "QUESTION",
"answer": [
"ANSWER"
],
"answerable": true,
"paragraphs": [
{
"title": "{FIRST SUPPORTING PASSAGE TITLE}",
"text": "{FIRST SUPPORTING PASSAGE TEXT}",
"is_supporting": true,
"idx": 0
},
{
"title": "{SECOND SUPPORTING PASSAGE TITLE}",
"text": "{SECOND SUPPORTING PASSAGE TEXT}",
"is_supporting": true,
"idx": 1
}
]
}
]
When preparing your data, you may need to chunk each passage, as longer passage may be too complex for the OpenIE process.
π¦ .
β-- π src/hipporag
β βββ π embedding_model # Implementation of all embedding models
β β βββ __init__.py # Getter function for get specific embedding model classes
| | βββ base.py # Base embedding model class `BaseEmbeddingModel` to inherit and `EmbeddingConfig`
| | βββ NVEmbedV2.py # Implementation of NV-Embed-v2 model
| | βββ ...
β βββ π evaluation # Implementation of all evaluation metrics
β β βββ __init__.py
| | βββ base.py # Base evaluation metric class `BaseMetric` to inherit
β β βββ qa_eval.py # Eval metrics for QA
β β βββ retrieval_eval.py # Eval metrics for retrieval
β βββ π information_extraction # Implementation of all information extraction models
β β βββ __init__.py
| | βββ openie_openai_gpt.py # Model for OpenIE with OpenAI GPT
| | βββ openie_vllm_offline.py # Model for OpenIE with LLMs deployed offline with vLLM
β βββ π llm # Classes for inference with large language models
β β βββ __init__.py # Getter function
| | βββ base.py # Config class for LLM inference and base LLM inference class to inherit
| | βββ openai_gpt.py # Class for inference with OpenAI GPT
| | βββ vllm_llama.py # Class for inference using a local vLLM server
| | βββ vllm_offline.py # Class for inference using the vLLM API directly
β βββ π prompts # Prompt templates and prompt template manager class
| β βββ π dspy_prompts # Prompts for filtering
| β β βββ ...
| β βββ π templates # All prompt templates for template manager to load
| β β βββ README.md # Documentations of usage of prompte template manager and prompt template files
| β β βββ __init__.py
| β β βββ triple_extraction.py
| β β βββ ...
β β βββ __init__.py
| | βββ linking.py # Instruction for linking
| | βββ prompt_template_manager.py # Implementation of prompt template manager
β βββ π utils # All utility functions used across this repo (the file name indicates its relevant usage)
β β βββ config_utils.py # We use only one config across all modules and its setup is specified here
| | βββ ...
β βββ __init__.py
β βββ HippoRAG.py # Highest level class for initiating retrieval, question answering, and evaluations
β βββ embedding_store.py # Storage database to load, manage and save embeddings for passages, entities and facts.
β βββ rerank.py # Reranking and filtering methods
β-- π examples
β βββ ...
β βββ ...
β-- π README.md
β-- π requirements.txt # Dependencies list
β-- π .gitignore # Files to exclude from Git
Questions or issues? File an issue or contact Bernal JimΓ©nez GutiΓ©rrez, Yiheng Shu, Yu Su, The Ohio State University
If you find this work useful, please consider citing our papers:
@misc{gutiΓ©rrez2025ragmemorynonparametriccontinual,
title={From RAG to Memory: Non-Parametric Continual Learning for Large Language Models},
author={Bernal JimΓ©nez GutiΓ©rrez and Yiheng Shu and Weijian Qi and Sizhe Zhou and Yu Su},
year={2025},
eprint={2502.14802},
archivePrefix={arXiv},
primaryClass={cs.CL},
url={https://arxiv.org/abs/2502.14802},
}
@inproceedings{gutiΓ©rrez2024hipporag,
title={HippoRAG: Neurobiologically Inspired Long-Term Memory for Large Language Models},
author={Bernal JimΓ©nez GutiΓ©rrez and Yiheng Shu and Yu Gu and Michihiro Yasunaga and Yu Su},
booktitle={The Thirty-eighth Annual Conference on Neural Information Processing Systems},
year={2024},
url={https://openreview.net/forum?id=hkujvAPVsg}
- [ ] Add support for more embedding models
- [ ] Add support for vector database integration
- [ ] Add support for embedding endpoints
Please feel free to open an issue or PR if you have any questions or suggestions.
For Tasks:
Click tags to check more tools for each tasksFor Jobs:
Alternative AI tools for HippoRAG
Similar Open Source Tools
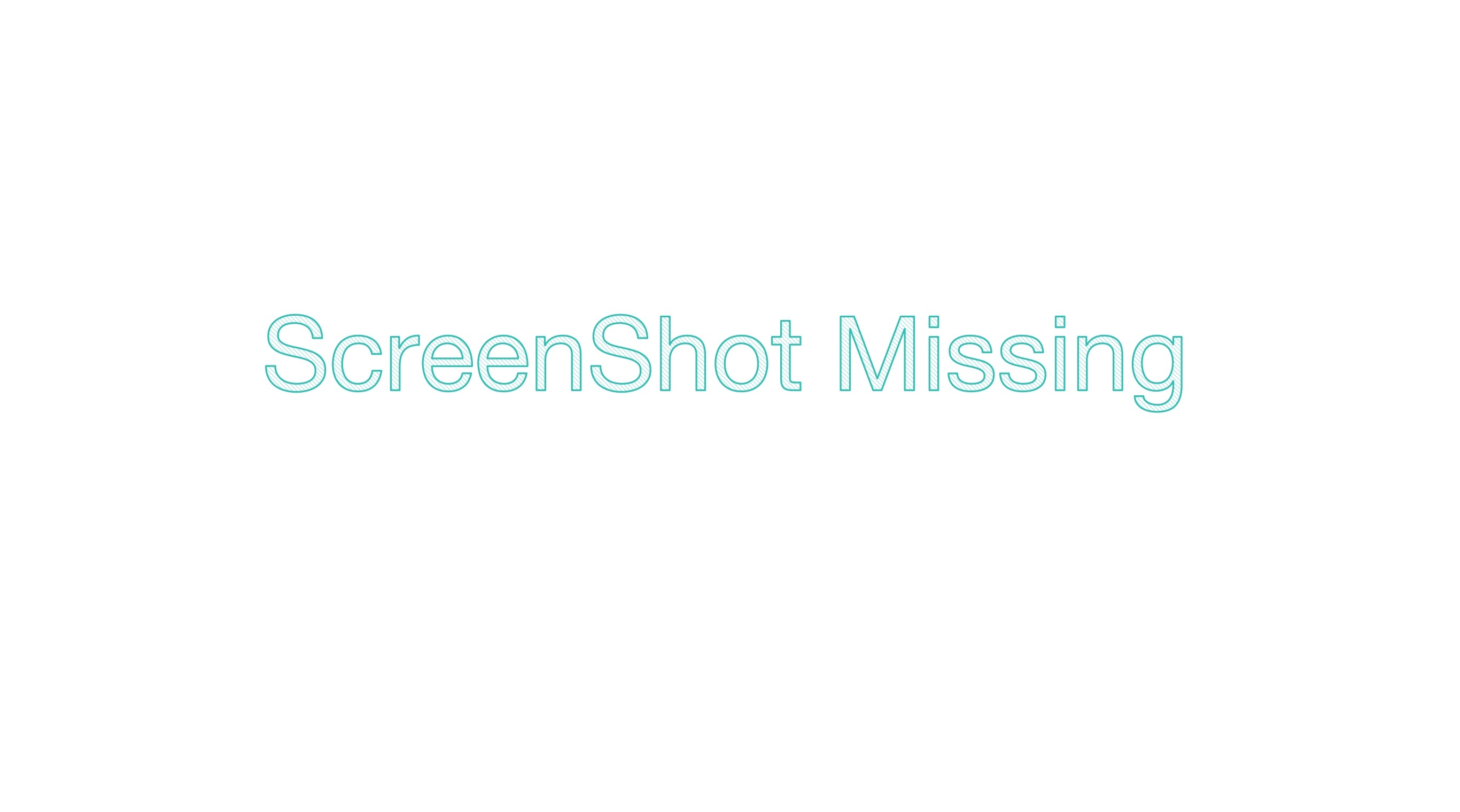
HippoRAG
HippoRAG is a novel retrieval augmented generation (RAG) framework inspired by the neurobiology of human long-term memory that enables Large Language Models (LLMs) to continuously integrate knowledge across external documents. It provides RAG systems with capabilities that usually require a costly and high-latency iterative LLM pipeline for only a fraction of the computational cost. The tool facilitates setting up retrieval corpus, indexing, and retrieval processes for LLMs, offering flexibility in choosing different online LLM APIs or offline LLM deployments through LangChain integration. Users can run retrieval on pre-defined queries or integrate directly with the HippoRAG API. The tool also supports reproducibility of experiments and provides data, baselines, and hyperparameter tuning scripts for research purposes.
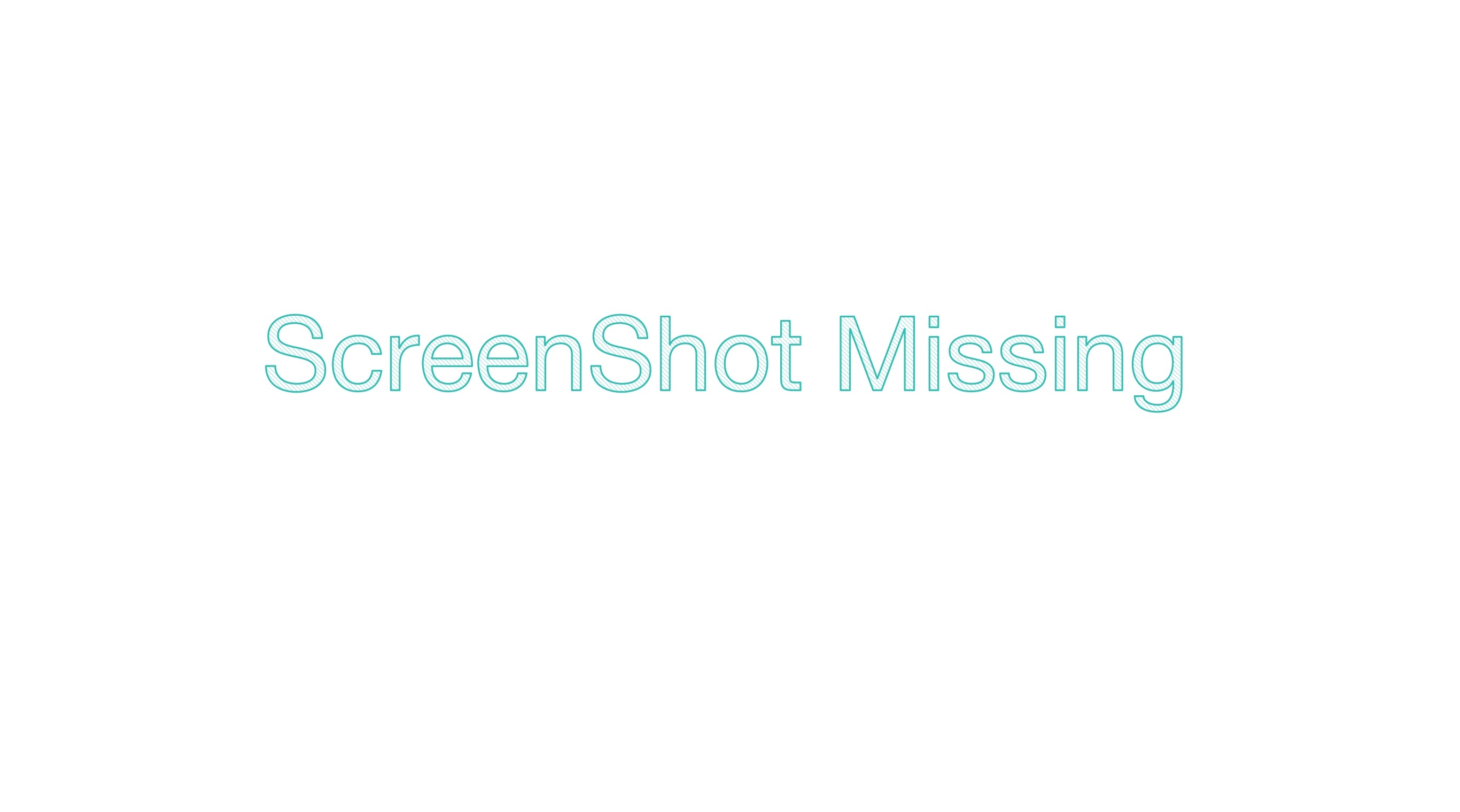
syncode
SynCode is a novel framework for the grammar-guided generation of Large Language Models (LLMs) that ensures syntactically valid output with respect to defined Context-Free Grammar (CFG) rules. It supports general-purpose programming languages like Python, Go, SQL, JSON, and more, allowing users to define custom grammars using EBNF syntax. The tool compares favorably to other constrained decoders and offers features like fast grammar-guided generation, compatibility with HuggingFace Language Models, and the ability to work with various decoding strategies.
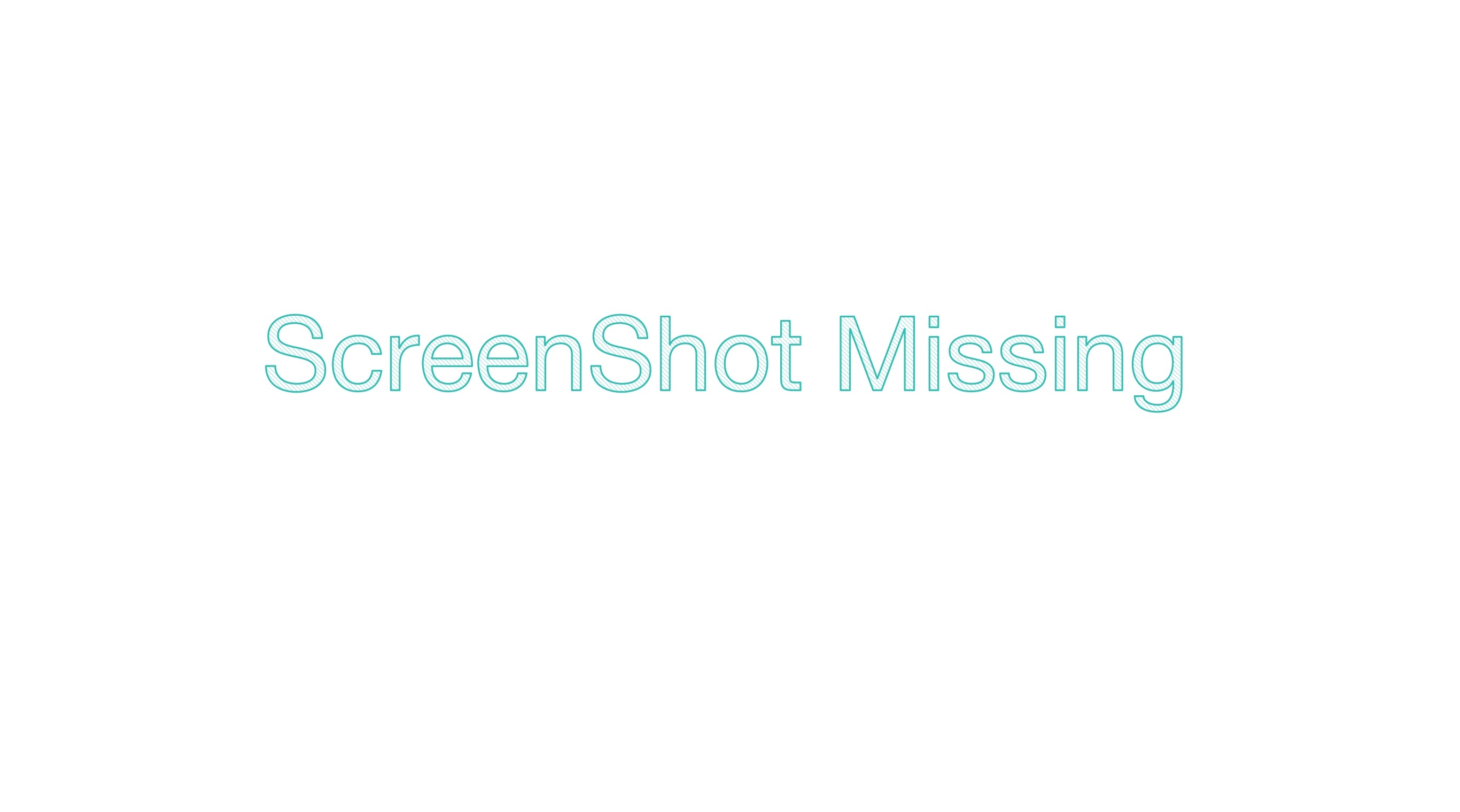
gfm-rag
The GFM-RAG is a graph foundation model-powered pipeline that combines graph neural networks to reason over knowledge graphs and retrieve relevant documents for question answering. It features a knowledge graph index, efficiency in multi-hop reasoning, generalizability to unseen datasets, transferability for fine-tuning, compatibility with agent-based frameworks, and interpretability of reasoning paths. The tool can be used for conducting retrieval and question answering tasks using pre-trained models or fine-tuning on custom datasets.
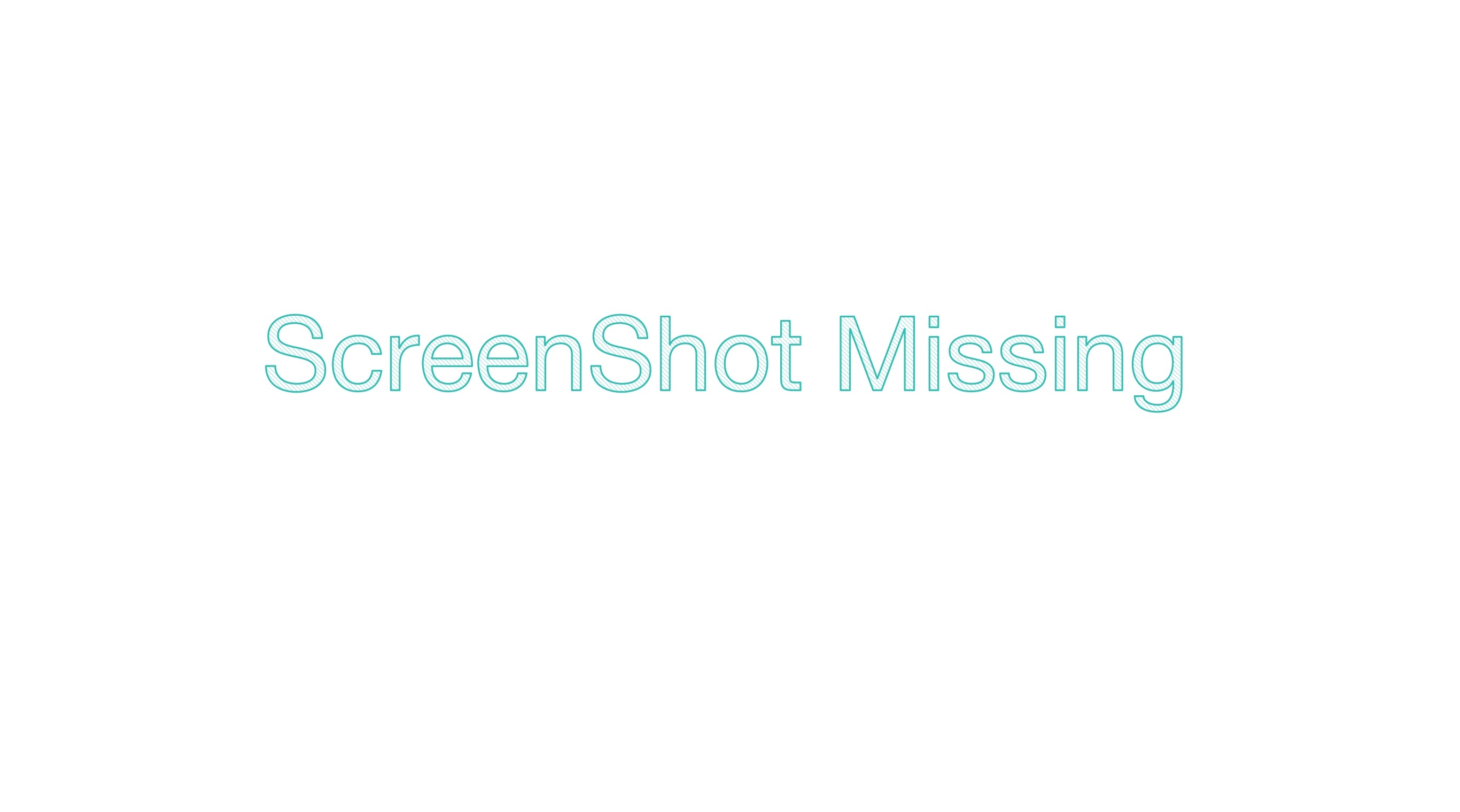
mlx-llm
mlx-llm is a library that allows you to run Large Language Models (LLMs) on Apple Silicon devices in real-time using Apple's MLX framework. It provides a simple and easy-to-use API for creating, loading, and using LLM models, as well as a variety of applications such as chatbots, fine-tuning, and retrieval-augmented generation.
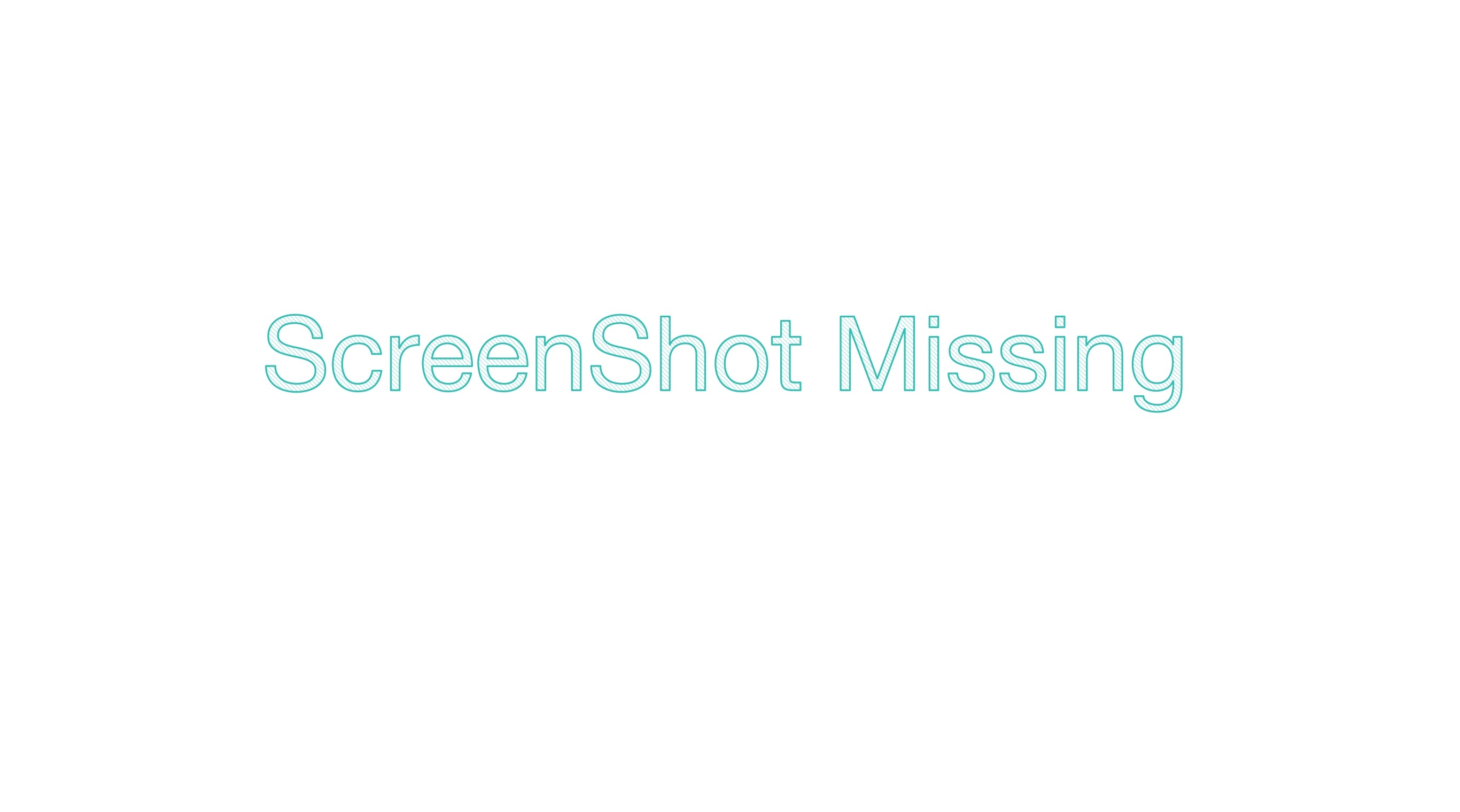
lionagi
LionAGI is a powerful intelligent workflow automation framework that introduces advanced ML models into any existing workflows and data infrastructure. It can interact with almost any model, run interactions in parallel for most models, produce structured pydantic outputs with flexible usage, automate workflow via graph based agents, use advanced prompting techniques, and more. LionAGI aims to provide a centralized agent-managed framework for "ML-powered tools coordination" and to dramatically lower the barrier of entries for creating use-case/domain specific tools. It is designed to be asynchronous only and requires Python 3.10 or higher.
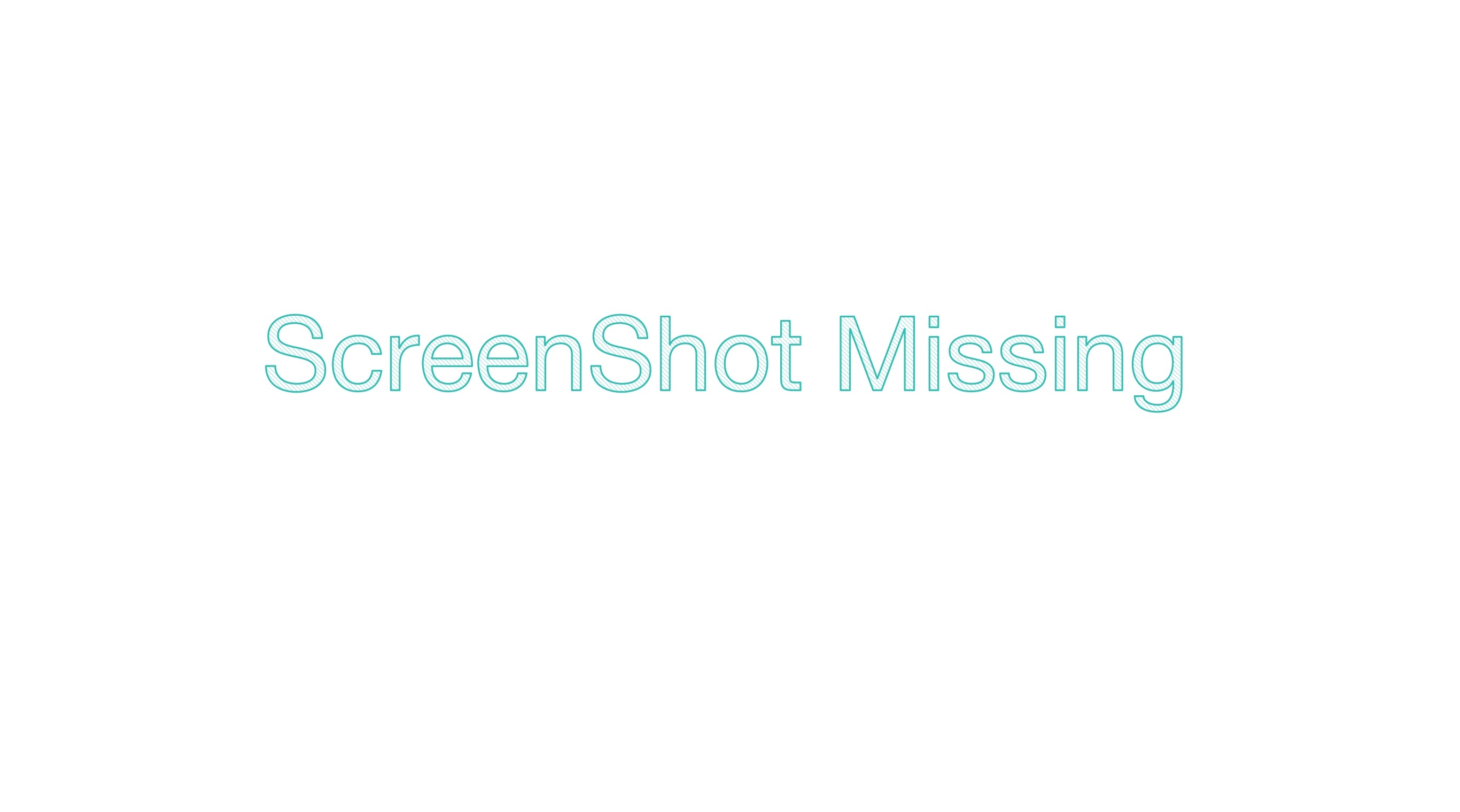
ModelCache
Codefuse-ModelCache is a semantic cache for large language models (LLMs) that aims to optimize services by introducing a caching mechanism. It helps reduce the cost of inference deployment, improve model performance and efficiency, and provide scalable services for large models. The project facilitates sharing and exchanging technologies related to large model semantic cache through open-source collaboration.
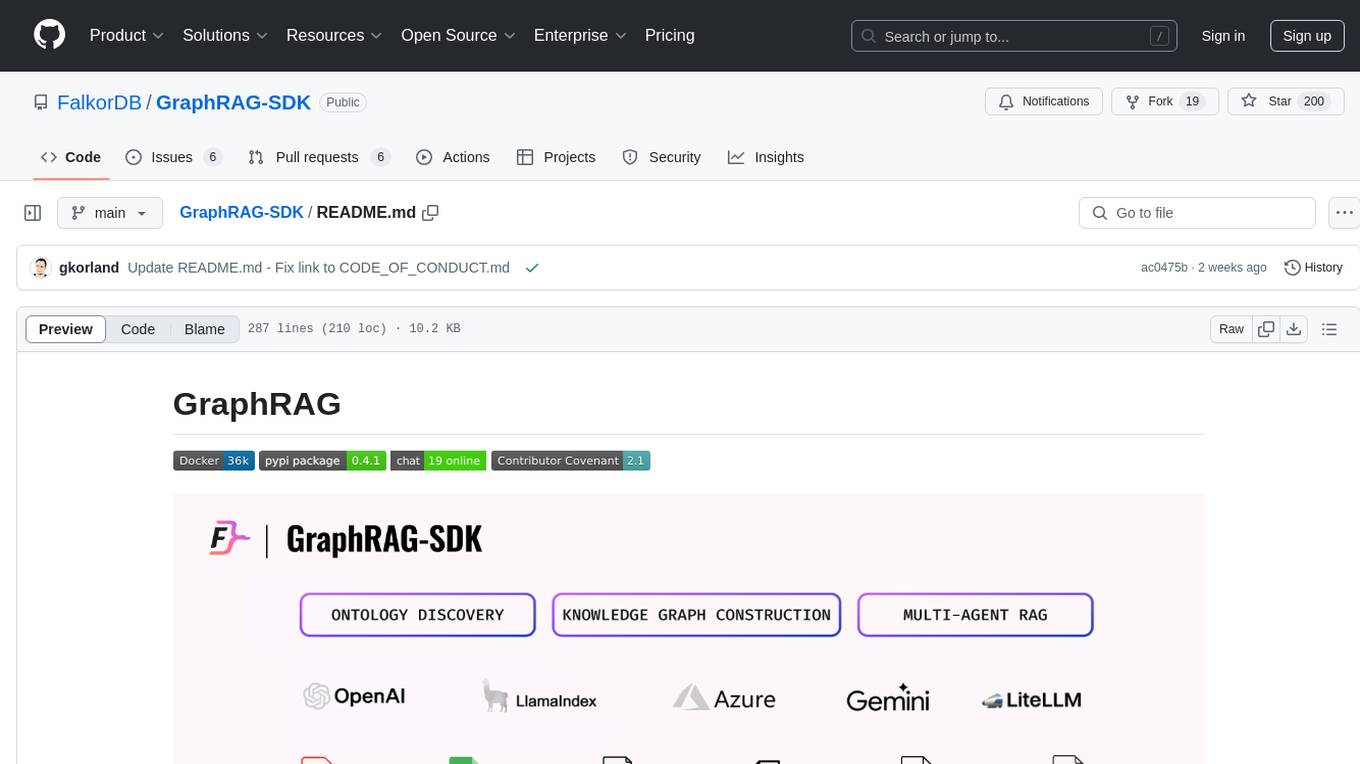
GraphRAG-SDK
Build fast and accurate GenAI applications with GraphRAG SDK, a specialized toolkit for building Graph Retrieval-Augmented Generation (GraphRAG) systems. It integrates knowledge graphs, ontology management, and state-of-the-art LLMs to deliver accurate, efficient, and customizable RAG workflows. The SDK simplifies the development process by automating ontology creation, knowledge graph agent creation, and query handling, enabling users to interact and query their knowledge graphs effectively. It supports multi-agent systems and orchestrates agents specialized in different domains. The SDK is optimized for FalkorDB, ensuring high performance and scalability for large-scale applications. By leveraging knowledge graphs, it enables semantic relationships and ontology-driven queries that go beyond standard vector similarity, enhancing retrieval-augmented generation capabilities.
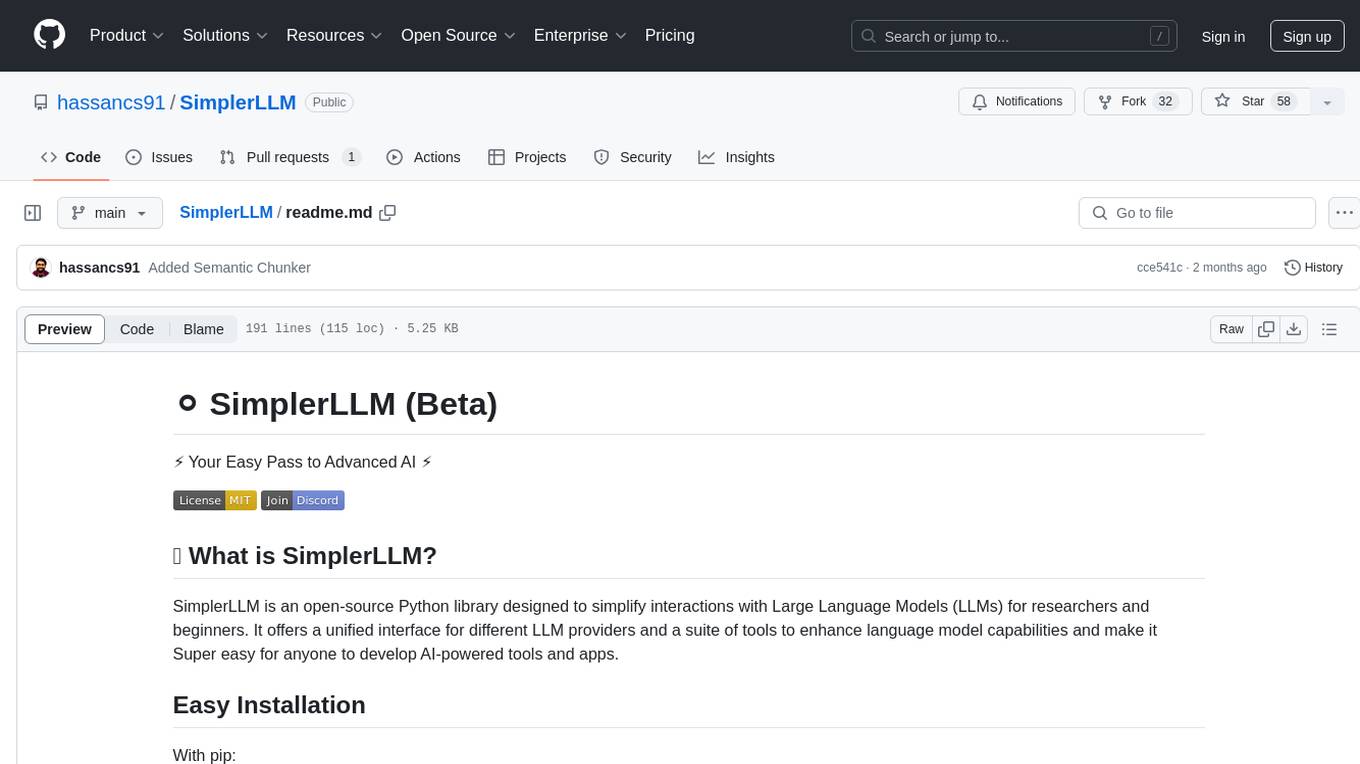
SimplerLLM
SimplerLLM is an open-source Python library that simplifies interactions with Large Language Models (LLMs) for researchers and beginners. It provides a unified interface for different LLM providers, tools for enhancing language model capabilities, and easy development of AI-powered tools and apps. The library offers features like unified LLM interface, generic text loader, RapidAPI connector, SERP integration, prompt template builder, and more. Users can easily set up environment variables, create LLM instances, use tools like SERP, generic text loader, calling RapidAPI APIs, and prompt template builder. Additionally, the library includes chunking functions to split texts into manageable chunks based on different criteria. Future updates will bring more tools, interactions with local LLMs, prompt optimization, response evaluation, GPT Trainer, document chunker, advanced document loader, integration with more providers, Simple RAG with SimplerVectors, integration with vector databases, agent builder, and LLM server.
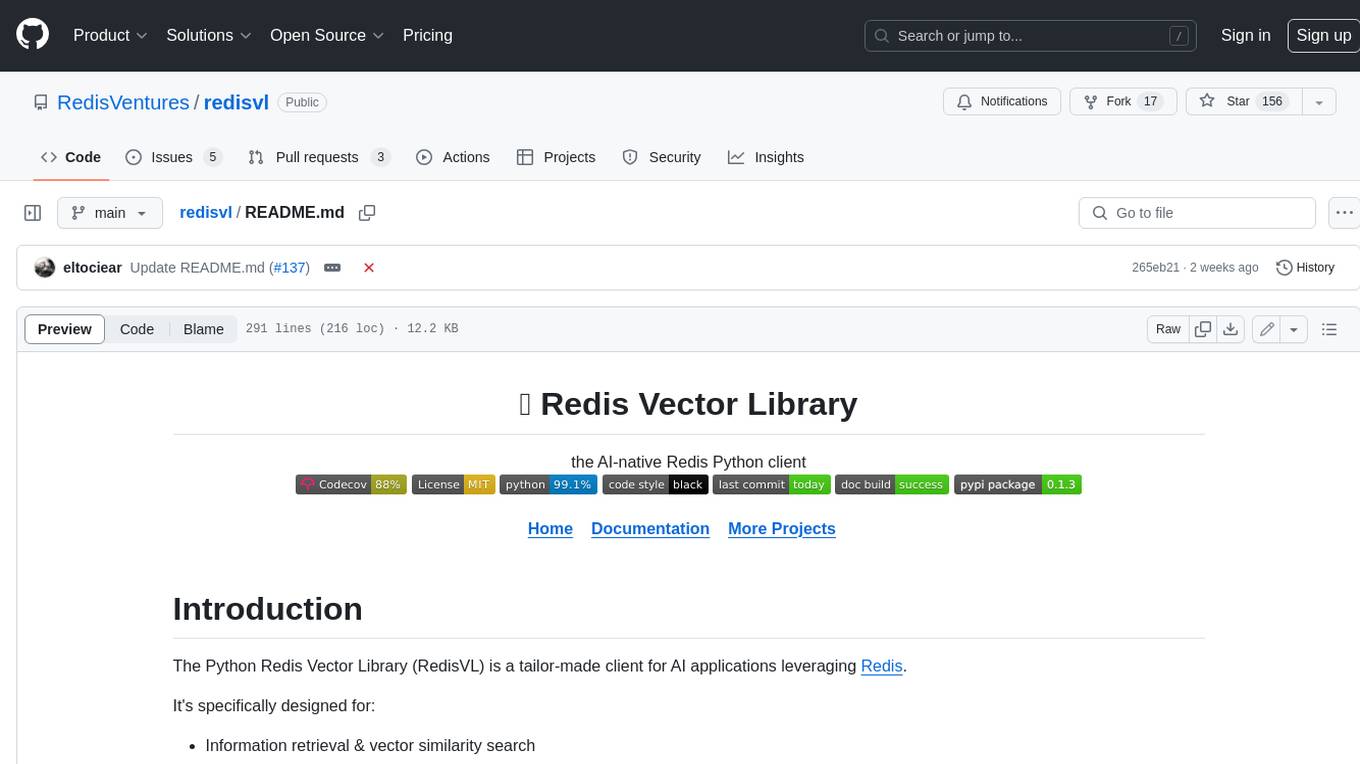
redisvl
Redis Vector Library (RedisVL) is a Python client library for building AI applications on top of Redis. It provides a high-level interface for managing vector indexes, performing vector search, and integrating with popular embedding models and providers. RedisVL is designed to make it easy for developers to build and deploy AI applications that leverage the speed, flexibility, and reliability of Redis.
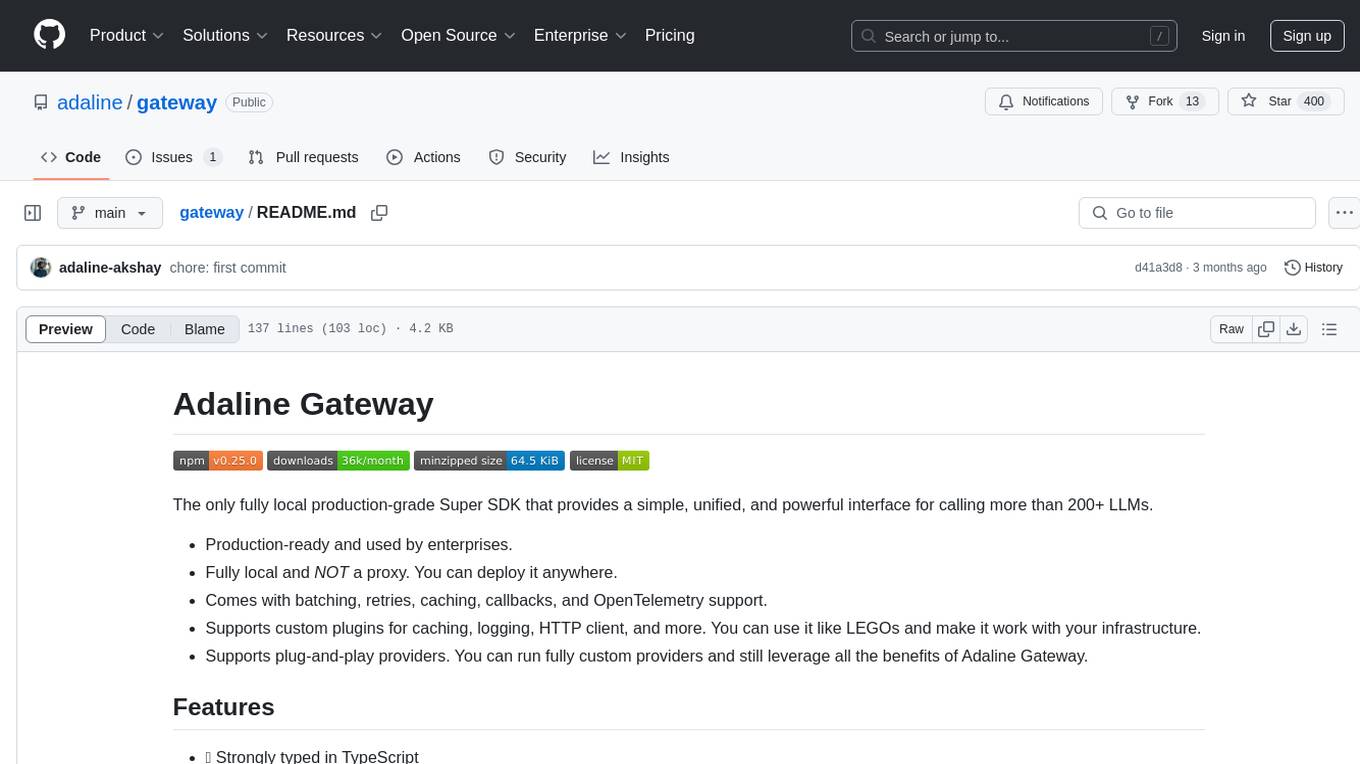
gateway
Adaline Gateway is a fully local production-grade Super SDK that offers a unified interface for calling over 200+ LLMs. It is production-ready, supports batching, retries, caching, callbacks, and OpenTelemetry. Users can create custom plugins and providers for seamless integration with their infrastructure.
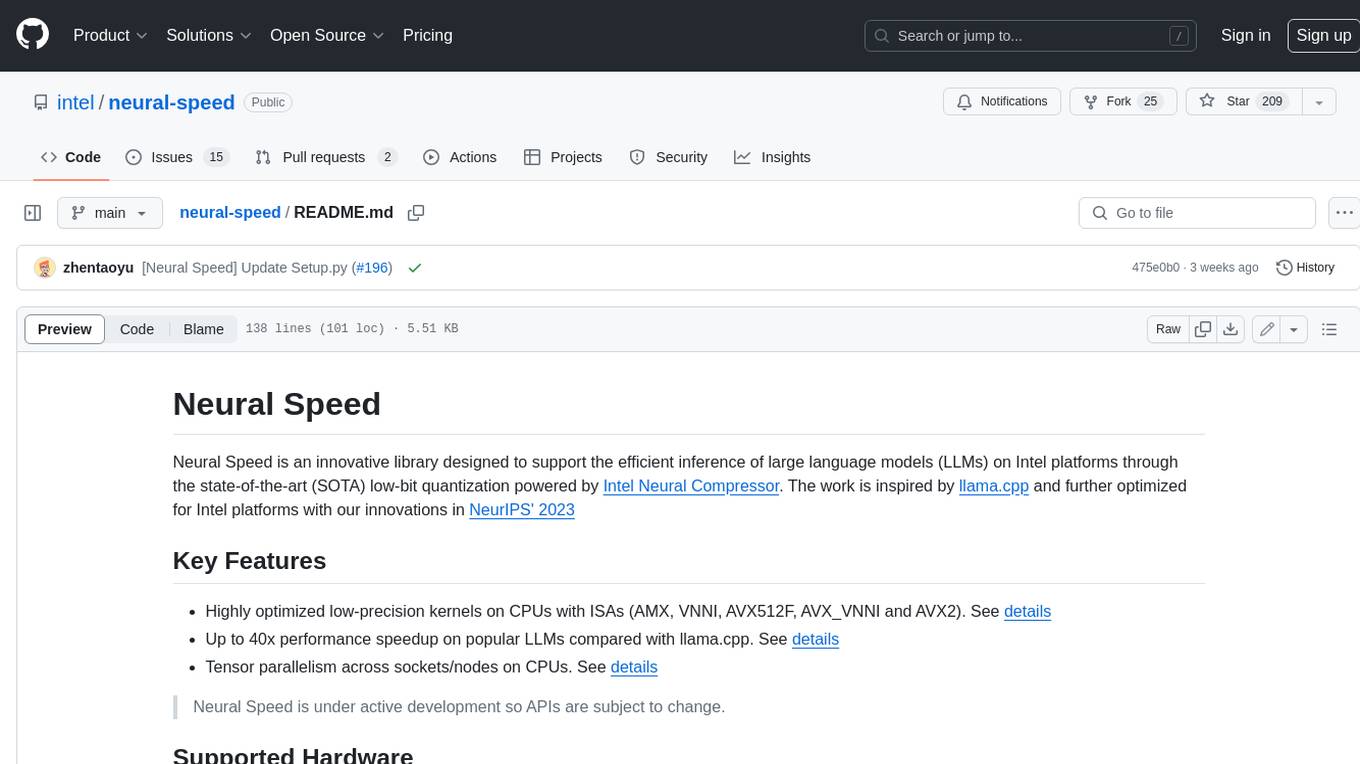
neural-speed
Neural Speed is an innovative library designed to support the efficient inference of large language models (LLMs) on Intel platforms through the state-of-the-art (SOTA) low-bit quantization powered by Intel Neural Compressor. The work is inspired by llama.cpp and further optimized for Intel platforms with our innovations in NeurIPS' 2023
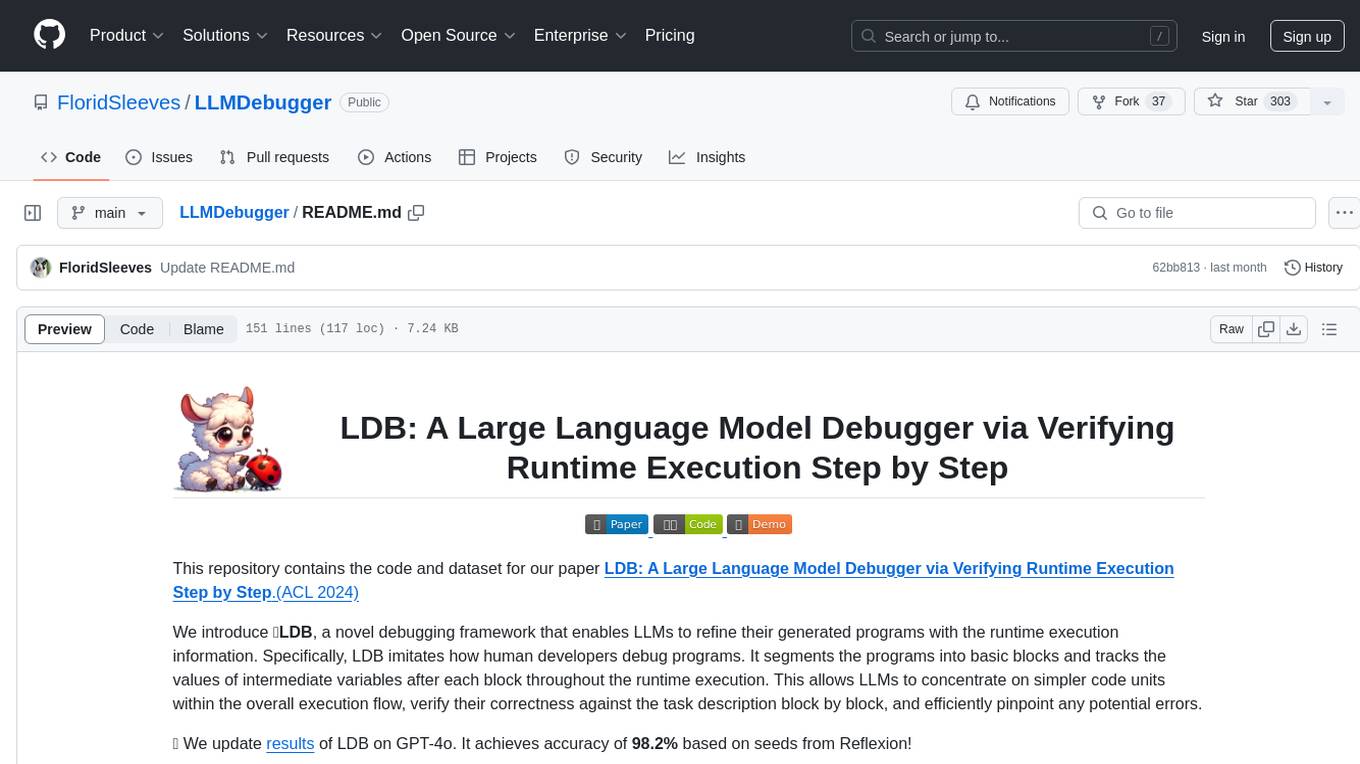
LLMDebugger
This repository contains the code and dataset for LDB, a novel debugging framework that enables Large Language Models (LLMs) to refine their generated programs by tracking the values of intermediate variables throughout the runtime execution. LDB segments programs into basic blocks, allowing LLMs to concentrate on simpler code units, verify correctness block by block, and pinpoint errors efficiently. The tool provides APIs for debugging and generating code with debugging messages, mimicking how human developers debug programs.
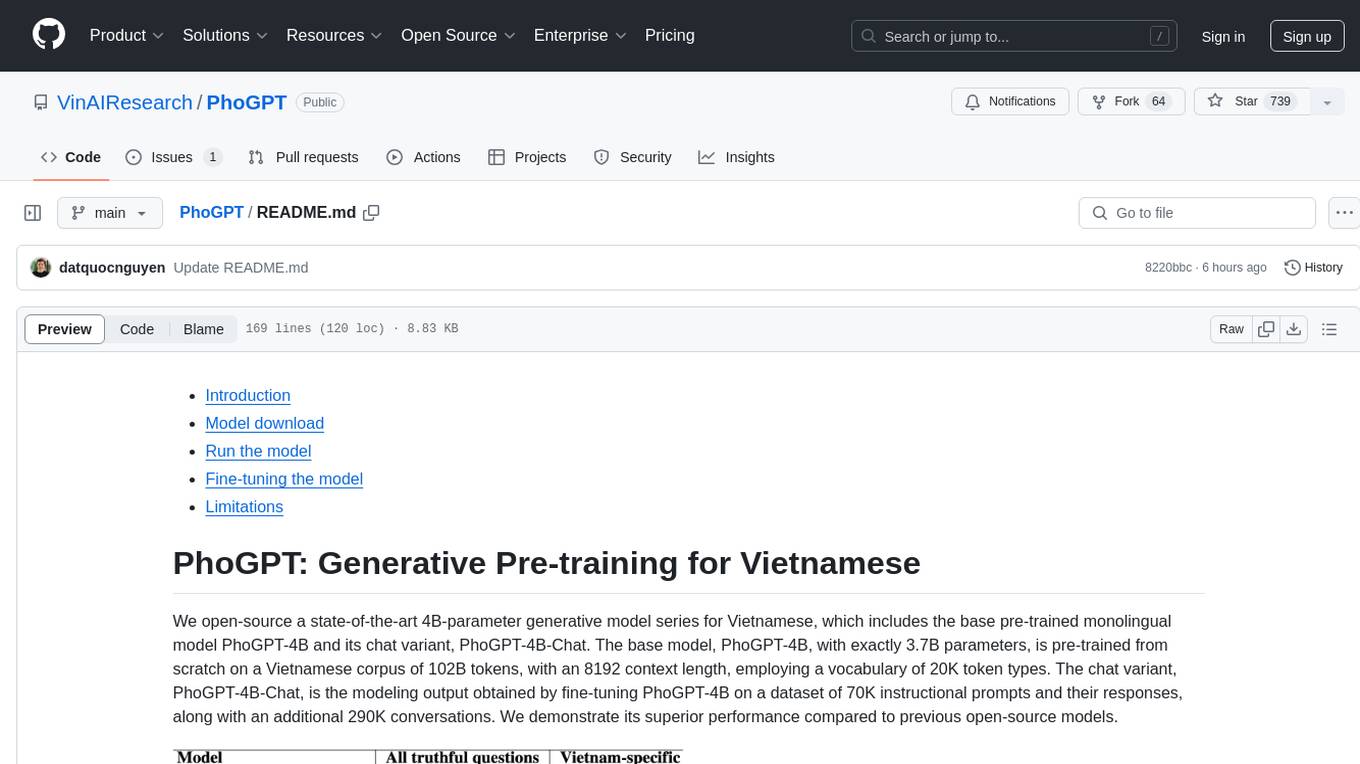
PhoGPT
PhoGPT is an open-source 4B-parameter generative model series for Vietnamese, including the base pre-trained monolingual model PhoGPT-4B and its chat variant, PhoGPT-4B-Chat. PhoGPT-4B is pre-trained from scratch on a Vietnamese corpus of 102B tokens, with an 8192 context length and a vocabulary of 20K token types. PhoGPT-4B-Chat is fine-tuned on instructional prompts and conversations, demonstrating superior performance. Users can run the model with inference engines like vLLM and Text Generation Inference, and fine-tune it using llm-foundry. However, PhoGPT has limitations in reasoning, coding, and mathematics tasks, and may generate harmful or biased responses.
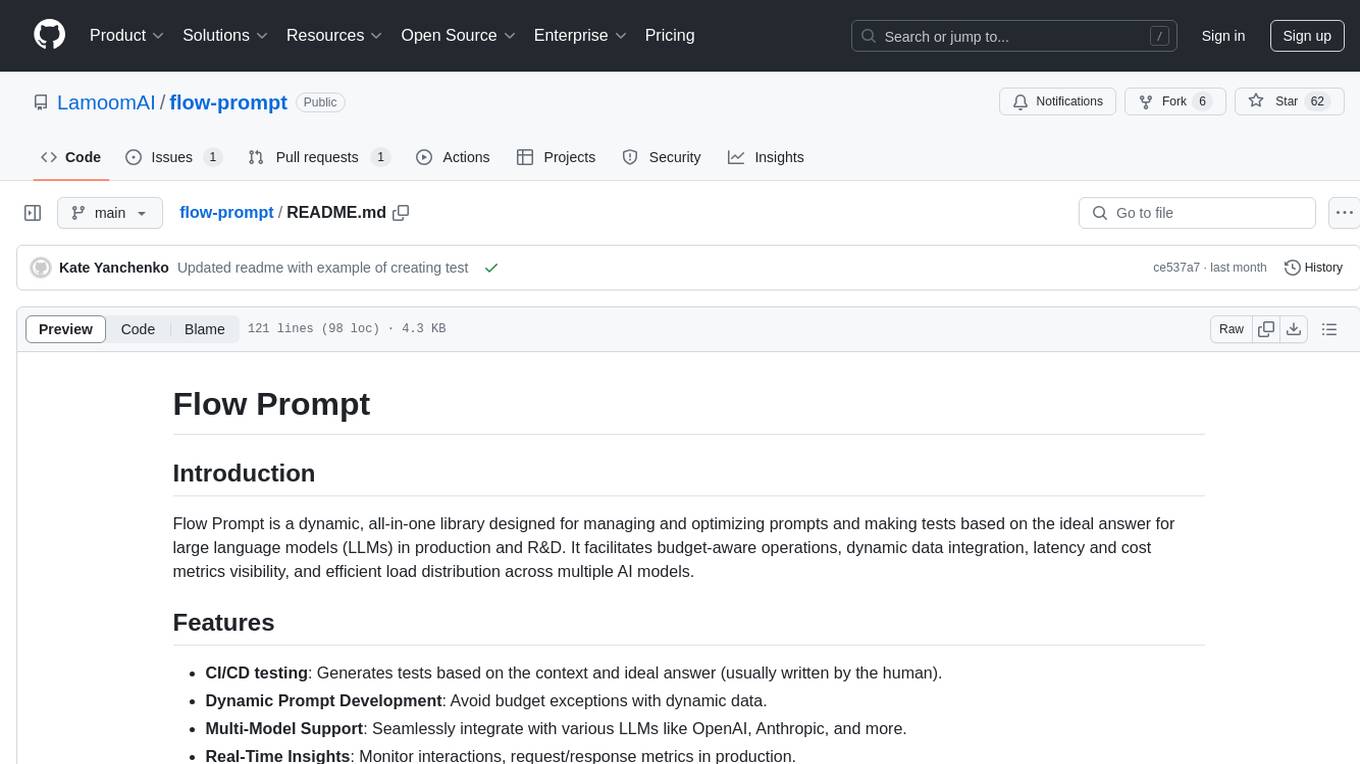
flow-prompt
Flow Prompt is a dynamic library for managing and optimizing prompts for large language models. It facilitates budget-aware operations, dynamic data integration, and efficient load distribution. Features include CI/CD testing, dynamic prompt development, multi-model support, real-time insights, and prompt testing and evolution.
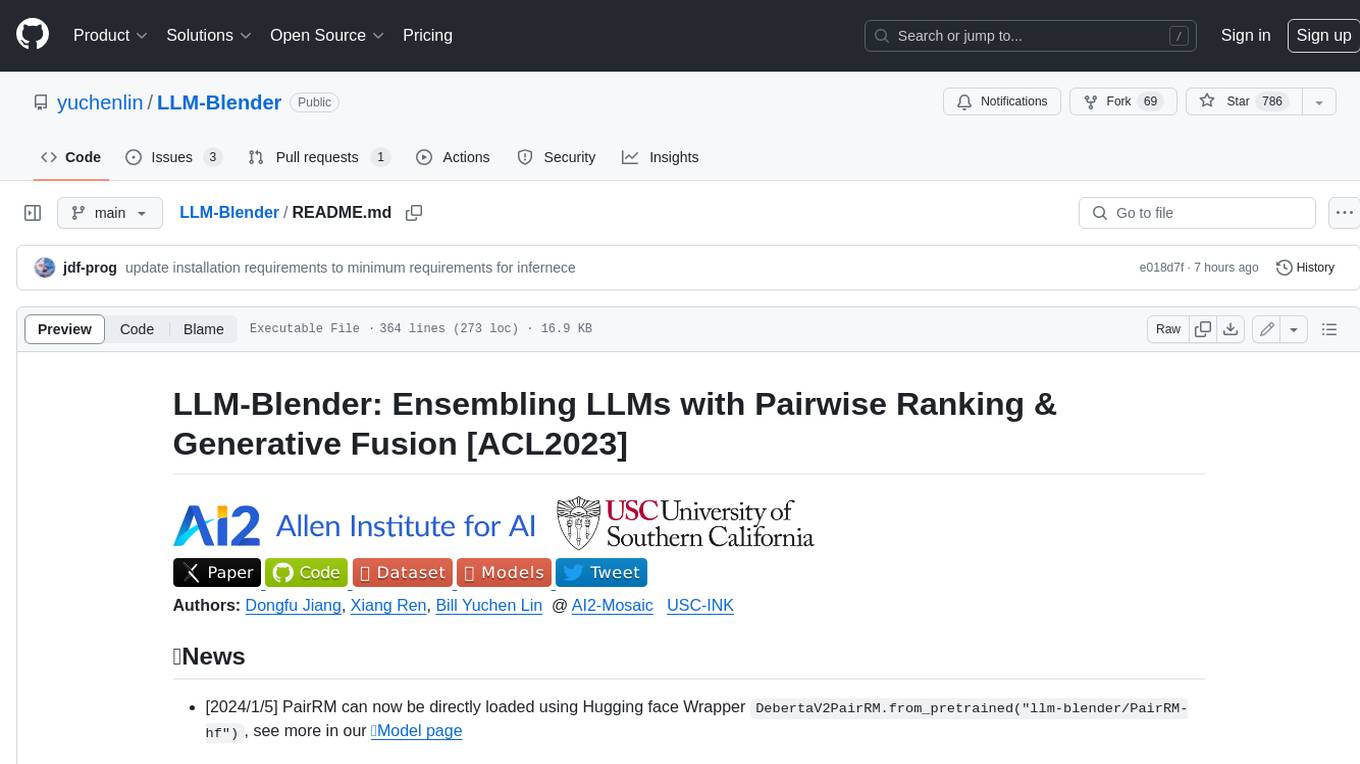
LLM-Blender
LLM-Blender is a framework for ensembling large language models (LLMs) to achieve superior performance. It consists of two modules: PairRanker and GenFuser. PairRanker uses pairwise comparisons to distinguish between candidate outputs, while GenFuser merges the top-ranked candidates to create an improved output. LLM-Blender has been shown to significantly surpass the best LLMs and baseline ensembling methods across various metrics on the MixInstruct benchmark dataset.
For similar tasks
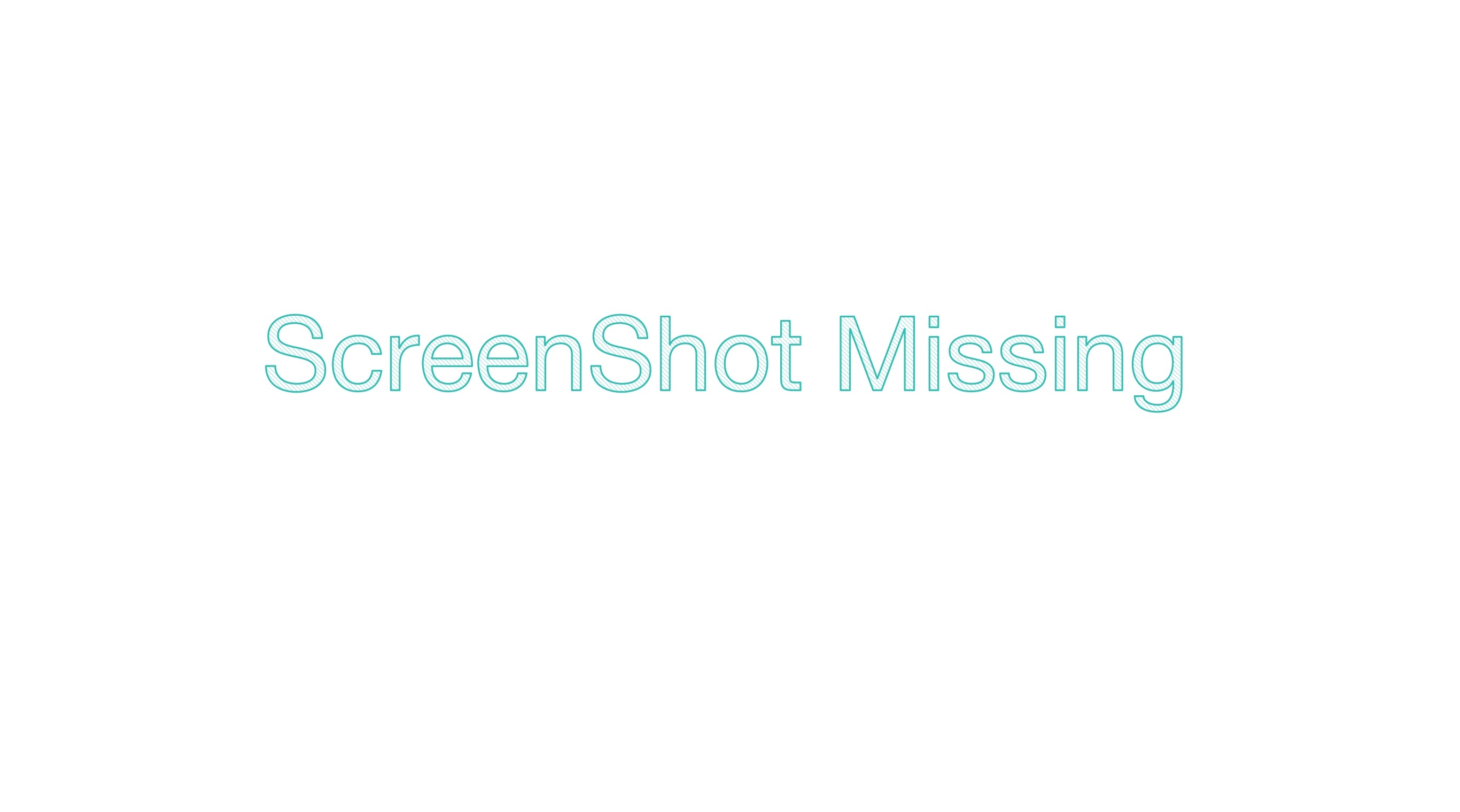
HippoRAG
HippoRAG is a novel retrieval augmented generation (RAG) framework inspired by the neurobiology of human long-term memory that enables Large Language Models (LLMs) to continuously integrate knowledge across external documents. It provides RAG systems with capabilities that usually require a costly and high-latency iterative LLM pipeline for only a fraction of the computational cost. The tool facilitates setting up retrieval corpus, indexing, and retrieval processes for LLMs, offering flexibility in choosing different online LLM APIs or offline LLM deployments through LangChain integration. Users can run retrieval on pre-defined queries or integrate directly with the HippoRAG API. The tool also supports reproducibility of experiments and provides data, baselines, and hyperparameter tuning scripts for research purposes.
For similar jobs
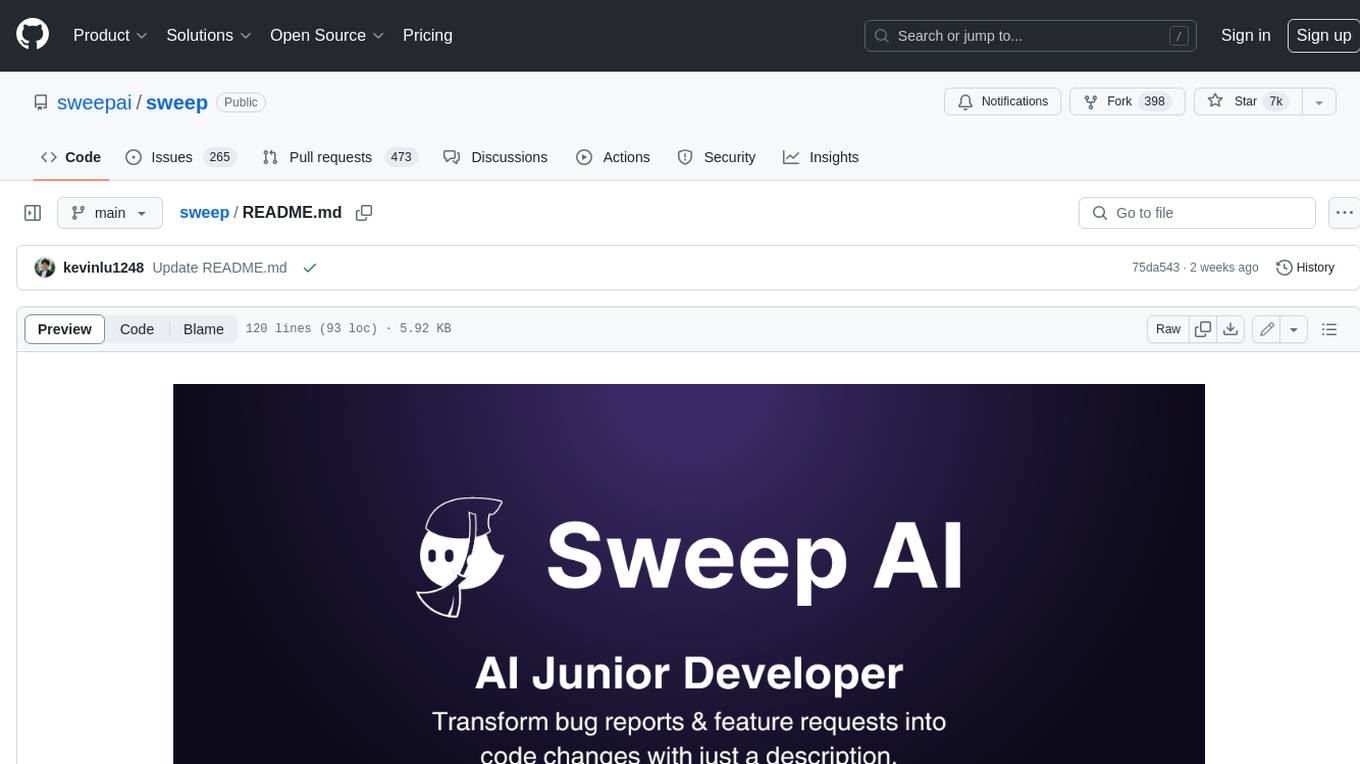
sweep
Sweep is an AI junior developer that turns bugs and feature requests into code changes. It automatically handles developer experience improvements like adding type hints and improving test coverage.
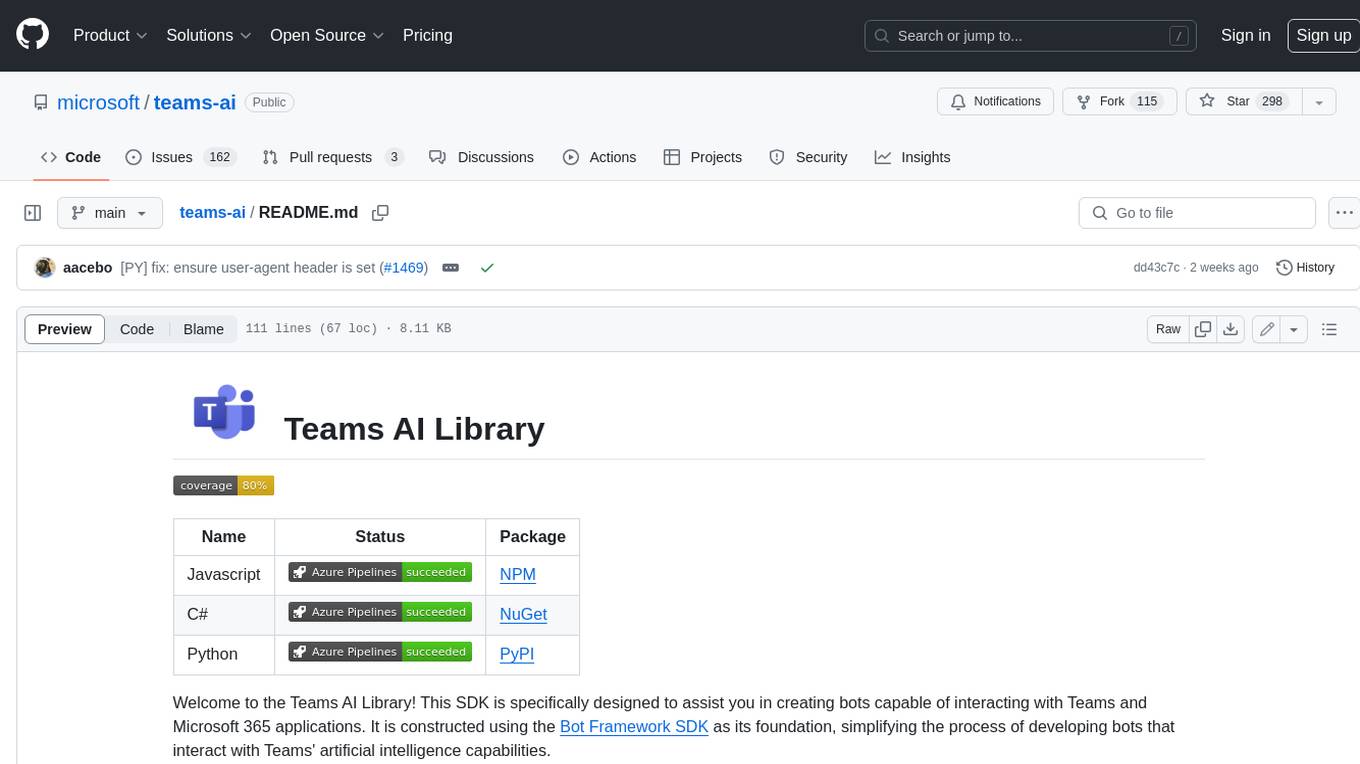
teams-ai
The Teams AI Library is a software development kit (SDK) that helps developers create bots that can interact with Teams and Microsoft 365 applications. It is built on top of the Bot Framework SDK and simplifies the process of developing bots that interact with Teams' artificial intelligence capabilities. The SDK is available for JavaScript/TypeScript, .NET, and Python.
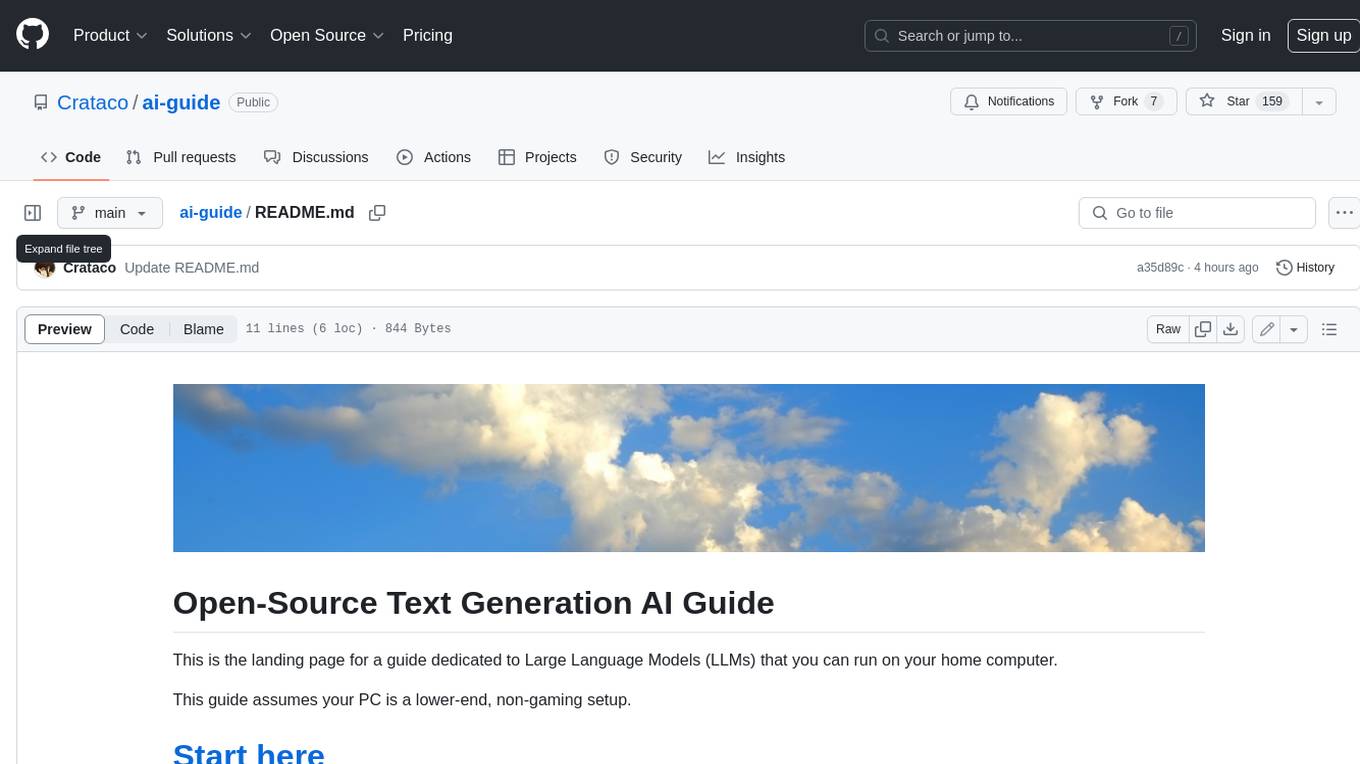
ai-guide
This guide is dedicated to Large Language Models (LLMs) that you can run on your home computer. It assumes your PC is a lower-end, non-gaming setup.
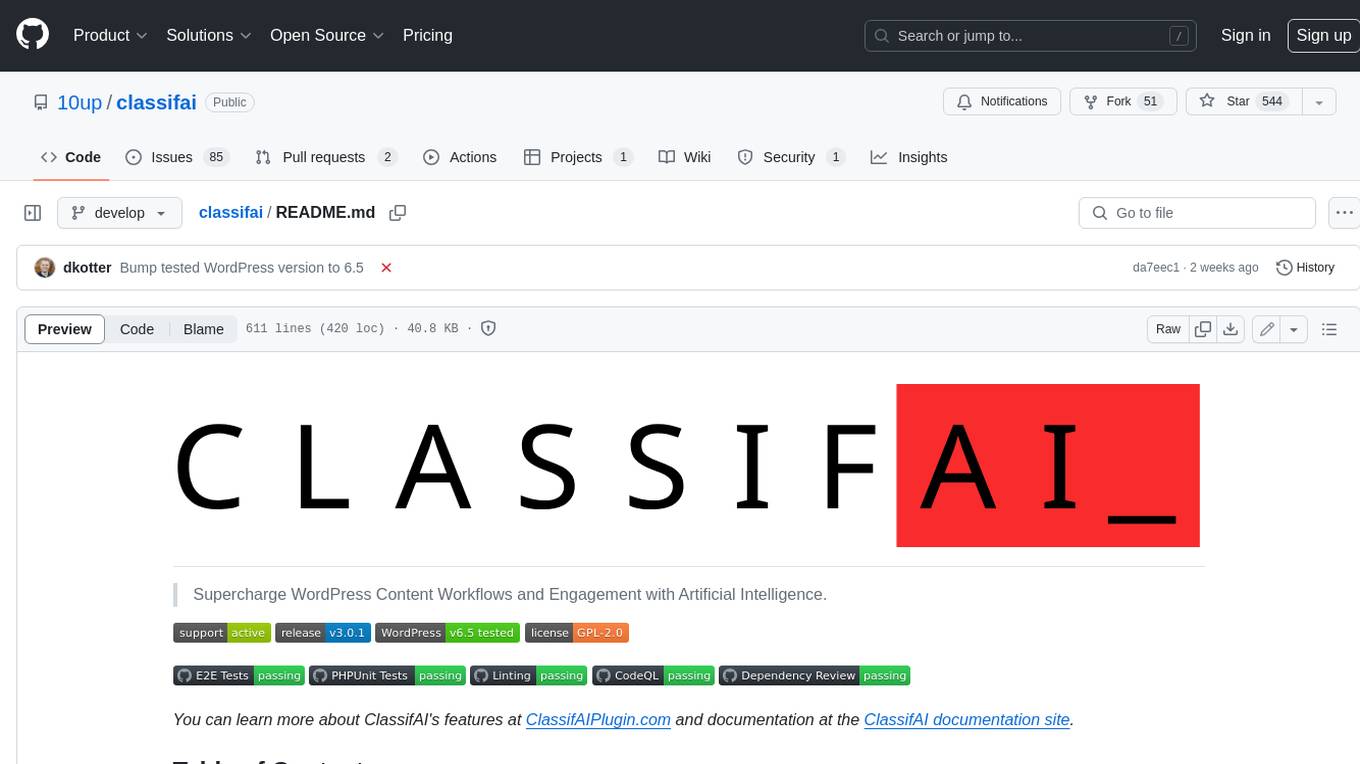
classifai
Supercharge WordPress Content Workflows and Engagement with Artificial Intelligence. Tap into leading cloud-based services like OpenAI, Microsoft Azure AI, Google Gemini and IBM Watson to augment your WordPress-powered websites. Publish content faster while improving SEO performance and increasing audience engagement. ClassifAI integrates Artificial Intelligence and Machine Learning technologies to lighten your workload and eliminate tedious tasks, giving you more time to create original content that matters.
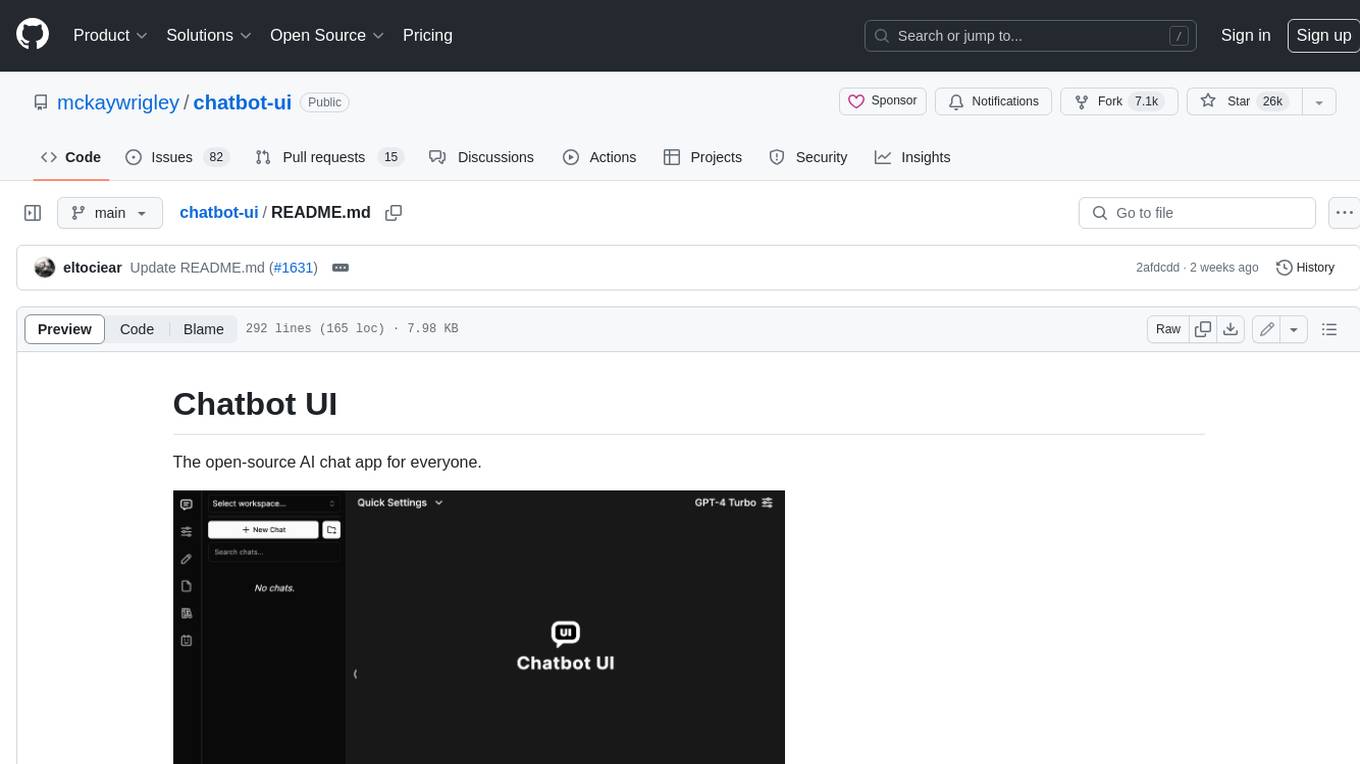
chatbot-ui
Chatbot UI is an open-source AI chat app that allows users to create and deploy their own AI chatbots. It is easy to use and can be customized to fit any need. Chatbot UI is perfect for businesses, developers, and anyone who wants to create a chatbot.
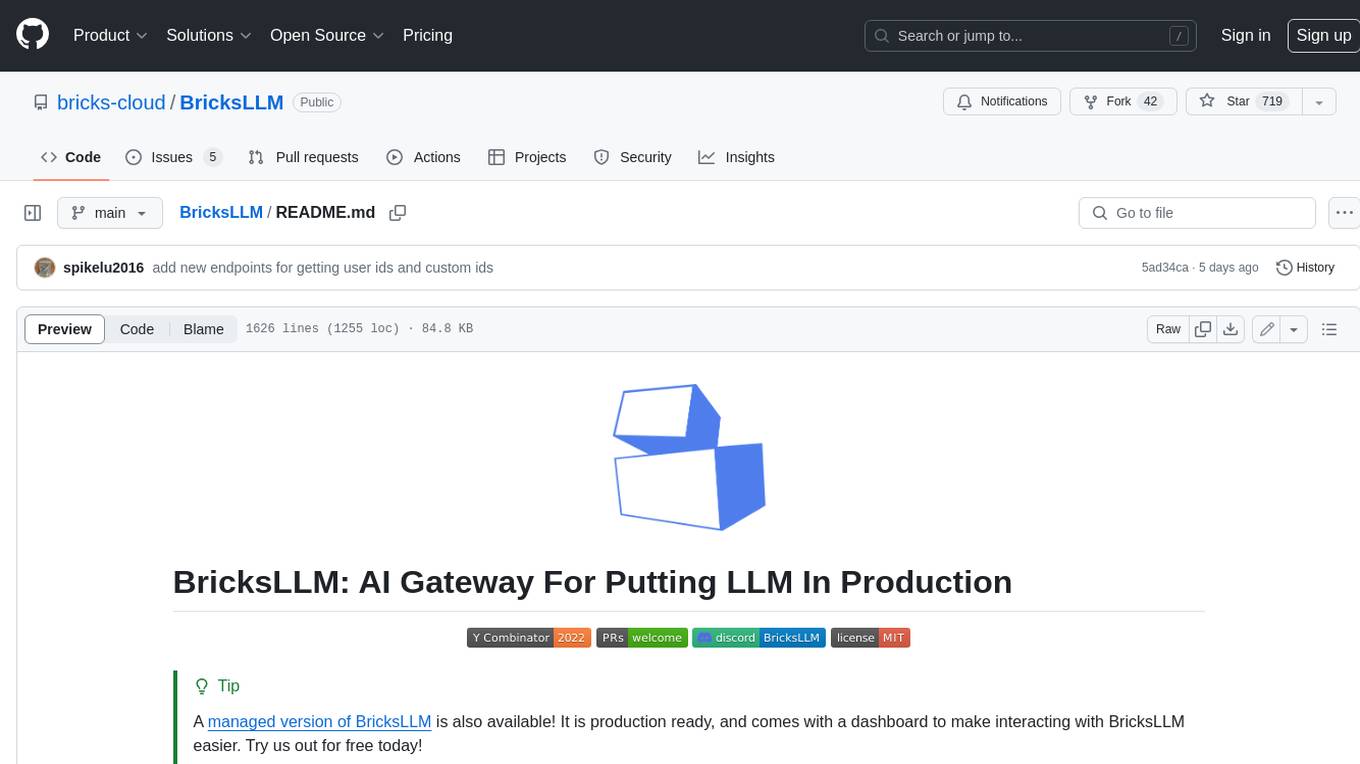
BricksLLM
BricksLLM is a cloud native AI gateway written in Go. Currently, it provides native support for OpenAI, Anthropic, Azure OpenAI and vLLM. BricksLLM aims to provide enterprise level infrastructure that can power any LLM production use cases. Here are some use cases for BricksLLM: * Set LLM usage limits for users on different pricing tiers * Track LLM usage on a per user and per organization basis * Block or redact requests containing PIIs * Improve LLM reliability with failovers, retries and caching * Distribute API keys with rate limits and cost limits for internal development/production use cases * Distribute API keys with rate limits and cost limits for students
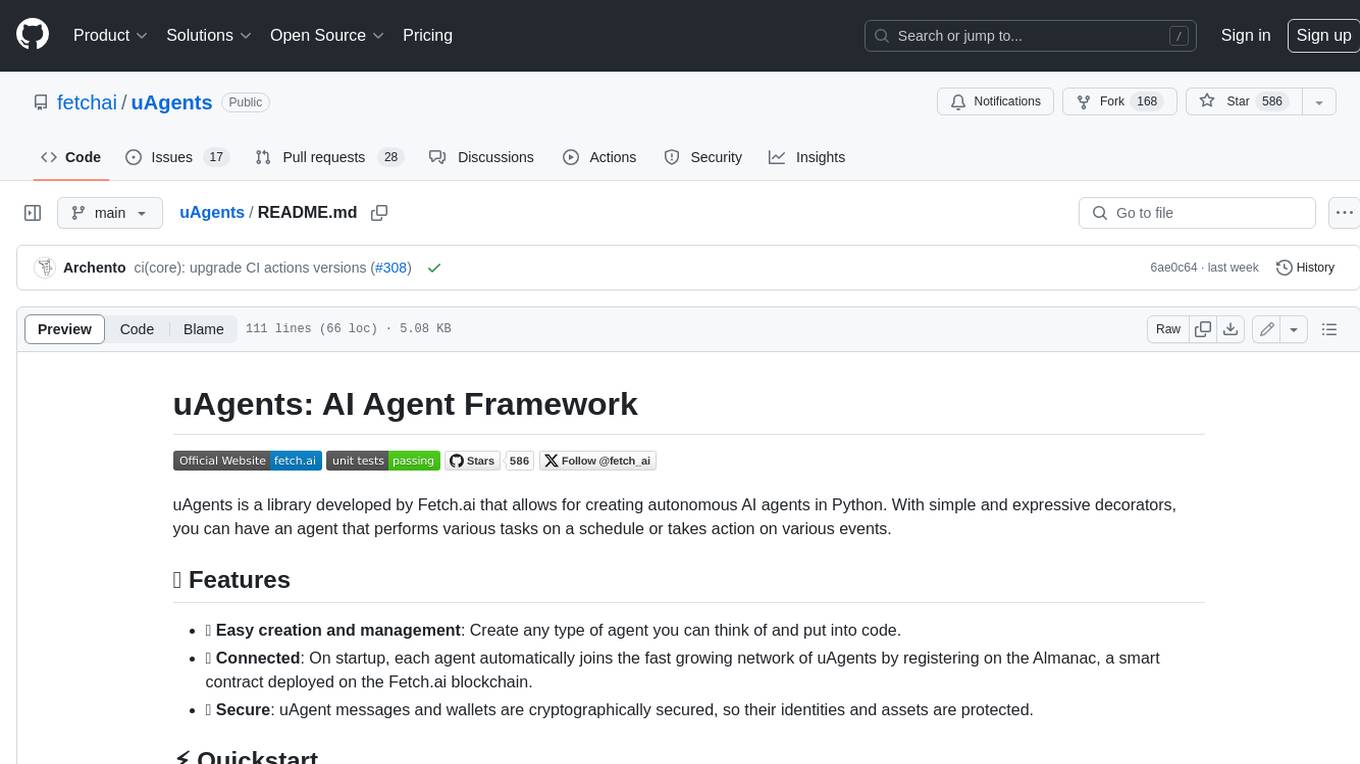
uAgents
uAgents is a Python library developed by Fetch.ai that allows for the creation of autonomous AI agents. These agents can perform various tasks on a schedule or take action on various events. uAgents are easy to create and manage, and they are connected to a fast-growing network of other uAgents. They are also secure, with cryptographically secured messages and wallets.
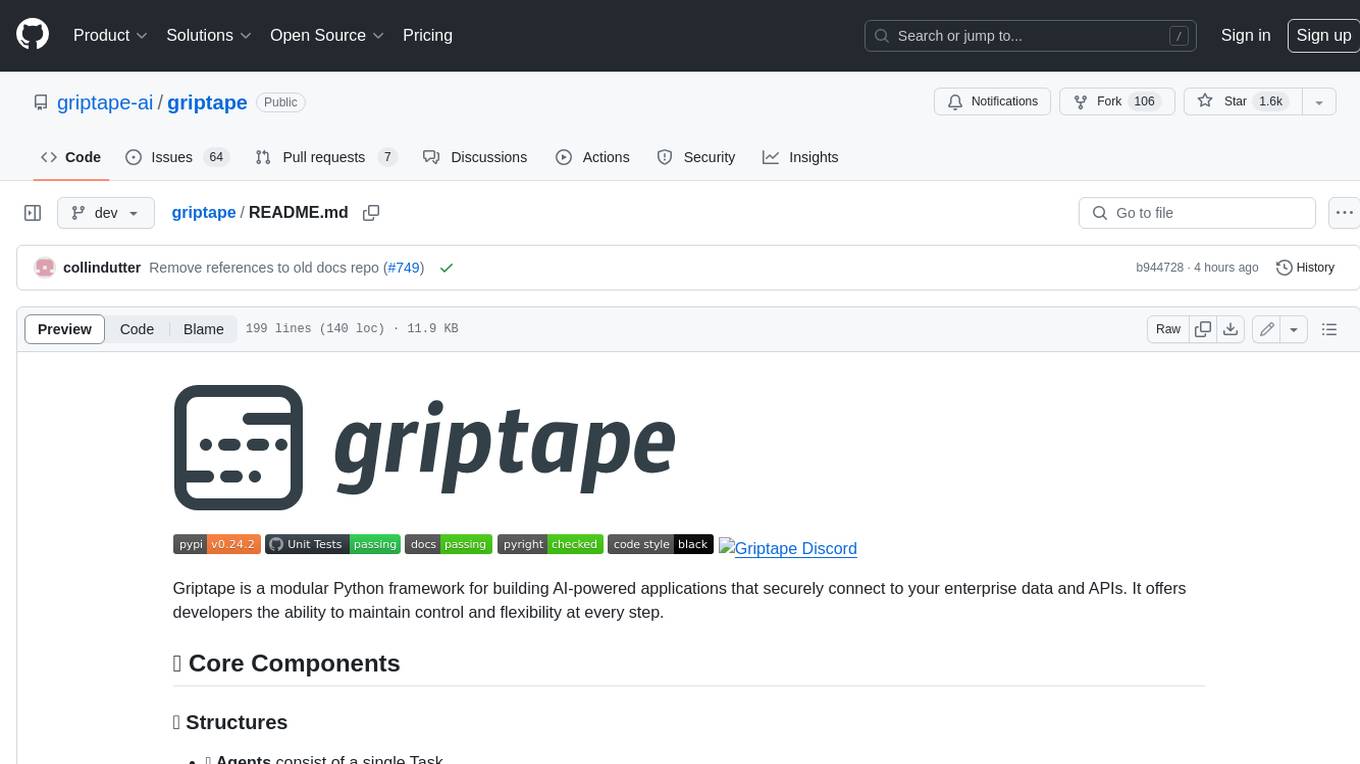
griptape
Griptape is a modular Python framework for building AI-powered applications that securely connect to your enterprise data and APIs. It offers developers the ability to maintain control and flexibility at every step. Griptape's core components include Structures (Agents, Pipelines, and Workflows), Tasks, Tools, Memory (Conversation Memory, Task Memory, and Meta Memory), Drivers (Prompt and Embedding Drivers, Vector Store Drivers, Image Generation Drivers, Image Query Drivers, SQL Drivers, Web Scraper Drivers, and Conversation Memory Drivers), Engines (Query Engines, Extraction Engines, Summary Engines, Image Generation Engines, and Image Query Engines), and additional components (Rulesets, Loaders, Artifacts, Chunkers, and Tokenizers). Griptape enables developers to create AI-powered applications with ease and efficiency.