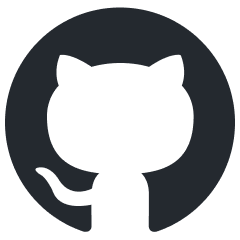
ModelCache
A LLM semantic caching system aiming to enhance user experience by reducing response time via cached query-result pairs.
Stars: 902
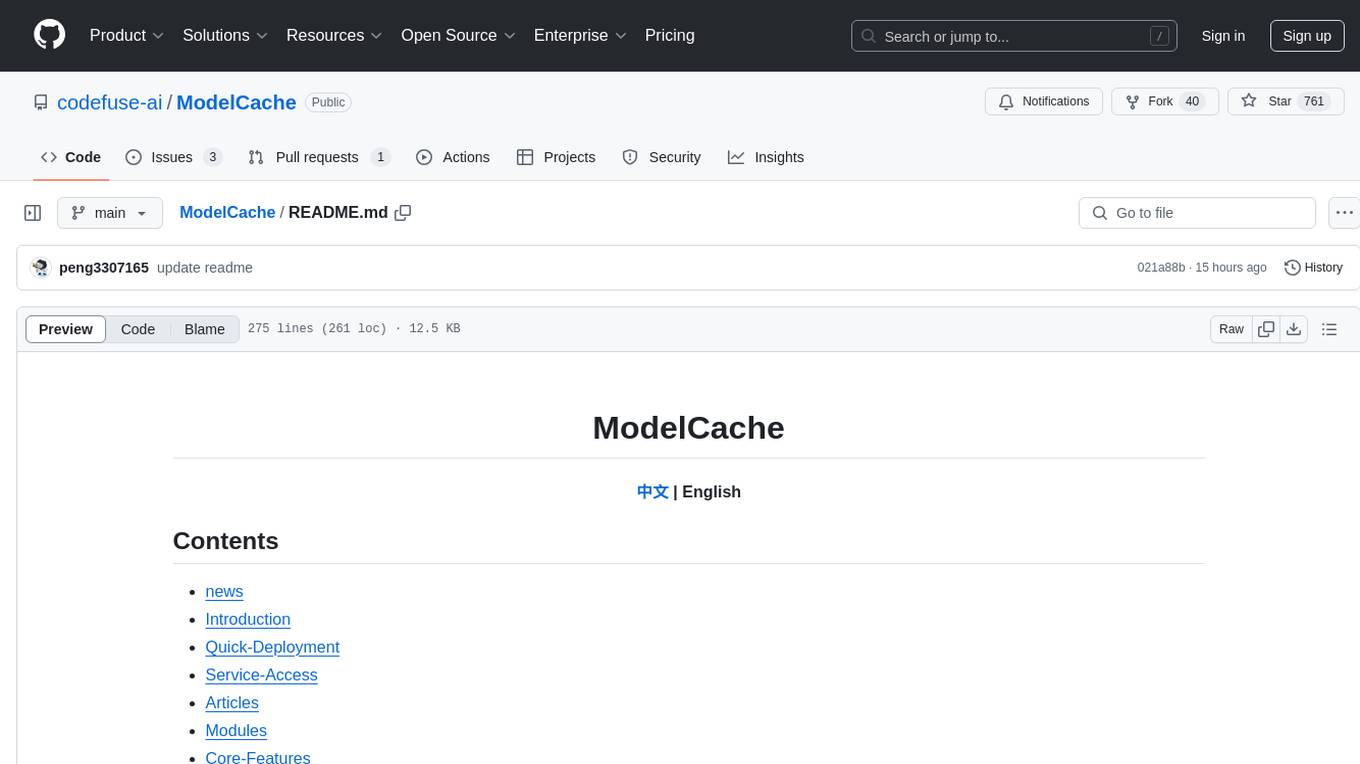
Codefuse-ModelCache is a semantic cache for large language models (LLMs) that aims to optimize services by introducing a caching mechanism. It helps reduce the cost of inference deployment, improve model performance and efficiency, and provide scalable services for large models. The project facilitates sharing and exchanging technologies related to large model semantic cache through open-source collaboration.
README:
δΈζ | English
- Contents
- News
- Architecture
- Quick start
- Visit the service
- Function comparison
- Features
- Todo List
- Acknowledgements
- Contributing
- π₯π₯[2024.10.22] Added tasks for 1024 developer day.
- π₯π₯[2024.04.09] Added Redis Search to store and retrieve embeddings in multi-tenant. This can reduce the interaction time between Cache and vector databases to 10ms.
- π₯π₯[2023.12.10] Integrated LLM embedding frameworks such as 'llmEmb', 'ONNX', 'PaddleNLP', 'FastText', and the image embedding framework 'timm' to bolster embedding functionality.
- π₯π₯[2023.11.20] Integrated local storage, such as sqlite and faiss. This enables you to initiate quick and convenient tests.
- [2023.08.26] codefuse-ModelCache...
Codefuse-ModelCache is a semantic cache for large language models (LLMs). By caching pre-generated model results, it reduces response time for similar requests and improves user experience.
This project aims to optimize services by introducing a caching mechanism. It helps businesses and research institutions reduce the cost of inference deployment, improve model performance and efficiency, and provide scalable services for large models. Through open-source, we aim to share and exchange technologies related to large model semantic cache.
You can find the start script in flask4modelcache.py
and flask4modelcache_demo.py
.
-
flask4modelcache_demo.py
: A quick test service that embeds SQLite and FAISS. No database configuration required. -
flask4modelcache.py
: The standard service that requires MySQL and Milvus configuration.
-
Python: V3.8 or above
-
Package installation
pip install -r requirements.txt
- Download the embedding model bin file from Hugging Face. Place it in the
model/text2vec-base-chinese
folder. - Start the backend service:
cd CodeFuse-ModelCache
python flask4modelcache_demo.py
Before you start standard service, do these steps:
-
Install MySQL and import the SQL file from
reference_doc/create_table.sql
. -
Install vector database Milvus.
-
Configure database access in:
modelcache/config/milvus_config.ini
modelcache/config/mysql_config.ini
-
Download the embedding model bin file from Hugging Face. Put it in
model/text2vec-base-chinese
. -
Start the backend service:
python flask4modelcache.py
The service provides three core RESTful API functionalities: Cache-Writing, Cache-Querying, and Cache-Clearing.
import json
import requests
url = 'http://127.0.0.1:5000/modelcache'
type = 'insert'
scope = {"model": "CODEGPT-1008"}
chat_info = [{"query": [{"role": "system", "content": "You are an AI code assistant and you must provide neutral and harmless answers to help users solve code-related problems."}, {"role": "user", "content": "δ½ ζ―θ°?"}],
"answer": "Hello, I am an intelligent assistant. How can I assist you?"}]
data = {'type': type, 'scope': scope, 'chat_info': chat_info}
headers = {"Content-Type": "application/json"}
res = requests.post(url, headers=headers, json=json.dumps(data))
import json
import requests
url = 'http://127.0.0.1:5000/modelcache'
type = 'query'
scope = {"model": "CODEGPT-1008"}
query = [{"role": "system", "content": "You are an AI code assistant and you must provide neutral and harmless answers to help users solve code-related problems."}, {"role": "user", "content": "Who are you?"}]
data = {'type': type, 'scope': scope, 'query': query}
headers = {"Content-Type": "application/json"}
res = requests.post(url, headers=headers, json=json.dumps(data))
import json
import requests
url = 'http://127.0.0.1:5000/modelcache'
type = 'remove'
scope = {"model": "CODEGPT-1008"}
remove_type = 'truncate_by_model'
data = {'type': type, 'scope': scope, 'remove_type': remove_type}
headers = {"Content-Type": "application/json"}
res = requests.post(url, headers=headers, json=json.dumps(data))
We've implemented several key updates to our repository. We've resolved network issues with Hugging Face and improved inference speed by introducing local embedding capabilities. Due to limitations in SqlAlchemy, we've redesigned our relational database interaction module for more flexible operations. We've added multi-tenancy support to ModelCache, recognizing the need for multiple users and models in LLM products. Lastly, we've made initial adjustments for better compatibility with system commands and multi-turn dialogues.
Module | Function | ||
---|---|---|---|
ModelCache | GPTCache | ||
Basic Interface | Data query interface | β | β |
Data writing interface | β | β | |
Embedding | Embedding model configuration | β | β |
Large model embedding layer | β | ||
BERT model long text processing | β | ||
Large model invocation | Decoupling from large models | β | |
Local loading of embedding model | β | ||
Data isolation | Model data isolation | β | β |
Hyperparameter isolation | |||
Databases | MySQL | β | β |
Milvus | β | β | |
OceanBase | β | ||
Session management | Single-turn dialogue | β | β |
System commands | β | ||
Multi-turn dialogue | β | ||
Data management | Data persistence | β | β |
One-click cache clearance | β | ||
Tenant management | Support for multi-tenancy | β | |
Milvus multi-collection capability | β | ||
Other | Long-short dialogue distinction | β |
In ModelCache, we incorporated the core principles of GPTCache. ModelCache has four modules: adapter, embedding, similarity, and data_manager.
- The adapter module orchestrates the business logic for various tasks, integrate the embedding, similarity, and data_manager modules.
- The embedding module converts text into semantic vector representations, and transforms user queries into vectors.
- The rank module ranks and evaluate the similarity of recalled vectors.
- The data_manager module manages the databases.
To make ModelCache more suitable for industrial use, we made several improvements to its architecture and functionality:
- [x] Architectural adjustment (lightweight integration):
- Embedded into LLM products using a Redis-like caching mode
- Provided semantic caching without interfering with LLM calls, security audits, and other functions
- Compatible with all LLM services
- [x] Multiple model loading:
- Supported local embedding model loading, and resolved Hugging Face network connectivity issues
- Supported loading embedding layers from various pre-trained models
- [x] Data isolation
- Environment isolation: Read different database configurations based on the environment. Isolate development, staging, and production environments.
- Multi-tenant data isolation: Dynamically create collections based on models for data isolation, addressing data separation issues in multi-model/service scenarios within large language model products
- [x] Supported system instruction: Adopted a concatenation approach to resolve issues with system instructions in the prompt paradigm.
- [x] Long and short text differentiation: Long texts bring more challenges for similarity assessment. Added differentiation between long and short texts, allowing for separate threshold configurations.
- [x] Milvus performance optimization: Adjusted Milvus consistency level to "Session" level for better performance.
- [x] Data management:
- One-click cache clearing to enable easy data management after model upgrades.
- Recall of hit queries for subsequent data analysis and model iteration reference.
- Asynchronous log write-back for data analysis and statistics
- Added model field and data statistics field to enhance features
- [ ] Register adapter for MilvusοΌBased on the "model" parameter in the scope, initialize the corresponding Collection and perform the load operation.
- [ ] Inference Optimization: Optimizing the speed of embedding inference, compatible with inference engines such as FasterTransformer, TurboTransformers, and ByteTransformer.
- [ ] Compatibility with Hugging Face models and ModelScope models, offering more methods for model loading.
- [ ] Support MongoDB
- [ ] Support ElasticSearch
- [ ] Adapts Faiss storage in multimodal scenarios.
- [ ] Add ranking model to refine the order of data after embedding recall.
- [ ] Supports FastAPI.
- [ ] Add visual interface to offer a more direct user experience.
This project has referenced the following open-source projects. We would like to express our gratitude to the projects and their developers for their contributions and research.
GPTCache
ModelCache is a captivating and invaluable project, whether you are an experienced developer or a novice just starting out, your contributions to this project are warmly welcomed. Your involvement in this project, be it through raising issues, providing suggestions, writing code, or documenting and creating examples, will enhance the project's quality and make a significant contribution to the open-source community.
For Tasks:
Click tags to check more tools for each tasksFor Jobs:
Alternative AI tools for ModelCache
Similar Open Source Tools
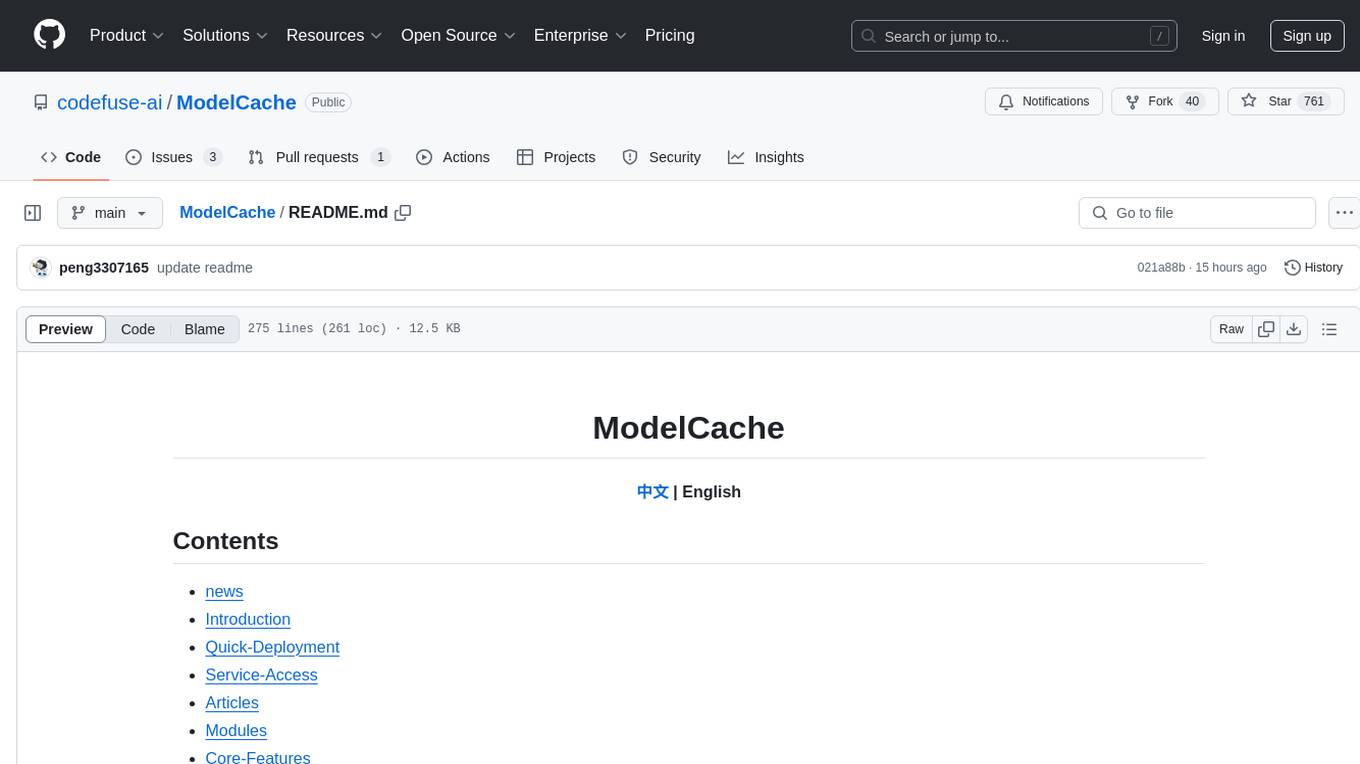
ModelCache
Codefuse-ModelCache is a semantic cache for large language models (LLMs) that aims to optimize services by introducing a caching mechanism. It helps reduce the cost of inference deployment, improve model performance and efficiency, and provide scalable services for large models. The project facilitates sharing and exchanging technologies related to large model semantic cache through open-source collaboration.
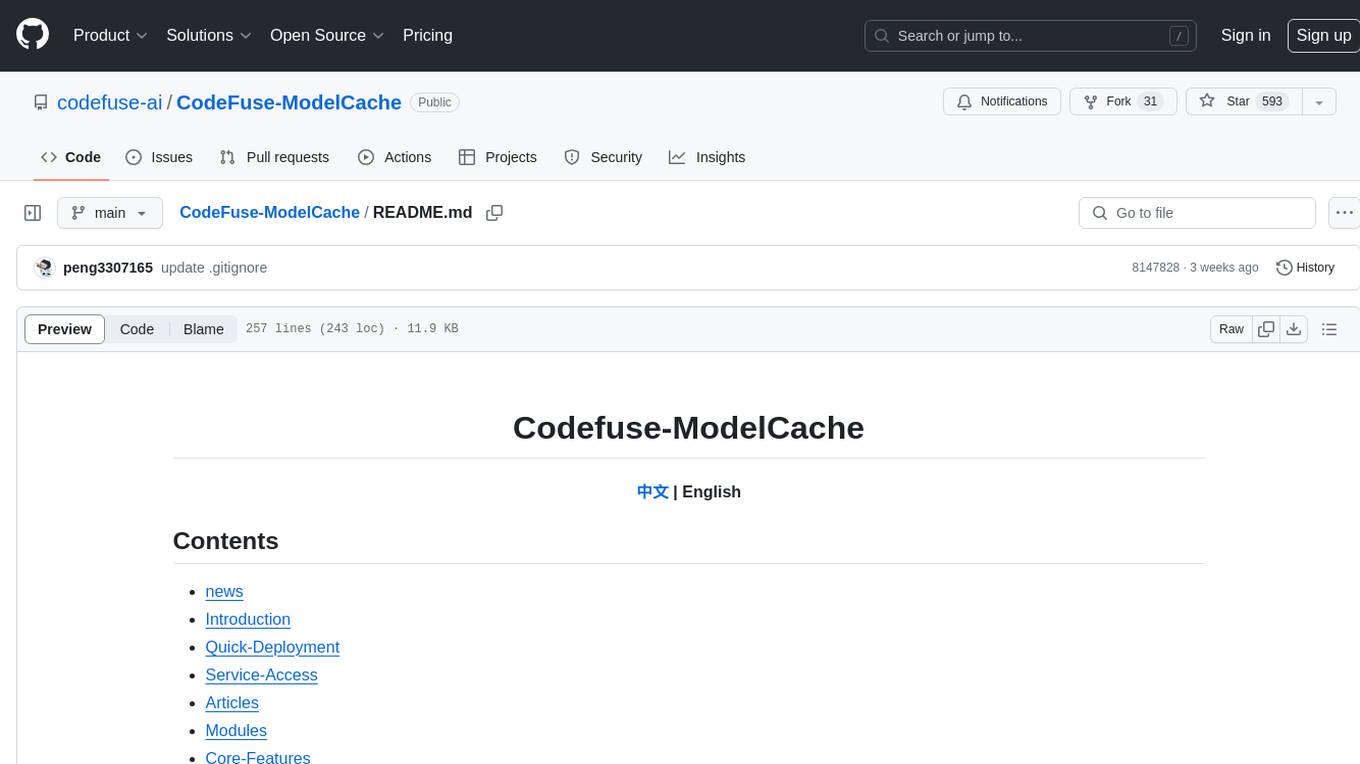
CodeFuse-ModelCache
Codefuse-ModelCache is a semantic cache for large language models (LLMs) that aims to optimize services by introducing a caching mechanism. It helps reduce the cost of inference deployment, improve model performance and efficiency, and provide scalable services for large models. The project caches pre-generated model results to reduce response time for similar requests and enhance user experience. It integrates various embedding frameworks and local storage options, offering functionalities like cache-writing, cache-querying, and cache-clearing through RESTful API. The tool supports multi-tenancy, system commands, and multi-turn dialogue, with features for data isolation, database management, and model loading schemes. Future developments include data isolation based on hyperparameters, enhanced system prompt partitioning storage, and more versatile embedding models and similarity evaluation algorithms.
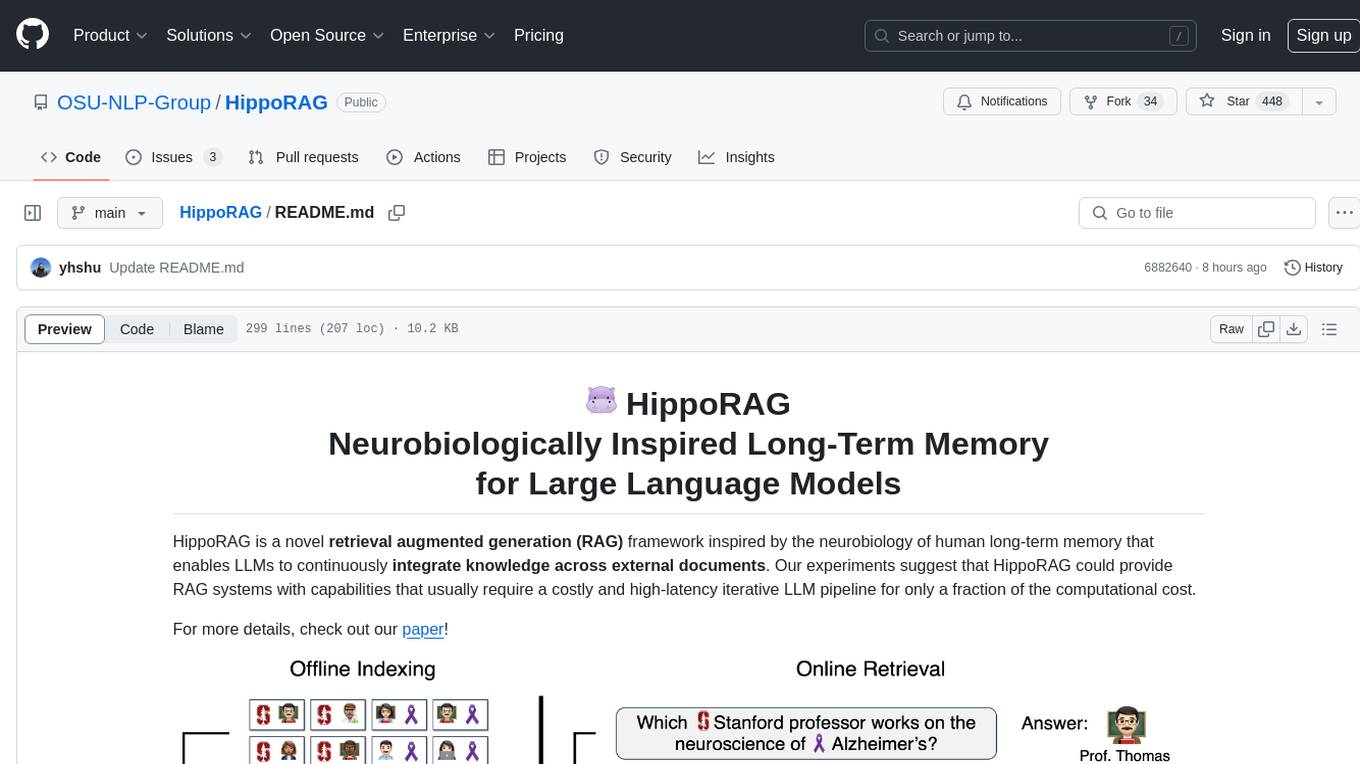
HippoRAG
HippoRAG is a novel retrieval augmented generation (RAG) framework inspired by the neurobiology of human long-term memory that enables Large Language Models (LLMs) to continuously integrate knowledge across external documents. It provides RAG systems with capabilities that usually require a costly and high-latency iterative LLM pipeline for only a fraction of the computational cost. The tool facilitates setting up retrieval corpus, indexing, and retrieval processes for LLMs, offering flexibility in choosing different online LLM APIs or offline LLM deployments through LangChain integration. Users can run retrieval on pre-defined queries or integrate directly with the HippoRAG API. The tool also supports reproducibility of experiments and provides data, baselines, and hyperparameter tuning scripts for research purposes.
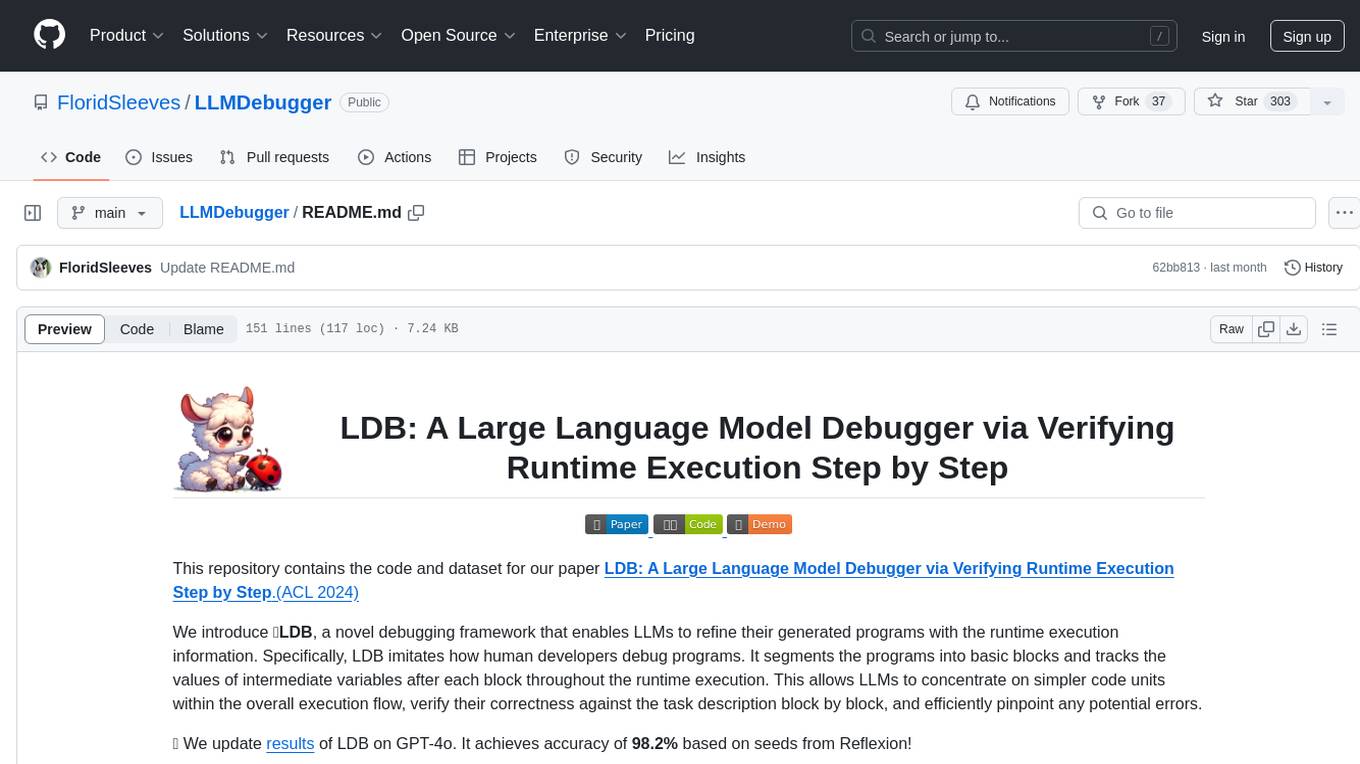
LLMDebugger
This repository contains the code and dataset for LDB, a novel debugging framework that enables Large Language Models (LLMs) to refine their generated programs by tracking the values of intermediate variables throughout the runtime execution. LDB segments programs into basic blocks, allowing LLMs to concentrate on simpler code units, verify correctness block by block, and pinpoint errors efficiently. The tool provides APIs for debugging and generating code with debugging messages, mimicking how human developers debug programs.
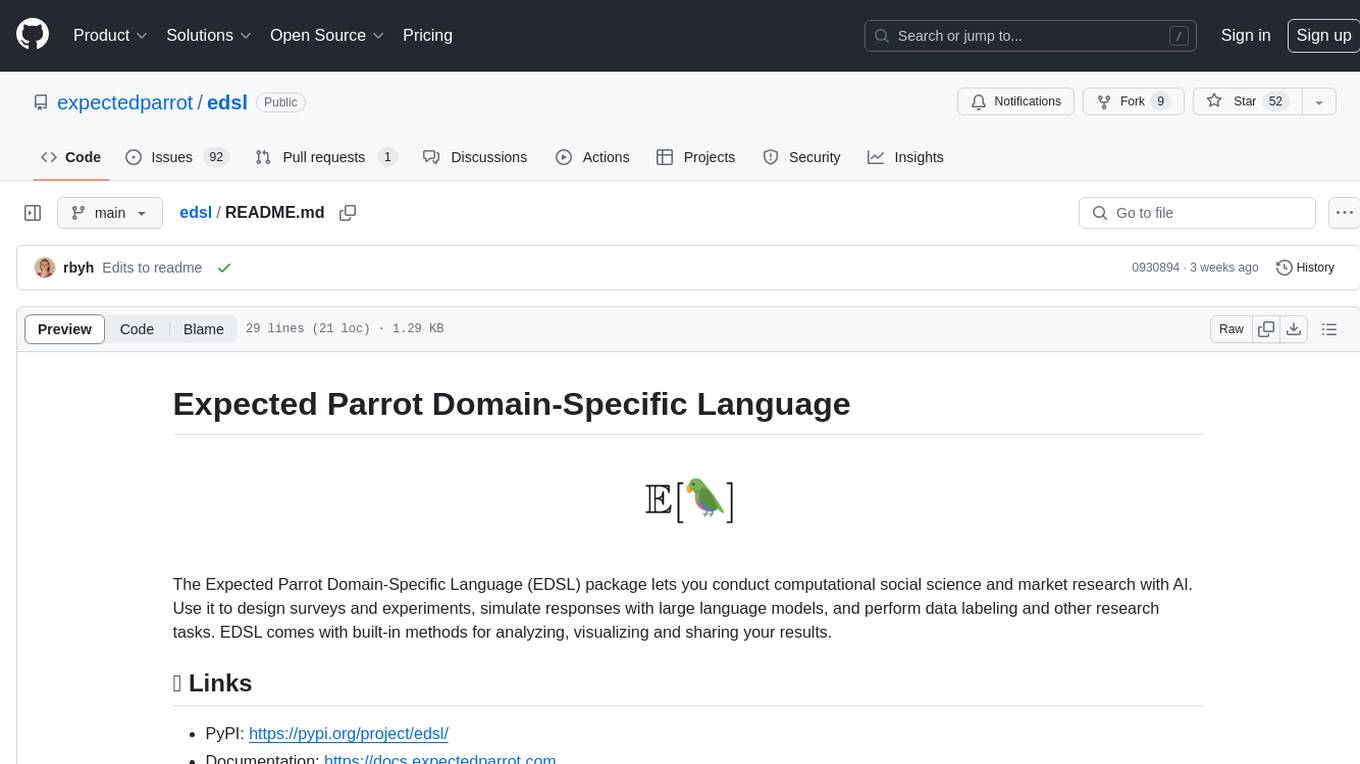
edsl
The Expected Parrot Domain-Specific Language (EDSL) package enables users to conduct computational social science and market research with AI. It facilitates designing surveys and experiments, simulating responses using large language models, and performing data labeling and other research tasks. EDSL includes built-in methods for analyzing, visualizing, and sharing research results. It is compatible with Python 3.9 - 3.11 and requires API keys for LLMs stored in a `.env` file.
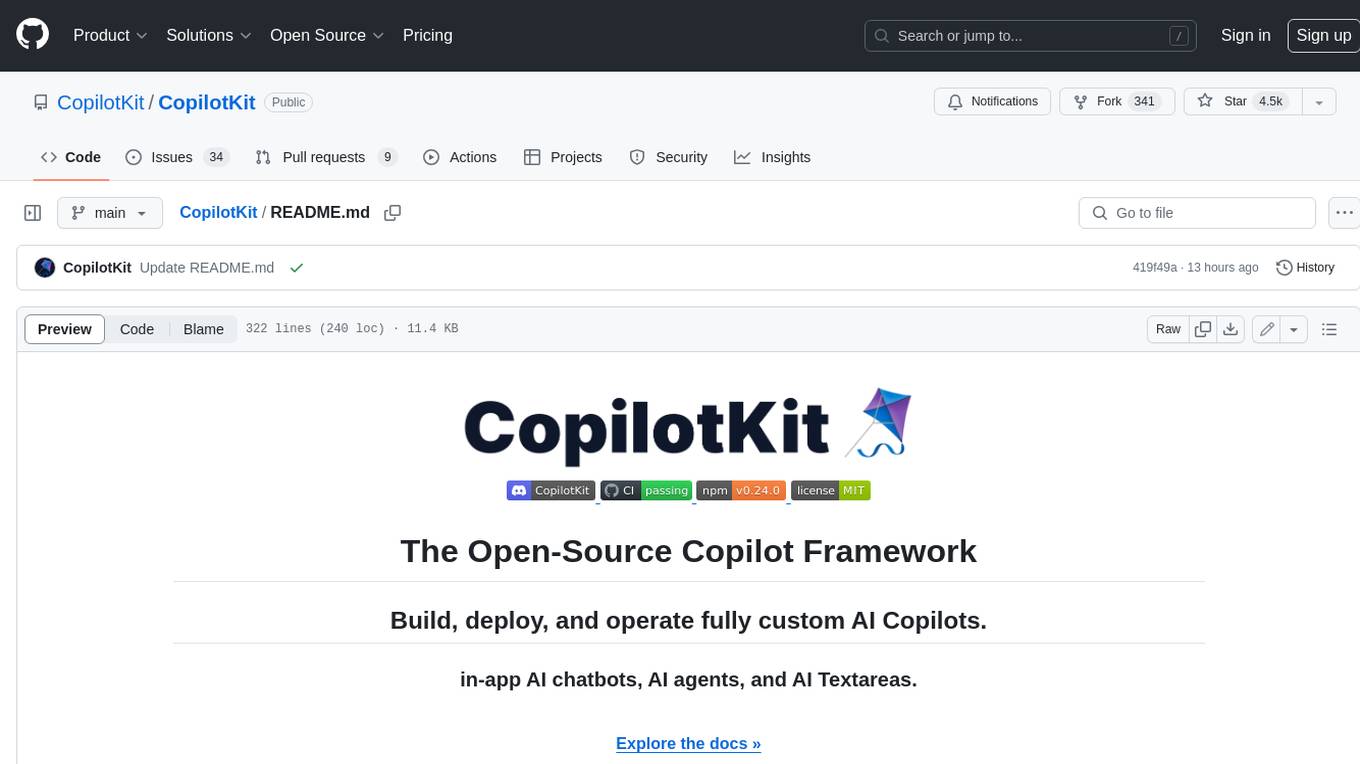
CopilotKit
CopilotKit is an open-source framework for building, deploying, and operating fully custom AI Copilots, including in-app AI chatbots, AI agents, and AI Textareas. It provides a set of components and entry points that allow developers to easily integrate AI capabilities into their applications. CopilotKit is designed to be flexible and extensible, so developers can tailor it to their specific needs. It supports a variety of use cases, including providing app-aware AI chatbots that can interact with the application state and take action, drop-in replacements for textareas with AI-assisted text generation, and in-app agents that can access real-time application context and take action within the application.
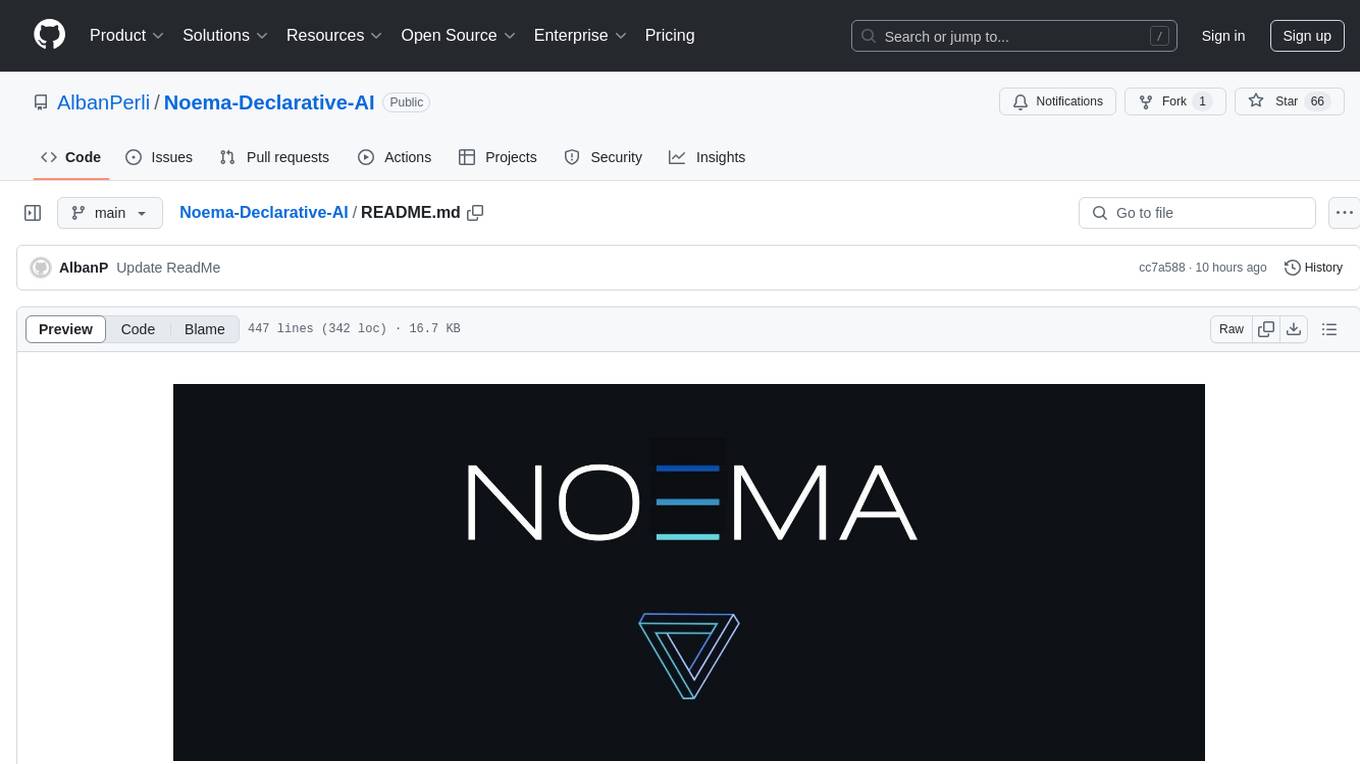
Noema-Declarative-AI
Noema is a framework that enables developers to control a language model and choose the path it will follow. It integrates Python with llm's generations, allowing users to use LLM as a thought interpreter rather than a source of truth. Noema is built on llama.cpp and guidance's shoulders. It applies the declarative programming paradigm to a language model, providing a way to represent functions, descriptions, and transformations. Users can create subjects, think about tasks, and generate content through generators, selectors, and code generators. Noema supports ReAct prompting, visualization, and semantic Python functionalities, offering a versatile tool for automating tasks and guiding language models.
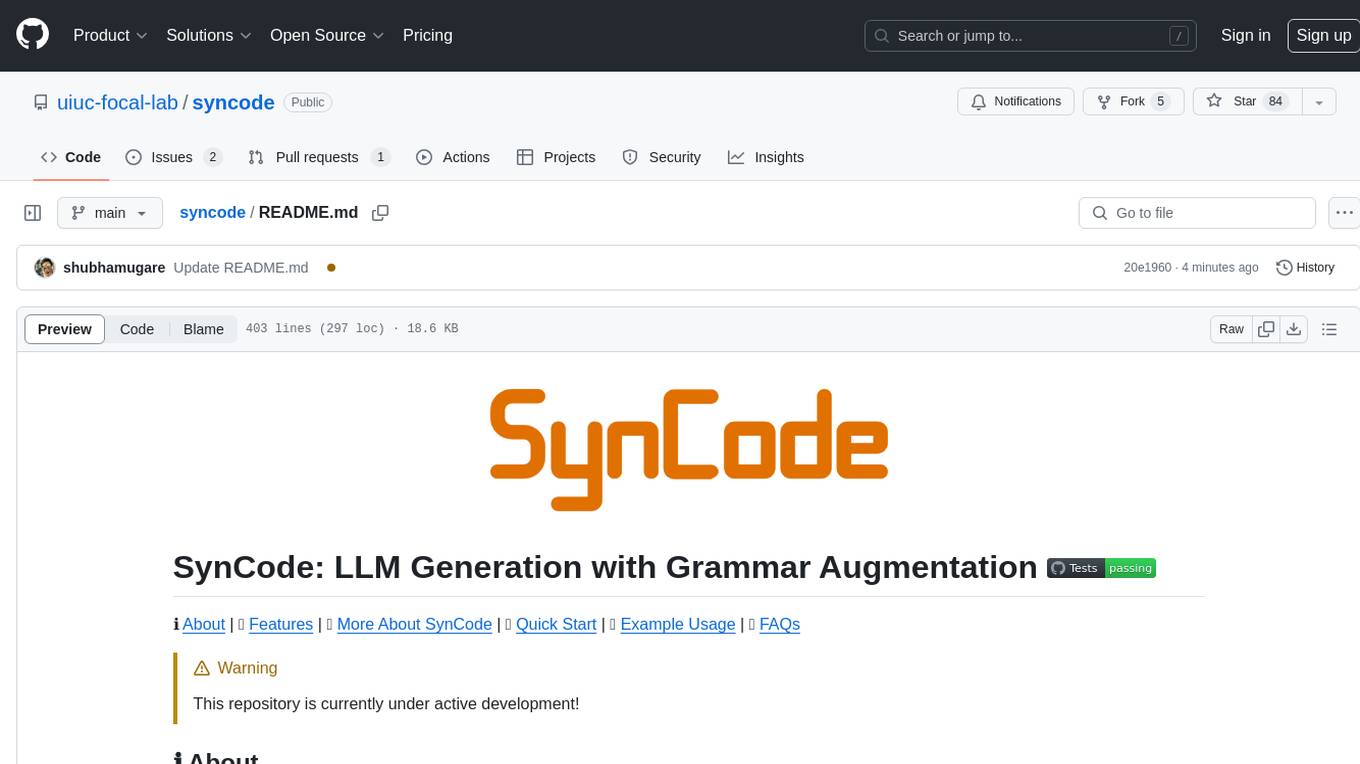
syncode
SynCode is a novel framework for the grammar-guided generation of Large Language Models (LLMs) that ensures syntactically valid output with respect to defined Context-Free Grammar (CFG) rules. It supports general-purpose programming languages like Python, Go, SQL, JSON, and more, allowing users to define custom grammars using EBNF syntax. The tool compares favorably to other constrained decoders and offers features like fast grammar-guided generation, compatibility with HuggingFace Language Models, and the ability to work with various decoding strategies.
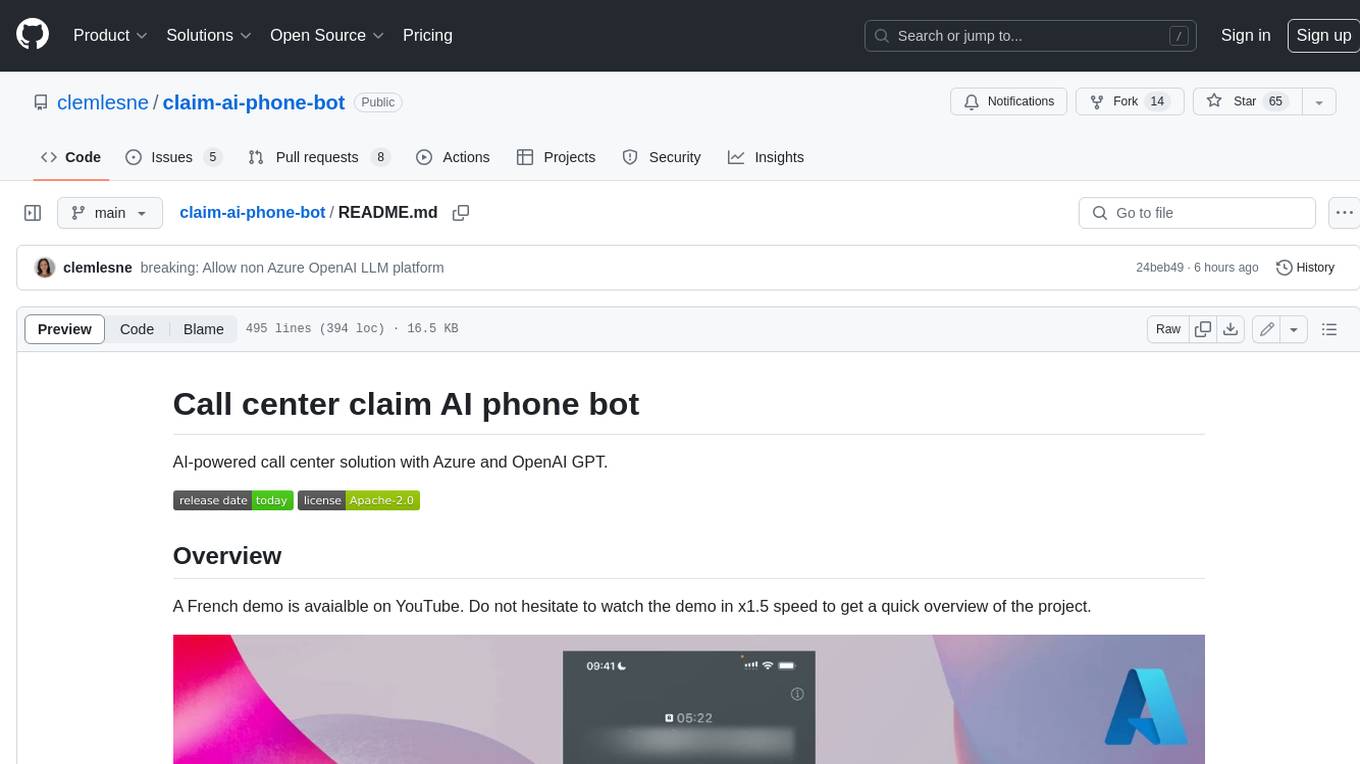
claim-ai-phone-bot
AI-powered call center solution with Azure and OpenAI GPT. The bot can answer calls, understand the customer's request, and provide relevant information or assistance. It can also create a todo list of tasks to complete the claim, and send a report after the call. The bot is customizable, and can be used in multiple languages.
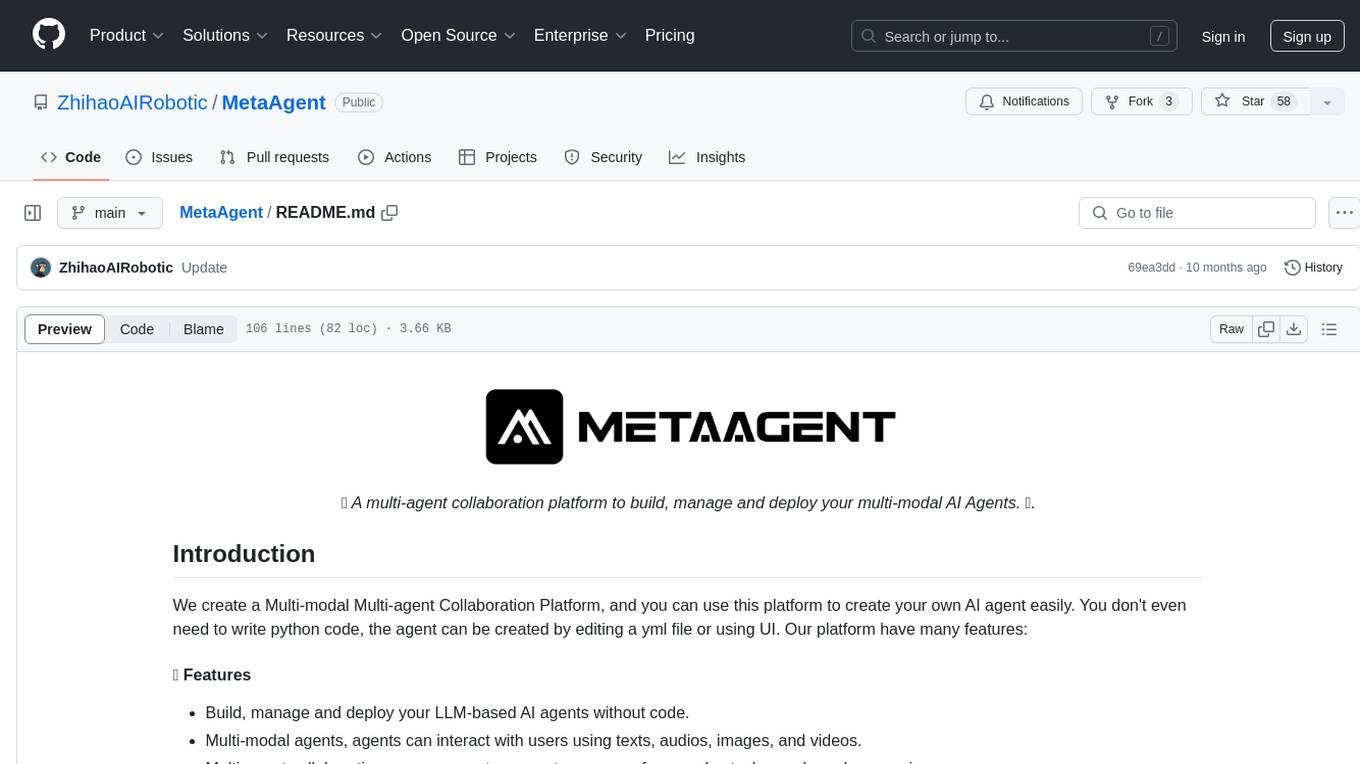
MetaAgent
MetaAgent is a multi-agent collaboration platform designed to build, manage, and deploy multi-modal AI agents without the need for coding. Users can easily create AI agents by editing a yml file or using the provided UI. The platform supports features such as building LLM-based AI agents, multi-modal interactions with users using texts, audios, images, and videos, creating a company of agents for complex tasks like drawing comics, vector database and knowledge embeddings, and upcoming features like UI for creating and using AI agents, fine-tuning, and RLHF. The tool simplifies the process of creating and deploying AI agents for various tasks.
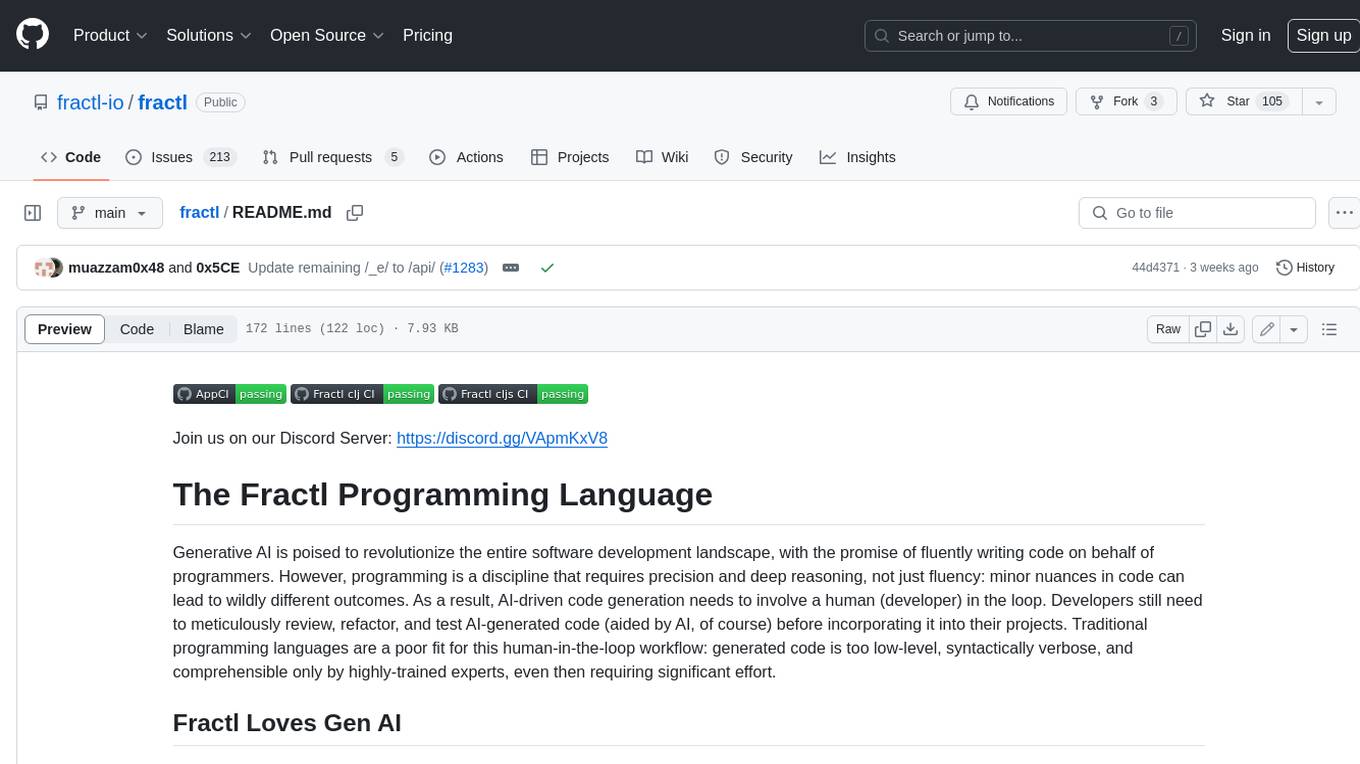
fractl
Fractl is a programming language designed for generative AI, making it easier for developers to work with AI-generated code. It features a data-oriented and declarative syntax, making it a better fit for generative AI-powered code generation. Fractl also bridges the gap between traditional programming and visual building, allowing developers to use multiple ways of building, including traditional coding, visual development, and code generation with generative AI. Key concepts in Fractl include a graph-based hierarchical data model, zero-trust programming, declarative dataflow, resolvers, interceptors, and entity-graph-database mapping.
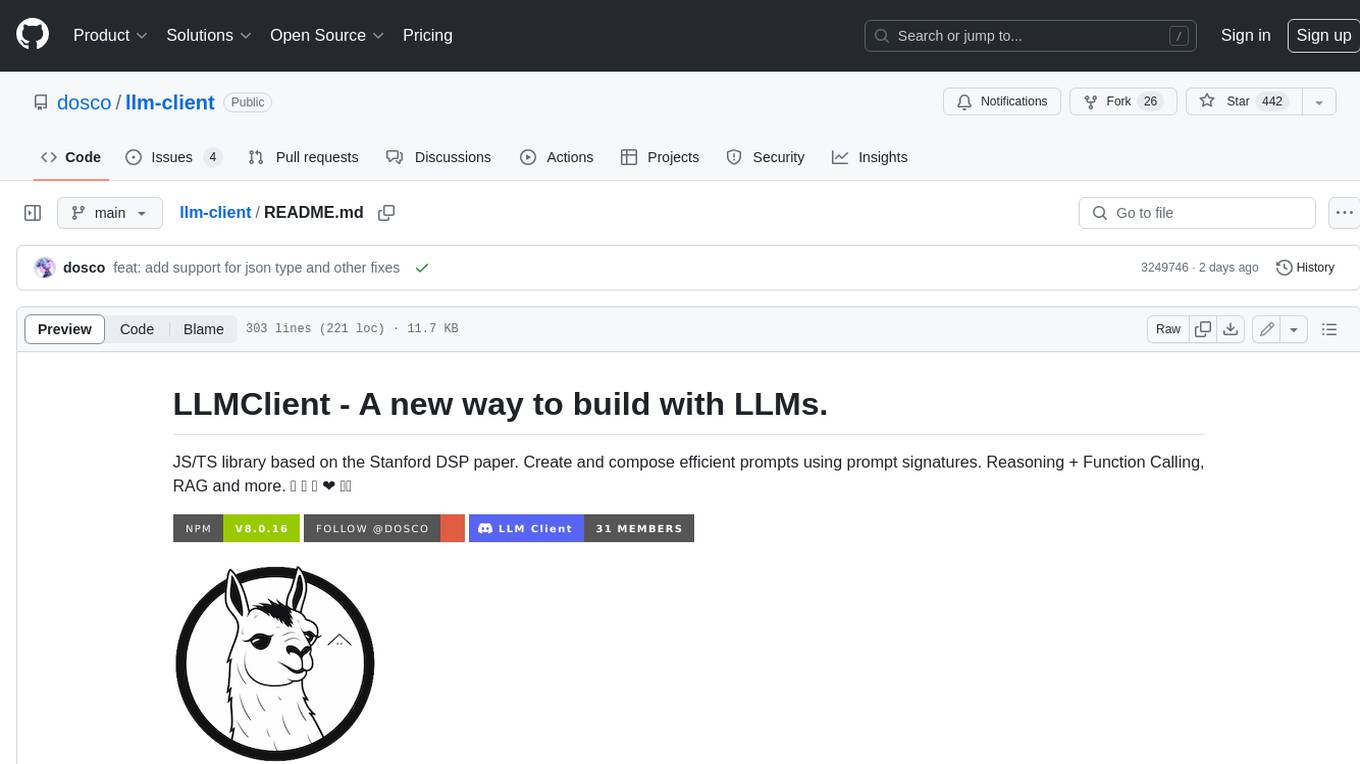
llm-client
LLMClient is a JavaScript/TypeScript library that simplifies working with large language models (LLMs) by providing an easy-to-use interface for building and composing efficient prompts using prompt signatures. These signatures enable the automatic generation of typed prompts, allowing developers to leverage advanced capabilities like reasoning, function calling, RAG, ReAcT, and Chain of Thought. The library supports various LLMs and vector databases, making it a versatile tool for a wide range of applications.
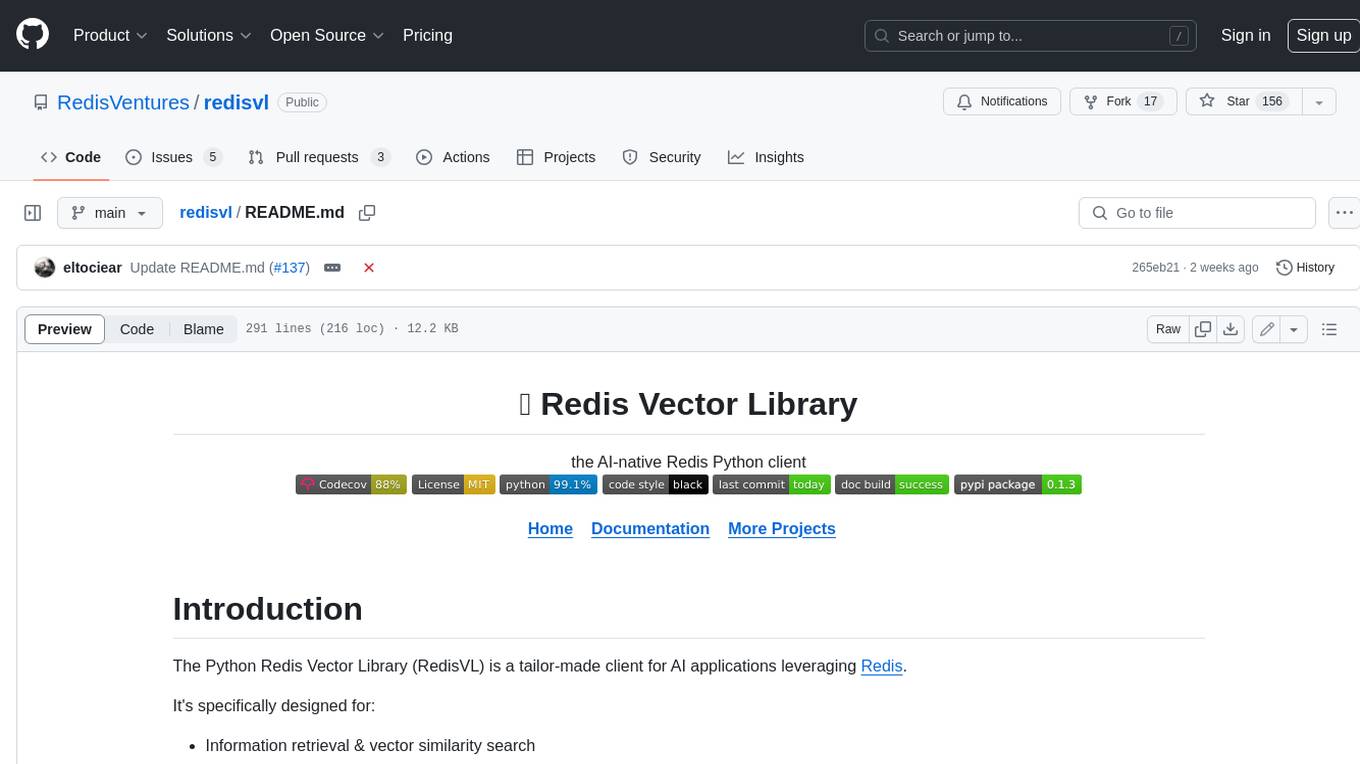
redisvl
Redis Vector Library (RedisVL) is a Python client library for building AI applications on top of Redis. It provides a high-level interface for managing vector indexes, performing vector search, and integrating with popular embedding models and providers. RedisVL is designed to make it easy for developers to build and deploy AI applications that leverage the speed, flexibility, and reliability of Redis.
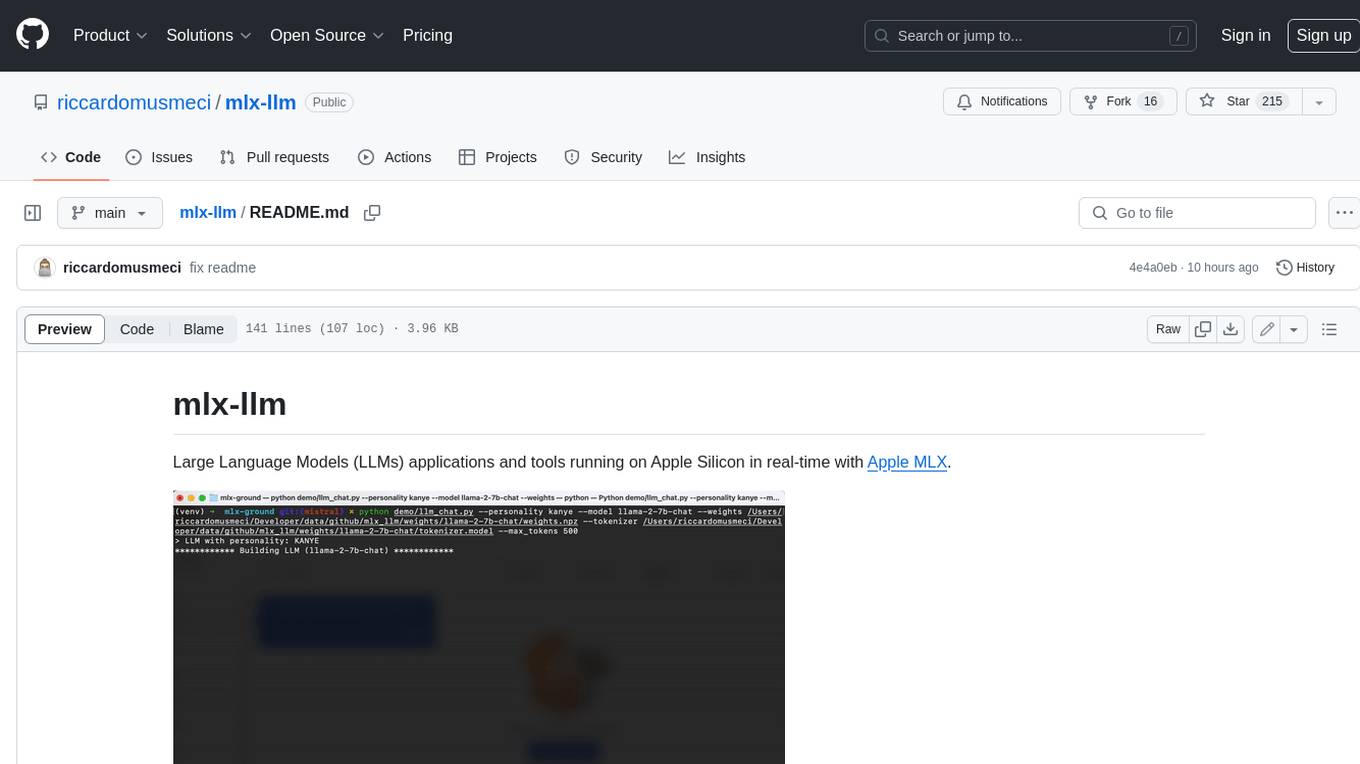
mlx-llm
mlx-llm is a library that allows you to run Large Language Models (LLMs) on Apple Silicon devices in real-time using Apple's MLX framework. It provides a simple and easy-to-use API for creating, loading, and using LLM models, as well as a variety of applications such as chatbots, fine-tuning, and retrieval-augmented generation.
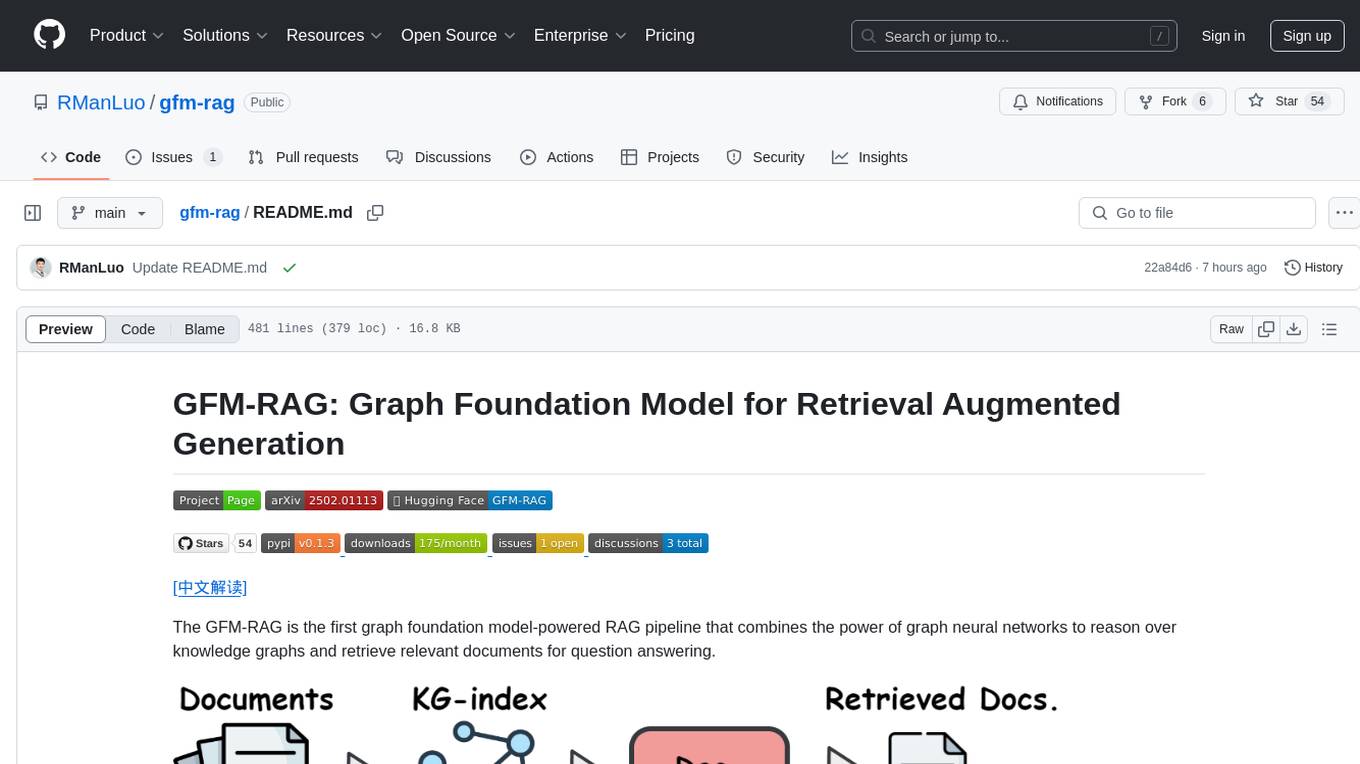
gfm-rag
The GFM-RAG is a graph foundation model-powered pipeline that combines graph neural networks to reason over knowledge graphs and retrieve relevant documents for question answering. It features a knowledge graph index, efficiency in multi-hop reasoning, generalizability to unseen datasets, transferability for fine-tuning, compatibility with agent-based frameworks, and interpretability of reasoning paths. The tool can be used for conducting retrieval and question answering tasks using pre-trained models or fine-tuning on custom datasets.
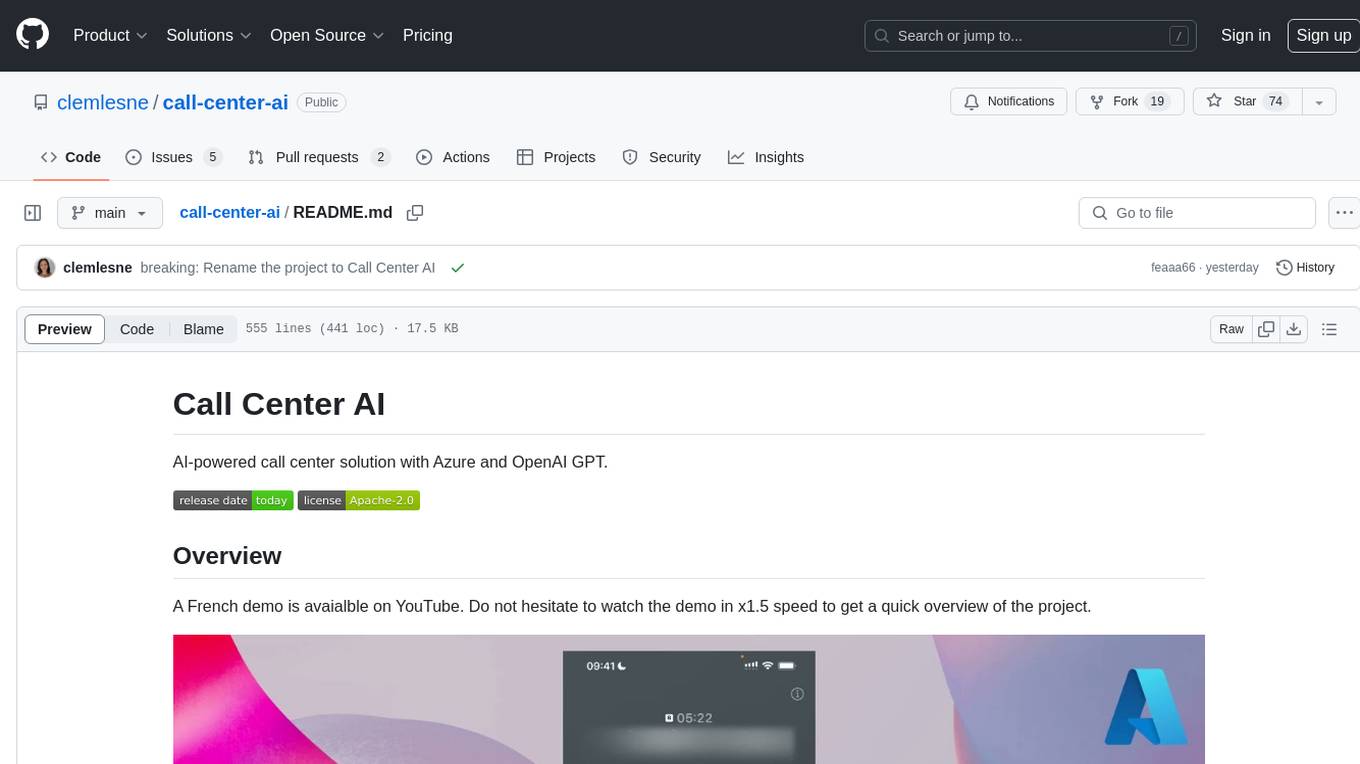
call-center-ai
Call Center AI is an AI-powered call center solution that leverages Azure and OpenAI GPT. It is a proof of concept demonstrating the integration of Azure Communication Services, Azure Cognitive Services, and Azure OpenAI to build an automated call center solution. The project showcases features like accessing claims on a public website, customer conversation history, language change during conversation, bot interaction via phone number, multiple voice tones, lexicon understanding, todo list creation, customizable prompts, content filtering, GPT-4 Turbo for customer requests, specific data schema for claims, documentation database access, SMS report sending, conversation resumption, and more. The system architecture includes components like RAG AI Search, SMS gateway, call gateway, moderation, Cosmos DB, event broker, GPT-4 Turbo, Redis cache, translation service, and more. The tool can be deployed remotely using GitHub Actions and locally with prerequisites like Azure environment setup, configuration file creation, and resource hosting. Advanced usage includes custom training data with AI Search, prompt customization, language customization, moderation level customization, claim data schema customization, OpenAI compatible model usage for the LLM, and Twilio integration for SMS.
For similar tasks
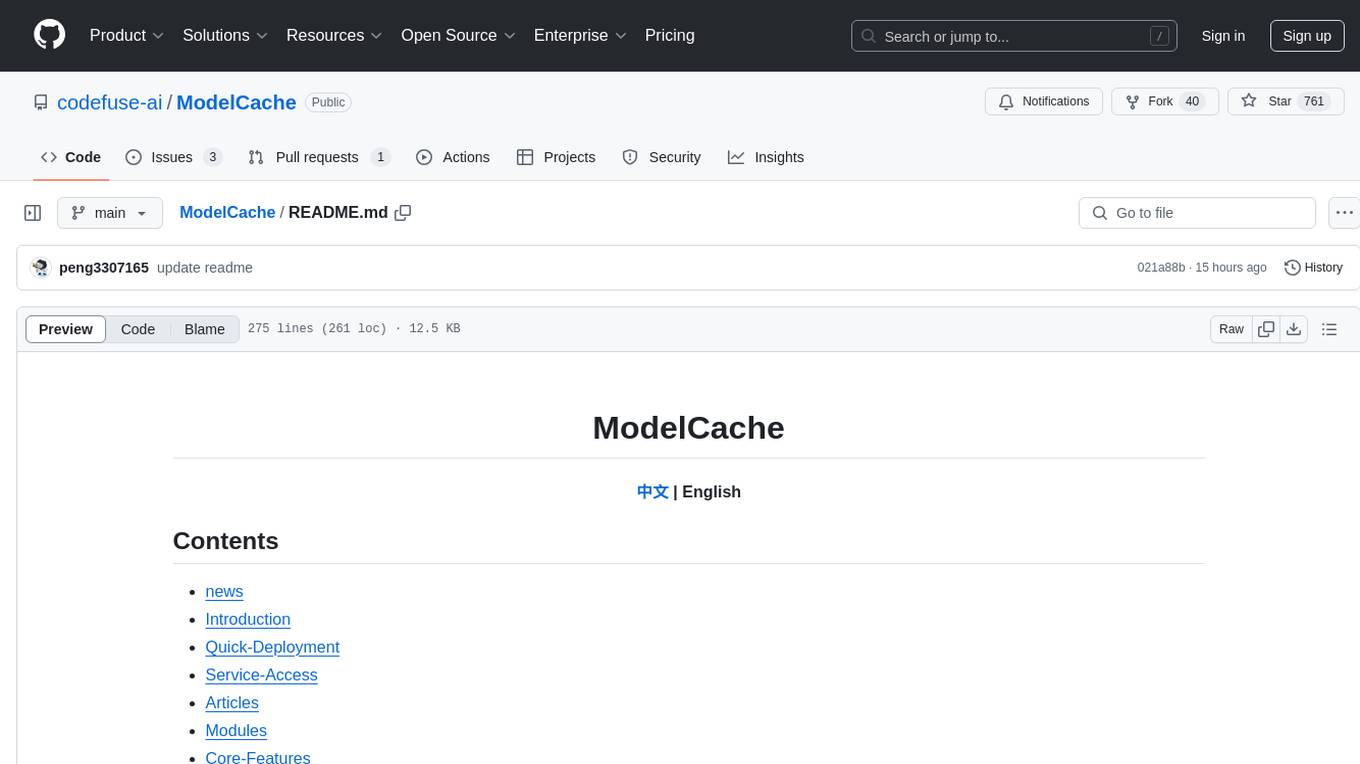
ModelCache
Codefuse-ModelCache is a semantic cache for large language models (LLMs) that aims to optimize services by introducing a caching mechanism. It helps reduce the cost of inference deployment, improve model performance and efficiency, and provide scalable services for large models. The project facilitates sharing and exchanging technologies related to large model semantic cache through open-source collaboration.
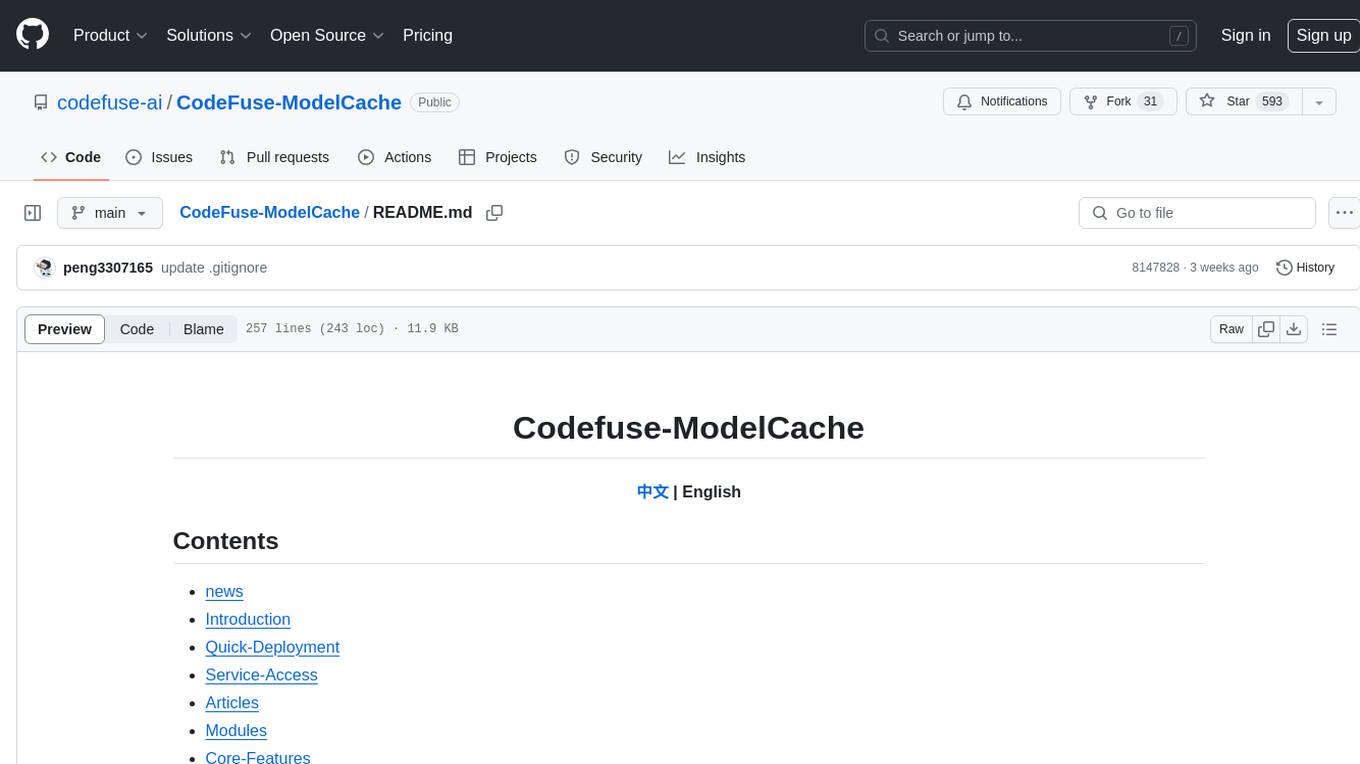
CodeFuse-ModelCache
Codefuse-ModelCache is a semantic cache for large language models (LLMs) that aims to optimize services by introducing a caching mechanism. It helps reduce the cost of inference deployment, improve model performance and efficiency, and provide scalable services for large models. The project caches pre-generated model results to reduce response time for similar requests and enhance user experience. It integrates various embedding frameworks and local storage options, offering functionalities like cache-writing, cache-querying, and cache-clearing through RESTful API. The tool supports multi-tenancy, system commands, and multi-turn dialogue, with features for data isolation, database management, and model loading schemes. Future developments include data isolation based on hyperparameters, enhanced system prompt partitioning storage, and more versatile embedding models and similarity evaluation algorithms.
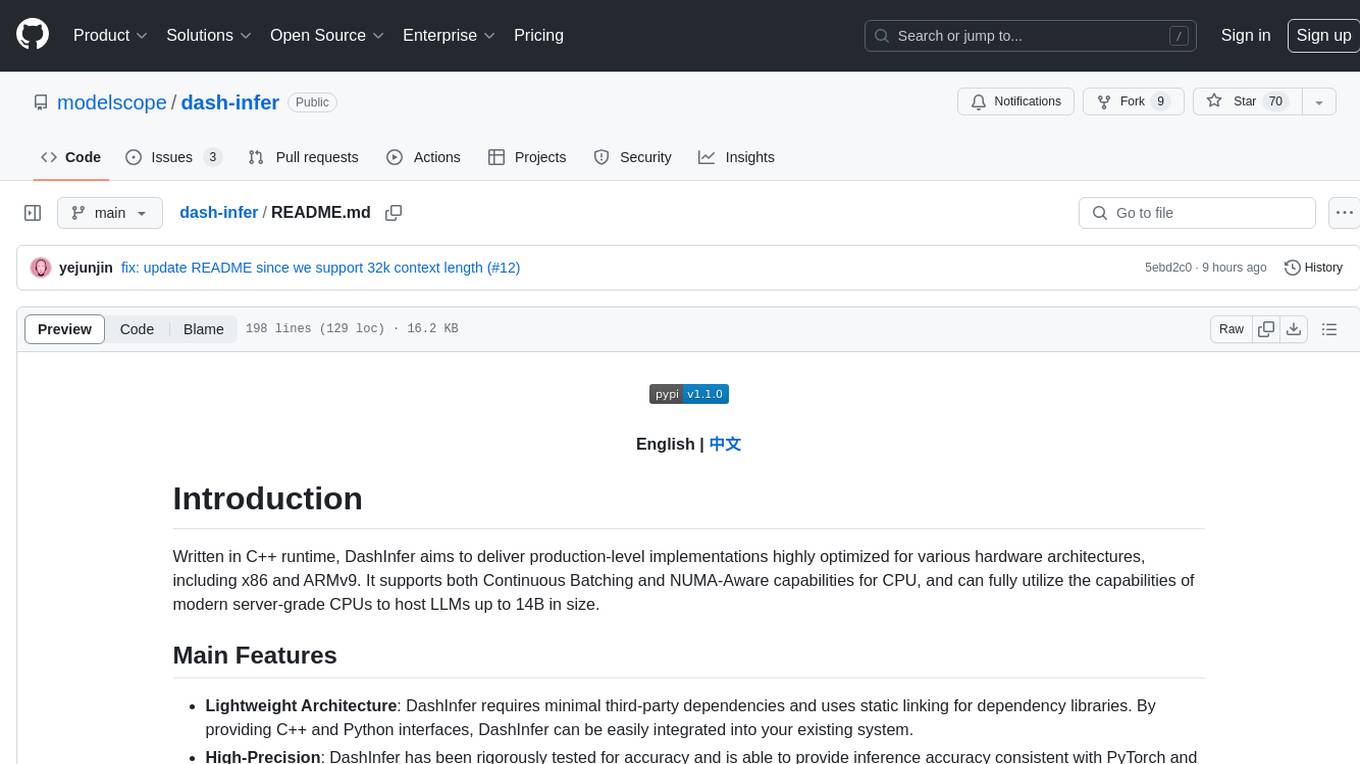
dash-infer
DashInfer is a C++ runtime tool designed to deliver production-level implementations highly optimized for various hardware architectures, including x86 and ARMv9. It supports Continuous Batching and NUMA-Aware capabilities for CPU, and can fully utilize modern server-grade CPUs to host large language models (LLMs) up to 14B in size. With lightweight architecture, high precision, support for mainstream open-source LLMs, post-training quantization, optimized computation kernels, NUMA-aware design, and multi-language API interfaces, DashInfer provides a versatile solution for efficient inference tasks. It supports x86 CPUs with AVX2 instruction set and ARMv9 CPUs with SVE instruction set, along with various data types like FP32, BF16, and InstantQuant. DashInfer also offers single-NUMA and multi-NUMA architectures for model inference, with detailed performance tests and inference accuracy evaluations available. The tool is supported on mainstream Linux server operating systems and provides documentation and examples for easy integration and usage.
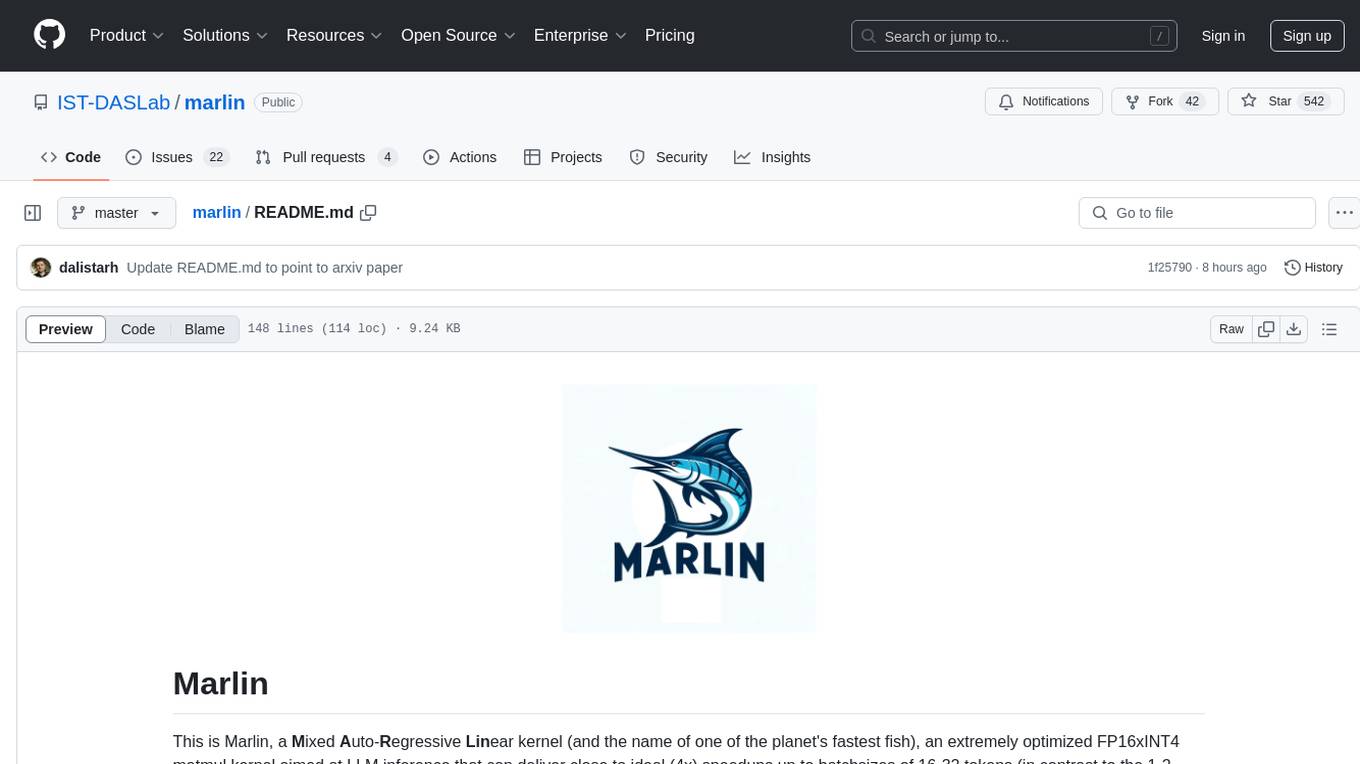
marlin
Marlin is a highly optimized FP16xINT4 matmul kernel designed for large language model (LLM) inference, offering close to ideal speedups up to batchsizes of 16-32 tokens. It is suitable for larger-scale serving, speculative decoding, and advanced multi-inference schemes like CoT-Majority. Marlin achieves optimal performance by utilizing various techniques and optimizations to fully leverage GPU resources, ensuring efficient computation and memory management.
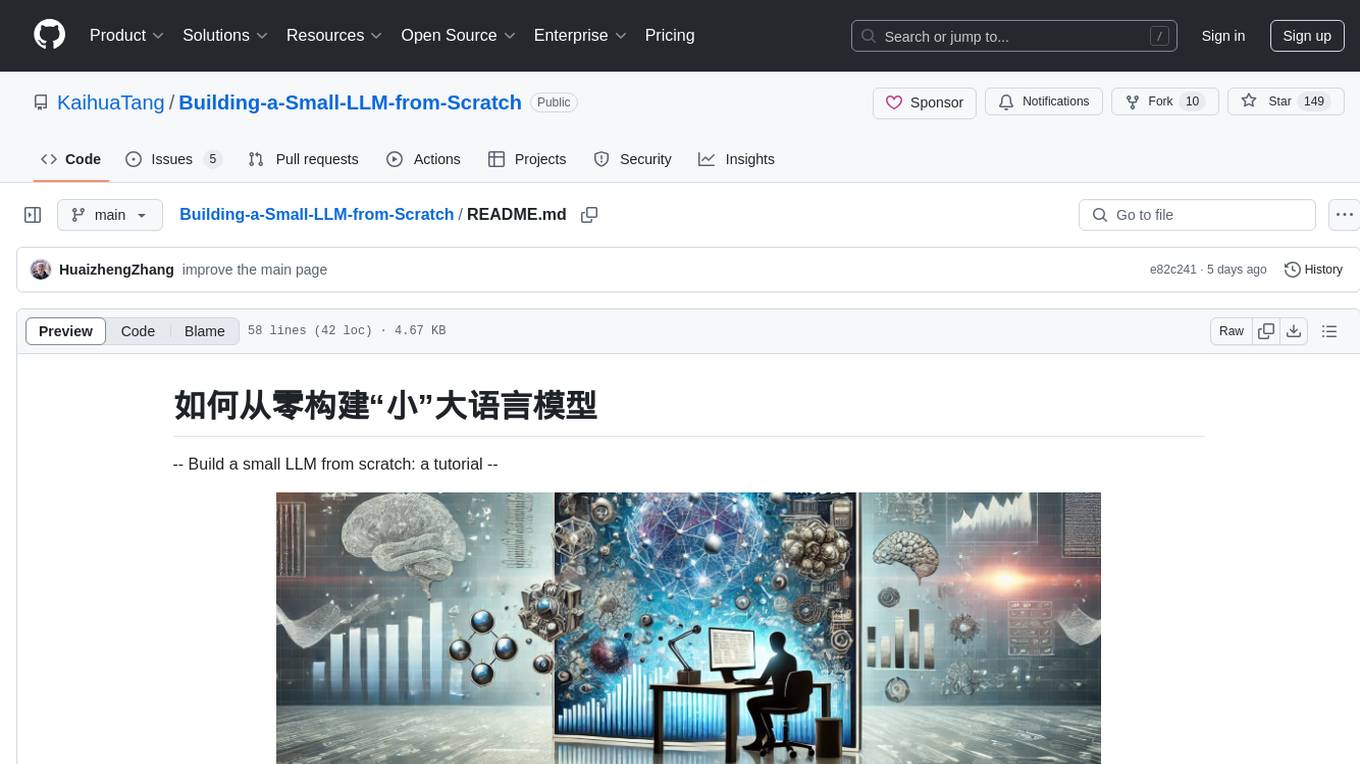
Building-a-Small-LLM-from-Scratch
This tutorial provides a comprehensive guide on building a small Large Language Model (LLM) from scratch using PyTorch. The author shares insights and experiences gained from working on LLM projects in the industry, aiming to help beginners understand the fundamental components of LLMs and training fine-tuning codes. The tutorial covers topics such as model structure overview, attention modules, optimization techniques, normalization layers, tokenizers, pretraining, and fine-tuning with dialogue data. It also addresses specific industry-related challenges and explores cutting-edge model concepts like DeepSeek network structure, causal attention, dynamic-to-static tensor conversion for ONNX inference, and performance optimizations for NPU chips. The series emphasizes hands-on practice with small models to enable local execution and plans to expand into multimodal language models and tensor parallel multi-card deployment.
For similar jobs
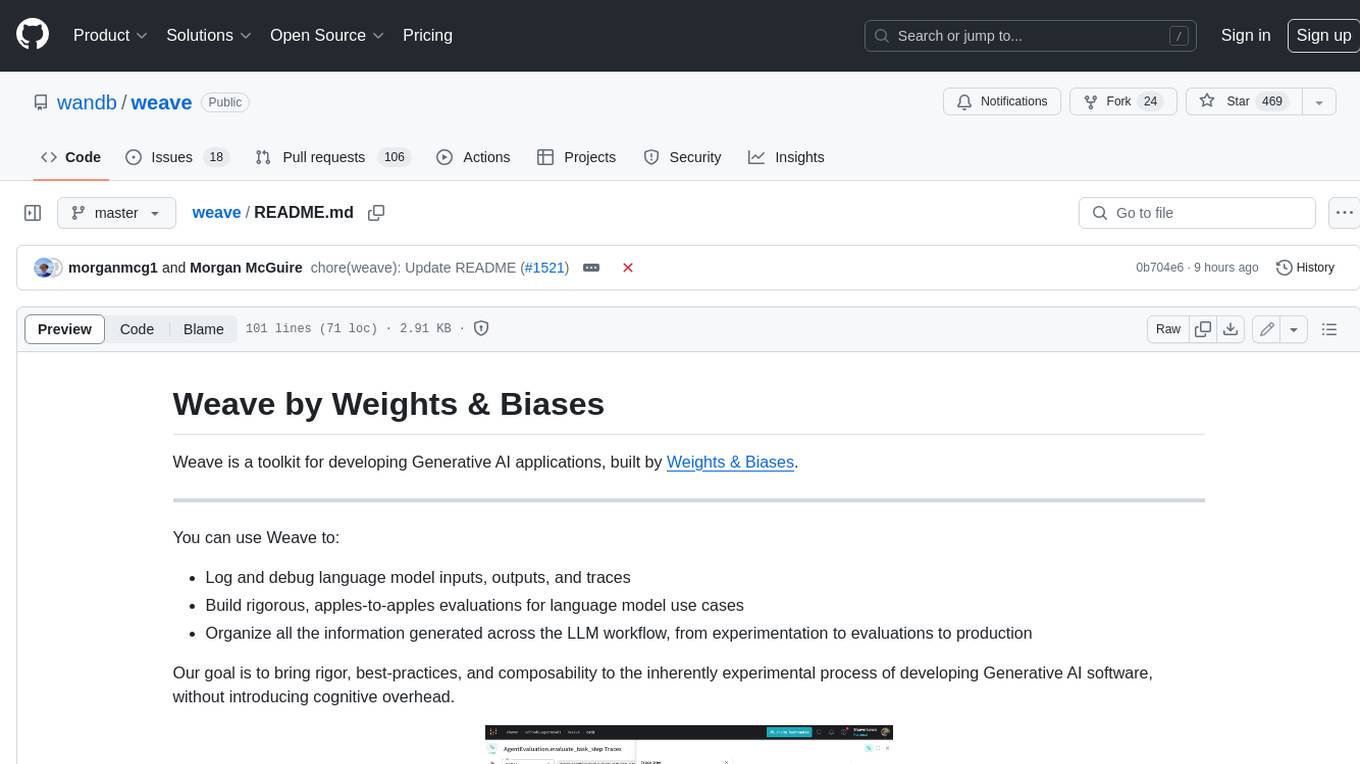
weave
Weave is a toolkit for developing Generative AI applications, built by Weights & Biases. With Weave, you can log and debug language model inputs, outputs, and traces; build rigorous, apples-to-apples evaluations for language model use cases; and organize all the information generated across the LLM workflow, from experimentation to evaluations to production. Weave aims to bring rigor, best-practices, and composability to the inherently experimental process of developing Generative AI software, without introducing cognitive overhead.
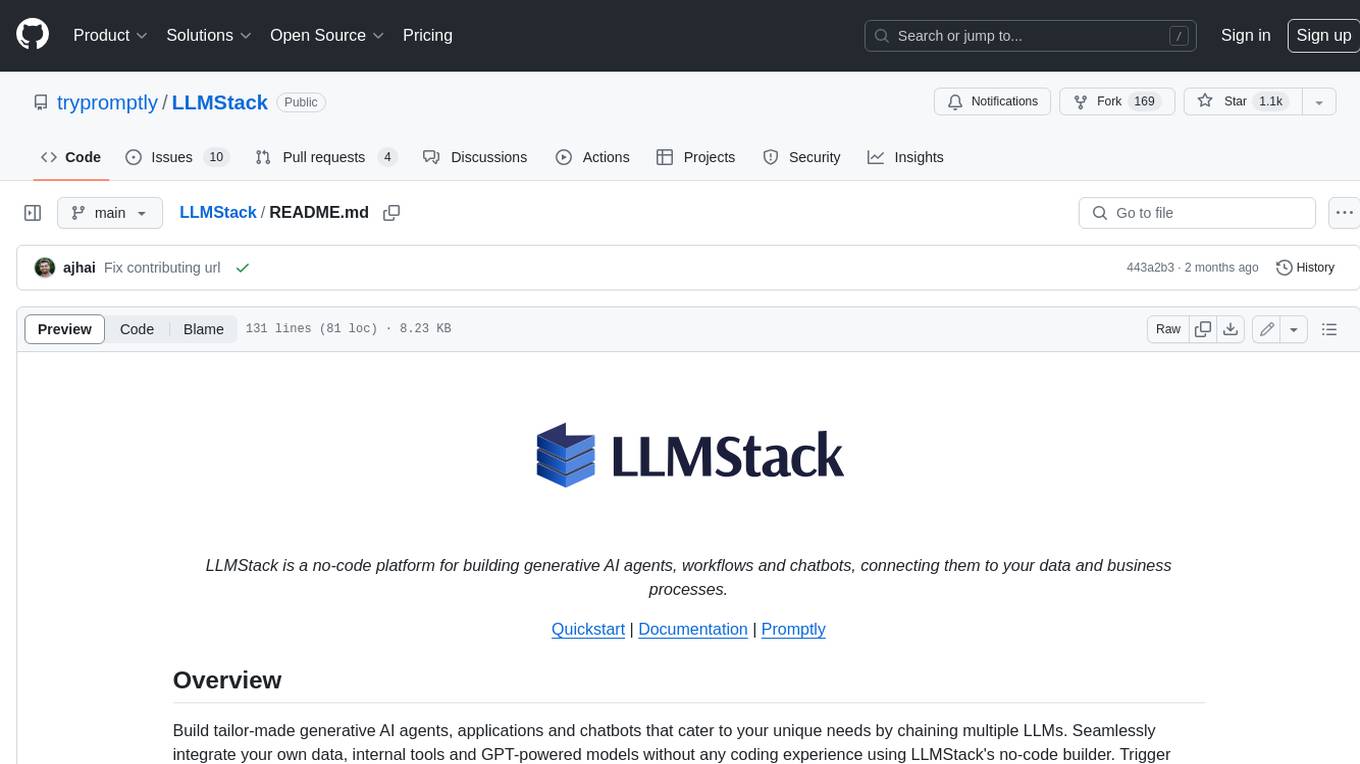
LLMStack
LLMStack is a no-code platform for building generative AI agents, workflows, and chatbots. It allows users to connect their own data, internal tools, and GPT-powered models without any coding experience. LLMStack can be deployed to the cloud or on-premise and can be accessed via HTTP API or triggered from Slack or Discord.
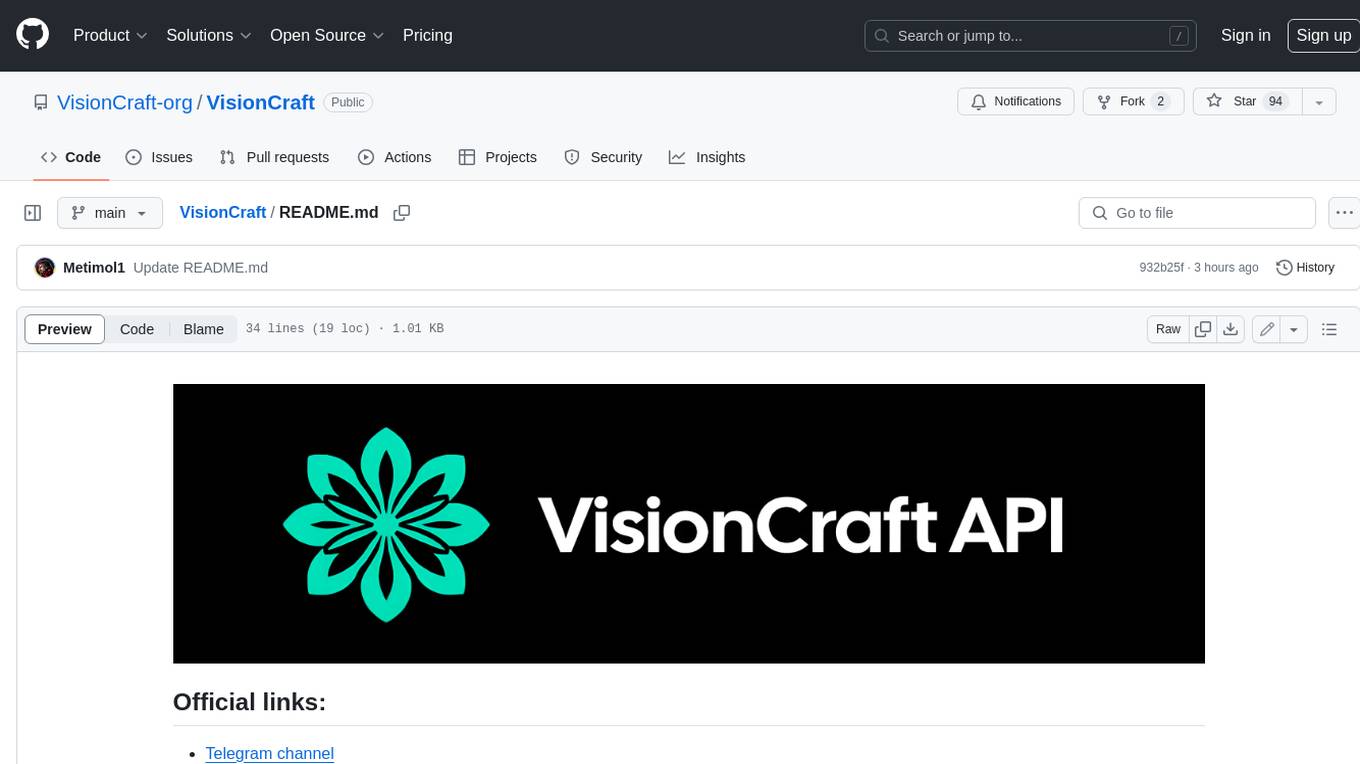
VisionCraft
The VisionCraft API is a free API for using over 100 different AI models. From images to sound.
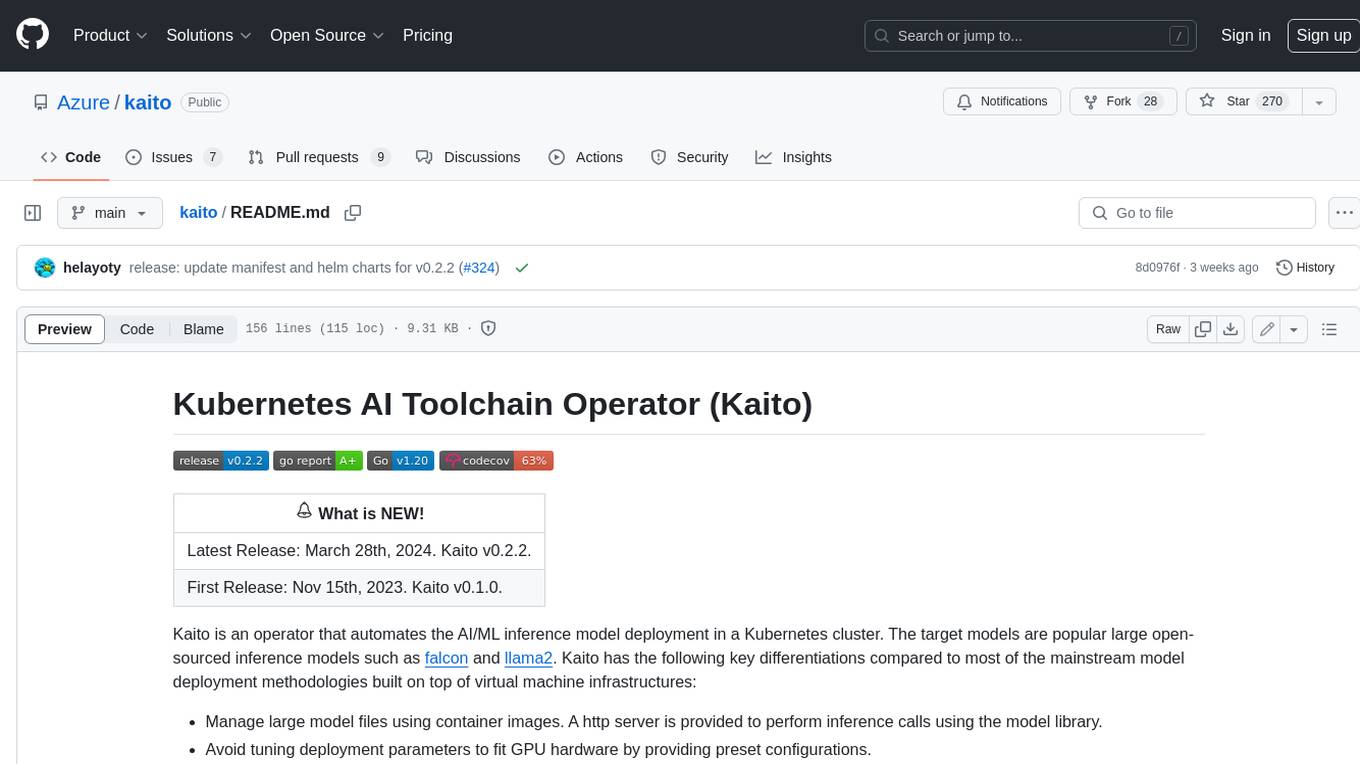
kaito
Kaito is an operator that automates the AI/ML inference model deployment in a Kubernetes cluster. It manages large model files using container images, avoids tuning deployment parameters to fit GPU hardware by providing preset configurations, auto-provisions GPU nodes based on model requirements, and hosts large model images in the public Microsoft Container Registry (MCR) if the license allows. Using Kaito, the workflow of onboarding large AI inference models in Kubernetes is largely simplified.
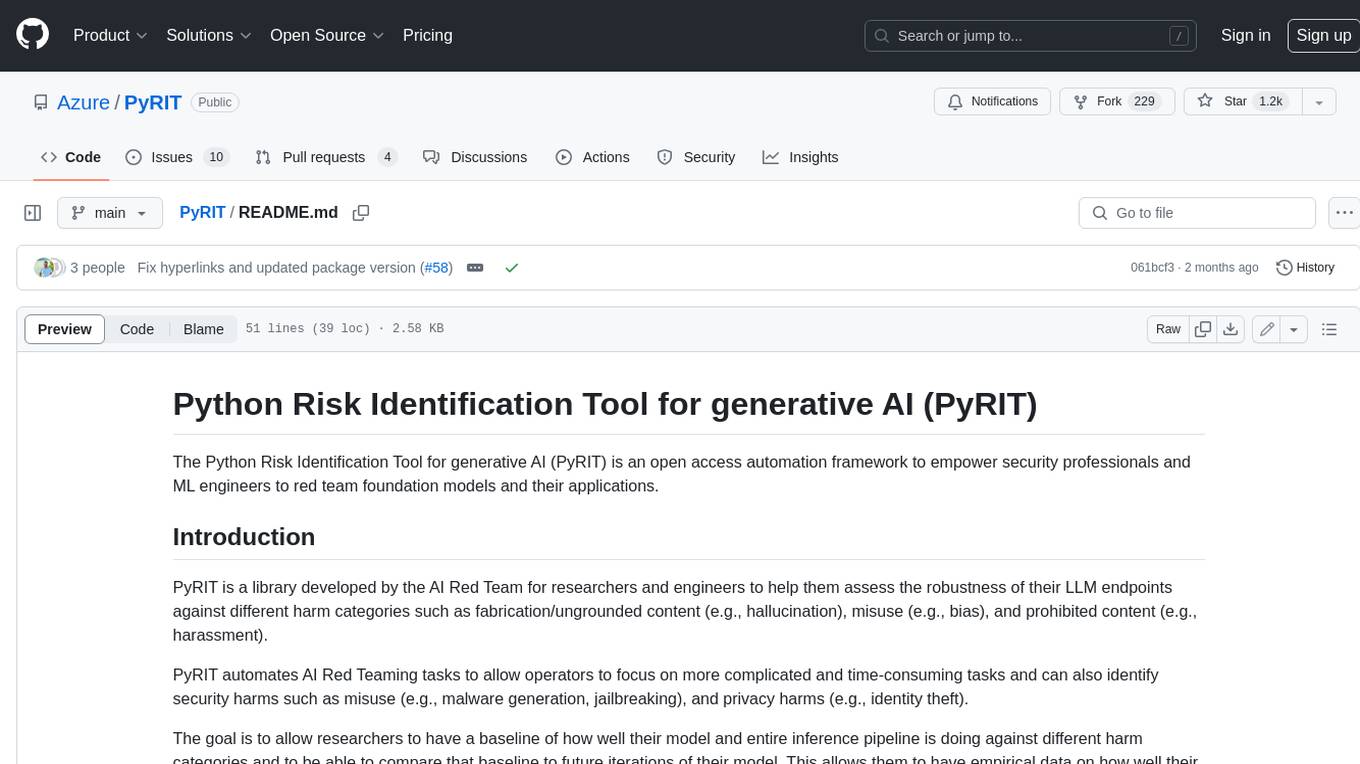
PyRIT
PyRIT is an open access automation framework designed to empower security professionals and ML engineers to red team foundation models and their applications. It automates AI Red Teaming tasks to allow operators to focus on more complicated and time-consuming tasks and can also identify security harms such as misuse (e.g., malware generation, jailbreaking), and privacy harms (e.g., identity theft). The goal is to allow researchers to have a baseline of how well their model and entire inference pipeline is doing against different harm categories and to be able to compare that baseline to future iterations of their model. This allows them to have empirical data on how well their model is doing today, and detect any degradation of performance based on future improvements.
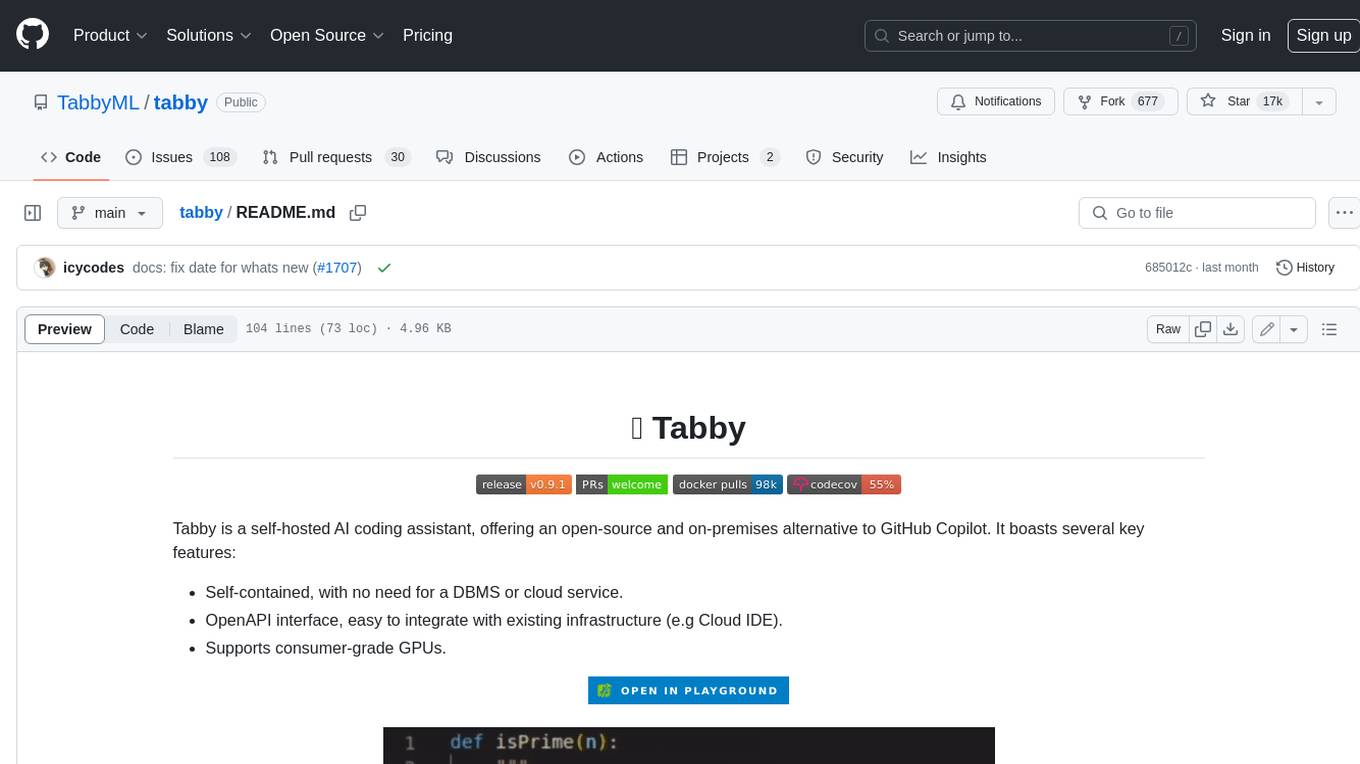
tabby
Tabby is a self-hosted AI coding assistant, offering an open-source and on-premises alternative to GitHub Copilot. It boasts several key features: * Self-contained, with no need for a DBMS or cloud service. * OpenAPI interface, easy to integrate with existing infrastructure (e.g Cloud IDE). * Supports consumer-grade GPUs.
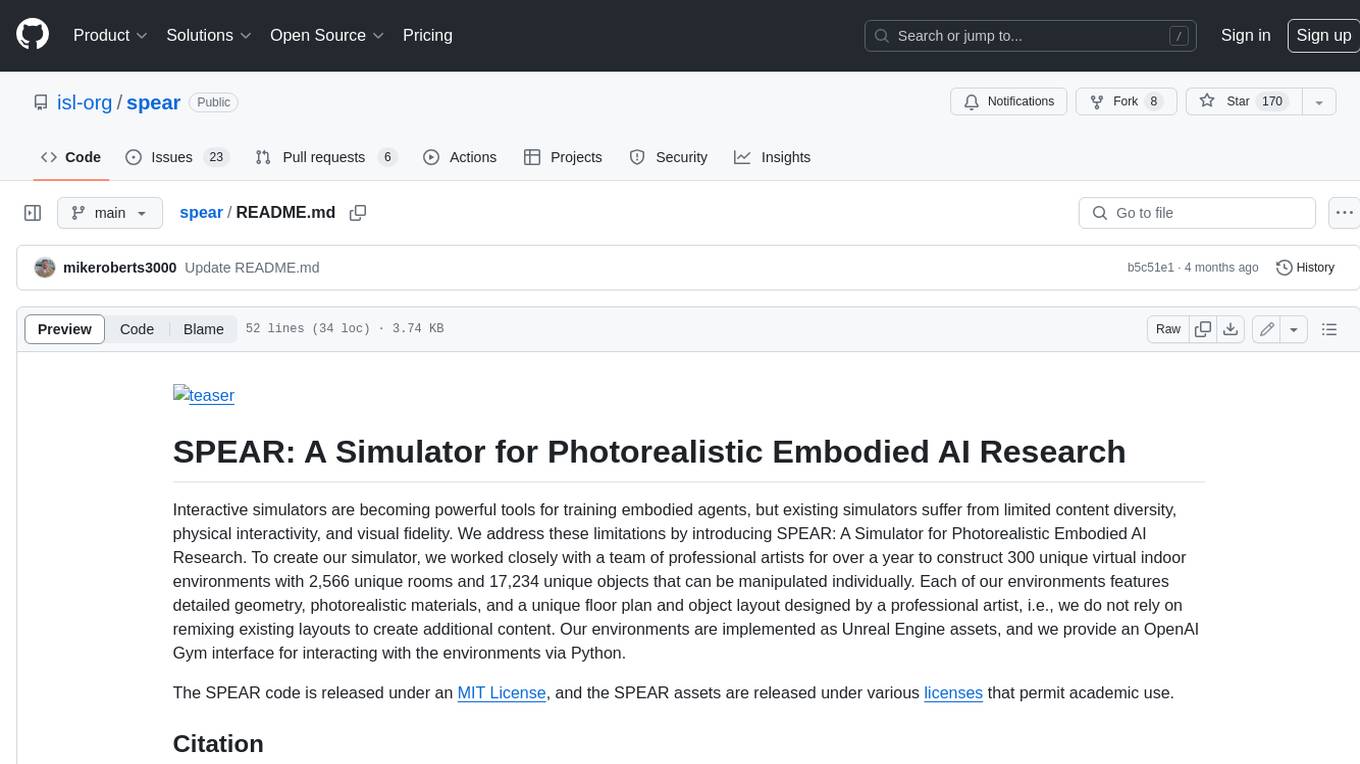
spear
SPEAR (Simulator for Photorealistic Embodied AI Research) is a powerful tool for training embodied agents. It features 300 unique virtual indoor environments with 2,566 unique rooms and 17,234 unique objects that can be manipulated individually. Each environment is designed by a professional artist and features detailed geometry, photorealistic materials, and a unique floor plan and object layout. SPEAR is implemented as Unreal Engine assets and provides an OpenAI Gym interface for interacting with the environments via Python.
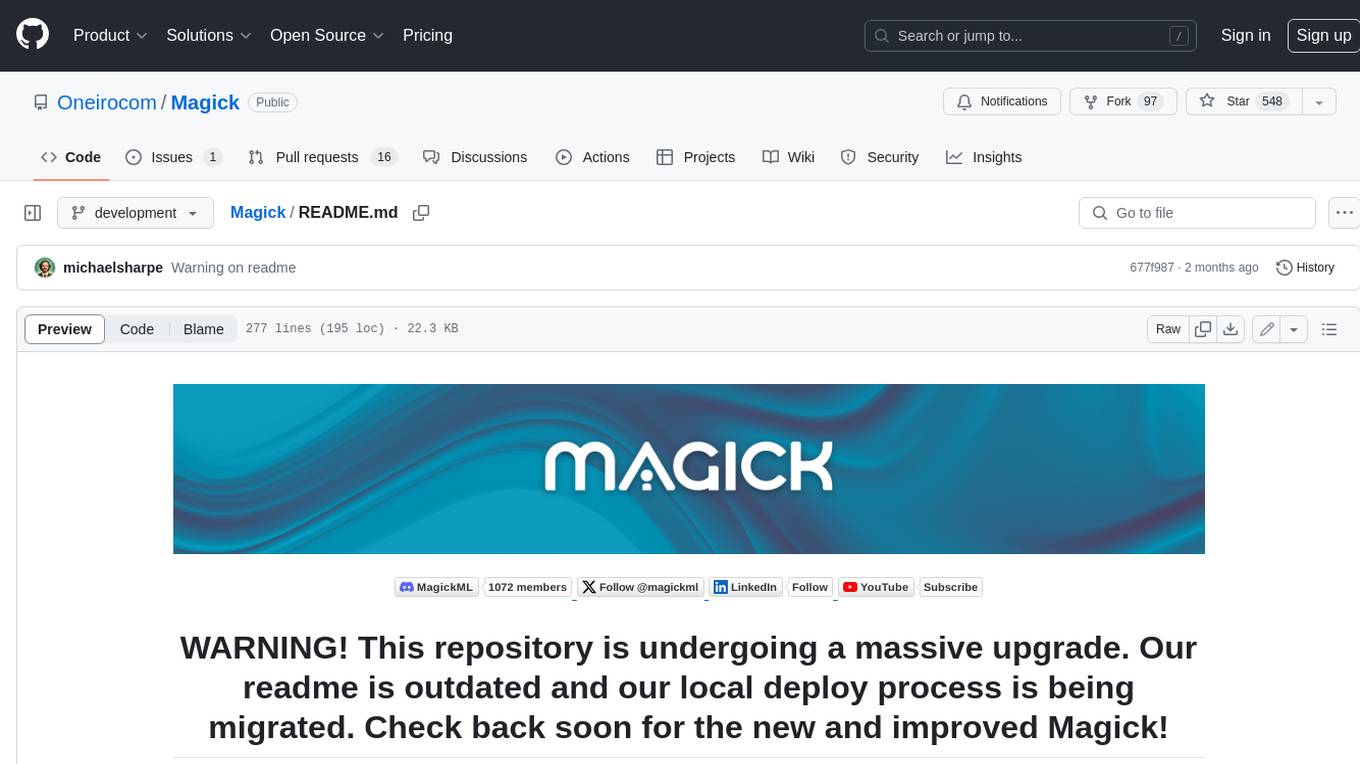
Magick
Magick is a groundbreaking visual AIDE (Artificial Intelligence Development Environment) for no-code data pipelines and multimodal agents. Magick can connect to other services and comes with nodes and templates well-suited for intelligent agents, chatbots, complex reasoning systems and realistic characters.