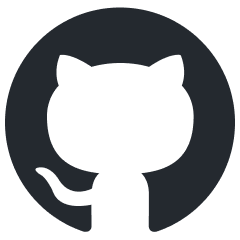
DB-GPT-Hub
A repository that contains models, datasets, and fine-tuning techniques for DB-GPT, with the purpose of enhancing model performance in Text-to-SQL
Stars: 1313
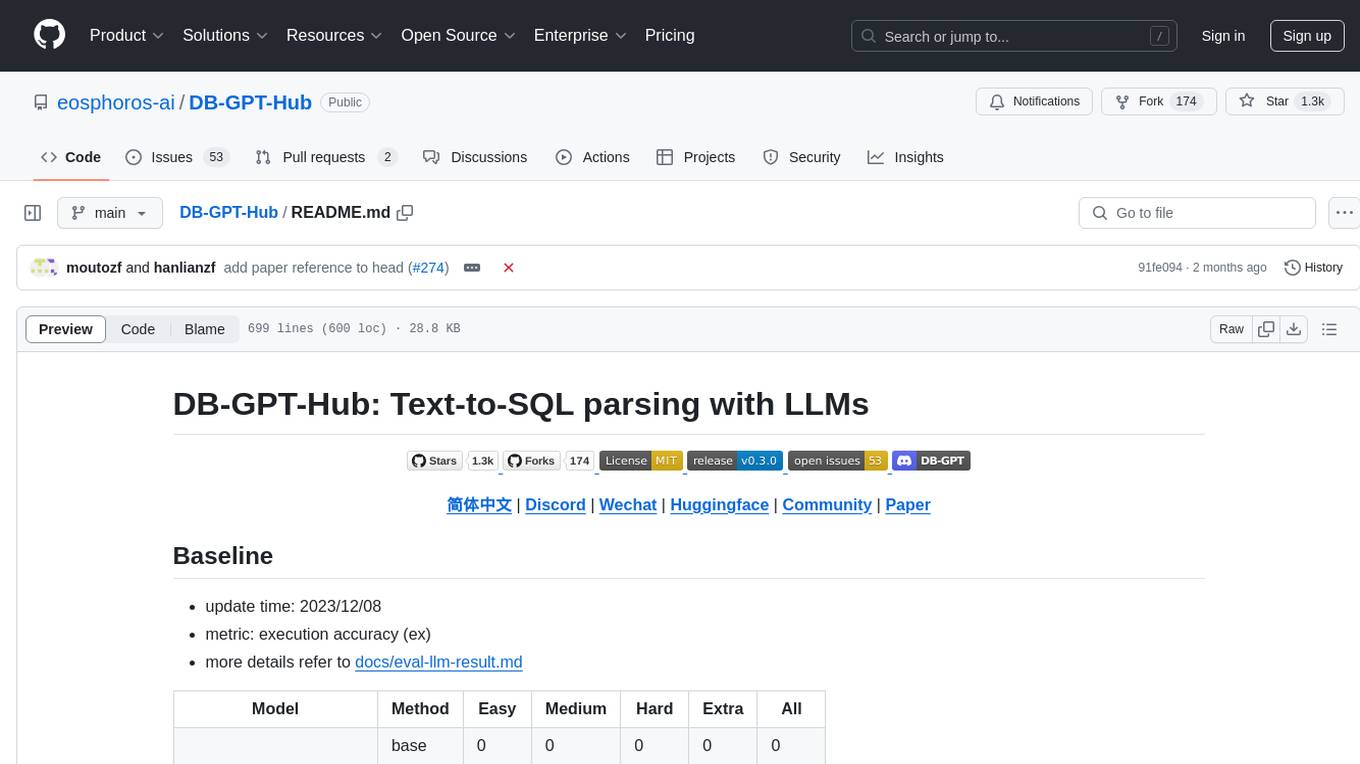
DB-GPT-Hub is an experimental project leveraging Large Language Models (LLMs) for Text-to-SQL parsing. It includes stages like data collection, preprocessing, model selection, construction, and fine-tuning of model weights. The project aims to enhance Text-to-SQL capabilities, reduce model training costs, and enable developers to contribute to improving Text-to-SQL accuracy. The ultimate goal is to achieve automated question-answering based on databases, allowing users to execute complex database queries using natural language descriptions. The project has successfully integrated multiple large models and established a comprehensive workflow for data processing, SFT model training, prediction output, and evaluation.
README:
- Support Text2NLU fine-tuning to improve semantic understanding accuracy.
- Support Text2GQL fine-tuning to generate graph query.
Text2SQL eval execution accuracy (ex) metric, and we will move this to src/dbgpt_hub_sql
- update time: 2023/12/08
- metric: execution accuracy (ex)
- more details refer to docs/eval-llm-result.md
Model | Method | Easy | Medium | Hard | Extra | All |
---|---|---|---|---|---|---|
base | 0 | 0 | 0 | 0 | 0 | |
Llama2-7B-Chat | lora | 0.887 | 0.641 | 0.489 | 0.331 | 0.626 |
qlora | 0.847 | 0.623 | 0.466 | 0.361 | 0.608 | |
base | 0 | 0 | 0 | 0 | 0 | |
Llama2-13B-Chat | lora | 0.907 | 0.729 | 0.552 | 0.343 | 0.68 |
qlora | 0.911 | 0.7 | 0.552 | 0.319 | 0.664 | |
base | 0.214 | 0.177 | 0.092 | 0.036 | 0.149 | |
CodeLlama-7B-Instruct | lora | 0.923 | 0.756 | 0.586 | 0.349 | 0.702 |
qlora | 0.911 | 0.751 | 0.598 | 0.331 | 0.696 | |
base | 0.698 | 0.601 | 0.408 | 0.271 | 0.539 | |
CodeLlama-13B-Instruct | lora | 0.94 | 0.789 | 0.684 | 0.404 | 0.746 |
qlora | 0.94 | 0.774 | 0.626 | 0.392 | 0.727 | |
base | 0.577 | 0.352 | 0.201 | 0.066 | 0.335 | |
Baichuan2-7B-Chat | lora | 0.871 | 0.63 | 0.448 | 0.295 | 0.603 |
qlora | 0.891 | 0.637 | 0.489 | 0.331 | 0.624 | |
base | 0.581 | 0.413 | 0.264 | 0.187 | 0.392 | |
Baichuan2-13B-Chat | lora | 0.903 | 0.702 | 0.569 | 0.392 | 0.678 |
qlora | 0.895 | 0.675 | 0.58 | 0.343 | 0.659 | |
base | 0.395 | 0.256 | 0.138 | 0.042 | 0.235 | |
Qwen-7B-Chat | lora | 0.855 | 0.688 | 0.575 | 0.331 | 0.652 |
qlora | 0.911 | 0.675 | 0.575 | 0.343 | 0.662 | |
base | 0.871 | 0.632 | 0.368 | 0.181 | 0.573 | |
Qwen-14B-Chat | lora | 0.895 | 0.702 | 0.552 | 0.331 | 0.663 |
qlora | 0.919 | 0.744 | 0.598 | 0.367 | 0.701 | |
base | 0 | 0 | 0 | 0 | 0 | |
ChatGLM3-6b | lora | 0.855 | 0.605 | 0.477 | 0.271 | 0.59 |
qlora | 0.843 | 0.603 | 0.506 | 0.211 | 0.581 |
- DB-GPT-Hub: Text-to-SQL parsing with LLMs
DB-GPT-Hub is an experimental project that leverages Large Language Models (LLMs) to achieve Text-to-SQL parsing. The project encompasses various stages, including data collection, data preprocessing, model selection and construction, and fine-tuning of model weights. Through these processes, our aim is to enhance Text-to-SQL capabilities while reducing model training costs, thus enabling more developers to contribute to improving Text-to-SQL accuracy. Our ultimate goal is to realize automated question-answering capabilities based on databases, allowing users to execute complex database queries using natural language descriptions.
To date, we have successfully integrated multiple large models and established a comprehensive workflow that includes data processing, Supervised Fine-Tuning (SFT) model training, prediction output, and evaluation. The code developed for this project is easily reusable within the project itself.
As of October 10, 2023, we have used this project to fine-tune the open-source 13B-sized model, incorporating more relevant data. Under zero-shot prompts and utilizing the Spider-based test-suite, we have achieved an execution accuracy rate of 0.764 for a database with a size of 1.27G. Additionally, the execution accuracy for the database pointed to by the Spider official website, with a size of 95M, stands at 0.825.
We enhance the Text-to-SQL performance by applying Supervised Fine-Tuning (SFT) on large language models.
The primary dataset for this project's examples is the Spider dataset:
- SPIDER: A complex text2sql dataset across domains, containing 10,181 natural language queries, 5,693 SQL distributed across 200 separate databases, covering 138 different domains.download link
Other text2sql datasets available:
-
WikiSQL: A large semantic parsing dataset consisting of 80,654 natural statement expressions and sql annotations of 24,241 tables. Each query in WikiSQL is limited to the same table and does not contain complex operations such as sorting, grouping The queries in WikiSQL are limited to the same table and do not include complex operations such as sorting, grouping, subqueries, etc.
-
CHASE: A cross-domain multi-round interactive text2sql Chinese dataset containing a list of 5,459 multi-round questions consisting of 17,940 <query, SQL> binary groups across 280 different domain databases.
-
BIRD-SQL: A large-scale cross-domain text-to-SQL benchmark in English, with a particular focus on large database content. The dataset contains 12,751 text-to-SQL data pairs and 95 databases with a total size of 33.4 GB across 37 occupational domains. The BIRD-SQL dataset bridges the gap between text-to-SQL research and real-world applications by exploring three additional challenges, namely dealing with large and messy database values, external knowledge inference and optimising SQL execution efficiency.
-
CoSQL: A corpus for building cross-domain conversational text-to-SQL systems. It is a conversational version of the Spider and SParC tasks. CoSQL consists of 30k+ rounds and 10k+ annotated SQL queries from Wizard-of-Oz's collection of 3k conversations querying 200 complex databases across 138 domains. Each conversation simulates a realistic DB query scenario in which a staff member explores the database as a user and a SQL expert uses SQL to retrieve answers, clarify ambiguous questions, or otherwise inform.
-
Following the processing template of NSQL, the dataset underwent basic processing, yielding approximately 20W dataset
DB-GPT-Hub currently supports the following base models:
- [x] CodeLlama
- [x] Baichuan2
- [x] LLaMa/LLaMa2
- [x] Falcon
- [x] Qwen
- [x] XVERSE
- [x] ChatGLM2
- [x] ChatGLM3
- [x] internlm
- [x] sqlcoder-7b(mistral)
- [x] sqlcoder2-15b(starcoder)
The model is fine-tuned based on a quantization bit of 4 using Quantized Learning over Redundant Architecture (QLoRA). The minimum hardware requirements for this can be referred to as follows:
Model Parameters | GPU RAM | CPU RAM | DISK |
---|---|---|---|
7b | 6GB | 3.6GB | 36.4GB |
13b | 13.4GB | 5.9GB | 60.2GB |
All the related parameters are set to the minimum, with a batch size of 1 and max length of 512. Based on experience, for better performance, it is recommended to set the related length values to 1024 or 2048.
git clone https://github.com/eosphoros-ai/DB-GPT-Hub.git
cd DB-GPT-Hub
conda create -n dbgpt_hub python=3.10
conda activate dbgpt_hub
cd src/dbgpt_hub_sql
pip install -e .
Firstly, install dbgpt-hub
with the following command
pip install dbgpt-hub
Then, set up the arguments and run the whole process.
from dbgpt_hub_sql.data_process import preprocess_sft_data
from dbgpt_hub_sql.train import start_sft
from dbgpt_hub_sql.predict import start_predict
from dbgpt_hub_sql.eval import start_evaluate
# Config the input datasets
data_folder = "dbgpt_hub_sql/data"
data_info = [
{
"data_source": "spider",
"train_file": ["train_spider.json", "train_others.json"],
"dev_file": ["dev.json"],
"tables_file": "tables.json",
"db_id_name": "db_id",
"is_multiple_turn": False,
"train_output": "spider_train.json",
"dev_output": "spider_dev.json",
}
]
# Config training parameters
train_args = {
"model_name_or_path": "codellama/CodeLlama-13b-Instruct-hf",
"do_train": True,
"dataset": "example_text2sql_train",
"max_source_length": 2048,
"max_target_length": 512,
"finetuning_type": "lora",
"lora_target": "q_proj,v_proj",
"template": "llama2",
"lora_rank": 64,
"lora_alpha": 32,
"output_dir": "dbgpt_hub_sql/output/adapter/CodeLlama-13b-sql-lora",
"overwrite_cache": True,
"overwrite_output_dir": True,
"per_device_train_batch_size": 1,
"gradient_accumulation_steps": 16,
"lr_scheduler_type": "cosine_with_restarts",
"logging_steps": 50,
"save_steps": 2000,
"learning_rate": 2e-4,
"num_train_epochs": 8,
"plot_loss": True,
"bf16": True,
}
# Config predict parameters
predict_args = {
"model_name_or_path": "codellama/CodeLlama-13b-Instruct-hf",
"template": "llama2",
"finetuning_type": "lora",
"checkpoint_dir": "dbgpt_hub_sql/output/adapter/CodeLlama-13b-sql-lora",
"predict_file_path": "dbgpt_hub_sql/data/eval_data/dev_sql.json",
"predict_out_dir": "dbgpt_hub_sql/output/",
"predicted_out_filename": "pred_sql.sql",
}
# Config evaluation parameters
evaluate_args = {
"input": "./dbgpt_hub_sql/output/pred/pred_sql_dev_skeleton.sql",
"gold": "./dbgpt_hub_sql/data/eval_data/gold.txt",
"gold_natsql": "./dbgpt_hub_sql/data/eval_data/gold_natsql2sql.txt",
"db": "./dbgpt_hub_sql/data/spider/database",
"table": "./dbgpt_hub_sql/data/eval_data/tables.json",
"table_natsql": "./dbgpt_hub_sql/data/eval_data/tables_for_natsql2sql.json",
"etype": "exec",
"plug_value": True,
"keep_distict": False,
"progress_bar_for_each_datapoint": False,
"natsql": False,
}
# Run the whole fine-tuning workflow
preprocess_sft_data(
data_folder = data_folder,
data_info = data_info
)
start_sft(train_args)
start_predict(predict_args)
start_evaluate(evaluate_args)
DB-GPT-Hub uses the information matching generation method for data preparation, i.e. the SQL + Repository generation method that combines table information. This method combines data table information to better understand the structure and relationships of the data table, and is suitable for generating SQL statements that meet the requirements.
Download the Spider dataset from the Spider dataset link. By default, after downloading and extracting the data, place it in the dbgpt_hub_sql/data directory, i.e., the path should be dbgpt_hub_sql/data/spider
.
For the data preprocessing part, simply run the following script :
## generate train and dev(eval) data
sh dbgpt_hub_sql/scripts/gen_train_eval_data.sh
In the directory dbgpt_hub_sql/data/
, you will find the newly generated training file example_text2sql_train.json and testing file example_text2sql_dev.json, containing 8659 and 1034 entries respectively. For the data used in subsequent fine-tuning, set the parameter file_name
value to the file name of the training set in dbgpt_hub_sql/data/dataset_info.json, such as example_text2sql_train.json
The data in the generated JSON looks something like this:
{
"db_id": "department_management",
"instruction": "I want you to act as a SQL terminal in front of an example database, you need only to return the sql command to me.Below is an instruction that describes a task, Write a response that appropriately completes the request.\n\"\n##Instruction:\ndepartment_management contains tables such as department, head, management. Table department has columns such as Department_ID, Name, Creation, Ranking, Budget_in_Billions, Num_Employees. Department_ID is the primary key.\nTable head has columns such as head_ID, name, born_state, age. head_ID is the primary key.\nTable management has columns such as department_ID, head_ID, temporary_acting. department_ID is the primary key.\nThe head_ID of management is the foreign key of head_ID of head.\nThe department_ID of management is the foreign key of Department_ID of department.\n\n",
"input": "###Input:\nHow many heads of the departments are older than 56 ?\n\n###Response:",
"output": "SELECT count(*) FROM head WHERE age > 56",
"history": []
},
The data processing code of chase
, cosql
and sparc
has been embedded in the data processing code of the project. After downloading the data set according to the above link, you only need to add in
dbgpt_hub_sql/configs/config.py Just loosen the corresponding code comment in SQL_DATA_INFO
.
The model fine-tuning supports both LoRA and QLoRA methods. We can run the following command to fine-tune the model. By default, with the parameter --quantization_bit, it uses the QLoRA fine-tuning method. To switch to LoRAs, simply remove the related parameter from the script. Run the command:
sh dbgpt_hub_sql/scripts/train_sft.sh
After fine-tuning, the model weights will be saved by default in the adapter folder, specifically in the dbgpt_hub_sql/output/adapter directory.
If you're using multi-GPU training and want to utilize deepseed, you should modify the default content in train_sft.sh. The change is:
CUDA_VISIBLE_DEVICES=0 python dbgpt_hub_sql/train/sft_train.py \
--quantization_bit 4 \
...
change to :
deepspeed --num_gpus 2 dbgpt_hub_sql/train/sft_train.py \
--deepspeed dbgpt_hub_sql/configs/ds_config.json \
--quantization_bit 4 \
...
if you need order card id
deepspeed --include localhost:0,1 dbgpt_hub_sql/train/sft_train.py \
--deepspeed dbgpt_hub_sql/configs/ds_config.json \
--quantization_bit 4 \
...
The other parts that are omitted (…) can be kept consistent. If you want to change the default deepseed configuration, go into the dbgpt_hub_sql/configs
directory and make changes to ds_config.json as needed,the default is stage2.
In the script, during fine-tuning, different models correspond to key parameters lora_target and template, as shown in the following table:
model name | lora_target | template |
---|---|---|
LLaMA-2 | q_proj,v_proj | llama2 |
CodeLlama-2 | q_proj,v_proj | llama2 |
Baichuan2 | W_pack | baichuan2 |
Qwen | c_attn | chatml |
sqlcoder-7b | q_proj,v_proj | mistral |
sqlcoder2-15b | c_attn | default |
InternLM | q_proj,v_proj | intern |
XVERSE | q_proj,v_proj | xverse |
ChatGLM2 | query_key_value | chatglm2 |
LLaMA | q_proj,v_proj | - |
BLOOM | query_key_value | - |
BLOOMZ | query_key_value | - |
Baichuan | W_pack | baichuan |
Falcon | query_key_value | - |
In train_sft.sh
, other key parameters are as follows:
quantization_bit: Indicates whether quantization is applied, with valid values being [4 or 8].
model_name_or_path: The path of the LLM (Large Language Model).
dataset: Specifies the name of the training dataset configuration, corresponding to the outer key value in dbgpt_hub_sql/data/dataset_info.json, such as example_text2sql.
max_source_length: The length of the text input into the model. If computing resources allow, it can be set as large as possible, like 1024 or 2048.
max_target_length: The length of the SQL content output by the model; 512 is generally sufficient.
output_dir: The output path of the Peft module during SFT (Supervised Fine-Tuning), set by default todbgpt_hub_sql/output/adapter/
.
per_device_train_batch_size: The size of the batch. If computing resources allow, it can be set larger; the default is 1.
gradient_accumulation_steps: The number of steps for accumulating gradients before an update.
save_steps: The number of steps at which model checkpoints are saved; it can be set to 100 by default.
num_train_epochs: The number of epochs for training the dataset.
Under the project directory ./dbgpt_hub_sql/output/pred/, this folder is the default output location for model predictions(if not exist, just mkdir).
sh ./dbgpt_hub_sql/scripts/predict_sft.sh
In the script, by default with the parameter --quantization_bit
, it predicts using QLoRA. Removing it switches to the LoRA prediction method.
The value of the parameter predicted_input_filename
is your predict test dataset file. --predicted_out_filename
is the file name of the model's predicted results.
You can find the second corresponding model weights from Huggingface hg-eosphoros-ai ,we uploaded the LoRA weights in October,which execution accuracy on the Spider evaluation set reached 0.789.
If you need to merge the weights of the trained base model and the fine-tuned Peft module to export a complete model, execute the following model export script:
sh ./dbgpt_hub_sql/scripts/export_merge.sh
Be sure to replace the parameter path values in the script with the paths corresponding to your project.
To evaluate model performance on the dataset, default is spider dev dataset. Run the following command:
python dbgpt_hub_sql/eval/evaluation.py --plug_value --input Your_model_pred_file
You can find the results of our latest review and part of experiment results here
Note: The database pointed to by the default code is a 95M database downloaded from [Spider official website] (https://yale-lily.github.io/spider). If you need to use Spider database (size 1.27G) in test-suite, please download the database in the link to the custom directory first, and run the above evaluation command which add parameters and values like --db Your_download_db_path
.
The whole process we will divide into three phases:
-
Stage 1:
- Set up the foundational framework, enabling an end-to-end workflow that encompasses data processing, model SFT (Single Fine-Tuning) training, prediction output, and evaluation using multiple large language models (LLMs). As of August 4th, 2023, the entire pipeline has been successfully established.
Currently, we offer support for the following features:
- [x] CodeLlama
- [x] Baichuan2
- [x] LLaMa/LLaMa2
- [x] Falcon
- [x] Qwen
- [x] XVERSE
- [x] ChatGLM2
- [x] ChatGLM3
- [x] internlm
- [x] sqlcoder-7b(mistral)
- [x] sqlcoder2-15b(starcoder)
-
Stage 2:
- [x] Optidmize model performance, and support fine-tuning more different models in various ways before
20231010
- [x] Optimize
prompts
- [x] Release evaluation results, and optimized models open to peers.
- [x] Optidmize model performance, and support fine-tuning more different models in various ways before
-
Stage 3:
- [ ] Inference speed optimization and improvement
- [ ] Targeted optimization and improvement of business scenarios and Chinese effects
- [ ] Optimized based on more papers, such as RESDSQL and others. Combined with our community's sibling projectAwesome-Text2SQLfor further enhancements..
If our work has provided even a small measure of assistance to you, please consider giving us a star. Your feedback and support serve as motivation for us to continue releasing more related work and improving our efforts. Thank you!
We warmly invite more individuals to join us and actively engage in various aspects of our project, such as datasets, model fine-tuning, performance evaluation, paper recommendations, and code reproduction. Please don't hesitate to open issues or pull requests (PRs), and we will be proactive in responding to your contributions.
Before submitting your code, please ensure that it is formatted according to the black style by using the following command:
black dbgpt_hub
If you have more time to execute more detailed type checking and style checking of your code, please use the following command:
pyright dbgpt_hub
pylint dbgpt_hub
If you have any questions or need further assistance, don't hesitate to reach out. We appreciate your involvement!
Our work is primarily based on the foundation of numerous open-source contributions. Thanks to the following open source projects
- Spider
- CoSQL
- Chase
- BIRD-SQL
- LLaMA
- BLOOM
- Falcon
- ChatGLM
- WizardLM
- text-to-sql-wizardcoder
- test-suite-sql-eval
- LLaMa-Efficient-Tuning
Thanks to all the contributors, especially @JBoRu who raised the issue which reminded us to add a new promising evaluation way, i.e. Test Suite. As the paper 《SQL-PALM: IMPROVED LARGE LANGUAGE MODEL ADAPTATION FOR TEXT-TO-SQL》 mentioned, "We consider two commonly-used evaluation metrics: execution accuracy (EX) and test-suite accuracy (TS). EX measures whether the SQL execution outcome matches ground truth (GT), whereas TS measures whether the SQL passes all EX evaluations for multiple tests, generated by database augmentation. Since EX contains false positives, we consider TS as a more reliable evaluation metric".
If you find DB-GPT-Hub
useful for your research or development, please cite the following paper:
@misc{zhou2024dbgpthub,
title={DB-GPT-Hub: Towards Open Benchmarking Text-to-SQL Empowered by Large Language Models},
author={Fan Zhou and Siqiao Xue and Danrui Qi and Wenhui Shi and Wang Zhao and Ganglin Wei and Hongyang Zhang and Caigai Jiang and Gangwei Jiang and Zhixuan Chu and Faqiang Chen},
year={2024},
eprint={2406.11434},
archivePrefix={arXiv},
primaryClass={id='cs.DB' full_name='Databases' is_active=True alt_name=None in_archive='cs' is_general=False description='Covers database management, datamining, and data processing. Roughly includes material in ACM Subject Classes E.2, E.5, H.0, H.2, and J.1.'}
}
The MIT License (MIT)
We are collaborating as a community, and if you have any ideas regarding our community work, please don't hesitate to get in touch with us. If you're interested in delving into an in-depth experiment and optimizing the DB-GPT-Hub subproject, you can reach out to 'wangzai' within the WeChat group. We wholeheartedly welcome your contributions to making it even better together!
For Tasks:
Click tags to check more tools for each tasksFor Jobs:
Alternative AI tools for DB-GPT-Hub
Similar Open Source Tools
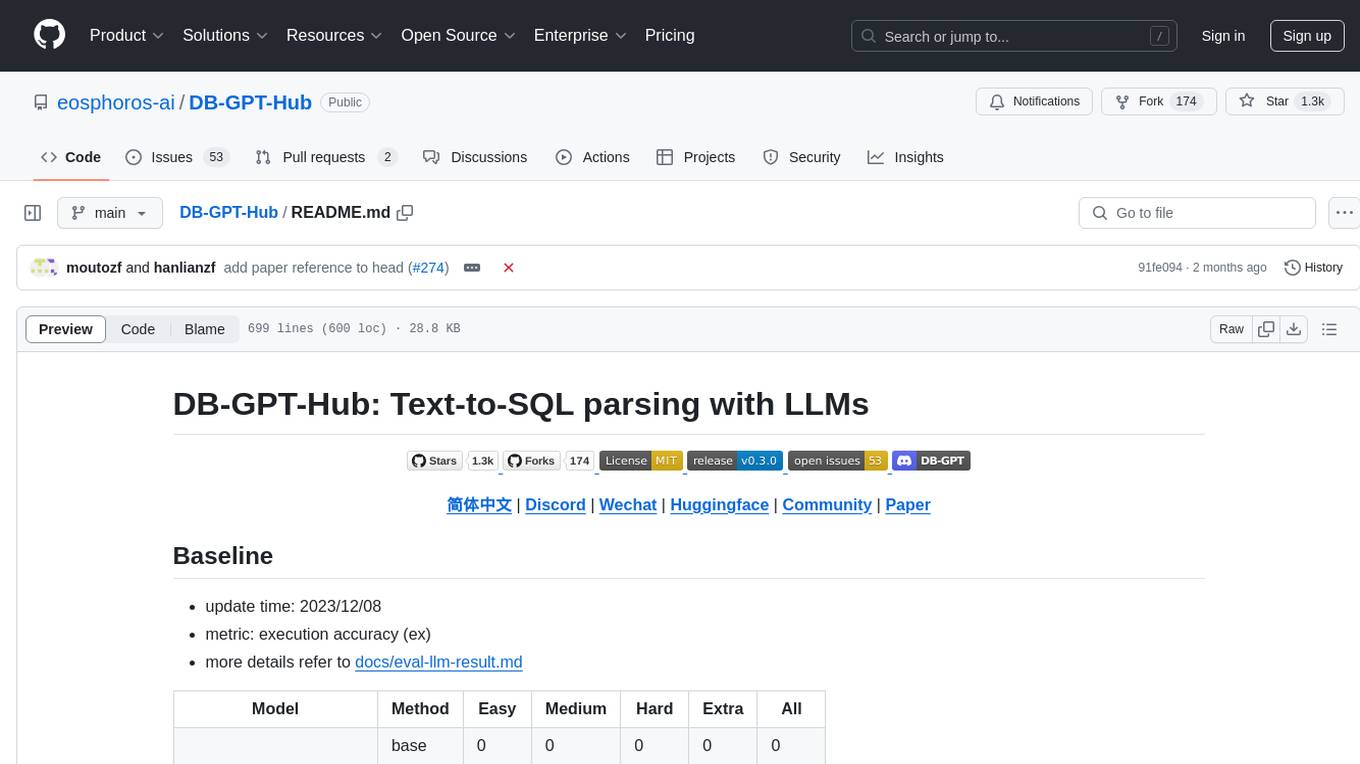
DB-GPT-Hub
DB-GPT-Hub is an experimental project leveraging Large Language Models (LLMs) for Text-to-SQL parsing. It includes stages like data collection, preprocessing, model selection, construction, and fine-tuning of model weights. The project aims to enhance Text-to-SQL capabilities, reduce model training costs, and enable developers to contribute to improving Text-to-SQL accuracy. The ultimate goal is to achieve automated question-answering based on databases, allowing users to execute complex database queries using natural language descriptions. The project has successfully integrated multiple large models and established a comprehensive workflow for data processing, SFT model training, prediction output, and evaluation.
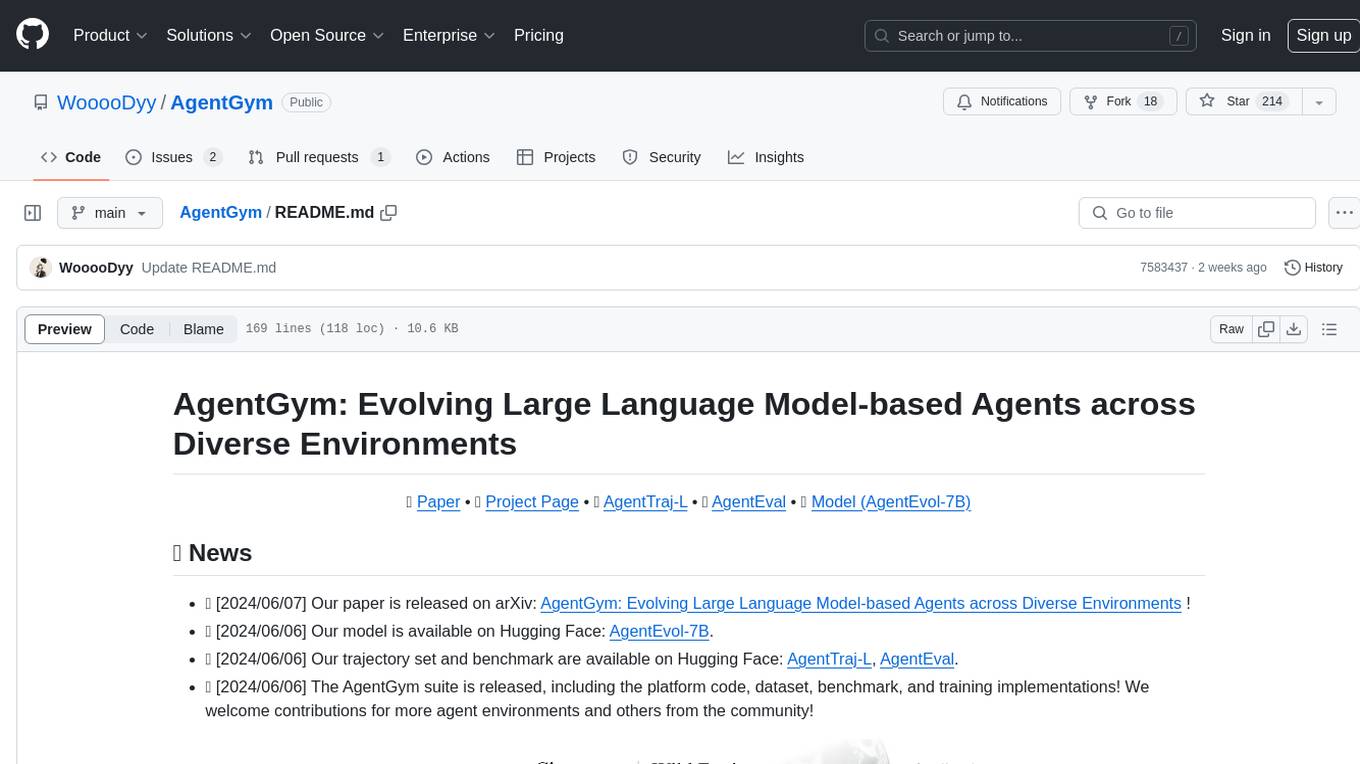
AgentGym
AgentGym is a framework designed to help the AI community evaluate and develop generally-capable Large Language Model-based agents. It features diverse interactive environments and tasks with real-time feedback and concurrency. The platform supports 14 environments across various domains like web navigating, text games, house-holding tasks, digital games, and more. AgentGym includes a trajectory set (AgentTraj) and a benchmark suite (AgentEval) to facilitate agent exploration and evaluation. The framework allows for agent self-evolution beyond existing data, showcasing comparable results to state-of-the-art models.
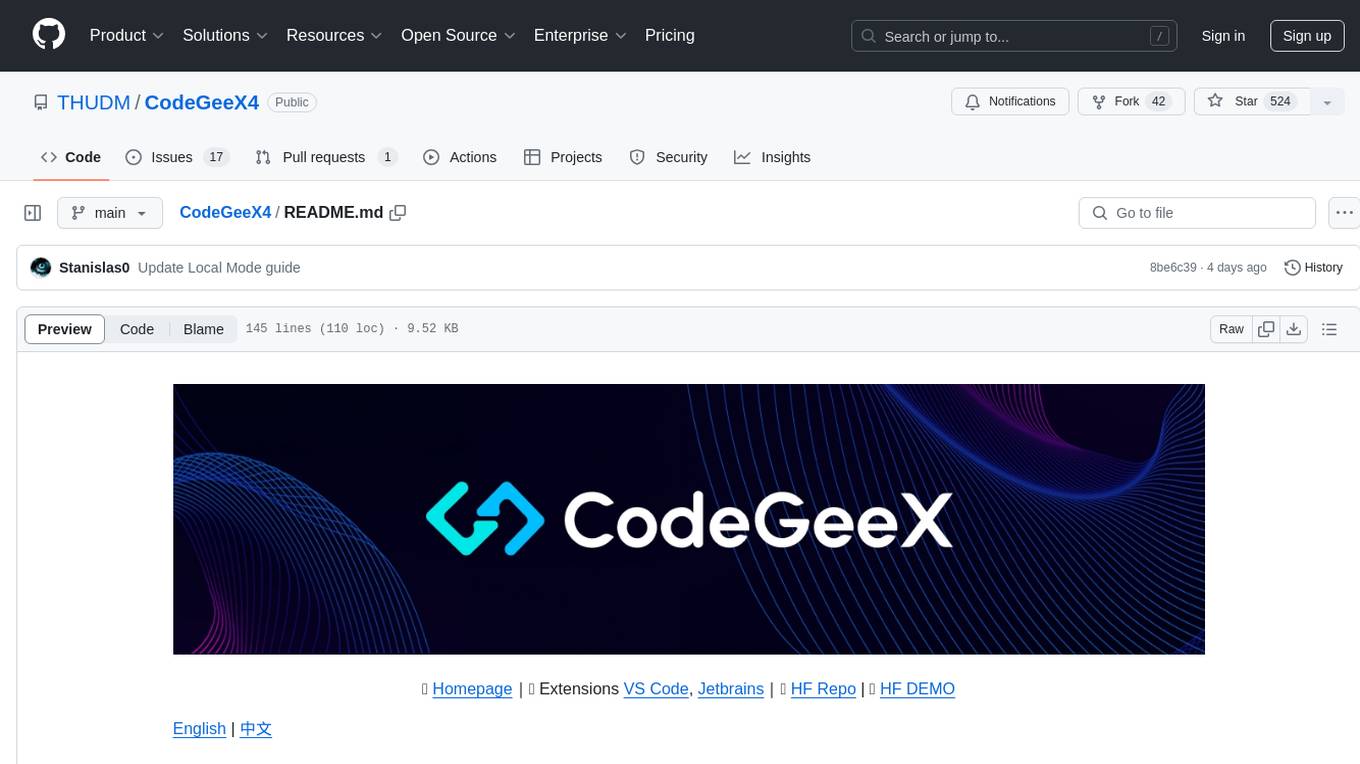
CodeGeeX4
CodeGeeX4-ALL-9B is an open-source multilingual code generation model based on GLM-4-9B, offering enhanced code generation capabilities. It supports functions like code completion, code interpreter, web search, function call, and repository-level code Q&A. The model has competitive performance on benchmarks like BigCodeBench and NaturalCodeBench, outperforming larger models in terms of speed and performance.
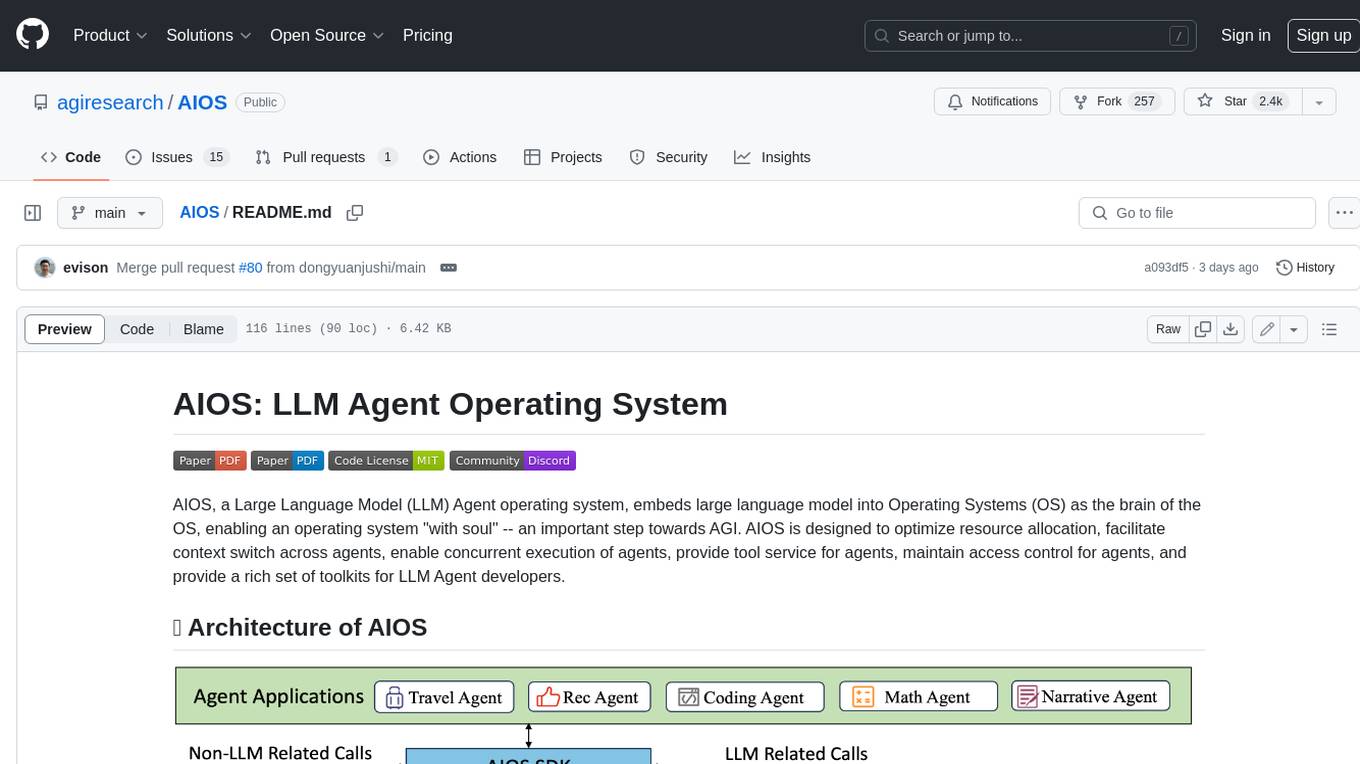
AIOS
AIOS, a Large Language Model (LLM) Agent operating system, embeds large language model into Operating Systems (OS) as the brain of the OS, enabling an operating system "with soul" -- an important step towards AGI. AIOS is designed to optimize resource allocation, facilitate context switch across agents, enable concurrent execution of agents, provide tool service for agents, maintain access control for agents, and provide a rich set of toolkits for LLM Agent developers.
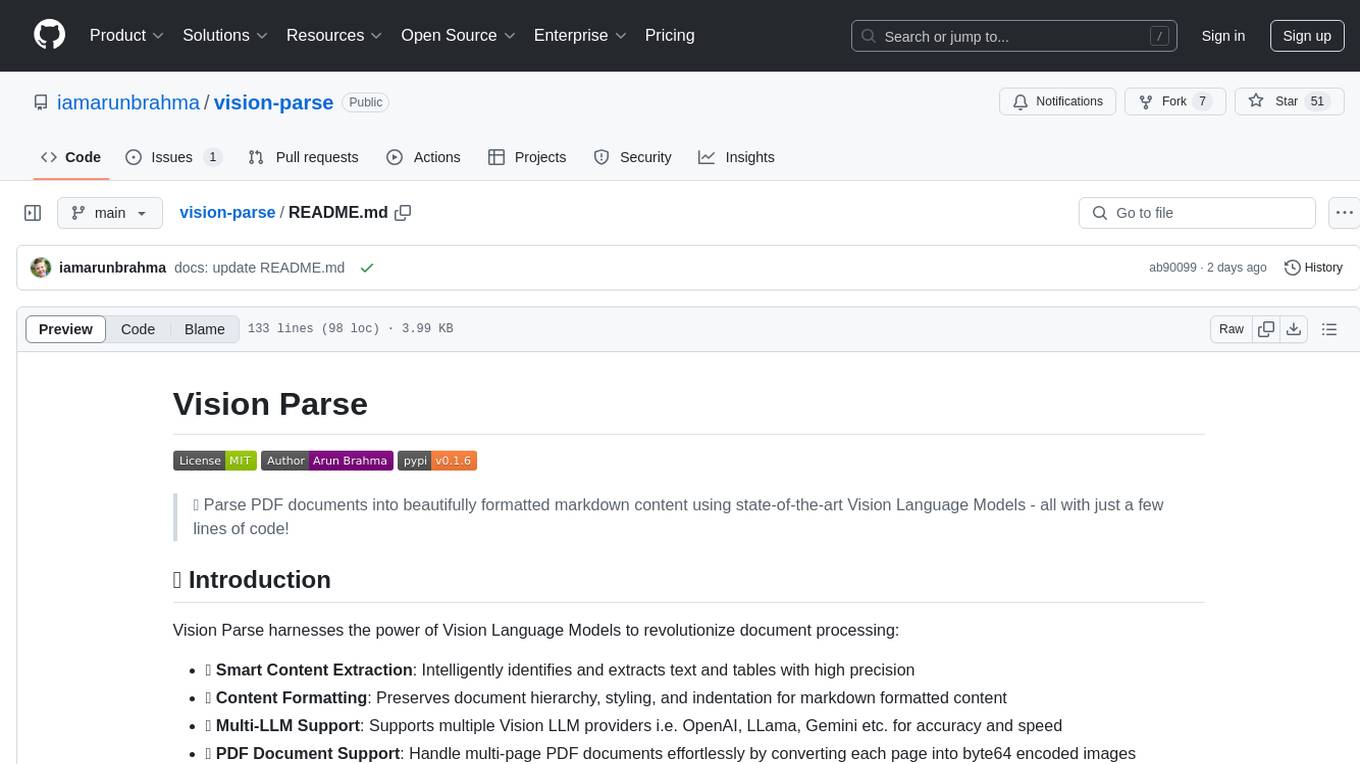
vision-parse
Vision Parse is a tool that leverages Vision Language Models to parse PDF documents into beautifully formatted markdown content. It offers smart content extraction, content formatting, multi-LLM support, PDF document support, and local model hosting using Ollama. Users can easily convert PDFs to markdown with high precision and preserve document hierarchy and styling. The tool supports multiple Vision LLM providers like OpenAI, LLama, and Gemini for accuracy and speed, making document processing efficient and effortless.
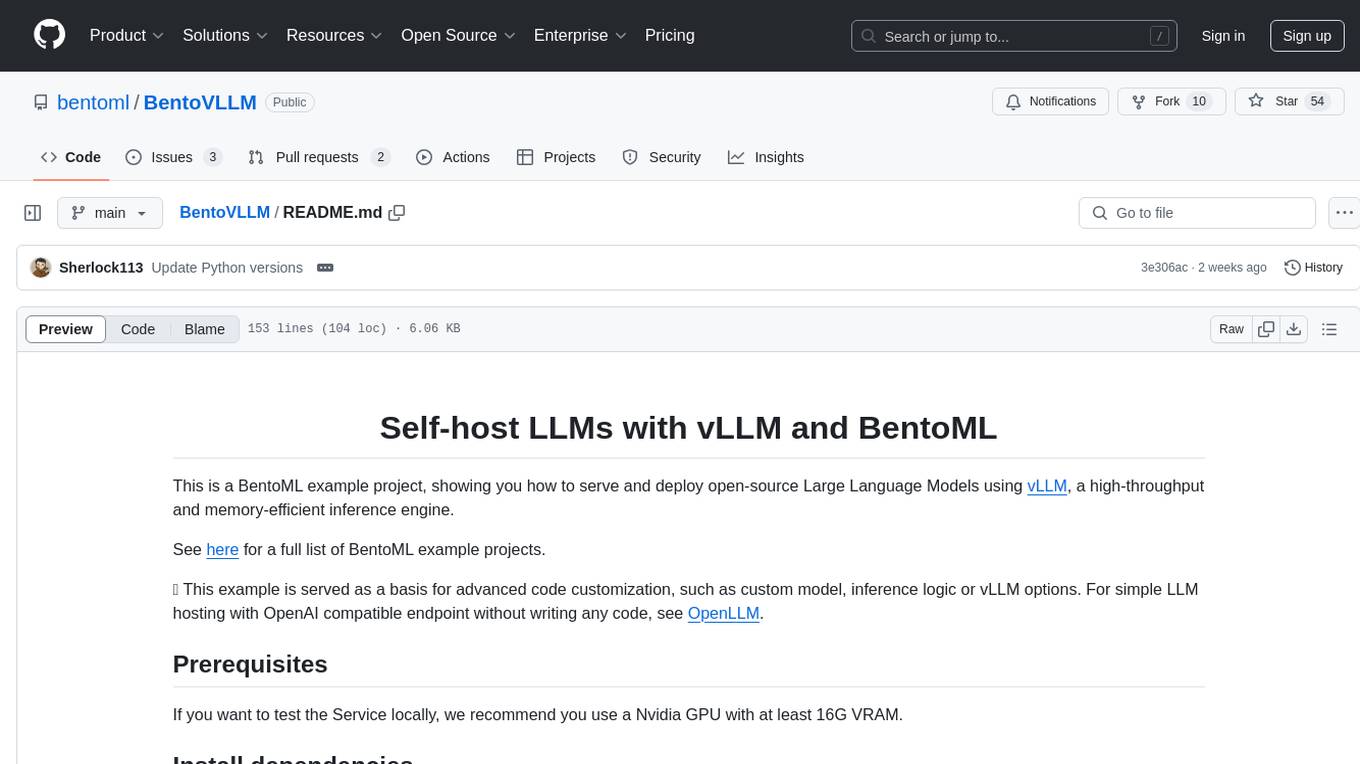
BentoVLLM
BentoVLLM is an example project demonstrating how to serve and deploy open-source Large Language Models using vLLM, a high-throughput and memory-efficient inference engine. It provides a basis for advanced code customization, such as custom models, inference logic, or vLLM options. The project allows for simple LLM hosting with OpenAI compatible endpoints without the need to write any code. Users can interact with the server using Swagger UI or other methods, and the service can be deployed to BentoCloud for better management and scalability. Additionally, the repository includes integration examples for different LLM models and tools.
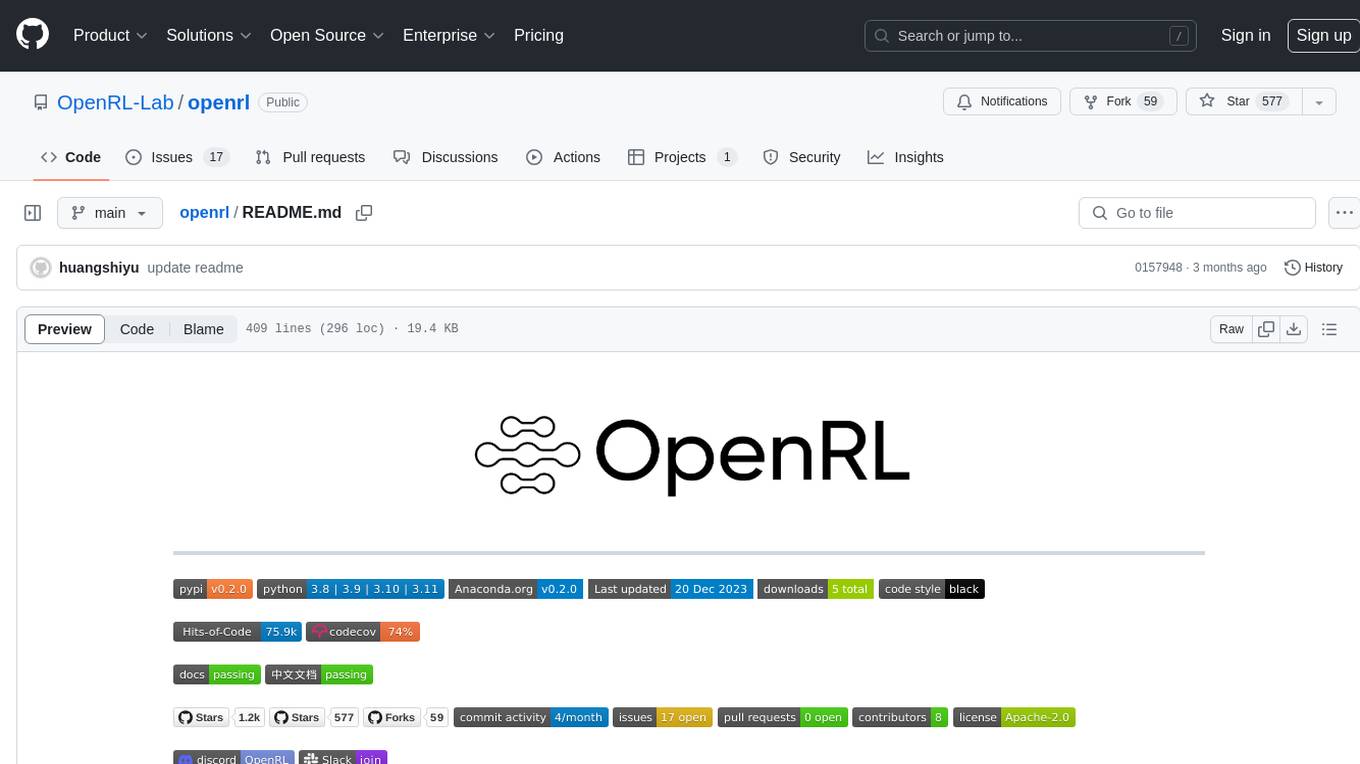
openrl
OpenRL is an open-source general reinforcement learning research framework that supports training for various tasks such as single-agent, multi-agent, offline RL, self-play, and natural language. Developed based on PyTorch, the goal of OpenRL is to provide a simple-to-use, flexible, efficient and sustainable platform for the reinforcement learning research community. It supports a universal interface for all tasks/environments, single-agent and multi-agent tasks, offline RL training with expert dataset, self-play training, reinforcement learning training for natural language tasks, DeepSpeed, Arena for evaluation, importing models and datasets from Hugging Face, user-defined environments, models, and datasets, gymnasium environments, callbacks, visualization tools, unit testing, and code coverage testing. It also supports various algorithms like PPO, DQN, SAC, and environments like Gymnasium, MuJoCo, Atari, and more.
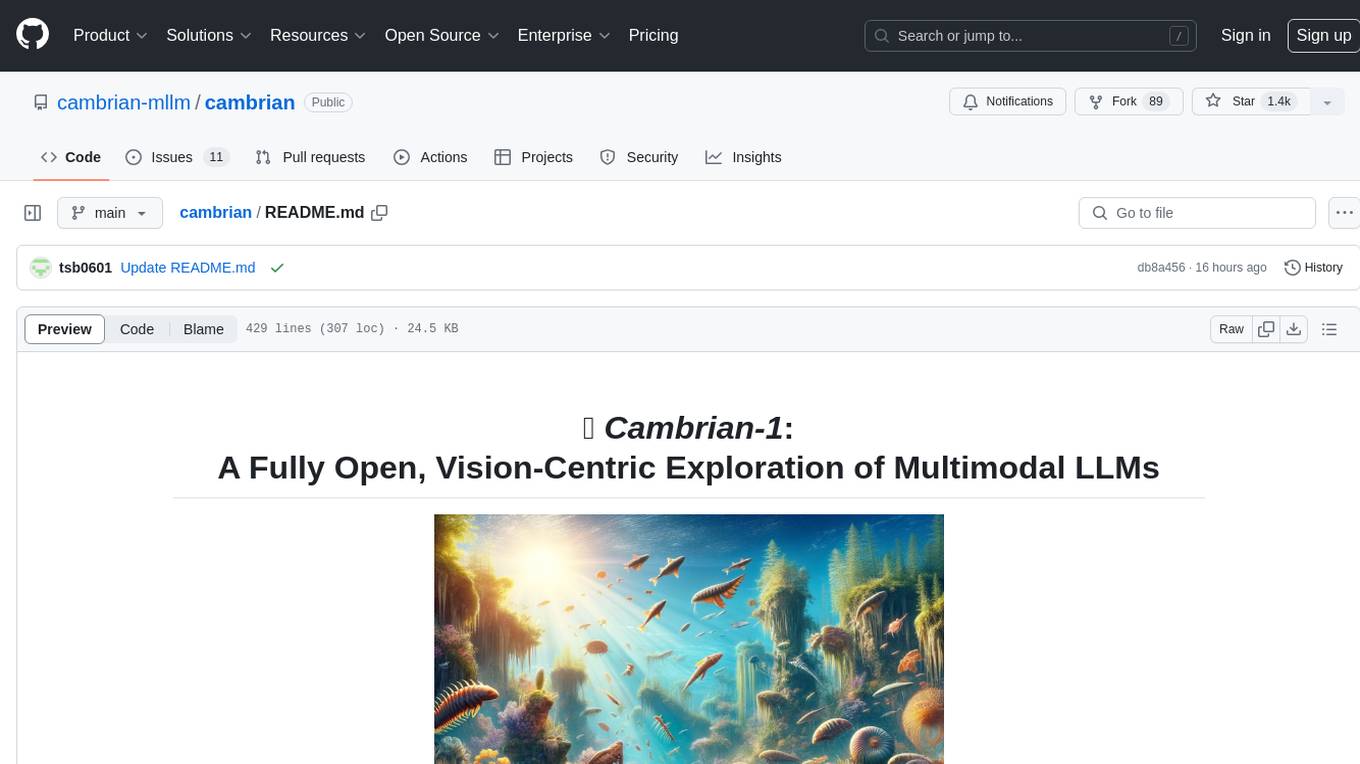
cambrian
Cambrian-1 is a fully open project focused on exploring multimodal Large Language Models (LLMs) with a vision-centric approach. It offers competitive performance across various benchmarks with models at different parameter levels. The project includes training configurations, model weights, instruction tuning data, and evaluation details. Users can interact with Cambrian-1 through a Gradio web interface for inference. The project is inspired by LLaVA and incorporates contributions from Vicuna, LLaMA, and Yi. Cambrian-1 is licensed under Apache 2.0 and utilizes datasets and checkpoints subject to their respective original licenses.
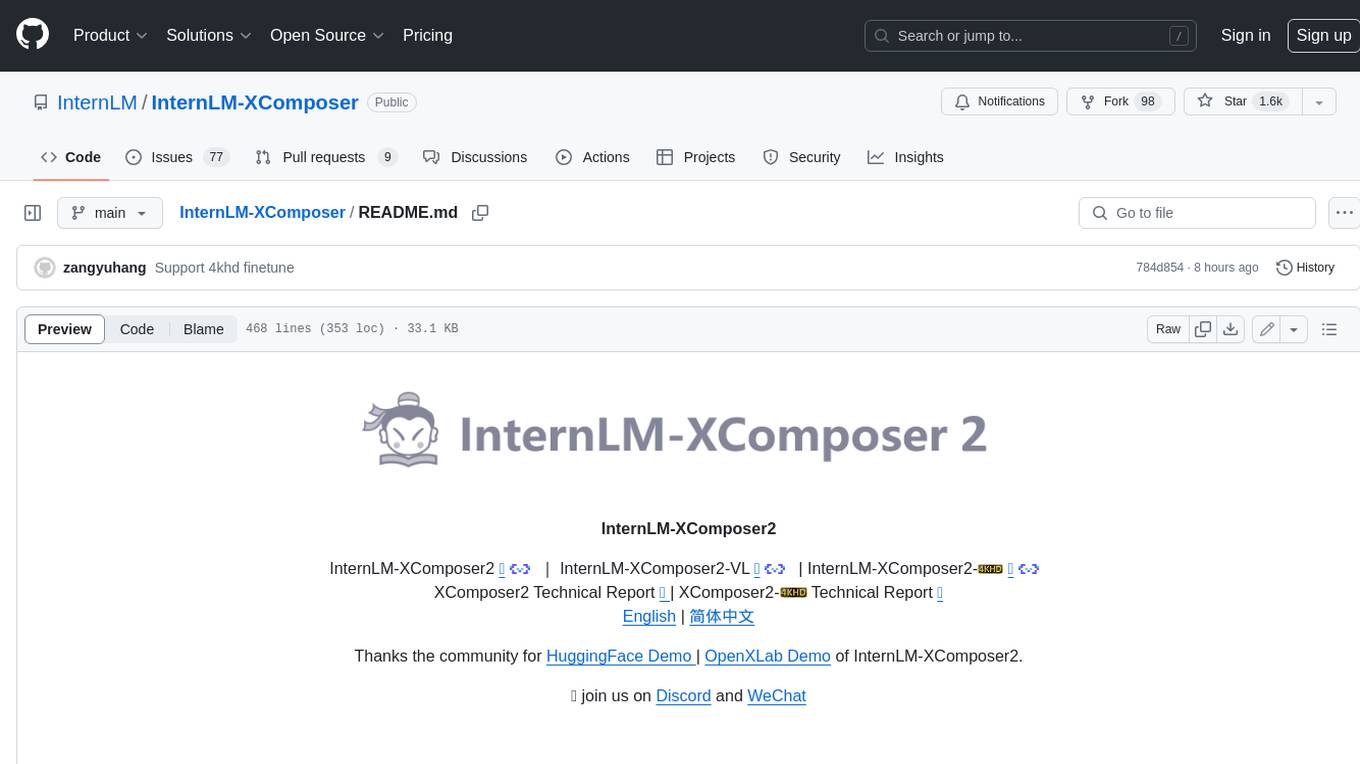
InternLM-XComposer
InternLM-XComposer2 is a groundbreaking vision-language large model (VLLM) based on InternLM2-7B excelling in free-form text-image composition and comprehension. It boasts several amazing capabilities and applications: * **Free-form Interleaved Text-Image Composition** : InternLM-XComposer2 can effortlessly generate coherent and contextual articles with interleaved images following diverse inputs like outlines, detailed text requirements and reference images, enabling highly customizable content creation. * **Accurate Vision-language Problem-solving** : InternLM-XComposer2 accurately handles diverse and challenging vision-language Q&A tasks based on free-form instructions, excelling in recognition, perception, detailed captioning, visual reasoning, and more. * **Awesome performance** : InternLM-XComposer2 based on InternLM2-7B not only significantly outperforms existing open-source multimodal models in 13 benchmarks but also **matches or even surpasses GPT-4V and Gemini Pro in 6 benchmarks** We release InternLM-XComposer2 series in three versions: * **InternLM-XComposer2-4KHD-7B** 🤗: The high-resolution multi-task trained VLLM model with InternLM-7B as the initialization of the LLM for _High-resolution understanding_ , _VL benchmarks_ and _AI assistant_. * **InternLM-XComposer2-VL-7B** 🤗 : The multi-task trained VLLM model with InternLM-7B as the initialization of the LLM for _VL benchmarks_ and _AI assistant_. **It ranks as the most powerful vision-language model based on 7B-parameter level LLMs, leading across 13 benchmarks.** * **InternLM-XComposer2-VL-1.8B** 🤗 : A lightweight version of InternLM-XComposer2-VL based on InternLM-1.8B. * **InternLM-XComposer2-7B** 🤗: The further instruction tuned VLLM for _Interleaved Text-Image Composition_ with free-form inputs. Please refer to Technical Report and 4KHD Technical Reportfor more details.
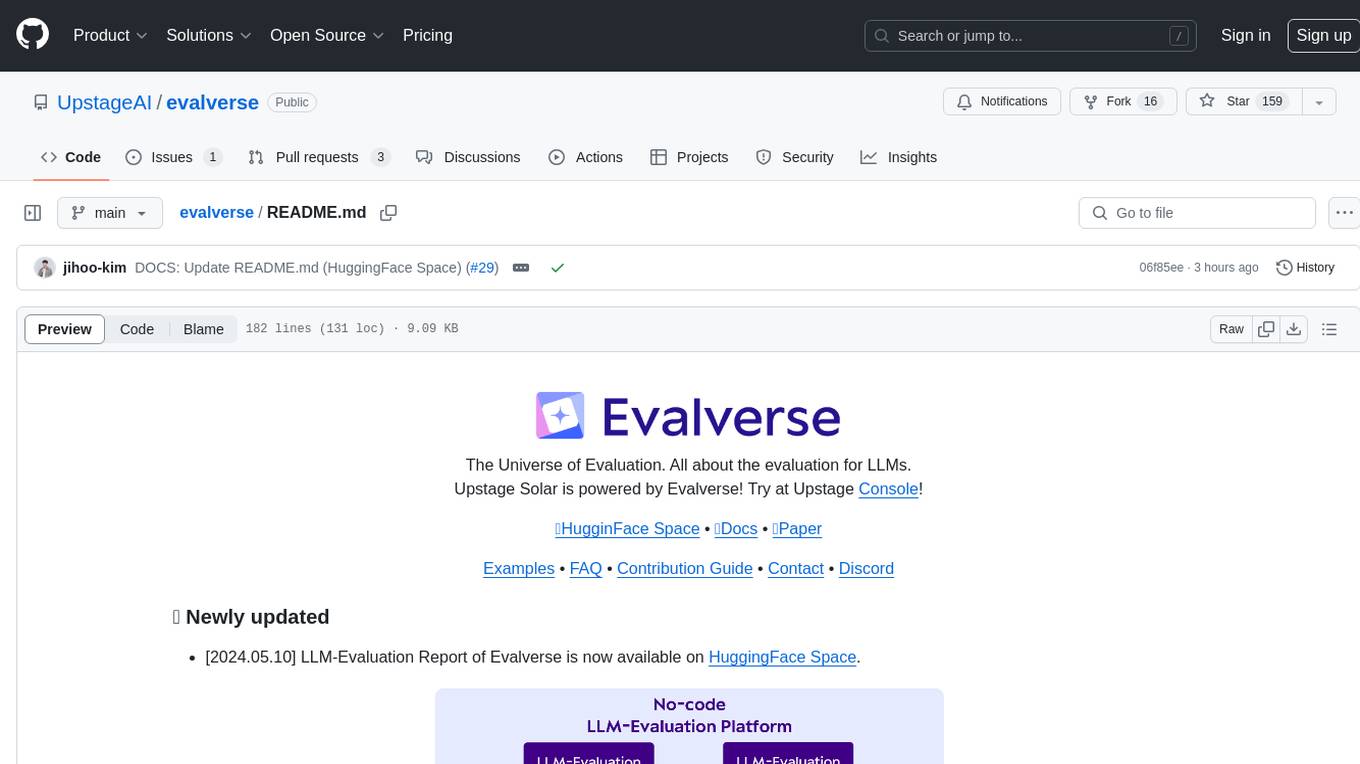
evalverse
Evalverse is an open-source project designed to support Large Language Model (LLM) evaluation needs. It provides a standardized and user-friendly solution for processing and managing LLM evaluations, catering to AI research engineers and scientists. Evalverse supports various evaluation methods, insightful reports, and no-code evaluation processes. Users can access unified evaluation with submodules, request evaluations without code via Slack bot, and obtain comprehensive reports with scores, rankings, and visuals. The tool allows for easy comparison of scores across different models and swift addition of new evaluation tools.
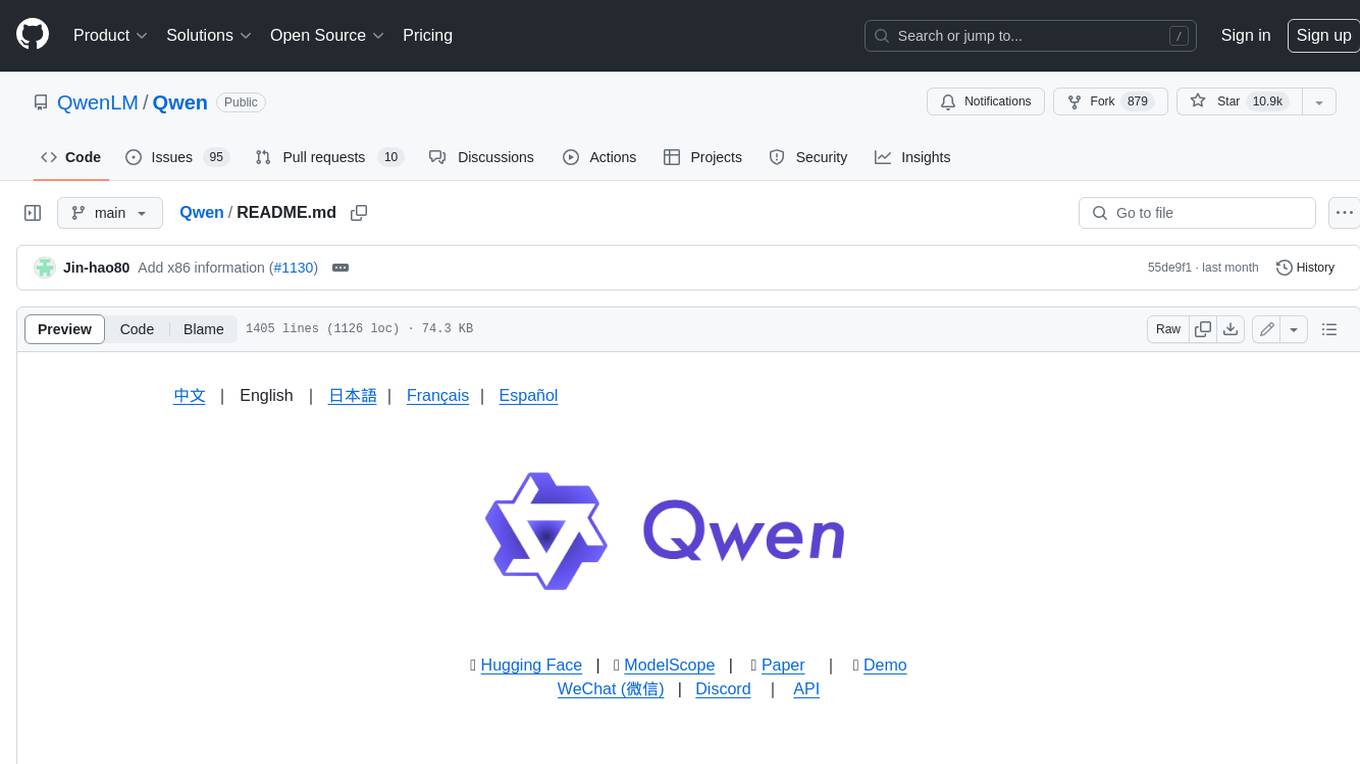
Qwen
Qwen is a series of large language models developed by Alibaba DAMO Academy. It outperforms the baseline models of similar model sizes on a series of benchmark datasets, e.g., MMLU, C-Eval, GSM8K, MATH, HumanEval, MBPP, BBH, etc., which evaluate the models’ capabilities on natural language understanding, mathematic problem solving, coding, etc. Qwen models outperform the baseline models of similar model sizes on a series of benchmark datasets, e.g., MMLU, C-Eval, GSM8K, MATH, HumanEval, MBPP, BBH, etc., which evaluate the models’ capabilities on natural language understanding, mathematic problem solving, coding, etc. Qwen-72B achieves better performance than LLaMA2-70B on all tasks and outperforms GPT-3.5 on 7 out of 10 tasks.
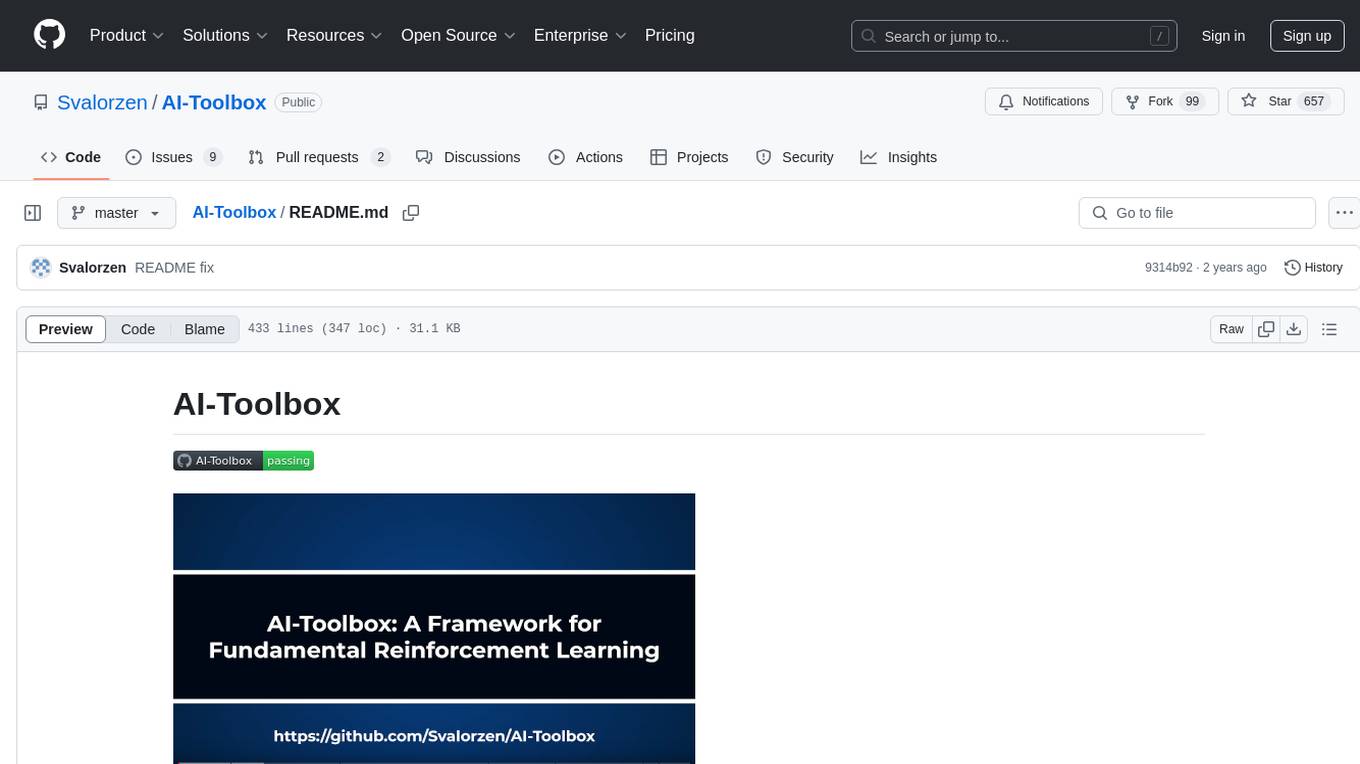
AI-Toolbox
AI-Toolbox is a C++ library aimed at representing and solving common AI problems, with a focus on MDPs, POMDPs, and related algorithms. It provides an easy-to-use interface that is extensible to many problems while maintaining readable code. The toolbox includes tutorials for beginners in reinforcement learning and offers Python bindings for seamless integration. It features utilities for combinatorics, polytopes, linear programming, sampling, distributions, statistics, belief updating, data structures, logging, seeding, and more. Additionally, it supports bandit/normal games, single agent MDP/stochastic games, single agent POMDP, and factored/joint multi-agent scenarios.
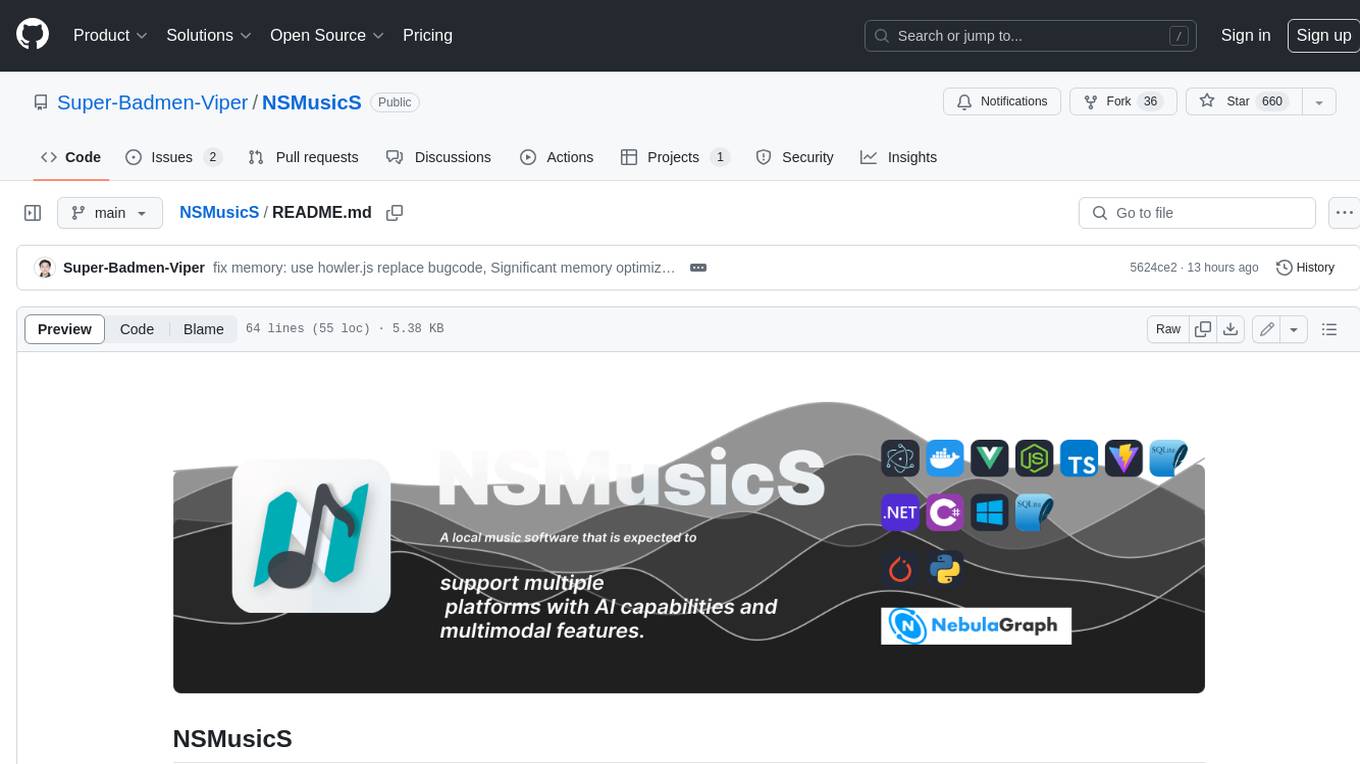
NSMusicS
NSMusicS is a local music software that is expected to support multiple platforms with AI capabilities and multimodal features. The goal of NSMusicS is to integrate various functions (such as artificial intelligence, streaming, music library management, cross platform, etc.), which can be understood as similar to Navidrome but with more features than Navidrome. It wants to become a plugin integrated application that can almost have all music functions.
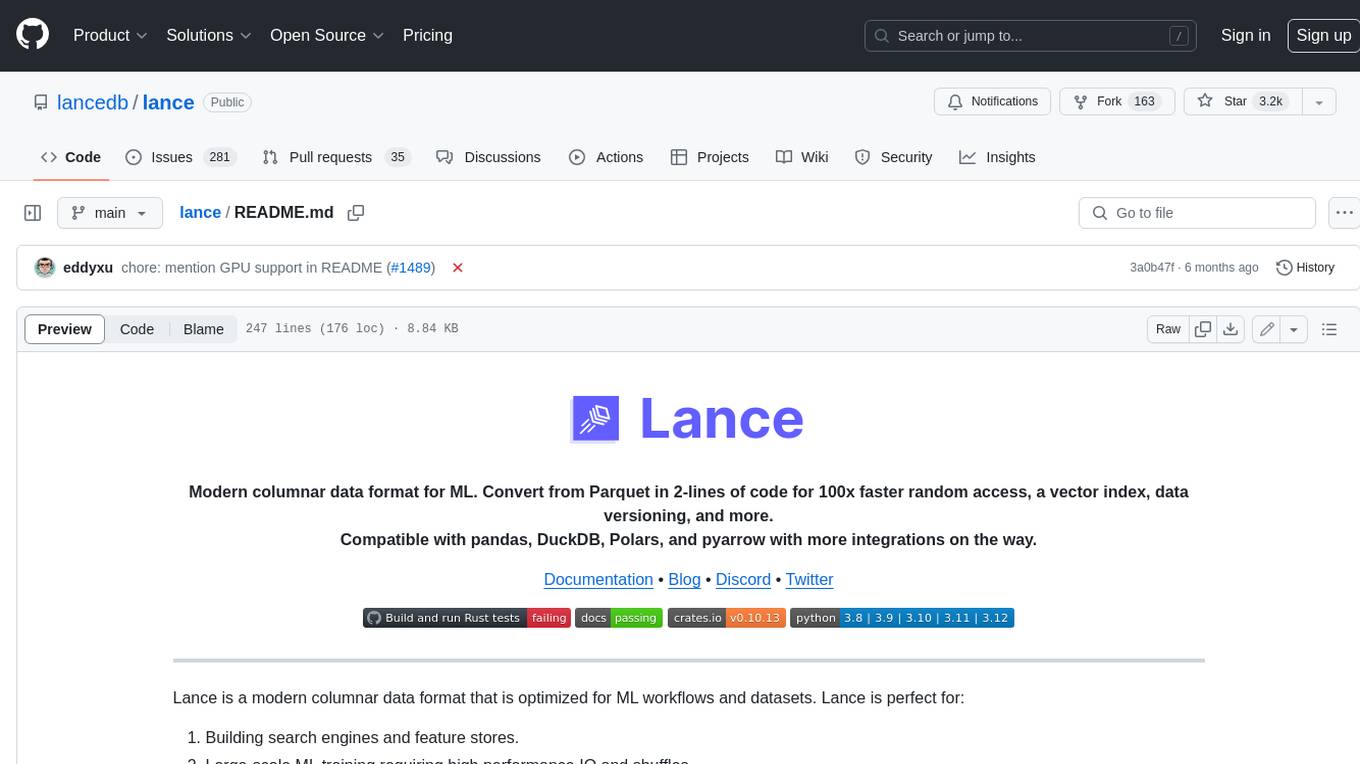
lance
Lance is a modern columnar data format optimized for ML workflows and datasets. It offers high-performance random access, vector search, zero-copy automatic versioning, and ecosystem integrations with Apache Arrow, Pandas, Polars, and DuckDB. Lance is designed to address the challenges of the ML development cycle, providing a unified data format for collection, exploration, analytics, feature engineering, training, evaluation, deployment, and monitoring. It aims to reduce data silos and streamline the ML development process.
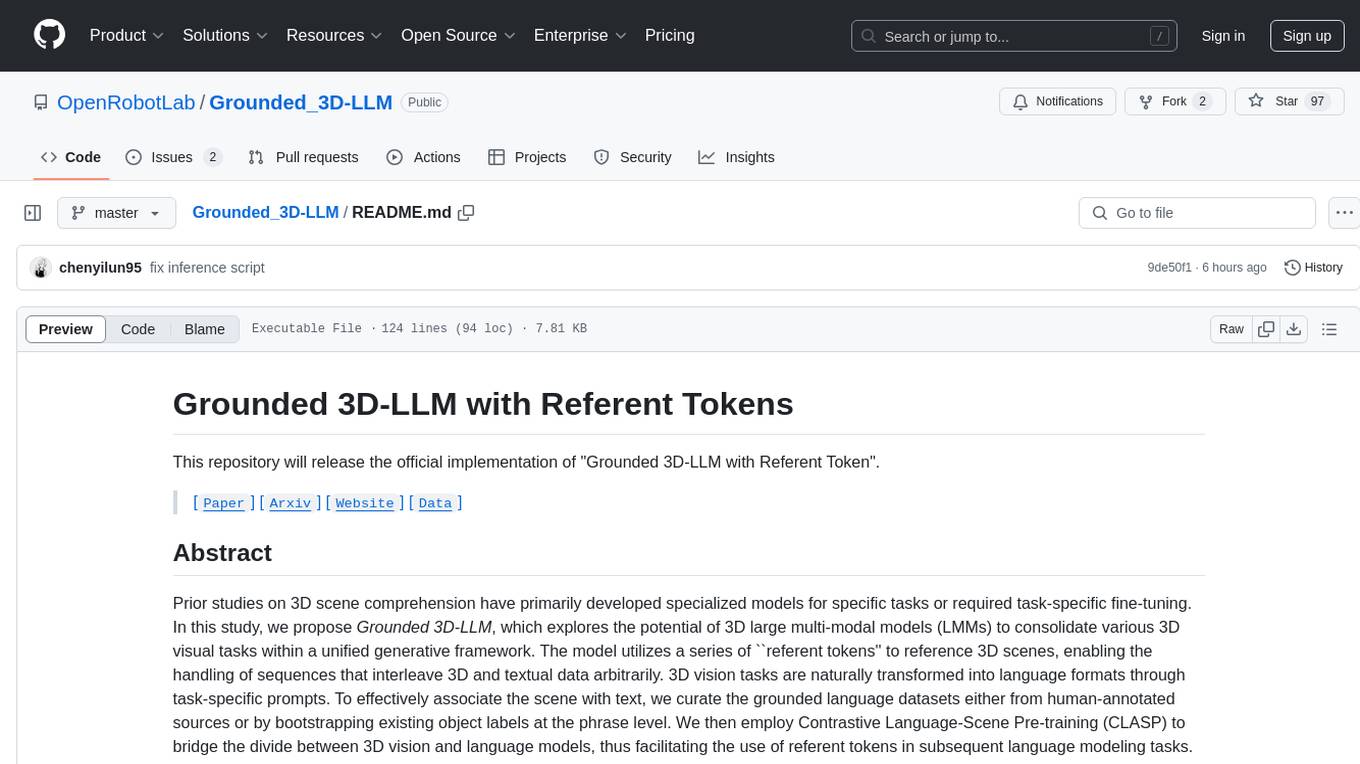
Grounded_3D-LLM
Grounded 3D-LLM is a unified generative framework that utilizes referent tokens to reference 3D scenes, enabling the handling of sequences that interleave 3D and textual data. It transforms 3D vision tasks into language formats through task-specific prompts, curating grounded language datasets and employing Contrastive Language-Scene Pre-training (CLASP) to bridge the gap between 3D vision and language models. The model covers tasks like 3D visual question answering, dense captioning, object detection, and language grounding.
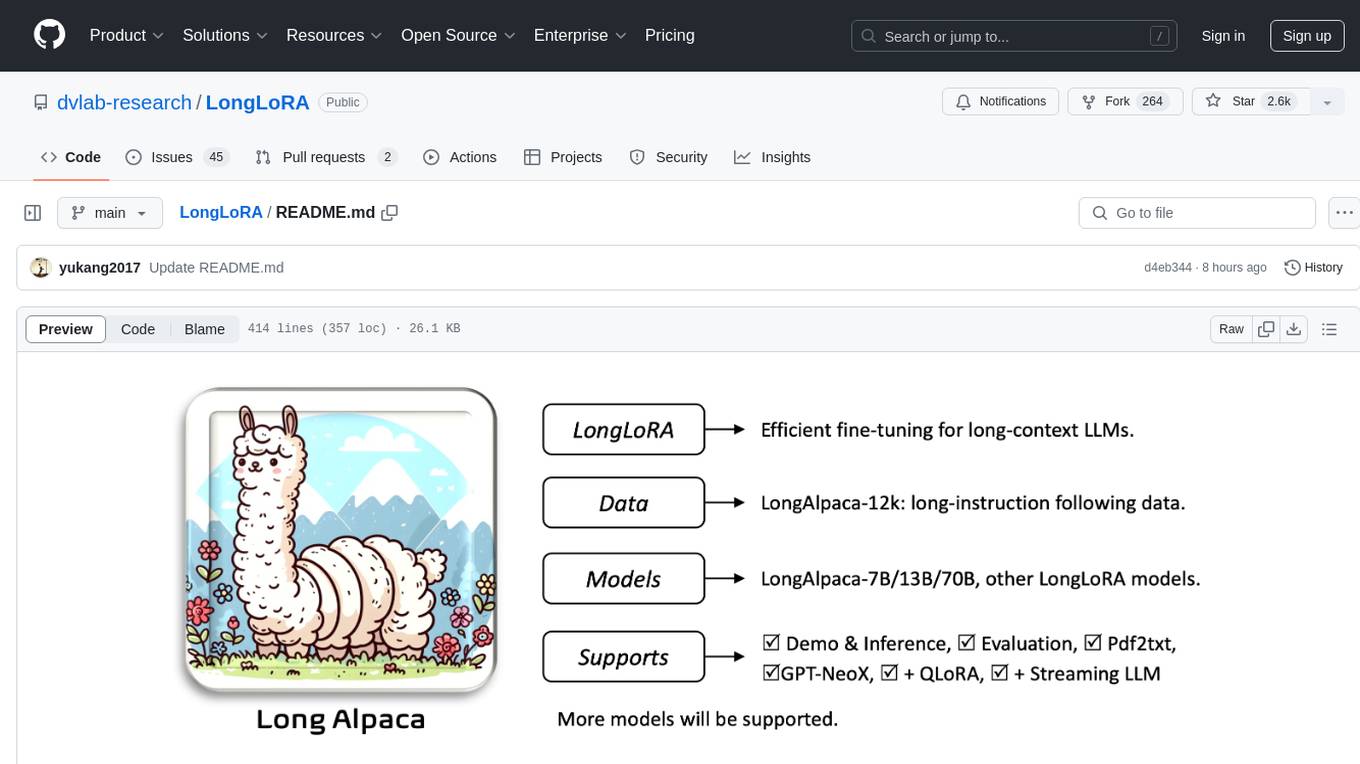
LongLoRA
LongLoRA is a tool for efficient fine-tuning of long-context large language models. It includes LongAlpaca data with long QA data collected and short QA sampled, models from 7B to 70B with context length from 8k to 100k, and support for GPTNeoX models. The tool supports supervised fine-tuning, context extension, and improved LoRA fine-tuning. It provides pre-trained weights, fine-tuning instructions, evaluation methods, local and online demos, streaming inference, and data generation via Pdf2text. LongLoRA is licensed under Apache License 2.0, while data and weights are under CC-BY-NC 4.0 License for research use only.
For similar tasks
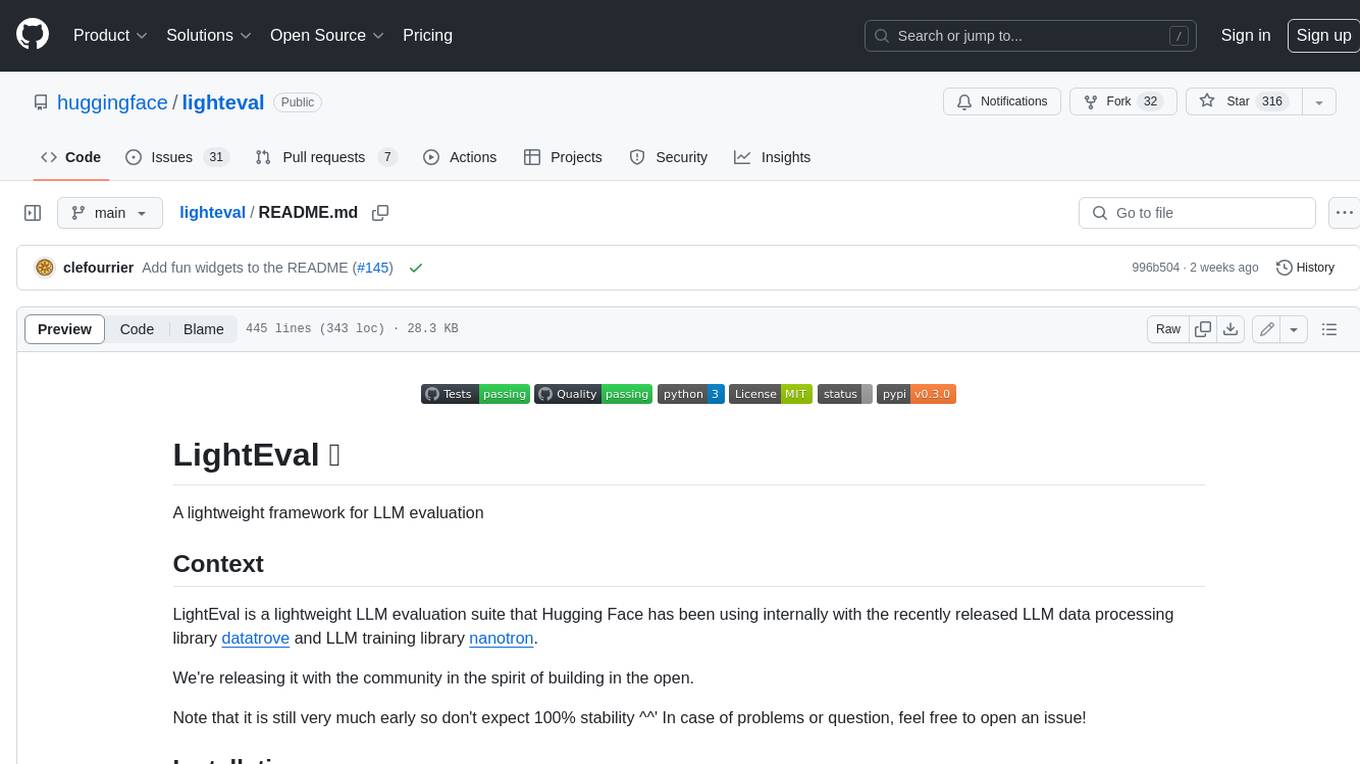
lighteval
LightEval is a lightweight LLM evaluation suite that Hugging Face has been using internally with the recently released LLM data processing library datatrove and LLM training library nanotron. We're releasing it with the community in the spirit of building in the open. Note that it is still very much early so don't expect 100% stability ^^' In case of problems or question, feel free to open an issue!
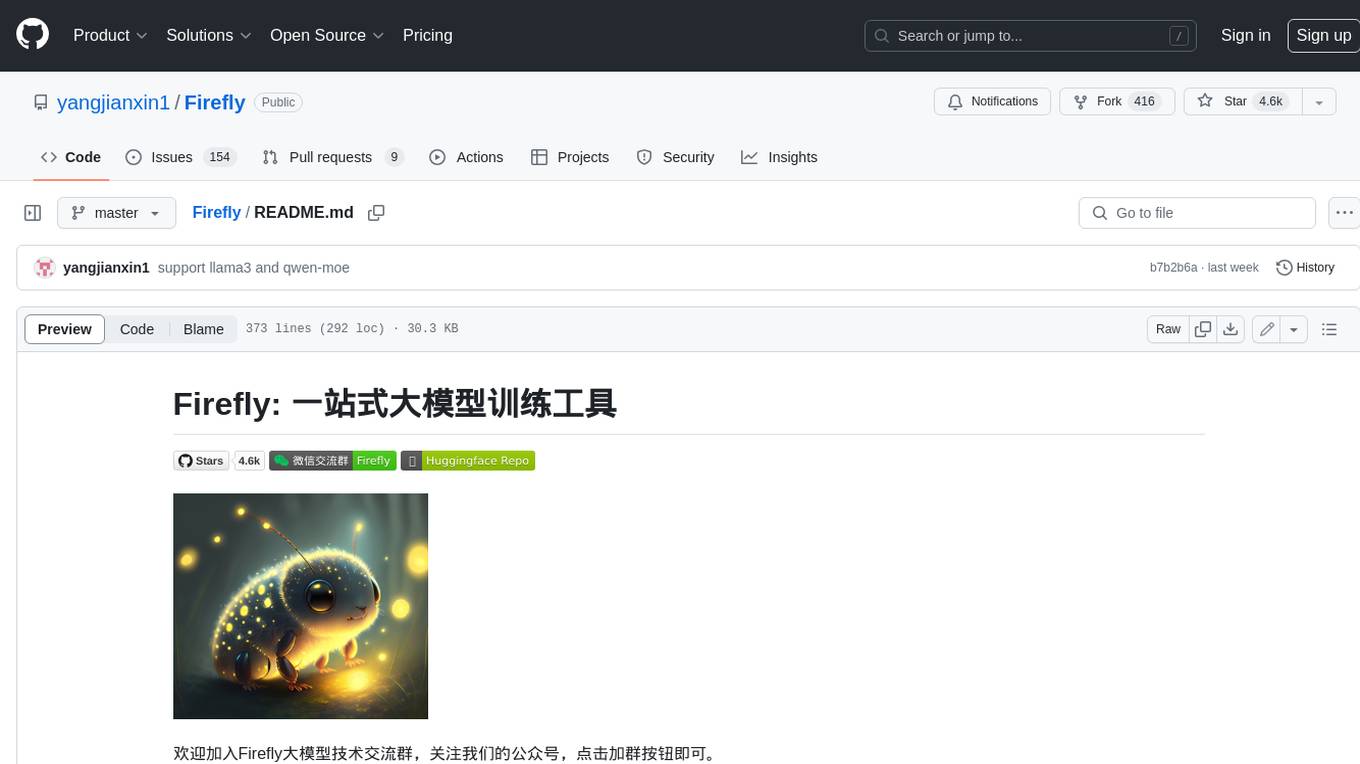
Firefly
Firefly is an open-source large model training project that supports pre-training, fine-tuning, and DPO of mainstream large models. It includes models like Llama3, Gemma, Qwen1.5, MiniCPM, Llama, InternLM, Baichuan, ChatGLM, Yi, Deepseek, Qwen, Orion, Ziya, Xverse, Mistral, Mixtral-8x7B, Zephyr, Vicuna, Bloom, etc. The project supports full-parameter training, LoRA, QLoRA efficient training, and various tasks such as pre-training, SFT, and DPO. Suitable for users with limited training resources, QLoRA is recommended for fine-tuning instructions. The project has achieved good results on the Open LLM Leaderboard with QLoRA training process validation. The latest version has significant updates and adaptations for different chat model templates.
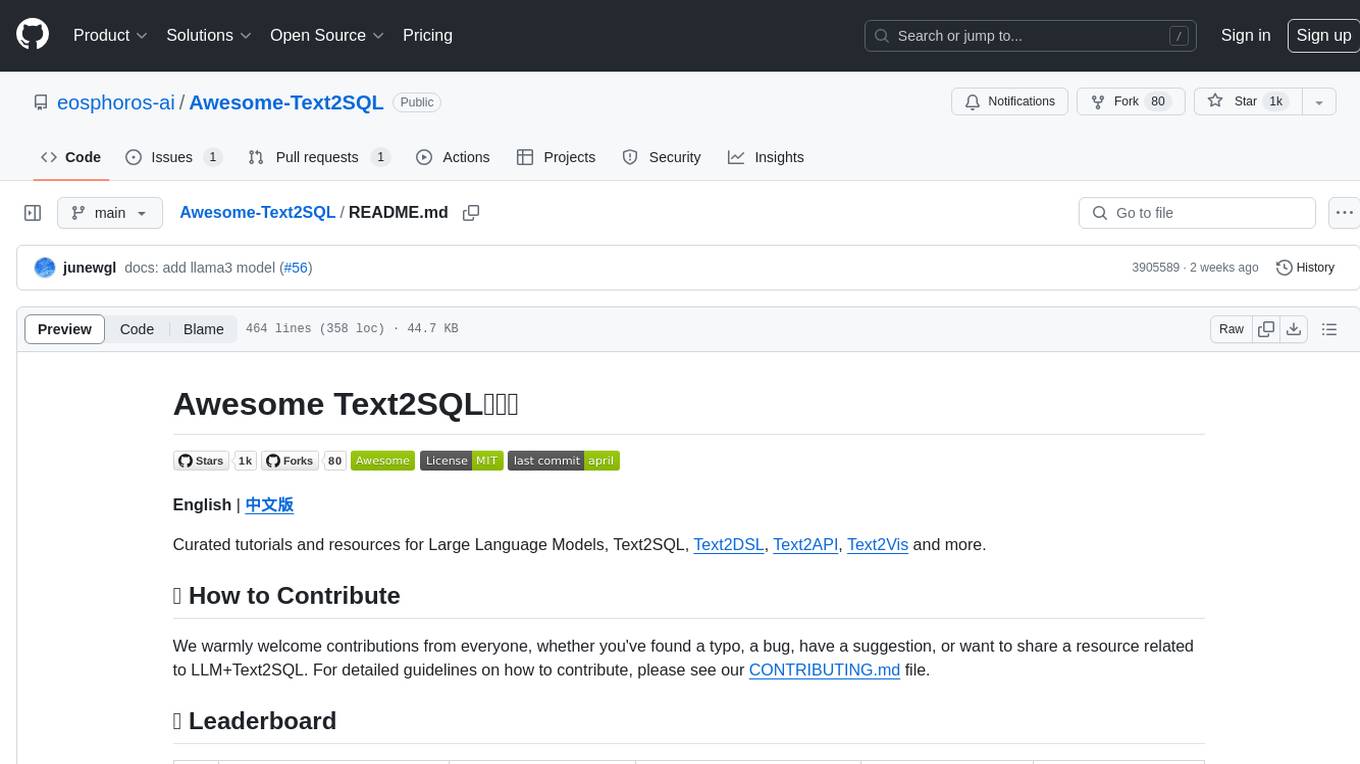
Awesome-Text2SQL
Awesome Text2SQL is a curated repository containing tutorials and resources for Large Language Models, Text2SQL, Text2DSL, Text2API, Text2Vis, and more. It provides guidelines on converting natural language questions into structured SQL queries, with a focus on NL2SQL. The repository includes information on various models, datasets, evaluation metrics, fine-tuning methods, libraries, and practice projects related to Text2SQL. It serves as a comprehensive resource for individuals interested in working with Text2SQL and related technologies.
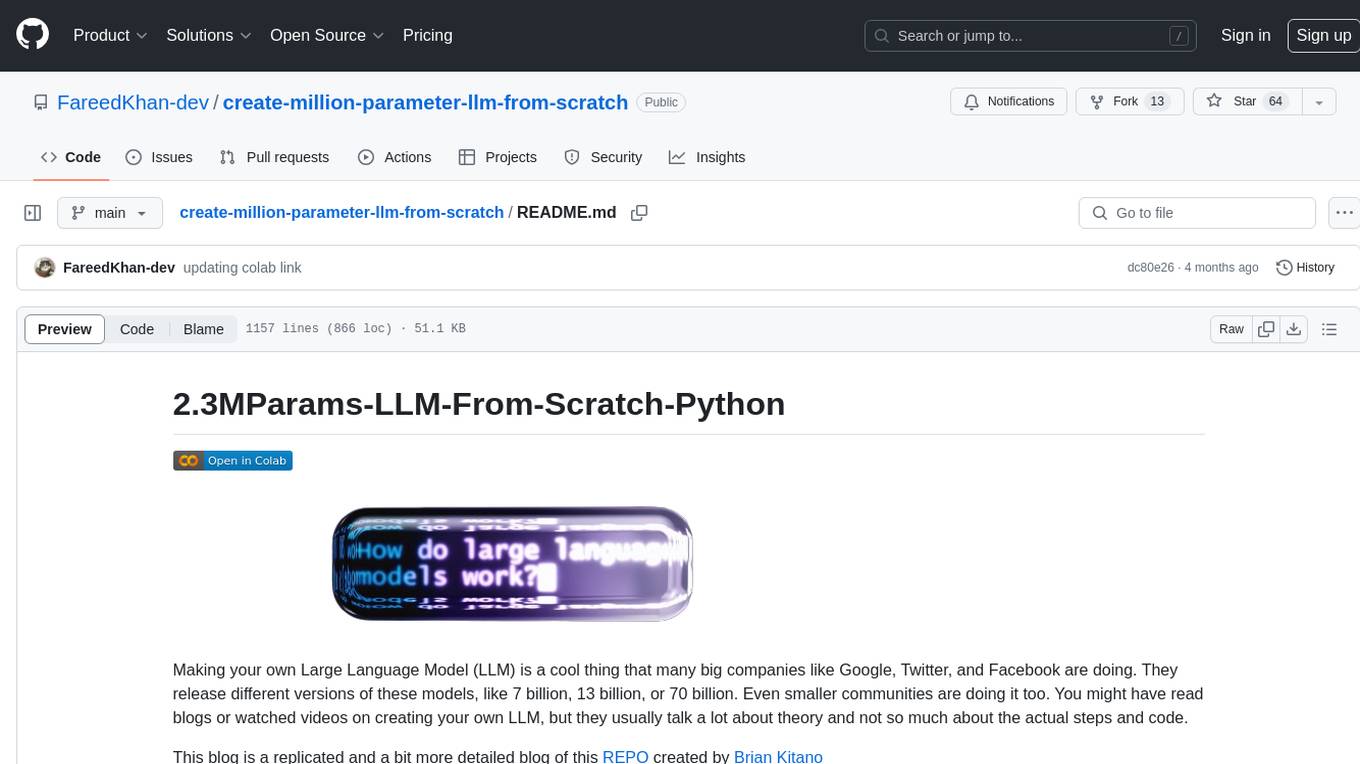
create-million-parameter-llm-from-scratch
The 'create-million-parameter-llm-from-scratch' repository provides a detailed guide on creating a Large Language Model (LLM) with 2.3 million parameters from scratch. The blog replicates the LLaMA approach, incorporating concepts like RMSNorm for pre-normalization, SwiGLU activation function, and Rotary Embeddings. The model is trained on a basic dataset to demonstrate the ease of creating a million-parameter LLM without the need for a high-end GPU.
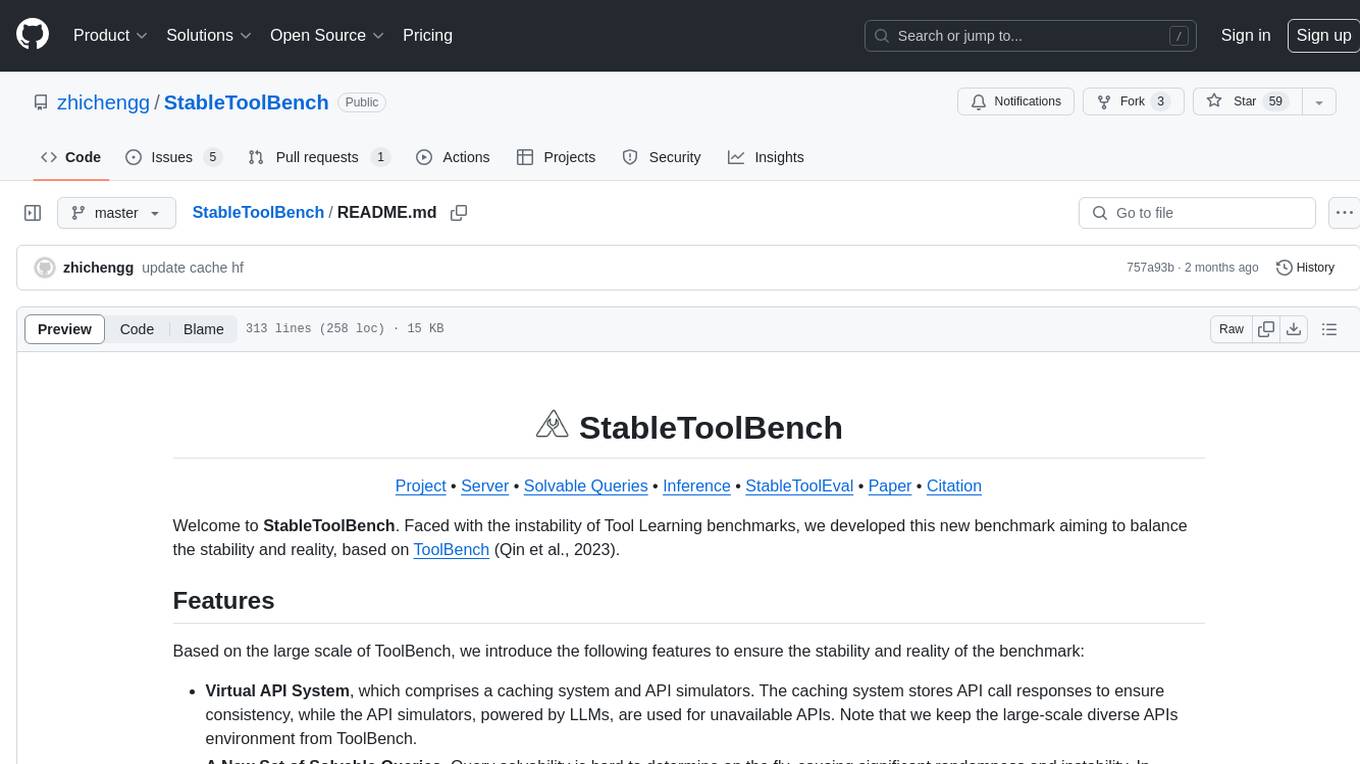
StableToolBench
StableToolBench is a new benchmark developed to address the instability of Tool Learning benchmarks. It aims to balance stability and reality by introducing features such as a Virtual API System with caching and API simulators, a new set of solvable queries determined by LLMs, and a Stable Evaluation System using GPT-4. The Virtual API Server can be set up either by building from source or using a prebuilt Docker image. Users can test the server using provided scripts and evaluate models with Solvable Pass Rate and Solvable Win Rate metrics. The tool also includes model experiments results comparing different models' performance.
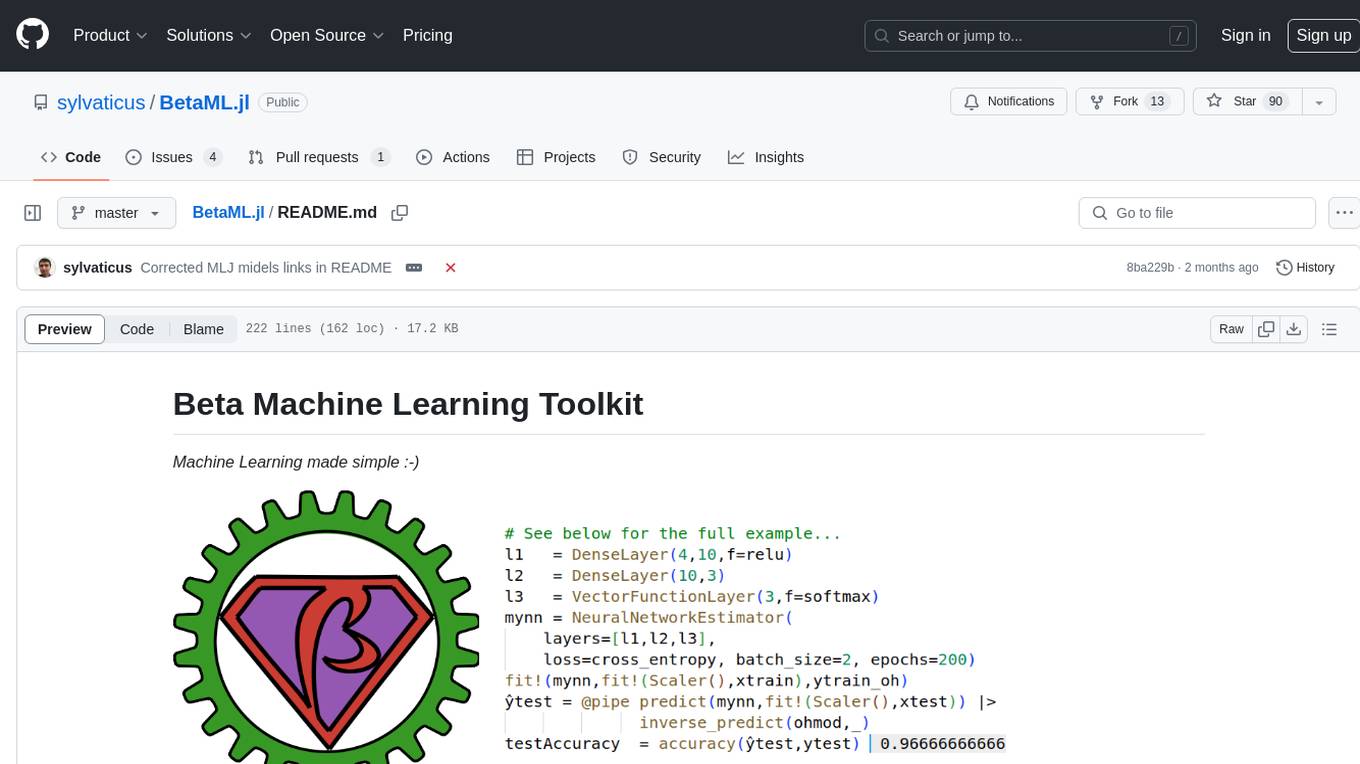
BetaML.jl
The Beta Machine Learning Toolkit is a package containing various algorithms and utilities for implementing machine learning workflows in multiple languages, including Julia, Python, and R. It offers a range of supervised and unsupervised models, data transformers, and assessment tools. The models are implemented entirely in Julia and are not wrappers for third-party models. Users can easily contribute new models or request implementations. The focus is on user-friendliness rather than computational efficiency, making it suitable for educational and research purposes.
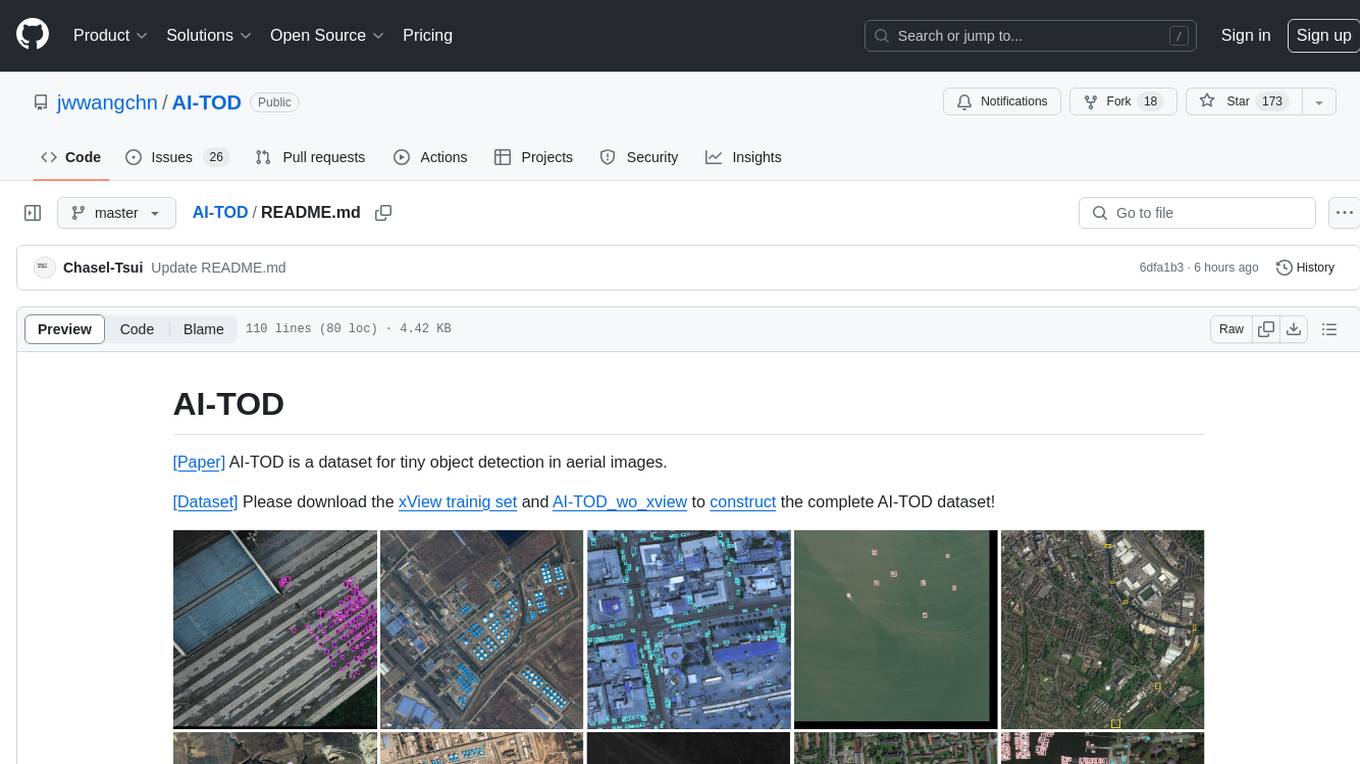
AI-TOD
AI-TOD is a dataset for tiny object detection in aerial images, containing 700,621 object instances across 28,036 images. Objects in AI-TOD are smaller with a mean size of 12.8 pixels compared to other aerial image datasets. To use AI-TOD, download xView training set and AI-TOD_wo_xview, then generate the complete dataset using the provided synthesis tool. The dataset is publicly available for academic and research purposes under CC BY-NC-SA 4.0 license.
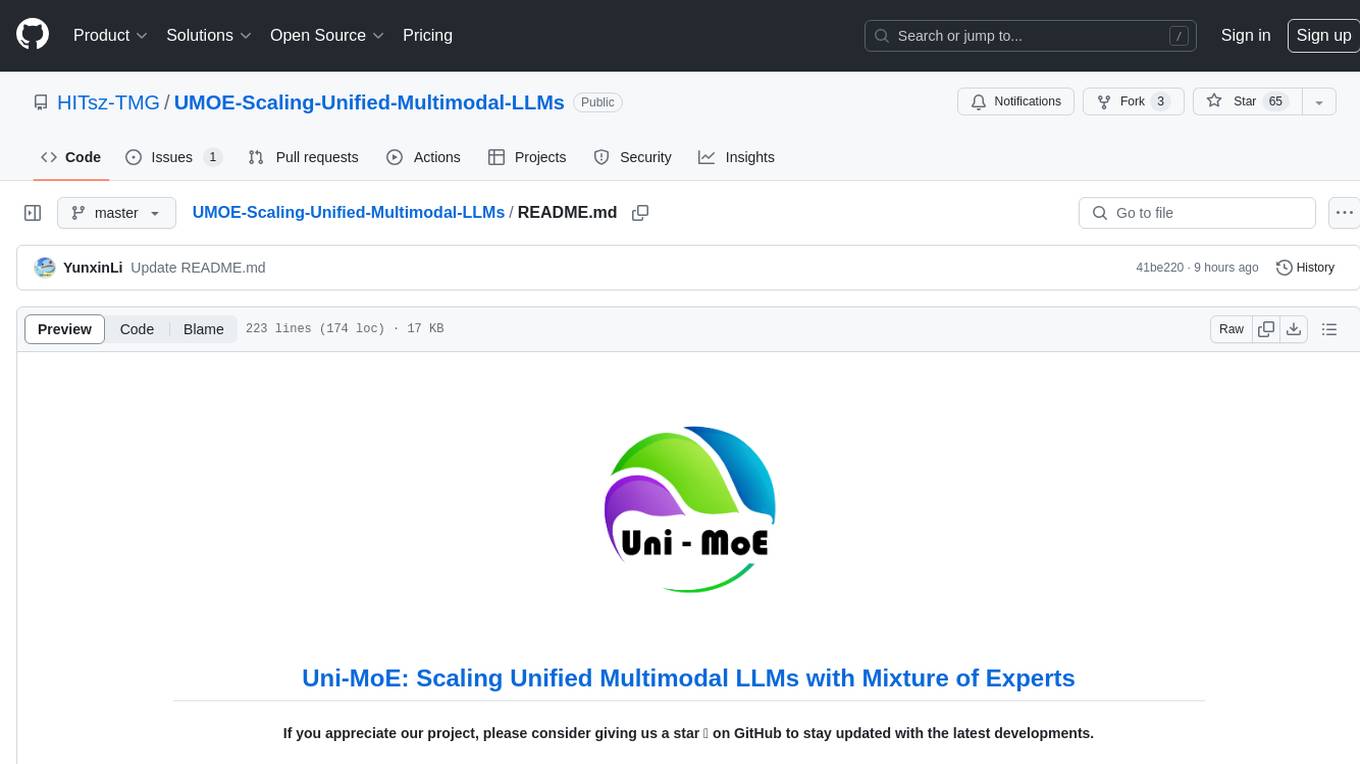
UMOE-Scaling-Unified-Multimodal-LLMs
Uni-MoE is a MoE-based unified multimodal model that can handle diverse modalities including audio, speech, image, text, and video. The project focuses on scaling Unified Multimodal LLMs with a Mixture of Experts framework. It offers enhanced functionality for training across multiple nodes and GPUs, as well as parallel processing at both the expert and modality levels. The model architecture involves three training stages: building connectors for multimodal understanding, developing modality-specific experts, and incorporating multiple trained experts into LLMs using the LoRA technique on mixed multimodal data. The tool provides instructions for installation, weights organization, inference, training, and evaluation on various datasets.
For similar jobs
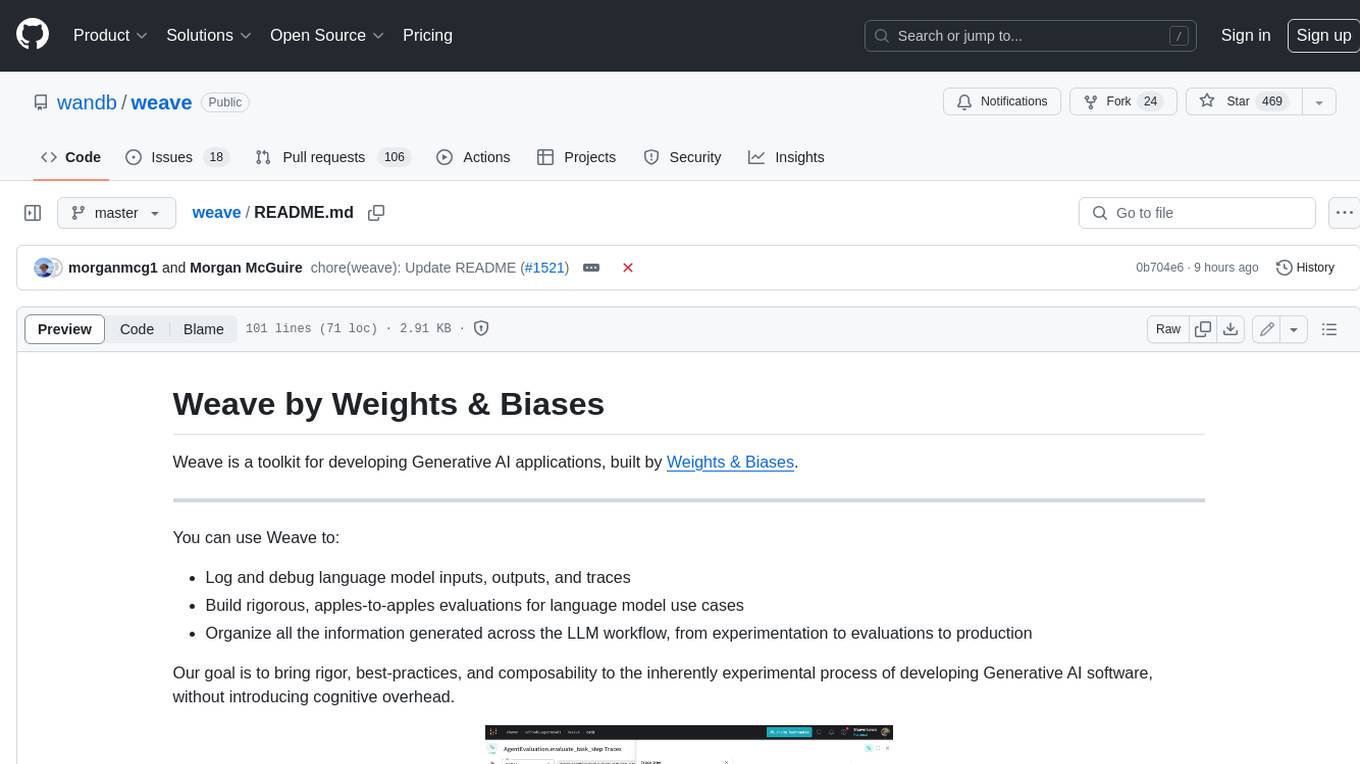
weave
Weave is a toolkit for developing Generative AI applications, built by Weights & Biases. With Weave, you can log and debug language model inputs, outputs, and traces; build rigorous, apples-to-apples evaluations for language model use cases; and organize all the information generated across the LLM workflow, from experimentation to evaluations to production. Weave aims to bring rigor, best-practices, and composability to the inherently experimental process of developing Generative AI software, without introducing cognitive overhead.
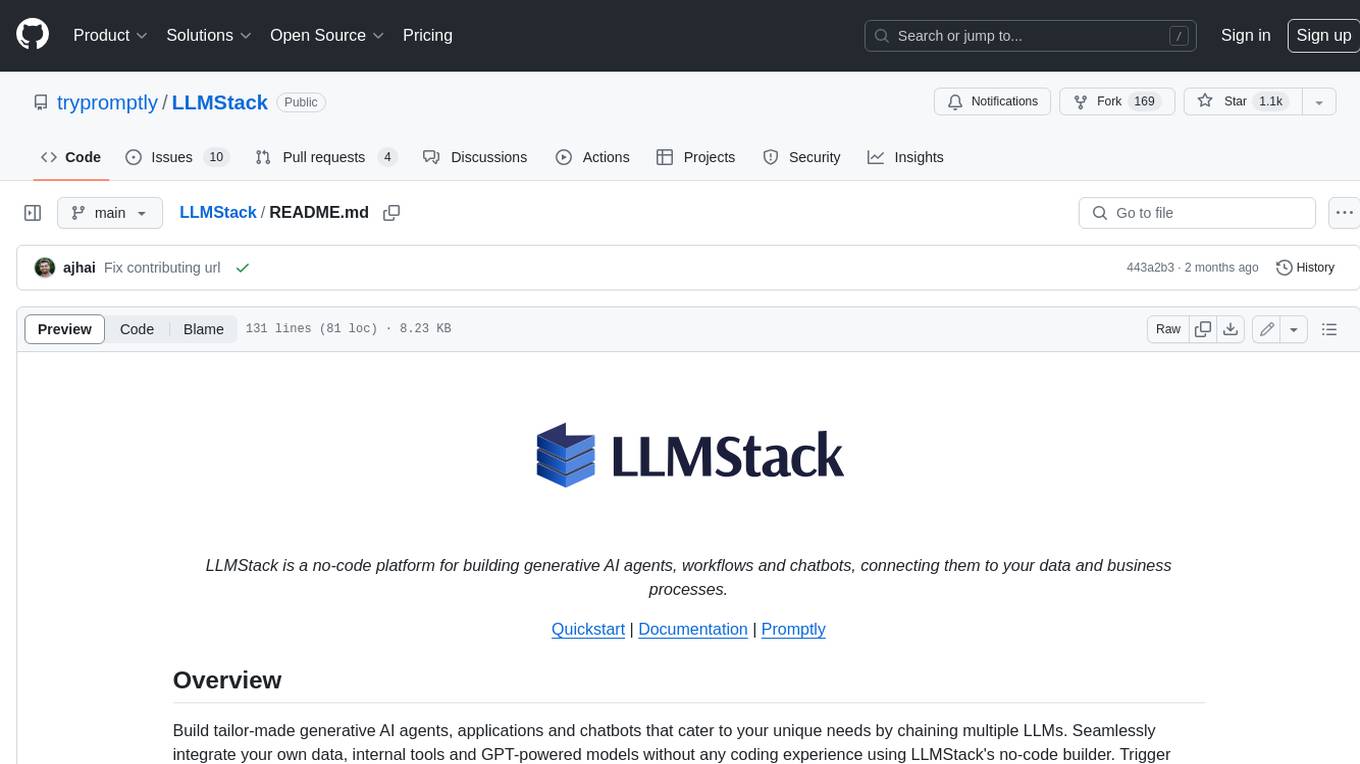
LLMStack
LLMStack is a no-code platform for building generative AI agents, workflows, and chatbots. It allows users to connect their own data, internal tools, and GPT-powered models without any coding experience. LLMStack can be deployed to the cloud or on-premise and can be accessed via HTTP API or triggered from Slack or Discord.
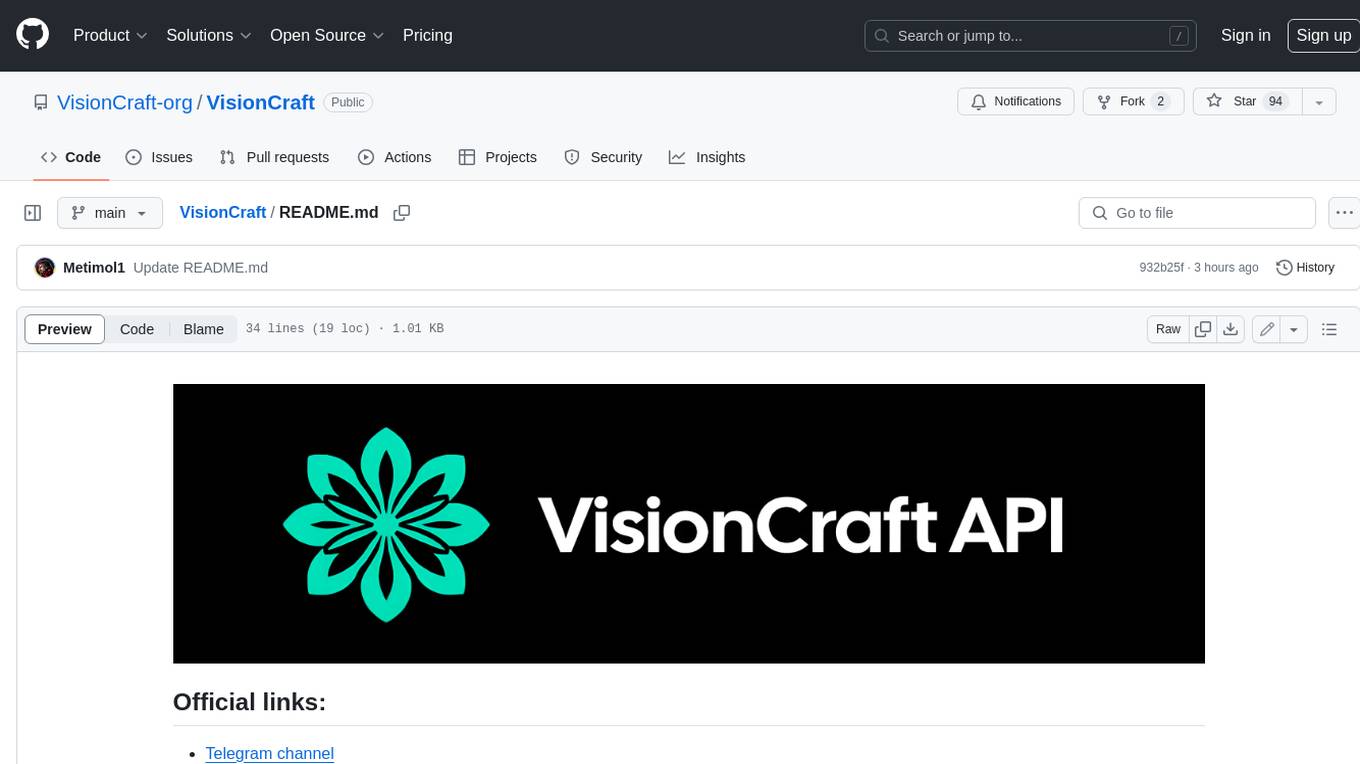
VisionCraft
The VisionCraft API is a free API for using over 100 different AI models. From images to sound.
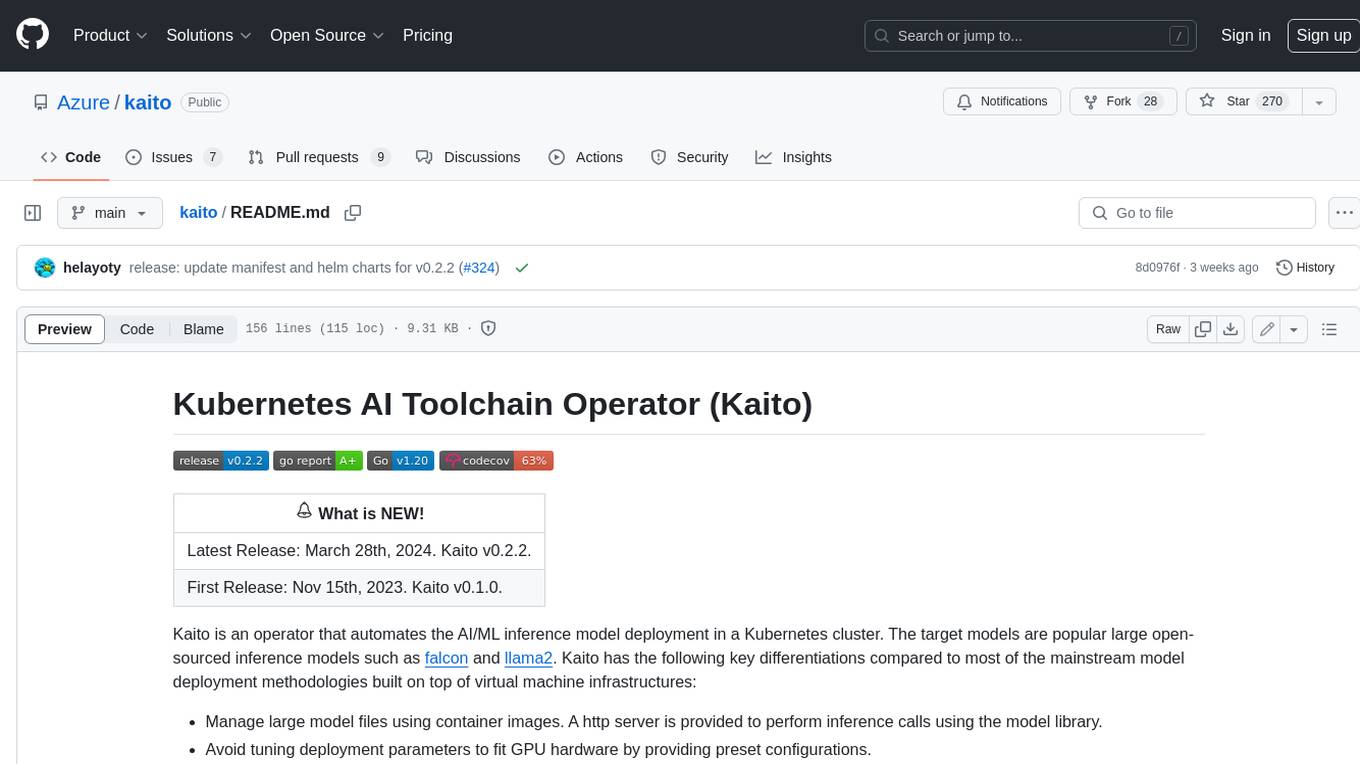
kaito
Kaito is an operator that automates the AI/ML inference model deployment in a Kubernetes cluster. It manages large model files using container images, avoids tuning deployment parameters to fit GPU hardware by providing preset configurations, auto-provisions GPU nodes based on model requirements, and hosts large model images in the public Microsoft Container Registry (MCR) if the license allows. Using Kaito, the workflow of onboarding large AI inference models in Kubernetes is largely simplified.
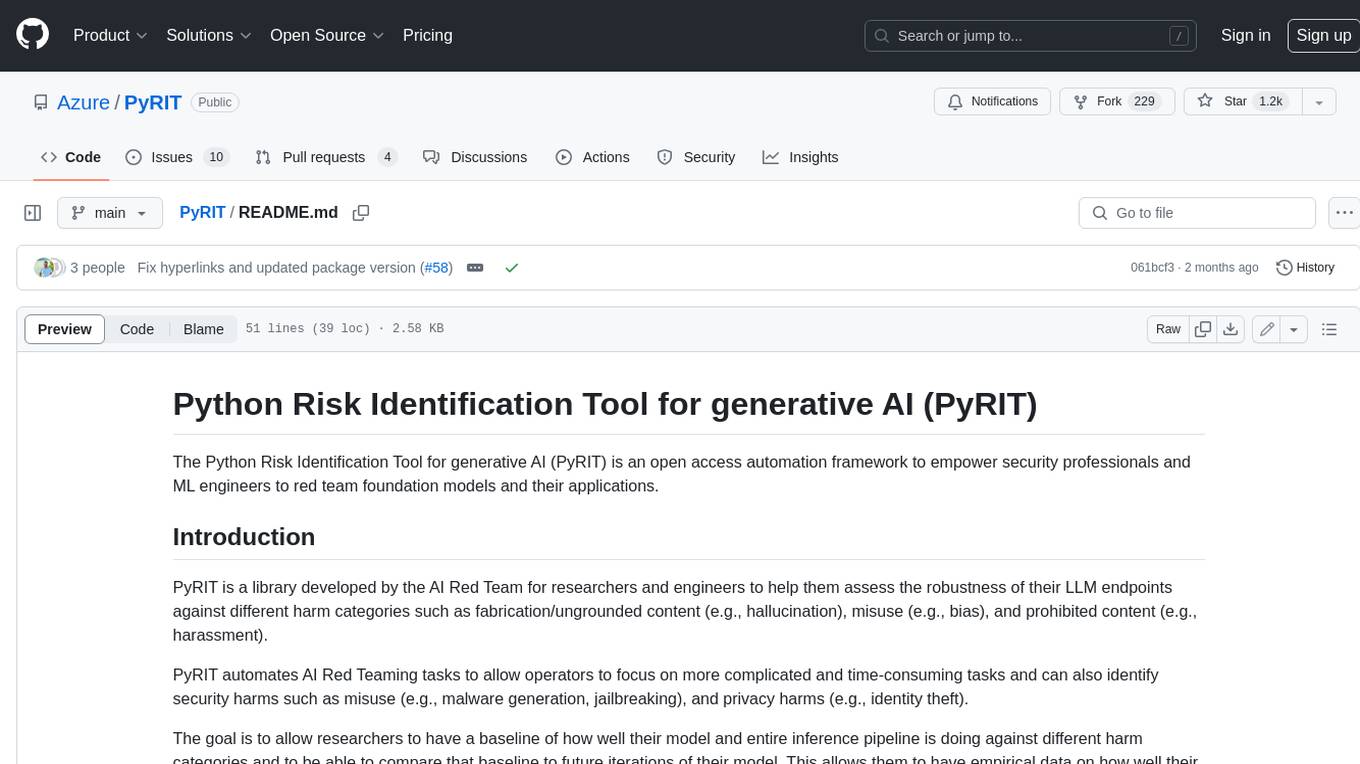
PyRIT
PyRIT is an open access automation framework designed to empower security professionals and ML engineers to red team foundation models and their applications. It automates AI Red Teaming tasks to allow operators to focus on more complicated and time-consuming tasks and can also identify security harms such as misuse (e.g., malware generation, jailbreaking), and privacy harms (e.g., identity theft). The goal is to allow researchers to have a baseline of how well their model and entire inference pipeline is doing against different harm categories and to be able to compare that baseline to future iterations of their model. This allows them to have empirical data on how well their model is doing today, and detect any degradation of performance based on future improvements.
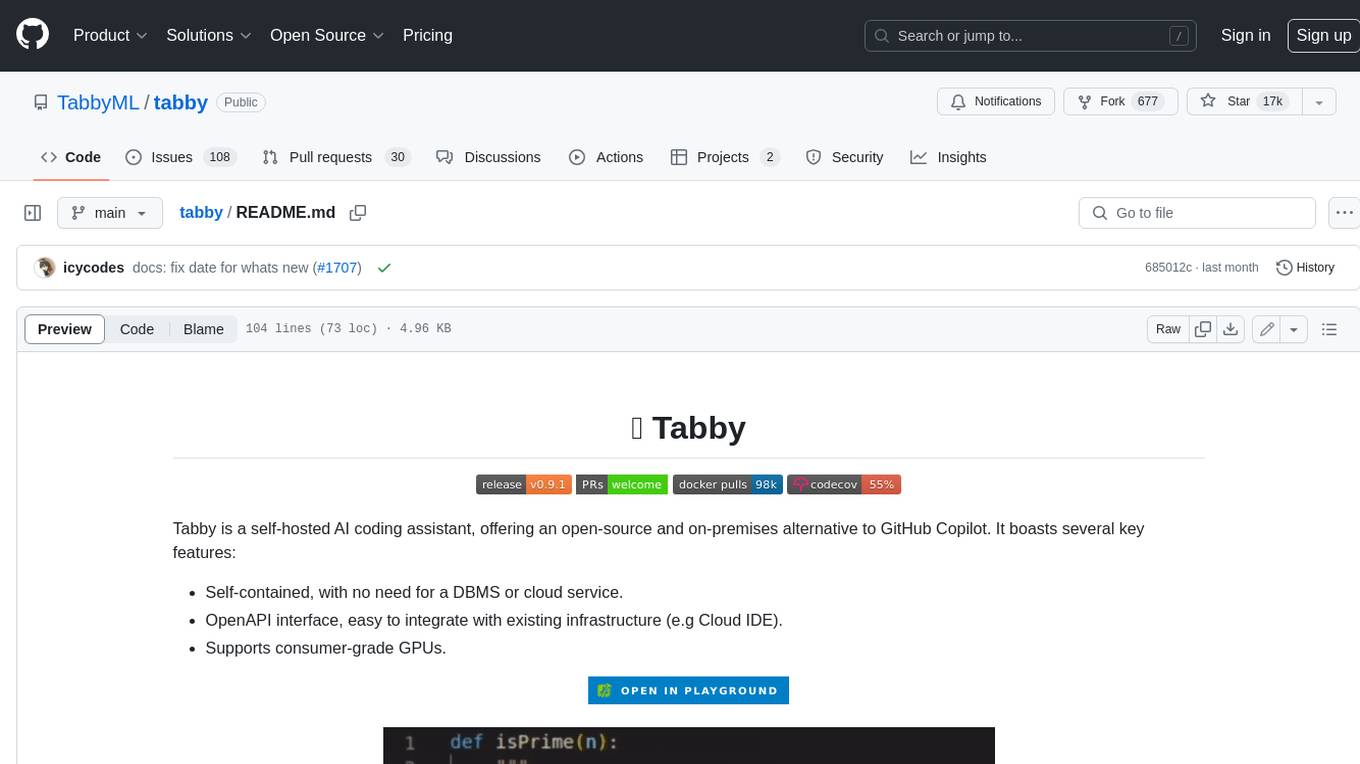
tabby
Tabby is a self-hosted AI coding assistant, offering an open-source and on-premises alternative to GitHub Copilot. It boasts several key features: * Self-contained, with no need for a DBMS or cloud service. * OpenAPI interface, easy to integrate with existing infrastructure (e.g Cloud IDE). * Supports consumer-grade GPUs.
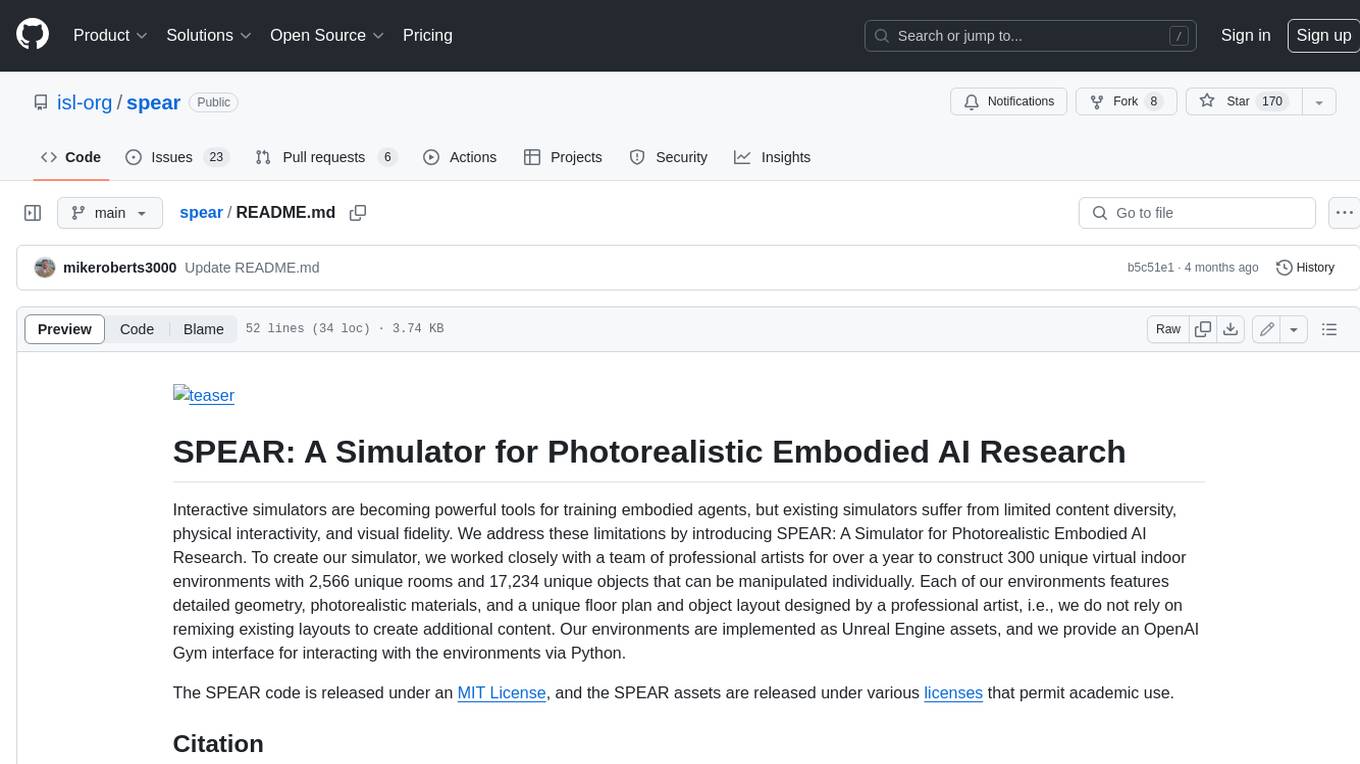
spear
SPEAR (Simulator for Photorealistic Embodied AI Research) is a powerful tool for training embodied agents. It features 300 unique virtual indoor environments with 2,566 unique rooms and 17,234 unique objects that can be manipulated individually. Each environment is designed by a professional artist and features detailed geometry, photorealistic materials, and a unique floor plan and object layout. SPEAR is implemented as Unreal Engine assets and provides an OpenAI Gym interface for interacting with the environments via Python.
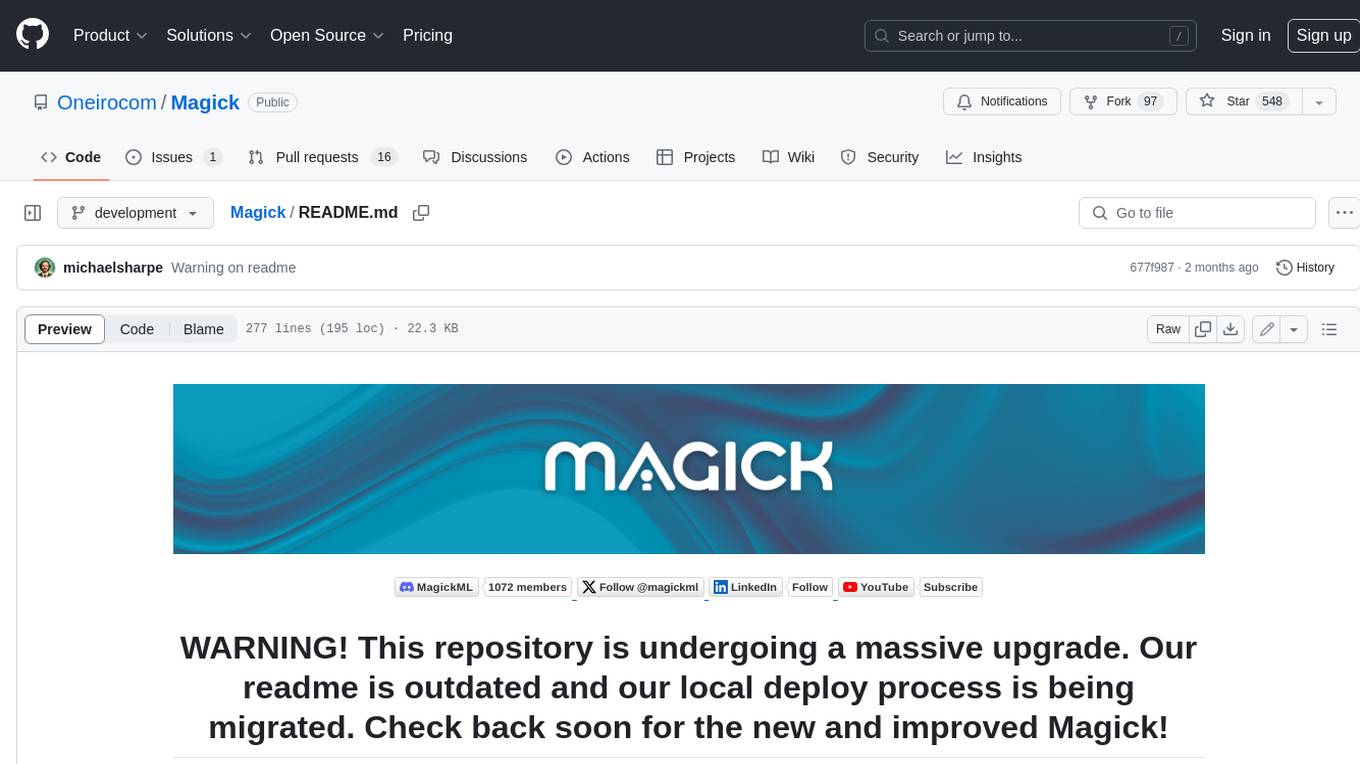
Magick
Magick is a groundbreaking visual AIDE (Artificial Intelligence Development Environment) for no-code data pipelines and multimodal agents. Magick can connect to other services and comes with nodes and templates well-suited for intelligent agents, chatbots, complex reasoning systems and realistic characters.