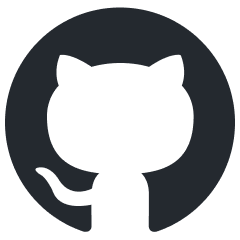
cellseg_models.pytorch
Encoder-Decoder Cell and Nuclei segmentation models
Stars: 69
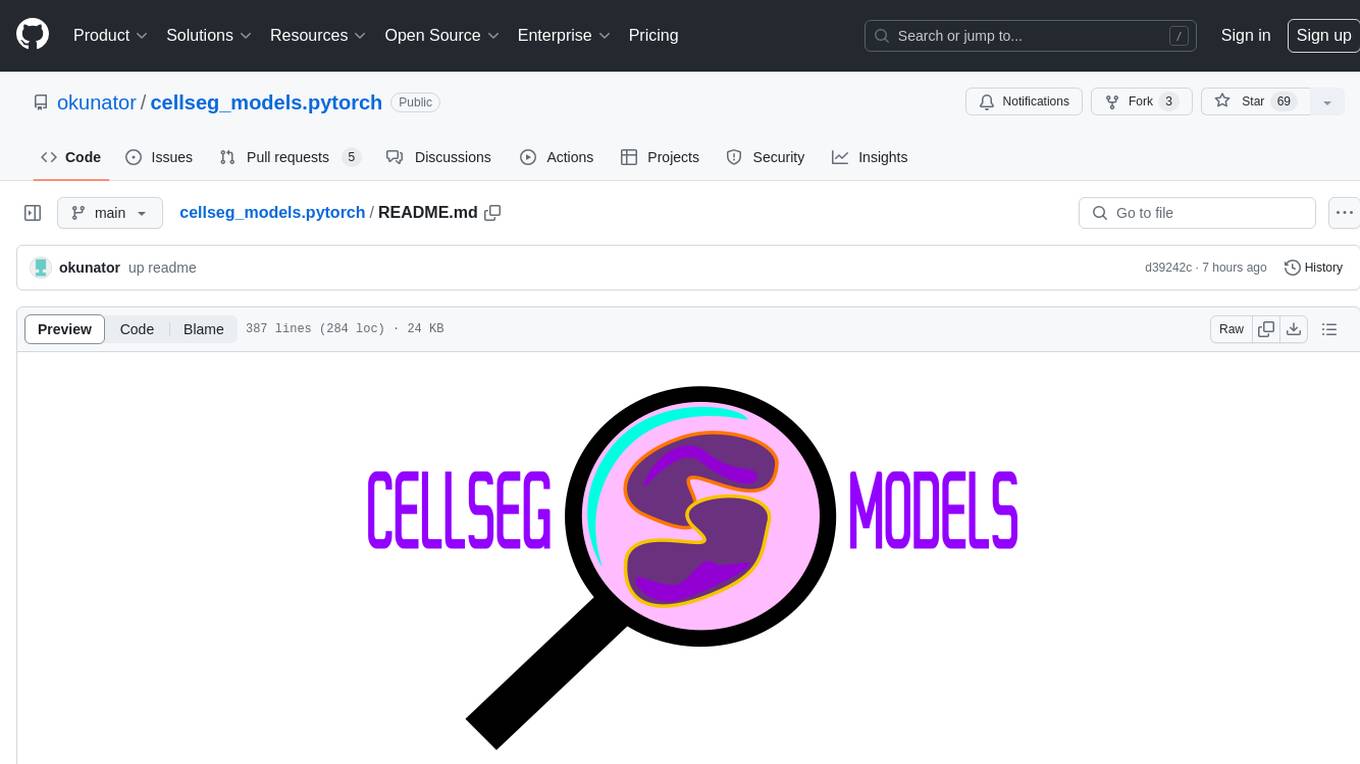
cellseg-models.pytorch is a Python library built upon PyTorch for 2D cell/nuclei instance segmentation models. It provides multi-task encoder-decoder architectures and post-processing methods for segmenting cell/nuclei instances. The library offers high-level API to define segmentation models, open-source datasets for training, flexibility to modify model components, sliding window inference, multi-GPU inference, benchmarking utilities, regularization techniques, and example notebooks for training and finetuning models with different backbones.
README:
cellseg-models.pytorch is a library built upon PyTorch that contains multi-task encoder-decoder architectures along with dedicated post-processing methods for segmenting cell/nuclei instances. As the name might suggest, this library is heavily inspired by segmentation_models.pytorch library for semantic segmentation.
- Now you can use any pre-trained image encoder from the timm library as the model backbone. (Given that they implement the
forward_intermediates
method, most of them do). - New example notebooks showing how to finetune Cellpose and Stardist with the new state-of-the-art foundation model backbones: UNI from the MahmoodLab, and Prov-GigaPath from Microsoft Research. Check out the notebooks here (UNI), and here (Prov-GigaPath).
- NOTE!: These foundation models are licensed under restrictive licences and you need to agree to the terms of any of the said models to get access to the weights. Once you have been granted access, you can run the above notebooks. You can request for access here: the model pages UNI and model pages Prov-GigaPath. These models may only be used for non-commercial, academic research purposes with proper attribution. Be sure that you have read and understood the terms before requesting access.
- High level API to define cell/nuclei instance segmentation models.
- 6 cell/nuclei instance segmentation model architectures and more to come.
- Open source datasets for training and benchmarking.
- Flexibility to modify the components of the model architectures.
- Sliding window inference for large images.
- Multi-GPU inference.
- All model architectures can be augmented to panoptic segmentation.
- Popular training losses and benchmarking metrics.
- Benchmarking utilities both for model latency & segmentation performance.
- Regularization techniques to tackle batch effects/domain shifts such as Strong Augment, Spectral decoupling, Label smoothing.
- Example notebooks to train models with lightning or accelerate.
- Example notebooks to finetune models with foundation model backbones such as UNI, Prov-GigaPath, and DINOv2.
pip install cellseg-models-pytorch
Model | Paper |
---|---|
[1] HoVer-Net | https://www.sciencedirect.com/science/article/pii/S1361841519301045?via%3Dihub |
[2] Cellpose | https://www.nature.com/articles/s41592-020-01018-x |
[3] Omnipose | https://www.biorxiv.org/content/10.1101/2021.11.03.467199v2 |
[4] Stardist | https://arxiv.org/abs/1806.03535 |
[5] CellVit-SAM | https://arxiv.org/abs/2306.15350.03535 |
[6] CPP-Net | https://arxiv.org/abs/2102.0686703535 |
Dataset | Paper |
---|---|
[7, 8] Pannuke | https://arxiv.org/abs/2003.10778 , https://link.springer.com/chapter/10.1007/978-3-030-23937-4_2 |
Finetuning CellPose with UNI backbone
-
Finetuning CellPose with UNI. Here we finetune the CellPose multi-class nuclei segmentation model with the foundation model
UNI
-image-encoder backbone (checkout UNI). The Pannuke dataset (fold 1 & fold 2) are used for training data and the fold 3 is used as validation data. The model is trained (with checkpointing) by utilizing accelerate by hugginface. NOTE that you need to have granted access to the UNI weights and agreed to the terms of the model to be able to run the notebook.
Finetuning Stardist with Prov-GigaPath backbone
-
Finetuning Stardist with Prov-GigaPath. Here we finetune the Stardist multi-class nuclei segmentation model with the foundation model
Prov-GigaPath
-image-encoder backbone (checkout Prov-GigaPath). The Pannuke dataset (fold 1 & fold 2) are used for training data and the fold 3 is used as validation data. The model is trained (with checkpointing) by utilizing accelerate by hugginface. NOTE that you need to have granted access to the Prov-GigaPath weights and agreed to the terms of the model to be able to run the notebook.
Finetuning CellPose with DINOv2 backbone
-
Finetuning CellPose with DINOv2 backbone for Pannuke. Here we finetune the CellPose multi-class nuclei segmentation model with a
LVD-142M
pretrainedDINOv2
backbone. The Pannuke dataset (fold 1 & fold 2) are used for training data and the fold 3 is used as validation data. The model is trained (with checkpointing) by utilizing lightning.
Finetuning CellVit-SAM with Pannuke
-
Finetuning CellVit-SAM with Pannuke. Here we finetune the CellVit-SAM multi-class nuclei segmentation model with a
SA-1B
pretrainedSAM
-image-encoder backbone (checkout SAM). The encoder is transformer basedVitDet
-model. The Pannuke dataset (fold 1 & fold 2) are used for training data and the fold 3 is used as validation data. The model is trained (with checkpointing) by utilizing accelerate by hugginface.
Training Hover-Net with Pannuke
-
Training Hover-Net with Pannuke. Here we train the
Hover-Net
nuclei segmentation model with animagenet
pretrainedresnet50
backbone from thetimm
library. The Pannuke dataset (fold 1 & fold 2) are used for training data and the fold 3 is used as validation data. The model is trained by utilizing lightning.
Training Stardist with Pannuke
-
Training Stardist with Pannuke. Here we train the
Stardist
multi-class nuclei segmentation model with animagenet
pretrainedefficientnetv2_s
backbone from thetimm
library. The Pannuke dataset (fold 1 & fold 2) are used for training data and the fold 3 is used as validation data. The model is trained by utilizing lightning.
Training CellPose with Pannuke
-
Training CellPose with Pannuke. Here we train the
CellPose
multi-class nuclei segmentation model with animagenet
pretrainedconvnext_small
backbone from thetimm
library. The Pannuke dataset (fold 1 & fold 2) are used for training data and the fold 3 is used as validation data. The model is trained (with checkpointing) by utilizing accelerate by hugginface.
Training OmniPose with Pannuke
-
Training OmniPose with Pannuke. Here we train the OmniPose multi-class nuclei segmentation model with an
imagenet
pretrainedfocalnet_small_lrf
backbone from thetimm
library. The Pannuke dataset (fold 1 & fold 2) are used for training data and the fold 3 is used as validation data. The model is trained (with checkpointing) by utilizing accelerate by hugginface.
Training CPP-Net with Pannuke
-
Training CPP-Net with Pannuke. Here we train the CPP-Net multi-class nuclei segmentation model with an
imagenet
pretrainedefficientnetv2_s
backbone from thetimm
library. The Pannuke dataset (fold 1 & fold 2) are used for training data and the fold 3 is used as validation data. The model is trained by utilizing lightning.
Benchmarking Cellpose Trained on Pannuke
-
Benchmarking Cellpose Trained on Pannuke. Here we run benchmarking for
Cellpose
that was trained on Pannuke. Both the model performance and latency benchmarking are covered.
Define Cellpose for cell segmentation.
import cellseg_models_pytorch as csmp
import torch
model = csmp.models.cellpose_base(type_classes=5)
x = torch.rand([1, 3, 256, 256])
# NOTE: the outputs still need post-processing.
y = model(x) # {"cellpose": [1, 2, 256, 256], "type": [1, 5, 256, 256]}
Define Cellpose for cell and tissue area segmentation (Panoptic segmentation).
import cellseg_models_pytorch as csmp
import torch
model = csmp.models.cellpose_plus(type_classes=5, sem_classes=3)
x = torch.rand([1, 3, 256, 256])
# NOTE: the outputs still need post-processing.
y = model(x) # {"cellpose": [1, 2, 256, 256], "type": [1, 5, 256, 256], "sem": [1, 3, 256, 256]}
Define panoptic Cellpose model with more flexibility.
import cellseg_models_pytorch as csmp
# the model will include two decoder branches.
decoders = ("cellpose", "sem")
# and in total three segmentation heads emerging from the decoders.
heads = {
"cellpose": {"cellpose": 2, "type": 5},
"sem": {"sem": 3}
}
model = csmp.CellPoseUnet(
decoders=decoders, # cellpose and semantic decoders
heads=heads, # three output heads
depth=5, # encoder depth
out_channels=(256, 128, 64, 32, 16), # num out channels at each decoder stage
layer_depths=(4, 4, 4, 4, 4), # num of conv blocks at each decoder layer
style_channels=256, # num of style vector channels
enc_name="resnet50", # timm encoder
enc_pretrain=True, # imagenet pretrained encoder
long_skip="unetpp", # unet++ long skips ("unet", "unetpp", "unet3p")
merge_policy="sum", # concatenate long skips ("cat", "sum")
short_skip="residual", # residual short skips ("basic", "residual", "dense")
normalization="bcn", # batch-channel-normalization.
activation="gelu", # gelu activation.
convolution="wsconv", # weight standardized conv.
attention="se", # squeeze-and-excitation attention.
pre_activate=False, # normalize and activation after convolution.
)
x = torch.rand([1, 3, 256, 256])
# NOTE: the outputs still need post-processing.
y = model(x) # {"cellpose": [1, 2, 256, 256], "type": [1, 5, 256, 256], "sem": [1, 3, 256, 256]}
Run HoVer-Net inference and post-processing with a sliding window approach.
import cellseg_models_pytorch as csmp
# define the model
model = csmp.models.hovernet_base(type_classes=5)
# define the final activations for each model output
out_activations = {"hovernet": "tanh", "type": "softmax", "inst": "softmax"}
# define whether to weight down the predictions at the image boundaries
# typically, models perform the poorest at the image boundaries and with
# overlapping patches this causes issues which can be overcome by down-
# weighting the prediction boundaries
out_boundary_weights = {"hovernet": True, "type": False, "inst": False}
# define the inferer
inferer = csmp.inference.SlidingWindowInferer(
model=model,
input_folder="/path/to/images/",
checkpoint_path="/path/to/model/weights/",
out_activations=out_activations,
out_boundary_weights=out_boundary_weights,
instance_postproc="hovernet", # THE POST-PROCESSING METHOD
normalization="percentile", # same normalization as in training
patch_size=(256, 256),
stride=128,
padding=80,
batch_size=8,
)
inferer.infer()
inferer.out_masks
# {"image1" :{"inst": [H, W], "type": [H, W]}, ..., "imageN" :{"inst": [H, W], "type": [H, W]}}
Generally, the model building API enables the effortless creation of hard-parameter sharing multi-task encoder-decoder CNN architectures. The general architectural schema is illustrated in the below image.
The class API enables the most flexibility in defining different model architectures. It borrows a lot from segmentation_models.pytorch models API.
Model classes:
csmp.CellPoseUnet
csmp.StarDistUnet
csmp.HoverNet
csmp.CellVitSAM
All of the models contain:
-
model.encoder
- pretrained timm backbone for feature extraction. -
model.{decoder_name}_decoder
- Models can have multiple decoders with unique names. -
model.{head_name}_seg_head
- Model decoders can have multiple segmentation heads with unique names. -
model.forward(x)
- forward pass. -
model.forward_features(x)
- forward pass of the encoder and decoders. Returns enc and dec features
Defining your own multi-task architecture
For example, to define a multi-task architecture that has resnet50
encoder, four decoders, and 5 output heads with CellPoseUnet
architectural components, we could do this:
import cellseg_models_pytorch as csmp
import torch
model = csmp.CellPoseUnet(
decoders=("cellpose", "dist", "contour", "sem"),
heads={
"cellpose": {"type": 5, "cellpose": 2},
"dist": {"dist": 1},
"contour": {"contour": 1},
"sem": {"sem": 4}
},
)
x = torch.rand([1, 3, 256, 256])
model(x)
# {
# "cellpose": [1, 2, 256, 256],
# "type": [1, 5, 256, 256],
# "dist": [1, 1, 256, 256],
# "contour": [1, 1, 256, 256],
# "sem": [1, 4, 256, 256]
# }
With the function API, you can build models with low effort by calling the below listed functions. Under the hood, the function API simply calls the above classes with pre-defined decoder and head names. The training and post-processing tools of this library are built around these names, thus, it is recommended to use the function API, although, it is a bit more rigid than the class API. Basically, the function API only lacks the ability to define the output-tasks of the model, but allows for all the rest as the class API.
Model functions | Output names | Task |
---|---|---|
csmp.models.cellpose_base |
"type" , "cellpose" , |
instance segmentation |
csmp.models.cellpose_plus |
"type" , "cellpose" , "sem" , |
panoptic segmentation |
csmp.models.omnipose_base |
"type" , "omnipose"
|
instance segmentation |
csmp.models.omnipose_plus |
"type" , "omnipose" , "sem" , |
panoptic segmentation |
csmp.models.hovernet_base |
"type" , "inst" , "hovernet"
|
instance segmentation |
csmp.models.hovernet_plus |
"type" , "inst" , "hovernet" , "sem"
|
panoptic segmentation |
csmp.models.hovernet_small |
"type" ,"hovernet"
|
instance segmentation |
csmp.models.hovernet_small_plus |
"type" , "hovernet" , "sem"
|
panoptic segmentation |
csmp.models.stardist_base |
"stardist" , "dist"
|
binary instance segmentation |
csmp.models.stardist_base_multiclass |
"stardist" , "dist" , "type"
|
instance segmentation |
csmp.models.stardist_plus |
"stardist" , "dist" , "type" , "sem"
|
panoptic segmentation |
csmp.models.cppnet_base |
"stardist_refined" , "dist"
|
binary instance segmentation |
csmp.models.cppnet_base_multiclass |
"stardist_refined" , "dist" , "type"
|
instance segmentation |
csmp.models.cppnet_plus |
"stardist_refined" , "dist" , "type" , "sem"
|
panoptic segmentation |
csmp.models.cellvit_sam_base |
"type" , "inst" , "hovernet"
|
instance segmentation |
csmp.models.cellvit_sam_plus |
"type" , "inst" , "hovernet" , "sem"
|
panoptic segmentation |
csmp.models.cellvit_sam_small |
"type" ,"hovernet"
|
instance segmentation |
csmp.models.cellvit_sam_small_plus |
"type" , "hovernet" , "sem"
|
panoptic segmentation |
- [1] S. Graham, Q. D. Vu, S. E. A. Raza, A. Azam, Y-W. Tsang, J. T. Kwak and N. Rajpoot. "HoVer-Net: Simultaneous Segmentation and Classification of Nuclei in Multi-Tissue Histology Images." Medical Image Analysis, Sept. 2019.
- [2] Stringer, C.; Wang, T.; Michaelos, M. & Pachitariu, M. Cellpose: a generalist algorithm for cellular segmentation Nature Methods, 2021, 18, 100-106
- [3] Cutler, K. J., Stringer, C., Wiggins, P. A., & Mougous, J. D. (2022). Omnipose: a high-precision morphology-independent solution for bacterial cell segmentation. bioRxiv. doi:10.1101/2021.11.03.467199
- [4] Uwe Schmidt, Martin Weigert, Coleman Broaddus, & Gene Myers (2018). Cell Detection with Star-Convex Polygons. In Medical Image Computing and Computer Assisted Intervention - MICCAI 2018 - 21st International Conference, Granada, Spain, September 16-20, 2018, Proceedings, Part II (pp. 265–273).
- [5] Hörst, F., Rempe, M., Heine, L., Seibold, C., Keyl, J., Baldini, G., Ugurel, S., Siveke, J., Grünwald, B., Egger, J., & Kleesiek, J. (2023). CellViT: Vision Transformers for Precise Cell Segmentation and Classification (Version 1). arXiv. https://doi.org/10.48550/ARXIV.2306.15350.
- [6] Chen, S., Ding, C., Liu, M., Cheng, J., & Tao, D. (2023). CPP-Net: Context-Aware Polygon Proposal Network for Nucleus Segmentation. In IEEE Transactions on Image Processing (Vol. 32, pp. 980–994). Institute of Electrical and Electronics Engineers (IEEE). https://doi.org/10.1109/tip.2023.3237013
- [7] Gamper, J., Koohbanani, N., Benet, K., Khuram, A., & Rajpoot, N. (2019) PanNuke: an open pan-cancer histology dataset for nuclei instance segmentation and classification. In European Congress on Digital Pathology (pp. 11-19).
- [8] Gamper, J., Koohbanani, N., Graham, S., Jahanifar, M., Khurram, S., Azam, A.,Hewitt, K., & Rajpoot, N. (2020). PanNuke Dataset Extension, Insights and Baselines. arXiv preprint arXiv:2003.10778.
- [9] Graham, S., Jahanifar, M., Azam, A., Nimir, M., Tsang, Y.W., Dodd, K., Hero, E., Sahota, H., Tank, A., Benes, K., & others (2021). Lizard: A Large-Scale Dataset for Colonic Nuclear Instance Segmentation and Classification. In Proceedings of the IEEE/CVF International Conference on Computer Vision (pp. 684-693).
@misc{csmp2022,
title={{cellseg_models.pytorch}: Cell/Nuclei Segmentation Models and Benchmark.},
author={Oskari Lehtonen},
howpublished = {\url{https://github.com/okunator/cellseg_models.pytorch}},
doi = {10.5281/zenodo.7064617}
year={2022}
}
This project is distributed under MIT License
The project contains code from the original cell segmentation and 3rd-party libraries that have permissive licenses:
If you find this library useful in your project, it is your responsibility to ensure you comply with the conditions of any dependent licenses. Please create an issue if you think something is missing regarding the licenses.
For Tasks:
Click tags to check more tools for each tasksFor Jobs:
Alternative AI tools for cellseg_models.pytorch
Similar Open Source Tools
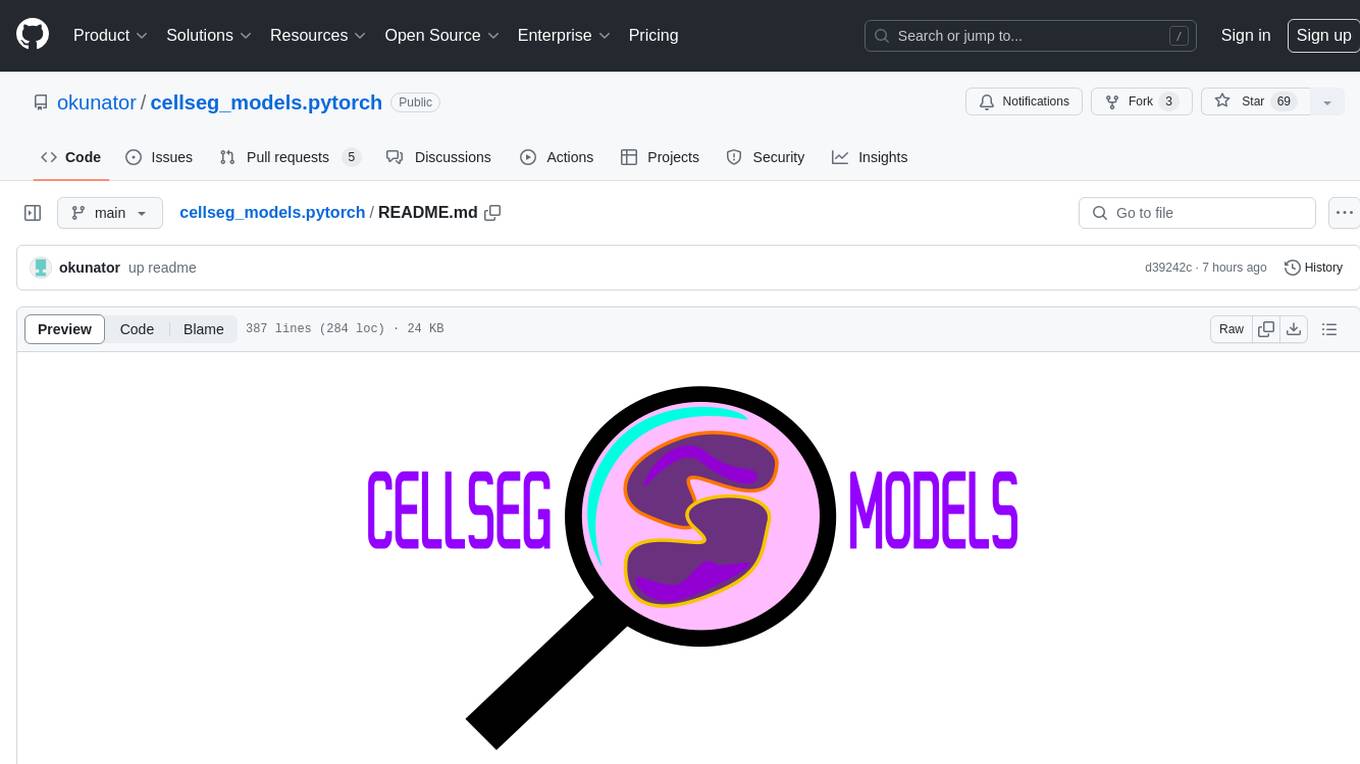
cellseg_models.pytorch
cellseg-models.pytorch is a Python library built upon PyTorch for 2D cell/nuclei instance segmentation models. It provides multi-task encoder-decoder architectures and post-processing methods for segmenting cell/nuclei instances. The library offers high-level API to define segmentation models, open-source datasets for training, flexibility to modify model components, sliding window inference, multi-GPU inference, benchmarking utilities, regularization techniques, and example notebooks for training and finetuning models with different backbones.
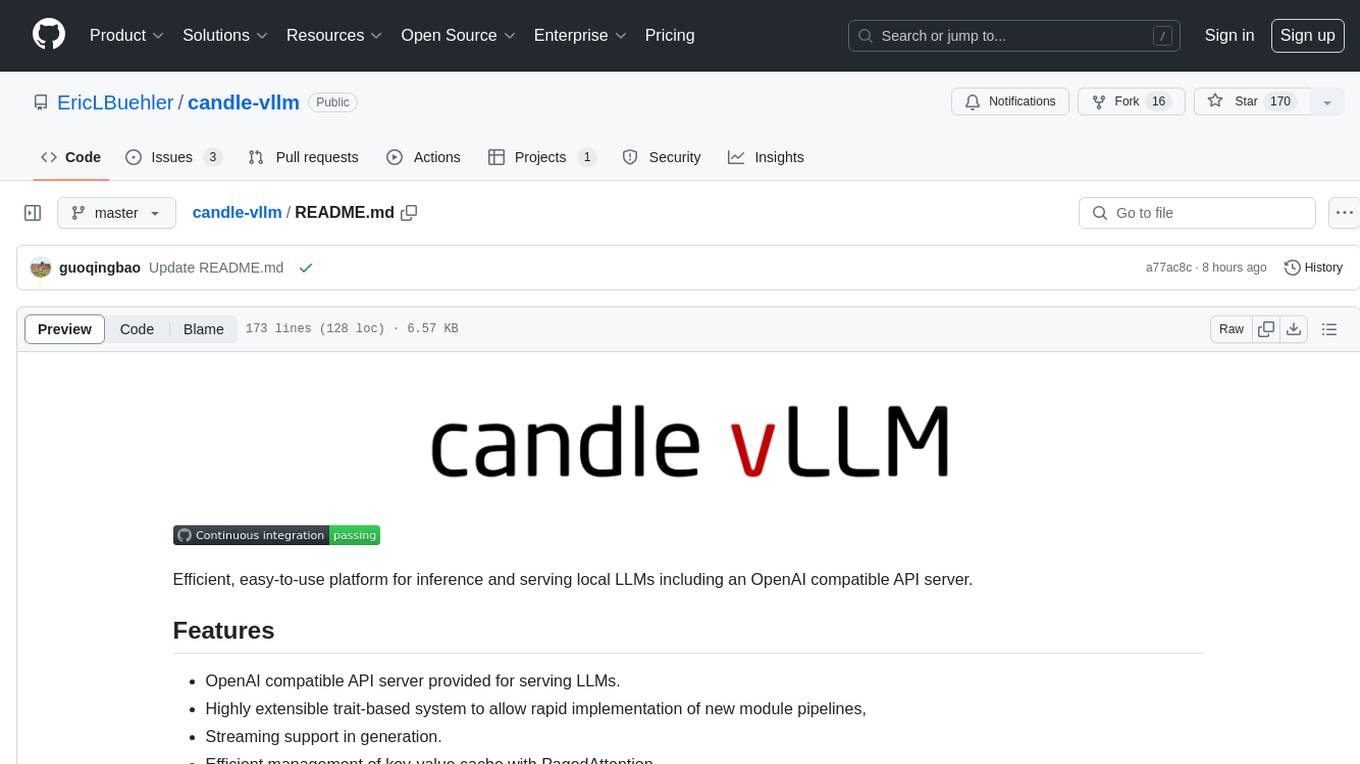
candle-vllm
Candle-vllm is an efficient and easy-to-use platform designed for inference and serving local LLMs, featuring an OpenAI compatible API server. It offers a highly extensible trait-based system for rapid implementation of new module pipelines, streaming support in generation, efficient management of key-value cache with PagedAttention, and continuous batching. The tool supports chat serving for various models and provides a seamless experience for users to interact with LLMs through different interfaces.
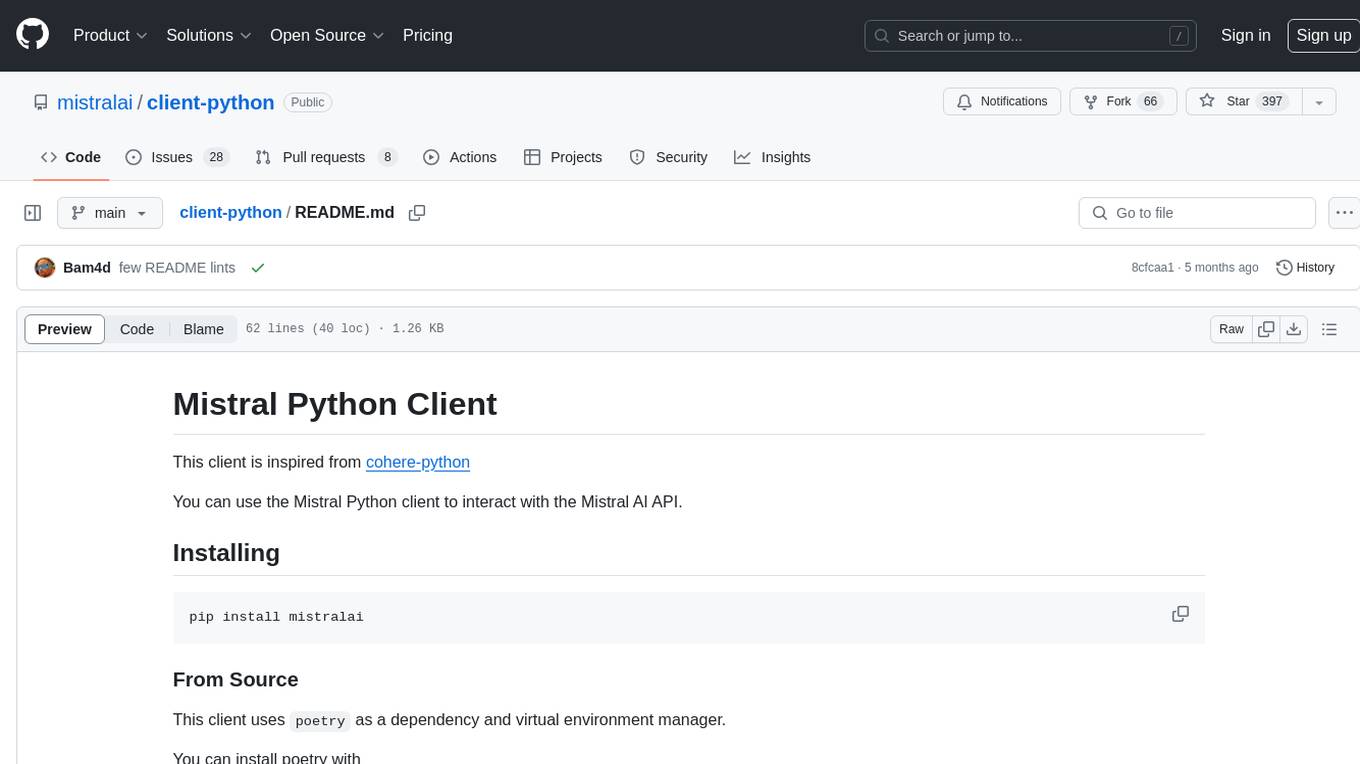
client-python
The Mistral Python Client is a tool inspired by cohere-python that allows users to interact with the Mistral AI API. It provides functionalities to access and utilize the AI capabilities offered by Mistral. Users can easily install the client using pip and manage dependencies using poetry. The client includes examples demonstrating how to use the API for various tasks, such as chat interactions. To get started, users need to obtain a Mistral API Key and set it as an environment variable. Overall, the Mistral Python Client simplifies the integration of Mistral AI services into Python applications.
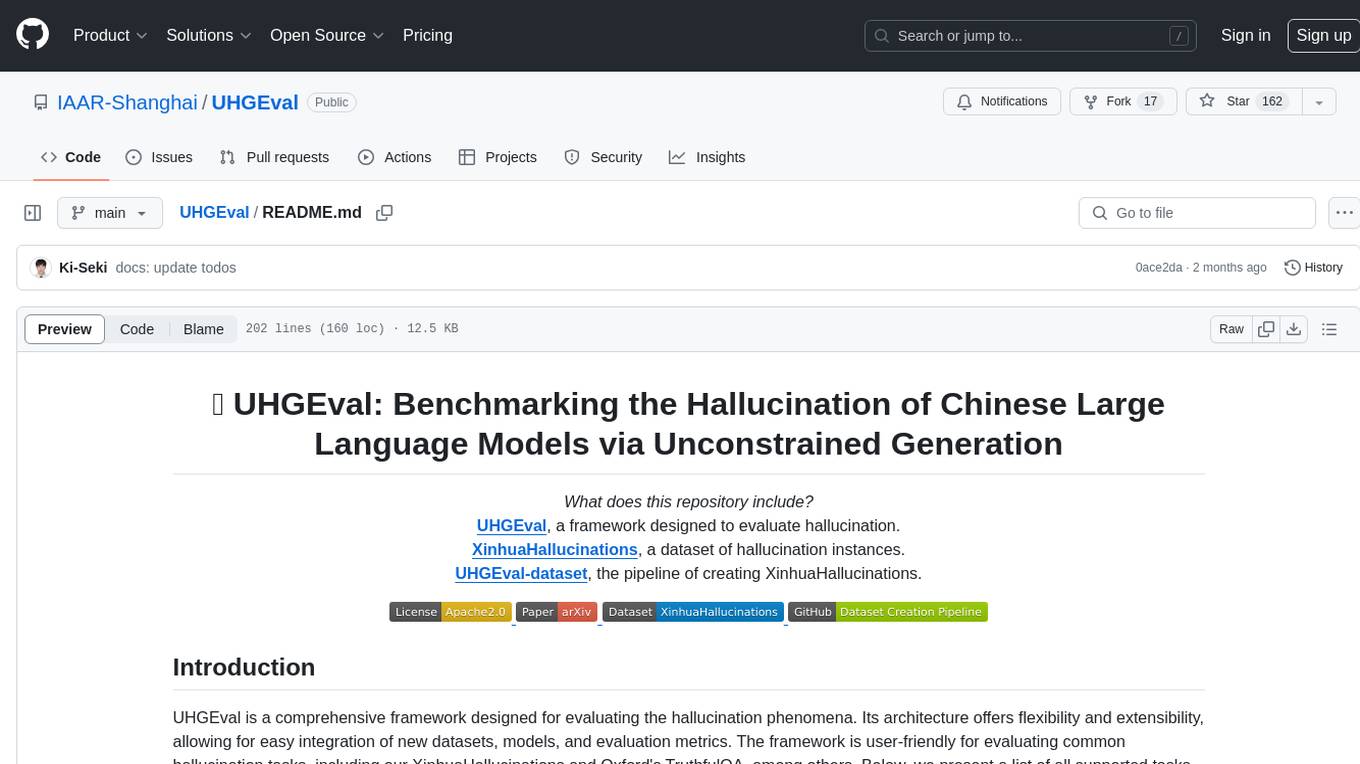
UHGEval
UHGEval is a comprehensive framework designed for evaluating the hallucination phenomena. It includes UHGEval, a framework for evaluating hallucination, XinhuaHallucinations dataset, and UHGEval-dataset pipeline for creating XinhuaHallucinations. The framework offers flexibility and extensibility for evaluating common hallucination tasks, supporting various models and datasets. Researchers can use the open-source pipeline to create customized datasets. Supported tasks include QA, dialogue, summarization, and multi-choice tasks.
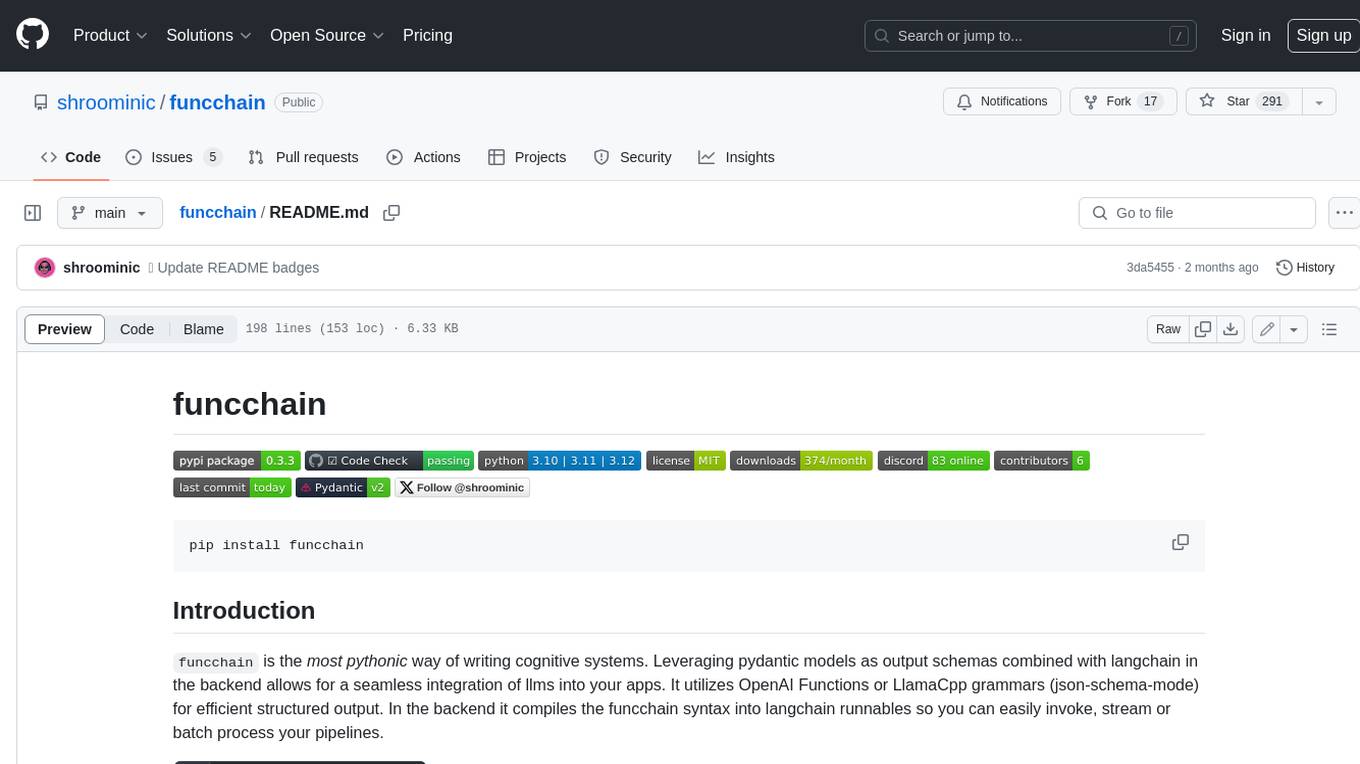
funcchain
Funcchain is a Python library that allows you to easily write cognitive systems by leveraging Pydantic models as output schemas and LangChain in the backend. It provides a seamless integration of LLMs into your apps, utilizing OpenAI Functions or LlamaCpp grammars (json-schema-mode) for efficient structured output. Funcchain compiles the Funcchain syntax into LangChain runnables, enabling you to invoke, stream, or batch process your pipelines effortlessly.
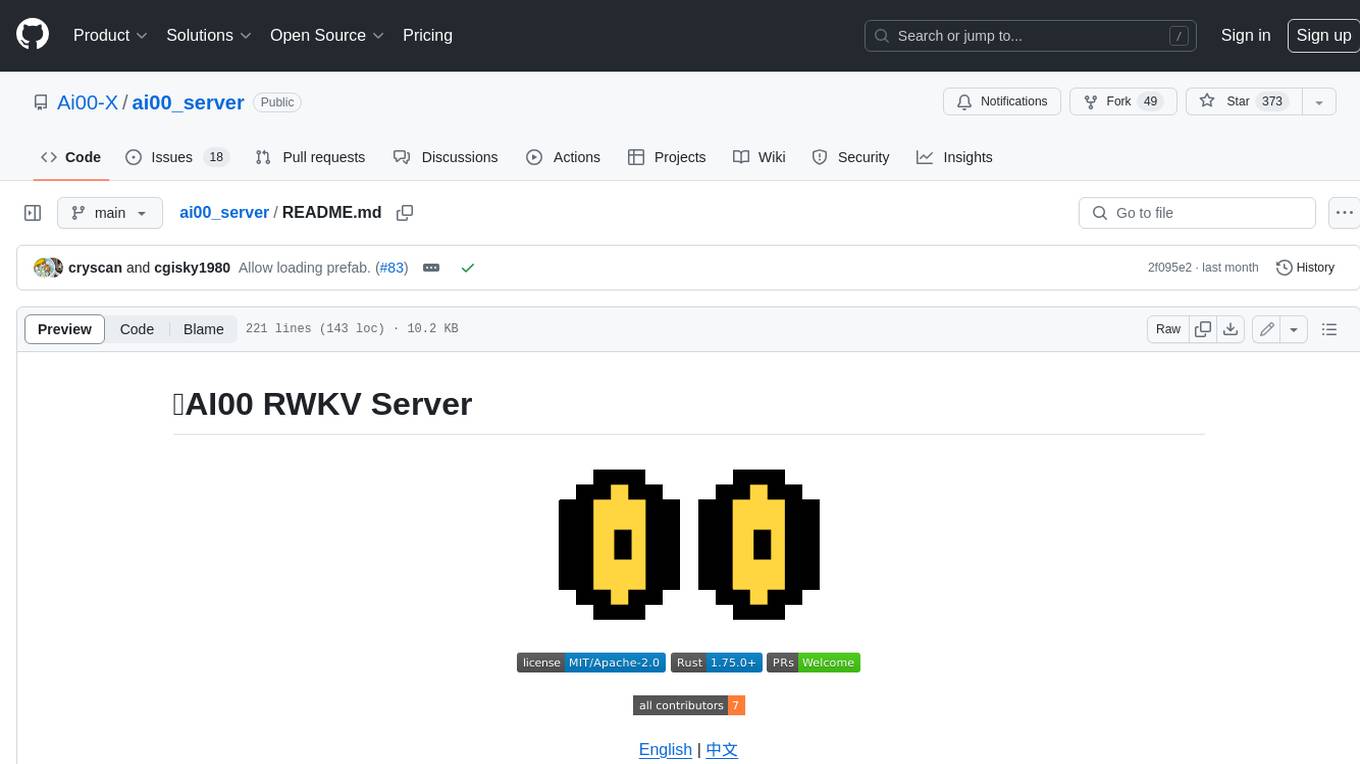
ai00_server
AI00 RWKV Server is an inference API server for the RWKV language model based upon the web-rwkv inference engine. It supports VULKAN parallel and concurrent batched inference and can run on all GPUs that support VULKAN. No need for Nvidia cards!!! AMD cards and even integrated graphics can be accelerated!!! No need for bulky pytorch, CUDA and other runtime environments, it's compact and ready to use out of the box! Compatible with OpenAI's ChatGPT API interface. 100% open source and commercially usable, under the MIT license. If you are looking for a fast, efficient, and easy-to-use LLM API server, then AI00 RWKV Server is your best choice. It can be used for various tasks, including chatbots, text generation, translation, and Q&A.
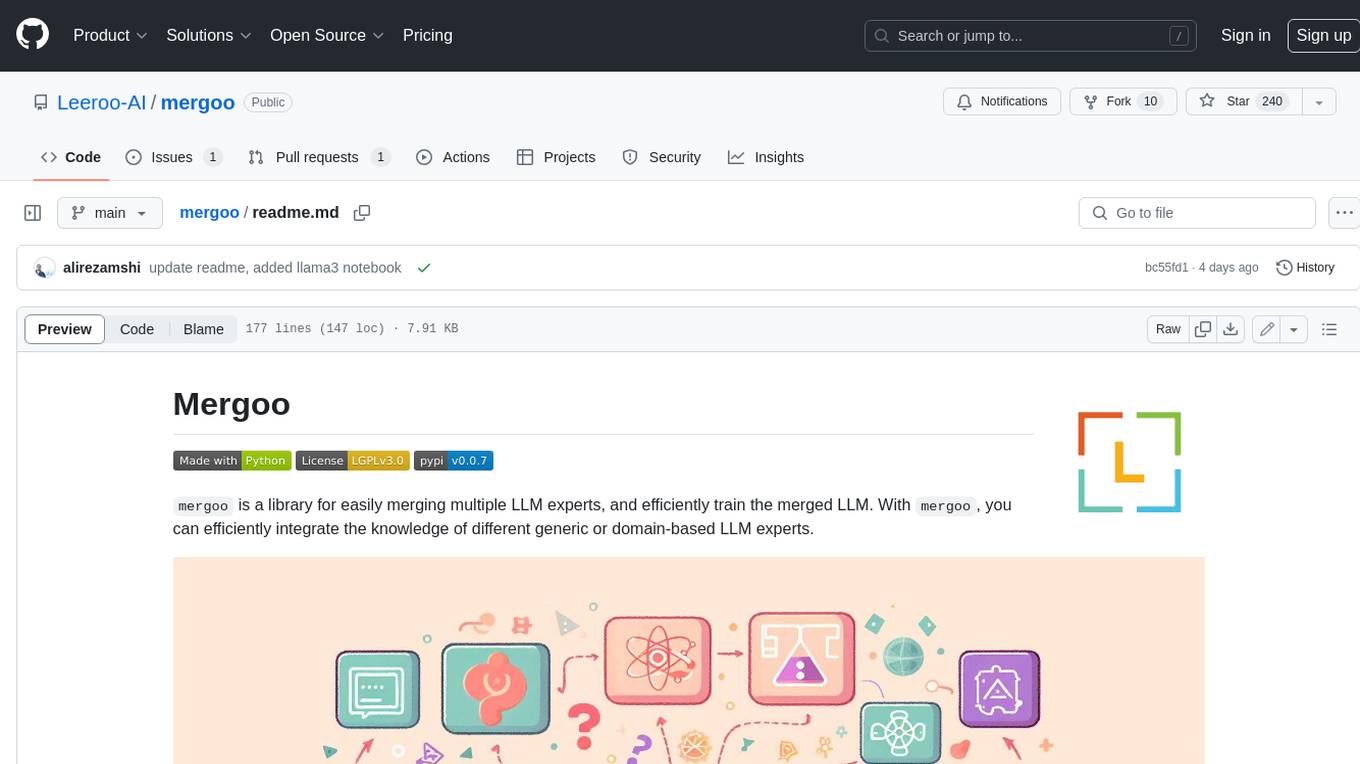
mergoo
Mergoo is a library for easily merging multiple LLM experts and efficiently training the merged LLM. With Mergoo, you can efficiently integrate the knowledge of different generic or domain-based LLM experts. Mergoo supports several merging methods, including Mixture-of-Experts, Mixture-of-Adapters, and Layer-wise merging. It also supports various base models, including LLaMa, Mistral, and BERT, and trainers, including Hugging Face Trainer, SFTrainer, and PEFT. Mergoo provides flexible merging for each layer and supports training choices such as only routing MoE layers or fully fine-tuning the merged LLM.
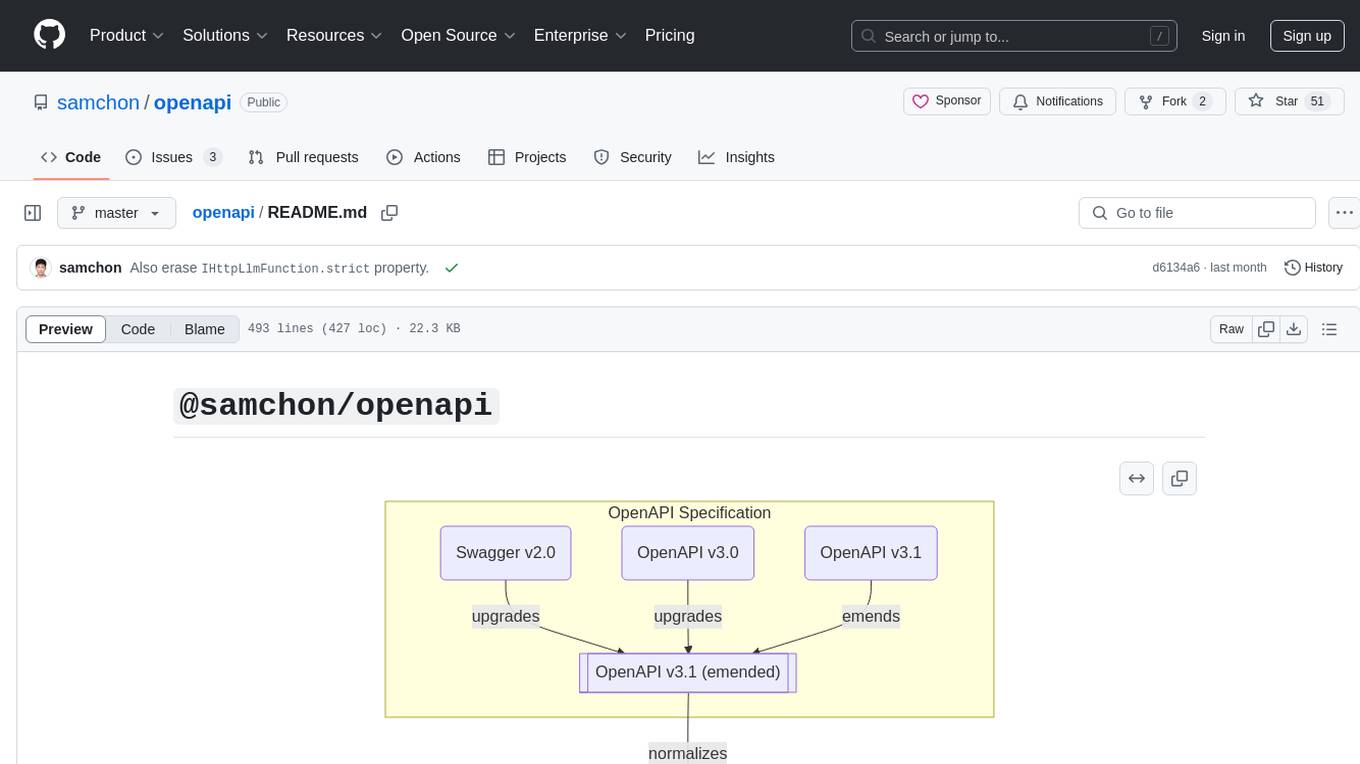
openapi
The `@samchon/openapi` repository is a collection of OpenAPI types and converters for various versions of OpenAPI specifications. It includes an 'emended' OpenAPI v3.1 specification that enhances clarity by removing ambiguous and duplicated expressions. The repository also provides an application composer for LLM (Large Language Model) function calling from OpenAPI documents, allowing users to easily perform LLM function calls based on the Swagger document. Conversions to different versions of OpenAPI documents are also supported, all based on the emended OpenAPI v3.1 specification. Users can validate their OpenAPI documents using the `typia` library with `@samchon/openapi` types, ensuring compliance with standard specifications.
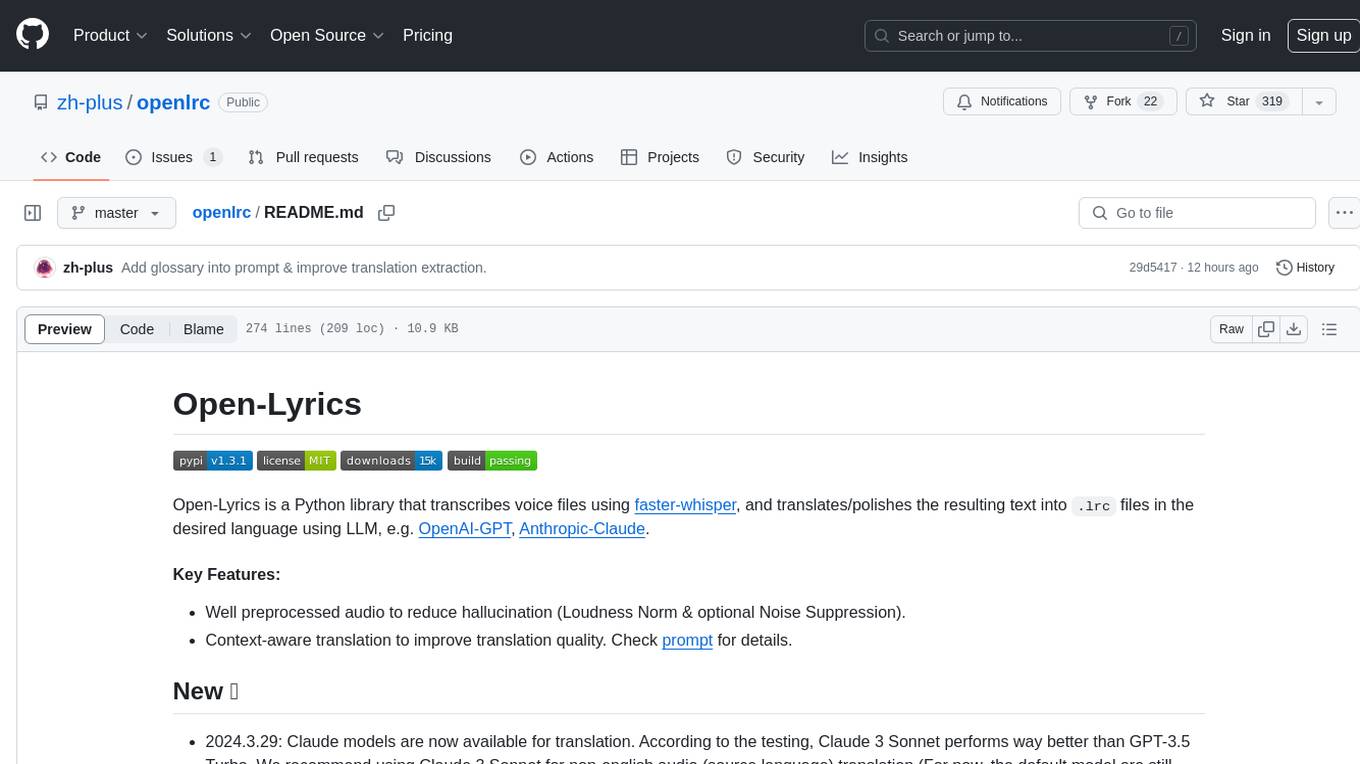
openlrc
Open-Lyrics is a Python library that transcribes voice files using faster-whisper and translates/polishes the resulting text into `.lrc` files in the desired language using LLM, e.g. OpenAI-GPT, Anthropic-Claude. It offers well preprocessed audio to reduce hallucination and context-aware translation to improve translation quality. Users can install the library from PyPI or GitHub and follow the installation steps to set up the environment. The tool supports GUI usage and provides Python code examples for transcription and translation tasks. It also includes features like utilizing context and glossary for translation enhancement, pricing information for different models, and a list of todo tasks for future improvements.
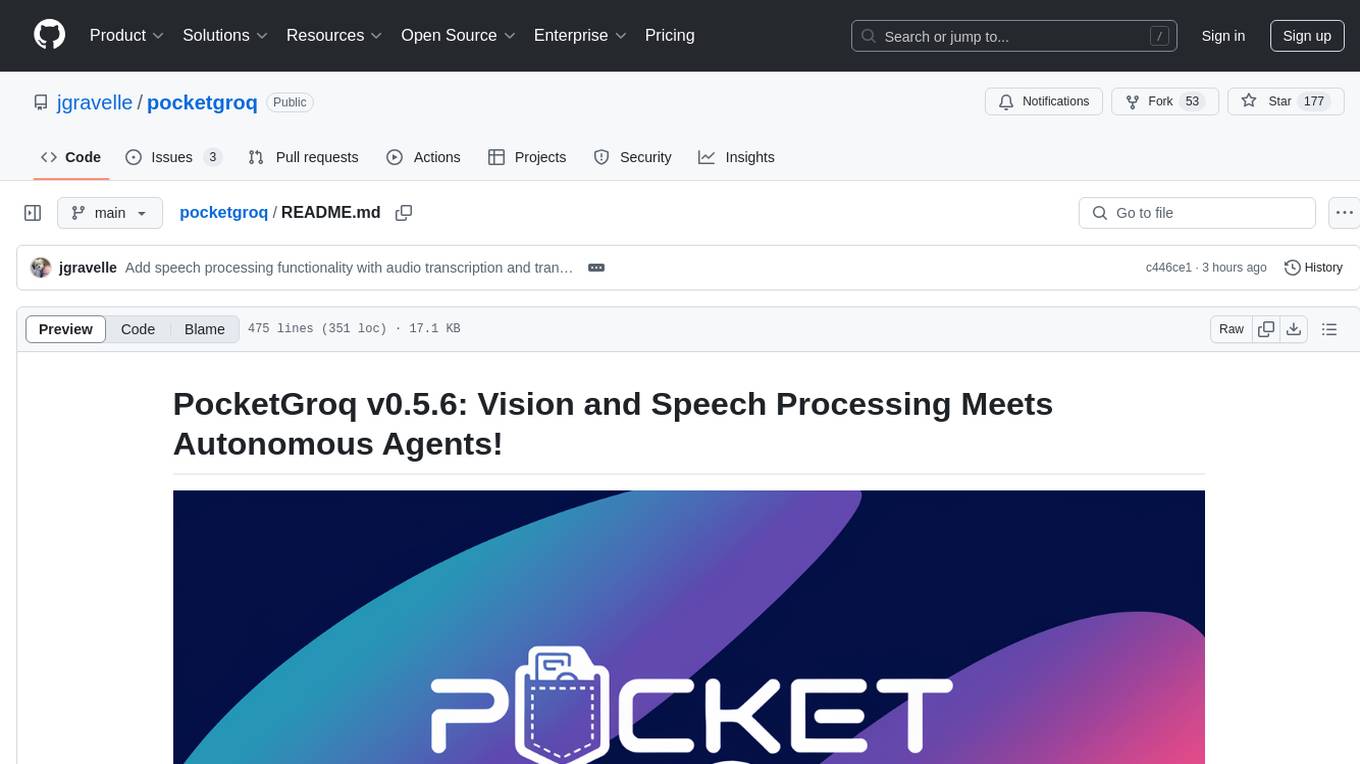
pocketgroq
PocketGroq is a tool that provides advanced functionalities for text generation, web scraping, web search, and AI response evaluation. It includes features like an Autonomous Agent for answering questions, web crawling and scraping capabilities, enhanced web search functionality, and flexible integration with Ollama server. Users can customize the agent's behavior, evaluate responses using AI, and utilize various methods for text generation, conversation management, and Chain of Thought reasoning. The tool offers comprehensive methods for different tasks, such as initializing RAG, error handling, and tool management. PocketGroq is designed to enhance development processes and enable the creation of AI-powered applications with ease.
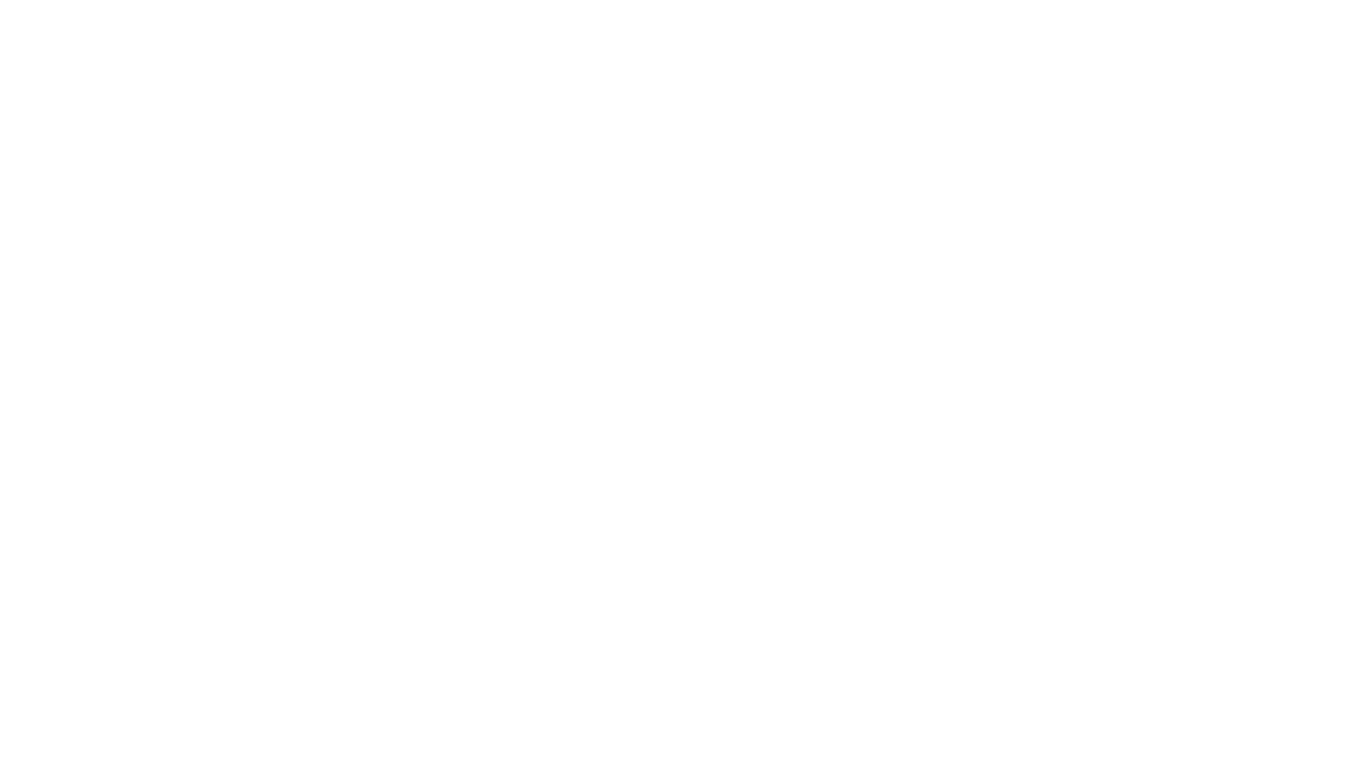
litdata
LitData is a tool designed for blazingly fast, distributed streaming of training data from any cloud storage. It allows users to transform and optimize data in cloud storage environments efficiently and intuitively, supporting various data types like images, text, video, audio, geo-spatial, and multimodal data. LitData integrates smoothly with frameworks such as LitGPT and PyTorch, enabling seamless streaming of data to multiple machines. Key features include multi-GPU/multi-node support, easy data mixing, pause & resume functionality, support for profiling, memory footprint reduction, cache size configuration, and on-prem optimizations. The tool also provides benchmarks for measuring streaming speed and conversion efficiency, along with runnable templates for different data types. LitData enables infinite cloud data processing by utilizing the Lightning.ai platform to scale data processing with optimized machines.
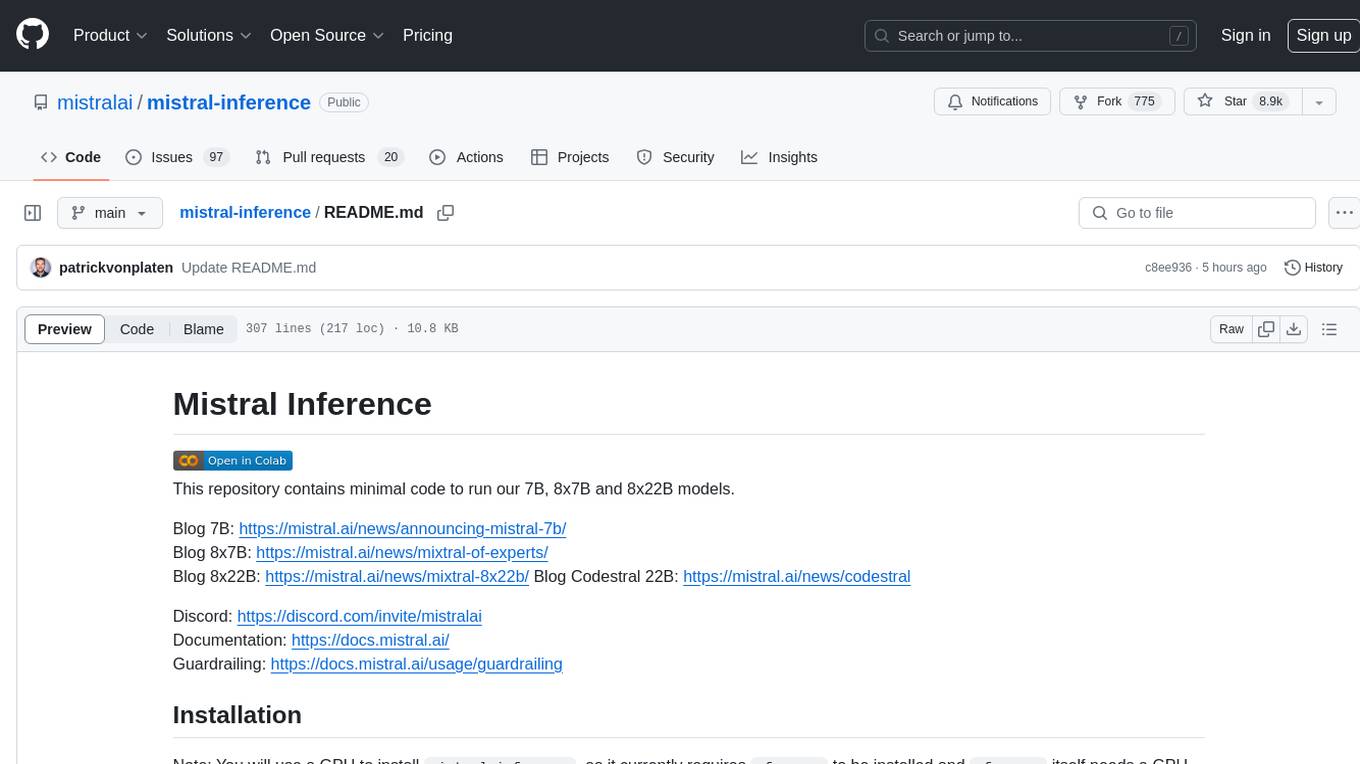
mistral-inference
Mistral Inference repository contains minimal code to run 7B, 8x7B, and 8x22B models. It provides model download links, installation instructions, and usage guidelines for running models via CLI or Python. The repository also includes information on guardrailing, model platforms, deployment, and references. Users can interact with models through commands like mistral-demo, mistral-chat, and mistral-common. Mistral AI models support function calling and chat interactions for tasks like testing models, chatting with models, and using Codestral as a coding assistant. The repository offers detailed documentation and links to blogs for further information.
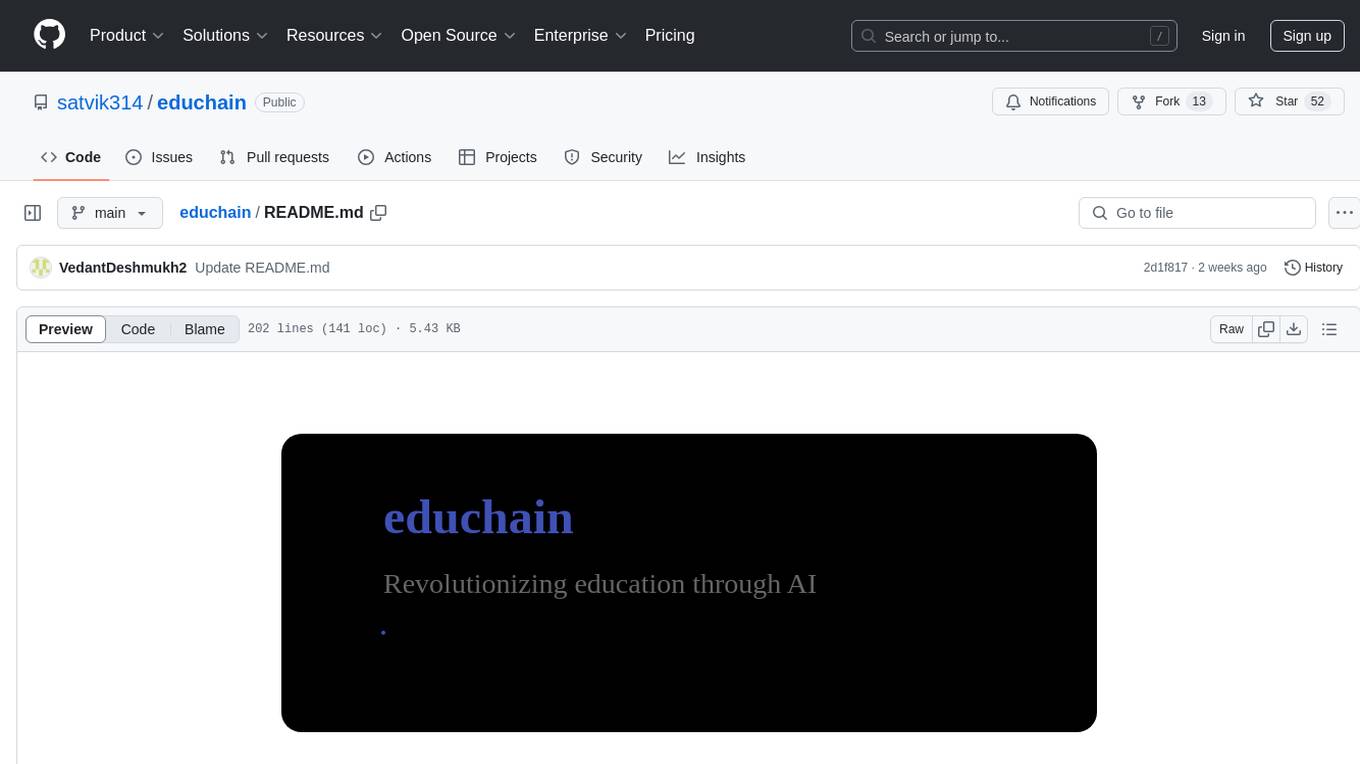
educhain
Educhain is a powerful Python package that leverages Generative AI to create engaging and personalized educational content. It enables users to generate multiple-choice questions, create lesson plans, and support various LLM models. Users can export questions to JSON, PDF, and CSV formats, customize prompt templates, and generate questions from text, PDF, URL files, youtube videos, and images. Educhain outperforms traditional methods in content generation speed and quality. It offers advanced configuration options and has a roadmap for future enhancements, including integration with popular Learning Management Systems and a mobile app for content generation on-the-go.
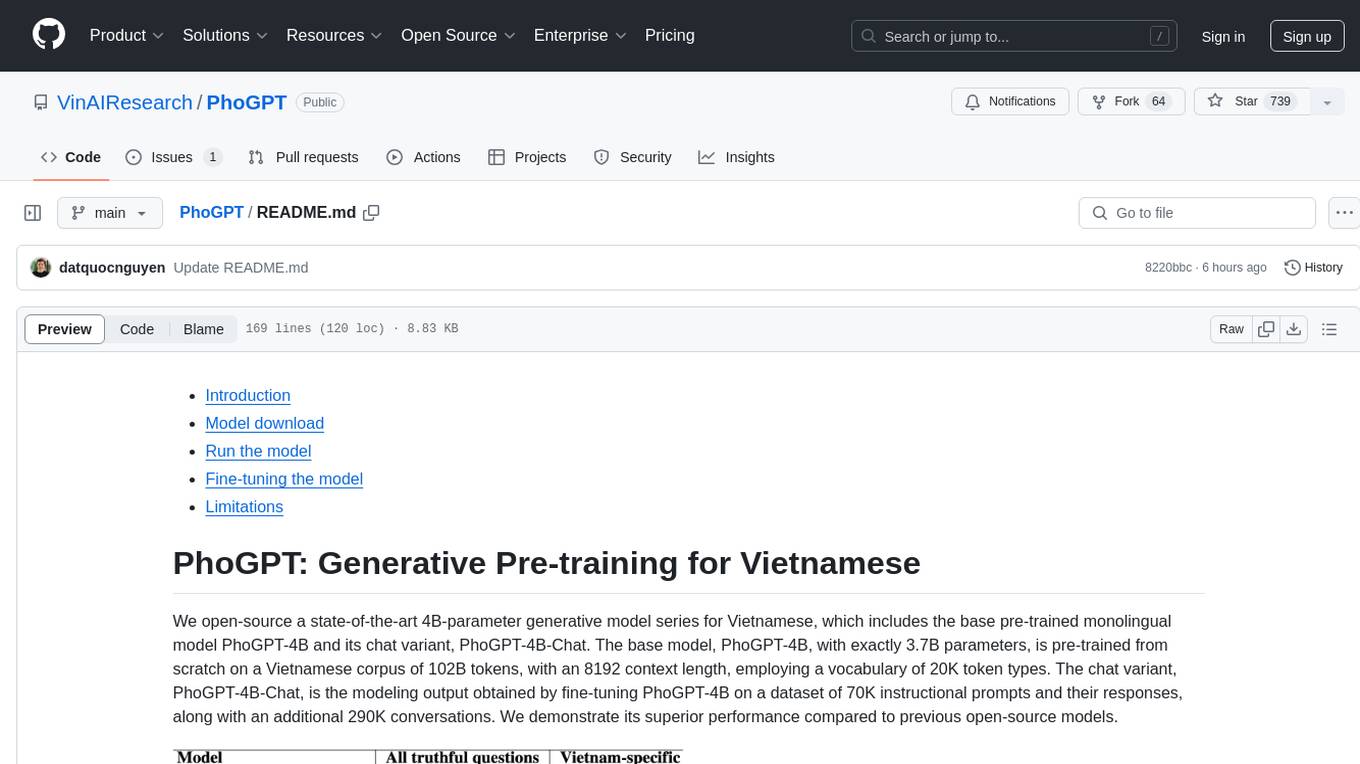
PhoGPT
PhoGPT is an open-source 4B-parameter generative model series for Vietnamese, including the base pre-trained monolingual model PhoGPT-4B and its chat variant, PhoGPT-4B-Chat. PhoGPT-4B is pre-trained from scratch on a Vietnamese corpus of 102B tokens, with an 8192 context length and a vocabulary of 20K token types. PhoGPT-4B-Chat is fine-tuned on instructional prompts and conversations, demonstrating superior performance. Users can run the model with inference engines like vLLM and Text Generation Inference, and fine-tune it using llm-foundry. However, PhoGPT has limitations in reasoning, coding, and mathematics tasks, and may generate harmful or biased responses.
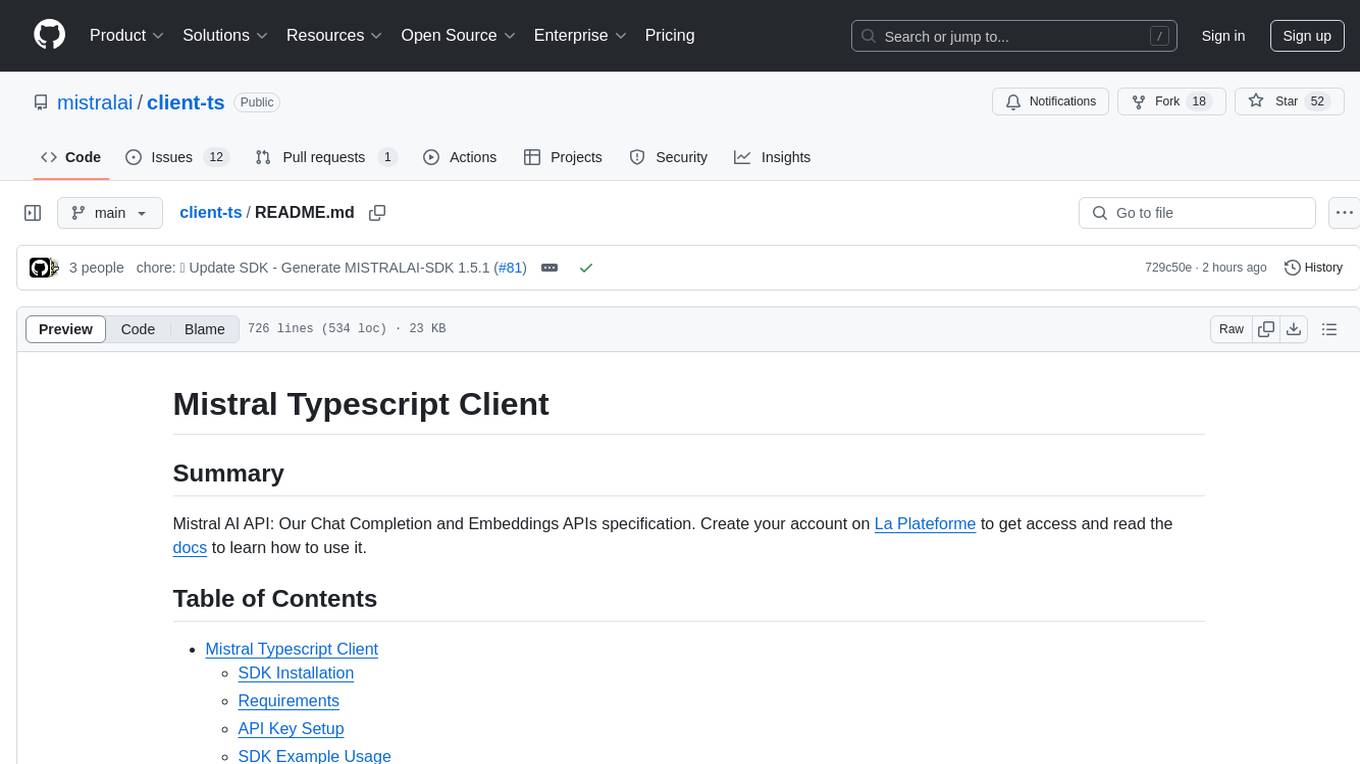
client-ts
Mistral Typescript Client is an SDK for Mistral AI API, providing Chat Completion and Embeddings APIs. It allows users to create chat completions, upload files, create agent completions, create embedding requests, and more. The SDK supports various JavaScript runtimes and provides detailed documentation on installation, requirements, API key setup, example usage, error handling, server selection, custom HTTP client, authentication, providers support, standalone functions, debugging, and contributions.
For similar tasks
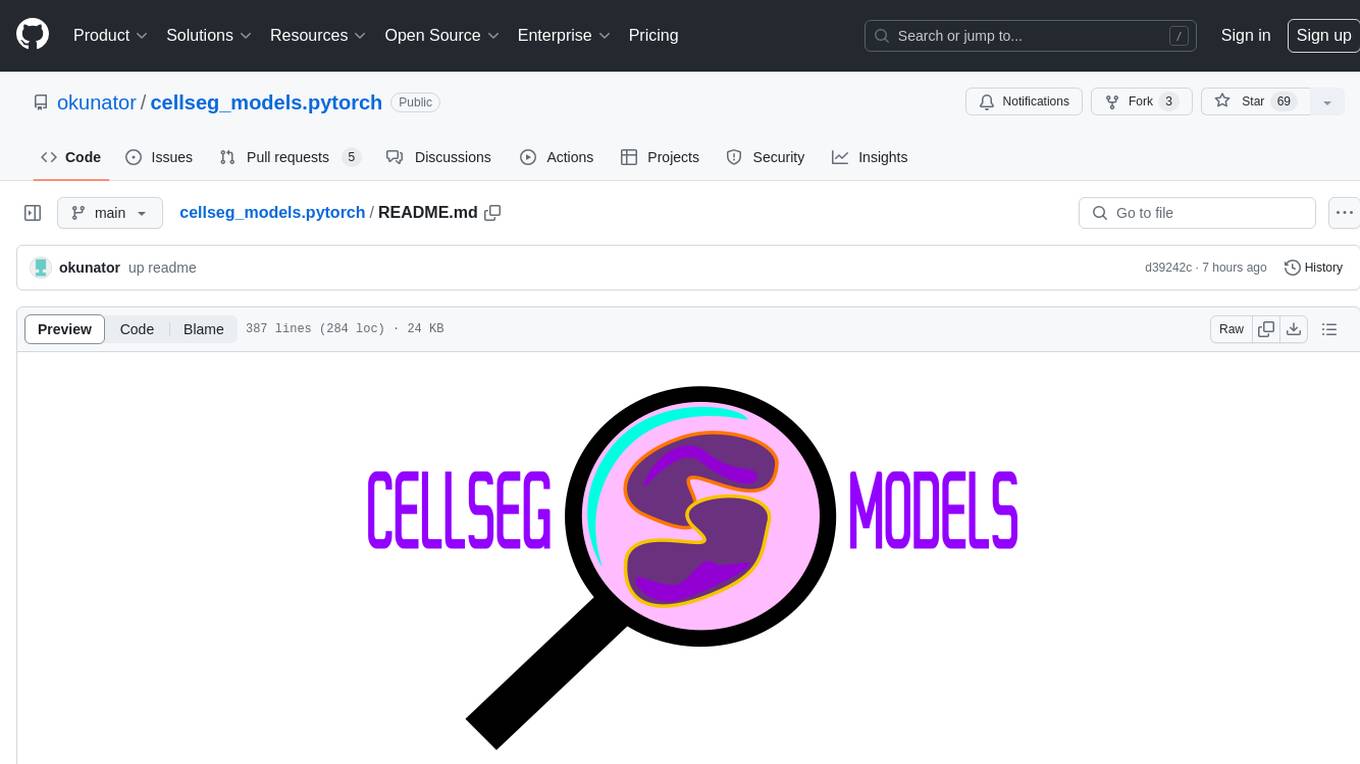
cellseg_models.pytorch
cellseg-models.pytorch is a Python library built upon PyTorch for 2D cell/nuclei instance segmentation models. It provides multi-task encoder-decoder architectures and post-processing methods for segmenting cell/nuclei instances. The library offers high-level API to define segmentation models, open-source datasets for training, flexibility to modify model components, sliding window inference, multi-GPU inference, benchmarking utilities, regularization techniques, and example notebooks for training and finetuning models with different backbones.
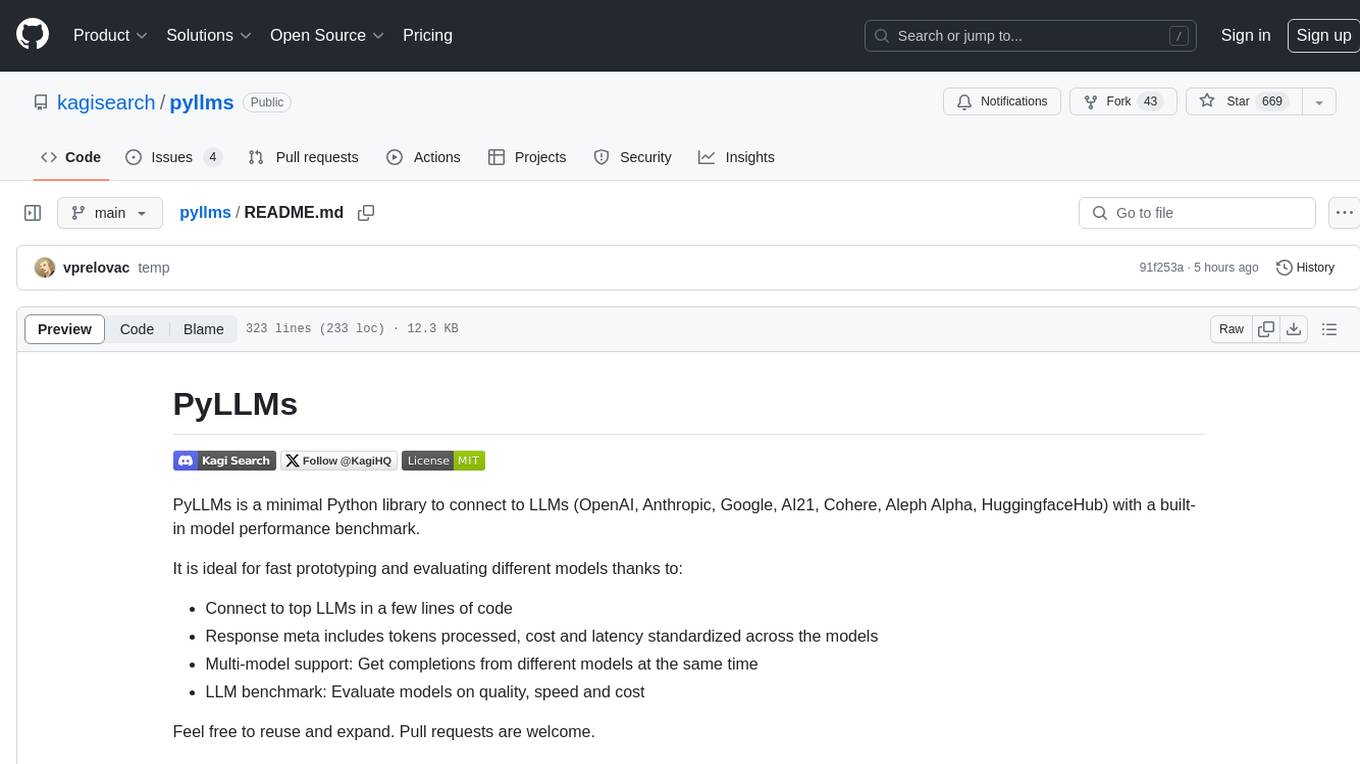
pyllms
PyLLMs is a minimal Python library designed to connect to various Language Model Models (LLMs) such as OpenAI, Anthropic, Google, AI21, Cohere, Aleph Alpha, and HuggingfaceHub. It provides a built-in model performance benchmark for fast prototyping and evaluating different models. Users can easily connect to top LLMs, get completions from multiple models simultaneously, and evaluate models on quality, speed, and cost. The library supports asynchronous completion, streaming from compatible models, and multi-model initialization for testing and comparison. Additionally, it offers features like passing chat history, system messages, counting tokens, and benchmarking models based on quality, speed, and cost.
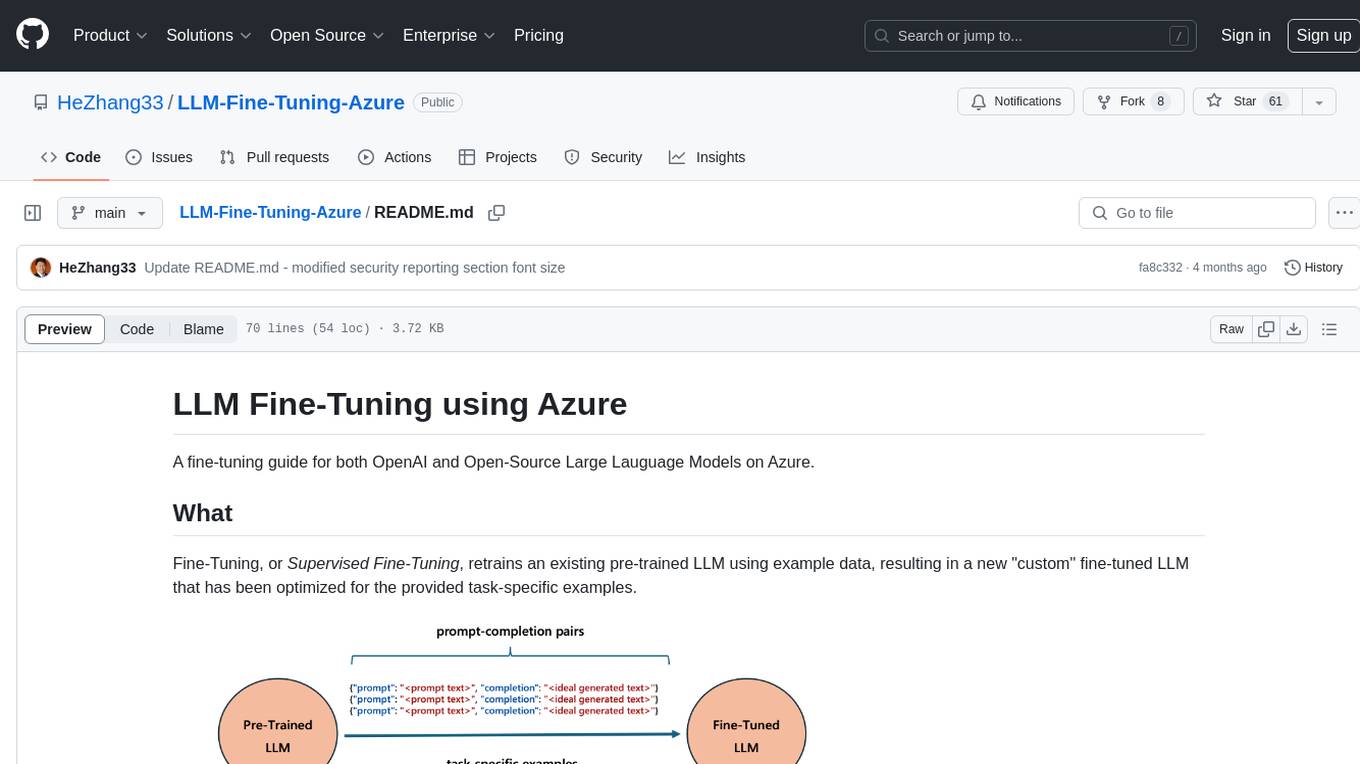
LLM-Fine-Tuning-Azure
A fine-tuning guide for both OpenAI and Open-Source Large Language Models on Azure. Fine-Tuning retrains an existing pre-trained LLM using example data, resulting in a new 'custom' fine-tuned LLM optimized for task-specific examples. Use cases include improving LLM performance on specific tasks and introducing information not well represented by the base LLM model. Suitable for cases where latency is critical, high accuracy is required, and clear evaluation metrics are available. Learning path includes labs for fine-tuning GPT and Llama2 models via Dashboards and Python SDK.
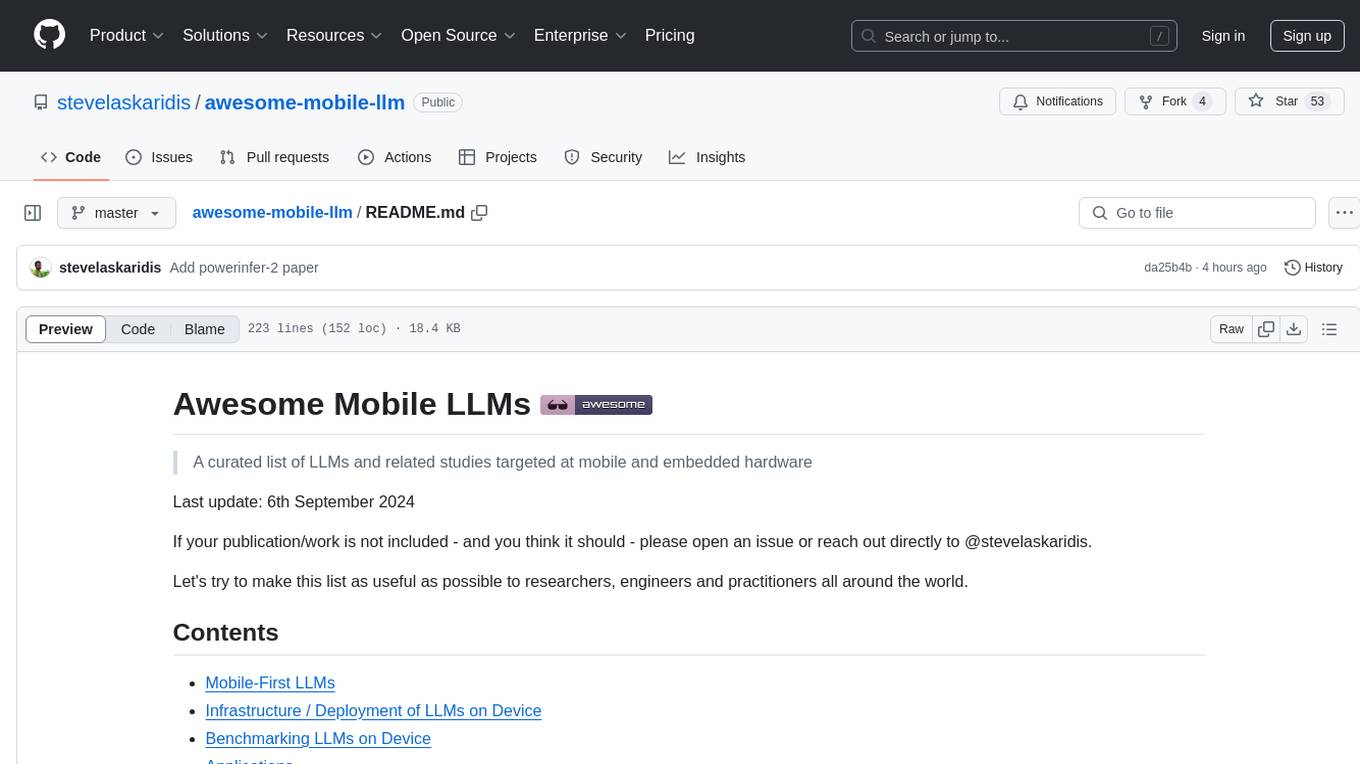
awesome-mobile-llm
Awesome Mobile LLMs is a curated list of Large Language Models (LLMs) and related studies focused on mobile and embedded hardware. The repository includes information on various LLM models, deployment frameworks, benchmarking efforts, applications, multimodal LLMs, surveys on efficient LLMs, training LLMs on device, mobile-related use-cases, industry announcements, and related repositories. It aims to be a valuable resource for researchers, engineers, and practitioners interested in mobile LLMs.
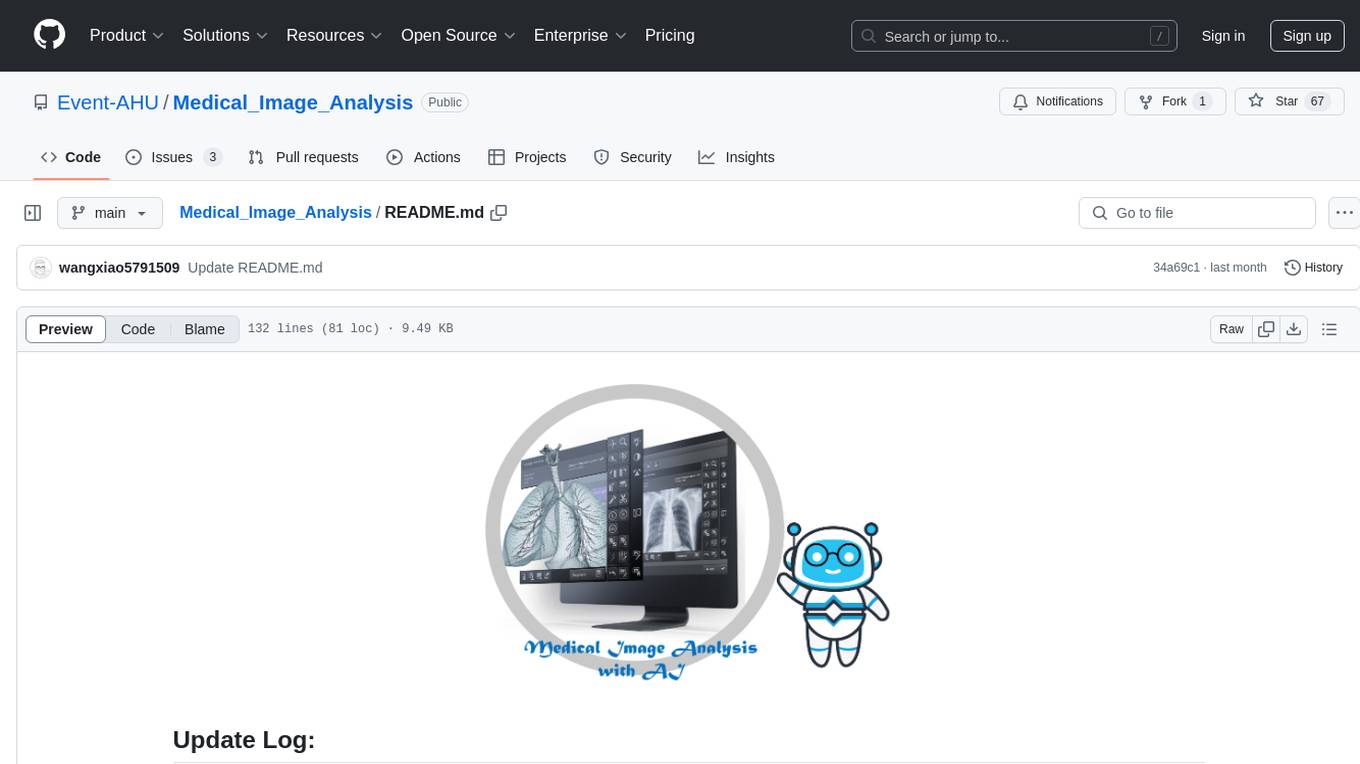
Medical_Image_Analysis
The Medical_Image_Analysis repository focuses on X-ray image-based medical report generation using large language models. It provides pre-trained models and benchmarks for CheXpert Plus dataset, context sample retrieval for X-ray report generation, and pre-training on high-definition X-ray images. The goal is to enhance diagnostic accuracy and reduce patient wait times by improving X-ray report generation through advanced AI techniques.
For similar jobs
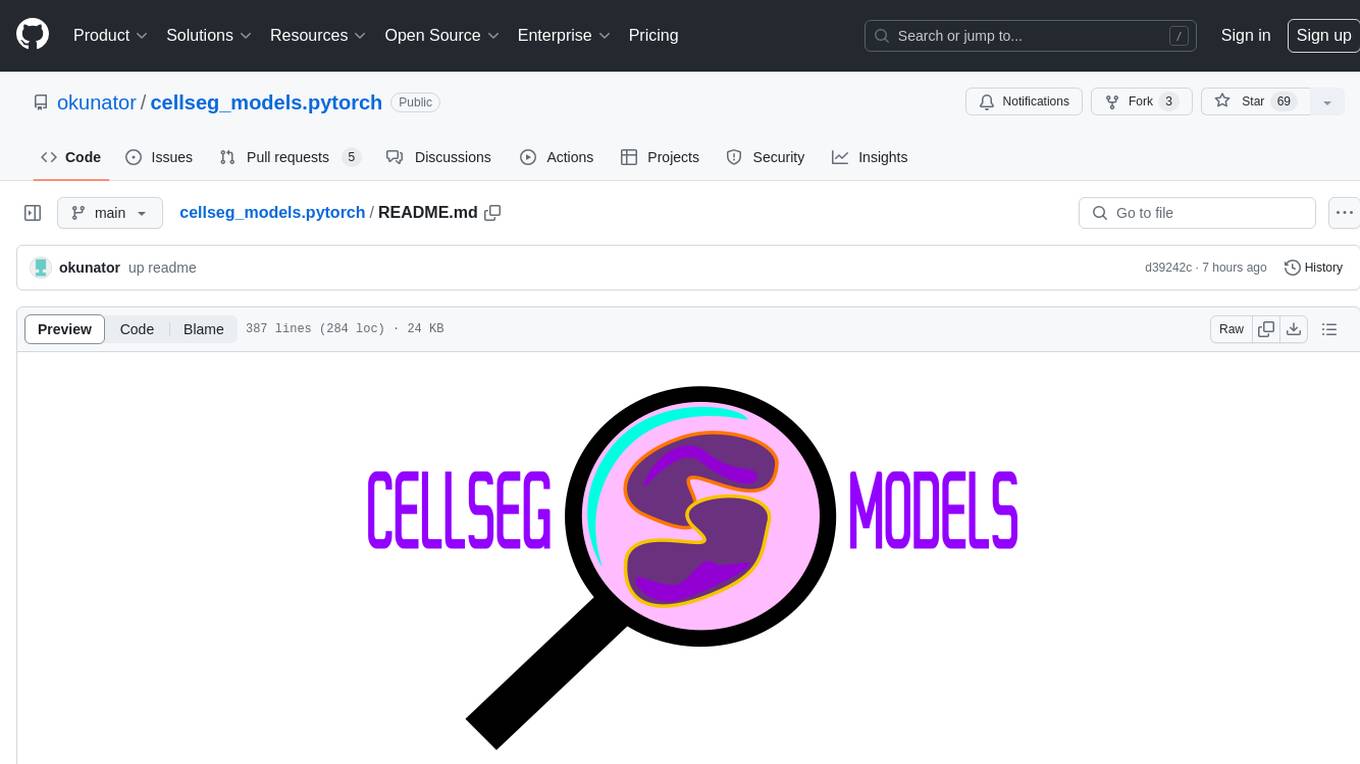
cellseg_models.pytorch
cellseg-models.pytorch is a Python library built upon PyTorch for 2D cell/nuclei instance segmentation models. It provides multi-task encoder-decoder architectures and post-processing methods for segmenting cell/nuclei instances. The library offers high-level API to define segmentation models, open-source datasets for training, flexibility to modify model components, sliding window inference, multi-GPU inference, benchmarking utilities, regularization techniques, and example notebooks for training and finetuning models with different backbones.
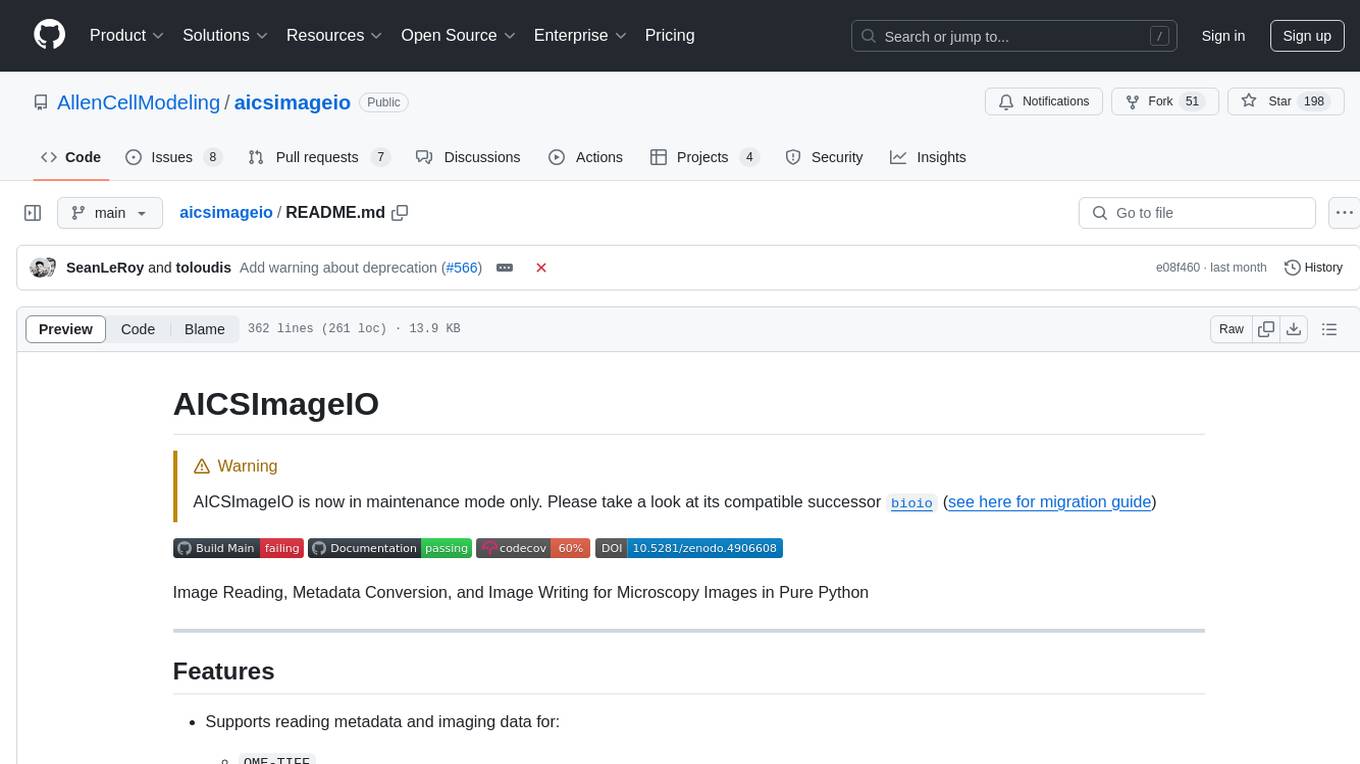
aicsimageio
AICSImageIO is a Python tool for Image Reading, Metadata Conversion, and Image Writing for Microscopy Images. It supports various file formats like OME-TIFF, TIFF, ND2, DV, CZI, LIF, PNG, GIF, and Bio-Formats. Users can read and write metadata and imaging data, work with different file systems like local paths, HTTP URLs, s3fs, and gcsfs. The tool provides functionalities for full image reading, delayed image reading, mosaic image reading, metadata reading, xarray coordinate plane attachment, cloud IO support, and saving to OME-TIFF. It also offers benchmarking and developer resources.
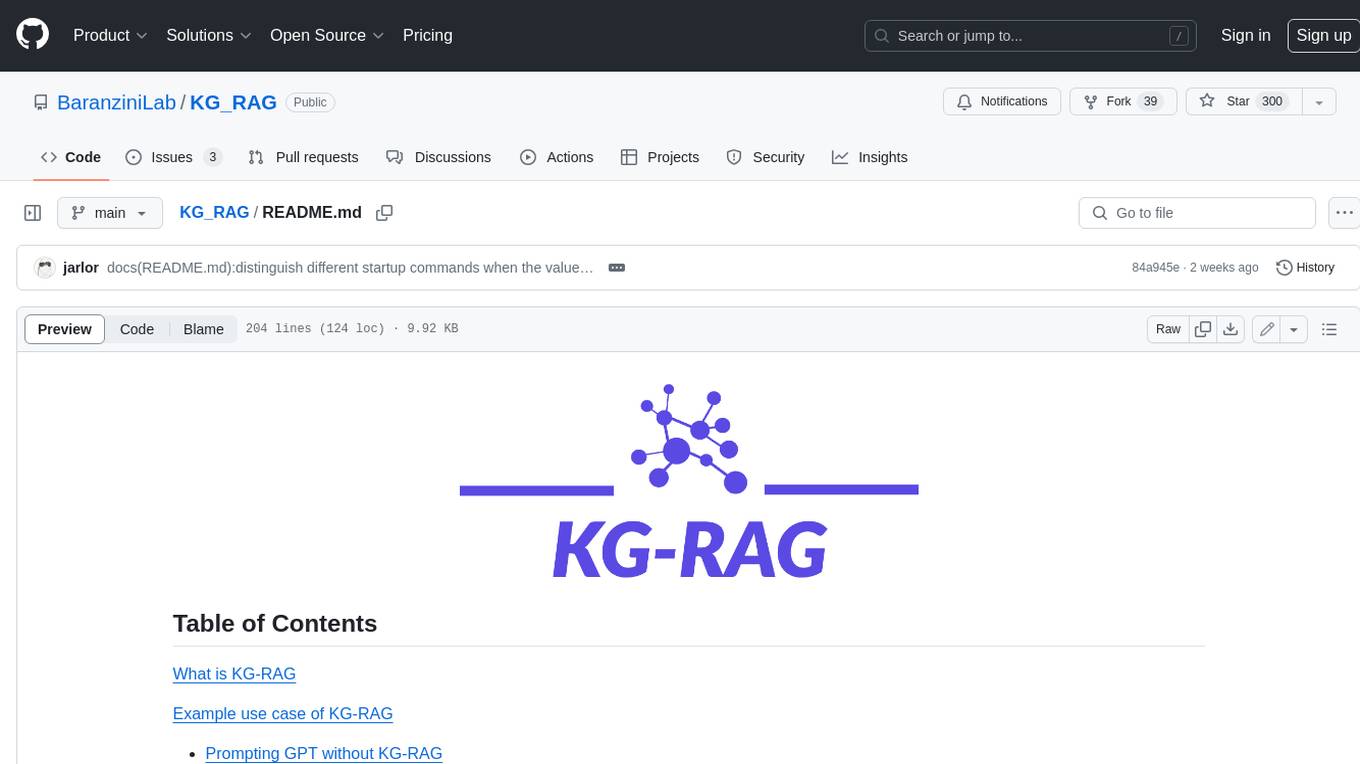
KG_RAG
KG-RAG (Knowledge Graph-based Retrieval Augmented Generation) is a task agnostic framework that combines the explicit knowledge of a Knowledge Graph (KG) with the implicit knowledge of a Large Language Model (LLM). KG-RAG extracts "prompt-aware context" from a KG, which is defined as the minimal context sufficient enough to respond to the user prompt. This framework empowers a general-purpose LLM by incorporating an optimized domain-specific 'prompt-aware context' from a biomedical KG. KG-RAG is specifically designed for running prompts related to Diseases.
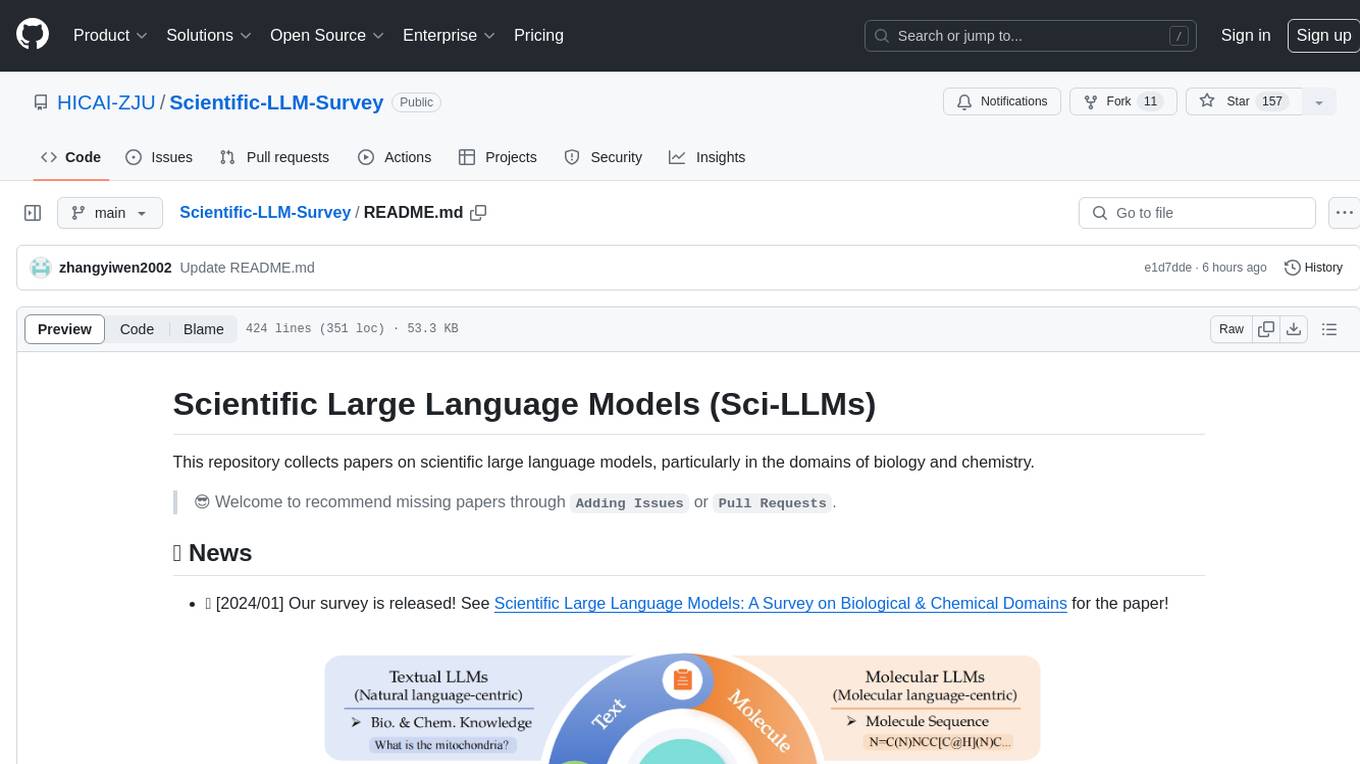
Scientific-LLM-Survey
Scientific Large Language Models (Sci-LLMs) is a repository that collects papers on scientific large language models, focusing on biology and chemistry domains. It includes textual, molecular, protein, and genomic languages, as well as multimodal language. The repository covers various large language models for tasks such as molecule property prediction, interaction prediction, protein sequence representation, protein sequence generation/design, DNA-protein interaction prediction, and RNA prediction. It also provides datasets and benchmarks for evaluating these models. The repository aims to facilitate research and development in the field of scientific language modeling.
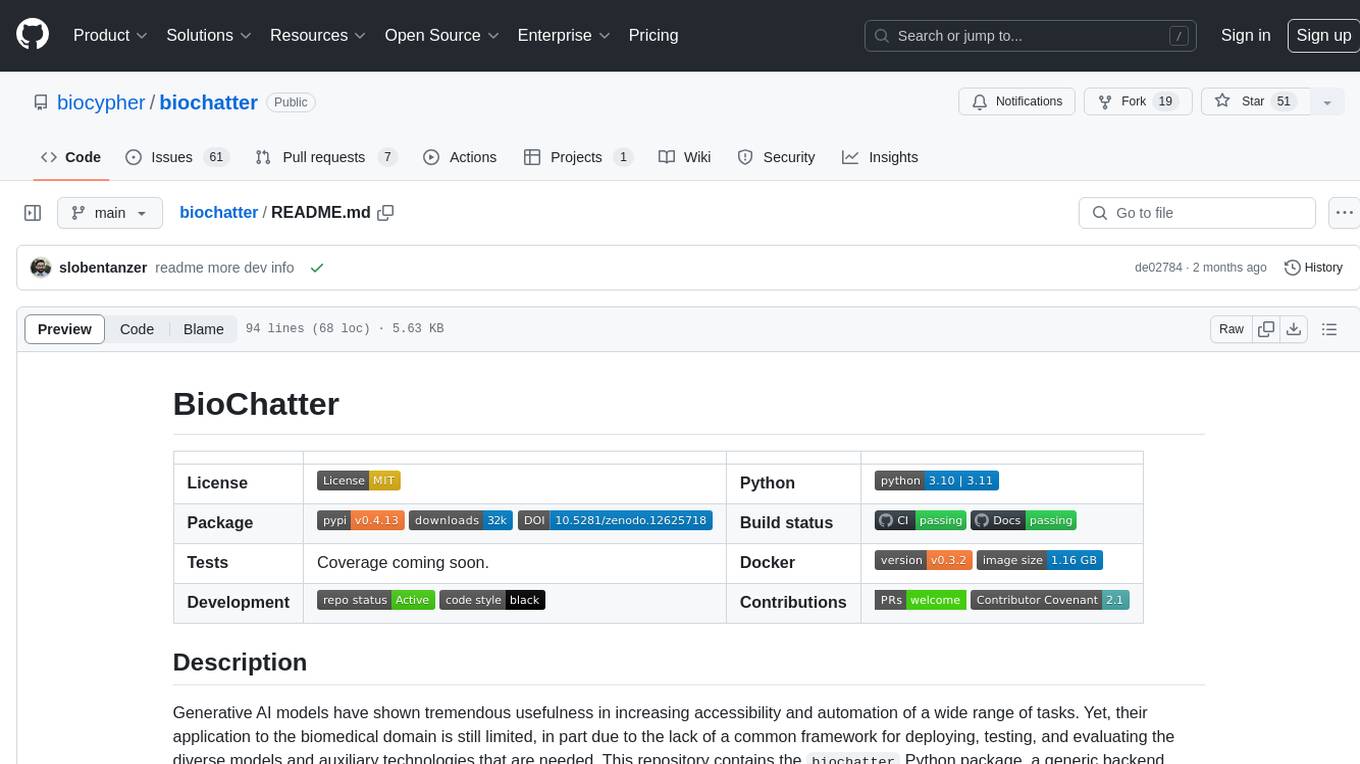
biochatter
Generative AI models have shown tremendous usefulness in increasing accessibility and automation of a wide range of tasks. This repository contains the `biochatter` Python package, a generic backend library for the connection of biomedical applications to conversational AI. It aims to provide a common framework for deploying, testing, and evaluating diverse models and auxiliary technologies in the biomedical domain. BioChatter is part of the BioCypher ecosystem, connecting natively to BioCypher knowledge graphs.
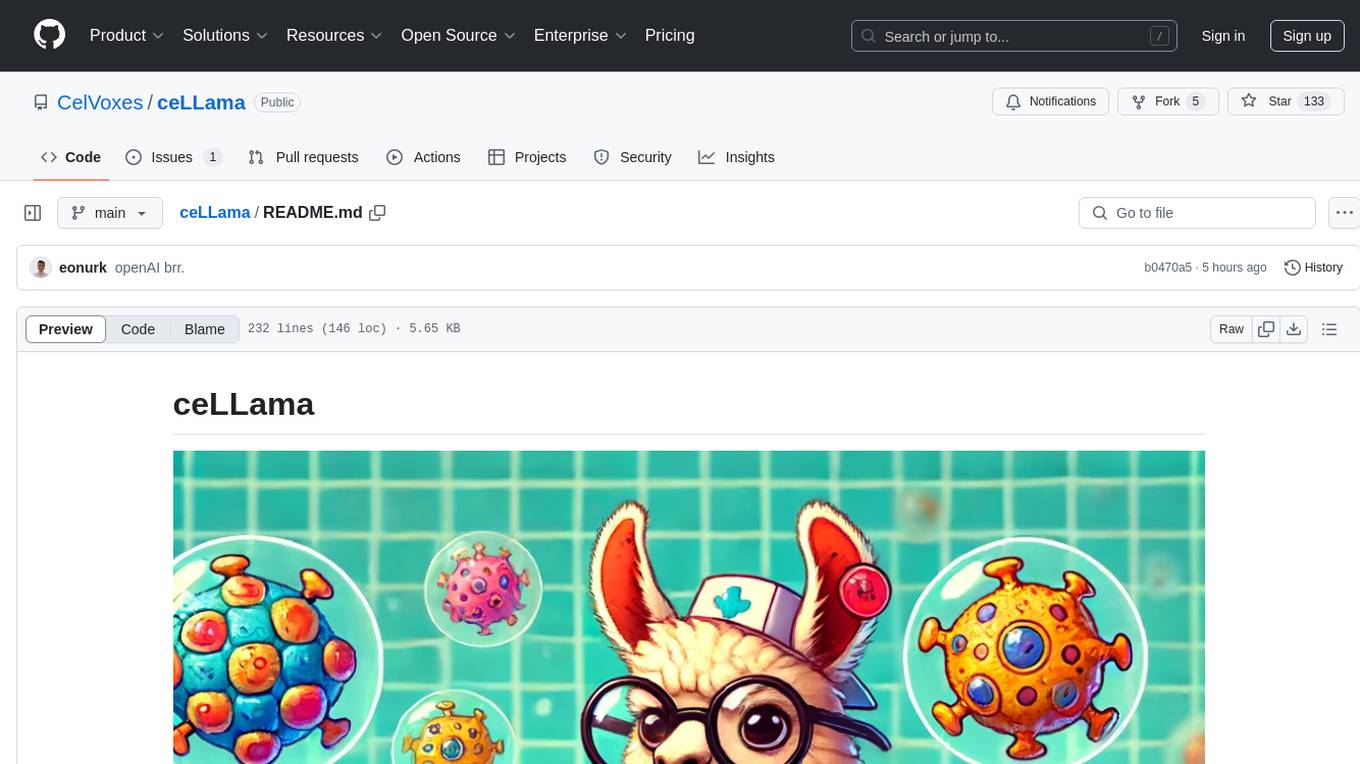
ceLLama
ceLLama is a streamlined automation pipeline for cell type annotations using large-language models (LLMs). It operates locally to ensure privacy, provides comprehensive analysis by considering negative genes, offers efficient processing speed, and generates customized reports. Ideal for quick and preliminary cell type checks.
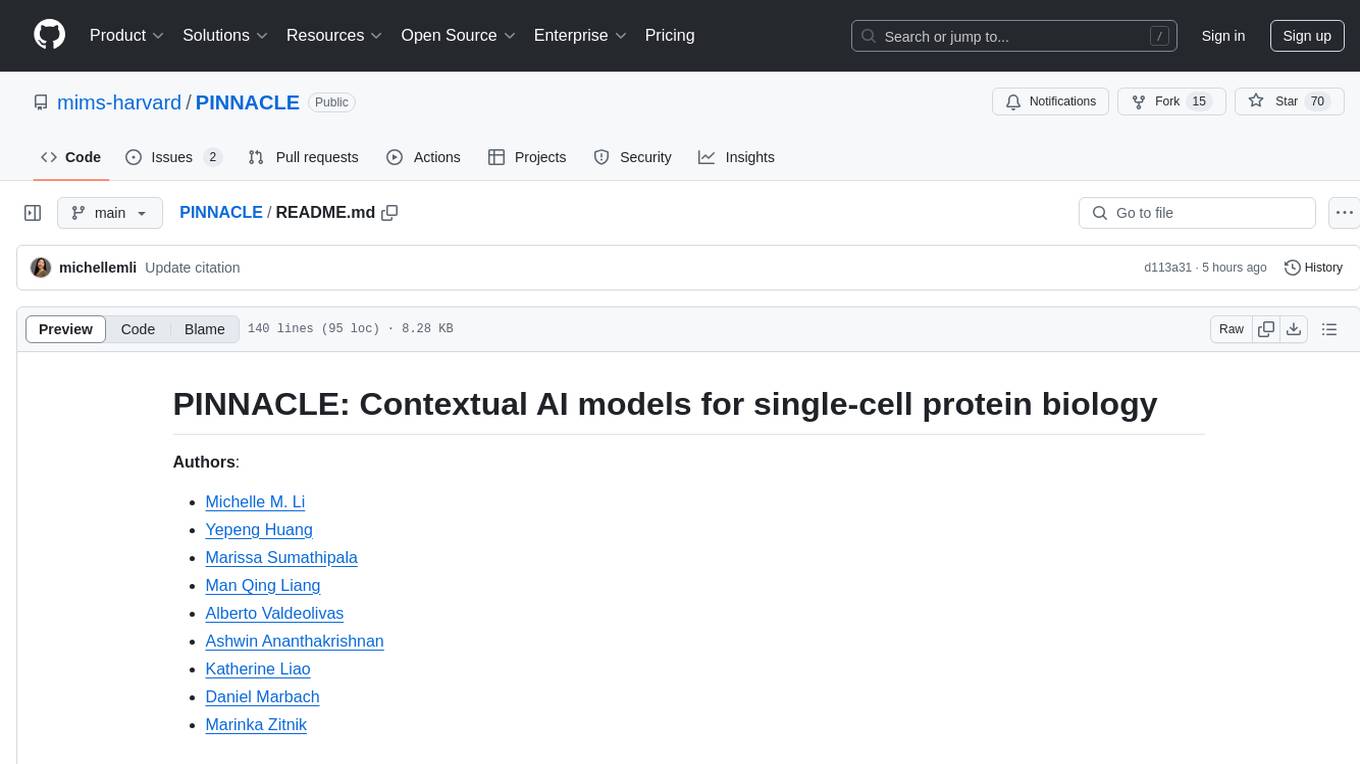
PINNACLE
PINNACLE is a flexible geometric deep learning approach that trains on contextualized protein interaction networks to generate context-aware protein representations. It provides protein representations split across various cell-type contexts from different tissues and organs. The tool can be fine-tuned to study the genomic effects of drugs and nominate promising protein targets and cell-type contexts for further investigation. PINNACLE exemplifies the paradigm of incorporating context-specific effects for studying biological systems, especially the impact of disease and therapeutics.
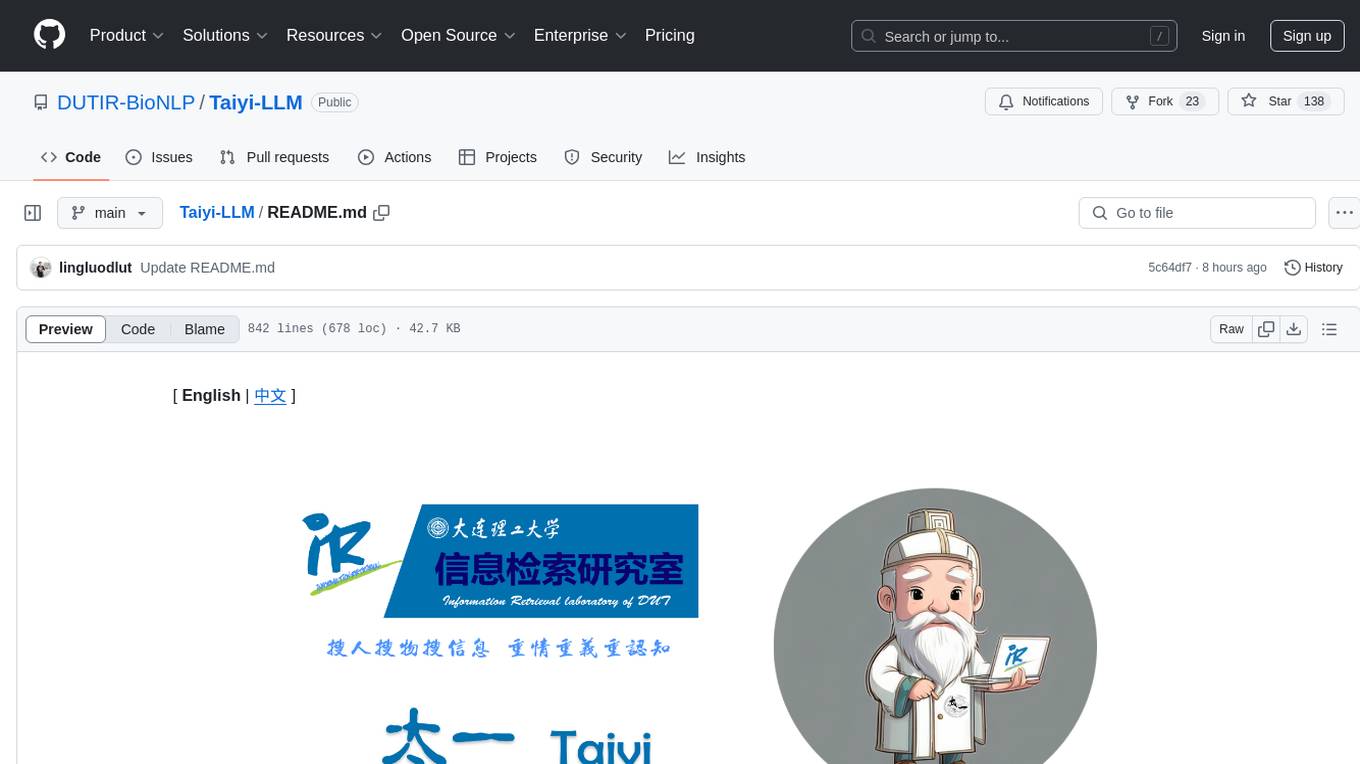
Taiyi-LLM
Taiyi (太一) is a bilingual large language model fine-tuned for diverse biomedical tasks. It aims to facilitate communication between healthcare professionals and patients, provide medical information, and assist in diagnosis, biomedical knowledge discovery, drug development, and personalized healthcare solutions. The model is based on the Qwen-7B-base model and has been fine-tuned using rich bilingual instruction data. It covers tasks such as question answering, biomedical dialogue, medical report generation, biomedical information extraction, machine translation, title generation, text classification, and text semantic similarity. The project also provides standardized data formats, model training details, model inference guidelines, and overall performance metrics across various BioNLP tasks.