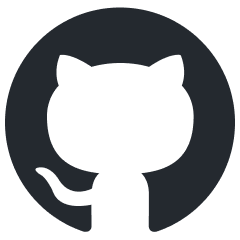
mergoo
A library for easily merging multiple LLM experts, and efficiently train the merged LLM.
Stars: 329
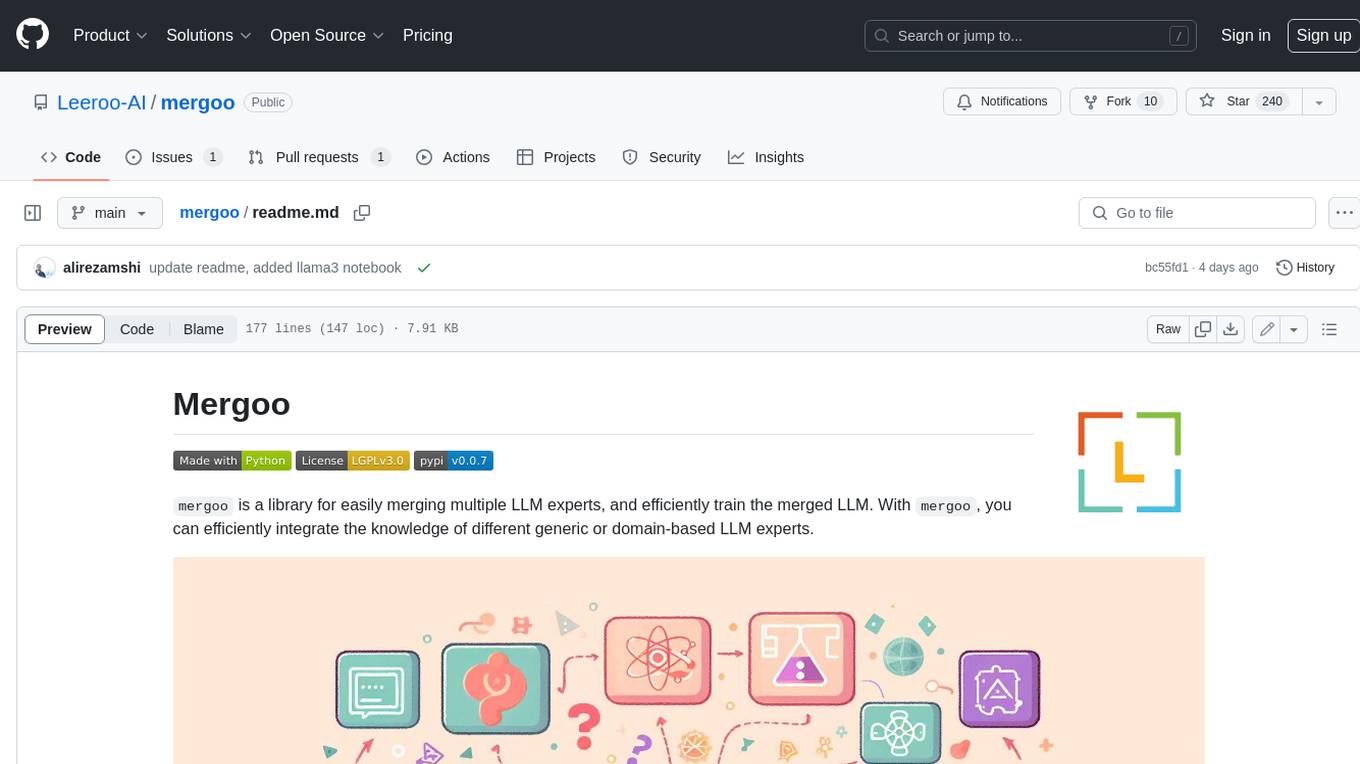
Mergoo is a library for easily merging multiple LLM experts and efficiently training the merged LLM. With Mergoo, you can efficiently integrate the knowledge of different generic or domain-based LLM experts. Mergoo supports several merging methods, including Mixture-of-Experts, Mixture-of-Adapters, and Layer-wise merging. It also supports various base models, including LLaMa, Mistral, and BERT, and trainers, including Hugging Face Trainer, SFTrainer, and PEFT. Mergoo provides flexible merging for each layer and supports training choices such as only routing MoE layers or fully fine-tuning the merged LLM.
README:
mergoo
is a library for easily merging multiple LLM experts, and efficiently train the merged LLM. With mergoo
, you can efficiently integrate the knowledge of different generic or domain-based LLM experts.
- Supports several merging methods: Mixture-of-Experts, Mixture-of-Adapters, and Layer-wise merging
- Flexible merging for each layer
- Base Models supported : Llama(including LLaMa3), Mistral, Phi3, and BERT
- Trainers supported : 🤗 Trainer, SFTrainer, PEFT
- Device Supported: CPU, MPS, GPU
- Training choices: Only Router of MoE layers, or Fully fine-tuning of Merged LLM
If you like the project, consider leaving a ⭐️
Install by pip:
pip install mergoo
Install latest unstable version on Github:
pip install git+https://github.com/Leeroo-AI/mergoo
Install it from the source:
git clone https://github.com/Leeroo-AI/mergoo
cd mergoo
pip install -e .
Specify the config for merging:
-
model_type
: type of base model. choices:mistral
,llama
, orbert
. -
num_experts_per_token
: Number of experts for each token of MoE. -
experts
: config for experts to merge. includesexpert_name
and Hugging Face 🤗model_id
. -
router_layers
: layers chosen for applying Mixture-of-Experts.
This is a sample config when merging fully fine-tuned LLM experts.
config = {
"model_type": "mistral",
"num_experts_per_tok": 2,
"experts": [
{"expert_name": "base_expert", "model_id": "mistralai/Mistral-7B-v0.1"},
{"expert_name": "expert_1", "model_id": "meta-math/MetaMath-Mistral-7B"},
{"expert_name": "expert_2", "model_id": "ajibawa-2023/Code-Mistral-7B"}
],
"router_layers": ["gate_proj", "up_proj", "down_proj"]
}
For the above example, we merged math and code mistral-based experts. Please refer to this notebook for further details!
This is a sample config when merging LoRA fine-tuned LLM experts. mergoo
builds a routing layer on top of LoRAs, resulting in a mixture of adapters.
config = {
"model_type": "mistral",
"num_experts_per_tok": 2,
"base_model": "mistralai/Mistral-7B-v0.1",
"experts": [
{"expert_name": "adapter_1", "model_id": "predibase/customer_support"},
{"expert_name": "adapter_2", "model_id": "predibase/customer_support_accounts"},
{"expert_name": "adapter_3", "model_id": "predibase/customer_support_orders"},
{"expert_name": "adapter_4", "model_id": "predibase/customer_support_payments"}
],
}
The expert_name
starts with adapter
instead of expert
. Please refer to this notebook for further details!
Following the config setup, mergoo
creates the merged LLM as:
import torch
from mergoo.compose_experts import ComposeExperts
# create checkpoint
model_id = "data/mistral_lora_moe"
expertmerger = ComposeExperts(config, torch_dtype=torch.float16)
expertmerger.compose()
expertmerger.save_checkpoint(model_id)
Now, you can easily train the merged LLM with Hugging Face Trainer:
from transformers import Trainer
from mergoo.models.modeling_mistral import MistralForCausalLM
model = MistralForCausalLM.from_pretrained("data/mistral_lora_moe")
# NOTE: 'gate' / router layers are untrained hence weight loading warning would appeare for them
trainer = Trainer( ... )
trainer.train()
After finishing the Quick Start guide, you can explore the tutorials below to further familiarize yourself with mergoo
.
Notebook | Details |
---|---|
MoE with fully fine-tuned LLM experts | Build a unifined Mixture-of-Experts model with fully fine-tuned experts. Inspired by BTX Research (Meta AI). |
MoE with LoRA fine-tuned experts | Build a Mixture of Adaptes expert. Inspired by xlora | Mixture-of-LoRAs | MoLE | PHATGOOSE | MoELoRA |
Hugging Face Blog | Deep dive into research details behind the merging methods of mergoo library |
LLaMa3-based Experts | Build your own MoE-style LLM experts by integrating LLaMa3-based domain experts |
Phi3-based Experts | Create MoE-style LLM architecture by merging Phi3-based fine-tuned models |
As an open-source library in a fast evolving domain, we welcome contributions, whether it is introducing new features, enhancing infrastructure, or improving documentation.
Here is mergoo
roadmap:
- [X] Support MoE for Transformer Block
- [X] Compatibility with Huggingface 🤗
- [X] Support Trainer, SFTrainer
- [X] Loading Unified Checkpoint in BTX
- [X] Feature: Convertible QKV linear layers
- [X] Feature: Convertible FF linear layers
- [X] Feature: Routers only for a list of decoder layers indexes
- [X] Sharded Safetensor Saving
- [X] Support experts based on LLaMa and Mistral
- [X] Support experts based on Phi3
- [X] Support Mixture of LORA Experts (Mixture of Adapters)
- [ ] Router Load balancing loss
- [ ] Lazy loading of tensors for low memory usage in Merging
- [ ] Support other Layer-wise merging methods, including Mergekit
- [ ] Support experts based on Gemma and Mamba
- [ ] Support flash-attention
- [ ] Support Mixture of Depths Transformer
Feel free to suggest new features and/or contribute to mergoo
roadmap!
🚀 We love to here your feedback, please join Leeroo community:
Have a question not listed here? Open a GitHub Issue or send us an email!
For Tasks:
Click tags to check more tools for each tasksFor Jobs:
Alternative AI tools for mergoo
Similar Open Source Tools
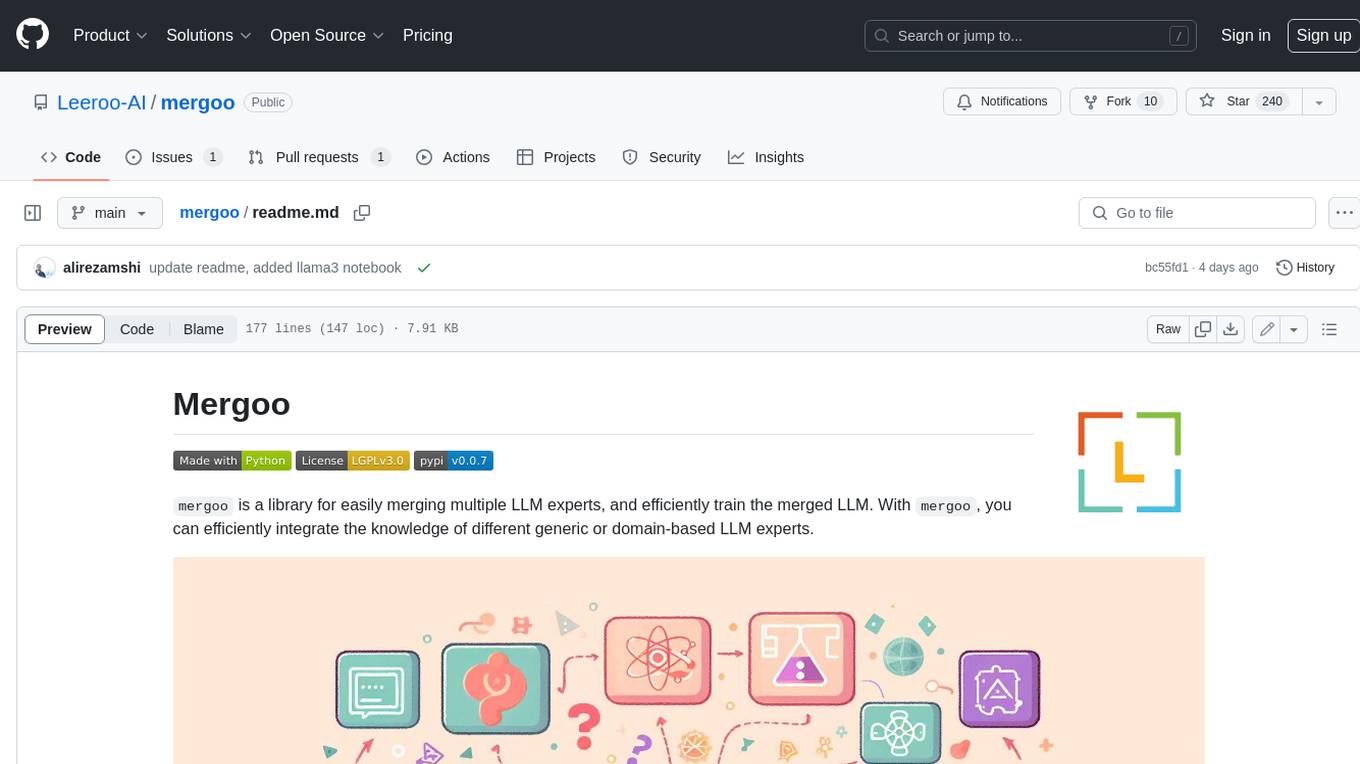
mergoo
Mergoo is a library for easily merging multiple LLM experts and efficiently training the merged LLM. With Mergoo, you can efficiently integrate the knowledge of different generic or domain-based LLM experts. Mergoo supports several merging methods, including Mixture-of-Experts, Mixture-of-Adapters, and Layer-wise merging. It also supports various base models, including LLaMa, Mistral, and BERT, and trainers, including Hugging Face Trainer, SFTrainer, and PEFT. Mergoo provides flexible merging for each layer and supports training choices such as only routing MoE layers or fully fine-tuning the merged LLM.
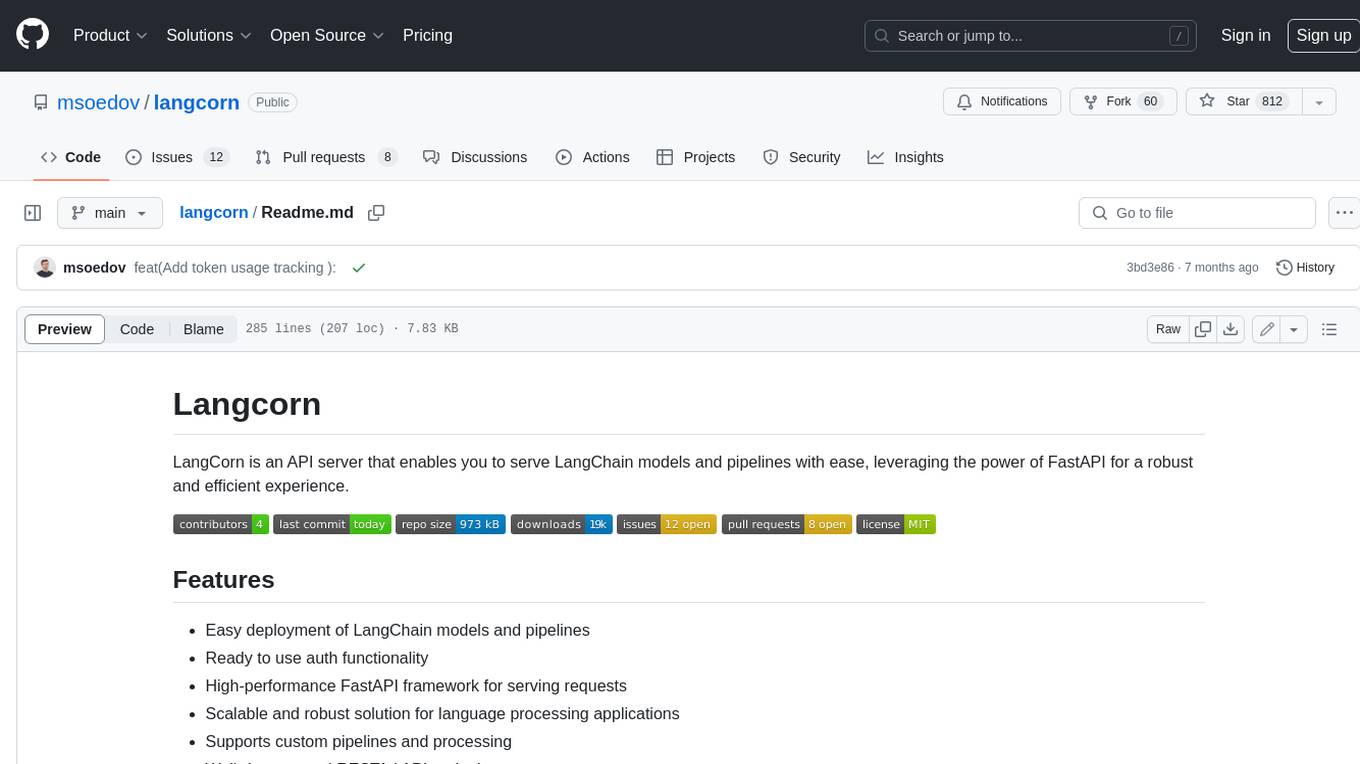
langcorn
LangCorn is an API server that enables you to serve LangChain models and pipelines with ease, leveraging the power of FastAPI for a robust and efficient experience. It offers features such as easy deployment of LangChain models and pipelines, ready-to-use authentication functionality, high-performance FastAPI framework for serving requests, scalability and robustness for language processing applications, support for custom pipelines and processing, well-documented RESTful API endpoints, and asynchronous processing for faster response times.
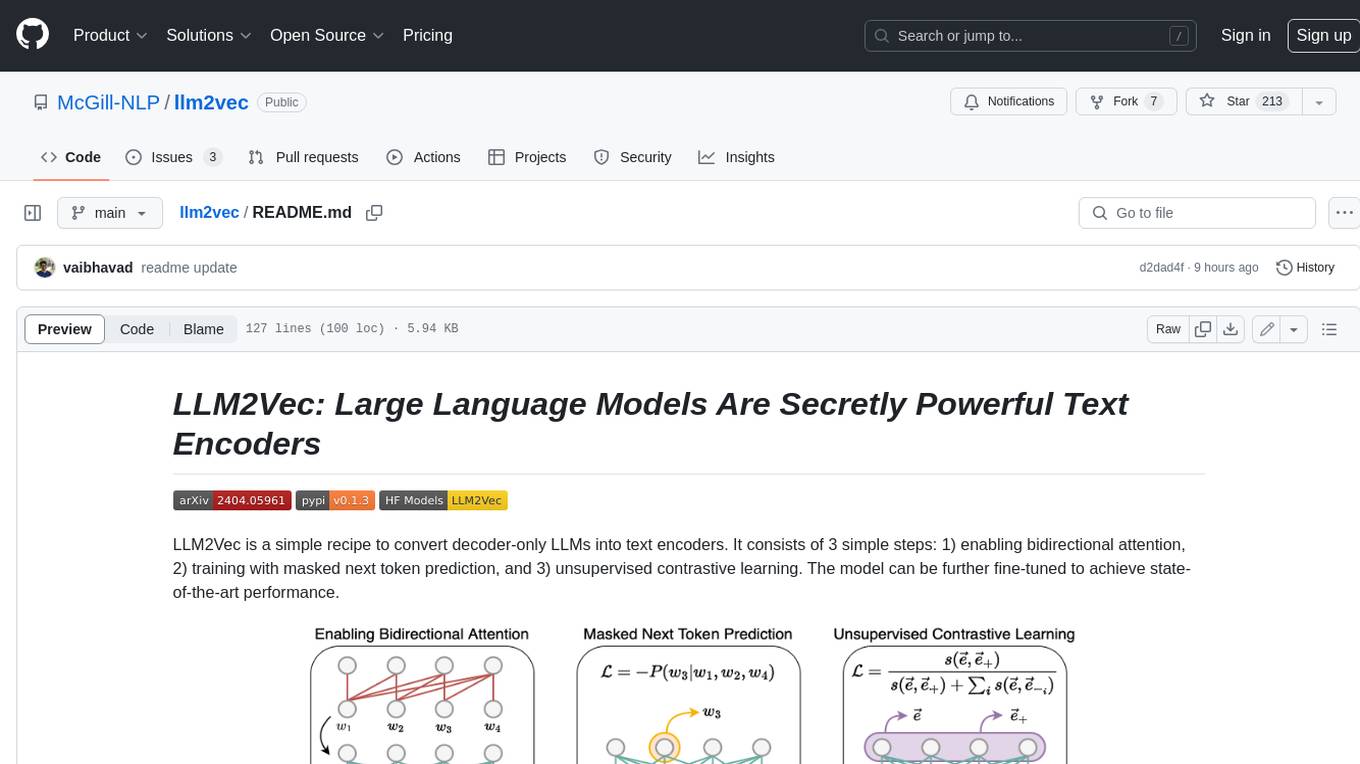
llm2vec
LLM2Vec is a simple recipe to convert decoder-only LLMs into text encoders. It consists of 3 simple steps: 1) enabling bidirectional attention, 2) training with masked next token prediction, and 3) unsupervised contrastive learning. The model can be further fine-tuned to achieve state-of-the-art performance.
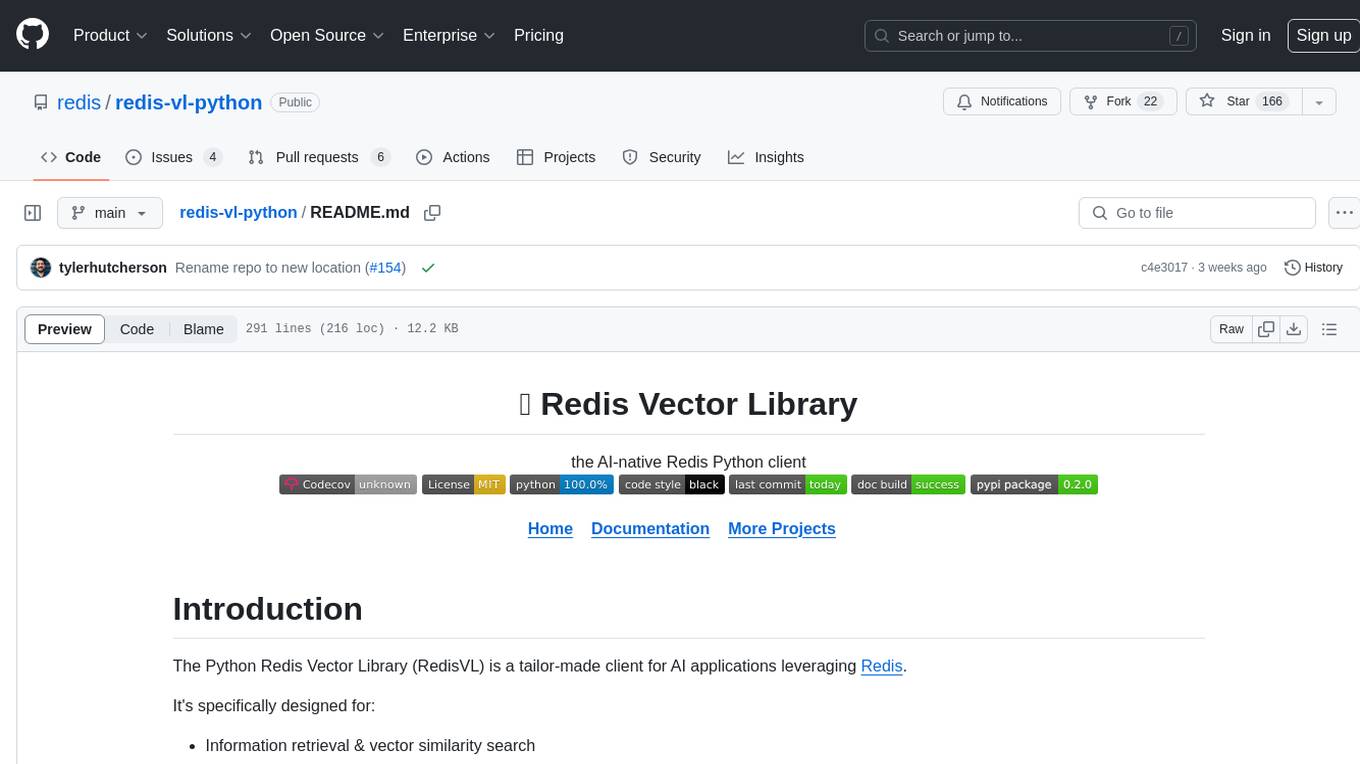
redis-vl-python
The Python Redis Vector Library (RedisVL) is a tailor-made client for AI applications leveraging Redis. It enhances applications with Redis' speed, flexibility, and reliability, incorporating capabilities like vector-based semantic search, full-text search, and geo-spatial search. The library bridges the gap between the emerging AI-native developer ecosystem and the capabilities of Redis by providing a lightweight, elegant, and intuitive interface. It abstracts the features of Redis into a grammar that is more aligned to the needs of today's AI/ML Engineers or Data Scientists.
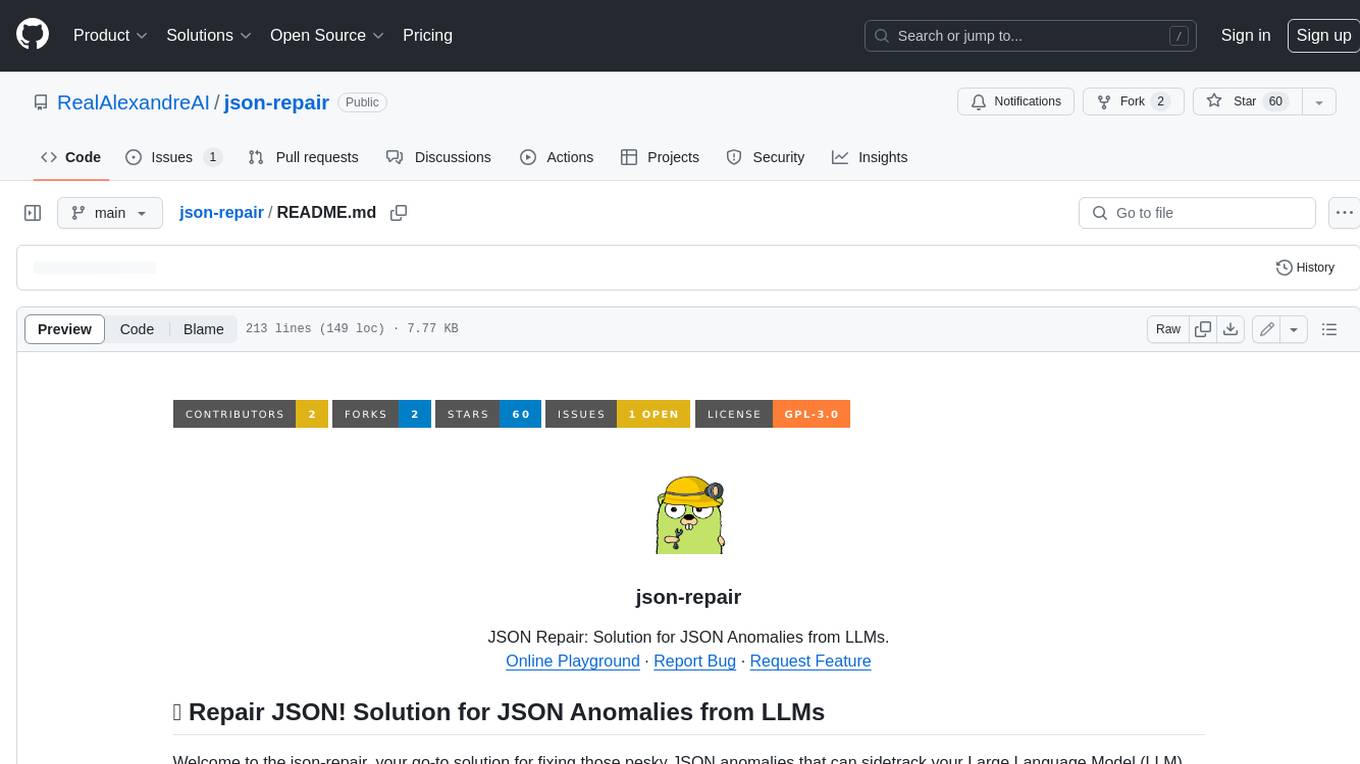
json-repair
JSON Repair is a toolkit designed to address JSON anomalies that can arise from Large Language Models (LLMs). It offers a comprehensive solution for repairing JSON strings, ensuring accuracy and reliability in your data processing. With its user-friendly interface and extensive capabilities, JSON Repair empowers developers to seamlessly integrate JSON repair into their workflows.
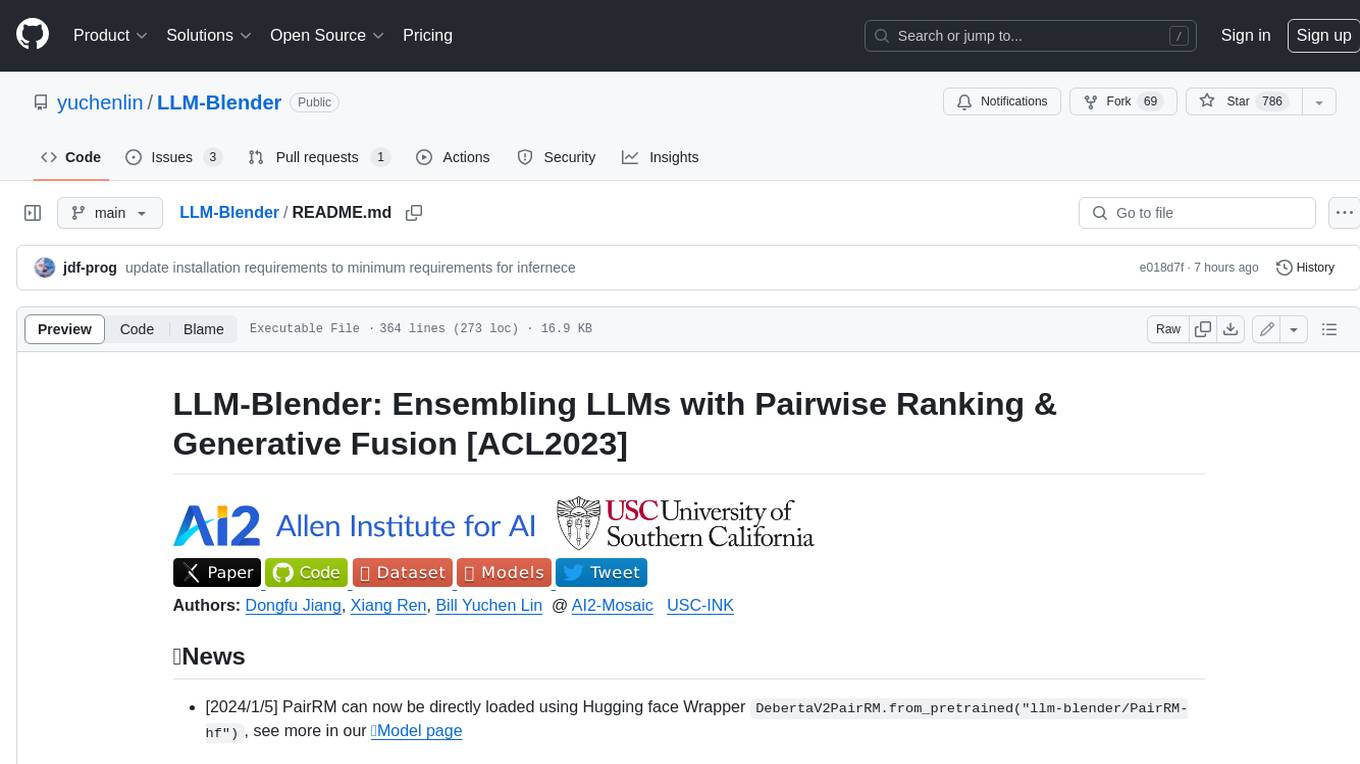
LLM-Blender
LLM-Blender is a framework for ensembling large language models (LLMs) to achieve superior performance. It consists of two modules: PairRanker and GenFuser. PairRanker uses pairwise comparisons to distinguish between candidate outputs, while GenFuser merges the top-ranked candidates to create an improved output. LLM-Blender has been shown to significantly surpass the best LLMs and baseline ensembling methods across various metrics on the MixInstruct benchmark dataset.
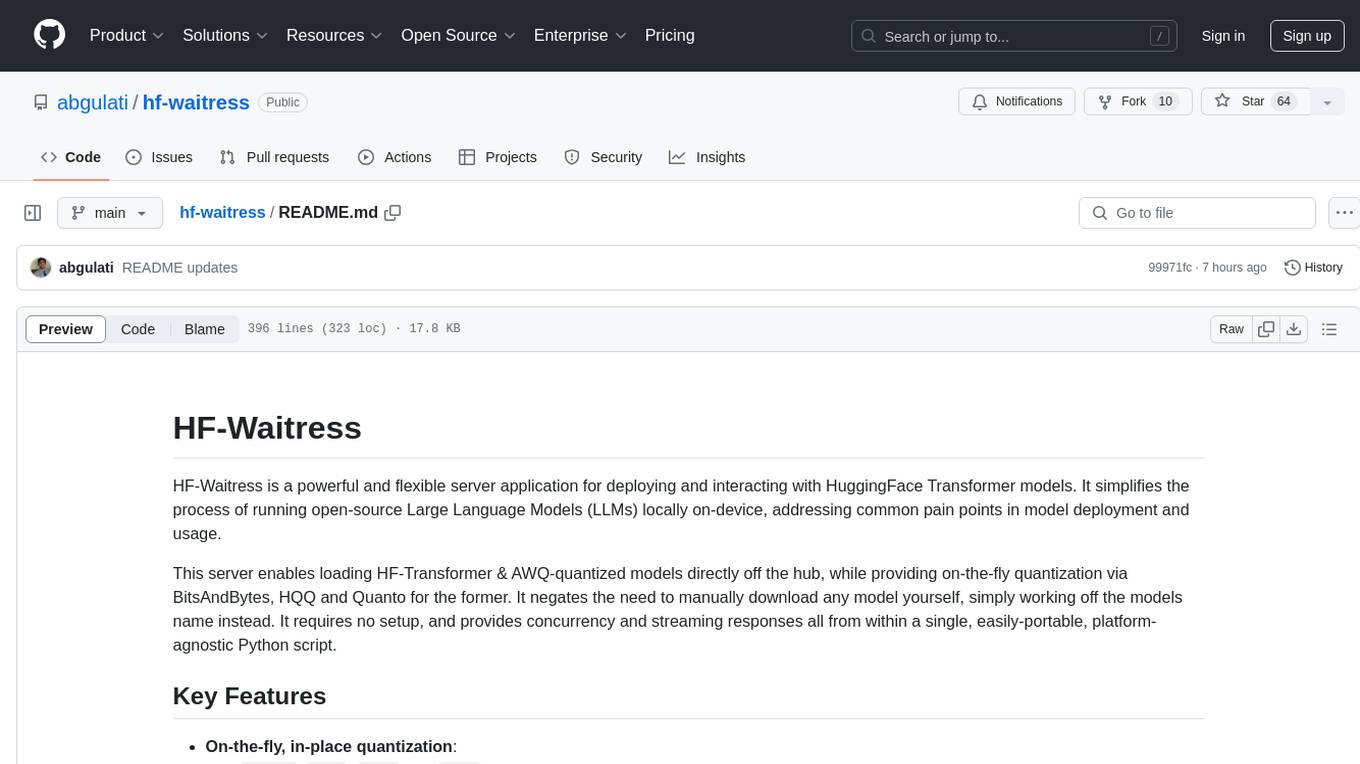
hf-waitress
HF-Waitress is a powerful server application for deploying and interacting with HuggingFace Transformer models. It simplifies running open-source Large Language Models (LLMs) locally on-device, providing on-the-fly quantization via BitsAndBytes, HQQ, and Quanto. It requires no manual model downloads, offers concurrency, streaming responses, and supports various hardware and platforms. The server uses a `config.json` file for easy configuration management and provides detailed error handling and logging.
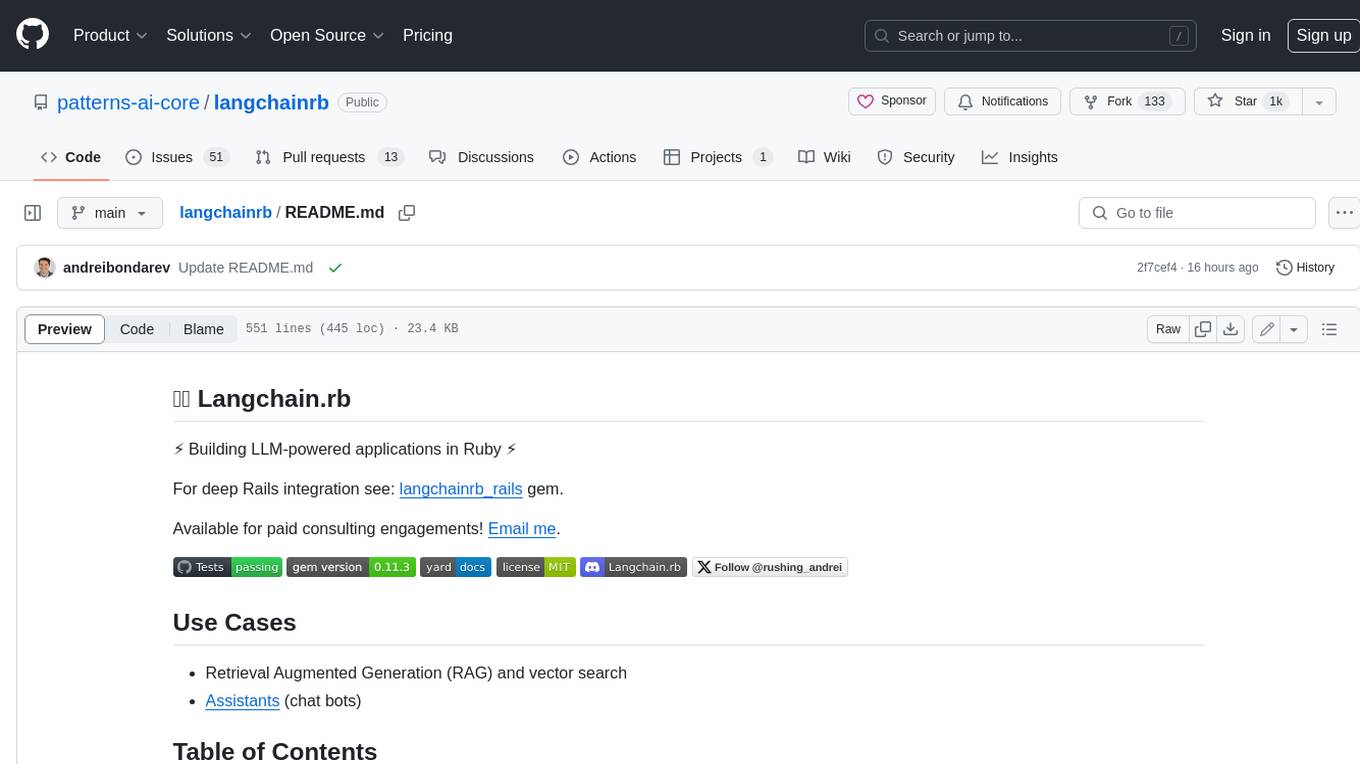
langchainrb
Langchain.rb is a Ruby library that makes it easy to build LLM-powered applications. It provides a unified interface to a variety of LLMs, vector search databases, and other tools, making it easy to build and deploy RAG (Retrieval Augmented Generation) systems and assistants. Langchain.rb is open source and available under the MIT License.
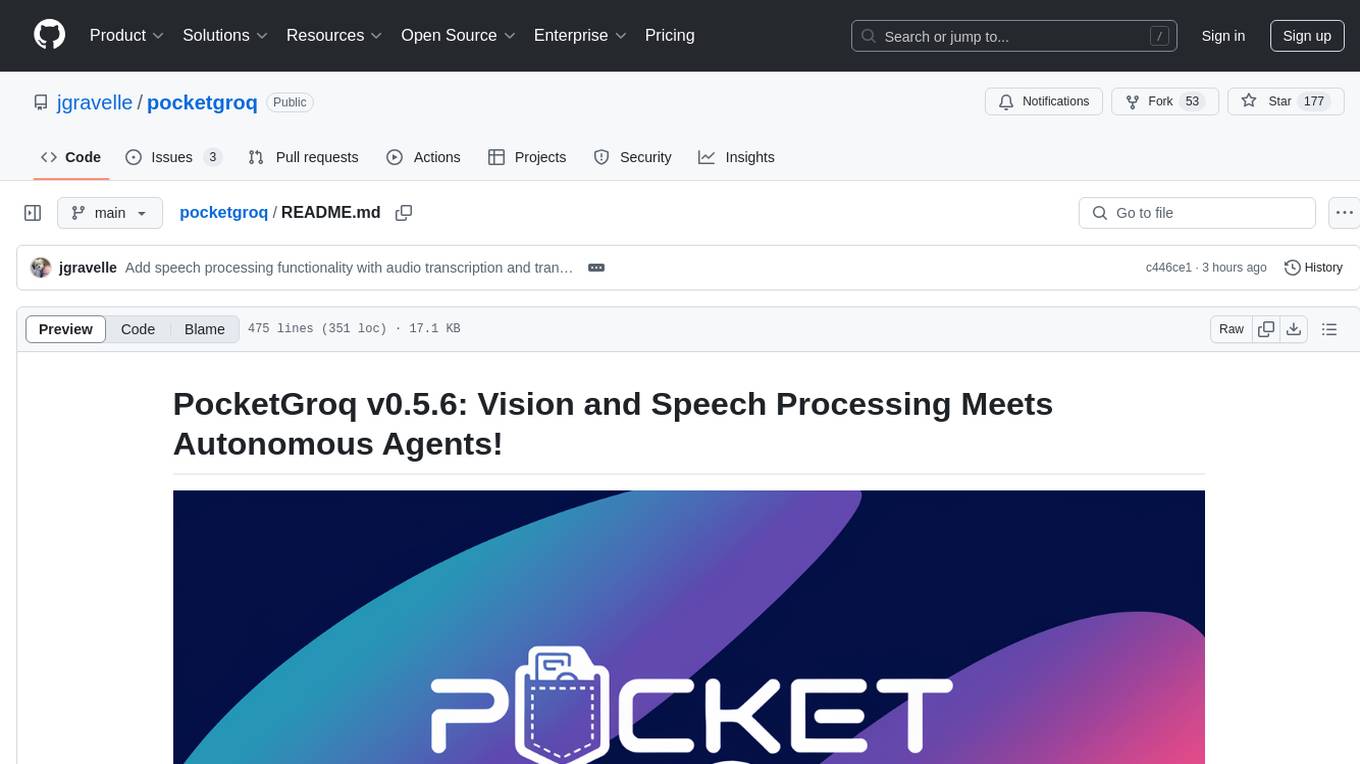
pocketgroq
PocketGroq is a tool that provides advanced functionalities for text generation, web scraping, web search, and AI response evaluation. It includes features like an Autonomous Agent for answering questions, web crawling and scraping capabilities, enhanced web search functionality, and flexible integration with Ollama server. Users can customize the agent's behavior, evaluate responses using AI, and utilize various methods for text generation, conversation management, and Chain of Thought reasoning. The tool offers comprehensive methods for different tasks, such as initializing RAG, error handling, and tool management. PocketGroq is designed to enhance development processes and enable the creation of AI-powered applications with ease.
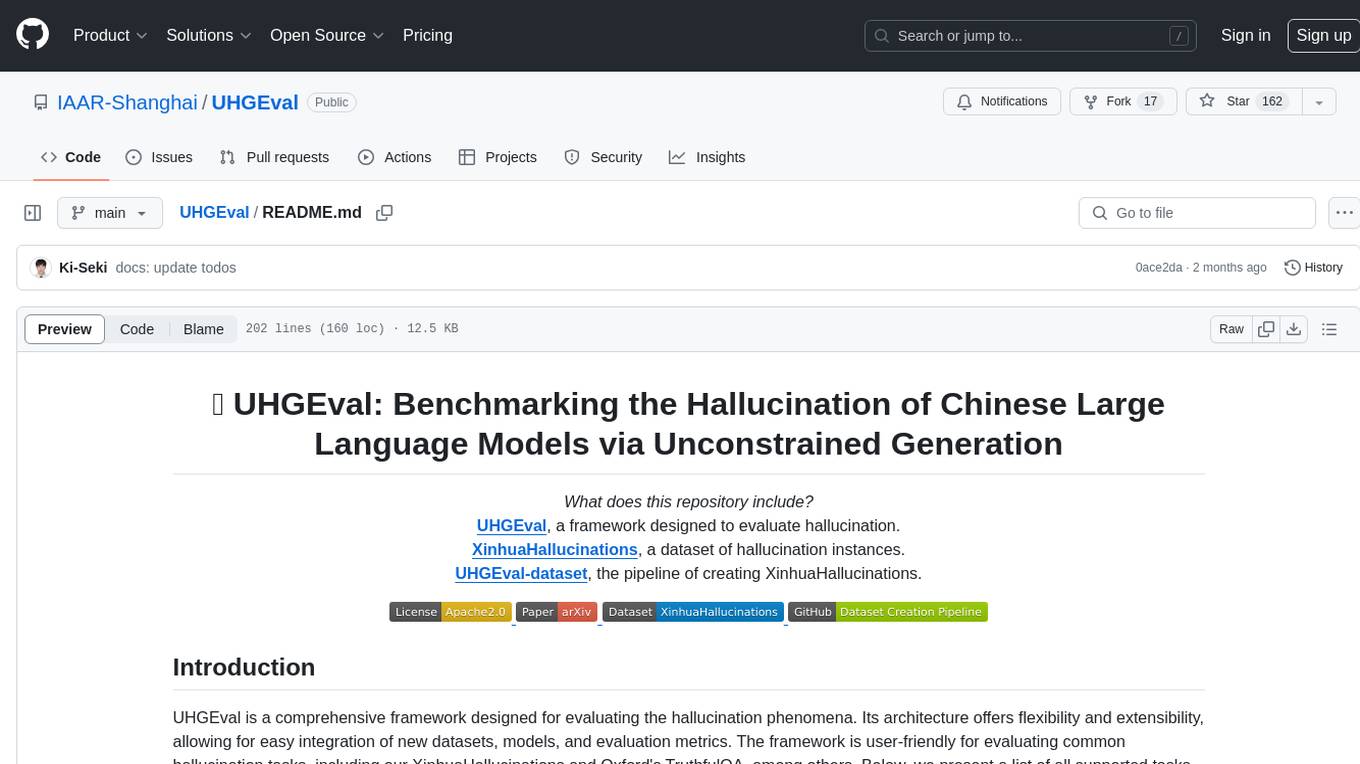
UHGEval
UHGEval is a comprehensive framework designed for evaluating the hallucination phenomena. It includes UHGEval, a framework for evaluating hallucination, XinhuaHallucinations dataset, and UHGEval-dataset pipeline for creating XinhuaHallucinations. The framework offers flexibility and extensibility for evaluating common hallucination tasks, supporting various models and datasets. Researchers can use the open-source pipeline to create customized datasets. Supported tasks include QA, dialogue, summarization, and multi-choice tasks.
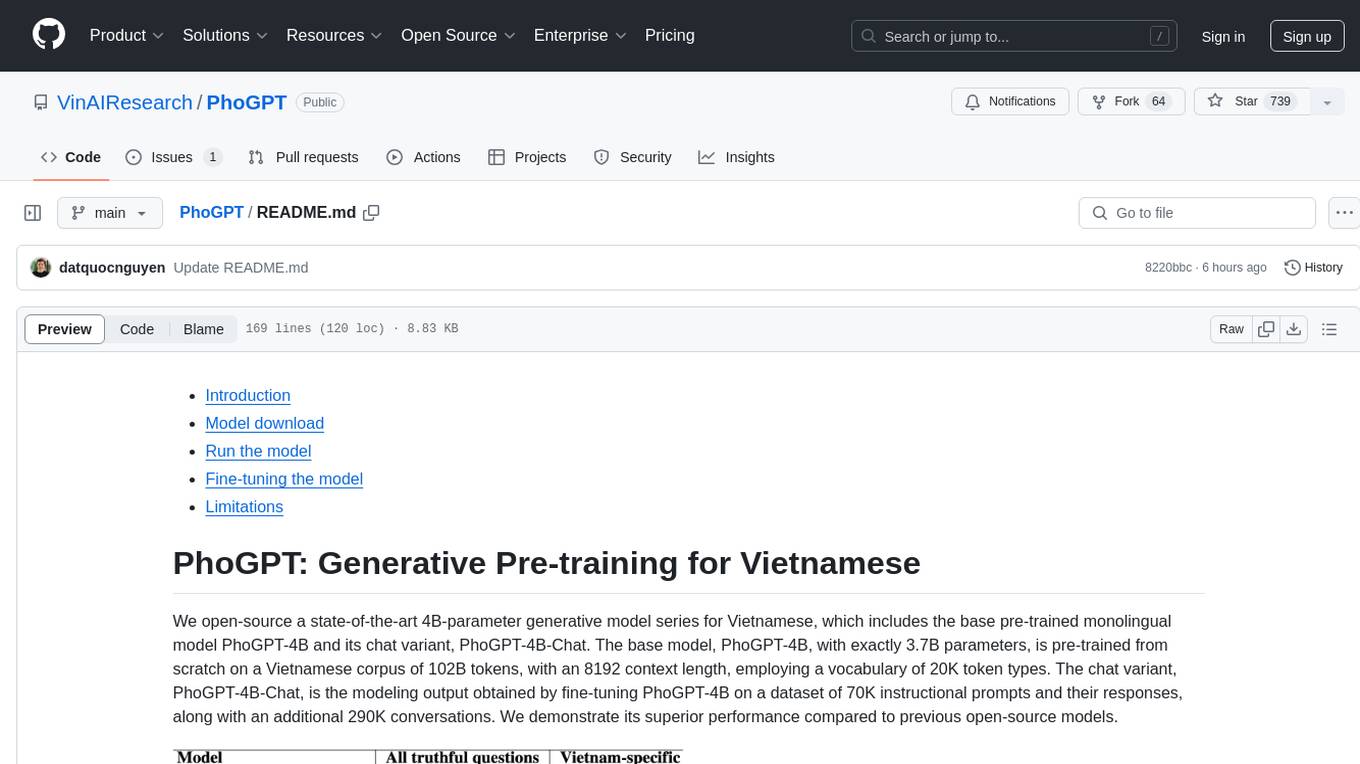
PhoGPT
PhoGPT is an open-source 4B-parameter generative model series for Vietnamese, including the base pre-trained monolingual model PhoGPT-4B and its chat variant, PhoGPT-4B-Chat. PhoGPT-4B is pre-trained from scratch on a Vietnamese corpus of 102B tokens, with an 8192 context length and a vocabulary of 20K token types. PhoGPT-4B-Chat is fine-tuned on instructional prompts and conversations, demonstrating superior performance. Users can run the model with inference engines like vLLM and Text Generation Inference, and fine-tune it using llm-foundry. However, PhoGPT has limitations in reasoning, coding, and mathematics tasks, and may generate harmful or biased responses.
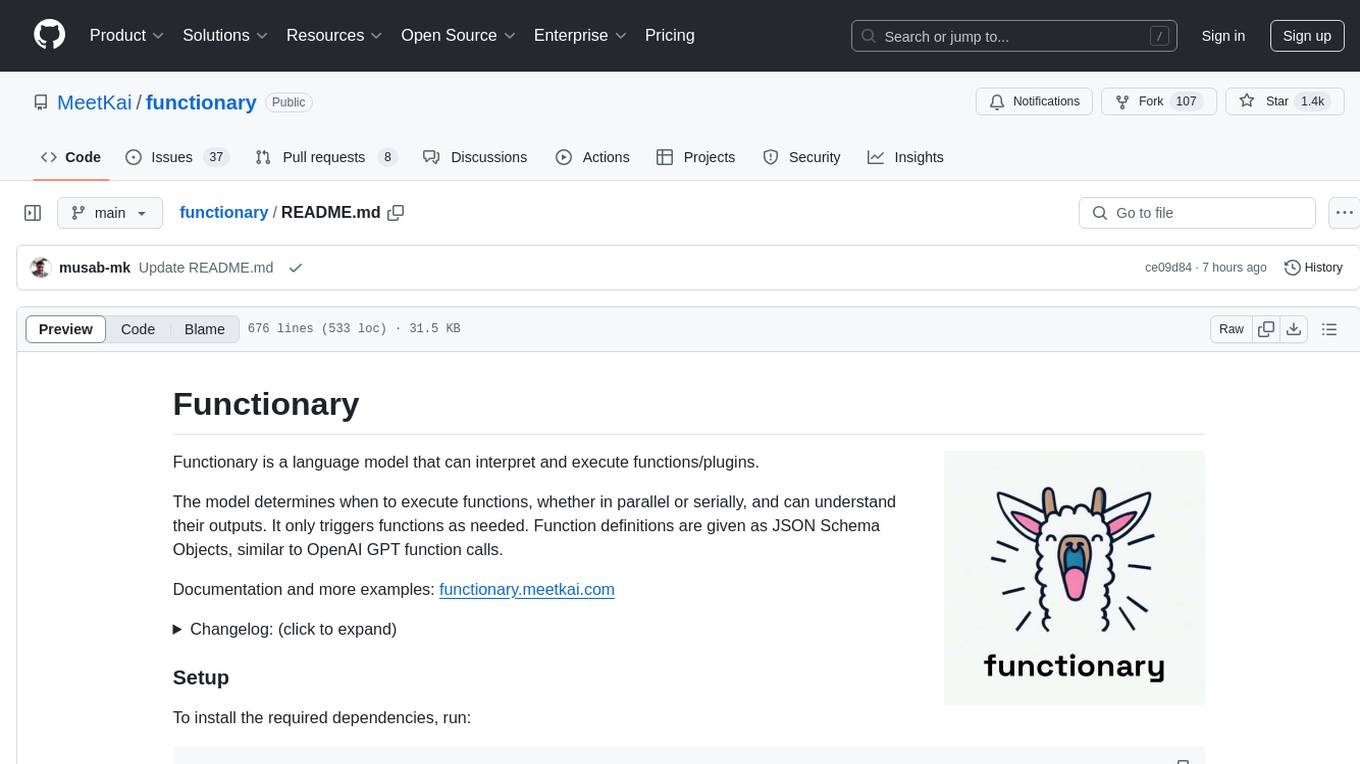
functionary
Functionary is a language model that interprets and executes functions/plugins. It determines when to execute functions, whether in parallel or serially, and understands their outputs. Function definitions are given as JSON Schema Objects, similar to OpenAI GPT function calls. It offers documentation and examples on functionary.meetkai.com. The newest model, meetkai/functionary-medium-v3.1, is ranked 2nd in the Berkeley Function-Calling Leaderboard. Functionary supports models with different context lengths and capabilities for function calling and code interpretation. It also provides grammar sampling for accurate function and parameter names. Users can deploy Functionary models serverlessly using Modal.com.
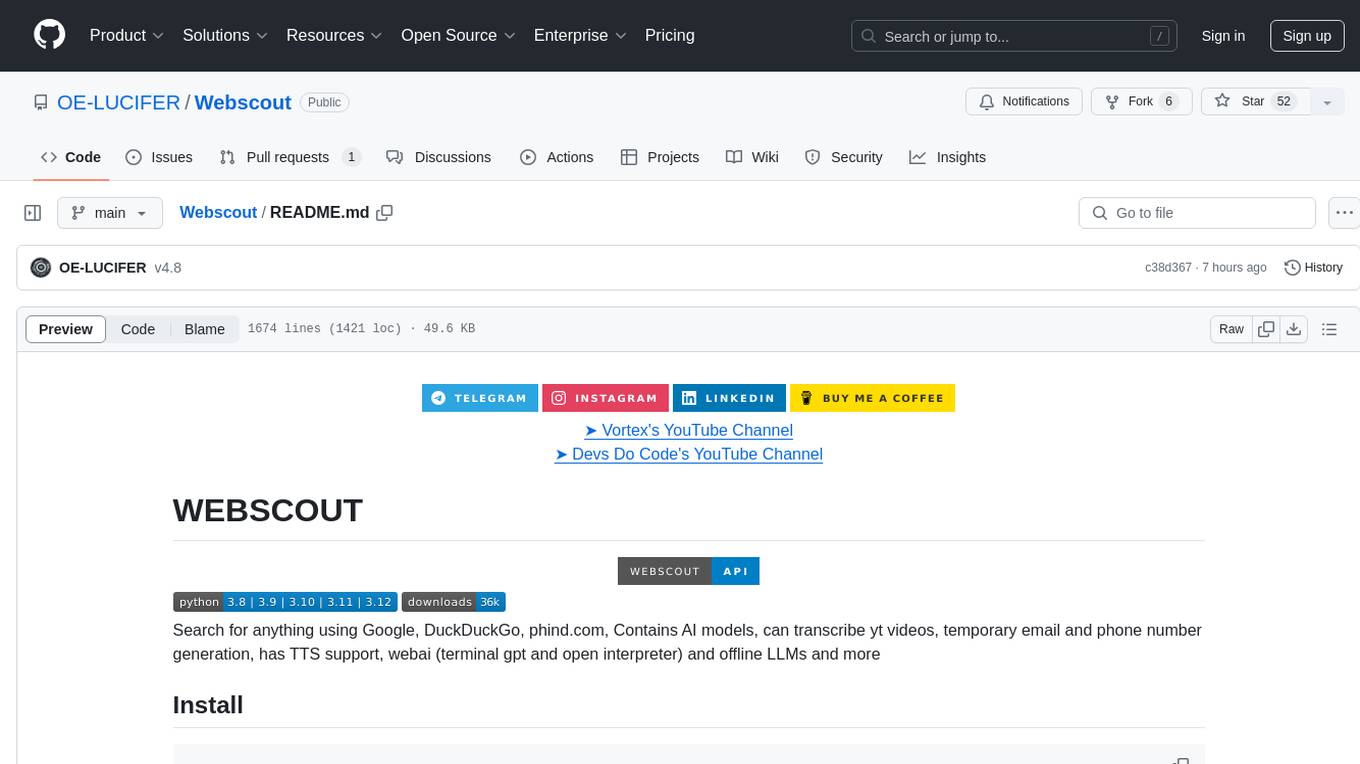
Webscout
WebScout is a versatile tool that allows users to search for anything using Google, DuckDuckGo, and phind.com. It contains AI models, can transcribe YouTube videos, generate temporary email and phone numbers, has TTS support, webai (terminal GPT and open interpreter), and offline LLMs. It also supports features like weather forecasting, YT video downloading, temp mail and number generation, text-to-speech, advanced web searches, and more.
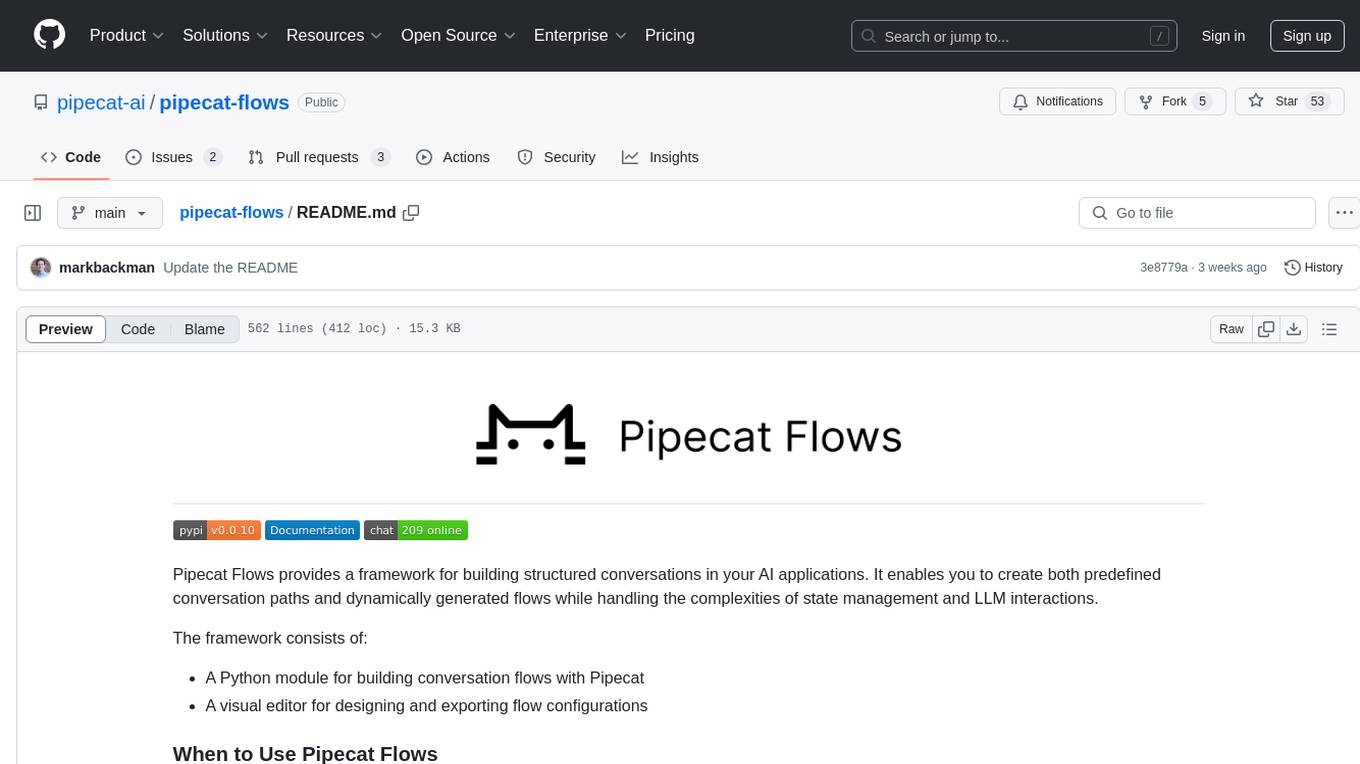
pipecat-flows
Pipecat Flows is a framework designed for building structured conversations in AI applications. It allows users to create both predefined conversation paths and dynamically generated flows, handling state management and LLM interactions. The framework includes a Python module for building conversation flows and a visual editor for designing and exporting flow configurations. Pipecat Flows is suitable for scenarios such as customer service scripts, intake forms, personalized experiences, and complex decision trees.
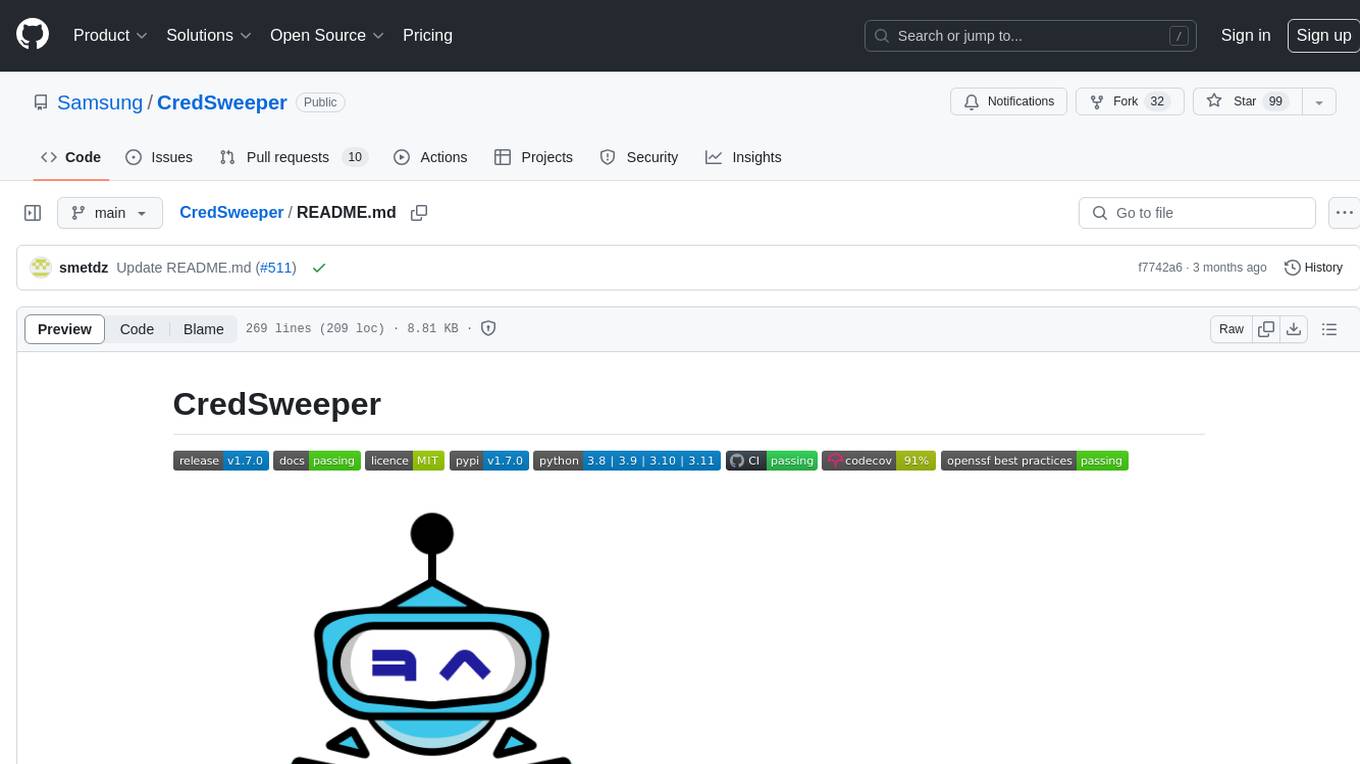
CredSweeper
CredSweeper is a tool designed to detect credentials like tokens, passwords, and API keys in directories or files. It helps users identify potential exposure of sensitive information by scanning lines, filtering, and utilizing an AI model. The tool reports lines containing possible credentials, their location, and the expected type of credential.
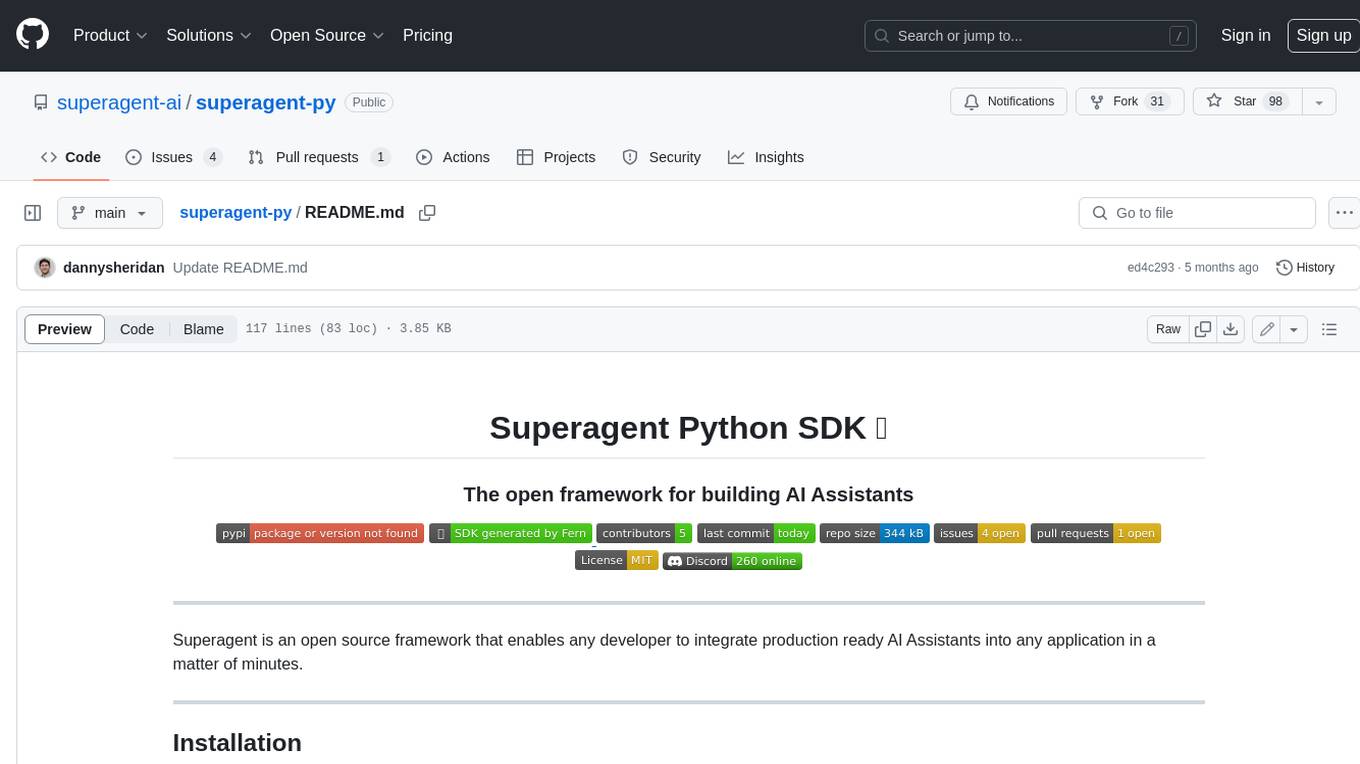
superagent-py
Superagent is an open-source framework that enables developers to integrate production-ready AI assistants into any application quickly and easily. It provides a Python SDK for interacting with the Superagent API, allowing developers to create, manage, and invoke AI agents. The SDK simplifies the process of building AI-powered applications, making it accessible to developers of all skill levels.
For similar tasks
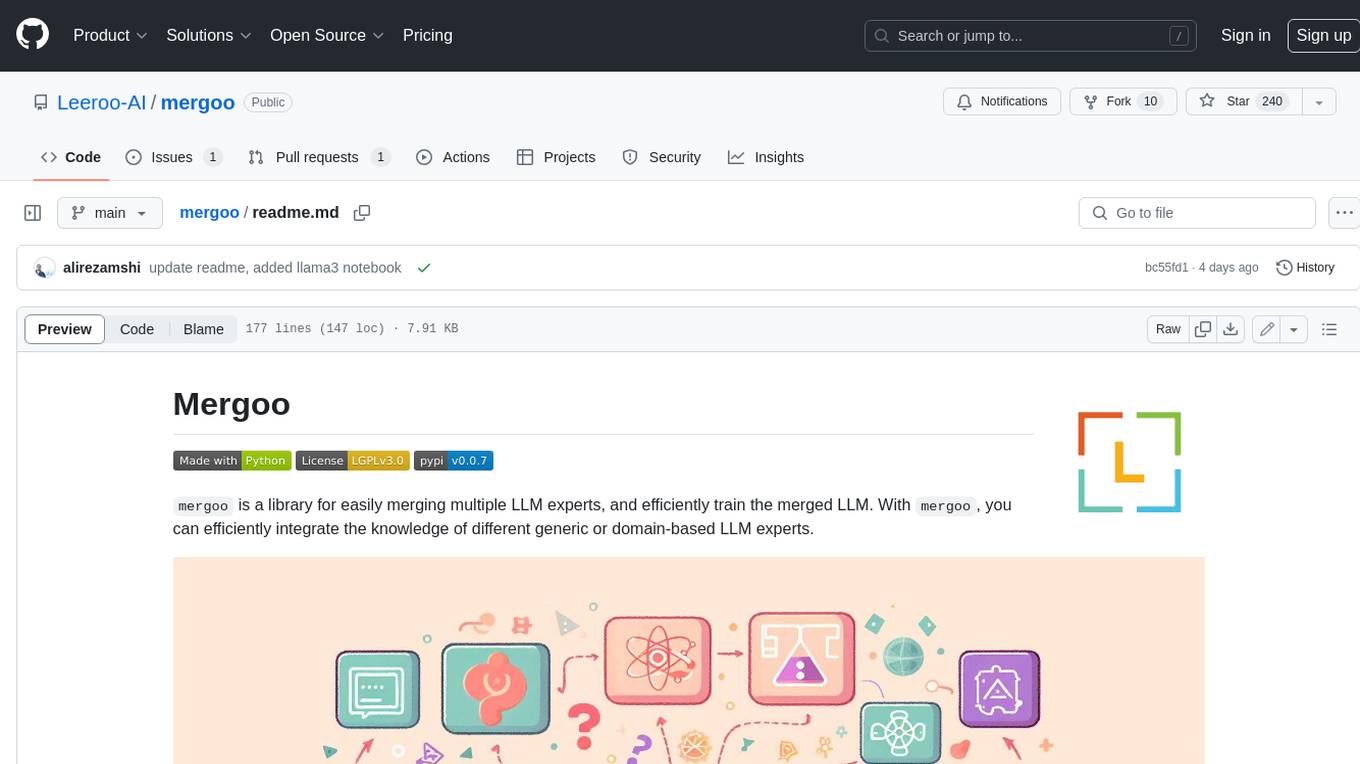
mergoo
Mergoo is a library for easily merging multiple LLM experts and efficiently training the merged LLM. With Mergoo, you can efficiently integrate the knowledge of different generic or domain-based LLM experts. Mergoo supports several merging methods, including Mixture-of-Experts, Mixture-of-Adapters, and Layer-wise merging. It also supports various base models, including LLaMa, Mistral, and BERT, and trainers, including Hugging Face Trainer, SFTrainer, and PEFT. Mergoo provides flexible merging for each layer and supports training choices such as only routing MoE layers or fully fine-tuning the merged LLM.
For similar jobs
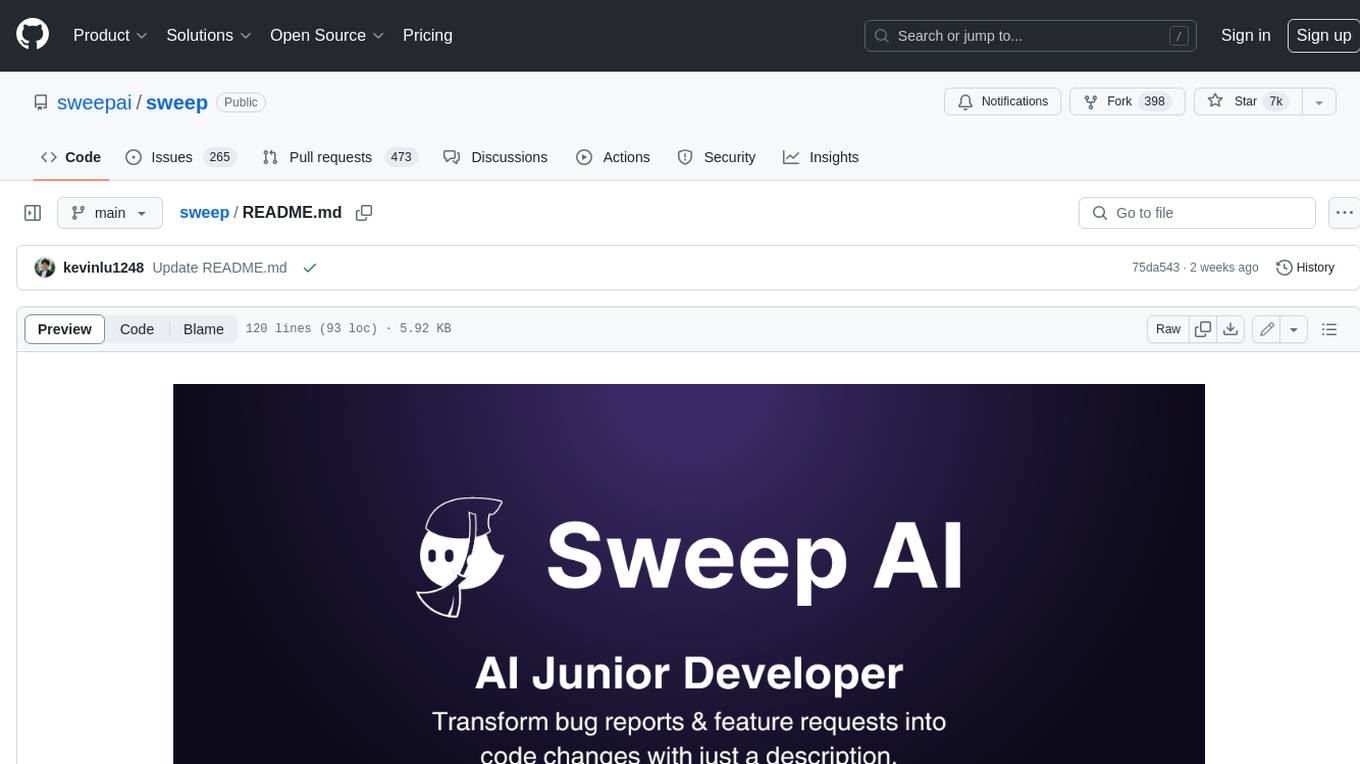
sweep
Sweep is an AI junior developer that turns bugs and feature requests into code changes. It automatically handles developer experience improvements like adding type hints and improving test coverage.
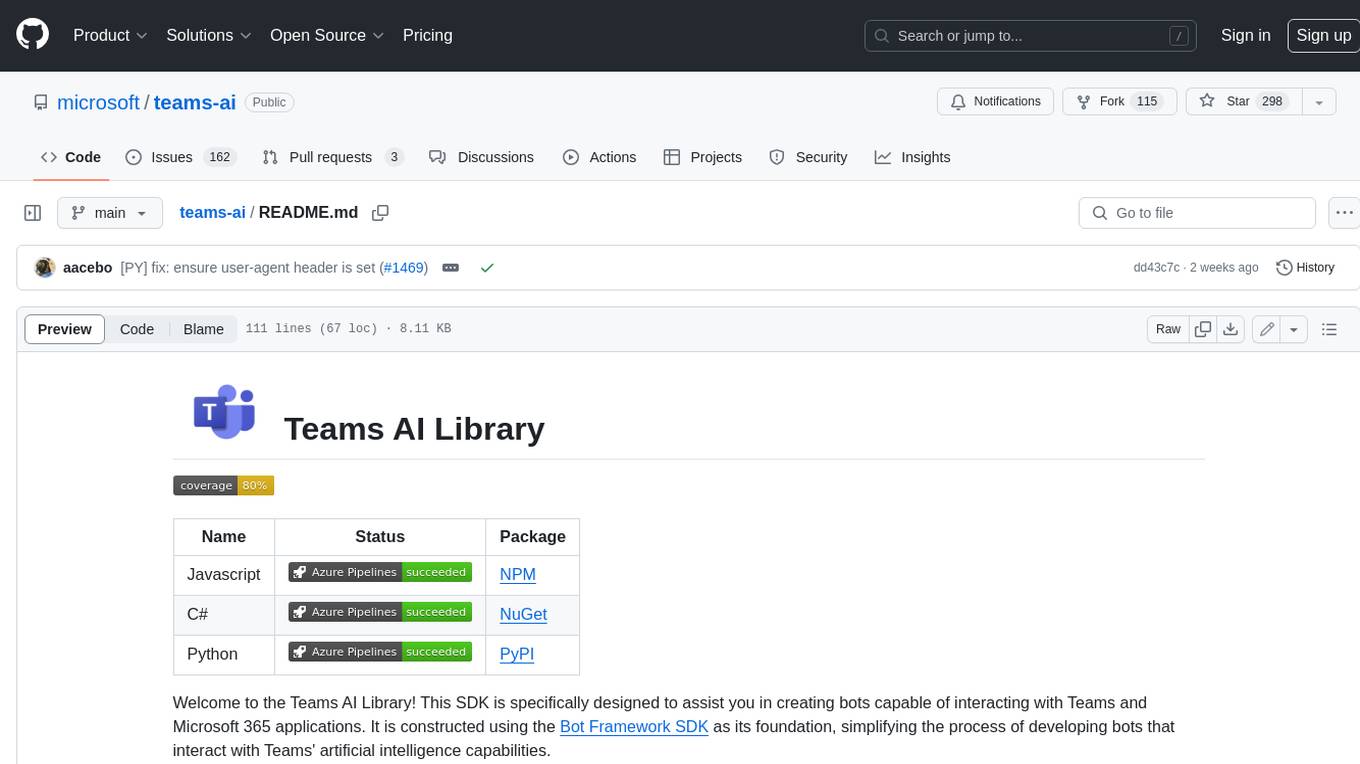
teams-ai
The Teams AI Library is a software development kit (SDK) that helps developers create bots that can interact with Teams and Microsoft 365 applications. It is built on top of the Bot Framework SDK and simplifies the process of developing bots that interact with Teams' artificial intelligence capabilities. The SDK is available for JavaScript/TypeScript, .NET, and Python.
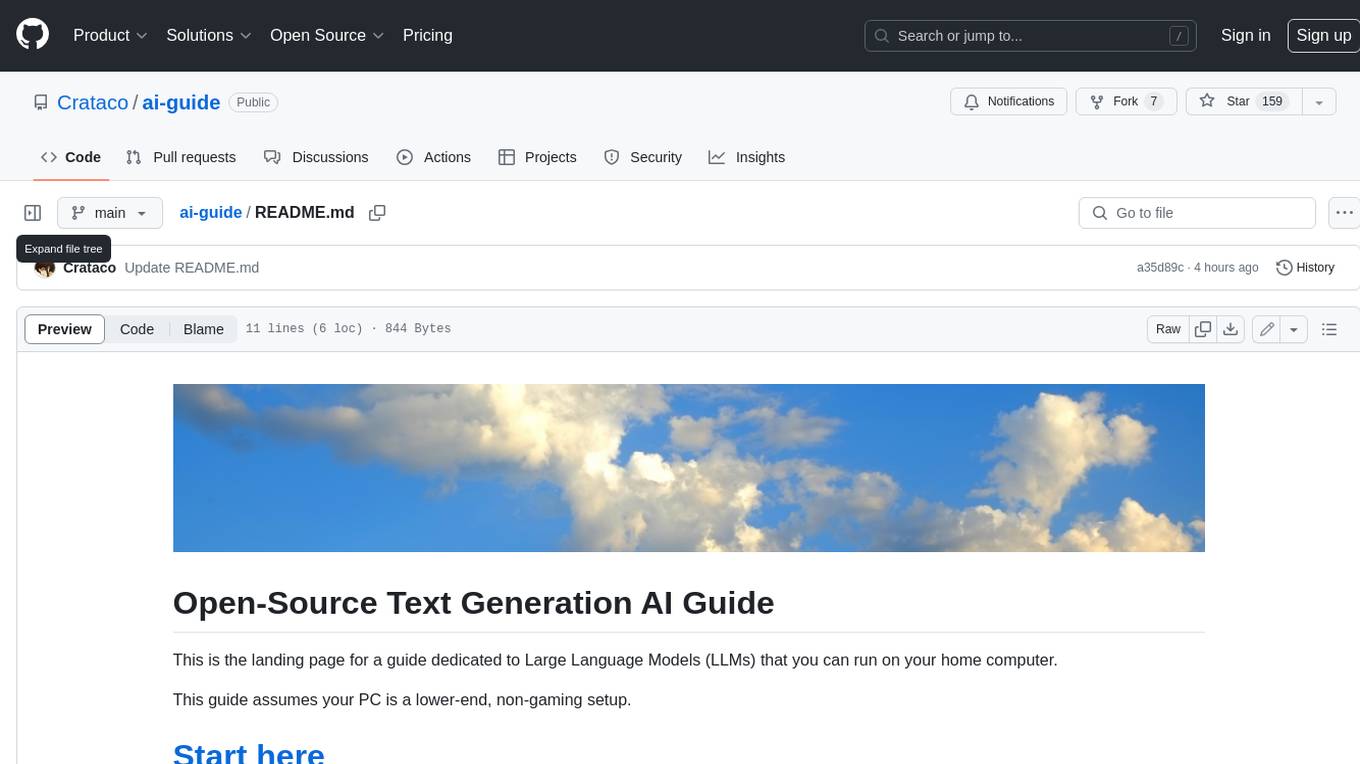
ai-guide
This guide is dedicated to Large Language Models (LLMs) that you can run on your home computer. It assumes your PC is a lower-end, non-gaming setup.
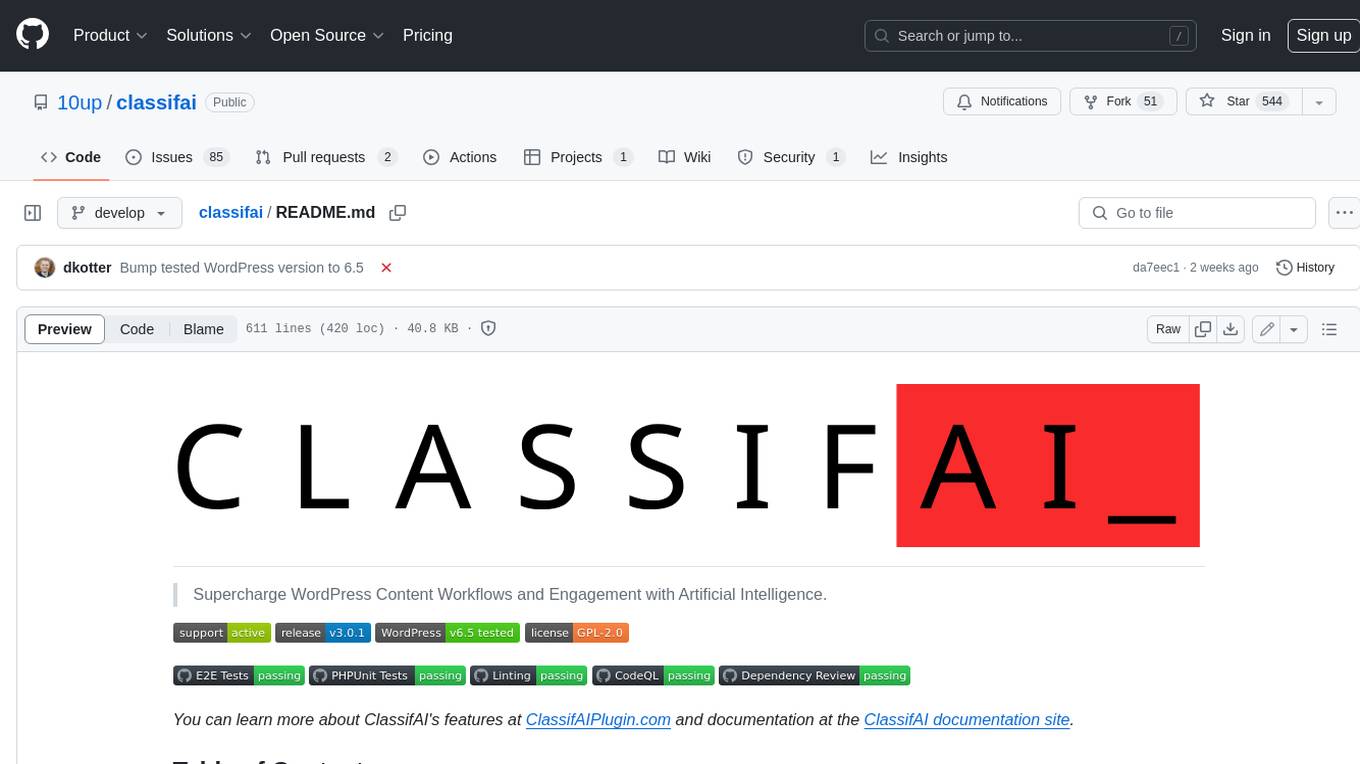
classifai
Supercharge WordPress Content Workflows and Engagement with Artificial Intelligence. Tap into leading cloud-based services like OpenAI, Microsoft Azure AI, Google Gemini and IBM Watson to augment your WordPress-powered websites. Publish content faster while improving SEO performance and increasing audience engagement. ClassifAI integrates Artificial Intelligence and Machine Learning technologies to lighten your workload and eliminate tedious tasks, giving you more time to create original content that matters.
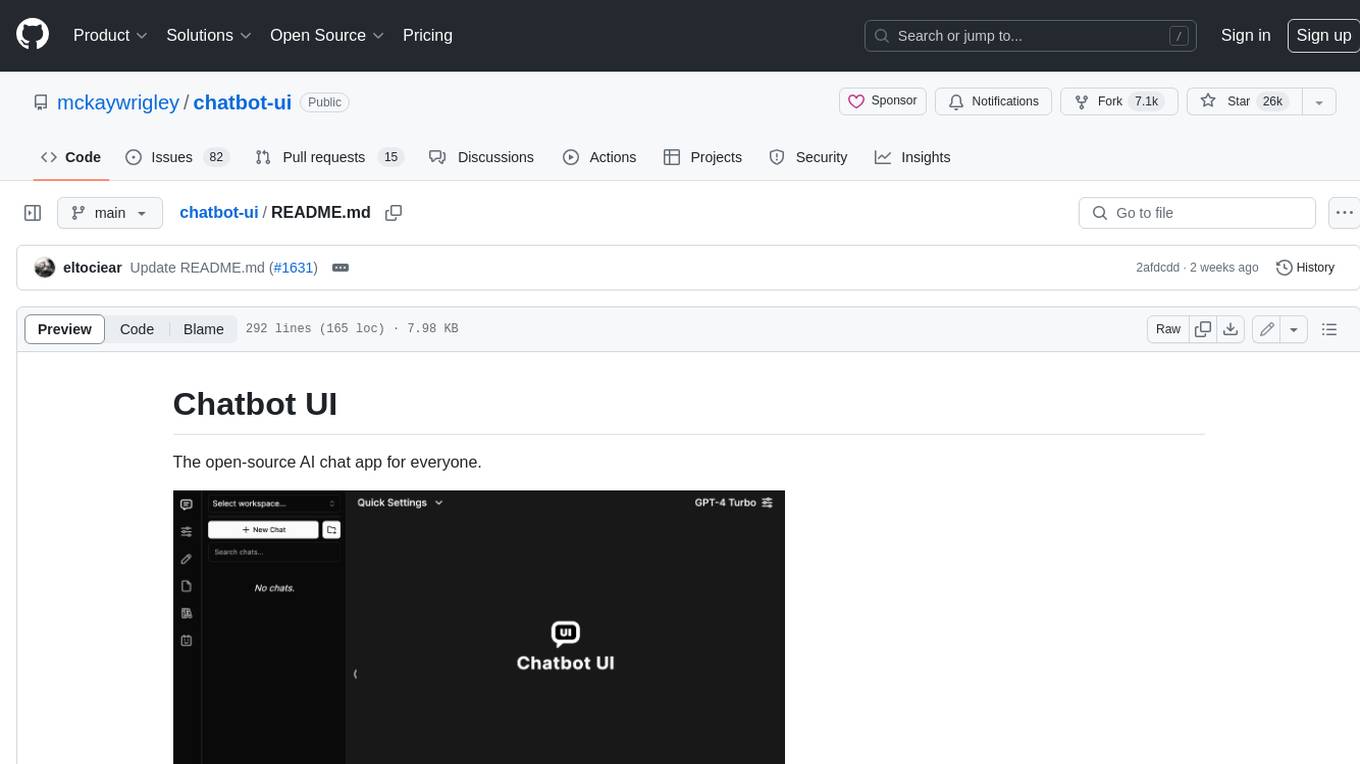
chatbot-ui
Chatbot UI is an open-source AI chat app that allows users to create and deploy their own AI chatbots. It is easy to use and can be customized to fit any need. Chatbot UI is perfect for businesses, developers, and anyone who wants to create a chatbot.
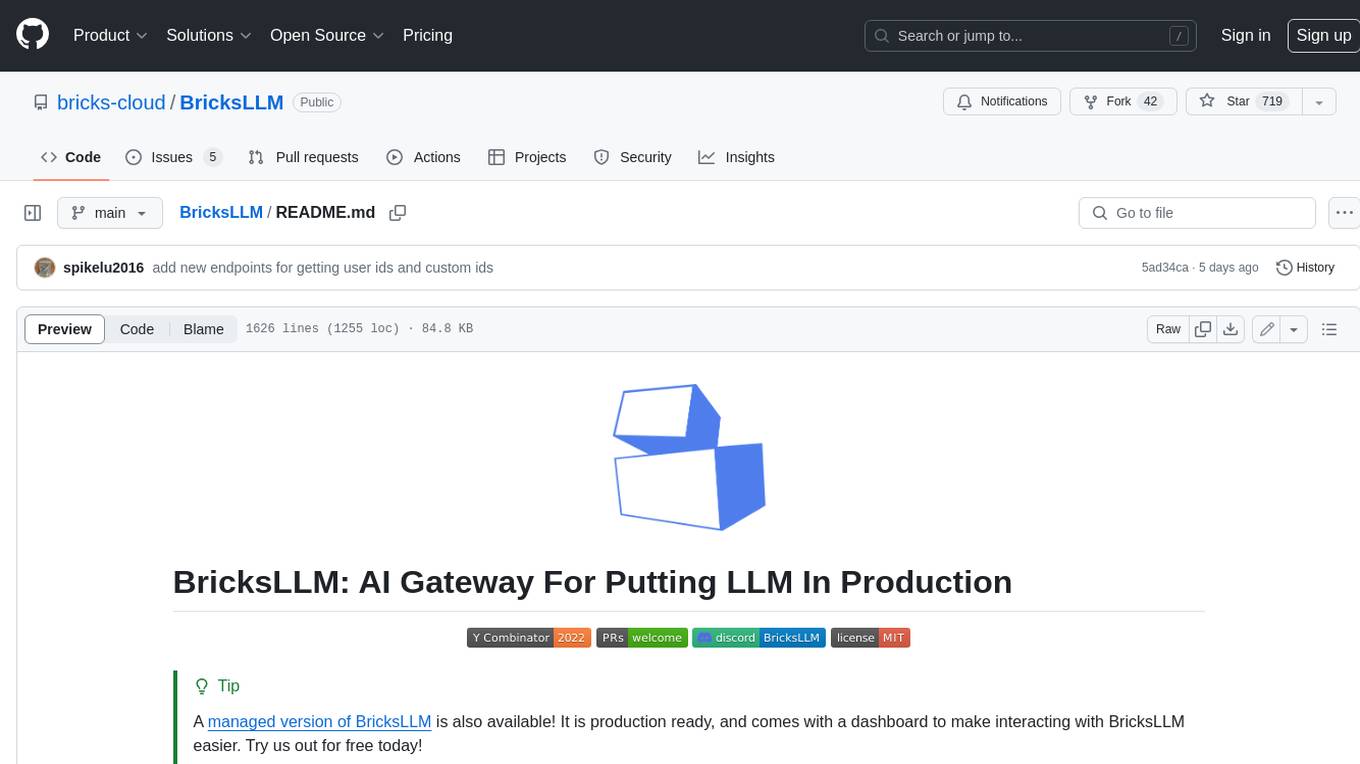
BricksLLM
BricksLLM is a cloud native AI gateway written in Go. Currently, it provides native support for OpenAI, Anthropic, Azure OpenAI and vLLM. BricksLLM aims to provide enterprise level infrastructure that can power any LLM production use cases. Here are some use cases for BricksLLM: * Set LLM usage limits for users on different pricing tiers * Track LLM usage on a per user and per organization basis * Block or redact requests containing PIIs * Improve LLM reliability with failovers, retries and caching * Distribute API keys with rate limits and cost limits for internal development/production use cases * Distribute API keys with rate limits and cost limits for students
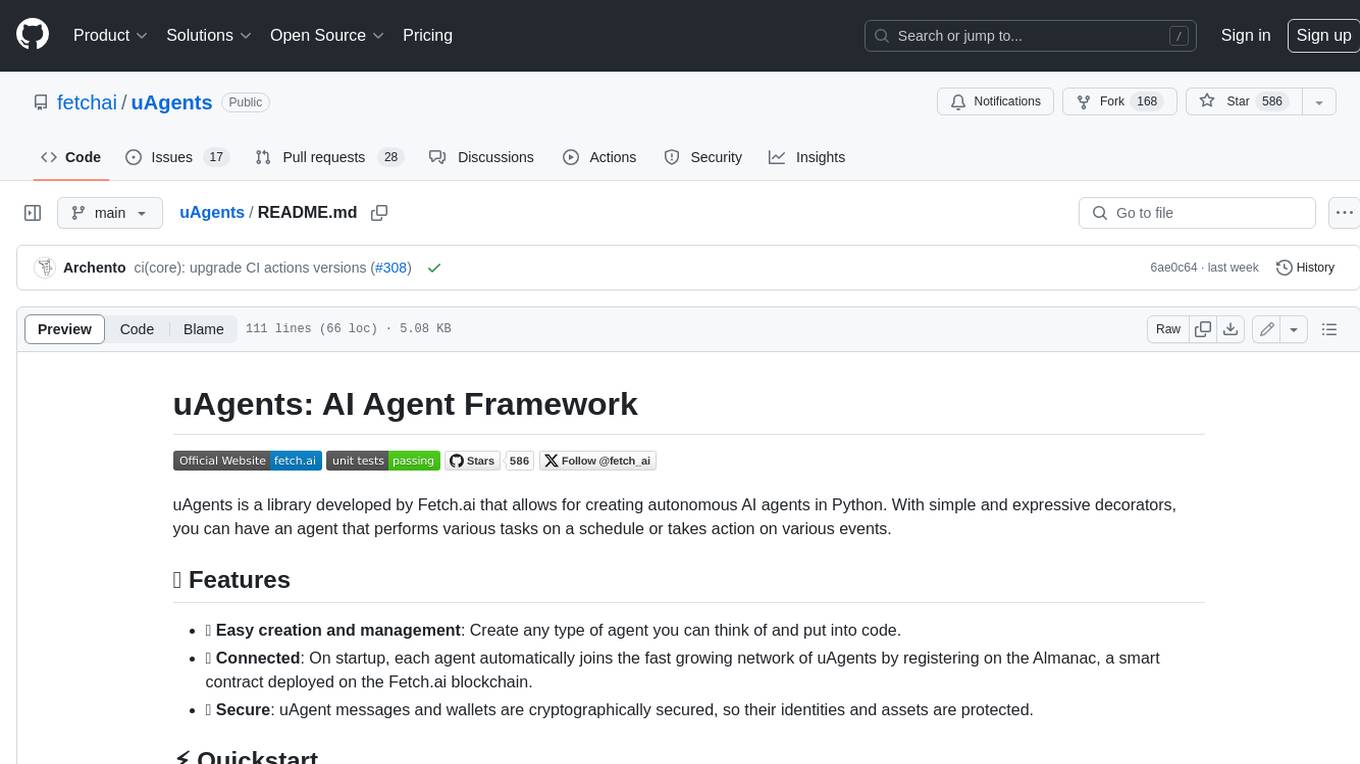
uAgents
uAgents is a Python library developed by Fetch.ai that allows for the creation of autonomous AI agents. These agents can perform various tasks on a schedule or take action on various events. uAgents are easy to create and manage, and they are connected to a fast-growing network of other uAgents. They are also secure, with cryptographically secured messages and wallets.
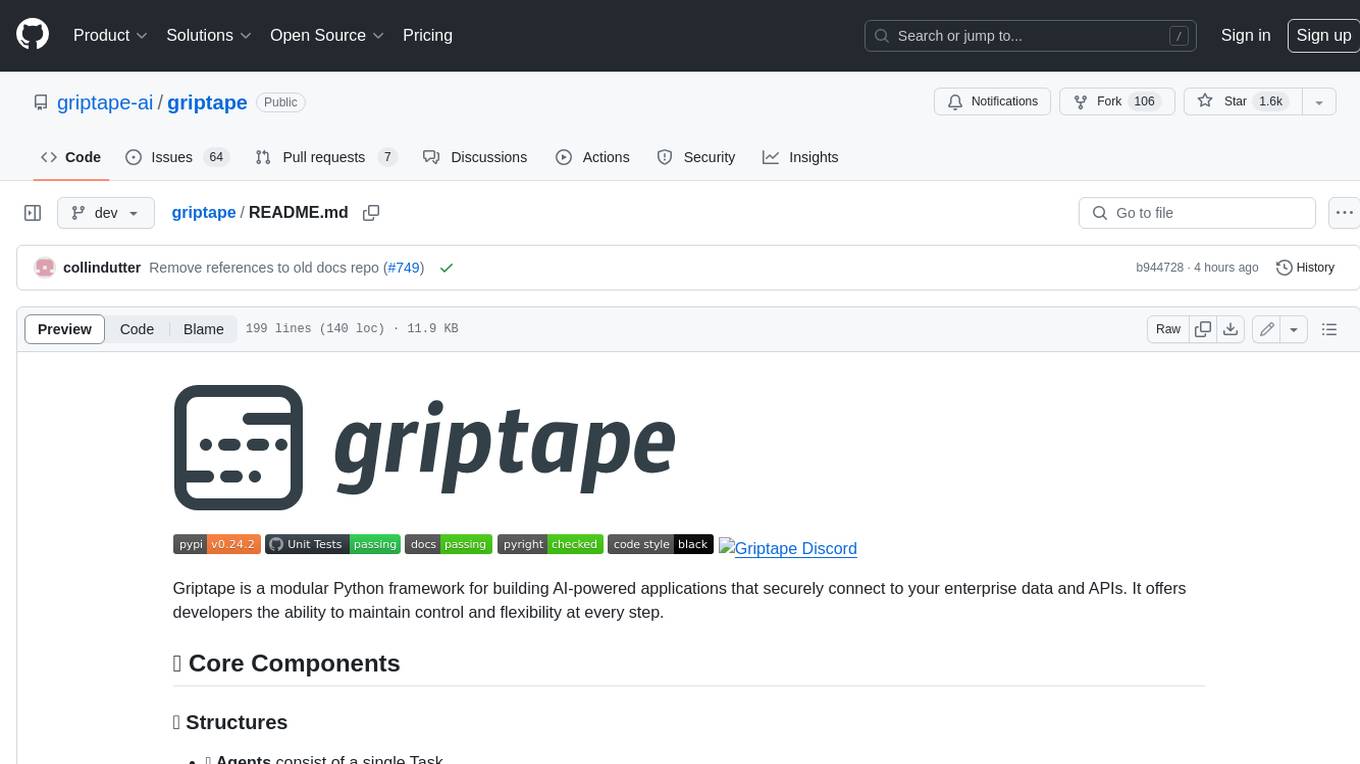
griptape
Griptape is a modular Python framework for building AI-powered applications that securely connect to your enterprise data and APIs. It offers developers the ability to maintain control and flexibility at every step. Griptape's core components include Structures (Agents, Pipelines, and Workflows), Tasks, Tools, Memory (Conversation Memory, Task Memory, and Meta Memory), Drivers (Prompt and Embedding Drivers, Vector Store Drivers, Image Generation Drivers, Image Query Drivers, SQL Drivers, Web Scraper Drivers, and Conversation Memory Drivers), Engines (Query Engines, Extraction Engines, Summary Engines, Image Generation Engines, and Image Query Engines), and additional components (Rulesets, Loaders, Artifacts, Chunkers, and Tokenizers). Griptape enables developers to create AI-powered applications with ease and efficiency.