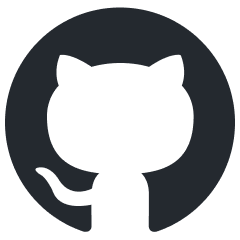
minuet-ai.el
💃 Dance with LLM in Your Code. Minuet offers code completion as-you-type from popular LLMs including OpenAI, Gemini, Claude, Ollama, Llama.cpp, Codestral, and more.
Stars: 155
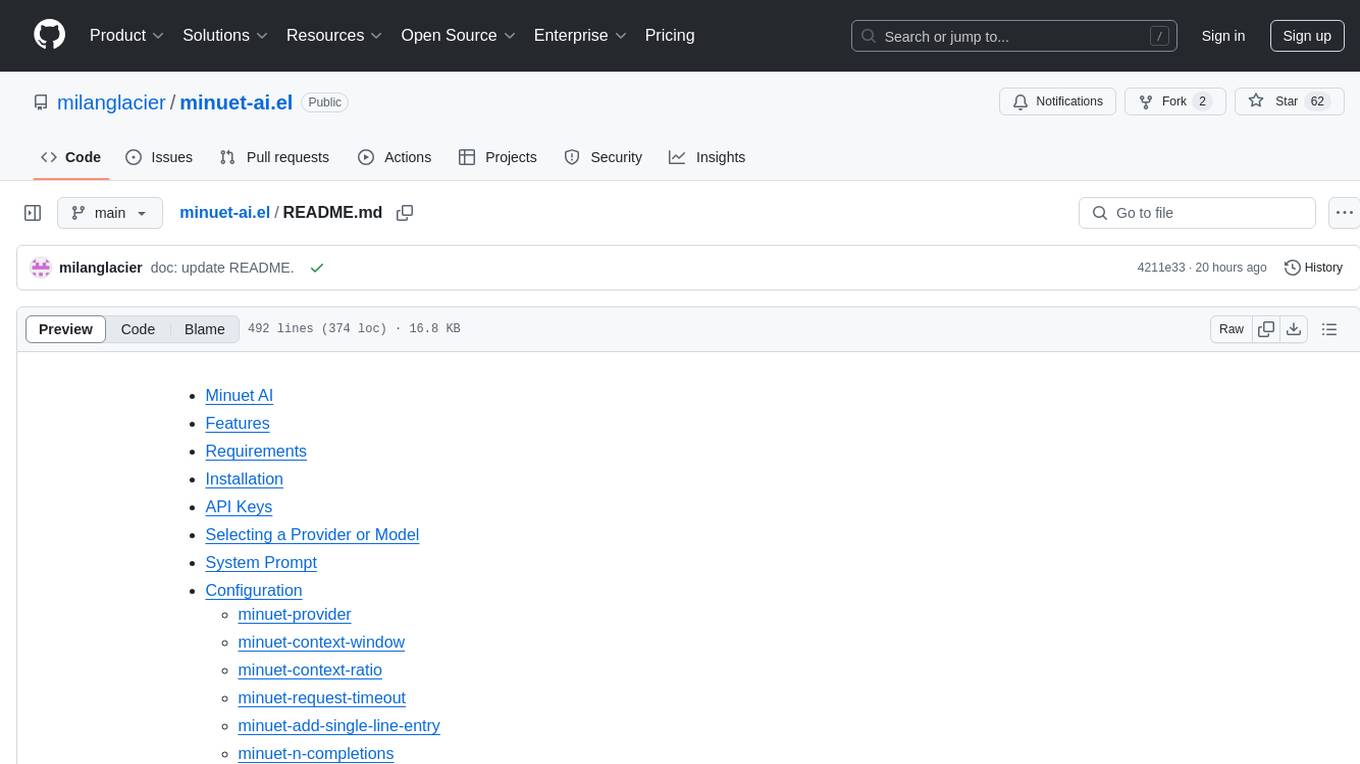
Minuet AI is a tool that brings the grace and harmony of a minuet to your coding process. It offers AI-powered code completion with specialized prompts and enhancements for chat-based LLMs, as well as Fill-in-the-middle (FIM) completion for compatible models. The tool supports multiple AI providers such as OpenAI, Claude, Gemini, Codestral, Ollama, and OpenAI-compatible providers. It provides customizable configuration options and streaming support for completion delivery even with slower LLMs.
README:
- Minuet
- Features
- Requirements
- Installation
- Quick Start: LLM Provider Examples
- API Keys
- Selecting a Provider or Model
- Prompt
- Configuration
- Provider Options
- Troubleshooting
- Acknowledgement
Minuet: Dance with LLM in Your Code 💃.
Minuet
brings the grace and harmony of a minuet to your coding process. Just
as dancers move during a minuet.
- LLM-powered code completion with dual modes:
- Specialized prompts and various enhancements for chat-based LLMs on code completion tasks.
- Fill-in-the-middle (FIM) completion for compatible models (DeepSeek, Codestral, and some Ollama models).
- Support for multiple LLM providers (OpenAI, Claude, Gemini, Codestral, Ollama, Llama.cpp and OpenAI-compatible providers)
- Customizable configuration options
- Streaming support to enable completion delivery even with slower LLMs
With minibuffer frontend:
With overlay ghost text frontend:
- emacs 29+ compiled with native JSON support (verify with
json-available-p
). - plz 0.9+
- dash
- An API key for at least one of the supported LLM providers
minuet
is available on MELPA and can be installed using your preferred package
managers.
;; install with package.el
(package-install 'minuet)
;; install with straight
(straight-use-package 'minuet)
(use-package minuet
:bind
(("M-y" . #'minuet-complete-with-minibuffer) ;; use minibuffer for completion
("M-i" . #'minuet-show-suggestion) ;; use overlay for completion
("C-c m" . #'minuet-configure-provider)
:map minuet-active-mode-map
;; These keymaps activate only when a minuet suggestion is displayed in the current buffer
("M-p" . #'minuet-previous-suggestion) ;; invoke completion or cycle to next completion
("M-n" . #'minuet-next-suggestion) ;; invoke completion or cycle to previous completion
("M-A" . #'minuet-accept-suggestion) ;; accept whole completion
;; Accept the first line of completion, or N lines with a numeric-prefix:
;; e.g. C-u 2 M-a will accepts 2 lines of completion.
("M-a" . #'minuet-accept-suggestion-line)
("M-e" . #'minuet-dismiss-suggestion))
:init
;; if you want to enable auto suggestion.
;; Note that you can manually invoke completions without enable minuet-auto-suggestion-mode
(add-hook 'prog-mode-hook #'minuet-auto-suggestion-mode)
:config
;; You can use M-x minuet-configure-provider to interactively configure provider and model
(setq minuet-provider 'openai-fim-compatible)
(minuet-set-optional-options minuet-openai-fim-compatible-options :max_tokens 64))
;; For Evil users: When defining `minuet-ative-mode-map` in insert
;; or normal states, the following one-liner is required.
;; (add-hook 'minuet-active-mode-hook #'evil-normalize-keymaps)
;; This is *not* necessary when defining `minuet-active-mode-map`.
;; To minimize frequent overhead, it is recommended to avoid adding
;; `evil-normalize-keymaps` to `minuet-active-mode-hook`. Instead,
;; bind keybindings directly within `minuet-active-mode-map` using
;; standard Emacs key sequences, such as `M-xxx`. This approach should
;; not conflict with Evil's keybindings, as Evil primarily avoids
;; using `M-xxx` bindings.
(use-package minuet
:config
(setq minuet-provider 'openai-fim-compatible)
(setq minuet-n-completions 1) ; recommended for Local LLM for resource saving
;; I recommend beginning with a small context window size and incrementally
;; expanding it, depending on your local computing power. A context window
;; of 512, serves as an good starting point to estimate your computing
;; power. Once you have a reliable estimate of your local computing power,
;; you should adjust the context window to a larger value.
(setq minuet-context-window 512)
(plist-put minuet-openai-fim-compatible-options :end-point "http://localhost:11434/v1/completions")
;; an arbitrary non-null environment variable as placeholder
(plist-put minuet-openai-fim-compatible-options :name "Ollama")
(plist-put minuet-openai-fim-compatible-options :api-key "TERM")
(plist-put minuet-openai-fim-compatible-options :model "qwen2.5-coder:3b")
(minuet-set-optional-options minuet-openai-fim-compatible-options :max_tokens 56))
(use-package minuet
:config
(setq minuet-provider 'openai-compatible)
(setq minuet-request-timeout 2.5)
(setq minuet-auto-suggestion-throttle-delay 1.5) ;; Increase to reduce costs and avoid rate limits
(setq minuet-auto-suggestion-debounce-delay 0.6) ;; Increase to reduce costs and avoid rate limits
(plist-put minuet-openai-compatible-options :end-point "https://openrouter.ai/api/v1/chat/completions")
(plist-put minuet-openai-compatible-options :api-key "OPENROUTER_API_KEY")
(plist-put minuet-openai-compatible-options :model "qwen/qwen2.5-32b-instruct")
;; Prioritize throughput for faster completion
(minuet-set-optional-options minuet-openai-compatible-options :provider '(:sort "throughput"))
(minuet-set-optional-options minuet-openai-compatible-options :max_tokens 128)
(minuet-set-optional-options minuet-openai-compatible-options :top_p 0.9))
First, launch the llama-server
with your chosen model.
Here's an example of a bash script to start the server if your system has less than 8GB of VRAM:
llama-server \
-hf ggml-org/Qwen2.5-Coder-1.5B-Q8_0-GGUF \
--port 8012 -ngl 99 -fa -ub 1024 -b 1024 \
--ctx-size 0 --cache-reuse 256
(use-package minuet
:config
(setq minuet-provider 'openai-fim-compatible)
(setq minuet-n-completions 1) ; recommended for Local LLM for resource saving
;; I recommend beginning with a small context window size and incrementally
;; expanding it, depending on your local computing power. A context window
;; of 512, serves as an good starting point to estimate your computing
;; power. Once you have a reliable estimate of your local computing power,
;; you should adjust the context window to a larger value.
(setq minuet-context-window 512)
(plist-put minuet-openai-fim-compatible-options :end-point "http://localhost:8012/v1/completions")
;; an arbitrary non-null environment variable as placeholder
(plist-put minuet-openai-fim-compatible-options :name "Llama.cpp")
(plist-put minuet-openai-fim-compatible-options :api-key "TERM")
;; The model is set by the llama-cpp server and cannot be altered
;; post-launch.
(plist-put minuet-openai-fim-compatible-options :model "PLACEHOLDER")
;; Llama.cpp does not support the `suffix` option in FIM completion.
;; Therefore, we must disable it and manually populate the special
;; tokens required for FIM completion.
(minuet-set-optional-options minuet-openai-fim-compatible-options :suffix nil :template)
(minuet-set-optional-options
minuet-openai-fim-compatible-options
:prompt
(defun minuet-llama-cpp-fim-qwen-prompt-function (ctx)
(format "<|fim_prefix|>%s\n%s<|fim_suffix|>%s<|fim_middle|>"
(plist-get ctx :language-and-tab)
(plist-get ctx :before-cursor)
(plist-get ctx :after-cursor)))
:template)
(minuet-set-optional-options minuet-openai-fim-compatible-options :max_tokens 56))
For additional example bash scripts to run llama.cpp based on your local computing power, please refer to recipes.md.
Minuet requires API keys to function. Set the following environment variables:
-
OPENAI_API_KEY
for OpenAI -
GEMINI_API_KEY
for Gemini -
ANTHROPIC_API_KEY
for Claude -
CODESTRAL_API_KEY
for Codestral - Custom environment variable for OpenAI-compatible services (as specified in your configuration)
Note: Provide the name of the environment variable to Minuet inside the
provider options, not the actual value. For instance, pass OPENAI_API_KEY
to
Minuet, not the value itself (e.g., sk-xxxx
).
If using Ollama, you need to assign an arbitrary, non-null environment variable as a placeholder for it to function.
Alternatively, you can provide a function that returns the API key. This function should return the result instantly as it will be called with each completion request.
;; Good
(plist-put minuet-openai-compatible-options :api-key "FIREWORKS_API_KEY")
(plist-put minuet-openai-compatible-options :api-key (defun my-fireworks-api-key () "sk-xxxx"))
;; Bad
(plist-put minuet-openai-compatible-options :api-key "sk-xxxxx")
The gemini-flash
and codestral
models offer high-quality output with free
and fast processing. For optimal quality (albeit slower generation speed),
consider using the deepseek-chat
model, which is compatible with both
openai-fim-compatible
and openai-compatible
providers. For local LLM
inference, you can deploy either qwen-2.5-coder
or deepseek-coder-v2
through
Ollama using the openai-fim-compatible
provider.
Note: as of January 27, 2025, the high server demand from deepseek may
significantly slow down the default provider used by Minuet
(openai-fim-compatible
with deepseek). We recommend trying alternative
providers instead.
See prompt for the default prompt used by minuet
and
instructions on customization.
Note that minuet
employs two distinct prompt systems:
- A system designed for chat-based LLMs (OpenAI, OpenAI-Compatible, Claude, and Gemini)
- A separate system designed for Codestral and OpenAI-FIM-compatible models
Below are commonly used configuration options. To view the complete list of
available settings, search for minuet
through the customize
interface.
Set the provider you want to use for completion with minuet, available options:
openai
, openai-compatible
, claude
, gemini
, openai-fim-compatible
, and
codestral
.
The default is openai-fim-compatible
using the deepseek endpoint.
You can use ollama
with either openai-compatible
or openai-fim-compatible
provider, depending on your model is a chat model or code completion (FIM)
model.
The maximum total characters of the context before and after cursor. This limits how much surrounding code is sent to the LLM for context.
The default is 16000, which roughly equates to 4000 tokens after tokenization.
Ratio of context before cursor vs after cursor. When the total characters exceed
the context window, this ratio determines how much context to keep before vs
after the cursor. A larger ratio means more context before the cursor will be
used. The ratio should between 0 and 1
, and default is 0.75
.
Maximum timeout in seconds for sending completion requests. In case of the
timeout, the incomplete completion items will be delivered. The default is 3
.
For minuet-complete-with-minibuffer
function, Whether to create additional
single-line completion items. When non-nil and a completion item has multiple
lines, create another completion item containing only its first line. This
option has no impact for overlay-based suggesion.
For FIM model, this is the number of requests to send. For chat LLM , this is
the number of completions encoded as part of the prompt. Note that when
minuet-add-single-line-entry
is true, the actual number of returned items may
exceed this value. Additionally, the LLM cannot guarantee the exact number of
completion items specified, as this parameter serves only as a prompt guideline.
The default is 3
.
If resource efficiency is imporant, it is recommended to set this value to 1
.
The delay in seconds before sending a completion request after typing stops. The
default is 0.4
seconds.
The minimum time in seconds between 2 completion requests. The default is 1.0
seconds.
You can customize the provider options using plist-put
, for example:
(with-eval-after-load 'minuet
;; change openai model to gpt-4o
(plist-put minuet-openai-options :model "gpt-4o")
;; change openai-compatible provider to use fireworks
(setq minuet-provider 'openai-compatible)
(plist-put minuet-openai-compatible-options :end-point "https://api.fireworks.ai/inference/v1/chat/completions")
(plist-put minuet-openai-compatible-options :api-key "FIREWORKS_API_KEY")
(plist-put minuet-openai-compatible-options :model "accounts/fireworks/models/llama-v3p3-70b-instruct")
)
To pass optional parameters (like max_tokens
and top_p
) to send to the curl
request, you can use function minuet-set-optional-options
:
(minuet-set-optional-options minuet-openai-options :max_tokens 256)
(minuet-set-optional-options minuet-openai-options :top_p 0.9)
Below is the default value:
(defvar minuet-openai-options
`(:model "gpt-4o-mini"
:api-key "OPENAI_API_KEY"
:system
(:template minuet-default-system-template
:prompt minuet-default-prompt
:guidelines minuet-default-guidelines
:n-completions-template minuet-default-n-completion-template)
:fewshots minuet-default-fewshots
:chat-input
(:template minuet-default-chat-input-template
:language-and-tab minuet--default-chat-input-language-and-tab-function
:context-before-cursor minuet--default-chat-input-before-cursor-function
:context-after-cursor minuet--default-chat-input-after-cursor-function)
:optional nil)
"config options for Minuet OpenAI provider")
Below is the default value:
(defvar minuet-claude-options
`(:model "claude-3-5-haiku-20241022"
:max_tokens 512
:api-key "ANTHROPIC_API_KEY"
:system
(:template minuet-default-system-template
:prompt minuet-default-prompt
:guidelines minuet-default-guidelines
:n-completions-template minuet-default-n-completion-template)
:fewshots minuet-default-fewshots
:chat-input
(:template minuet-default-chat-input-template
:language-and-tab minuet--default-chat-input-language-and-tab-function
:context-before-cursor minuet--default-chat-input-before-cursor-function
:context-after-cursor minuet--default-chat-input-after-cursor-function)
:optional nil)
"config options for Minuet Claude provider")
Codestral is a text completion model, not a chat model, so the system prompt and
few shot examples does not apply. Note that you should use the
CODESTRAL_API_KEY
, not the MISTRAL_API_KEY
, as they are using different
endpoint. To use the Mistral endpoint, simply modify the end_point
and
api_key
parameters in the configuration.
Below is the default value:
(defvar minuet-codestral-options
'(:model "codestral-latest"
:end-point "https://codestral.mistral.ai/v1/fim/completions"
:api-key "CODESTRAL_API_KEY"
:template (:prompt minuet--default-fim-prompt-function
:suffix minuet--default-fim-suffix-function)
:optional nil)
"config options for Minuet Codestral provider")
The following configuration is not the default, but recommended to prevent request timeout from outputing too many tokens.
(minuet-set-optional-options minuet-codestral-options :stop ["\n\n"])
(minuet-set-optional-options minuet-codestral-options :max_tokens 256)
You should register the account and use the service from Google AI Studio instead of Google Cloud. You can get an API key via their Google API page.
The following config is the default.
(defvar minuet-gemini-options
`(:model "gemini-2.0-flash"
:api-key "GEMINI_API_KEY"
:system
(:template minuet-default-system-template
:prompt minuet-default-prompt-prefix-first
:guidelines minuet-default-guidelines
:n-completions-template minuet-default-n-completion-template)
:fewshots minuet-default-fewshots-prefix-first
:chat-input
(:template minuet-default-chat-input-template-prefix-first
:language-and-tab minuet--default-chat-input-language-and-tab-function
:context-before-cursor minuet--default-chat-input-before-cursor-function
:context-after-cursor minuet--default-chat-input-after-cursor-function)
:optional nil)
"config options for Minuet Gemini provider")
The following configuration is not the default, but recommended to prevent request timeout from outputing too many tokens. You can also adjust the safety settings following the example:
(minuet-set-optional-options minuet-gemini-options
:generationConfig
'(:maxOutputTokens 256
:topP 0.9))
(minuet-set-optional-options minuet-gemini-options
:safetySettings
[(:category "HARM_CATEGORY_DANGEROUS_CONTENT"
:threshold "BLOCK_NONE")
(:category "HARM_CATEGORY_HATE_SPEECH"
:threshold "BLOCK_NONE")
(:category "HARM_CATEGORY_HARASSMENT"
:threshold "BLOCK_NONE")
(:category "HARM_CATEGORY_SEXUALLY_EXPLICIT"
:threshold "BLOCK_NONE")])
Use any providers compatible with OpenAI's chat completion API.
For example, you can set the end_point
to
http://localhost:11434/v1/chat/completions
to use ollama
.
The following config is the default.
(defvar minuet-openai-compatible-options
`(:end-point "https://openrouter.ai/api/v1/chat/completions"
:api-key "OPENROUTER_API_KEY"
:model "qwen/qwen2.5-32b-instruct"
:system
(:template minuet-default-system-template
:prompt minuet-default-prompt
:guidelines minuet-default-guidelines
:n-completions-template minuet-default-n-completion-template)
:fewshots minuet-default-fewshots
:chat-input
(:template minuet-default-chat-input-template
:language-and-tab minuet--default-chat-input-language-and-tab-function
:context-before-cursor minuet--default-chat-input-before-cursor-function
:context-after-cursor minuet--default-chat-input-after-cursor-function)
:optional nil)
"Config options for Minuet OpenAI compatible provider.")
The following configuration is not the default, but recommended to prevent request timeout from outputing too many tokens.
(minuet-set-optional-options minuet-openai-compatible-options :max_tokens 256)
(minuet-set-optional-options minuet-openai-compatible-options :top_p 0.9)
Use any provider compatible with OpenAI's completion API. This request uses the
text /completions
endpoint, not /chat/completions
endpoint, so system
prompts and few-shot examples are not applicable.
For example, you can set the end_point
to
http://localhost:11434/v1/completions
to use ollama
, or set it to
http://localhost:8012/v1/completions
to use llama.cpp
.
Additionally, for Ollama users, it is essential to verify whether the model's
template supports FIM completion. For example, qwen2.5-coder offers FIM support,
as suggested in its
template. However
it may come as a surprise to some users that, deepseek-coder
does not support
the FIM template, and you should use deepseek-coder-v2
instead.
The following config is the default.
(defvar minuet-openai-fim-compatible-options
'(:model "deepseek-chat"
:end-point "https://api.deepseek.com/beta/completions"
:api-key "DEEPSEEK_API_KEY"
:name "Deepseek"
:template (:prompt minuet--default-fim-prompt-function
:suffix minuet--default-fim-suffix-function)
:optional nil)
"config options for Minuet OpenAI FIM compatible provider")
The following configuration is not the default, but recommended to prevent request timeout from outputing too many tokens.
(minuet-set-optional-options minuet-openai-fim-compatible-options :max_tokens 256)
(minuet-set-optional-options minuet-openai-fim-compatible-options :top_p 0.9)
For example bash scripts to run llama.cpp based on your local computing power,
please refer to recipes.md. Note that the model for llama.cpp
must be determined when you launch the llama.cpp
server and cannot be changed
thereafter.
If your setup failed, there are two most likely reasons:
- You may set the API key incorrectly. Checkout the API Key section to see how to correctly specify the API key.
- You are using a model or a context window that is too large, causing
completion items to timeout before returning any tokens. This is particularly
common with local LLM. It is recommended to start with the following settings
to have a better understanding of your provider's inference speed.
- Begin by testing with manual completions.
- Use a smaller context window (e.g.,
context-window = 768
) - Use a smaller model
- Set a longer request timeout (e.g.,
request-timeout = 5
)
To diagnose issues, examine the buffer content from *minuet*
.
- continue.dev: not a emacs plugin, but I find a lot LLM models from here.
- llama.vim: Reference for CLI parameters used to launch the llama-cpp server.
For Tasks:
Click tags to check more tools for each tasksFor Jobs:
Alternative AI tools for minuet-ai.el
Similar Open Source Tools
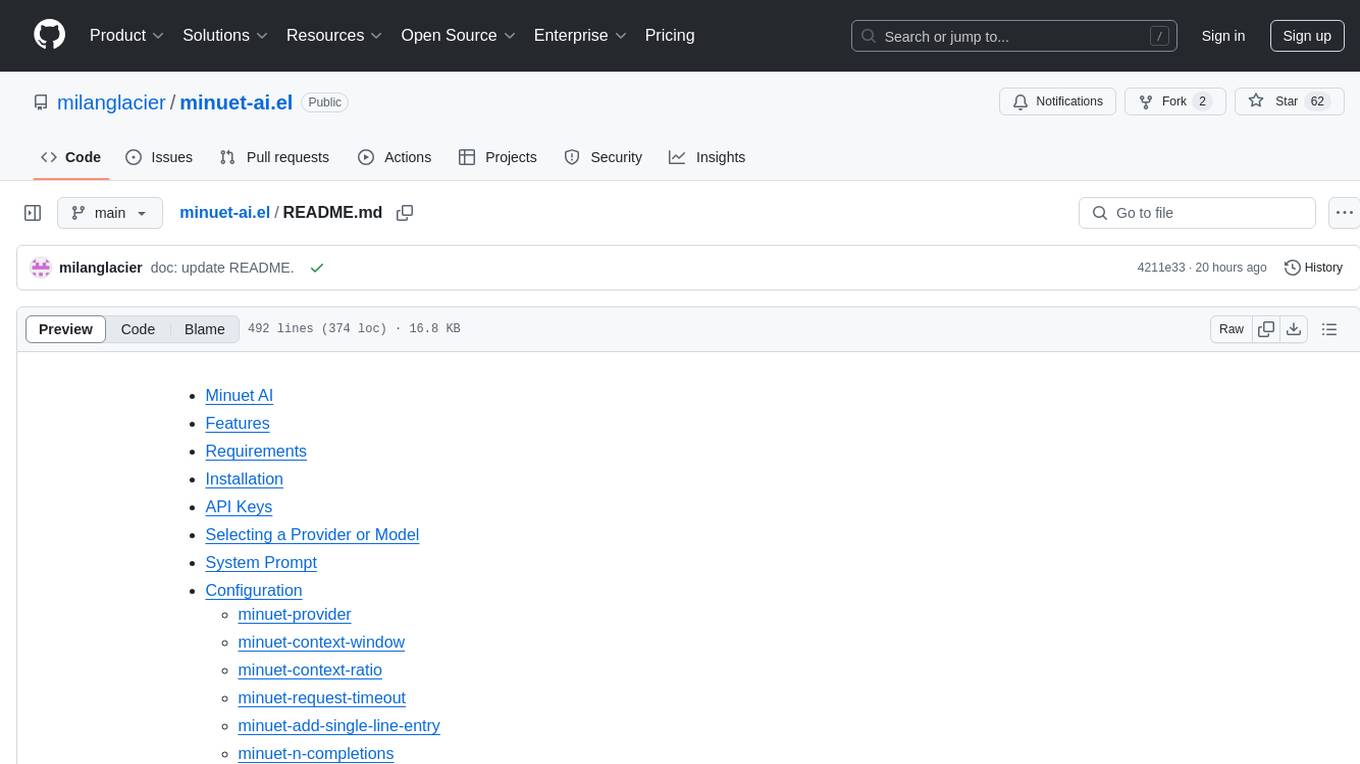
minuet-ai.el
Minuet AI is a tool that brings the grace and harmony of a minuet to your coding process. It offers AI-powered code completion with specialized prompts and enhancements for chat-based LLMs, as well as Fill-in-the-middle (FIM) completion for compatible models. The tool supports multiple AI providers such as OpenAI, Claude, Gemini, Codestral, Ollama, and OpenAI-compatible providers. It provides customizable configuration options and streaming support for completion delivery even with slower LLMs.
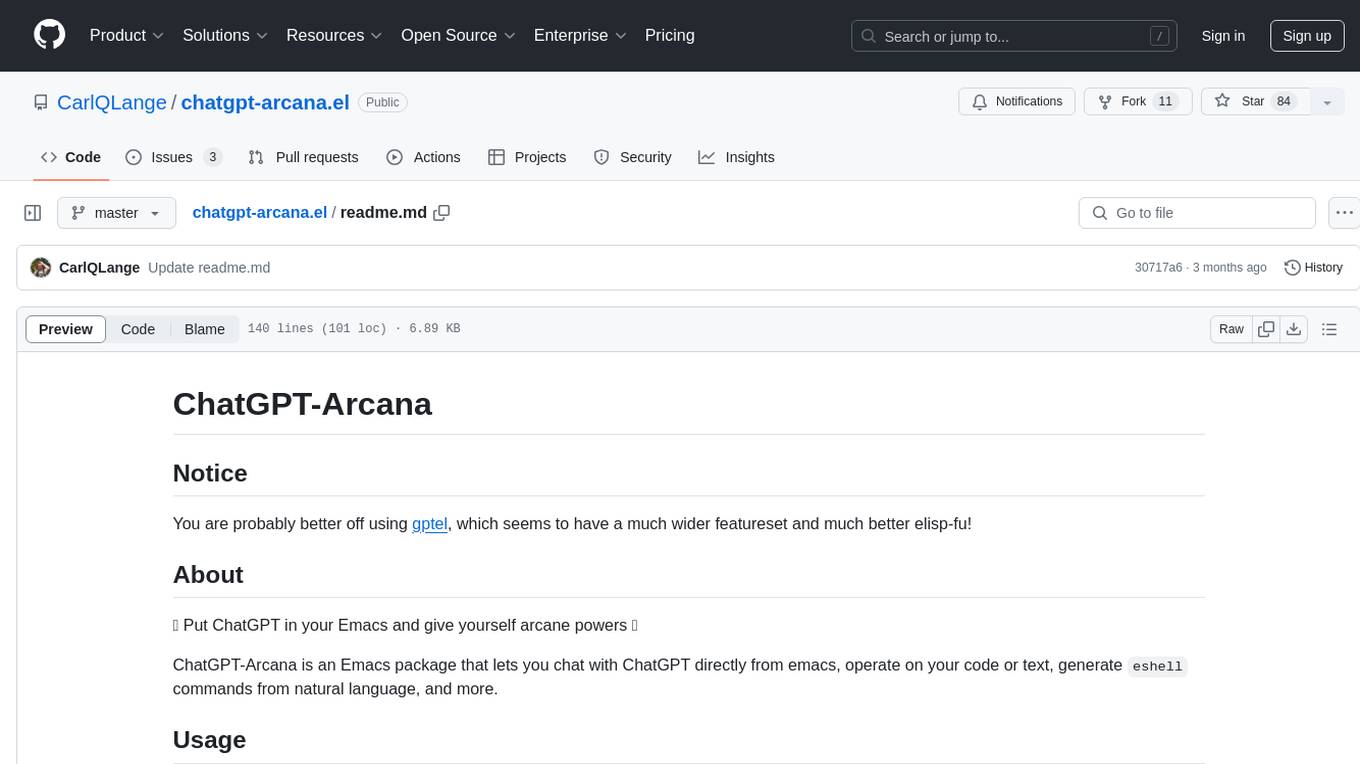
chatgpt-arcana.el
ChatGPT-Arcana is an Emacs package that allows users to interact with ChatGPT directly from Emacs, enabling tasks such as chatting with GPT, operating on code or text, generating eshell commands from natural language, fixing errors, writing commit messages, and creating agents for web search and code evaluation. The package requires an API key from OpenAI's GPT-3 model and offers various interactive functions for enhancing productivity within Emacs.
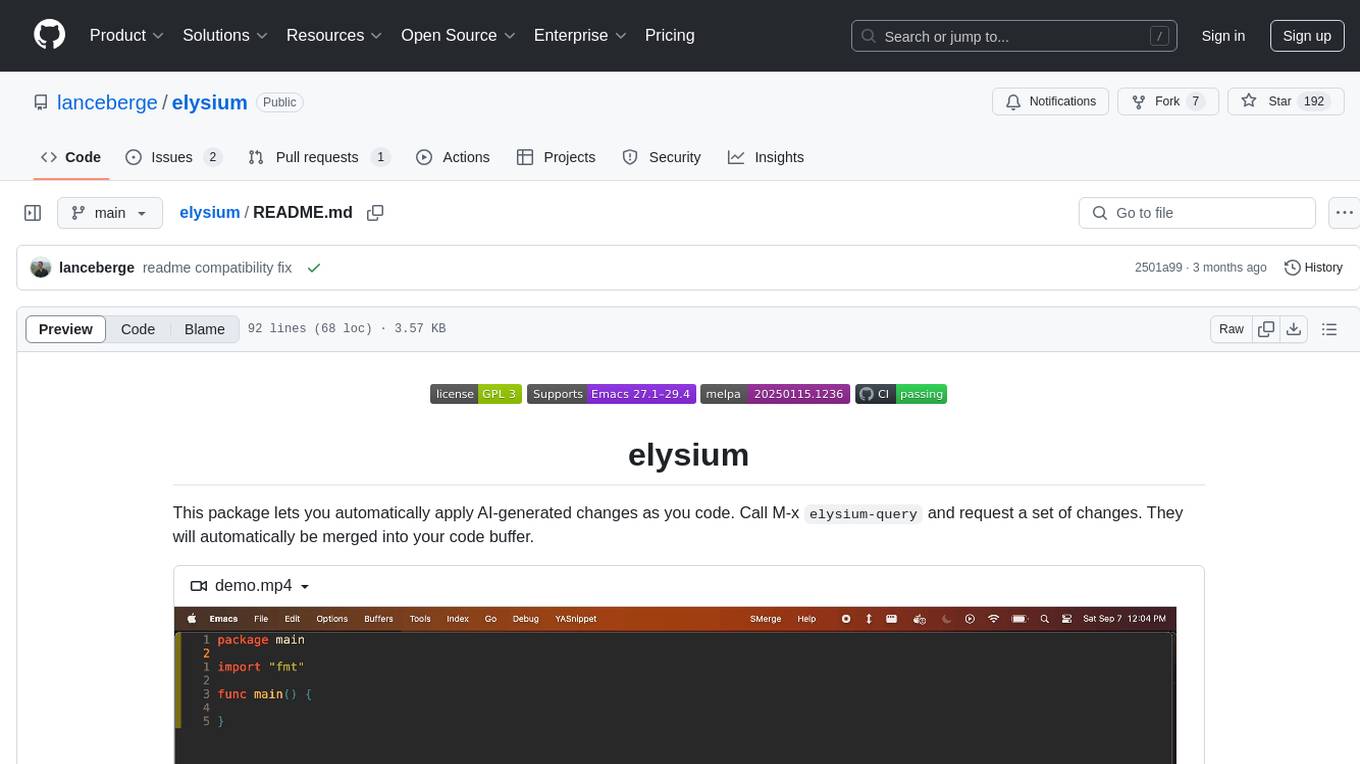
elysium
Elysium is an Emacs package that allows users to automatically apply AI-generated changes while coding. By calling `elysium-query`, users can request a set of changes that will be merged into the code buffer. The tool supports making queries on a specific region without leaving the code buffer. It uses the `gptel` backend and currently recommends using the Claude 3-5 Sonnet model for generating code. Users can customize the window size and style of the Elysium buffer. The tool also provides functions to keep or discard AI-suggested changes and navigate conflicting hunks with `smerge-mode`.
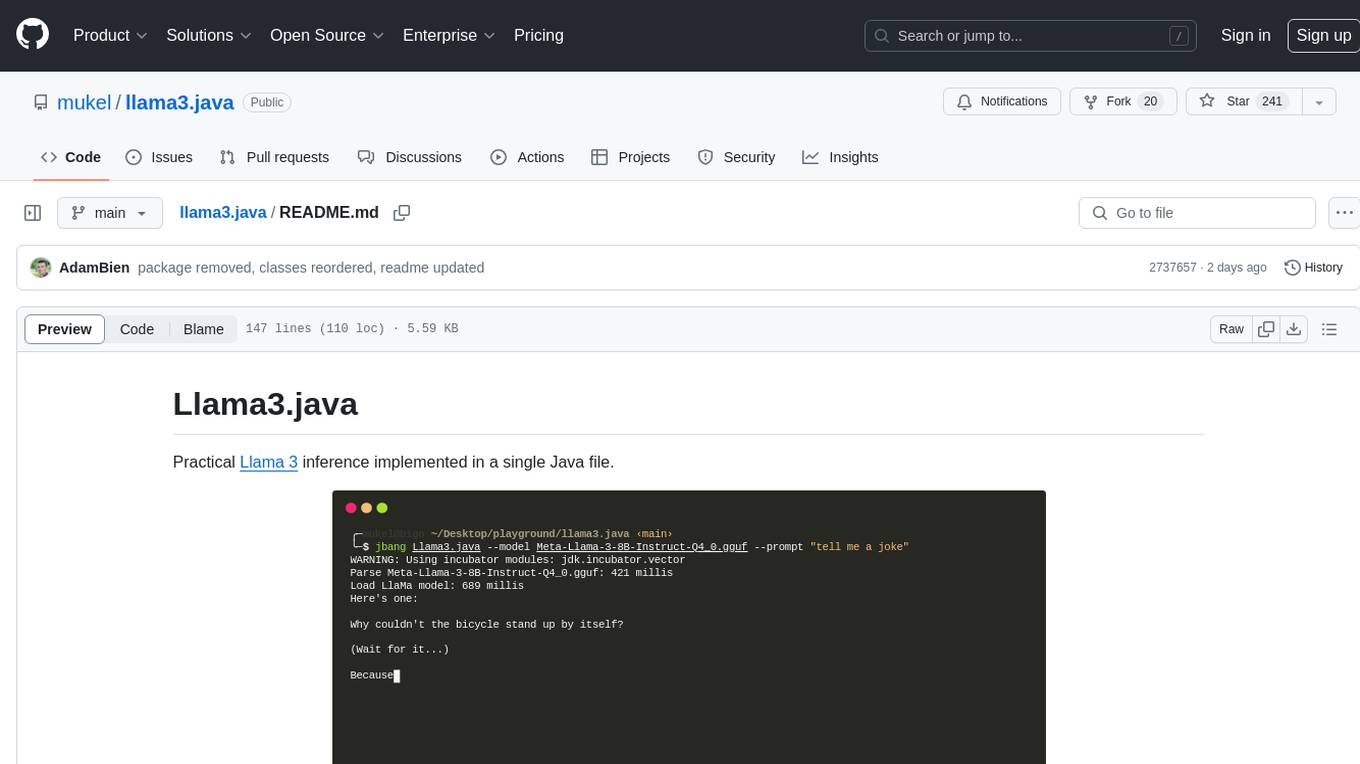
llama3.java
Llama3.java is a practical Llama 3 inference tool implemented in a single Java file. It serves as the successor of llama2.java and is designed for testing and tuning compiler optimizations and features on the JVM, especially for the Graal compiler. The tool features a GGUF format parser, Llama 3 tokenizer, Grouped-Query Attention inference, support for Q8_0 and Q4_0 quantizations, fast matrix-vector multiplication routines using Java's Vector API, and a simple CLI with 'chat' and 'instruct' modes. Users can download quantized .gguf files from huggingface.co for model usage and can also manually quantize to pure 'Q4_0'. The tool requires Java 21+ and supports running from source or building a JAR file for execution. Performance benchmarks show varying tokens/s rates for different models and implementations on different hardware setups.
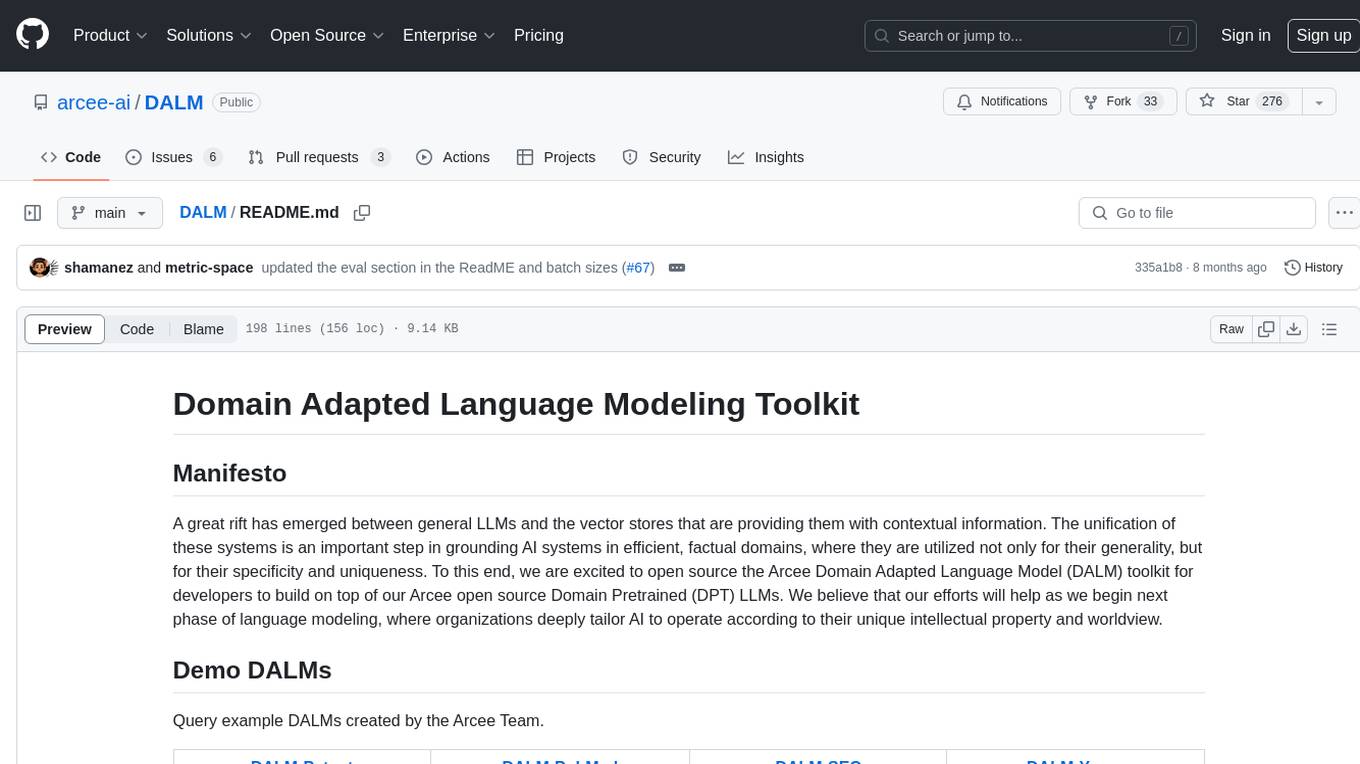
DALM
The DALM (Domain Adapted Language Modeling) toolkit is designed to unify general LLMs with vector stores to ground AI systems in efficient, factual domains. It provides developers with tools to build on top of Arcee's open source Domain Pretrained LLMs, enabling organizations to deeply tailor AI according to their unique intellectual property and worldview. The toolkit contains code for fine-tuning a fully differential Retrieval Augmented Generation (RAG-end2end) architecture, incorporating in-batch negative concept alongside RAG's marginalization for efficiency. It includes training scripts for both retriever and generator models, evaluation scripts, data processing codes, and synthetic data generation code.
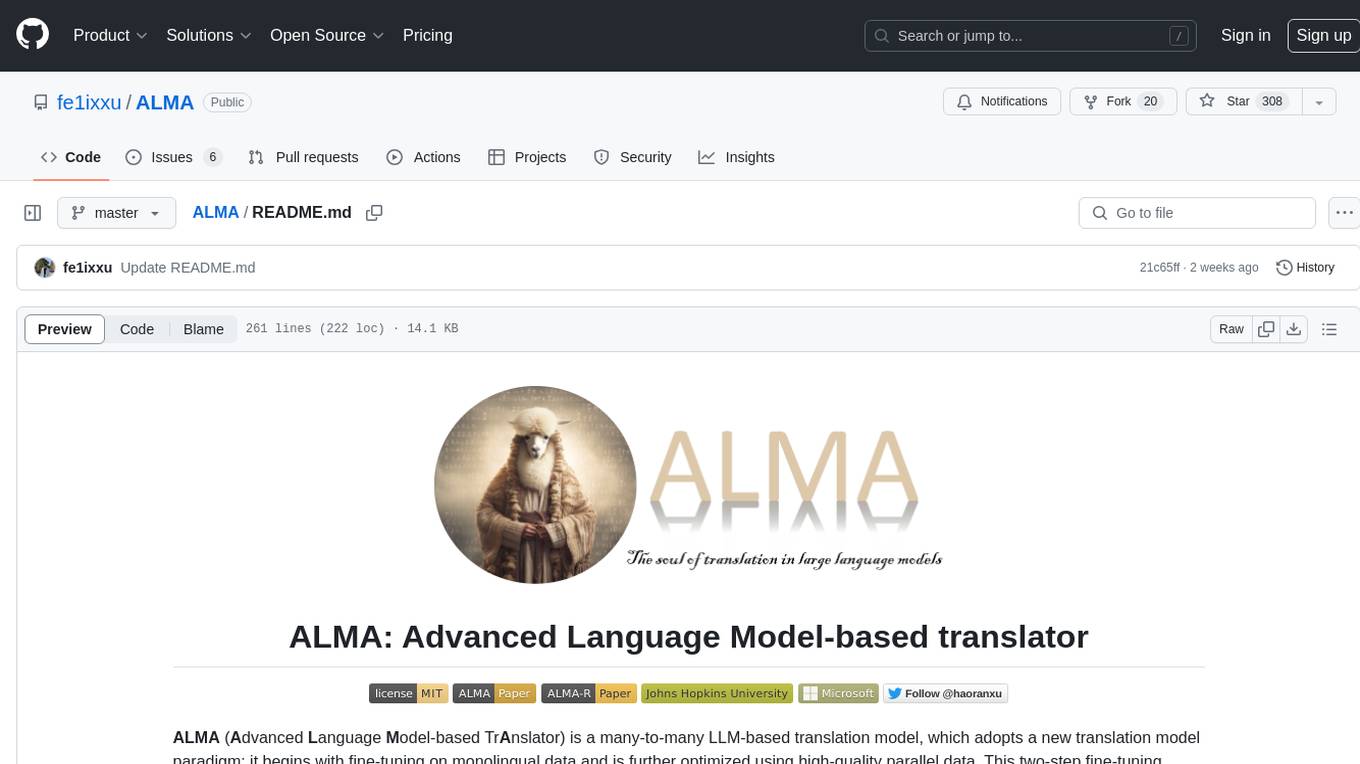
ALMA
ALMA (Advanced Language Model-based Translator) is a many-to-many LLM-based translation model that utilizes a two-step fine-tuning process on monolingual and parallel data to achieve strong translation performance. ALMA-R builds upon ALMA models with LoRA fine-tuning and Contrastive Preference Optimization (CPO) for even better performance, surpassing GPT-4 and WMT winners. The repository provides ALMA and ALMA-R models, datasets, environment setup, evaluation scripts, training guides, and data information for users to leverage these models for translation tasks.
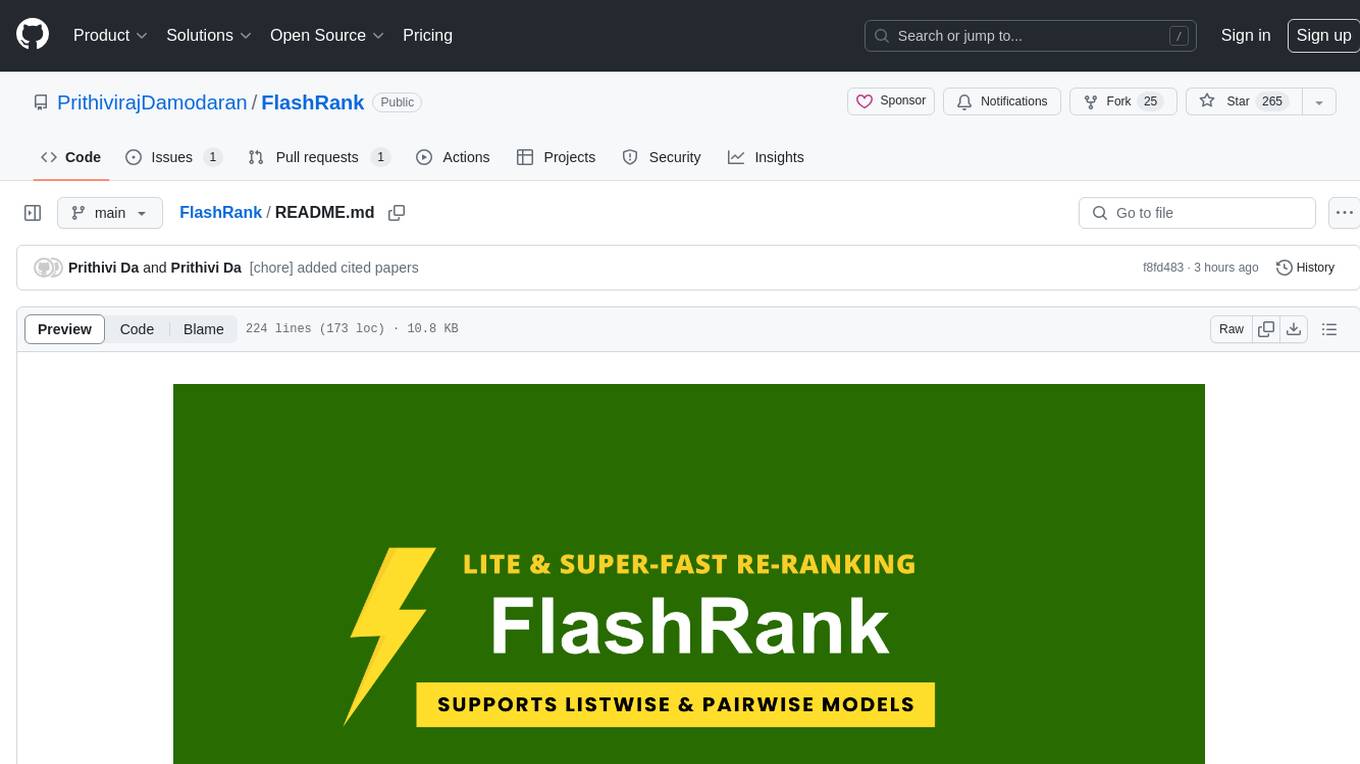
FlashRank
FlashRank is an ultra-lite and super-fast Python library designed to add re-ranking capabilities to existing search and retrieval pipelines. It is based on state-of-the-art Language Models (LLMs) and cross-encoders, offering support for pairwise/pointwise rerankers and listwise LLM-based rerankers. The library boasts the tiniest reranking model in the world (~4MB) and runs on CPU without the need for Torch or Transformers. FlashRank is cost-conscious, with a focus on low cost per invocation and smaller package size for efficient serverless deployments. It supports various models like ms-marco-TinyBERT, ms-marco-MiniLM, rank-T5-flan, ms-marco-MultiBERT, and more, with plans for future model additions. The tool is ideal for enhancing search precision and speed in scenarios where lightweight models with competitive performance are preferred.
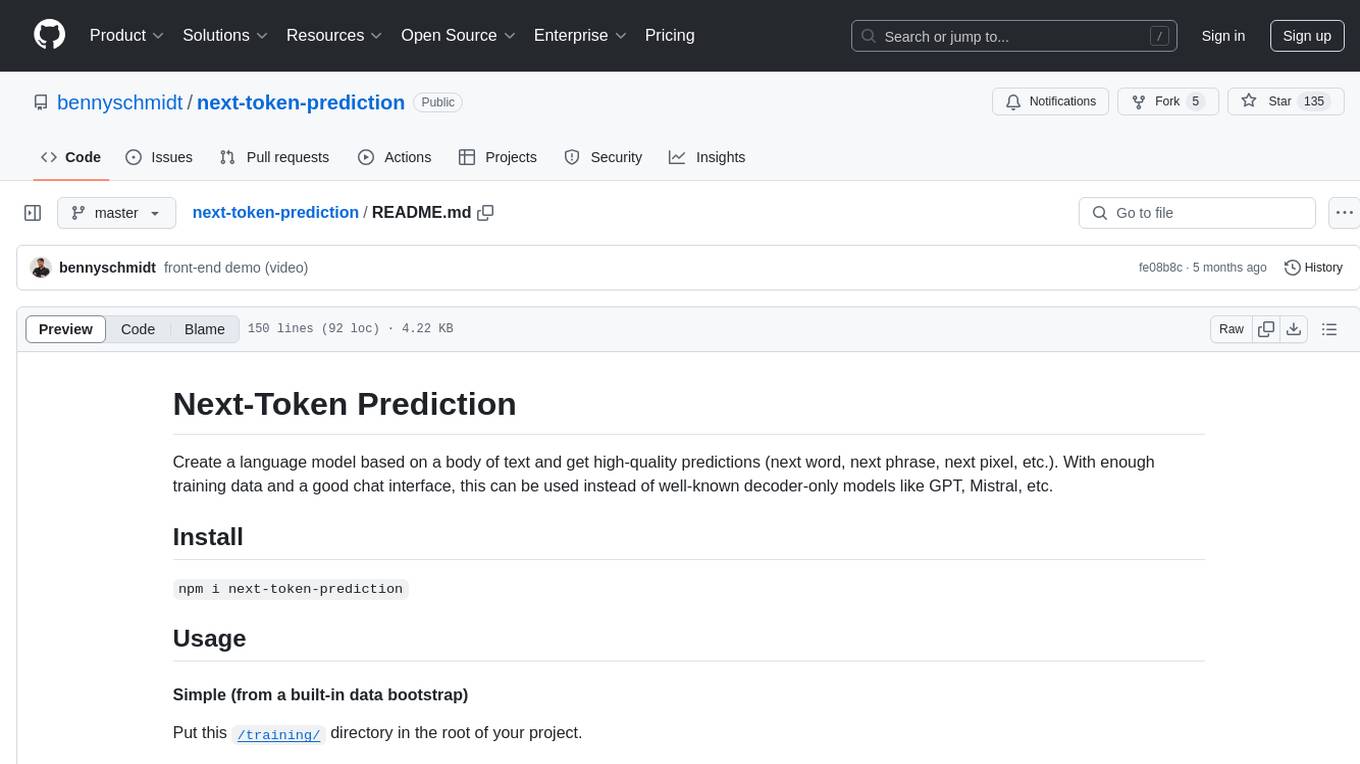
next-token-prediction
Next-Token Prediction is a language model tool that allows users to create high-quality predictions for the next word, phrase, or pixel based on a body of text. It can be used as an alternative to well-known decoder-only models like GPT and Mistral. The tool provides options for simple usage with built-in data bootstrap or advanced customization by providing training data or creating it from .txt files. It aims to simplify methodologies, provide autocomplete, autocorrect, spell checking, search/lookup functionalities, and create pixel and audio transformers for various prediction formats.
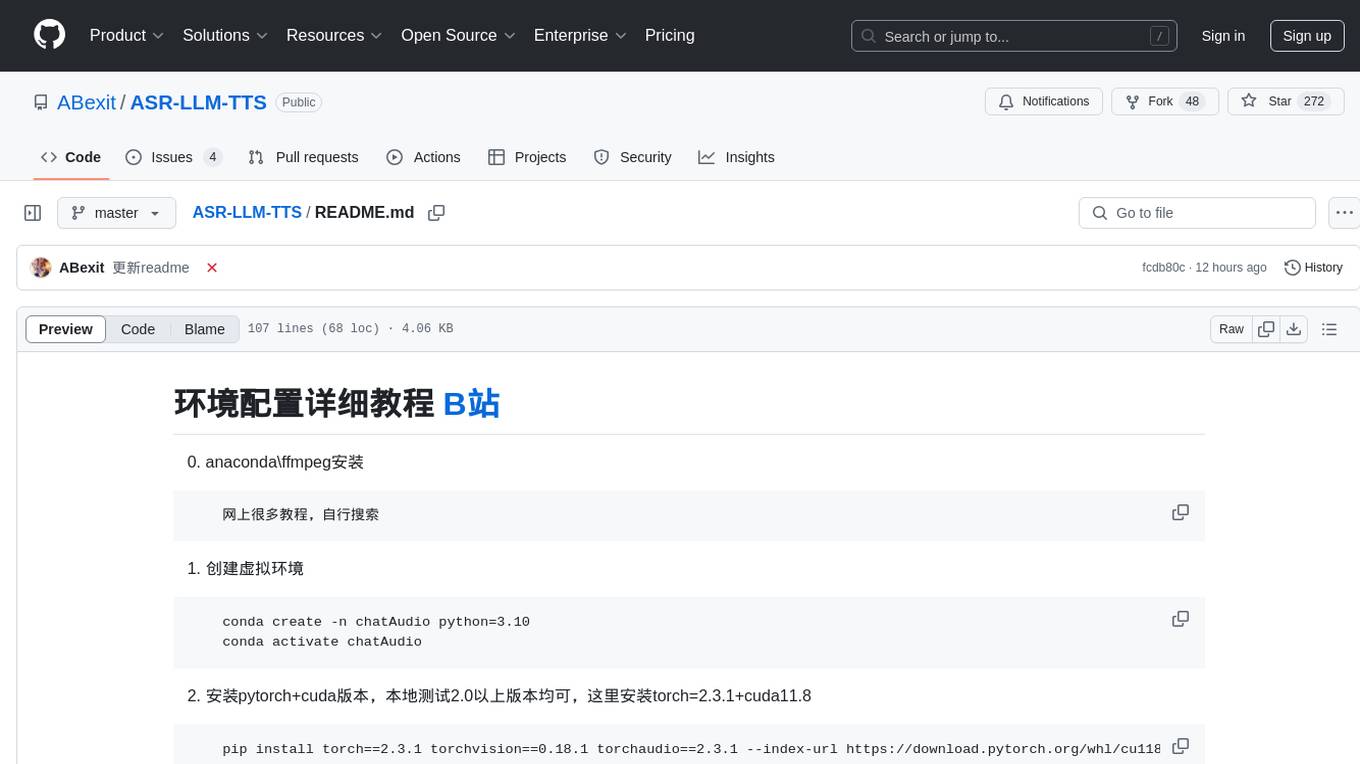
ASR-LLM-TTS
ASR-LLM-TTS is a repository that provides detailed tutorials for setting up the environment, including installing anaconda, ffmpeg, creating virtual environments, and installing necessary libraries such as pytorch, torchaudio, edge-tts, funasr, and more. It also introduces features like voiceprint recognition, custom wake words, and conversation history memory. The repository combines CosyVoice for speech synthesis, SenceVoice for speech recognition, and QWen2.5 for dialogue understanding. It offers multiple speech synthesis methods including CoosyVoice, pyttsx3, and edgeTTS, with scripts for interactive inference provided. The repository aims to enable real-time speech interaction and multi-modal interactions involving audio and video.
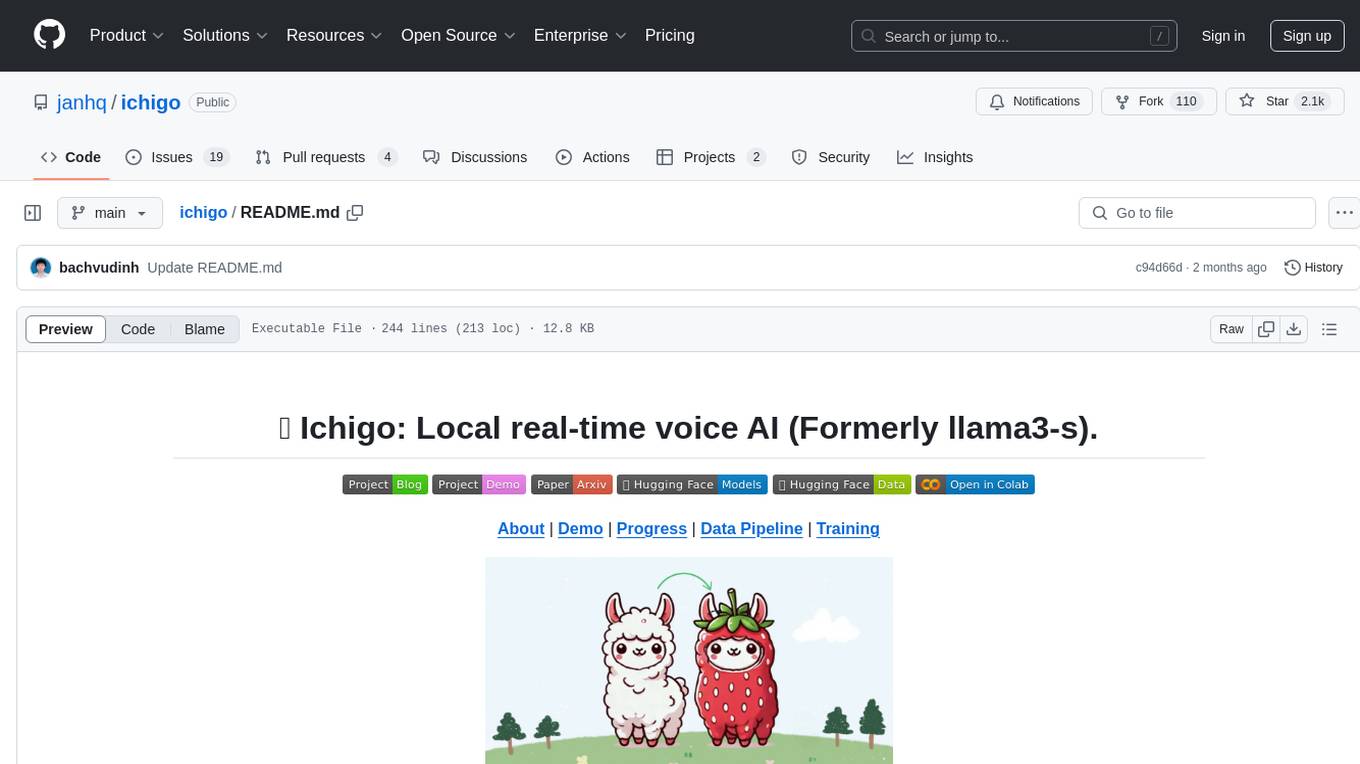
ichigo
Ichigo is a local real-time voice AI tool that uses an early fusion technique to extend a text-based LLM to have native 'listening' ability. It is an open research experiment with improved multiturn capabilities and the ability to refuse processing inaudible queries. The tool is designed for open data, open weight, on-device Siri-like functionality, inspired by Meta's Chameleon paper. Ichigo offers a web UI demo and Gradio web UI for users to interact with the tool. It has achieved enhanced MMLU scores, stronger context handling, advanced noise management, and improved multi-turn capabilities for a robust user experience.
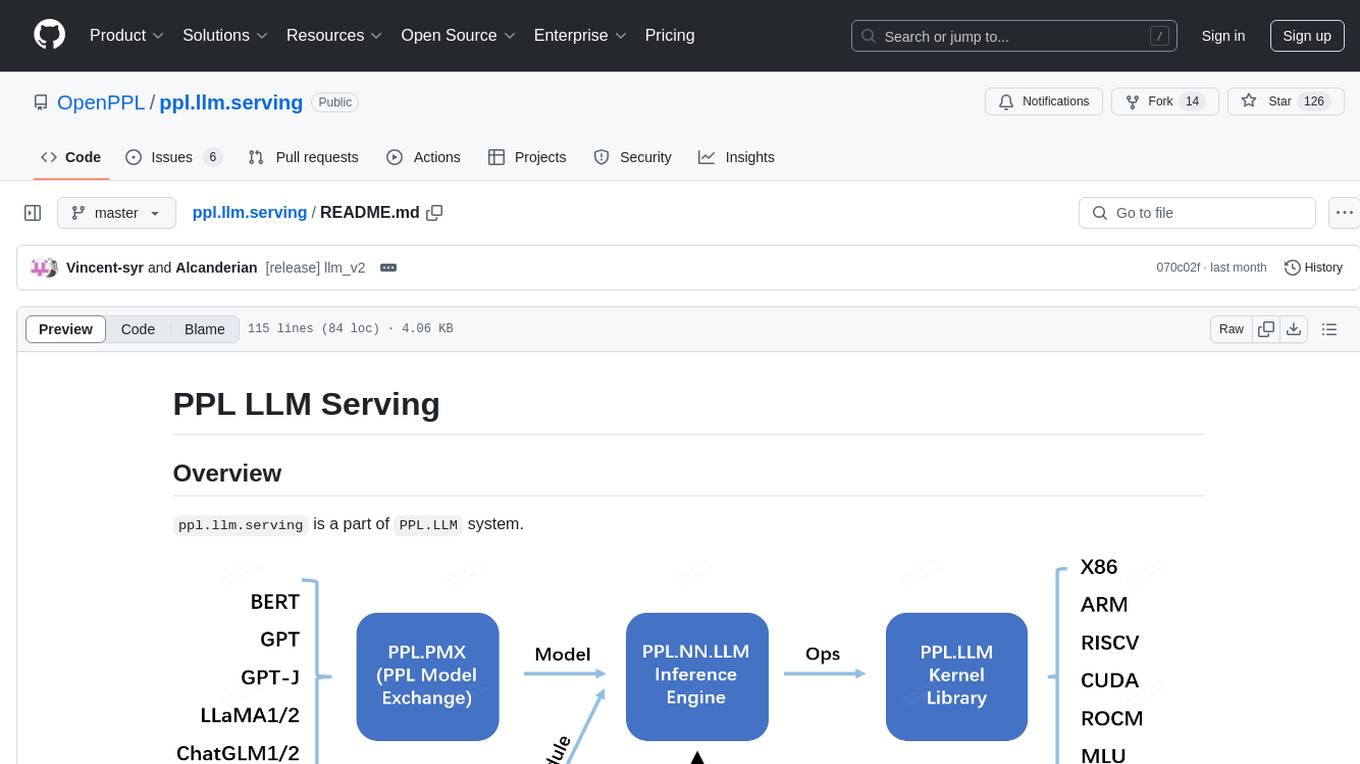
ppl.llm.serving
ppl.llm.serving is a serving component for Large Language Models (LLMs) within the PPL.LLM system. It provides a server based on gRPC and supports inference for LLaMA. The repository includes instructions for prerequisites, quick start guide, model exporting, server setup, client usage, benchmarking, and offline inference. Users can refer to the LLaMA Guide for more details on using this serving component.
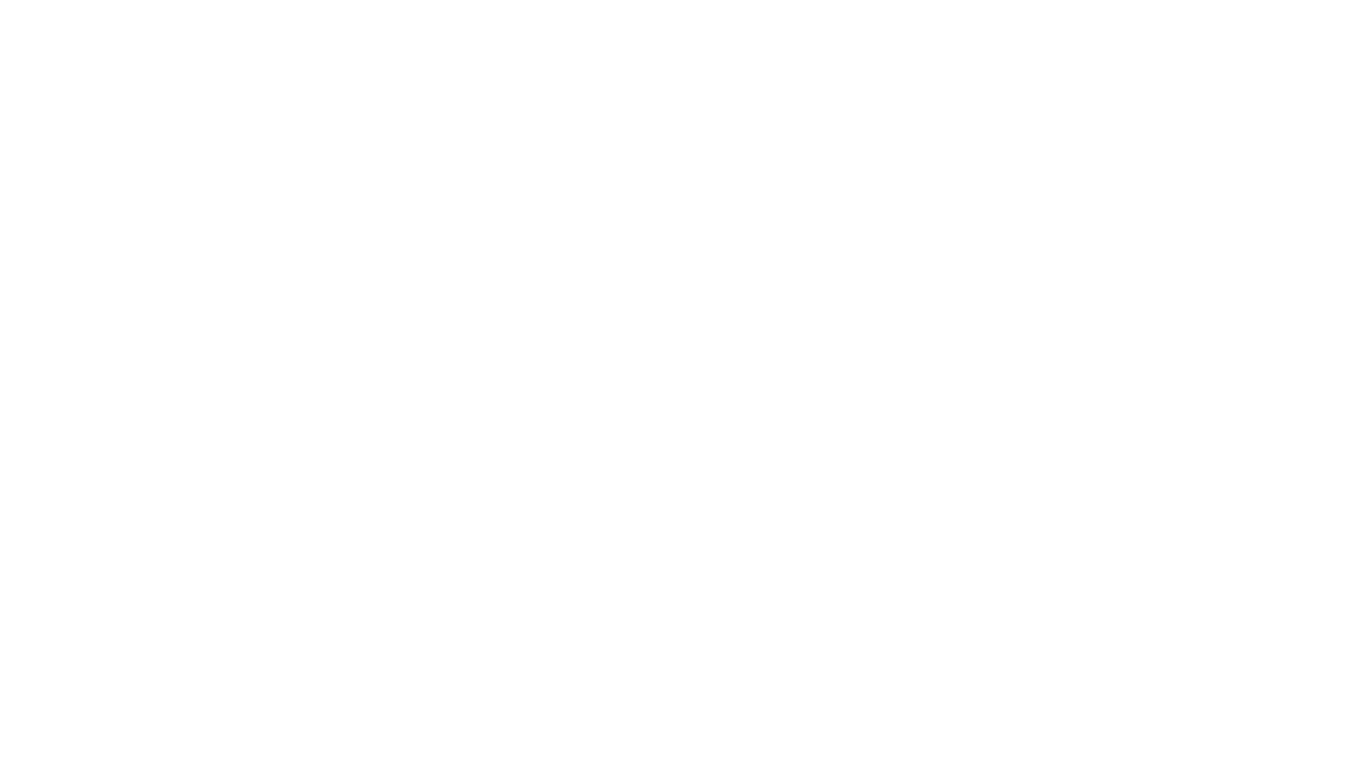
raglite
RAGLite is a Python toolkit for Retrieval-Augmented Generation (RAG) with PostgreSQL or SQLite. It offers configurable options for choosing LLM providers, database types, and rerankers. The toolkit is fast and permissive, utilizing lightweight dependencies and hardware acceleration. RAGLite provides features like PDF to Markdown conversion, multi-vector chunk embedding, optimal semantic chunking, hybrid search capabilities, adaptive retrieval, and improved output quality. It is extensible with a built-in Model Context Protocol server, customizable ChatGPT-like frontend, document conversion to Markdown, and evaluation tools. Users can configure RAGLite for various tasks like configuring, inserting documents, running RAG pipelines, computing query adapters, evaluating performance, running MCP servers, and serving frontends.
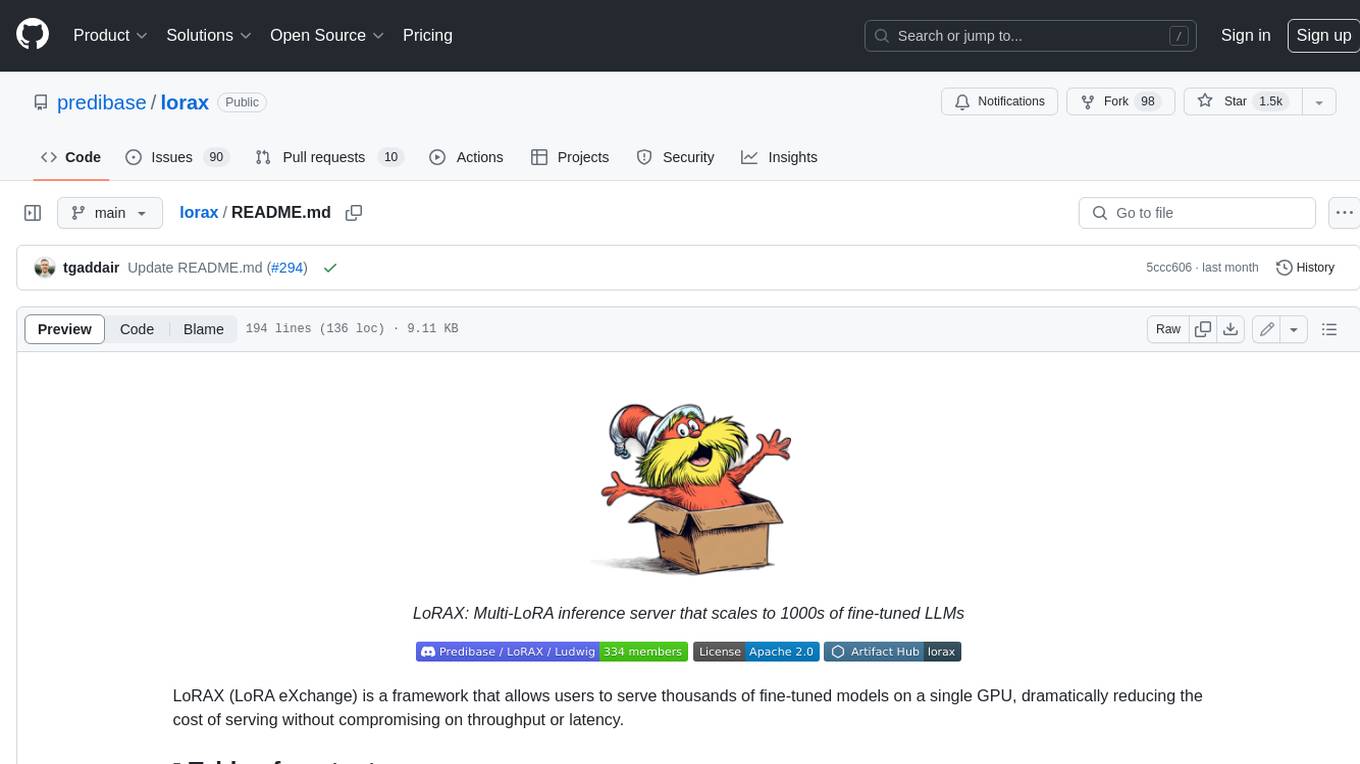
lorax
LoRAX is a framework that allows users to serve thousands of fine-tuned models on a single GPU, dramatically reducing the cost of serving without compromising on throughput or latency. It features dynamic adapter loading, heterogeneous continuous batching, adapter exchange scheduling, optimized inference, and is ready for production with prebuilt Docker images, Helm charts for Kubernetes, Prometheus metrics, and distributed tracing with Open Telemetry. LoRAX supports a number of Large Language Models as the base model including Llama, Mistral, and Qwen, and any of the linear layers in the model can be adapted via LoRA and loaded in LoRAX.
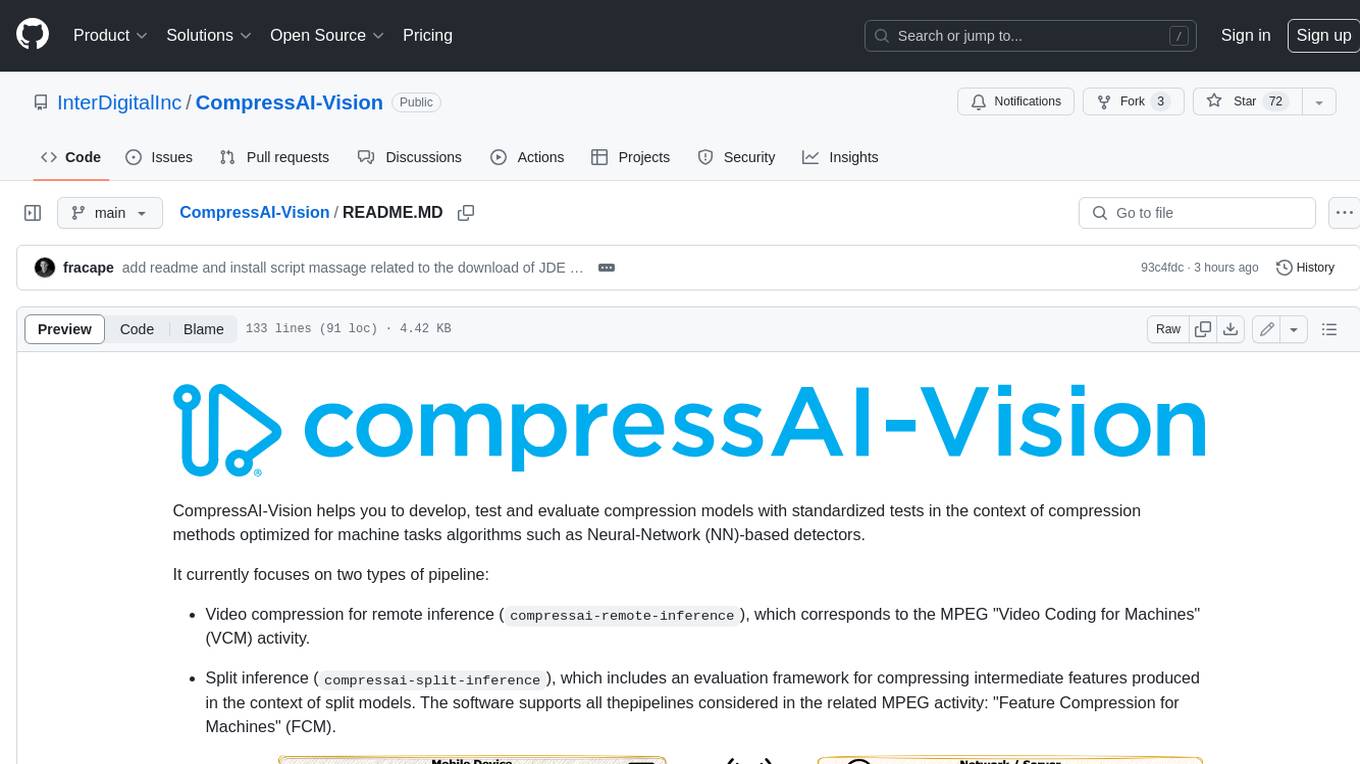
CompressAI-Vision
CompressAI-Vision is a tool that helps you develop, test, and evaluate compression models with standardized tests in the context of compression methods optimized for machine tasks algorithms such as Neural-Network (NN)-based detectors. It currently focuses on two types of pipeline: Video compression for remote inference (`compressai-remote-inference`), which corresponds to the MPEG "Video Coding for Machines" (VCM) activity. Split inference (`compressai-split-inference`), which includes an evaluation framework for compressing intermediate features produced in the context of split models. The software supports all the pipelines considered in the related MPEG activity: "Feature Compression for Machines" (FCM).
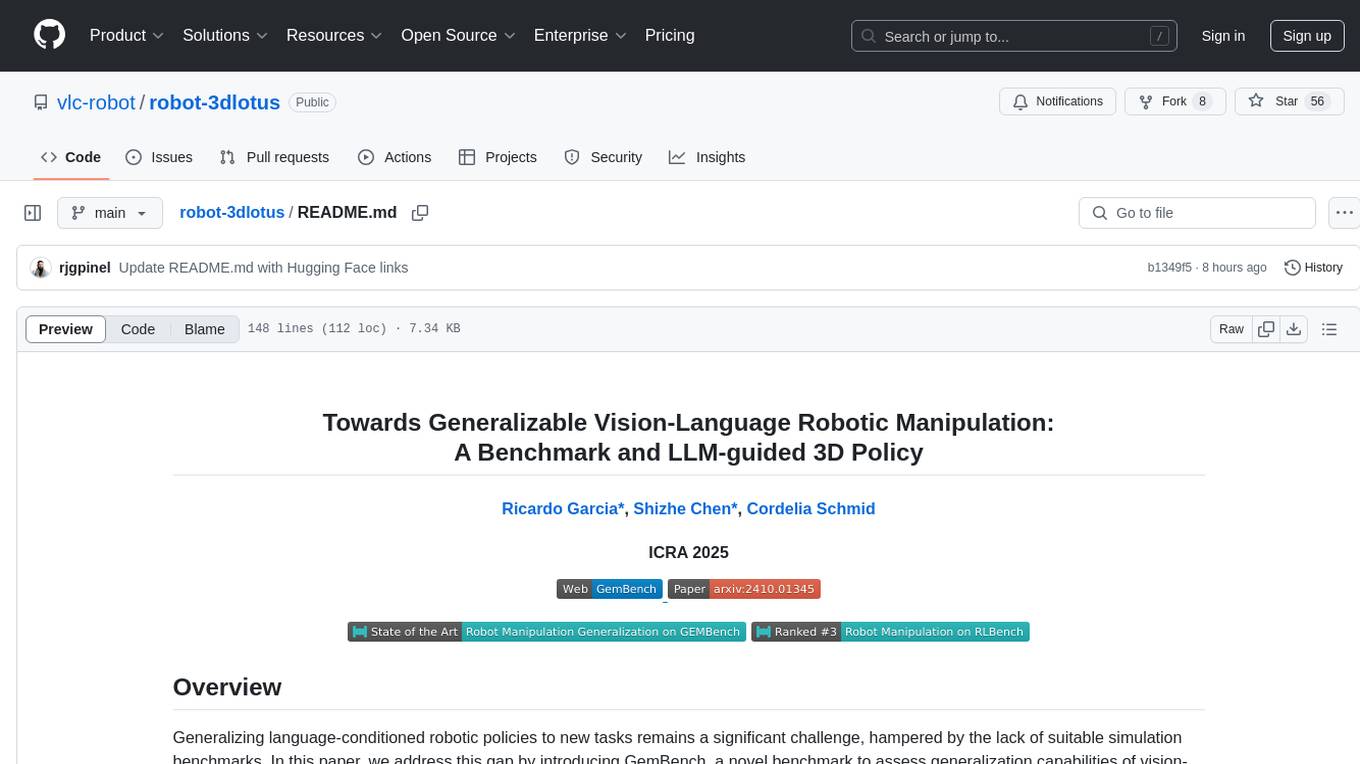
robot-3dlotus
Towards Generalizable Vision-Language Robotic Manipulation: A Benchmark and LLM-guided 3D Policy is a research project focusing on addressing the challenge of generalizing language-conditioned robotic policies to new tasks. The project introduces GemBench, a benchmark to evaluate the generalization capabilities of vision-language robotic manipulation policies. It also presents the 3D-LOTUS approach, which leverages rich 3D information for action prediction conditioned on language. Additionally, the project introduces 3D-LOTUS++, a framework that integrates 3D-LOTUS's motion planning capabilities with the task planning capabilities of LLMs and the object grounding accuracy of VLMs to achieve state-of-the-art performance on novel tasks in robotic manipulation.
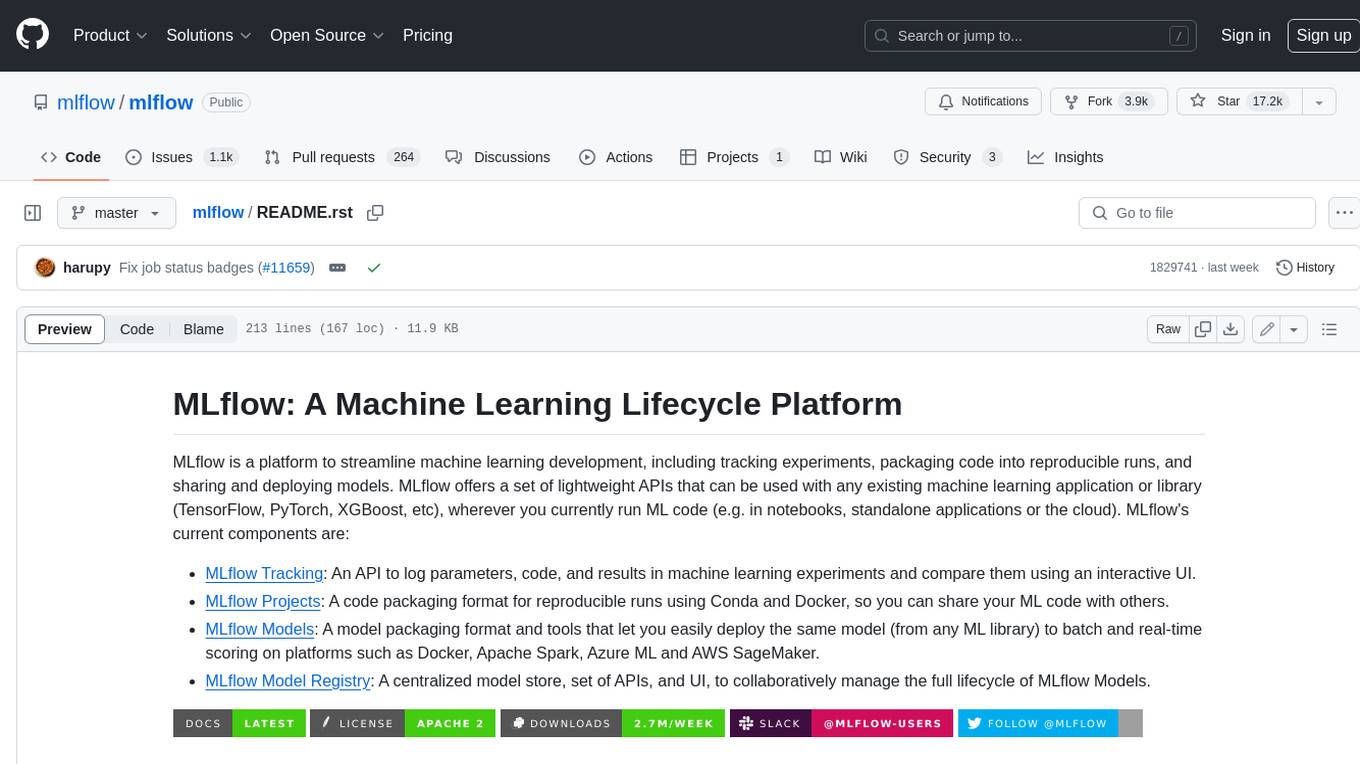
mlflow
MLflow is a platform to streamline machine learning development, including tracking experiments, packaging code into reproducible runs, and sharing and deploying models. MLflow offers a set of lightweight APIs that can be used with any existing machine learning application or library (TensorFlow, PyTorch, XGBoost, etc), wherever you currently run ML code (e.g. in notebooks, standalone applications or the cloud). MLflow's current components are:
* `MLflow Tracking
For similar tasks
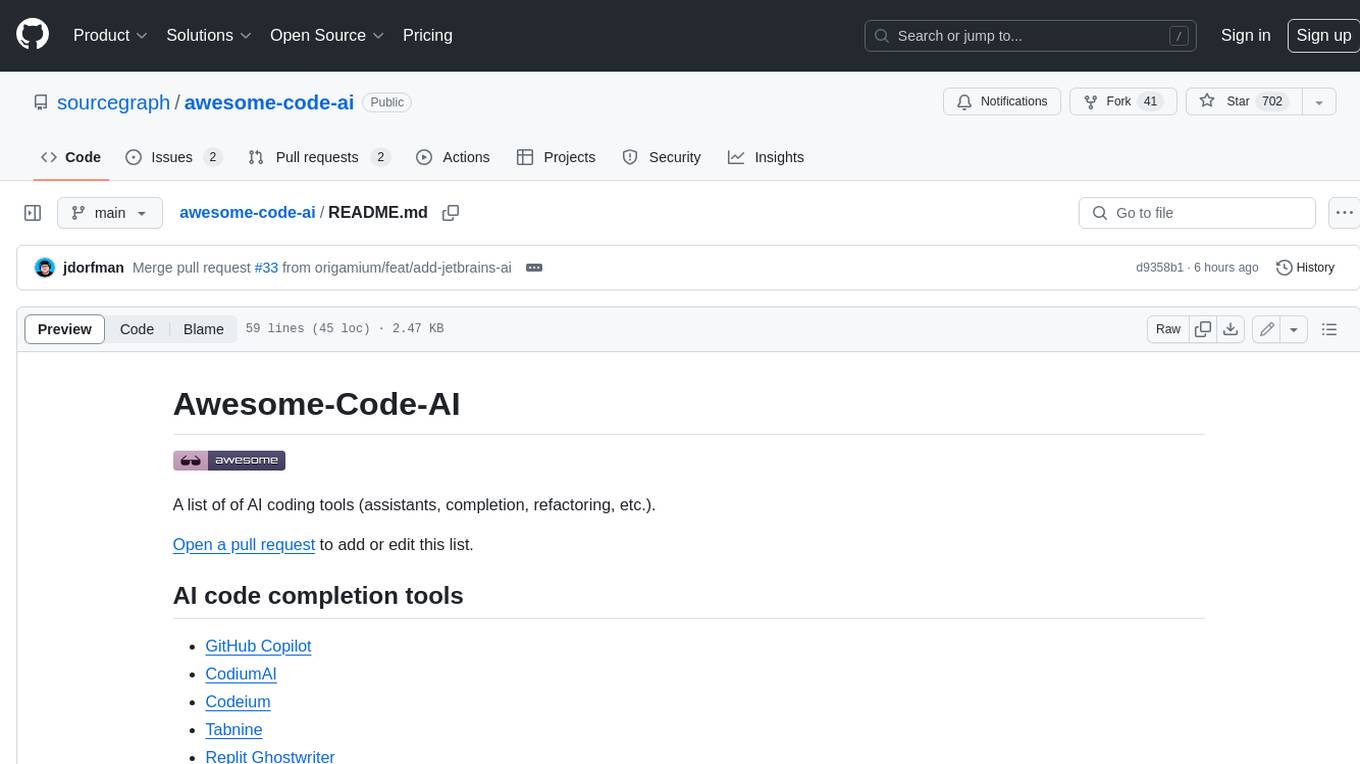
awesome-code-ai
A curated list of AI coding tools, including code completion, refactoring, and assistants. This list includes both open-source and commercial tools, as well as tools that are still in development. Some of the most popular AI coding tools include GitHub Copilot, CodiumAI, Codeium, Tabnine, and Replit Ghostwriter.
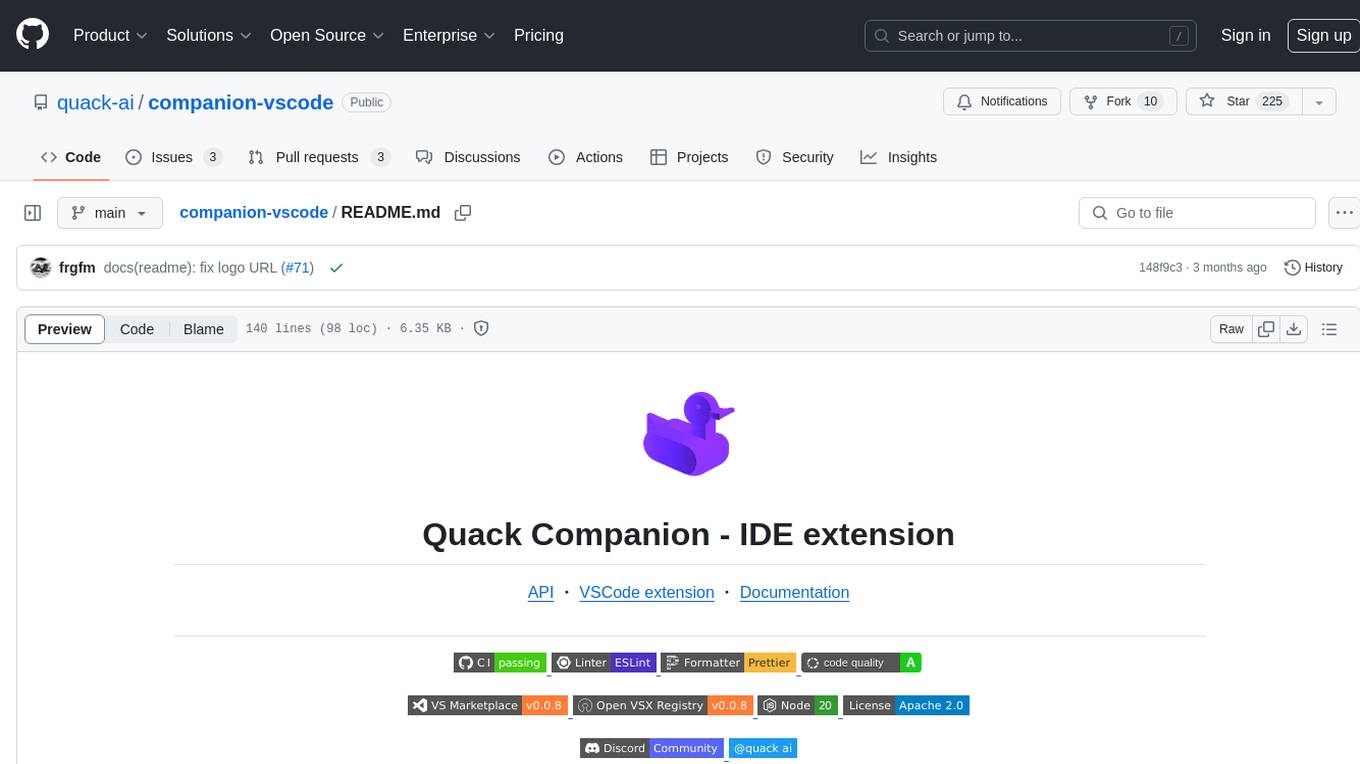
companion-vscode
Quack Companion is a VSCode extension that provides smart linting, code chat, and coding guideline curation for developers. It aims to enhance the coding experience by offering a new tab with features like curating software insights with the team, code chat similar to ChatGPT, smart linting, and upcoming code completion. The extension focuses on creating a smooth contribution experience for developers by turning contribution guidelines into a live pair coding experience, helping developers find starter contribution opportunities, and ensuring alignment between contribution goals and project priorities. Quack collects limited telemetry data to improve its services and products for developers, with options for anonymization and disabling telemetry available to users.
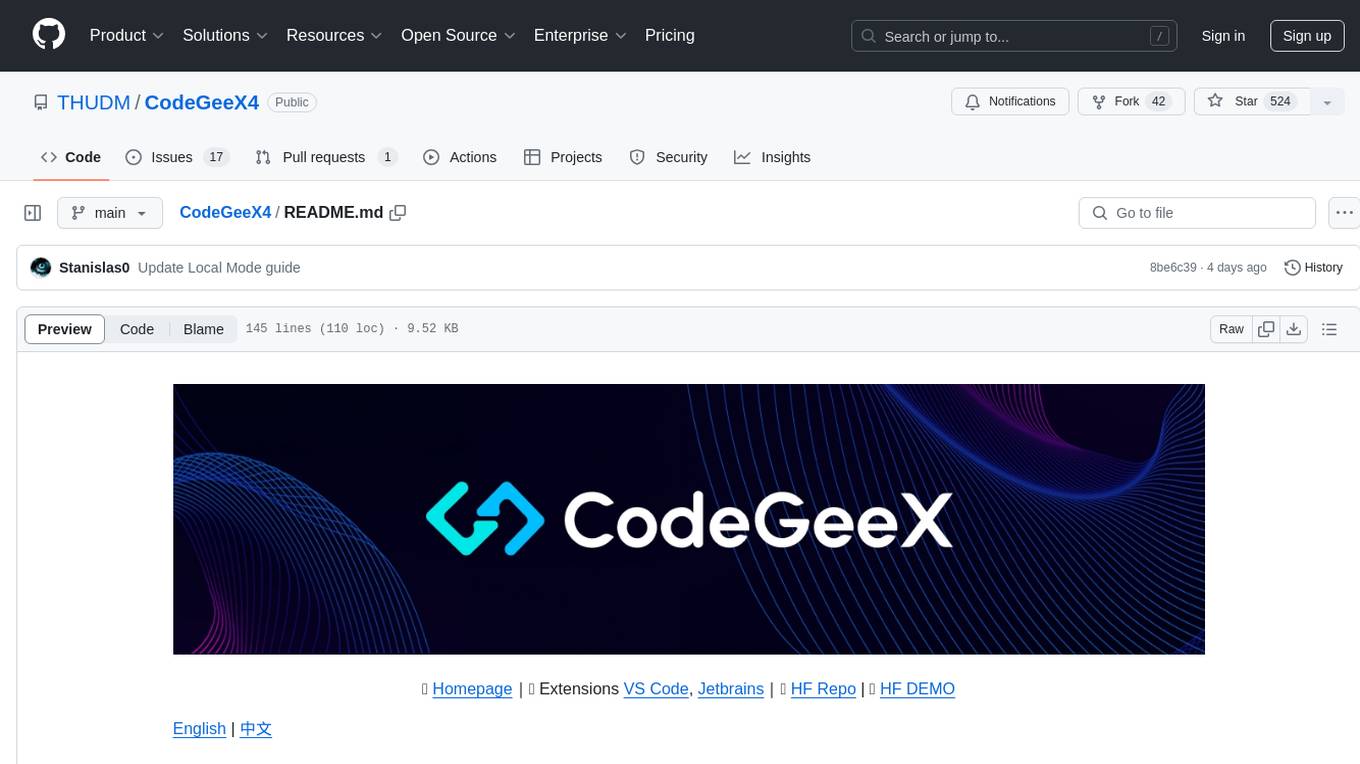
CodeGeeX4
CodeGeeX4-ALL-9B is an open-source multilingual code generation model based on GLM-4-9B, offering enhanced code generation capabilities. It supports functions like code completion, code interpreter, web search, function call, and repository-level code Q&A. The model has competitive performance on benchmarks like BigCodeBench and NaturalCodeBench, outperforming larger models in terms of speed and performance.
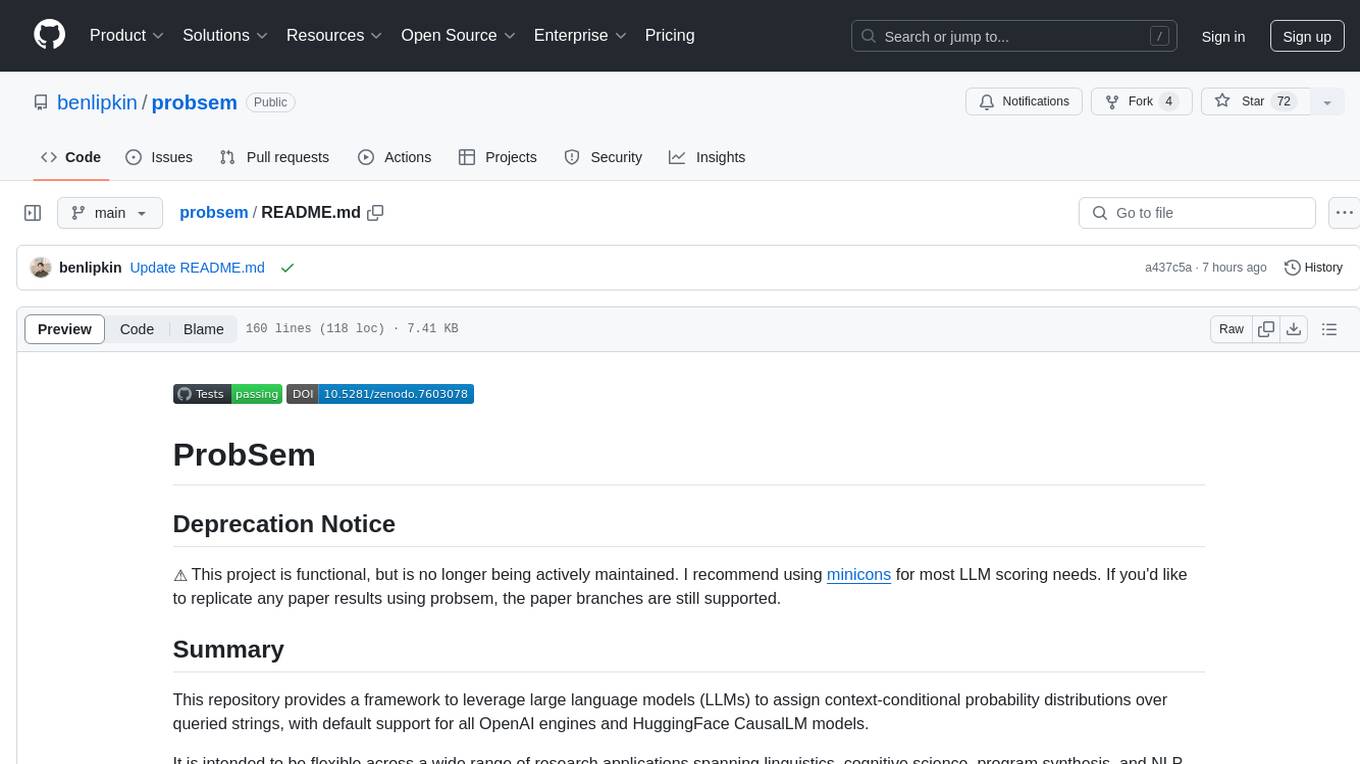
probsem
ProbSem is a repository that provides a framework to leverage large language models (LLMs) for assigning context-conditional probability distributions over queried strings. It supports OpenAI engines and HuggingFace CausalLM models, and is flexible for research applications in linguistics, cognitive science, program synthesis, and NLP. Users can define prompts, contexts, and queries to derive probability distributions over possible completions, enabling tasks like cloze completion, multiple-choice QA, semantic parsing, and code completion. The repository offers CLI and API interfaces for evaluation, with options to customize models, normalize scores, and adjust temperature for probability distributions.
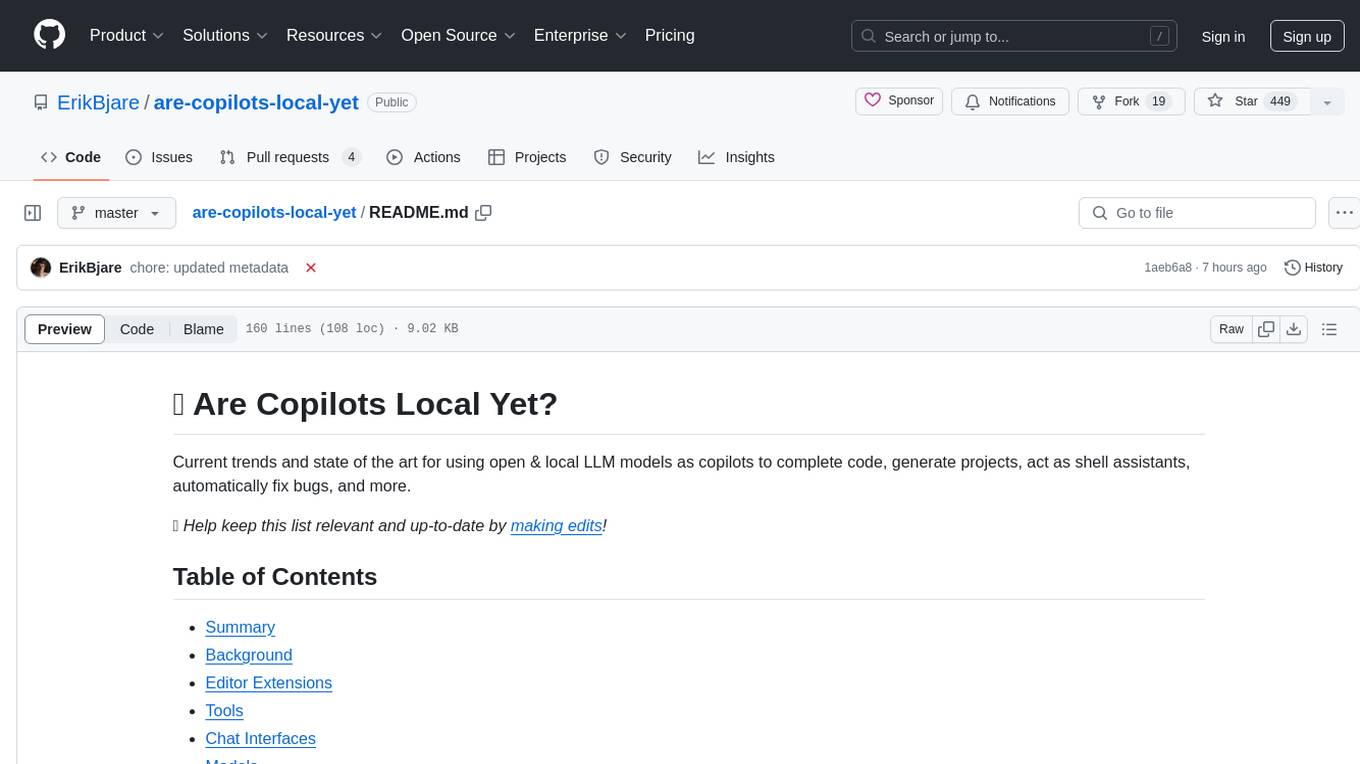
are-copilots-local-yet
Current trends and state of the art for using open & local LLM models as copilots to complete code, generate projects, act as shell assistants, automatically fix bugs, and more. This document is a curated list of local Copilots, shell assistants, and related projects, intended to be a resource for those interested in a survey of the existing tools and to help developers discover the state of the art for projects like these.
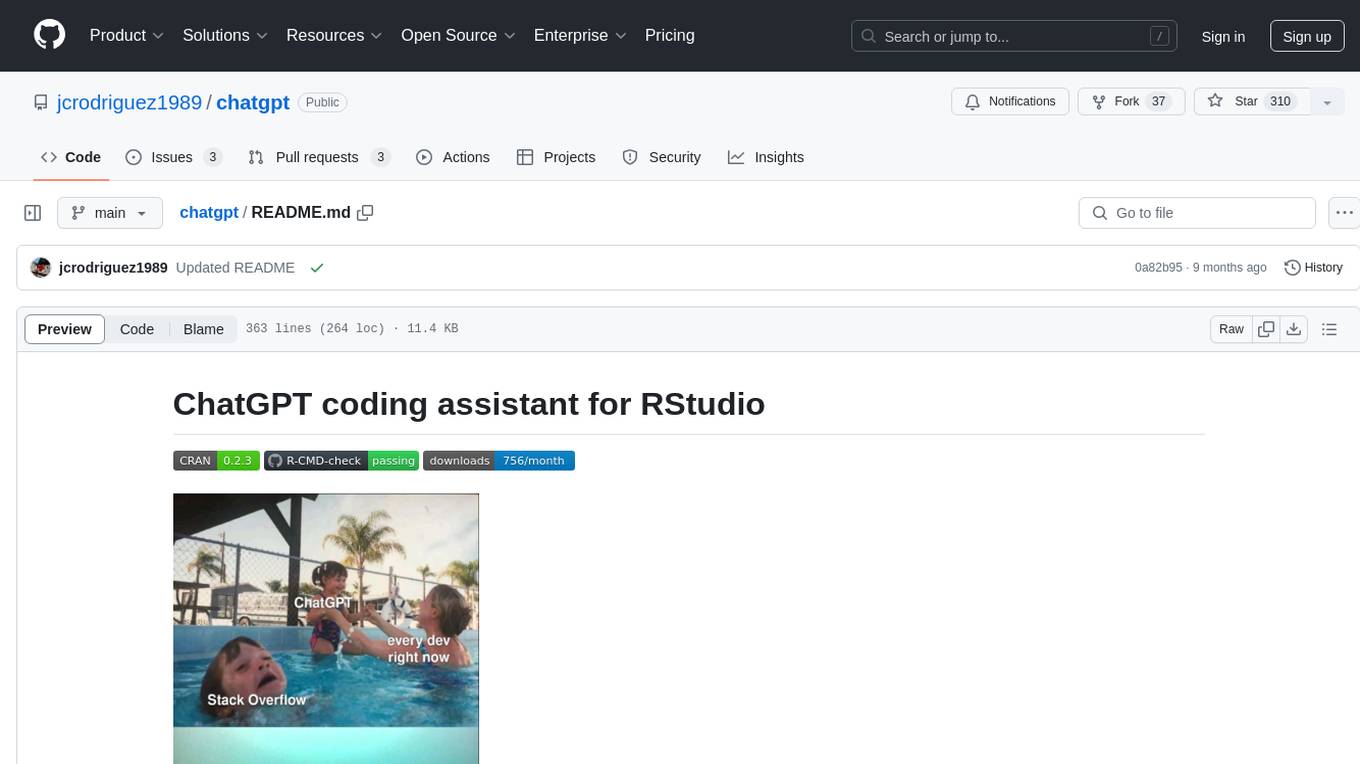
chatgpt
The ChatGPT R package provides a set of features to assist in R coding. It includes addins like Ask ChatGPT, Comment selected code, Complete selected code, Create unit tests, Create variable name, Document code, Explain selected code, Find issues in the selected code, Optimize selected code, and Refactor selected code. Users can interact with ChatGPT to get code suggestions, explanations, and optimizations. The package helps in improving coding efficiency and quality by providing AI-powered assistance within the RStudio environment.
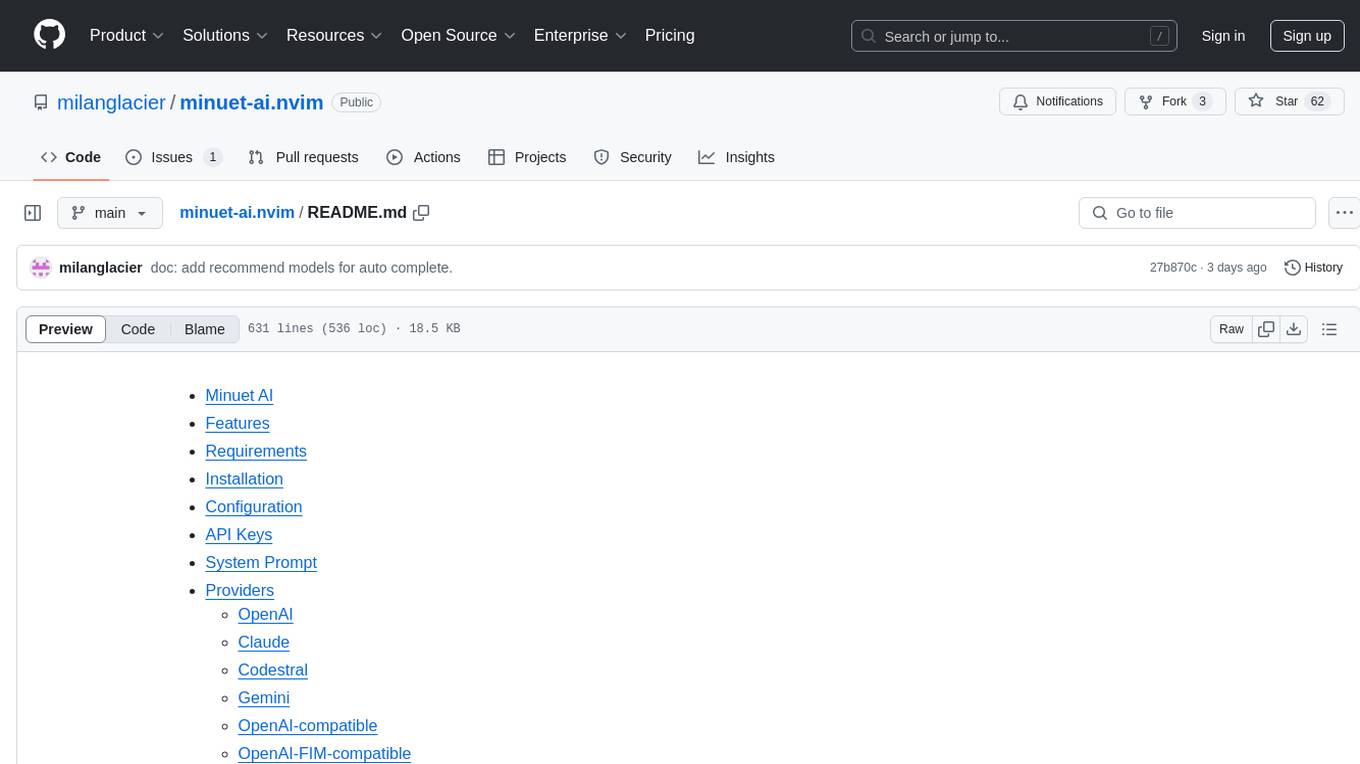
minuet-ai.nvim
Minuet AI is a Neovim plugin that integrates with nvim-cmp to provide AI-powered code completion using multiple AI providers such as OpenAI, Claude, Gemini, Codestral, and Huggingface. It offers customizable configuration options and streaming support for completion delivery. Users can manually invoke completion or use cost-effective models for auto-completion. The plugin requires API keys for supported AI providers and allows customization of system prompts. Minuet AI also supports changing providers, toggling auto-completion, and provides solutions for input delay issues. Integration with lazyvim is possible, and future plans include implementing RAG on the codebase and virtual text UI support.
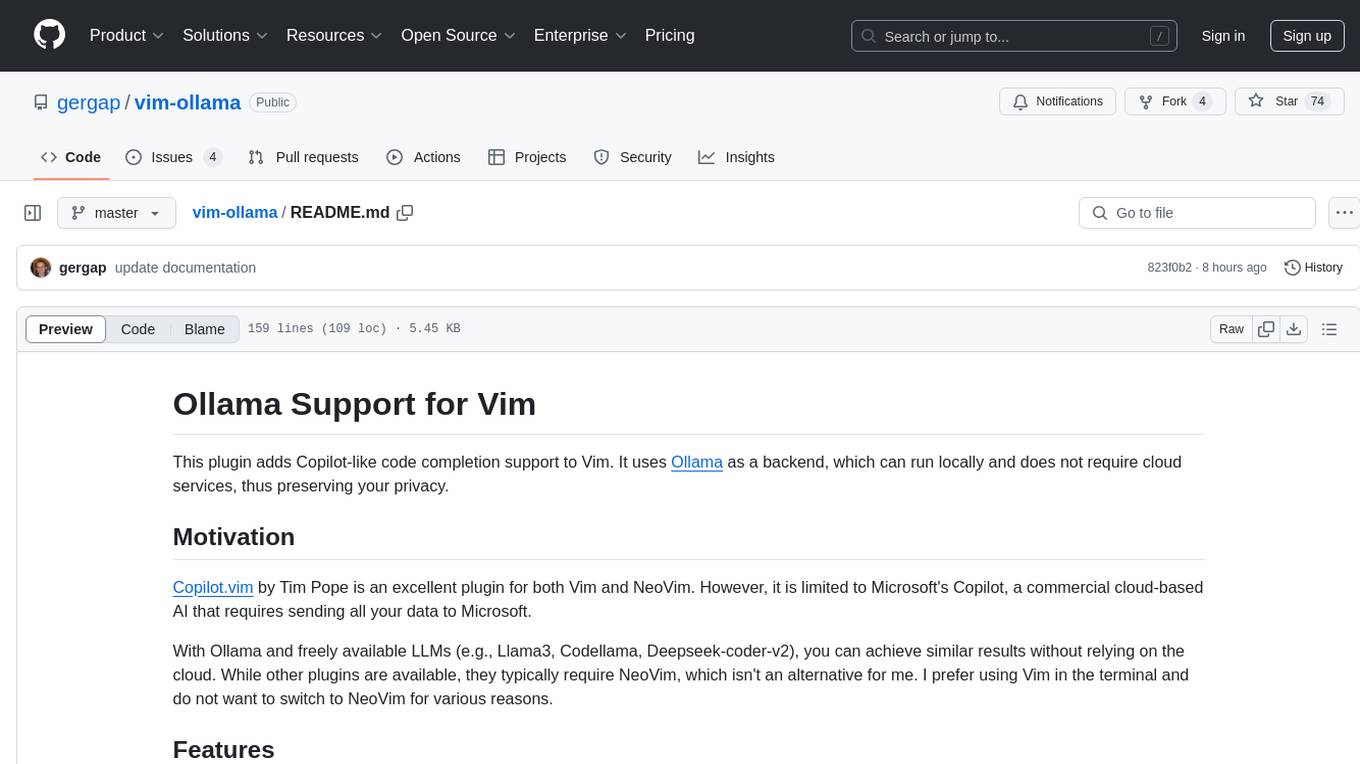
vim-ollama
The 'vim-ollama' plugin for Vim adds Copilot-like code completion support using Ollama as a backend, enabling intelligent AI-based code completion and integrated chat support for code reviews. It does not rely on cloud services, preserving user privacy. The plugin communicates with Ollama via Python scripts for code completion and interactive chat, supporting Vim only. Users can configure LLM models for code completion tasks and interactive conversations, with detailed installation and usage instructions provided in the README.
For similar jobs
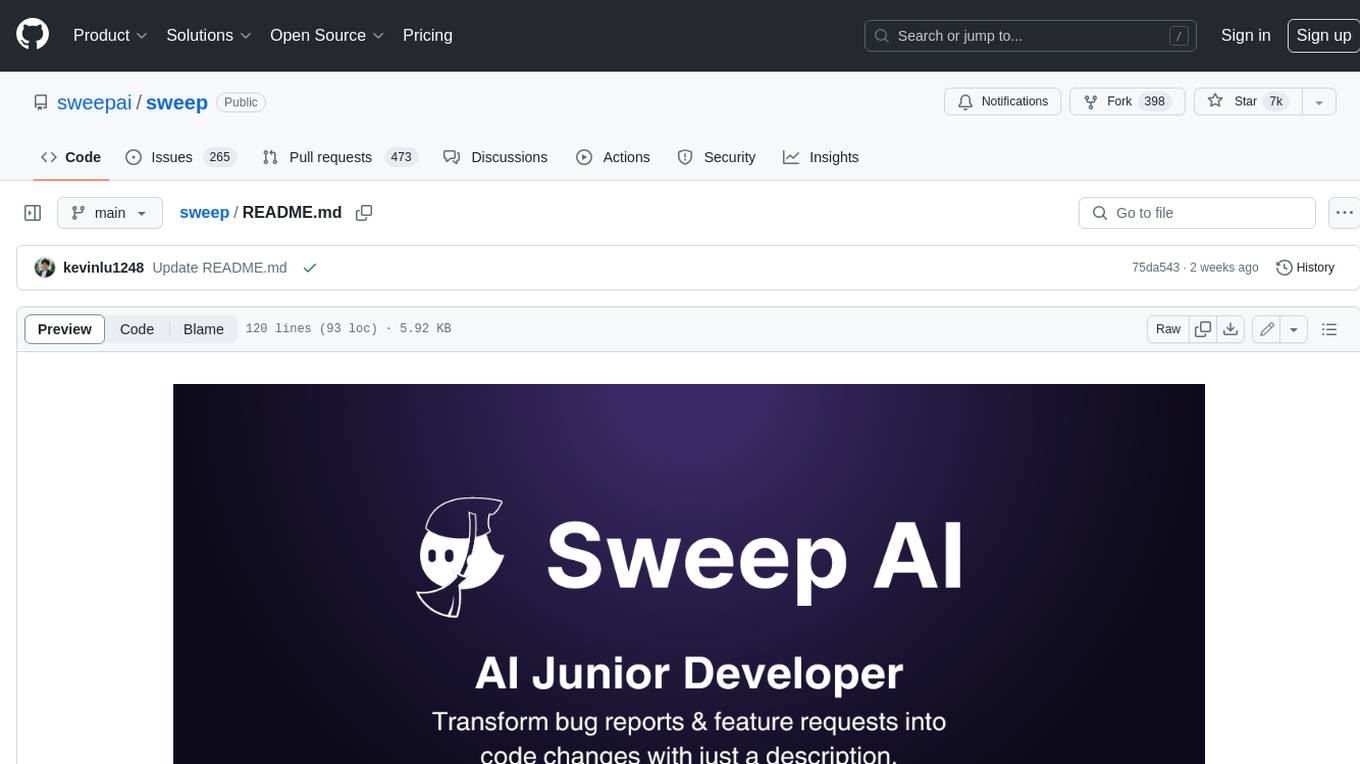
sweep
Sweep is an AI junior developer that turns bugs and feature requests into code changes. It automatically handles developer experience improvements like adding type hints and improving test coverage.
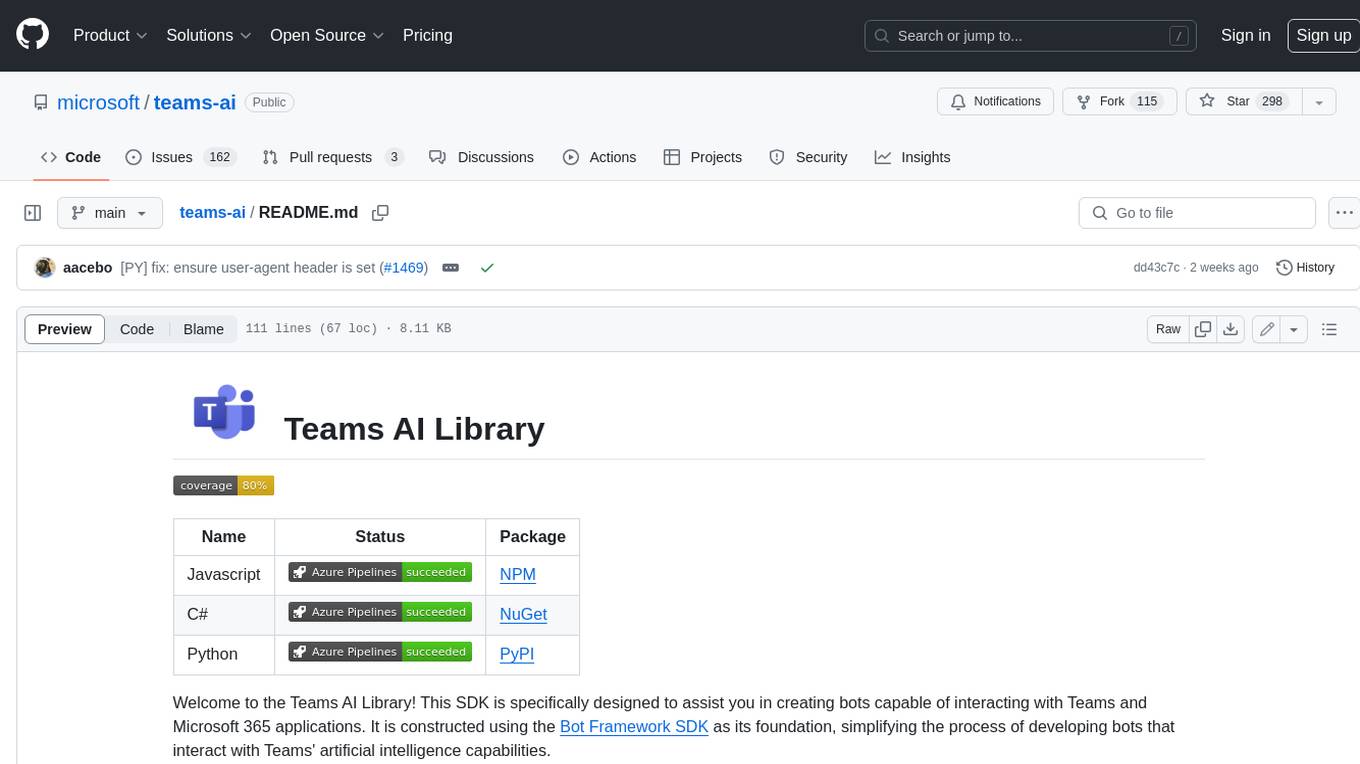
teams-ai
The Teams AI Library is a software development kit (SDK) that helps developers create bots that can interact with Teams and Microsoft 365 applications. It is built on top of the Bot Framework SDK and simplifies the process of developing bots that interact with Teams' artificial intelligence capabilities. The SDK is available for JavaScript/TypeScript, .NET, and Python.
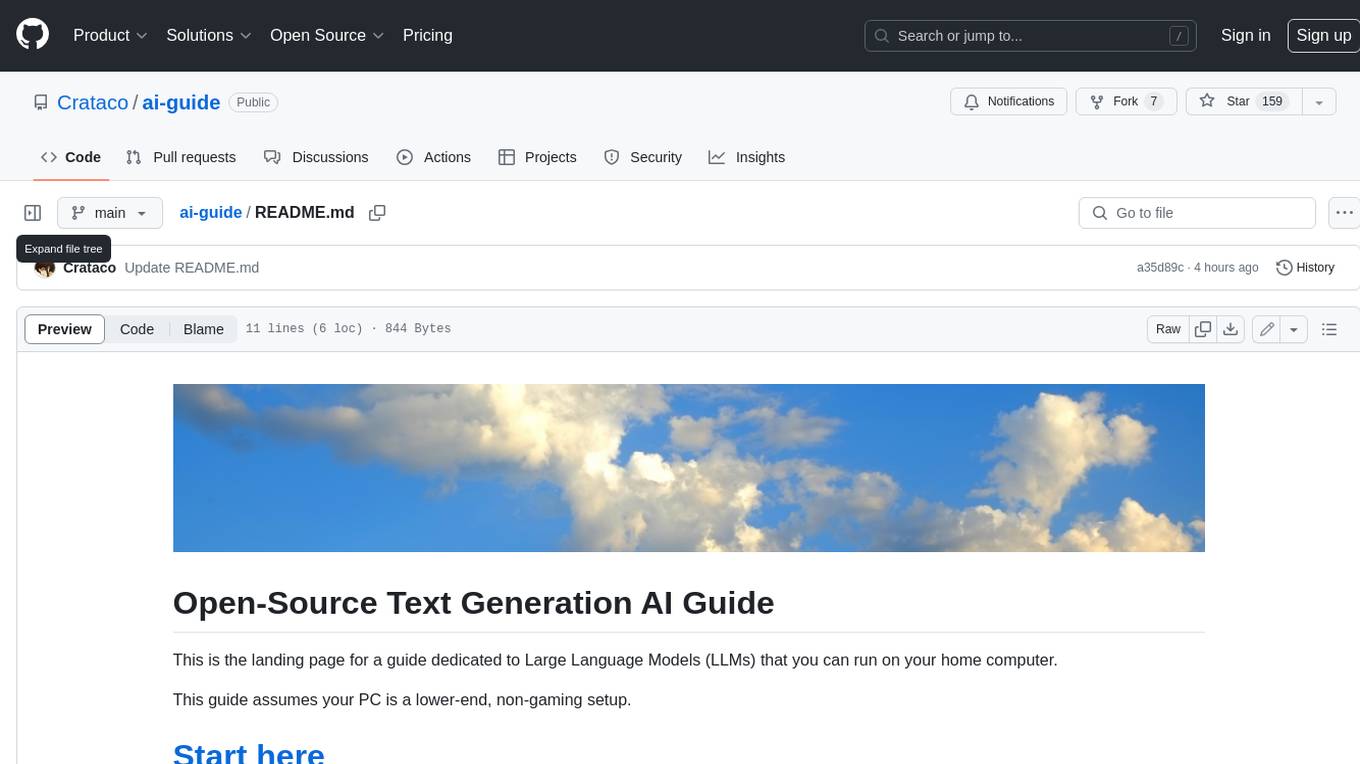
ai-guide
This guide is dedicated to Large Language Models (LLMs) that you can run on your home computer. It assumes your PC is a lower-end, non-gaming setup.
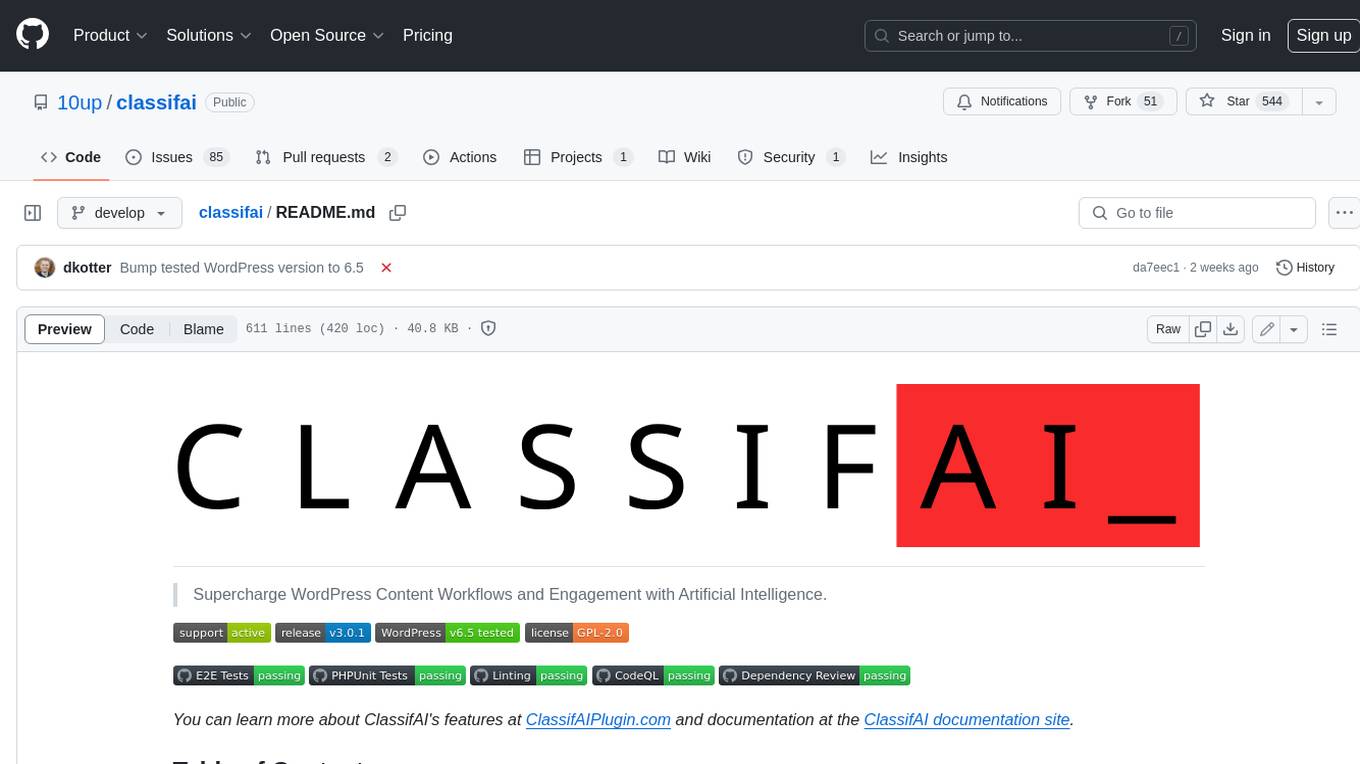
classifai
Supercharge WordPress Content Workflows and Engagement with Artificial Intelligence. Tap into leading cloud-based services like OpenAI, Microsoft Azure AI, Google Gemini and IBM Watson to augment your WordPress-powered websites. Publish content faster while improving SEO performance and increasing audience engagement. ClassifAI integrates Artificial Intelligence and Machine Learning technologies to lighten your workload and eliminate tedious tasks, giving you more time to create original content that matters.
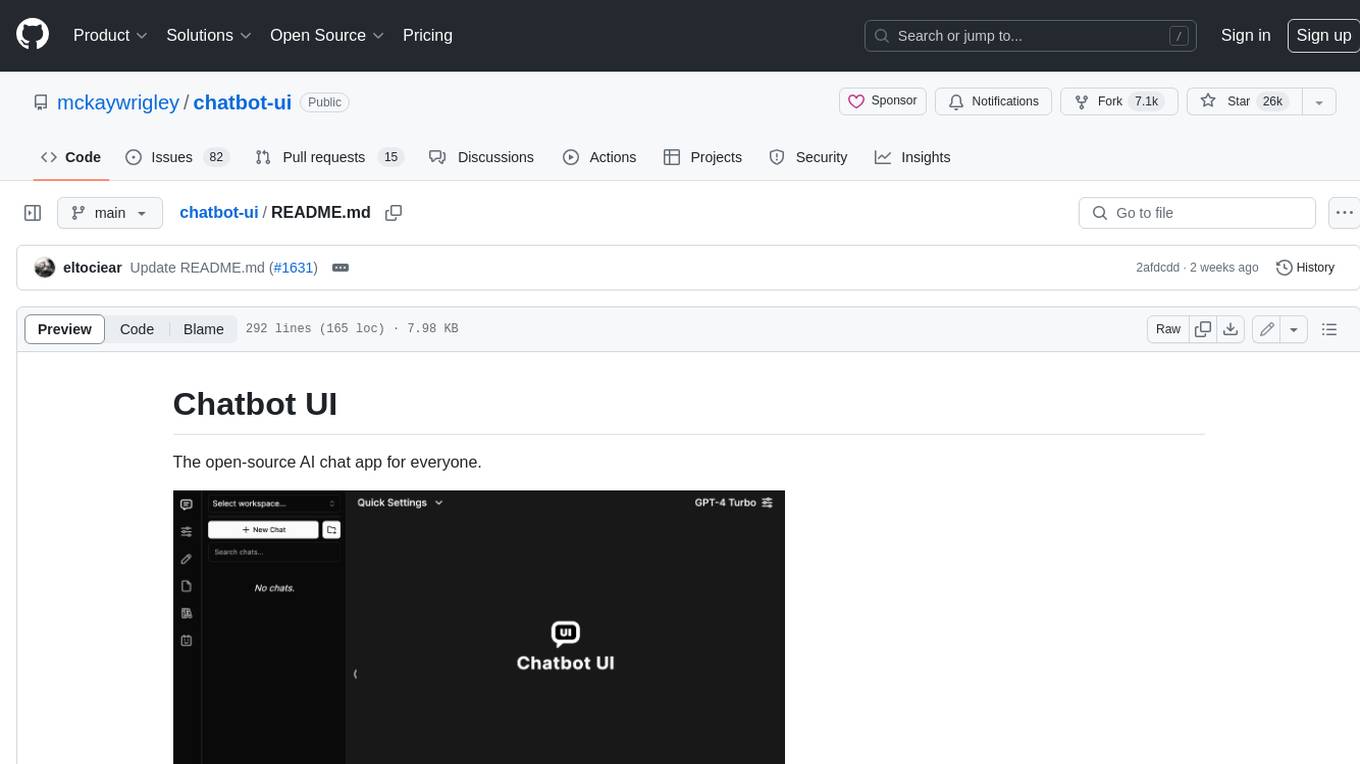
chatbot-ui
Chatbot UI is an open-source AI chat app that allows users to create and deploy their own AI chatbots. It is easy to use and can be customized to fit any need. Chatbot UI is perfect for businesses, developers, and anyone who wants to create a chatbot.
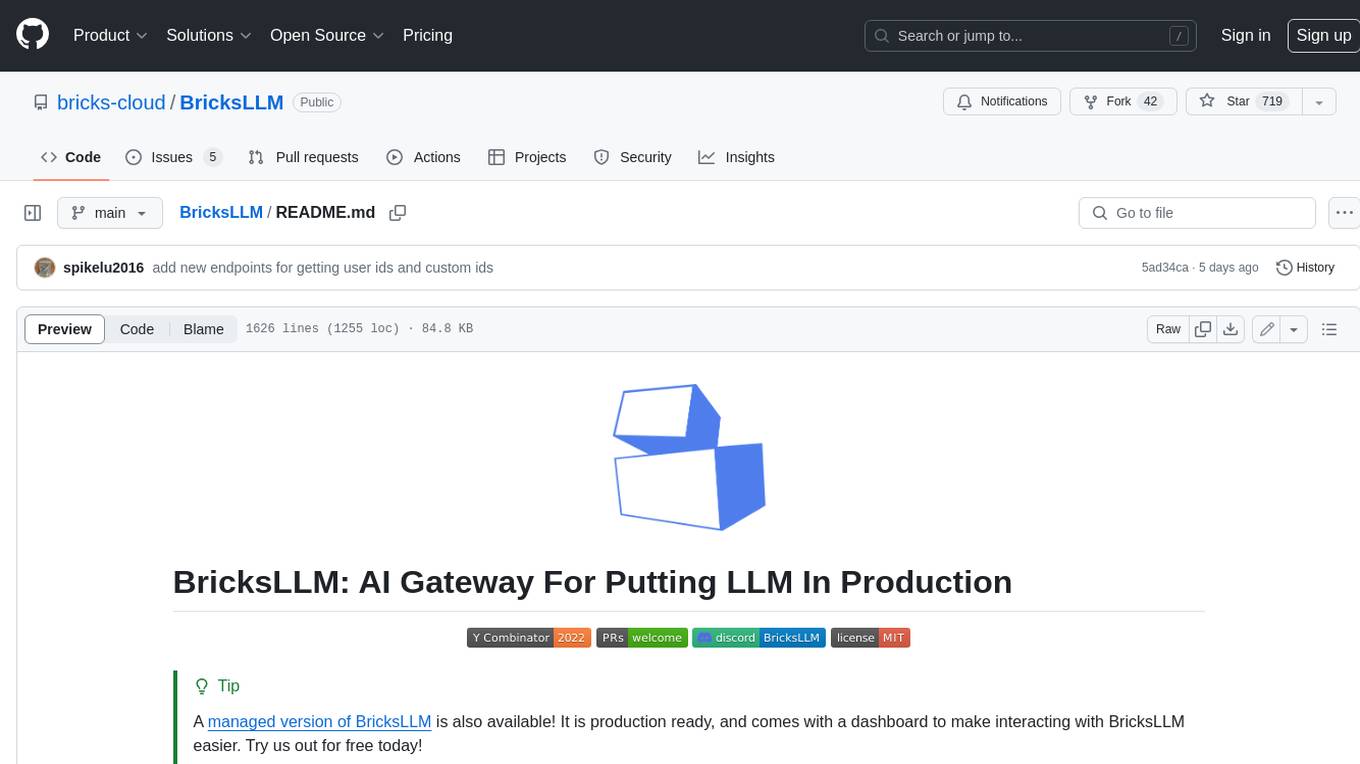
BricksLLM
BricksLLM is a cloud native AI gateway written in Go. Currently, it provides native support for OpenAI, Anthropic, Azure OpenAI and vLLM. BricksLLM aims to provide enterprise level infrastructure that can power any LLM production use cases. Here are some use cases for BricksLLM: * Set LLM usage limits for users on different pricing tiers * Track LLM usage on a per user and per organization basis * Block or redact requests containing PIIs * Improve LLM reliability with failovers, retries and caching * Distribute API keys with rate limits and cost limits for internal development/production use cases * Distribute API keys with rate limits and cost limits for students
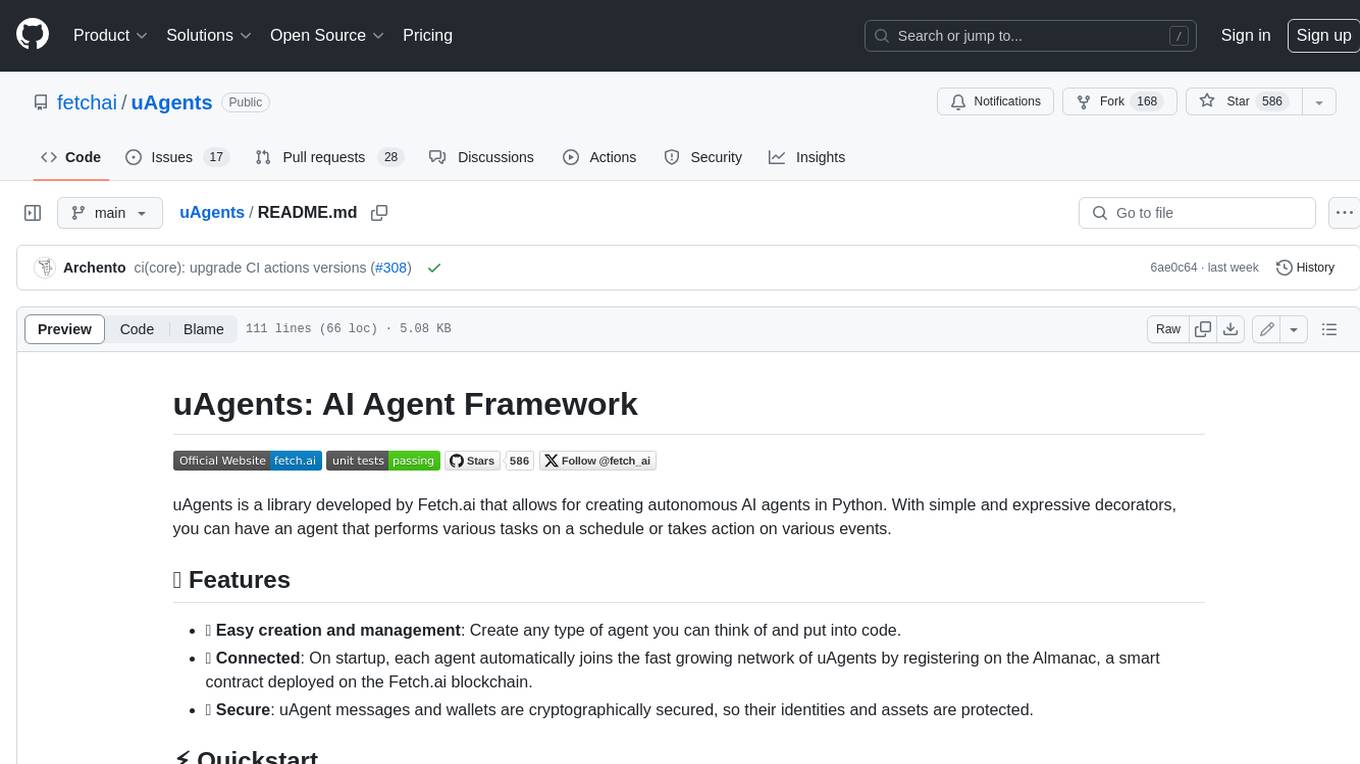
uAgents
uAgents is a Python library developed by Fetch.ai that allows for the creation of autonomous AI agents. These agents can perform various tasks on a schedule or take action on various events. uAgents are easy to create and manage, and they are connected to a fast-growing network of other uAgents. They are also secure, with cryptographically secured messages and wallets.
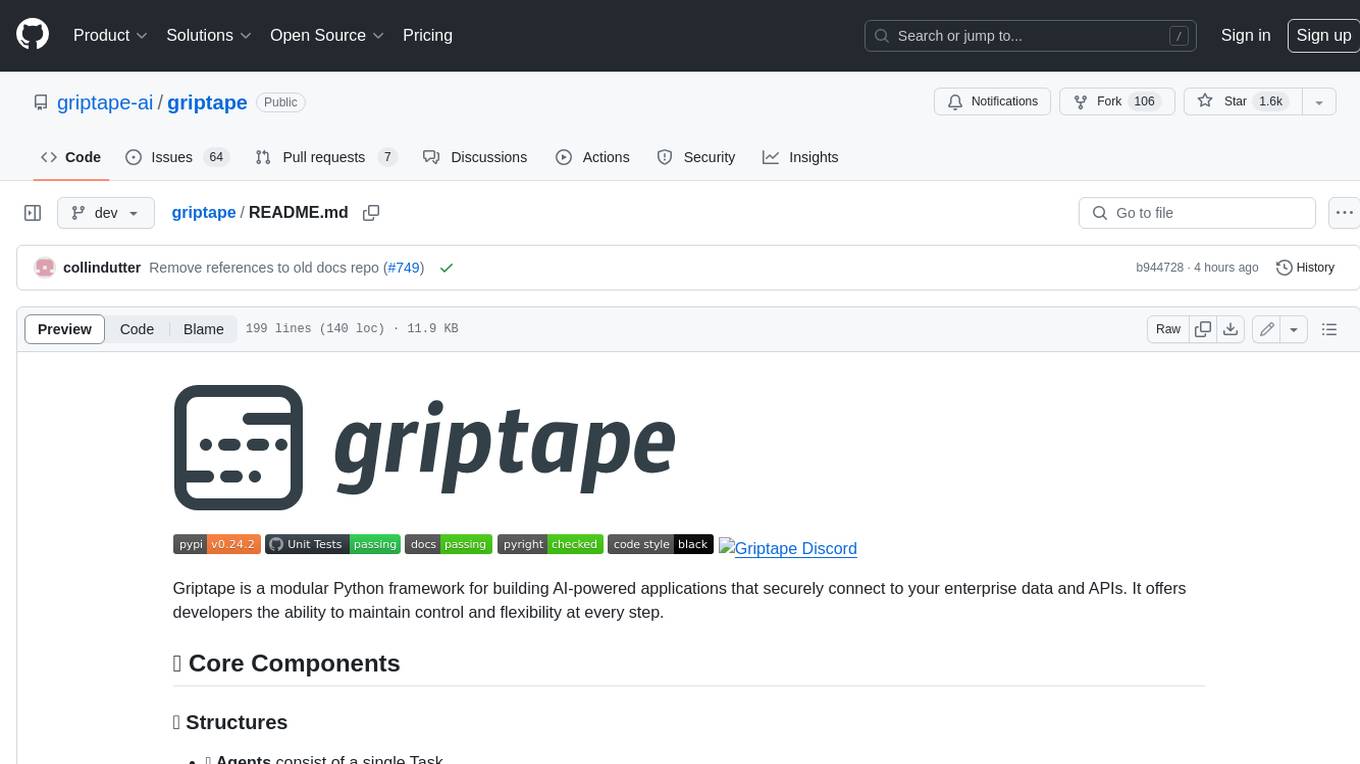
griptape
Griptape is a modular Python framework for building AI-powered applications that securely connect to your enterprise data and APIs. It offers developers the ability to maintain control and flexibility at every step. Griptape's core components include Structures (Agents, Pipelines, and Workflows), Tasks, Tools, Memory (Conversation Memory, Task Memory, and Meta Memory), Drivers (Prompt and Embedding Drivers, Vector Store Drivers, Image Generation Drivers, Image Query Drivers, SQL Drivers, Web Scraper Drivers, and Conversation Memory Drivers), Engines (Query Engines, Extraction Engines, Summary Engines, Image Generation Engines, and Image Query Engines), and additional components (Rulesets, Loaders, Artifacts, Chunkers, and Tokenizers). Griptape enables developers to create AI-powered applications with ease and efficiency.