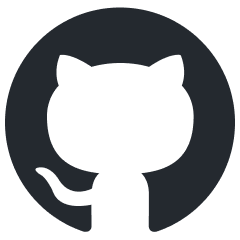
next-token-prediction
Next-token prediction in JavaScript — build fast language and diffusion models.
Stars: 135
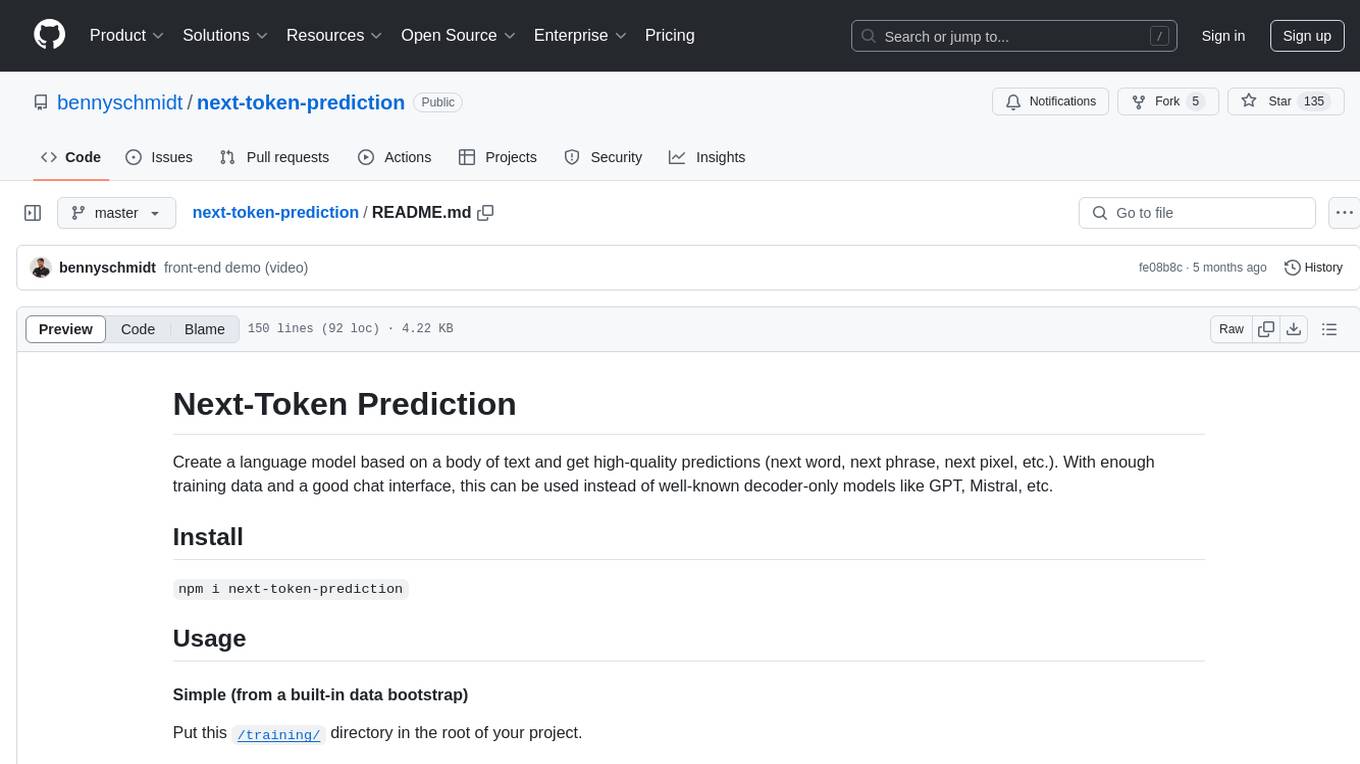
Next-Token Prediction is a language model tool that allows users to create high-quality predictions for the next word, phrase, or pixel based on a body of text. It can be used as an alternative to well-known decoder-only models like GPT and Mistral. The tool provides options for simple usage with built-in data bootstrap or advanced customization by providing training data or creating it from .txt files. It aims to simplify methodologies, provide autocomplete, autocorrect, spell checking, search/lookup functionalities, and create pixel and audio transformers for various prediction formats.
README:
Create a language model based on a body of text and get high-quality predictions (next word, next phrase, next pixel, etc.). With enough training data and a good chat interface, this can be used instead of well-known decoder-only models like GPT, Mistral, etc.
npm i next-token-prediction
Put this /training/
directory in the root of your project.
Now you just need to create your app's index.js
file and run it. Your model will start training on the .txt files located in /training/documents/
. After training is complete it will run these 4 queries:
const { Language: LM } = require('next-token-prediction');
const MyLanguageModel = async () => {
const agent = await LM({
bootstrap: true
});
// Predict the next word
agent.getTokenPrediction('what');
// Predict the next 5 words
agent.getTokenSequencePrediction('what is', 5);
// Complete the phrase
agent.complete('hopefully');
// Get a top k sample of completion predictions
agent.getCompletions('The sun');
};
MyLanguageModel();
Put this /training/
directory in the root of your project.
Because training data was committed to this repo, you can optionally skip training, and just use the bootstrapped training data, like this:
const { dirname } = require('path');
const __root = dirname(require.main.filename);
const { Language: LM } = require('next-token-prediction');
const OpenSourceBooksDataset = require(`${__root}/training/datasets/OpenSourceBooks`);
const MyLanguageModel = async () => {
const agent = await LM({
dataset: OpenSourceBooksDataset
});
// Complete the phrase
agent.complete('hopefully');
};
MyLanguageModel();
Or, train on your own provided text files:
const { dirname } = require('path');
const __root = dirname(require.main.filename);
const { Language: LM } = require('next-token-prediction');
const MyLanguageModel = () => {
// The following .txt files should exist in a `/training/documents/`
// directory in the root of your project
const agent = await LM({
files: [
'marie-antoinette',
'pride-and-prejudice',
'to-kill-a-mockingbird',
'basic-algebra',
'a-history-of-war',
'introduction-to-c-programming'
]
});
// Complete the phrase
agent.complete('hopefully');
};
MyLanguageModel();
Run tests
npm test
With more training data you can get more suggestions, eventually hitting a tipping point where it can complete anything.
3Blue1Brown video on YouTube:
Watch: YouTube
- Provide a high-quality text prediction library for:
- autocomplete
- autocorrect
- spell checking
- search/lookup
-
Create pixel and audio transformers for other prediction formats
-
Demystify LLMs & simplify methodologies
-
Make a high-quality, free/open chat-focused LLM in JavaScript, and an equally sophisticated image-focused diffusion model. Working on this here.
For Tasks:
Click tags to check more tools for each tasksFor Jobs:
Alternative AI tools for next-token-prediction
Similar Open Source Tools
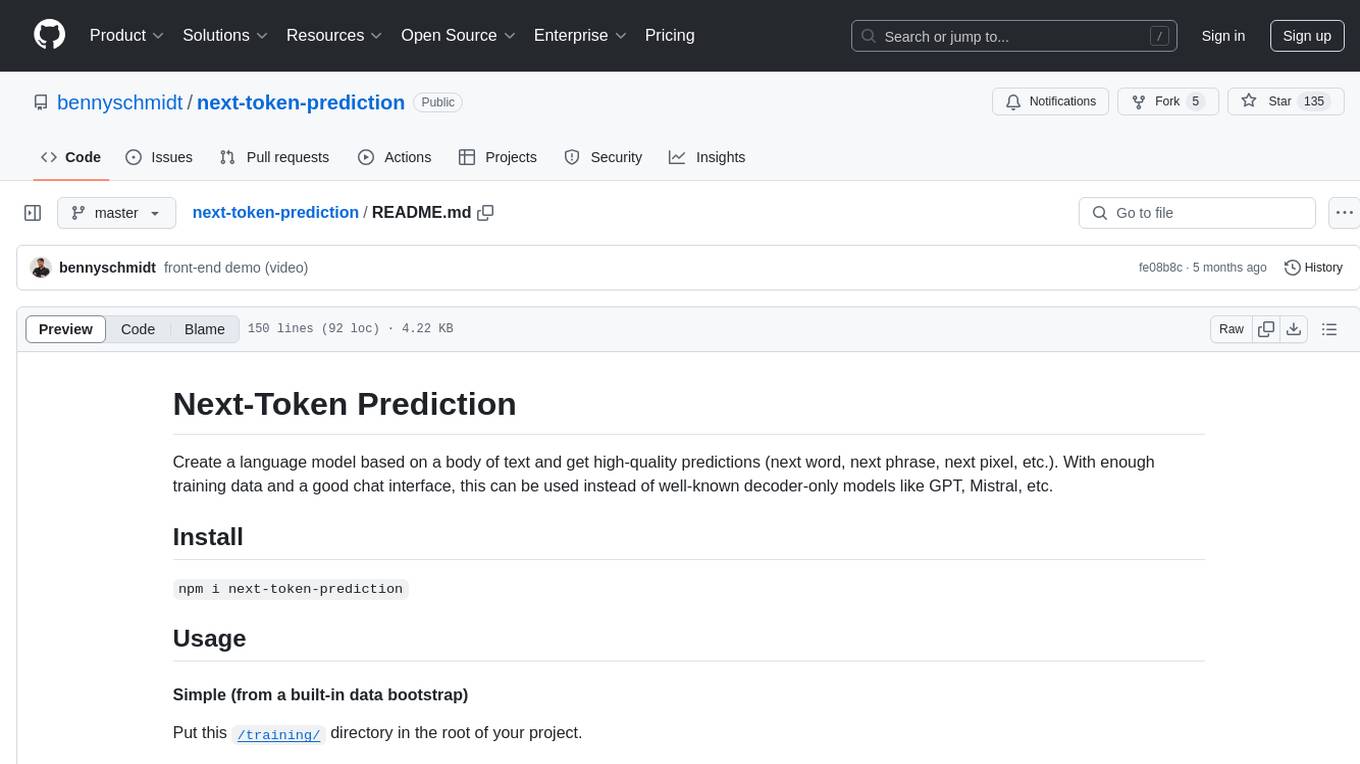
next-token-prediction
Next-Token Prediction is a language model tool that allows users to create high-quality predictions for the next word, phrase, or pixel based on a body of text. It can be used as an alternative to well-known decoder-only models like GPT and Mistral. The tool provides options for simple usage with built-in data bootstrap or advanced customization by providing training data or creating it from .txt files. It aims to simplify methodologies, provide autocomplete, autocorrect, spell checking, search/lookup functionalities, and create pixel and audio transformers for various prediction formats.
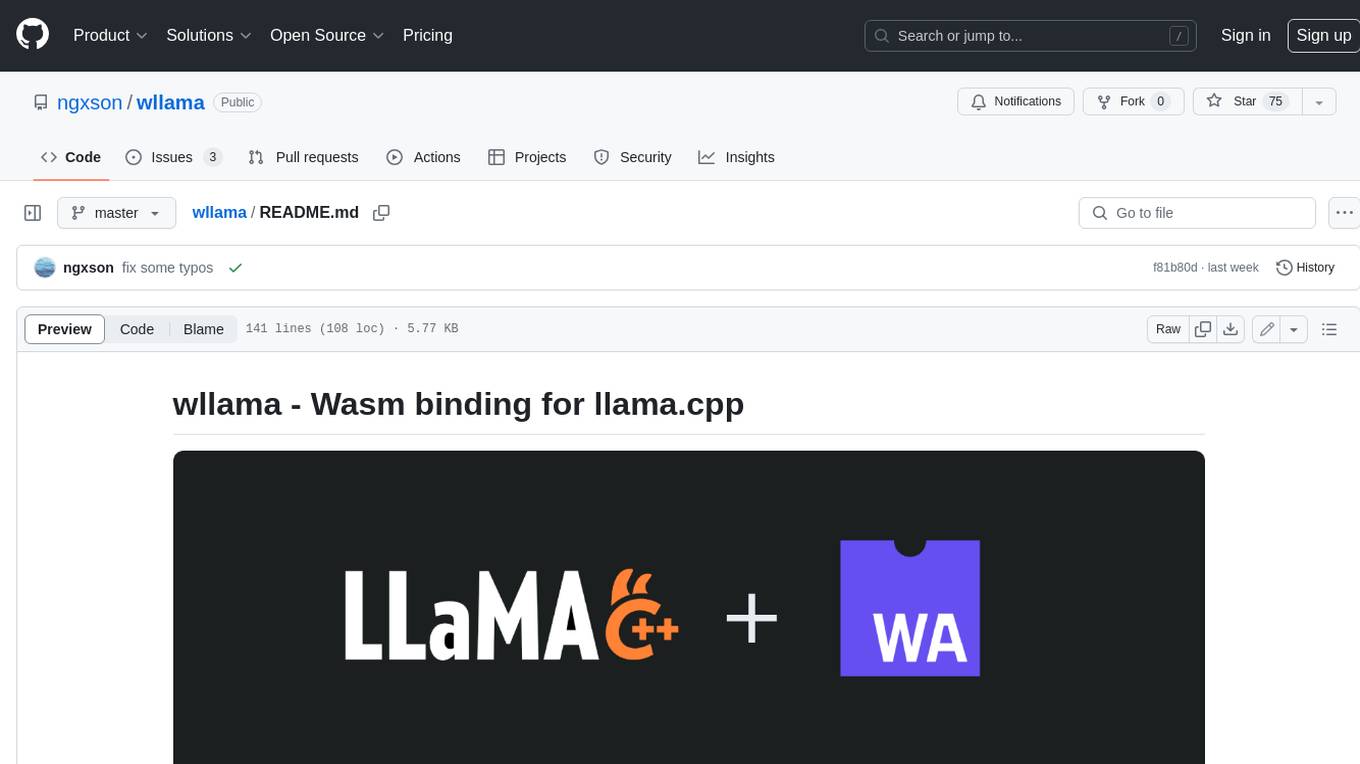
wllama
Wllama is a WebAssembly binding for llama.cpp, a high-performance and lightweight language model library. It enables you to run inference directly on the browser without the need for a backend or GPU. Wllama provides both high-level and low-level APIs, allowing you to perform various tasks such as completions, embeddings, tokenization, and more. It also supports model splitting, enabling you to load large models in parallel for faster download. With its Typescript support and pre-built npm package, Wllama is easy to integrate into your React Typescript projects.
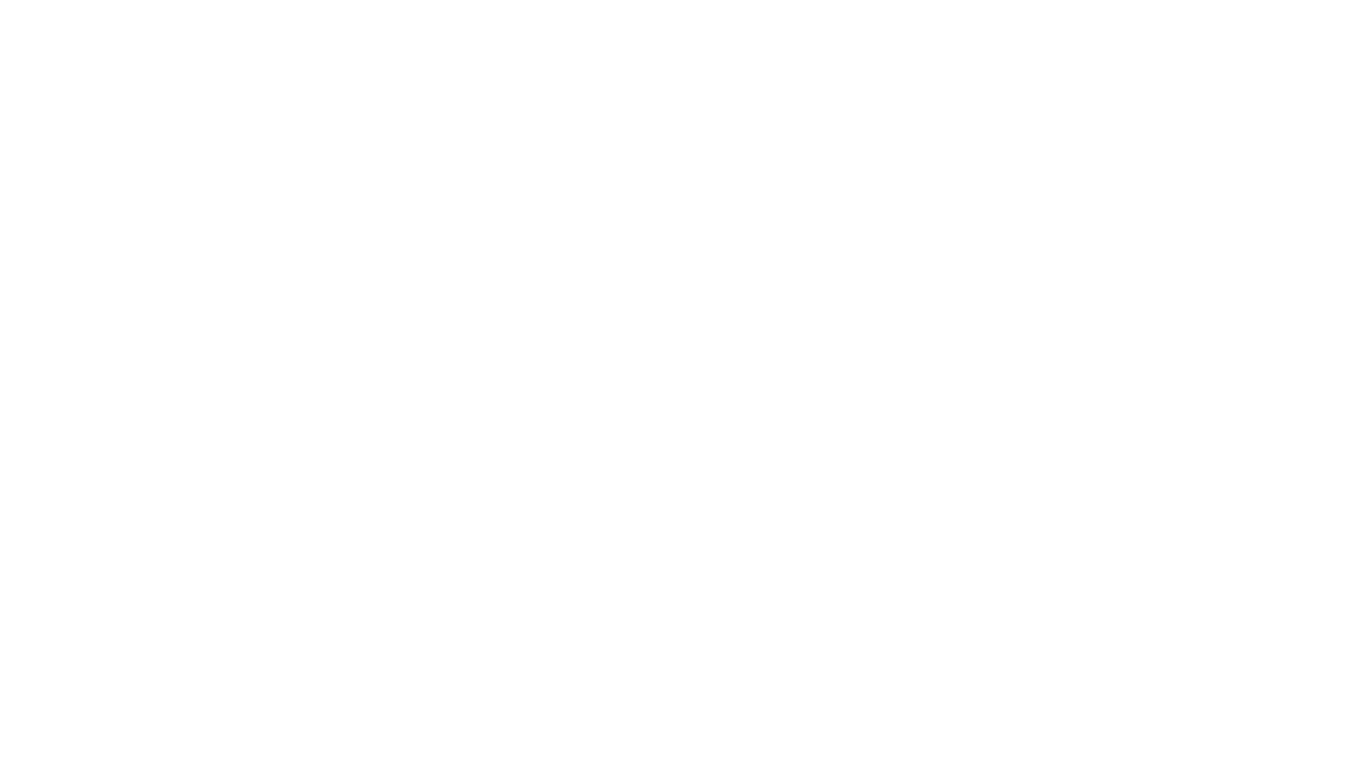
raglite
RAGLite is a Python toolkit for Retrieval-Augmented Generation (RAG) with PostgreSQL or SQLite. It offers configurable options for choosing LLM providers, database types, and rerankers. The toolkit is fast and permissive, utilizing lightweight dependencies and hardware acceleration. RAGLite provides features like PDF to Markdown conversion, multi-vector chunk embedding, optimal semantic chunking, hybrid search capabilities, adaptive retrieval, and improved output quality. It is extensible with a built-in Model Context Protocol server, customizable ChatGPT-like frontend, document conversion to Markdown, and evaluation tools. Users can configure RAGLite for various tasks like configuring, inserting documents, running RAG pipelines, computing query adapters, evaluating performance, running MCP servers, and serving frontends.
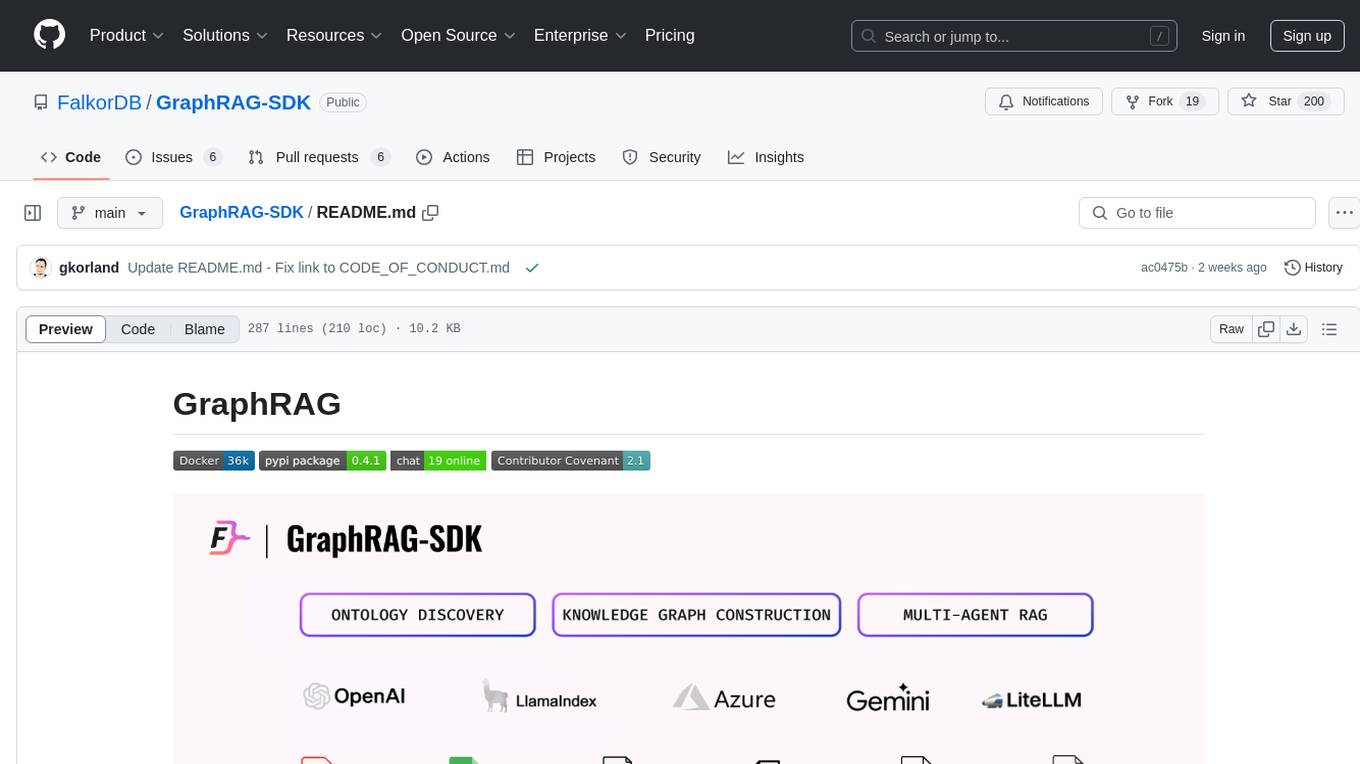
GraphRAG-SDK
Build fast and accurate GenAI applications with GraphRAG SDK, a specialized toolkit for building Graph Retrieval-Augmented Generation (GraphRAG) systems. It integrates knowledge graphs, ontology management, and state-of-the-art LLMs to deliver accurate, efficient, and customizable RAG workflows. The SDK simplifies the development process by automating ontology creation, knowledge graph agent creation, and query handling, enabling users to interact and query their knowledge graphs effectively. It supports multi-agent systems and orchestrates agents specialized in different domains. The SDK is optimized for FalkorDB, ensuring high performance and scalability for large-scale applications. By leveraging knowledge graphs, it enables semantic relationships and ontology-driven queries that go beyond standard vector similarity, enhancing retrieval-augmented generation capabilities.
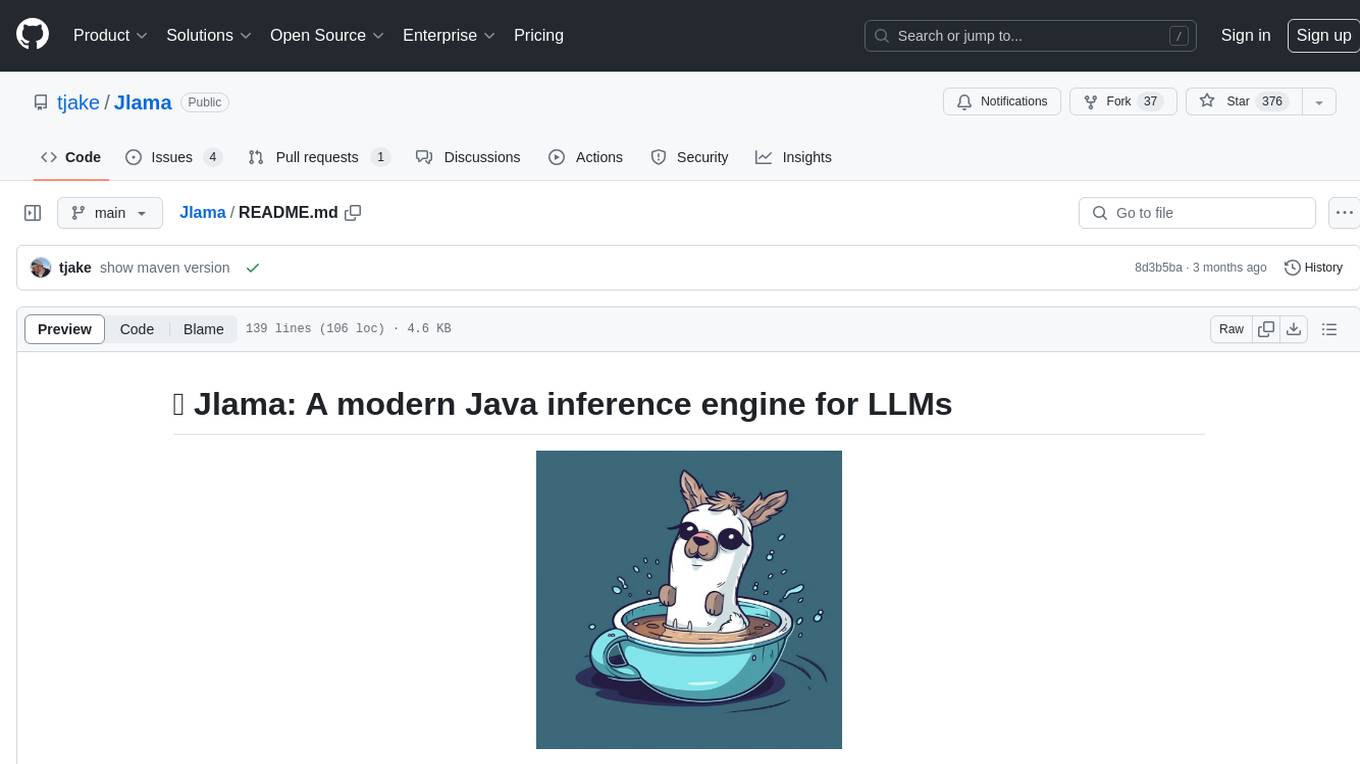
Jlama
Jlama is a modern Java inference engine designed for large language models. It supports various model types such as Gemma, Llama, Mistral, GPT-2, BERT, and more. The tool implements features like Flash Attention, Mixture of Experts, and supports different model quantization formats. Built with Java 21 and utilizing the new Vector API for faster inference, Jlama allows users to add LLM inference directly to their Java applications. The tool includes a CLI for running models, a simple UI for chatting with LLMs, and examples for different model types.
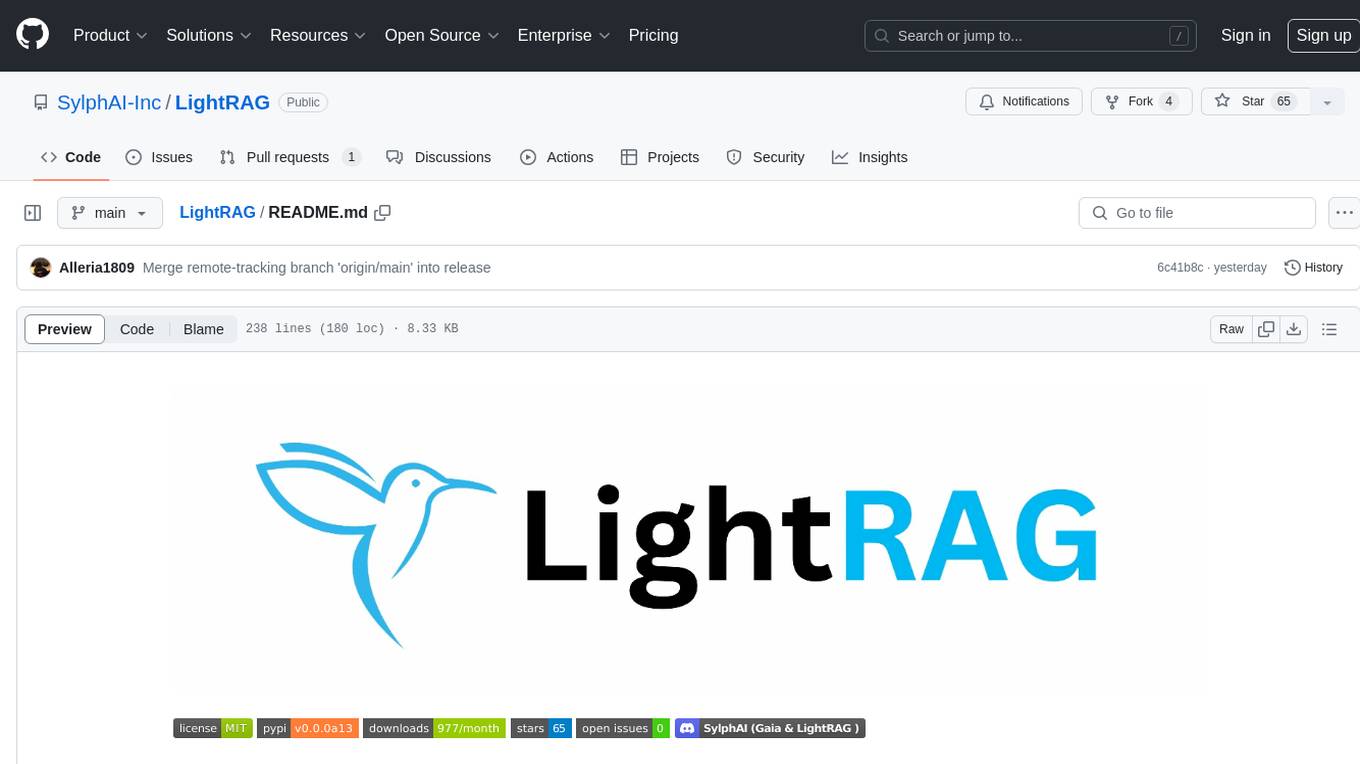
LightRAG
LightRAG is a PyTorch library designed for building and optimizing Retriever-Agent-Generator (RAG) pipelines. It follows principles of simplicity, quality, and optimization, offering developers maximum customizability with minimal abstraction. The library includes components for model interaction, output parsing, and structured data generation. LightRAG facilitates tasks like providing explanations and examples for concepts through a question-answering pipeline.
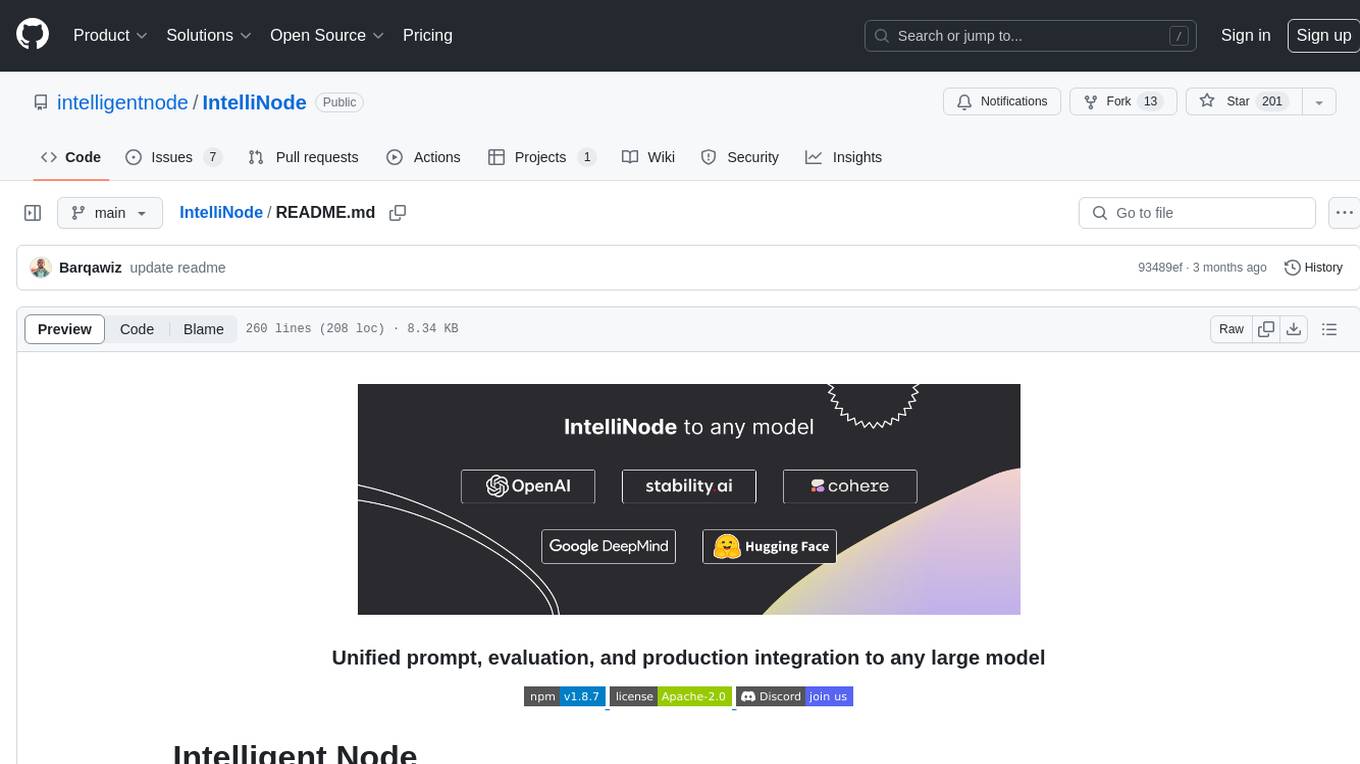
IntelliNode
IntelliNode is a javascript module that integrates cutting-edge AI models like ChatGPT, LLaMA, WaveNet, Gemini, and Stable diffusion into projects. It offers functions for generating text, speech, and images, as well as semantic search, multi-model evaluation, and chatbot capabilities. The module provides a wrapper layer for low-level model access, a controller layer for unified input handling, and a function layer for abstract functionality tailored to various use cases.
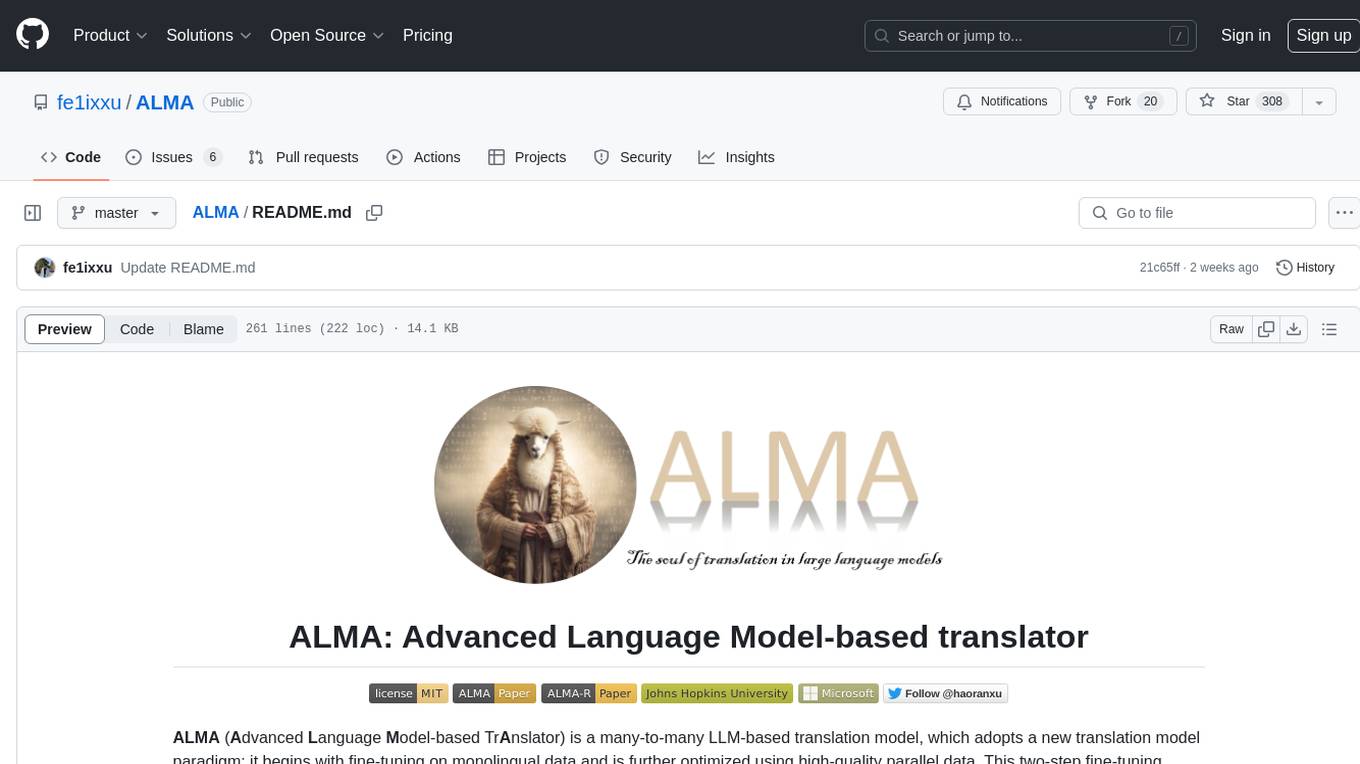
ALMA
ALMA (Advanced Language Model-based Translator) is a many-to-many LLM-based translation model that utilizes a two-step fine-tuning process on monolingual and parallel data to achieve strong translation performance. ALMA-R builds upon ALMA models with LoRA fine-tuning and Contrastive Preference Optimization (CPO) for even better performance, surpassing GPT-4 and WMT winners. The repository provides ALMA and ALMA-R models, datasets, environment setup, evaluation scripts, training guides, and data information for users to leverage these models for translation tasks.
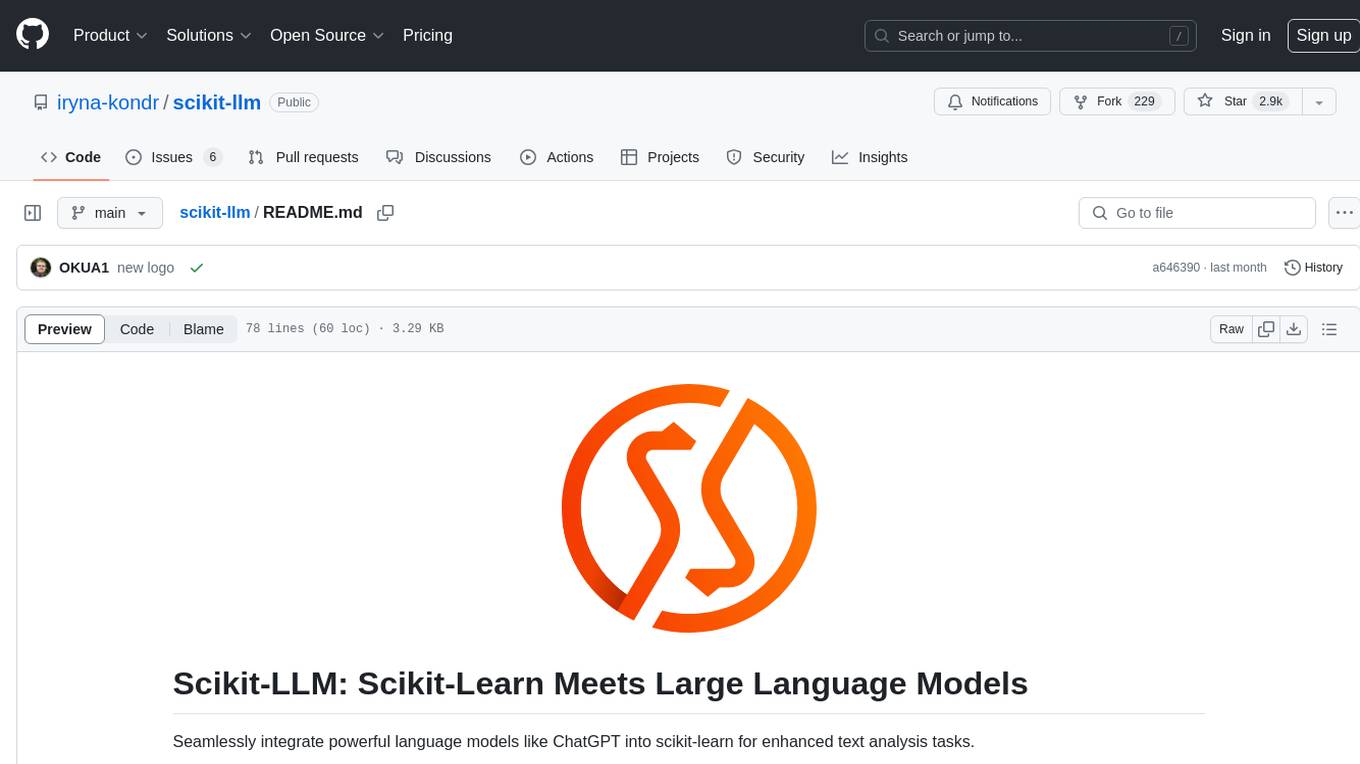
scikit-llm
Scikit-LLM is a tool that seamlessly integrates powerful language models like ChatGPT into scikit-learn for enhanced text analysis tasks. It allows users to leverage large language models for various text analysis applications within the familiar scikit-learn framework. The tool simplifies the process of incorporating advanced language processing capabilities into machine learning pipelines, enabling users to benefit from the latest advancements in natural language processing.
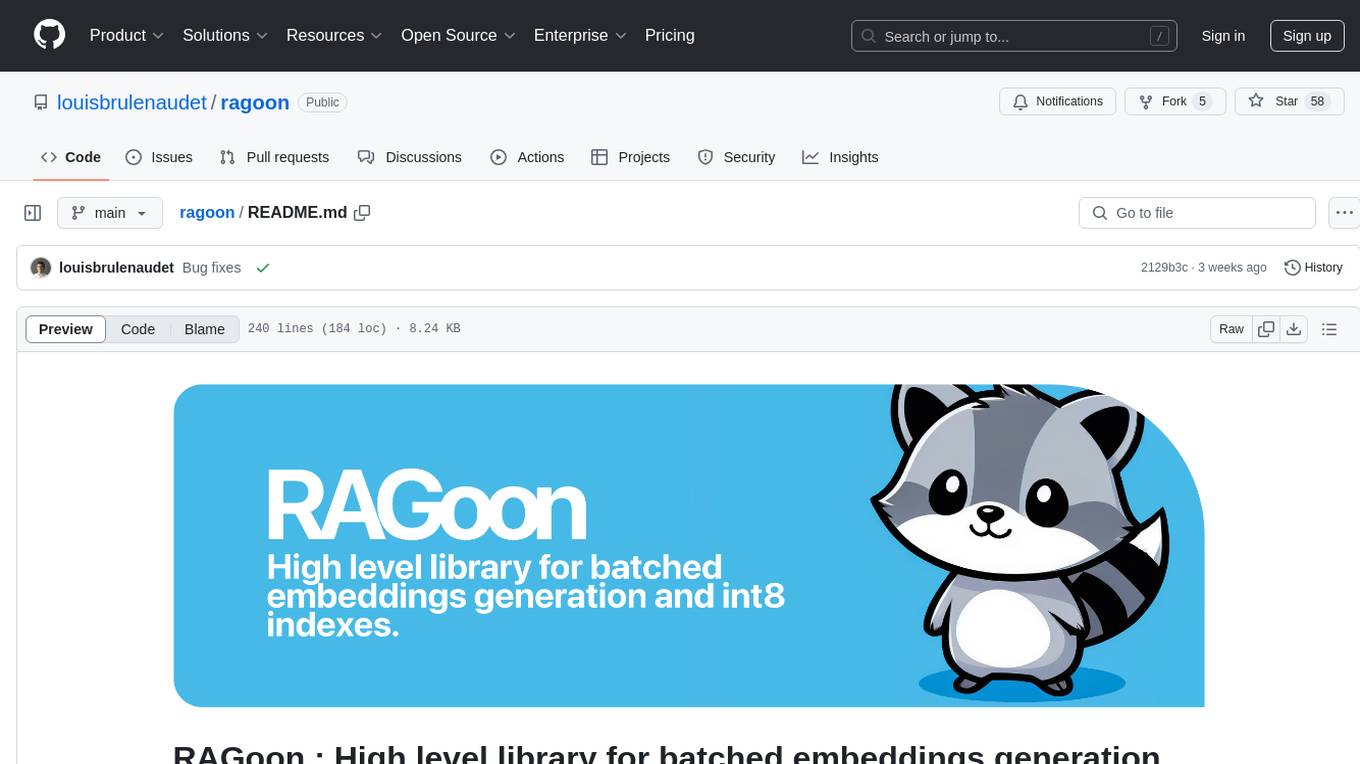
ragoon
RAGoon is a high-level library designed for batched embeddings generation, fast web-based RAG (Retrieval-Augmented Generation) processing, and quantized indexes processing. It provides NLP utilities for multi-model embedding production, high-dimensional vector visualization, and enhancing language model performance through search-based querying, web scraping, and data augmentation techniques.
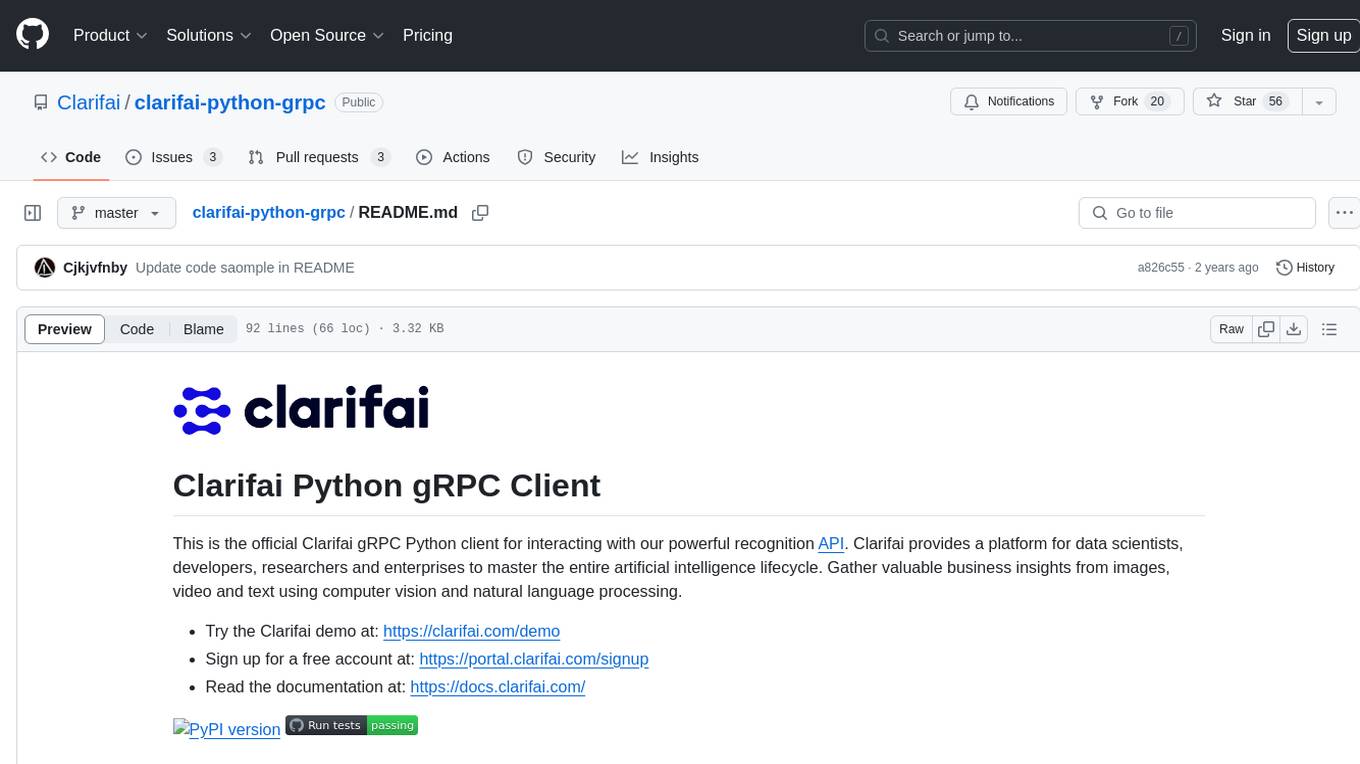
clarifai-python-grpc
This is the official Clarifai gRPC Python client for interacting with their recognition API. Clarifai offers a platform for data scientists, developers, researchers, and enterprises to utilize artificial intelligence for image, video, and text analysis through computer vision and natural language processing. The client allows users to authenticate, predict concepts in images, and access various functionalities provided by the Clarifai API. It follows a versioning scheme that aligns with the backend API updates and includes specific instructions for installation and troubleshooting. Users can explore the Clarifai demo, sign up for an account, and refer to the documentation for detailed information.
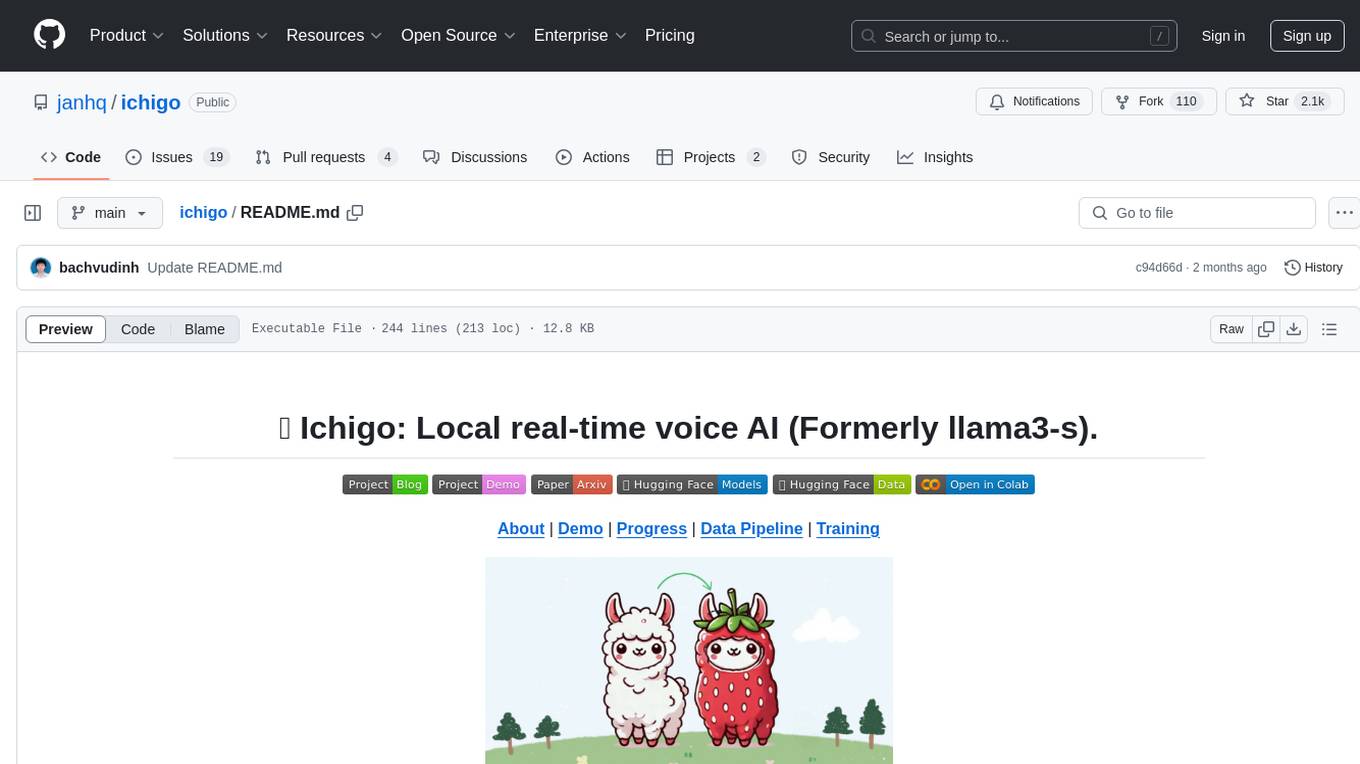
ichigo
Ichigo is a local real-time voice AI tool that uses an early fusion technique to extend a text-based LLM to have native 'listening' ability. It is an open research experiment with improved multiturn capabilities and the ability to refuse processing inaudible queries. The tool is designed for open data, open weight, on-device Siri-like functionality, inspired by Meta's Chameleon paper. Ichigo offers a web UI demo and Gradio web UI for users to interact with the tool. It has achieved enhanced MMLU scores, stronger context handling, advanced noise management, and improved multi-turn capabilities for a robust user experience.
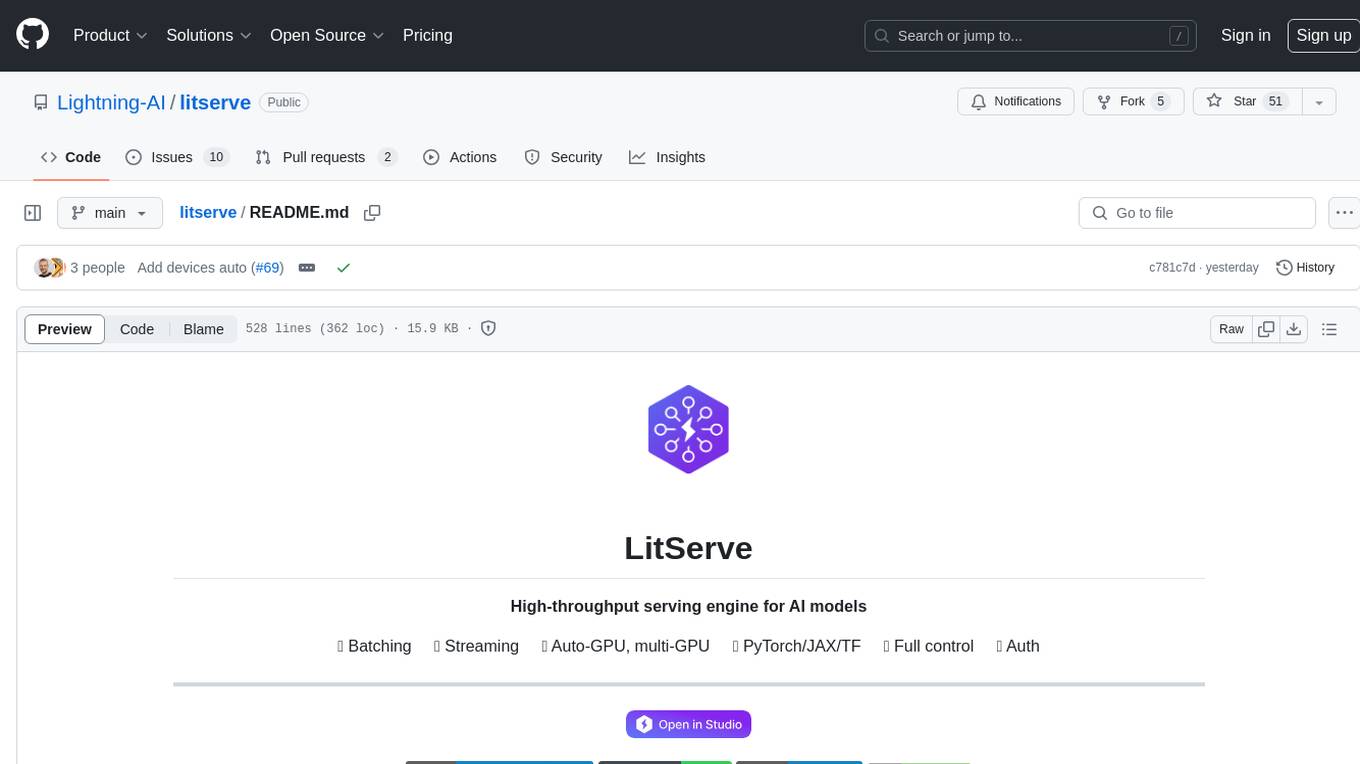
litserve
LitServe is a high-throughput serving engine for deploying AI models at scale. It generates an API endpoint for a model, handles batching, streaming, autoscaling across CPU/GPUs, and more. Built for enterprise scale, it supports every framework like PyTorch, JAX, Tensorflow, and more. LitServe is designed to let users focus on model performance, not the serving boilerplate. It is like PyTorch Lightning for model serving but with broader framework support and scalability.
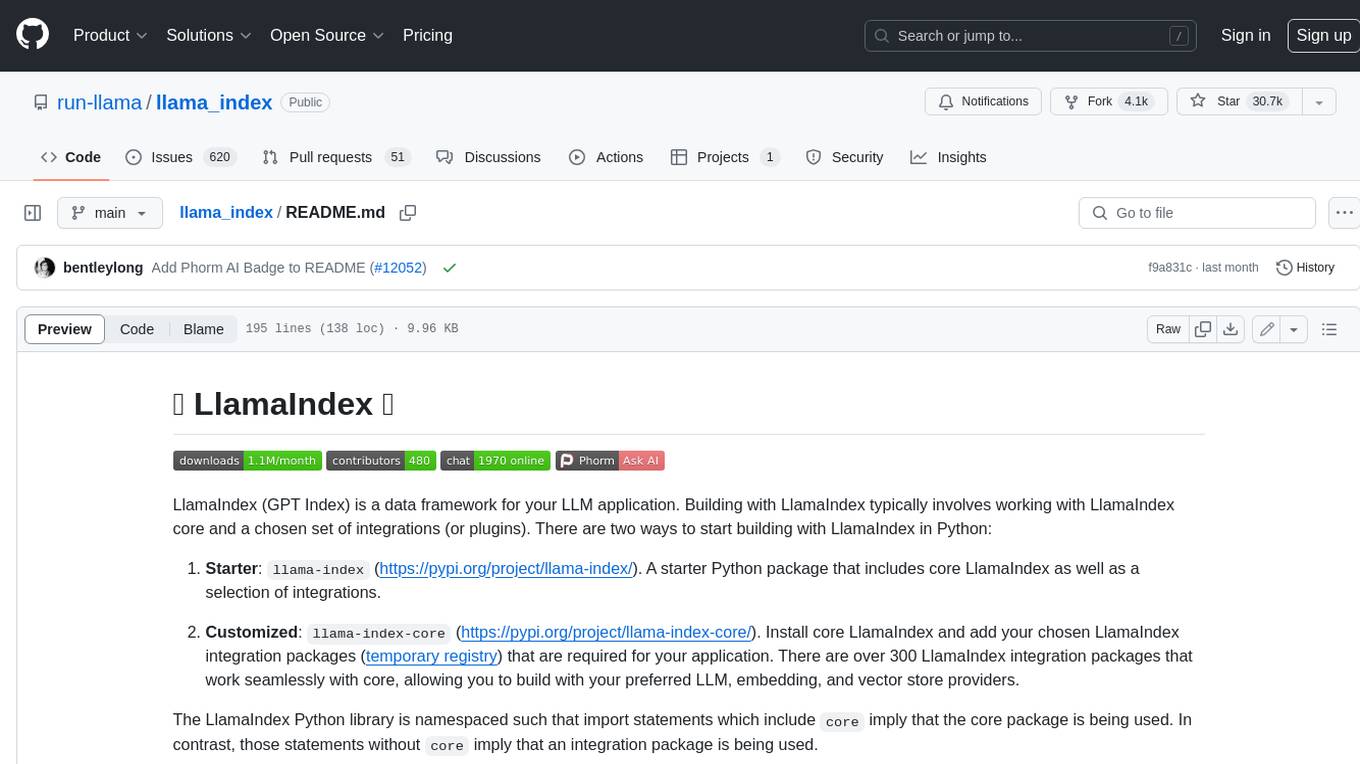
llama_index
LlamaIndex is a data framework for building LLM applications. It provides tools for ingesting, structuring, and querying data, as well as integrating with LLMs and other tools. LlamaIndex is designed to be easy to use for both beginner and advanced users, and it provides a comprehensive set of features for building LLM applications.
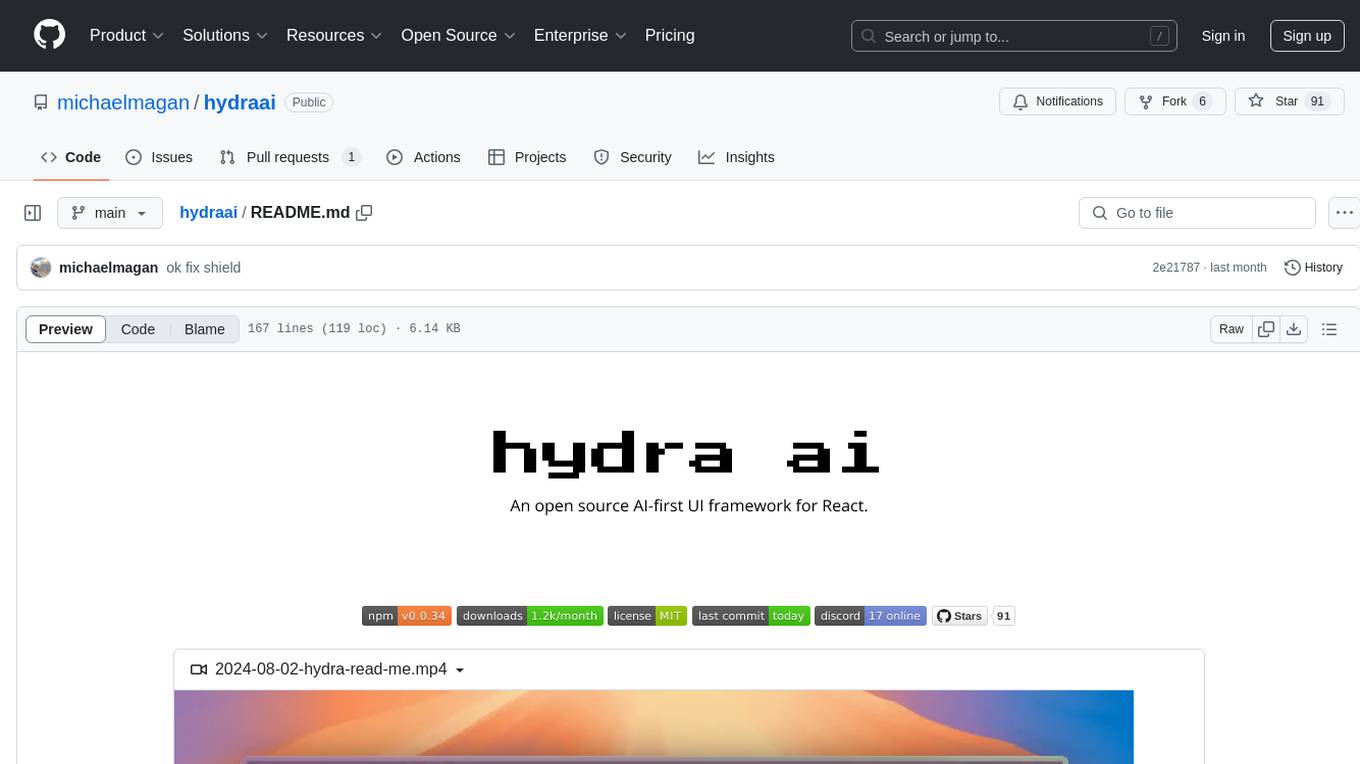
hydraai
Generate React components on-the-fly at runtime using AI. Register your components, and let Hydra choose when to show them in your App. Hydra development is still early, and patterns for different types of components and apps are still being developed. Join the discord to chat with the developers. Expects to be used in a NextJS project. Components that have function props do not work.
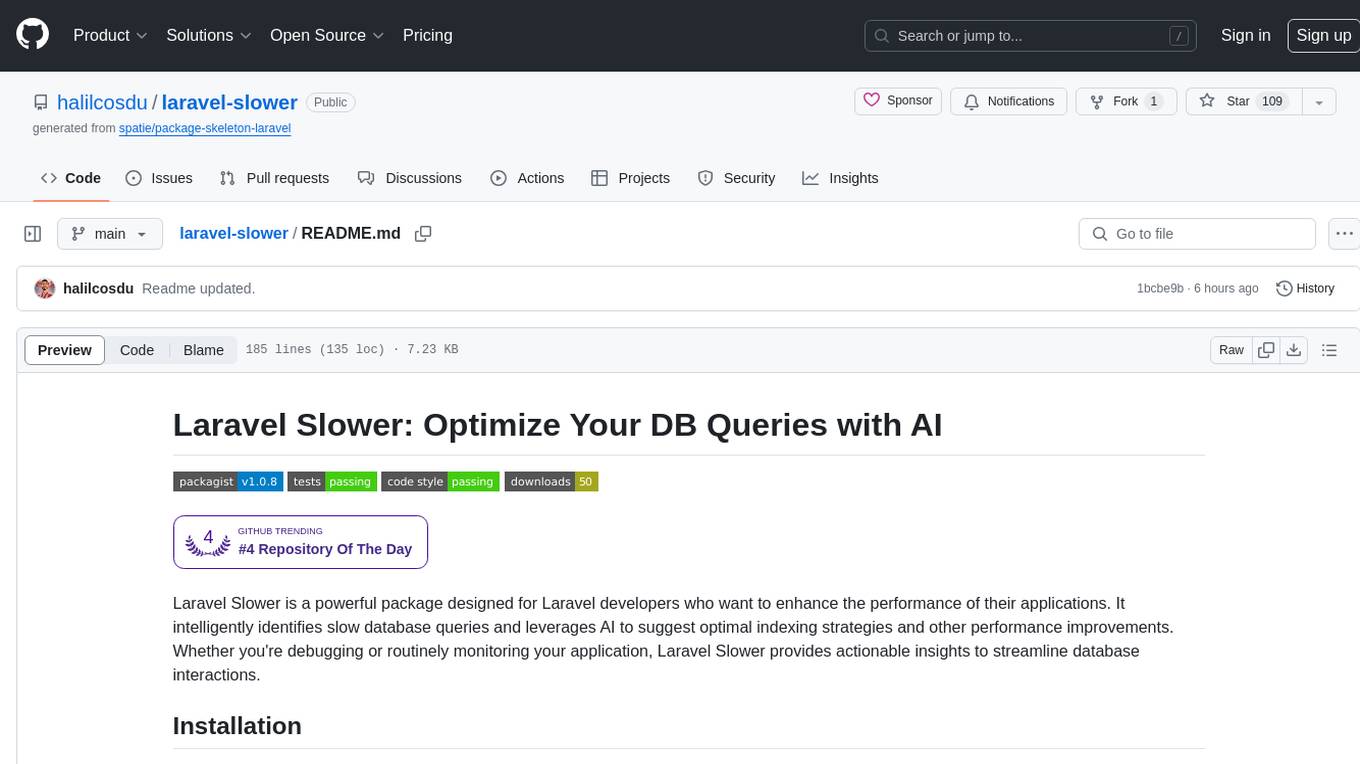
laravel-slower
Laravel Slower is a powerful package designed for Laravel developers to optimize the performance of their applications by identifying slow database queries and providing AI-driven suggestions for optimal indexing strategies and performance improvements. It offers actionable insights for debugging and monitoring database interactions, enhancing efficiency and scalability.
For similar tasks
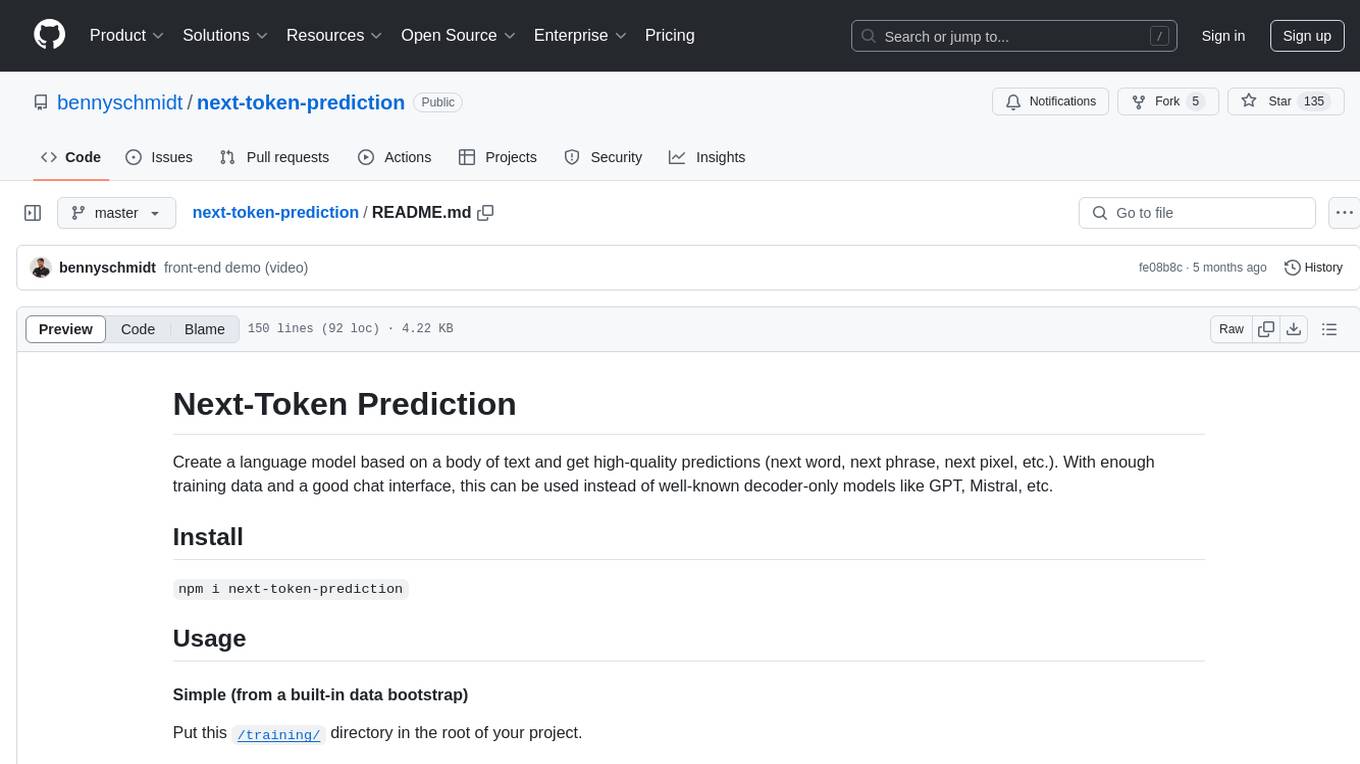
next-token-prediction
Next-Token Prediction is a language model tool that allows users to create high-quality predictions for the next word, phrase, or pixel based on a body of text. It can be used as an alternative to well-known decoder-only models like GPT and Mistral. The tool provides options for simple usage with built-in data bootstrap or advanced customization by providing training data or creating it from .txt files. It aims to simplify methodologies, provide autocomplete, autocorrect, spell checking, search/lookup functionalities, and create pixel and audio transformers for various prediction formats.
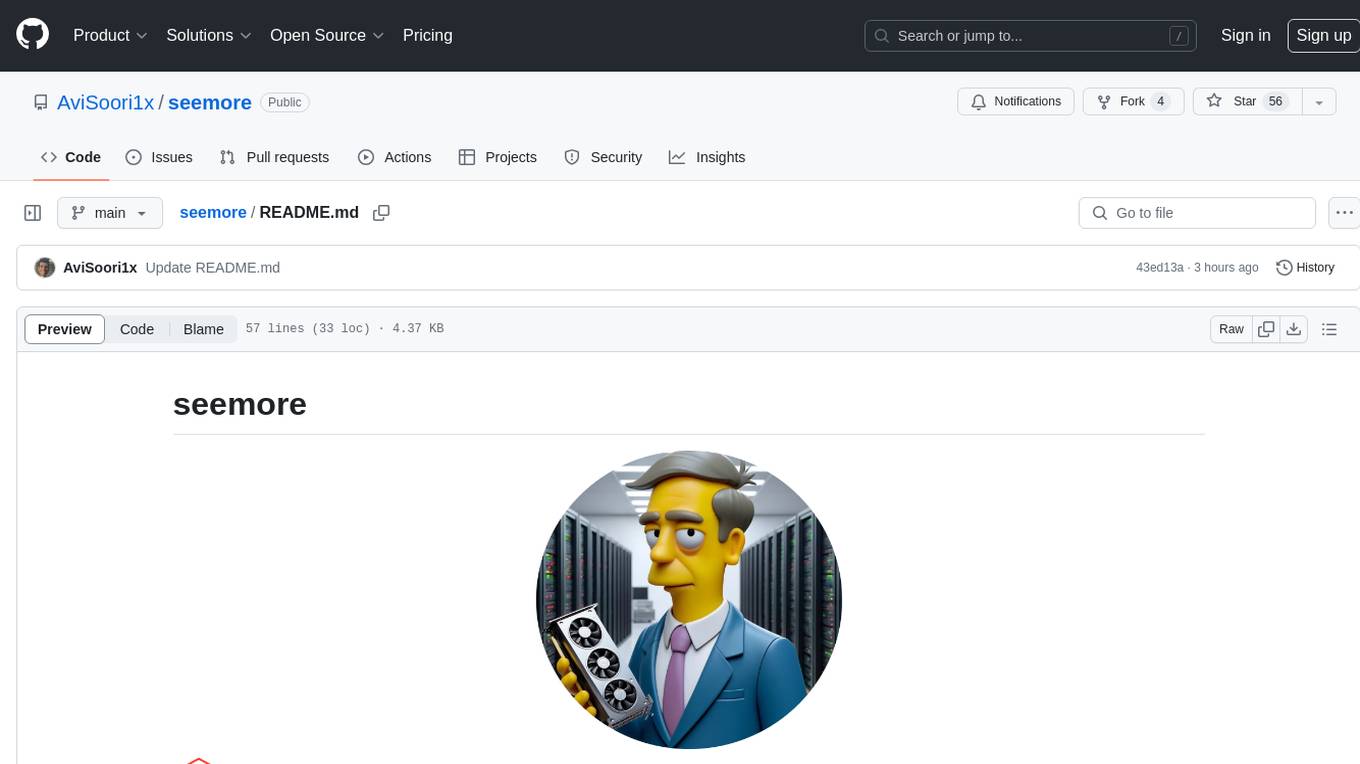
seemore
seemore is a vision language model developed in Pytorch, implementing components like image encoder, vision-language projector, and decoder language model. The model is built from scratch, including attention mechanisms and patch creation. It is designed for readability and hackability, with the intention to be improved upon. The implementation is based on public publications and borrows attention mechanism from makemore by Andrej Kapathy. The code was developed on Databricks using a single A100 for compute, and MLFlow is used for tracking metrics. The tool aims to provide a simplistic version of vision language models like Grok 1.5/GPT-4 Vision, suitable for experimentation and learning.
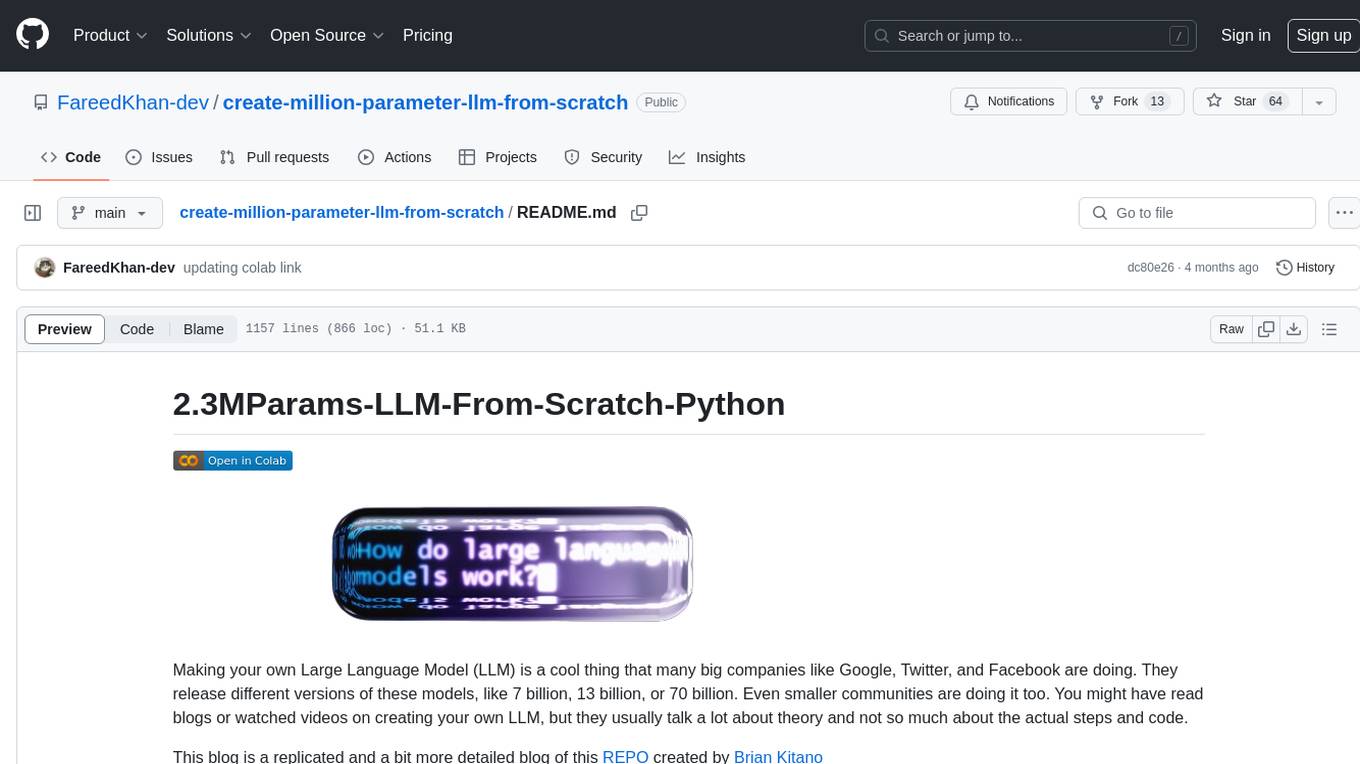
create-million-parameter-llm-from-scratch
The 'create-million-parameter-llm-from-scratch' repository provides a detailed guide on creating a Large Language Model (LLM) with 2.3 million parameters from scratch. The blog replicates the LLaMA approach, incorporating concepts like RMSNorm for pre-normalization, SwiGLU activation function, and Rotary Embeddings. The model is trained on a basic dataset to demonstrate the ease of creating a million-parameter LLM without the need for a high-end GPU.
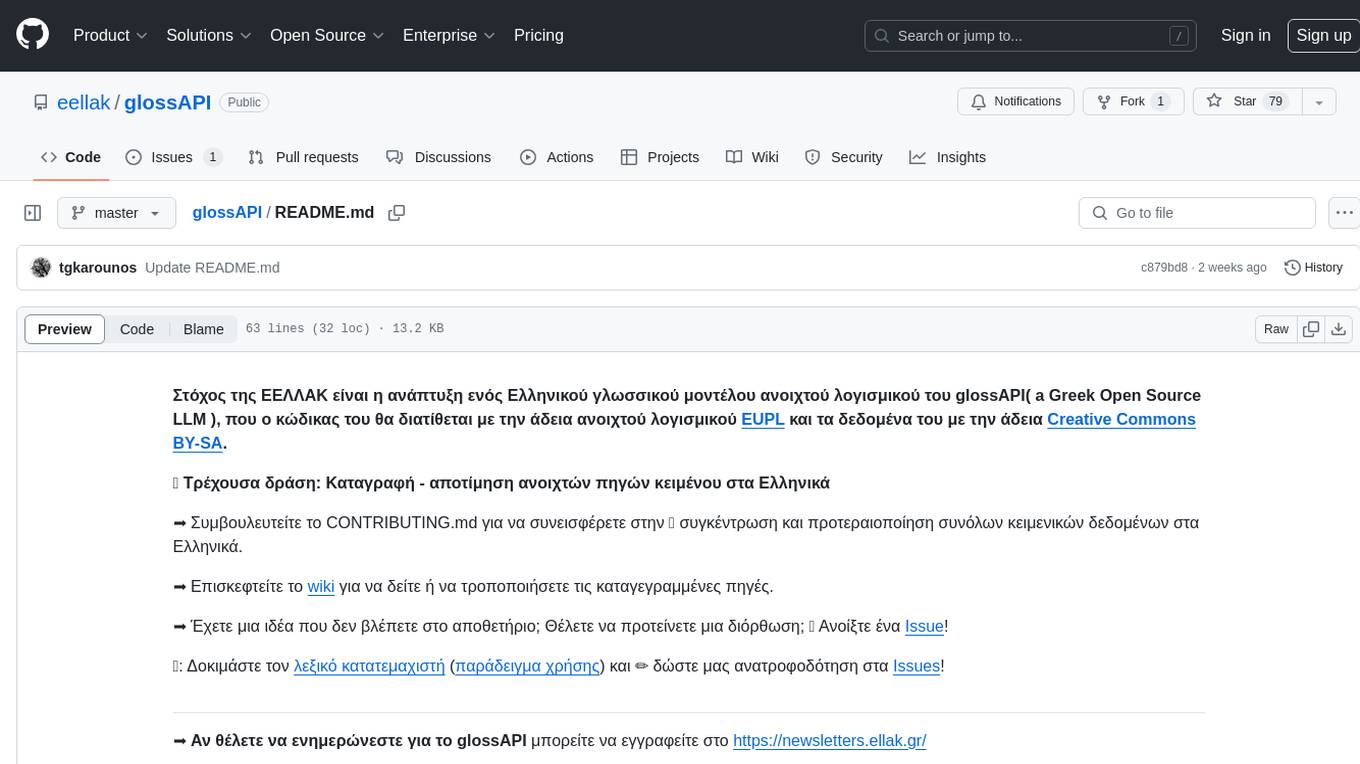
glossAPI
The glossAPI project aims to develop a Greek language model as open-source software, with code licensed under EUPL and data under Creative Commons BY-SA. The project focuses on collecting and evaluating open text sources in Greek, with efforts to prioritize and gather textual data sets. The project encourages contributions through the CONTRIBUTING.md file and provides resources in the wiki for viewing and modifying recorded sources. It also welcomes ideas and corrections through issue submissions. The project emphasizes the importance of open standards, ethically secured data, privacy protection, and addressing digital divides in the context of artificial intelligence and advanced language technologies.
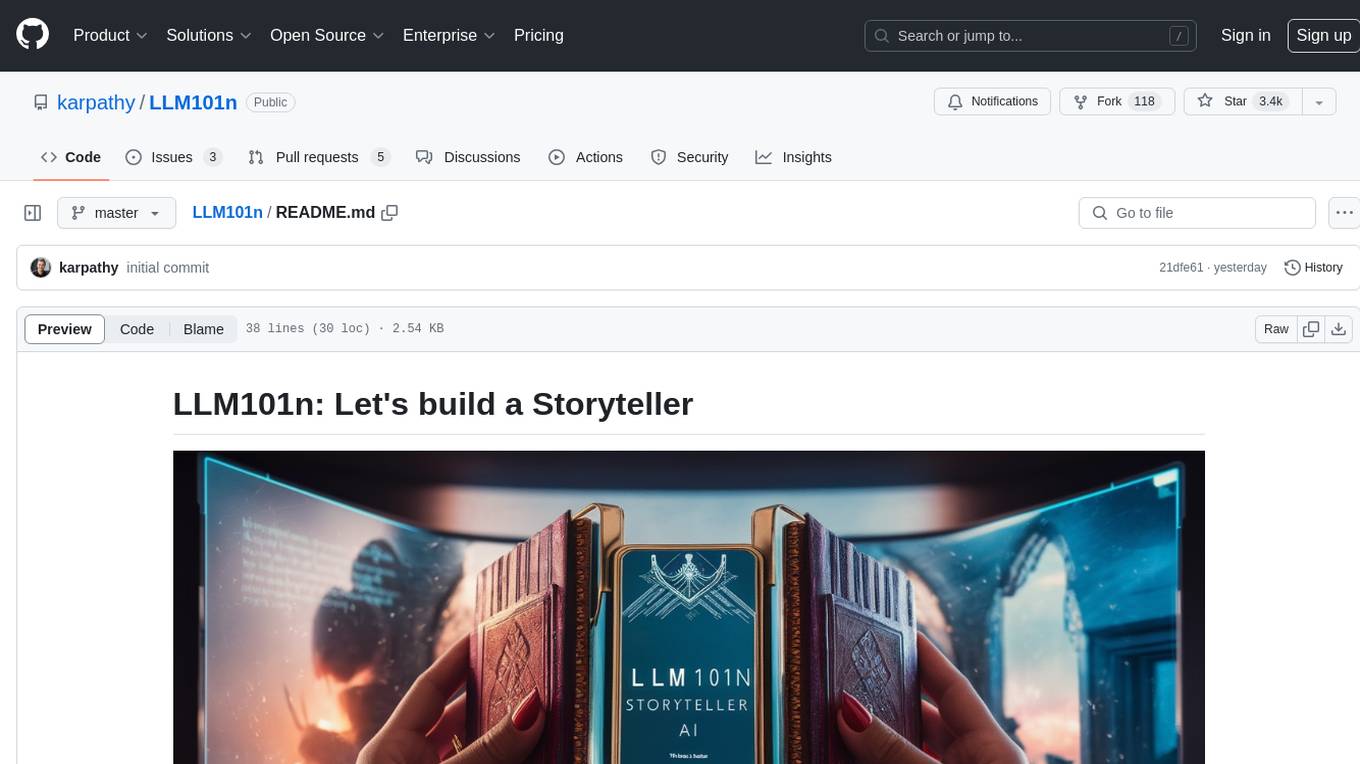
LLM101n
LLM101n is a course focused on building a Storyteller AI Large Language Model (LLM) from scratch in Python, C, and CUDA. The course covers various topics such as language modeling, machine learning, attention mechanisms, tokenization, optimization, device usage, precision training, distributed optimization, datasets, inference, finetuning, deployment, and multimodal applications. Participants will gain a deep understanding of AI, LLMs, and deep learning through hands-on projects and practical examples.
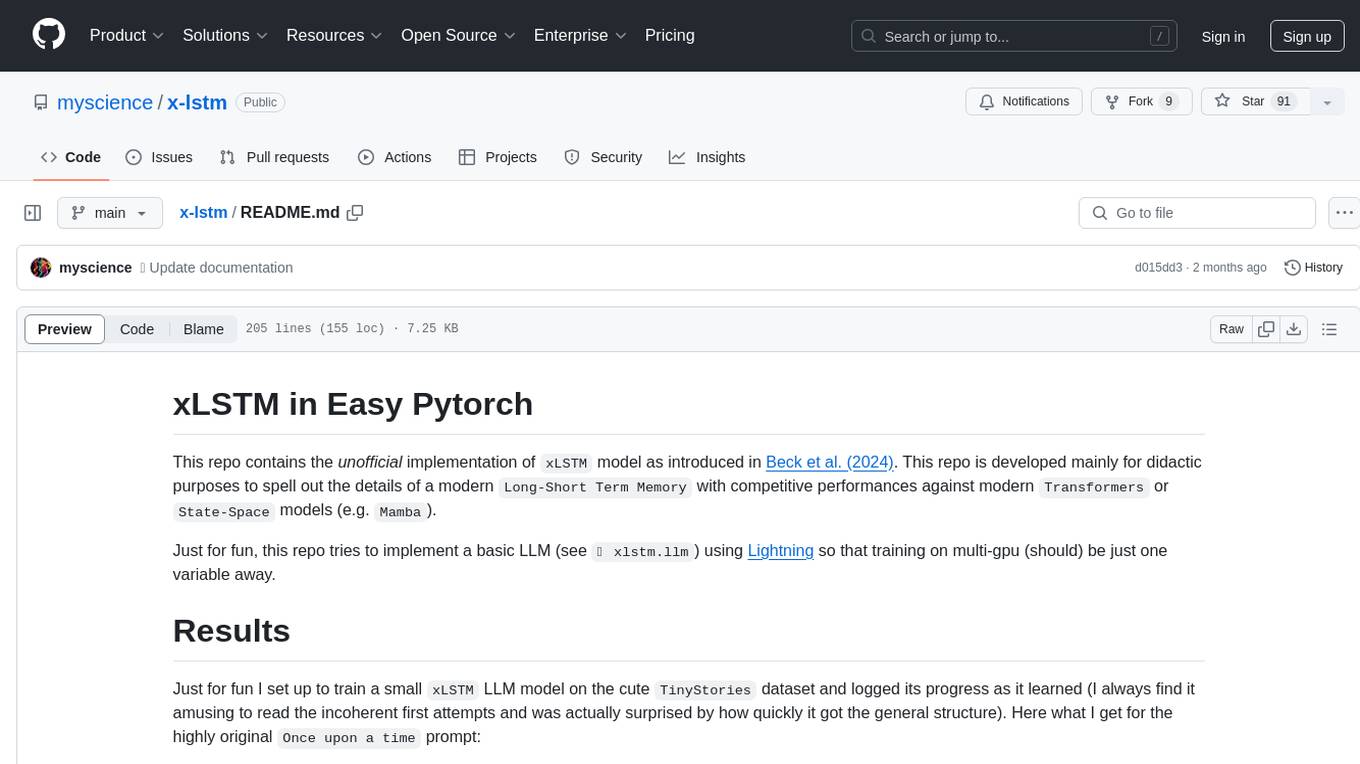
x-lstm
This repository contains an unofficial implementation of the xLSTM model introduced in Beck et al. (2024). It serves as a didactic tool to explain the details of a modern Long-Short Term Memory model with competitive performance against Transformers or State-Space models. The repository also includes a Lightning-based implementation of a basic LLM for multi-GPU training. It provides modules for scalar-LSTM and matrix-LSTM, as well as an xLSTM LLM built using Pytorch Lightning for easy training on multi-GPUs.
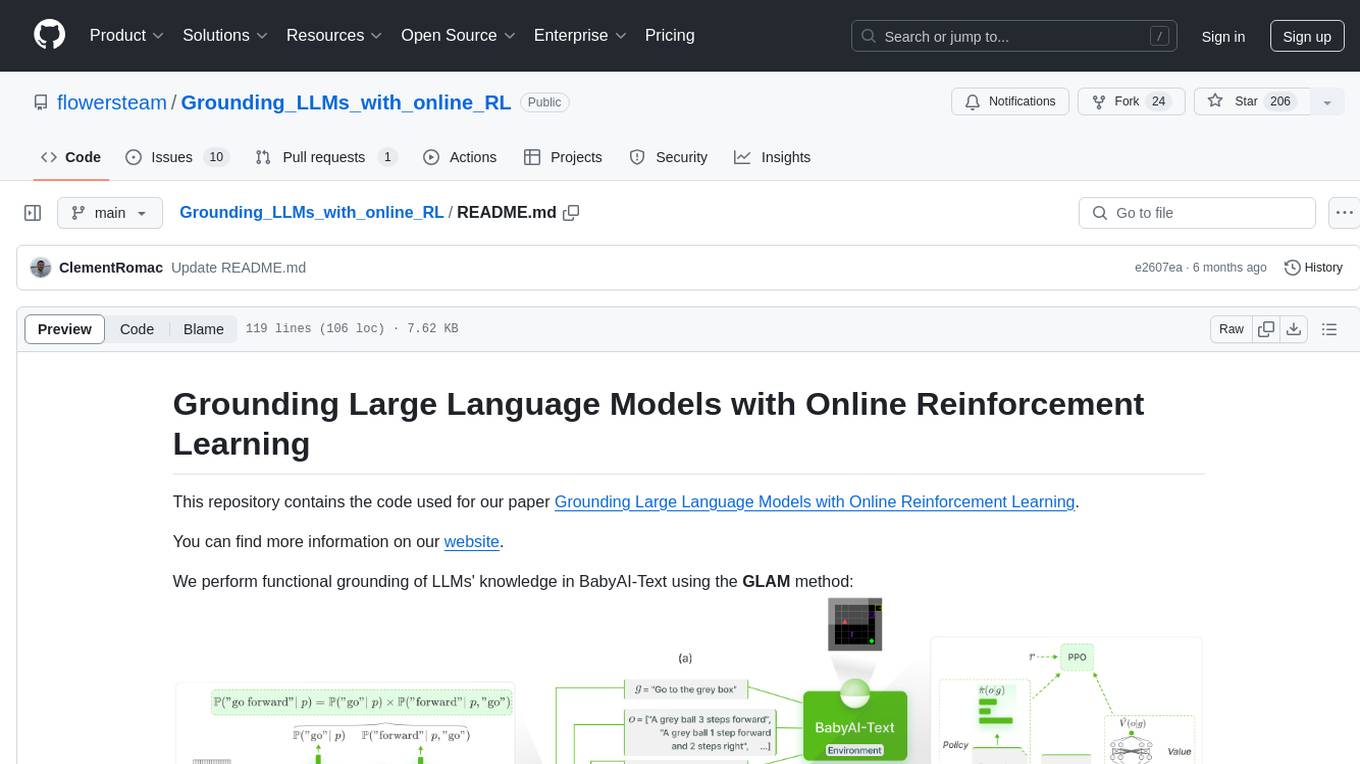
Grounding_LLMs_with_online_RL
This repository contains code for grounding large language models' knowledge in BabyAI-Text using the GLAM method. It includes the BabyAI-Text environment, code for experiments, and training agents. The repository is structured with folders for the environment, experiments, agents, configurations, SLURM scripts, and training scripts. Installation steps involve creating a conda environment, installing PyTorch, required packages, BabyAI-Text, and Lamorel. The launch process involves using Lamorel with configs and training scripts. Users can train a language model and evaluate performance on test episodes using provided scripts and config entries.
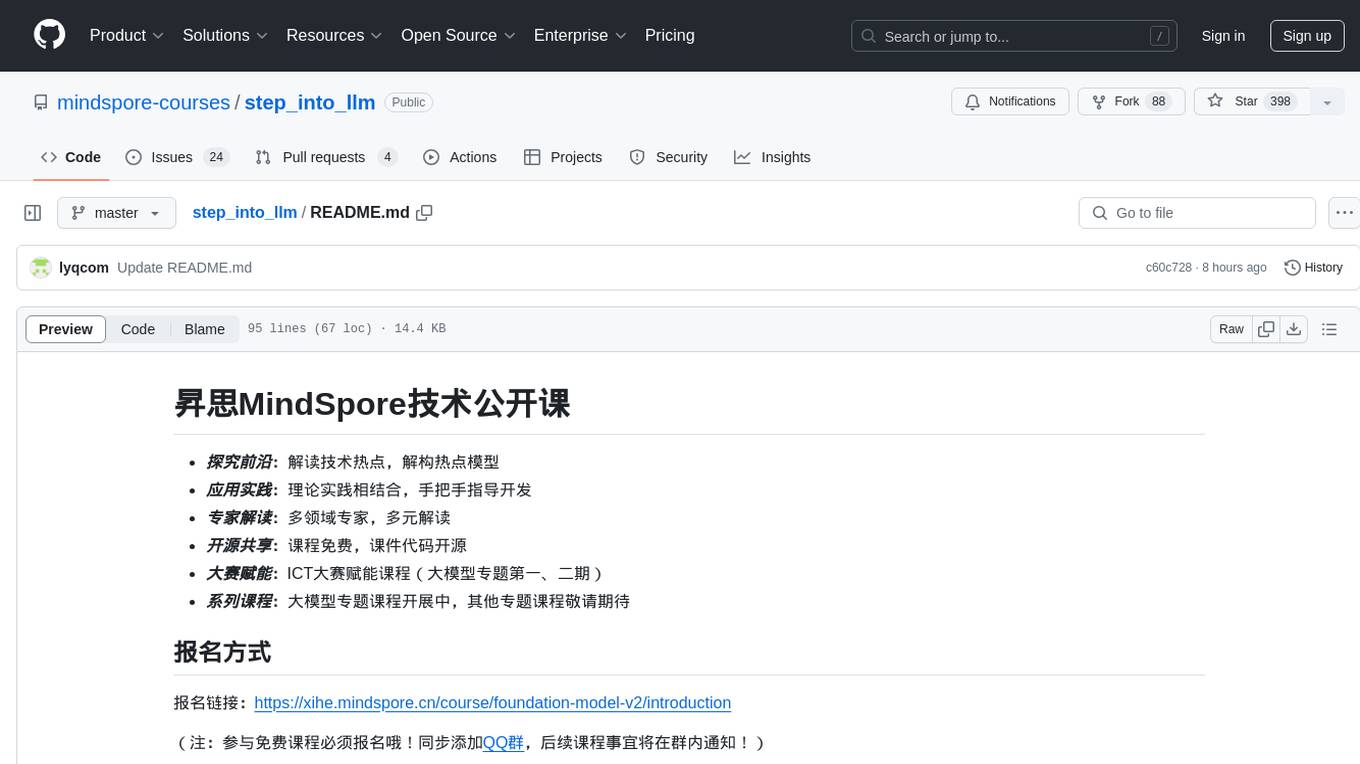
step_into_llm
The 'step_into_llm' repository is dedicated to the 昇思MindSpore technology open class, which focuses on exploring cutting-edge technologies, combining theory with practical applications, expert interpretations, open sharing, and empowering competitions. The repository contains course materials, including slides and code, for the ongoing second phase of the course. It covers various topics related to large language models (LLMs) such as Transformer, BERT, GPT, GPT2, and more. The course aims to guide developers interested in LLMs from theory to practical implementation, with a special emphasis on the development and application of large models.
For similar jobs
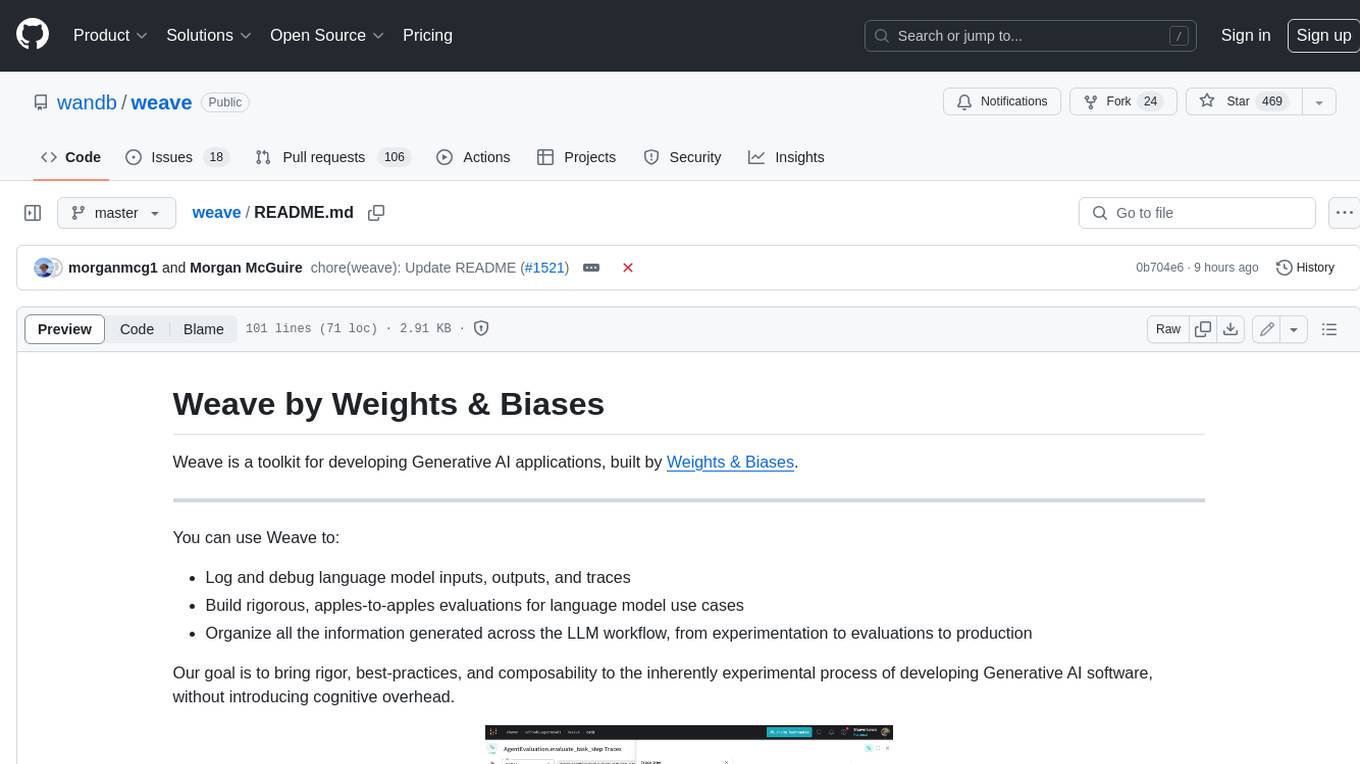
weave
Weave is a toolkit for developing Generative AI applications, built by Weights & Biases. With Weave, you can log and debug language model inputs, outputs, and traces; build rigorous, apples-to-apples evaluations for language model use cases; and organize all the information generated across the LLM workflow, from experimentation to evaluations to production. Weave aims to bring rigor, best-practices, and composability to the inherently experimental process of developing Generative AI software, without introducing cognitive overhead.
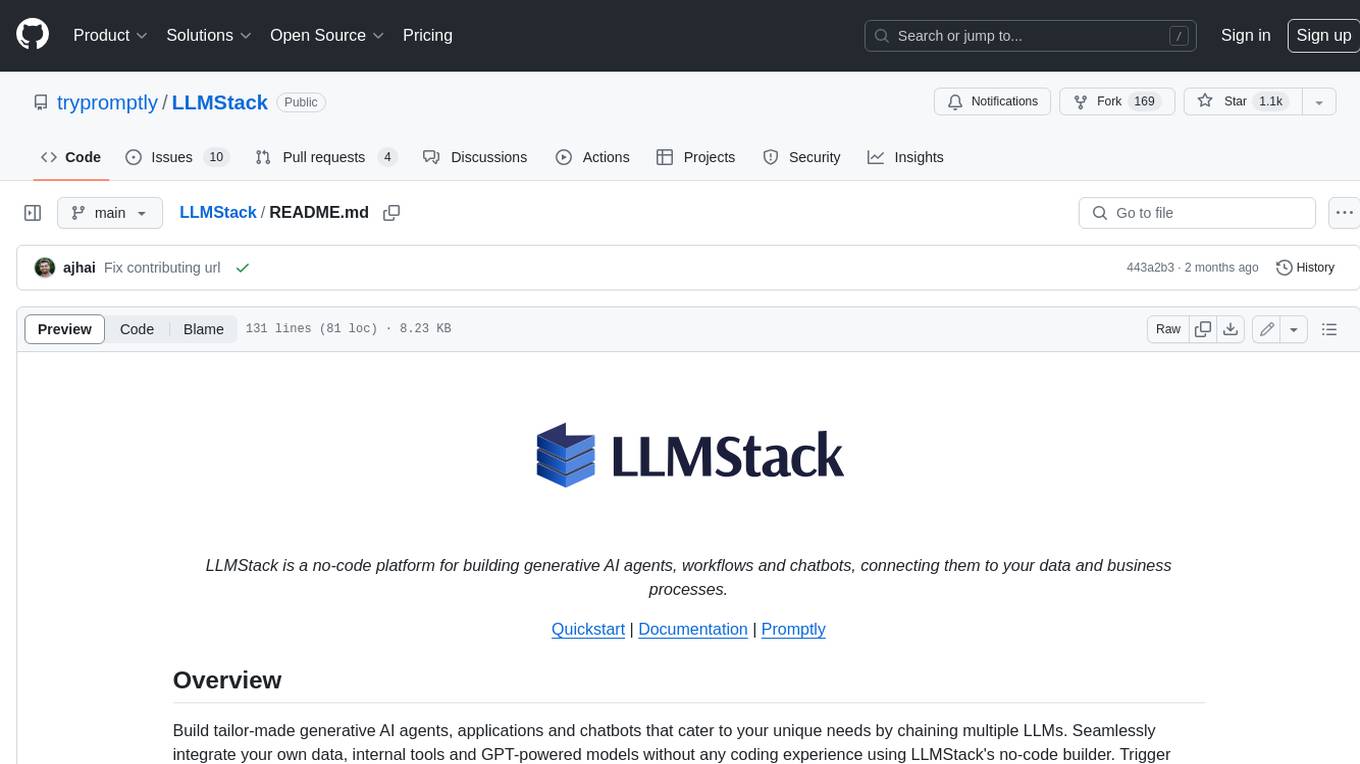
LLMStack
LLMStack is a no-code platform for building generative AI agents, workflows, and chatbots. It allows users to connect their own data, internal tools, and GPT-powered models without any coding experience. LLMStack can be deployed to the cloud or on-premise and can be accessed via HTTP API or triggered from Slack or Discord.
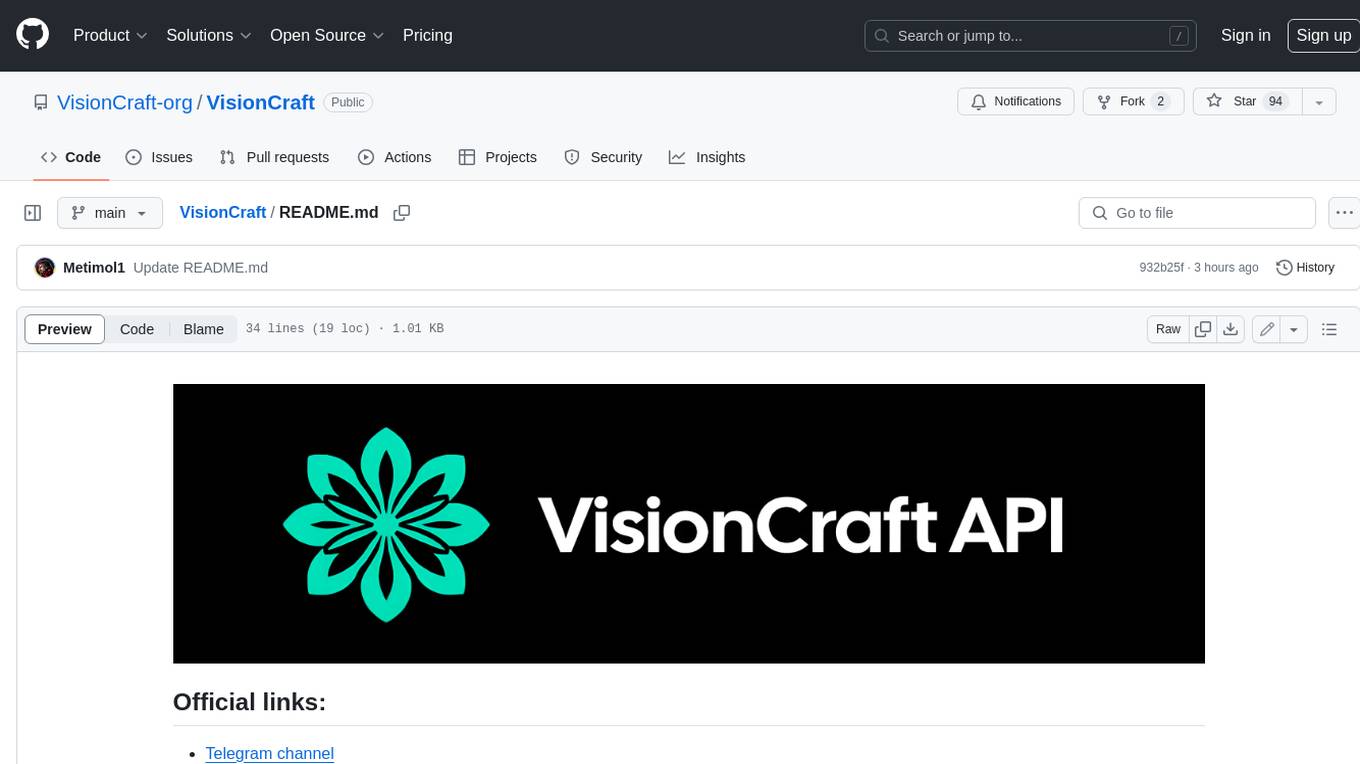
VisionCraft
The VisionCraft API is a free API for using over 100 different AI models. From images to sound.
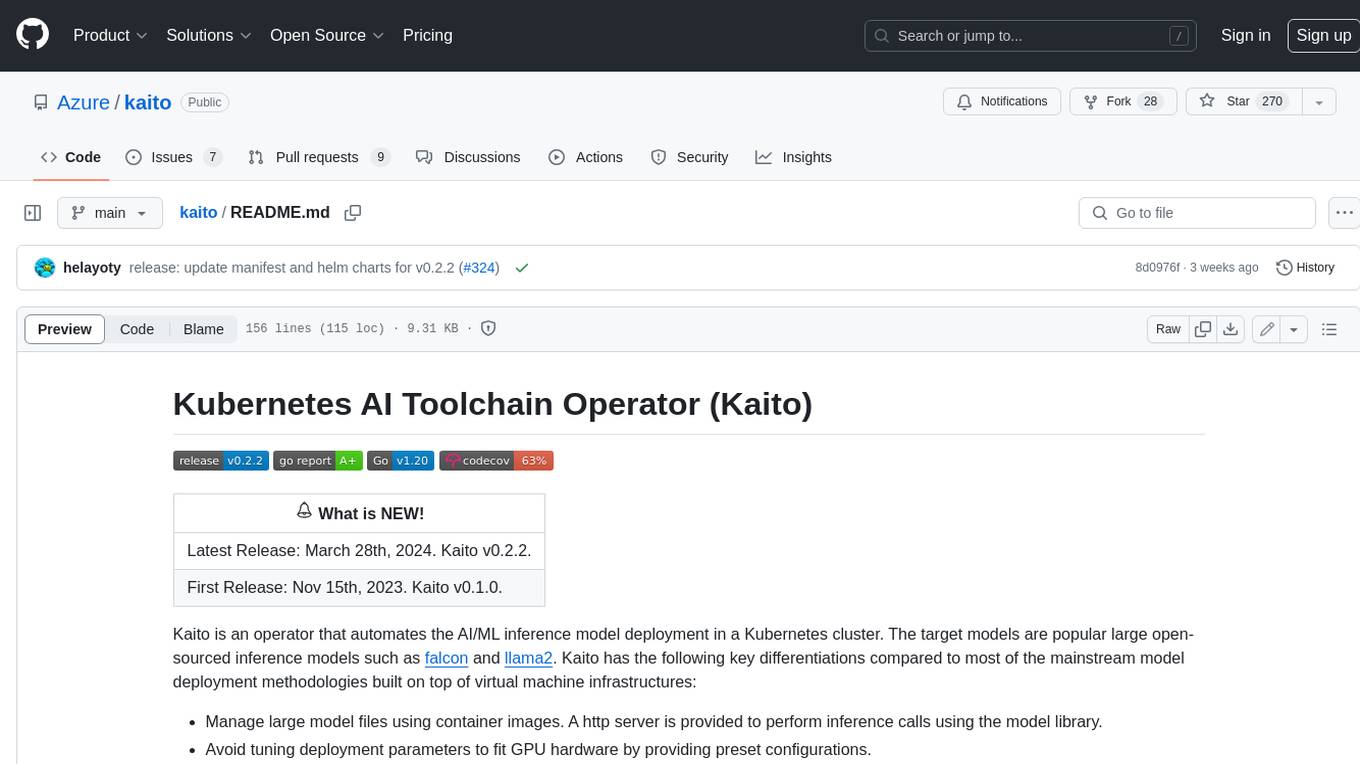
kaito
Kaito is an operator that automates the AI/ML inference model deployment in a Kubernetes cluster. It manages large model files using container images, avoids tuning deployment parameters to fit GPU hardware by providing preset configurations, auto-provisions GPU nodes based on model requirements, and hosts large model images in the public Microsoft Container Registry (MCR) if the license allows. Using Kaito, the workflow of onboarding large AI inference models in Kubernetes is largely simplified.
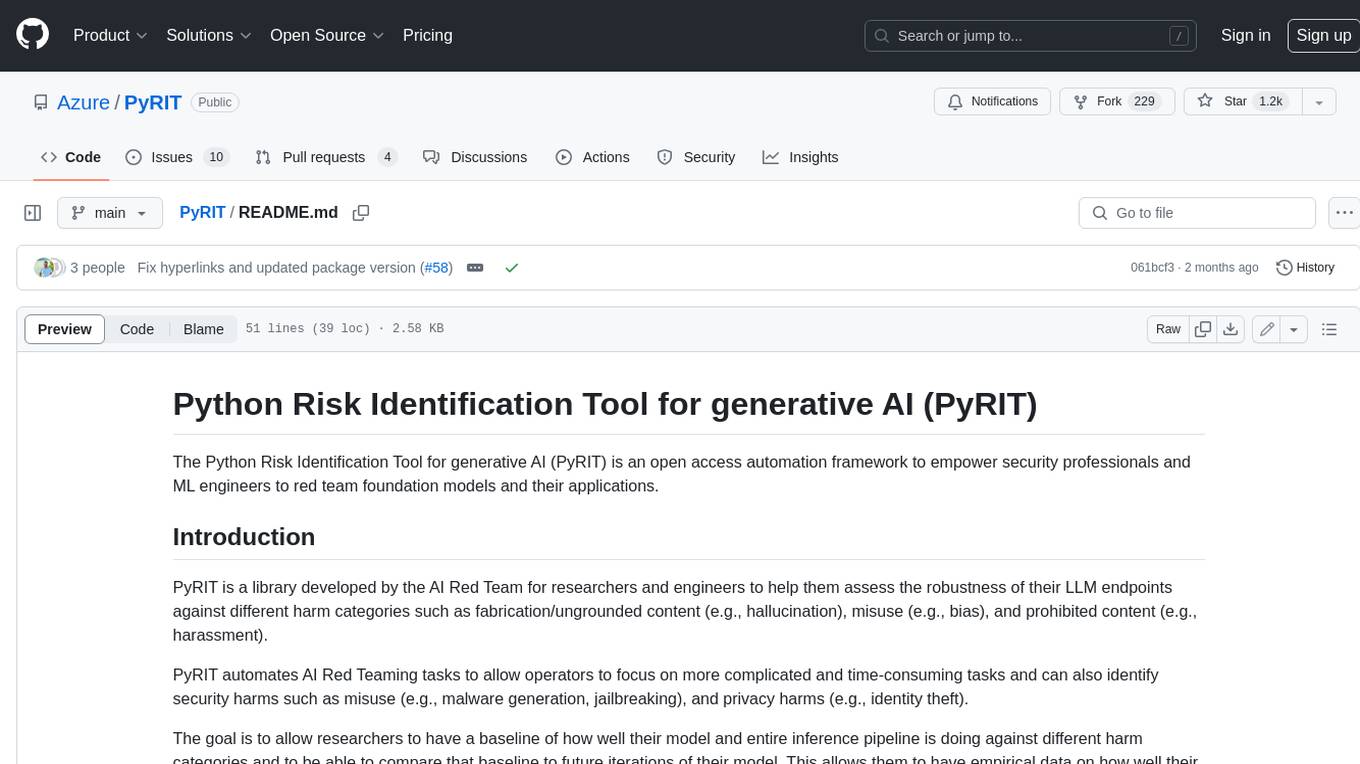
PyRIT
PyRIT is an open access automation framework designed to empower security professionals and ML engineers to red team foundation models and their applications. It automates AI Red Teaming tasks to allow operators to focus on more complicated and time-consuming tasks and can also identify security harms such as misuse (e.g., malware generation, jailbreaking), and privacy harms (e.g., identity theft). The goal is to allow researchers to have a baseline of how well their model and entire inference pipeline is doing against different harm categories and to be able to compare that baseline to future iterations of their model. This allows them to have empirical data on how well their model is doing today, and detect any degradation of performance based on future improvements.
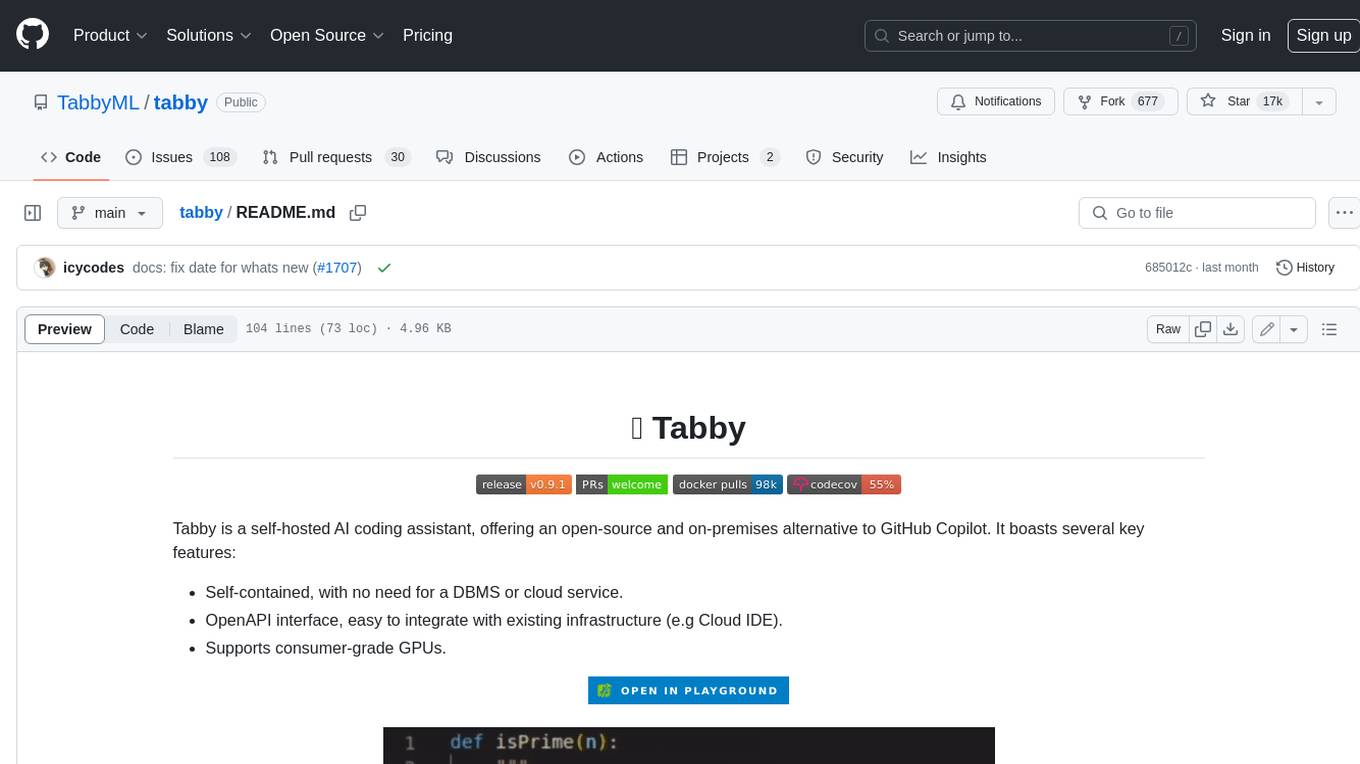
tabby
Tabby is a self-hosted AI coding assistant, offering an open-source and on-premises alternative to GitHub Copilot. It boasts several key features: * Self-contained, with no need for a DBMS or cloud service. * OpenAPI interface, easy to integrate with existing infrastructure (e.g Cloud IDE). * Supports consumer-grade GPUs.
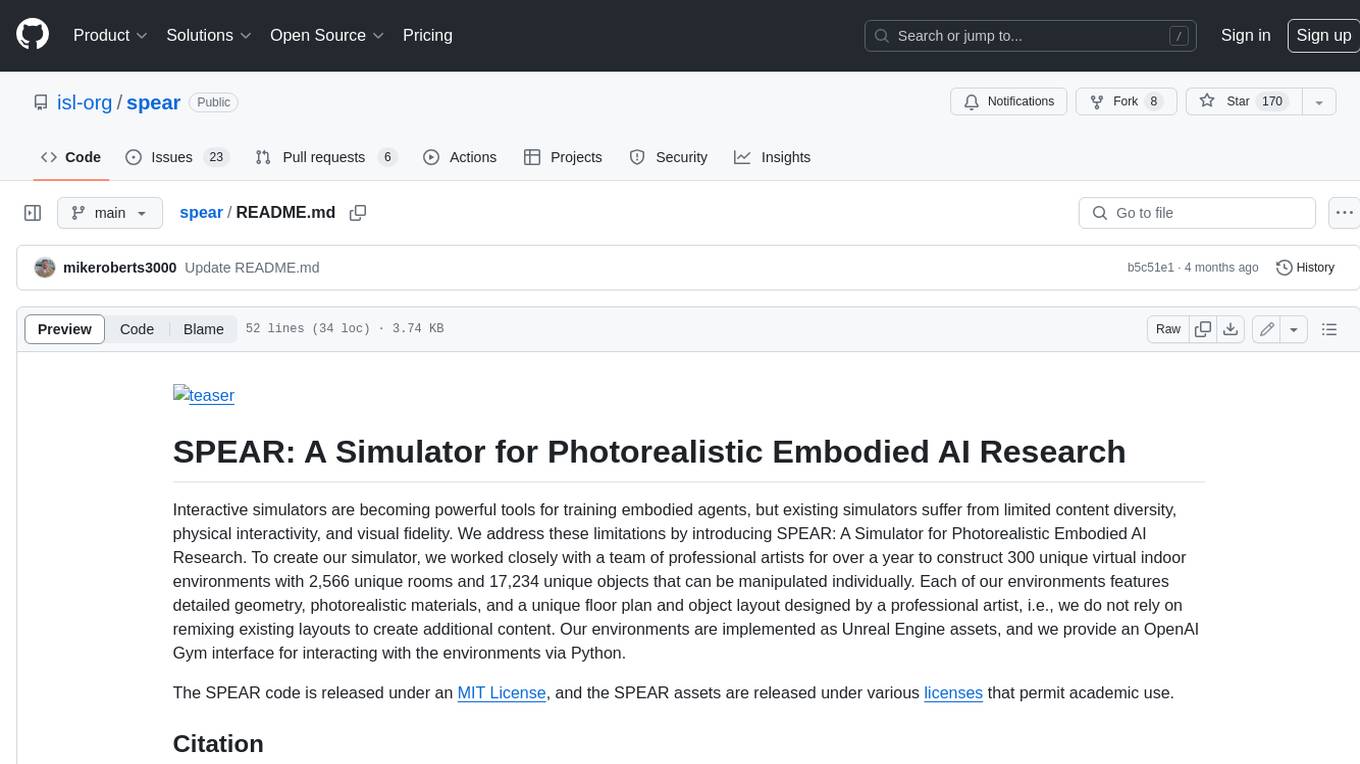
spear
SPEAR (Simulator for Photorealistic Embodied AI Research) is a powerful tool for training embodied agents. It features 300 unique virtual indoor environments with 2,566 unique rooms and 17,234 unique objects that can be manipulated individually. Each environment is designed by a professional artist and features detailed geometry, photorealistic materials, and a unique floor plan and object layout. SPEAR is implemented as Unreal Engine assets and provides an OpenAI Gym interface for interacting with the environments via Python.
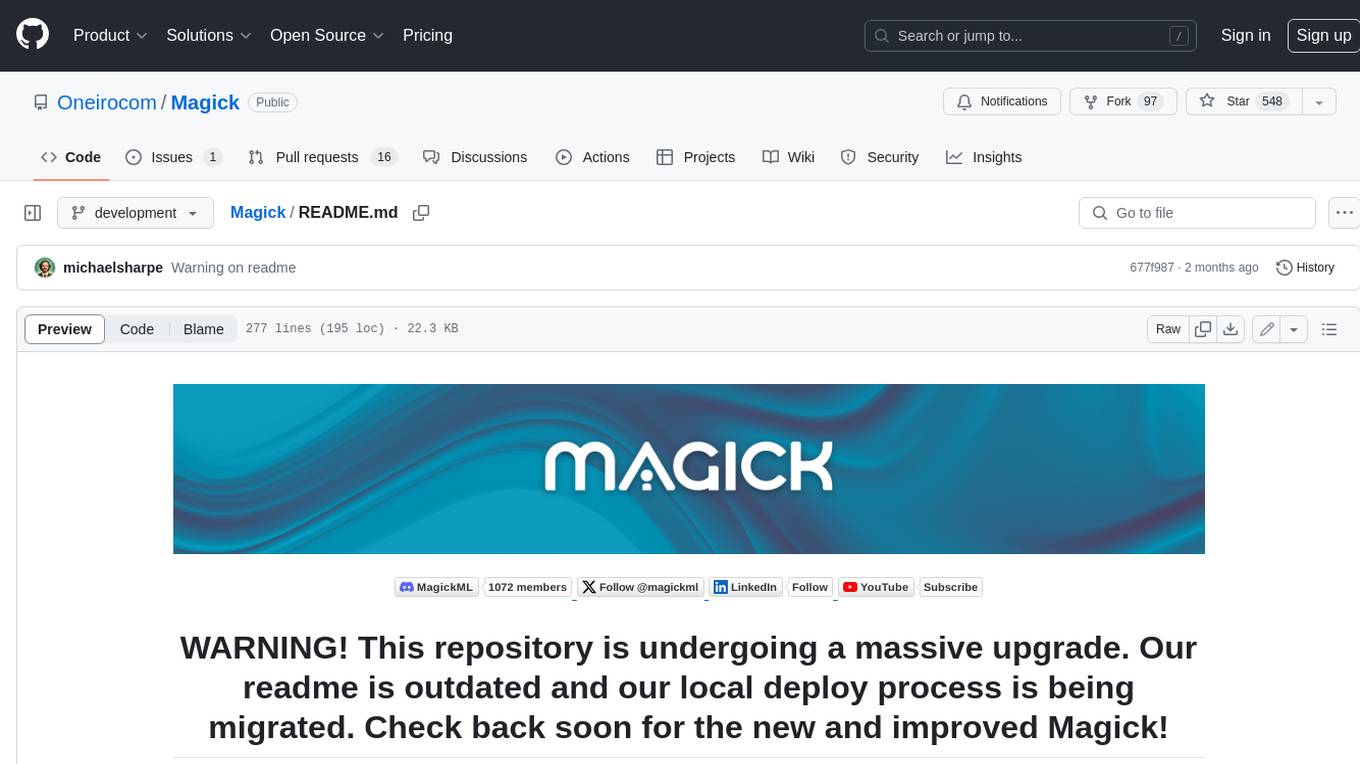
Magick
Magick is a groundbreaking visual AIDE (Artificial Intelligence Development Environment) for no-code data pipelines and multimodal agents. Magick can connect to other services and comes with nodes and templates well-suited for intelligent agents, chatbots, complex reasoning systems and realistic characters.