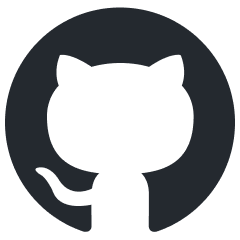
simple_GRPO
A very simple GRPO implement for reproducing r1-like LLM thinking.
Stars: 605
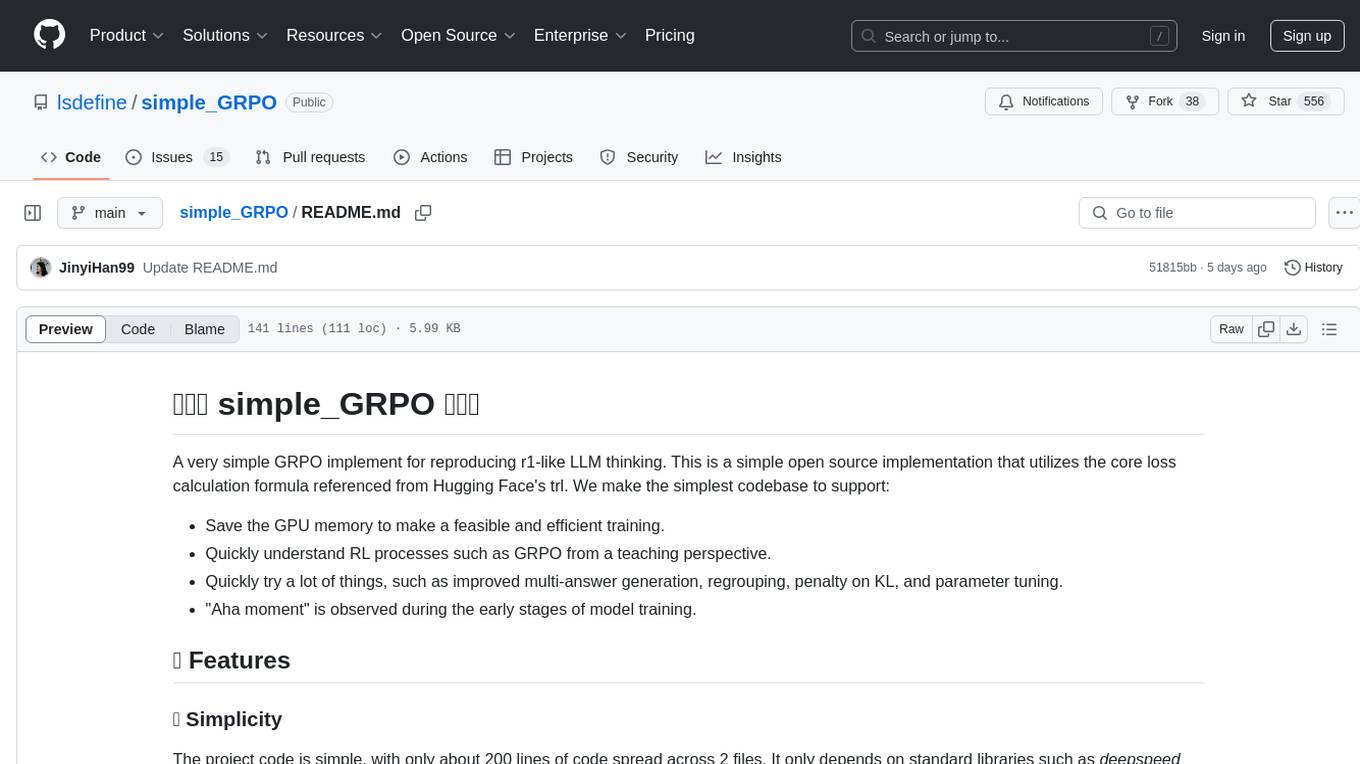
simple_GRPO is a very simple implementation of the GRPO algorithm for reproducing r1-like LLM thinking. It provides a codebase that supports saving GPU memory, understanding RL processes, trying various improvements like multi-answer generation, regrouping, penalty on KL, and parameter tuning. The project focuses on simplicity, performance, and core loss calculation based on Hugging Face's trl. It offers a straightforward setup with minimal dependencies and efficient training on multiple GPUs.
README:
A very simple GRPO implement for reproducing r1-like LLM thinking. This is a simple open source implementation that utilizes the core loss calculation formula referenced from Hugging Face's trl. We make the simplest codebase to support:
- Save the GPU memory to make a feasible and efficient training.
- Quickly understand RL processes such as GRPO from a teaching perspective.
- Quickly try a lot of things, such as improved multi-answer generation, regrouping, penalty on KL, and parameter tuning.
- "Aha moment" is observed during the early stages of model training.
- 2025/02/19: Added a loss triton implementation, which has a little speedup, but you can choose not to use it. See simple_grpo_v1 fold
- 2025/02/19: Added regroup version, implemented sampling of generated data on ref_server. See regroup_ver fold
- 2025/02/27: Added vllm package to accelerate the inference.
The project code is simple, with only about 200 lines of code spread across 2 files. It only depends on standard libraries such as deepspeed and torch, without requiring dependencies like ray. It is designed to allow for more complex interventions.
The reference model part is decoupled, which allows it to be run on different GPUs (even on a different machine with 4090). This avoids having the reference model and the training model on the same GPU, preventing multiple copies created by torchβs multiprocessing, and enabling training of a 7B model on 80G A800.
Training completed in under 1 hour on 1*A800 GPUs. Both Qwen2.5-7B and Qwen2.5-3B exhibited an "Aha moment" within the first 30 optimization steps.
The loss calculation formula is based on Hugging Face's trl. We extend our gratitude to Hugging Face for their contribution.
The runtime environment is in the requirements.txt so you can
pip install -r requirements.txt
At least two GPUs are needed.
Run the following command:
CUDA_VISIBLE_DEVICES=7 python ref_server.py
This just uses one GPU to collect and run the reference model.
In grpo_vllm_one.py, set the generation device index βrelative to the visible devicesβ in next step:
gen_device = 1
Then, open another bash:
CUDA_VISIBLE_DEVICES=2,3,4,5,6 deepspeed grpo_vllm_one.py
- Runtime Environment
- Hardware Setup: 2ΓA800 (80GB) GPUs
- Configuration:
- Training: 1 GPU with Zero-Stage 2 optimization
- Inference: Dedicated 1 GPU (3090/4090 compatible)
- Training Performance
Model | Steps | Time |
---|---|---|
Qwen2.5-3B | 60 | 12m 34s |
Qwen2.5-7B | 60 | 16m 40s |
2.1 Qwen2.5-3B
- Accuracy:
- Stabilizes above 60% after 5 optimization steps
- Peaks at ~70%
- Format Compliance:
- Reaches β100% after 30 steps
2.2 Qwen2.5-7B
- Accuracy:
- Maintains >90% throughout training
- Format Compliance:
- Achieves 100% within 30 steps
- Convergence: Both metrics show rapid convergence
- Qwen2.5-3B (Step 20)
<think>
... To find out how many more eggs Camden painted than Arnold, we subtract the number of eggs painted by Arnold from the number of eggs painted by Camden. So, 21 - 28 = -7. However, this result doesn't make sense in the context of the problem, as Camden cannot have painted fewer eggs than Arnold. Let's reconsider the initial solution steps: we should verify if the initial assumption about the relationship between Camden and Sarah's eggs is correct. ...</think>
<answer>
-7</answer>
- Qwen2.5-7B (Step 20)
<think>
... Therefore, Joanne gathered 350 apples from the average trees. However, this doesn't seem right because the total should be 500 and we've already accounted for 150, leaving room only for 350 from the average trees, which contradicts the total. Let's reassess. ...</think>
<answer>
350</answer>
- Answer generation may be invalid due to a group containing all wrong answers or all correct answers. We need group reorganization and better answer generation.
- GPU memory is still tight if it generates long cots. We have to split the groups to make the batch smaller.
We have implemented and are testing these features. They will be available soon.
This project is led by Dr. Jiaqing Liang and Professor Yanghua Xiao from KnowledgeWorks Lab, Fudan University. The core development team includes Ph.D. candidate Jinyi Han, Master's student Xinyi Wang, and other contributors. We gratefully acknowledge their dedication to this work.
If you find the code in our project useful, please consider citing our work as follows:
@misc{KW-R1,
author = {Jiaqing Liang, Jinyi Han, Xinyi Wang, Zishang Jiang, Chengyuan Xiong, Boyu Zhu, Jie Shi, Weijia Li, Tingyun Li, Yanghua Xiao},
title = {KW-R1: A Simple Implementation of the GRPO Algorithm},
year = {2025},
publisher = {GitHub},
journal = {GitHub repository},
howpublished = {\url{https://github.com/lsdefine/simple_GRPO}},
}
For Tasks:
Click tags to check more tools for each tasksFor Jobs:
Alternative AI tools for simple_GRPO
Similar Open Source Tools
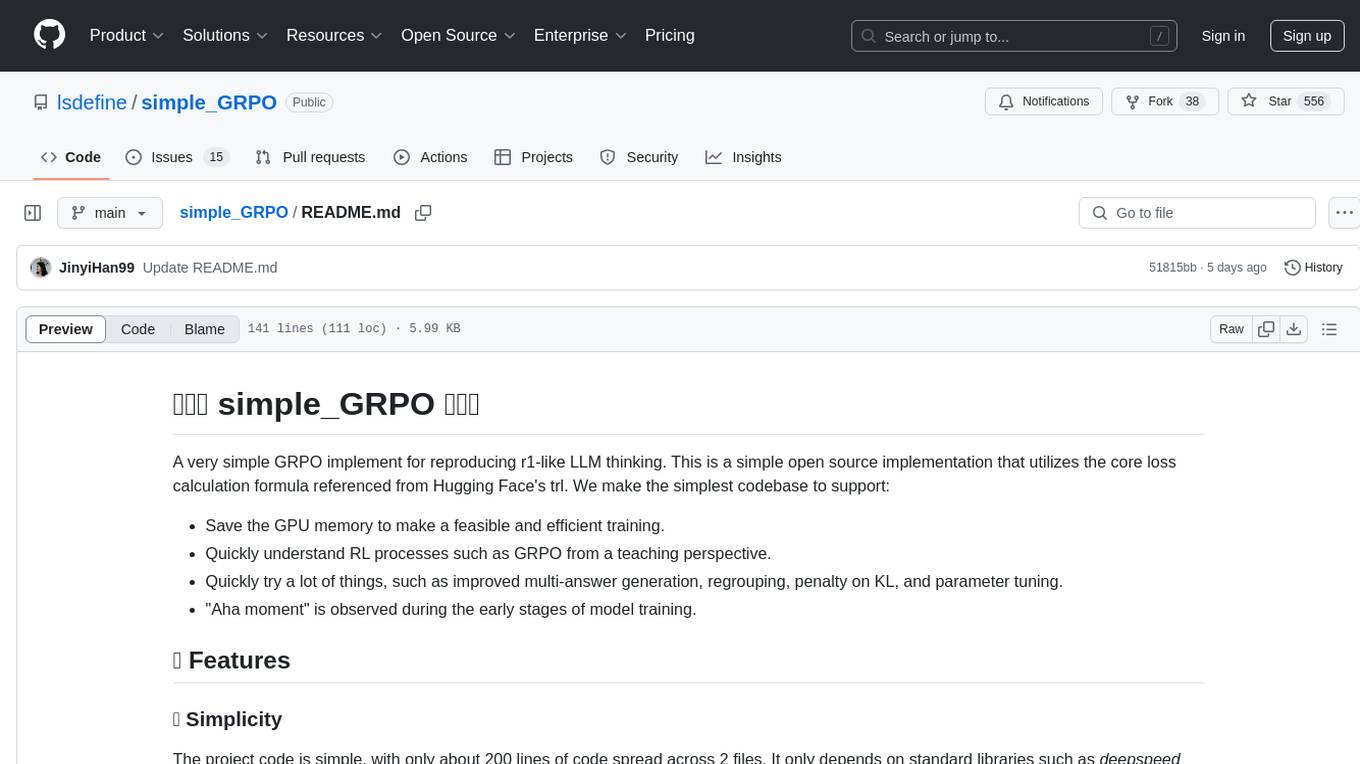
simple_GRPO
simple_GRPO is a very simple implementation of the GRPO algorithm for reproducing r1-like LLM thinking. It provides a codebase that supports saving GPU memory, understanding RL processes, trying various improvements like multi-answer generation, regrouping, penalty on KL, and parameter tuning. The project focuses on simplicity, performance, and core loss calculation based on Hugging Face's trl. It offers a straightforward setup with minimal dependencies and efficient training on multiple GPUs.
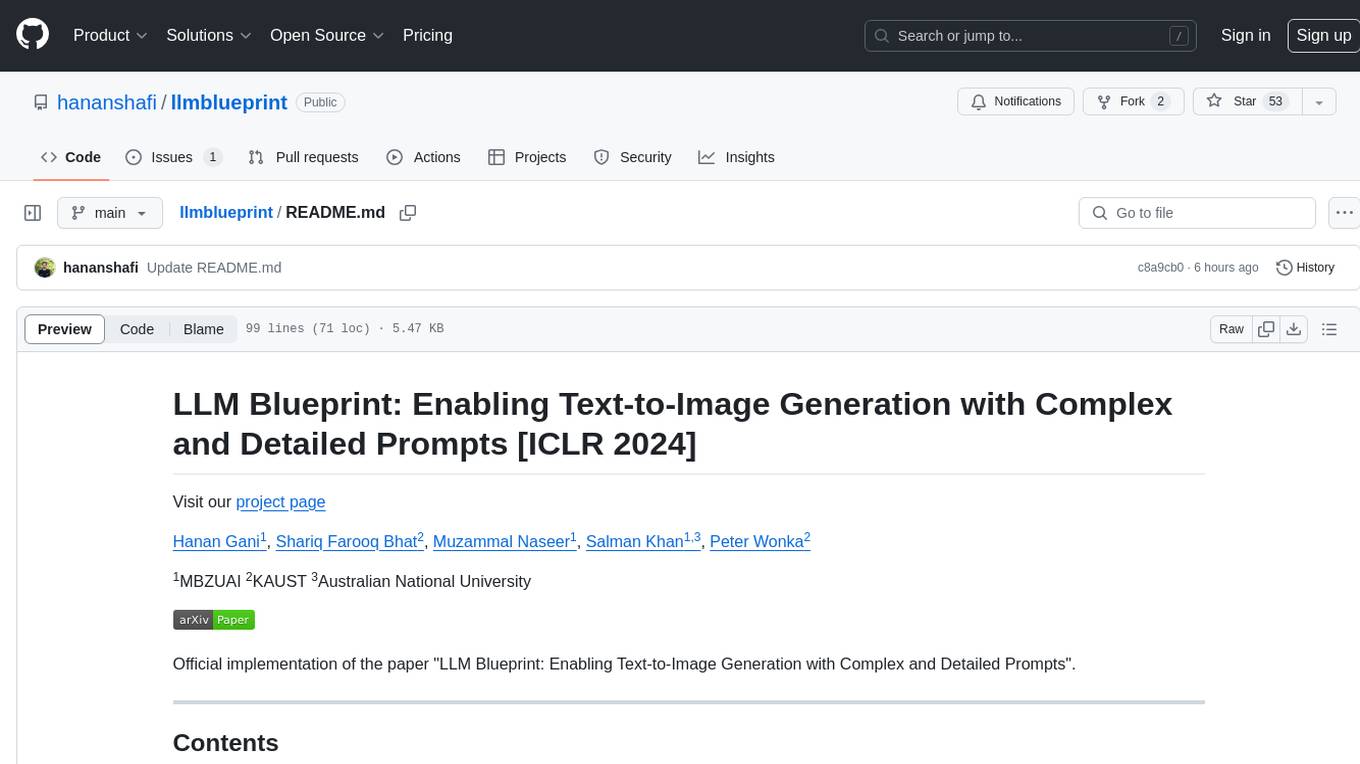
llmblueprint
LLM Blueprint is an official implementation of a paper that enables text-to-image generation with complex and detailed prompts. It leverages Large Language Models (LLMs) to extract critical components from text prompts, including bounding box coordinates for foreground objects, detailed textual descriptions for individual objects, and a succinct background context. The tool operates in two phases: Global Scene Generation creates an initial scene using object layouts and background context, and an Iterative Refinement Scheme refines box-level content to align with textual descriptions, ensuring consistency and improving recall compared to baseline diffusion models.
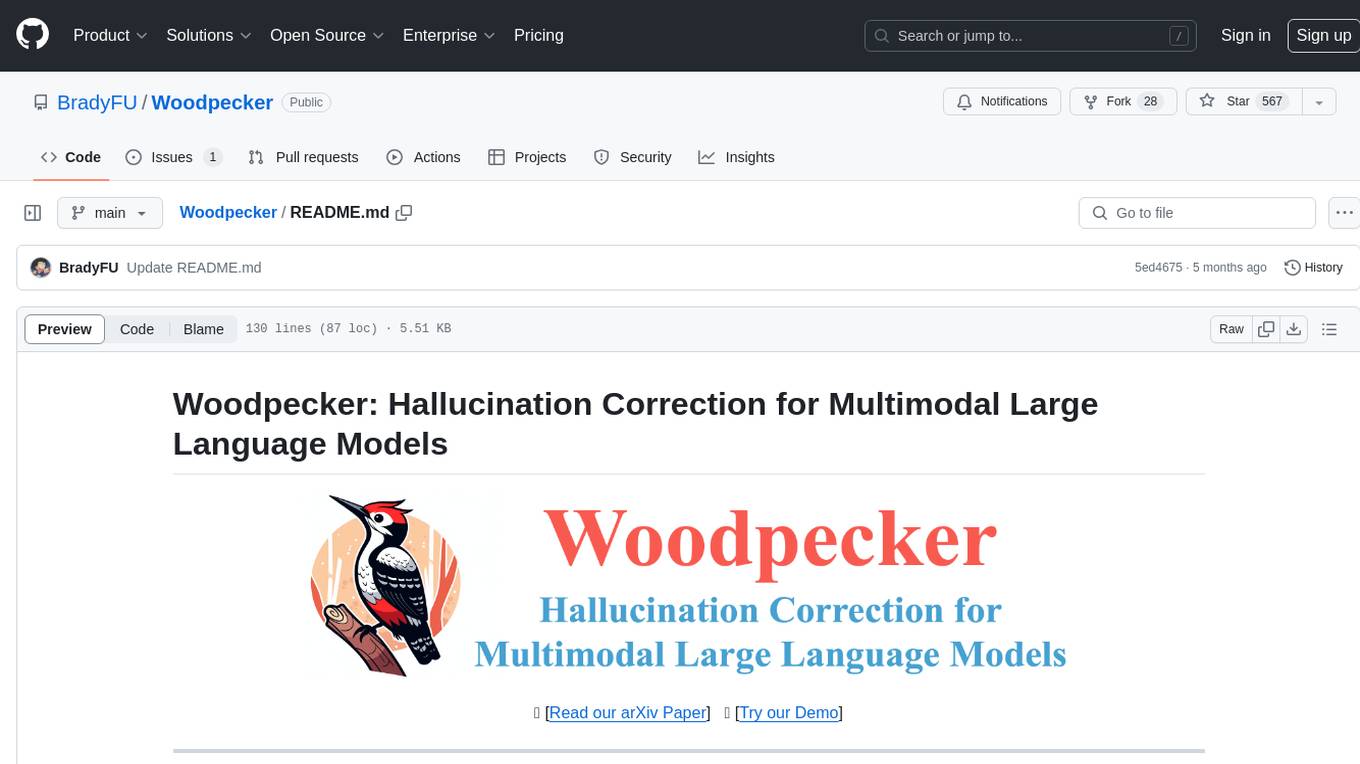
Woodpecker
Woodpecker is a tool designed to correct hallucinations in Multimodal Large Language Models (MLLMs) by introducing a training-free method that picks out and corrects inconsistencies between generated text and image content. It consists of five stages: key concept extraction, question formulation, visual knowledge validation, visual claim generation, and hallucination correction. Woodpecker can be easily integrated with different MLLMs and provides interpretable results by accessing intermediate outputs of the stages. The tool has shown significant improvements in accuracy over baseline models like MiniGPT-4 and mPLUG-Owl.
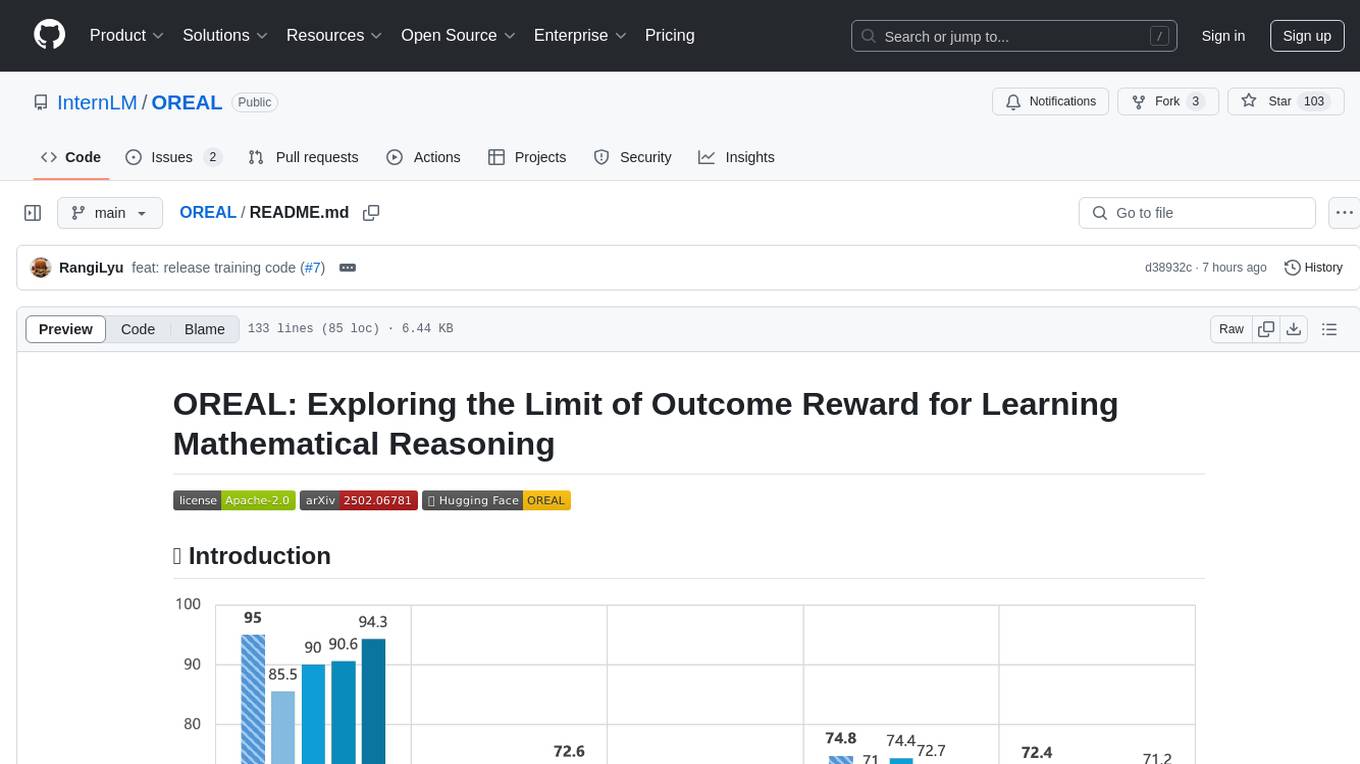
OREAL
OREAL is a reinforcement learning framework designed for mathematical reasoning tasks, aiming to achieve optimal performance through outcome reward-based learning. The framework utilizes behavior cloning, reshaping rewards, and token-level reward models to address challenges in sparse rewards and partial correctness. OREAL has achieved significant results, with a 7B model reaching 94.0 pass@1 accuracy on MATH-500 and surpassing previous 32B models. The tool provides training tutorials and Hugging Face model repositories for easy access and implementation.
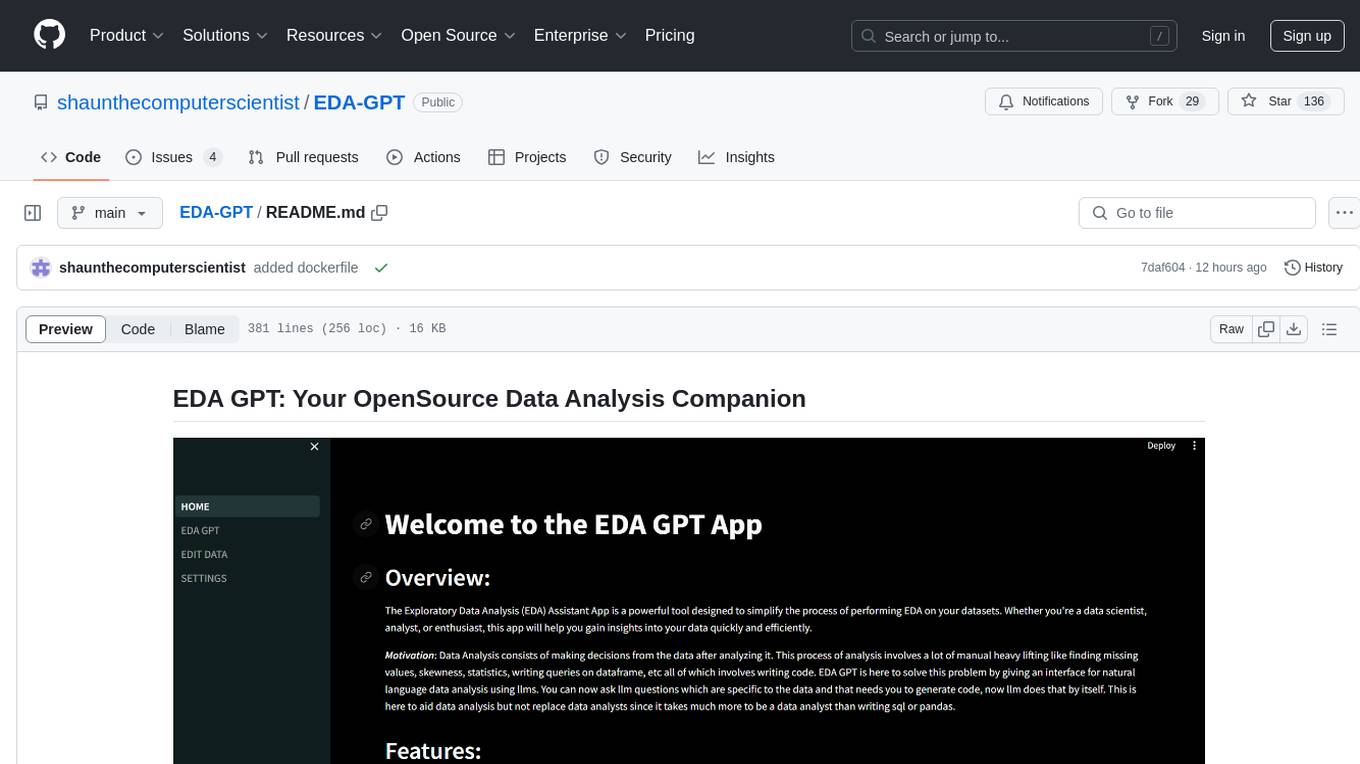
EDA-GPT
EDA GPT is an open-source data analysis companion that offers a comprehensive solution for structured and unstructured data analysis. It streamlines the data analysis process, empowering users to explore, visualize, and gain insights from their data. EDA GPT supports analyzing structured data in various formats like CSV, XLSX, and SQLite, generating graphs, and conducting in-depth analysis of unstructured data such as PDFs and images. It provides a user-friendly interface, powerful features, and capabilities like comparing performance with other tools, analyzing large language models, multimodal search, data cleaning, and editing. The tool is optimized for maximal parallel processing, searching internet and documents, and creating analysis reports from structured and unstructured data.
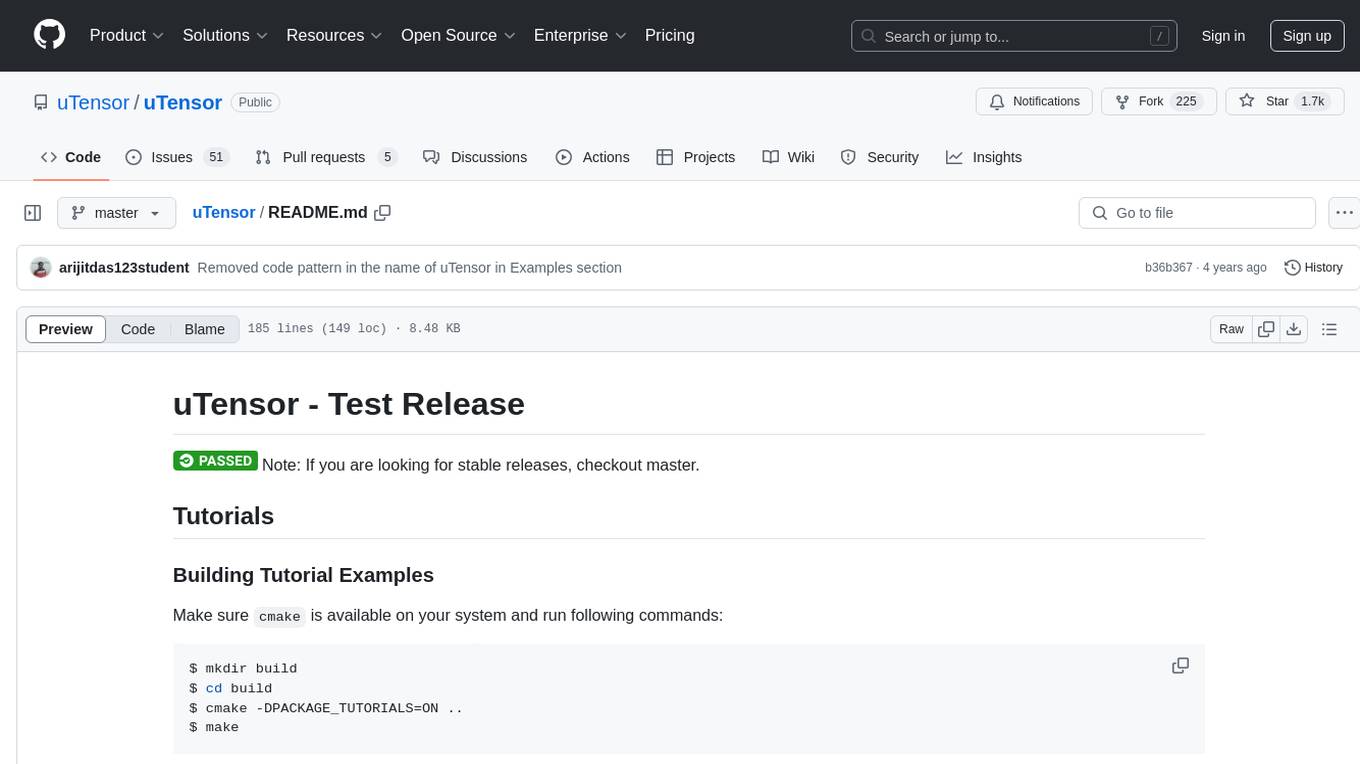
uTensor
uTensor is an extremely light-weight machine learning inference framework built on Tensorflow and optimized for Arm targets. It consists of a runtime library and an offline tool that handles most of the model translation work. The core runtime is only ~2KB. The workflow involves constructing and training a model in Tensorflow, then using uTensor to produce C++ code for inferencing. The runtime ensures system safety, guarantees RAM usage, and focuses on clear, concise, and debuggable code. The high-level API simplifies tensor handling and operator execution for embedded systems.
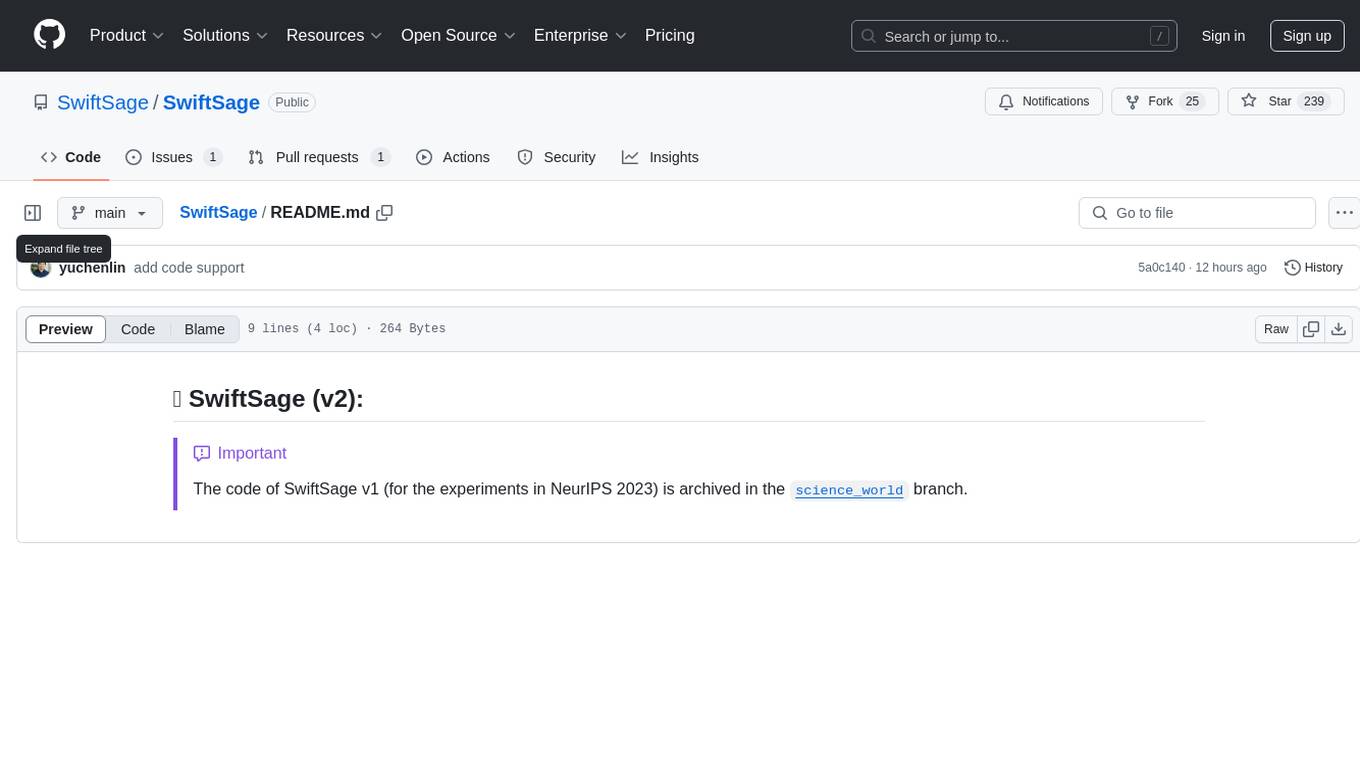
SwiftSage
SwiftSage is a tool designed for conducting experiments in the field of machine learning and artificial intelligence. It provides a platform for researchers and developers to implement and test various algorithms and models. The tool is particularly useful for exploring new ideas and conducting experiments in a controlled environment. SwiftSage aims to streamline the process of developing and testing machine learning models, making it easier for users to iterate on their ideas and achieve better results. With its user-friendly interface and powerful features, SwiftSage is a valuable tool for anyone working in the field of AI and ML.
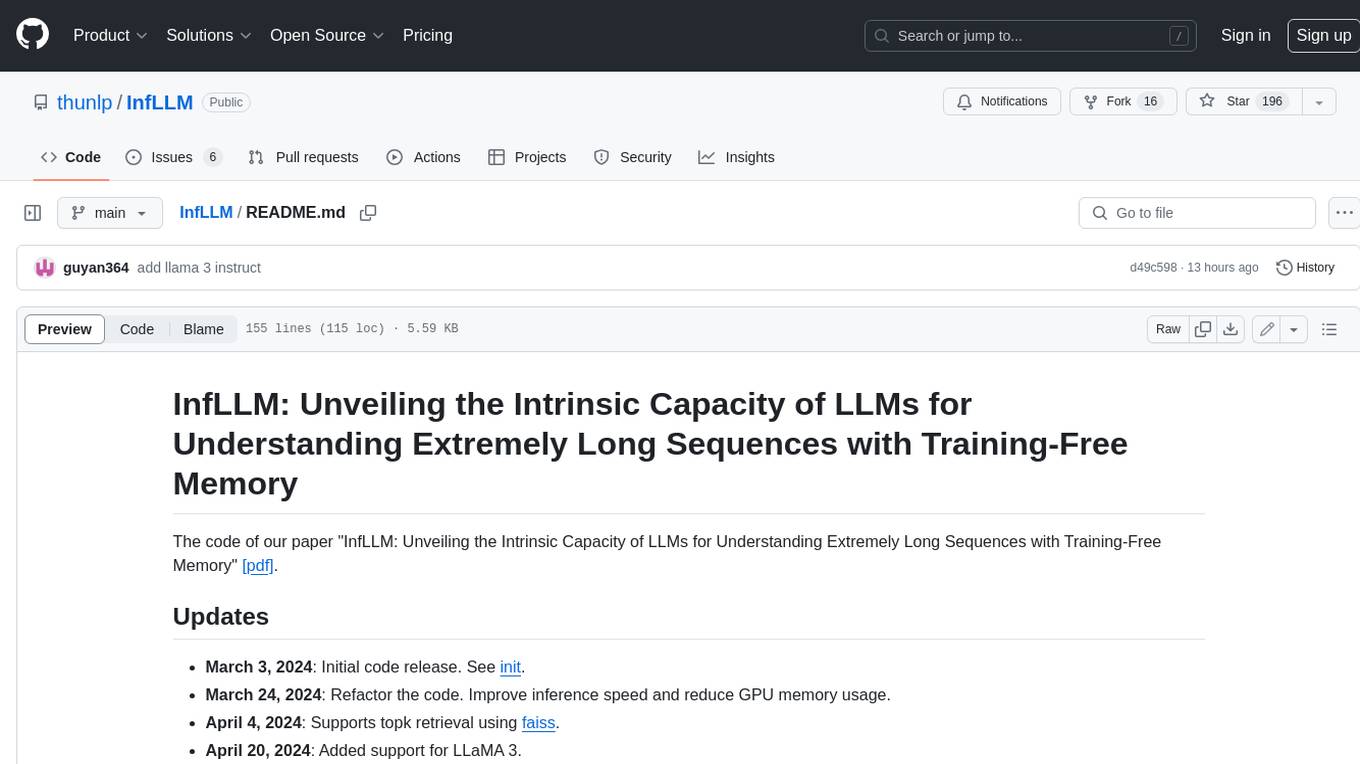
InfLLM
InfLLM is a training-free memory-based method that unveils the intrinsic ability of LLMs to process streaming long sequences. It stores distant contexts into additional memory units and employs an efficient mechanism to lookup token-relevant units for attention computation. Thereby, InfLLM allows LLMs to efficiently process long sequences while maintaining the ability to capture long-distance dependencies. Without any training, InfLLM enables LLMs pre-trained on sequences of a few thousand tokens to achieve superior performance than competitive baselines continually training these LLMs on long sequences. Even when the sequence length is scaled to 1, 024K, InfLLM still effectively captures long-distance dependencies.
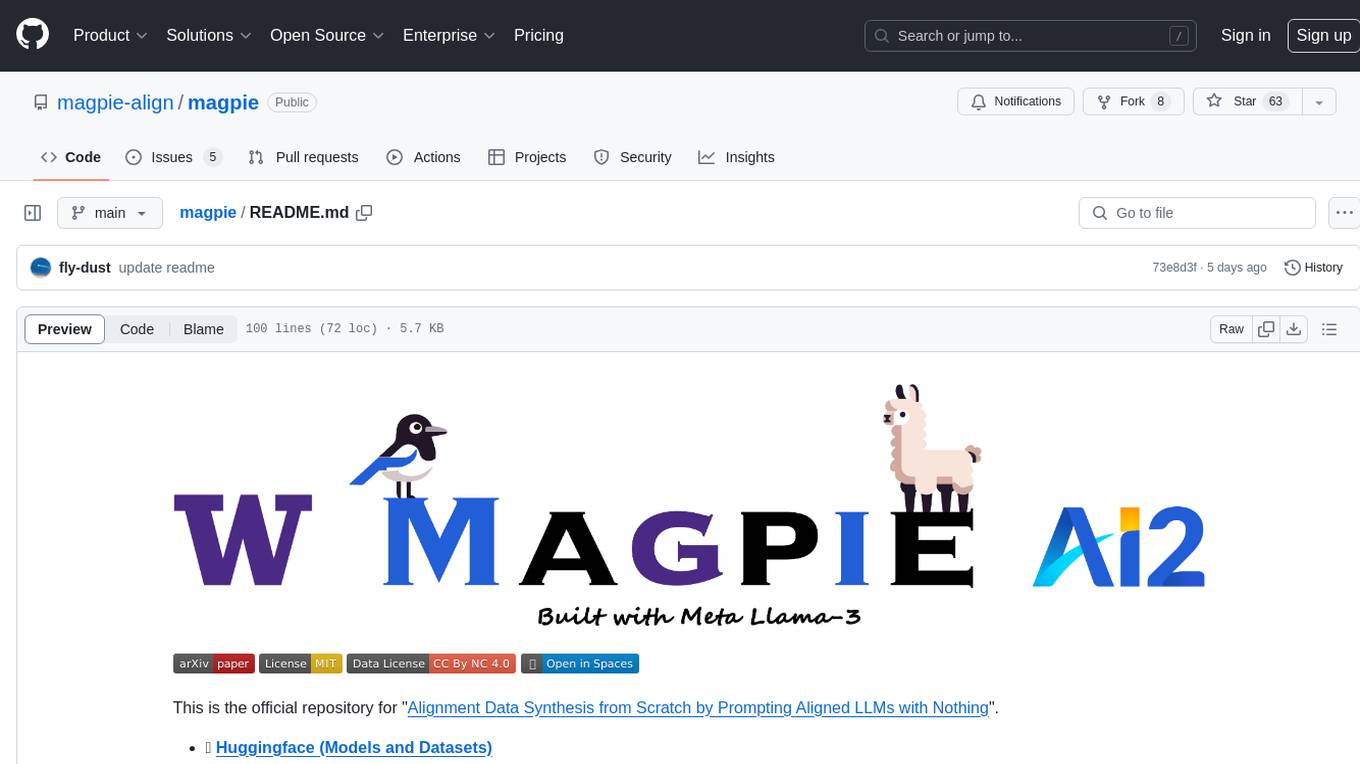
magpie
This is the official repository for 'Alignment Data Synthesis from Scratch by Prompting Aligned LLMs with Nothing'. Magpie is a tool designed to synthesize high-quality instruction data at scale by extracting it directly from an aligned Large Language Models (LLMs). It aims to democratize AI by generating large-scale alignment data and enhancing the transparency of model alignment processes. Magpie has been tested on various model families and can be used to fine-tune models for improved performance on alignment benchmarks such as AlpacaEval, ArenaHard, and WildBench.
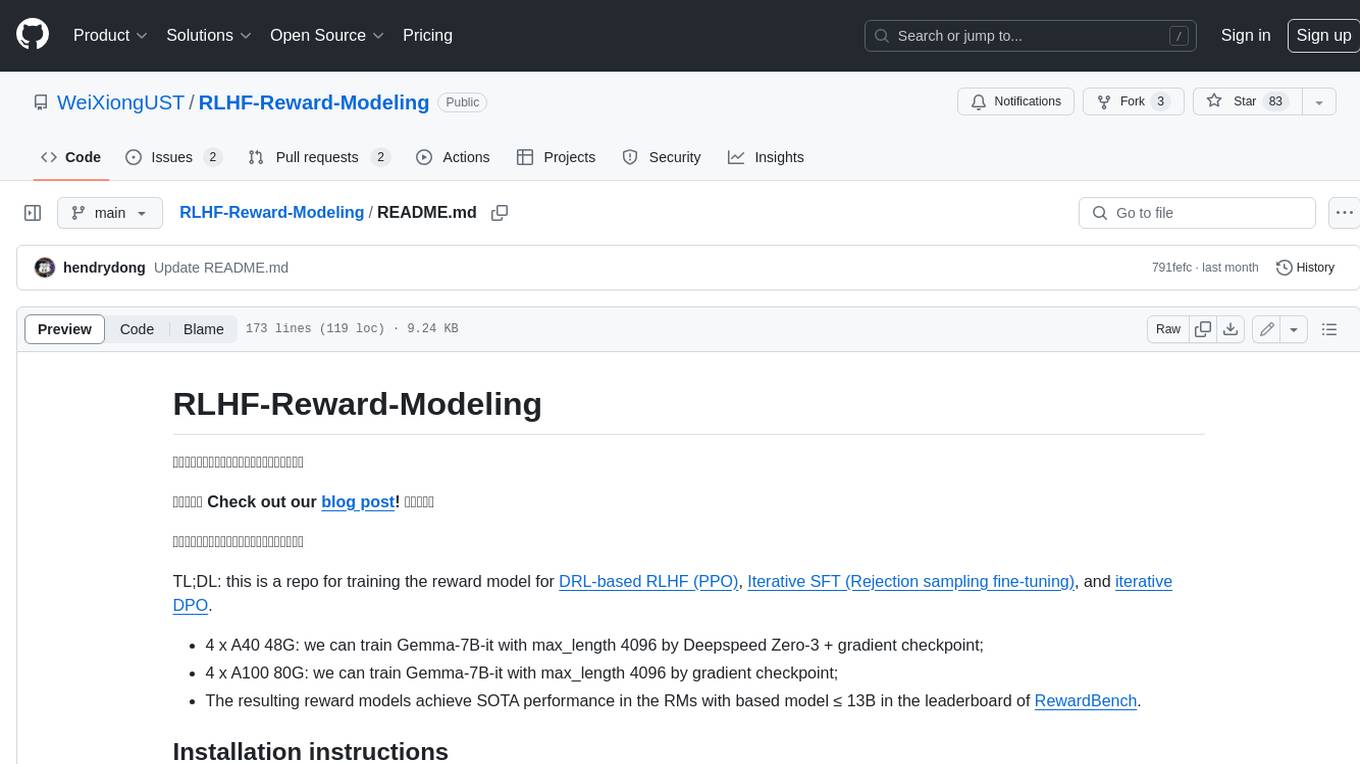
RLHF-Reward-Modeling
This repository contains code for training reward models for Deep Reinforcement Learning-based Reward-modulated Hierarchical Fine-tuning (DRL-based RLHF), Iterative Selection Fine-tuning (Rejection sampling fine-tuning), and iterative Decision Policy Optimization (DPO). The reward models are trained using a Bradley-Terry model based on the Gemma and Mistral language models. The resulting reward models achieve state-of-the-art performance on the RewardBench leaderboard for reward models with base models of up to 13B parameters.
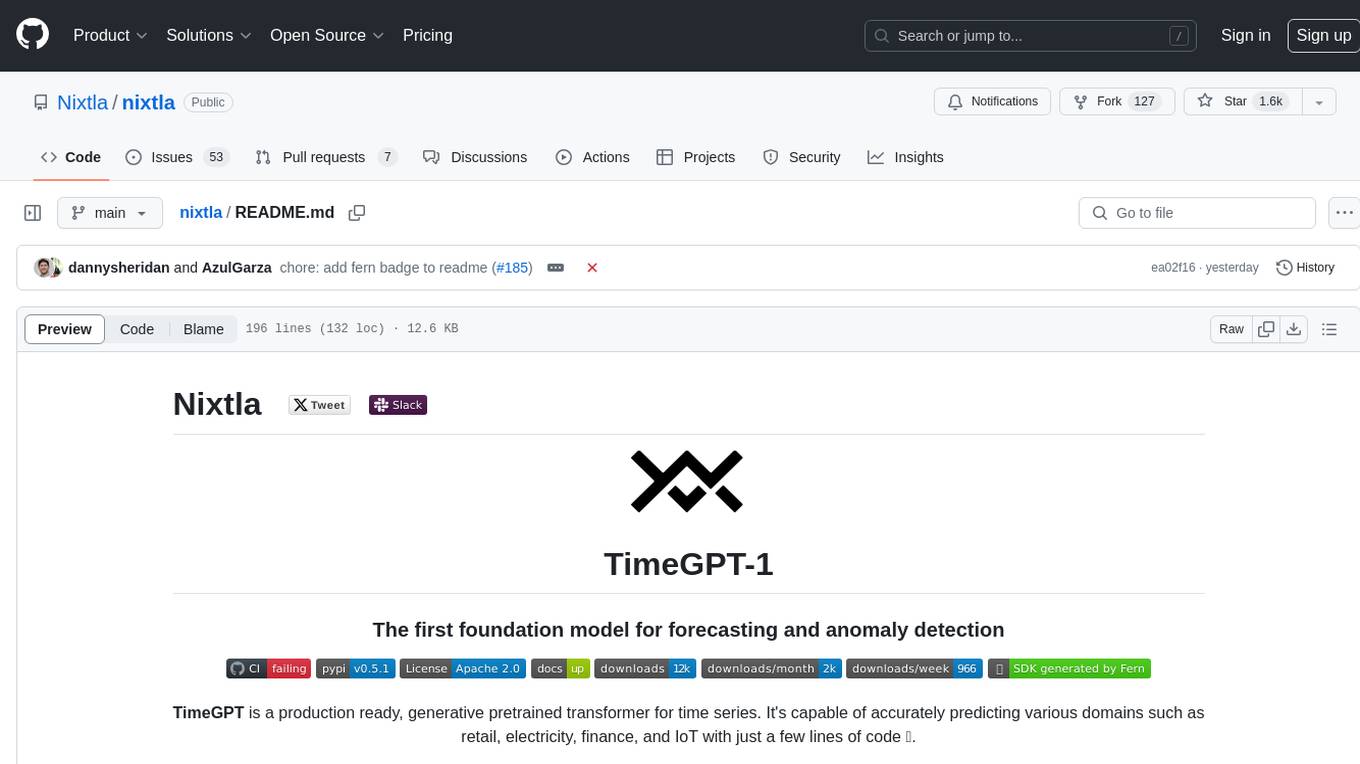
nixtla
Nixtla is a production-ready generative pretrained transformer for time series forecasting and anomaly detection. It can accurately predict various domains such as retail, electricity, finance, and IoT with just a few lines of code. TimeGPT introduces a paradigm shift with its standout performance, efficiency, and simplicity, making it accessible even to users with minimal coding experience. The model is based on self-attention and is independently trained on a vast time series dataset to minimize forecasting error. It offers features like zero-shot inference, fine-tuning, API access, adding exogenous variables, multiple series forecasting, custom loss function, cross-validation, prediction intervals, and handling irregular timestamps.
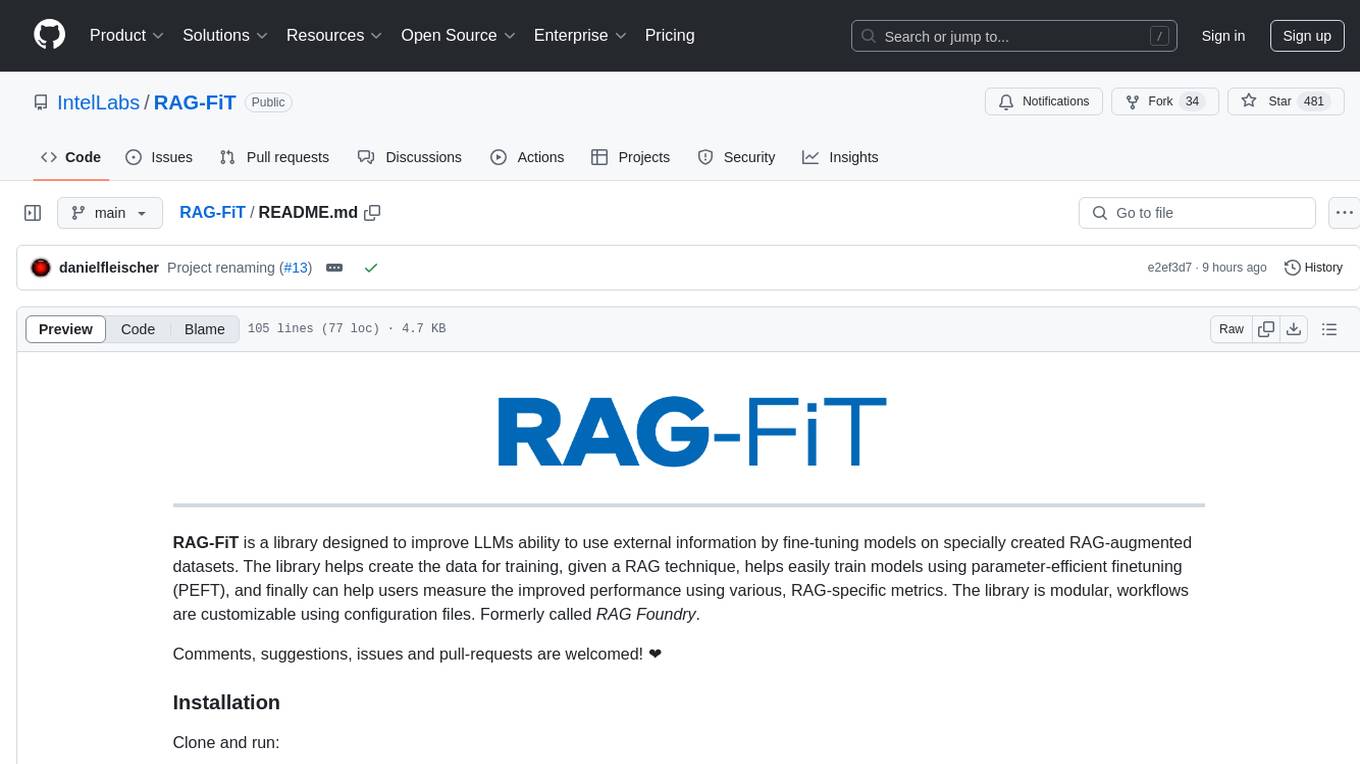
RAG-FiT
RAG-FiT is a library designed to improve Language Models' ability to use external information by fine-tuning models on specially created RAG-augmented datasets. The library assists in creating training data, training models using parameter-efficient finetuning (PEFT), and evaluating performance using RAG-specific metrics. It is modular, customizable via configuration files, and facilitates fast prototyping and experimentation with various RAG settings and configurations.
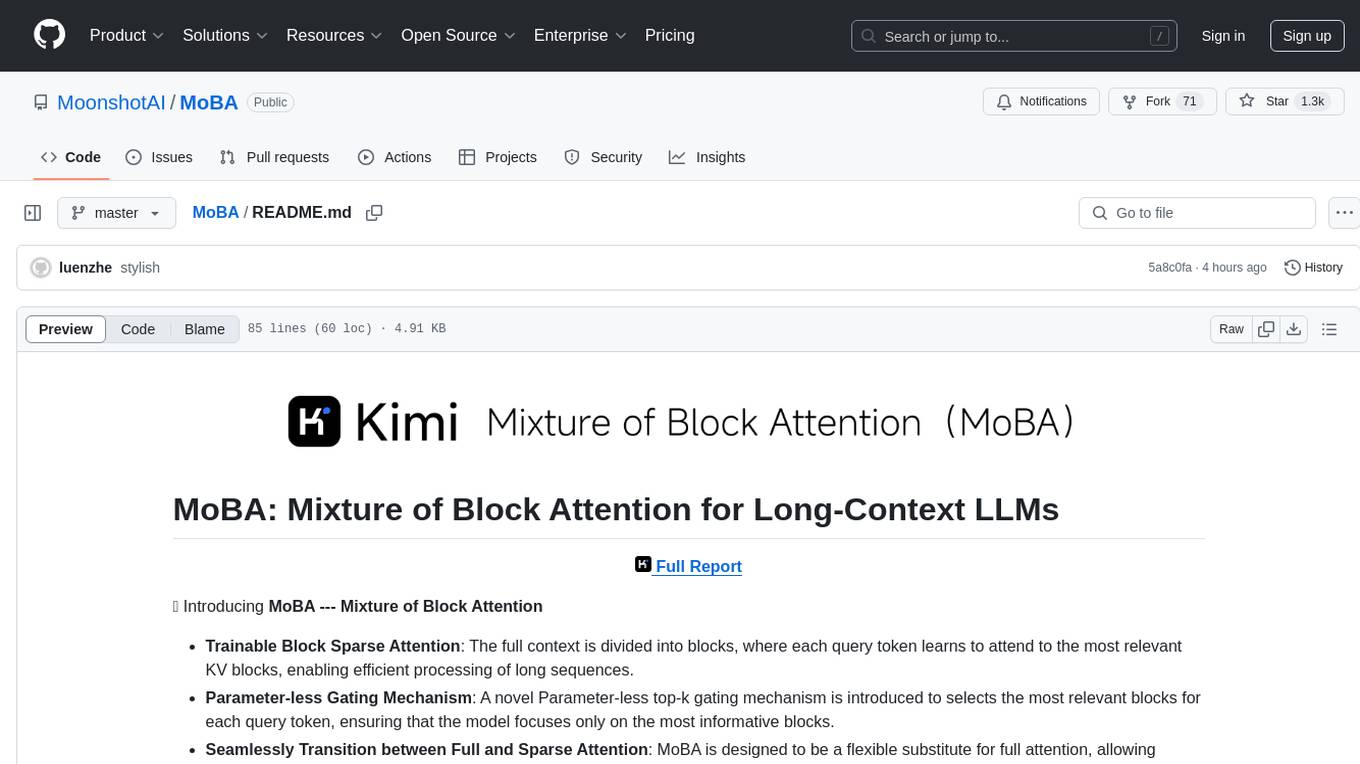
MoBA
MoBA (Mixture of Block Attention) is an innovative approach for long-context language models, enabling efficient processing of long sequences by dividing the full context into blocks and introducing a parameter-less gating mechanism. It allows seamless transitions between full and sparse attention modes, enhancing efficiency without compromising performance. MoBA has been deployed to support long-context requests and demonstrates significant advancements in efficient attention computation for large language models.
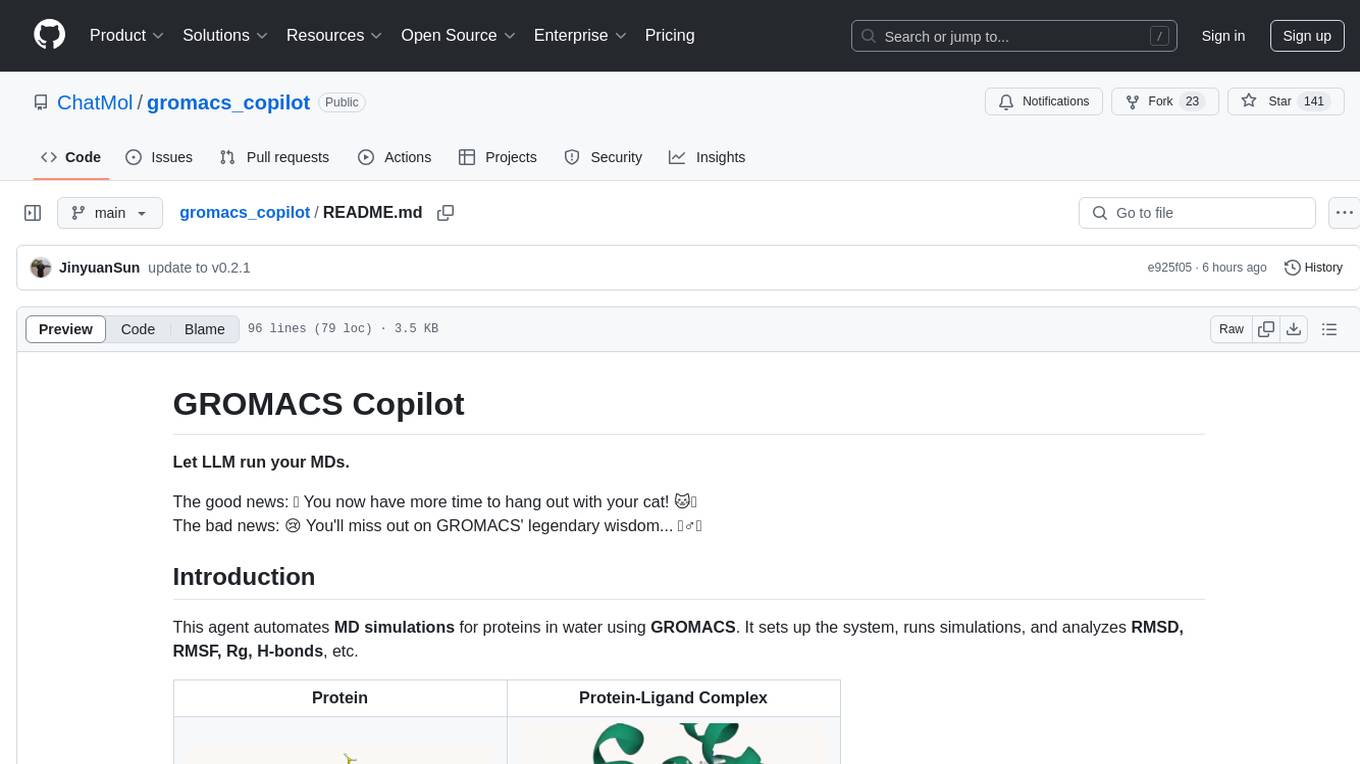
gromacs_copilot
GROMACS Copilot is an agent designed to automate molecular dynamics simulations for proteins in water using GROMACS. It handles system setup, simulation execution, and result analysis automatically, providing outputs such as RMSD, RMSF, Rg, and H-bonds. Users can interact with the agent through prompts and API keys from DeepSeek and OpenAI. The tool aims to simplify the process of running MD simulations, allowing users to focus on other tasks while it handles the technical aspects of the simulations.
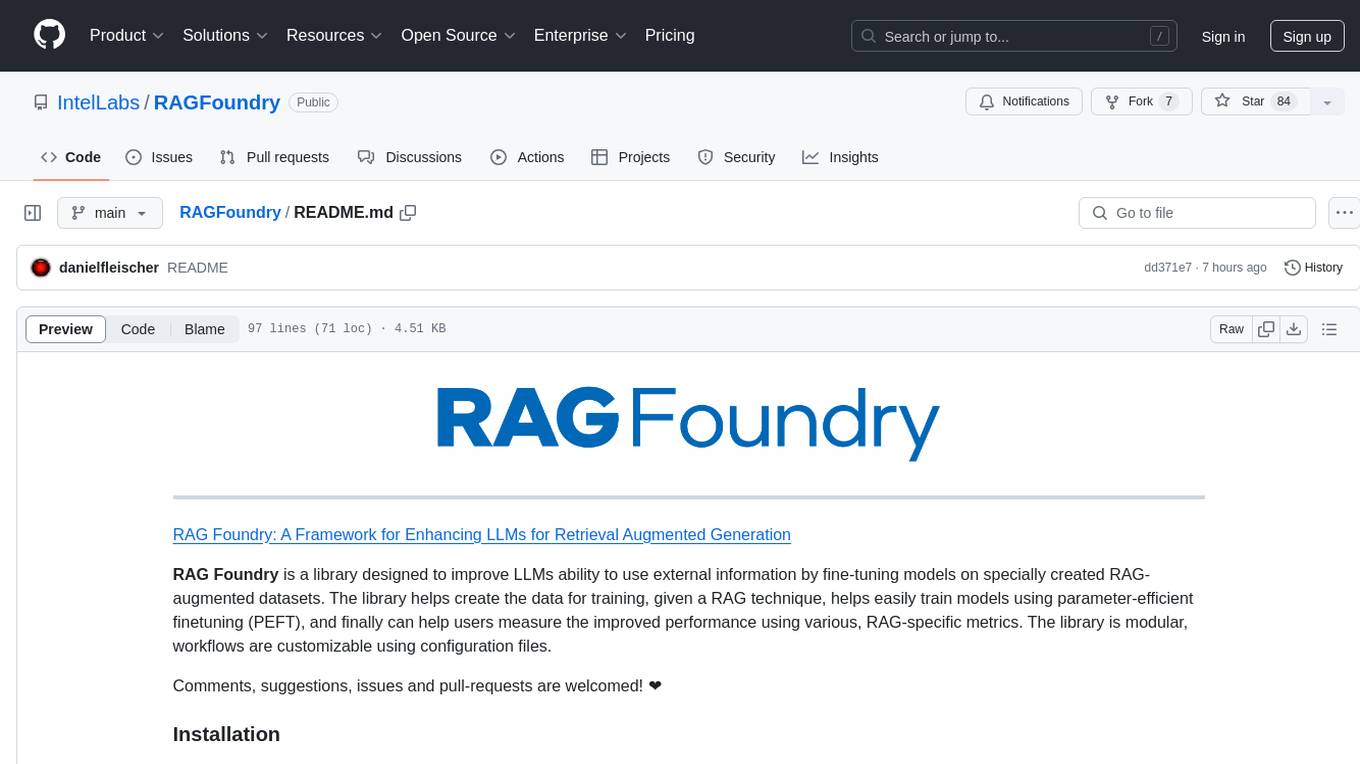
RAGFoundry
RAG Foundry is a library designed to enhance Large Language Models (LLMs) by fine-tuning models on RAG-augmented datasets. It helps create training data, train models using parameter-efficient finetuning (PEFT), and measure performance using RAG-specific metrics. The library is modular, customizable using configuration files, and facilitates prototyping with various RAG settings and configurations for tasks like data processing, retrieval, training, inference, and evaluation.
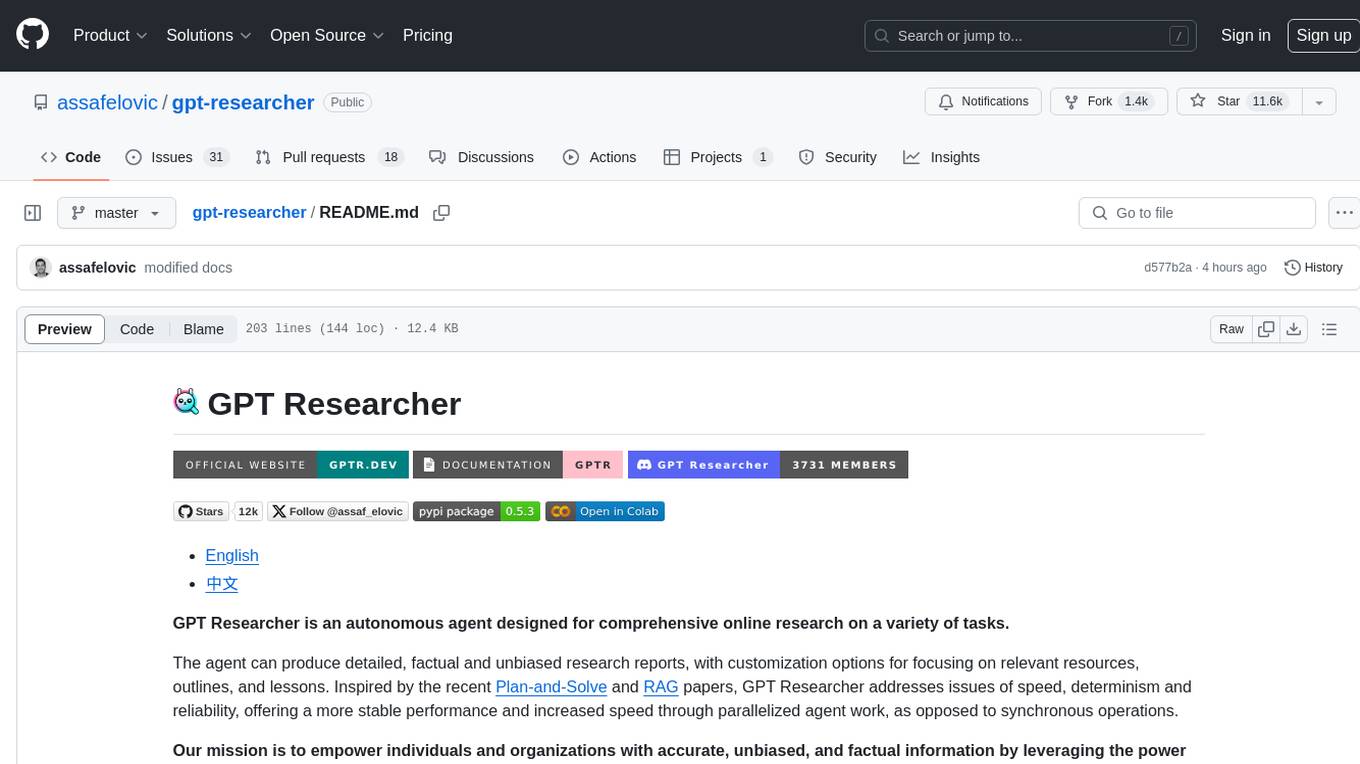
gpt-researcher
GPT Researcher is an autonomous agent designed for comprehensive online research on a variety of tasks. It can produce detailed, factual, and unbiased research reports with customization options. The tool addresses issues of speed, determinism, and reliability by leveraging parallelized agent work. The main idea involves running 'planner' and 'execution' agents to generate research questions, seek related information, and create research reports. GPT Researcher optimizes costs and completes tasks in around 3 minutes. Features include generating long research reports, aggregating web sources, an easy-to-use web interface, scraping web sources, and exporting reports to various formats.
For similar tasks
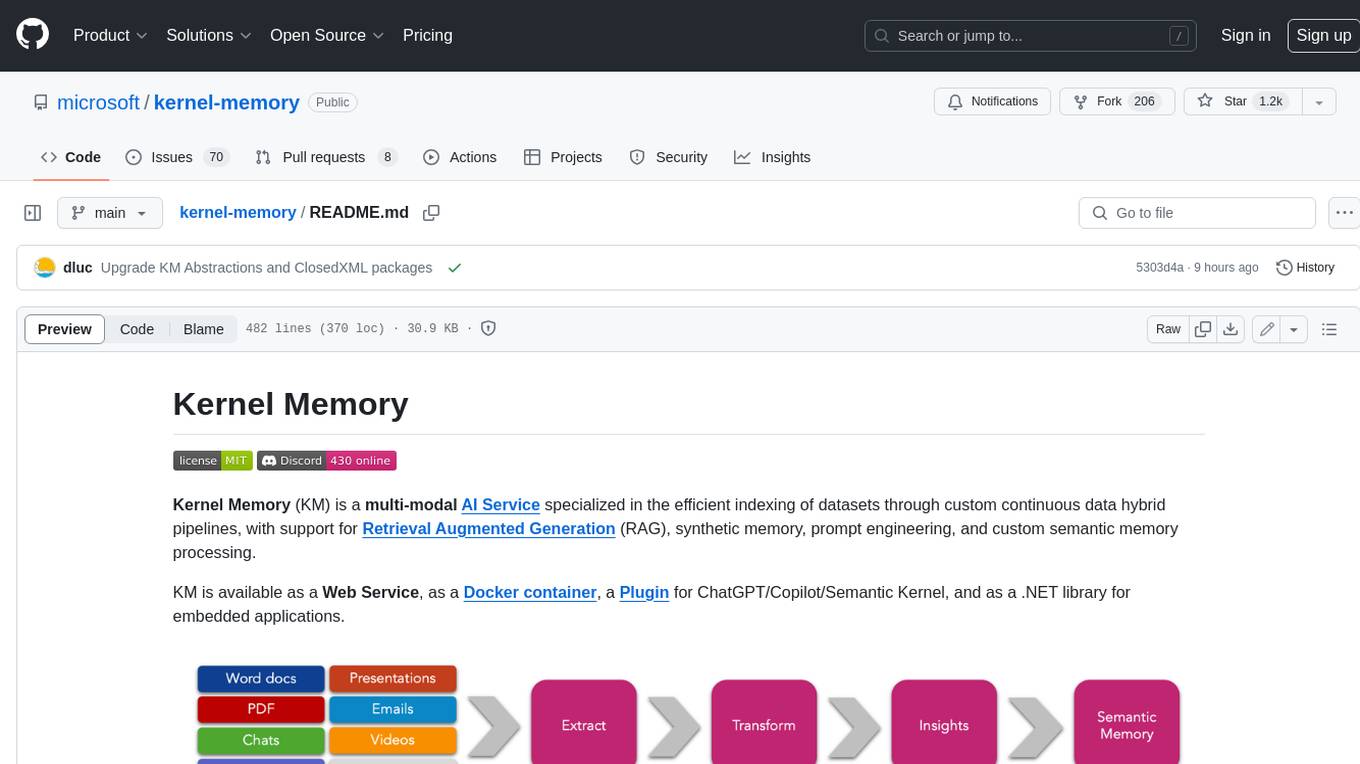
kernel-memory
Kernel Memory (KM) is a multi-modal AI Service specialized in the efficient indexing of datasets through custom continuous data hybrid pipelines, with support for Retrieval Augmented Generation (RAG), synthetic memory, prompt engineering, and custom semantic memory processing. KM is available as a Web Service, as a Docker container, a Plugin for ChatGPT/Copilot/Semantic Kernel, and as a .NET library for embedded applications. Utilizing advanced embeddings and LLMs, the system enables Natural Language querying for obtaining answers from the indexed data, complete with citations and links to the original sources. Designed for seamless integration as a Plugin with Semantic Kernel, Microsoft Copilot and ChatGPT, Kernel Memory enhances data-driven features in applications built for most popular AI platforms.
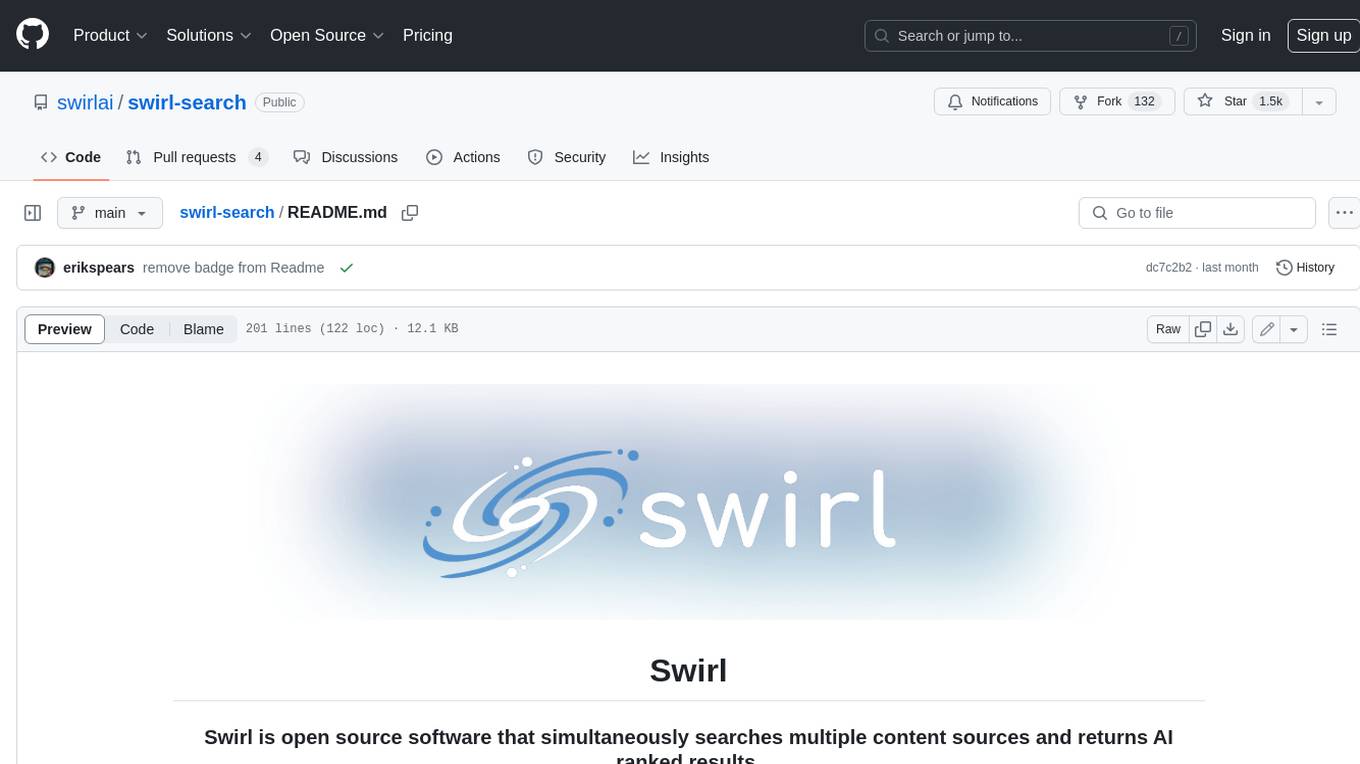
swirl-search
Swirl is an open-source software that allows users to simultaneously search multiple content sources and receive AI-ranked results. It connects to various data sources, including databases, public data services, and enterprise sources, and utilizes AI and LLMs to generate insights and answers based on the user's data. Swirl is easy to use, requiring only the download of a YML file, starting in Docker, and searching with Swirl. Users can add credentials to preloaded SearchProviders to access more sources. Swirl also offers integration with ChatGPT as a configured AI model. It adapts and distributes user queries to anything with a search API, re-ranking the unified results using Large Language Models without extracting or indexing anything. Swirl includes five Google Programmable Search Engines (PSEs) to get users up and running quickly. Key features of Swirl include Microsoft 365 integration, SearchProvider configurations, query adaptation, synchronous or asynchronous search federation, optional subscribe feature, pipelining of Processor stages, results stored in SQLite3 or PostgreSQL, built-in Query Transformation support, matching on word stems and handling of stopwords, duplicate detection, re-ranking of unified results using Cosine Vector Similarity, result mixers, page through all results requested, sample data sets, optional spell correction, optional search/result expiration service, easily extensible Connector and Mixer objects, and a welcoming community for collaboration and support.
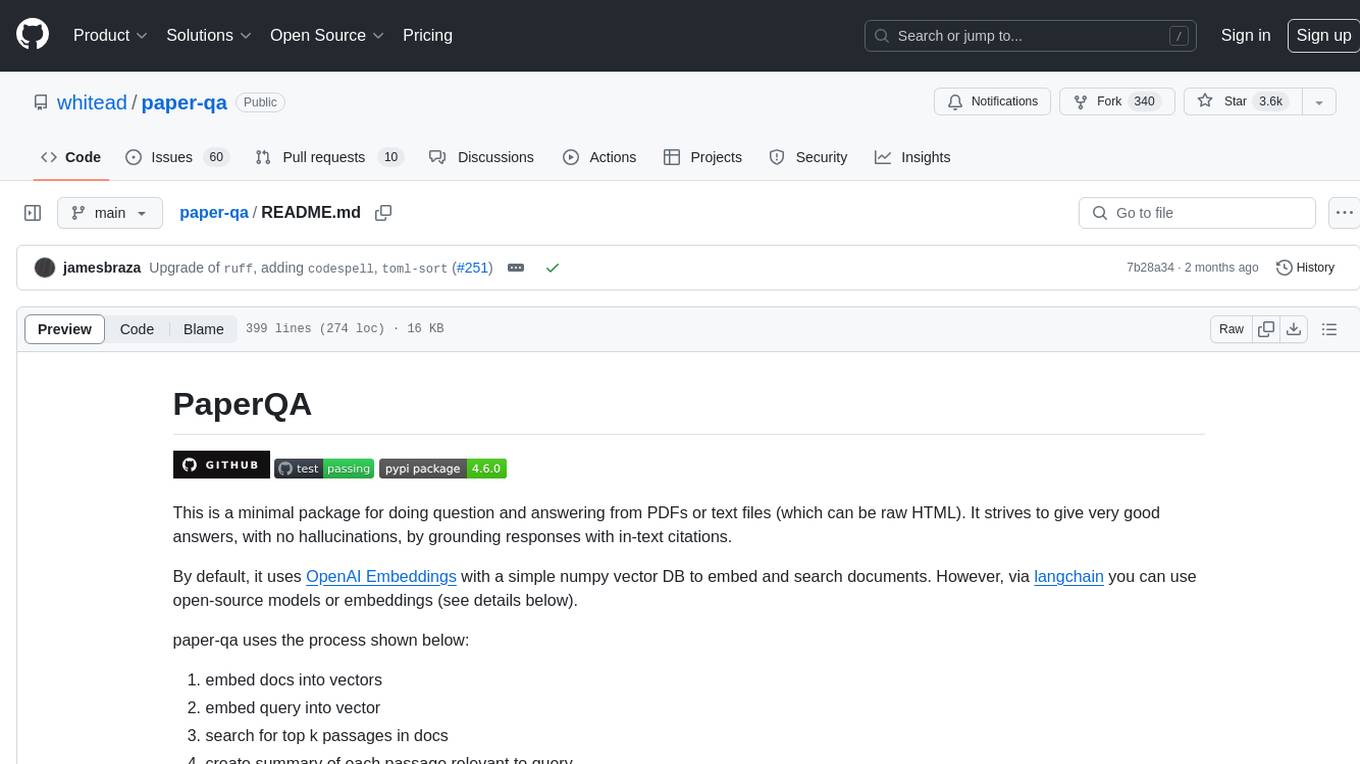
paper-qa
PaperQA is a minimal package for question and answering from PDFs or text files, providing very good answers with in-text citations. It uses OpenAI Embeddings to embed and search documents, and follows a process of embedding docs and queries, searching for top passages, creating summaries, scoring and selecting relevant summaries, putting summaries into prompt, and generating answers. Users can customize prompts and use various models for embeddings and LLMs. The tool can be used asynchronously and supports adding documents from paths, files, or URLs.
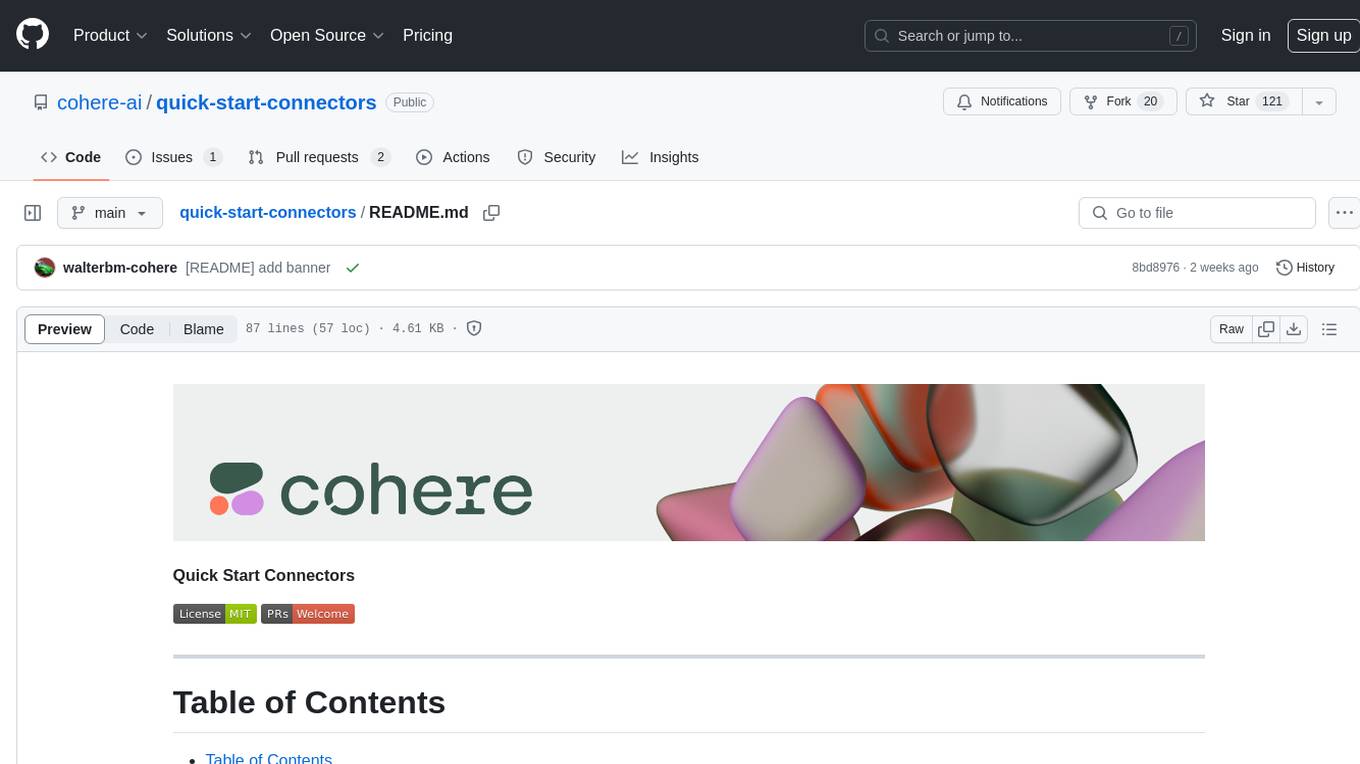
quick-start-connectors
Cohere's Build-Your-Own-Connector framework allows integration of Cohere's Command LLM via the Chat API endpoint to any datastore/software holding text information with a search endpoint. Enables user queries grounded in proprietary information. Use-cases include question/answering, knowledge working, comms summary, and research. Repository provides code for popular datastores and a template connector. Requires Python 3.11+ and Poetry. Connectors can be built and deployed using Docker. Environment variables set authorization values. Pre-commits for linting. Connectors tailored to integrate with Cohere's Chat API for creating chatbots. Connectors return documents as JSON objects for Cohere's API to generate answers with citations.
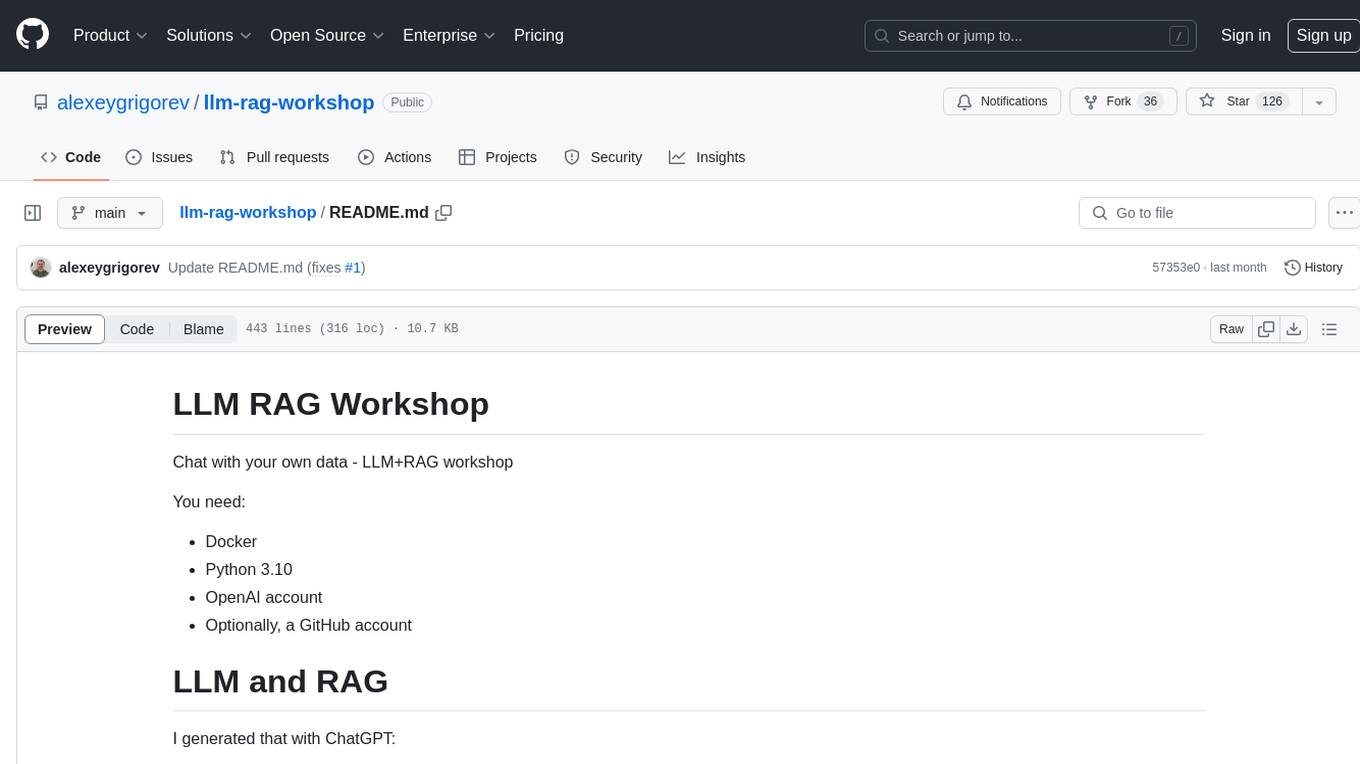
llm-rag-workshop
The LLM RAG Workshop repository provides a workshop on using Large Language Models (LLMs) and Retrieval-Augmented Generation (RAG) to generate and understand text in a human-like manner. It includes instructions on setting up the environment, indexing Zoomcamp FAQ documents, creating a Q&A system, and using OpenAI for generation based on retrieved information. The repository focuses on enhancing language model responses with retrieved information from external sources, such as document databases or search engines, to improve factual accuracy and relevance of generated text.
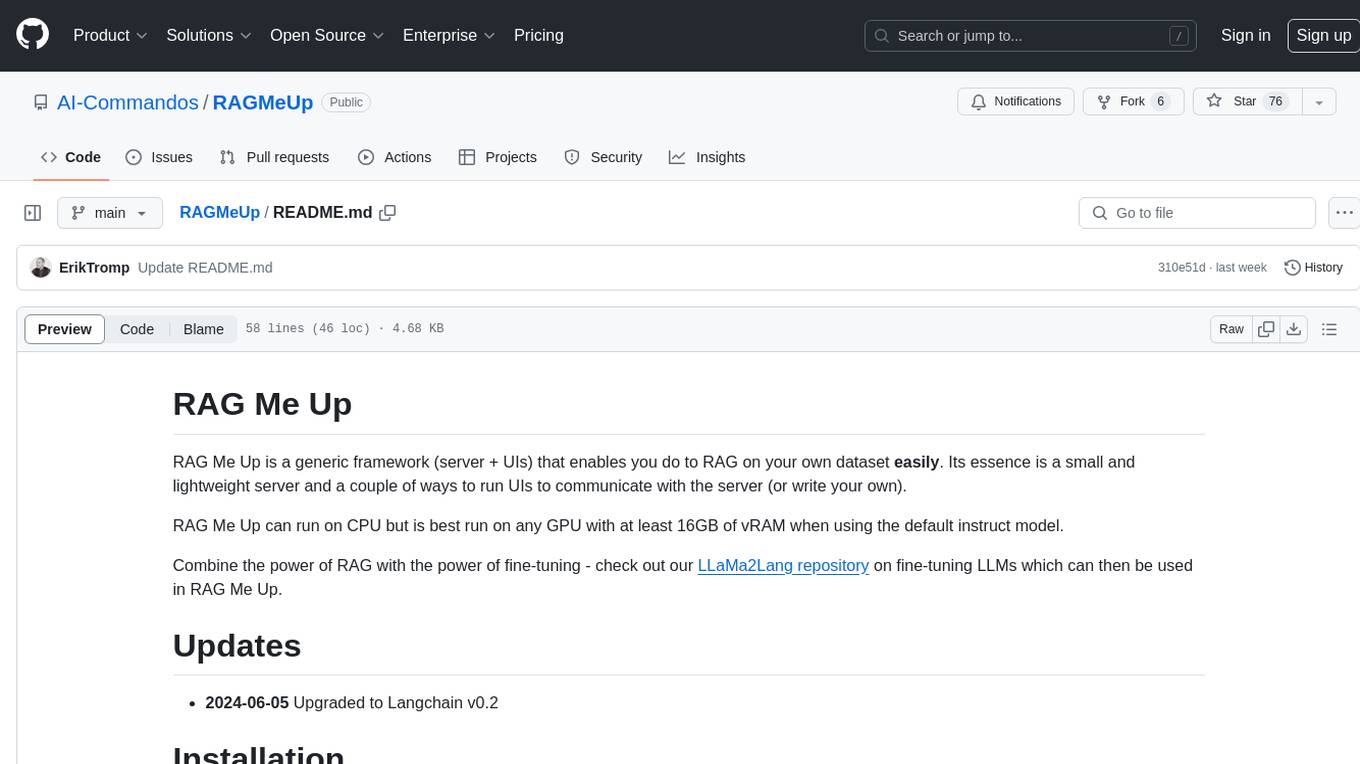
RAGMeUp
RAG Me Up is a generic framework that enables users to perform Retrieve and Generate (RAG) on their own dataset easily. It consists of a small server and UIs for communication. Best run on GPU with 16GB vRAM. Users can combine RAG with fine-tuning using LLaMa2Lang repository. The tool allows configuration for LLM, data, LLM parameters, prompt, and document splitting. Funding is sought to democratize AI and advance its applications.
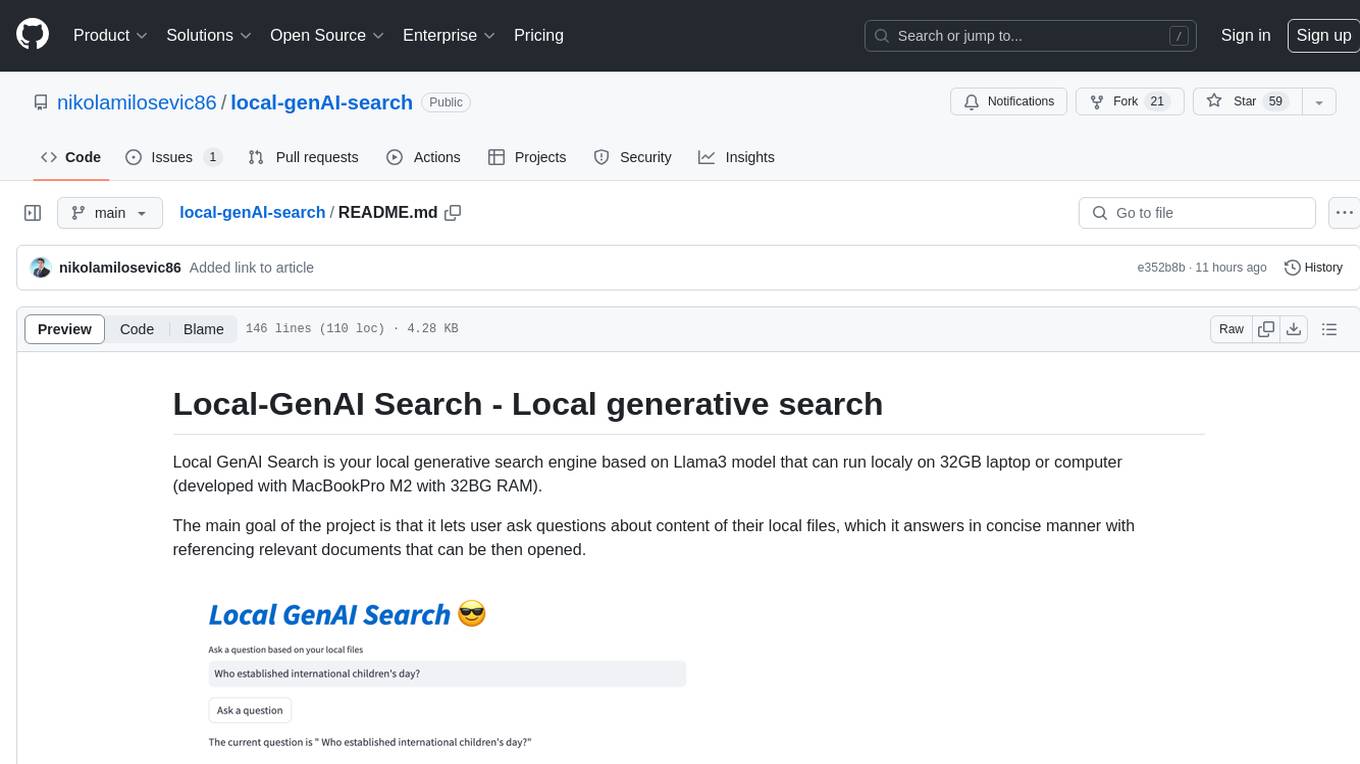
local-genAI-search
Local-GenAI Search is a local generative search engine powered by the Llama3 model, allowing users to ask questions about their local files and receive concise answers with relevant document references. It utilizes MS MARCO embeddings for semantic search and can run locally on a 32GB laptop or computer. The tool can be used to index local documents, search for information, and provide generative search services through a user interface.
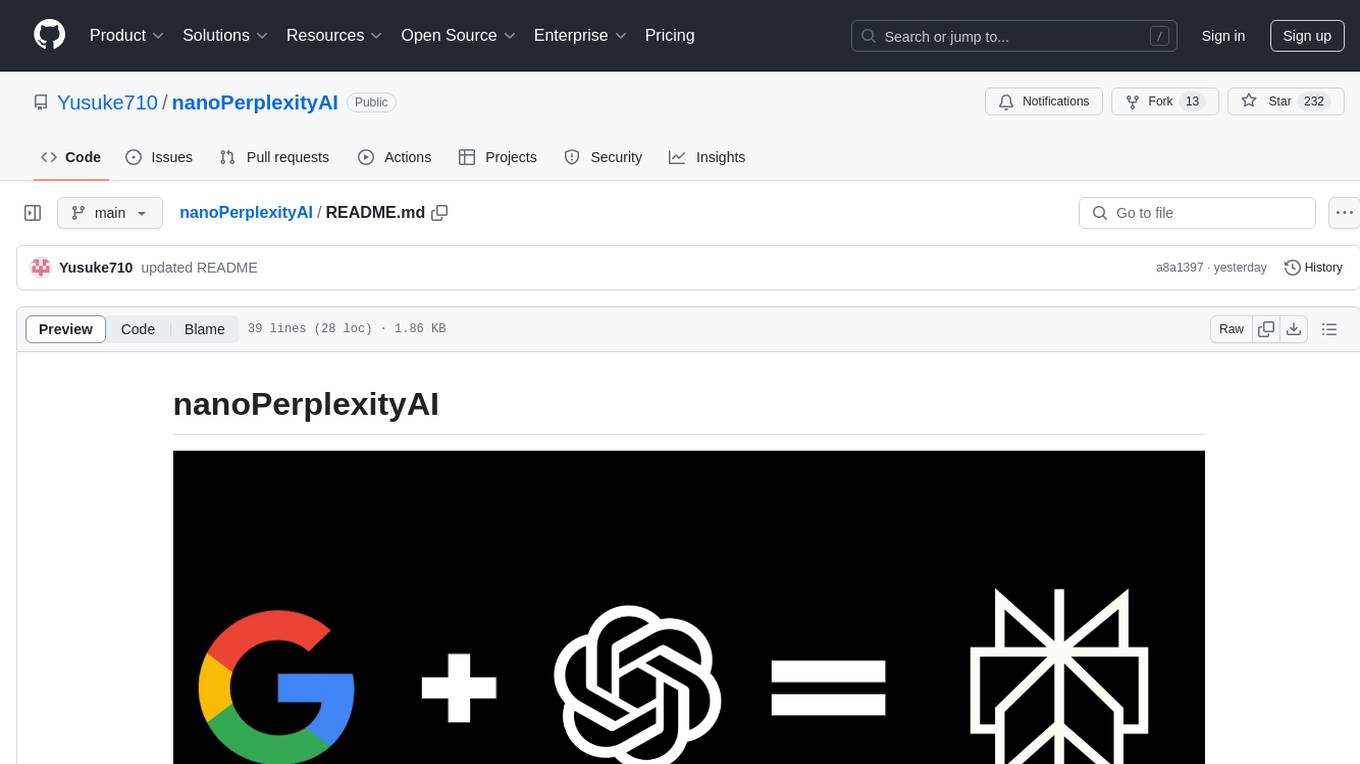
nanoPerplexityAI
nanoPerplexityAI is an open-source implementation of a large language model service that fetches information from Google. It involves a simple architecture where the user query is checked by the language model, reformulated for Google search, and an answer is generated and saved in a markdown file. The tool requires minimal setup and is designed for easy visualization of answers.
For similar jobs
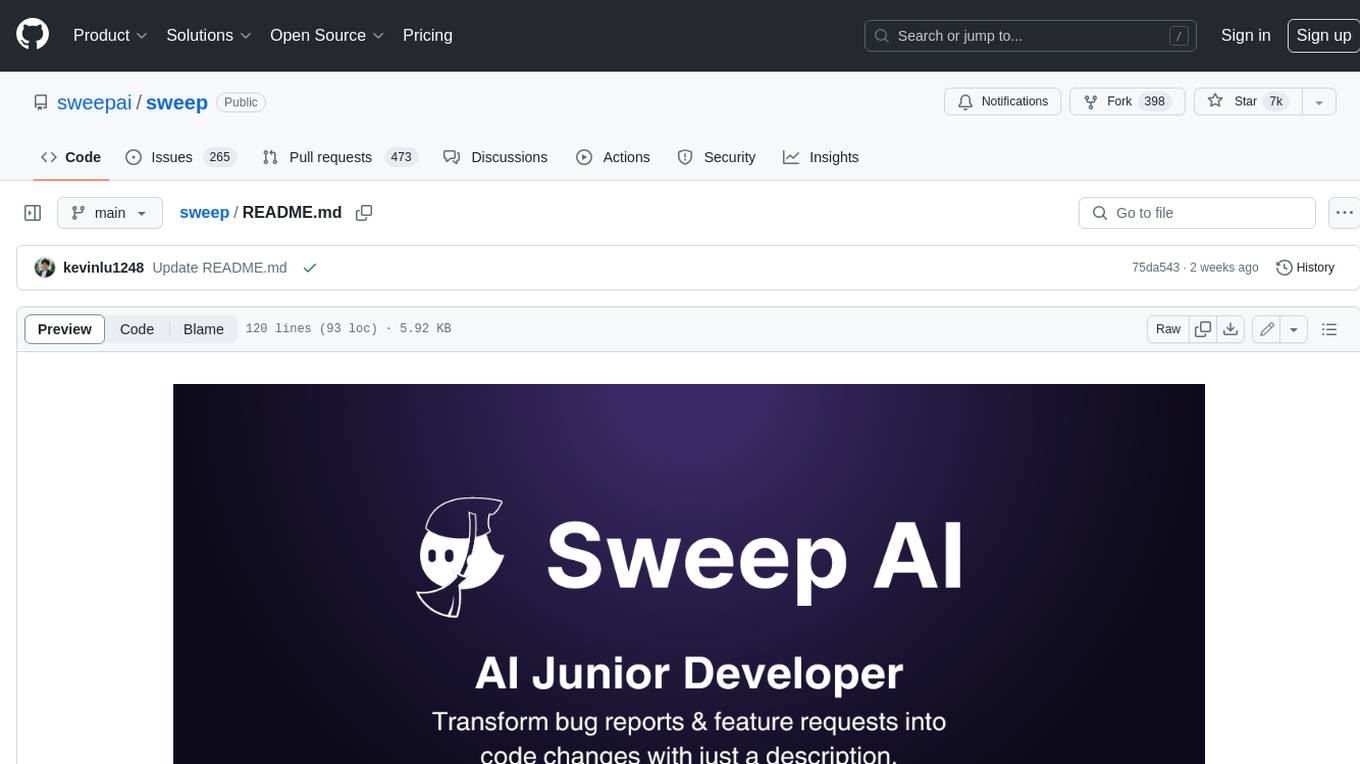
sweep
Sweep is an AI junior developer that turns bugs and feature requests into code changes. It automatically handles developer experience improvements like adding type hints and improving test coverage.
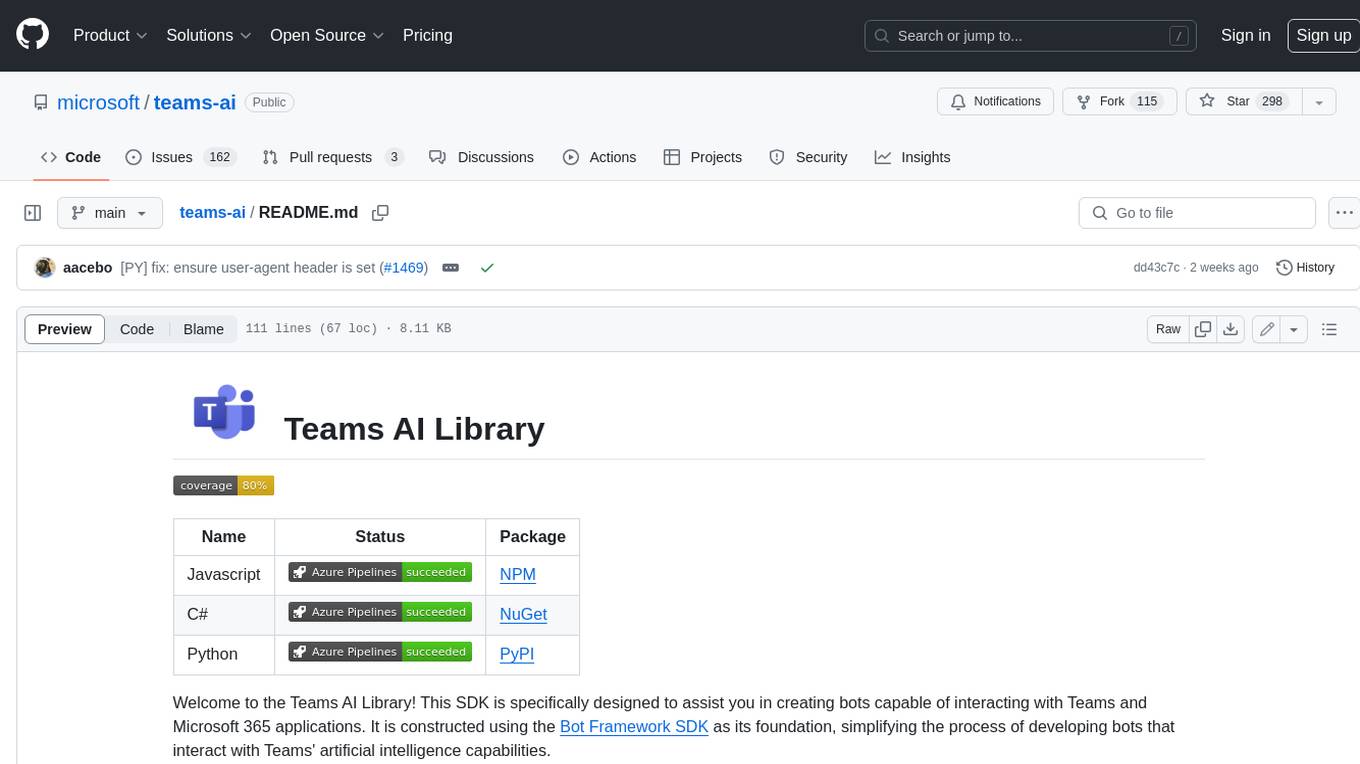
teams-ai
The Teams AI Library is a software development kit (SDK) that helps developers create bots that can interact with Teams and Microsoft 365 applications. It is built on top of the Bot Framework SDK and simplifies the process of developing bots that interact with Teams' artificial intelligence capabilities. The SDK is available for JavaScript/TypeScript, .NET, and Python.
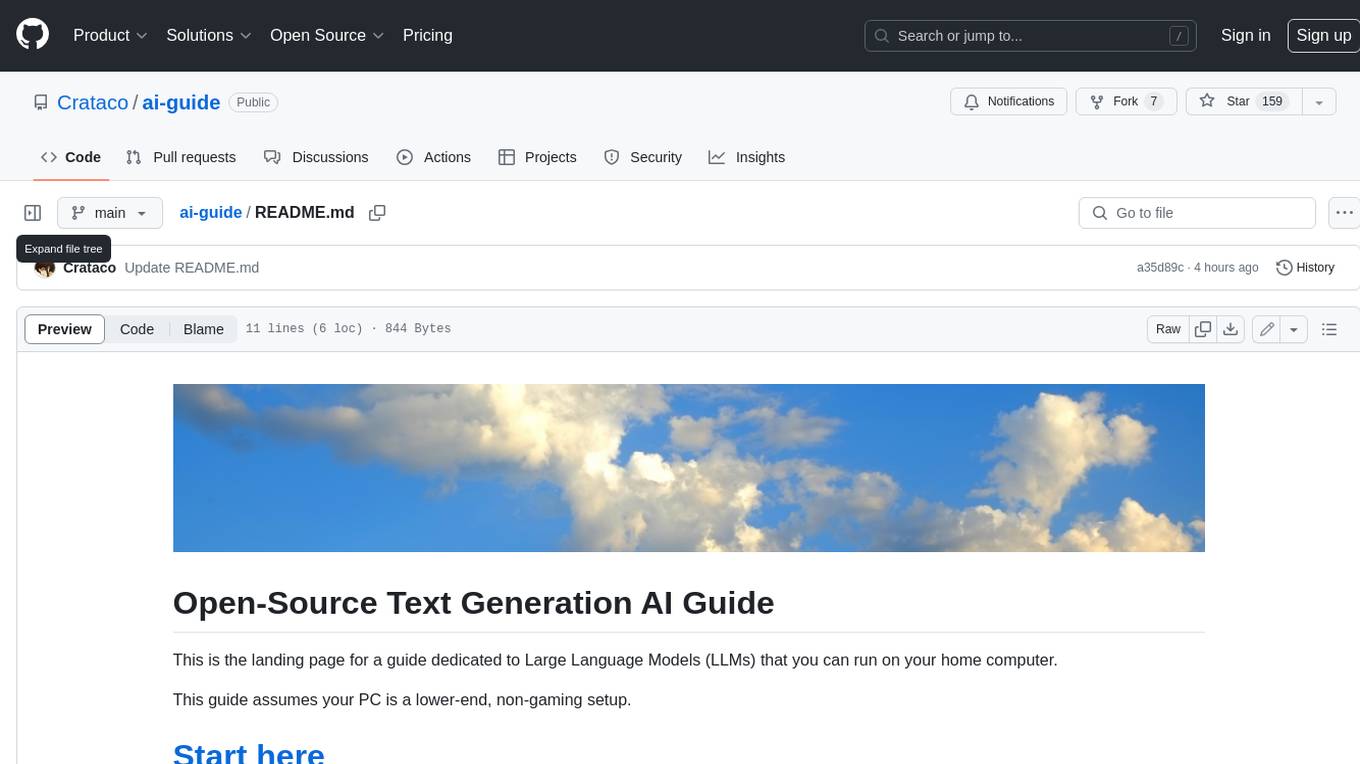
ai-guide
This guide is dedicated to Large Language Models (LLMs) that you can run on your home computer. It assumes your PC is a lower-end, non-gaming setup.
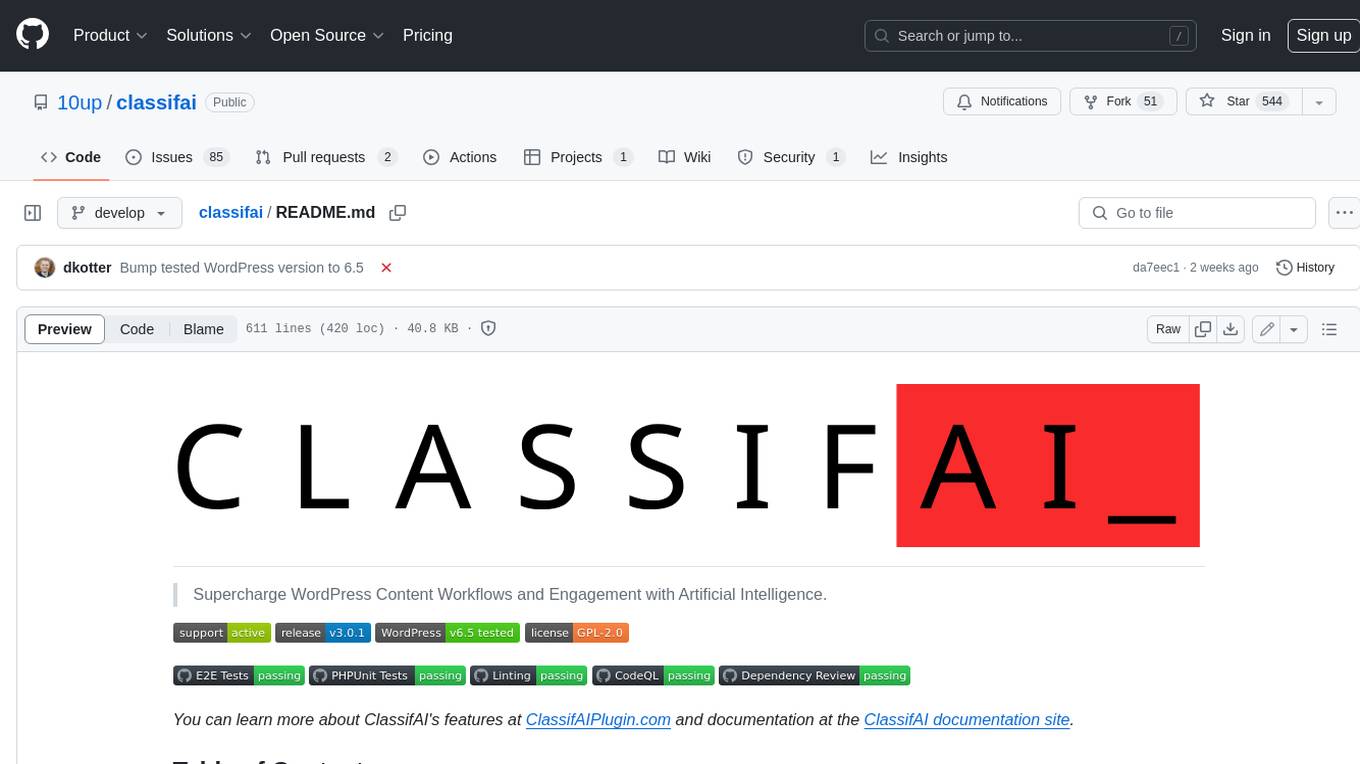
classifai
Supercharge WordPress Content Workflows and Engagement with Artificial Intelligence. Tap into leading cloud-based services like OpenAI, Microsoft Azure AI, Google Gemini and IBM Watson to augment your WordPress-powered websites. Publish content faster while improving SEO performance and increasing audience engagement. ClassifAI integrates Artificial Intelligence and Machine Learning technologies to lighten your workload and eliminate tedious tasks, giving you more time to create original content that matters.
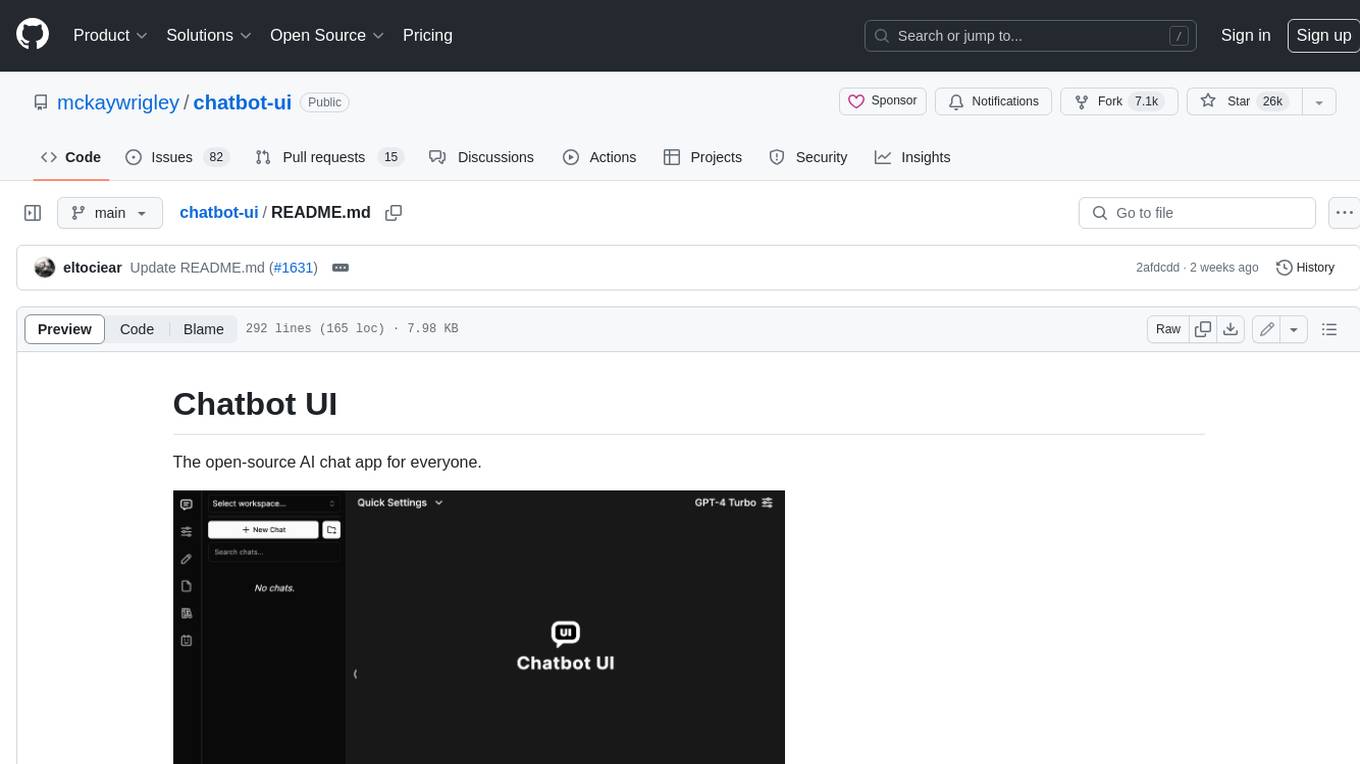
chatbot-ui
Chatbot UI is an open-source AI chat app that allows users to create and deploy their own AI chatbots. It is easy to use and can be customized to fit any need. Chatbot UI is perfect for businesses, developers, and anyone who wants to create a chatbot.
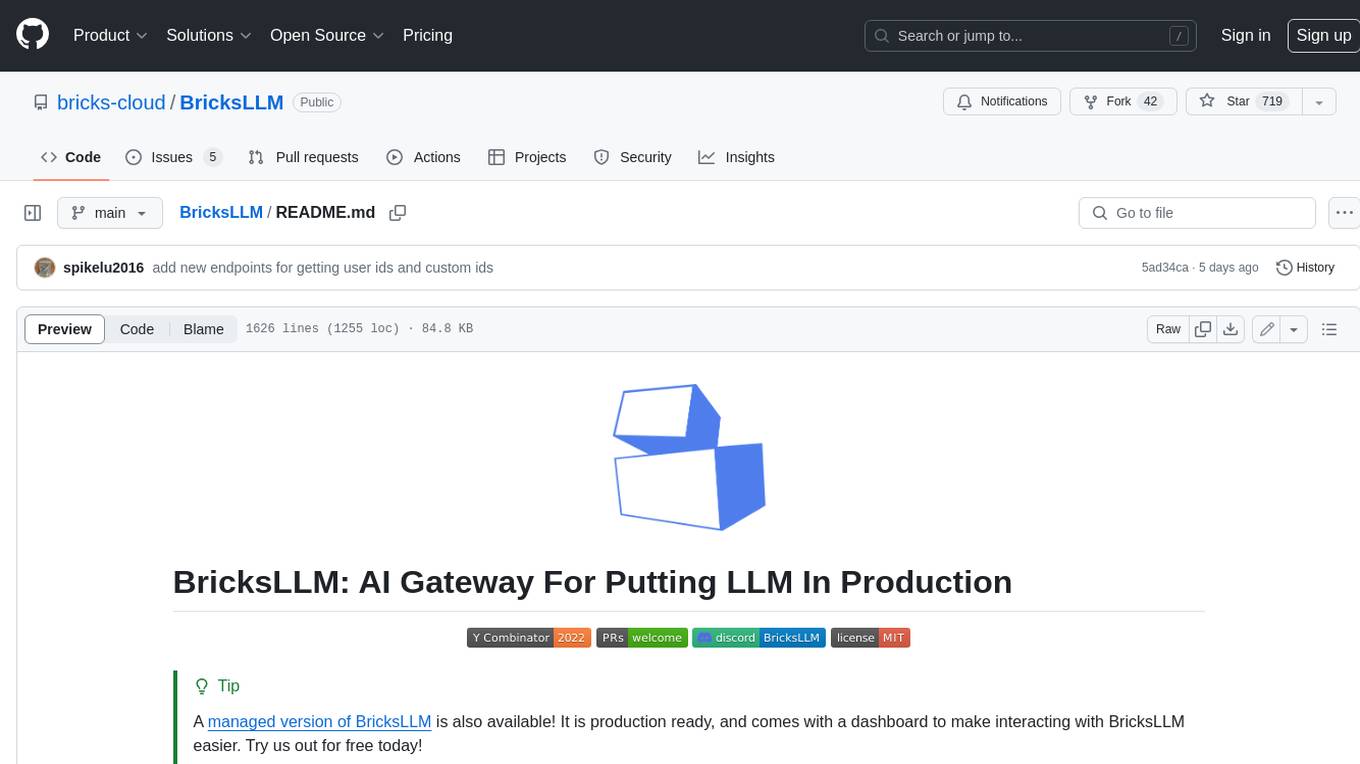
BricksLLM
BricksLLM is a cloud native AI gateway written in Go. Currently, it provides native support for OpenAI, Anthropic, Azure OpenAI and vLLM. BricksLLM aims to provide enterprise level infrastructure that can power any LLM production use cases. Here are some use cases for BricksLLM: * Set LLM usage limits for users on different pricing tiers * Track LLM usage on a per user and per organization basis * Block or redact requests containing PIIs * Improve LLM reliability with failovers, retries and caching * Distribute API keys with rate limits and cost limits for internal development/production use cases * Distribute API keys with rate limits and cost limits for students
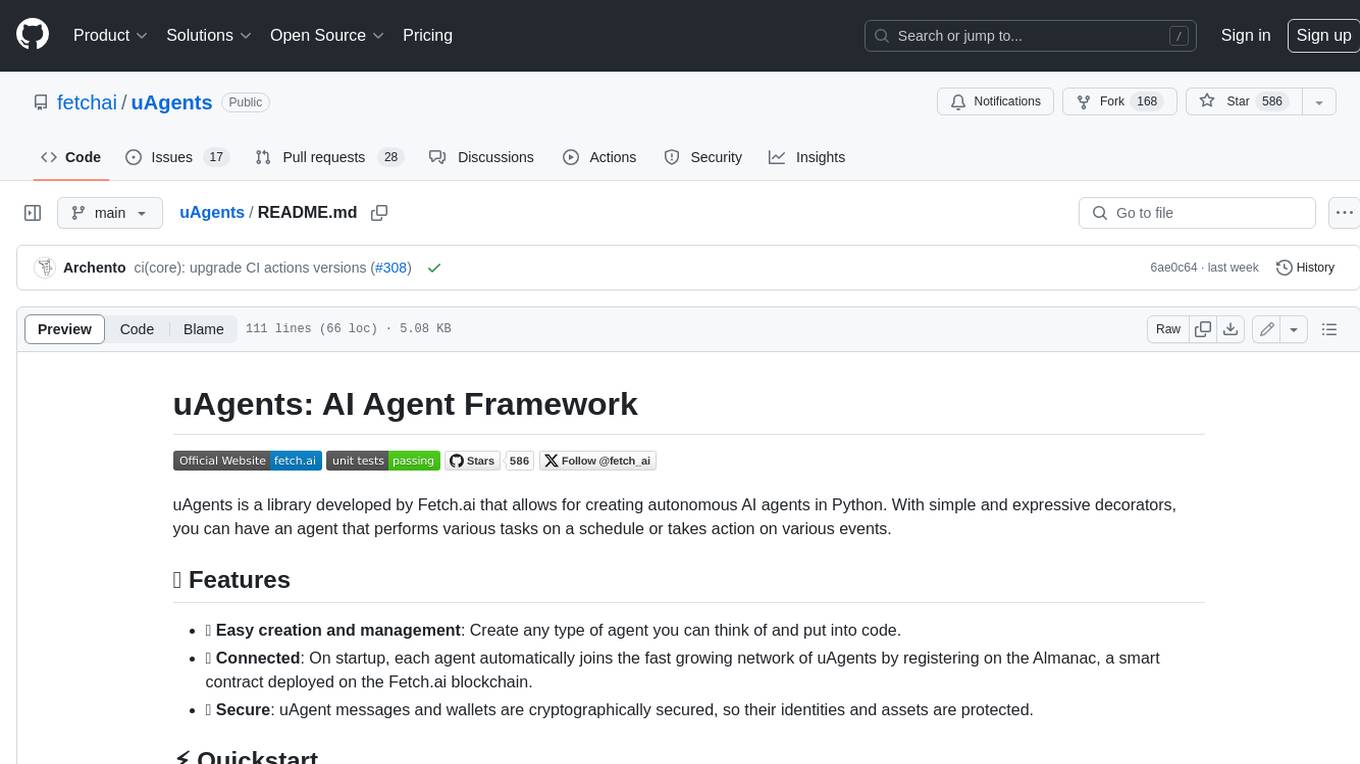
uAgents
uAgents is a Python library developed by Fetch.ai that allows for the creation of autonomous AI agents. These agents can perform various tasks on a schedule or take action on various events. uAgents are easy to create and manage, and they are connected to a fast-growing network of other uAgents. They are also secure, with cryptographically secured messages and wallets.
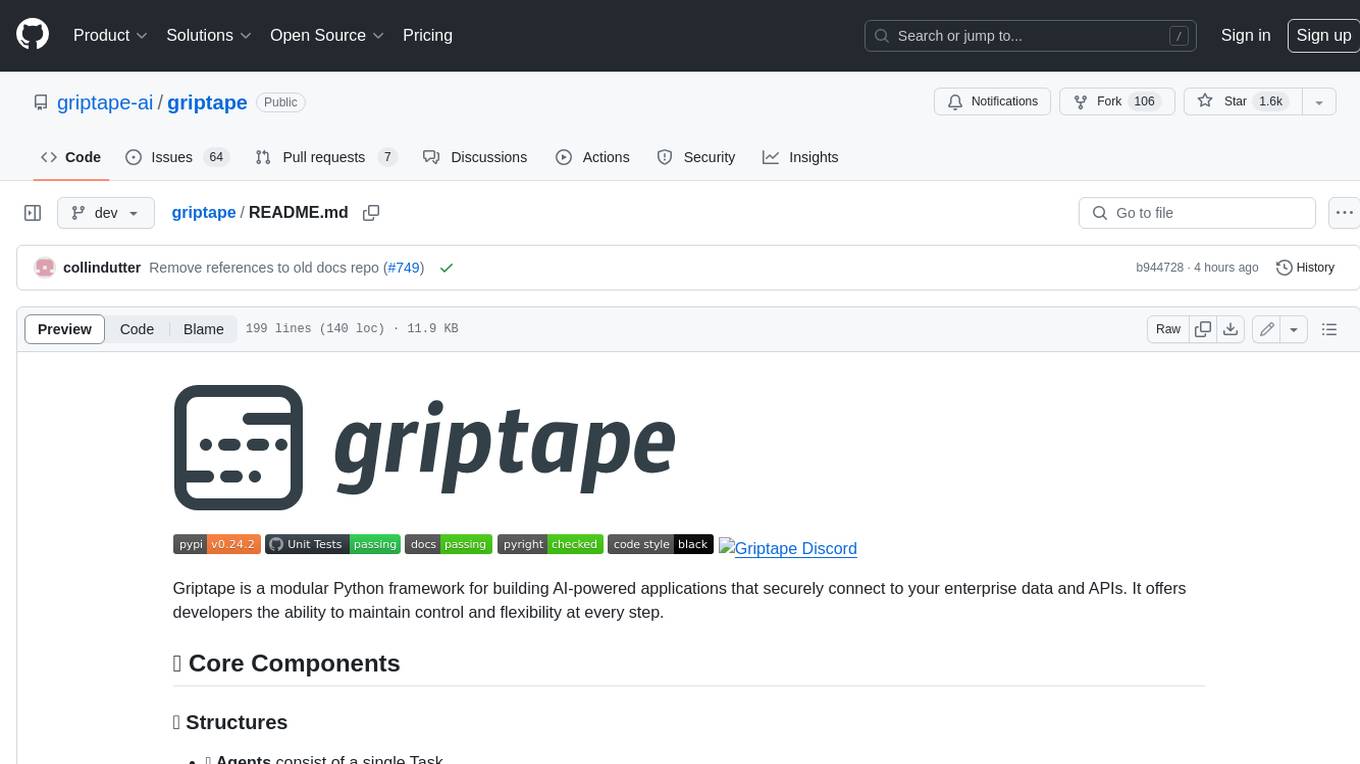
griptape
Griptape is a modular Python framework for building AI-powered applications that securely connect to your enterprise data and APIs. It offers developers the ability to maintain control and flexibility at every step. Griptape's core components include Structures (Agents, Pipelines, and Workflows), Tasks, Tools, Memory (Conversation Memory, Task Memory, and Meta Memory), Drivers (Prompt and Embedding Drivers, Vector Store Drivers, Image Generation Drivers, Image Query Drivers, SQL Drivers, Web Scraper Drivers, and Conversation Memory Drivers), Engines (Query Engines, Extraction Engines, Summary Engines, Image Generation Engines, and Image Query Engines), and additional components (Rulesets, Loaders, Artifacts, Chunkers, and Tokenizers). Griptape enables developers to create AI-powered applications with ease and efficiency.