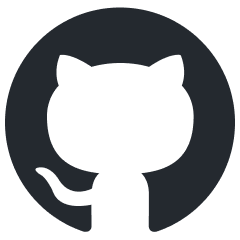
leettools
AI Search tools.
Stars: 283
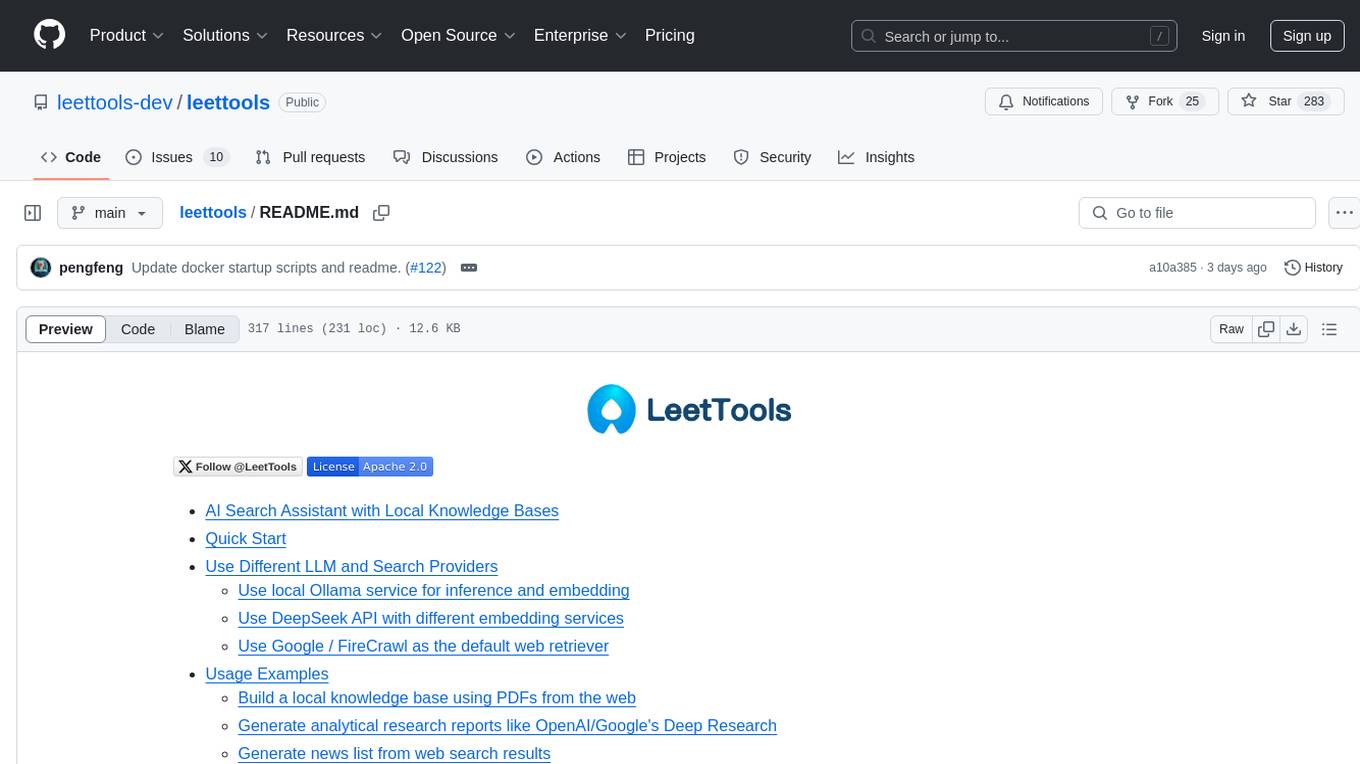
LeetTools is an AI search assistant that can perform highly customizable search workflows and generate customized format results based on both web and local knowledge bases. It provides an automated document pipeline for data ingestion, indexing, and storage, allowing users to focus on implementing workflows without worrying about infrastructure. LeetTools can run with minimal resource requirements on the command line with configurable LLM settings and supports different databases for various functions. Users can configure different functions in the same workflow to use different LLM providers and models.
README:
- AI Search Assistant with Local Knowledge Bases
- Quick Start
- Use Different LLM and Search Providers
- Usage Examples
- Main Components
- Community
LeetTools is an AI search assistant that can perform highly customizable search workflows and generate customized format results based on both web and local knowledge bases. With an automated document pipeline that handles data ingestion, indexing, and storage, we can focus on implementing the workflow without worrying about the underlying infrastructure.
LeetTools can run with minimal resource requirements on the command line with a DuckDB-backend and configurable LLM settings. It can also use other dedicated databases for different functions, e.g., we can use MongoDB for document storage, Milvus for vector search, and Neo4j for graph search. We can configure different functions in the same workflow to use different LLM providers and models.
Here is an illustration of the LeetTools digest flow where it can search the web (or local KB) and generate a digest article from the search results:
And here is an example output article generated by the digest flow for the query How does Ollama work?.
Currently LeetTools provides the following workflows:
- answer : Answer the query directly with source references (similar to Perplexity). ๐
- digest : Generate a multi-section digest article from search results (similar to Google Deep Research). ๐
- search : Search for top segements that match the query. ๐
- news : Generate a list of news items for the specified topic. ๐
- extract : Extract and store structured data for given schema. ๐
- opinions: Generate sentiment analysis and facts from the search results. ๐
Before you start
-
.env file: We can use any OpenAI-compatible LLM endpoint, such as local Ollama service or public provider such as Gemini or DeepSeek. we can switch the service easily by defining environment variables or switching .env files.
-
LeetHome: By default the data is saved under ${HOME}/leettools, you can set a different LeetHome environment variable to change the location:
% export LEET_HOME=<your_leet_home>
% mkdir -p ${LEET_HOME}
๐ New: Run LeetTools Web UI with Docker ๐
LeetTools now provides a Docker container that includes the web UI. You can start the container by running the following command:
docker/start.sh
This will start the LeetTools service and the web UI. You can access the web UI at http://localhost:3000. The web UI app is currently under development and not open sourced yet. We plan to open source it in the near future.
Run with pip
If you are using an OpenAI compatible LLM endpoint, you can install and run LeetTools with pip as follows (using Conda/Venv is recommended):
% conda create -y -n leettools python=3.11
% conda activate leettools
% pip install leettools
% export EDS_LLM_API_KEY=<your_api_key>
% leet flow -t answer -q "How does GraphRAG work?" -k graphrag -l info
The above flow -t answer
command will run the answer
flow with the query "How does
GraphRAG work?" and save the scraped web pages to the knowledge base graphrag
. The
-l info
option will show the essential log messages.
The default API endpoint is set to the OpenAI API endpoint, which you can modify by
changing the EDS_DEFAULT_LLM_BASE_URL
environment variable:
% export EDS_DEFAULT_LLM_BASE_URL=https://api.openai.com/v1
Run with source code
% git clone https://github.com/leettools-dev/leettools.git
% cd leettools
% conda create -y -n leettools python=3.11
% conda activate leettools
% pip install -r requirements.txt
% pip install -e .
# add the script path to the path
% export PATH=`pwd`/scripts:${PATH}
% export EDS_LLM_API_KEY=<your_api_key>
% leet flow -t answer -q "How does GraphRAG work?" -k graphrag -l info
We can run LeetTools with different env files to use different LLM providers and other related settings.
# you may need to pull the models first
% ollama pull llama3.2
% ollama pull nomic-embed-text
% ollama serve
% cat > .env.ollama <<EOF
EDS_DEFAULT_LLM_BASE_URL=http://localhost:11434/v1
EDS_LLM_API_KEY=dummy-llm-api-key
EDS_DEFAULT_INFERENCE_MODEL=llama3.2
EDS_DEFAULT_EMBEDDING_MODEL=nomic-embed-text
EDS_EMBEDDING_MODEL_DIMENSION=768
EOF
# Then run the command with the -e option to specify the .env file to use
% leet flow -e .env.ollama -t answer -q "How does GraphRAG work?" -k graphrag.ollama -l info
For another example, since DeepSeek does not provide an embedding endpoint yet, we can
use the "EDS_DEFAULT_DENSE_EMBEDDER" setting to specify a local embedder with a default
all-MiniLM-L6-v2
model:
### to you can put the settings in the .env.deepseek file
% cat > .env.deepseek <<EOF
LEET_HOME=</Users/myhome/leettools>
EDS_DEFAULT_LLM_BASE_URL=https://api.deepseek.com/v1
EDS_LLM_API_KEY=<your-api-key>
EDS_DEFAULT_INFERENCE_MODEL=deepseek-chat
EDS_DEFAULT_DENSE_EMBEDDER=dense_embedder_local_mem
EOF
# Then run the command with the -e option to specify the .env file to use
% leet flow -e .env.deepseek -t answer -q "How does GraphRAG work?" -k graphrag -l info
If you want to use another API provider (OpenAI compatible) for embedding, say a local Ollama embedder, you can set the embedding endpoint URL and API key separately as follows:
% cat > .env.deepseek <<EOF
EDS_DEFAULT_LLM_BASE_URL=https://api.deepseek.com/v1
EDS_LLM_API_KEY=<your-api-key>
EDS_DEFAULT_INFERENCE_MODEL=deepseek-chat
# this specifies to use an OpenAI compatible embedding endpoint
EDS_DEFAULT_DENSE_EMBEDDER=dense_embedder_openai
# the following specifies the embedding endpoint URL and model to use
EDS_DEFAULT_EMBEDDING_BASE_URL=http://localhost:11434/v1
EDS_DEFAULT_EMBEDDING_MODEL=nomic-embed-text
EDS_EMBEDDING_MODEL_DIMENSION=768
EOF
The search engine is google
by default, which can be set by the following environment
variable:
export EDS_WEB_RETRIEVER=google
export EDS_SEARCH_API_URL=https://www.googleapis.com/customsearch/v1
export EDS_GOOGLE_CX_KEY=<your-google-cx-key>
export EDS_GOOGLE_API_KEY=<your-google-api-key>
We can also use the FireCrawl search as the default web retriever instead of the default Google search by setting the following environment variables:
export EDS_WEB_RETRIEVER=firecrawl
export EDS_FIRECRAWL_API_URL=https://api.firecrawl.dev
export EDS_FIRECRAWL_API_KEY=your_firecrawl_api_key
Here is a detailed example of using FireCrawl with Ollama to run a deep research.
By default we provide a shared proxy search service that can be used for testing purposes. Users should use their own search services for production use.
We can build a local knowledge base with PDFs from the web. Suppose we have set up the local Ollama service as described above, now we can use the following commands to build a local knowledge base with PDFs from the web:
# create a KB with a URL
# the book downloaded here is "Foundations of Large Language Models"
# it has 231 pages and take some time to process
% leet kb add-url -e .env.ollama -k llmbook -r "https://arxiv.org/pdf/2501.09223"
# now you can query the KB with any topic you want to explore
% leet kb flow -e .env.ollama -t answer -k llmbook -l info \
-q "How does LLM Finetuning process work?"
We have a more detailed example to show how to use the local Ollama service with the DeepSeek-r1:1.5B model to build a local knowledge base.
We can generate analytical research reports like OpenAI/Google's Deep Research by using
the digest
flow. Here is an example:
% leet flow -e .env.fireworks -t digest -k aijob.fireworks \
-p search_max_results=30 -p days_limit=360 \
-q "How will agentic AI and generative AI affect our non-tech jobs?" \
-l info -o outputs/aijob.fireworks.md
An example of the output is available here, and the tutorial to use the DeepSeek API from fireworks.ai for the above command is available here.
We can create a knowledge base with a web search with a date limit, and then generate a list of news items from the KB. Here is an example:
leet flow -t news -q "LLM GenAI Startups" -k genai -l info\
-p days_limit=3 -p search_iteration=3 -p search_max_results=100 \
-o llm_genai_news.md
The query retrieves the latest web pages from the past 3 days up to 100 search result page
and generates a list of news items from the search results. The output is saved to
the llm_genai_news.md
file. An example of the output is available here.
The main components of the backend include:
- ๐ Automated document pipeline to ingest, convert, chunk, embed, and index documents.
- ๐๏ธ Knowledge base to manage and serve the indexed documents.
- ๐ Search and retrieval library to fetch documents from the web or local KB.
- ๐ค Workflow engine to implement search-based AI workflows.
- โ Configuration system to support dynamic configurations used for every component.
- ๐ Query history system to manage the history and the context of the queries.
- ๐ป Scheduler for automatic execution of the pipeline tasks.
- ๐งฉ Accounting system to track the usage of the LLM APIs.
The architecture of the document pipeline is shown below:
See the Documentation for more details.
Acknowledgements
Right now we are using the following open source libraries and tools (not limited to):
We plan to add more plugins for different components to support different workloads.
Get help and support
Please feel free to connect with us using the discussion section.
Contributing
Please read Contributing to LeetTools for details.
License
LeetTools is licensed under the Apache License, Version 2.0. See LICENSE for the full license text.
For Tasks:
Click tags to check more tools for each tasksFor Jobs:
Alternative AI tools for leettools
Similar Open Source Tools
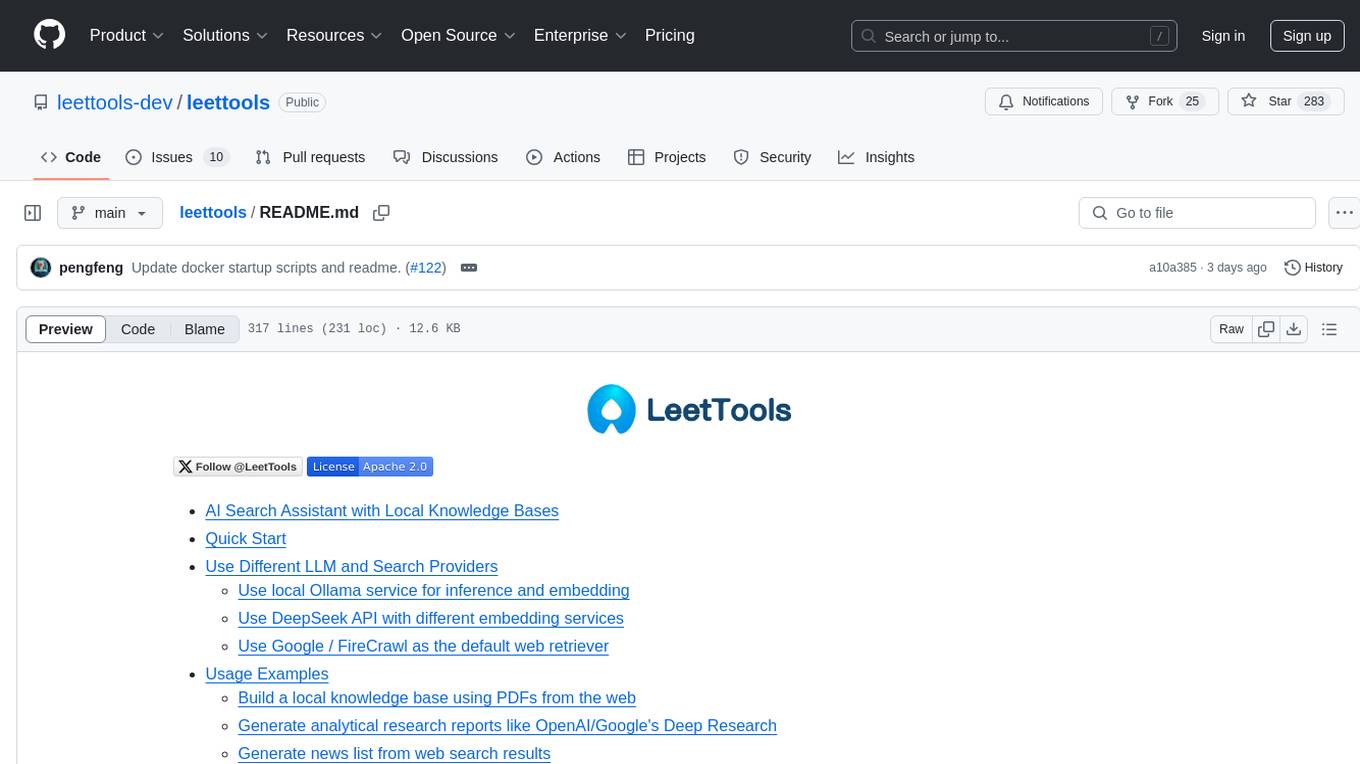
leettools
LeetTools is an AI search assistant that can perform highly customizable search workflows and generate customized format results based on both web and local knowledge bases. It provides an automated document pipeline for data ingestion, indexing, and storage, allowing users to focus on implementing workflows without worrying about infrastructure. LeetTools can run with minimal resource requirements on the command line with configurable LLM settings and supports different databases for various functions. Users can configure different functions in the same workflow to use different LLM providers and models.
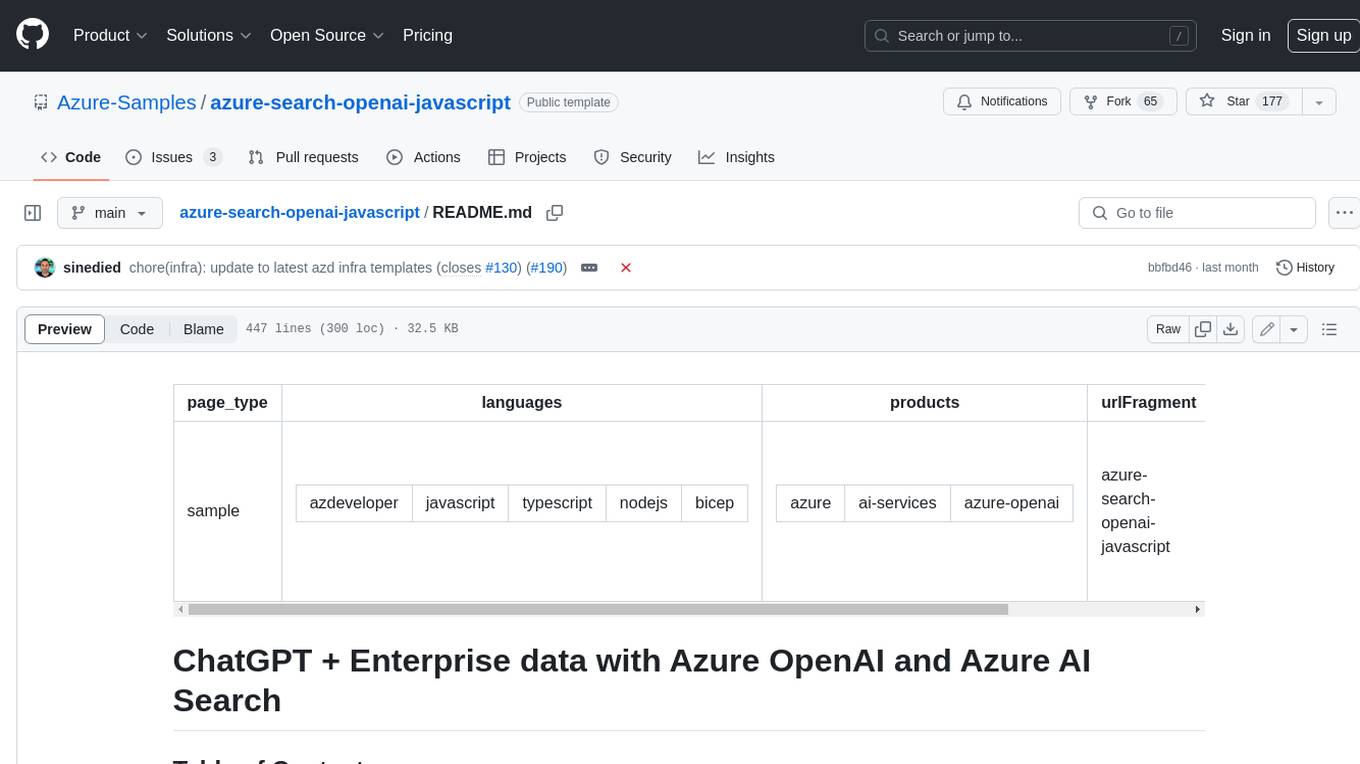
azure-search-openai-javascript
This sample demonstrates a few approaches for creating ChatGPT-like experiences over your own data using the Retrieval Augmented Generation pattern. It uses Azure OpenAI Service to access the ChatGPT model (gpt-35-turbo), and Azure AI Search for data indexing and retrieval.
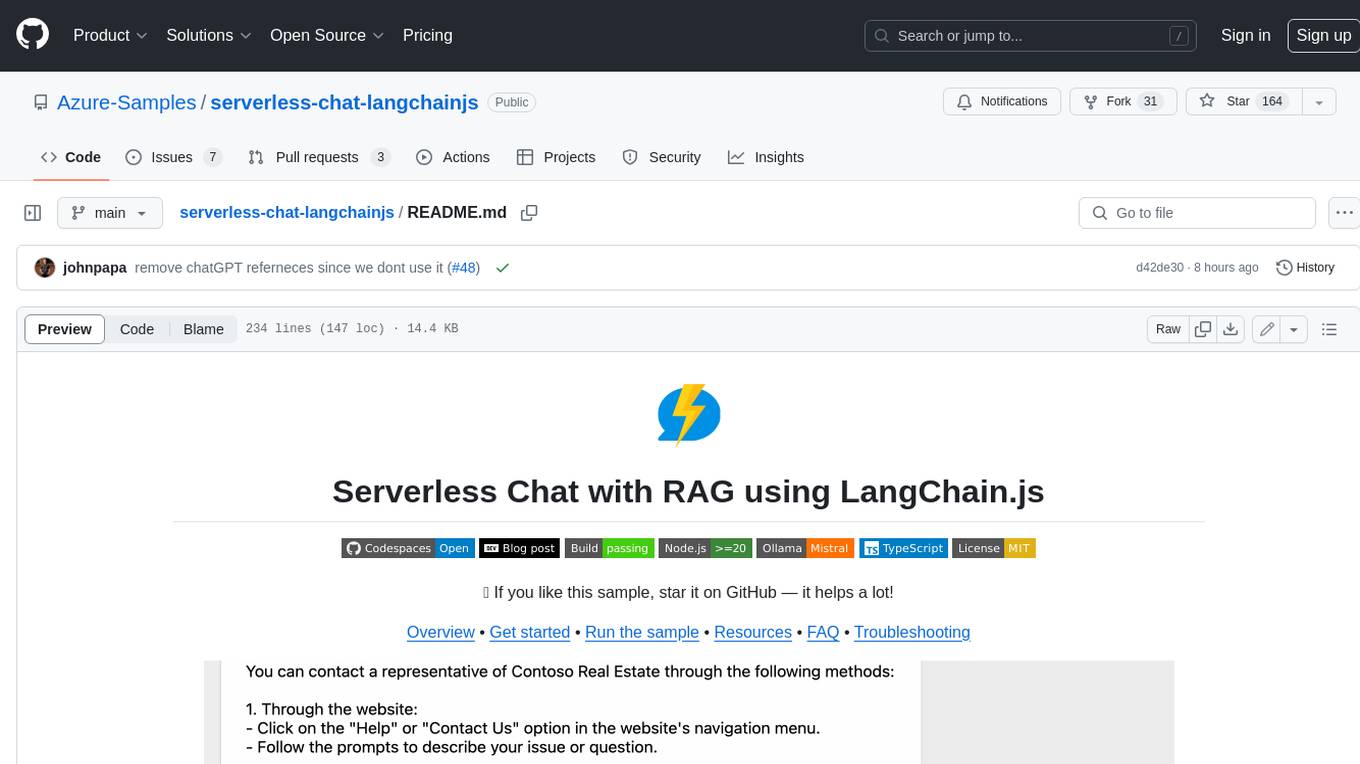
serverless-chat-langchainjs
This sample shows how to build a serverless chat experience with Retrieval-Augmented Generation using LangChain.js and Azure. The application is hosted on Azure Static Web Apps and Azure Functions, with Azure Cosmos DB for MongoDB vCore as the vector database. You can use it as a starting point for building more complex AI applications.
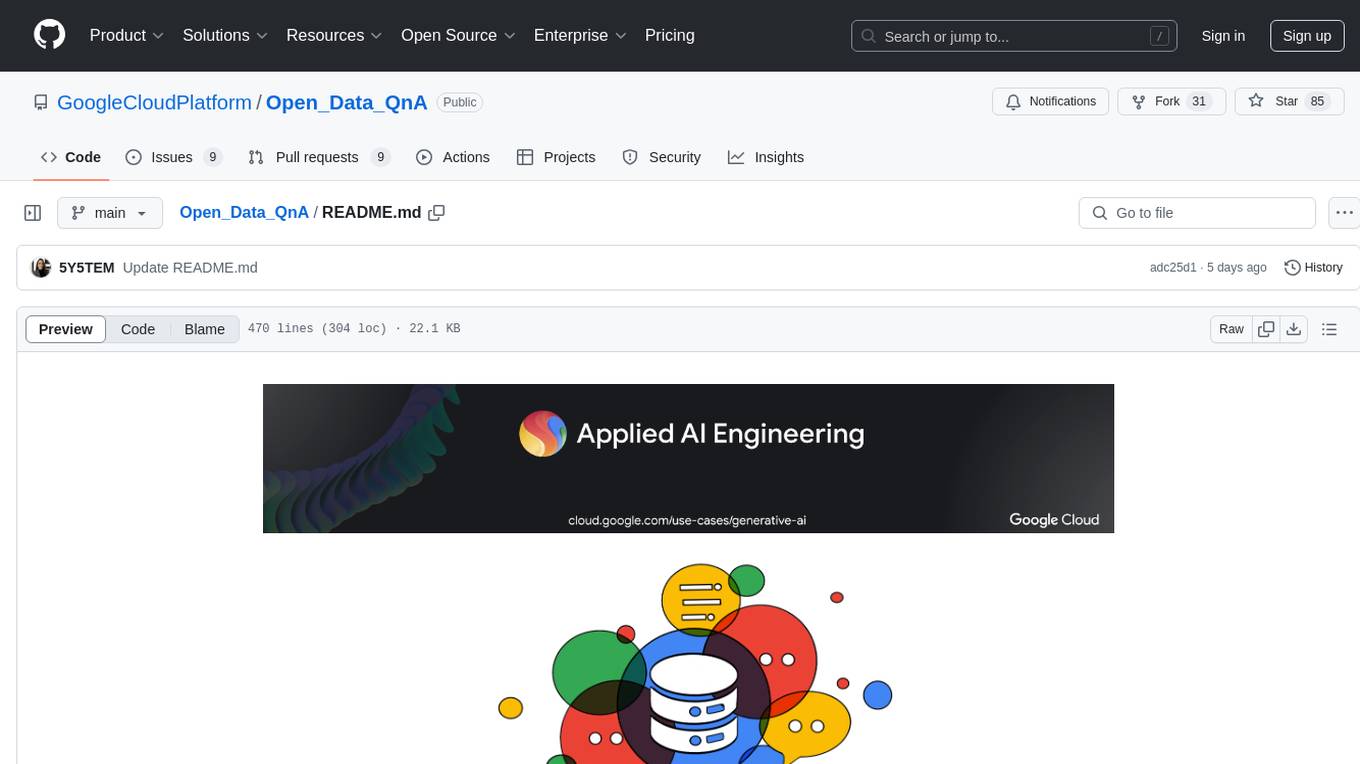
Open_Data_QnA
Open Data QnA is a Python library that allows users to interact with their PostgreSQL or BigQuery databases in a conversational manner, without needing to write SQL queries. The library leverages Large Language Models (LLMs) to bridge the gap between human language and database queries, enabling users to ask questions in natural language and receive informative responses. It offers features such as conversational querying with multiturn support, table grouping, multi schema/dataset support, SQL generation, query refinement, natural language responses, visualizations, and extensibility. The library is built on a modular design and supports various components like Database Connectors, Vector Stores, and Agents for SQL generation, validation, debugging, descriptions, embeddings, responses, and visualizations.
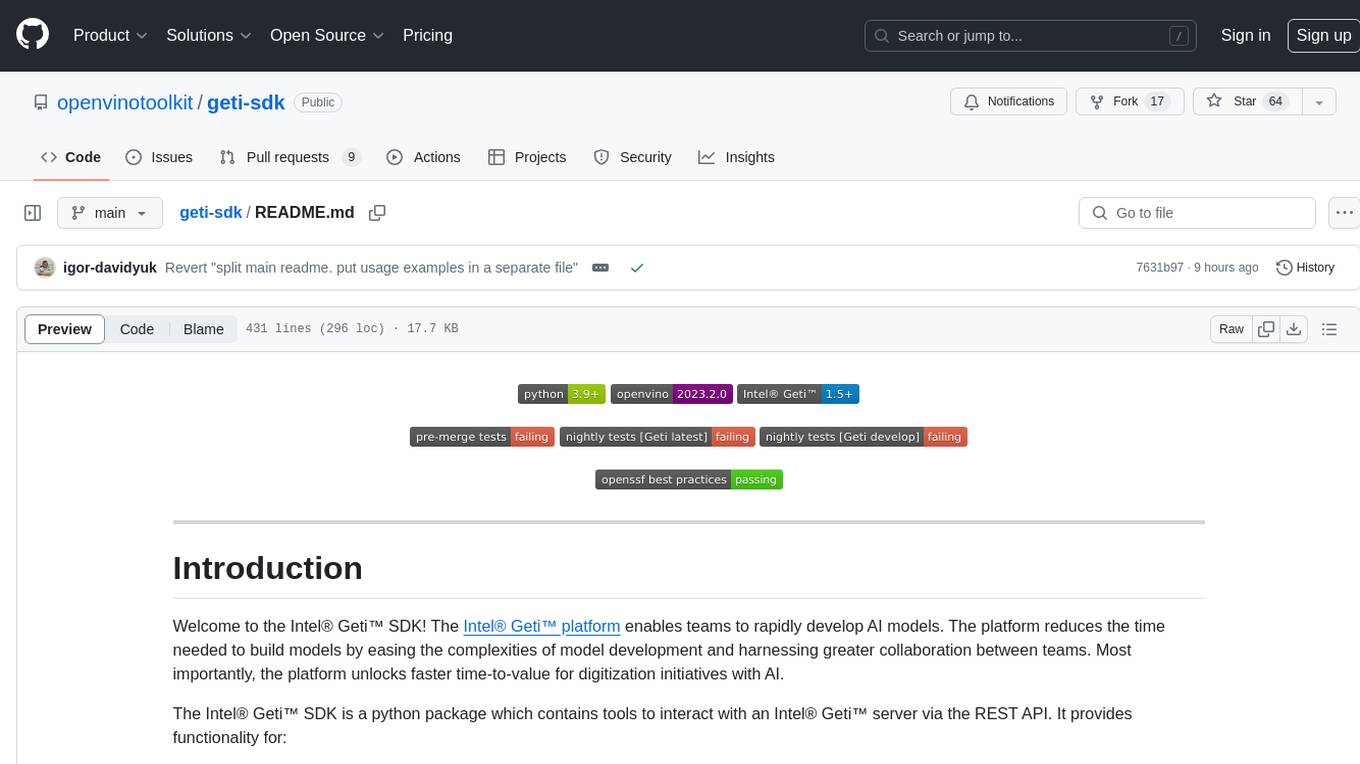
geti-sdk
The Intelยฎ Getiโข SDK is a python package that enables teams to rapidly develop AI models by easing the complexities of model development and enhancing collaboration between teams. It provides tools to interact with an Intelยฎ Getiโข server via the REST API, allowing for project creation, downloading, uploading, deploying for local inference with OpenVINO, setting project and model configuration, launching and monitoring training jobs, and media upload and prediction. The SDK also includes tutorial-style Jupyter notebooks demonstrating its usage.
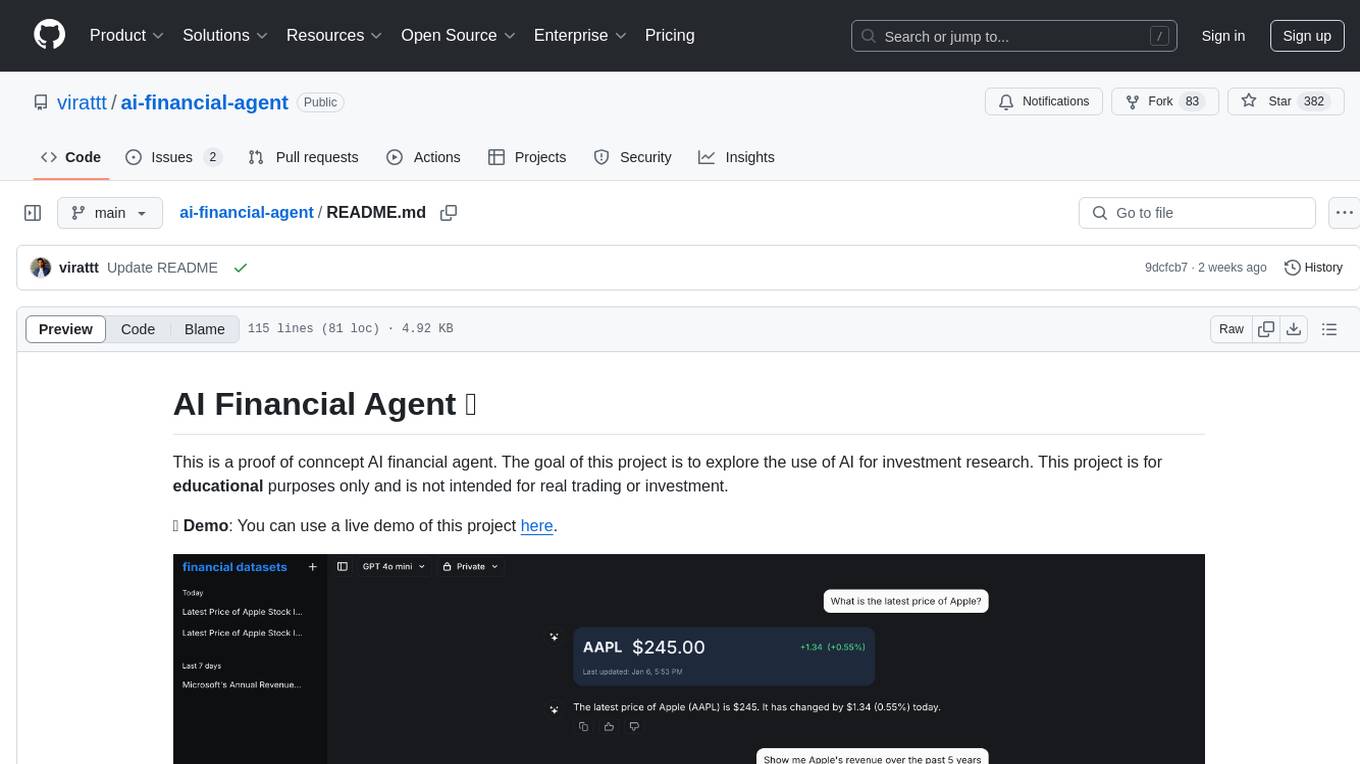
ai-financial-agent
AI Financial Agent is a proof of concept project exploring the use of AI for investment research. It provides an AI SDK with a unified API for generating text and structured objects, along with access to real-time and historical stock market data optimized for AI financial agents. The project includes features like dynamic chat interfaces, support for multiple model providers, and styling with Tailwind CSS. Users can deploy their own version of the AI Financial Agent using Vercel and GitHub integration.
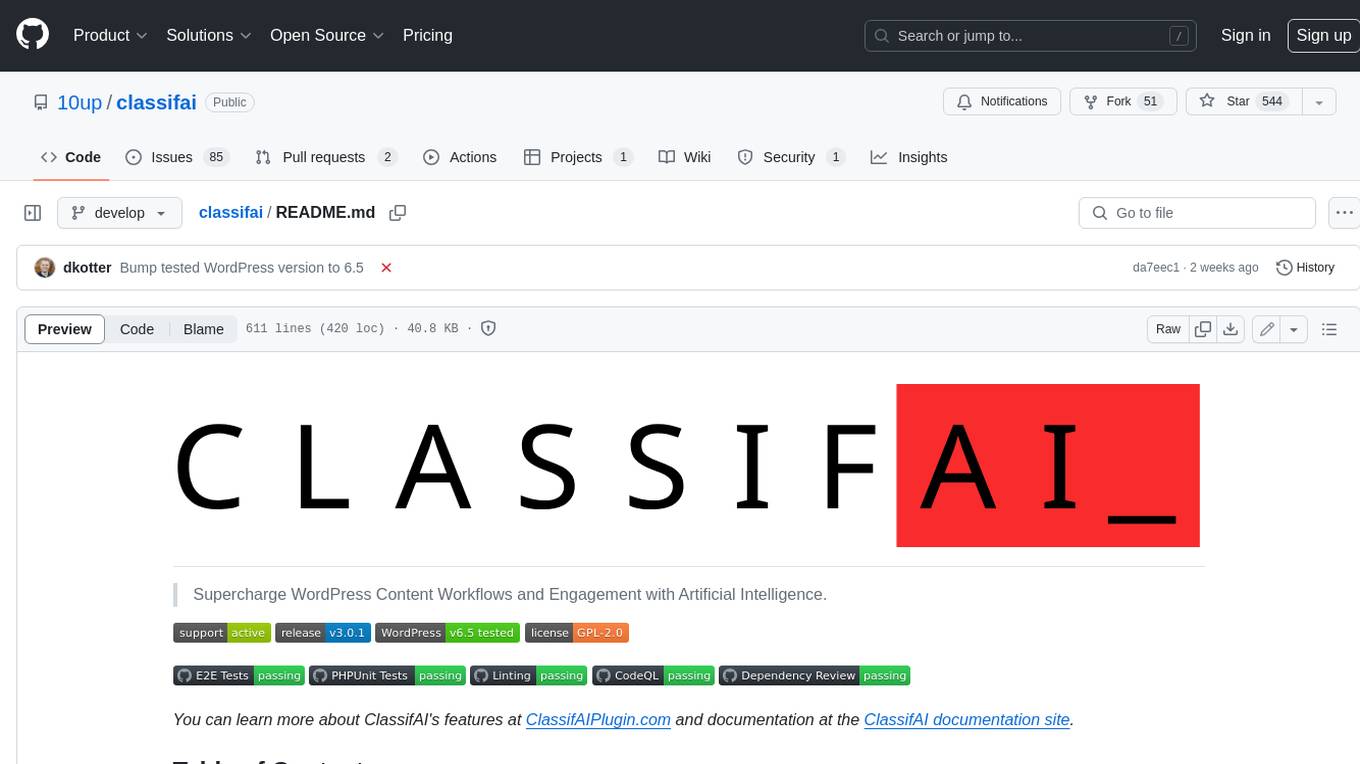
classifai
Supercharge WordPress Content Workflows and Engagement with Artificial Intelligence. Tap into leading cloud-based services like OpenAI, Microsoft Azure AI, Google Gemini and IBM Watson to augment your WordPress-powered websites. Publish content faster while improving SEO performance and increasing audience engagement. ClassifAI integrates Artificial Intelligence and Machine Learning technologies to lighten your workload and eliminate tedious tasks, giving you more time to create original content that matters.
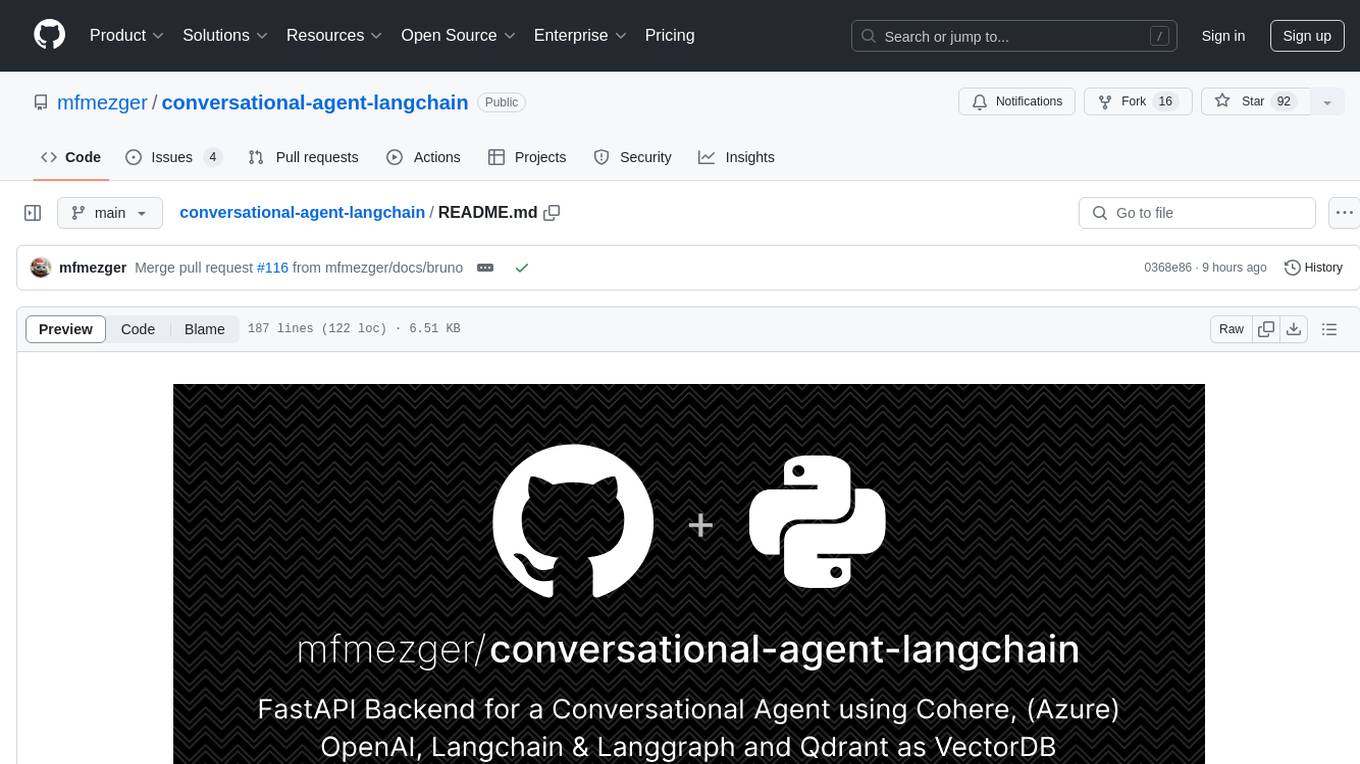
conversational-agent-langchain
This repository contains a Rest-Backend for a Conversational Agent that allows embedding documents, semantic search, QA based on documents, and document processing with Large Language Models. It uses Aleph Alpha and OpenAI Large Language Models to generate responses to user queries, includes a vector database, and provides a REST API built with FastAPI. The project also features semantic search, secret management for API keys, installation instructions, and development guidelines for both backend and frontend components.
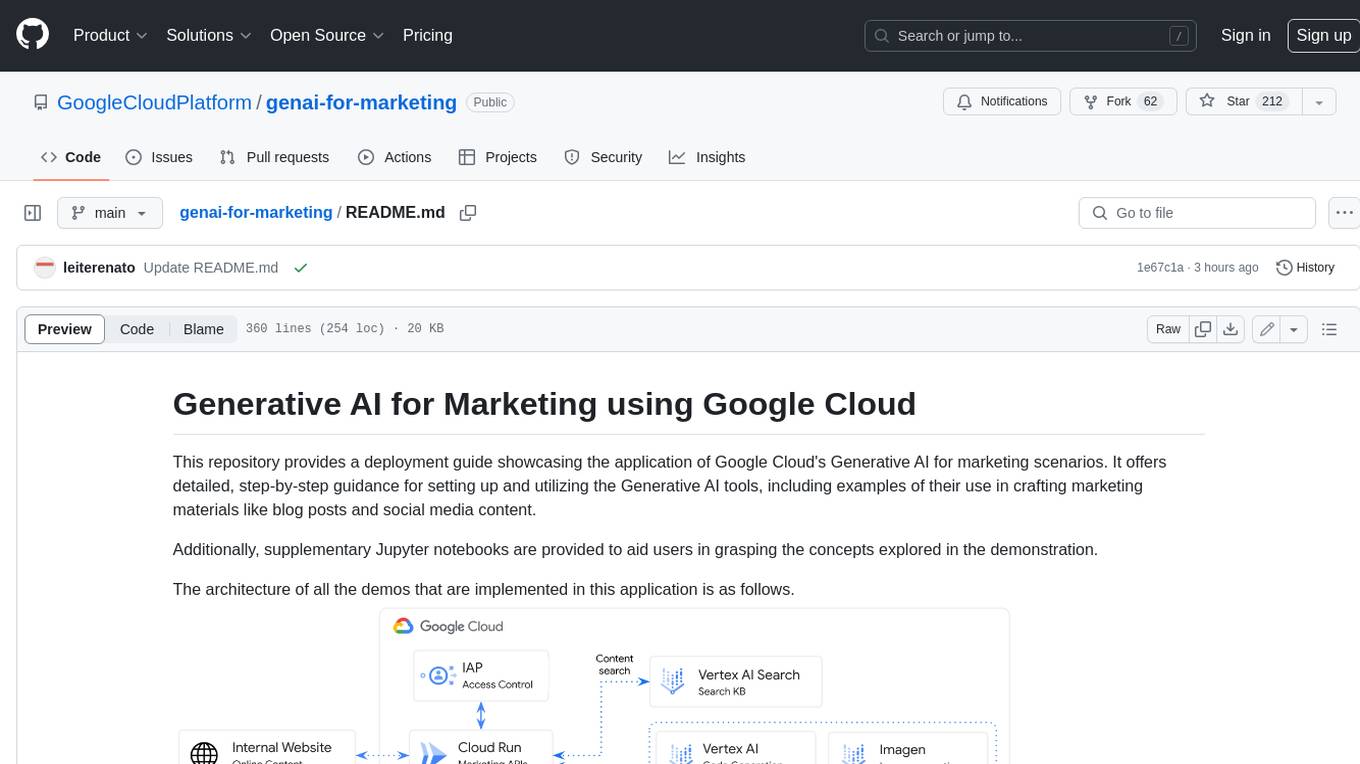
genai-for-marketing
This repository provides a deployment guide for utilizing Google Cloud's Generative AI tools in marketing scenarios. It includes step-by-step instructions, examples of crafting marketing materials, and supplementary Jupyter notebooks. The demos cover marketing insights, audience analysis, trendspotting, content search, content generation, and workspace integration. Users can access and visualize marketing data, analyze trends, improve search experience, and generate compelling content. The repository structure includes backend APIs, frontend code, sample notebooks, templates, and installation scripts.
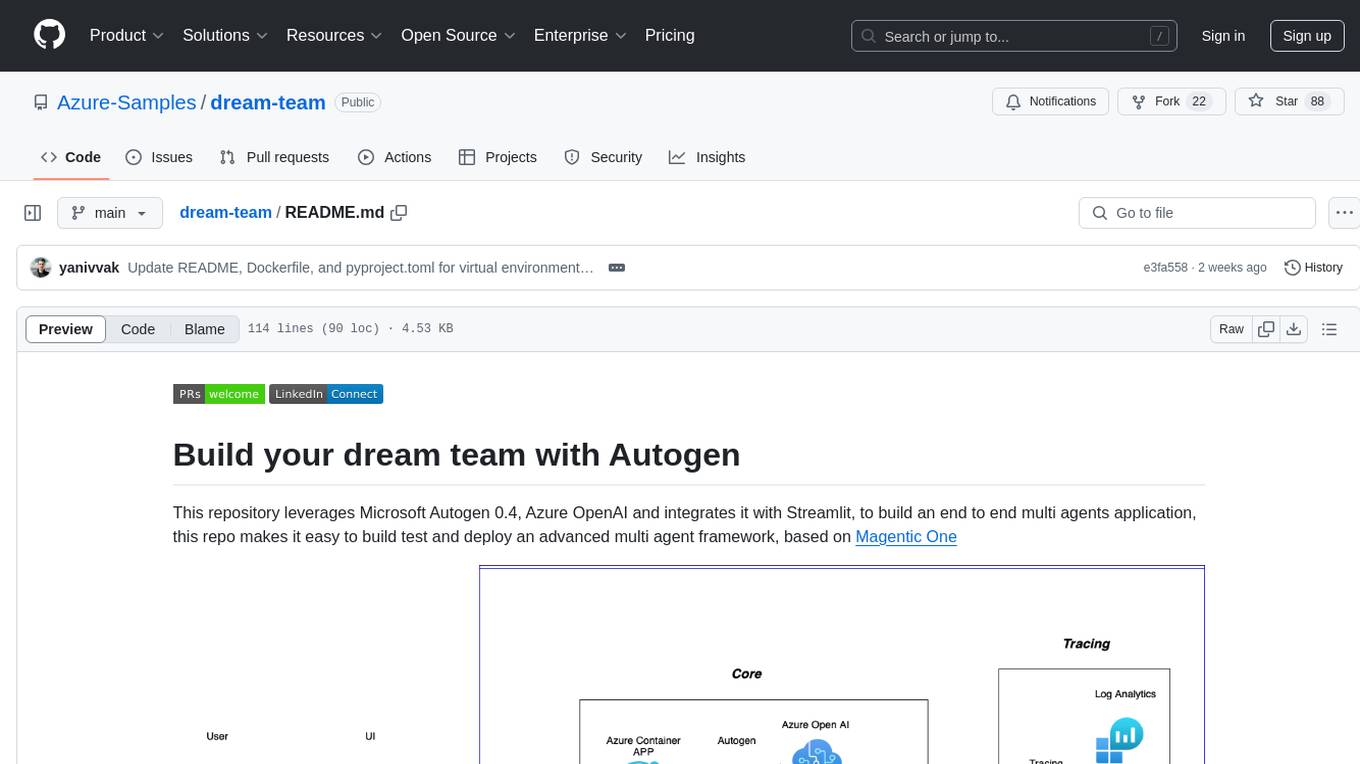
dream-team
Build your dream team with Autogen is a repository that leverages Microsoft Autogen 0.4, Azure OpenAI, and Streamlit to create an end-to-end multi-agent application. It provides an advanced multi-agent framework based on Magentic One, with features such as a friendly UI, single-line deployment, secure code execution, managed identities, and observability & debugging tools. Users can deploy Azure resources and the app with simple commands, work locally with virtual environments, install dependencies, update configurations, and run the application. The repository also offers resources for learning more about building applications with Autogen.
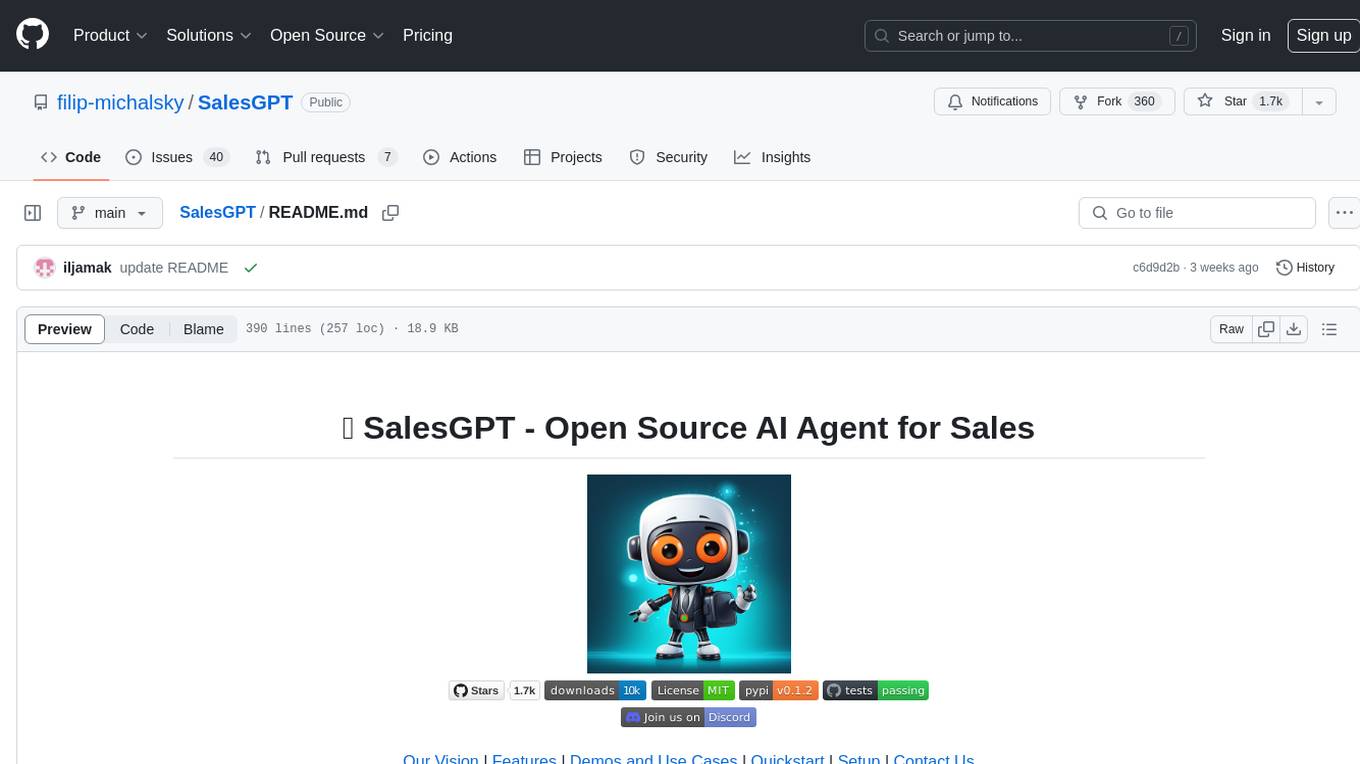
SalesGPT
SalesGPT is an open-source AI agent designed for sales, utilizing context-awareness and LLMs to work across various communication channels like voice, email, and texting. It aims to enhance sales conversations by understanding the stage of the conversation and providing tools like product knowledge base to reduce errors. The agent can autonomously generate payment links, handle objections, and close sales. It also offers features like automated email communication, meeting scheduling, and integration with various LLMs for customization. SalesGPT is optimized for low latency in voice channels and ensures human supervision where necessary. The tool provides enterprise-grade security and supports LangSmith tracing for monitoring and evaluation of intelligent agents built on LLM frameworks.
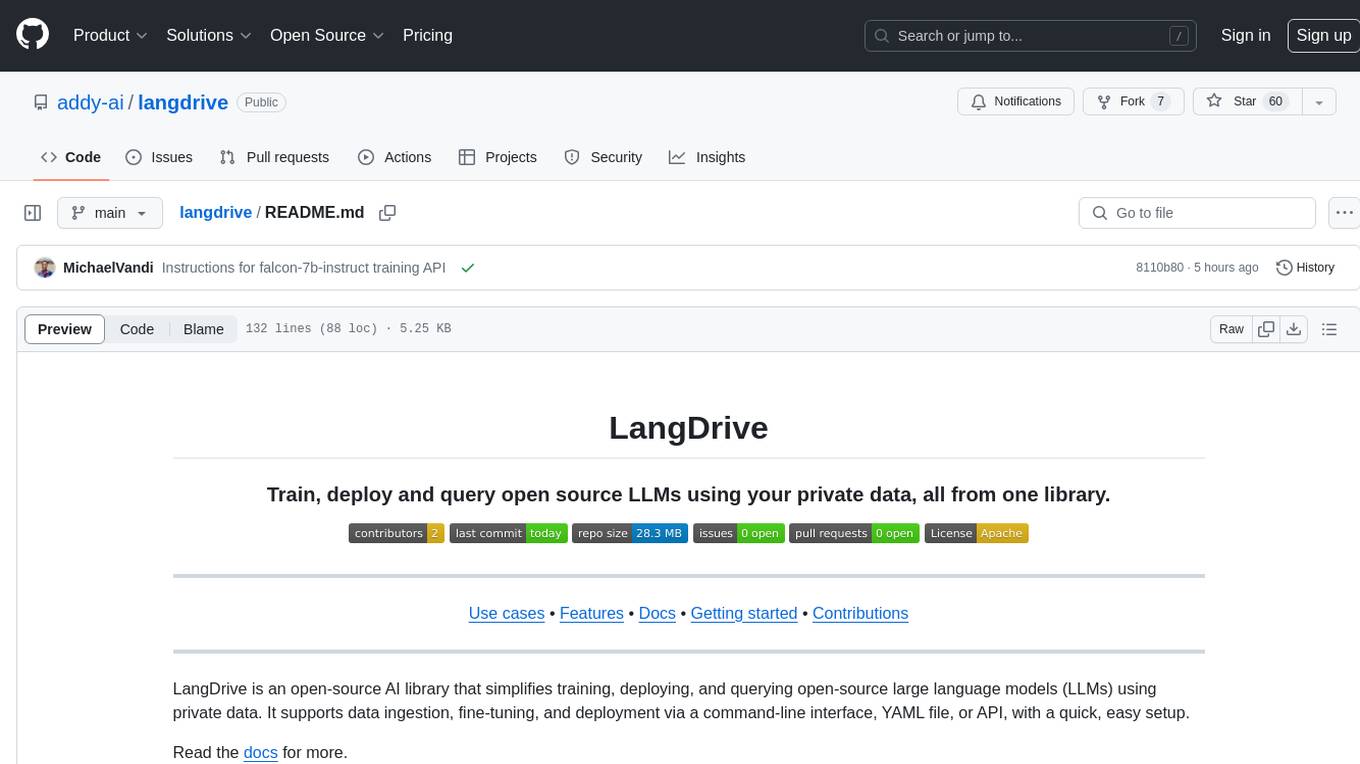
langdrive
LangDrive is an open-source AI library that simplifies training, deploying, and querying open-source large language models (LLMs) using private data. It supports data ingestion, fine-tuning, and deployment via a command-line interface, YAML file, or API, with a quick, easy setup. Users can build AI applications such as question/answering systems, chatbots, AI agents, and content generators. The library provides features like data connectors for ingestion, fine-tuning of LLMs, deployment to Hugging Face hub, inference querying, data utilities for CRUD operations, and APIs for model access. LangDrive is designed to streamline the process of working with LLMs and making AI development more accessible.
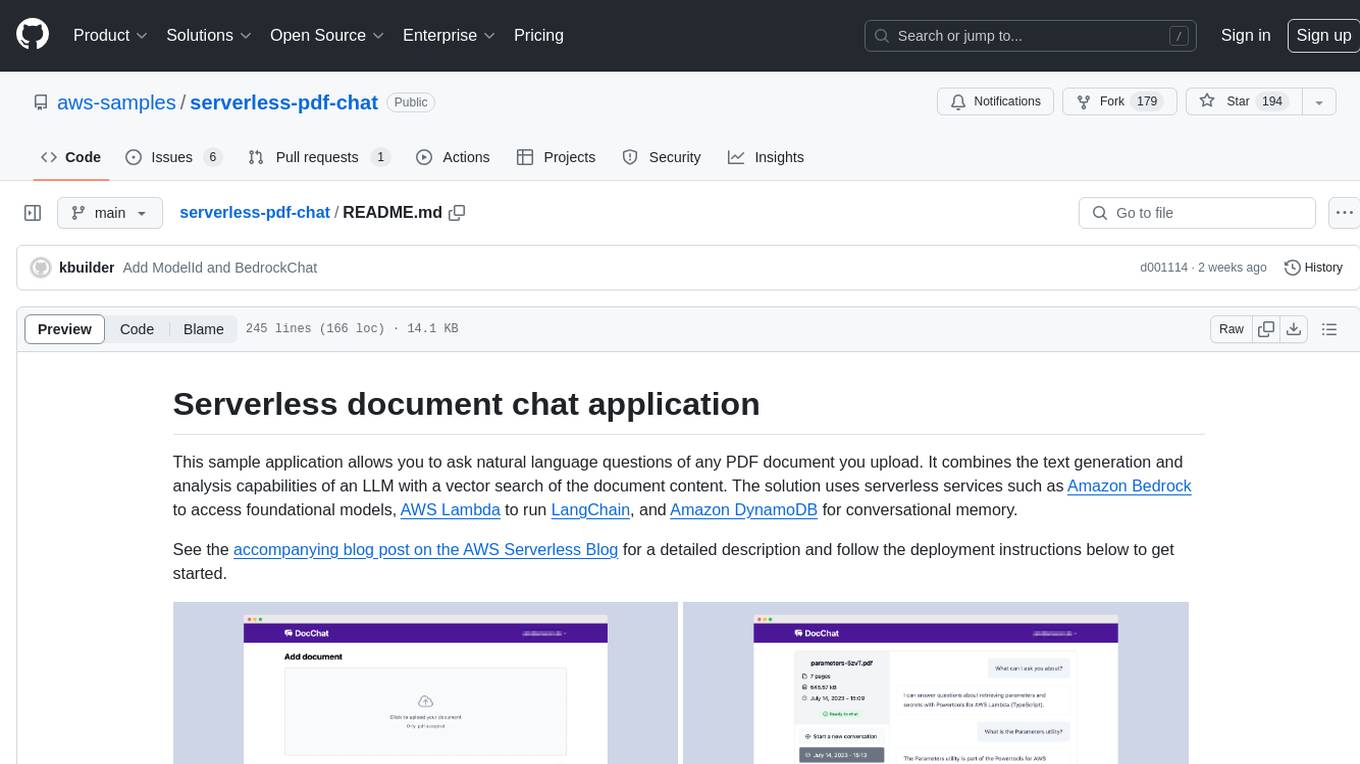
serverless-pdf-chat
The serverless-pdf-chat repository contains a sample application that allows users to ask natural language questions of any PDF document they upload. It leverages serverless services like Amazon Bedrock, AWS Lambda, and Amazon DynamoDB to provide text generation and analysis capabilities. The application architecture involves uploading a PDF document to an S3 bucket, extracting metadata, converting text to vectors, and using a LangChain to search for information related to user prompts. The application is not intended for production use and serves as a demonstration and educational tool.
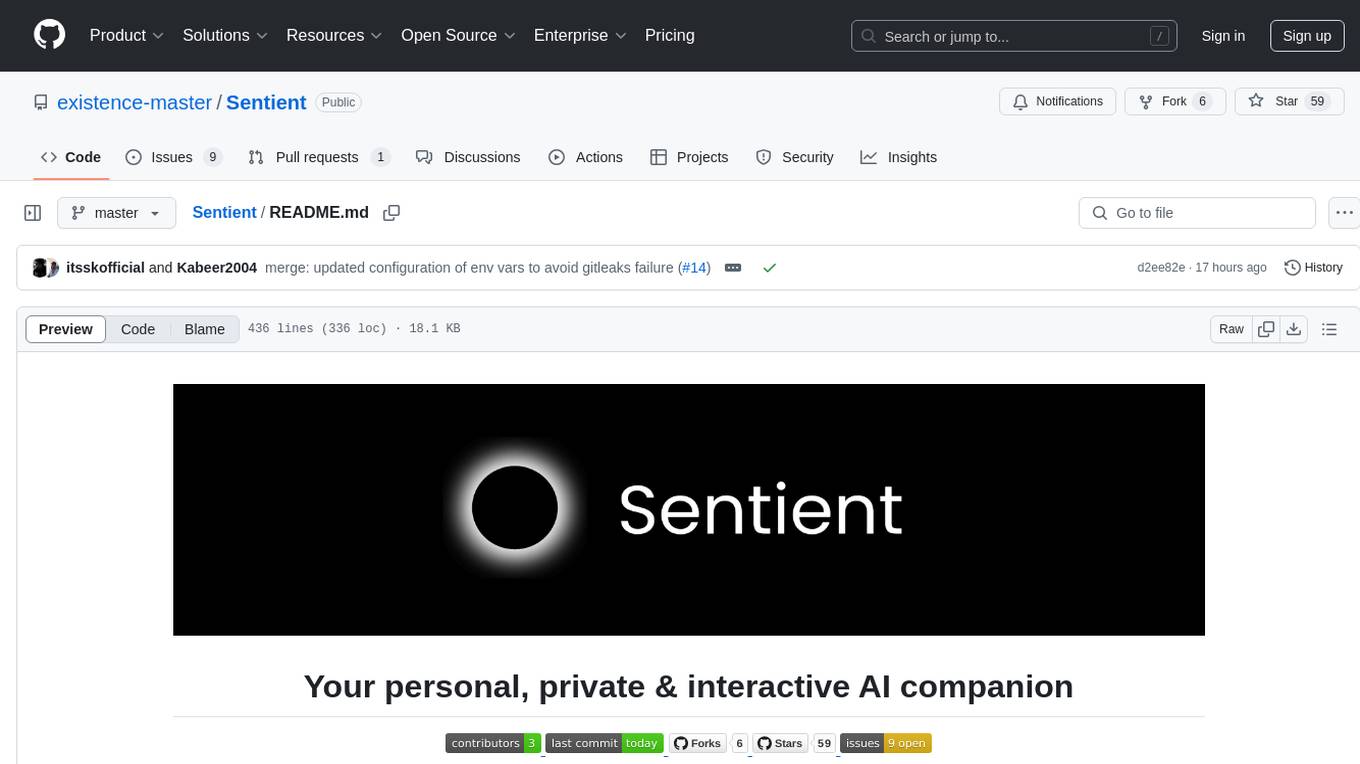
Sentient
Sentient is a personal, private, and interactive AI companion developed by Existence. The project aims to build a completely private AI companion that is deeply personalized and context-aware of the user. It utilizes automation and privacy to create a true companion for humans. The tool is designed to remember information about the user and use it to respond to queries and perform various actions. Sentient features a local and private environment, MBTI personality test, integrations with LinkedIn, Reddit, and more, self-managed graph memory, web search capabilities, multi-chat functionality, and auto-updates for the app. The project is built using technologies like ElectronJS, Next.js, TailwindCSS, FastAPI, Neo4j, and various APIs.
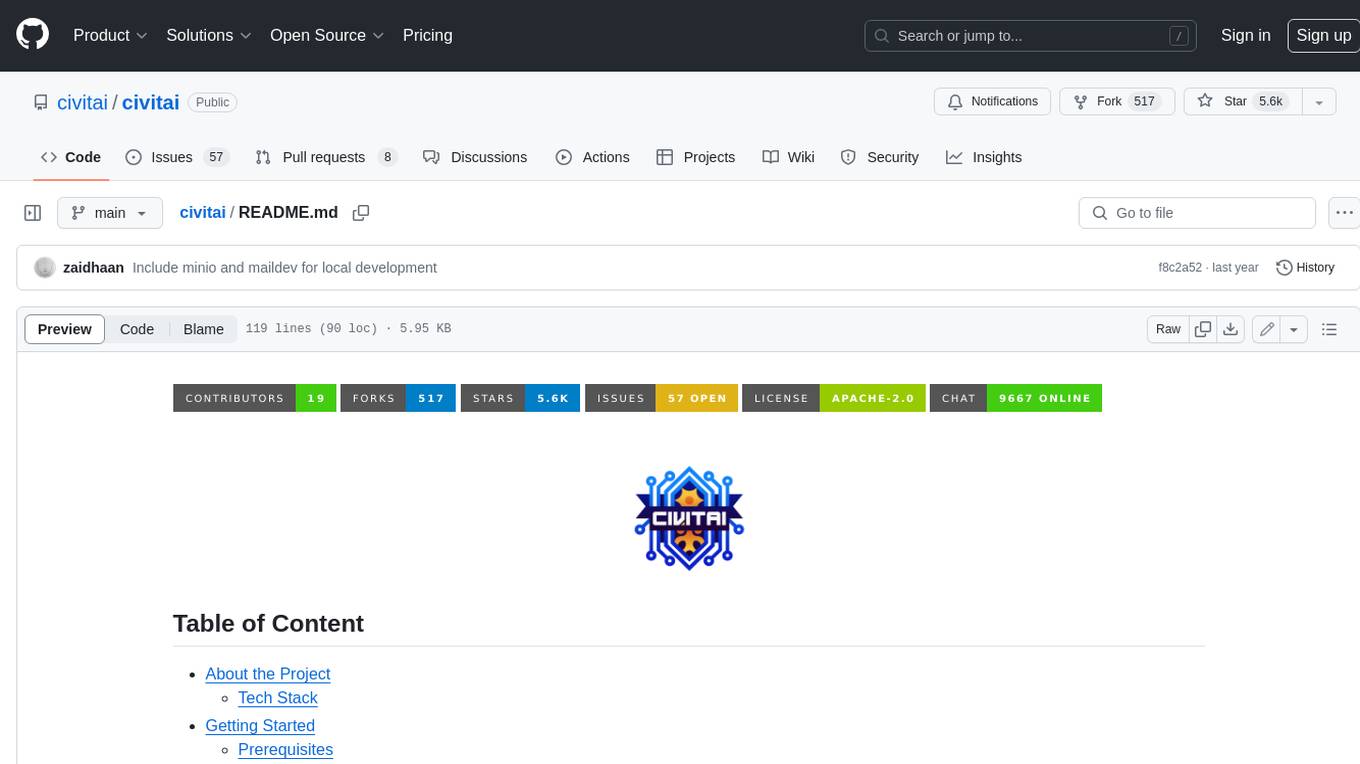
civitai
Civitai is a platform where people can share their stable diffusion models (textual inversions, hypernetworks, aesthetic gradients, VAEs, and any other crazy stuff people do to customize their AI generations), collaborate with others to improve them, and learn from each other's work. The platform allows users to create an account, upload their models, and browse models that have been shared by others. Users can also leave comments and feedback on each other's models to facilitate collaboration and knowledge sharing.
For similar tasks
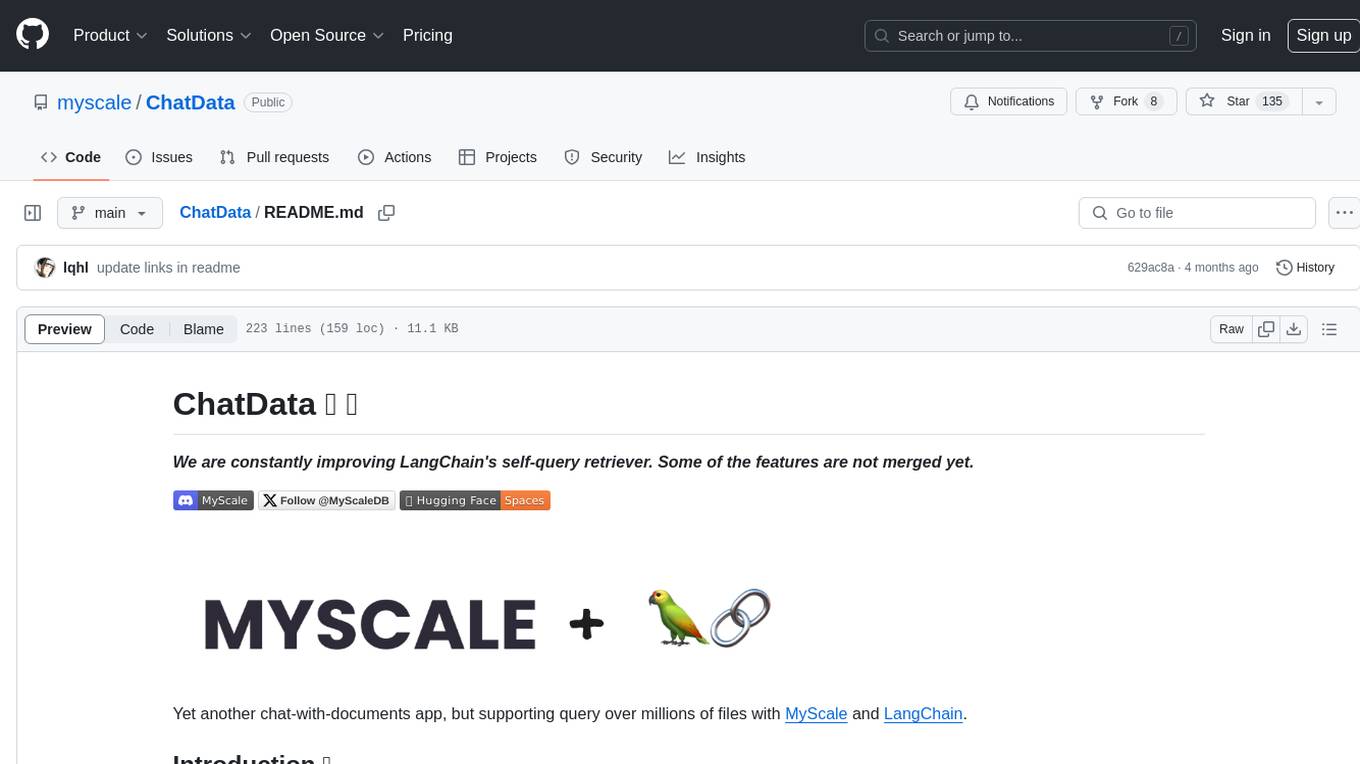
ChatData
ChatData is a robust chat-with-documents application designed to extract information and provide answers by querying the MyScale free knowledge base or uploaded documents. It leverages the Retrieval Augmented Generation (RAG) framework, millions of Wikipedia pages, and arXiv papers. Features include self-querying retriever, VectorSQL, session management, and building a personalized knowledge base. Users can effortlessly navigate vast data, explore academic papers, and research documents. ChatData empowers researchers, students, and knowledge enthusiasts to unlock the true potential of information retrieval.
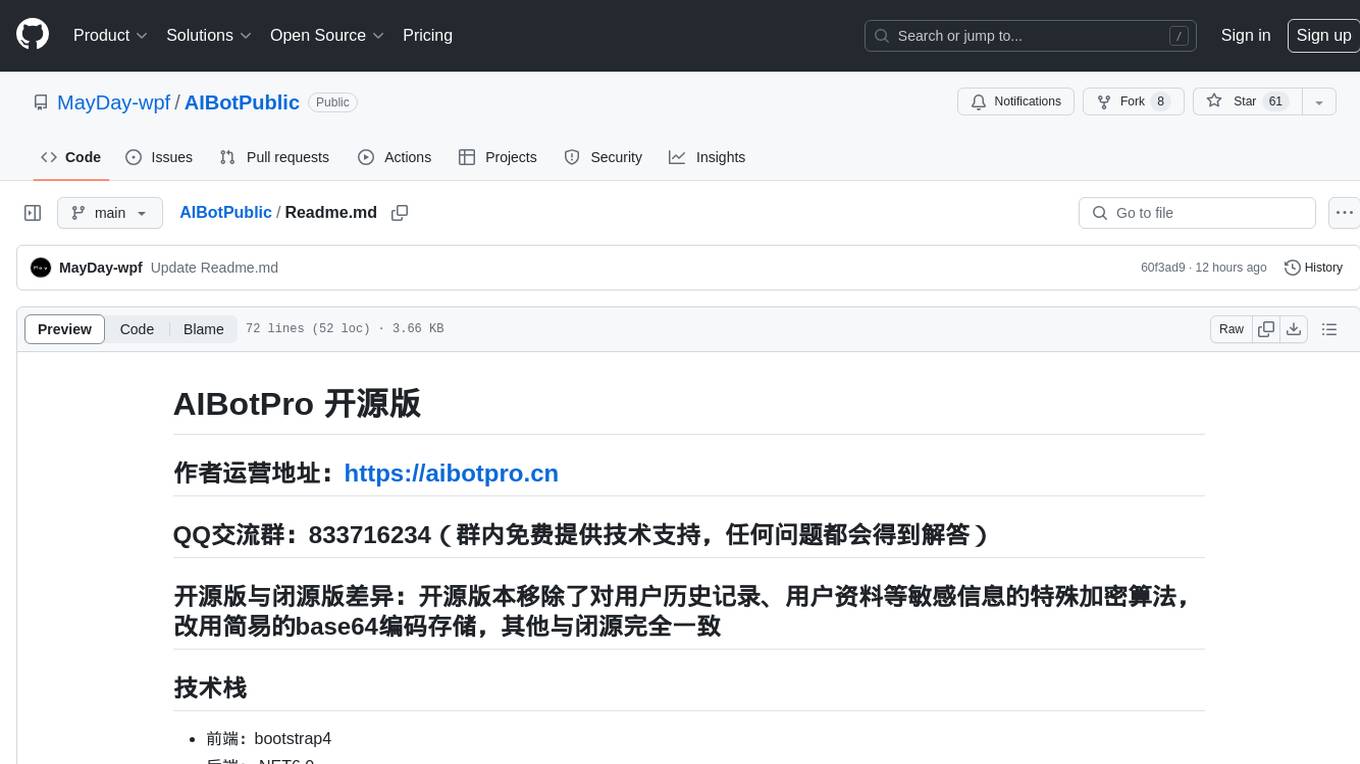
AIBotPublic
AIBotPublic is an open-source version of AIBotPro, a comprehensive AI tool that provides various features such as knowledge base construction, AI drawing, API hosting, and more. It supports custom plugins and parallel processing of multiple files. The tool is built using bootstrap4 for the frontend, .NET6.0 for the backend, and utilizes technologies like SqlServer, Redis, and Milvus for database and vector database functionalities. It integrates third-party dependencies like Baidu AI OCR, Milvus C# SDK, Google Search, and more to enhance its capabilities.
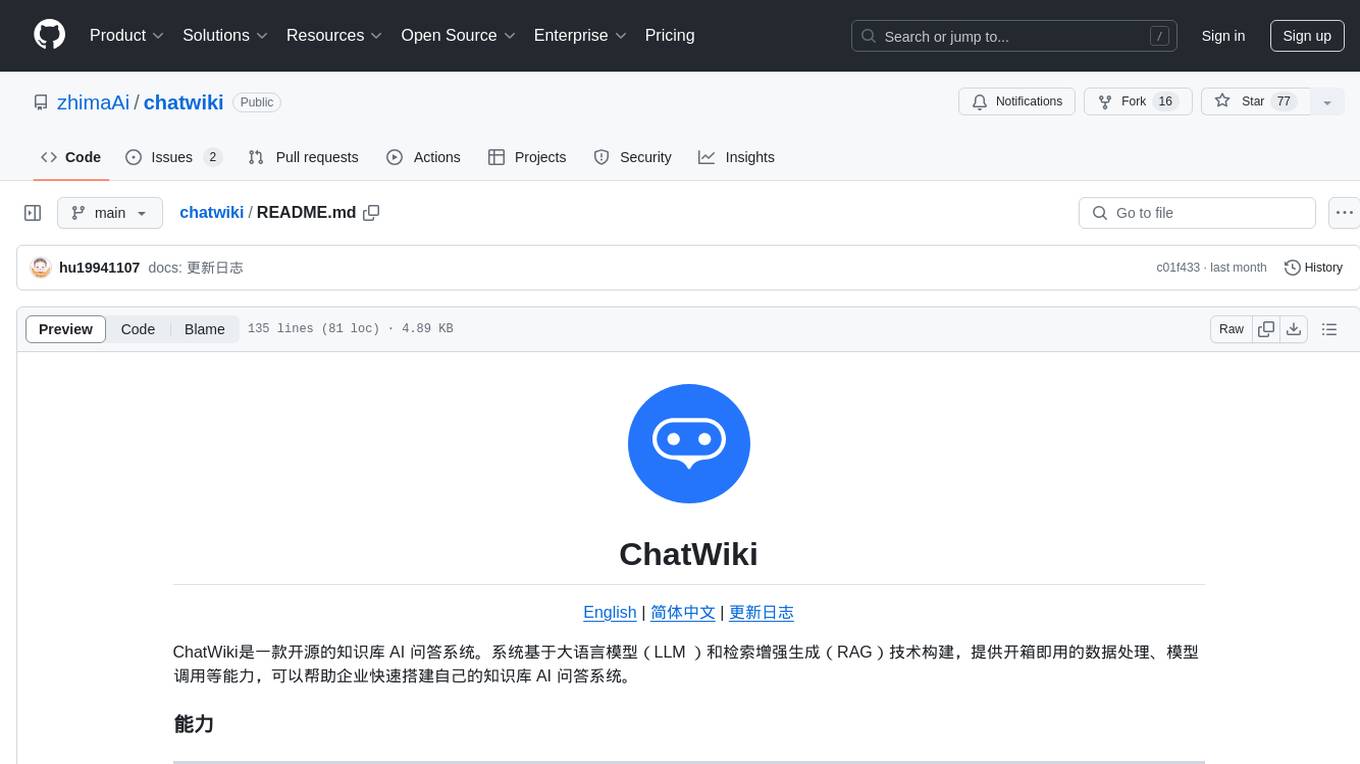
chatwiki
ChatWiki is an open-source knowledge base AI question-answering system. It is built on large language models (LLM) and retrieval-augmented generation (RAG) technologies, providing out-of-the-box data processing, model invocation capabilities, and helping enterprises quickly build their own knowledge base AI question-answering systems. It offers exclusive AI question-answering system, easy integration of models, data preprocessing, simple user interface design, and adaptability to different business scenarios.
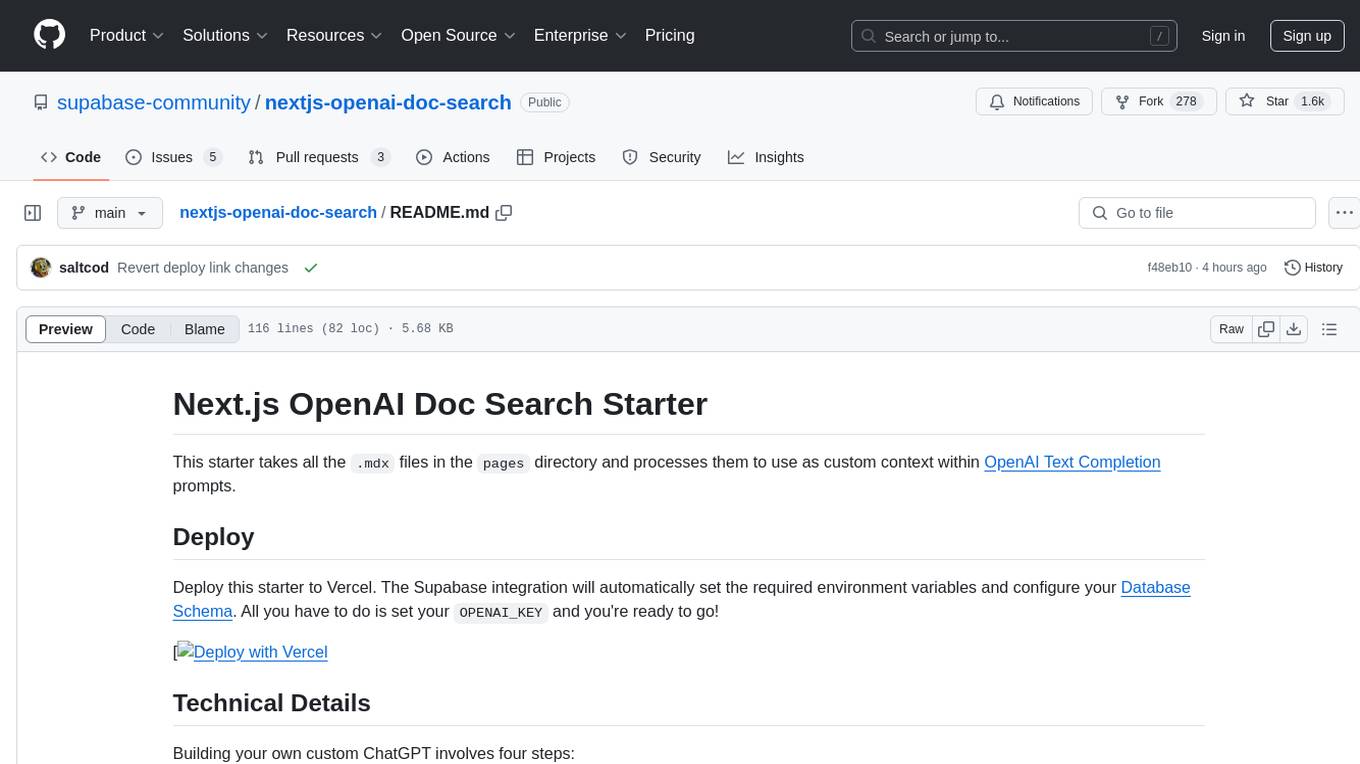
nextjs-openai-doc-search
This starter project is designed to process `.mdx` files in the `pages` directory to use as custom context within OpenAI Text Completion prompts. It involves building a custom ChatGPT style doc search powered by Next.js, OpenAI, and Supabase. The project includes steps for pre-processing knowledge base, storing embeddings in Postgres, performing vector similarity search, and injecting content into OpenAI GPT-3 text completion prompt.
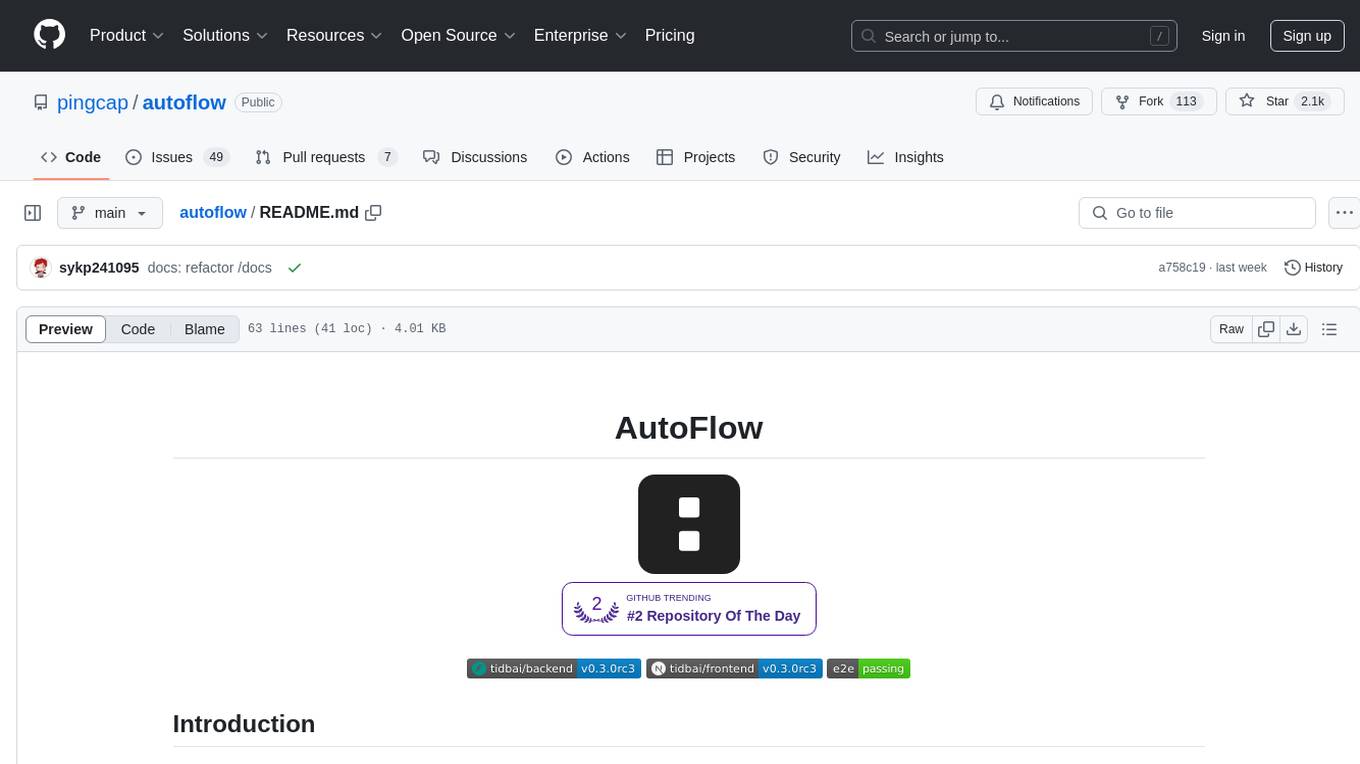
autoflow
AutoFlow is an open source graph rag based knowledge base tool built on top of TiDB Vector and LlamaIndex and DSPy. It features a Perplexity-style Conversational Search page and an Embeddable JavaScript Snippet for easy integration into websites. The tool allows for comprehensive coverage and streamlined search processes through sitemap URL scraping.
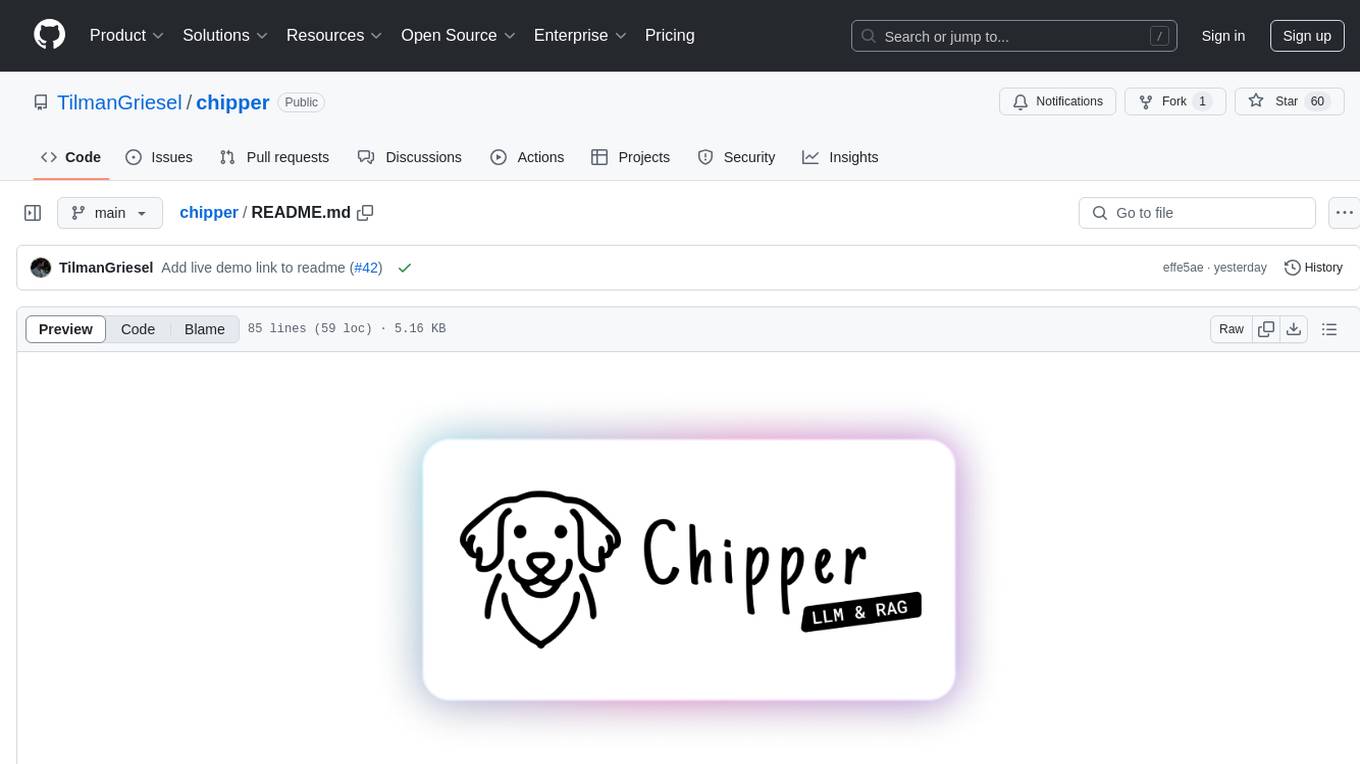
chipper
Chipper provides a web interface, CLI, and architecture for pipelines, document chunking, web scraping, and query workflows. It is built with Haystack, Ollama, Hugging Face, Docker, Tailwind, and ElasticSearch, running locally or as a Dockerized service. Originally created to assist in creative writing, it now offers features like local Ollama and Hugging Face API, ElasticSearch embeddings, document splitting, web scraping, audio transcription, user-friendly CLI, and Docker deployment. The project aims to be educational, beginner-friendly, and a playground for AI exploration and innovation.
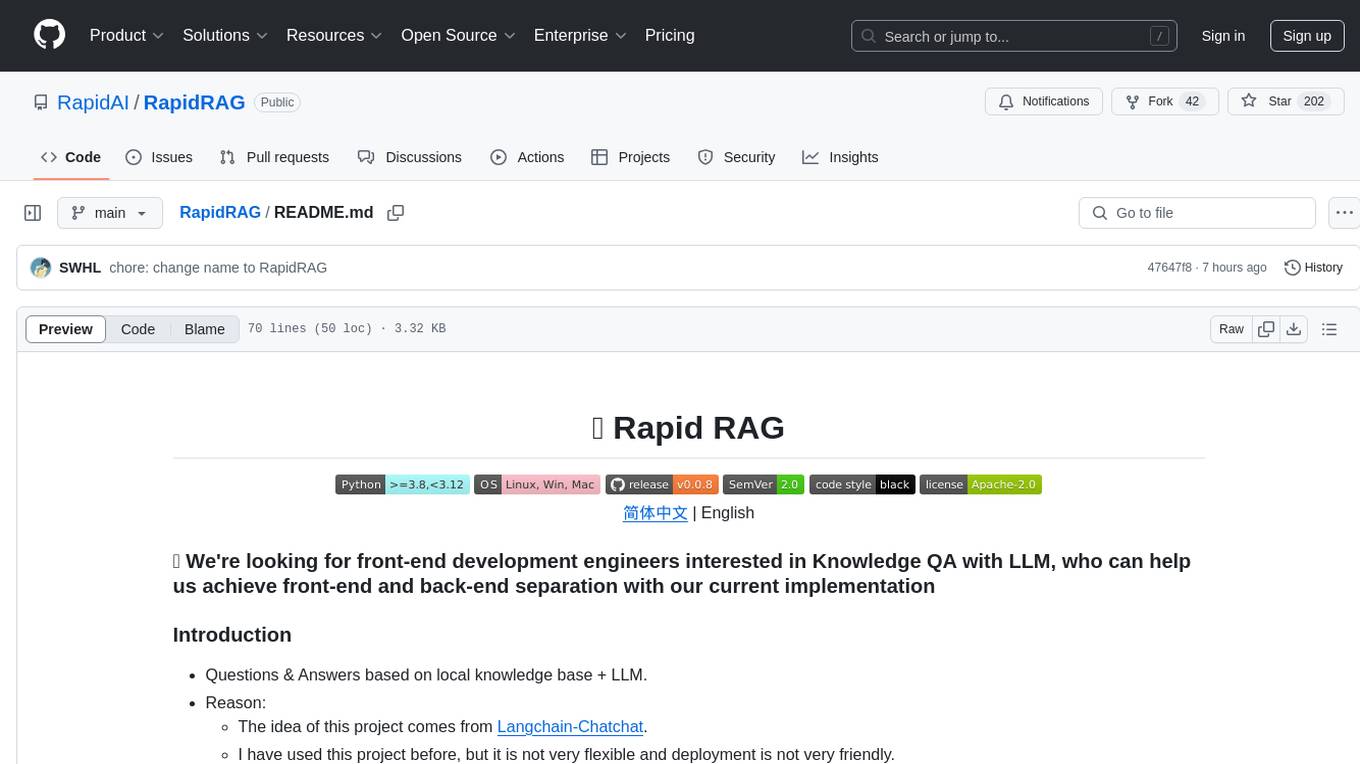
RapidRAG
RapidRAG is a project focused on Knowledge QA with LLM, combining Questions & Answers based on local knowledge base with a large language model. The project aims to provide a flexible and deployment-friendly solution for building a knowledge question answering system. It is modularized, allowing easy replacement of parts and simple code understanding. The tool supports various document formats and can utilize CPU for most parts, with the large language model interface requiring separate deployment.
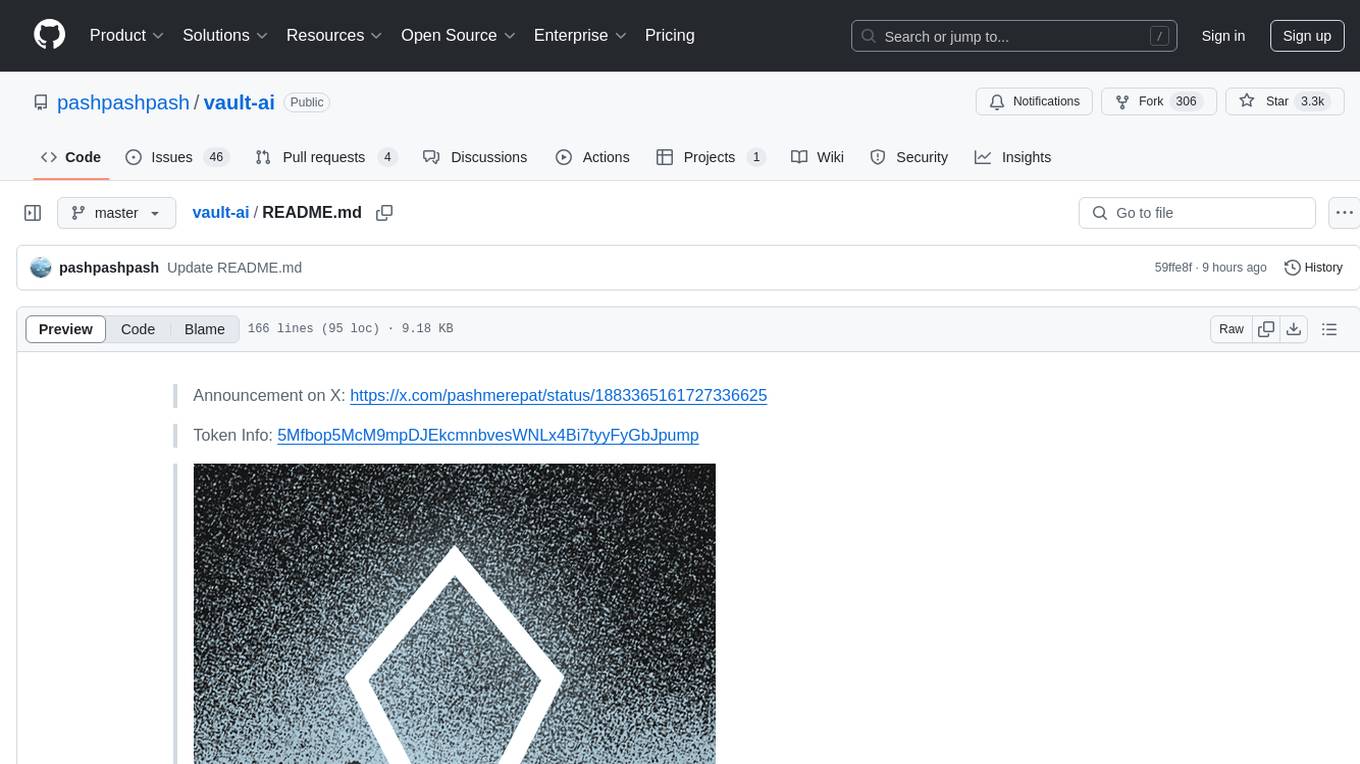
vault-ai
OP Vault is a tool that leverages the OP Stack (OpenAI + Pinecone Vector Database) to allow users to upload custom knowledgebase files and ask questions about their contents. It provides a user-friendly Golang server and React frontend for querying human-readable content like books and documents, making it valuable for knowledge extraction and question-answering. Users can upload entire libraries, receive specific answers with file and section references, and explore the power of the OP Stack in a practical interface.
For similar jobs
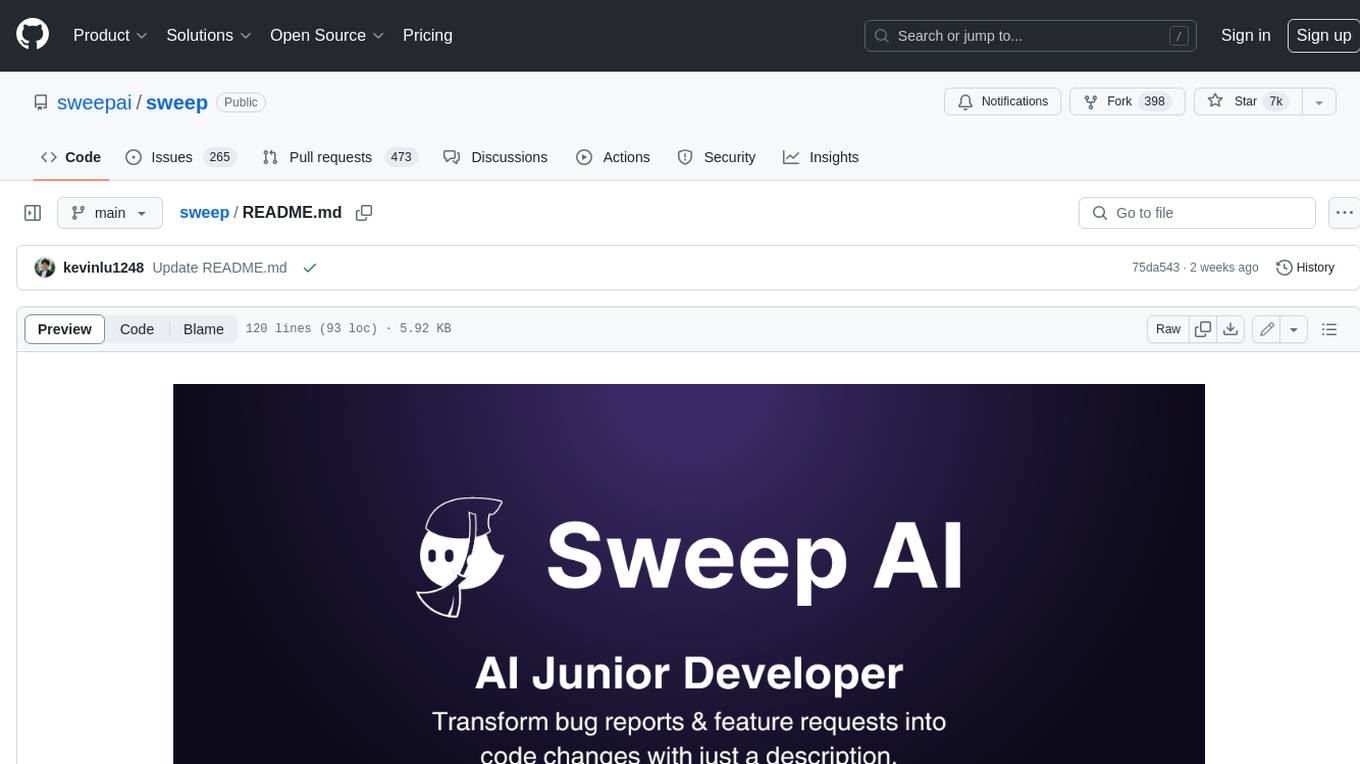
sweep
Sweep is an AI junior developer that turns bugs and feature requests into code changes. It automatically handles developer experience improvements like adding type hints and improving test coverage.
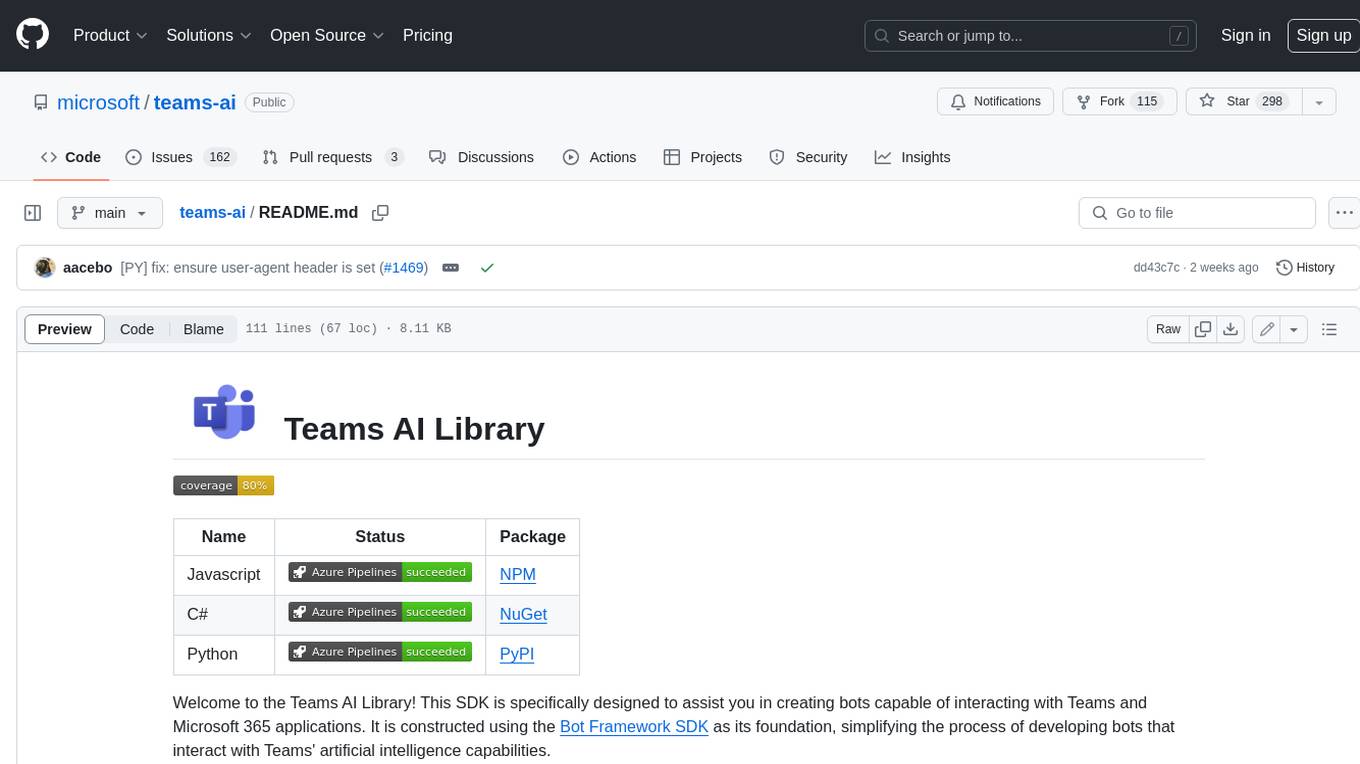
teams-ai
The Teams AI Library is a software development kit (SDK) that helps developers create bots that can interact with Teams and Microsoft 365 applications. It is built on top of the Bot Framework SDK and simplifies the process of developing bots that interact with Teams' artificial intelligence capabilities. The SDK is available for JavaScript/TypeScript, .NET, and Python.
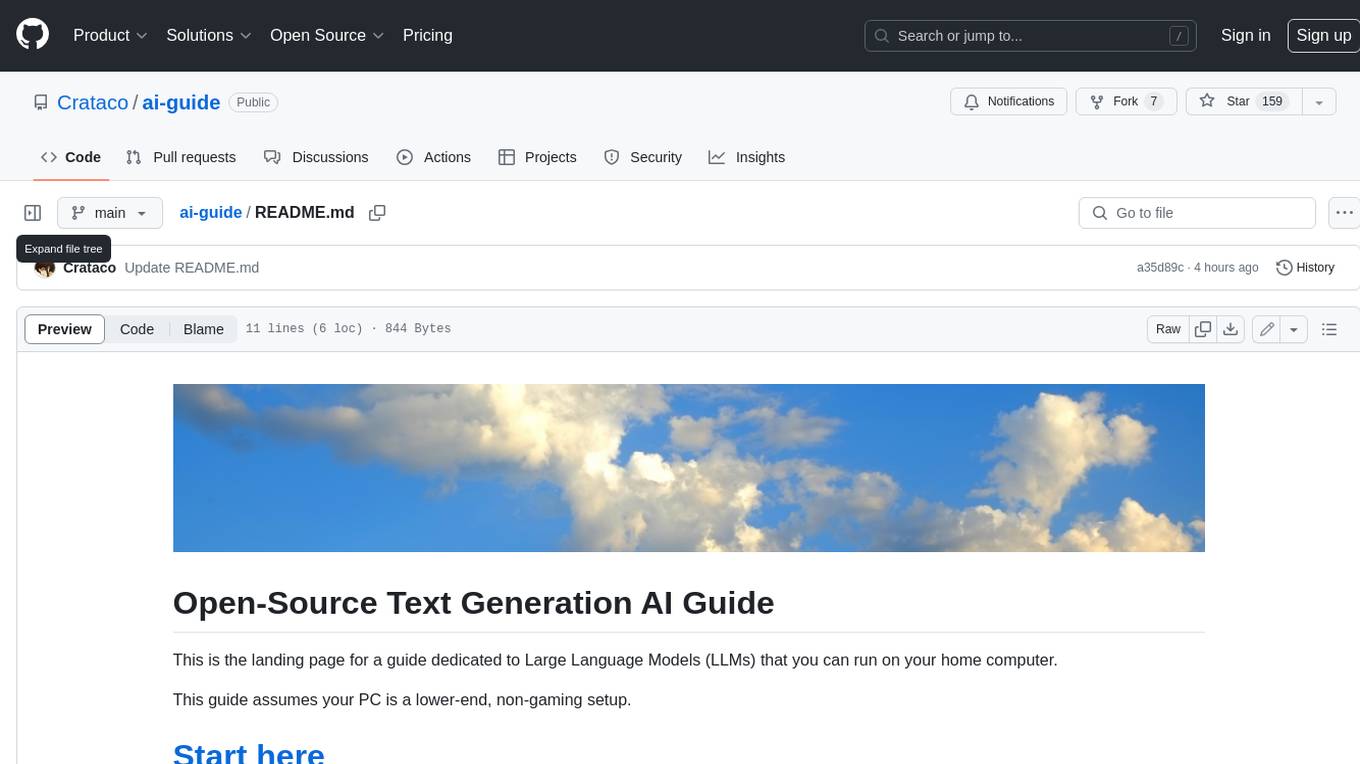
ai-guide
This guide is dedicated to Large Language Models (LLMs) that you can run on your home computer. It assumes your PC is a lower-end, non-gaming setup.
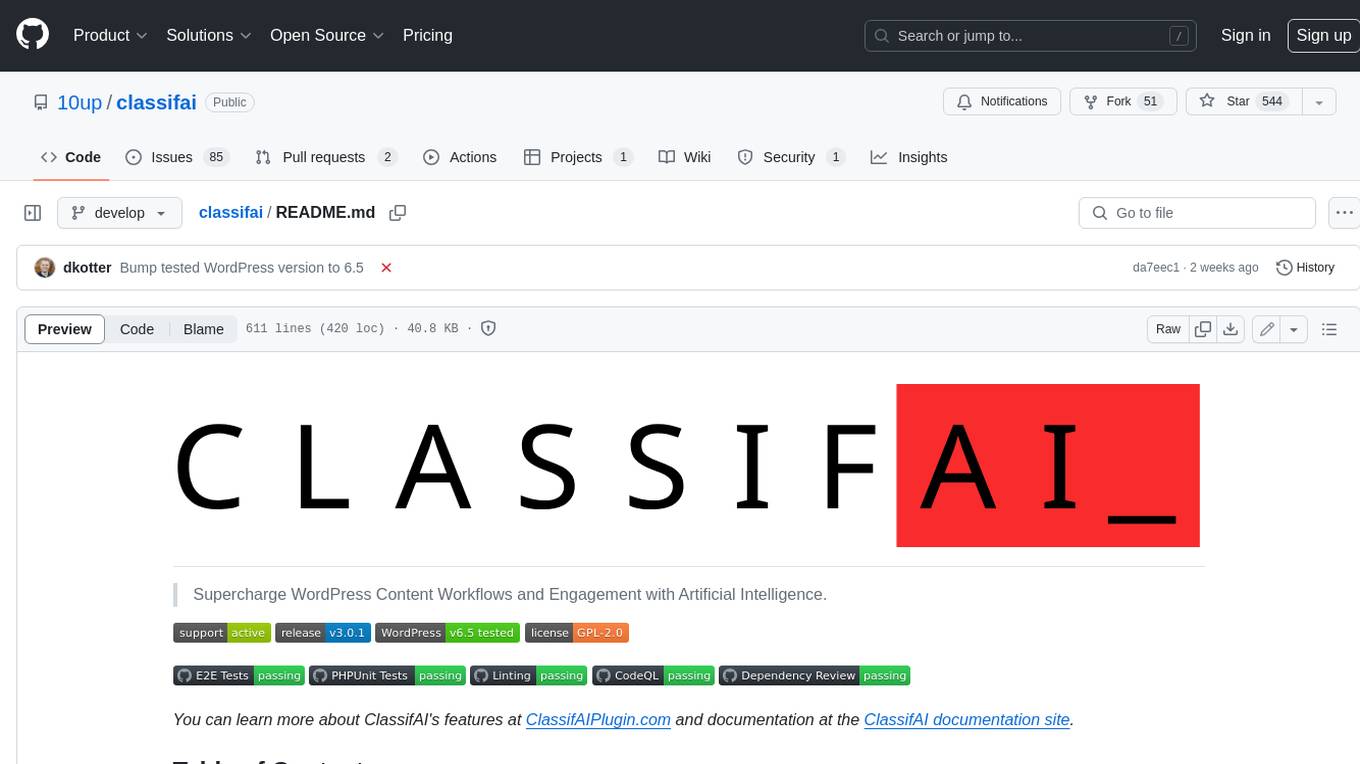
classifai
Supercharge WordPress Content Workflows and Engagement with Artificial Intelligence. Tap into leading cloud-based services like OpenAI, Microsoft Azure AI, Google Gemini and IBM Watson to augment your WordPress-powered websites. Publish content faster while improving SEO performance and increasing audience engagement. ClassifAI integrates Artificial Intelligence and Machine Learning technologies to lighten your workload and eliminate tedious tasks, giving you more time to create original content that matters.
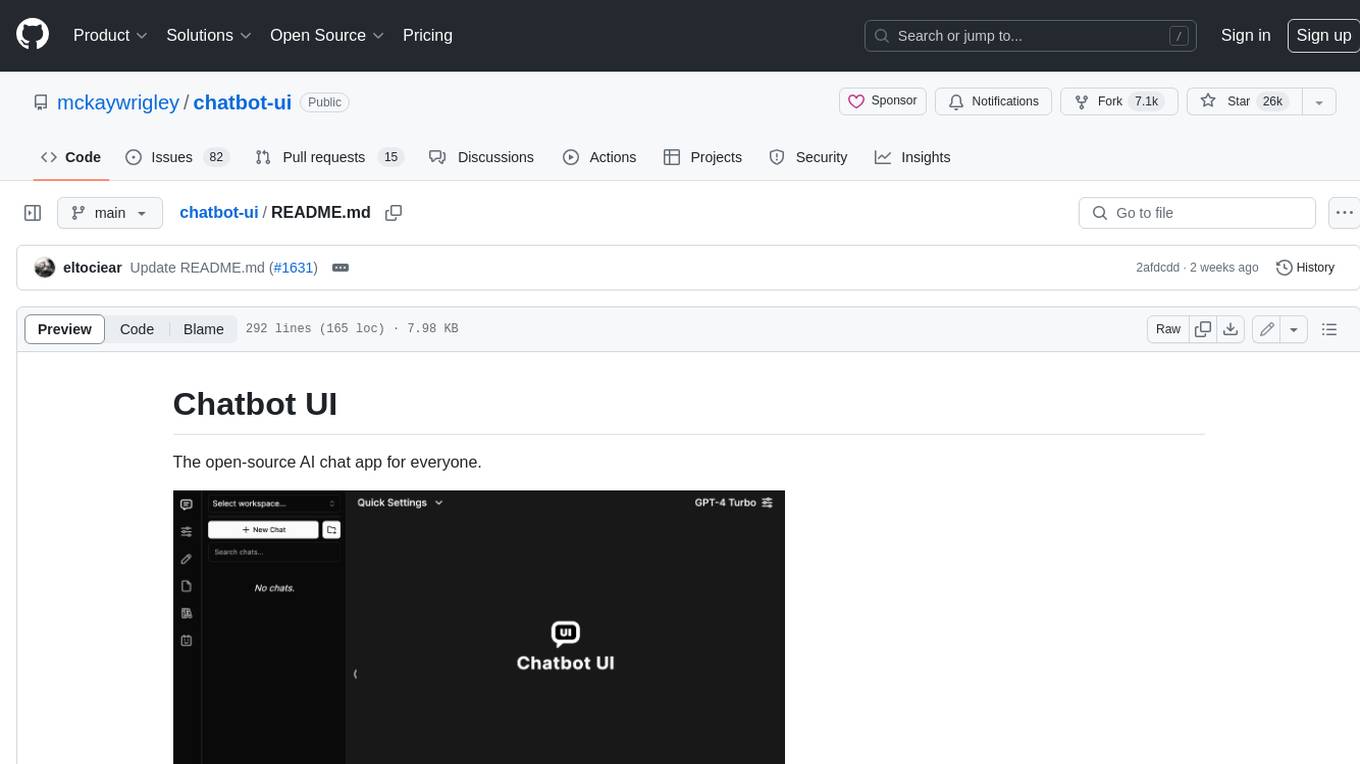
chatbot-ui
Chatbot UI is an open-source AI chat app that allows users to create and deploy their own AI chatbots. It is easy to use and can be customized to fit any need. Chatbot UI is perfect for businesses, developers, and anyone who wants to create a chatbot.
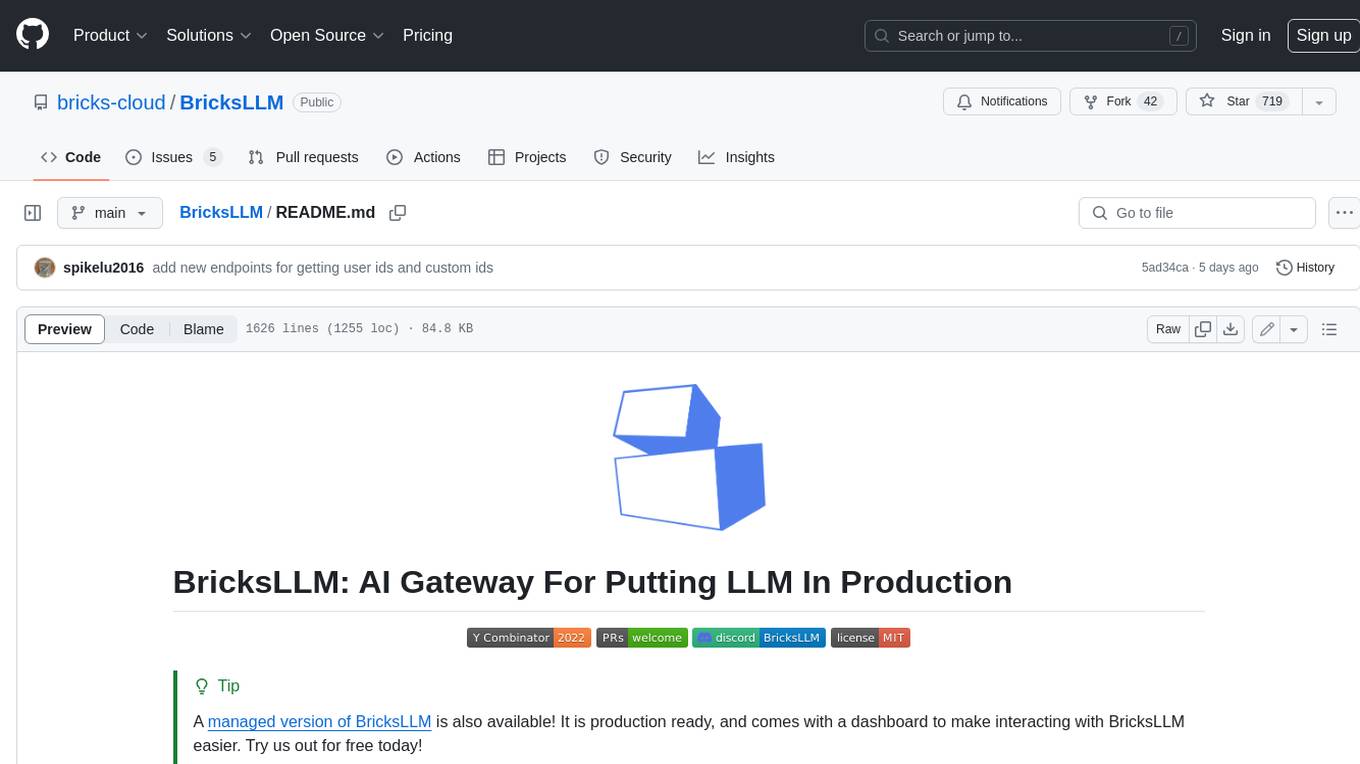
BricksLLM
BricksLLM is a cloud native AI gateway written in Go. Currently, it provides native support for OpenAI, Anthropic, Azure OpenAI and vLLM. BricksLLM aims to provide enterprise level infrastructure that can power any LLM production use cases. Here are some use cases for BricksLLM: * Set LLM usage limits for users on different pricing tiers * Track LLM usage on a per user and per organization basis * Block or redact requests containing PIIs * Improve LLM reliability with failovers, retries and caching * Distribute API keys with rate limits and cost limits for internal development/production use cases * Distribute API keys with rate limits and cost limits for students
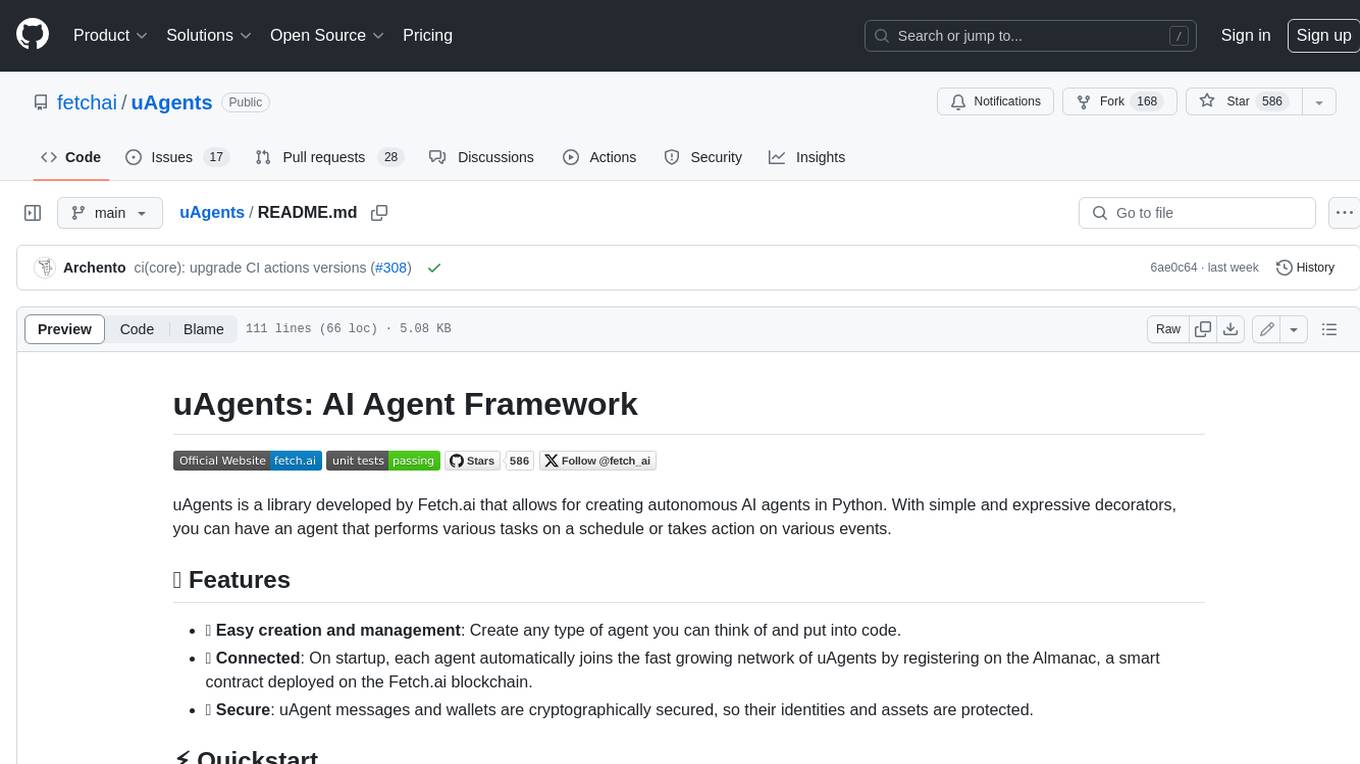
uAgents
uAgents is a Python library developed by Fetch.ai that allows for the creation of autonomous AI agents. These agents can perform various tasks on a schedule or take action on various events. uAgents are easy to create and manage, and they are connected to a fast-growing network of other uAgents. They are also secure, with cryptographically secured messages and wallets.
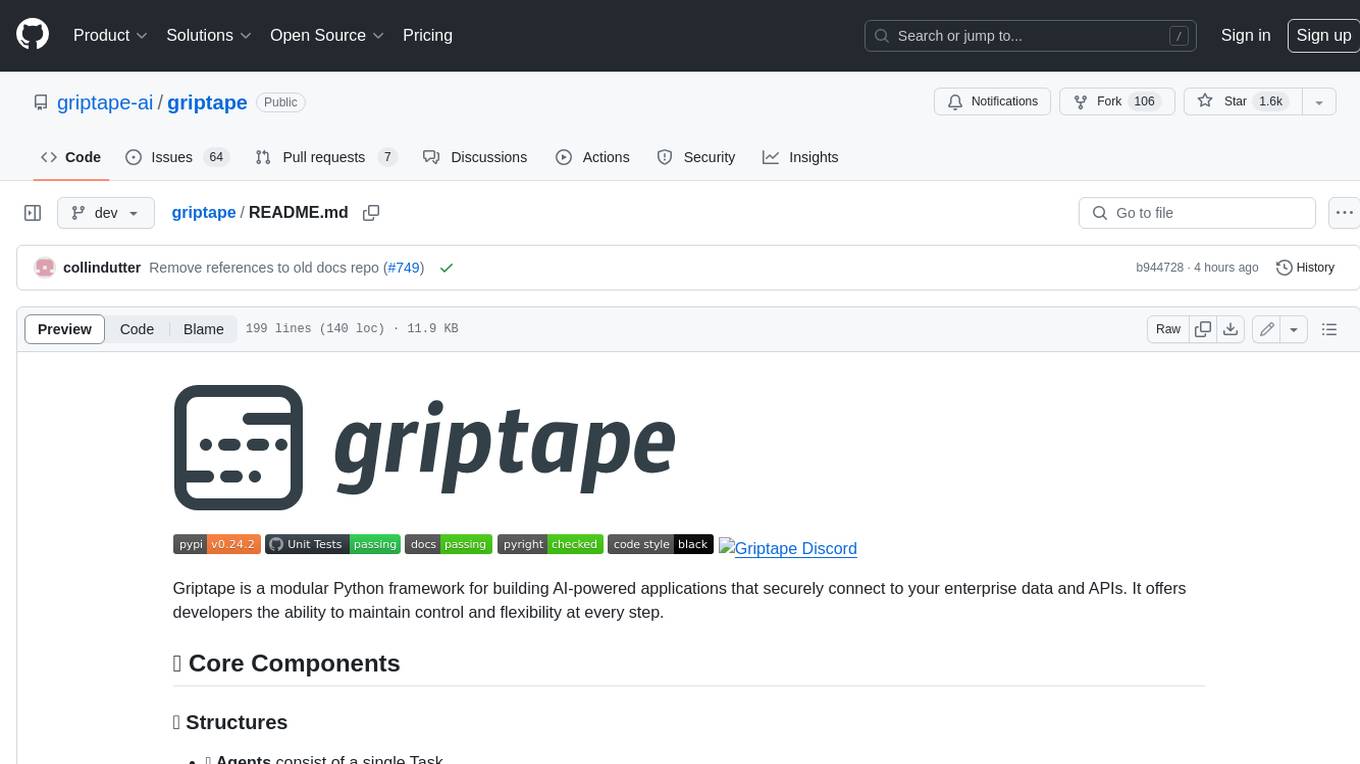
griptape
Griptape is a modular Python framework for building AI-powered applications that securely connect to your enterprise data and APIs. It offers developers the ability to maintain control and flexibility at every step. Griptape's core components include Structures (Agents, Pipelines, and Workflows), Tasks, Tools, Memory (Conversation Memory, Task Memory, and Meta Memory), Drivers (Prompt and Embedding Drivers, Vector Store Drivers, Image Generation Drivers, Image Query Drivers, SQL Drivers, Web Scraper Drivers, and Conversation Memory Drivers), Engines (Query Engines, Extraction Engines, Summary Engines, Image Generation Engines, and Image Query Engines), and additional components (Rulesets, Loaders, Artifacts, Chunkers, and Tokenizers). Griptape enables developers to create AI-powered applications with ease and efficiency.