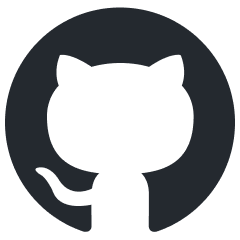
gemini-ai
A Ruby Gem for interacting with Gemini through Vertex AI, Generative Language API, or AI Studio, Google's generative AI services.
Stars: 85
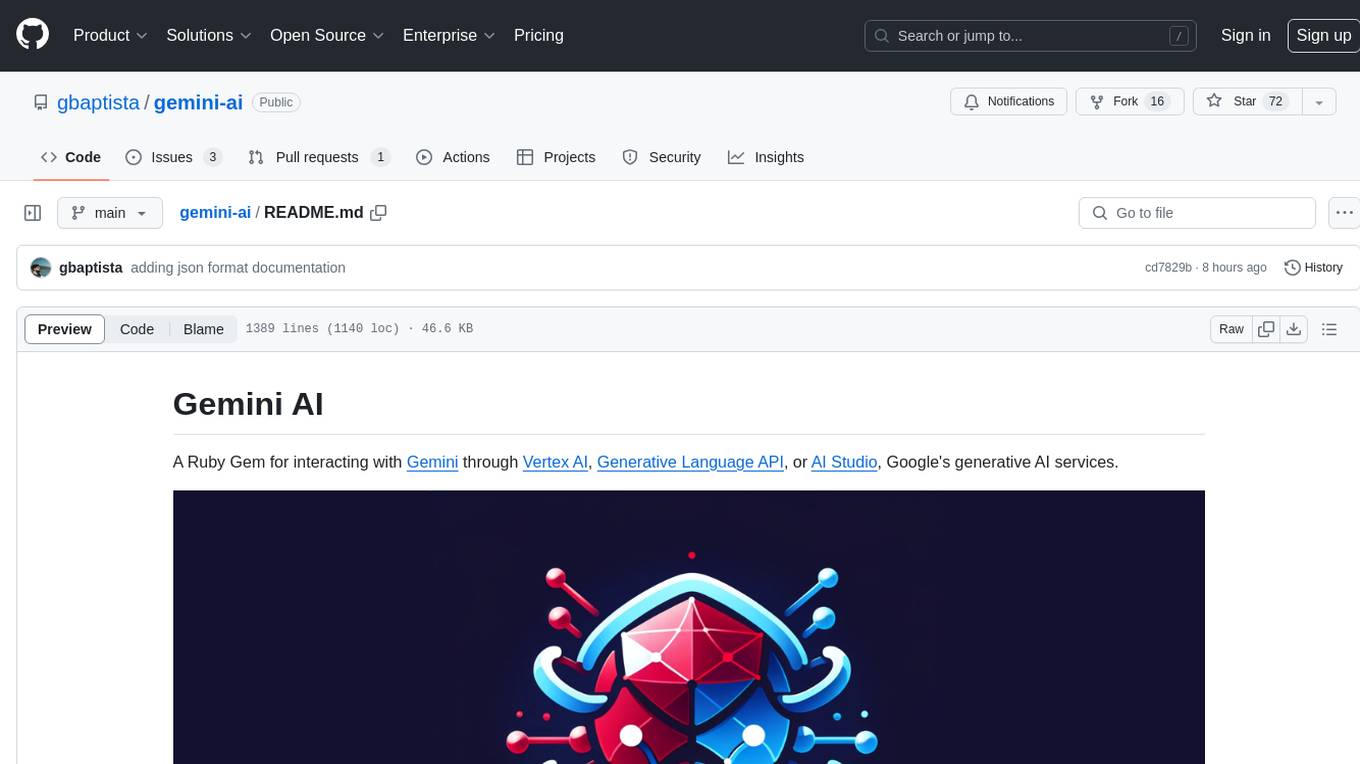
Gemini AI is a Ruby Gem designed to provide low-level access to Google's generative AI services through Vertex AI, Generative Language API, or AI Studio. It allows users to interact with Gemini to build abstractions on top of it. The Gem provides functionalities for tasks such as generating content, embeddings, predictions, and more. It supports streaming capabilities, server-sent events, safety settings, system instructions, JSON format responses, and tools (functions) calling. The Gem also includes error handling, development setup, publishing to RubyGems, updating the README, and references to resources for further learning.
README:
A Ruby Gem for interacting with Gemini through Vertex AI, Generative Language API, or AI Studio, Google's generative AI services.
This Gem is designed to provide low-level access to Gemini, enabling people to build abstractions on top of it. If you are interested in more high-level abstractions or more user-friendly tools, you may want to consider Nano Bots π π€.
gem 'gemini-ai', '~> 4.2.0'
require 'gemini-ai'
# With an API key
client = Gemini.new(
credentials: {
service: 'generative-language-api',
api_key: ENV['GOOGLE_API_KEY']
},
options: { model: 'gemini-pro', server_sent_events: true }
)
# With a Service Account Credentials File
client = Gemini.new(
credentials: {
service: 'vertex-ai-api',
file_path: 'google-credentials.json',
region: 'us-east4'
},
options: { model: 'gemini-pro', server_sent_events: true }
)
# With the Service Account Credentials File contents
client = Gemini.new(
credentials: {
service: 'vertex-ai-api',
file_contents: File.read('google-credentials.json'),
# file_contents: ENV['GOOGLE_CREDENTIALS_FILE_CONTENTS'],
region: 'us-east4'
},
options: { model: 'gemini-pro', server_sent_events: true }
)
# With Application Default Credentials
client = Gemini.new(
credentials: {
service: 'vertex-ai-api',
region: 'us-east4'
},
options: { model: 'gemini-pro', server_sent_events: true }
)
result = client.stream_generate_content({
contents: { role: 'user', parts: { text: 'hi!' } }
})
Result:
[{ 'candidates' =>
[{ 'content' => {
'role' => 'model',
'parts' => [{ 'text' => 'Hello! How may I assist you?' }]
},
'finishReason' => 'STOP',
'safetyRatings' =>
[{ 'category' => 'HARM_CATEGORY_HARASSMENT', 'probability' => 'NEGLIGIBLE' },
{ 'category' => 'HARM_CATEGORY_HATE_SPEECH', 'probability' => 'NEGLIGIBLE' },
{ 'category' => 'HARM_CATEGORY_SEXUALLY_EXPLICIT', 'probability' => 'NEGLIGIBLE' },
{ 'category' => 'HARM_CATEGORY_DANGEROUS_CONTENT', 'probability' => 'NEGLIGIBLE' }] }],
'usageMetadata' => {
'promptTokenCount' => 2,
'candidatesTokenCount' => 8,
'totalTokenCount' => 10
} }]
- TL;DR and Quick Start
- Index
- Setup
- Available Models
- Usage
- Development
- Resources and References
- Disclaimer
gem install gemini-ai -v 4.2.0
gem 'gemini-ai', '~> 4.2.0'
- Option 1: API Key (Generative Language API)
- Option 2: Service Account Credentials File (Vertex AI API)
- Option 3: Application Default Credentials (Vertex AI API)
- Required Data
β οΈ DISCLAIMER: Be careful with what you are doing, and never trust others' code related to this. These commands and instructions alter the level of access to your Google Cloud Account, and running them naively can lead to security risks as well as financial risks. People with access to your account can use it to steal data or incur charges. Run these commands at your own responsibility and due diligence; expect no warranties from the contributors of this project.
You need a Google Cloud Project, and then you can generate an API Key through the Google Cloud Console here.
You also need to enable the Generative Language API service in your Google Cloud Console, which can be done here.
Alternatively, you can generate an API Key through Google AI Studio here. However, this approach will automatically create a project for you in your Google Cloud Account.
You need a Google Cloud Project and a Service Account to use Vertex AI API.
After creating them, you need to enable the Vertex AI API for your project by clicking Enable
here: Vertex AI API.
You can create credentials for your Service Account here, where you will be able to download a JSON file named google-credentials.json
that should have content similar to this:
{
"type": "service_account",
"project_id": "YOUR_PROJECT_ID",
"private_key_id": "a00...",
"private_key": "-----BEGIN PRIVATE KEY-----\n...\n-----END PRIVATE KEY-----\n",
"client_email": "PROJECT_ID@PROJECT_ID.iam.gserviceaccount.com",
"client_id": "000...",
"auth_uri": "https://accounts.google.com/o/oauth2/auth",
"token_uri": "https://oauth2.googleapis.com/token",
"auth_provider_x509_cert_url": "https://www.googleapis.com/oauth2/v1/certs",
"client_x509_cert_url": "https://www.googleapis.com/robot/v1/metadata/x509/..."
}
You need to have the necessary policies (roles/aiplatform.user
and possibly roles/ml.admin
) in place to use the Vertex AI API.
You can add them by navigating to the IAM Console and clicking on the "Edit principal" (βοΈ pencil icon) next to your Service Account.
Alternatively, you can add them through the gcloud CLI as follows:
gcloud projects add-iam-policy-binding PROJECT_ID \
--member='serviceAccount:PROJECT_ID@PROJECT_ID.iam.gserviceaccount.com' \
--role='roles/aiplatform.user'
Some people reported having trouble accessing the API, and adding the role roles/ml.admin
fixed it:
gcloud projects add-iam-policy-binding PROJECT_ID \
--member='serviceAccount:PROJECT_ID@PROJECT_ID.iam.gserviceaccount.com' \
--role='roles/ml.admin'
If you are not using a Service Account:
gcloud projects add-iam-policy-binding PROJECT_ID \
--member='user:[email protected]' \
--role='roles/aiplatform.user'
gcloud projects add-iam-policy-binding PROJECT_ID \
--member='user:[email protected]' \
--role='roles/ml.admin'
Similar to Option 2, but you don't need to download a google-credentials.json
. Application Default Credentials automatically find credentials based on the application environment.
For local development, you can generate your default credentials using the gcloud CLI as follows:
gcloud auth application-default login
For more details about alternative methods and different environments, check the official documentation: Set up Application Default Credentials
After choosing an option, you should have all the necessary data and access to use Gemini.
Option 1, for API Key:
{
service: 'generative-language-api',
api_key: 'GOOGLE_API_KEY'
}
Remember that hardcoding your API key in code is unsafe; it's preferable to use environment variables:
{
service: 'generative-language-api',
api_key: ENV['GOOGLE_API_KEY']
}
Option 2: For the Service Account, provide a google-credentials.json
file and a REGION
:
{
service: 'vertex-ai-api',
file_path: 'google-credentials.json',
region: 'us-east4'
}
Alternatively, you can pass the file contents instead of the path:
{
service: 'vertex-ai-api',
file_contents: File.read('google-credentials.json'),
region: 'us-east4'
}
{
service: 'vertex-ai-api',
file_contents: ENV['GOOGLE_CREDENTIALS_FILE_CONTENTS'],
region: 'us-east4'
}
Option 3: For Application Default Credentials, omit both the api_key
and the file_path
:
{
service: 'vertex-ai-api',
region: 'us-east4'
}
As of the writing of this README, the following regions support Gemini:
Iowa (us-central1)
Las Vegas, Nevada (us-west4)
MontrΓ©al, Canada (northamerica-northeast1)
Northern Virginia (us-east4)
Oregon (us-west1)
Seoul, Korea (asia-northeast3)
Singapore (asia-southeast1)
Tokyo, Japan (asia-northeast1)
You can follow here if new regions are available: Gemini API
You might want to explicitly set a Google Cloud Project ID, which you can do as follows:
{
service: 'vertex-ai-api',
project_id: 'PROJECT_ID'
}
By default, the gem uses the v1
version of the APIs. You may want to use a different version:
# With an API key
client = Gemini.new(
credentials: {
service: 'generative-language-api',
api_key: ENV['GOOGLE_API_KEY'],
version: 'v1beta'
},
options: { model: 'gemini-pro', server_sent_events: true }
)
# With a Service Account Credentials File
client = Gemini.new(
credentials: {
service: 'vertex-ai-api',
file_path: 'google-credentials.json',
region: 'us-east4',
version: 'v1beta'
},
options: { model: 'gemini-pro', server_sent_events: true }
)
# With the Service Account Credentials File contents
client = Gemini.new(
credentials: {
service: 'vertex-ai-api',
file_contents: File.read('google-credentials.json'),
# file_contents: ENV['GOOGLE_CREDENTIALS_FILE_CONTENTS'],
region: 'us-east4'
},
options: { model: 'gemini-pro', server_sent_events: true }
)
# With Application Default Credentials
client = Gemini.new(
credentials: {
service: 'vertex-ai-api',
region: 'us-east4',
version: 'v1beta'
},
options: { model: 'gemini-pro', server_sent_events: true }
)
These models are accessible to the repository author as of June 2025 in the us-east4
region. Access to models may vary by region, user, and account. All models here are expected to work, if you can access them. This is just a reference of what a "typical" user may expect to have access to right away:
Model | Vertex AI | Generative Language |
---|---|---|
gemini-pro-vision | β | π |
gemini-pro | β | β |
gemini-1.5-pro-preview-0514 | β | π |
gemini-1.5-pro-preview-0409 | β | π |
gemini-1.5-pro | β | β |
gemini-1.5-flash-preview-0514 | β | π |
gemini-1.5-flash | β | β |
gemini-1.0-pro-vision-latest | π | π |
gemini-1.0-pro-vision-001 | β | π |
gemini-1.0-pro-vision | β | π |
gemini-1.0-pro-latest | π | β |
gemini-1.0-pro-002 | β | π |
gemini-1.0-pro-001 | β | β |
gemini-1.0-pro | β | β |
gemini-ultra | π | π |
gemini-1.0-ultra | π | π |
gemini-1.0-ultra-001 | π | π |
text-embedding-preview-0514 | π | π |
text-embedding-preview-0409 | π | π |
text-embedding-004 | β | β |
embedding-001 | π | β |
text-multilingual-embedding-002 | β | π |
textembedding-gecko-multilingual@001 | β | π |
textembedding-gecko-multilingual@latest | β | π |
textembedding-gecko@001 | β | π |
textembedding-gecko@002 | β | π |
textembedding-gecko@003 | β | π |
textembedding-gecko@latest | β | π |
You can follow new models at:
This is the code used for generating this table that you can use to explore your own access.
Ensure that you have all the required data for authentication.
Create a new client:
require 'gemini-ai'
# With an API key
client = Gemini.new(
credentials: {
service: 'generative-language-api',
api_key: ENV['GOOGLE_API_KEY']
},
options: { model: 'gemini-pro', server_sent_events: true }
)
# With a Service Account Credentials File
client = Gemini.new(
credentials: {
service: 'vertex-ai-api',
file_path: 'google-credentials.json',
region: 'us-east4'
},
options: { model: 'gemini-pro', server_sent_events: true }
)
# With the Service Account Credentials File contents
client = Gemini.new(
credentials: {
service: 'vertex-ai-api',
file_contents: File.read('google-credentials.json'),
# file_contents: ENV['GOOGLE_CREDENTIALS_FILE_CONTENTS'],
region: 'us-east4'
},
options: { model: 'gemini-pro', server_sent_events: true }
)
# With Application Default Credentials
client = Gemini.new(
credentials: {
service: 'vertex-ai-api',
region: 'us-east4'
},
options: { model: 'gemini-pro', server_sent_events: true }
)
Ensure that you have enabled Server-Sent Events before using blocks for streaming:
client.stream_generate_content(
{ contents: { role: 'user', parts: { text: 'hi!' } } }
) do |event, parsed, raw|
puts event
end
Event:
{ 'candidates' =>
[{ 'content' => {
'role' => 'model',
'parts' => [{ 'text' => 'Hello! How may I assist you?' }]
},
'finishReason' => 'STOP',
'safetyRatings' =>
[{ 'category' => 'HARM_CATEGORY_HARASSMENT', 'probability' => 'NEGLIGIBLE' },
{ 'category' => 'HARM_CATEGORY_HATE_SPEECH', 'probability' => 'NEGLIGIBLE' },
{ 'category' => 'HARM_CATEGORY_SEXUALLY_EXPLICIT', 'probability' => 'NEGLIGIBLE' },
{ 'category' => 'HARM_CATEGORY_DANGEROUS_CONTENT', 'probability' => 'NEGLIGIBLE' }] }],
'usageMetadata' => {
'promptTokenCount' => 2,
'candidatesTokenCount' => 8,
'totalTokenCount' => 10
} }
You can use stream_generate_content
without events:
result = client.stream_generate_content(
{ contents: { role: 'user', parts: { text: 'hi!' } } }
)
In this case, the result will be an array with all the received events:
[{ 'candidates' =>
[{ 'content' => {
'role' => 'model',
'parts' => [{ 'text' => 'Hello! How may I assist you?' }]
},
'finishReason' => 'STOP',
'safetyRatings' =>
[{ 'category' => 'HARM_CATEGORY_HARASSMENT', 'probability' => 'NEGLIGIBLE' },
{ 'category' => 'HARM_CATEGORY_HATE_SPEECH', 'probability' => 'NEGLIGIBLE' },
{ 'category' => 'HARM_CATEGORY_SEXUALLY_EXPLICIT', 'probability' => 'NEGLIGIBLE' },
{ 'category' => 'HARM_CATEGORY_DANGEROUS_CONTENT', 'probability' => 'NEGLIGIBLE' }] }],
'usageMetadata' => {
'promptTokenCount' => 2,
'candidatesTokenCount' => 8,
'totalTokenCount' => 10
} }]
You can mix both as well:
result = client.stream_generate_content(
{ contents: { role: 'user', parts: { text: 'hi!' } } }
) do |event, parsed, raw|
puts event
end
result = client.generate_content(
{ contents: { role: 'user', parts: { text: 'hi!' } } }
)
Result:
{ 'candidates' =>
[{ 'content' => { 'parts' => [{ 'text' => 'Hello! How can I assist you today?' }], 'role' => 'model' },
'finishReason' => 'STOP',
'index' => 0,
'safetyRatings' =>
[{ 'category' => 'HARM_CATEGORY_SEXUALLY_EXPLICIT', 'probability' => 'NEGLIGIBLE' },
{ 'category' => 'HARM_CATEGORY_HATE_SPEECH', 'probability' => 'NEGLIGIBLE' },
{ 'category' => 'HARM_CATEGORY_HARASSMENT', 'probability' => 'NEGLIGIBLE' },
{ 'category' => 'HARM_CATEGORY_DANGEROUS_CONTENT', 'probability' => 'NEGLIGIBLE' }] }],
'promptFeedback' =>
{ 'safetyRatings' =>
[{ 'category' => 'HARM_CATEGORY_SEXUALLY_EXPLICIT', 'probability' => 'NEGLIGIBLE' },
{ 'category' => 'HARM_CATEGORY_HATE_SPEECH', 'probability' => 'NEGLIGIBLE' },
{ 'category' => 'HARM_CATEGORY_HARASSMENT', 'probability' => 'NEGLIGIBLE' },
{ 'category' => 'HARM_CATEGORY_DANGEROUS_CONTENT', 'probability' => 'NEGLIGIBLE' }] } }
As of the writing of this README, only the generative-language-api
service supports the generate_content
method; vertex-ai-api
does not.
Vertex AI API generates embeddings through the predict
method (documentation), and you need a client set up to use an embedding model (e.g. text-embedding-004
):
result = client.predict(
{ instances: [{ content: 'What is life?' }],
parameters: { autoTruncate: true } }
)
Result:
{ 'predictions' =>
[{ 'embeddings' =>
{ 'statistics' => { 'truncated' => false, 'token_count' => 4 },
'values' =>
[-0.006861076690256596,
0.00020840796059928834,
-0.028549950569868088,
# ...
0.0020092015620321035,
0.03279878571629524,
-0.014905261807143688] } }],
'metadata' => { 'billableCharacterCount' => 11 } }
Generative Language API generates embeddings through the embed_content
method (documentation), and you need a client set up to use an embedding model (e.g. text-embedding-004
):
result = client.embed_content(
{ content: { parts: [{ text: 'What is life?' }] } }
)
Result:
{ 'embedding' =>
{ 'values' =>
[-0.0065307906,
-0.0001632607,
-0.028370803,
0.0019950708,
0.032798845,
-0.014878989] } }
result = client.stream_generate_content({
contents: { role: 'user', parts: { text: 'hi!' } }
})
Result:
[{ 'candidates' =>
[{ 'content' => {
'role' => 'model',
'parts' => [{ 'text' => 'Hello! How may I assist you?' }]
},
'finishReason' => 'STOP',
'safetyRatings' =>
[{ 'category' => 'HARM_CATEGORY_HARASSMENT', 'probability' => 'NEGLIGIBLE' },
{ 'category' => 'HARM_CATEGORY_HATE_SPEECH', 'probability' => 'NEGLIGIBLE' },
{ 'category' => 'HARM_CATEGORY_SEXUALLY_EXPLICIT', 'probability' => 'NEGLIGIBLE' },
{ 'category' => 'HARM_CATEGORY_DANGEROUS_CONTENT', 'probability' => 'NEGLIGIBLE' }] }],
'usageMetadata' => {
'promptTokenCount' => 2,
'candidatesTokenCount' => 8,
'totalTokenCount' => 10
} }]
Courtesy of Unsplash
Switch to the gemini-pro-vision
model:
client = Gemini.new(
credentials: { service: 'vertex-ai-api', region: 'us-east4' },
options: { model: 'gemini-pro-vision', server_sent_events: true }
)
Then, encode the image as Base64 and add its MIME type:
require 'base64'
result = client.stream_generate_content(
{ contents: [
{ role: 'user', parts: [
{ text: 'Please describe this image.' },
{ inline_data: {
mime_type: 'image/jpeg',
data: Base64.strict_encode64(File.read('piano.jpg'))
} }
] }
] }
)
The result:
[{ 'candidates' =>
[{ 'content' =>
{ 'role' => 'model',
'parts' =>
[{ 'text' =>
' A black and white image of an old piano. The piano is an upright model, with the keys on the right side of the image. The piano is' }] },
'safetyRatings' =>
[{ 'category' => 'HARM_CATEGORY_HARASSMENT', 'probability' => 'NEGLIGIBLE' },
{ 'category' => 'HARM_CATEGORY_HATE_SPEECH', 'probability' => 'NEGLIGIBLE' },
{ 'category' => 'HARM_CATEGORY_SEXUALLY_EXPLICIT', 'probability' => 'NEGLIGIBLE' },
{ 'category' => 'HARM_CATEGORY_DANGEROUS_CONTENT', 'probability' => 'NEGLIGIBLE' }] }] },
{ 'candidates' =>
[{ 'content' => { 'role' => 'model', 'parts' => [{ 'text' => ' sitting on a tiled floor. There is a small round object on the top of the piano.' }] },
'finishReason' => 'STOP',
'safetyRatings' =>
[{ 'category' => 'HARM_CATEGORY_HARASSMENT', 'probability' => 'NEGLIGIBLE' },
{ 'category' => 'HARM_CATEGORY_HATE_SPEECH', 'probability' => 'NEGLIGIBLE' },
{ 'category' => 'HARM_CATEGORY_SEXUALLY_EXPLICIT', 'probability' => 'NEGLIGIBLE' },
{ 'category' => 'HARM_CATEGORY_DANGEROUS_CONTENT', 'probability' => 'NEGLIGIBLE' }] }],
'usageMetadata' => { 'promptTokenCount' => 263, 'candidatesTokenCount' => 50, 'totalTokenCount' => 313 } }]
https://gist.github.com/assets/29520/f82bccbf-02d2-4899-9c48-eb8a0a5ef741
ALT: A white and gold cup is being filled with coffee. The coffee is dark and rich. The cup is sitting on a black surface. The background is blurred.
Courtesy of Pexels
Switch to the gemini-pro-vision
model:
client = Gemini.new(
credentials: { service: 'vertex-ai-api', region: 'us-east4' },
options: { model: 'gemini-pro-vision', server_sent_events: true }
)
Then, encode the video as Base64 and add its MIME type:
require 'base64'
result = client.stream_generate_content(
{ contents: [
{ role: 'user', parts: [
{ text: 'Please describe this video.' },
{ inline_data: {
mime_type: 'video/mp4',
data: Base64.strict_encode64(File.read('coffee.mp4'))
} }
] }
] }
)
The result:
[{"candidates"=>
[{"content"=>
{"role"=>"model",
"parts"=>
[{"text"=>
" A white and gold cup is being filled with coffee. The coffee is dark and rich. The cup is sitting on a black surface. The background is blurred"}]},
"safetyRatings"=>
[{"category"=>"HARM_CATEGORY_HARASSMENT", "probability"=>"NEGLIGIBLE"},
{"category"=>"HARM_CATEGORY_HATE_SPEECH", "probability"=>"NEGLIGIBLE"},
{"category"=>"HARM_CATEGORY_SEXUALLY_EXPLICIT", "probability"=>"NEGLIGIBLE"},
{"category"=>"HARM_CATEGORY_DANGEROUS_CONTENT", "probability"=>"NEGLIGIBLE"}]}],
"usageMetadata"=>{"promptTokenCount"=>1037, "candidatesTokenCount"=>31, "totalTokenCount"=>1068}},
{"candidates"=>
[{"content"=>{"role"=>"model", "parts"=>[{"text"=>"."}]},
"finishReason"=>"STOP",
"safetyRatings"=>
[{"category"=>"HARM_CATEGORY_HARASSMENT", "probability"=>"NEGLIGIBLE"},
{"category"=>"HARM_CATEGORY_HATE_SPEECH", "probability"=>"NEGLIGIBLE"},
{"category"=>"HARM_CATEGORY_SEXUALLY_EXPLICIT", "probability"=>"NEGLIGIBLE"},
{"category"=>"HARM_CATEGORY_DANGEROUS_CONTENT", "probability"=>"NEGLIGIBLE"}]}],
"usageMetadata"=>{"promptTokenCount"=>1037, "candidatesTokenCount"=>32, "totalTokenCount"=>1069}}]
Server-Sent Events (SSE) is a technology that allows certain endpoints to offer streaming capabilities, such as creating the impression that "the model is typing along with you," rather than delivering the entire answer all at once.
You can set up the client to use Server-Sent Events (SSE) for all supported endpoints:
client = Gemini.new(
credentials: { ... },
options: { model: 'gemini-pro', server_sent_events: true }
)
Or, you can decide on a request basis:
client.stream_generate_content(
{ contents: { role: 'user', parts: { text: 'hi!' } } },
server_sent_events: true
)
With Server-Sent Events (SSE) enabled, you can use a block to receive partial results via events. This feature is particularly useful for methods that offer streaming capabilities, such as stream_generate_content
:
client.stream_generate_content(
{ contents: { role: 'user', parts: { text: 'hi!' } } }
) do |event, parsed, raw|
puts event
end
Event:
{ 'candidates' =>
[{ 'content' => {
'role' => 'model',
'parts' => [{ 'text' => 'Hello! How may I assist you?' }]
},
'finishReason' => 'STOP',
'safetyRatings' =>
[{ 'category' => 'HARM_CATEGORY_HARASSMENT', 'probability' => 'NEGLIGIBLE' },
{ 'category' => 'HARM_CATEGORY_HATE_SPEECH', 'probability' => 'NEGLIGIBLE' },
{ 'category' => 'HARM_CATEGORY_SEXUALLY_EXPLICIT', 'probability' => 'NEGLIGIBLE' },
{ 'category' => 'HARM_CATEGORY_DANGEROUS_CONTENT', 'probability' => 'NEGLIGIBLE' }] }],
'usageMetadata' => {
'promptTokenCount' => 2,
'candidatesTokenCount' => 8,
'totalTokenCount' => 10
} }
Even though streaming methods utilize Server-Sent Events (SSE), using this feature doesn't necessarily mean streaming data. For example, when generate_content
is called with SSE enabled, you will receive all the data at once in a single event, rather than through multiple partial events. This occurs because generate_content
isn't designed for streaming, even though it is capable of utilizing Server-Sent Events.
Method calls will hang until the server-sent events finish, so even without providing a block, you can obtain the final results of the received events:
result = client.stream_generate_content(
{ contents: { role: 'user', parts: { text: 'hi!' } } },
server_sent_events: true
)
Result:
[{ 'candidates' =>
[{ 'content' => {
'role' => 'model',
'parts' => [{ 'text' => 'Hello! How may I assist you?' }]
},
'finishReason' => 'STOP',
'safetyRatings' =>
[{ 'category' => 'HARM_CATEGORY_HARASSMENT', 'probability' => 'NEGLIGIBLE' },
{ 'category' => 'HARM_CATEGORY_HATE_SPEECH', 'probability' => 'NEGLIGIBLE' },
{ 'category' => 'HARM_CATEGORY_SEXUALLY_EXPLICIT', 'probability' => 'NEGLIGIBLE' },
{ 'category' => 'HARM_CATEGORY_DANGEROUS_CONTENT', 'probability' => 'NEGLIGIBLE' }] }],
'usageMetadata' => {
'promptTokenCount' => 2,
'candidatesTokenCount' => 8,
'totalTokenCount' => 10
} }]
Depending on the service, you can use the generate_content
method, which does not stream the answer.
You can also use methods designed for streaming without necessarily processing partial events; instead, you can wait for the result of all received events:
result = client.stream_generate_content({
contents: { role: 'user', parts: { text: 'hi!' } },
server_sent_events: false
})
Result:
[{ 'candidates' =>
[{ 'content' => {
'role' => 'model',
'parts' => [{ 'text' => 'Hello! How may I assist you?' }]
},
'finishReason' => 'STOP',
'safetyRatings' =>
[{ 'category' => 'HARM_CATEGORY_HARASSMENT', 'probability' => 'NEGLIGIBLE' },
{ 'category' => 'HARM_CATEGORY_HATE_SPEECH', 'probability' => 'NEGLIGIBLE' },
{ 'category' => 'HARM_CATEGORY_SEXUALLY_EXPLICIT', 'probability' => 'NEGLIGIBLE' },
{ 'category' => 'HARM_CATEGORY_DANGEROUS_CONTENT', 'probability' => 'NEGLIGIBLE' }] }],
'usageMetadata' => {
'promptTokenCount' => 2,
'candidatesTokenCount' => 8,
'totalTokenCount' => 10
} }]
To maintain a back-and-forth conversation, you need to append the received responses and build a history for your requests:
result = client.stream_generate_content(
{ contents: [
{ role: 'user', parts: { text: 'Hi! My name is Purple.' } },
{ role: 'model', parts: { text: "Hello Purple! It's nice to meet you." } },
{ role: 'user', parts: { text: "What's my name?" } }
] }
)
Result:
[{ 'candidates' =>
[{ 'content' =>
{ 'role' => 'model',
'parts' => [
{ 'text' => "Purple.\n\nYou told me your name was Purple in your first message to me.\n\nIs there anything" }
] },
'safetyRatings' =>
[{ 'category' => 'HARM_CATEGORY_HARASSMENT', 'probability' => 'NEGLIGIBLE' },
{ 'category' => 'HARM_CATEGORY_HATE_SPEECH', 'probability' => 'NEGLIGIBLE' },
{ 'category' => 'HARM_CATEGORY_SEXUALLY_EXPLICIT', 'probability' => 'NEGLIGIBLE' },
{ 'category' => 'HARM_CATEGORY_DANGEROUS_CONTENT', 'probability' => 'NEGLIGIBLE' }] }] },
{ 'candidates' =>
[{ 'content' => { 'role' => 'model', 'parts' => [{ 'text' => ' else I can help you with today, Purple?' }] },
'finishReason' => 'STOP',
'safetyRatings' =>
[{ 'category' => 'HARM_CATEGORY_HARASSMENT', 'probability' => 'NEGLIGIBLE' },
{ 'category' => 'HARM_CATEGORY_HATE_SPEECH', 'probability' => 'NEGLIGIBLE' },
{ 'category' => 'HARM_CATEGORY_SEXUALLY_EXPLICIT', 'probability' => 'NEGLIGIBLE' },
{ 'category' => 'HARM_CATEGORY_DANGEROUS_CONTENT', 'probability' => 'NEGLIGIBLE' }] }],
'usageMetadata' => {
'promptTokenCount' => 24,
'candidatesTokenCount' => 31,
'totalTokenCount' => 55
} }]
You can configure safety attributes for your requests.
Harm Categories:
HARM_CATEGORY_UNSPECIFIED
,HARM_CATEGORY_HARASSMENT
,HARM_CATEGORY_HATE_SPEECH
,HARM_CATEGORY_SEXUALLY_EXPLICIT
,HARM_CATEGORY_DANGEROUS_CONTENT
.
Thresholds:
BLOCK_NONE
,BLOCK_ONLY_HIGH
,BLOCK_MEDIUM_AND_ABOVE
,BLOCK_LOW_AND_ABOVE
,HARM_BLOCK_THRESHOLD_UNSPECIFIED
.
Example:
client.stream_generate_content(
{
contents: { role: 'user', parts: { text: 'hi!' } },
safetySettings: [
{
category: 'HARM_CATEGORY_UNSPECIFIED',
threshold: 'BLOCK_ONLY_HIGH'
},
{
category: 'HARM_CATEGORY_HARASSMENT',
threshold: 'BLOCK_ONLY_HIGH'
},
{
category: 'HARM_CATEGORY_HATE_SPEECH',
threshold: 'BLOCK_ONLY_HIGH'
},
{
category: 'HARM_CATEGORY_SEXUALLY_EXPLICIT',
threshold: 'BLOCK_ONLY_HIGH'
},
{
category: 'HARM_CATEGORY_DANGEROUS_CONTENT',
threshold: 'BLOCK_ONLY_HIGH'
}
]
}
)
Google started to block the usage of BLOCK_NONE
unless:
User has requested a restricted HarmBlockThreshold setting BLOCK_NONE. You can get access either (a) through an allowlist via your Google account team, or (b) by switching your account type to monthly invoiced billing via this instruction: https://cloud.google.com/billing/docs/how-to/invoiced-billing
Some models support system instructions:
client.stream_generate_content(
{ contents: { role: 'user', parts: { text: 'Hi! Who are you?' } },
system_instruction: { role: 'user', parts: { text: 'Your name is Neko.' } } }
)
Output:
Hi! I'm Neko, a factual language model from Google AI.
client.stream_generate_content(
{ contents: { role: 'user', parts: { text: 'Hi! Who are you?' } },
system_instruction: {
role: 'user', parts: [
{ text: 'You are a cat.' },
{ text: 'Your name is Neko.' }
]
} }
)
Output:
Meow! I'm Neko, a fluffy and playful cat. :3
You can count tokens and preview how many tokens a request is expected to consume:
client.count_tokens(
{ contents: { role: 'user', parts: { text: 'hi!' } } }
)
Output for Generative Language API:
{ 'totalTokens' => 3 }
Output for Vertex AI API:
{ 'totalTokens' => 2, 'totalBillableCharacters' => 3 }
As of the writing of this README, only the
vertex-ai-api
service andgemini
models version1.5
support this feature.
The Gemini API provides a configuration parameter to request a response in JSON format:
require 'json'
result = client.stream_generate_content(
{
contents: {
role: 'user',
parts: {
text: 'List 3 random colors.'
}
},
generation_config: {
response_mime_type: 'application/json'
}
}
)
json_string = result
.map { |response| response.dig('candidates', 0, 'content', 'parts') }
.map { |parts| parts.map { |part| part['text'] }.join }
.join
puts JSON.parse(json_string).inspect
Output:
{ 'colors' => ['Dark Salmon', 'Indigo', 'Lavender'] }
While Gemini 1.5 Flash models only accept a text description of the JSON schema you want returned, the Gemini 1.5 Pro models let you pass a schema object (or a Python type equivalent), and the model output will strictly follow that schema. This is also known as controlled generation or constrained decoding.
You can also provide a JSON Schema for the expected JSON output:
require 'json'
result = client.stream_generate_content(
{
contents: {
role: 'user',
parts: {
text: 'List 3 random colors.'
}
},
generation_config: {
response_mime_type: 'application/json',
response_schema: {
type: 'object',
properties: {
colors: {
type: 'array',
items: {
type: 'object',
properties: {
name: {
type: 'string'
}
}
}
}
}
}
}
}
)
json_string = result
.map { |response| response.dig('candidates', 0, 'content', 'parts') }
.map { |parts| parts.map { |part| part['text'] }.join }
.join
puts JSON.parse(json_string).inspect
Output:
{ 'colors' => [
{ 'name' => 'Lavender Blush' },
{ 'name' => 'Medium Turquoise' },
{ 'name' => 'Dark Slate Gray' }
] }
These models are accessible to the repository author as of June 2025 in the us-east4
region. Access to models may vary by region, user, and account.
- β Does not support JSON mode.
- π‘ Supports JSON mode but not Schema.
- β Supports JSON mode and Schema.
- π I don't have access to the model.
Model | Vertex AI | Generative Language |
---|---|---|
gemini-pro-vision | β | π |
gemini-pro | π‘ | β |
gemini-1.5-pro-preview-0514 | β | π |
gemini-1.5-pro-preview-0409 | β | π |
gemini-1.5-pro | β | β |
gemini-1.5-flash-preview-0514 | π‘ | π |
gemini-1.5-flash | π‘ | β |
gemini-1.0-pro-vision-latest | π | π |
gemini-1.0-pro-vision-001 | β | π |
gemini-1.0-pro-vision | β | π |
gemini-1.0-pro-latest | π | β |
gemini-1.0-pro-002 | π‘ | π |
gemini-1.0-pro-001 | β | β |
gemini-1.0-pro | π‘ | β |
gemini-ultra | π | π |
gemini-1.0-ultra | π | π |
gemini-1.0-ultra-001 | π | π |
As of the writing of this README, only the
vertex-ai-api
service and thegemini-pro
model supports tools (functions) calls.
You can provide specifications for tools (functions) using JSON Schema to generate potential calls to them:
input = {
tools: {
function_declarations: [
{
name: 'date_and_time',
description: 'Returns the current date and time in the ISO 8601 format for a given timezone.',
parameters: {
type: 'object',
properties: {
timezone: {
type: 'string',
description: 'A string represents the timezone to be used for providing a datetime, following the IANA (Internet Assigned Numbers Authority) Time Zone Database. Examples include "Asia/Tokyo" and "Europe/Paris". If not provided, the default timezone is the user\'s current timezone.'
}
}
}
}
]
},
contents: [
{ role: 'user', parts: { text: 'What time is it?' } }
]
}
result = client.stream_generate_content(input)
Which may return a request to perform a call:
[{ 'candidates' =>
[{ 'content' => {
'role' => 'model',
'parts' => [{ 'functionCall' => {
'name' => 'date_and_time',
'args' => { 'timezone' => 'local' }
} }]
},
'finishReason' => 'STOP',
'safetyRatings' =>
[{ 'category' => 'HARM_CATEGORY_HARASSMENT', 'probability' => 'NEGLIGIBLE' },
{ 'category' => 'HARM_CATEGORY_HATE_SPEECH', 'probability' => 'NEGLIGIBLE' },
{ 'category' => 'HARM_CATEGORY_SEXUALLY_EXPLICIT', 'probability' => 'NEGLIGIBLE' },
{ 'category' => 'HARM_CATEGORY_DANGEROUS_CONTENT', 'probability' => 'NEGLIGIBLE' }] }],
'usageMetadata' => { 'promptTokenCount' => 5, 'totalTokenCount' => 5 } }]
Based on these results, you can perform the requested calls and provide their outputs:
gem 'tzinfo', '~> 2.0', '>= 2.0.6'
require 'tzinfo'
require 'time'
function_calls = result.dig(0, 'candidates', 0, 'content', 'parts').filter do |part|
part.key?('functionCall')
end
function_parts = []
function_calls.each do |function_call|
next unless function_call['functionCall']['name'] == 'date_and_time'
timezone = function_call.dig('functionCall', 'args', 'timezone')
time = if !timezone.nil? && timezone != '' && timezone.downcase != 'local'
TZInfo::Timezone.get(timezone).now
else
Time.now
end
function_output = time.iso8601
function_parts << {
functionResponse: {
name: function_call['functionCall']['name'],
response: {
name: function_call['functionCall']['name'],
content: function_output
}
}
}
end
input[:contents] << result.dig(0, 'candidates', 0, 'content')
input[:contents] << { role: 'function', parts: function_parts }
This will be equivalent to the following final input:
{ tools: { function_declarations: [
{ name: 'date_and_time',
description: 'Returns the current date and time in the ISO 8601 format for a given timezone.',
parameters: {
type: 'object',
properties: {
timezone: {
type: 'string',
description: "A string represents the timezone to be used for providing a datetime, following the IANA (Internet Assigned Numbers Authority) Time Zone Database. Examples include \"Asia/Tokyo\" and \"Europe/Paris\". If not provided, the default timezone is the user's current timezone."
}
}
} }
] },
contents: [
{ role: 'user', parts: { text: 'What time is it?' } },
{ role: 'model',
parts: [
{ functionCall: { name: 'date_and_time', args: { timezone: 'local' } } }
] },
{ role: 'function',
parts: [{ functionResponse: {
name: 'date_and_time',
response: {
name: 'date_and_time',
content: '2023-12-13T21:15:11-03:00'
}
} }] }
] }
With the input properly arranged, you can make another request:
result = client.stream_generate_content(input)
Which will result in:
[{ 'candidates' =>
[{ 'content' => { 'role' => 'model', 'parts' => [{ 'text' => 'It is 21:15.' }] },
'finishReason' => 'STOP',
'safetyRatings' =>
[{ 'category' => 'HARM_CATEGORY_HARASSMENT', 'probability' => 'NEGLIGIBLE' },
{ 'category' => 'HARM_CATEGORY_HATE_SPEECH', 'probability' => 'NEGLIGIBLE' },
{ 'category' => 'HARM_CATEGORY_SEXUALLY_EXPLICIT', 'probability' => 'NEGLIGIBLE' },
{ 'category' => 'HARM_CATEGORY_DANGEROUS_CONTENT', 'probability' => 'NEGLIGIBLE' }] }],
'usageMetadata' => { 'promptTokenCount' => 5, 'candidatesTokenCount' => 9, 'totalTokenCount' => 14 } }]
Google may launch a new endpoint that we haven't covered in the Gem yet. If that's the case, you may still be able to use it through the request
method. For example, stream_generate_content
is just a wrapper for models/gemini-pro:streamGenerateContent
(Generative Language API) or publishers/google/models/gemini-pro:streamGenerateContent
(Vertex AI API), which you can call directly like this:
# Generative Language API
result = client.request(
'models/gemini-pro:streamGenerateContent',
{ contents: { role: 'user', parts: { text: 'hi!' } } },
request_method: 'POST',
server_sent_events: true
)
# Vertex AI API
result = client.request(
'publishers/google/models/gemini-pro:streamGenerateContent',
{ contents: { role: 'user', parts: { text: 'hi!' } } },
request_method: 'POST',
server_sent_events: true
)
To enable streaming, the gem uses Faraday with the Typhoeus adapter by default.
You can use a different adapter if you want:
require 'faraday/net_http'
client = Gemini.new(
credentials: { service: 'vertex-ai-api', region: 'us-east4' },
options: {
model: 'gemini-pro',
connection: { adapter: :net_http }
}
)
You can set the maximum number of seconds to wait for the request to complete with the timeout
option:
client = Gemini.new(
credentials: { service: 'vertex-ai-api', region: 'us-east4' },
options: {
model: 'gemini-pro',
connection: { request: { timeout: 5 } }
}
)
You can also have more fine-grained control over Faraday's Request Options if you prefer:
client = Gemini.new(
credentials: { service: 'vertex-ai-api', region: 'us-east4' },
options: {
model: 'gemini-pro',
connection: {
request: {
timeout: 5,
open_timeout: 5,
read_timeout: 5,
write_timeout: 5
}
}
}
)
require 'gemini-ai'
begin
client.stream_generate_content({
contents: { role: 'user', parts: { text: 'hi!' } }
})
rescue Gemini::Errors::GeminiError => error
puts error.class # Gemini::Errors::RequestError
puts error.message # 'the server responded with status 500'
puts error.payload
# { contents: [{ role: 'user', parts: { text: 'hi!' } }],
# generationConfig: { candidateCount: 1 },
# ...
# }
puts error.request
# #<Faraday::ServerError response={:status=>500, :headers...
end
require 'gemini-ai/errors'
begin
client.stream_generate_content({
contents: { role: 'user', parts: { text: 'hi!' } }
})
rescue GeminiError => error
puts error.class # Gemini::Errors::RequestError
end
GeminiError
MissingProjectIdError
UnsupportedServiceError
ConflictingCredentialsError
BlockWithoutServerSentEventsError
RequestError
bundle
rubocop -A
rspec
bundle exec ruby spec/tasks/run-available-models.rb
bundle exec ruby spec/tasks/run-embed.rb
bundle exec ruby spec/tasks/run-generate.rb
bundle exec ruby spec/tasks/run-json.rb
bundle exec ruby spec/tasks/run-safety.rb
bundle exec ruby spec/tasks/run-system.rb
This Gem is designed to provide low-level access to Gemini, enabling people to build abstractions on top of it. If you are interested in more high-level abstractions or more user-friendly tools, you may want to consider Nano Bots π π€.
gem build gemini-ai.gemspec
gem signin
gem push gemini-ai-4.2.0.gem
Install Babashka:
curl -s https://raw.githubusercontent.com/babashka/babashka/master/install | sudo bash
Update the template.md
file and then:
bb tasks/generate-readme.clj
Trick for automatically updating the README.md
when template.md
changes:
sudo pacman -S inotify-tools # Arch / Manjaro
sudo apt-get install inotify-tools # Debian / Ubuntu / Raspberry Pi OS
sudo dnf install inotify-tools # Fedora / CentOS / RHEL
while inotifywait -e modify template.md; do bb tasks/generate-readme.clj; done
Trick for Markdown Live Preview:
pip install -U markdown_live_preview
mlp README.md -p 8076
These resources and references may be useful throughout your learning process.
- Google AI for Developers
- Get started with the Gemini API
- Getting Started with the Vertex AI Gemini API with cURL
- Gemini API Documentation
- Vertex AI API Documentation
- Google models
- Google DeepMind Gemini
- Stream responses from Generative AI models
- Function calling
This is not an official Google project, nor is it affiliated with Google in any way.
This software is distributed under the MIT License. This license includes a disclaimer of warranty. Moreover, the authors assume no responsibility for any damage or costs that may result from using this project. Use the Gemini AI Ruby Gem at your own risk.
For Tasks:
Click tags to check more tools for each tasksFor Jobs:
Alternative AI tools for gemini-ai
Similar Open Source Tools
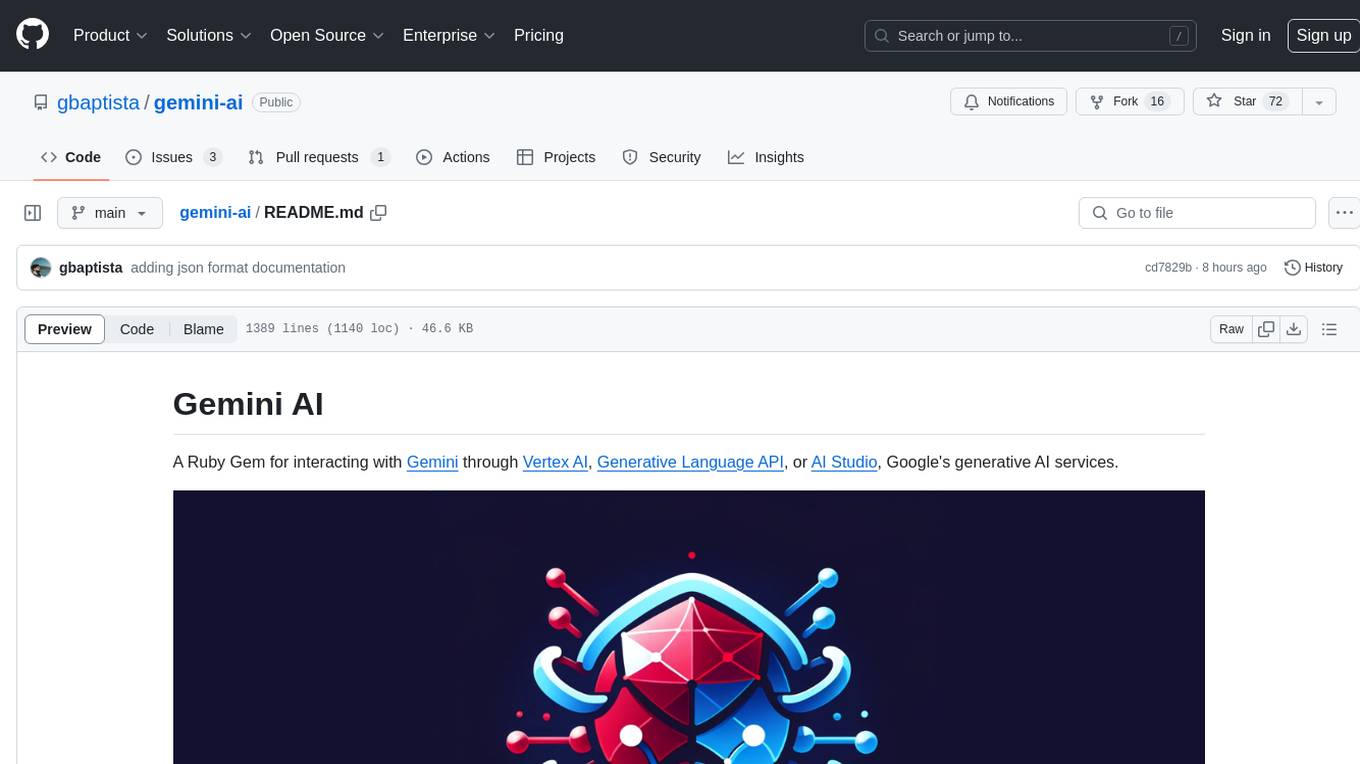
gemini-ai
Gemini AI is a Ruby Gem designed to provide low-level access to Google's generative AI services through Vertex AI, Generative Language API, or AI Studio. It allows users to interact with Gemini to build abstractions on top of it. The Gem provides functionalities for tasks such as generating content, embeddings, predictions, and more. It supports streaming capabilities, server-sent events, safety settings, system instructions, JSON format responses, and tools (functions) calling. The Gem also includes error handling, development setup, publishing to RubyGems, updating the README, and references to resources for further learning.
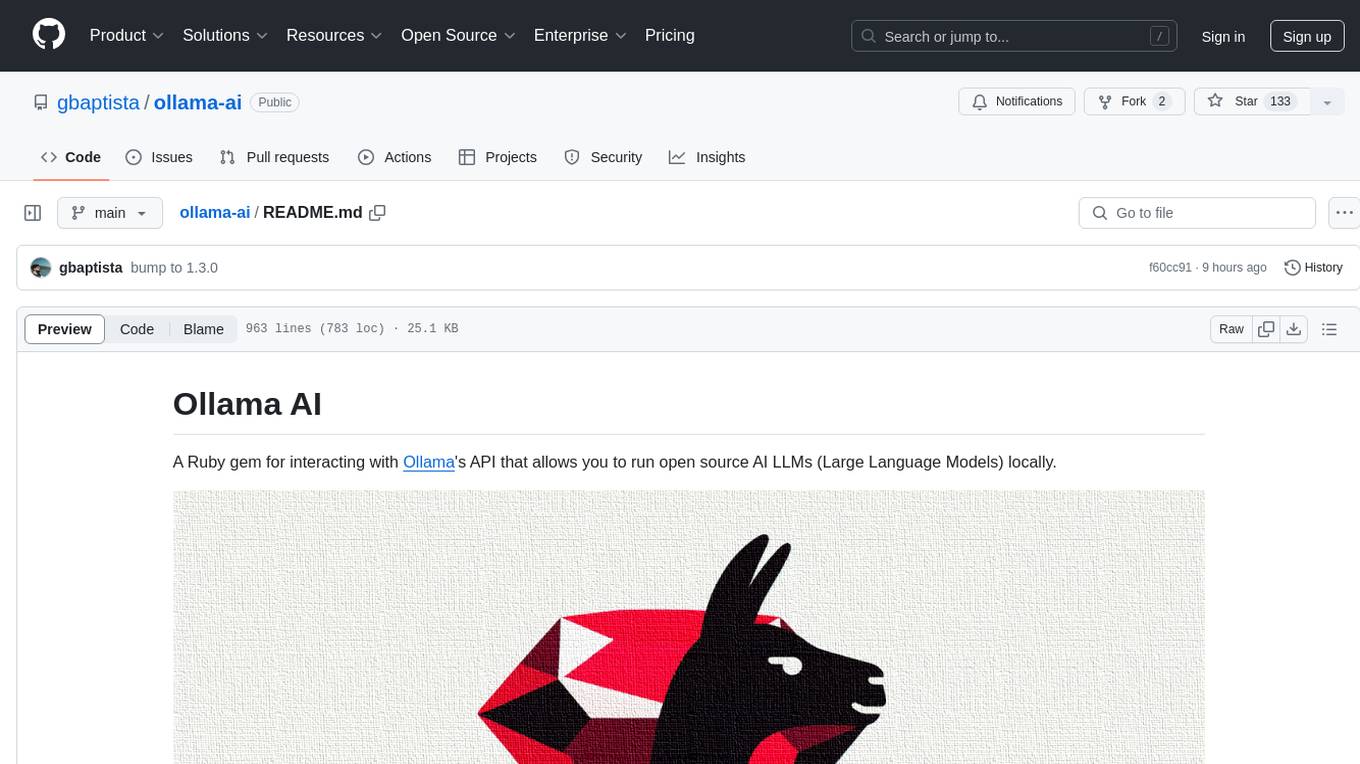
ollama-ai
Ollama AI is a Ruby gem designed to interact with Ollama's API, allowing users to run open source AI LLMs (Large Language Models) locally. The gem provides low-level access to Ollama, enabling users to build abstractions on top of it. It offers methods for generating completions, chat interactions, embeddings, creating and managing models, and more. Users can also work with text and image data, utilize Server-Sent Events for streaming capabilities, and handle errors effectively. Ollama AI is not an official Ollama project and is distributed under the MIT License.
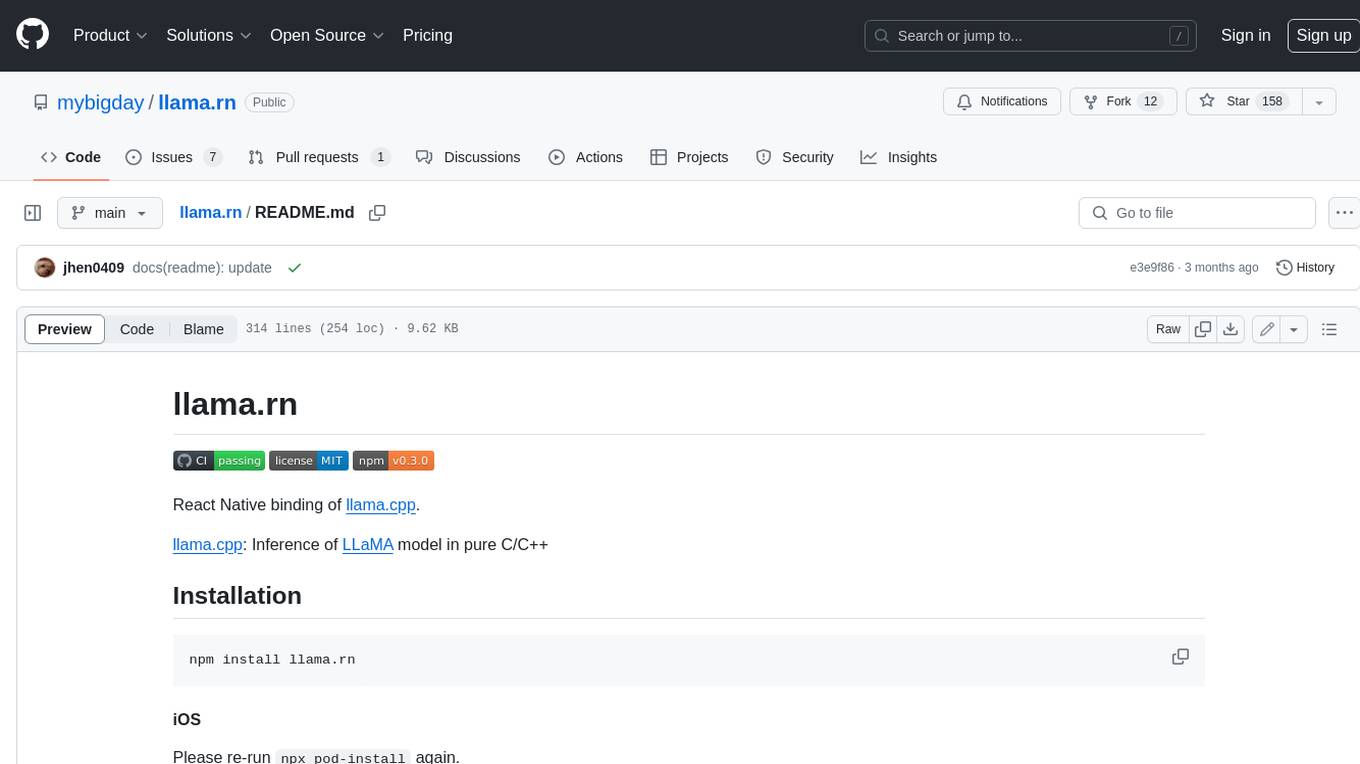
llama.rn
React Native binding of llama.cpp, which is an inference of LLaMA model in pure C/C++. This tool allows you to use the LLaMA model in your React Native applications for various tasks such as text completion, tokenization, detokenization, and embedding. It provides a convenient interface to interact with the LLaMA model and supports features like grammar sampling and mocking for testing purposes.
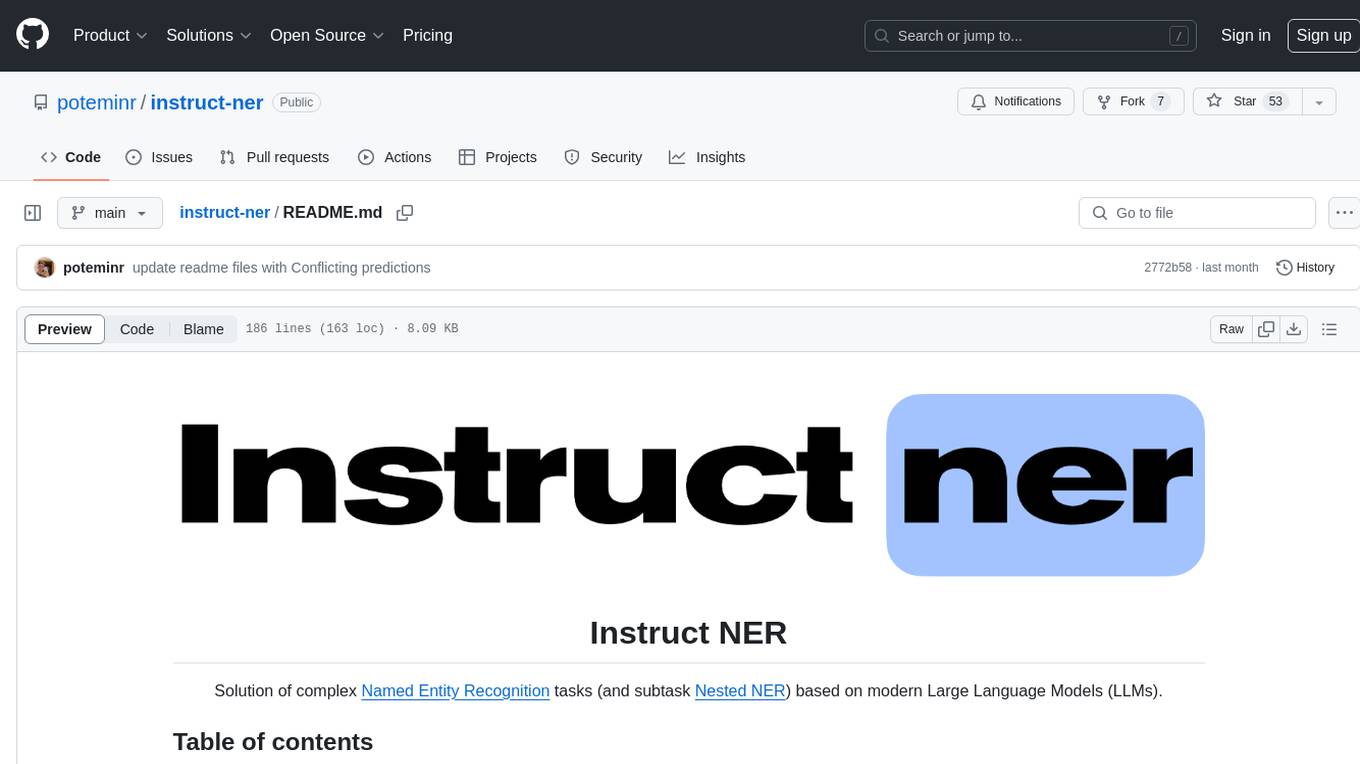
instruct-ner
Instruct NER is a solution for complex Named Entity Recognition tasks, including Nested NER, based on modern Large Language Models (LLMs). It provides tools for dataset creation, training, automatic metric calculation, inference, error analysis, and model implementation. Users can create instructions for LLM, build dictionaries with labels, and generate model input templates. The tool supports various entity types and datasets, such as RuDReC, NEREL-BIO, CoNLL-2003, and MultiCoNER II. It offers training scripts for LLMs and metric calculation functions. Instruct NER models like Llama, Mistral, T5, and RWKV are implemented, with HuggingFace models available for adaptation and merging.
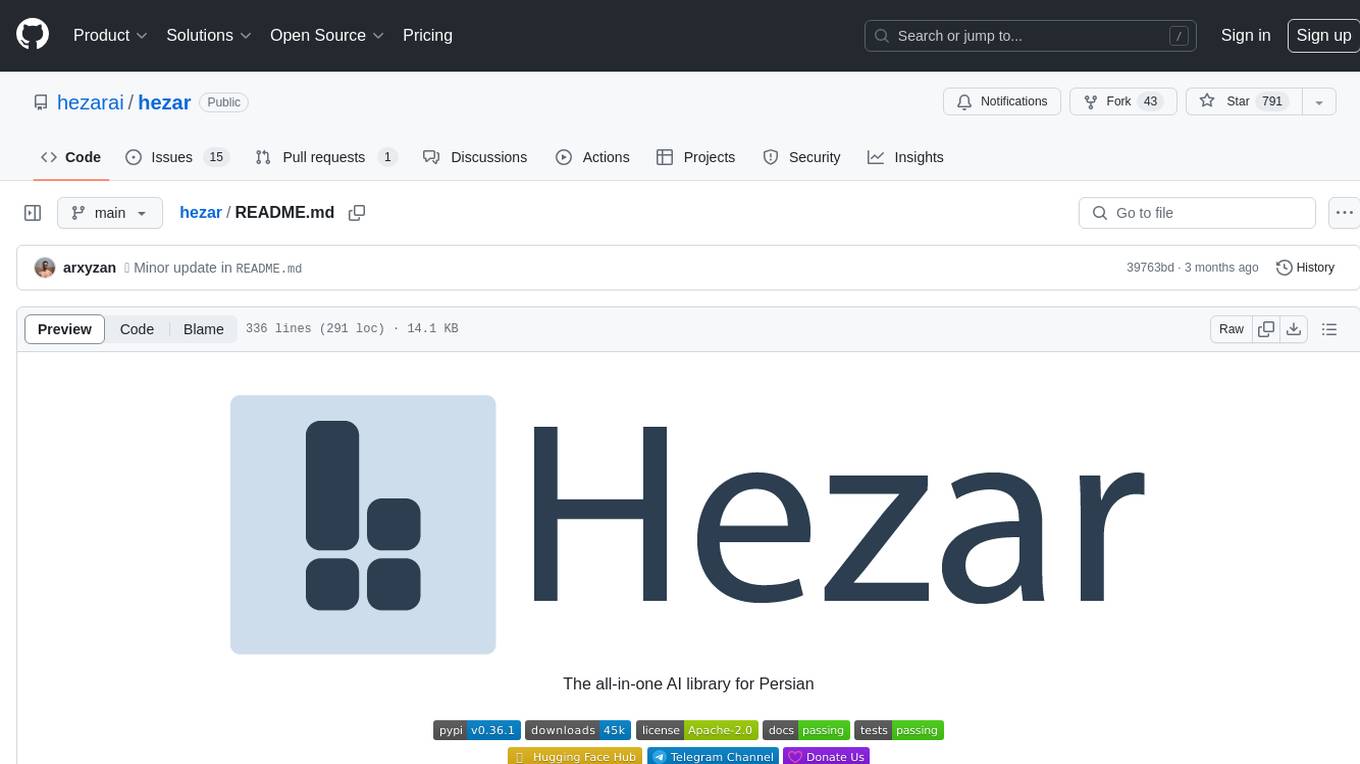
hezar
Hezar is an all-in-one AI library designed specifically for the Persian community. It brings together various AI models and tools, making it easy to use AI with just a few lines of code. The library seamlessly integrates with Hugging Face Hub, offering a developer-friendly interface and task-based model interface. In addition to models, Hezar provides tools like word embeddings, tokenizers, feature extractors, and more. It also includes supplementary ML tools for deployment, benchmarking, and optimization.
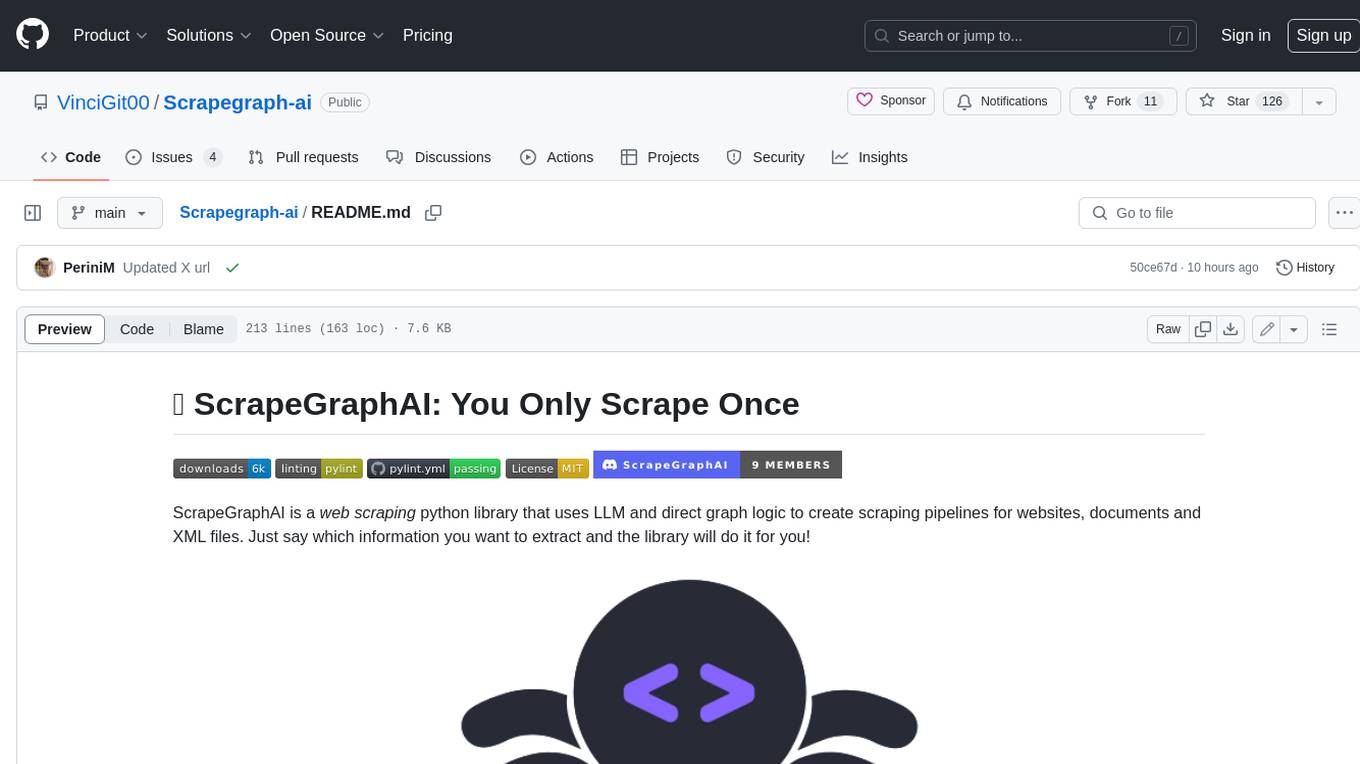
Scrapegraph-ai
ScrapeGraphAI is a Python library that uses Large Language Models (LLMs) and direct graph logic to create web scraping pipelines for websites, documents, and XML files. It allows users to extract specific information from web pages by providing a prompt describing the desired data. ScrapeGraphAI supports various LLMs, including Ollama, OpenAI, Gemini, and Docker, enabling users to choose the most suitable model for their needs. The library provides a user-friendly interface through its `SmartScraper` class, which simplifies the process of building and executing scraping pipelines. ScrapeGraphAI is open-source and available on GitHub, with extensive documentation and examples to guide users. It is particularly useful for researchers and data scientists who need to extract structured data from web pages for analysis and exploration.
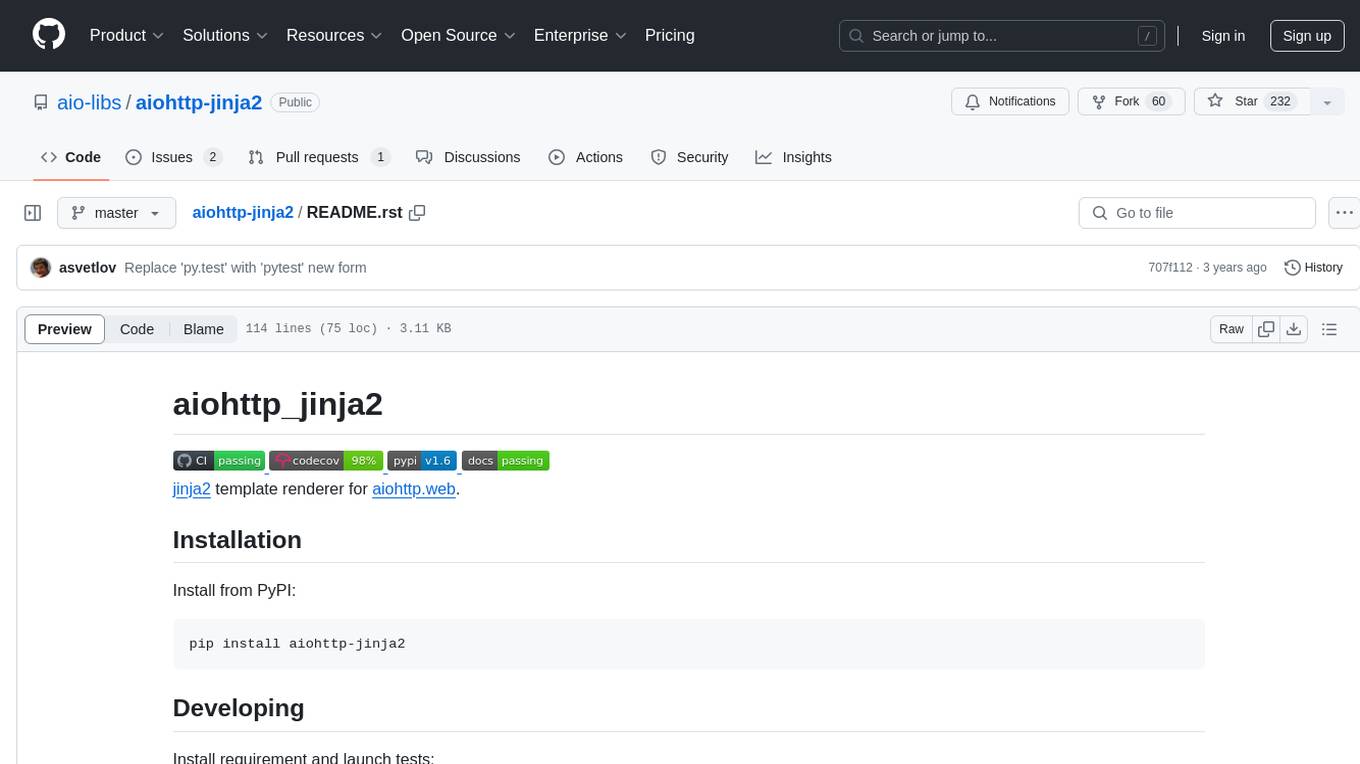
aiohttp-jinja2
aiohttp_jinja2 is a Jinja2 template renderer for aiohttp.web, allowing users to render templates in web applications built with aiohttp. It provides a convenient way to set up Jinja2 environment, use template engine in web handlers, and perform complex processing like setting response headers. The tool simplifies the process of rendering HTML text based on templates and passing context data to templates for dynamic content generation.
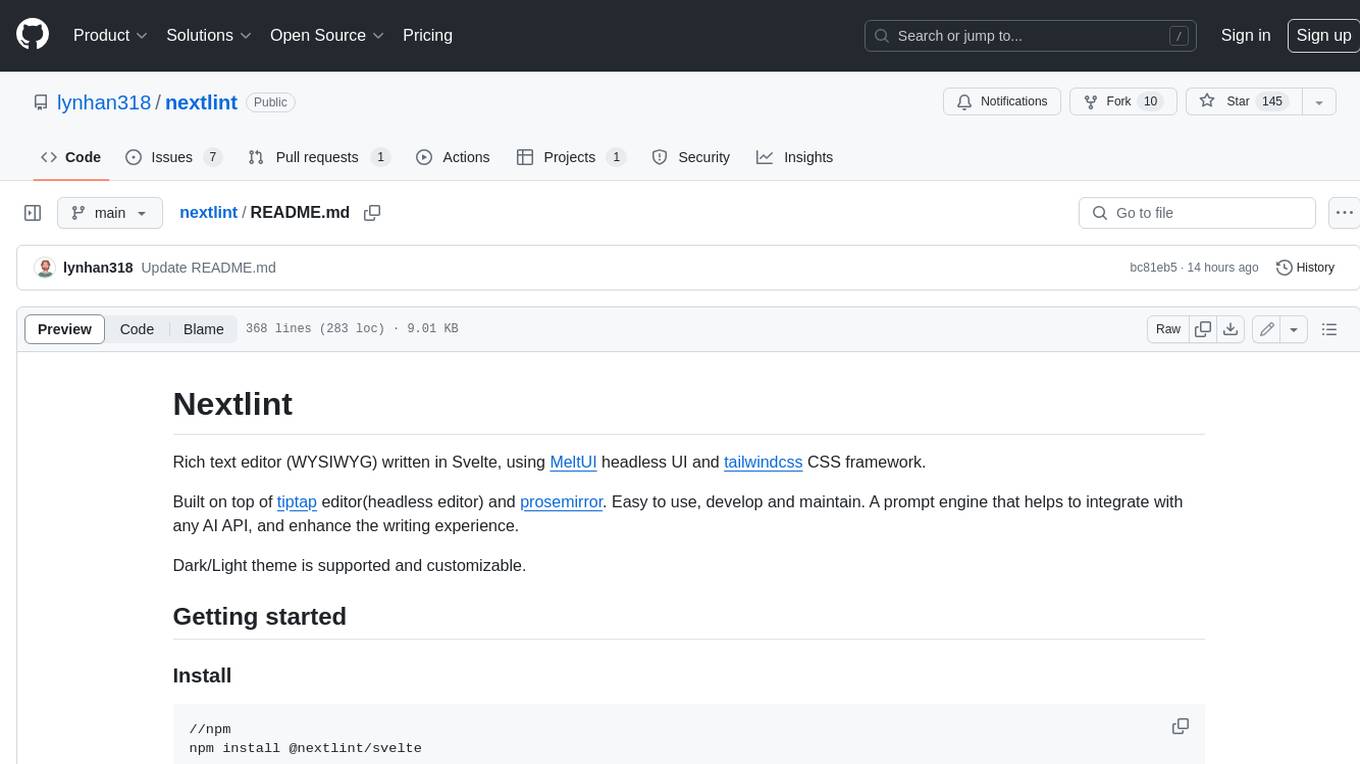
nextlint
Nextlint is a rich text editor (WYSIWYG) written in Svelte, using MeltUI headless UI and tailwindcss CSS framework. It is built on top of tiptap editor (headless editor) and prosemirror. Nextlint is easy to use, develop, and maintain. It has a prompt engine that helps to integrate with any AI API and enhance the writing experience. Dark/Light theme is supported and customizable.
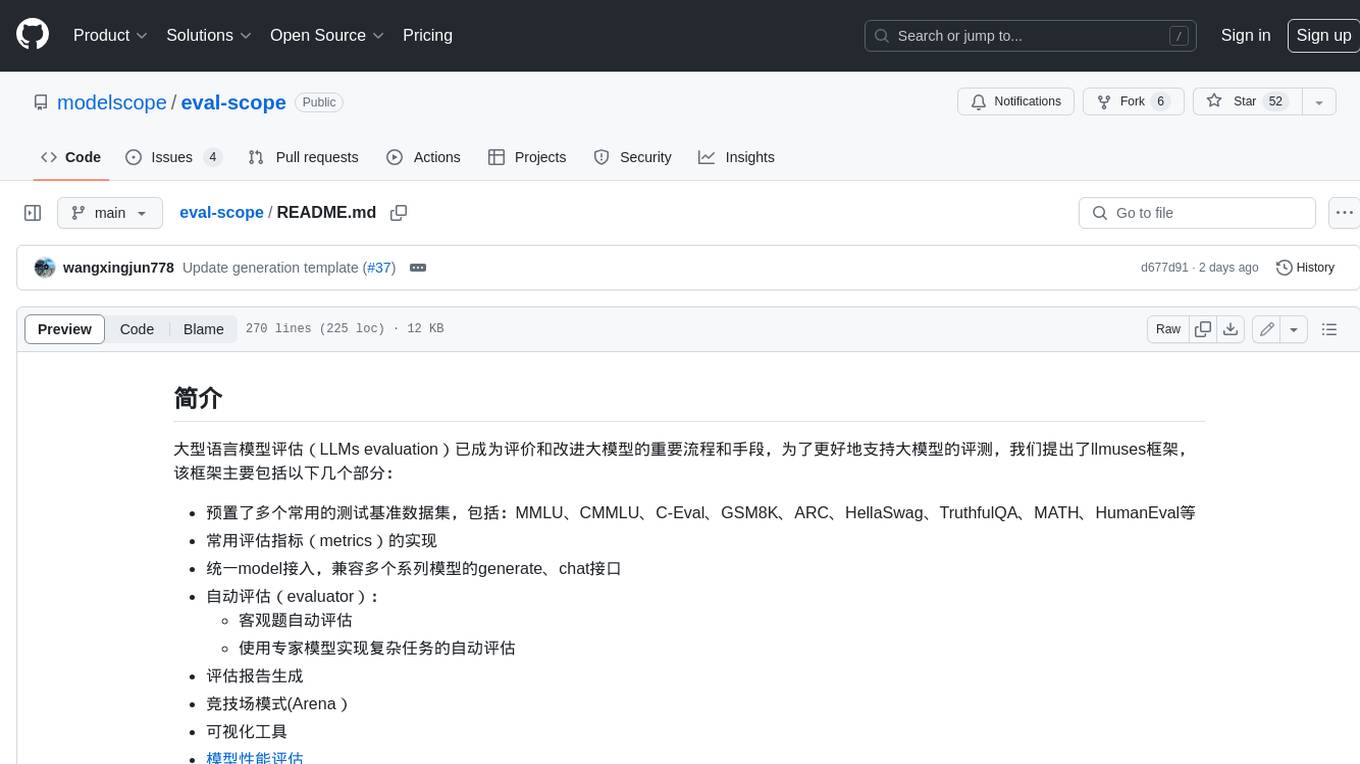
eval-scope
Eval-Scope is a framework for evaluating and improving large language models (LLMs). It provides a set of commonly used test datasets, metrics, and a unified model interface for generating and evaluating LLM responses. Eval-Scope also includes an automatic evaluator that can score objective questions and use expert models to evaluate complex tasks. Additionally, it offers a visual report generator, an arena mode for comparing multiple models, and a variety of other features to support LLM evaluation and development.
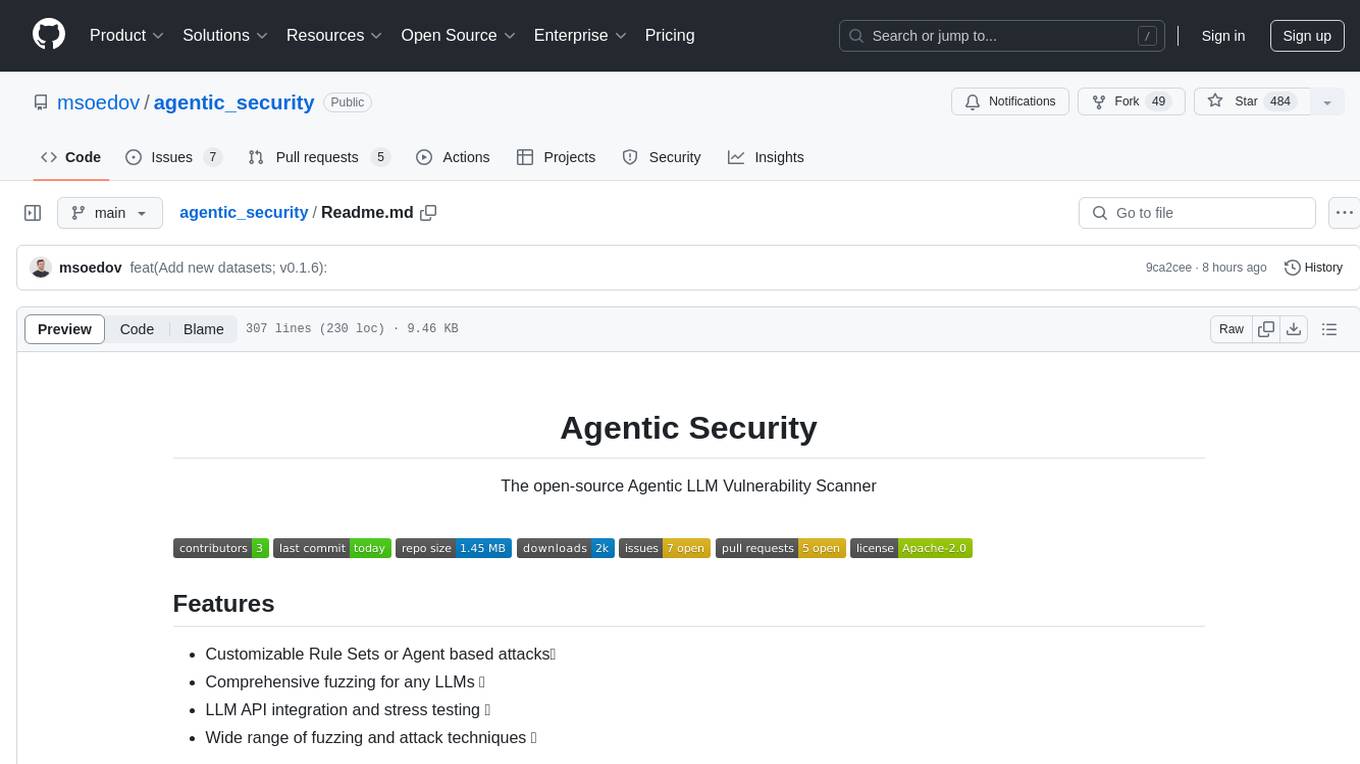
agentic_security
Agentic Security is an open-source vulnerability scanner designed for safety scanning, offering customizable rule sets and agent-based attacks. It provides comprehensive fuzzing for any LLMs, LLM API integration, and stress testing with a wide range of fuzzing and attack techniques. The tool is not a foolproof solution but aims to enhance security measures against potential threats. It offers installation via pip and supports quick start commands for easy setup. Users can utilize the tool for LLM integration, adding custom datasets, running CI checks, extending dataset collections, and dynamic datasets with mutations. The tool also includes a probe endpoint for integration testing. The roadmap includes expanding dataset variety, introducing new attack vectors, developing an attacker LLM, and integrating OWASP Top 10 classification.
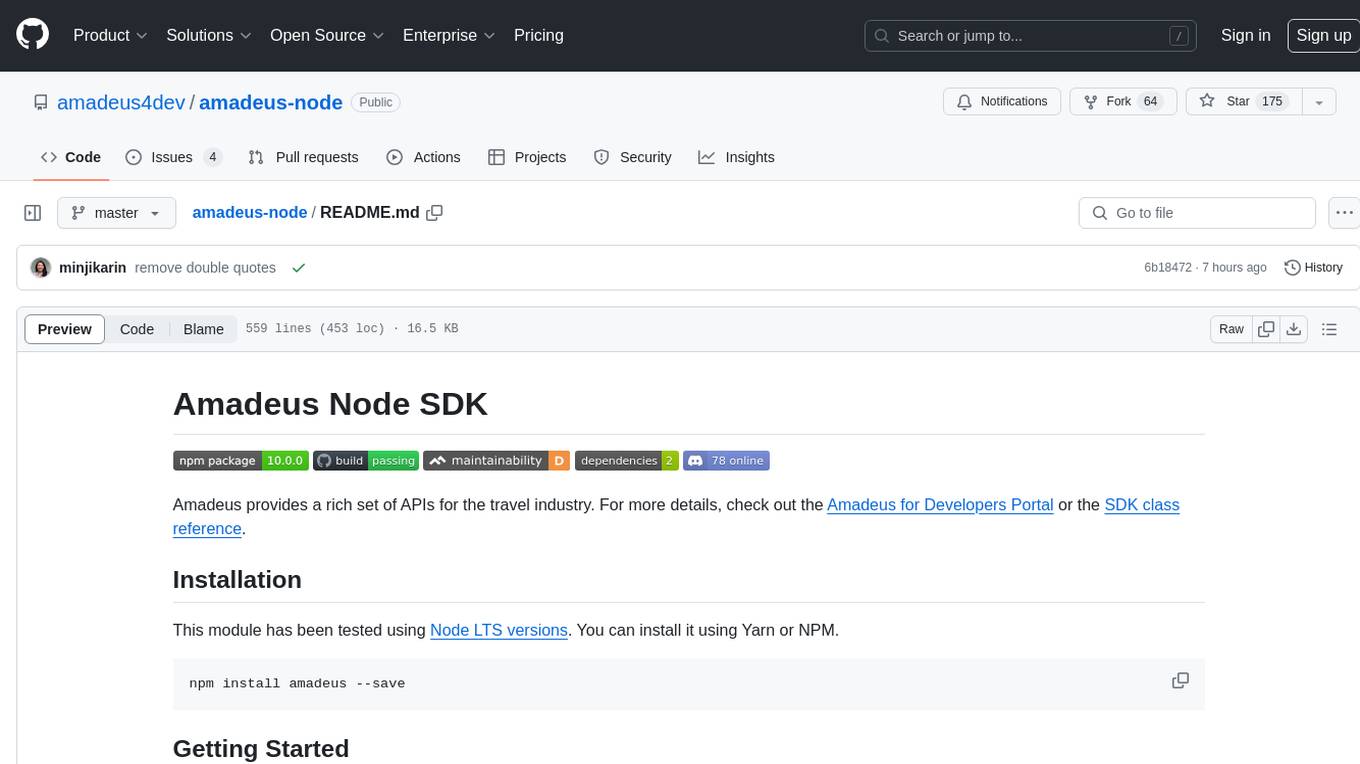
amadeus-node
Amadeus Node SDK provides a rich set of APIs for the travel industry. It allows developers to interact with various endpoints related to flights, hotels, activities, and more. The SDK simplifies making API calls, handling promises, pagination, logging, and debugging. It supports a wide range of functionalities such as flight search, booking, seat maps, flight status, points of interest, hotel search, sentiment analysis, trip predictions, and more. Developers can easily integrate the SDK into their Node.js applications to access Amadeus APIs and build travel-related applications.
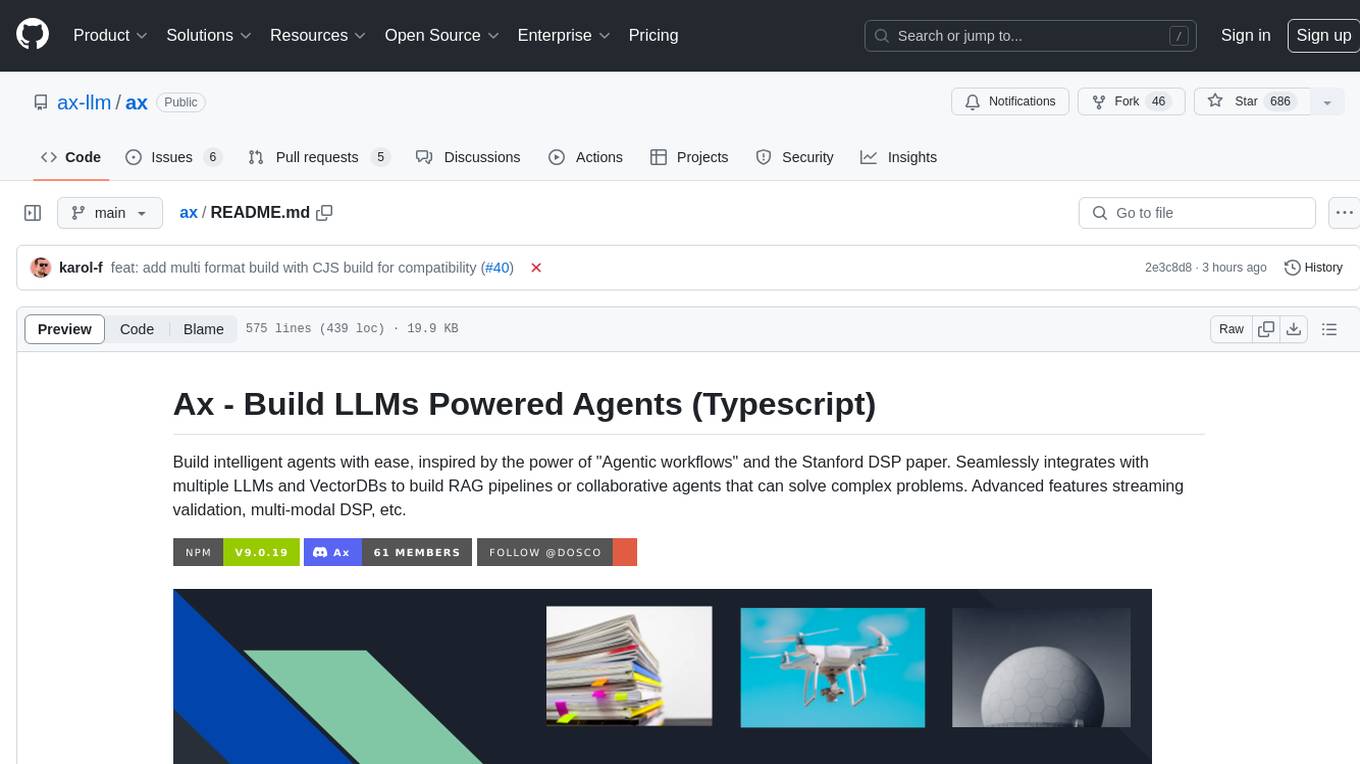
ax
Ax is a Typescript library that allows users to build intelligent agents inspired by agentic workflows and the Stanford DSP paper. It seamlessly integrates with multiple Large Language Models (LLMs) and VectorDBs to create RAG pipelines or collaborative agents capable of solving complex problems. The library offers advanced features such as streaming validation, multi-modal DSP, and automatic prompt tuning using optimizers. Users can easily convert documents of any format to text, perform smart chunking, embedding, and querying, and ensure output validation while streaming. Ax is production-ready, written in Typescript, and has zero dependencies.
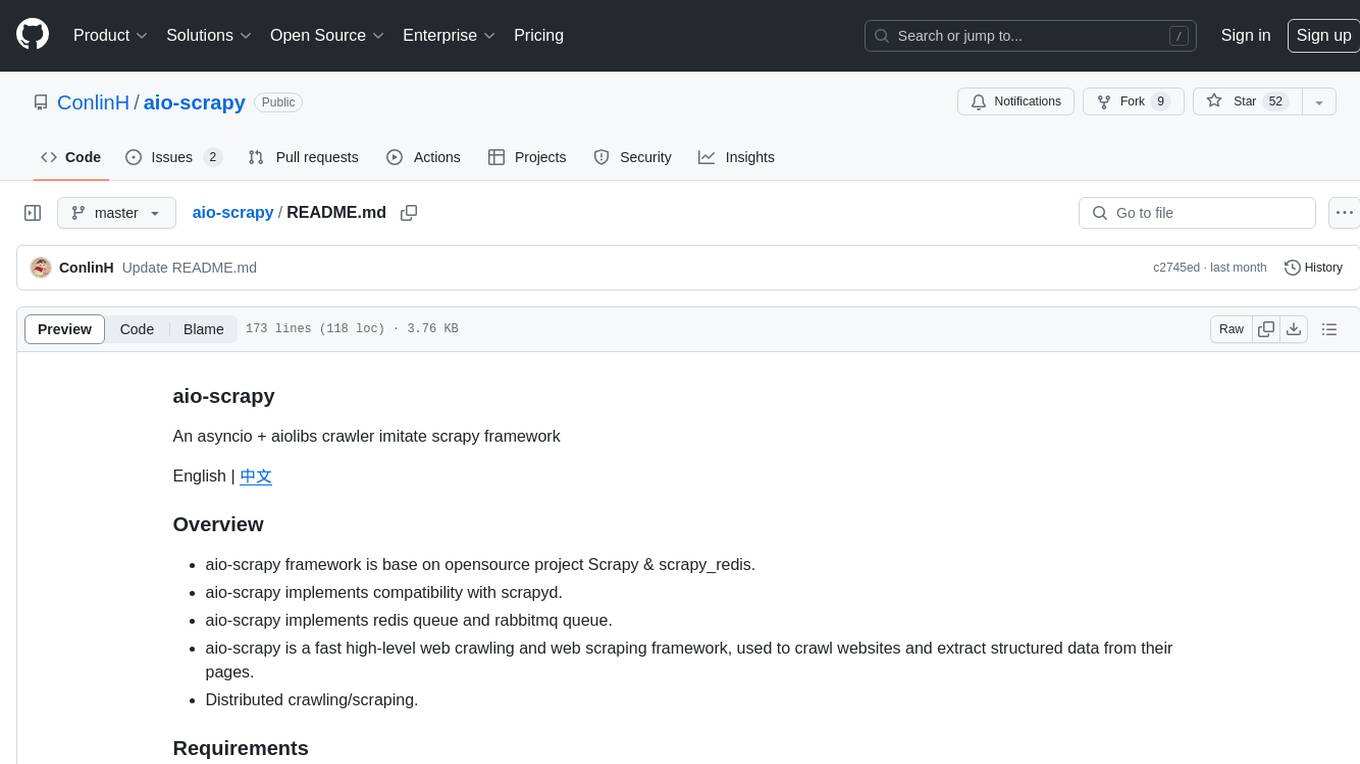
aio-scrapy
Aio-scrapy is an asyncio-based web crawling and web scraping framework inspired by Scrapy. It supports distributed crawling/scraping, implements compatibility with scrapyd, and provides options for using redis queue and rabbitmq queue. The framework is designed for fast extraction of structured data from websites. Aio-scrapy requires Python 3.9+ and is compatible with Linux, Windows, macOS, and BSD systems.
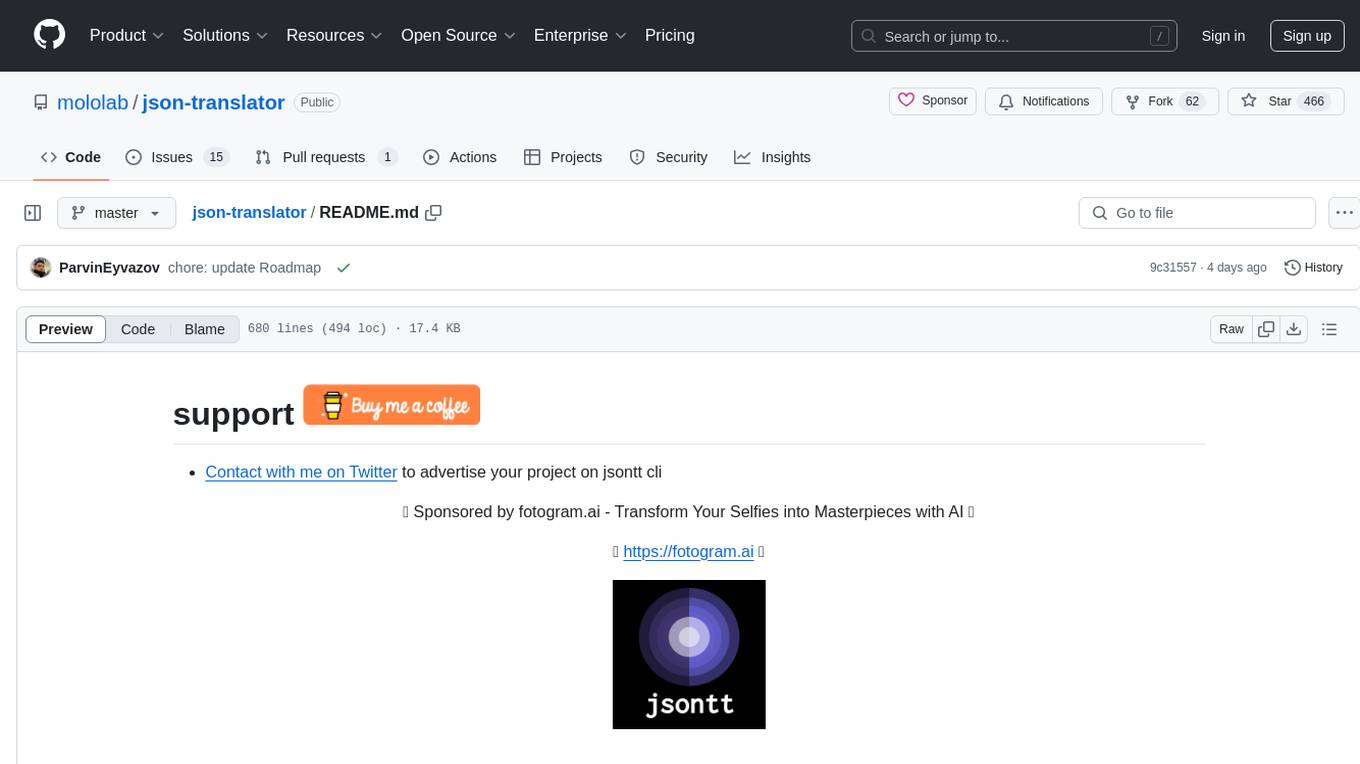
json-translator
The json-translator repository provides a free tool to translate JSON/YAML files or JSON objects into different languages using various translation modules. It supports CLI usage and package support, allowing users to translate words, sentences, JSON objects, and JSON files. The tool also offers multi-language translation, ignoring specific words, and safe translation practices. Users can contribute to the project by updating CLI, translation functions, JSON operations, and more. The roadmap includes features like Libre Translate option, Argos Translate option, Bing Translate option, and support for additional translation modules.
For similar tasks
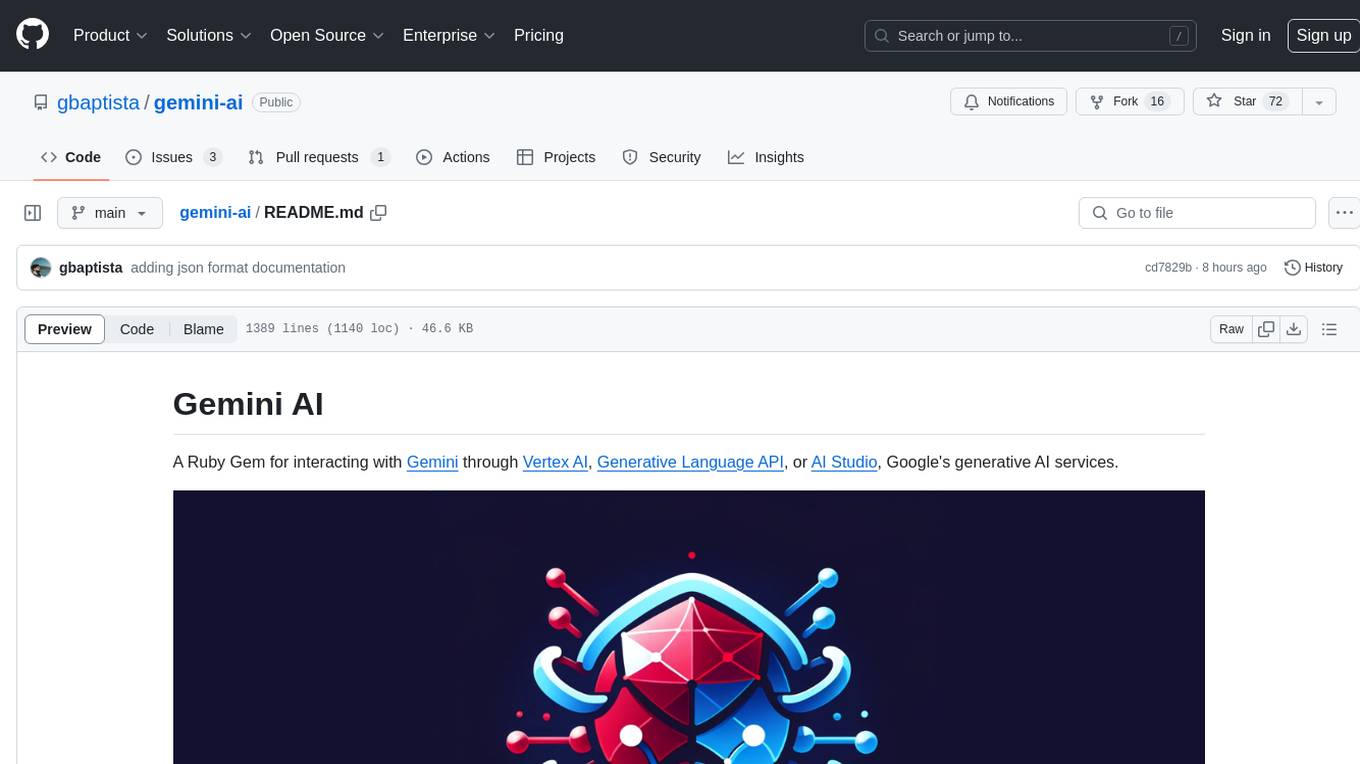
gemini-ai
Gemini AI is a Ruby Gem designed to provide low-level access to Google's generative AI services through Vertex AI, Generative Language API, or AI Studio. It allows users to interact with Gemini to build abstractions on top of it. The Gem provides functionalities for tasks such as generating content, embeddings, predictions, and more. It supports streaming capabilities, server-sent events, safety settings, system instructions, JSON format responses, and tools (functions) calling. The Gem also includes error handling, development setup, publishing to RubyGems, updating the README, and references to resources for further learning.
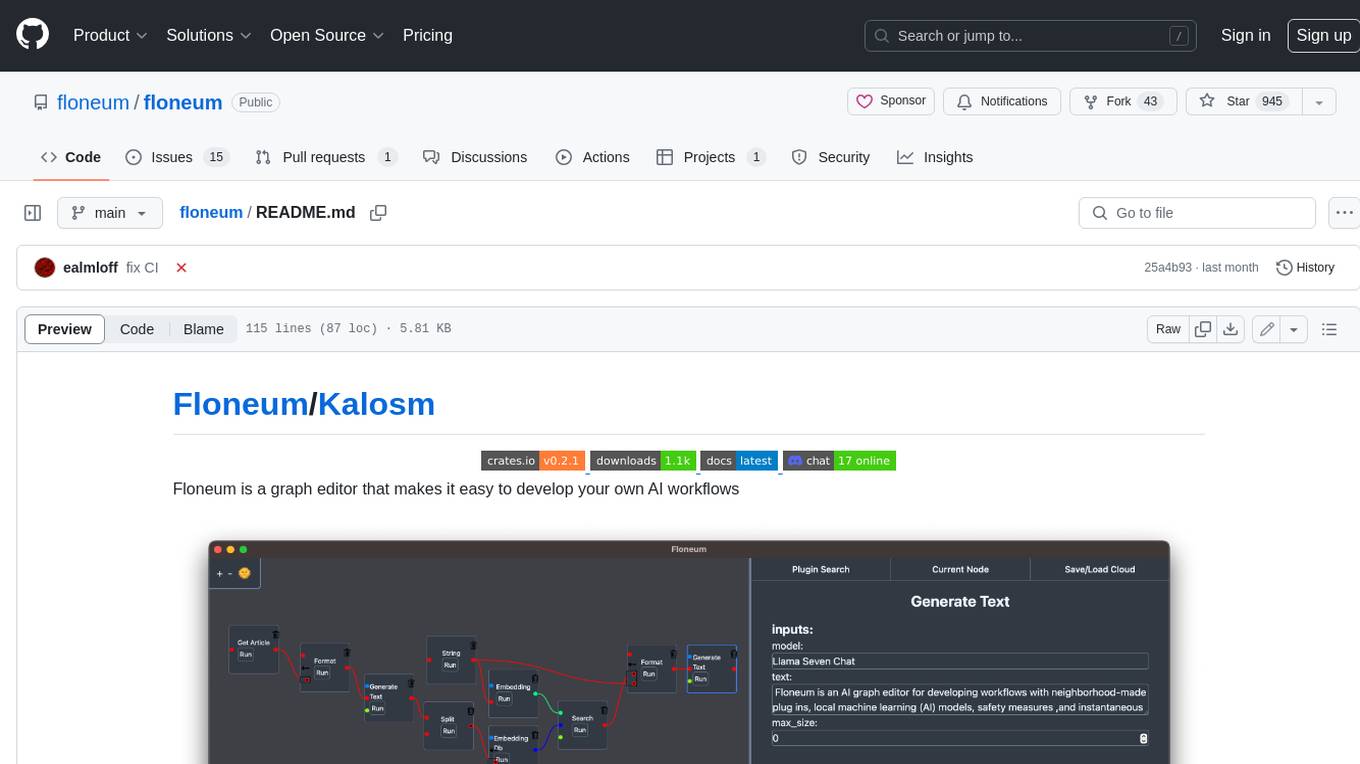
floneum
Floneum is a graph editor that makes it easy to develop your own AI workflows. It uses large language models (LLMs) to run AI models locally, without any external dependencies or even a GPU. This makes it easy to use LLMs with your own data, without worrying about privacy. Floneum also has a plugin system that allows you to improve the performance of LLMs and make them work better for your specific use case. Plugins can be used in any language that supports web assembly, and they can control the output of LLMs with a process similar to JSONformer or guidance.
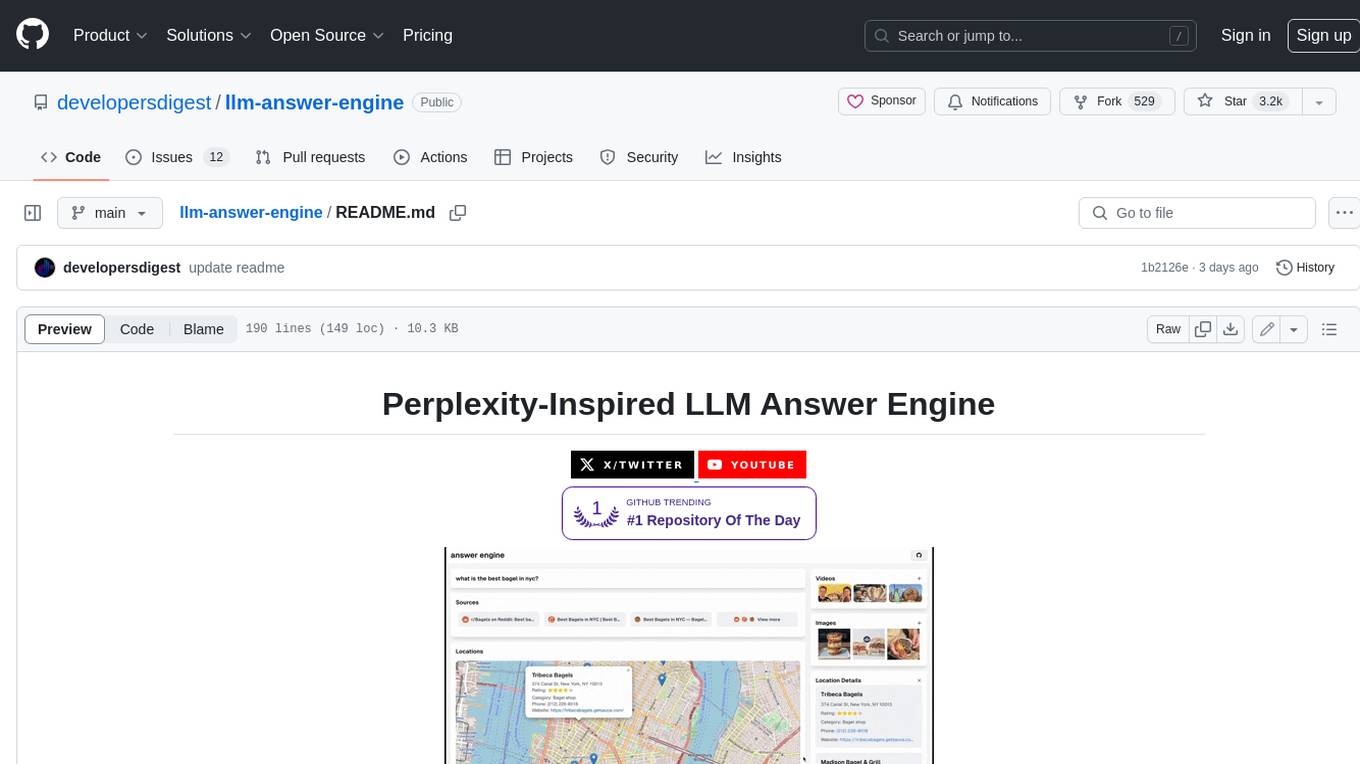
llm-answer-engine
This repository contains the code and instructions needed to build a sophisticated answer engine that leverages the capabilities of Groq, Mistral AI's Mixtral, Langchain.JS, Brave Search, Serper API, and OpenAI. Designed to efficiently return sources, answers, images, videos, and follow-up questions based on user queries, this project is an ideal starting point for developers interested in natural language processing and search technologies.
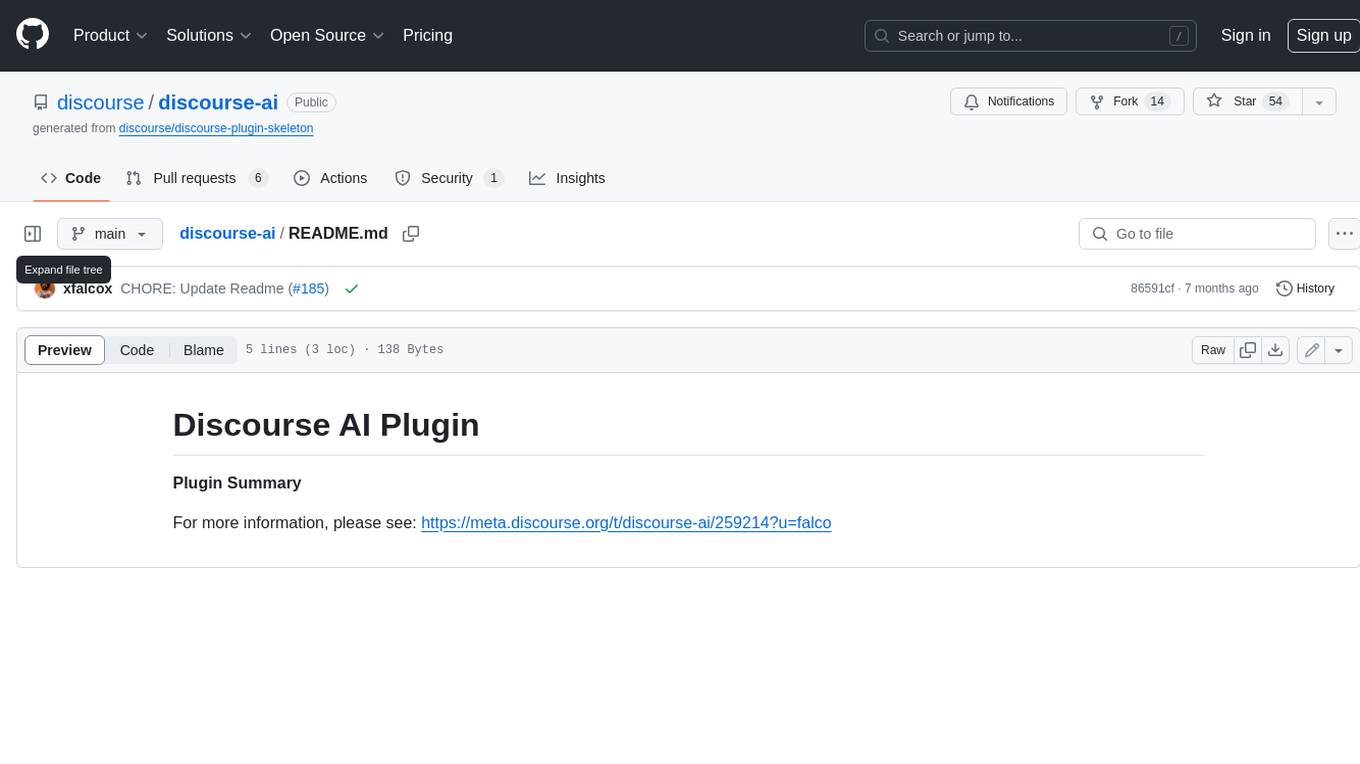
discourse-ai
Discourse AI is a plugin for the Discourse forum software that uses artificial intelligence to improve the user experience. It can automatically generate content, moderate posts, and answer questions. This can free up moderators and administrators to focus on other tasks, and it can help to create a more engaging and informative community.
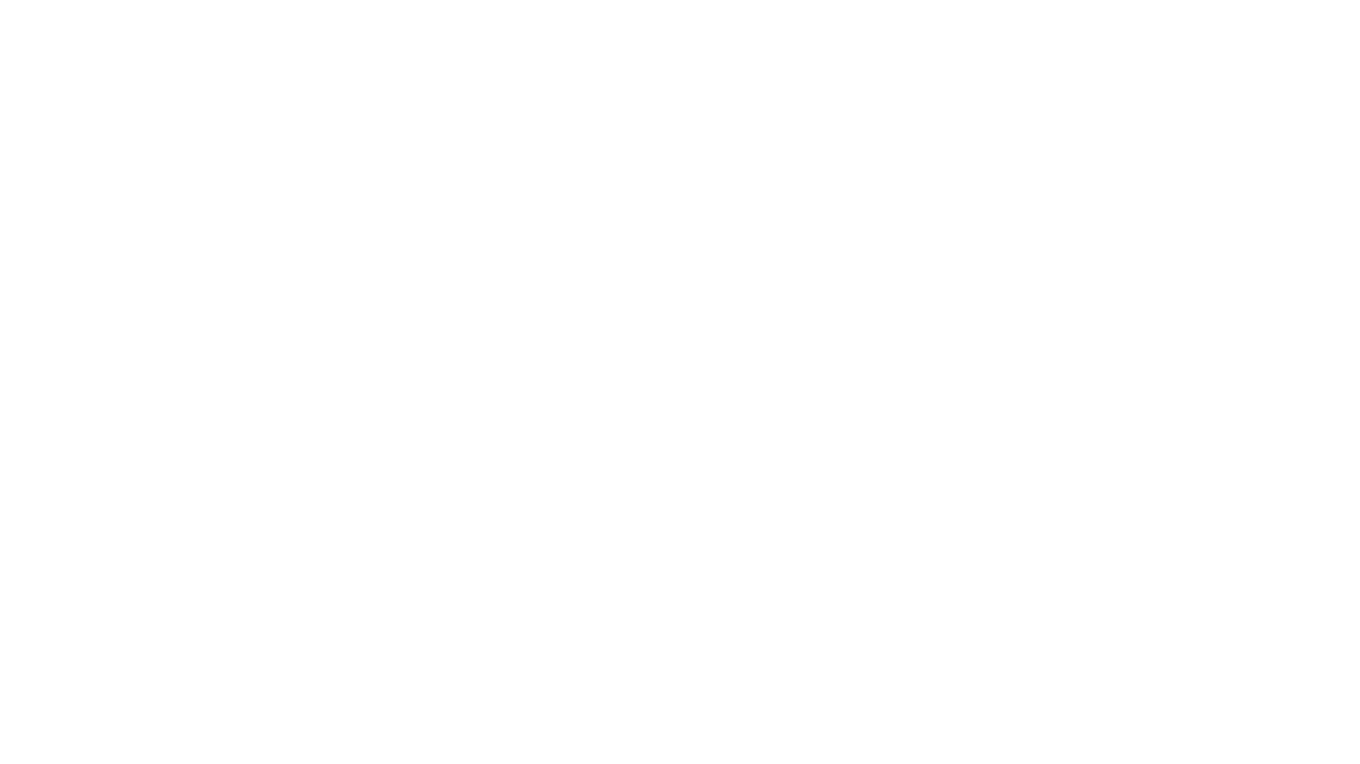
Gemini-API
Gemini-API is a reverse-engineered asynchronous Python wrapper for Google Gemini web app (formerly Bard). It provides features like persistent cookies, ImageFx support, extension support, classified outputs, official flavor, and asynchronous operation. The tool allows users to generate contents from text or images, have conversations across multiple turns, retrieve images in response, generate images with ImageFx, save images to local files, use Gemini extensions, check and switch reply candidates, and control log level.
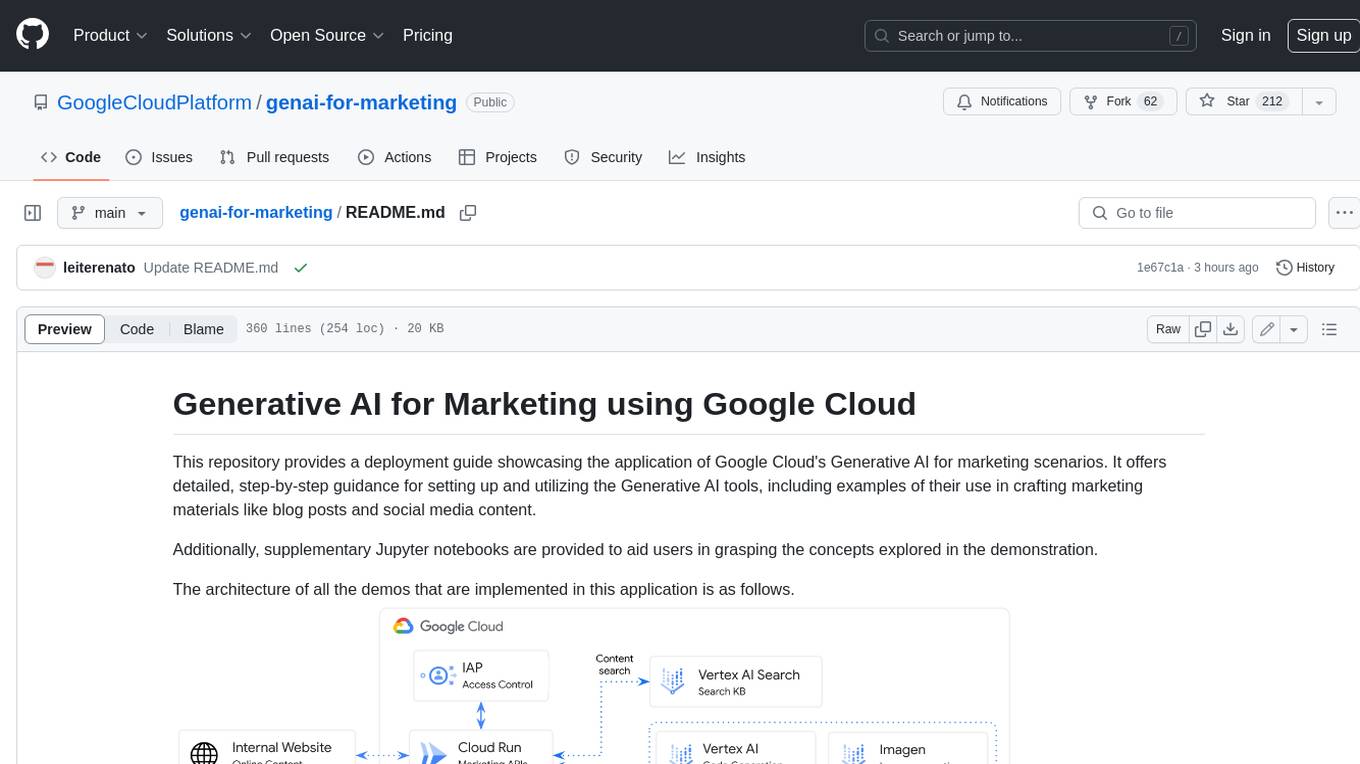
genai-for-marketing
This repository provides a deployment guide for utilizing Google Cloud's Generative AI tools in marketing scenarios. It includes step-by-step instructions, examples of crafting marketing materials, and supplementary Jupyter notebooks. The demos cover marketing insights, audience analysis, trendspotting, content search, content generation, and workspace integration. Users can access and visualize marketing data, analyze trends, improve search experience, and generate compelling content. The repository structure includes backend APIs, frontend code, sample notebooks, templates, and installation scripts.
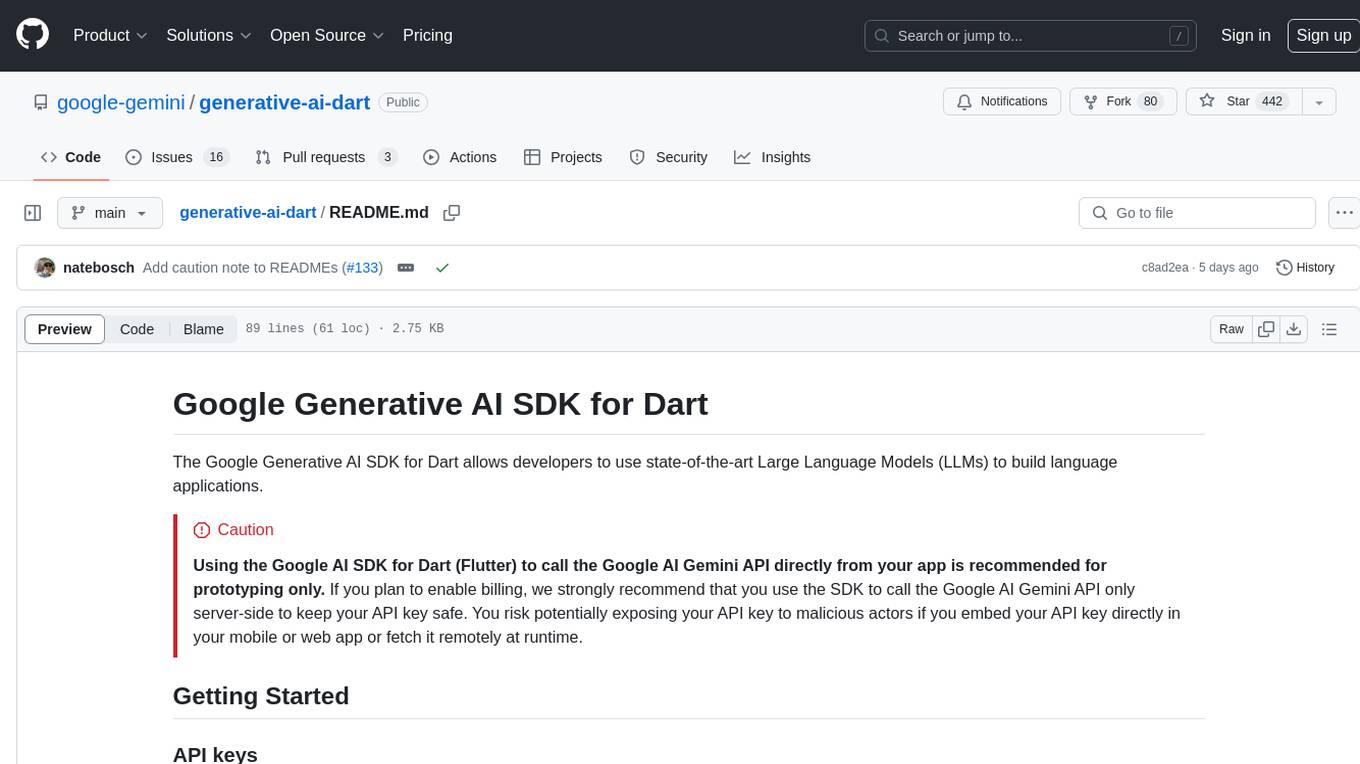
generative-ai-dart
The Google Generative AI SDK for Dart enables developers to utilize cutting-edge Large Language Models (LLMs) for creating language applications. It provides access to the Gemini API for generating content using state-of-the-art models. Developers can integrate the SDK into their Dart or Flutter applications to leverage powerful AI capabilities. It is recommended to use the SDK for server-side API calls to ensure the security of API keys and protect against potential key exposure in mobile or web apps.
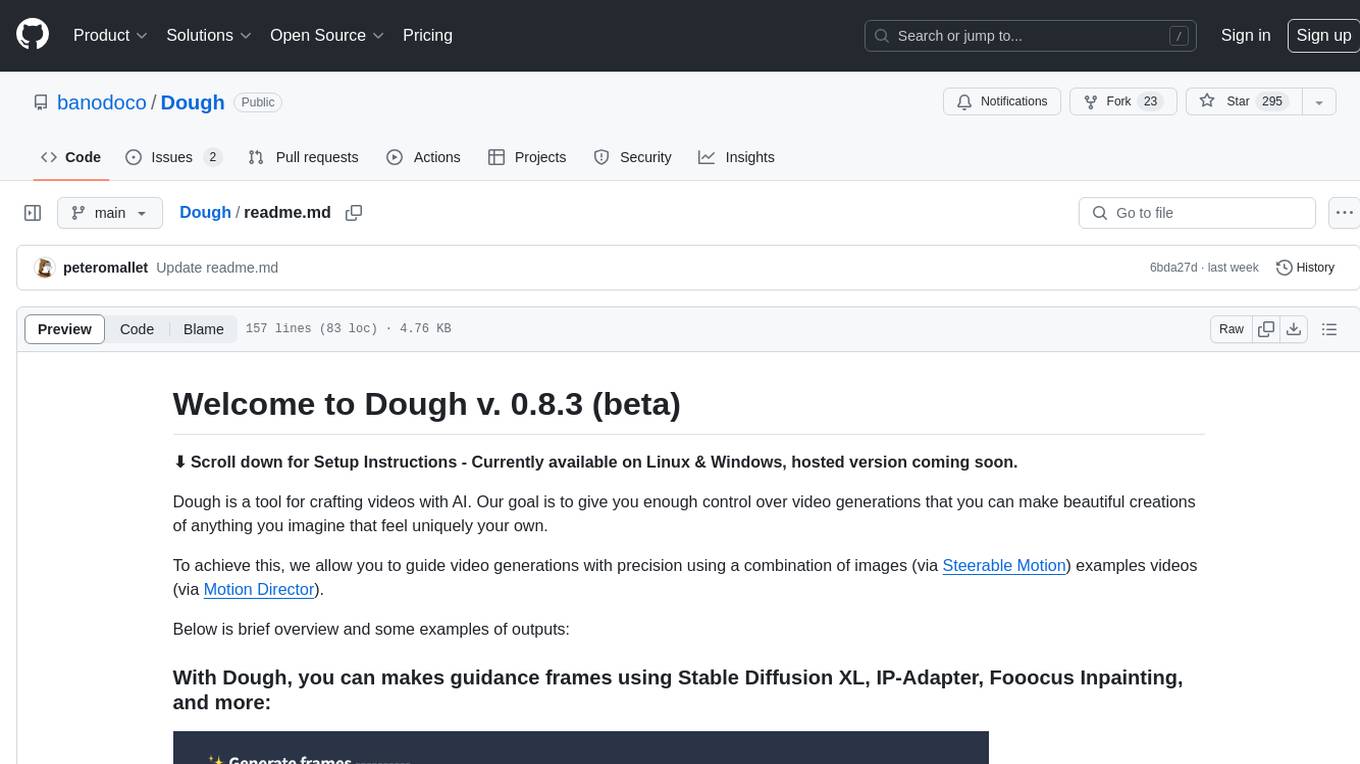
Dough
Dough is a tool for crafting videos with AI, allowing users to guide video generations with precision using images and example videos. Users can create guidance frames, assemble shots, and animate them by defining parameters and selecting guidance videos. The tool aims to help users make beautiful and unique video creations, providing control over the generation process. Setup instructions are available for Linux and Windows platforms, with detailed steps for installation and running the app.
For similar jobs
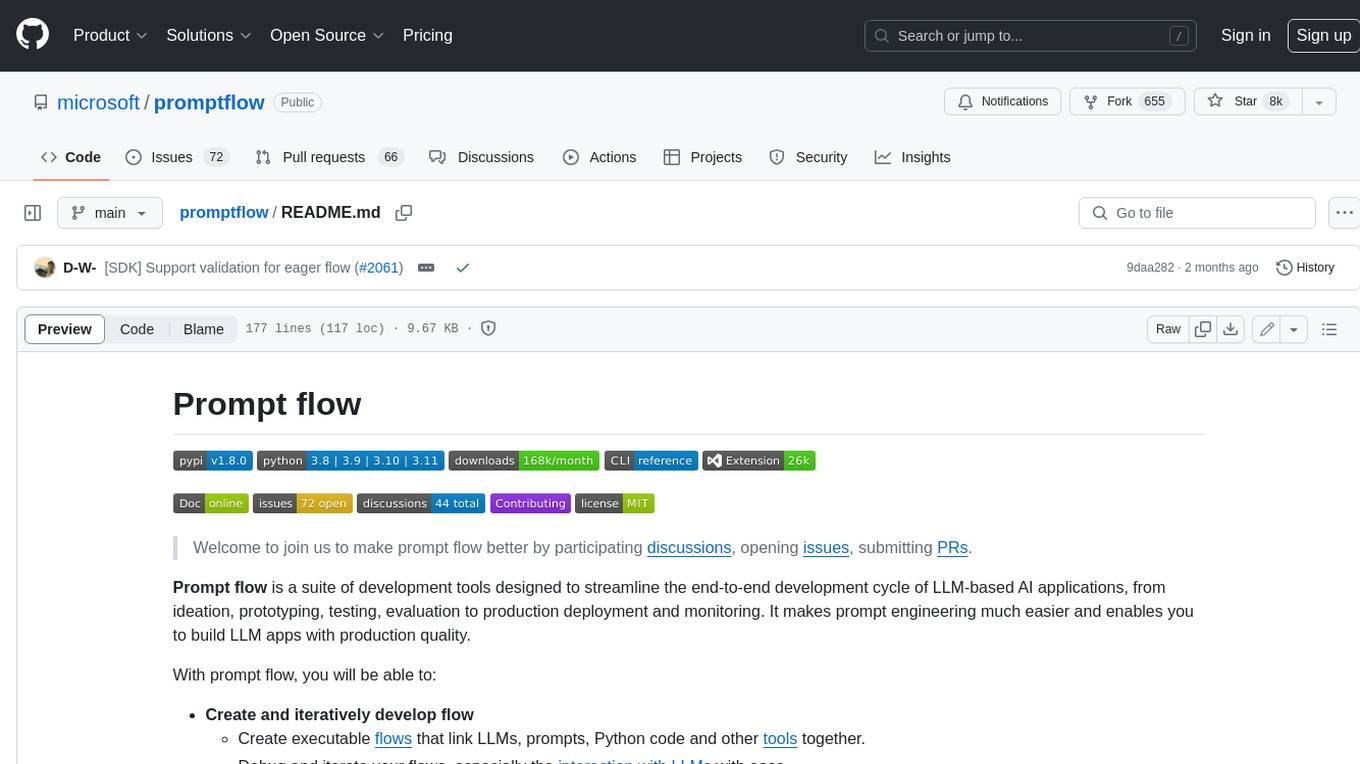
promptflow
**Prompt flow** is a suite of development tools designed to streamline the end-to-end development cycle of LLM-based AI applications, from ideation, prototyping, testing, evaluation to production deployment and monitoring. It makes prompt engineering much easier and enables you to build LLM apps with production quality.
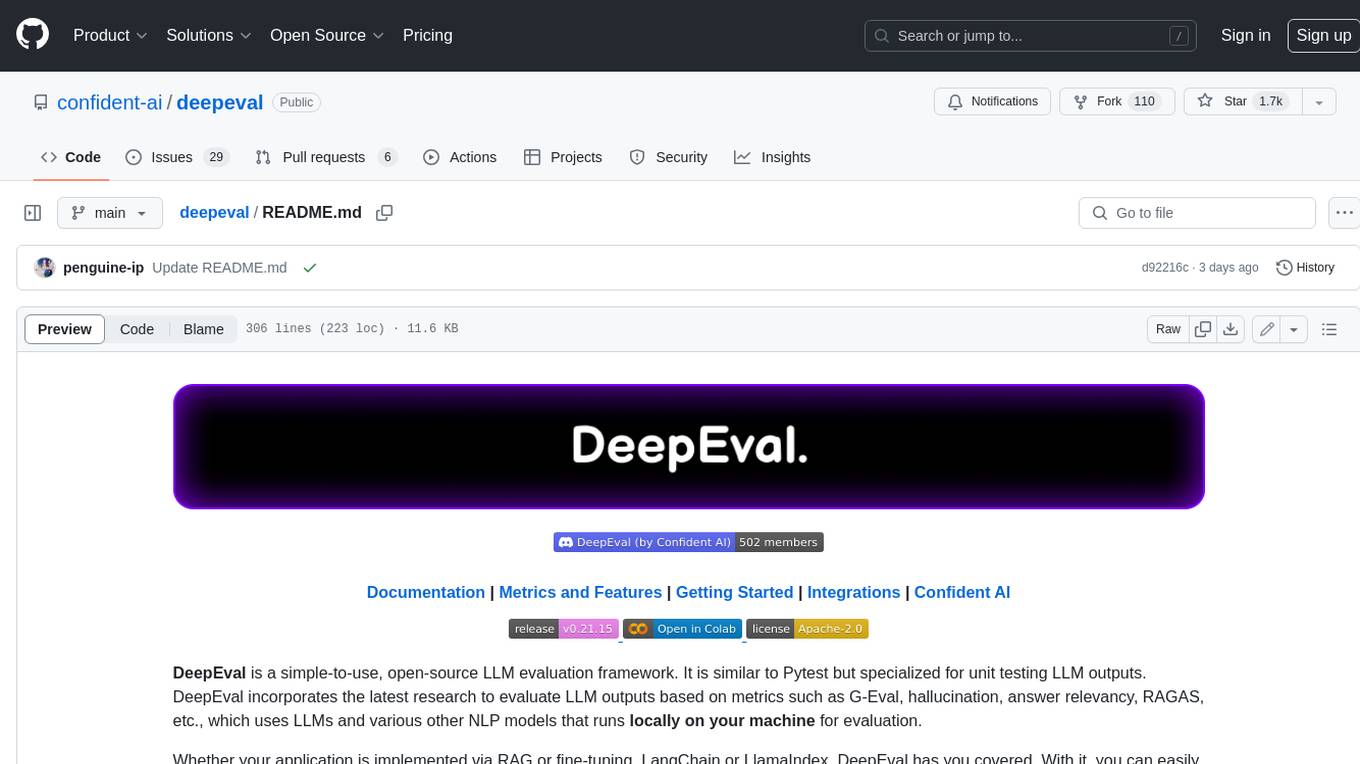
deepeval
DeepEval is a simple-to-use, open-source LLM evaluation framework specialized for unit testing LLM outputs. It incorporates various metrics such as G-Eval, hallucination, answer relevancy, RAGAS, etc., and runs locally on your machine for evaluation. It provides a wide range of ready-to-use evaluation metrics, allows for creating custom metrics, integrates with any CI/CD environment, and enables benchmarking LLMs on popular benchmarks. DeepEval is designed for evaluating RAG and fine-tuning applications, helping users optimize hyperparameters, prevent prompt drifting, and transition from OpenAI to hosting their own Llama2 with confidence.
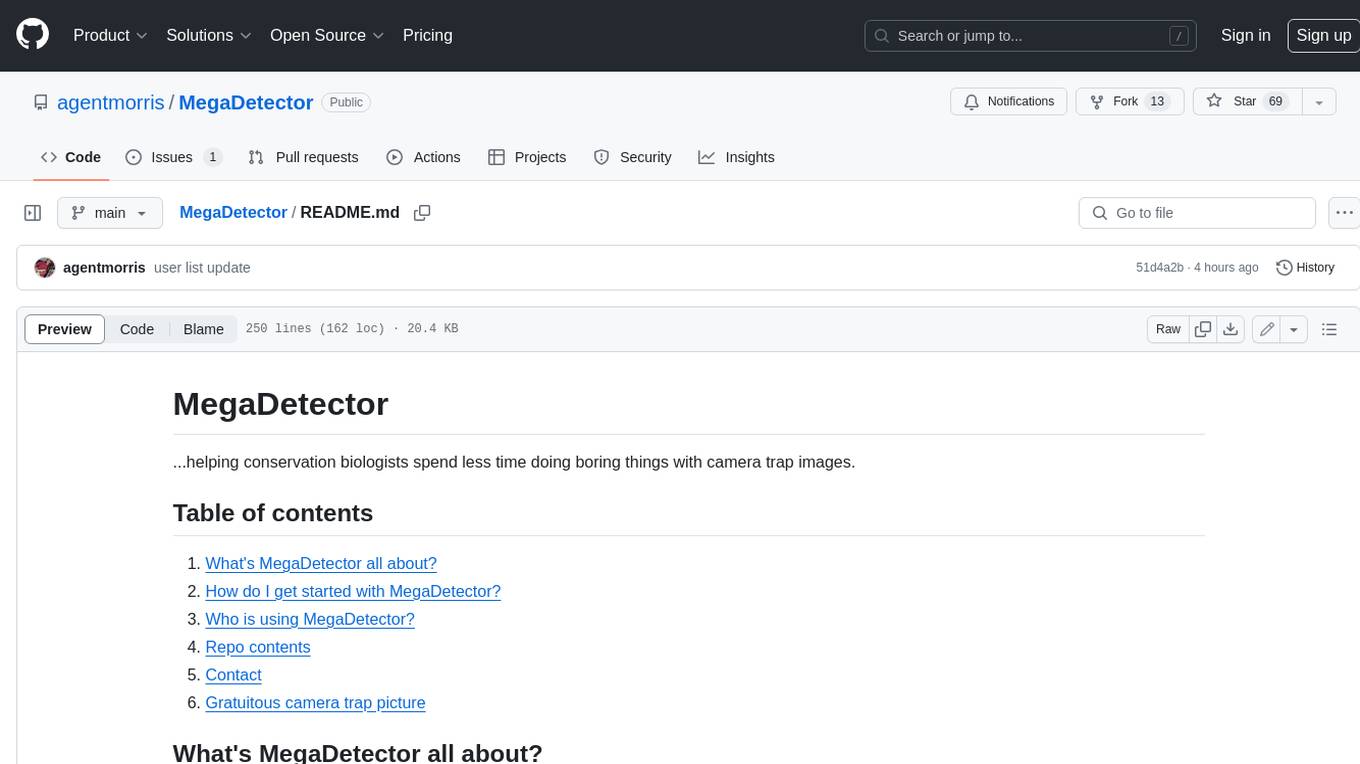
MegaDetector
MegaDetector is an AI model that identifies animals, people, and vehicles in camera trap images (which also makes it useful for eliminating blank images). This model is trained on several million images from a variety of ecosystems. MegaDetector is just one of many tools that aims to make conservation biologists more efficient with AI. If you want to learn about other ways to use AI to accelerate camera trap workflows, check out our of the field, affectionately titled "Everything I know about machine learning and camera traps".
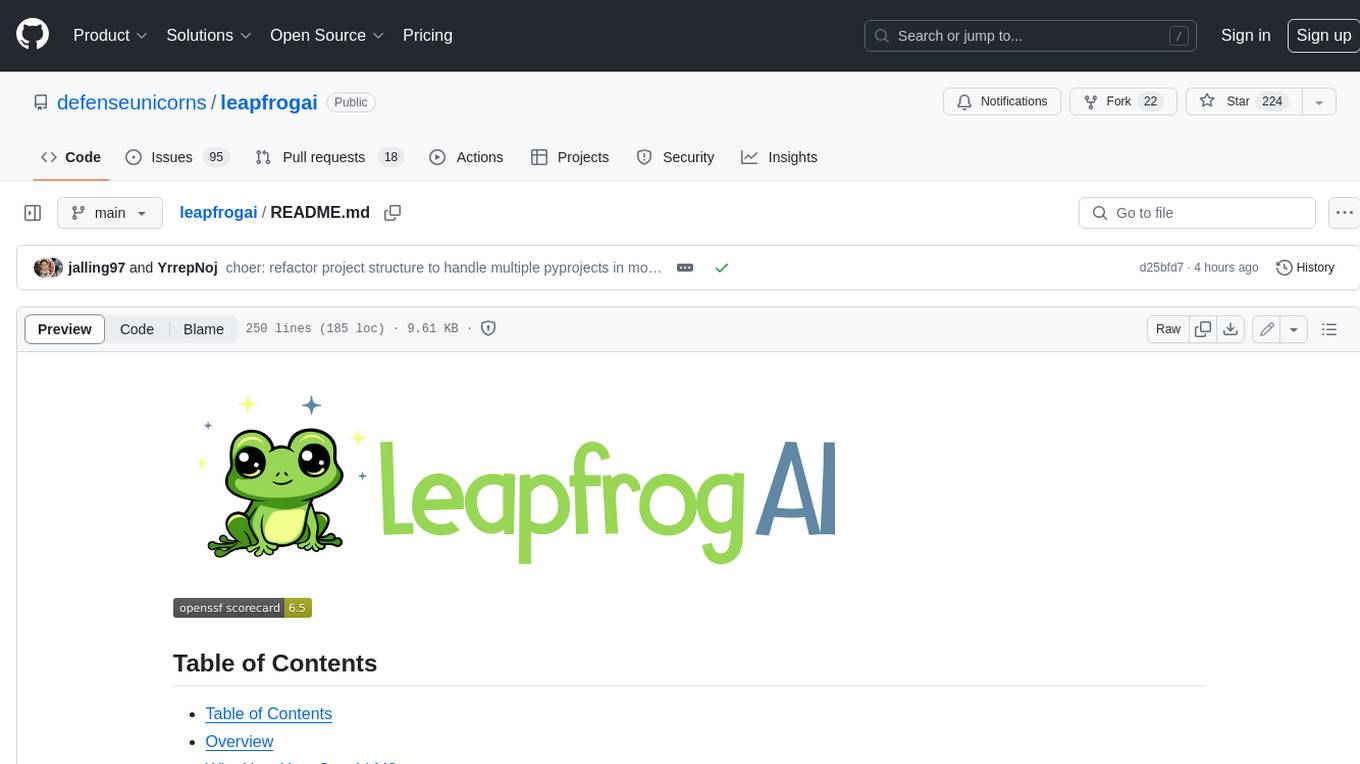
leapfrogai
LeapfrogAI is a self-hosted AI platform designed to be deployed in air-gapped resource-constrained environments. It brings sophisticated AI solutions to these environments by hosting all the necessary components of an AI stack, including vector databases, model backends, API, and UI. LeapfrogAI's API closely matches that of OpenAI, allowing tools built for OpenAI/ChatGPT to function seamlessly with a LeapfrogAI backend. It provides several backends for various use cases, including llama-cpp-python, whisper, text-embeddings, and vllm. LeapfrogAI leverages Chainguard's apko to harden base python images, ensuring the latest supported Python versions are used by the other components of the stack. The LeapfrogAI SDK provides a standard set of protobuffs and python utilities for implementing backends and gRPC. LeapfrogAI offers UI options for common use-cases like chat, summarization, and transcription. It can be deployed and run locally via UDS and Kubernetes, built out using Zarf packages. LeapfrogAI is supported by a community of users and contributors, including Defense Unicorns, Beast Code, Chainguard, Exovera, Hypergiant, Pulze, SOSi, United States Navy, United States Air Force, and United States Space Force.
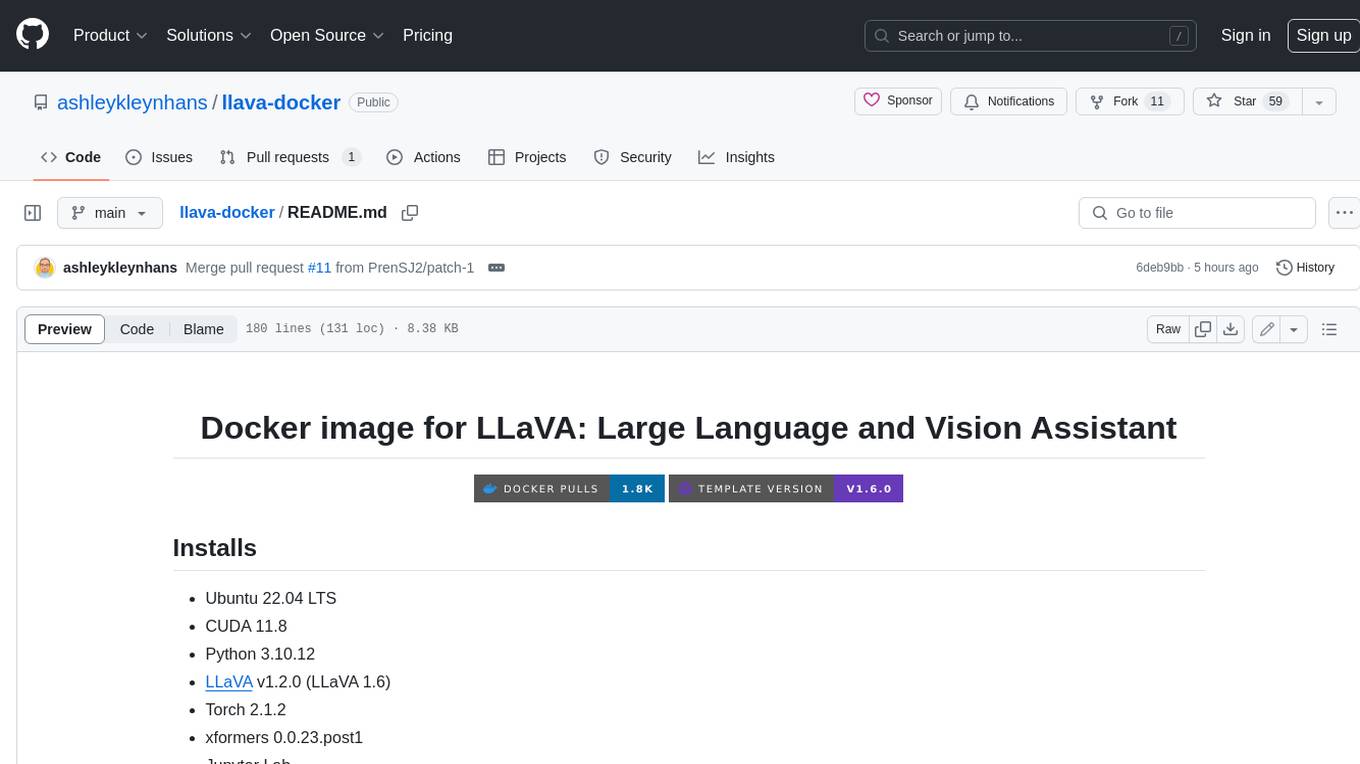
llava-docker
This Docker image for LLaVA (Large Language and Vision Assistant) provides a convenient way to run LLaVA locally or on RunPod. LLaVA is a powerful AI tool that combines natural language processing and computer vision capabilities. With this Docker image, you can easily access LLaVA's functionalities for various tasks, including image captioning, visual question answering, text summarization, and more. The image comes pre-installed with LLaVA v1.2.0, Torch 2.1.2, xformers 0.0.23.post1, and other necessary dependencies. You can customize the model used by setting the MODEL environment variable. The image also includes a Jupyter Lab environment for interactive development and exploration. Overall, this Docker image offers a comprehensive and user-friendly platform for leveraging LLaVA's capabilities.
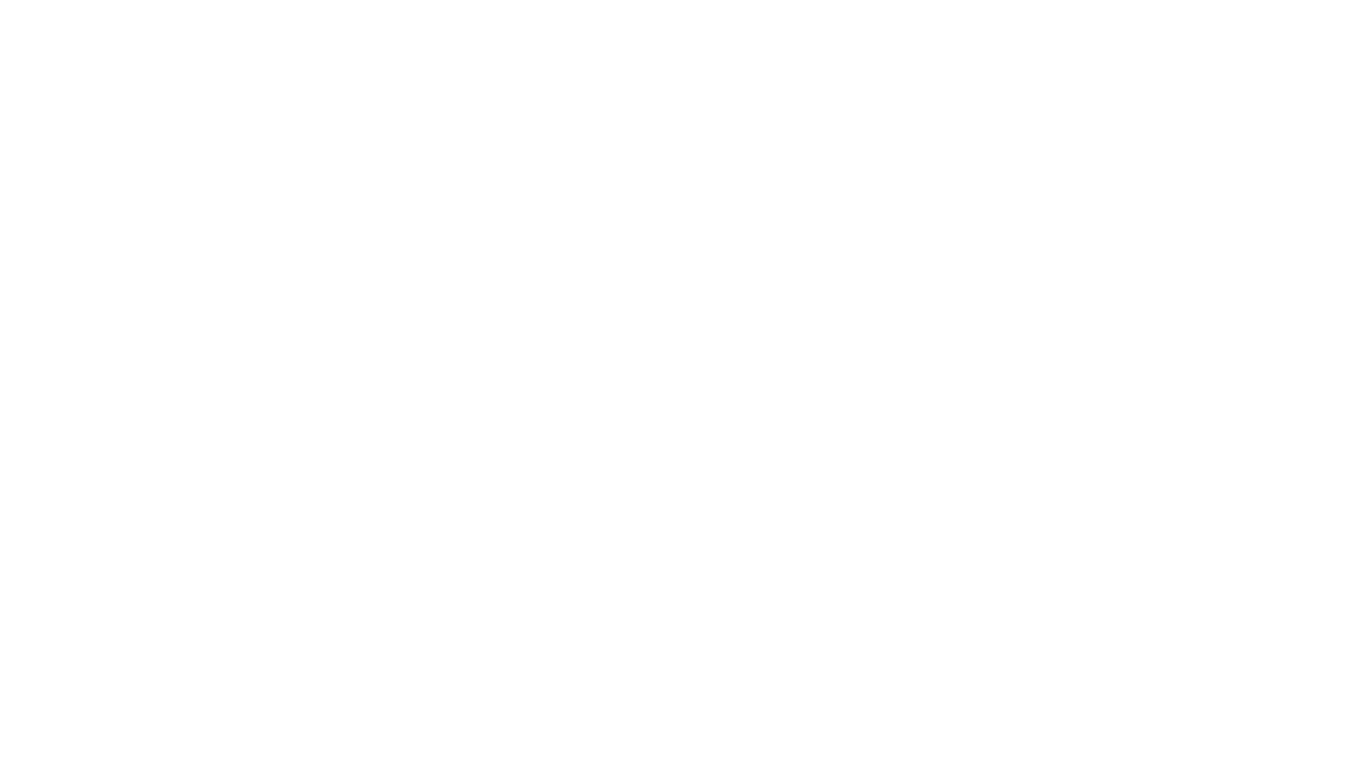
carrot
The 'carrot' repository on GitHub provides a list of free and user-friendly ChatGPT mirror sites for easy access. The repository includes sponsored sites offering various GPT models and services. Users can find and share sites, report errors, and access stable and recommended sites for ChatGPT usage. The repository also includes a detailed list of ChatGPT sites, their features, and accessibility options, making it a valuable resource for ChatGPT users seeking free and unlimited GPT services.
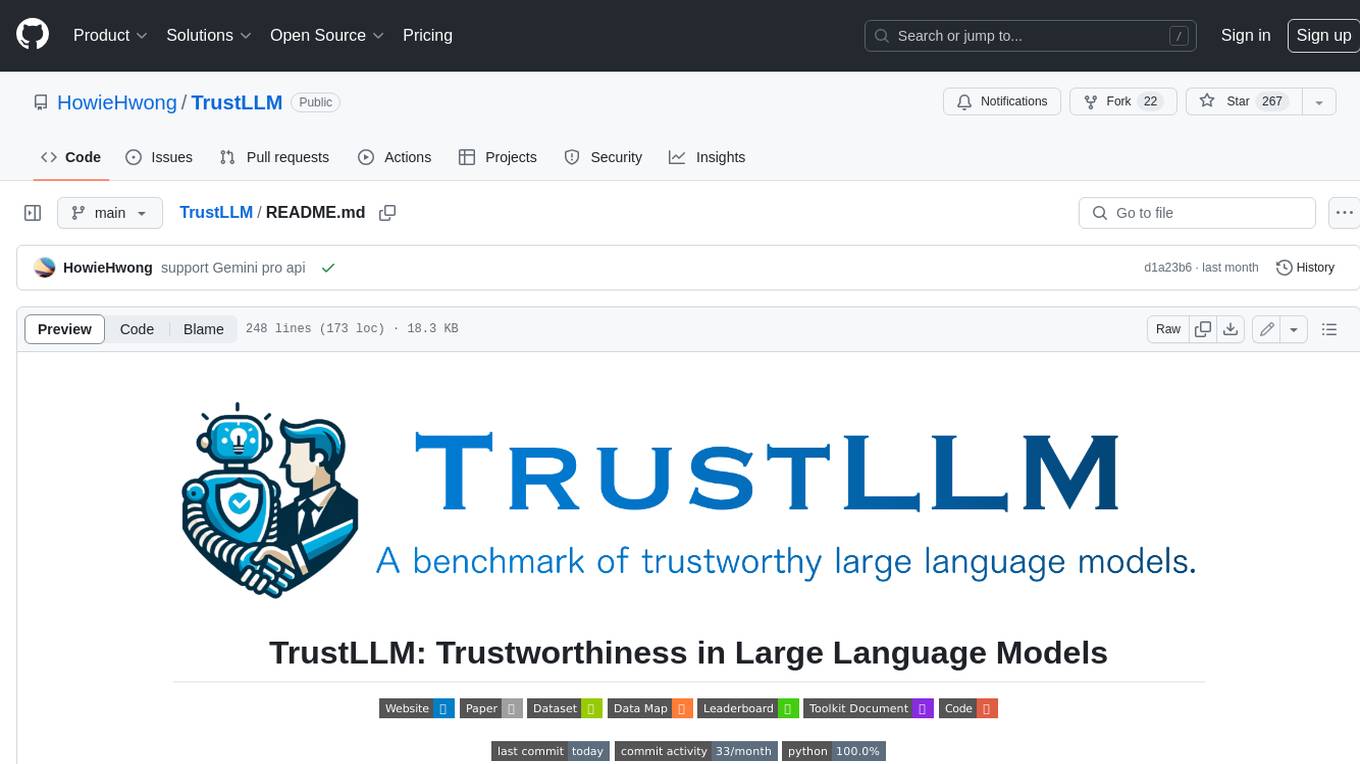
TrustLLM
TrustLLM is a comprehensive study of trustworthiness in LLMs, including principles for different dimensions of trustworthiness, established benchmark, evaluation, and analysis of trustworthiness for mainstream LLMs, and discussion of open challenges and future directions. Specifically, we first propose a set of principles for trustworthy LLMs that span eight different dimensions. Based on these principles, we further establish a benchmark across six dimensions including truthfulness, safety, fairness, robustness, privacy, and machine ethics. We then present a study evaluating 16 mainstream LLMs in TrustLLM, consisting of over 30 datasets. The document explains how to use the trustllm python package to help you assess the performance of your LLM in trustworthiness more quickly. For more details about TrustLLM, please refer to project website.
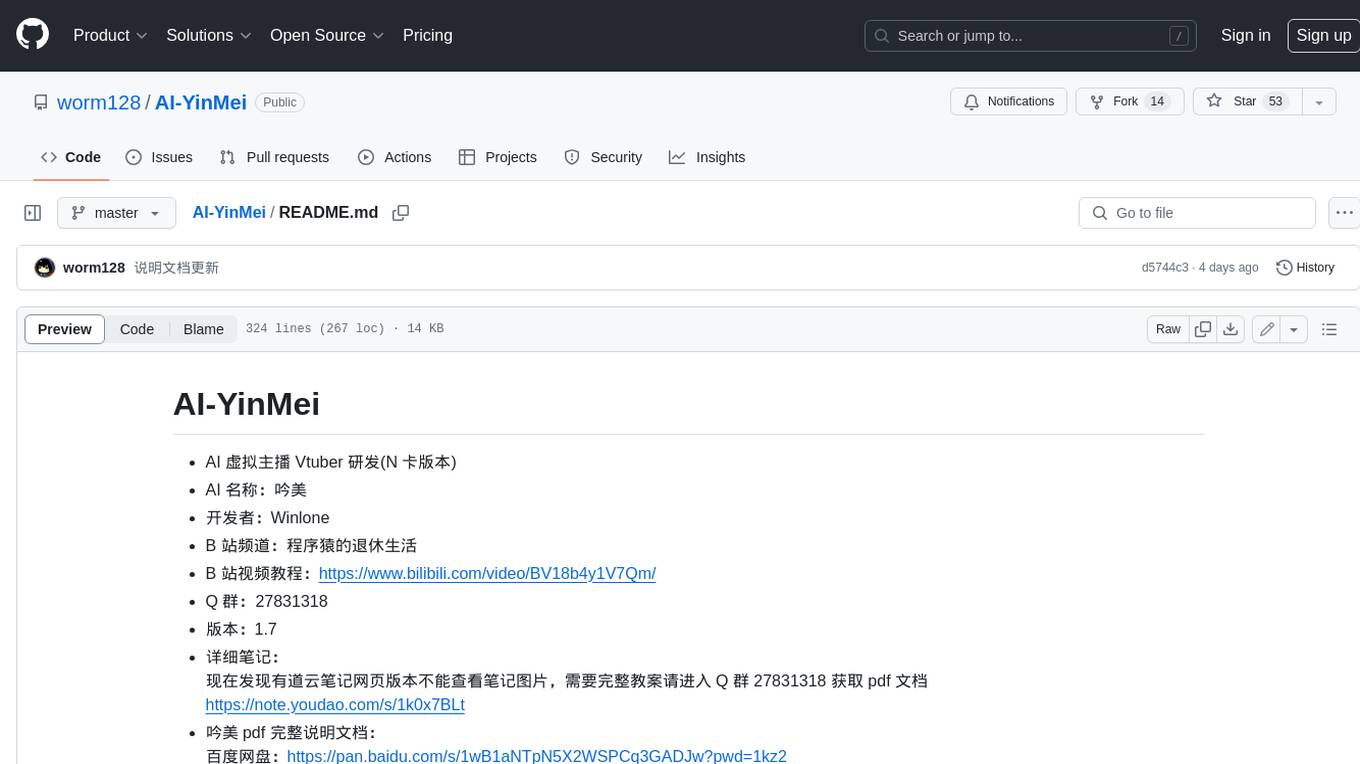
AI-YinMei
AI-YinMei is an AI virtual anchor Vtuber development tool (N card version). It supports fastgpt knowledge base chat dialogue, a complete set of solutions for LLM large language models: [fastgpt] + [one-api] + [Xinference], supports docking bilibili live broadcast barrage reply and entering live broadcast welcome speech, supports Microsoft edge-tts speech synthesis, supports Bert-VITS2 speech synthesis, supports GPT-SoVITS speech synthesis, supports expression control Vtuber Studio, supports painting stable-diffusion-webui output OBS live broadcast room, supports painting picture pornography public-NSFW-y-distinguish, supports search and image search service duckduckgo (requires magic Internet access), supports image search service Baidu image search (no magic Internet access), supports AI reply chat box [html plug-in], supports AI singing Auto-Convert-Music, supports playlist [html plug-in], supports dancing function, supports expression video playback, supports head touching action, supports gift smashing action, supports singing automatic start dancing function, chat and singing automatic cycle swing action, supports multi scene switching, background music switching, day and night automatic switching scene, supports open singing and painting, let AI automatically judge the content.