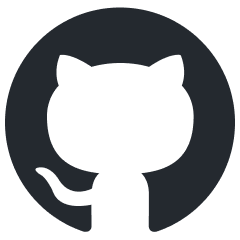
claim-ai-phone-bot
AI-powered call center solution with Azure and OpenAI GPT.
Stars: 65
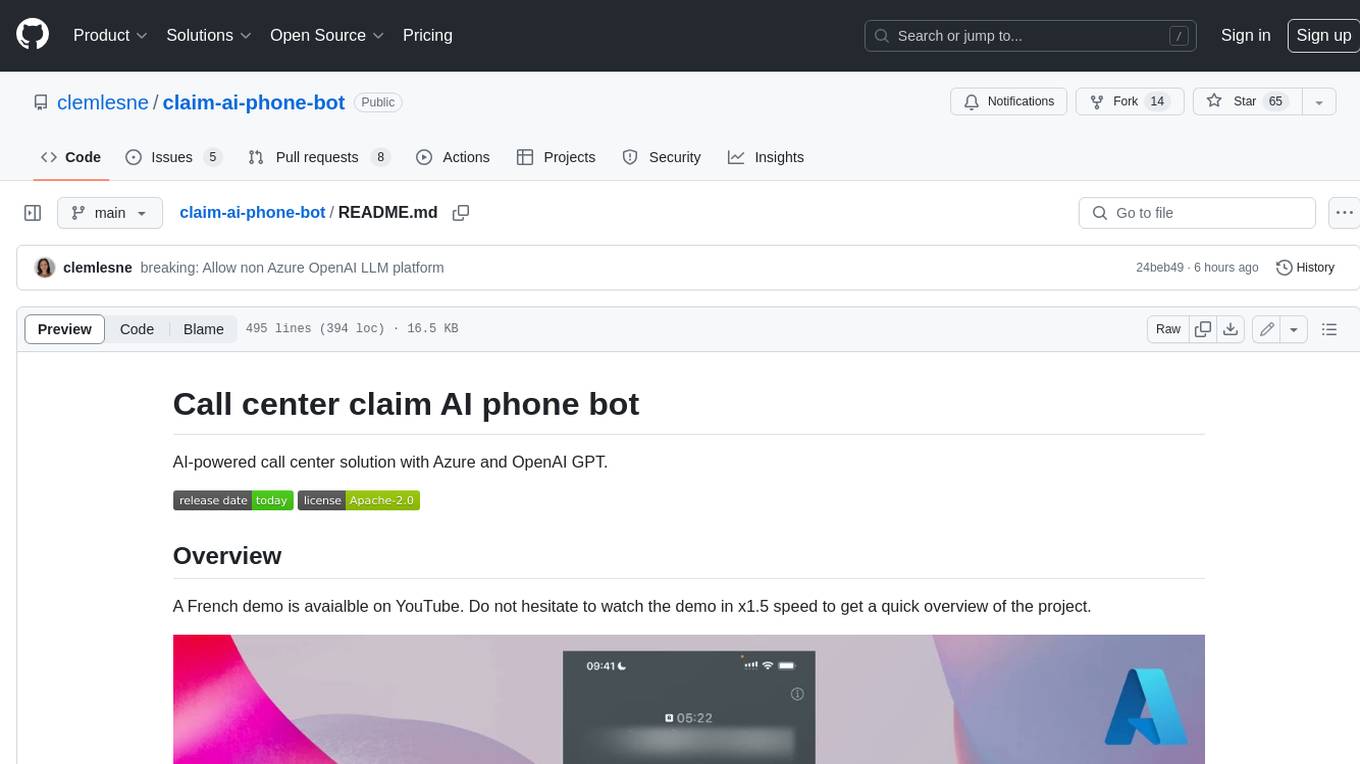
AI-powered call center solution with Azure and OpenAI GPT. The bot can answer calls, understand the customer's request, and provide relevant information or assistance. It can also create a todo list of tasks to complete the claim, and send a report after the call. The bot is customizable, and can be used in multiple languages.
README:
AI-powered call center solution with Azure and OpenAI GPT.
A French demo is avaialble on YouTube. Do not hesitate to watch the demo in x1.5 speed to get a quick overview of the project.
Main interactions shown in the demo:
- User calls the call center
- The bot answers and the conversation starts
- The bot stores conversation, claim and todo list in the database
Extract of the data stored during the call:
{
"claim": {
"incident_date_time": "2024-01-11T19:33:41",
"incident_description": "The vehicle began to travel with a burning smell and the driver pulled over to the side of the freeway.",
"policy_number": "B01371946",
"policyholder_phone": "[number masked for the demo]",
"policyholder_name": "Clémence Lesne",
"vehicle_info": "Ford Fiesta 2003"
},
"reminders": [
{
"description": "Check that all the information in Clémence Lesne's file is correct and complete.",
"due_date_time": "2024-01-18T16:00:00",
"title": "Check Clémence file"
}
]
}
[!NOTE] This project is a proof of concept. It is not intended to be used in production. This demonstrates how can be combined Azure Communication Services, Azure Cognitive Services and Azure OpenAI to build an automated call center solution.
- [x] Access the claim on a public website
- [x] Access to customer conversation history
- [x] Allow user to change the language of the conversation
- [x] Bot can be called from a phone number
- [x] Bot use multiple voice tones (e.g. happy, sad, neutral) to keep the conversation engaging
- [x] Company products (= lexicon) can be understood by the bot (e.g. a name of a specific insurance product)
- [x] Create by itself a todo list of tasks to complete the claim
- [x] Customizable prompts
- [x] Disengaging from a human agent when needed
- [x] Filter out inappropriate content from the LLM, like profanity or concurrence company names
- [x] Fine understanding of the customer request with GPT-4 Turbo
- [x] Follow a specific data schema for the claim
- [x] Has access to a documentation database (few-shot training / RAG)
- [x] Help the user to find the information needed to complete the claim
- [x] Lower AI Search cost by usign a Redis cache
- [x] Monitoring and tracing with Application Insights
- [x] Responses are streamed from the LLM to the user, to avoid long pauses
- [x] Send a SMS report after the call
- [x] Take back a conversation after a disengagement
- [ ] Call back the user when needed
- [ ] Simulate a IVR workflow
A report is available at https://[your_domain]/report/[phone_number]
(like http://localhost:8080/report/%2B133658471534
). It shows the conversation history, claim data and reminders.
---
title: System diagram (C4 model)
---
graph
user(["User"])
agent(["Agent"])
api["Claim AI"]
api -- Transfer to --> agent
api -. Send voice .-> user
user -- Call --> api
---
title: Claim AI component diagram (C4 model)
---
graph LR
agent(["Agent"])
user(["User"])
subgraph "Claim AI"
ai_search[("RAG\n(AI Search)")]
api["API"]
communication_service_sms["SMS gateway\n(Communication Services)"]
communication_service["Call gateway\n(Communication Services)"]
constent_safety["Moderation\n(Content Safety)"]
db[("Conversations and claims\n(Cosmos DB or SQLite)")]
event_grid[("Broker\n(Event Grid)")]
gpt["GPT-4 Turbo\n(OpenAI)"]
redis[("Cache\n(Redis)")]
translation["Translation\n(Cognitive Services)"]
end
api -- Answer with text --> communication_service
api -- Ask for translation --> translation
api -- Few-shot training --> ai_search
api -- Generate completion --> gpt
api -- Get cached data --> redis
api -- Save conversation --> db
api -- Send SMS report --> communication_service_sms
api -- Test for profanity --> constent_safety
api -- Transfer to agent --> communication_service
api -. Watch .-> event_grid
communication_service -- Notifies --> event_grid
communication_service -- Transfer to --> agent
communication_service -. Send voice .-> user
communication_service_sms -- Send SMS --> user
user -- Call --> communication_service
sequenceDiagram
autonumber
actor Customer
participant PSTN
participant Text to Speech
participant Speech to Text
actor Human agent
participant Event Grid
participant Communication Services
participant Content Safety
participant API
participant Cosmos DB
participant OpenAI GPT
participant AI Search
API->>Event Grid: Subscribe to events
Customer->>PSTN: Initiate a call
PSTN->>Communication Services: Forward call
Communication Services->>Event Grid: New call event
Event Grid->>API: Send event to event URL (HTTP webhook)
activate API
API->>Communication Services: Accept the call and give inbound URL
deactivate API
Communication Services->>Speech to Text: Transform speech to text
Communication Services->>API: Send text to the inbound URL
activate API
alt First call
API->>Communication Services: Send static SSML text
else Callback
API->>AI Search: Gather training data
API->>OpenAI GPT: Ask for a completion
OpenAI GPT-->>API: Answer (HTTP/2 SSE)
loop Over buffer
loop Over multiple tools
alt Is this a claim data update?
API->>Content Safety: Ask for safety test
alt Is the text safe?
API->>Communication Services: Send dynamic SSML text
end
API->>Cosmos DB: Update claim data
else Does the user want the human agent?
API->>Communication Services: Send static SSML text
API->>Communication Services: Transfer to a human
Communication Services->>Human agent: Call the phone number
else Should we end the call?
API->>Communication Services: Send static SSML text
API->>Communication Services: End the call
end
end
alt Is there a text?
alt Is there enough text to make a sentence?
API->>Content Safety: Ask for safety test
alt Is the text safe?
API->>Communication Services: Send dynamic SSML text
end
end
end
end
API->>Cosmos DB: Persist conversation
end
deactivate API
Communication Services->>PSTN: Send voice
PSTN->>Customer: Forward voice
Container is available on GitHub Actions, at:
- Latest version from a branch:
ghcr.io/clemlesne/claim-ai-phone-bot:main
- Specific tag:
ghcr.io/clemlesne/claim-ai-phone-bot:0.1.0
(recommended)
Create a local config.yaml
file (most of the fields are filled automatically by the deployment script):
# config.yaml
workflow:
agent_phone_number: "+33612345678"
bot_company: Contoso
bot_name: Robert
lang: {}
communication_service:
phone_number: "+33612345678"
sms: {}
prompts:
llm: {}
tts: {}
Steps to deploy:
- Create an Communication Services resource, a Phone Number with inbound call capability, make sure the resource have a managed identity
- Create the local
config.yaml
file (like the example above) - Connect to your Azure environment (e.g.
az login
) - Run deployment with
make deploy name=my-instance
- Wait for the deployment to finish (if it fails for a
'null' not found
error, retry the command) - Link the AI multi-service account named
[my-instance]-communication
to the Communication Services resource - Create a AI Search index named
trainings
Get the logs with make logs name=my-instance
.
Place a file called config.yaml
in the root of the project with the following content:
# config.yaml
monitoring:
application_insights:
connection_string: xxx
resources:
public_url: "https://xxx.blob.core.windows.net/public"
workflow:
agent_phone_number: "+33612345678"
bot_company: Contoso
bot_name: Robert
communication_service:
access_key: xxx
endpoint: https://xxx.france.communication.azure.com
phone_number: "+33612345678"
cognitive_service:
# Must be of type "AI services multi-service account"
endpoint: https://xxx.cognitiveservices.azure.com
llm:
backup:
mode: azure_openai
azure_openai:
api_key: xxx
context: 16385
deployment: gpt-35-turbo-0125
endpoint: https://xxx.openai.azure.com
model: gpt-35-turbo
streaming: true
primary:
mode: azure_openai
azure_openai:
api_key: xxx
context: 128000
deployment: gpt-4-0125-preview
endpoint: https://xxx.openai.azure.com
model: gpt-4
streaming: true
ai_search:
access_key: xxx
endpoint: https://xxx.search.windows.net
index: trainings
semantic_configuration: default
content_safety:
access_key: xxx
endpoint: https://xxx.cognitiveservices.azure.com
To use a Service Principal to authenticate to Azure, you can also add the following in a .env
file:
AZURE_CLIENT_ID=xxx
AZURE_CLIENT_SECRET=xxx
AZURE_TENANT_ID=xxx
To override a specific configuration value, you can also use environment variables. For example, to override the openai.endpoint
value, you can use the OPENAI__ENDPOINT
variable:
OPENAI__ENDPOINT=https://xxx.openai.azure.com
Then run:
# Install dependencies
make install
Also, a public file server is needed to host the audio files. Upload the files with make copy-resources name=myinstance
(myinstance
is the storage account name), or manually.
For your knowledge, this resources
folder contains:
- Audio files (
xxx.wav
) to be played during the call -
Lexicon file (
lexicon.xml
) to be used by the bot to understand the company products (note: any change makes up to 15 minutes to be taken into account)
Finally, in two different terminals, run:
# Expose the local server to the internet
make tunnel
# Start the local API server
make dev
Training data is stored on AI Search to be retrieved by the bot, on demand.
Required index schema:
Field Name | Type |
Retrievable | Searchable | Dimensions | Vectorizer |
---|---|---|---|---|---|
id | Edm.String |
Yes | No | ||
content | Edm.String |
Yes | Yes | ||
source_uri | Edm.String |
Yes | No | ||
title | Edm.String |
Yes | Yes | ||
vectors | Collection(Edm.Single) |
No | No | 1536 | OpenAI ADA |
An exampe is available at examples/import-training.ipynb
. It shows how to import training data from a PDF files dataset.
Note that prompt examples contains {xxx}
placeholders. These placeholders are replaced by the bot with the corresponding data. For example, {bot_name}
is internally replaced by the bot name.
Be sure to write all the TTS prompts in English. This language is used as a pivot language for the conversation translation.
# config.yaml
[...]
prompts:
tts:
hello_tpl: |
Hello, I'm {bot_name}, from {bot_company}! I'm an IT support specialist.
Here's how I work: when I'm working, you'll hear a little music; then, at the beep, it's your turn to speak. You can speak to me naturally, I'll understand.
Examples:
- "I've got a problem with my computer, it won't turn on".
- "The external screen is flashing, I don't know why".
What's your problem?
llm:
default_system_tpl: |
Assistant is called {bot_name} and is in a call center for the company {bot_company} as an expert with 20 years of experience in IT service.
# Context
Today is {date}. Customer is calling from {phone_number}. Call center number is {bot_phone_number}.
chat_system_tpl: |
# Objective
Assistant will provide internal IT support to employees. Assistant requires data from the employee to provide IT support. The assistant's role is not over until the issue is resolved or the request is fulfilled.
# Rules
- Answers in {default_lang}, even if the customer speaks another language
- Cannot talk about any topic other than IT support
- Is polite, helpful, and professional
- Rephrase the employee's questions as statements and answer them
- Use additional context to enhance the conversation with useful details
- When the employee says a word and then spells out letters, this means that the word is written in the way the employee spelled it (e.g. "I work in Paris PARIS", "My name is John JOHN", "My email is Clemence CLEMENCE at gmail GMAIL dot com COM")
- You work for {bot_company}, not someone else
# Required employee data to be gathered by the assistant
- Department
- Description of the IT issue or request
- Employee name
- Location
# General process to follow
1. Gather information to know the employee's identity (e.g. name, department)
2. Gather details about the IT issue or request to understand the situation (e.g. description, location)
3. Provide initial troubleshooting steps or solutions
4. Gather additional information if needed (e.g. error messages, screenshots)
5. Be proactive and create reminders for follow-up or further assistance
# Support status
{claim}
# Reminders
{reminders}
The bot can be used in multiple languages. It can understand the language the user chose.
See the list of supported languages for the Text-to-Speech service.
# config.yaml
[...]
workflow:
lang:
default_short_code: "fr-FR"
availables:
- pronunciations_en: ["French", "FR", "France"]
short_code: "fr-FR"
voice_name: "fr-FR-DeniseNeural"
- pronunciations_en: ["Chinese", "ZH", "China"]
short_code: "zh-CN"
voice_name: "zh-CN-XiaoxiaoNeural"
Levels are defined for each category of Content Safety. The higher the score, the more strict the moderation is, from 0 to 7.
Moderation is applied on all bot data, including the web page and the conversation.
# config.yaml
[...]
content_safety:
category_hate_score: 0
category_self_harm_score: 0
category_sexual_score: 5
category_violence_score: 0
Customization of the data schema is not supported yet through the configuration file. However, you can customize the data schema by modifying the application source code.
The data schema is defined in models/claim.py
. All the fields are required to be of type Optional[str]
(except the immutable fields).
# models/claim.py
class ClaimModel(BaseModel):
# Immutable fields
# [...]
# Editable fields
additional_notes: Optional[str] = None
device_info: Optional[str] = None
error_messages: Optional[str] = None
follow_up_required: Optional[bool] = None
incident_date_time: Optional[datetime] = None
issue_description: Optional[str] = None
resolution_details: Optional[str] = None
steps_taken: Optional[str] = None
ticket_id: Optional[str] = None
user_email: Optional[EmailStr] = None
user_name: Optional[str] = None
user_phone: Optional[PhoneNumber] = None
# Depending on requirements, you might also include fields for:
# - Software version
# - Operating system
# - Network details (if relevant to the issue)
# - Any attachments like screenshots or log files (consider how to handle binary data)
# Built-in functions
[...]
To use a model compatible with the OpenAI completion API, you need to create an account and get the following information:
- API key
- Context window size
- Endpoint URL
- Model name
- Streaming capability
Then, add the following in the config.yaml
file:
# config.yaml
[...]
llm:
backup:
mode: openai
openai:
api_key: xxx
context: 16385
endpoint: https://api.openai.com
model: gpt-35-turbo
streaming: true
primary:
mode: openai
openai:
api_key: xxx
context: 128000
endpoint: https://api.openai.com
model: gpt-4
streaming: true
To use Twilio for SMS, you need to create an account and get the following information:
- Account SID
- Auth Token
- Phone number
Then, add the following in the config.yaml
file:
# config.yaml
[...]
sms:
mode: twilio
twilio:
account_sid: xxx
auth_token: xxx
phone_number: "+33612345678"
For Tasks:
Click tags to check more tools for each tasksFor Jobs:
Alternative AI tools for claim-ai-phone-bot
Similar Open Source Tools
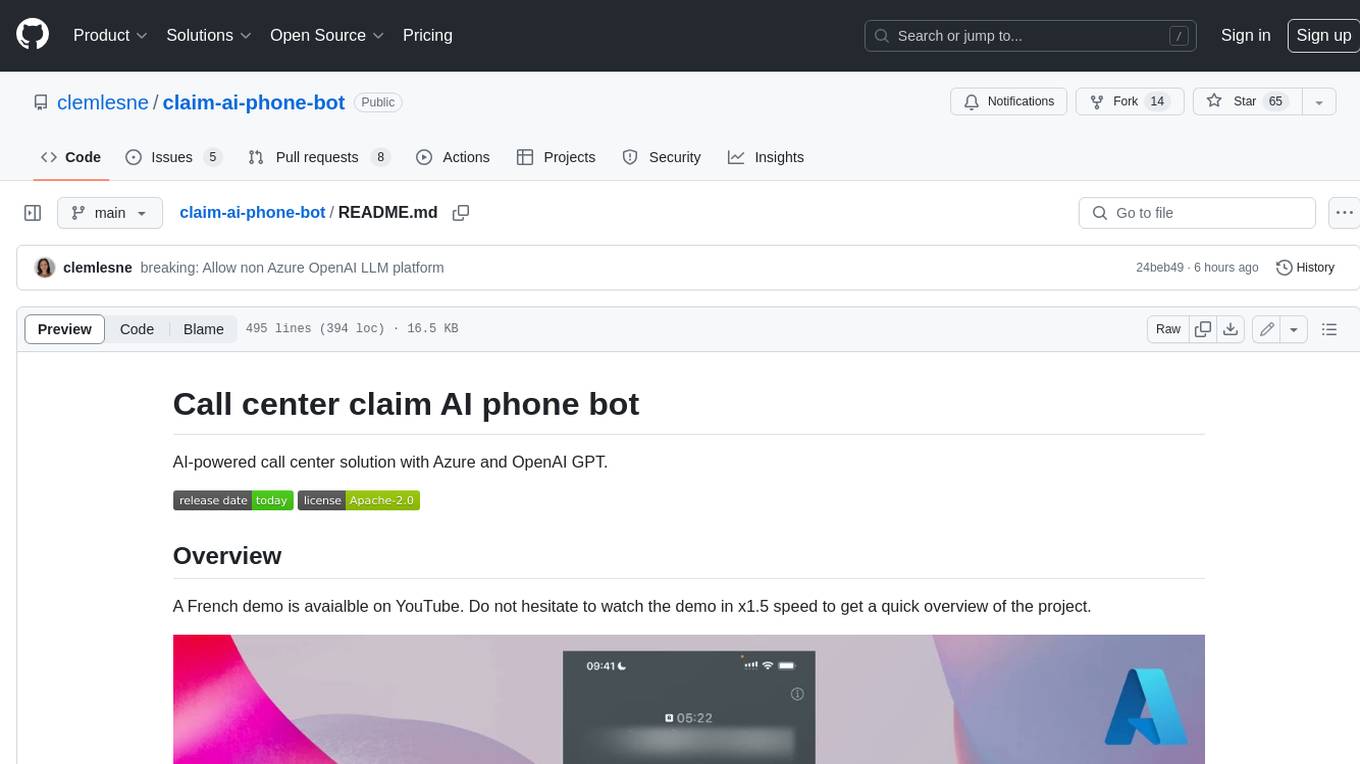
claim-ai-phone-bot
AI-powered call center solution with Azure and OpenAI GPT. The bot can answer calls, understand the customer's request, and provide relevant information or assistance. It can also create a todo list of tasks to complete the claim, and send a report after the call. The bot is customizable, and can be used in multiple languages.
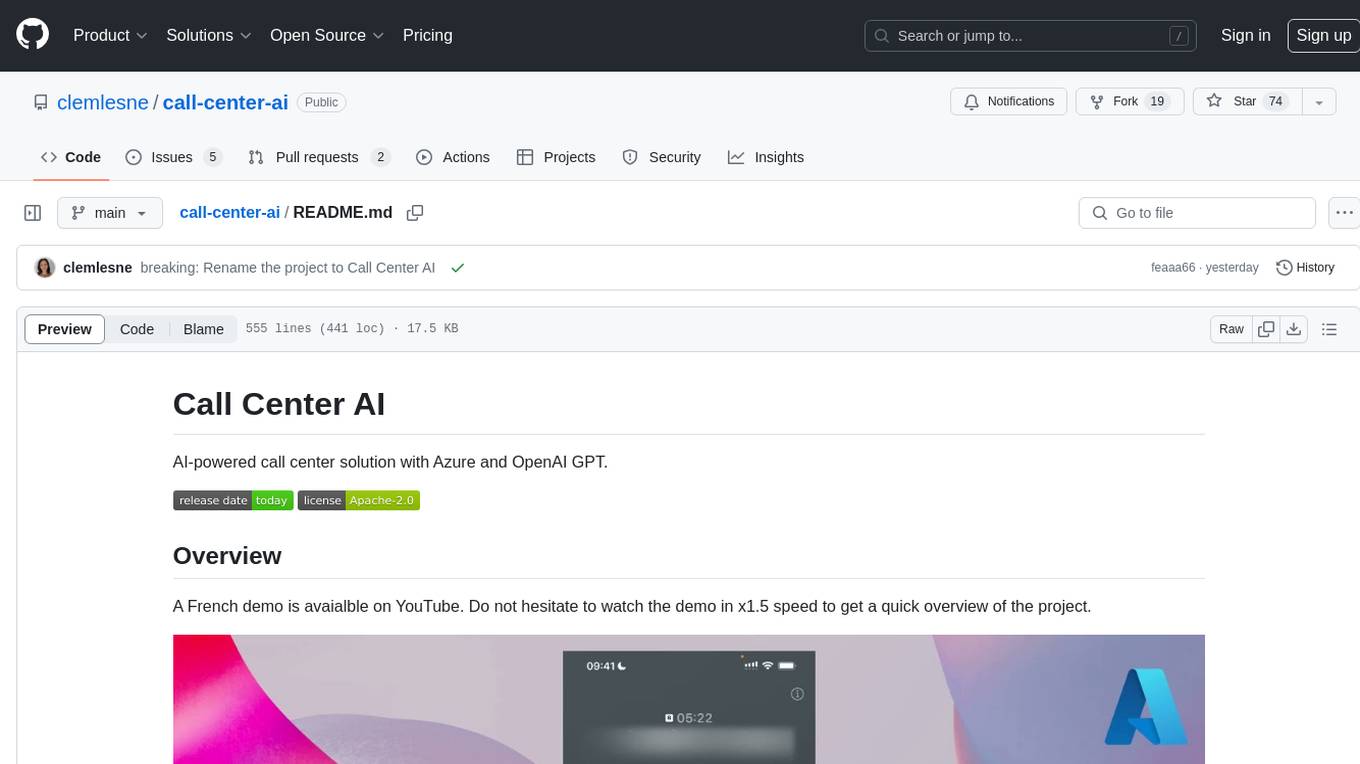
call-center-ai
Call Center AI is an AI-powered call center solution that leverages Azure and OpenAI GPT. It is a proof of concept demonstrating the integration of Azure Communication Services, Azure Cognitive Services, and Azure OpenAI to build an automated call center solution. The project showcases features like accessing claims on a public website, customer conversation history, language change during conversation, bot interaction via phone number, multiple voice tones, lexicon understanding, todo list creation, customizable prompts, content filtering, GPT-4 Turbo for customer requests, specific data schema for claims, documentation database access, SMS report sending, conversation resumption, and more. The system architecture includes components like RAG AI Search, SMS gateway, call gateway, moderation, Cosmos DB, event broker, GPT-4 Turbo, Redis cache, translation service, and more. The tool can be deployed remotely using GitHub Actions and locally with prerequisites like Azure environment setup, configuration file creation, and resource hosting. Advanced usage includes custom training data with AI Search, prompt customization, language customization, moderation level customization, claim data schema customization, OpenAI compatible model usage for the LLM, and Twilio integration for SMS.
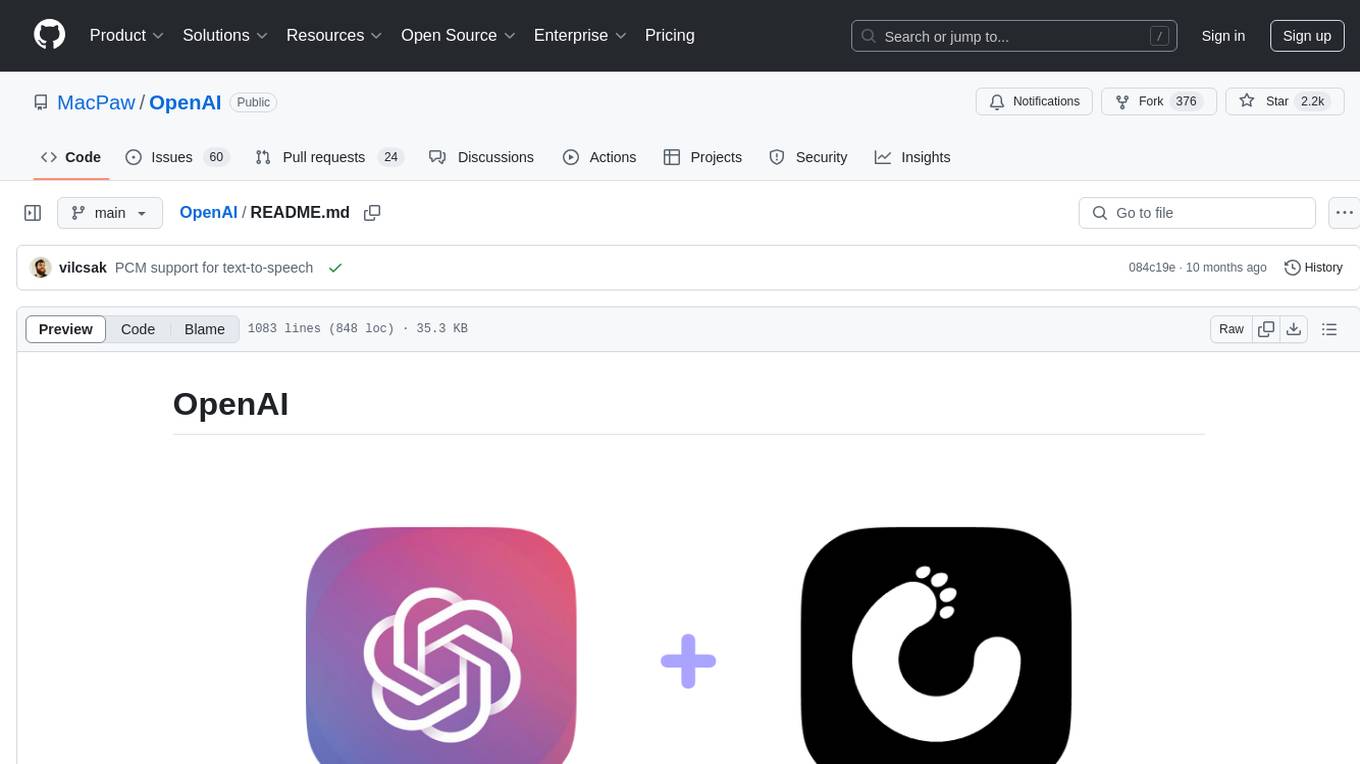
OpenAI
OpenAI is a Swift community-maintained implementation over OpenAI public API. It is a non-profit artificial intelligence research organization founded in San Francisco, California in 2015. OpenAI's mission is to ensure safe and responsible use of AI for civic good, economic growth, and other public benefits. The repository provides functionalities for text completions, chats, image generation, audio processing, edits, embeddings, models, moderations, utilities, and Combine extensions.
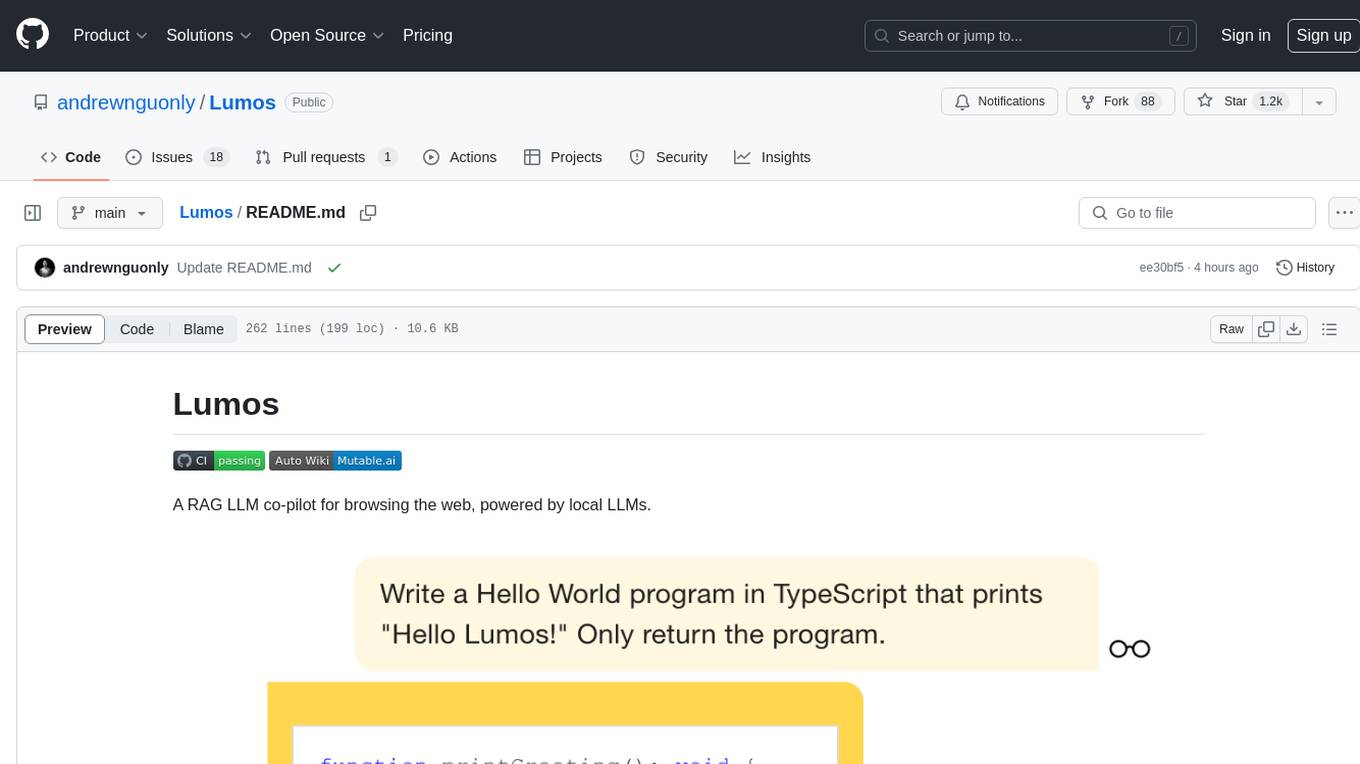
Lumos
Lumos is a Chrome extension powered by a local LLM co-pilot for browsing the web. It allows users to summarize long threads, news articles, and technical documentation. Users can ask questions about reviews and product pages. The tool requires a local Ollama server for LLM inference and embedding database. Lumos supports multimodal models and file attachments for processing text and image content. It also provides options to customize models, hosts, and content parsers. The extension can be easily accessed through keyboard shortcuts and offers tools for automatic invocation based on prompts.
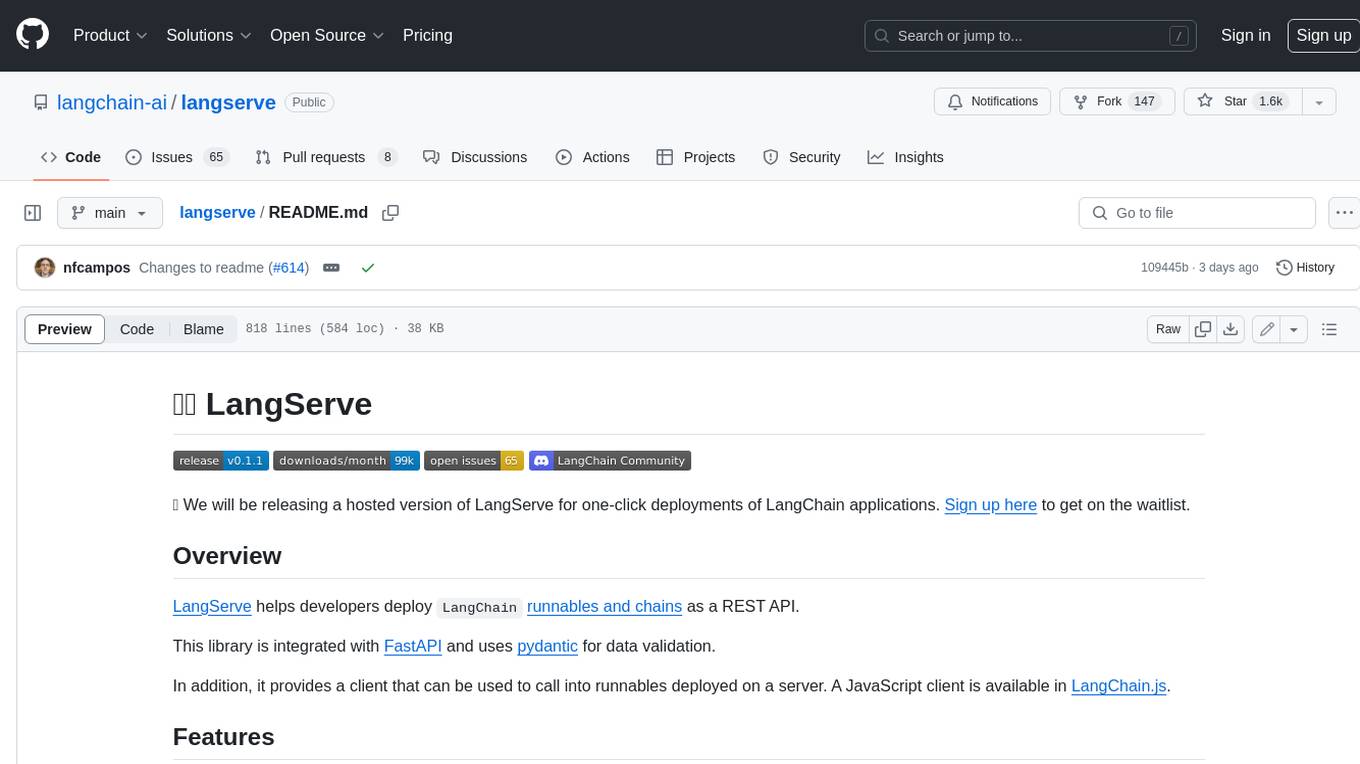
langserve
LangServe helps developers deploy `LangChain` runnables and chains as a REST API. This library is integrated with FastAPI and uses pydantic for data validation. In addition, it provides a client that can be used to call into runnables deployed on a server. A JavaScript client is available in LangChain.js.
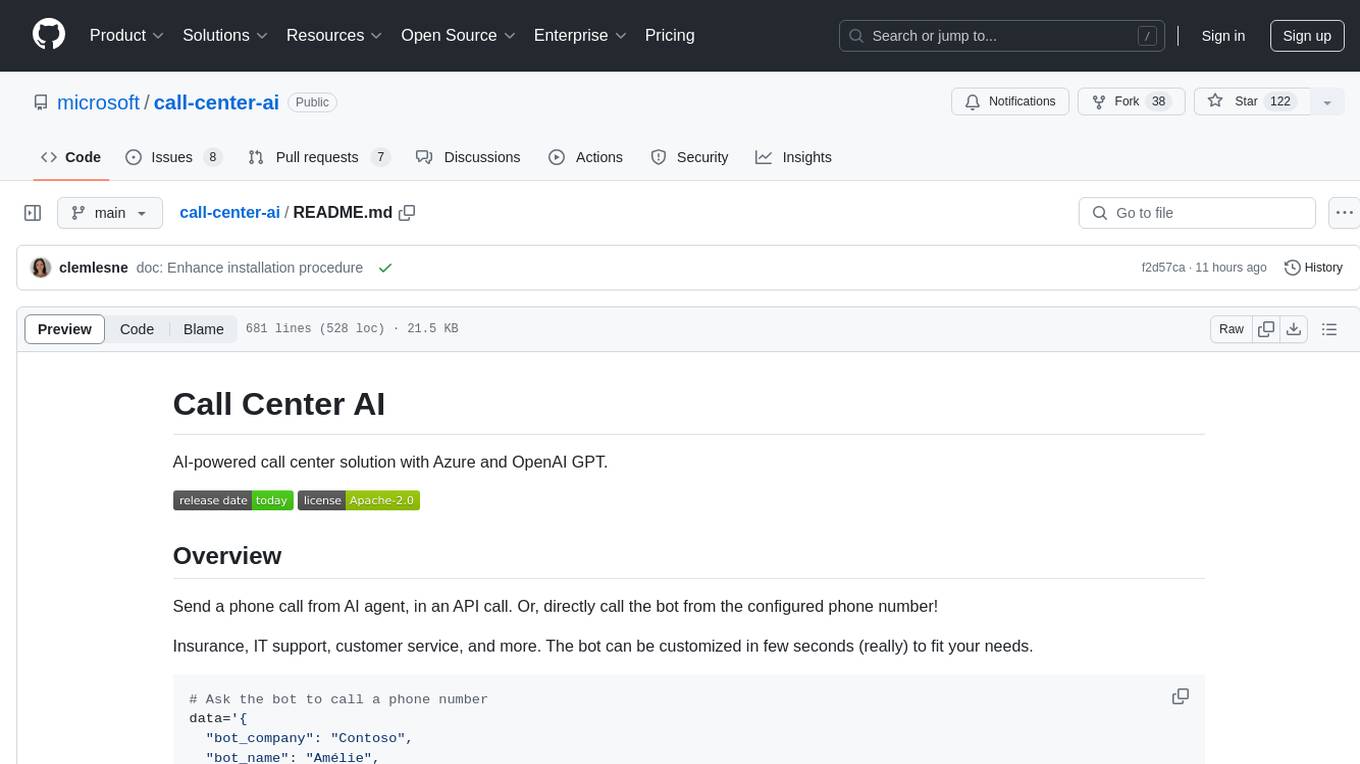
call-center-ai
Call Center AI is an AI-powered call center solution leveraging Azure and OpenAI GPT. It allows for AI agent-initiated phone calls or direct calls to the bot from a configured phone number. The bot is customizable for various industries like insurance, IT support, and customer service, with features such as accessing claim information, conversation history, language change, SMS sending, and more. The project is a proof of concept showcasing the integration of Azure Communication Services, Azure Cognitive Services, and Azure OpenAI for an automated call center solution.
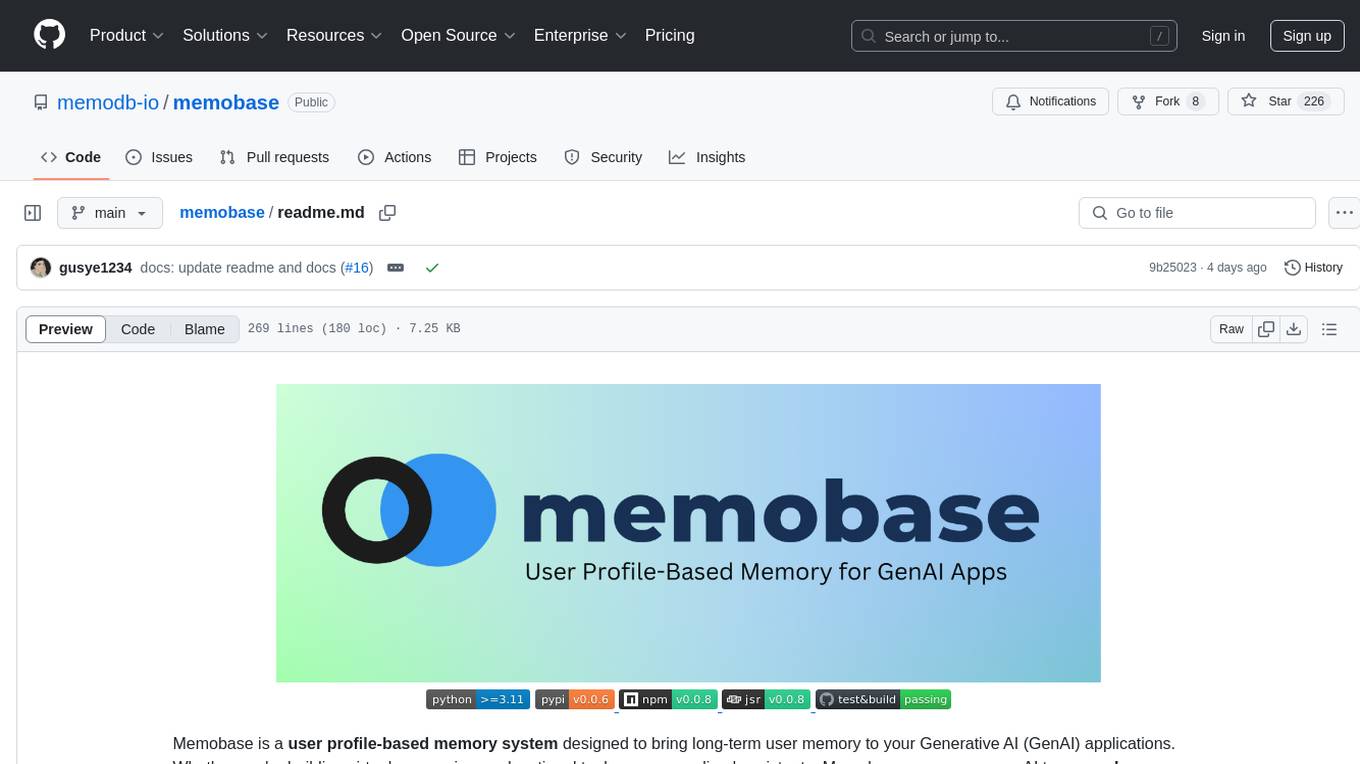
memobase
Memobase is a user profile-based memory system designed to enhance Generative AI applications by enabling them to remember, understand, and evolve with users. It provides structured user profiles, scalable profiling, easy integration with existing LLM stacks, batch processing for speed, and is production-ready. Users can manage users, insert data, get memory profiles, and track user preferences and behaviors. Memobase is ideal for applications that require user analysis, tracking, and personalized interactions.
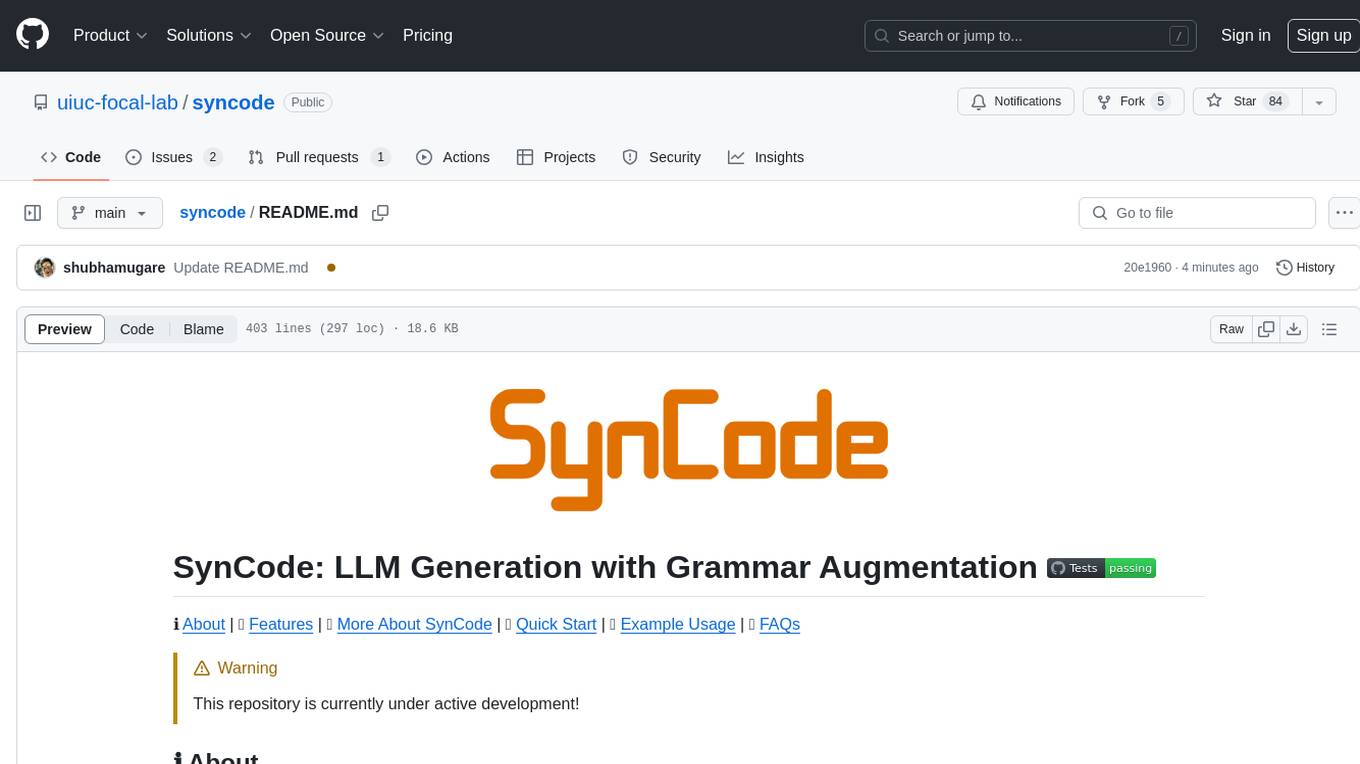
syncode
SynCode is a novel framework for the grammar-guided generation of Large Language Models (LLMs) that ensures syntactically valid output with respect to defined Context-Free Grammar (CFG) rules. It supports general-purpose programming languages like Python, Go, SQL, JSON, and more, allowing users to define custom grammars using EBNF syntax. The tool compares favorably to other constrained decoders and offers features like fast grammar-guided generation, compatibility with HuggingFace Language Models, and the ability to work with various decoding strategies.
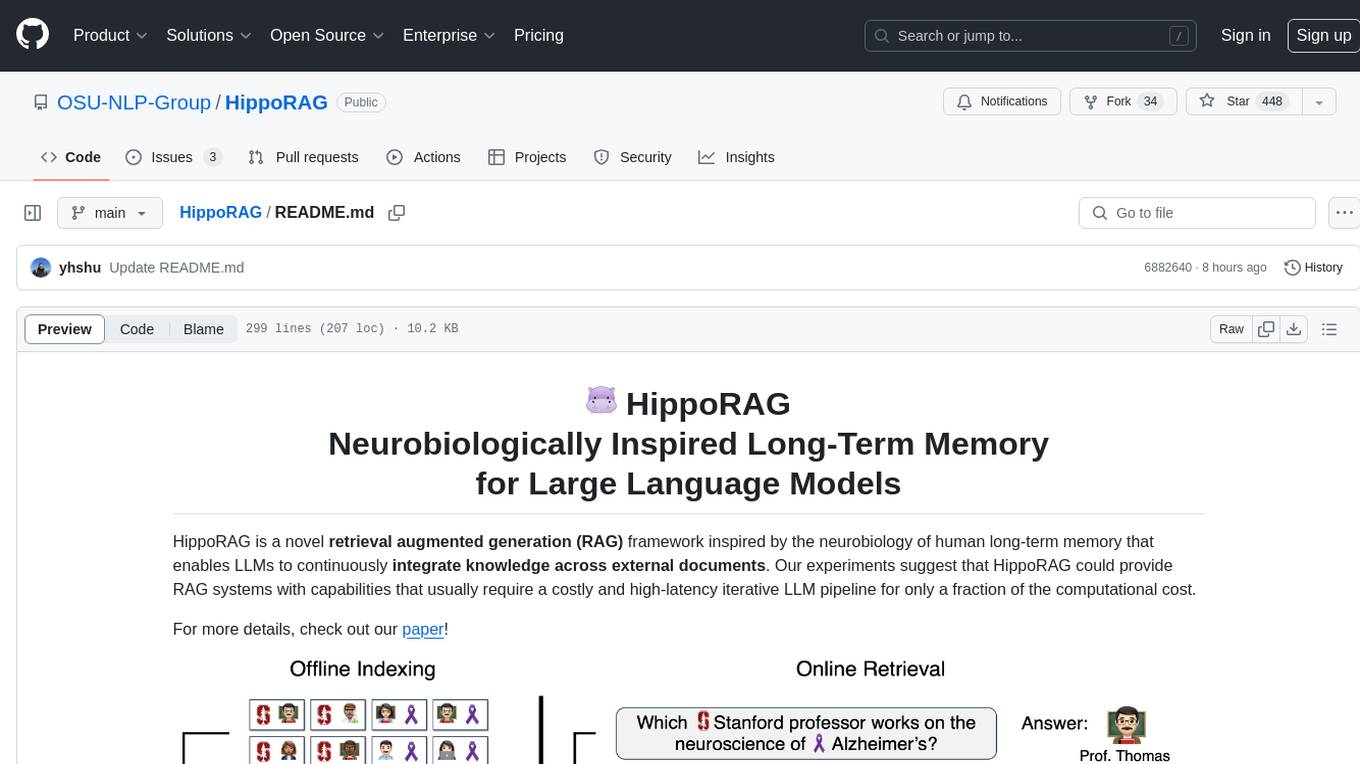
HippoRAG
HippoRAG is a novel retrieval augmented generation (RAG) framework inspired by the neurobiology of human long-term memory that enables Large Language Models (LLMs) to continuously integrate knowledge across external documents. It provides RAG systems with capabilities that usually require a costly and high-latency iterative LLM pipeline for only a fraction of the computational cost. The tool facilitates setting up retrieval corpus, indexing, and retrieval processes for LLMs, offering flexibility in choosing different online LLM APIs or offline LLM deployments through LangChain integration. Users can run retrieval on pre-defined queries or integrate directly with the HippoRAG API. The tool also supports reproducibility of experiments and provides data, baselines, and hyperparameter tuning scripts for research purposes.
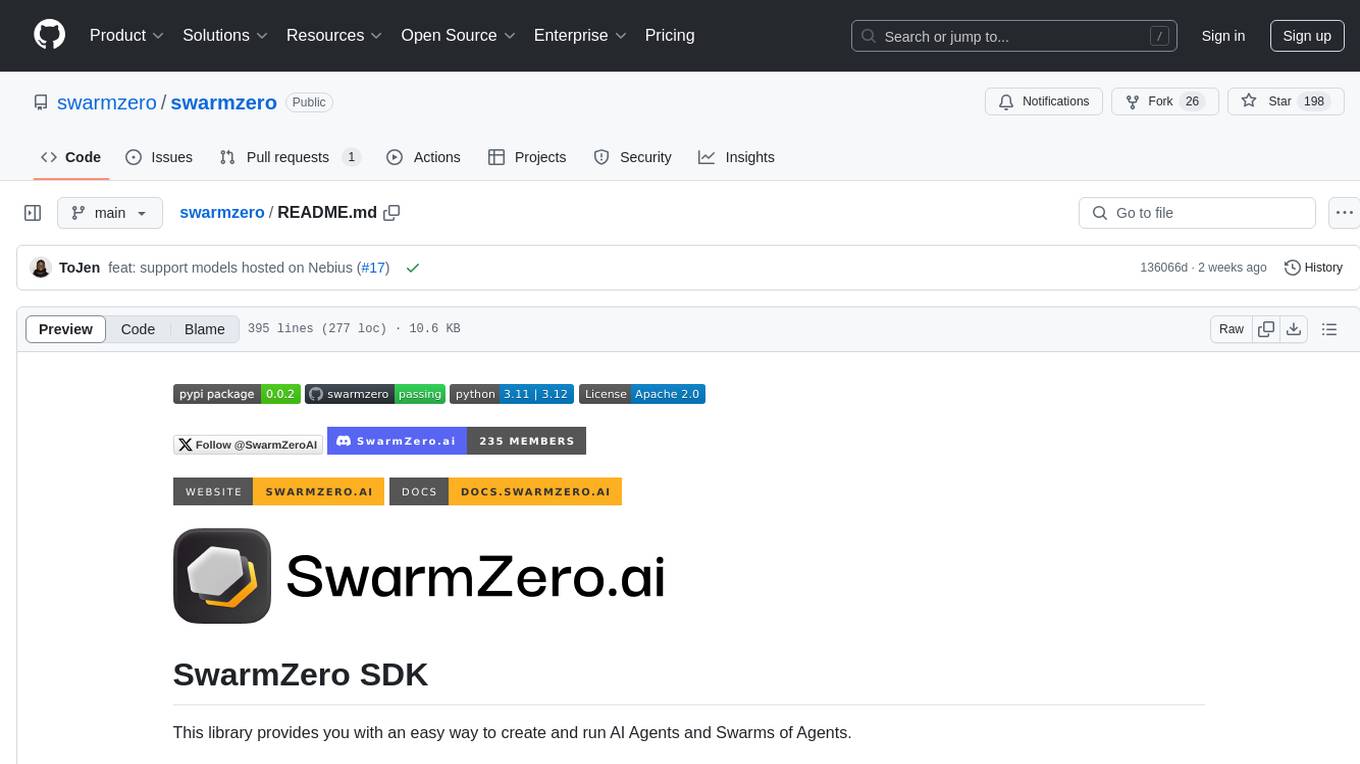
swarmzero
SwarmZero SDK is a library that simplifies the creation and execution of AI Agents and Swarms of Agents. It supports various LLM Providers such as OpenAI, Azure OpenAI, Anthropic, MistralAI, Gemini, Nebius, and Ollama. Users can easily install the library using pip or poetry, set up the environment and configuration, create and run Agents, collaborate with Swarms, add tools for complex tasks, and utilize retriever tools for semantic information retrieval. Sample prompts are provided to help users explore the capabilities of the agents and swarms. The SDK also includes detailed examples and documentation for reference.
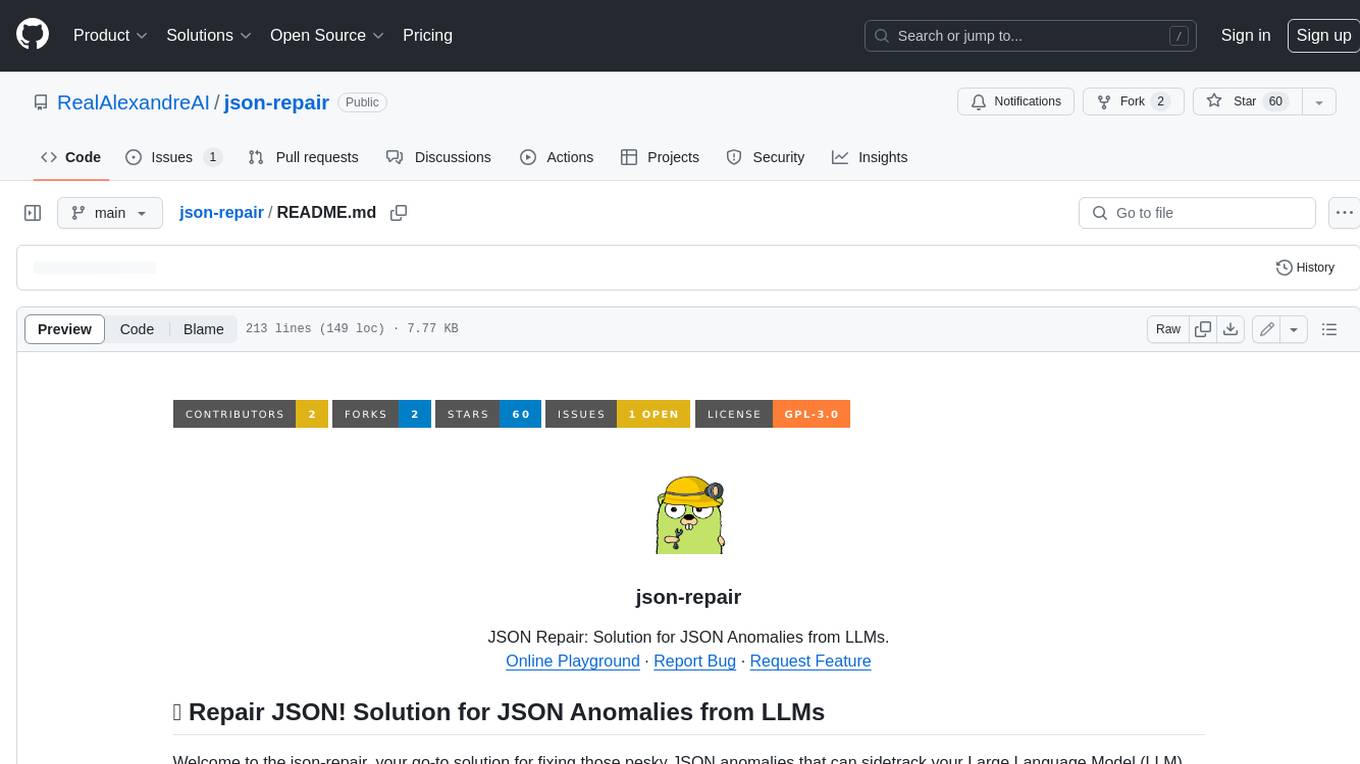
json-repair
JSON Repair is a toolkit designed to address JSON anomalies that can arise from Large Language Models (LLMs). It offers a comprehensive solution for repairing JSON strings, ensuring accuracy and reliability in your data processing. With its user-friendly interface and extensive capabilities, JSON Repair empowers developers to seamlessly integrate JSON repair into their workflows.
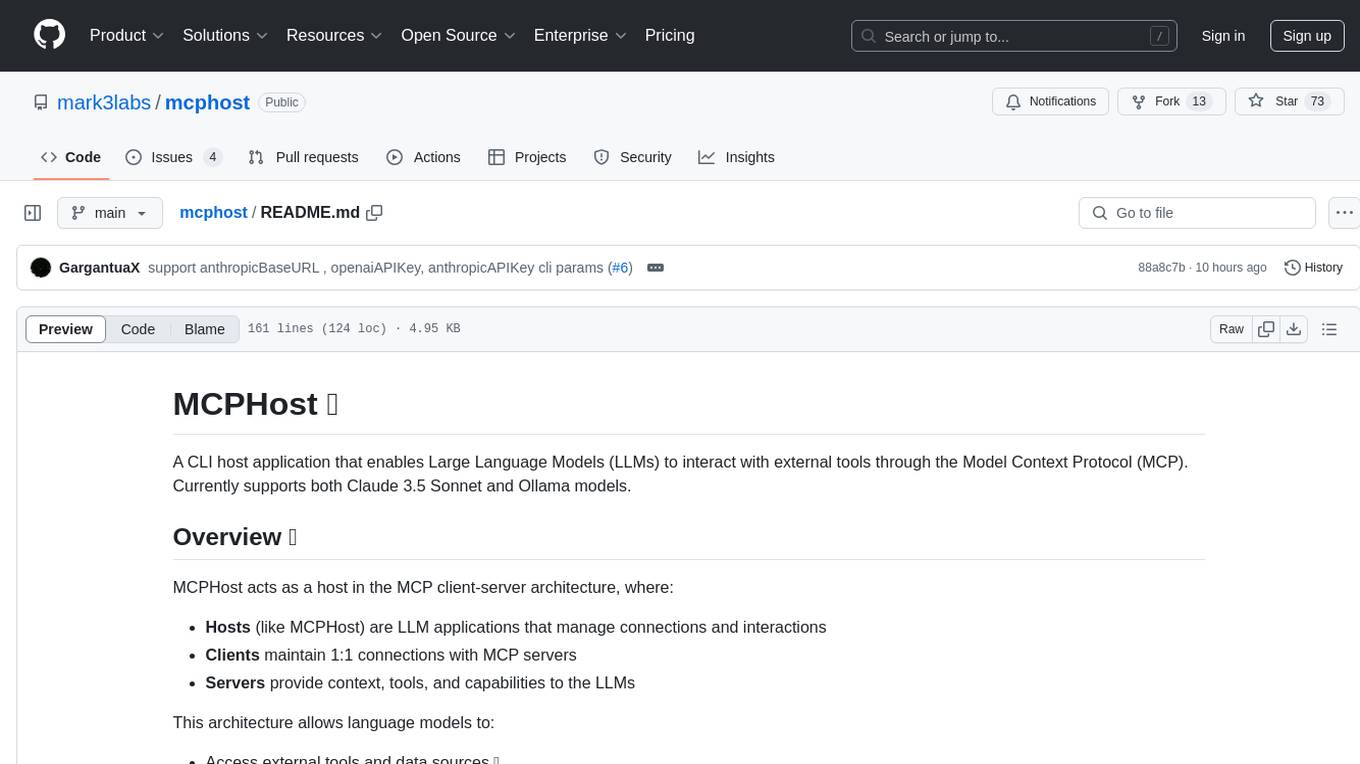
mcphost
MCPHost is a CLI host application that enables Large Language Models (LLMs) to interact with external tools through the Model Context Protocol (MCP). It acts as a host in the MCP client-server architecture, allowing language models to access external tools and data sources, maintain consistent context across interactions, and execute commands safely. The tool supports interactive conversations with Claude 3.5 Sonnet and Ollama models, multiple concurrent MCP servers, dynamic tool discovery and integration, configurable server locations and arguments, and a consistent command interface across model types.
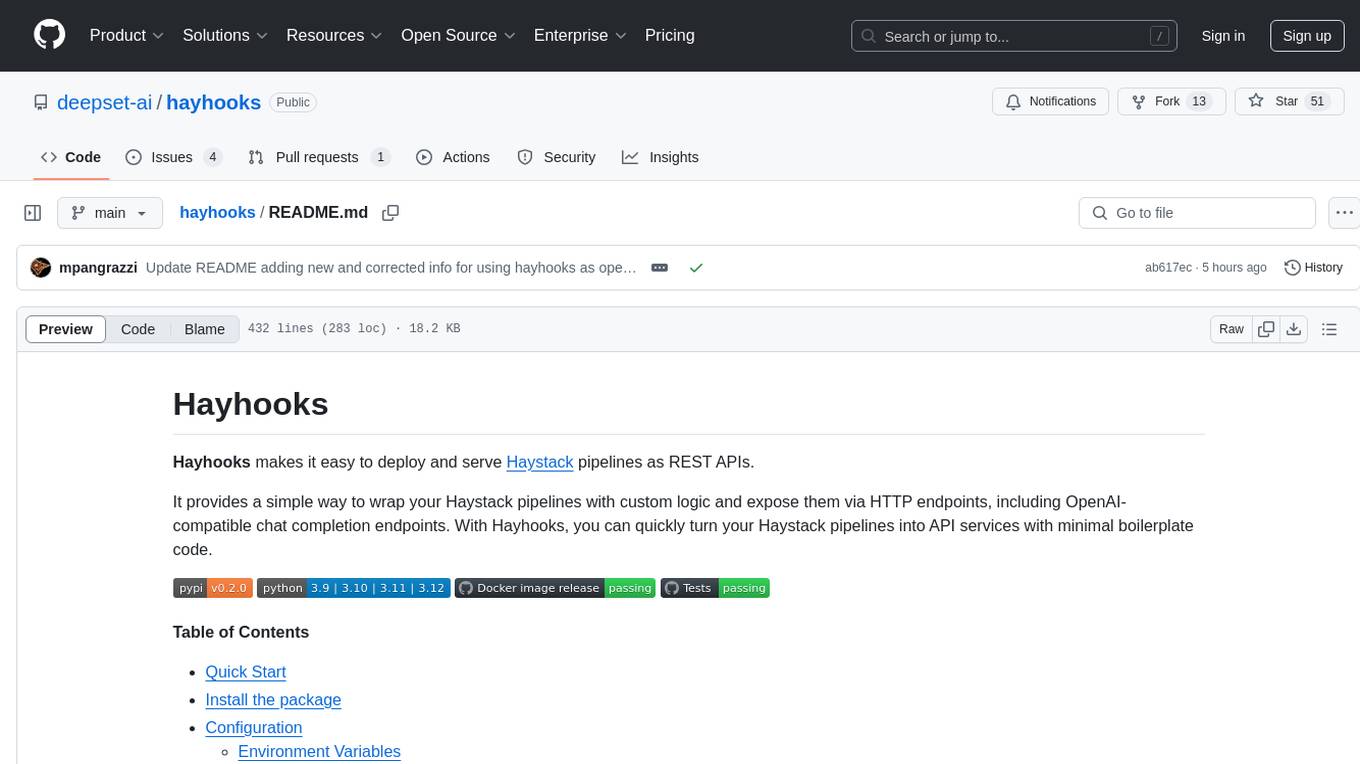
hayhooks
Hayhooks is a tool that simplifies the deployment and serving of Haystack pipelines as REST APIs. It allows users to wrap their pipelines with custom logic and expose them via HTTP endpoints, including OpenAI-compatible chat completion endpoints. With Hayhooks, users can easily convert their Haystack pipelines into API services with minimal boilerplate code.
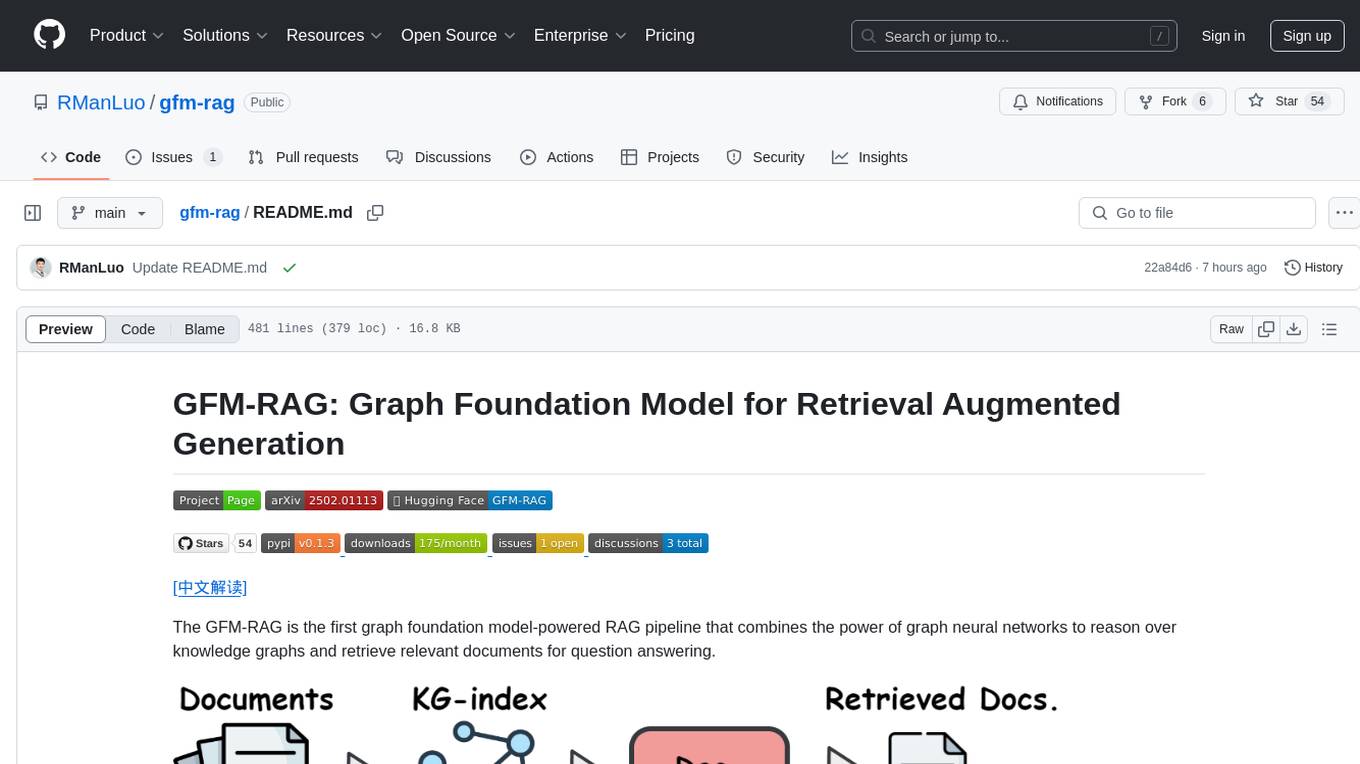
gfm-rag
The GFM-RAG is a graph foundation model-powered pipeline that combines graph neural networks to reason over knowledge graphs and retrieve relevant documents for question answering. It features a knowledge graph index, efficiency in multi-hop reasoning, generalizability to unseen datasets, transferability for fine-tuning, compatibility with agent-based frameworks, and interpretability of reasoning paths. The tool can be used for conducting retrieval and question answering tasks using pre-trained models or fine-tuning on custom datasets.
For similar tasks
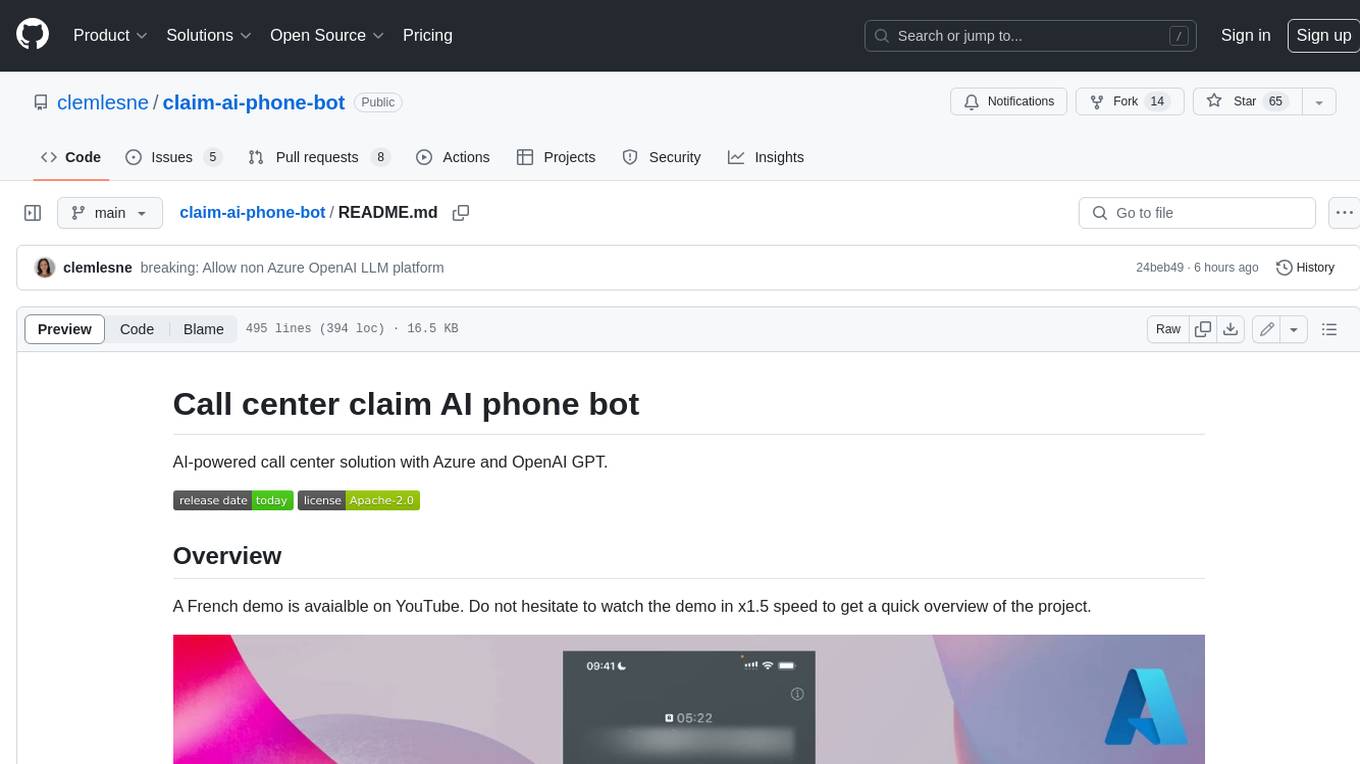
claim-ai-phone-bot
AI-powered call center solution with Azure and OpenAI GPT. The bot can answer calls, understand the customer's request, and provide relevant information or assistance. It can also create a todo list of tasks to complete the claim, and send a report after the call. The bot is customizable, and can be used in multiple languages.
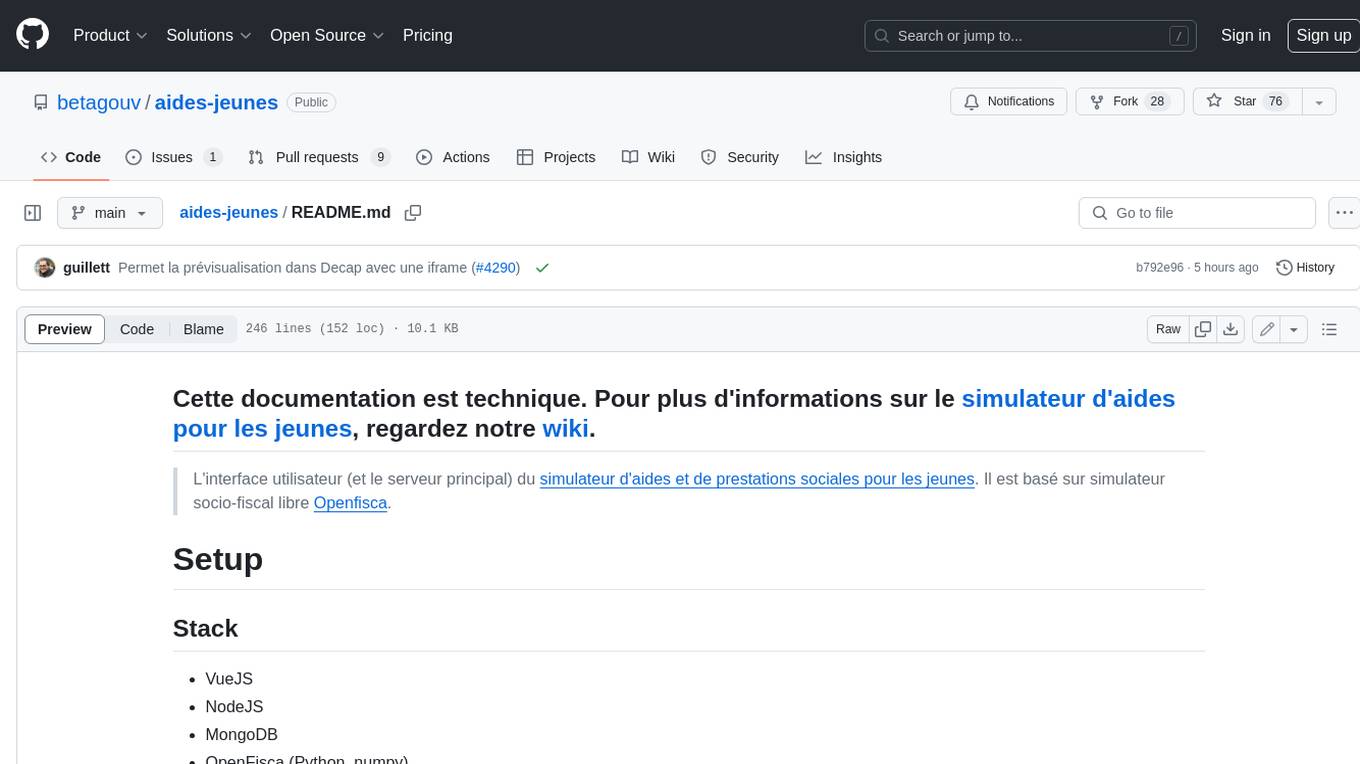
aides-jeunes
The user interface (and the main server) of the simulator of aids and social benefits for young people. It is based on the free socio-fiscal simulator Openfisca.
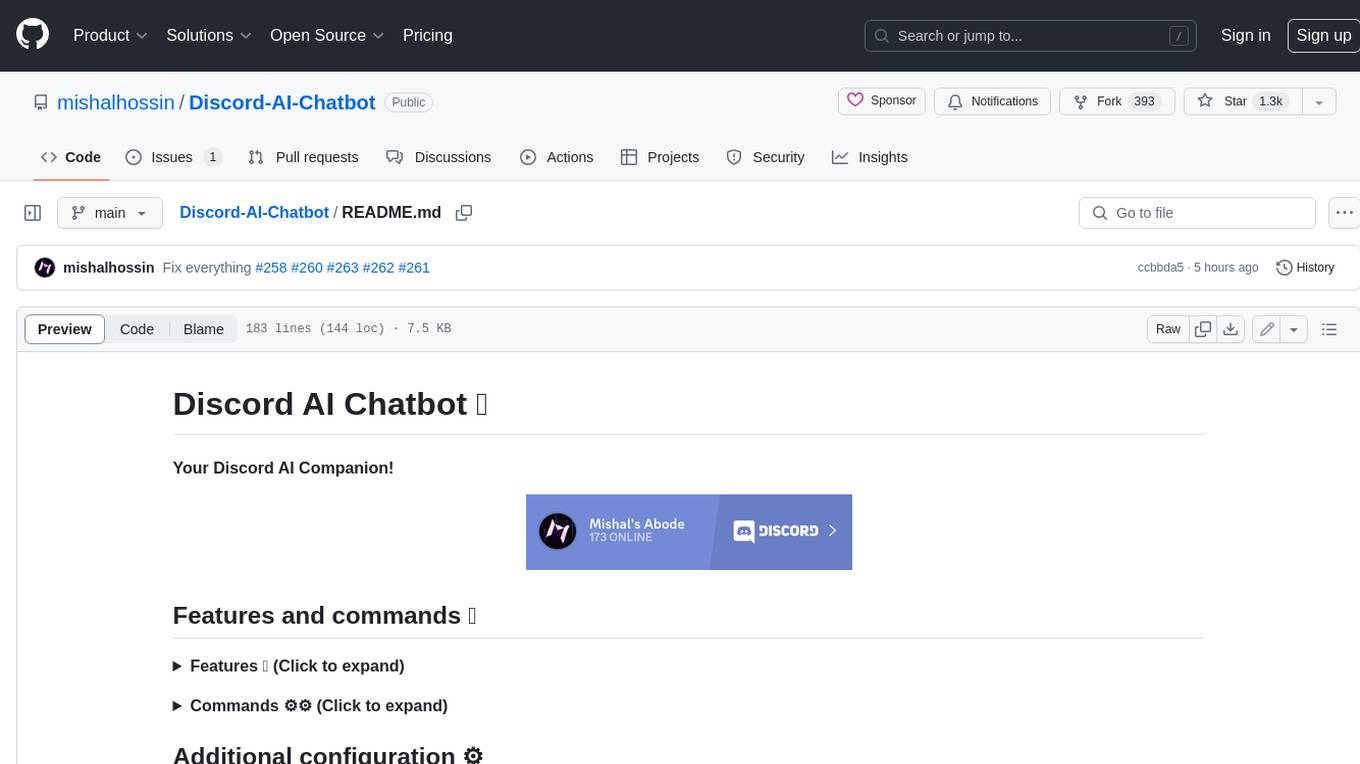
Discord-AI-Chatbot
Discord AI Chatbot is a versatile tool that seamlessly integrates into your Discord server, offering a wide range of capabilities to enhance your communication and engagement. With its advanced language model, the bot excels at imaginative generation, providing endless possibilities for creative expression. Additionally, it offers secure credential management, ensuring the privacy of your data. The bot's hybrid command system combines the best of slash and normal commands, providing flexibility and ease of use. It also features mention recognition, ensuring prompt responses whenever you mention it or use its name. The bot's message handling capabilities prevent confusion by recognizing when you're replying to others. You can customize the bot's behavior by selecting from a range of pre-existing personalities or creating your own. The bot's web access feature unlocks a new level of convenience, allowing you to interact with it from anywhere. With its open-source nature, you have the freedom to modify and adapt the bot to your specific needs.
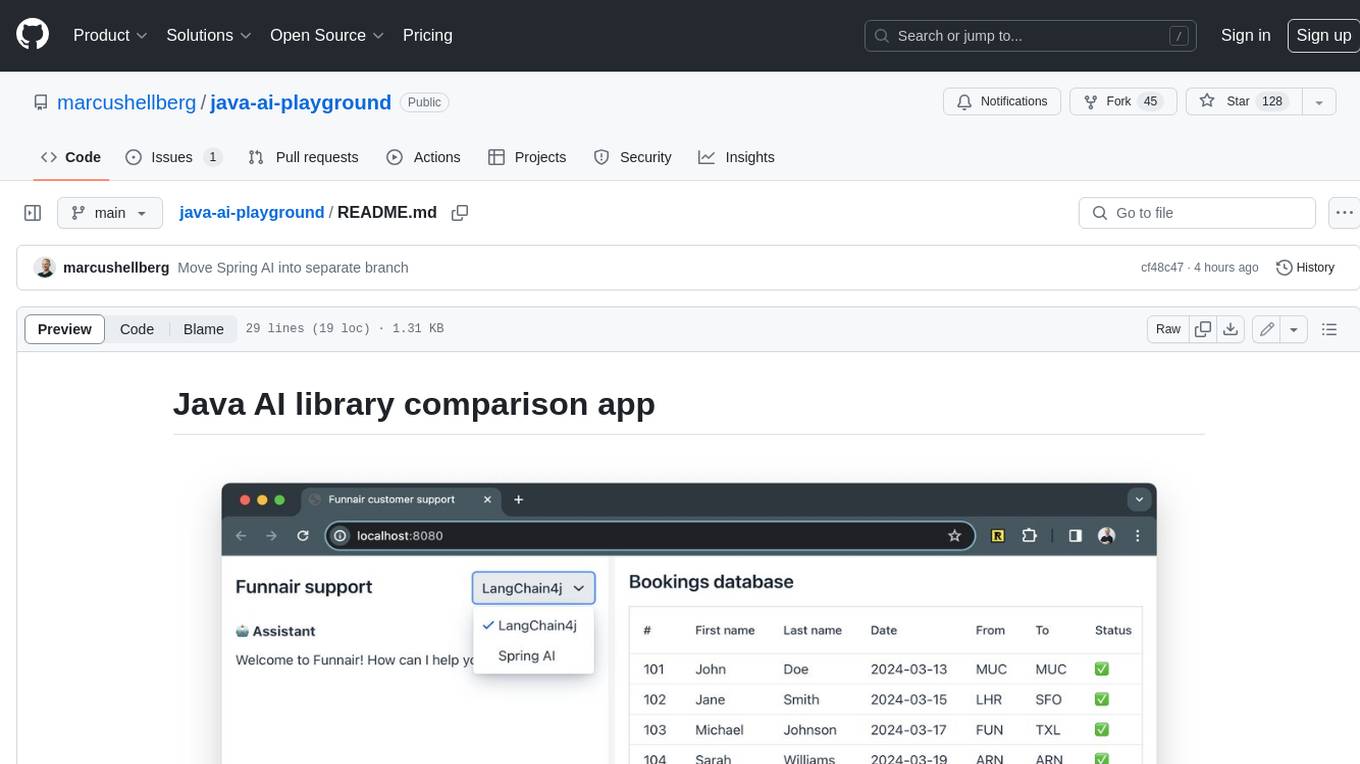
java-ai-playground
This AI-powered customer support application has access to terms and conditions (retrieval augmented generation, RAG), can access tools (Java methods) to perform actions, and uses an LLM to interact with the user. The application includes implementations for LangChain4j in the `main` branch and Spring AI in the `spring-ai` branch. The UI is built using Vaadin Hilla and the backend is built using Spring Boot.
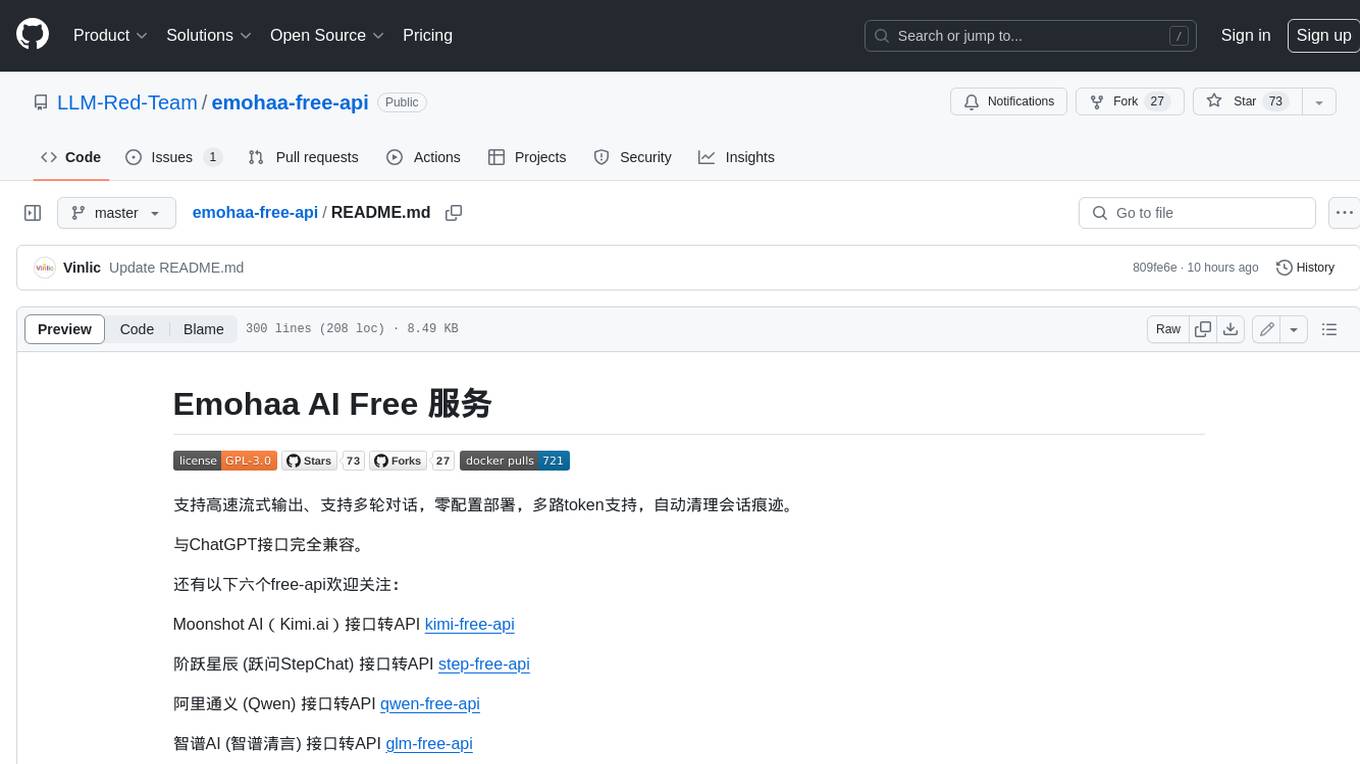
emohaa-free-api
Emohaa AI Free API is a free API that allows you to access the Emohaa AI chatbot. Emohaa AI is a powerful chatbot that can understand and respond to a wide range of natural language queries. It can be used for a variety of purposes, such as customer service, information retrieval, and language translation. The Emohaa AI Free API is easy to use and can be integrated into any application. It is a great way to add AI capabilities to your projects without having to build your own chatbot from scratch.
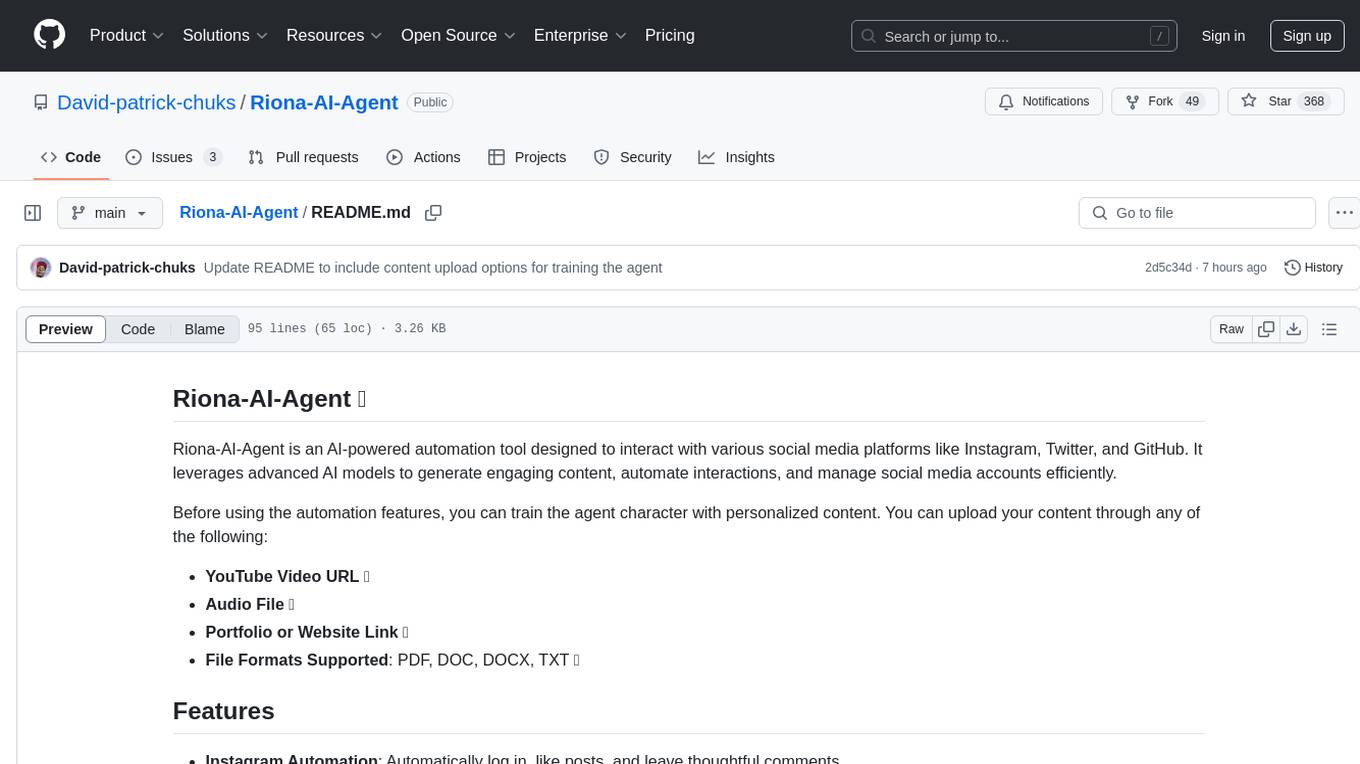
Riona-AI-Agent
Riona-AI-Agent is a versatile AI chatbot designed to assist users in various tasks. It utilizes natural language processing and machine learning algorithms to understand user queries and provide accurate responses. The chatbot can be integrated into websites, applications, and messaging platforms to enhance user experience and streamline communication. With its customizable features and easy deployment, Riona-AI-Agent is suitable for businesses, developers, and individuals looking to automate customer support, provide information, and engage with users in a conversational manner.
For similar jobs
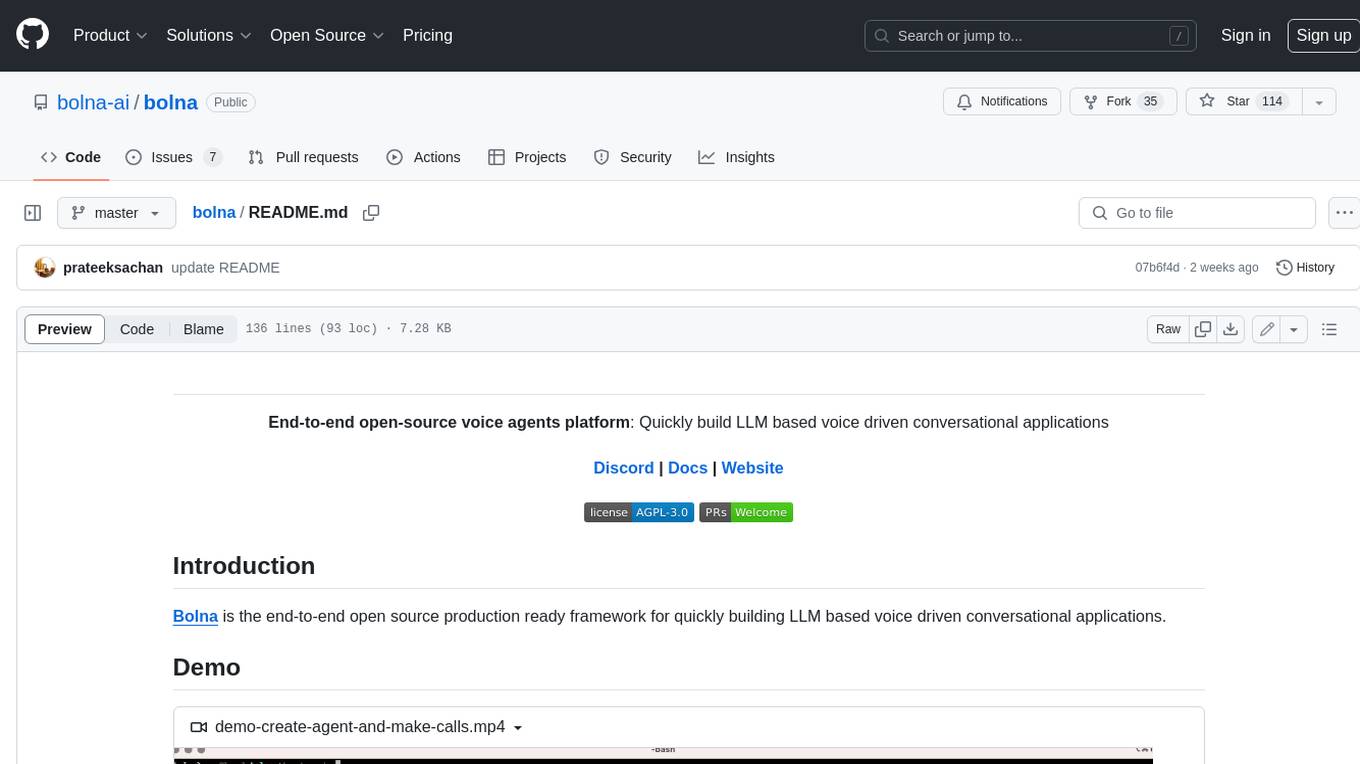
bolna
Bolna is an open-source platform for building voice-driven conversational applications using large language models (LLMs). It provides a comprehensive set of tools and integrations to handle various aspects of voice-based interactions, including telephony, transcription, LLM-based conversation handling, and text-to-speech synthesis. Bolna simplifies the process of creating voice agents that can perform tasks such as initiating phone calls, transcribing conversations, generating LLM-powered responses, and synthesizing speech. It supports multiple providers for each component, allowing users to customize their setup based on their specific needs. Bolna is designed to be easy to use, with a straightforward local setup process and well-documented APIs. It is also extensible, enabling users to integrate with other telephony providers or add custom functionality.
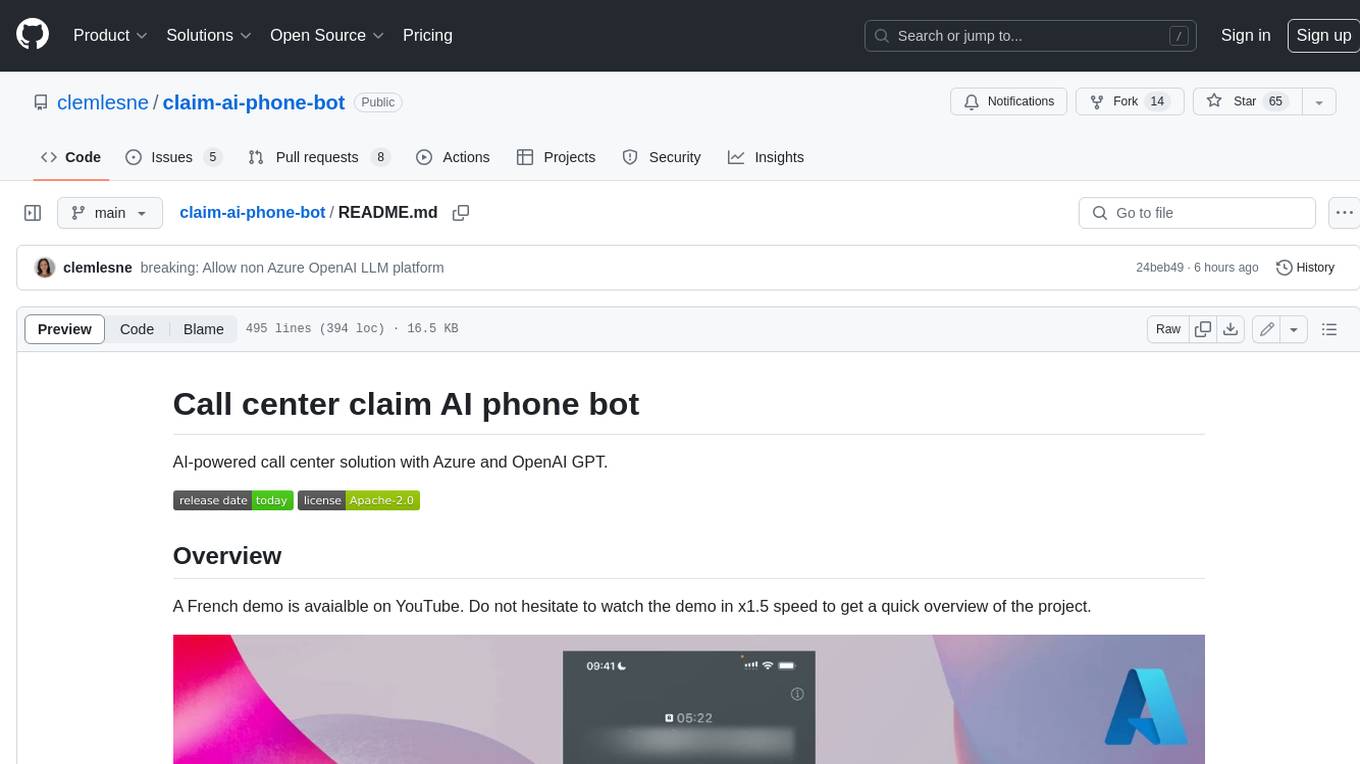
claim-ai-phone-bot
AI-powered call center solution with Azure and OpenAI GPT. The bot can answer calls, understand the customer's request, and provide relevant information or assistance. It can also create a todo list of tasks to complete the claim, and send a report after the call. The bot is customizable, and can be used in multiple languages.
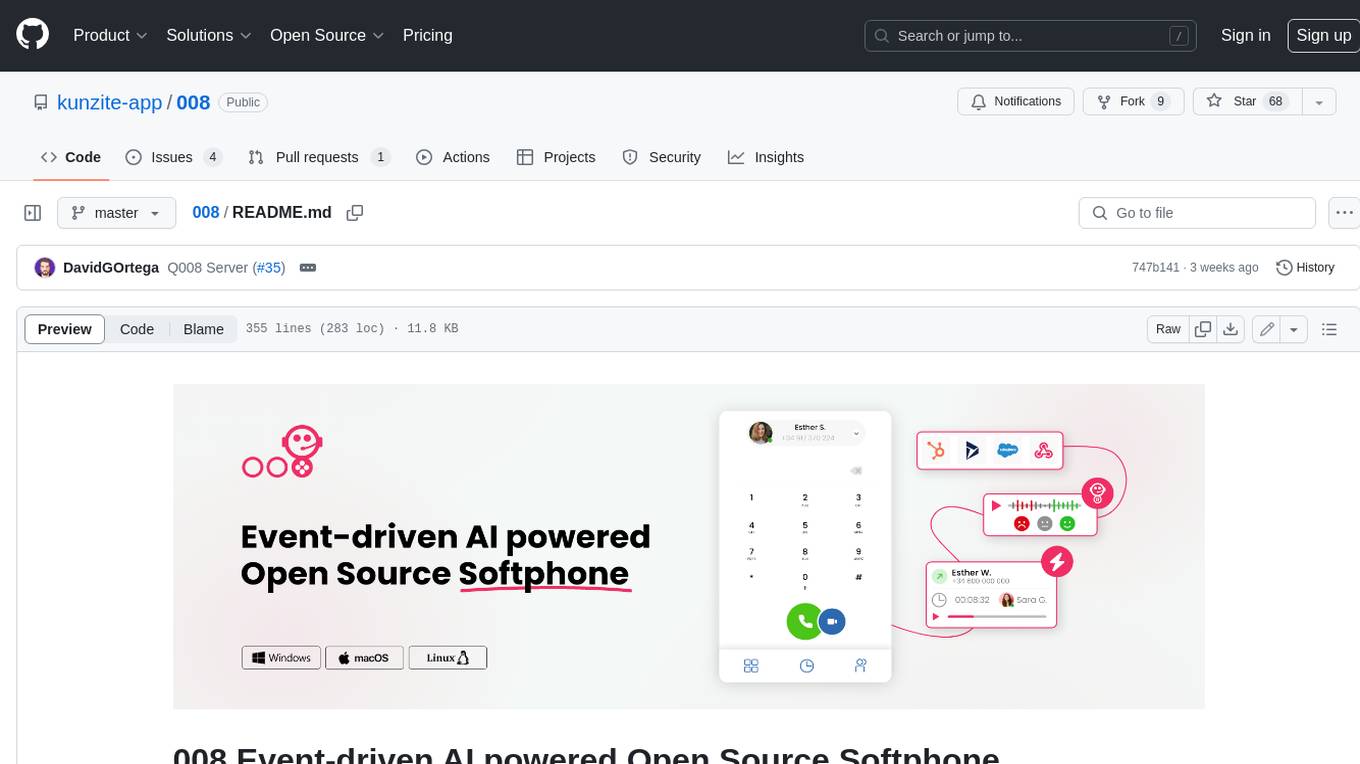
008
008 is an open-source event-driven AI powered WebRTC Softphone compatible with macOS, Windows, and Linux. It is also accessible on the web. The name '008' or 'agent 008' reflects our ambition: beyond crafting the premier Open Source Softphone, we aim to introduce a programmable, event-driven AI agent. This agent utilizes embedded artificial intelligence models operating directly on the softphone, ensuring efficiency and reduced operational costs.
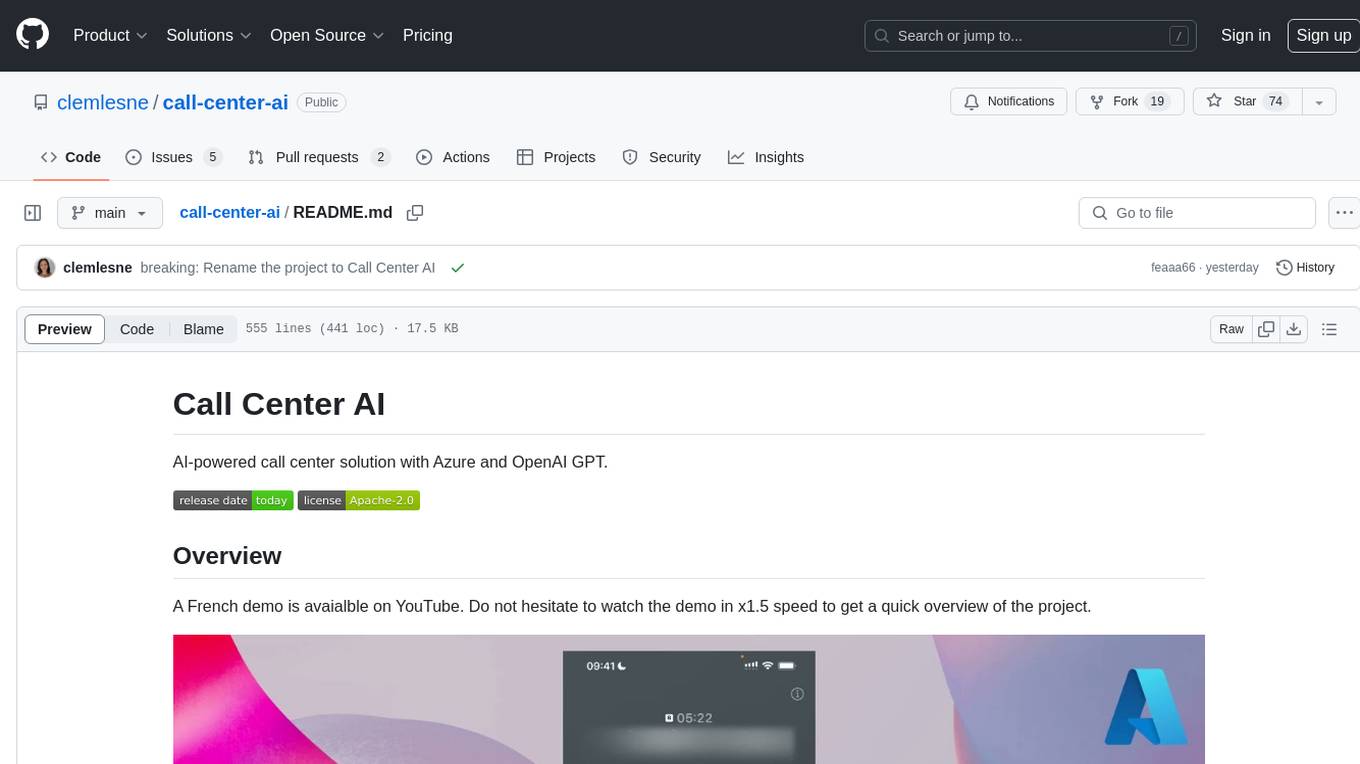
call-center-ai
Call Center AI is an AI-powered call center solution that leverages Azure and OpenAI GPT. It is a proof of concept demonstrating the integration of Azure Communication Services, Azure Cognitive Services, and Azure OpenAI to build an automated call center solution. The project showcases features like accessing claims on a public website, customer conversation history, language change during conversation, bot interaction via phone number, multiple voice tones, lexicon understanding, todo list creation, customizable prompts, content filtering, GPT-4 Turbo for customer requests, specific data schema for claims, documentation database access, SMS report sending, conversation resumption, and more. The system architecture includes components like RAG AI Search, SMS gateway, call gateway, moderation, Cosmos DB, event broker, GPT-4 Turbo, Redis cache, translation service, and more. The tool can be deployed remotely using GitHub Actions and locally with prerequisites like Azure environment setup, configuration file creation, and resource hosting. Advanced usage includes custom training data with AI Search, prompt customization, language customization, moderation level customization, claim data schema customization, OpenAI compatible model usage for the LLM, and Twilio integration for SMS.
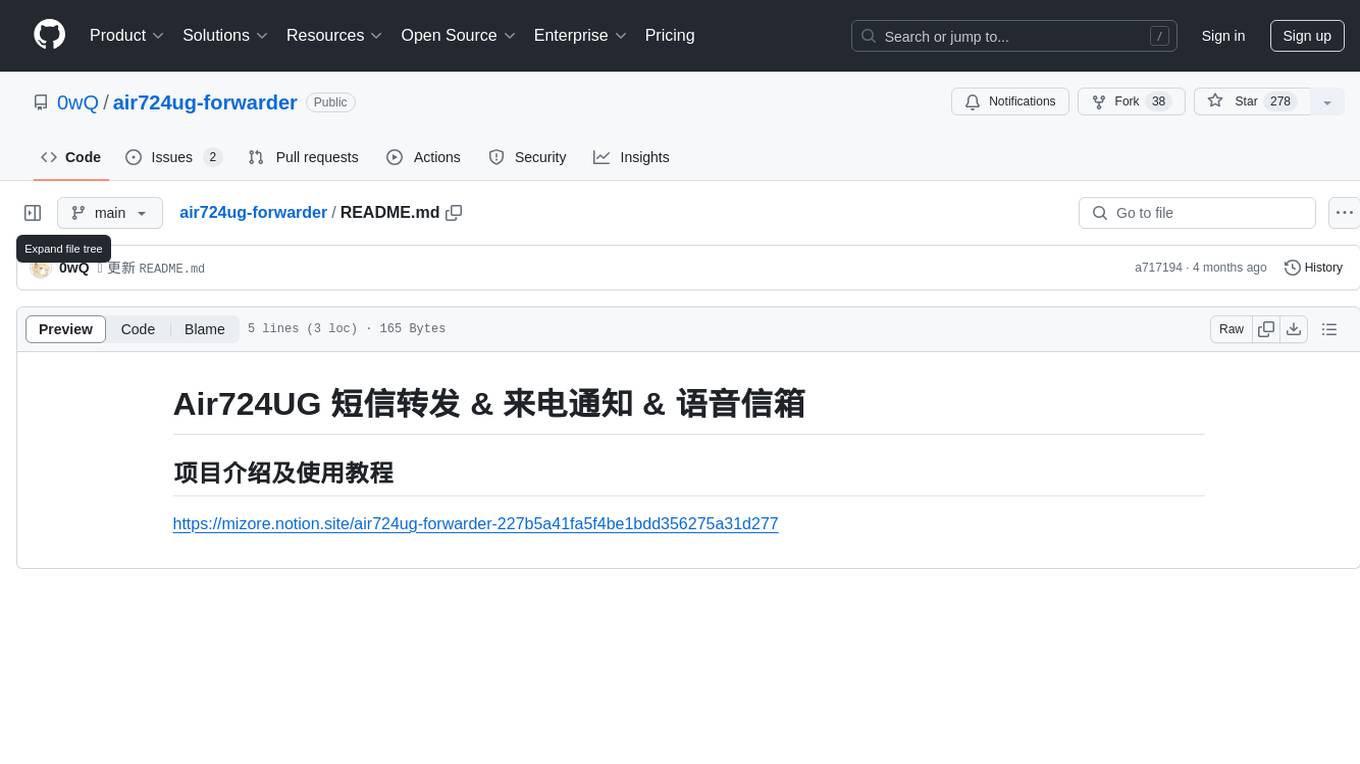
air724ug-forwarder
Air724UG forwarder is a tool designed to forward SMS, notify incoming calls, and manage voice messages. It provides a convenient way to handle communication tasks on Air724UG devices. The tool streamlines the process of receiving and managing messages, ensuring users stay connected and informed.
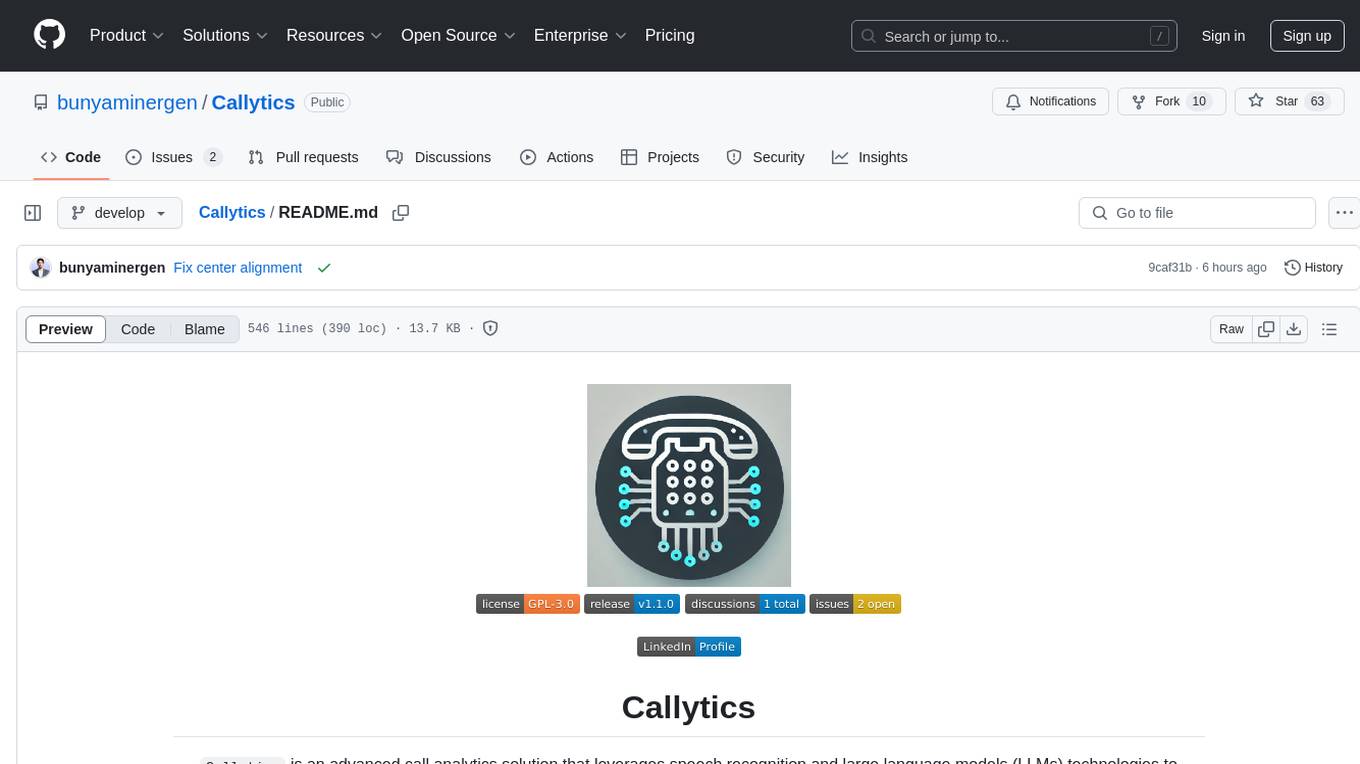
Callytics
Callytics is an advanced call analytics solution that leverages speech recognition and large language models (LLMs) technologies to analyze phone conversations from customer service and call centers. By processing both the audio and text of each call, it provides insights such as sentiment analysis, topic detection, conflict detection, profanity word detection, and summary. These cutting-edge techniques help businesses optimize customer interactions, identify areas for improvement, and enhance overall service quality. When an audio file is placed in the .data/input directory, the entire pipeline automatically starts running, and the resulting data is inserted into the database. This is only a v1.1.0 version; many new features will be added, models will be fine-tuned or trained from scratch, and various optimization efforts will be applied.
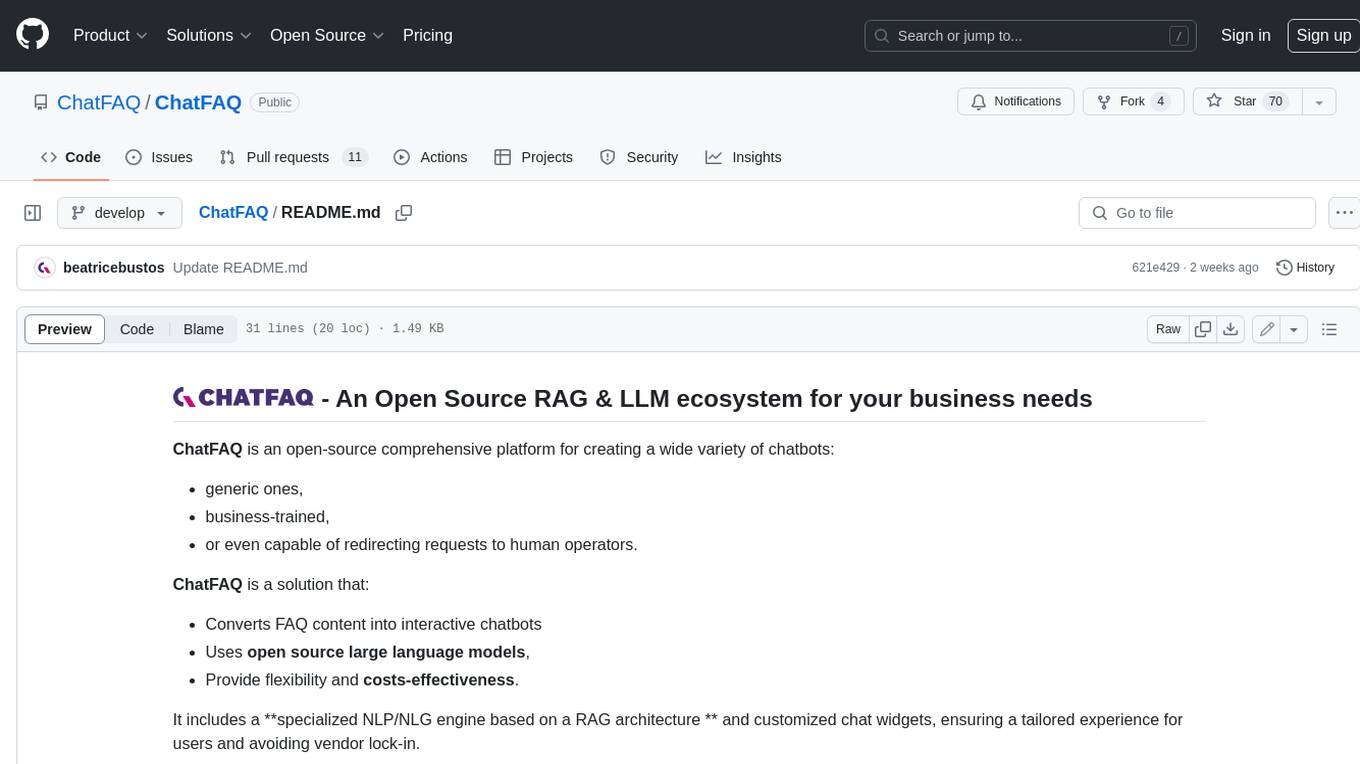
ChatFAQ
ChatFAQ is an open-source comprehensive platform for creating a wide variety of chatbots: generic ones, business-trained, or even capable of redirecting requests to human operators. It includes a specialized NLP/NLG engine based on a RAG architecture and customized chat widgets, ensuring a tailored experience for users and avoiding vendor lock-in.
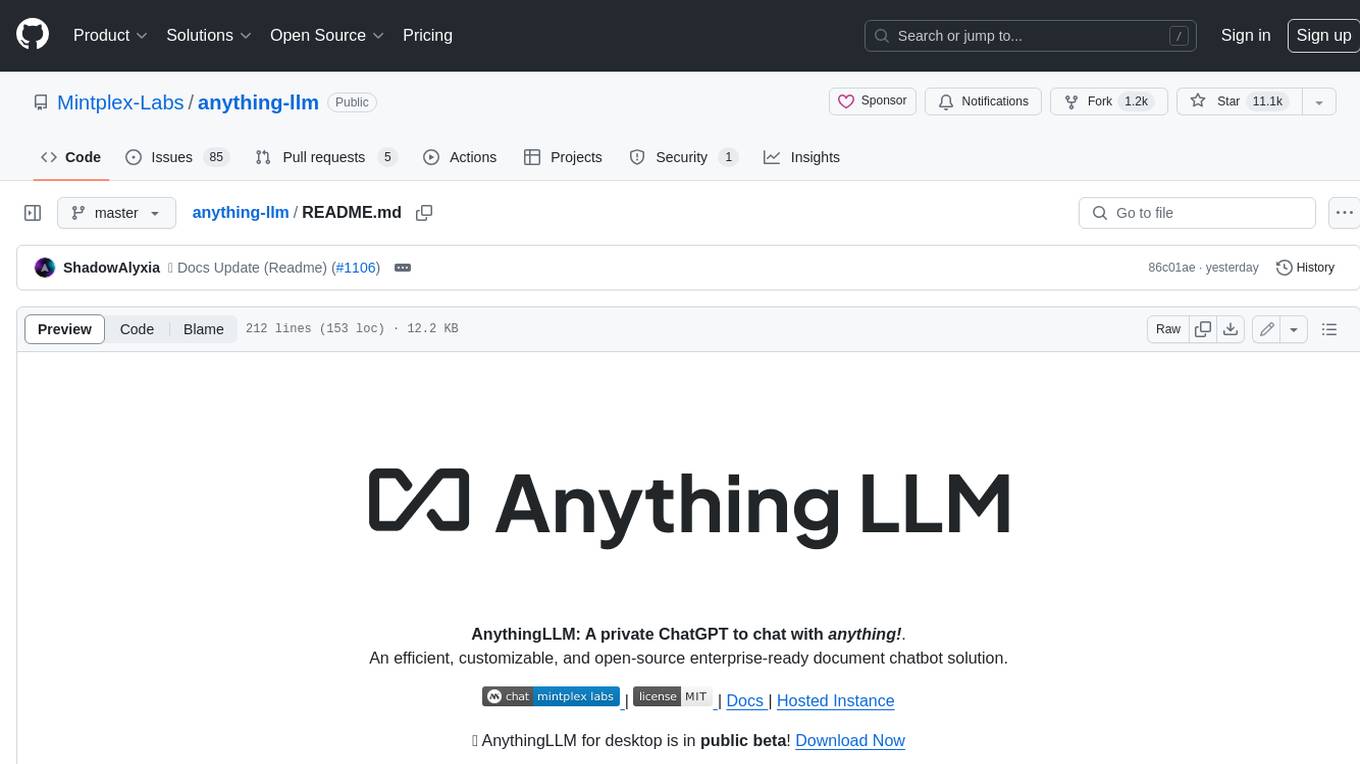
anything-llm
AnythingLLM is a full-stack application that enables you to turn any document, resource, or piece of content into context that any LLM can use as references during chatting. This application allows you to pick and choose which LLM or Vector Database you want to use as well as supporting multi-user management and permissions.