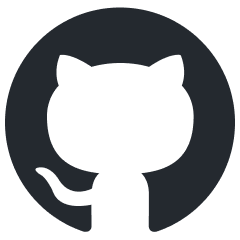
TestSpark
TestSpark - a plugin for generating unit tests. TestSpark natively integrates different AI-based test generation tools and techniques in the IDE. Started by SERG TU Delft. Currently under implementation by JetBrains Research (Software Testing Research) for research purposes.
Stars: 56
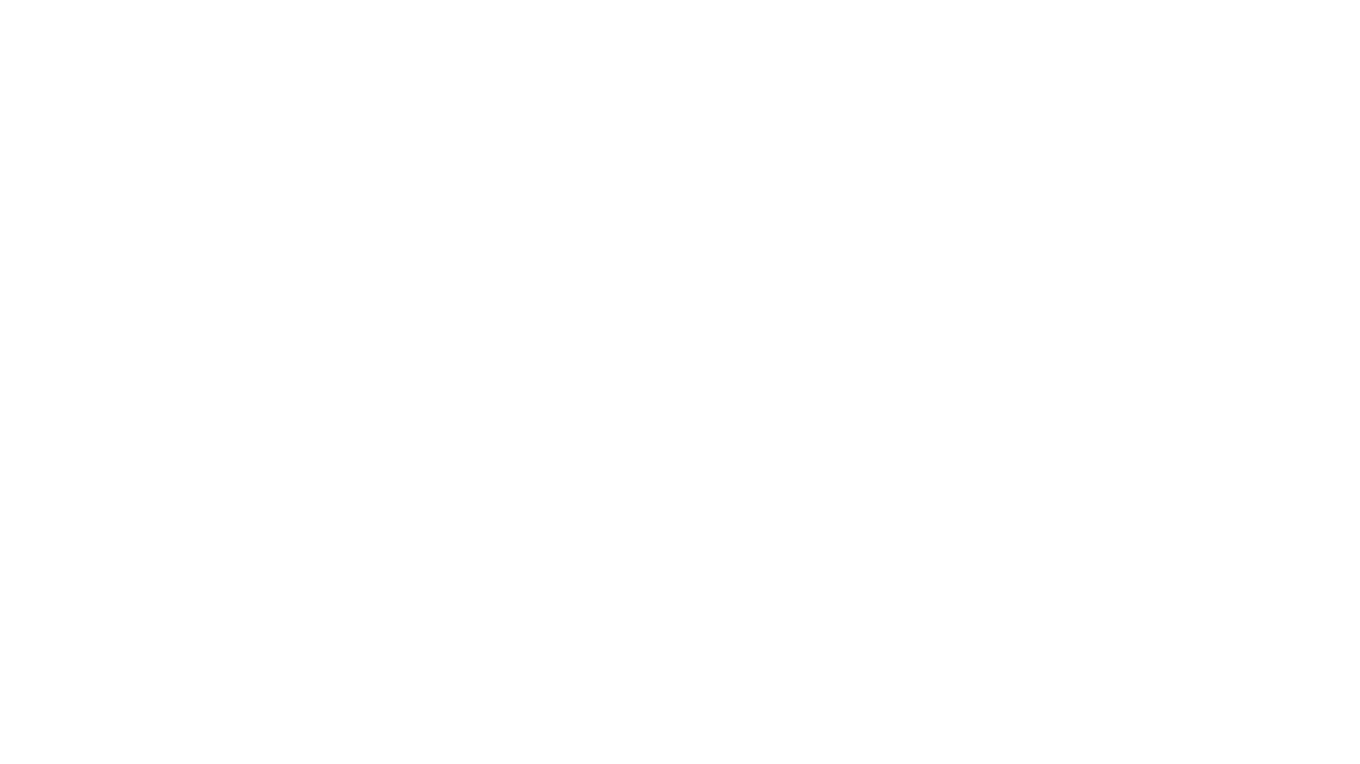
TestSpark is a plugin for generating unit tests that integrates AI-based test generation tools. It supports LLM-based test generation using OpenAI, HuggingFace, and JetBrains internal AI Assistant platform, as well as local search-based test generation using EvoSuite. Users can configure test generation settings, interact with test cases, view coverage statistics, and integrate tests into projects. The plugin is designed for experimental use to augment existing test suites, not replace manual test writing.
README:
TestSpark is a plugin for generating unit tests. TestSpark natively integrates different AI-based test generation tools and techniques in the IDE.
TestSpark currently supports two test generation strategies:
- LLM-based test generation (using OpenAI, HuggingFace, and JetBrains internal AI Assistant platform)
- Local search-based test generation (using EvoSuite)
For this type of test generation, TestSpark sends request to different Large Language Models. Also, it automatically checks if tests are valid before presenting it to users.
This feature needs a token from OpenAI, HuggingFace, or the AI Assistant platform.
- Supports Java and Kotlin.
- Generates unit tests for capturing failures.
- Generate tests for Java classes, methods, and single lines.
For this type of test generation, TestSpark uses EvoSuite, which is the most powerful search-based local test generator.
- Supports up to Java 11.
- Generates tests for different test criteria: line coverage, branch coverage, I/O diversity, exception coverage, mutation score.
- Generates unit tests for capturing failures.
- Generate tests for Java classes, methods, and single lines.
Initially implemented by CISELab at SERG @ TU Delft, TestSpark is currently developed and maintained by ICTL at JetBrains Research.
TestSpark is currently designed to serve as an experimental tool. Please keep in mind that tests generated by TestSpark are meant to augment your existing test suites. They are not meant to replace writing tests manually.
If you are running the plugin for the first time, checkout the Settings section.
-
Using IDE built-in plugin system:
Settings/Preferences > Plugins > Marketplace > Search for "TestSpark" > Install Plugin
-
Manually:
Download the latest release and install it manually using Settings/Preferences > Plugins > ⚙️ > Install plugin from disk...
- Generating Tests
- Working with Test Cases
- Coverage
- Integrating tests into the project
- Settings
- Disable K2 for Kotlin Test Generation
- Telemetry
To initiate the generation process, right-click on the part of the code for which tests need to be generated and select TestSpark
.
After that, a window will open where users need to configure generation settings.
Firstly users need to select the test generator (LLM-based test generator or EvoSuite
, which is a Local search-based test generator).
Also, in this window, it is necessary to select the part of the code for which tests need to be generated. The selection consists of no more than three items -- class/interface
, method/constructor
(if applicable), line
(if applicable).
After clicking the Next
button, the plugin provides the opportunity to configure the basic parameters of the selected generator. Advanced parameter settings can be done in Settings. All settings, both in Settings
and in this window, are saved, so you can disable the ability to configure generators before each generation process to perform this process more quickly.
In the case of LLM, two additional pages are provided for basic settings.
In the first page, users configure LLM Platform
, LLM Token
, LLM Model
, LLM JUnit version
, and Prompt Selection
. More detailed descriptions of each item can be found in Settings
.
After that, in the next page, you can provide some test samples for LLM.
Tests can be entered manually.
Also, tests can be chosen tests from the current project.
Test Cases can be modified, reset to their initial state, and deleted.
For EvoSuite
, you need to enter the local path to Java 11 and select the generation algorithm, after which the generation process will start.
After configuring the test generators, click the OK
button, after which the generation process will start, and a list of generated test cases will appear on the right side of the IDE.
During the test generation, users can observe the current state of the generation process.
After receiving the results, the user can interact with the test cases in various ways. They can view the result (whether it's passed or failed), also select, delete, modify, reset, like/dislike, fix by LLM and execute the tests to update the results.
Hitting the "Apply to test suite" button will add the selected tests to a test class of your choice.
Additionally, the top row of the tool window has buttons for selecting all tests, deselecting all tests, running all tests and removing them. The user also has an overview of how many tests they currently have selected and passed.
Users can select test cases.
Users can remove test cases.
Users can modify the code of test cases.
Users can reset the code to its original.
Users can reset the code to the last run.
Users can run the test to update the execution result.
Effortlessly identify passed and failed test cases with green and red color highlights for instant result comprehension is available. In case of failure, it is possible to find out the current error.
Users can copy the test.
Users can like/dislike the test for future analysis and improvement of the generation process.
Users can send a request to LLM with modification which users prefer for the test case.
Users can choose a default query, the list of which is set up in the Settings
.
Users can also manually punch in a new request.
Once a test suite is generated, basic statistics about it can be seen in the tool window, coverage
tab. The statistics include line coverage, branch coverage, weak mutation coverage. The table adjusts dynamically - it only calculates the statistics for the selected tests in the test suite.
Once test are generated, the lines which are covered by the tests will be highlighted (default color: green). The gutter next to the lines will have a green rectangle as well. If the rectangle is clicked, a popup will show the names of the tests which cover the selected line. If any of the test names are clicked, the corresponding test in the toolwindow will be highlighted with the same accent color. The effect lasts 10 seconds. Coverage visualisation adjusts dynamically - it only shows results for the tests that are selected in the TestSpark tab.
The tests can be added to an existing file:
Or to a new file:
The plugin is configured mainly through the Settings menu. The plugin settings can be found under Settings > Tools > TestSpark. Here, the user is able to select options for the plugin:
Before running the plugin for the first time, we highly recommend going to the Environment settings
section of TestSpark settings. The settings include compilation path (path to compiled code) and compilation command. Both commands have defaults. However, we recommend especially that you check compilation command. For this command the user requires maven, gradle or any other builder program which can be accessed via command. Leaving this field with a faulty value may cause unintended behaviour.
The plugin supports changing the color for coverage visualisation and killed mutants visualisation (one setting for both). To change the color, go to Settings > Tools > TestSpark and use the color picker under Accessibility settings
:
The plugin has been designed with translation in mind. The vast majority of the plugins labels, tooltips, messages, etc. is stored in .property files. For more information on translation, refer to the contributing readme.
The settings submenu Settings > Tools > TestSpark > EvoSuite allows the user to tweak EvoSuite parameters to their liking.
At the moment EvoSuite can be executed only with Java 11, so if the user has a more modern version by default, it is necessary to download Java 11 and set the path to the java file.
To accelerate the test generation process, users can disable the display of the EvoSuite Setup Page
.
EvoSuite
has hundreds of parameters, not all can be packed in a settings menu. However, the most commonly used and rational settings were added here:
The settings submenu Settings > Tools > TestSpark > LLM allows the user to tweak LLM parameters to their liking.
Selecting a platform to interact with the LLM. By default, only OpenAI is available, but for JetBrains employees there is an option to interact via Graize. More details in the TestSpark for JetBrains employees section.
Users have to set their own token for LLM, the plugin does not provide a default option.
Once the correct token is entered, it will be possible to select an LLM model for test generation.
In addition to the token, users are recommended to configure settings for the LLM process.
To expedite the test generation process, users can disable the display of the LLM Setup Page
.
Additionally, they can also disable the display of the LLM Samples Page
.
Users can customize the list of default requests to the LLM in test cases.
The plugin uses JUnit to generate tests. It is possible to select the JUnit version and prioritize the versions used by the current project.
Users have the opportunity to adjust the prompt that is sent to the LLM platform.
To create a request to the LLM, it is necessary to provide a prompt which contains information about the details of the generation. The most necessary data are located in the mandatory section, without them the prompt is not valid.
Users can modify, create new templates, delete and use different variants in practice.
❗ Pro tip: don't forget to hit the "save" button at the bottom. ❗
One of the biggest future plans of our client is to leverage the data that is gathered by TestSpark’s telemetry. This will help them with future research, including the development of an interactive way of using EvoSuite. The general idea behind this feature is to learn from the stored user corrections in order to improve test generation.
To opt into telemetry, go to Settings > Tools > TestSpark and tick the Enable telemetry
checkbox. If you want, change the directory where telemetry is stored.
JetBrains employees have the ability to send queries to OpenAI models through the Grazie platform.
-
To include test generation using Grazie in the build process, you need to pass Space username and token as properties:
gradle buildPlugin -Dspace.username=<USERNAME> -Dspace.pass=<TOKEN>
. -
To include test generation using Grazie in the runIdeForUiTests process, you need to pass Space username and token as properties:
gradle runIdeForUiTests -Dspace.username=<USERNAME> -Dspace.pass=<TOKEN>
. -
<TOKEN>
is generated by Space, which has access toAutomatically generating unit tests
maven packages.
Store Space username and token in ~/.gradle/gradle.properties
...
spaceUsername=<USERNAME>
spacePassword=<TOKEN>
...
LLM Settings with Grazie platform option:
The plugin is Open-Source and publicly hosted on github. Anyone can look into the code and suggest changes. You can find the plugin page here.
In addition, learn more about the structure of the plugin here.
Plugin based on the IntelliJ Platform Plugin Template.
For Tasks:
Click tags to check more tools for each tasksFor Jobs:
Alternative AI tools for TestSpark
Similar Open Source Tools
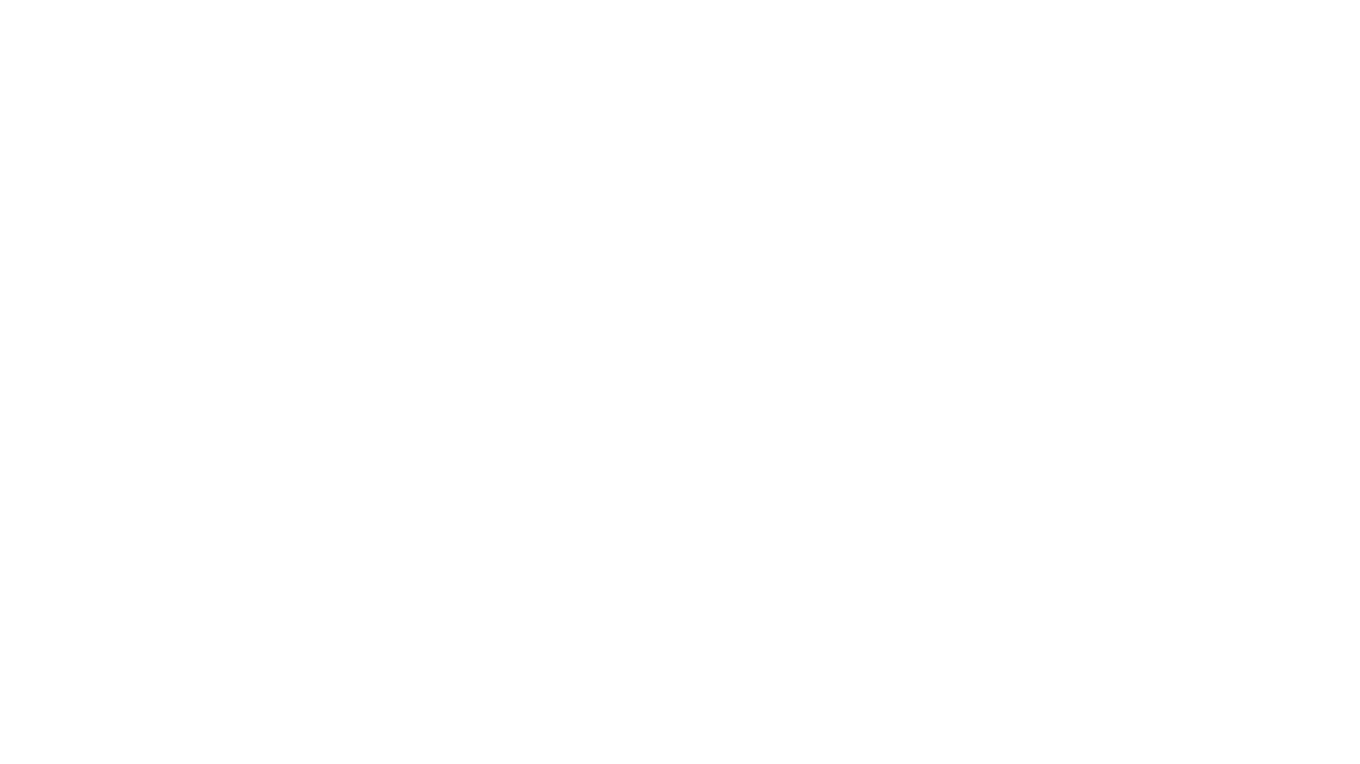
TestSpark
TestSpark is a plugin for generating unit tests that integrates AI-based test generation tools. It supports LLM-based test generation using OpenAI, HuggingFace, and JetBrains internal AI Assistant platform, as well as local search-based test generation using EvoSuite. Users can configure test generation settings, interact with test cases, view coverage statistics, and integrate tests into projects. The plugin is designed for experimental use to augment existing test suites, not replace manual test writing.
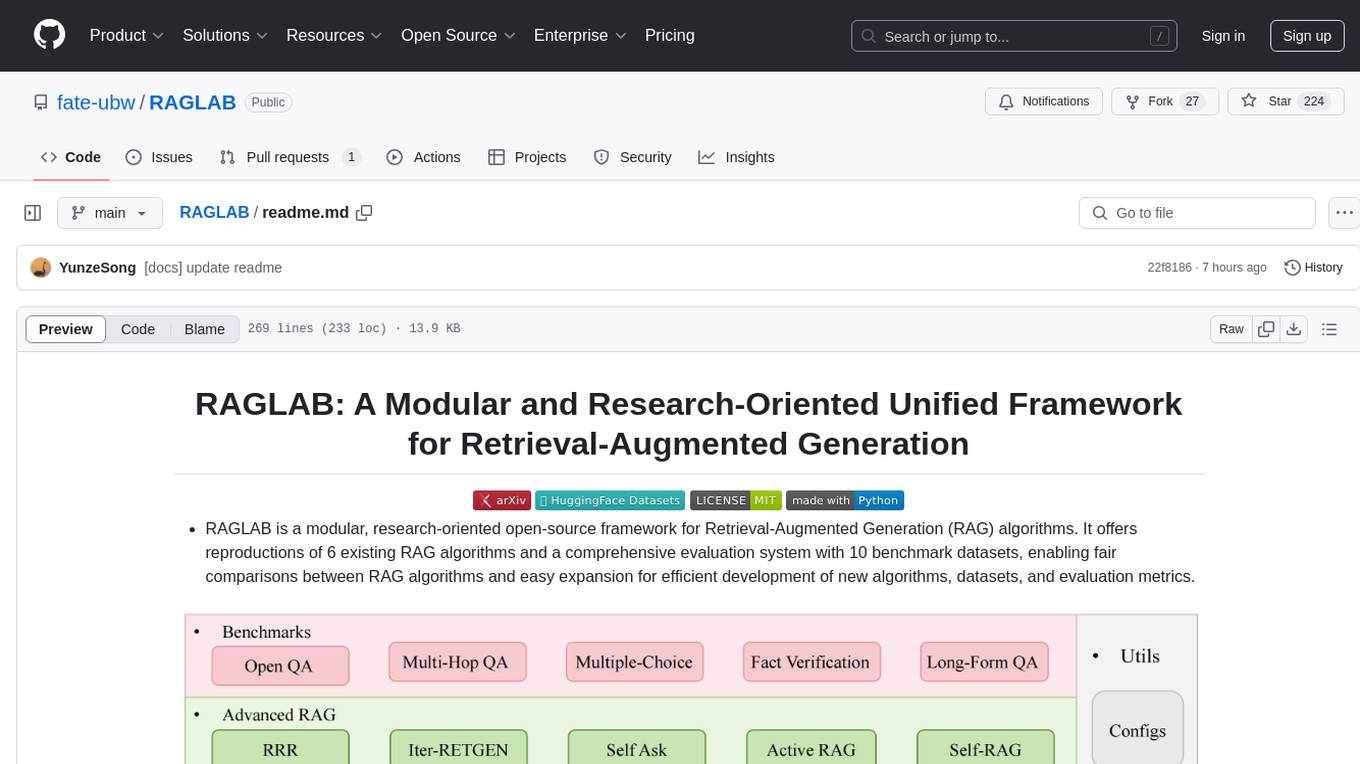
RAGLAB
RAGLAB is a modular, research-oriented open-source framework for Retrieval-Augmented Generation (RAG) algorithms. It offers reproductions of 6 existing RAG algorithms and a comprehensive evaluation system with 10 benchmark datasets, enabling fair comparisons between RAG algorithms and easy expansion for efficient development of new algorithms, datasets, and evaluation metrics. The framework supports the entire RAG pipeline, provides advanced algorithm implementations, fair comparison platform, efficient retriever client, versatile generator support, and flexible instruction lab. It also includes features like Interact Mode for quick understanding of algorithms and Evaluation Mode for reproducing paper results and scientific research.
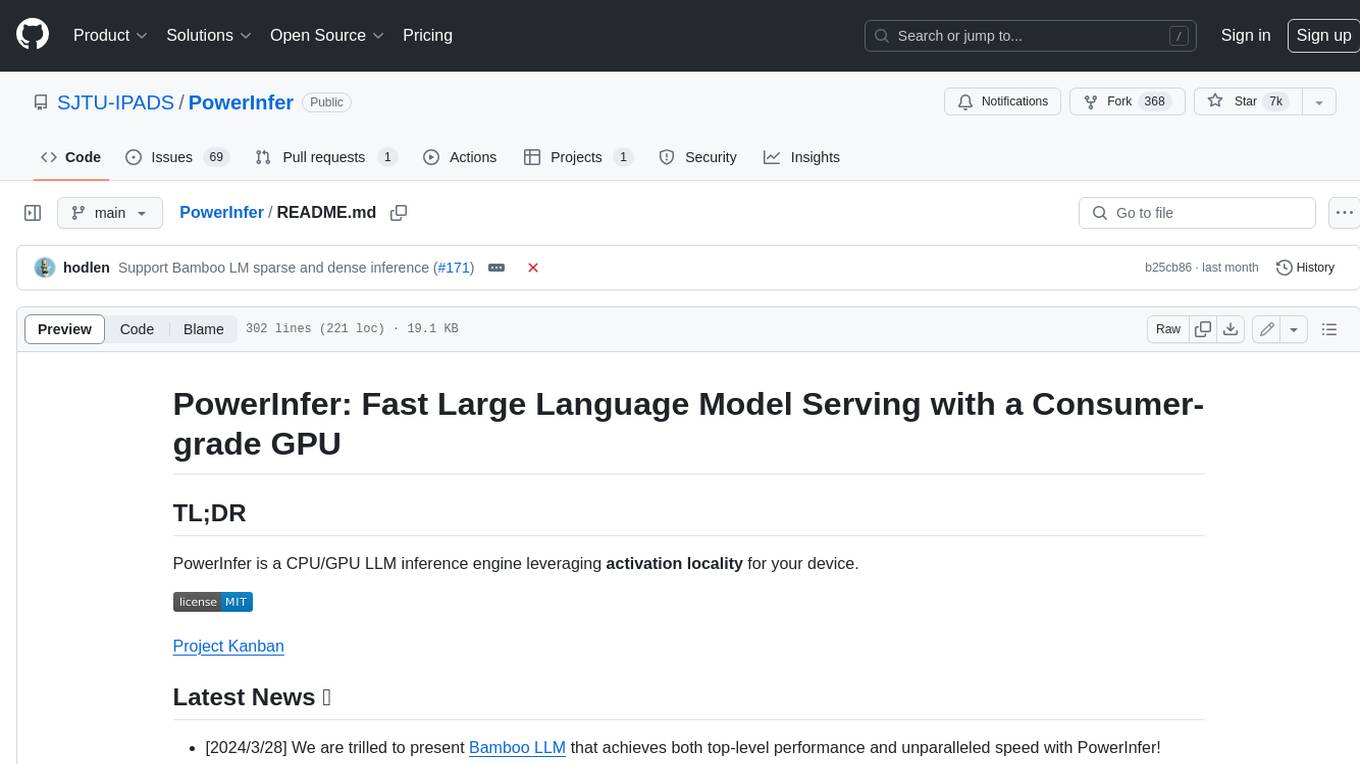
PowerInfer
PowerInfer is a high-speed Large Language Model (LLM) inference engine designed for local deployment on consumer-grade hardware, leveraging activation locality to optimize efficiency. It features a locality-centric design, hybrid CPU/GPU utilization, easy integration with popular ReLU-sparse models, and support for various platforms. PowerInfer achieves high speed with lower resource demands and is flexible for easy deployment and compatibility with existing models like Falcon-40B, Llama2 family, ProSparse Llama2 family, and Bamboo-7B.
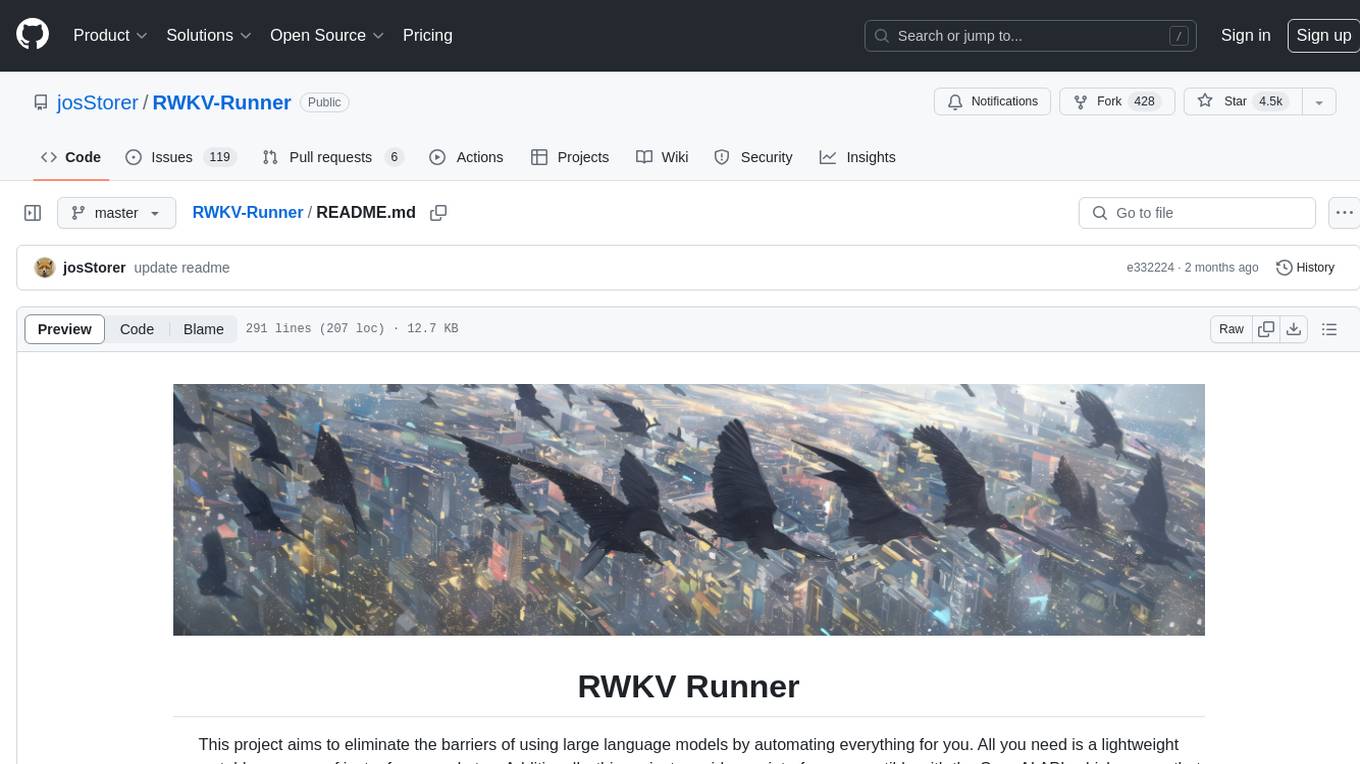
RWKV-Runner
RWKV Runner is a project designed to simplify the usage of large language models by automating various processes. It provides a lightweight executable program and is compatible with the OpenAI API. Users can deploy the backend on a server and use the program as a client. The project offers features like model management, VRAM configurations, user-friendly chat interface, WebUI option, parameter configuration, model conversion tool, download management, LoRA Finetune, and multilingual localization. It can be used for various tasks such as chat, completion, composition, and model inspection.
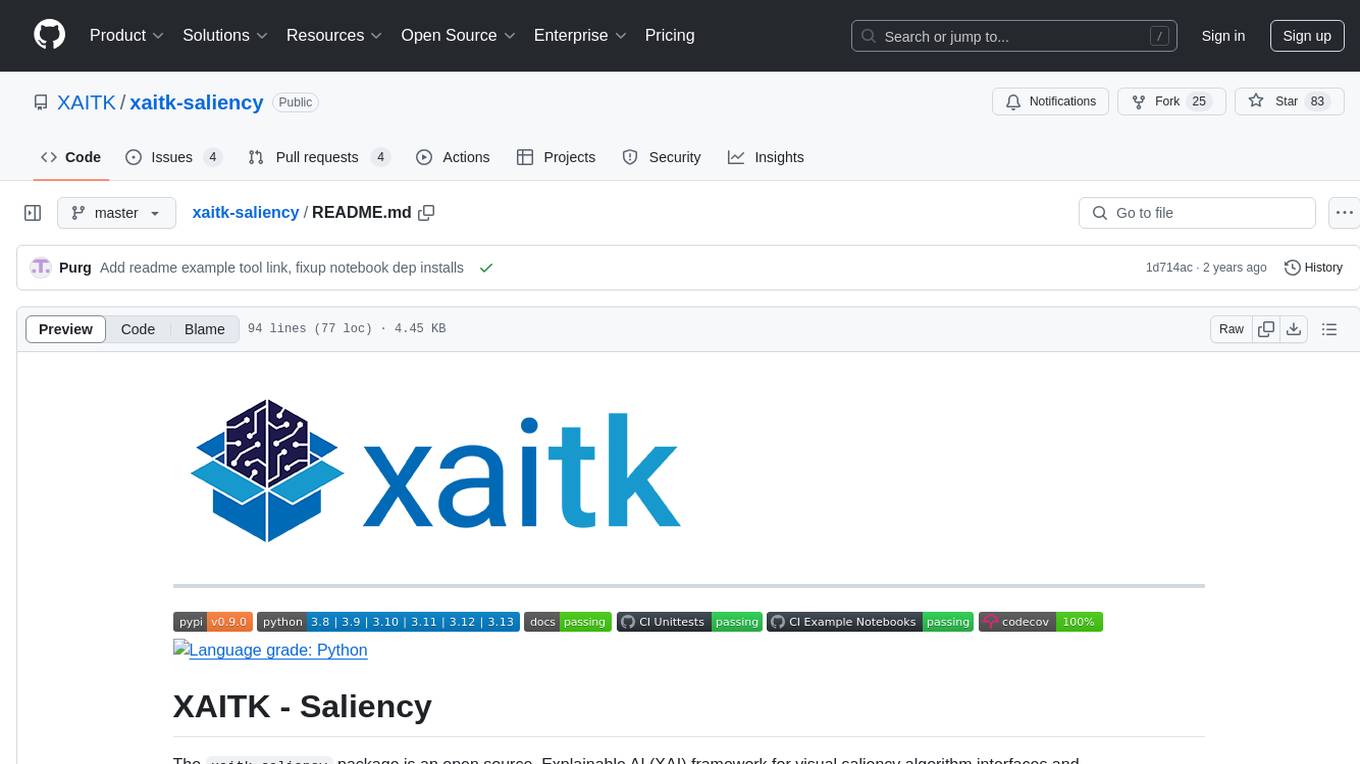
xaitk-saliency
The `xaitk-saliency` package is an open source Explainable AI (XAI) framework for visual saliency algorithm interfaces and implementations, designed for analytics and autonomy applications. It provides saliency algorithms for various image understanding tasks such as image classification, image similarity, object detection, and reinforcement learning. The toolkit targets data scientists and developers who aim to incorporate visual saliency explanations into their workflow or product, offering both direct accessibility for experimentation and modular integration into systems and applications through Strategy and Adapter patterns. The package includes documentation, examples, and a demonstration tool for visual saliency generation in a user-interface.
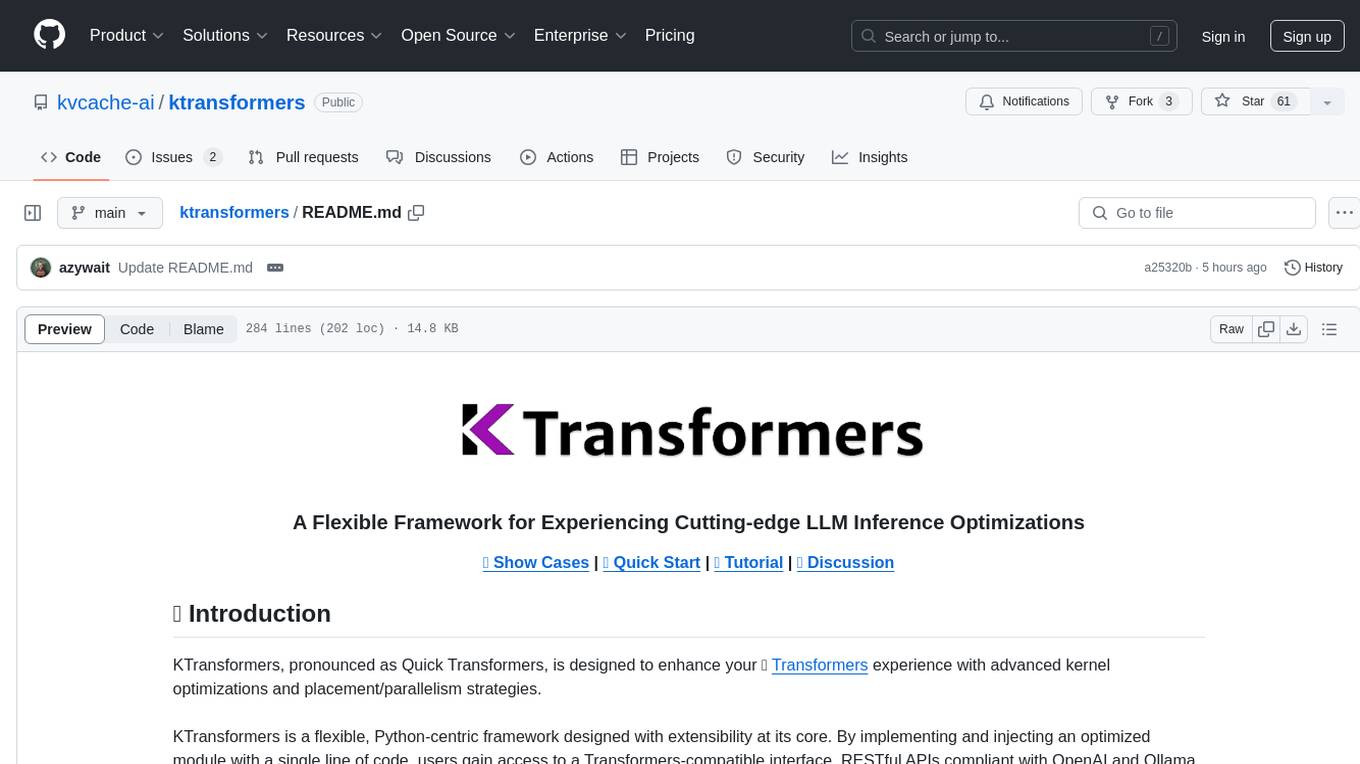
ktransformers
KTransformers is a flexible Python-centric framework designed to enhance the user's experience with advanced kernel optimizations and placement/parallelism strategies for Transformers. It provides a Transformers-compatible interface, RESTful APIs compliant with OpenAI and Ollama, and a simplified ChatGPT-like web UI. The framework aims to serve as a platform for experimenting with innovative LLM inference optimizations, focusing on local deployments constrained by limited resources and supporting heterogeneous computing opportunities like GPU/CPU offloading of quantized models.
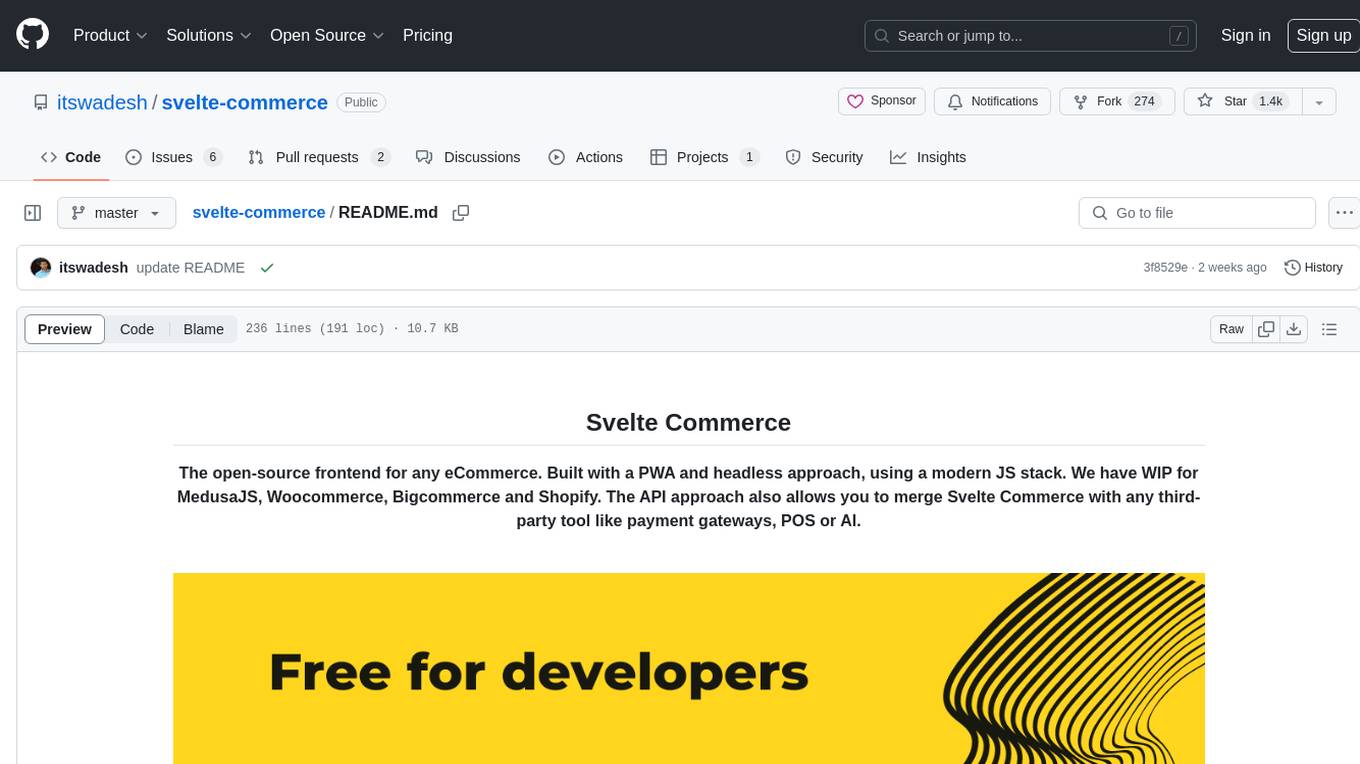
svelte-commerce
Svelte Commerce is an open-source frontend for eCommerce, utilizing a PWA and headless approach with a modern JS stack. It supports integration with various eCommerce backends like MedusaJS, Woocommerce, Bigcommerce, and Shopify. The API flexibility allows seamless connection with third-party tools such as payment gateways, POS systems, and AI services. Svelte Commerce offers essential eCommerce features, is both SSR and SPA, superfast, and free to download and modify. Users can easily deploy it on Netlify or Vercel with zero configuration. The tool provides features like headless commerce, authentication, cart & checkout, TailwindCSS styling, server-side rendering, proxy + API integration, animations, lazy loading, search functionality, faceted filters, and more.
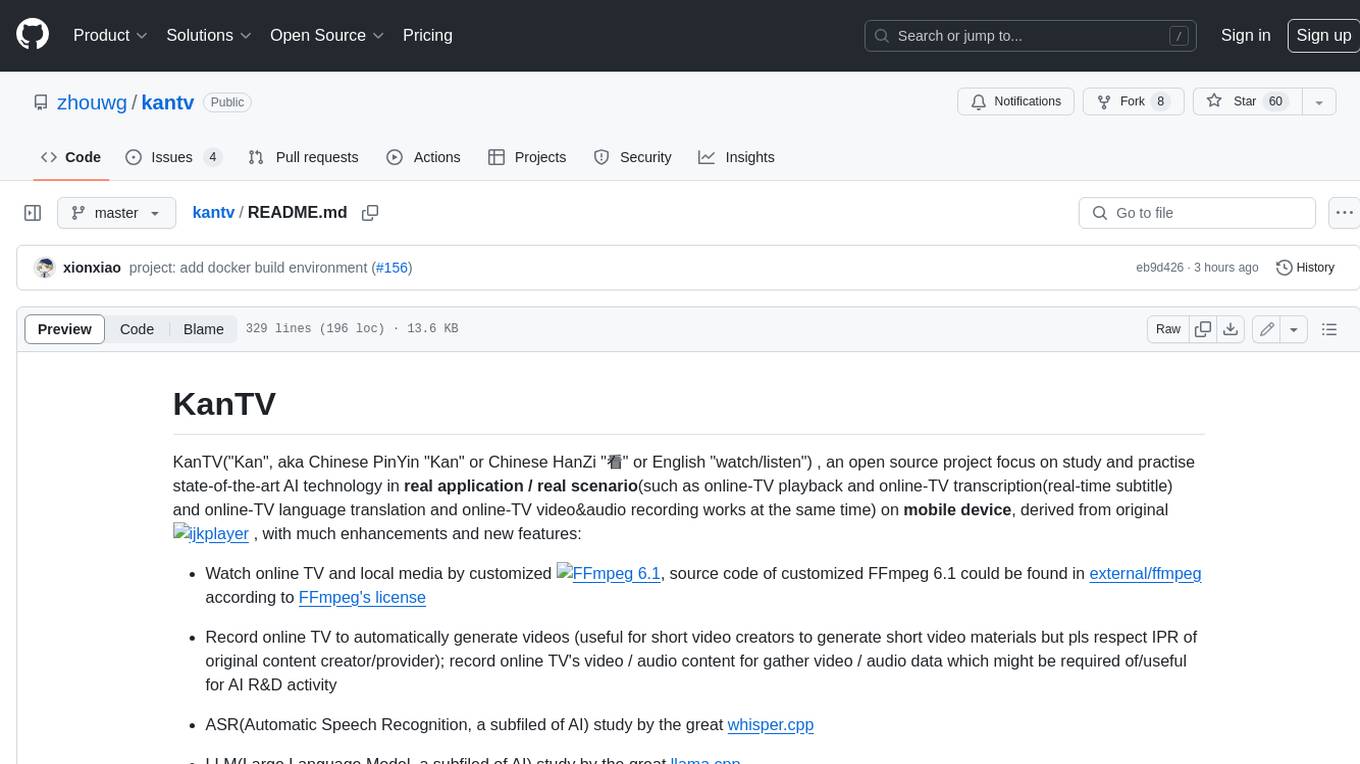
kantv
KanTV is an open-source project that focuses on studying and practicing state-of-the-art AI technology in real applications and scenarios, such as online TV playback, transcription, translation, and video/audio recording. It is derived from the original ijkplayer project and includes many enhancements and new features, including: * Watching online TV and local media using a customized FFmpeg 6.1. * Recording online TV to automatically generate videos. * Studying ASR (Automatic Speech Recognition) using whisper.cpp. * Studying LLM (Large Language Model) using llama.cpp. * Studying SD (Text to Image by Stable Diffusion) using stablediffusion.cpp. * Generating real-time English subtitles for English online TV using whisper.cpp. * Running/experiencing LLM on Xiaomi 14 using llama.cpp. * Setting up a customized playlist and using the software to watch the content for R&D activity. * Refactoring the UI to be closer to a real commercial Android application (currently only supports English). Some goals of this project are: * To provide a well-maintained "workbench" for ASR researchers interested in practicing state-of-the-art AI technology in real scenarios on mobile devices (currently focusing on Android). * To provide a well-maintained "workbench" for LLM researchers interested in practicing state-of-the-art AI technology in real scenarios on mobile devices (currently focusing on Android). * To create an Android "turn-key project" for AI experts/researchers (who may not be familiar with regular Android software development) to focus on device-side AI R&D activity, where part of the AI R&D activity (algorithm improvement, model training, model generation, algorithm validation, model validation, performance benchmark, etc.) can be done very easily using Android Studio IDE and a powerful Android phone.
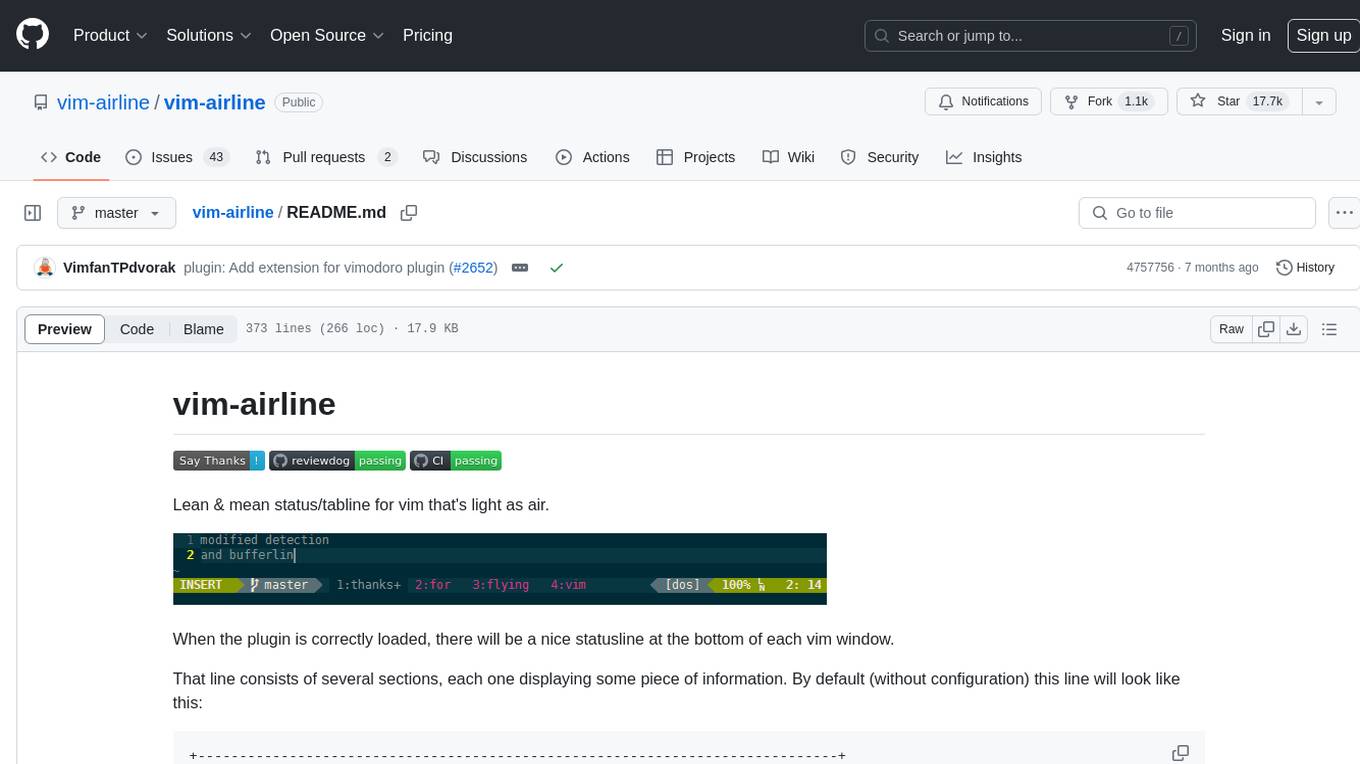
vim-airline
Vim-airline is a lean and mean status/tabline plugin for Vim that provides a nice statusline at the bottom of each Vim window. It consists of several sections displaying information such as mode, environment status, filename, filetype, file encoding, and current position in the file. The plugin is highly customizable and integrates with various plugins, providing a tiny core with extensibility in mind. It is optimized for speed, supports multiple themes, and integrates seamlessly with other plugins. Vim-airline is written in 100% Vimscript, eliminating the need for Python. The plugin aims to be stable and includes a unit testing suite for reliability.
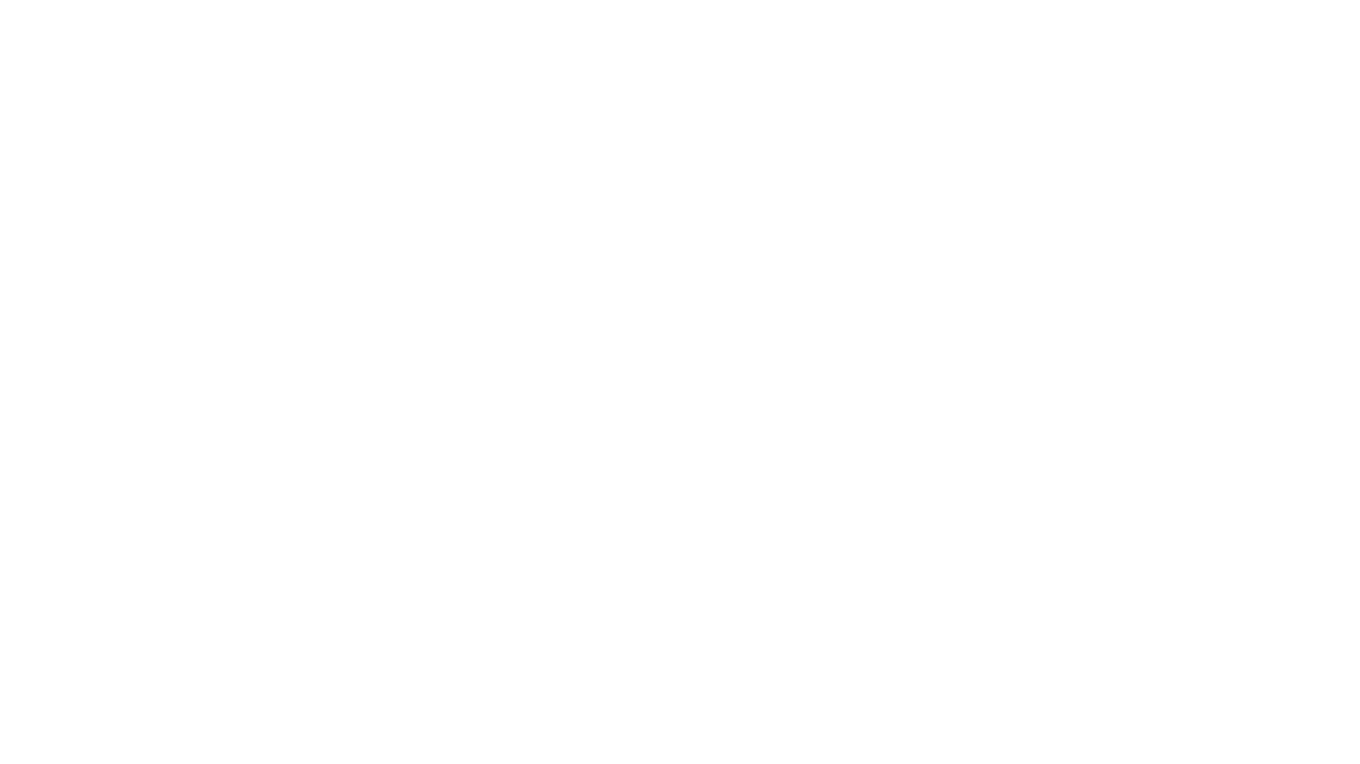
serverless-rag-demo
The serverless-rag-demo repository showcases a solution for building a Retrieval Augmented Generation (RAG) system using Amazon Opensearch Serverless Vector DB, Amazon Bedrock, Llama2 LLM, and Falcon LLM. The solution leverages generative AI powered by large language models to generate domain-specific text outputs by incorporating external data sources. Users can augment prompts with relevant context from documents within a knowledge library, enabling the creation of AI applications without managing vector database infrastructure. The repository provides detailed instructions on deploying the RAG-based solution, including prerequisites, architecture, and step-by-step deployment process using AWS Cloudshell.
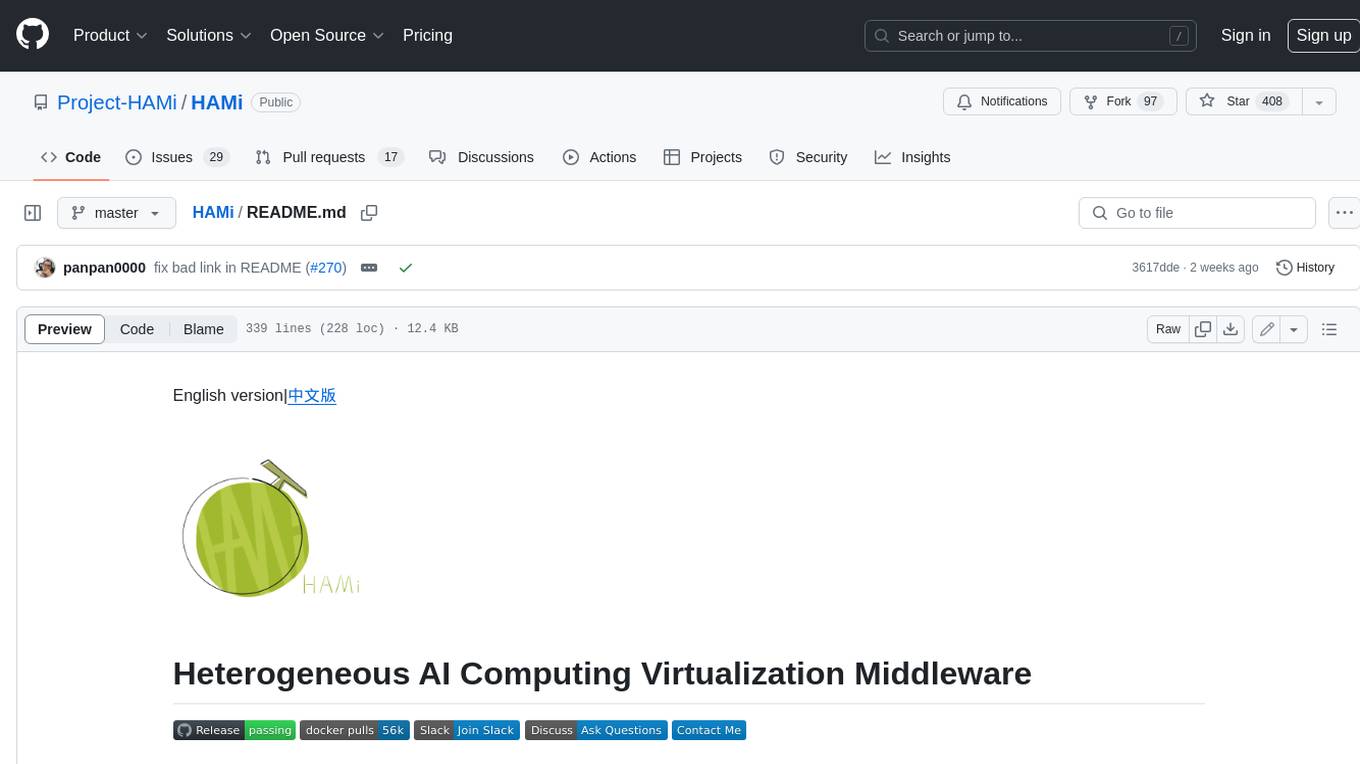
HAMi
HAMi is a Heterogeneous AI Computing Virtualization Middleware designed to manage Heterogeneous AI Computing Devices in a Kubernetes cluster. It allows for device sharing, device memory control, device type specification, and device UUID specification. The tool is easy to use and does not require modifying task YAML files. It includes features like hard limits on device memory, partial device allocation, streaming multiprocessor limits, and core usage specification. HAMi consists of components like a mutating webhook, scheduler extender, device plugins, and in-container virtualization techniques. It is suitable for scenarios requiring device sharing, specific device memory allocation, GPU balancing, low utilization optimization, and scenarios needing multiple small GPUs. The tool requires prerequisites like NVIDIA drivers, CUDA version, nvidia-docker, Kubernetes version, glibc version, and helm. Users can install, upgrade, and uninstall HAMi, submit tasks, and monitor cluster information. The tool's roadmap includes supporting additional AI computing devices, video codec processing, and Multi-Instance GPUs (MIG).
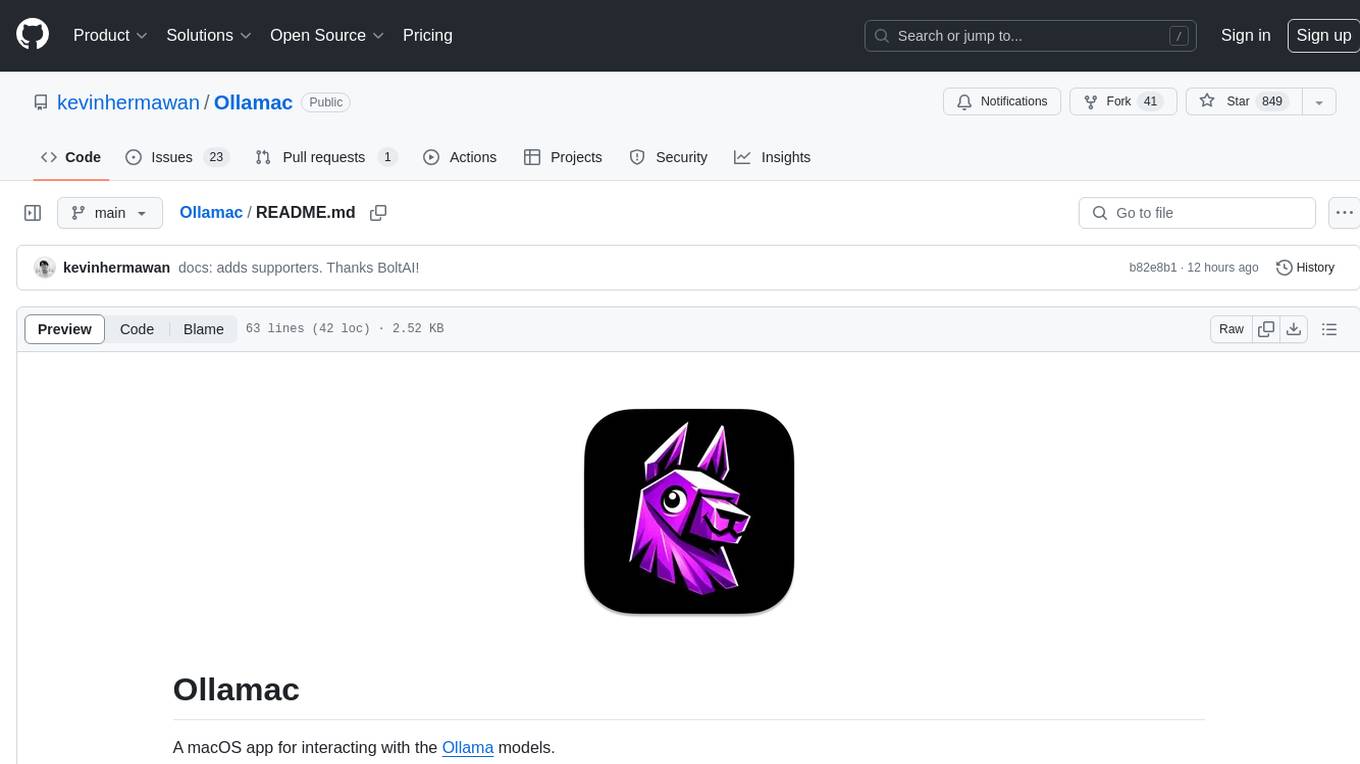
Ollamac
Ollamac is a macOS app designed for interacting with Ollama models. It is optimized for macOS, allowing users to easily use any model from the Ollama library. The app features a user-friendly interface, chat archive for saving interactions, and real-time communication using HTTP streaming technology. Ollamac is open-source, enabling users to contribute to its development and enhance its capabilities. It requires macOS 14 or later and the Ollama system to be installed on the user's Mac with at least one Ollama model downloaded.
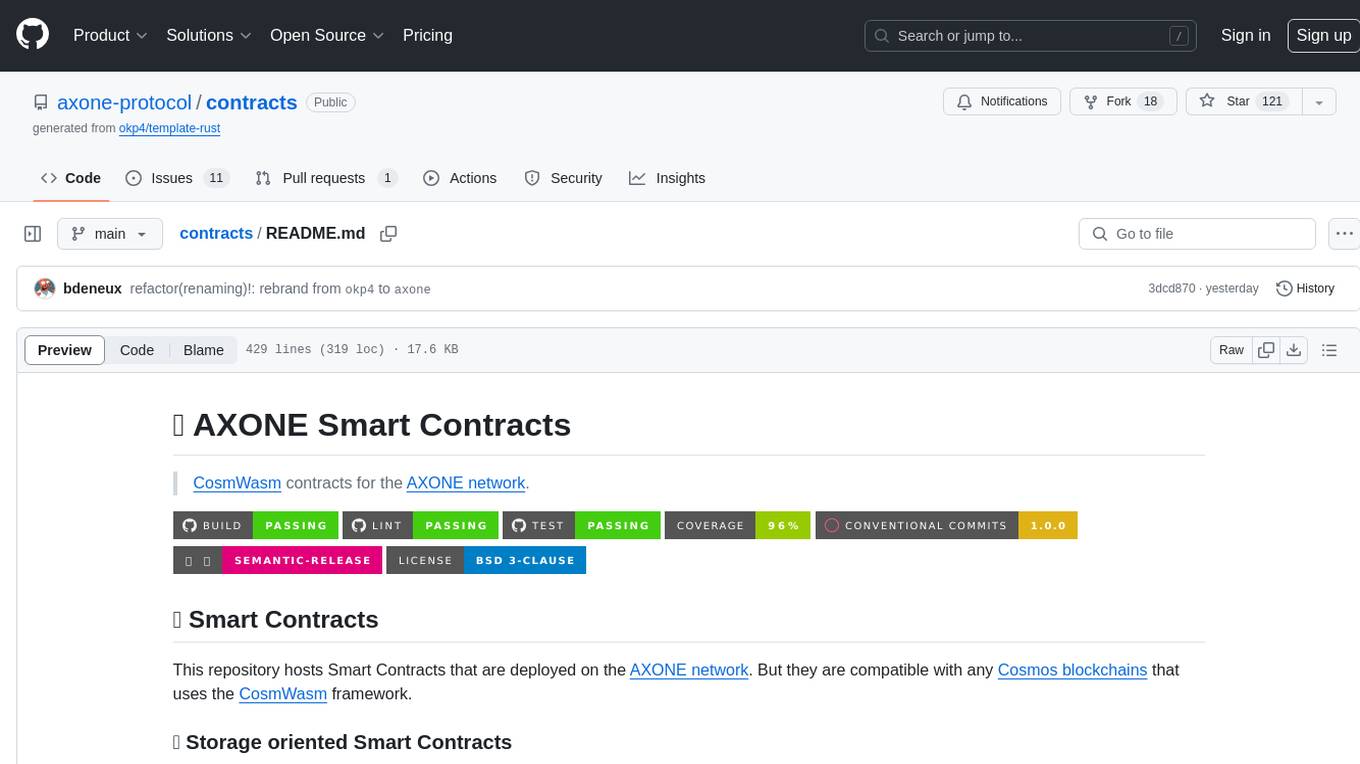
contracts
AXONE Smart Contracts repository hosts Smart Contracts for the AXONE network, compatible with any Cosmos blockchains using the CosmWasm framework. It includes storage, sovereignty, and resource management oriented Smart Contracts. Each contract has different functionalities and maturity stages, with detailed tech documentation and emojis indicating maturity levels. The repository provides tools for building, testing, deploying, and interacting with Smart Contracts, along with guidelines for contributing and community engagement.
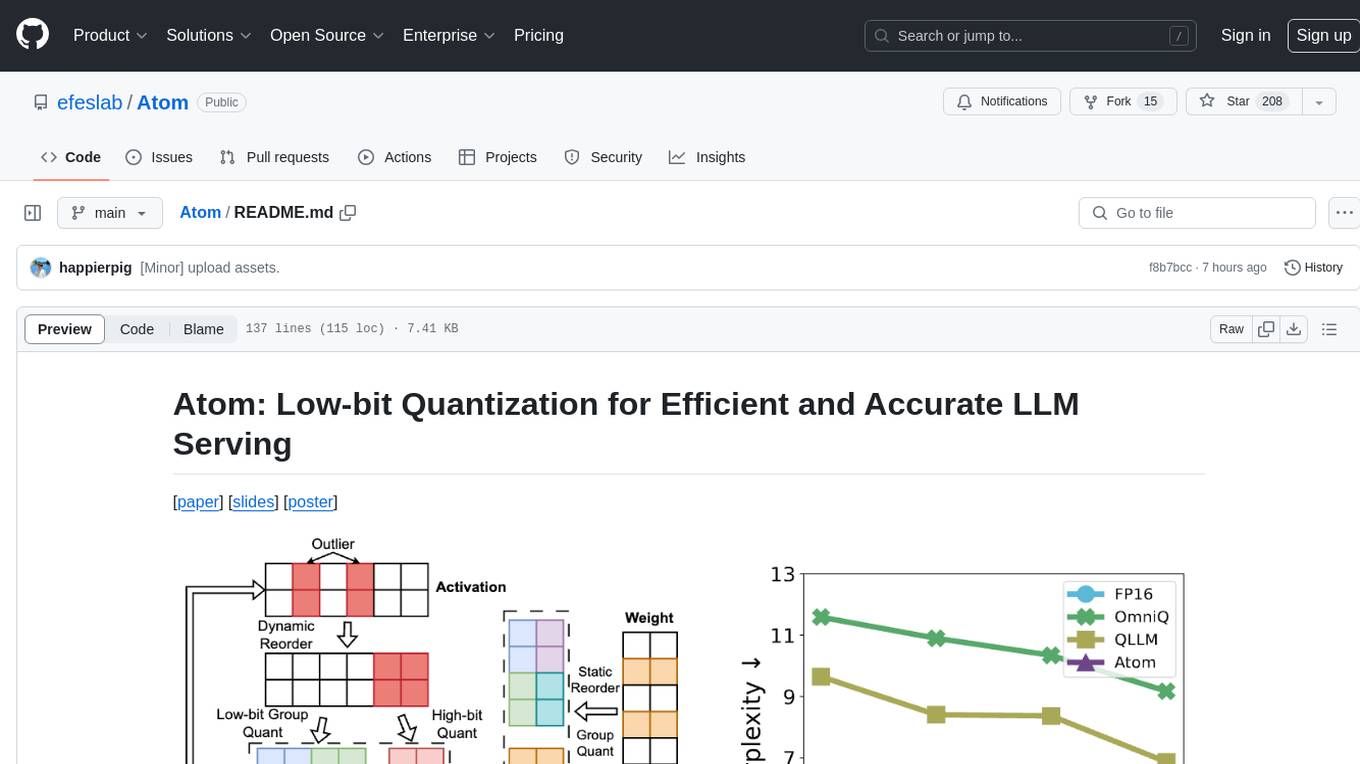
Atom
Atom is an accurate low-bit weight-activation quantization algorithm that combines mixed-precision, fine-grained group quantization, dynamic activation quantization, KV-cache quantization, and efficient CUDA kernels co-design. It introduces a low-bit quantization method, Atom, to maximize Large Language Models (LLMs) serving throughput with negligible accuracy loss. The codebase includes evaluation of perplexity and zero-shot accuracy, kernel benchmarking, and end-to-end evaluation. Atom significantly boosts serving throughput by using low-bit operators and reduces memory consumption via low-bit quantization.
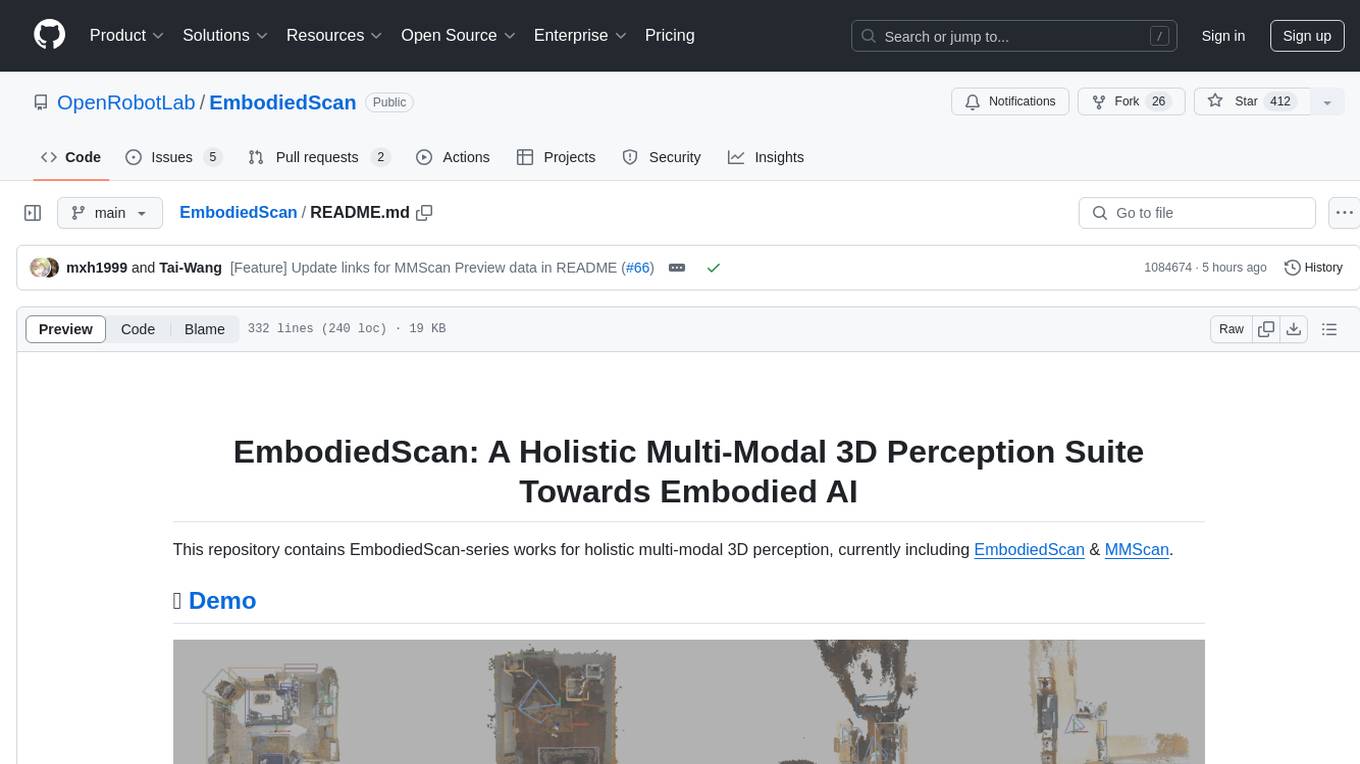
EmbodiedScan
EmbodiedScan is a holistic multi-modal 3D perception suite designed for embodied AI. It introduces a multi-modal, ego-centric 3D perception dataset and benchmark for holistic 3D scene understanding. The dataset includes over 5k scans with 1M ego-centric RGB-D views, 1M language prompts, 160k 3D-oriented boxes spanning 760 categories, and dense semantic occupancy with 80 common categories. The suite includes a baseline framework named Embodied Perceptron, capable of processing multi-modal inputs for 3D perception tasks and language-grounded tasks.
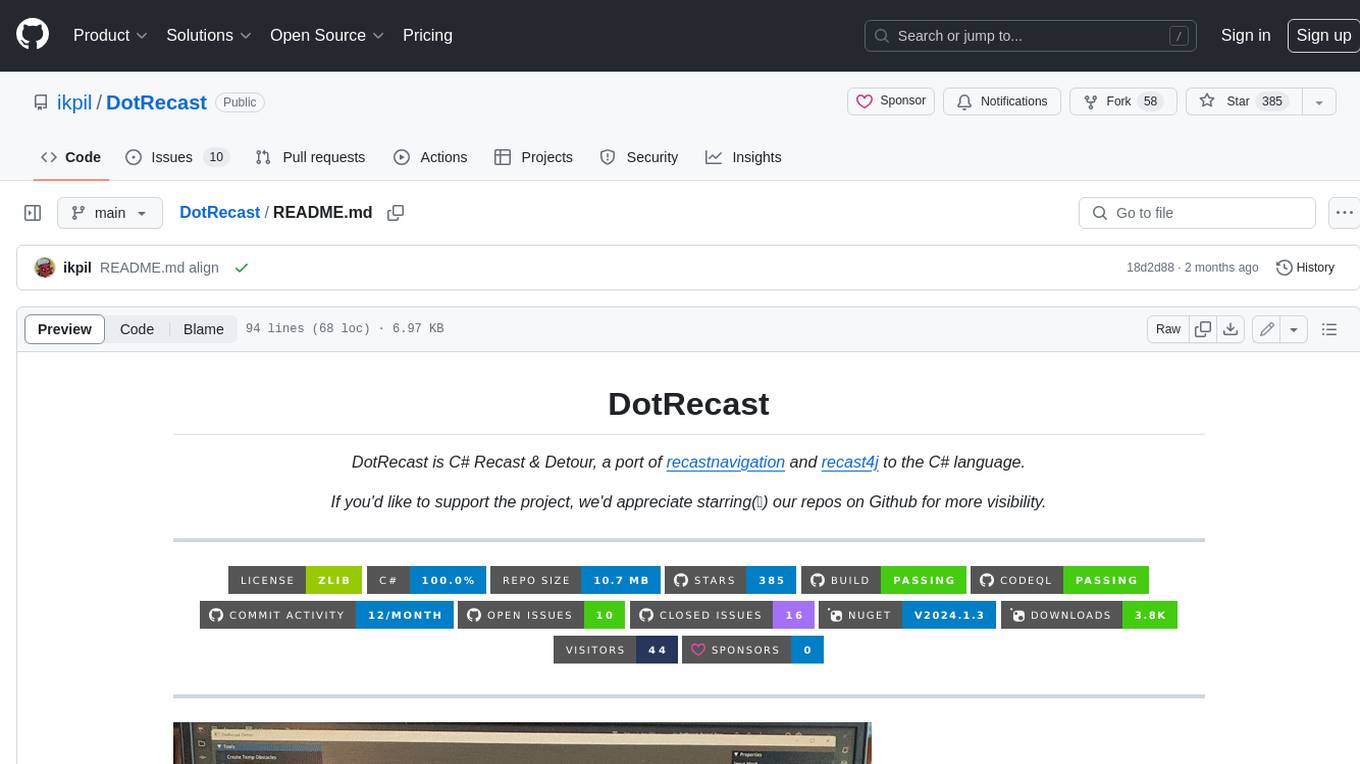
DotRecast
DotRecast is a C# port of Recast & Detour, a navigation library used in many AAA and indie games and engines. It provides automatic navmesh generation, fast turnaround times, detailed customization options, and is dependency-free. Recast Navigation is divided into multiple modules, each contained in its own folder: - DotRecast.Core: Core utils - DotRecast.Recast: Navmesh generation - DotRecast.Detour: Runtime loading of navmesh data, pathfinding, navmesh queries - DotRecast.Detour.TileCache: Navmesh streaming. Useful for large levels and open-world games - DotRecast.Detour.Crowd: Agent movement, collision avoidance, and crowd simulation - DotRecast.Detour.Dynamic: Robust support for dynamic nav meshes combining pre-built voxels with dynamic objects which can be freely added and removed - DotRecast.Detour.Extras: Simple tool to import navmeshes created with A* Pathfinding Project - DotRecast.Recast.Toolset: All modules - DotRecast.Recast.Demo: Standalone, comprehensive demo app showcasing all aspects of Recast & Detour's functionality - Tests: Unit tests Recast constructs a navmesh through a multi-step mesh rasterization process: 1. First Recast rasterizes the input triangle meshes into voxels. 2. Voxels in areas where agents would not be able to move are filtered and removed. 3. The walkable areas described by the voxel grid are then divided into sets of polygonal regions. 4. The navigation polygons are generated by re-triangulating the generated polygonal regions into a navmesh. You can use Recast to build a single navmesh, or a tiled navmesh. Single meshes are suitable for many simple, static cases and are easy to work with. Tiled navmeshes are more complex to work with but better support larger, more dynamic environments. Tiled meshes enable advanced Detour features like re-baking, hierarchical path-planning, and navmesh data-streaming.
For similar tasks
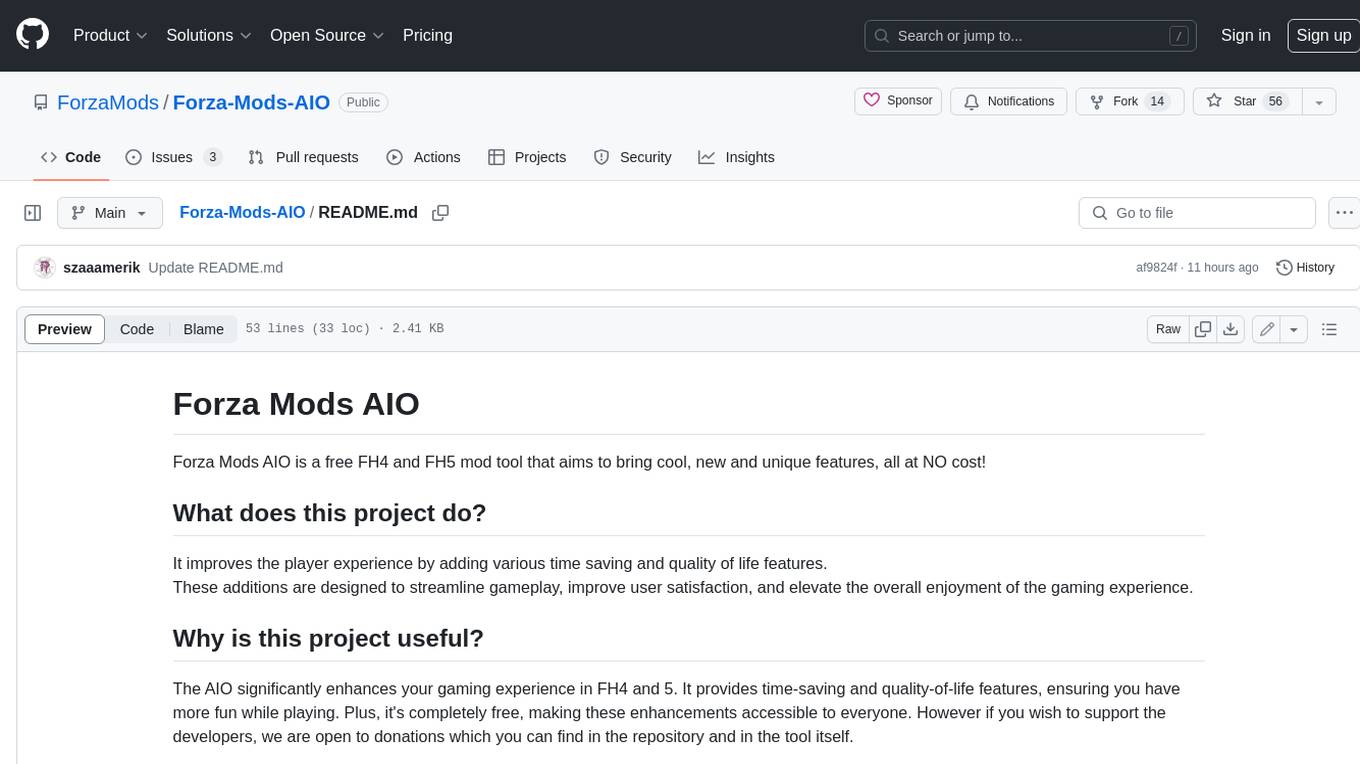
Forza-Mods-AIO
Forza Mods AIO is a free and open-source tool that enhances the gaming experience in Forza Horizon 4 and 5. It offers a range of time-saving and quality-of-life features, making gameplay more enjoyable and efficient. The tool is designed to streamline various aspects of the game, improving user satisfaction and overall enjoyment.
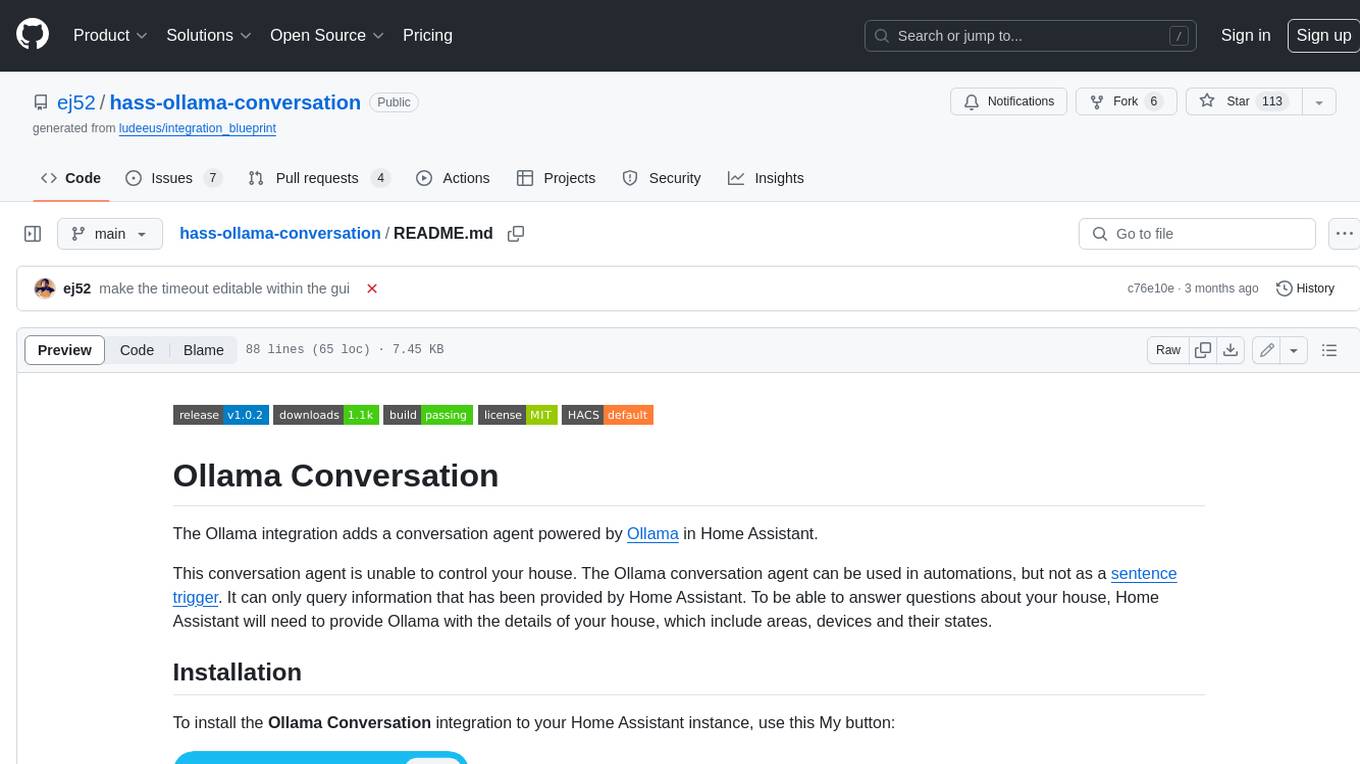
hass-ollama-conversation
The Ollama Conversation integration adds a conversation agent powered by Ollama in Home Assistant. This agent can be used in automations to query information provided by Home Assistant about your house, including areas, devices, and their states. Users can install the integration via HACS and configure settings such as API timeout, model selection, context size, maximum tokens, and other parameters to fine-tune the responses generated by the AI language model. Contributions to the project are welcome, and discussions can be held on the Home Assistant Community platform.
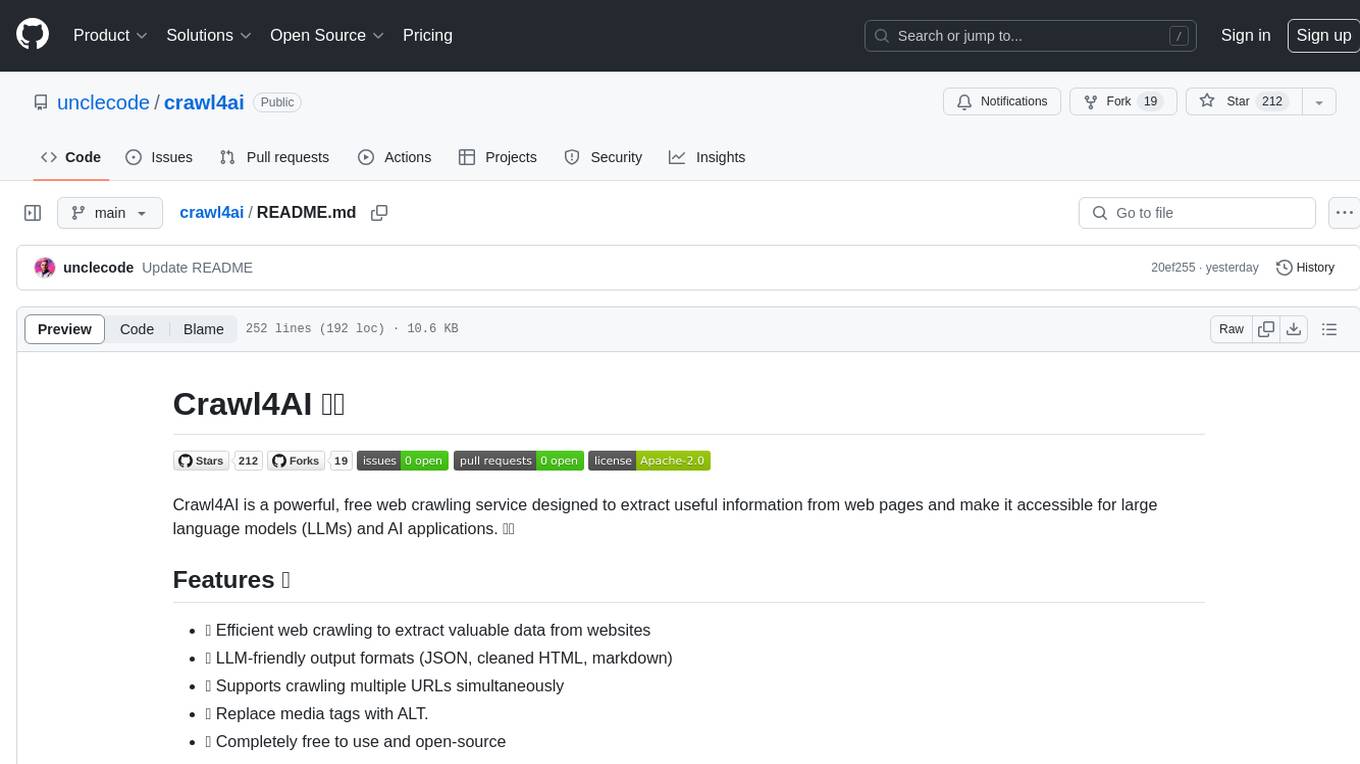
crawl4ai
Crawl4AI is a powerful and free web crawling service that extracts valuable data from websites and provides LLM-friendly output formats. It supports crawling multiple URLs simultaneously, replaces media tags with ALT, and is completely free to use and open-source. Users can integrate Crawl4AI into Python projects as a library or run it as a standalone local server. The tool allows users to crawl and extract data from specified URLs using different providers and models, with options to include raw HTML content, force fresh crawls, and extract meaningful text blocks. Configuration settings can be adjusted in the `crawler/config.py` file to customize providers, API keys, chunk processing, and word thresholds. Contributions to Crawl4AI are welcome from the open-source community to enhance its value for AI enthusiasts and developers.
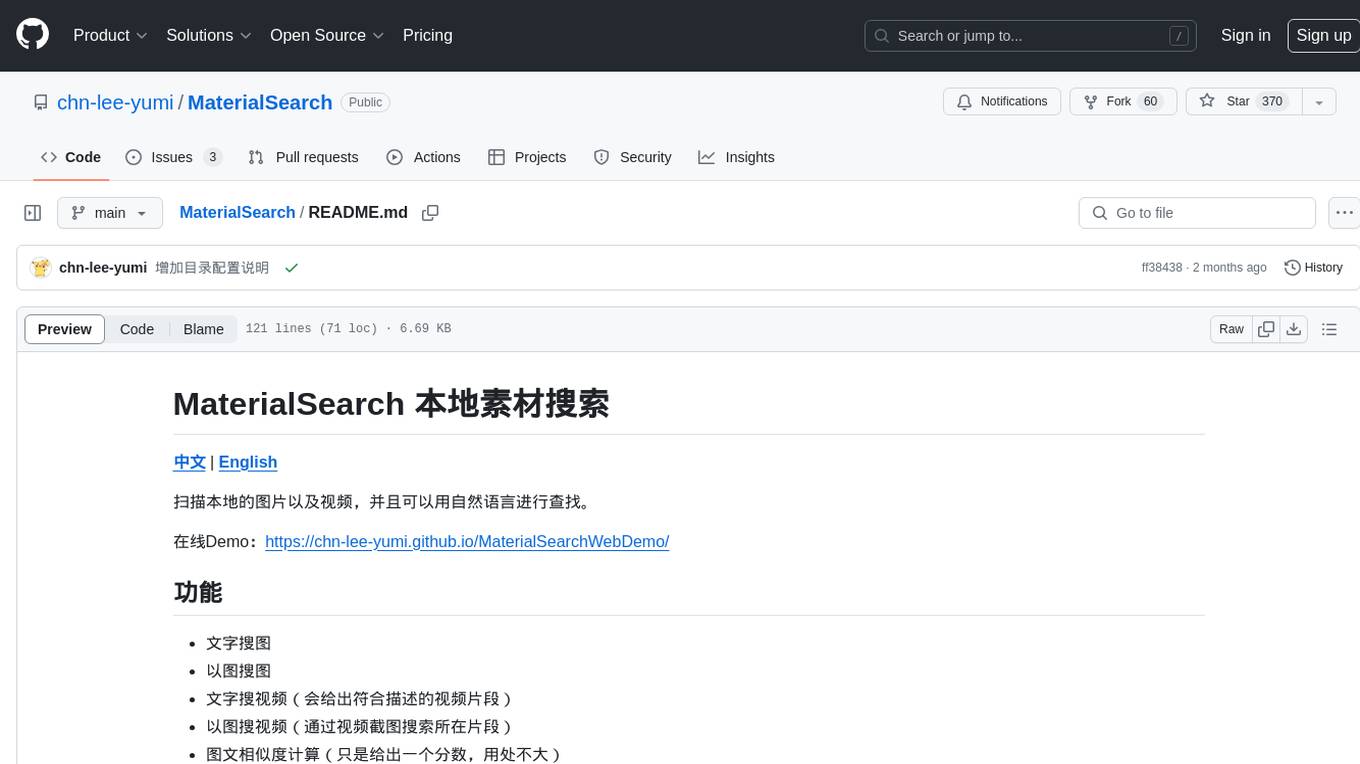
MaterialSearch
MaterialSearch is a tool for searching local images and videos using natural language. It provides functionalities such as text search for images, image search for images, text search for videos (providing matching video clips), image search for videos (searching for the segment in a video through a screenshot), image-text similarity calculation, and Pexels video search. The tool can be deployed through the source code or Docker image, and it supports GPU acceleration. Users can configure the tool through environment variables or a .env file. The tool is still under development, and configurations may change frequently. Users can report issues or suggest improvements through issues or pull requests.
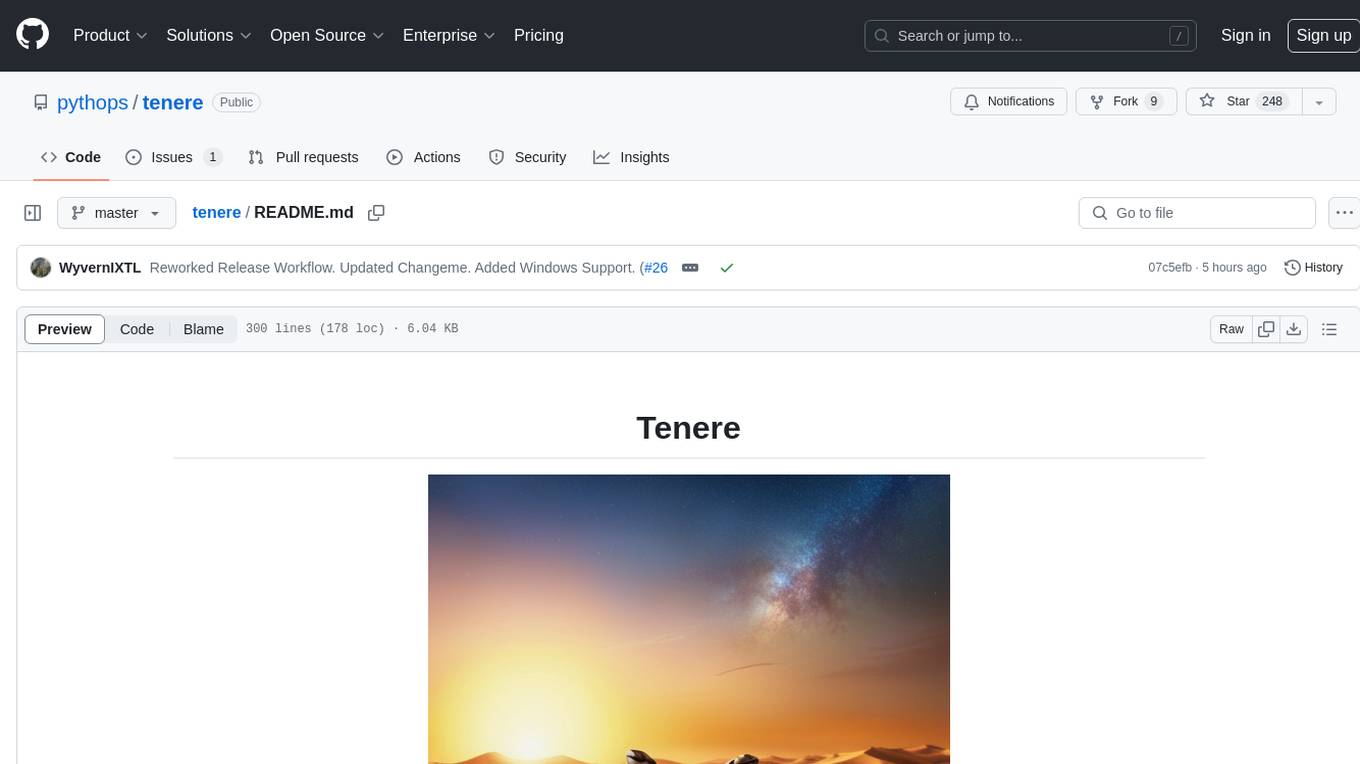
tenere
Tenere is a TUI interface for Language Model Libraries (LLMs) written in Rust. It provides syntax highlighting, chat history, saving chats to files, Vim keybindings, copying text from/to clipboard, and supports multiple backends. Users can configure Tenere using a TOML configuration file, set key bindings, and use different LLMs such as ChatGPT, llama.cpp, and ollama. Tenere offers default key bindings for global and prompt modes, with features like starting a new chat, saving chats, scrolling, showing chat history, and quitting the app. Users can interact with the prompt in different modes like Normal, Visual, and Insert, with various key bindings for navigation, editing, and text manipulation.
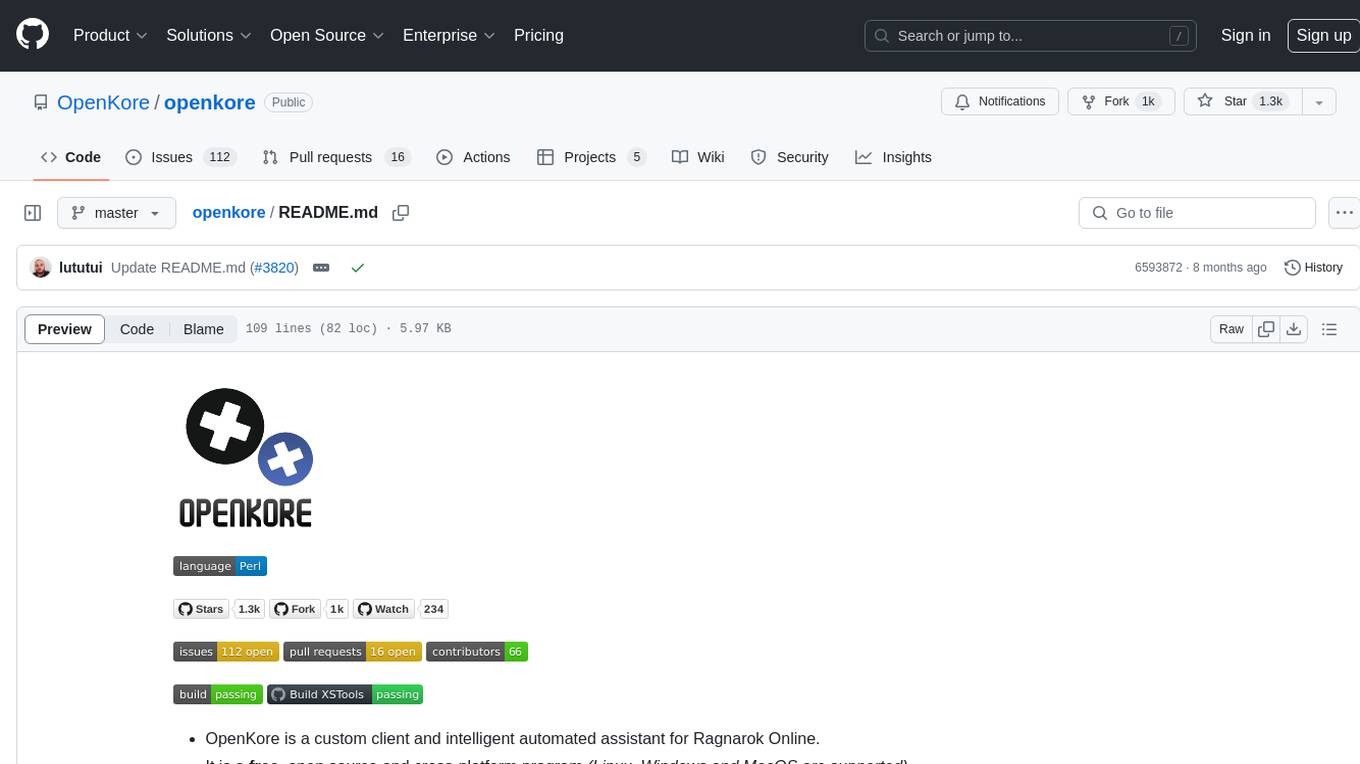
openkore
OpenKore is a custom client and intelligent automated assistant for Ragnarok Online. It is a free, open source, and cross-platform program (Linux, Windows, and MacOS are supported). To run OpenKore, you need to download and extract it or clone the repository using Git. Configure OpenKore according to the documentation and run openkore.pl to start. The tool provides a FAQ section for troubleshooting, guidelines for reporting issues, and information about botting status on official servers. OpenKore is developed by a global team, and contributions are welcome through pull requests. Various community resources are available for support and communication. Users are advised to comply with the GNU General Public License when using and distributing the software.
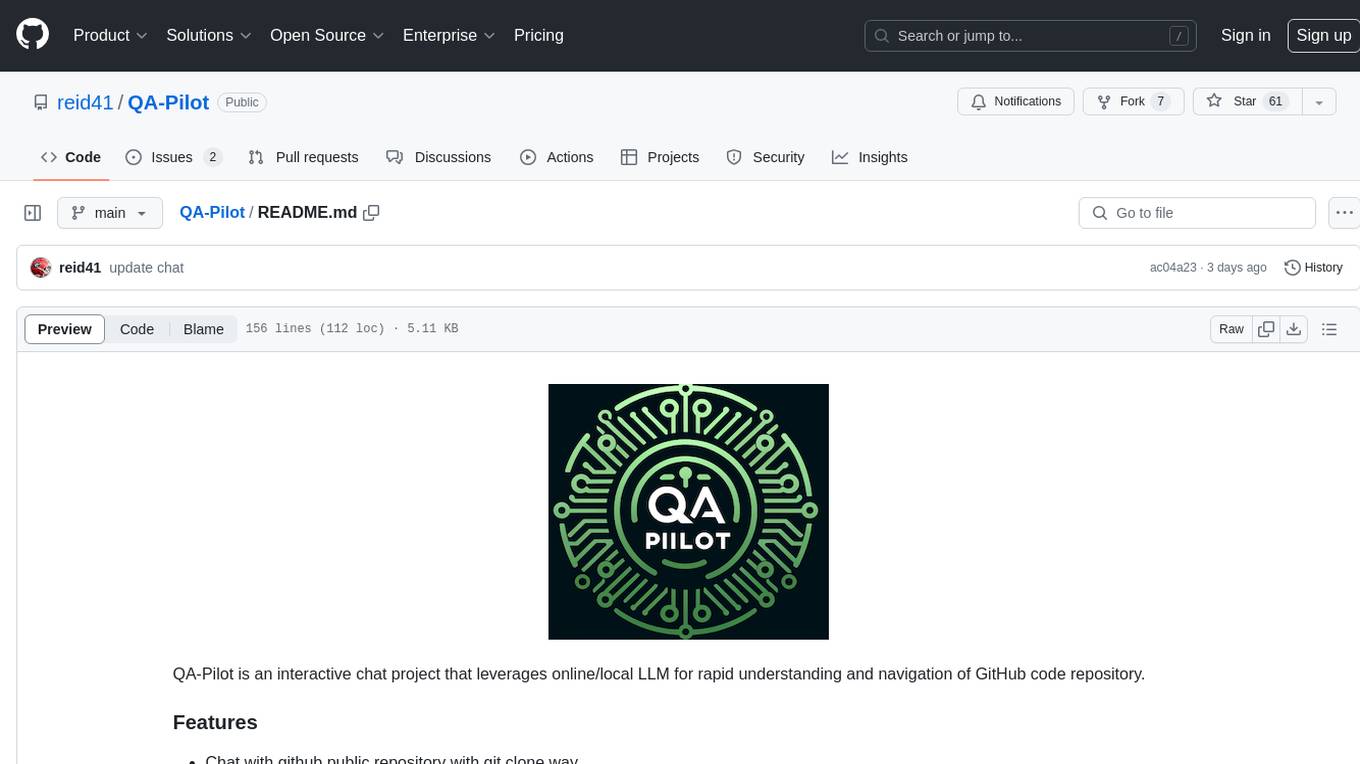
QA-Pilot
QA-Pilot is an interactive chat project that leverages online/local LLM for rapid understanding and navigation of GitHub code repository. It allows users to chat with GitHub public repositories using a git clone approach, store chat history, configure settings easily, manage multiple chat sessions, and quickly locate sessions with a search function. The tool integrates with `codegraph` to view Python files and supports various LLM models such as ollama, openai, mistralai, and localai. The project is continuously updated with new features and improvements, such as converting from `flask` to `fastapi`, adding `localai` API support, and upgrading dependencies like `langchain` and `Streamlit` to enhance performance.
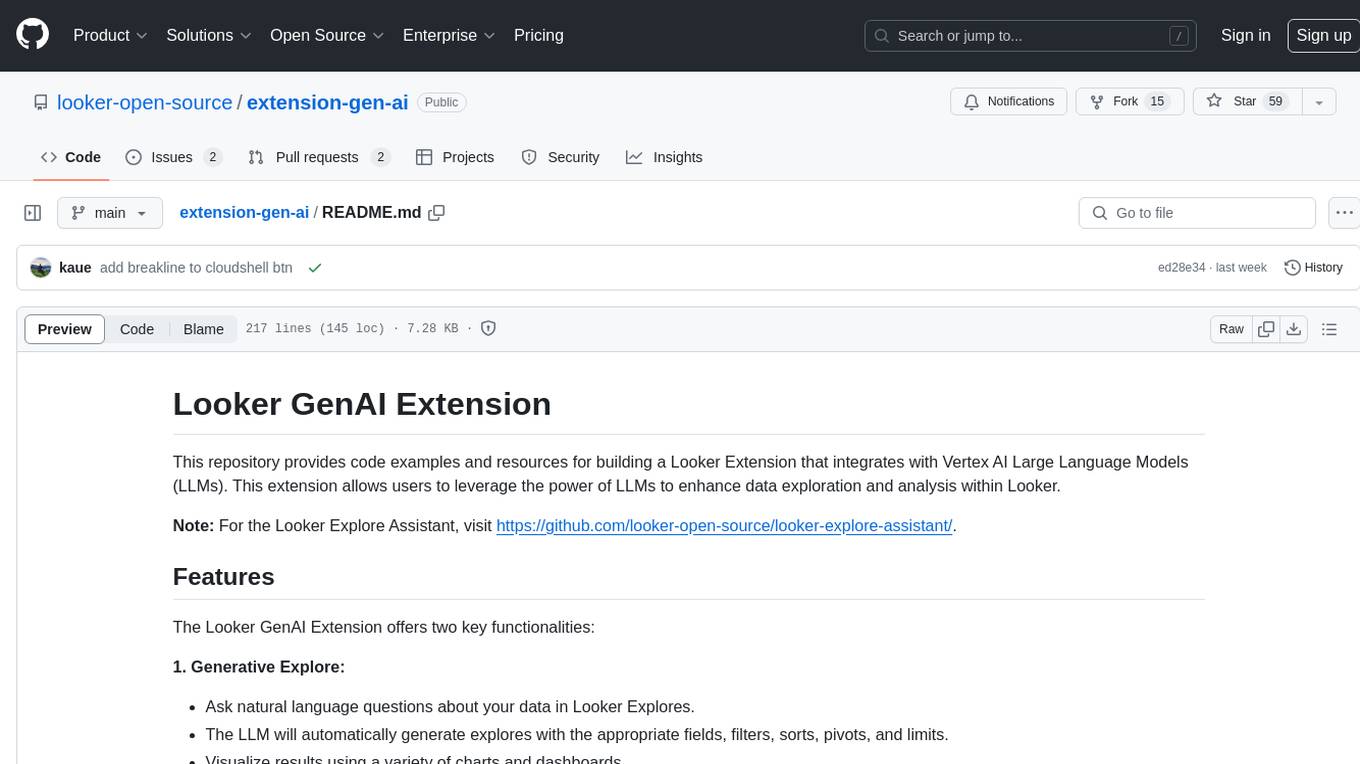
extension-gen-ai
The Looker GenAI Extension provides code examples and resources for building a Looker Extension that integrates with Vertex AI Large Language Models (LLMs). Users can leverage the power of LLMs to enhance data exploration and analysis within Looker. The extension offers generative explore functionality to ask natural language questions about data and generative insights on dashboards to analyze data by asking questions. It leverages components like BQML Remote Models, BQML Remote UDF with Vertex AI, and Custom Fine Tune Model for different integration options. Deployment involves setting up infrastructure with Terraform and deploying the Looker Extension by creating a Looker project, copying extension files, configuring BigQuery connection, connecting to Git, and testing the extension. Users can save example prompts and configure user settings for the extension. Development of the Looker Extension environment includes installing dependencies, starting the development server, and building for production.
For similar jobs
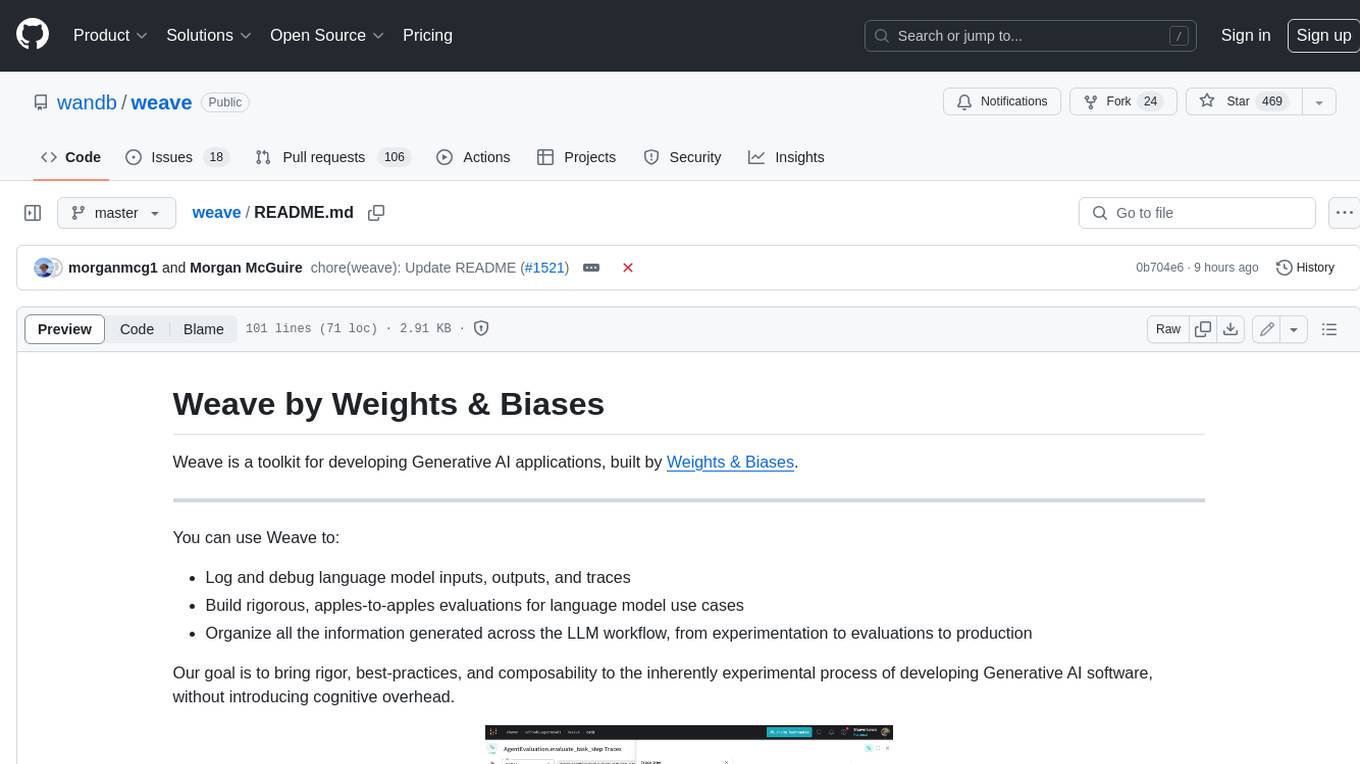
weave
Weave is a toolkit for developing Generative AI applications, built by Weights & Biases. With Weave, you can log and debug language model inputs, outputs, and traces; build rigorous, apples-to-apples evaluations for language model use cases; and organize all the information generated across the LLM workflow, from experimentation to evaluations to production. Weave aims to bring rigor, best-practices, and composability to the inherently experimental process of developing Generative AI software, without introducing cognitive overhead.
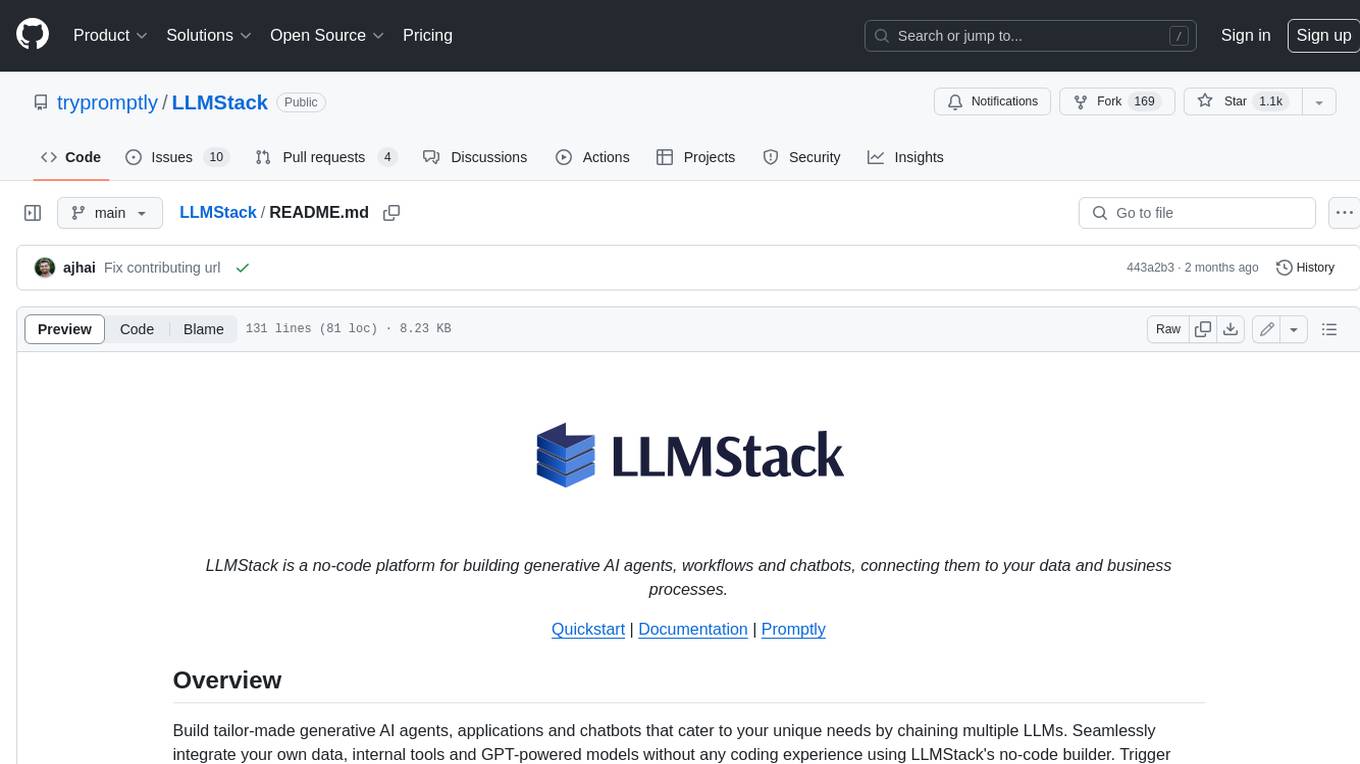
LLMStack
LLMStack is a no-code platform for building generative AI agents, workflows, and chatbots. It allows users to connect their own data, internal tools, and GPT-powered models without any coding experience. LLMStack can be deployed to the cloud or on-premise and can be accessed via HTTP API or triggered from Slack or Discord.
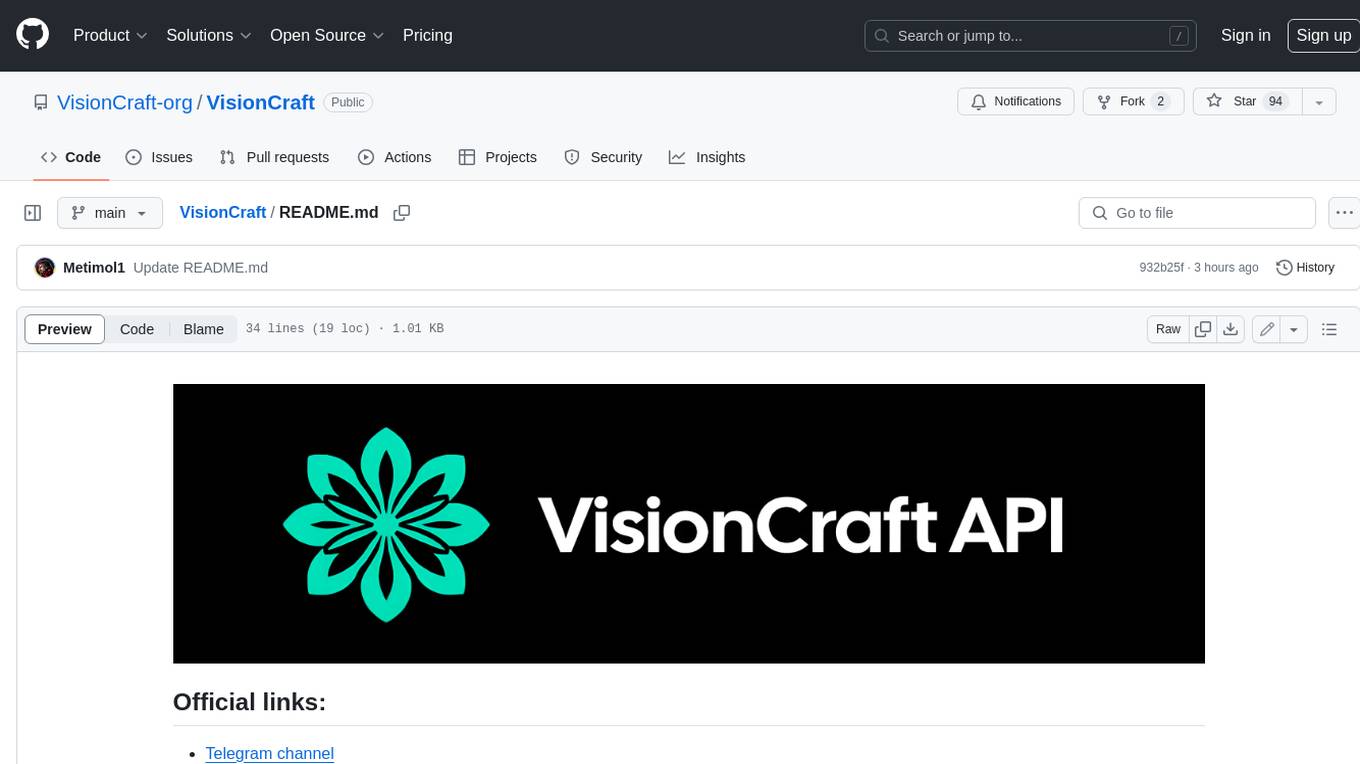
VisionCraft
The VisionCraft API is a free API for using over 100 different AI models. From images to sound.
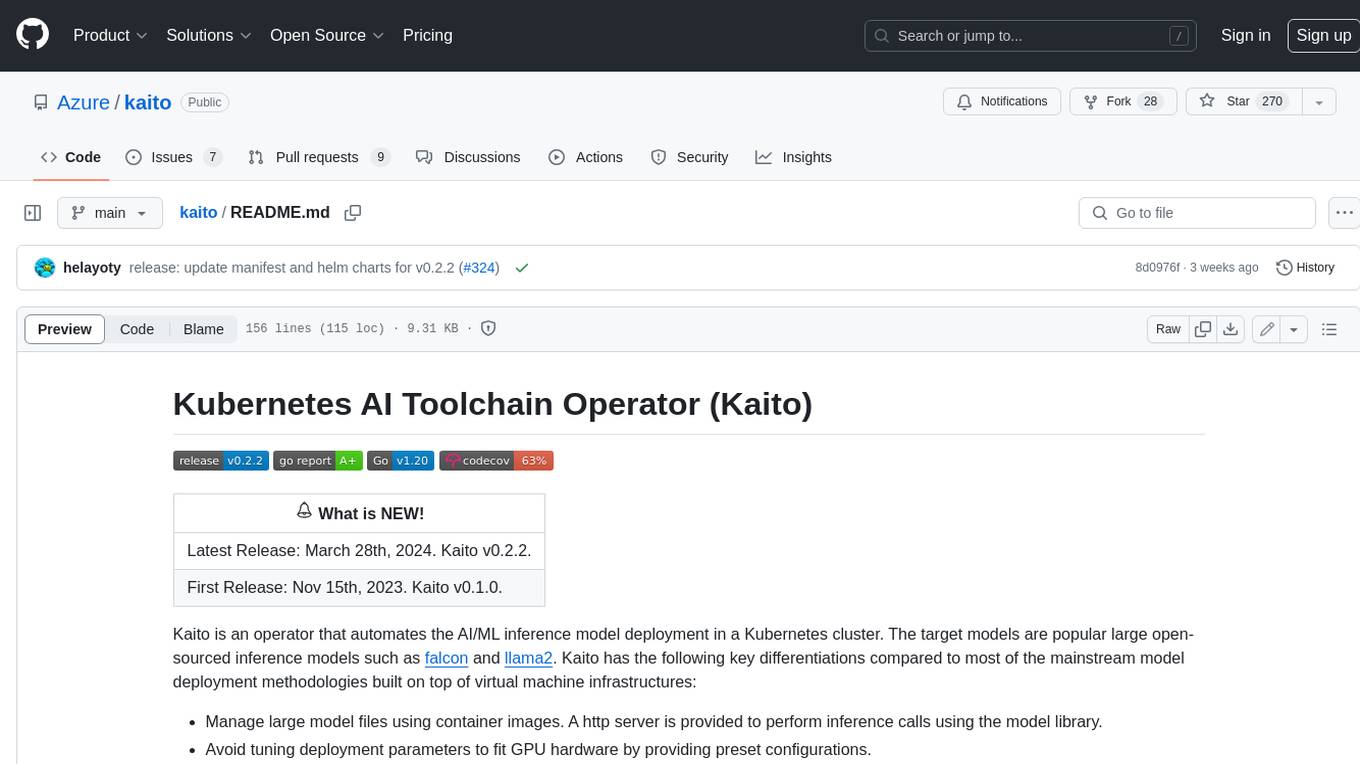
kaito
Kaito is an operator that automates the AI/ML inference model deployment in a Kubernetes cluster. It manages large model files using container images, avoids tuning deployment parameters to fit GPU hardware by providing preset configurations, auto-provisions GPU nodes based on model requirements, and hosts large model images in the public Microsoft Container Registry (MCR) if the license allows. Using Kaito, the workflow of onboarding large AI inference models in Kubernetes is largely simplified.
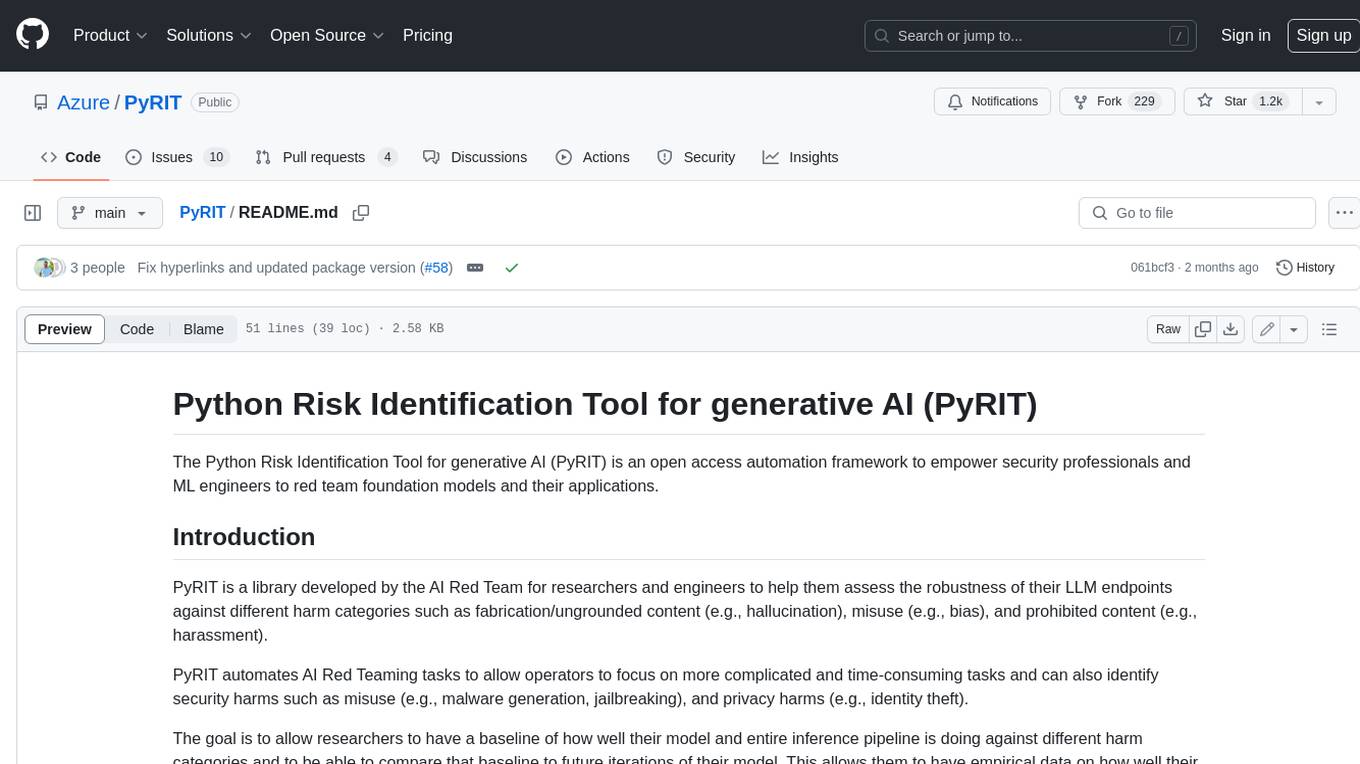
PyRIT
PyRIT is an open access automation framework designed to empower security professionals and ML engineers to red team foundation models and their applications. It automates AI Red Teaming tasks to allow operators to focus on more complicated and time-consuming tasks and can also identify security harms such as misuse (e.g., malware generation, jailbreaking), and privacy harms (e.g., identity theft). The goal is to allow researchers to have a baseline of how well their model and entire inference pipeline is doing against different harm categories and to be able to compare that baseline to future iterations of their model. This allows them to have empirical data on how well their model is doing today, and detect any degradation of performance based on future improvements.
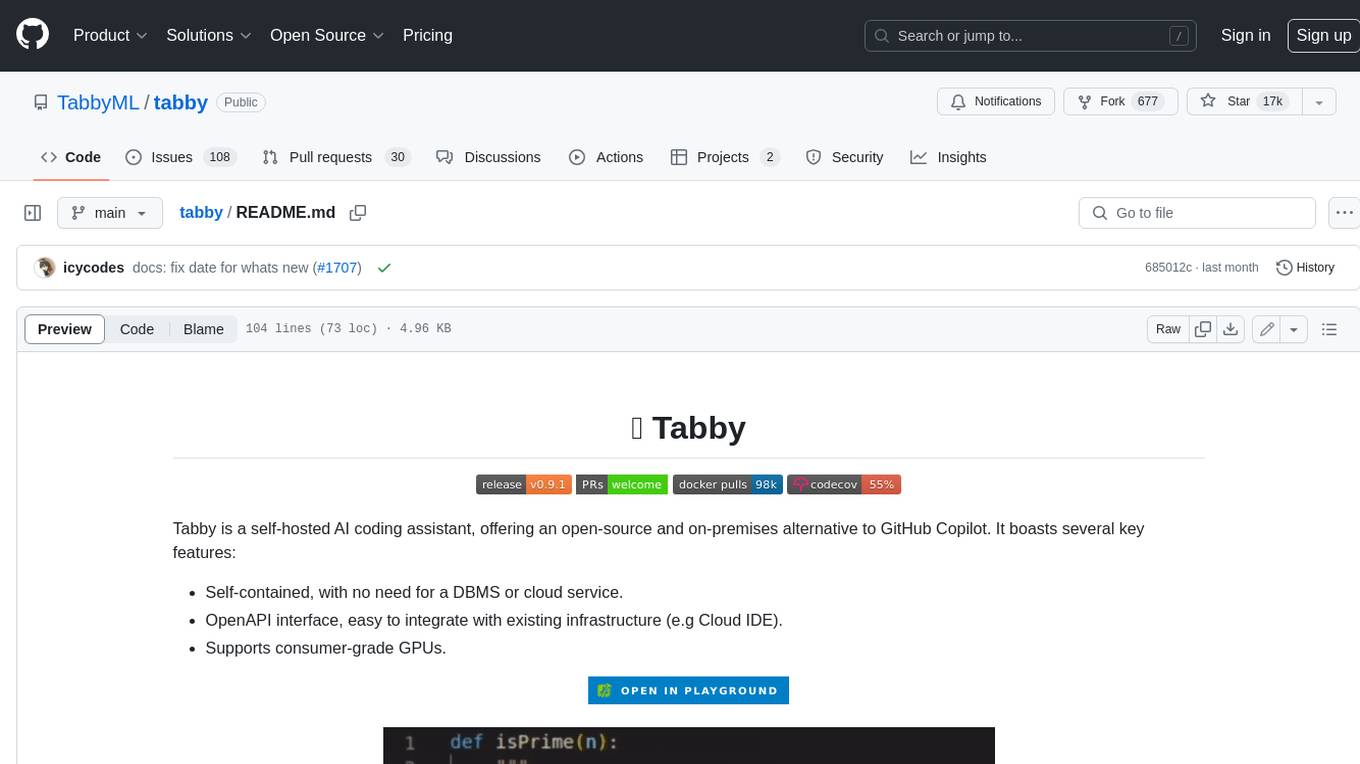
tabby
Tabby is a self-hosted AI coding assistant, offering an open-source and on-premises alternative to GitHub Copilot. It boasts several key features: * Self-contained, with no need for a DBMS or cloud service. * OpenAPI interface, easy to integrate with existing infrastructure (e.g Cloud IDE). * Supports consumer-grade GPUs.
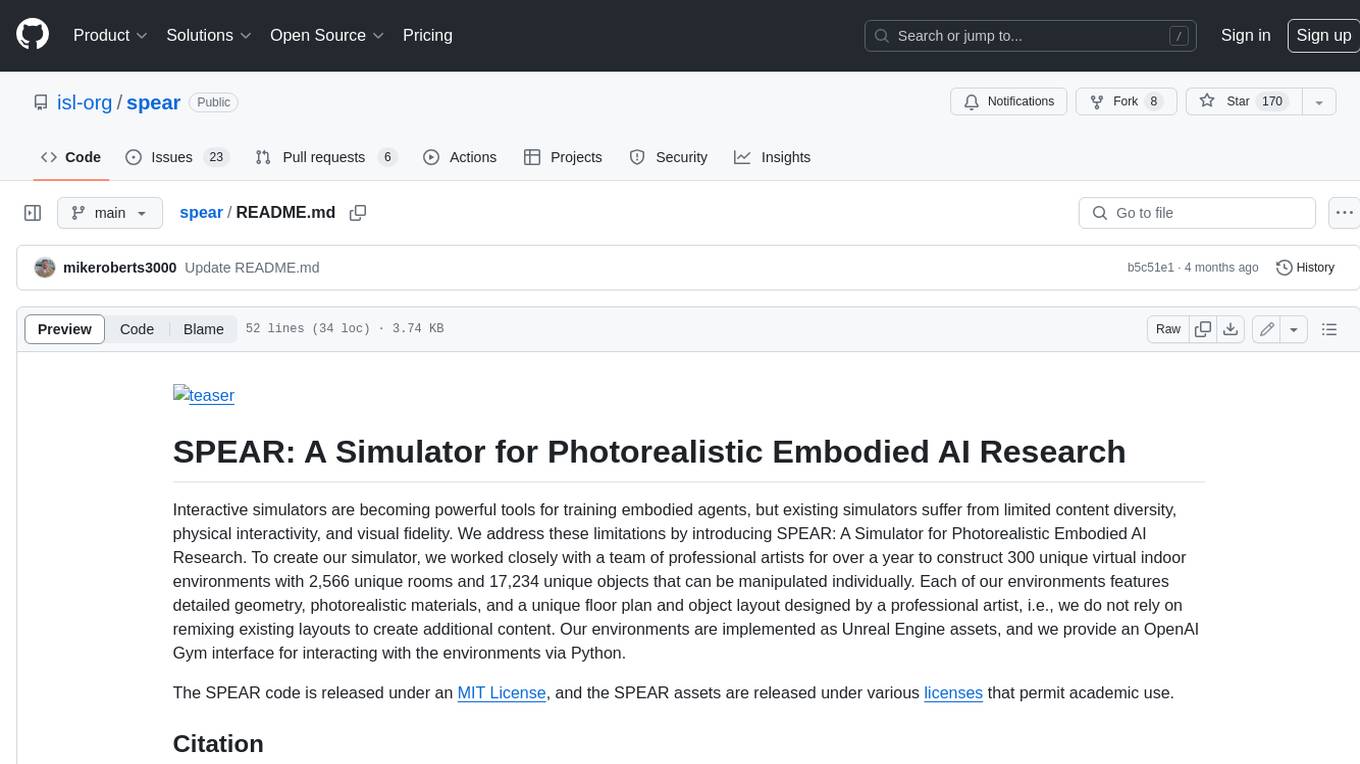
spear
SPEAR (Simulator for Photorealistic Embodied AI Research) is a powerful tool for training embodied agents. It features 300 unique virtual indoor environments with 2,566 unique rooms and 17,234 unique objects that can be manipulated individually. Each environment is designed by a professional artist and features detailed geometry, photorealistic materials, and a unique floor plan and object layout. SPEAR is implemented as Unreal Engine assets and provides an OpenAI Gym interface for interacting with the environments via Python.
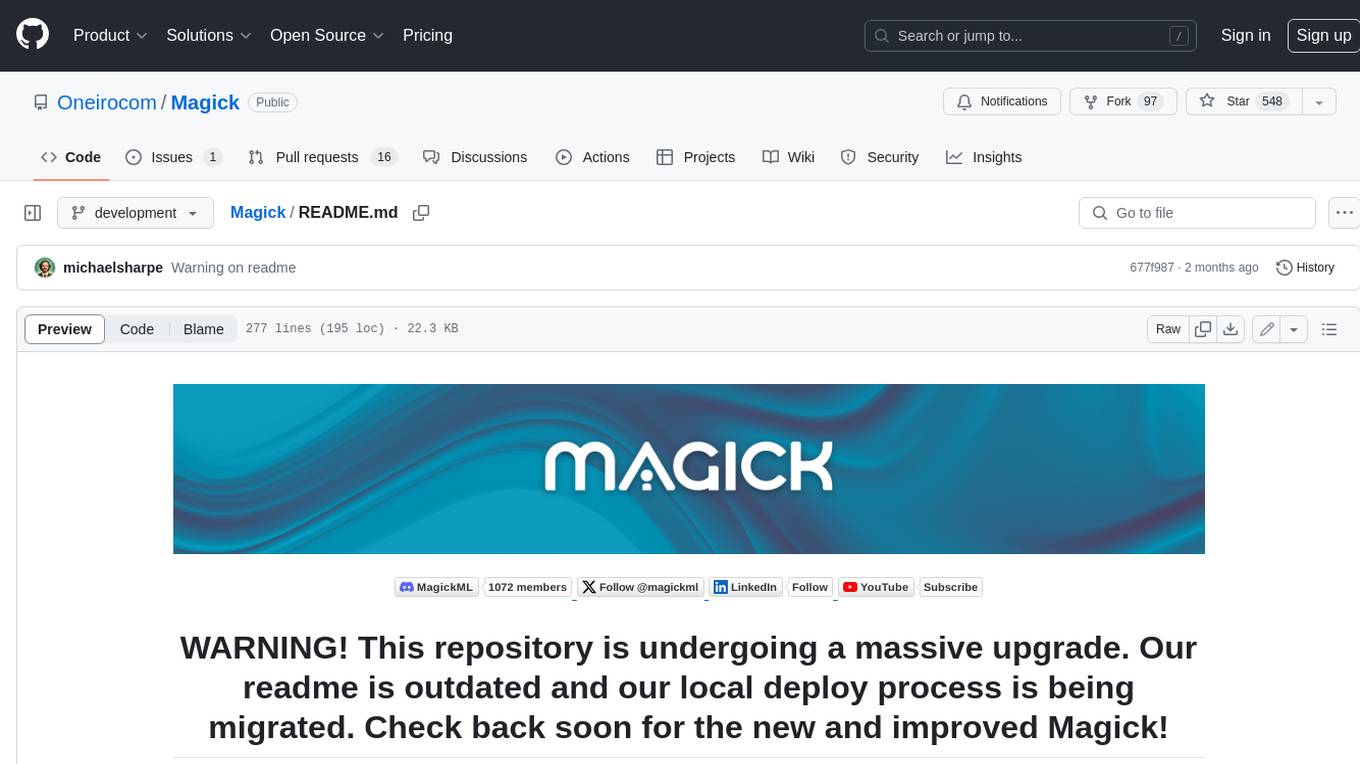
Magick
Magick is a groundbreaking visual AIDE (Artificial Intelligence Development Environment) for no-code data pipelines and multimodal agents. Magick can connect to other services and comes with nodes and templates well-suited for intelligent agents, chatbots, complex reasoning systems and realistic characters.