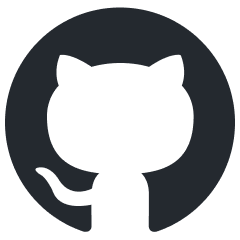
CEO-Agentic-AI-Framework
An ultra-lightweight agentic AI framework based on the ReAct paradigm.
Stars: 252
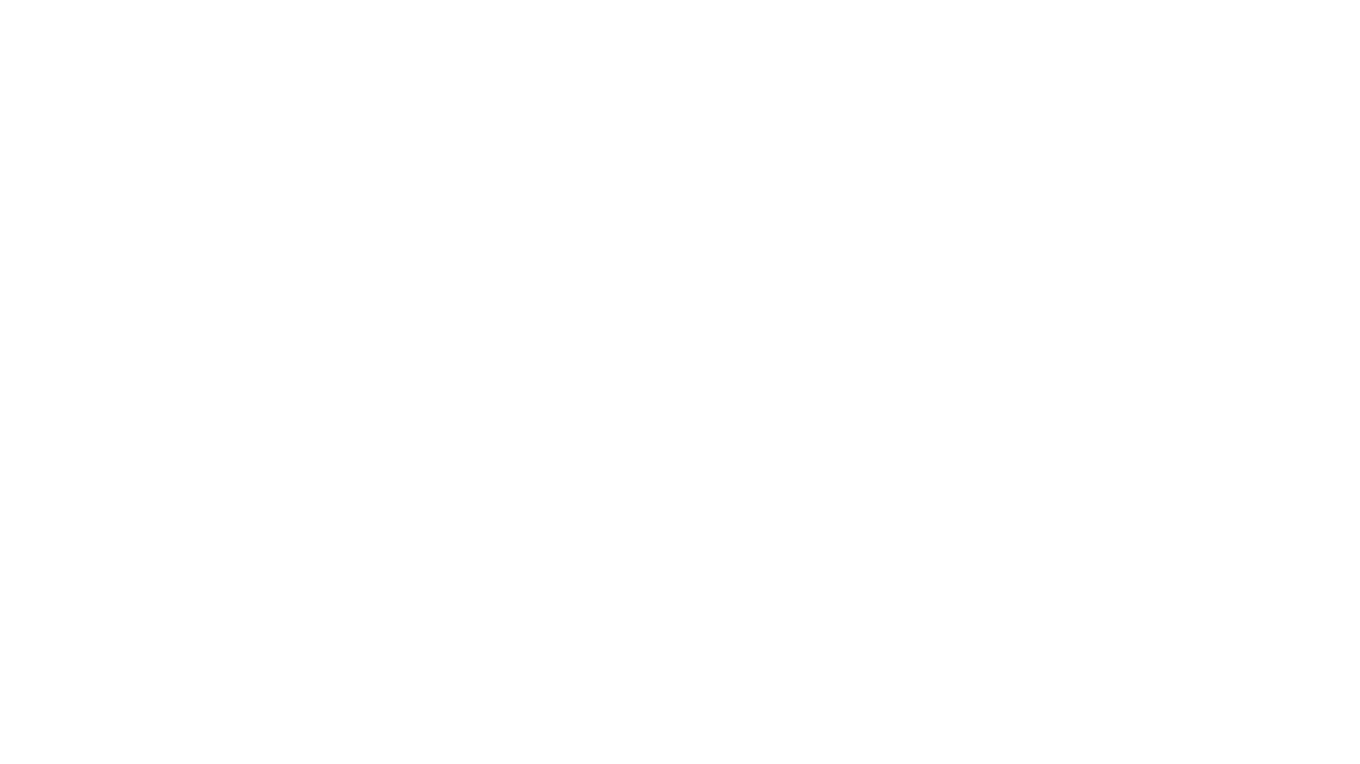
CEO-Agentic-AI-Framework is an ultra-lightweight Agentic AI framework based on the ReAct paradigm. It supports mainstream LLMs and is stronger than Swarm. The framework allows users to build their own agents, assign tasks, and interact with them through a set of predefined abilities. Users can customize agent personalities, grant and deprive abilities, and assign queries for specific tasks. CEO also supports multi-agent collaboration scenarios, where different agents with distinct capabilities can work together to achieve complex tasks. The framework provides a quick start guide, examples, and detailed documentation for seamless integration into research projects.
README:
An ultra-lightweight agentic AI framework based on the ReAct paradigm.
-
From PYPI
pip install ceo-py
-
From Github
Download .whl first then run
pip install ./ceo_py-x.x.x-py3-none-any.whl
If you are incorporating the CEO
framework into your research, please remember to properly cite it to acknowledge its contribution to your work.
Если вы интегрируете фреймворк CEO
в своё исследование, пожалуйста, не забудьте правильно сослаться на него, указывая его вклад в вашу работу.
もしあなたが研究に CEO
フレームワークを組み入れているなら、その貢献を認めるために適切に引用することを忘れないでください.
如果您正在將 CEO
框架整合到您的研究中,請務必正確引用它,以聲明它對您工作的貢獻.
@software {CEO,
author = {Zihao Wu},
title = {CEO: An ultra-lightweight agentic AI framework based on the ReAct paradigm},
publisher = {Github},
howpublished = {\url{https://github.com/vortezwohl/CEO-Agentic-AI-Framework}},
year = {2024},
date = {2024-10-25}
}
To start building your own agent, follow the steps listed.
-
set environmental variable
OPENAI_API_KEY
# .env OPENAI_API_KEY=sk-...
-
bring in SDKs from
CEO
-
Agent
lets you instantiate an agent. -
Personality
is an enumeration class used for customizing personalities of agents.-
Personality.PRUDENT
makes the agent's behavior more cautious. -
Personality.INQUISITIVE
encourages the agent to be more proactive in trying and exploring.
-
-
get_openai_model
gives you aBaseChatModel
as thought engine. -
@ability(brain: BaseChatModel, cache: bool = True, cache_dir: str = '')
is a decorator which lets you declare a function as anAbility
. -
@agentic(agent: Agent)
is a decorator which lets you declare a function as anAgenticAbility
.
from ceo import ( Agent, Personality, get_openai_model, ability, agentic )
-
-
declare functions as basic abilities
@ability def calculator(expr: str) -> float: # this function only accepts a single math expression return simplify(expr) @ability def write_file(filename: str, content: str) -> str: with open(filename, 'w', encoding='utf-8') as f: f.write(content) return f'{content} written to {filename}.'
-
instantiate an agent
You can grant abilities to agents while instantiating them.
model = get_openai_model() agent = Agent(abilities=[calculator, write_file], brain=model, name='CEO', personality=Personality.INQUISITIVE)
-
You can also grant more abilities to agents later:
agent.grant_ability(calculator)
or
agent.grant_abilities([calculator])
-
To deprive abilities:
agent.deprive_ability(calculator)
or
agent.deprive_abilities([calculator])
You can change an agent's personality using method
change_personality(personality: Personality)
agent.change_personality(Personality.PRUDENT)
-
-
assign a request to your agent
agent.assign("Here is a sphere with radius of (1 * 9.5 / 2 * 2) cm and pi here is 3.14159, find the area and volume respectively then write the results into a file called 'result.txt'.")
-
leave the rest to your agent
response = agent.just_do_it() print(response)
ceo
also supports multi-agent collaboration scenario, declare a function as agent calling ability with@agentic(agent: Agent)
, then grant it to an agent. See example.
-
-
Find the surface area and volume of a sphere and write the results into a file.
import logging from ceo import ( Agent, Personality, get_openai_model, ability ) from sympy import simplify from dotenv import load_dotenv load_dotenv() logging.getLogger('ceo').setLevel(logging.DEBUG) @ability def calculator(expr: str) -> float: # this function only accepts a single math expression return simplify(expr) @ability def write_file(filename: str, content: str) -> str: with open(filename, 'w', encoding='utf-8') as f: f.write(content) return f'{content} written to {filename}.' if __name__ == '__main__': ceo = Agent(abilities=[calculator, write_file], brain=get_openai_model(), name='CEO', personality=Personality.INQUISITIVE) radius = '(10.01 * 10.36 * 3.33 / 2 * 16)' # 2762.663904 pi = 3.14159 output_file = 'result.txt' request = f"Here is a sphere with radius of {radius} cm and pi here is {pi}, find the area and volume respectively then write the results into a file called '{output_file}'." result = ceo.assign(request).just_do_it() # area = 95910378.2949379, volume = 88322713378.13666 print(result)
# result.txt Surface Area: 95910378.29 cm², Volume: 88322713378.14 cm³
# stdout [DEBUG] 2025-01-12 01:33:50,780 ceo : Agent: CEO; Expected steps: 4; Request: "Here is a sphere with radius of (10.01 * 10.36 * 3.33 / 2 * 16) cm and pi here is 3.14159, find the area and volume respectively then write the results into a file called 'result.txt'."; [DEBUG] 2025-01-12 01:34:05,491 ceo : Agent: CEO; Memory size: 1; Memory update: I used the calculator ability to evaluate the mathematical expression '(10.01 * 10.36 * 3.33 / 2 * 16)', and the result is 2762.66390400000, which indicates the computed value of the expression.; [DEBUG] 2025-01-12 01:34:12,953 ceo : Agent: CEO; Memory size: 2; Memory update: I used the calculator ability to evaluate the expression '4 * 3.14159 * (2762.66390400000^2)', and the result is 95910378.2949379, which represents the calculated value of the mathematical expression.; [DEBUG] 2025-01-12 01:34:22,476 ceo : Agent: CEO; Memory size: 3; Memory update: I used the calculator ability to evaluate the expression '(4/3) * 3.14159 * (2762.66390400000^3)', and the result shows '88322713378.1367', which indicates the computed volume of a sphere with the given radius.; [DEBUG] 2025-01-12 01:34:34,233 ceo : Agent: CEO; Memory size: 4; Memory update: I used the write_file ability to create or overwrite a file named 'result.txt' with the specified content detailing the surface area and volume. The result confirms that the content was successfully written to the file.; [DEBUG] 2025-01-12 01:34:47,573 ceo : Agent: CEO; Conclusion: Your request has been fully achieved. The calculations for the surface area and volume were performed correctly, and the results were successfully written to the file 'result.txt'.; [DEBUG] 2025-01-12 01:34:47,573 ceo : Agent: CEO; Step count: 4; Time used: 59.79923509992659 seconds; {'success': True, 'conclusion': "Your request has been fully achieved. The calculations for the surface area and volume were performed correctly, and the results were successfully written to the file 'result.txt'.", 'raw_response': "--THOUGHT-PROCESS-- \n(Initial calculation) [Calculate radius]: The radius was calculated as (10.01 * 10.36 * 3.33 / 2 * 16) resulting in 2762.66390400000 cm. (--SUCCESS--) \n(After: Calculate radius) [Calculate surface area]: The surface area was calculated using the formula '4 * π * r²', yielding 95910378.2949379 cm². (--SUCCESS--) \n(After: Calculate surface area) [Calculate volume]: The volume was calculated using the formula '(4/3) * π * r³', resulting in 88322713378.1367 cm³. (--SUCCESS--) \n(After: Calculate volume) [Write results to file]: The results were successfully written to 'result.txt' with the correct surface area and volume. (--SUCCESS--) \n\nBased on above assessments, here is my conclusion: \n--CONCLUSION-- \nYour request has been fully achieved. The calculations for the surface area and volume were performed correctly, and the results were successfully written to the file 'result.txt'. \n--END--", 'misc': {'time_used': 59.79923509992659, 'step_count': 4}}
-
-
-
Ask the suitable agents to find the surface area and volume of a sphere and write the results into a file.
import logging from sympy import simplify from dotenv import load_dotenv from ceo import ( Agent, Personality, get_openai_model, agentic, ability ) load_dotenv() logging.getLogger('ceo').setLevel(logging.DEBUG) model = get_openai_model() @ability def calculator(expr: str) -> float: # this function only accepts a single math expression return simplify(expr) @ability def write_file(filename: str, content: str) -> str: with open(filename, 'w', encoding='utf-8') as f: f.write(content) return f'{content} written to {filename}.' jack = Agent(abilities=[calculator], brain=model, name='Jack', personality=Personality.INQUISITIVE) tylor = Agent(abilities=[write_file], brain=model, name='Tylor', personality=Personality.PRUDENT) @agentic(jack) def agent1(): return @agentic(tylor) def agent2(): return if __name__ == '__main__': ceo = Agent(abilities=[agent1, agent2], brain=model, name='CEO', personality=Personality.INQUISITIVE) radius = '(10.01 * 10.36 * 3.33 / 2 * 16)' # 2762.663904 pi = 3.14159 output_file = 'result.txt' request = f"Here is a sphere with radius of {radius} cm and pi here is {pi}, find the area and volume respectively then write the results into a file called '{output_file}'." result = ceo.assign(request).just_do_it() # area = 95910378.2949379, volume = 88322713378.13666 print(result)
In multi-agent collaboration scenario, you can assign different personalities to each distinct agent. For example, in the aforementioned script, Jack's capability is to perform calculations. I want him to try more and explore more, so Jack's personality is set to
Personality.INQUISITIVE
. On the other hand, Taylor's capability is to create and write files. For operations involving interaction with the external file system, I want him to be more cautious, so Taylor's personality is set toPersonality.PRUDENT
.# result.txt Surface Area: 95910378.2949379 cm² Volume: 88322713378.1367 cm³
# stdout [DEBUG] 2025-01-12 01:41:36,139 ceo : Agent: CEO; Expected steps: 4; Request: "Here is a sphere with radius of (10.01 * 10.36 * 3.33 / 2 * 16) cm and pi here is 3.14159, find the area and volume respectively then write the results into a file called 'result.txt'."; [DEBUG] 2025-01-12 01:41:41,870 ceo : Agent: Jack; Memory brought in: 0; [DEBUG] 2025-01-12 01:41:45,642 ceo : Agent: Jack; Expected steps: 3; Request: "Here is a sphere with radius of (10.01 * 10.36 * 3.33 / 2 * 16) cm and pi here is 3.14159, find the area and volume respectively then write the results into a file called 'result.txt'."; [DEBUG] 2025-01-12 01:41:52,758 ceo : Agent: Jack; Memory size: 1; Memory update: I used the calculator ability to evaluate the expression '(10.01 * 10.36 * 3.33 / 2 * 16)', and the result is '2762.66390400000', which indicates the computed value of the mathematical expression.; [DEBUG] 2025-01-12 01:42:03,734 ceo : Agent: Jack; Memory size: 2; Memory update: I used the calculator ability to evaluate the expression '4 * 3.14159 * (2762.66390400000^2)', and the result shows '95910378.2949379', which indicates the computed value of the mathematical expression.; [DEBUG] 2025-01-12 01:42:10,908 ceo : Agent: Jack; Memory size: 3; Memory update: I used the calculator ability to evaluate the expression '(4/3) * 3.14159 * (2762.66390400000^3)', and the result shows '88322713378.1367', which indicates the computed volume of a sphere with a radius of approximately 2762.66.; [DEBUG] 2025-01-12 01:42:22,210 ceo : Agent: Jack; Conclusion: Your request has not been fully achieved. I successfully calculated the radius, surface area, and volume of the sphere, but there is no record of writing these results into the file 'result.txt'.; [DEBUG] 2025-01-12 01:42:22,210 ceo : Agent: Jack; Step count: 3; Time used: 40.33986619999632 seconds; [DEBUG] 2025-01-12 01:42:29,049 ceo : Agent: CEO; Memory size: 1; Memory update: I used the __AgenticAbility__talk_to_Jack ability to ask Jack for a favor. Jack successfully calculated the radius, surface area, and volume of a sphere based on the given expression, but failed to write the results to 'result.txt', resulting in an incomplete request.; [DEBUG] 2025-01-12 01:42:44,713 ceo : Agent: Tylor; Memory brought in: 1; [DEBUG] 2025-01-12 01:42:47,498 ceo : Agent: Tylor; Expected steps: 1; Request: "Here is a sphere with radius of (10.01 * 10.36 * 3.33 / 2 * 16) cm and pi here is 3.14159, find the area and volume respectively then write the results into a file called 'result.txt'."; [DEBUG] 2025-01-12 01:42:56,955 ceo : Agent: Tylor; Memory size: 2; Memory update: I used the write_file ability to write the specified content about surface area and volume to a file named 'result.txt'. The result confirms that the content was successfully written to the file.; [DEBUG] 2025-01-12 01:43:06,851 ceo : Agent: Tylor; Conclusion: Your request has been fully achieved. The surface area and volume of the sphere were calculated successfully, and the results were written to 'result.txt'. Results: Surface Area: 95910378.2949379 cm² Volume: 88322713378.1367 cm³; [DEBUG] 2025-01-12 01:43:06,851 ceo : Agent: Tylor; Step count: 1; Time used: 22.138400600058958 seconds; [DEBUG] 2025-01-12 01:43:12,070 ceo : Agent: CEO; Memory size: 2; Memory update: I used the __AgenticAbility__talk_to_Tylor ability to ask Tylor for a favor, which involved calculating the surface area and volume of a sphere based on given dimensions. The result indicates success, confirming that the calculations were performed accurately and the results were saved to 'result.txt'.; [DEBUG] 2025-01-12 01:43:20,457 ceo : Agent: CEO; Conclusion: Your request has been fully achieved. The surface area and volume of the sphere were calculated successfully, and the results were written to 'result.txt'. Results: Surface Area: 95910378.2949379 cm² Volume: 88322713378.1367 cm³; [DEBUG] 2025-01-12 01:43:20,457 ceo : Agent: CEO; Step count: 2; Time used: 108.47770960000344 seconds; {'success': True, 'conclusion': "Your request has been fully achieved. The surface area and volume of the sphere were calculated successfully, and the results were written to 'result.txt'. \nResults: \nSurface Area: 95910378.2949379 cm² \nVolume: 88322713378.1367 cm³", 'raw_response': "--THOUGHT-PROCESS-- \n(Initial calculation) [Calculating radius]: I evaluated the expression '(10.01 * 10.36 * 3.33 / 2 * 16)' and obtained '2762.66390400000'. (--SUCCESS--) \n(Area calculation) [Calculating surface area]: I evaluated the expression '4 * 3.14159 * (2762.66390400000^2)' and obtained '95910378.2949379'. (--SUCCESS--) \n(Volume calculation) [Calculating volume]: I evaluated the expression '(4/3) * 3.14159 * (2762.66390400000^3)' and obtained '88322713378.1367'. (--SUCCESS--) \n(Write results) [Writing to file]: Tylor successfully wrote the results to 'result.txt'. (--SUCCESS--) \n\nBased on above assessments, here is my conclusion: \n--CONCLUSION-- \nYour request has been fully achieved. The surface area and volume of the sphere were calculated successfully, and the results were written to 'result.txt'. \nResults: \nSurface Area: 95910378.2949379 cm² \nVolume: 88322713378.1367 cm³ \n--END-- ", 'misc': {'time_used': 108.47770960000344, 'step_count': 2}}
-
For Tasks:
Click tags to check more tools for each tasksFor Jobs:
Alternative AI tools for CEO-Agentic-AI-Framework
Similar Open Source Tools
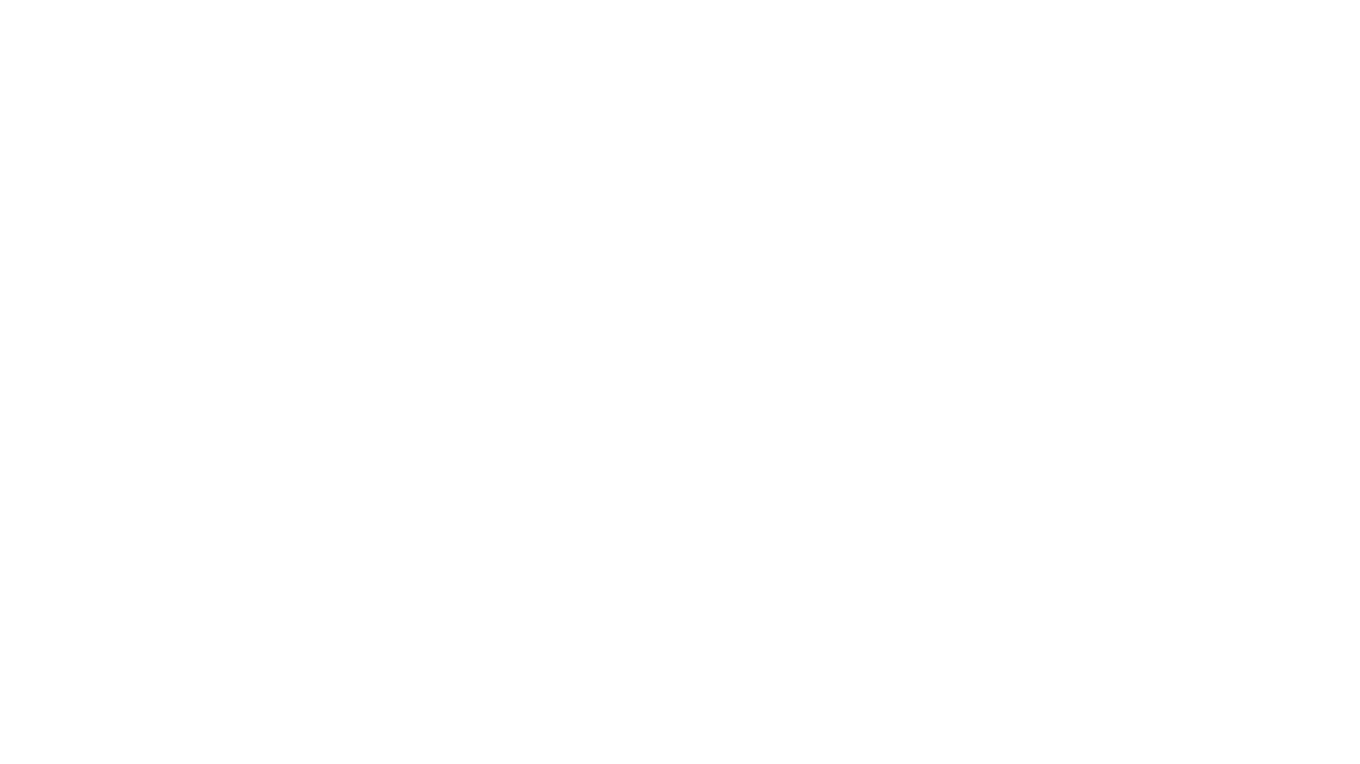
CEO-Agentic-AI-Framework
CEO-Agentic-AI-Framework is an ultra-lightweight Agentic AI framework based on the ReAct paradigm. It supports mainstream LLMs and is stronger than Swarm. The framework allows users to build their own agents, assign tasks, and interact with them through a set of predefined abilities. Users can customize agent personalities, grant and deprive abilities, and assign queries for specific tasks. CEO also supports multi-agent collaboration scenarios, where different agents with distinct capabilities can work together to achieve complex tasks. The framework provides a quick start guide, examples, and detailed documentation for seamless integration into research projects.
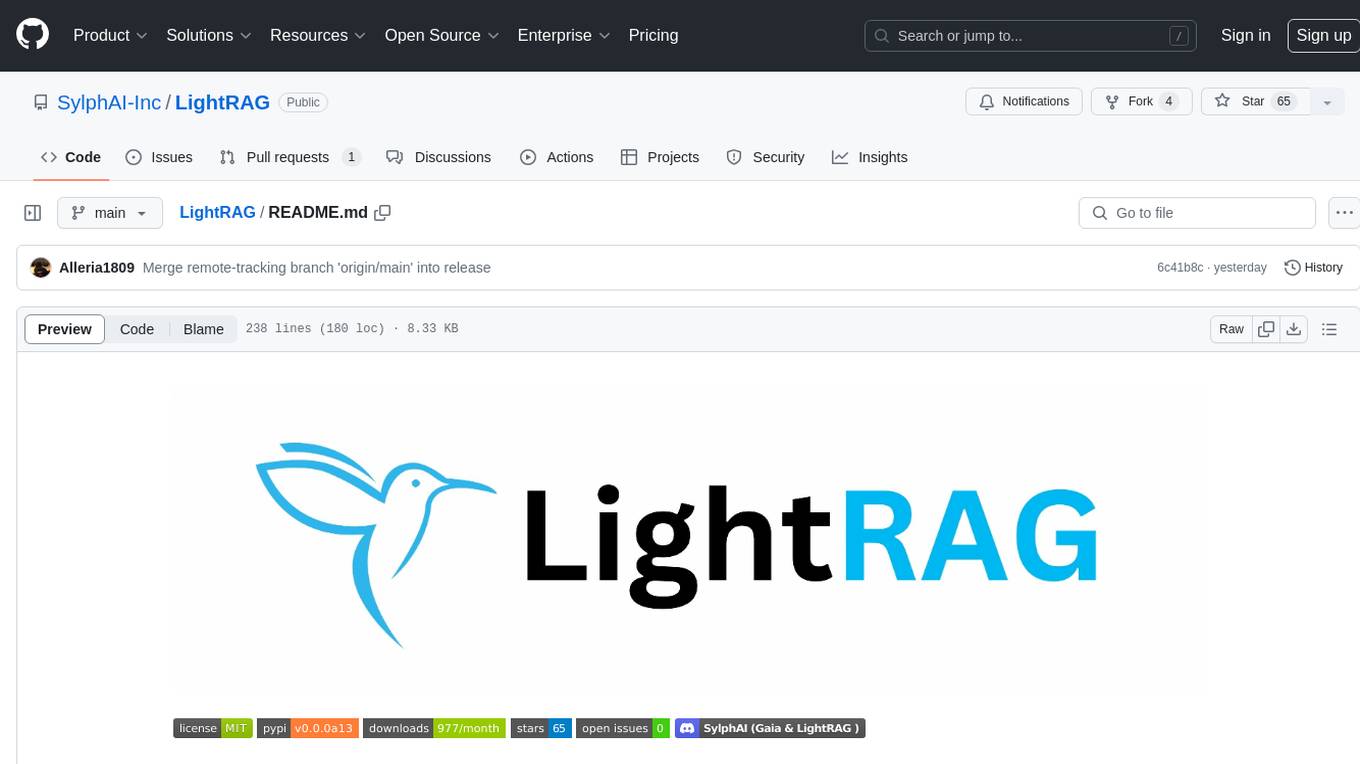
LightRAG
LightRAG is a PyTorch library designed for building and optimizing Retriever-Agent-Generator (RAG) pipelines. It follows principles of simplicity, quality, and optimization, offering developers maximum customizability with minimal abstraction. The library includes components for model interaction, output parsing, and structured data generation. LightRAG facilitates tasks like providing explanations and examples for concepts through a question-answering pipeline.
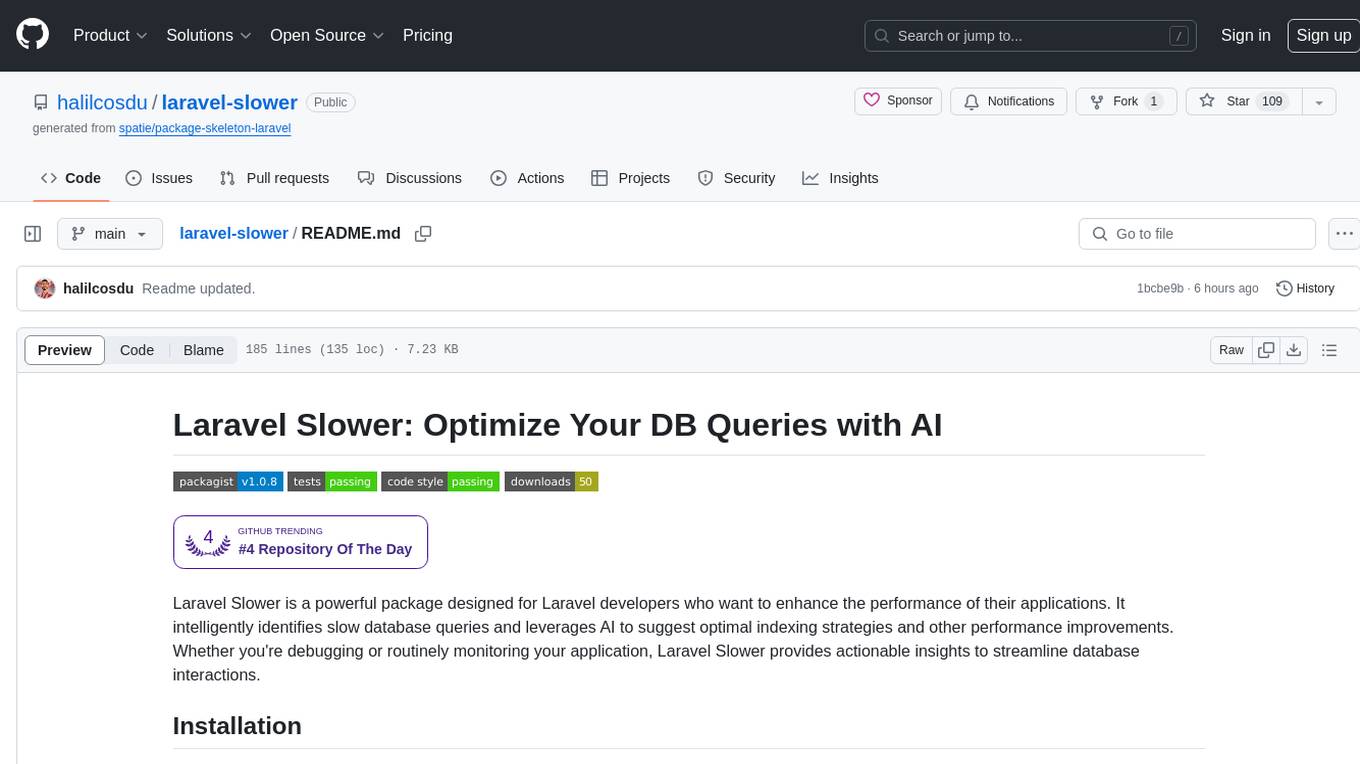
laravel-slower
Laravel Slower is a powerful package designed for Laravel developers to optimize the performance of their applications by identifying slow database queries and providing AI-driven suggestions for optimal indexing strategies and performance improvements. It offers actionable insights for debugging and monitoring database interactions, enhancing efficiency and scalability.
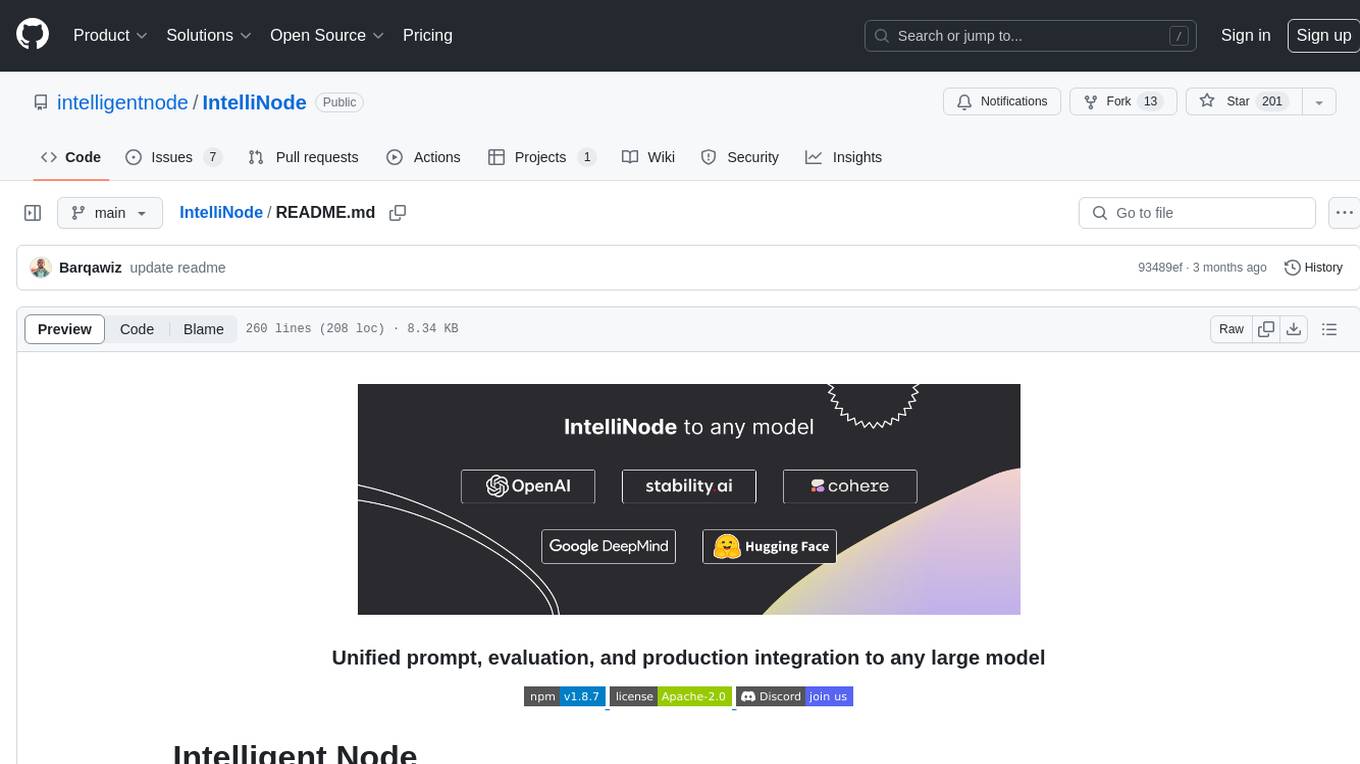
IntelliNode
IntelliNode is a javascript module that integrates cutting-edge AI models like ChatGPT, LLaMA, WaveNet, Gemini, and Stable diffusion into projects. It offers functions for generating text, speech, and images, as well as semantic search, multi-model evaluation, and chatbot capabilities. The module provides a wrapper layer for low-level model access, a controller layer for unified input handling, and a function layer for abstract functionality tailored to various use cases.
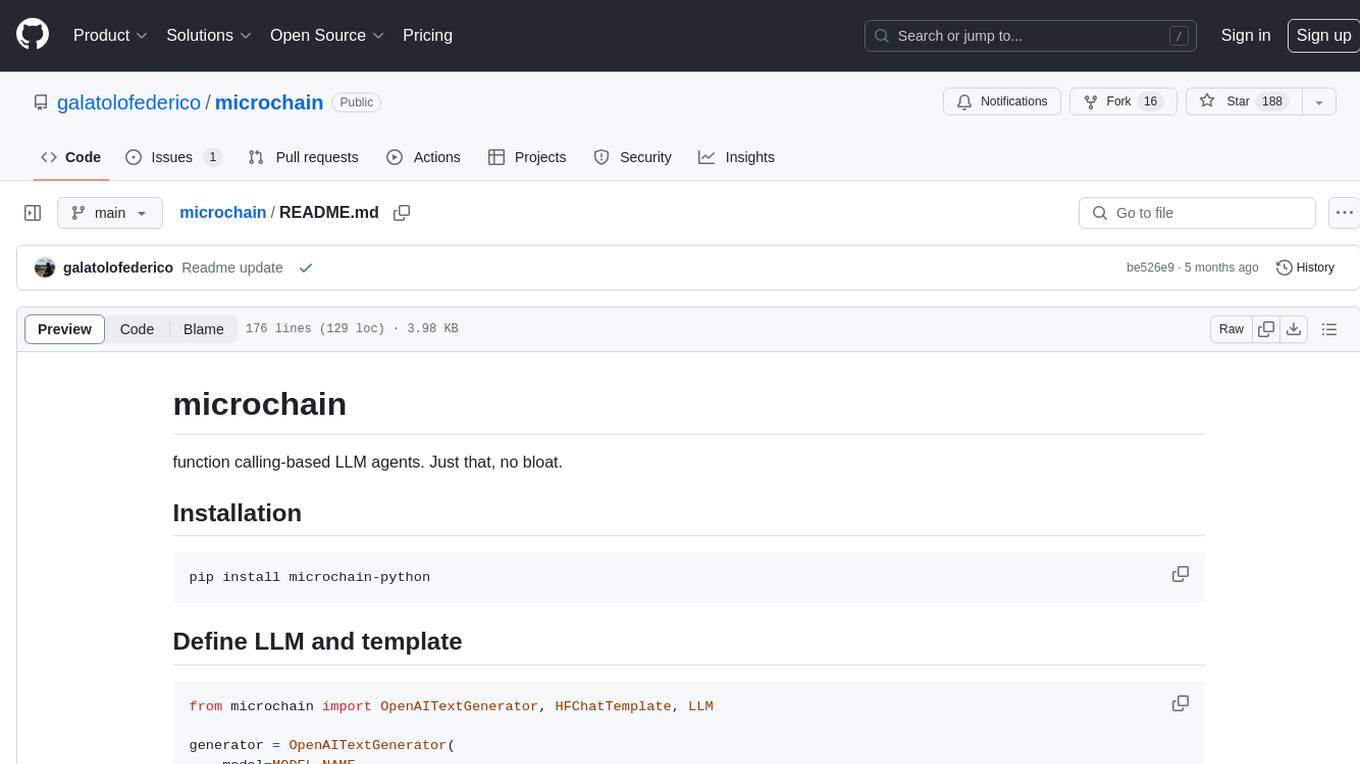
microchain
Microchain is a function calling-based LLM agents tool with no bloat. It allows users to define LLM and templates, use various functions like Sum and Product, and create LLM agents for specific tasks. The tool provides a simple and efficient way to interact with OpenAI models and create conversational agents for various applications.
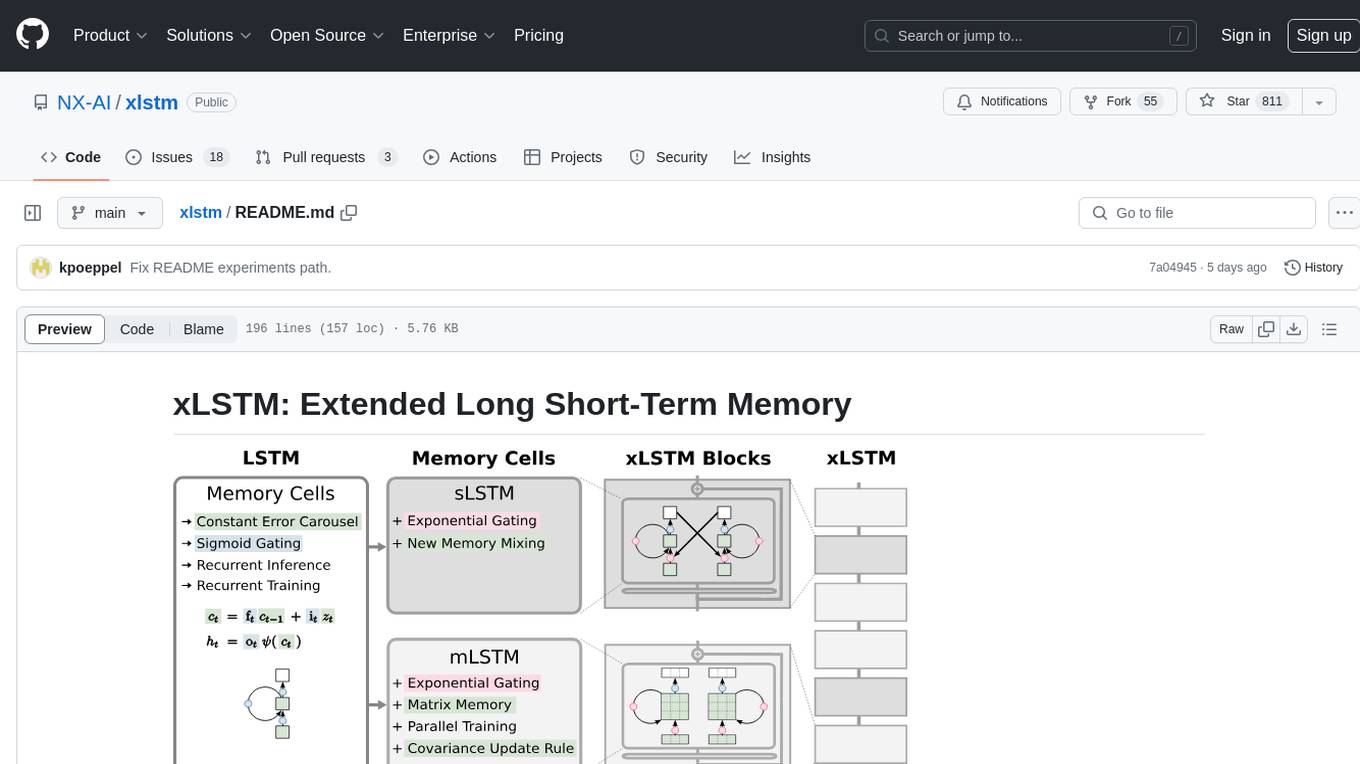
xlstm
xLSTM is a new Recurrent Neural Network architecture based on ideas of the original LSTM. Through Exponential Gating with appropriate normalization and stabilization techniques and a new Matrix Memory it overcomes the limitations of the original LSTM and shows promising performance on Language Modeling when compared to Transformers or State Space Models. The package is based on PyTorch and was tested for versions >=1.8. For the CUDA version of xLSTM, you need Compute Capability >= 8.0. The xLSTM tool provides two main components: xLSTMBlockStack for non-language applications or integrating in other architectures, and xLSTMLMModel for language modeling or other token-based applications.
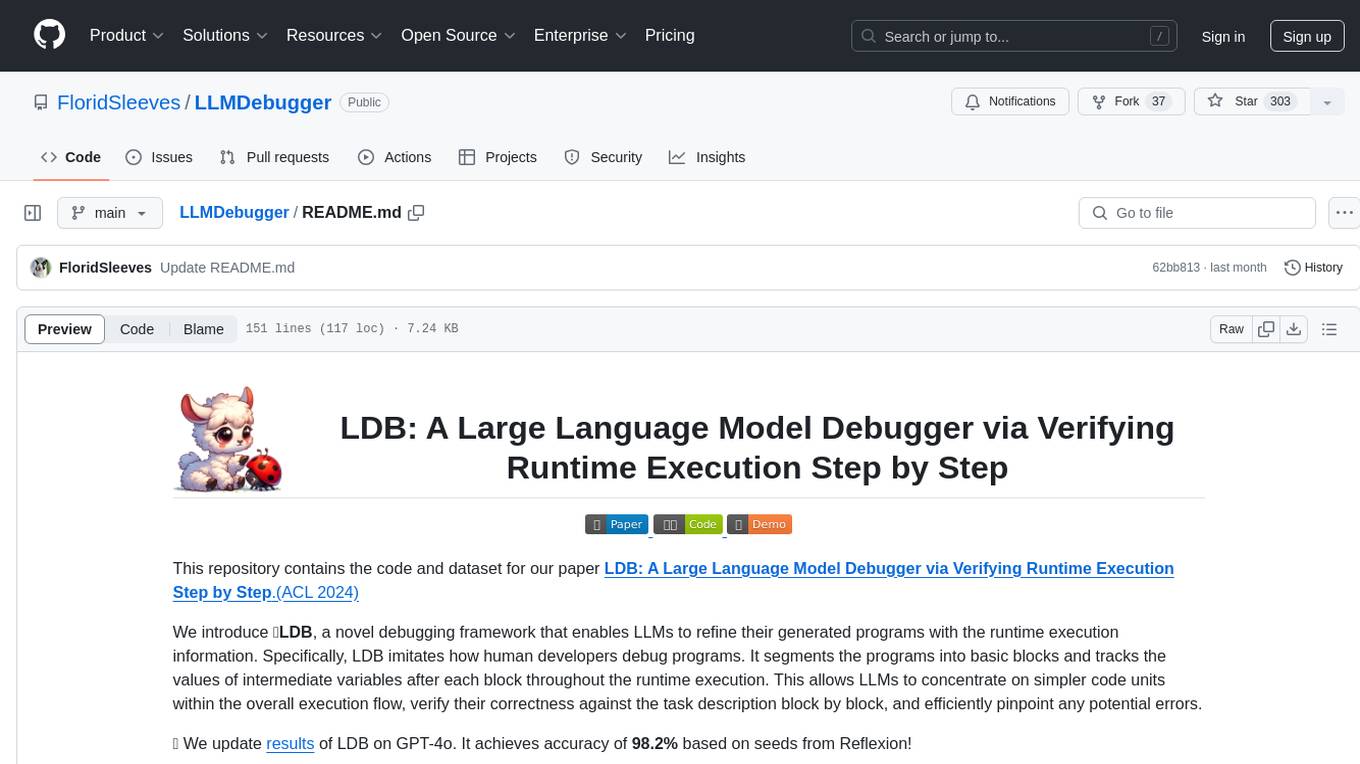
LLMDebugger
This repository contains the code and dataset for LDB, a novel debugging framework that enables Large Language Models (LLMs) to refine their generated programs by tracking the values of intermediate variables throughout the runtime execution. LDB segments programs into basic blocks, allowing LLMs to concentrate on simpler code units, verify correctness block by block, and pinpoint errors efficiently. The tool provides APIs for debugging and generating code with debugging messages, mimicking how human developers debug programs.
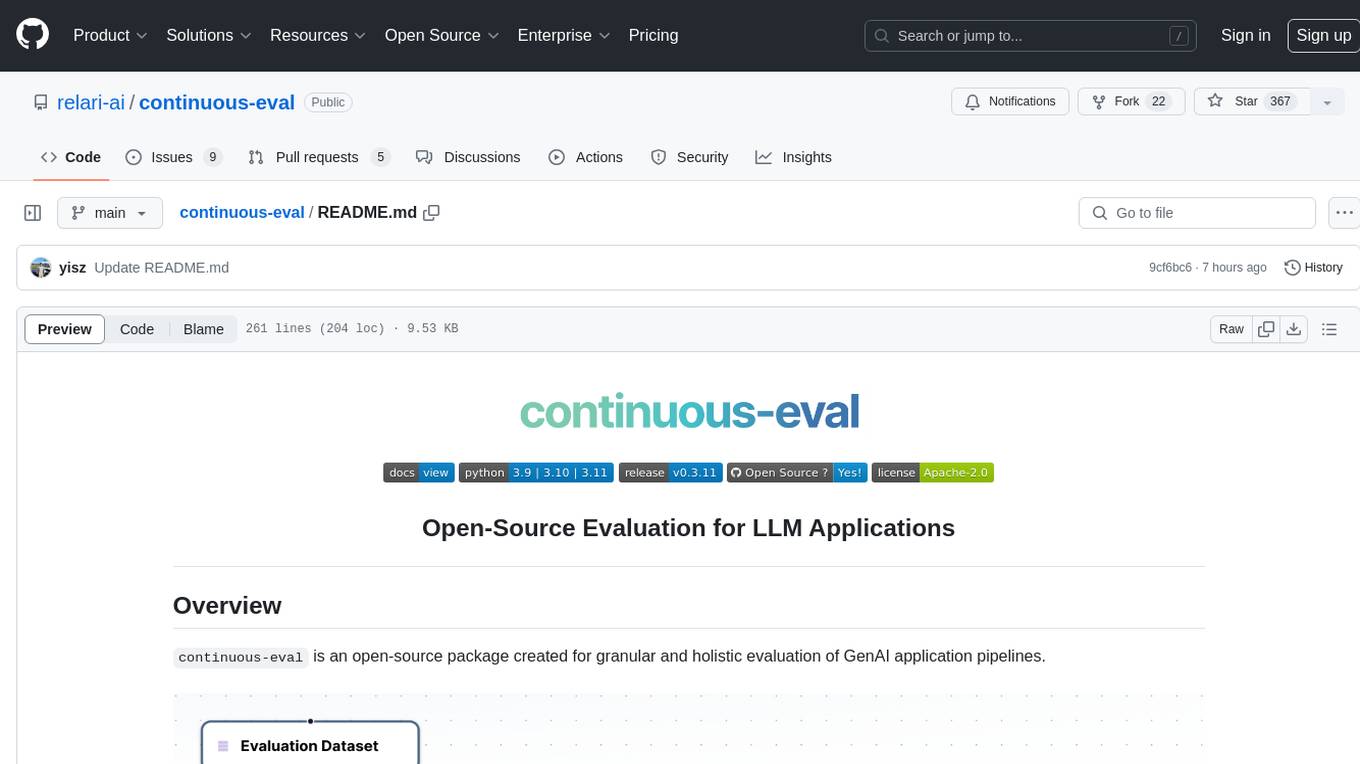
continuous-eval
Open-Source Evaluation for LLM Applications. `continuous-eval` is an open-source package created for granular and holistic evaluation of GenAI application pipelines. It offers modularized evaluation, a comprehensive metric library covering various LLM use cases, the ability to leverage user feedback in evaluation, and synthetic dataset generation for testing pipelines. Users can define their own metrics by extending the Metric class. The tool allows running evaluation on a pipeline defined with modules and corresponding metrics. Additionally, it provides synthetic data generation capabilities to create user interaction data for evaluation or training purposes.
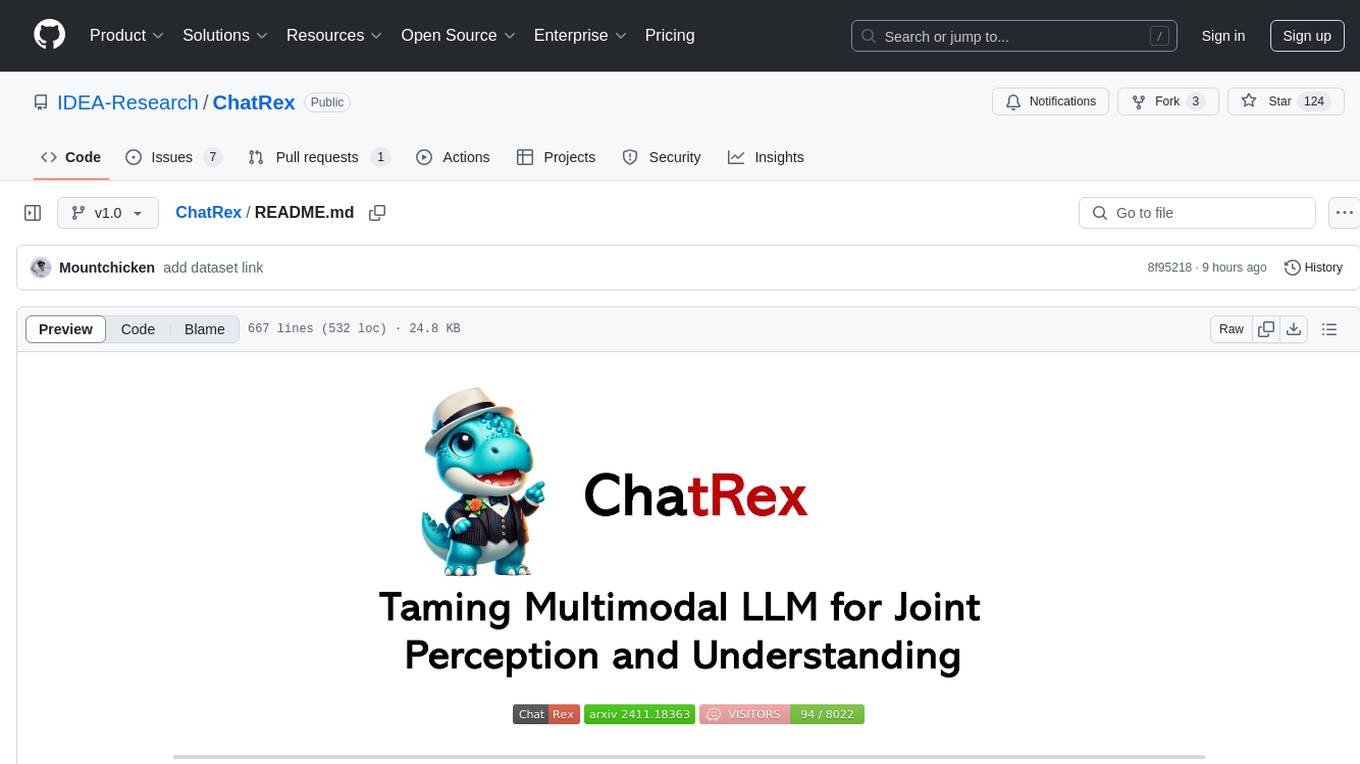
ChatRex
ChatRex is a Multimodal Large Language Model (MLLM) designed to seamlessly integrate fine-grained object perception and robust language understanding. By adopting a decoupled architecture with a retrieval-based approach for object detection and leveraging high-resolution visual inputs, ChatRex addresses key challenges in perception tasks. It is powered by the Rexverse-2M dataset with diverse image-region-text annotations. ChatRex can be applied to various scenarios requiring fine-grained perception, such as object detection, grounded conversation, grounded image captioning, and region understanding.
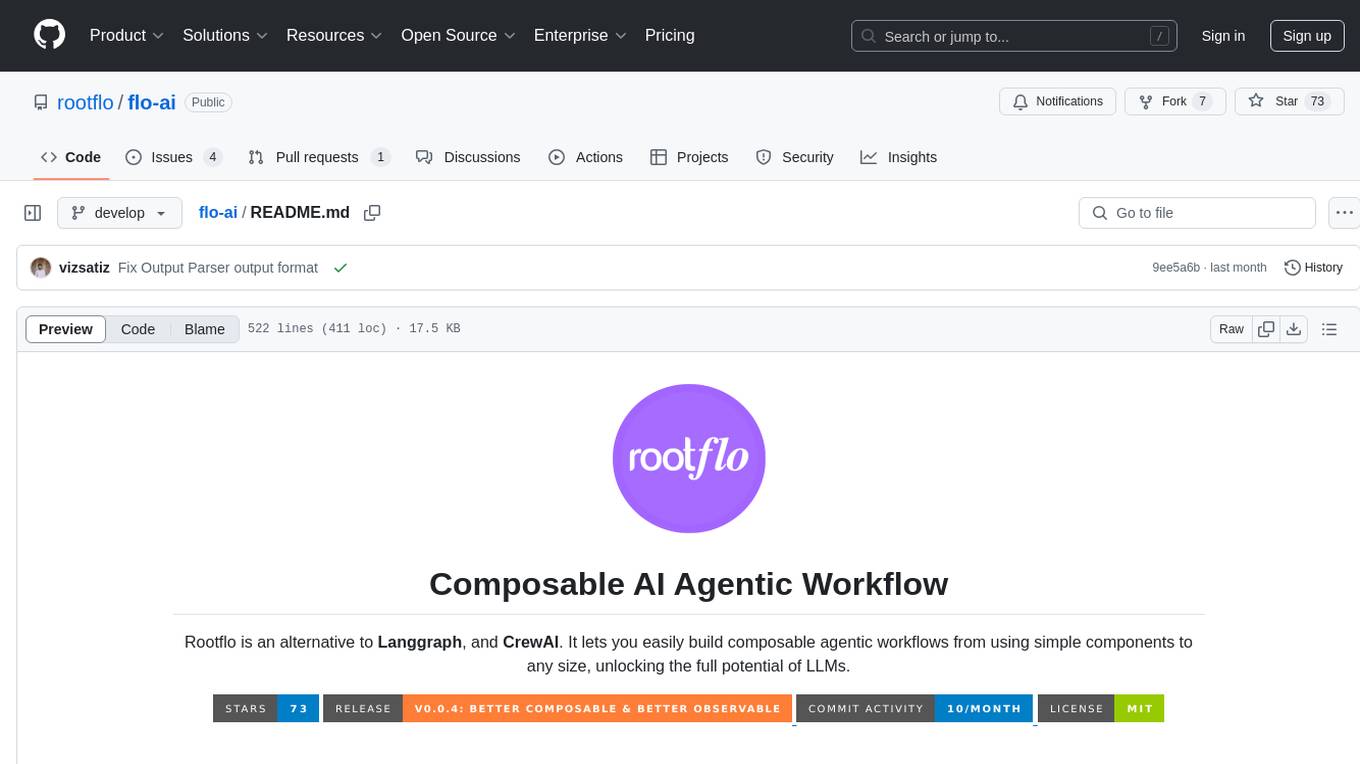
flo-ai
Flo AI is a Python framework that enables users to build production-ready AI agents and teams with minimal code. It allows users to compose complex AI architectures using pre-built components while maintaining the flexibility to create custom components. The framework supports composable, production-ready, YAML-first, and flexible AI systems. Users can easily create AI agents and teams, manage teams of AI agents working together, and utilize built-in support for Retrieval-Augmented Generation (RAG) and compatibility with Langchain tools. Flo AI also provides tools for output parsing and formatting, tool logging, data collection, and JSON output collection. It is MIT Licensed and offers detailed documentation, tutorials, and examples for AI engineers and teams to accelerate development, maintainability, scalability, and testability of AI systems.
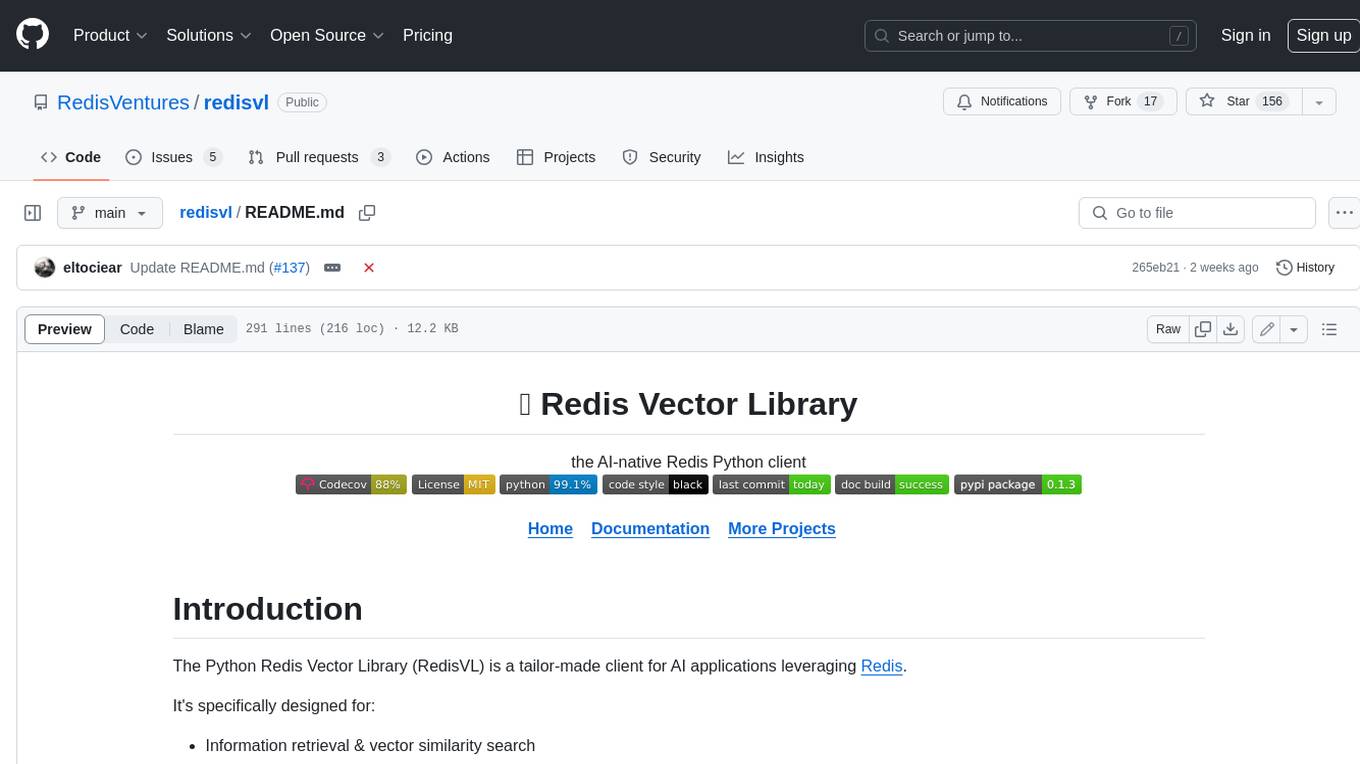
redisvl
Redis Vector Library (RedisVL) is a Python client library for building AI applications on top of Redis. It provides a high-level interface for managing vector indexes, performing vector search, and integrating with popular embedding models and providers. RedisVL is designed to make it easy for developers to build and deploy AI applications that leverage the speed, flexibility, and reliability of Redis.
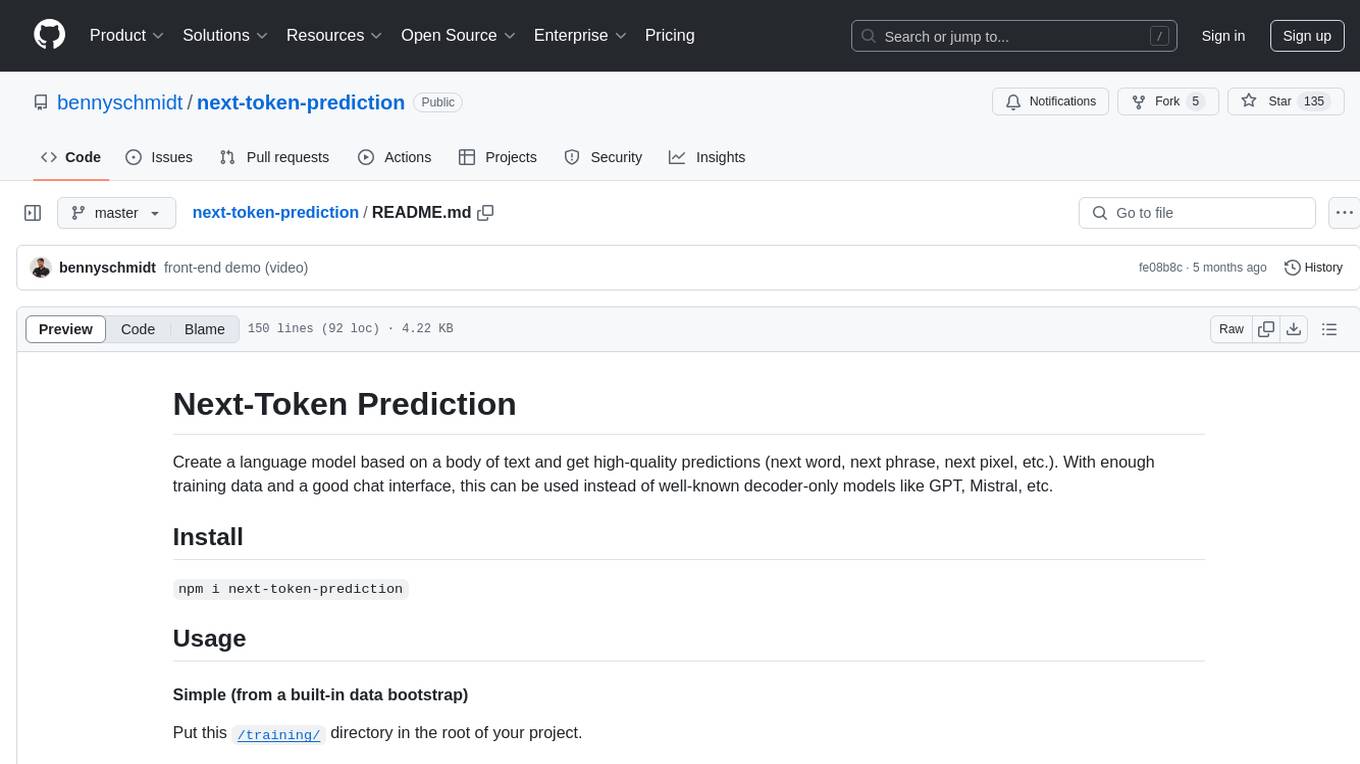
next-token-prediction
Next-Token Prediction is a language model tool that allows users to create high-quality predictions for the next word, phrase, or pixel based on a body of text. It can be used as an alternative to well-known decoder-only models like GPT and Mistral. The tool provides options for simple usage with built-in data bootstrap or advanced customization by providing training data or creating it from .txt files. It aims to simplify methodologies, provide autocomplete, autocorrect, spell checking, search/lookup functionalities, and create pixel and audio transformers for various prediction formats.
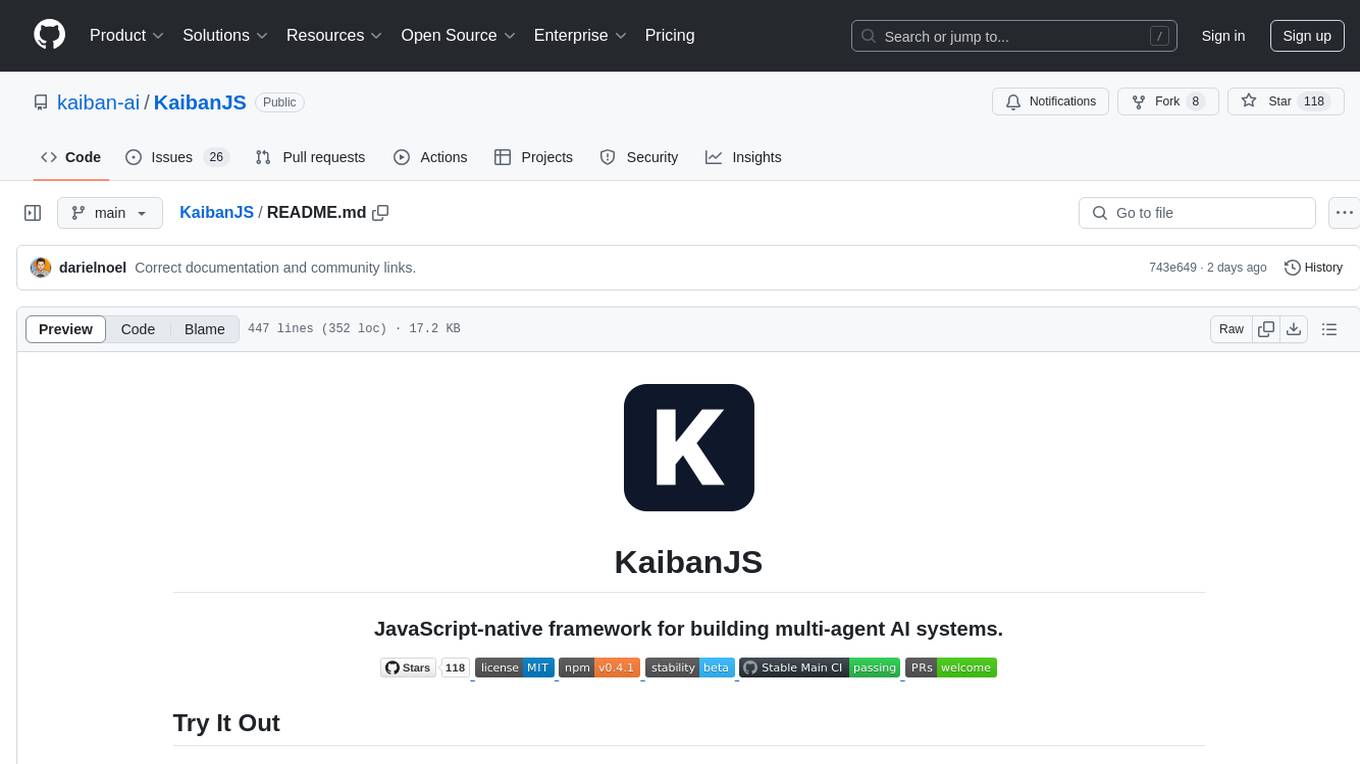
KaibanJS
KaibanJS is a JavaScript-native framework for building multi-agent AI systems. It enables users to create specialized AI agents with distinct roles and goals, manage tasks, and coordinate teams efficiently. The framework supports role-based agent design, tool integration, multiple LLMs support, robust state management, observability and monitoring features, and a real-time agentic Kanban board for visualizing AI workflows. KaibanJS aims to empower JavaScript developers with a user-friendly AI framework tailored for the JavaScript ecosystem, bridging the gap in the AI race for non-Python developers.
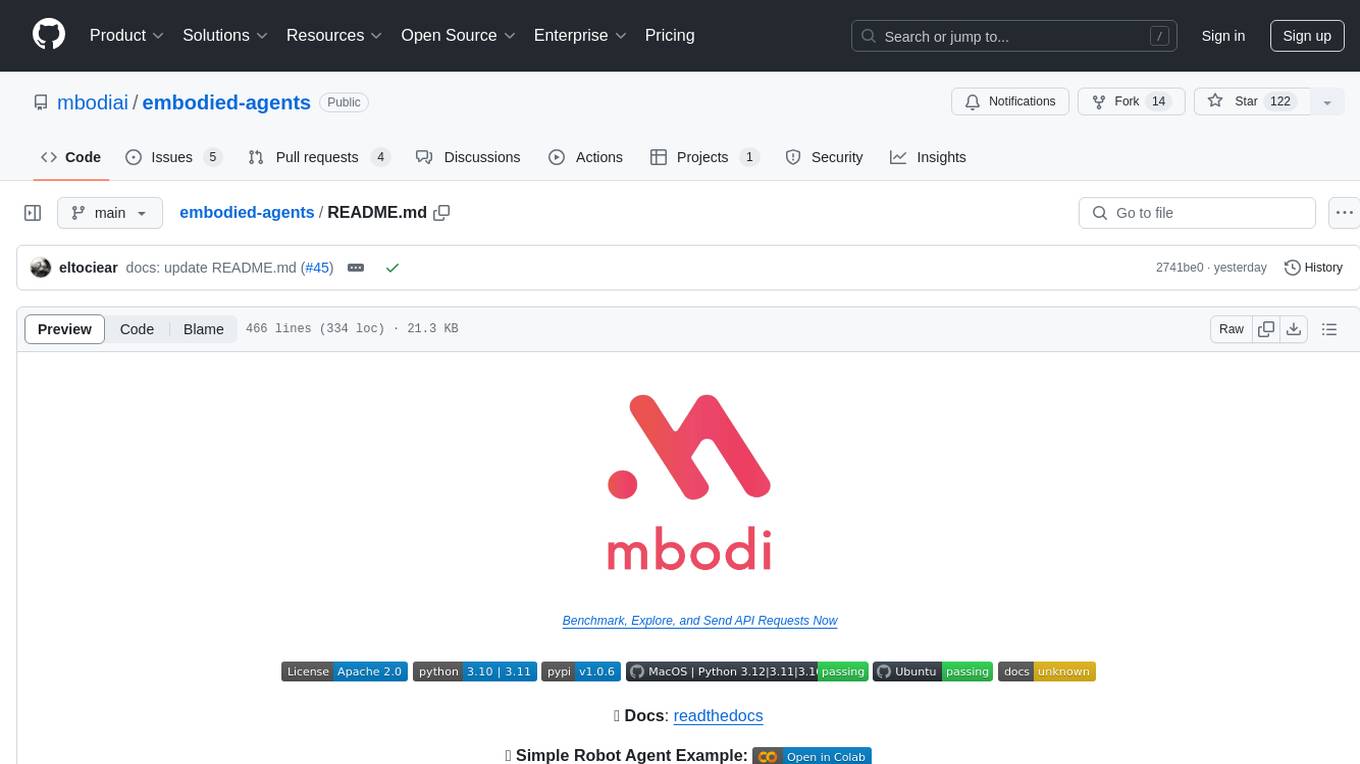
embodied-agents
Embodied Agents is a toolkit for integrating large multi-modal models into existing robot stacks with just a few lines of code. It provides consistency, reliability, scalability, and is configurable to any observation and action space. The toolkit is designed to reduce complexities involved in setting up inference endpoints, converting between different model formats, and collecting/storing datasets. It aims to facilitate data collection and sharing among roboticists by providing Python-first abstractions that are modular, extensible, and applicable to a wide range of tasks. The toolkit supports asynchronous and remote thread-safe agent execution for maximal responsiveness and scalability, and is compatible with various APIs like HuggingFace Spaces, Datasets, Gymnasium Spaces, Ollama, and OpenAI. It also offers automatic dataset recording and optional uploads to the HuggingFace hub.
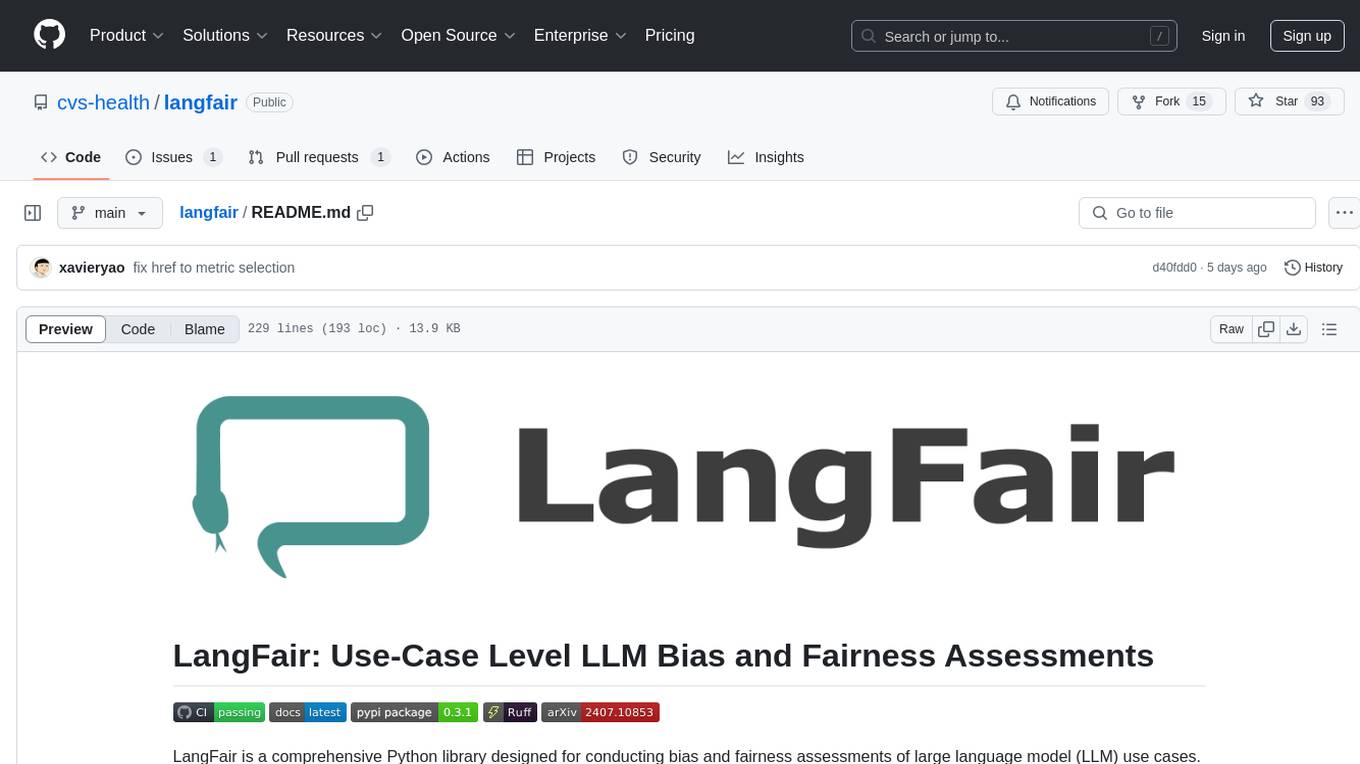
langfair
LangFair is a Python library for bias and fairness assessments of large language models (LLMs). It offers a comprehensive framework for choosing bias and fairness metrics, demo notebooks, and a technical playbook. Users can tailor evaluations to their use cases with a Bring Your Own Prompts approach. The focus is on output-based metrics practical for governance audits and real-world testing.
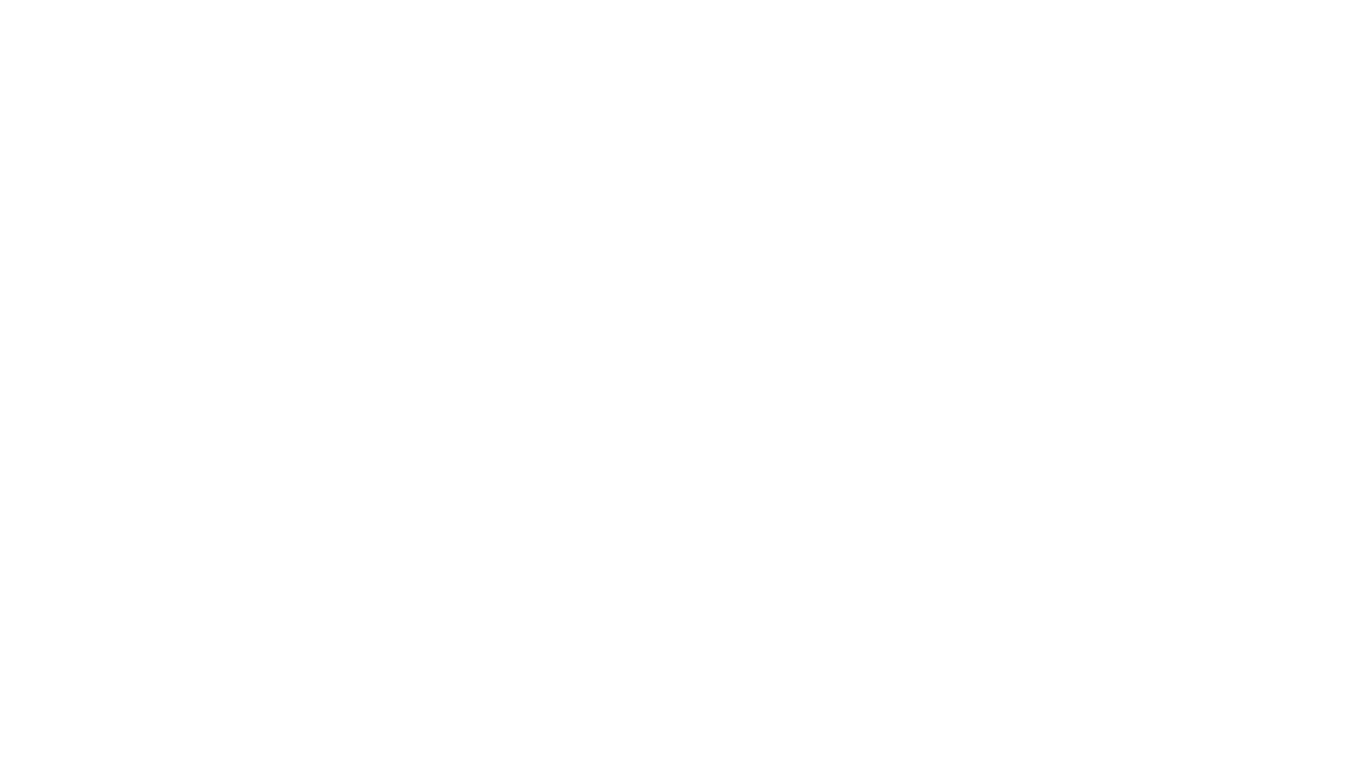
rl
TorchRL is an open-source Reinforcement Learning (RL) library for PyTorch. It provides pytorch and **python-first** , low and high level abstractions for RL that are intended to be **efficient** , **modular** , **documented** and properly **tested**. The code is aimed at supporting research in RL. Most of it is written in python in a highly modular way, such that researchers can easily swap components, transform them or write new ones with little effort.
For similar tasks
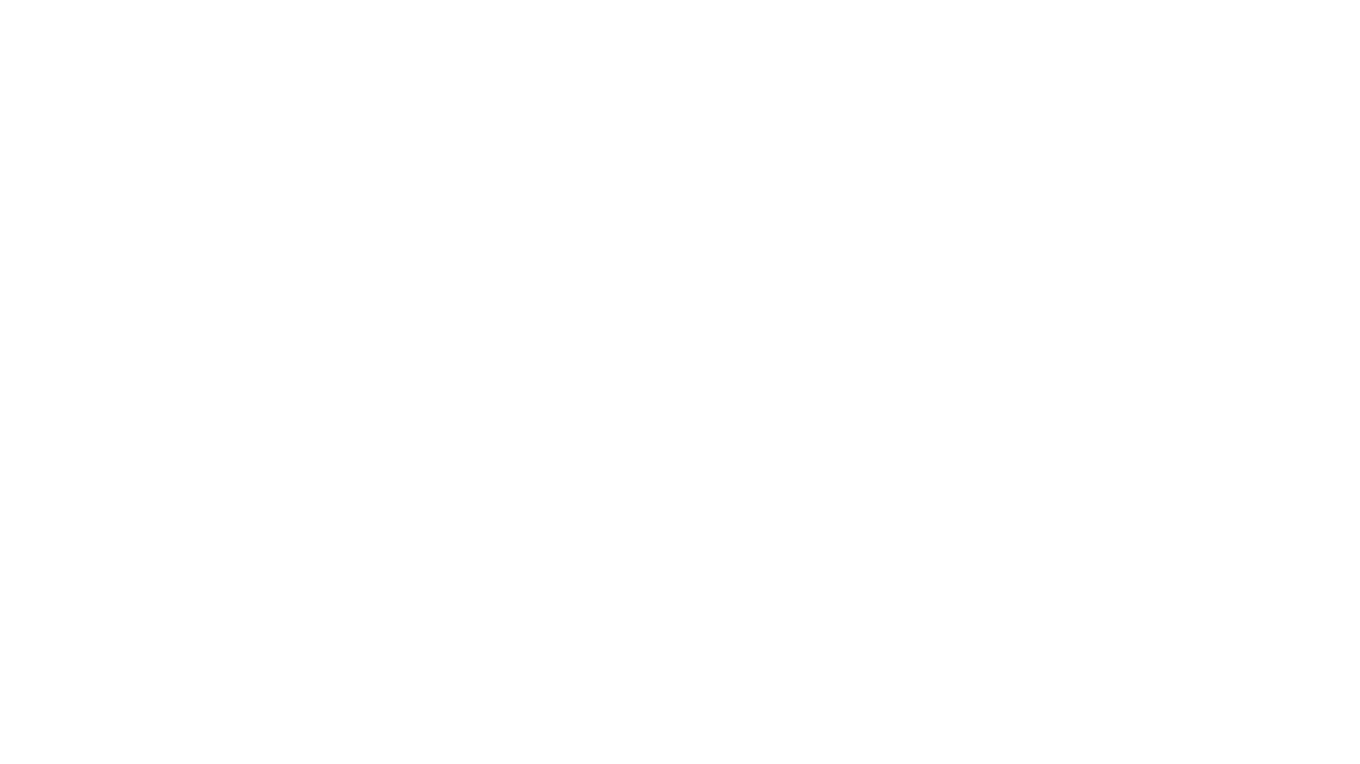
CEO-Agentic-AI-Framework
CEO-Agentic-AI-Framework is an ultra-lightweight Agentic AI framework based on the ReAct paradigm. It supports mainstream LLMs and is stronger than Swarm. The framework allows users to build their own agents, assign tasks, and interact with them through a set of predefined abilities. Users can customize agent personalities, grant and deprive abilities, and assign queries for specific tasks. CEO also supports multi-agent collaboration scenarios, where different agents with distinct capabilities can work together to achieve complex tasks. The framework provides a quick start guide, examples, and detailed documentation for seamless integration into research projects.
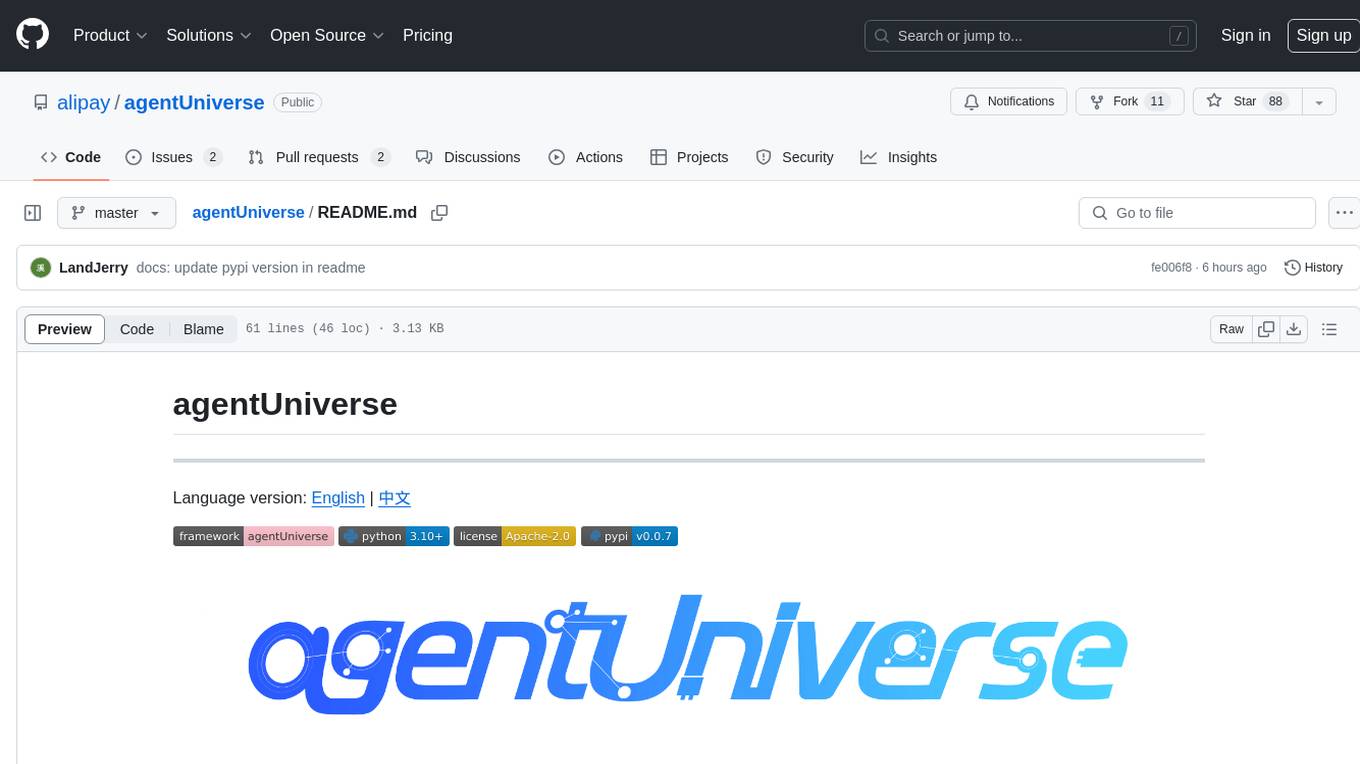
agentUniverse
agentUniverse is a framework for developing applications powered by multi-agent based on large language model. It provides essential components for building single agent and multi-agent collaboration mechanism for customizing collaboration patterns. Developers can easily construct multi-agent applications and share pattern practices from different fields. The framework includes pre-installed collaboration patterns like PEER and DOE for complex task breakdown and data-intensive tasks.
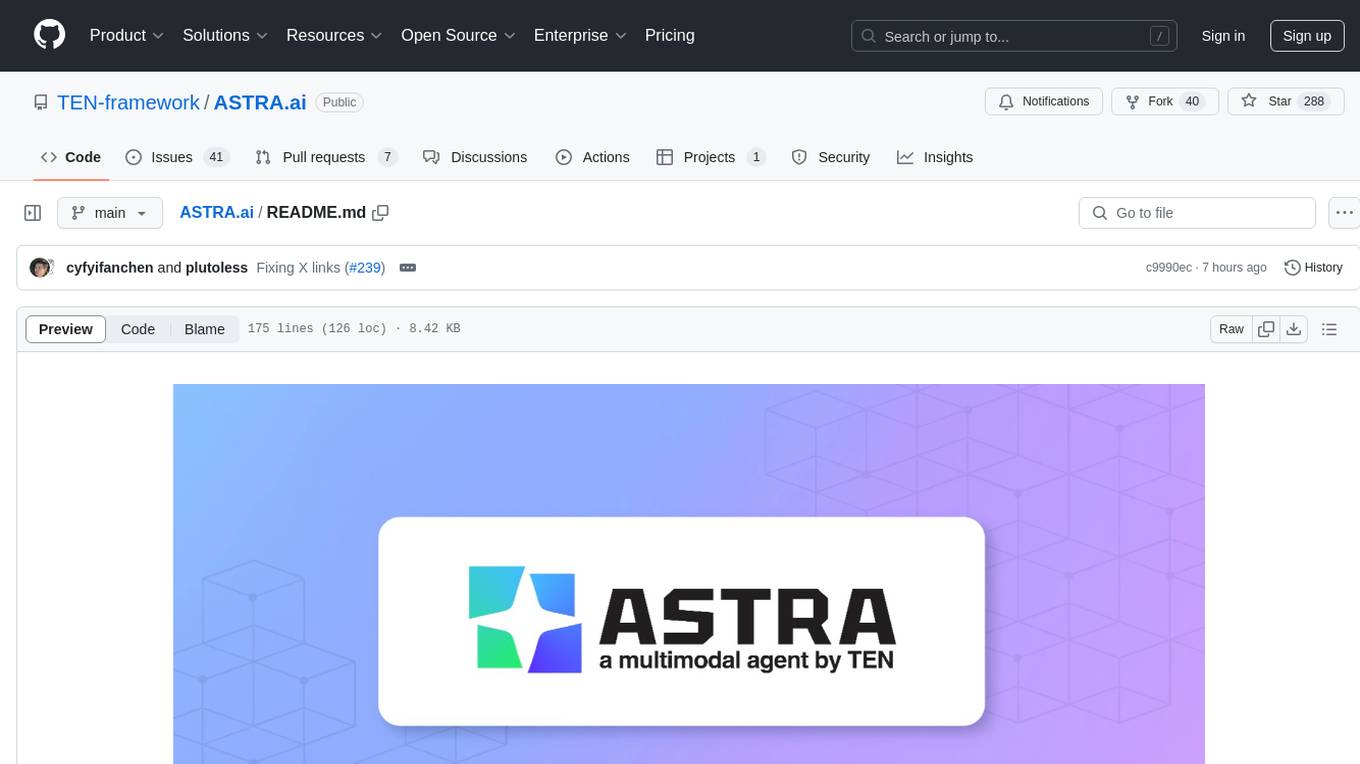
ASTRA.ai
Astra.ai is a multimodal agent powered by TEN, showcasing its capabilities in speech, vision, and reasoning through RAG from local documentation. It provides a platform for developing AI agents with features like RTC transportation, extension store, workflow builder, and local deployment. Users can build and test agents locally using Docker and Node.js, with prerequisites including Agora App ID, Azure's speech-to-text and text-to-speech API keys, and OpenAI API key. The platform offers advanced customization options through config files and API keys setup, enabling users to create and deploy their AI agents for various tasks.
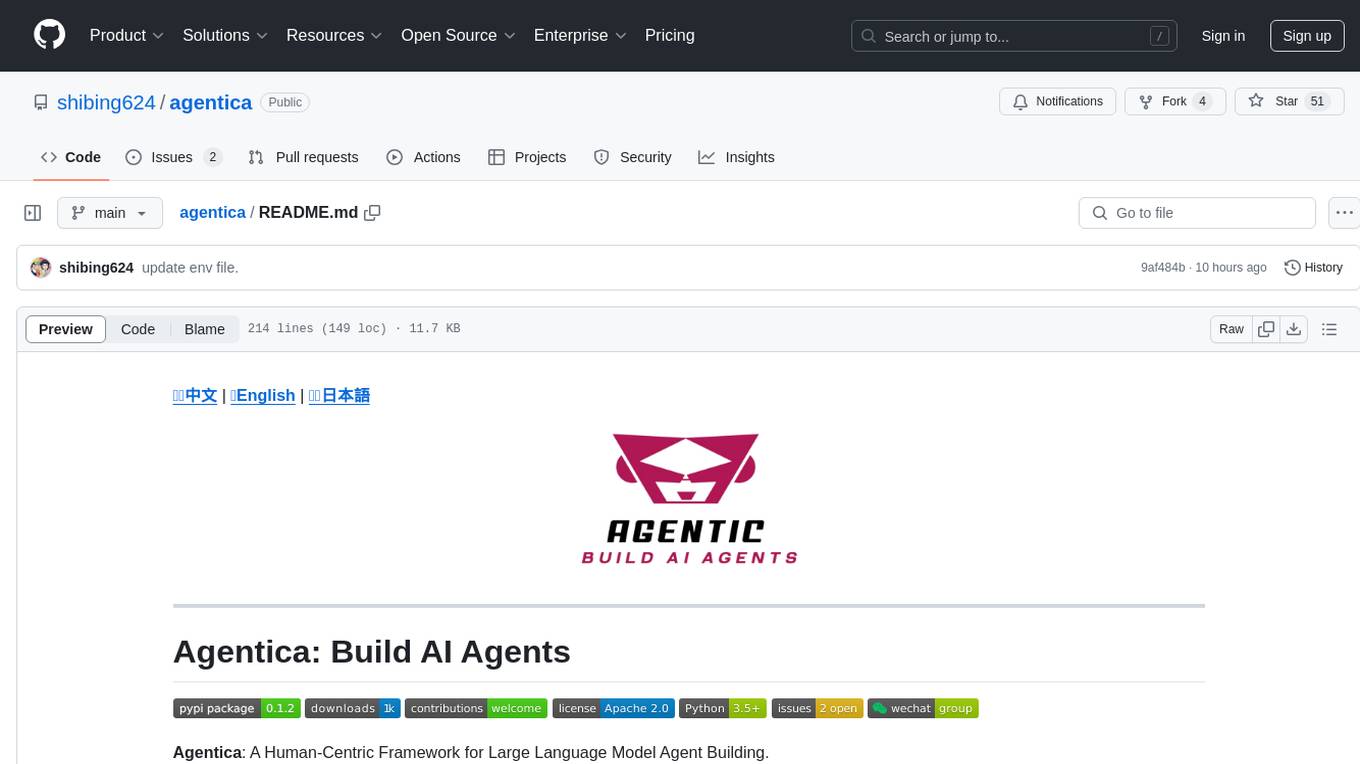
agentica
Agentica is a human-centric framework for building large language model agents. It provides functionalities for planning, memory management, tool usage, and supports features like reflection, planning and execution, RAG, multi-agent, multi-role, and workflow. The tool allows users to quickly code and orchestrate agents, customize prompts, and make API calls to various services. It supports API calls to OpenAI, Azure, Deepseek, Moonshot, Claude, Ollama, and Together. Agentica aims to simplify the process of building AI agents by providing a user-friendly interface and a range of functionalities for agent development.
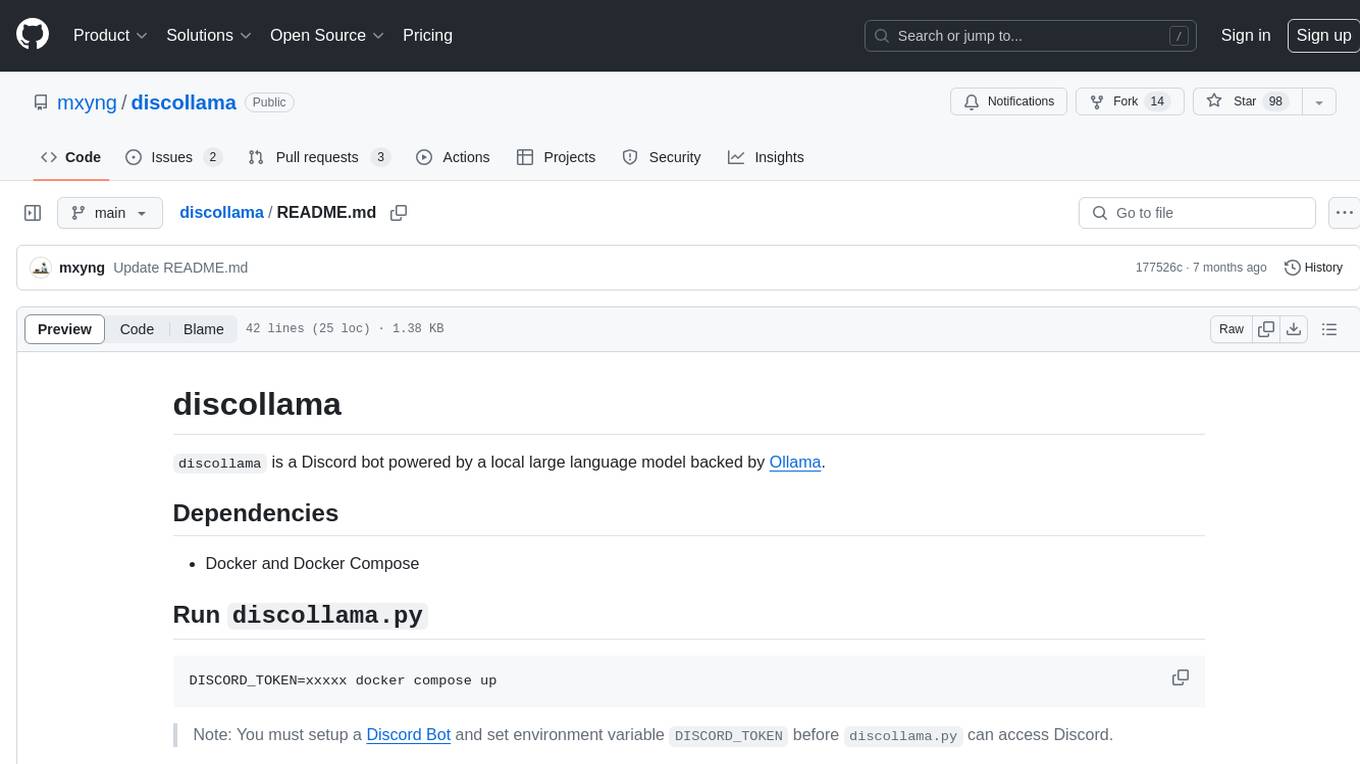
discollama
Discollama is a Discord bot powered by a local large language model backed by Ollama. It allows users to interact with the bot in Discord by mentioning it in a message to start a new conversation or in a reply to a previous response to continue an ongoing conversation. The bot requires Docker and Docker Compose to run, and users need to set up a Discord Bot and environment variable DISCORD_TOKEN before using discollama.py. Additionally, an Ollama server is needed, and users can customize the bot's personality by creating a custom model using Modelfile and running 'ollama create'.
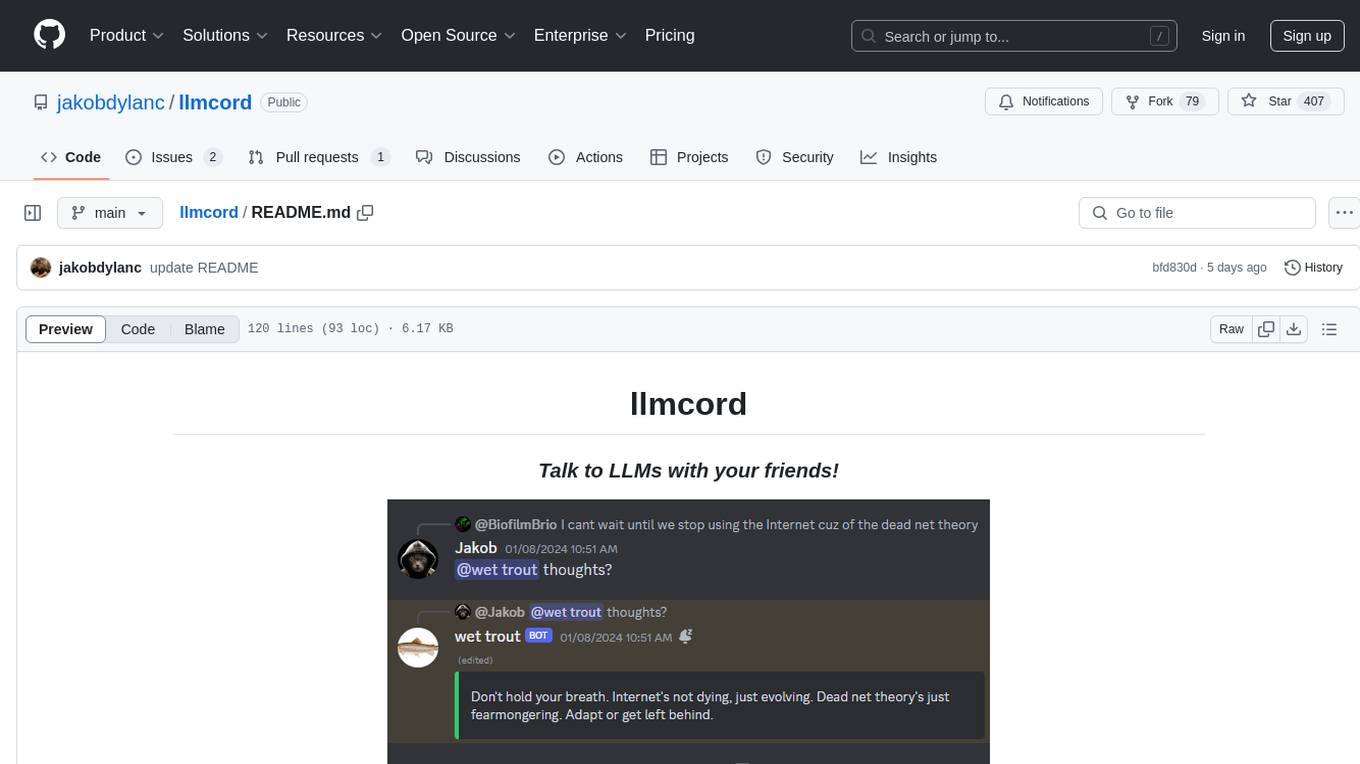
llmcord
llmcord is a Discord bot that transforms Discord into a collaborative LLM frontend, allowing users to interact with various LLM models. It features a reply-based chat system that enables branching conversations, supports remote and local LLM models, allows image and text file attachments, offers customizable personality settings, and provides streamed responses. The bot is fully asynchronous, efficient in managing message data, and offers hot reloading config. With just one Python file and around 200 lines of code, llmcord provides a seamless experience for engaging with LLMs on Discord.
For similar jobs
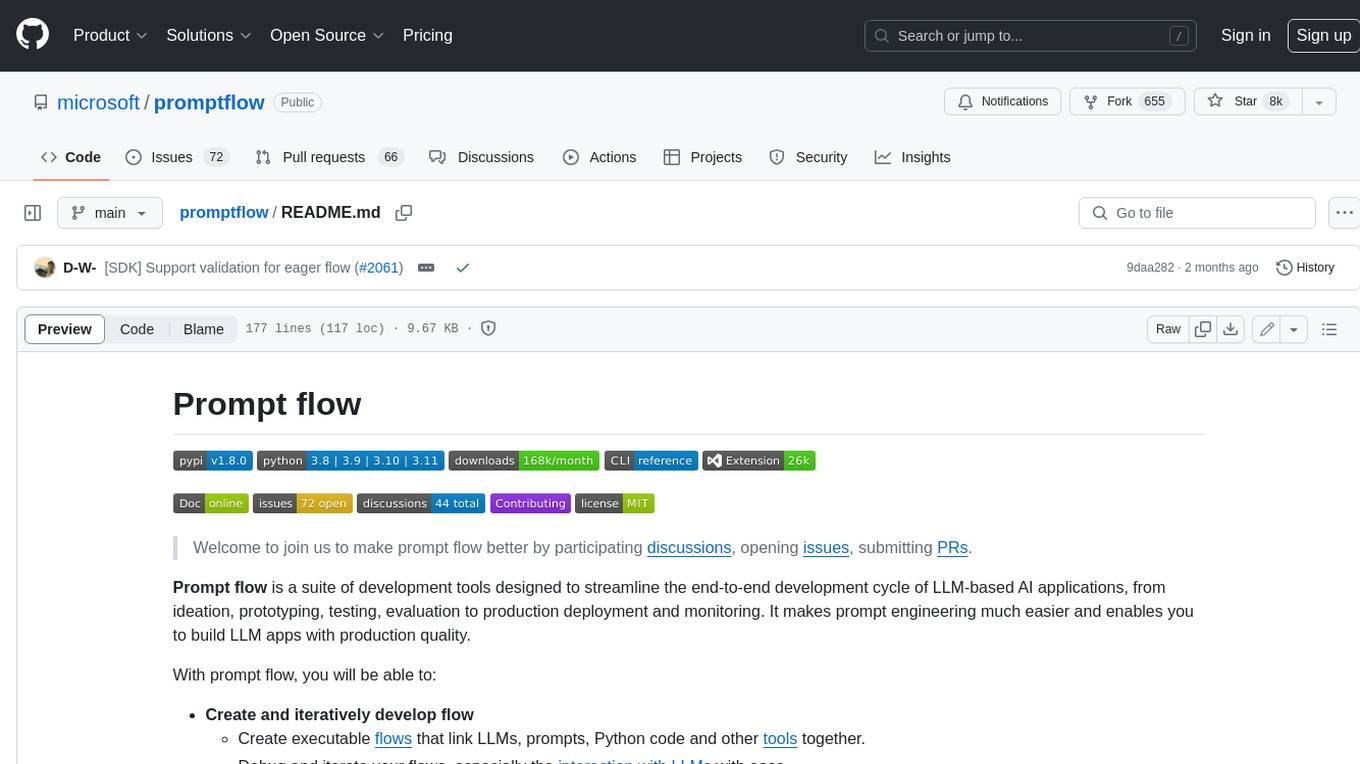
promptflow
**Prompt flow** is a suite of development tools designed to streamline the end-to-end development cycle of LLM-based AI applications, from ideation, prototyping, testing, evaluation to production deployment and monitoring. It makes prompt engineering much easier and enables you to build LLM apps with production quality.
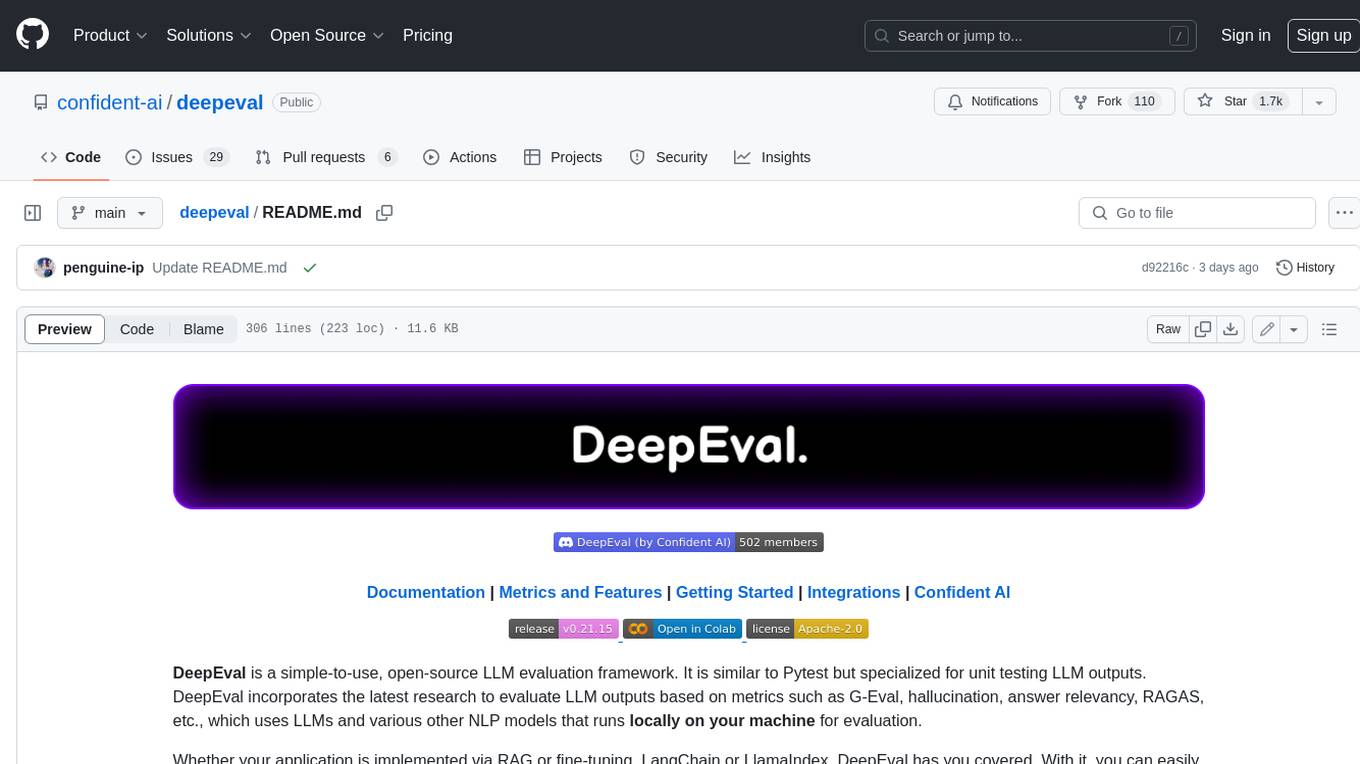
deepeval
DeepEval is a simple-to-use, open-source LLM evaluation framework specialized for unit testing LLM outputs. It incorporates various metrics such as G-Eval, hallucination, answer relevancy, RAGAS, etc., and runs locally on your machine for evaluation. It provides a wide range of ready-to-use evaluation metrics, allows for creating custom metrics, integrates with any CI/CD environment, and enables benchmarking LLMs on popular benchmarks. DeepEval is designed for evaluating RAG and fine-tuning applications, helping users optimize hyperparameters, prevent prompt drifting, and transition from OpenAI to hosting their own Llama2 with confidence.
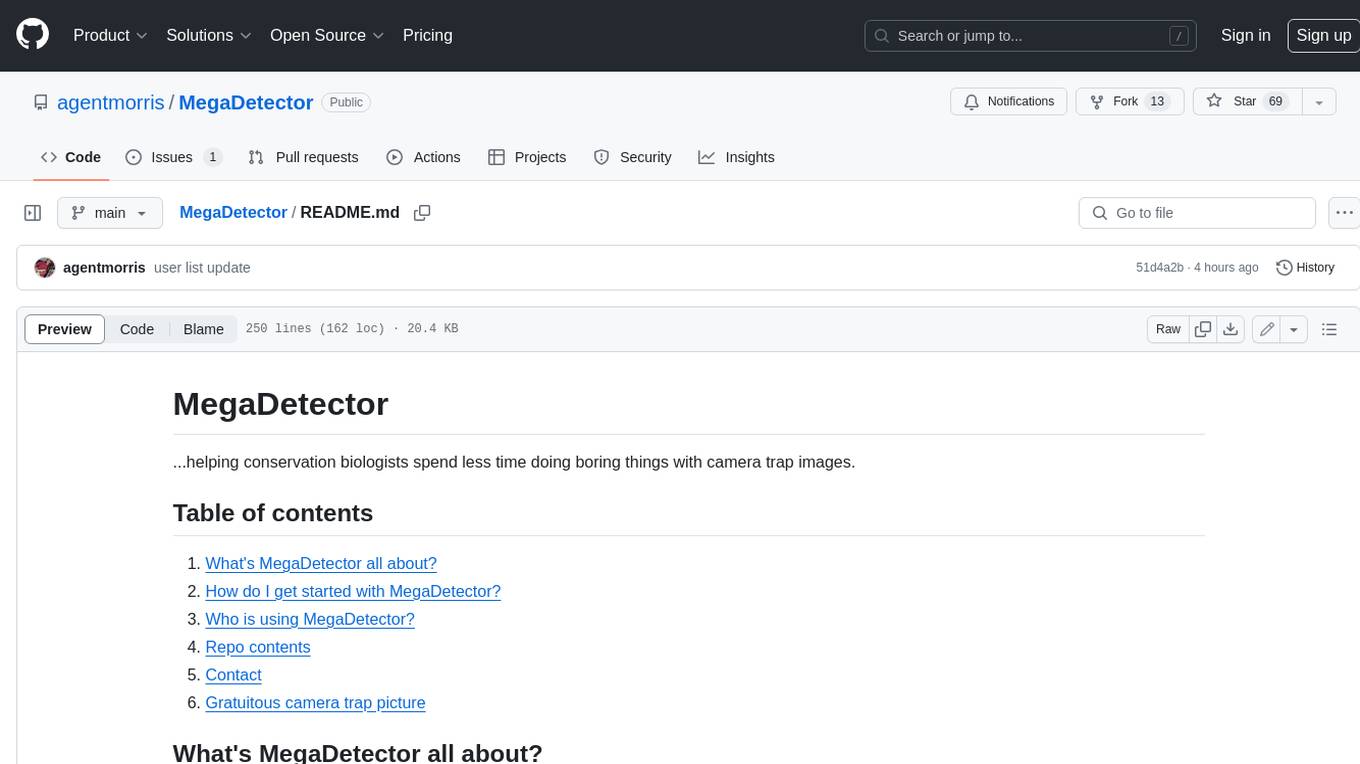
MegaDetector
MegaDetector is an AI model that identifies animals, people, and vehicles in camera trap images (which also makes it useful for eliminating blank images). This model is trained on several million images from a variety of ecosystems. MegaDetector is just one of many tools that aims to make conservation biologists more efficient with AI. If you want to learn about other ways to use AI to accelerate camera trap workflows, check out our of the field, affectionately titled "Everything I know about machine learning and camera traps".
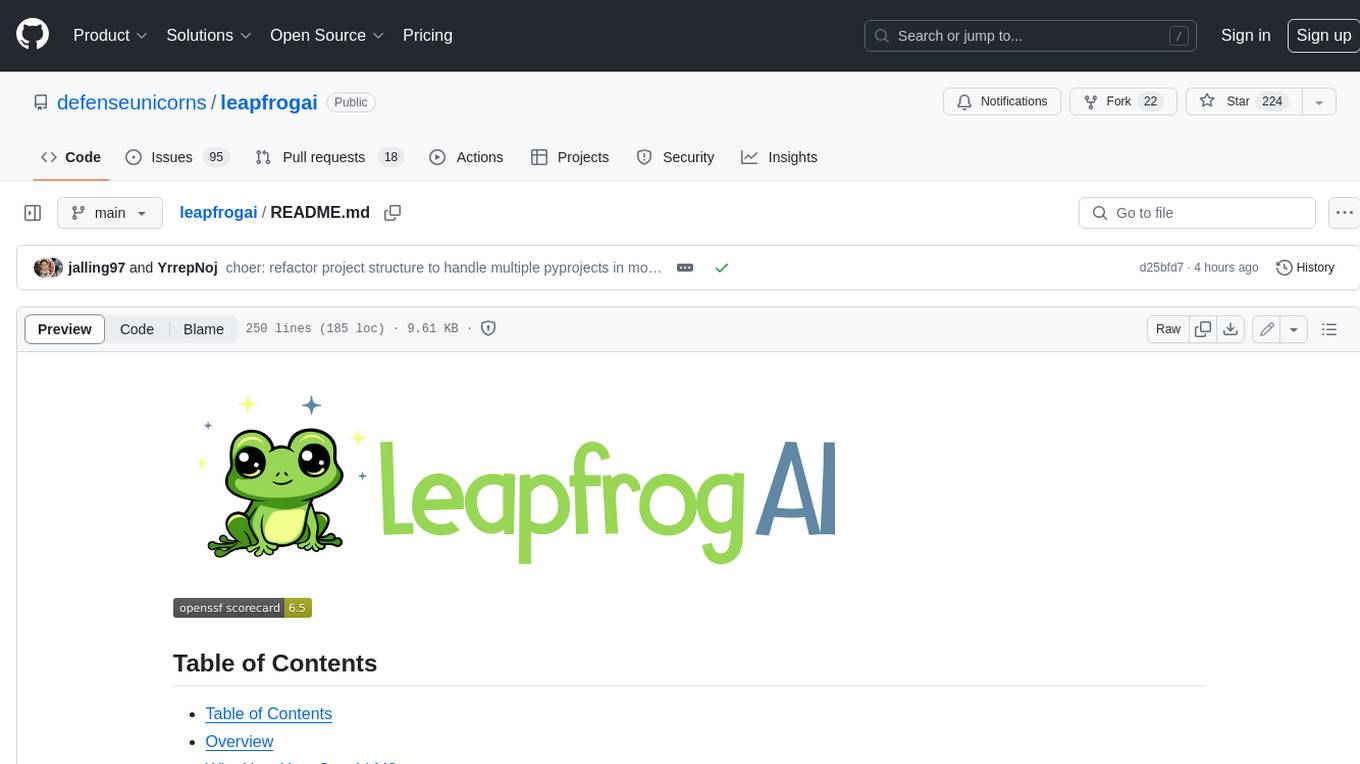
leapfrogai
LeapfrogAI is a self-hosted AI platform designed to be deployed in air-gapped resource-constrained environments. It brings sophisticated AI solutions to these environments by hosting all the necessary components of an AI stack, including vector databases, model backends, API, and UI. LeapfrogAI's API closely matches that of OpenAI, allowing tools built for OpenAI/ChatGPT to function seamlessly with a LeapfrogAI backend. It provides several backends for various use cases, including llama-cpp-python, whisper, text-embeddings, and vllm. LeapfrogAI leverages Chainguard's apko to harden base python images, ensuring the latest supported Python versions are used by the other components of the stack. The LeapfrogAI SDK provides a standard set of protobuffs and python utilities for implementing backends and gRPC. LeapfrogAI offers UI options for common use-cases like chat, summarization, and transcription. It can be deployed and run locally via UDS and Kubernetes, built out using Zarf packages. LeapfrogAI is supported by a community of users and contributors, including Defense Unicorns, Beast Code, Chainguard, Exovera, Hypergiant, Pulze, SOSi, United States Navy, United States Air Force, and United States Space Force.
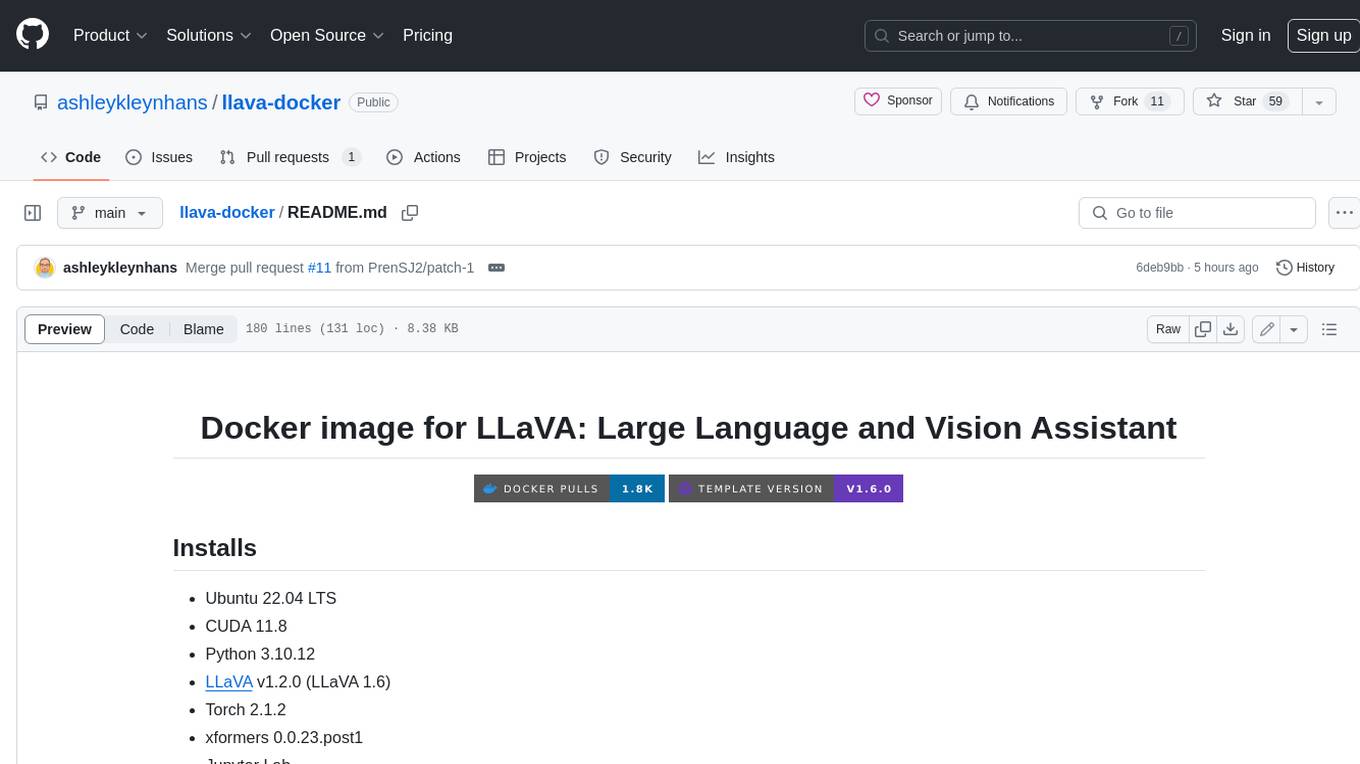
llava-docker
This Docker image for LLaVA (Large Language and Vision Assistant) provides a convenient way to run LLaVA locally or on RunPod. LLaVA is a powerful AI tool that combines natural language processing and computer vision capabilities. With this Docker image, you can easily access LLaVA's functionalities for various tasks, including image captioning, visual question answering, text summarization, and more. The image comes pre-installed with LLaVA v1.2.0, Torch 2.1.2, xformers 0.0.23.post1, and other necessary dependencies. You can customize the model used by setting the MODEL environment variable. The image also includes a Jupyter Lab environment for interactive development and exploration. Overall, this Docker image offers a comprehensive and user-friendly platform for leveraging LLaVA's capabilities.
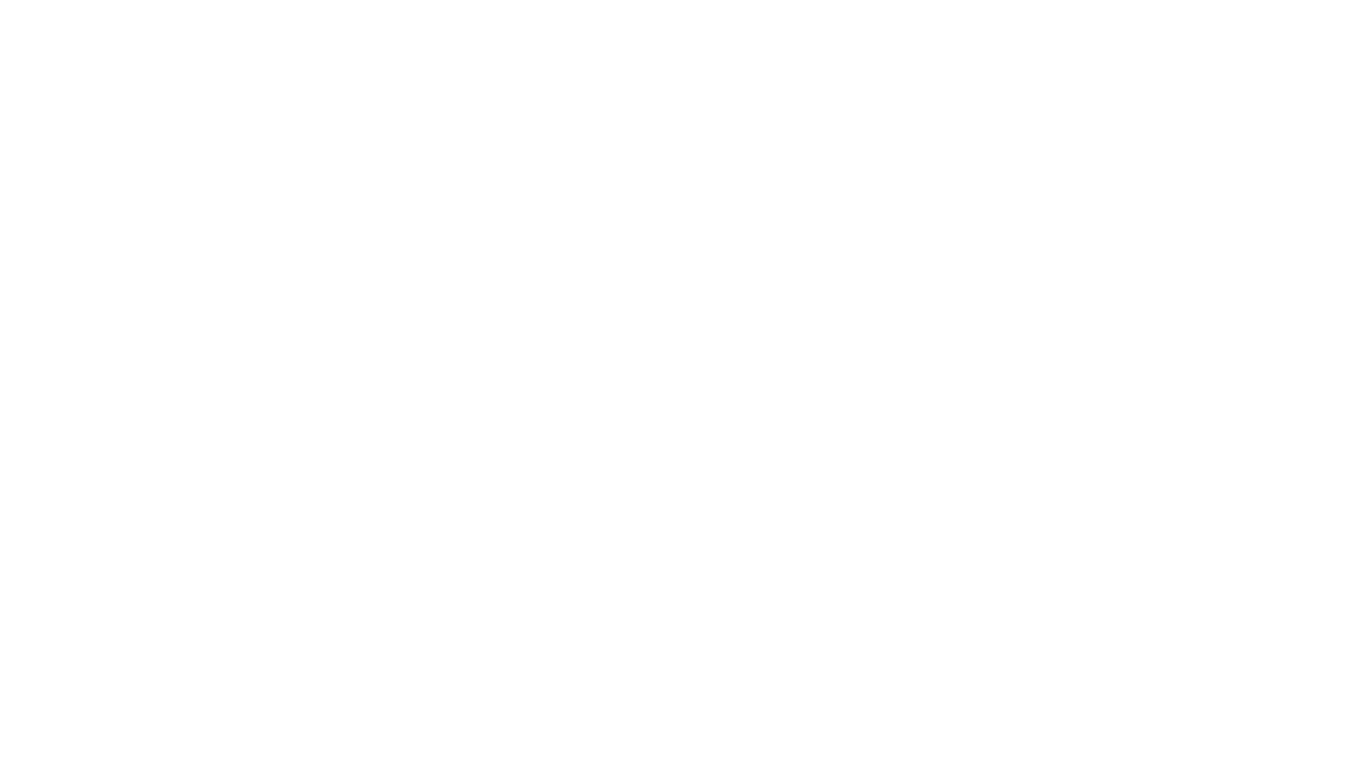
carrot
The 'carrot' repository on GitHub provides a list of free and user-friendly ChatGPT mirror sites for easy access. The repository includes sponsored sites offering various GPT models and services. Users can find and share sites, report errors, and access stable and recommended sites for ChatGPT usage. The repository also includes a detailed list of ChatGPT sites, their features, and accessibility options, making it a valuable resource for ChatGPT users seeking free and unlimited GPT services.
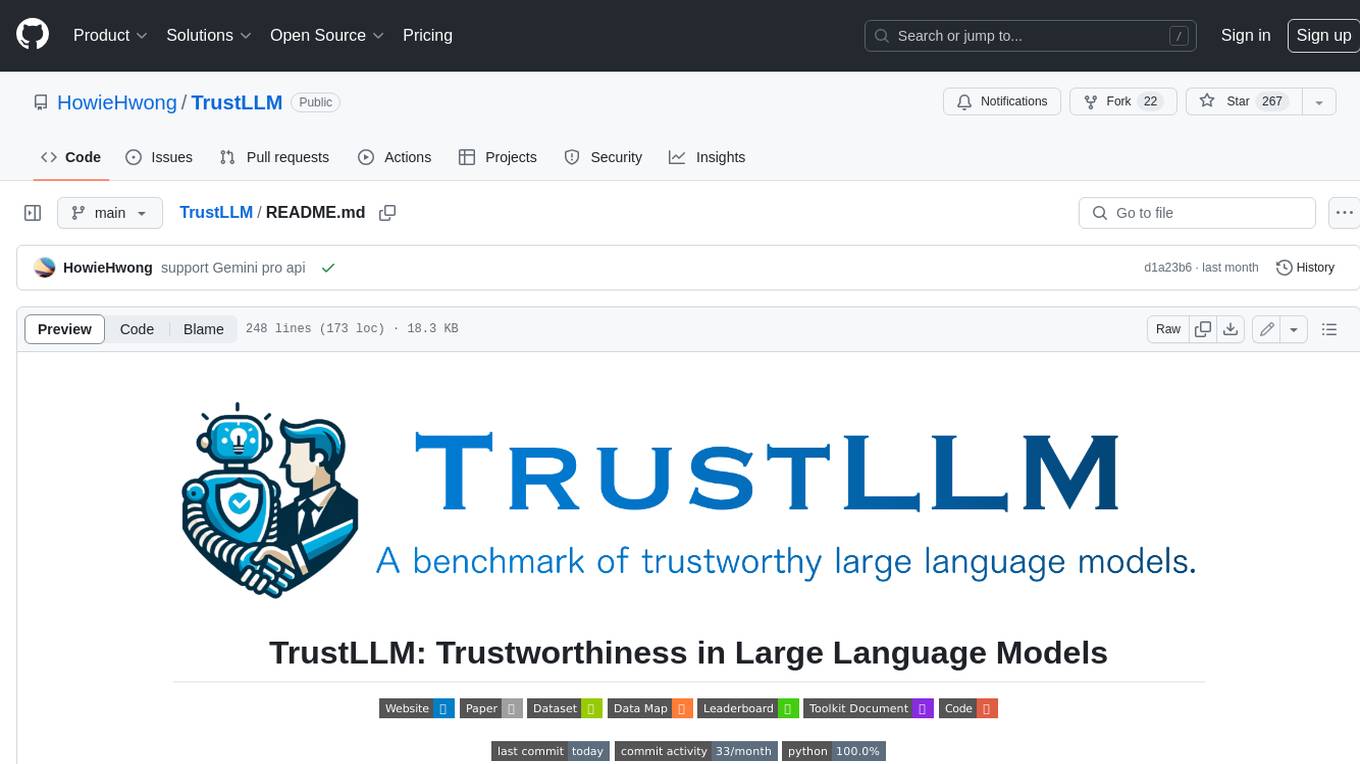
TrustLLM
TrustLLM is a comprehensive study of trustworthiness in LLMs, including principles for different dimensions of trustworthiness, established benchmark, evaluation, and analysis of trustworthiness for mainstream LLMs, and discussion of open challenges and future directions. Specifically, we first propose a set of principles for trustworthy LLMs that span eight different dimensions. Based on these principles, we further establish a benchmark across six dimensions including truthfulness, safety, fairness, robustness, privacy, and machine ethics. We then present a study evaluating 16 mainstream LLMs in TrustLLM, consisting of over 30 datasets. The document explains how to use the trustllm python package to help you assess the performance of your LLM in trustworthiness more quickly. For more details about TrustLLM, please refer to project website.
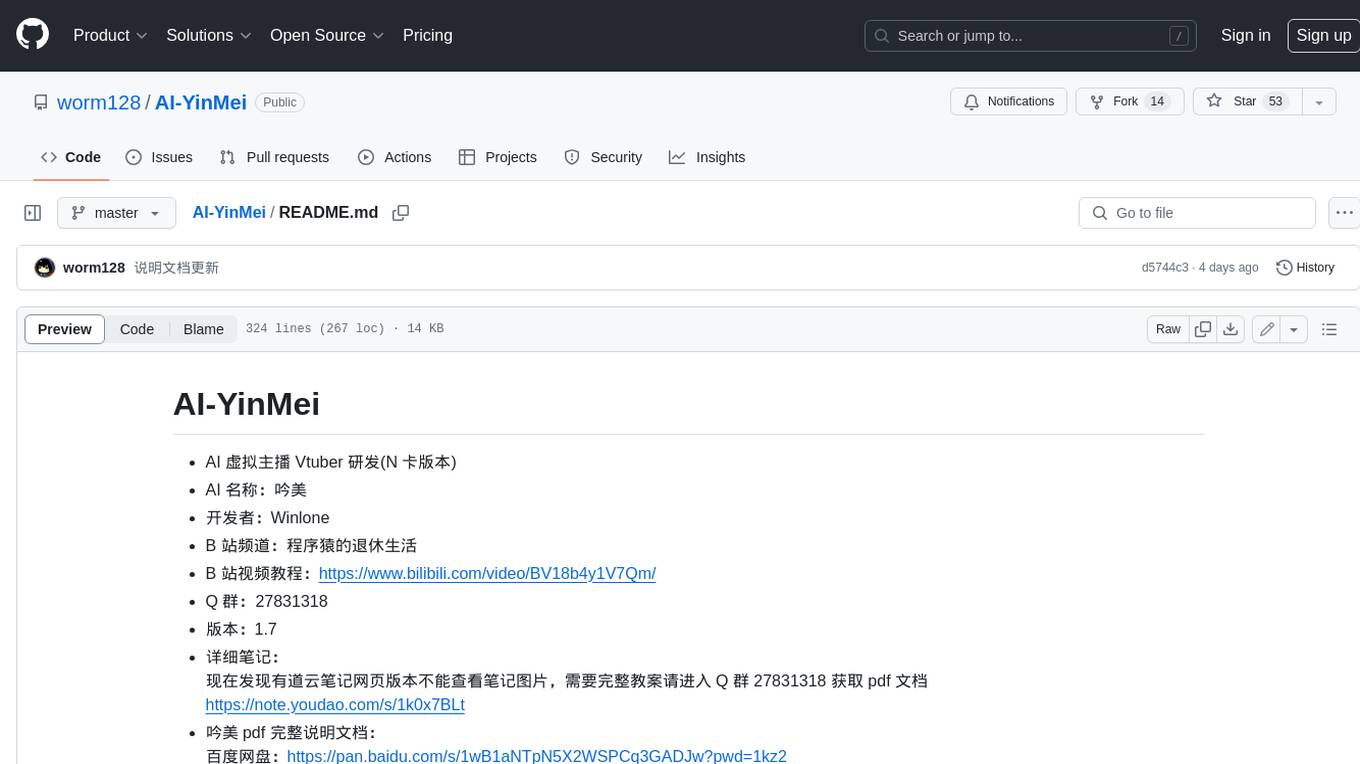
AI-YinMei
AI-YinMei is an AI virtual anchor Vtuber development tool (N card version). It supports fastgpt knowledge base chat dialogue, a complete set of solutions for LLM large language models: [fastgpt] + [one-api] + [Xinference], supports docking bilibili live broadcast barrage reply and entering live broadcast welcome speech, supports Microsoft edge-tts speech synthesis, supports Bert-VITS2 speech synthesis, supports GPT-SoVITS speech synthesis, supports expression control Vtuber Studio, supports painting stable-diffusion-webui output OBS live broadcast room, supports painting picture pornography public-NSFW-y-distinguish, supports search and image search service duckduckgo (requires magic Internet access), supports image search service Baidu image search (no magic Internet access), supports AI reply chat box [html plug-in], supports AI singing Auto-Convert-Music, supports playlist [html plug-in], supports dancing function, supports expression video playback, supports head touching action, supports gift smashing action, supports singing automatic start dancing function, chat and singing automatic cycle swing action, supports multi scene switching, background music switching, day and night automatic switching scene, supports open singing and painting, let AI automatically judge the content.