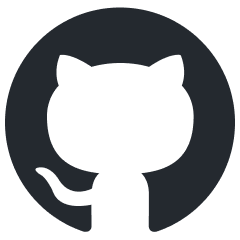
Cherry_LLM
[NAACL'24] Self-data filtering of LLM instruction-tuning data using a novel perplexity-based difficulty score, without using any other models
Stars: 271
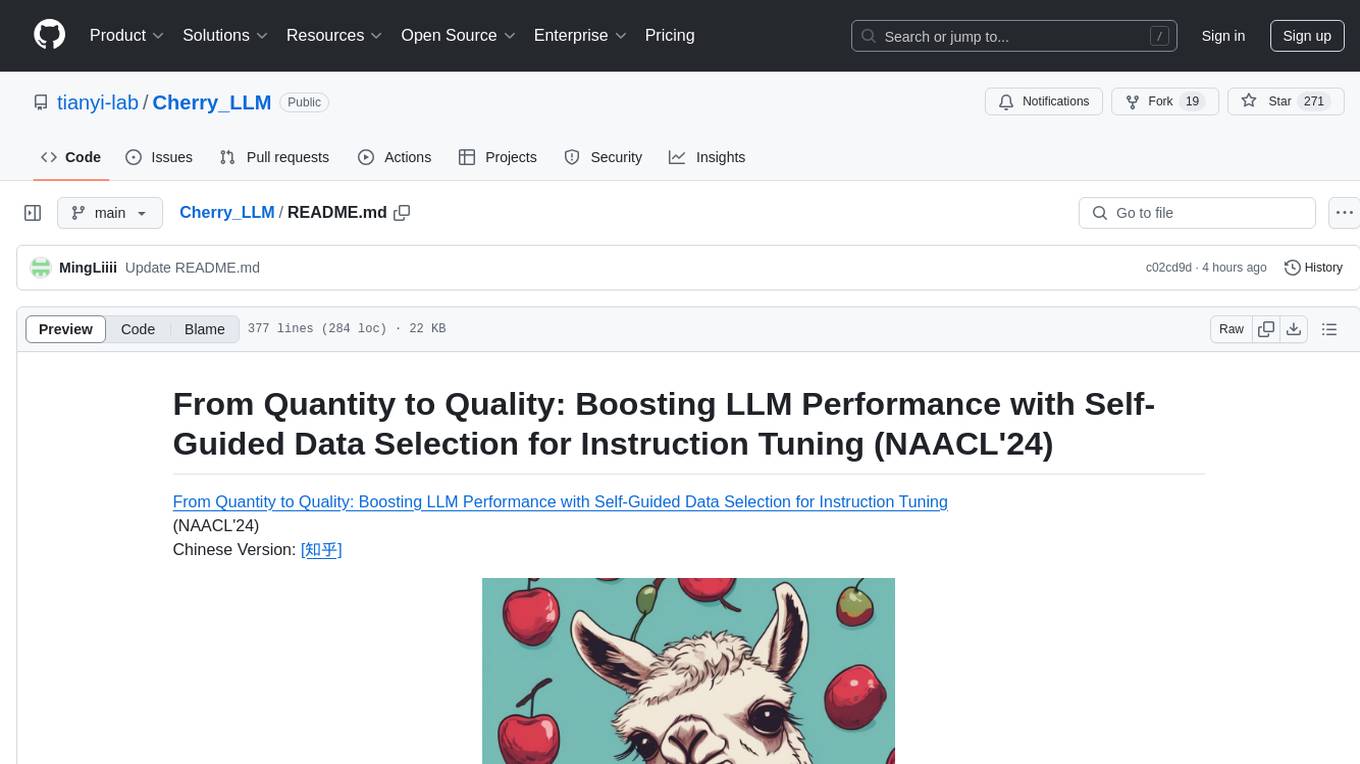
Cherry Data Selection project introduces a self-guided methodology for LLMs to autonomously discern and select cherry samples from open-source datasets, minimizing manual curation and cost for instruction tuning. The project focuses on selecting impactful training samples ('cherry data') to enhance LLM instruction tuning by estimating instruction-following difficulty. The method involves phases like 'Learning from Brief Experience', 'Evaluating Based on Experience', and 'Retraining from Self-Guided Experience' to improve LLM performance.
README:
From Quantity to Quality: Boosting LLM Performance with Self-Guided Data Selection for Instruction Tuning (NAACL'24)
From Quantity to Quality: Boosting LLM Performance with Self-Guided Data Selection for Instruction Tuning
(NAACL'24)
Chinese Version: [知乎]
This is the repo for the Cherry Data Selection project, which introduces a self-guided methodology for LLMs to autonomously discern and select cherry samples from vast open-source datasets, effectively minimizing manual curation and potential cost for instruction tuning an LLM.
The repo contains:
- The cherry data used for fine-tuning the model, cherry_data_v1 represents the cherry data obtained based on the llama-1 model.
- The model checkpoints that were trained using our cherry data.
- The code for selecting cherry data from the existing instruction-tuning dataset.
(Feel free to email Ming (Homepage, Email) for any questions or feedback.)
- [2024/03] Our paper has been accepted to the NAACL 2024 main conference!
- [2024/02] We released the Superfiltering, which reveals the strong consistency between small and large LLMs in perceiving and evaluating the difficulty of instruction tuning data and utilizes a small LM, e.g., GPT-2 (124M), to effectively and efficiently select data for instruction tuning.
- [2023/12] An updated code for calculating the statistics for IFD scores, please check Reflection-Tuning Code for Selection.
- [2023/12] The statistics necessary for calculating IFD scores on Alpaca and WizardLM on llama2-7b and llama2-13b were released, please check: Alpaca llama2 7b, Alpaca llama2 13b, WizardLM70k llama2 7b, WizardLM70k llama2 13b.
- [2023/11] We added some results on llama2-7b and llama2-13b, further showing the generalizability of our method.
- [2023/09] We partially reconstructed the repo structure and added some results on llama2.
- [2023/09] We released codes for evaluating the performance between two LLMs by using GPT4 or chatGPT.
- [2023/09] We released codes for this project.
- Overview
- Highlights
- Install
- Run Code
- Data and Model Weights V1
- Data and Model Weights V2
- Evaluation
- Performance Comparison
- Prompt
- Hyperparameters
- ToDo
- Citation
- Our Related Works
Our study puts forth a method for autonomously sifting through expansive open-source datasets to discover the most impactful training samples. We coin these samples as "cherry data", designating those data fragments that hold the potential to exponentially enhance LLM instruction tuning. At the heart of our research is the hypothesis that during their preliminary training stages with carefully chosen instruction data, LLMs can develop an intrinsic capability to discern instructions. This foundational understanding equips them with the discernment to assess the quality of broader datasets thus making it possible to estimate the instruction-following difficulty in a self-guided manner.
Initially, the model is familiarized with a fraction of the target dataset during the "Learning from Brief Experience" phase. This preliminary knowledge paves the way for the subsequent "Evaluating Based on Experience" phase, where we meticulously evaluate the model's response generation. To estimate the difficulty of a given example, we propose a novel metric called Instruction-Following Difficulty (IFD) score in which both models' capability to generate a response to a given instruction and the models' capability to generate a response directly are measured and compared. By calculating Instruction-Following Difficulty (IFD) scores, we quantify the challenge each sample presents to the model. Harnessing these insights, the "Retraining from Self-Guided Experience" phase utilizes cherry data with standout IFD scores to hone the model, culminating in our superior cherry models. The net result is a model that aligns more adeptly with instructions, ensuring enhanced performance.
- The selection of cherry data in this project is entirely self-guided and does not need ANY extra outside models, ranging from BERT to chatGPT.
- We use approximately 5% or 10% of the data to have comparable performances to the models trained on full data, which is experimented on the Alpaca and WizardLM datasets.
- The IFD score proposed by us can divide the samples into better or relatively bad ones, which might provide insight into the types of data good for instruction tuning.
- (Selective Reflection-Tuning) The IFD scores and the reversed version can be utilized to construct better data! In Reflection-Tuning Code for Selection, we proposed the Teacher-Student Collaboration pipeline to construct a training set customized for the student.
- (Superfiltering) The IFD scores calculated by LLMs with different sizes share strong consistencies! Thus you can utilize a really small language model like GPT2 to select the data for instruction tuning, which would be super fast and efficient! Please see Superfiltering for details.
Install the dependencies with pip install -r requirements.txt
Note: This requirements.txt
is originated from the Stanford Alpaca. If you are using a different code base with PyTorch installed, we recommend you manually install the below packages and do not need to install from requirements.txt
pip install tqdm
pip install scikit-learn
- Select Pre-Experienced Data
python cherry_seletion/data_analysis.py \
--data_path data/alpaca_data.json \
--save_path alpaca_data_pre.pt \
--model_name_or_path <your_path_to_hf_converted_llama_ckpt_and_tokenizer> \
--max_length 512 \
--prompt alpaca \
--mod pre
--data_path
: The targeted dataset in the Alpaca format
--save_path
: The path to save the .pt
file containing embeddings or scores
--prompt
: The prompt type used for training and selecting data, can choose between alpaca
or wiz
--mod
: pre
used for getting needed embeddings or scores on selecting pre-experienced samples and cherry
used for cherry
python cherry_seletion/data_by_cluster.py \
--pt_data_path alpaca_data_pre.pt \
--json_data_path data/alpaca_data.json \
--json_save_path alpaca_data_pre.json \
--sample_num 10 \
--kmeans_num_clusters 100 \
--low_th 25 \
--up_th 75
--pt_data_path
: The .pt
file from previous step containing needed embeddings or scores
--json_data_path
: The targeted dataset in the Alpaca format
--json_save_path
: The path to save the selected pre-experienced samples
--sample_num
: How many samples will be selected in each cluster
--kmeans_num_clusters
: How many clusters will be generated by K-Means
--low_th
and --up_th
: The lower and Upper threshold for selecting samples within each cluster
-
Train Pre-Experienced Model
-
Select Cherry Data
python cherry_seletion/data_analysis.py \
--data_path data/alpaca_data.json \
--save_path alpaca_data_cherry.pt \
--model_name_or_path <your_path_pre_experienced_model> \
--max_length 512 \
--prompt alpaca \
--mod cherry
python cherry_seletion/data_by_IFD.py \
--pt_data_path alpaca_data_cherry.pt \
--json_data_path data/alpaca_data.json \
--json_save_path alpaca_data_cherry.json \
--max_length 512 \
--sample_rate 0.06 \
--prompt alpaca
--sample_rate
: How many cherry samples you would like to select? You can also use --sample_number
to set the exact number of samples.
- Train Cherry Model
The following table provides a comparison between our cherry models and baseline models on the Huggingface Open LLM Leaderboard and AlpacaEval Leaderboard.
These results are based on cherry_data_v1. The prompt and training hyperparameters can be found in the Hyperparameters section.
These results verify the effectiveness of our method, which can be used to select the most valuable data samples for instruction tuning.
Avg | ARC | HellaSwag | MMLU | TruthfulQA | AlpacaEval | Data | Model | |||
---|---|---|---|---|---|---|---|---|---|---|
Alpaca | 50.21 | 42.65 | 76.91 | 41.73 | 39.55 | 26.46 | / | / | ||
5% Alpaca | 52.06 | 53.92 | 79.49 | 36.51 | 38.33 | 34.74 | [Link] | [hf-Link] | ||
10% Alpaca | / | / | / | / | / | / | [Link] | [hf-Link] | ||
15% Alpaca | / | / | / | / | / | / | [Link] | [hf-Link] | ||
WizardLM | 54.18 | 51.60 | 77.70 | 42.70 | 44.70 | 67.64 | / | / | ||
WizardLM* | 52.79 | 53.07 | 77.44 | 37.75 | 42.90 | 61.99 | [hf-Link] | [hf-Link] | ||
10% WizardLM | 51.59 | 52.90 | 78.95 | 33.08 | 41.41 | 61.44 | [Link] | [hf-Link] | ||
20% WizardLM | / | / | / | / | / | / | [Link] | [hf-Link] | ||
20% WizardLM | / | / | / | / | / | / | [Link] | [hf-Link] | ||
40% WizardLM | 52.83 | 53.07 | 77.79 | 35.29 | 45.17 | 65.09 | [Link] | [hf-Link] | ||
Also, the WizardLM filter script is provided here: [Link]
Thanks to the FastChat and flash-attention, we are able to run our experiments with longer length.
The above results are directly using cherry_data_v1 for finetuning the llama-2-7B model, with the length of 2048, and using original vicuna prompts.
Avg | ARC | HellaSwag | MMLU | TruthfulQA | AlpacaEval | Data | Model | |||
---|---|---|---|---|---|---|---|---|---|---|
WizardLM | 57.09 | 54.18 | 79.25 | 46.92 | 48.01 | 66.08 | / | [Link] | ||
10% WizardLM | 57.57 | 54.86 | 80.46 | 45.74 | 49.20 | 71.36 | [Link] | [Link] | ||
20% WizardLM | / | / | / | / | / | / | [Link] | [Link] | ||
20% WizardLM | 58.50 | 55.97 | 80.40 | 46.87 | 50.76 | 72.57 | [Link] | [Link] | ||
40% WizardLM | 58.00 | 56.23 | 80.22 | 46.15 | 49.37 | 70.52 | [Link] | [Link] | ||
Note: WizardLM in the above table is our implementation using FastChat code, prompt, and configuration.
Note: Due to the hardware limit, all our models are using the 7B model.
Note: For these llama2 models, we still use the cherry_data_v1 to ensure the effectiveness of our data. We will soon make the cherry_data_v2 which is based on llama2 available.
In this section, all the IFD scores are calculated on llama2-7b or llama2-13b models by using Vicuna's prompt. The training of pre-experienced models is discarded for more efficient usage. The performances are promising in the llama2 model even without a pre-experienced model, indicating the proficiency of our proposed IFD scores.
Avg | ARC | HellaSwag | MMLU | TruthfulQA | AlpacaEval | Data | Model | |||
---|---|---|---|---|---|---|---|---|---|---|
Alpaca-7b (llama2) | 55.25 | 54.35 | 78.65 | 47.02 | 40.98 | 27.75 | / | / | ||
5% Alpaca-7b (llama2) | 55.78 | 57.94 | 80.37 | 44.91 | 40.62 | 36.78 | / | / | ||
10% Alpaca-7b (llama2) | 56.31 | 58.02 | 80.42 | 46.64 | 40.18 | / | / | / | ||
15% Alpaca-7b (llama2) | 56.37 | 57.42 | 80.68 | 46.40 | 40.95 | / | / | / | ||
Alpaca-13b (llama2) | 58.78 | 57.59 | 81.98 | 54.05 | 41.49 | 35.00 | / | / | ||
5% Alpaca-13b (llama2) | 61.21 | 62.37 | 84.00 | 55.65 | 42.82 | 46.82 | / | / | ||
10% Alpaca-13b (llama2) | 61.02 | 62.97 | 83.88 | 55.29 | 41.93 | / | / | / | ||
15% Alpaca-13b (llama2) | 61.23 | 62.37 | 83.48 | 55.56 | 43.42 | / | / | / |
All the above models are trained using FastChat code and prompt.
Data with IFD scores will be released soon.
We release the codes and data for using GPT4 or chatGPT to evaluate and compare the performance between two LLMs. This method greatly eliminates the potential position bias of GPT4 and chatGPT. For details, please see AlpaGasus or our paper. We thank @Lichang-Chen and AlpaGasus repo for sharing the evaluation codes.
To use this code, please follow the below scripts:
bash scripts/do_eval_generation.sh
: The model automatically generates the responses for a given instruction in test datasets.
bash scripts/do_eval_generation_wrap.sh
: Wrap the response files of LLMs being compared.
bash scripts/do_eval.sh
: Use GPT4 or chatGPT for the evaluation.
bash scripts/do_review_eval_score.sh
: Parse the results and draw the figure.
More detailed illustrations will be updated. Feel free to drop me an email if you are urgent about it.
Comparing our models trained on selected data with models trained on full data. (a) Comparison between our model with 5% Alpaca data and the official Alpaca model. (b) Comparison between our model with 10% WizardLM data and the reimplemented WizardLM model. (c) Comparison between our model with 40% WizardLM data and the official WizardLM model. All these experiments use GPT4 as the judge. Each horizontal bar represents a comparison in a specific test set.
We used the following prompts for fine-tuning the cherry models with Alpaca data:
- for examples with a non-empty input field:
Below is an instruction that describes a task, paired with an input that provides further context. Write a response that appropriately completes the request.
### Instruction:
{instruction}
### Input:
{input}
### Response:
- for examples with an empty input field:
Below is an instruction that describes a task. Write a response that appropriately completes the request.
### Instruction:
{instruction}
### Response:
We used the following prompts for fine-tuning the cherry models with Wizard data:
{instruction}
### Response:
Hyperparameter | Global Batch Size | Learning rate | Epochs | Max length | Weight decay | Warmup Rate |
---|---|---|---|---|---|---|
Cherry Models V1 (Alpaca) | 128 | 2e-5 | 3 | 512 | 0 | 0.03 |
Cherry Models V1 (WizardLM) | 128 | 2e-5 | 3 | 1024 | 0 | 0.03 |
--- | ---: | ---: | ---: | ---: | ---: | ---: |
Cherry Models V2 7B | 128 | 2e-5 | 3 | 2048 | 0 | 0.03 |
Cherry Models V2 13B | 128 | 1e-5 | 5 | 2048 | 0 | 0.03 |
- [x] Release the code, data, and models.
- [x] Release the evaluation code for comparison.
- [x] Train Cherry WizardLM with the length of 2048.
- [x] Implement our method on llama 2 models.
- [x] Modify the paper
Please consider citing our paper if you think our codes, data, or models are useful. Thank you!
@inproceedings{li-etal-2024-quantity,
title = "From Quantity to Quality: Boosting {LLM} Performance with Self-Guided Data Selection for Instruction Tuning",
author = "Li, Ming and
Zhang, Yong and
Li, Zhitao and
Chen, Jiuhai and
Chen, Lichang and
Cheng, Ning and
Wang, Jianzong and
Zhou, Tianyi and
Xiao, Jing",
editor = "Duh, Kevin and
Gomez, Helena and
Bethard, Steven",
booktitle = "Proceedings of the 2024 Conference of the North American Chapter of the Association for Computational Linguistics: Human Language Technologies (Volume 1: Long Papers)",
month = jun,
year = "2024",
address = "Mexico City, Mexico",
publisher = "Association for Computational Linguistics",
url = "https://aclanthology.org/2024.naacl-long.421",
pages = "7595--7628",
}
@inproceedings{li-etal-2024-superfiltering,
title = "Superfiltering: Weak-to-Strong Data Filtering for Fast Instruction-Tuning",
author = "Li, Ming and
Zhang, Yong and
He, Shwai and
Li, Zhitao and
Zhao, Hongyu and
Wang, Jianzong and
Cheng, Ning and
Zhou, Tianyi",
editor = "Ku, Lun-Wei and
Martins, Andre and
Srikumar, Vivek",
booktitle = "Proceedings of the 62nd Annual Meeting of the Association for Computational Linguistics (Volume 1: Long Papers)",
month = aug,
year = "2024",
address = "Bangkok, Thailand",
publisher = "Association for Computational Linguistics",
url = "https://aclanthology.org/2024.acl-long.769",
pages = "14255--14273",
}
@inproceedings{li-etal-2024-selective,
title = "Selective Reflection-Tuning: Student-Selected Data Recycling for {LLM} Instruction-Tuning",
author = "Li, Ming and
Chen, Lichang and
Chen, Jiuhai and
He, Shwai and
Gu, Jiuxiang and
Zhou, Tianyi",
editor = "Ku, Lun-Wei and
Martins, Andre and
Srikumar, Vivek",
booktitle = "Findings of the Association for Computational Linguistics ACL 2024",
month = aug,
year = "2024",
address = "Bangkok, Thailand and virtual meeting",
publisher = "Association for Computational Linguistics",
url = "https://aclanthology.org/2024.findings-acl.958",
pages = "16189--16211",
}
@inproceedings{li2023reflectiontuning,
title={Reflection-Tuning: Recycling Data for Better Instruction-Tuning},
author={Ming Li and Lichang Chen and Jiuhai Chen and Shwai He and Tianyi Zhou},
booktitle={NeurIPS 2023 Workshop on Instruction Tuning and Instruction Following},
year={2023},
url={https://openreview.net/forum?id=xaqoZZqkPU}
}
If you are interested in Data Selection for Instruction Tuning, please see Cherry_LLM and Superfiltering.
If you are interested in human/LLM-free Data Augmentation for Instruction Tuning, please see Mosaic-IT and RuleR.
If you are interested in Data Improvement for Instruction Tuning, please see Reflection_Tuning.
If you are interested in Knowledge Distillation in the LLM era, please see this Survey.
For Tasks:
Click tags to check more tools for each tasksFor Jobs:
Alternative AI tools for Cherry_LLM
Similar Open Source Tools
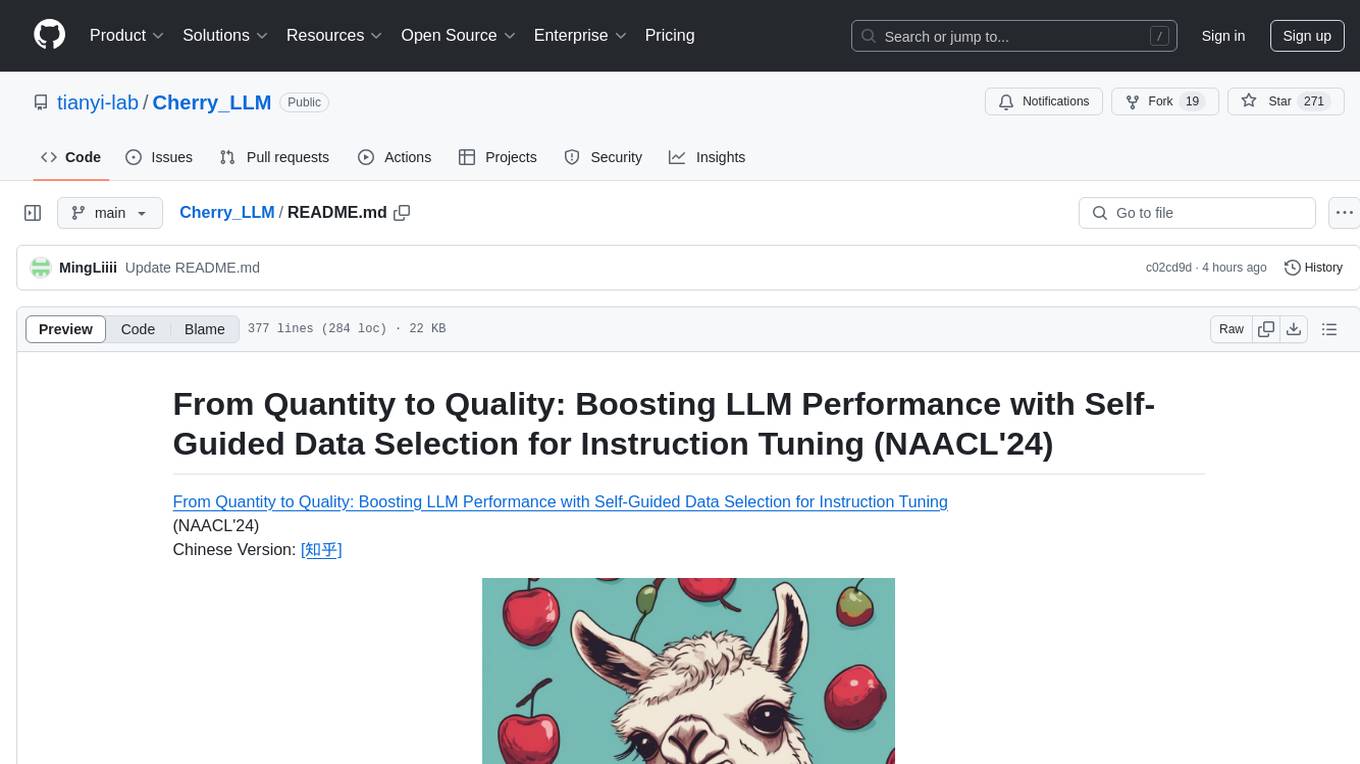
Cherry_LLM
Cherry Data Selection project introduces a self-guided methodology for LLMs to autonomously discern and select cherry samples from open-source datasets, minimizing manual curation and cost for instruction tuning. The project focuses on selecting impactful training samples ('cherry data') to enhance LLM instruction tuning by estimating instruction-following difficulty. The method involves phases like 'Learning from Brief Experience', 'Evaluating Based on Experience', and 'Retraining from Self-Guided Experience' to improve LLM performance.
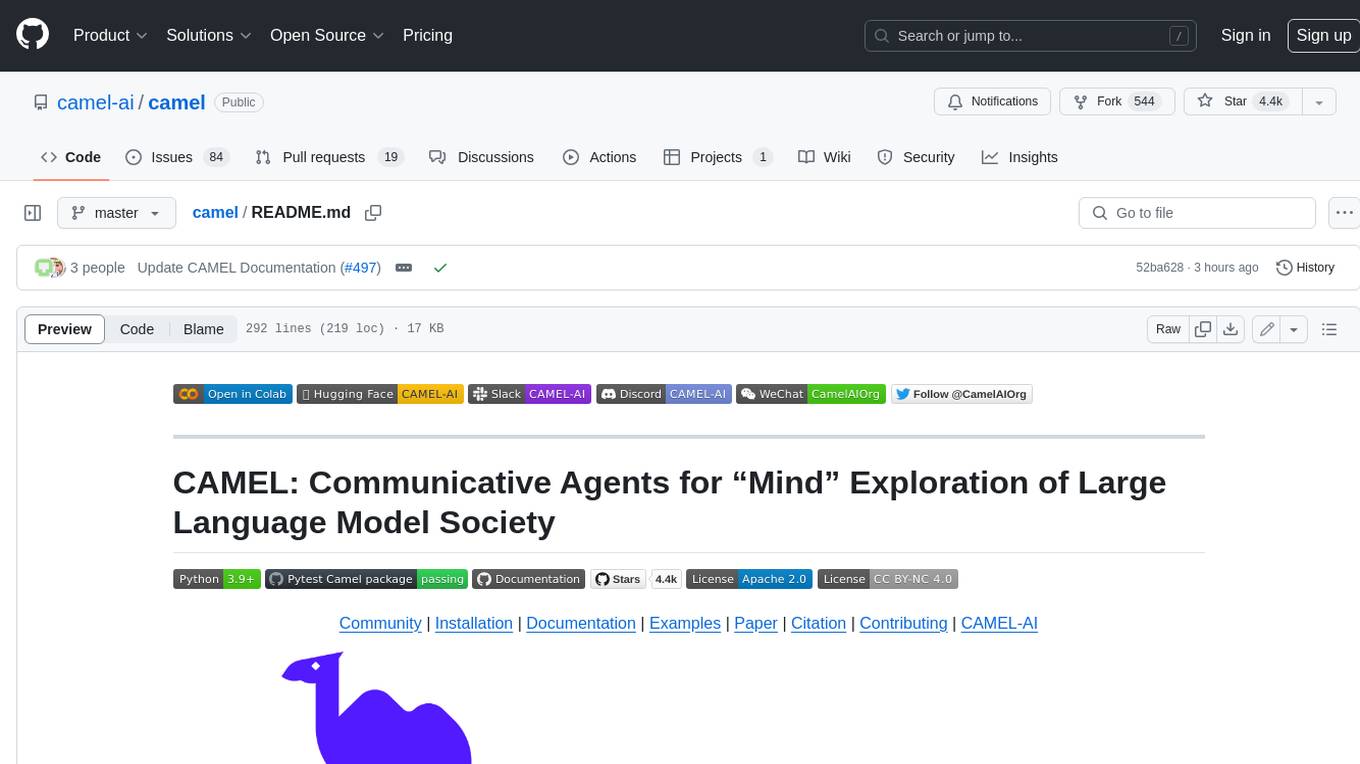
camel
CAMEL is an open-source library designed for the study of autonomous and communicative agents. We believe that studying these agents on a large scale offers valuable insights into their behaviors, capabilities, and potential risks. To facilitate research in this field, we implement and support various types of agents, tasks, prompts, models, and simulated environments.
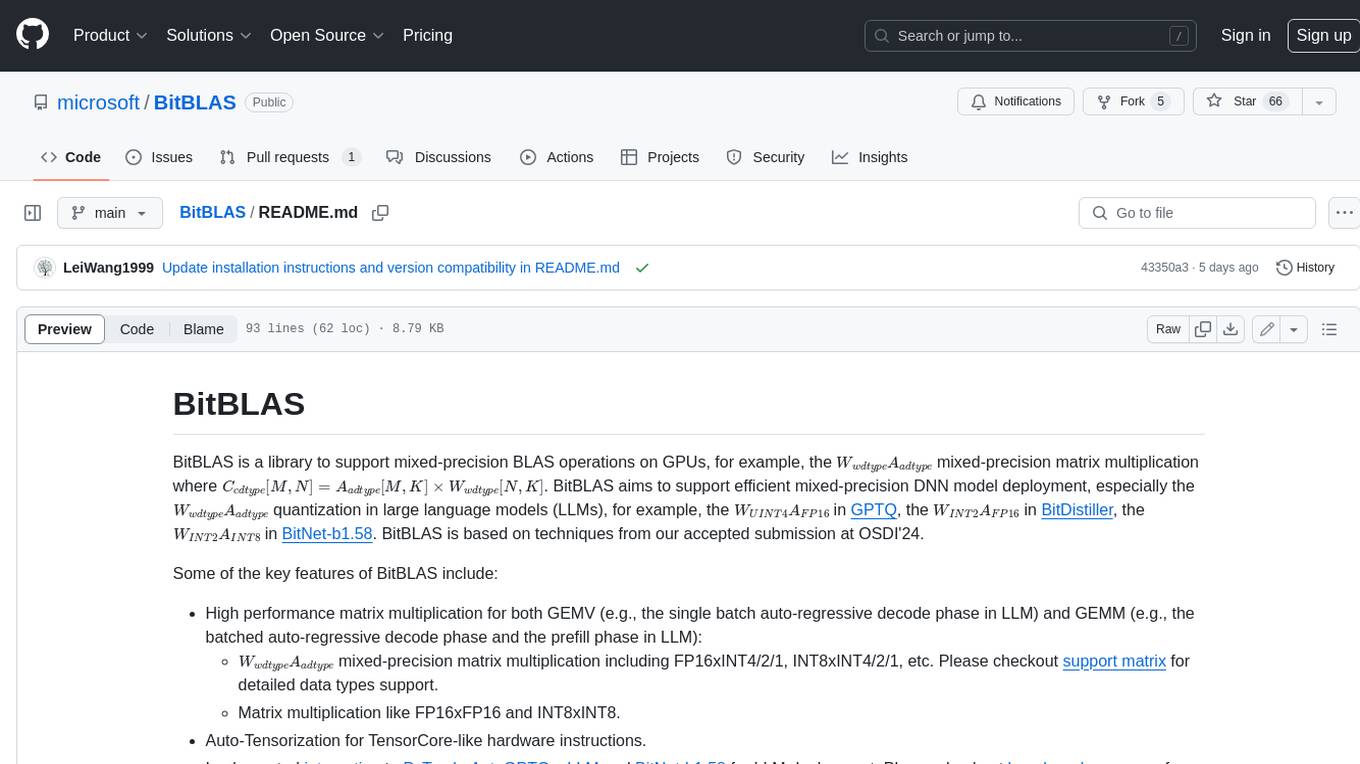
BitBLAS
BitBLAS is a library for mixed-precision BLAS operations on GPUs, for example, the $W_{wdtype}A_{adtype}$ mixed-precision matrix multiplication where $C_{cdtype}[M, N] = A_{adtype}[M, K] \times W_{wdtype}[N, K]$. BitBLAS aims to support efficient mixed-precision DNN model deployment, especially the $W_{wdtype}A_{adtype}$ quantization in large language models (LLMs), for example, the $W_{UINT4}A_{FP16}$ in GPTQ, the $W_{INT2}A_{FP16}$ in BitDistiller, the $W_{INT2}A_{INT8}$ in BitNet-b1.58. BitBLAS is based on techniques from our accepted submission at OSDI'24.
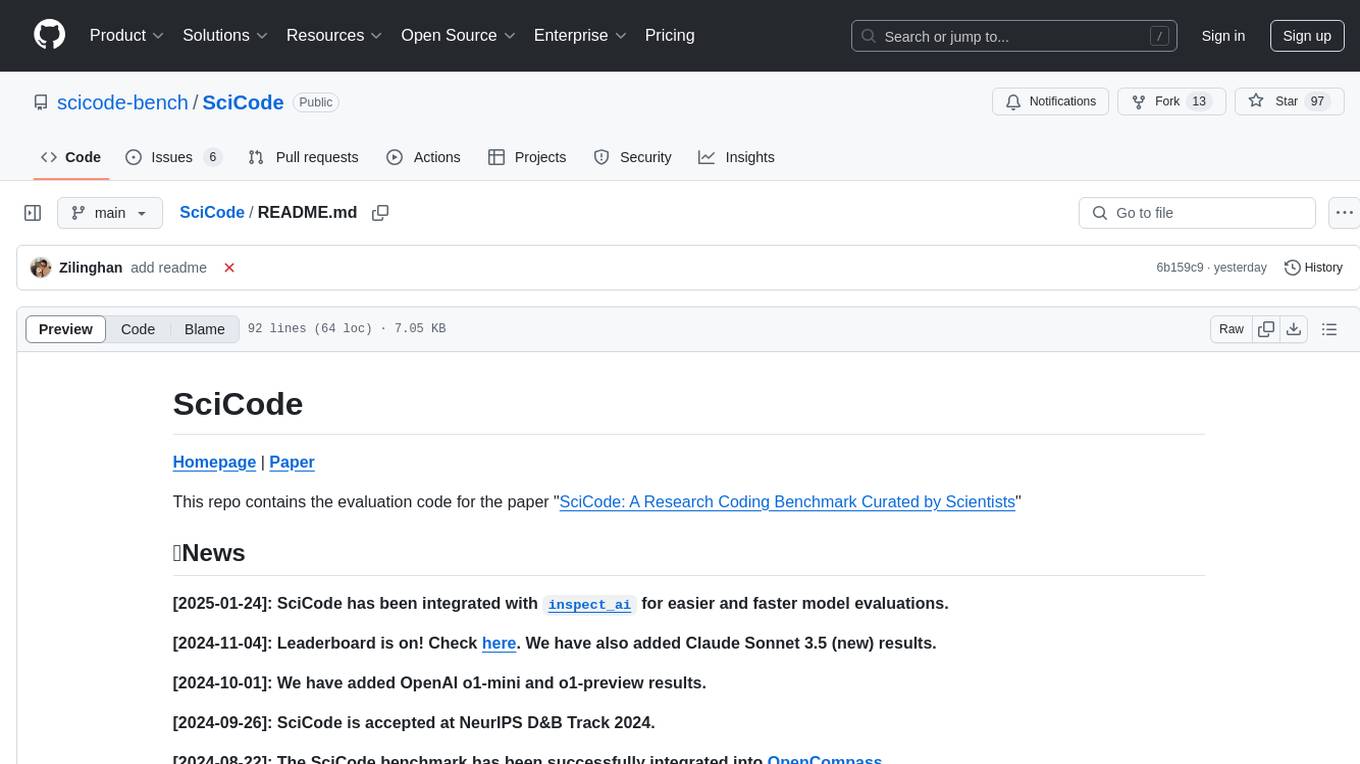
SciCode
SciCode is a challenging benchmark designed to evaluate the capabilities of language models (LMs) in generating code for solving realistic scientific research problems. It contains 338 subproblems decomposed from 80 challenging main problems across 16 subdomains from 6 domains. The benchmark offers optional descriptions specifying useful scientific background information and scientist-annotated gold-standard solutions and test cases for evaluation. SciCode demonstrates a realistic workflow of identifying critical science concepts and facts and transforming them into computation and simulation code, aiming to help showcase LLMs' progress towards assisting scientists and contribute to the future building and evaluation of scientific AI.
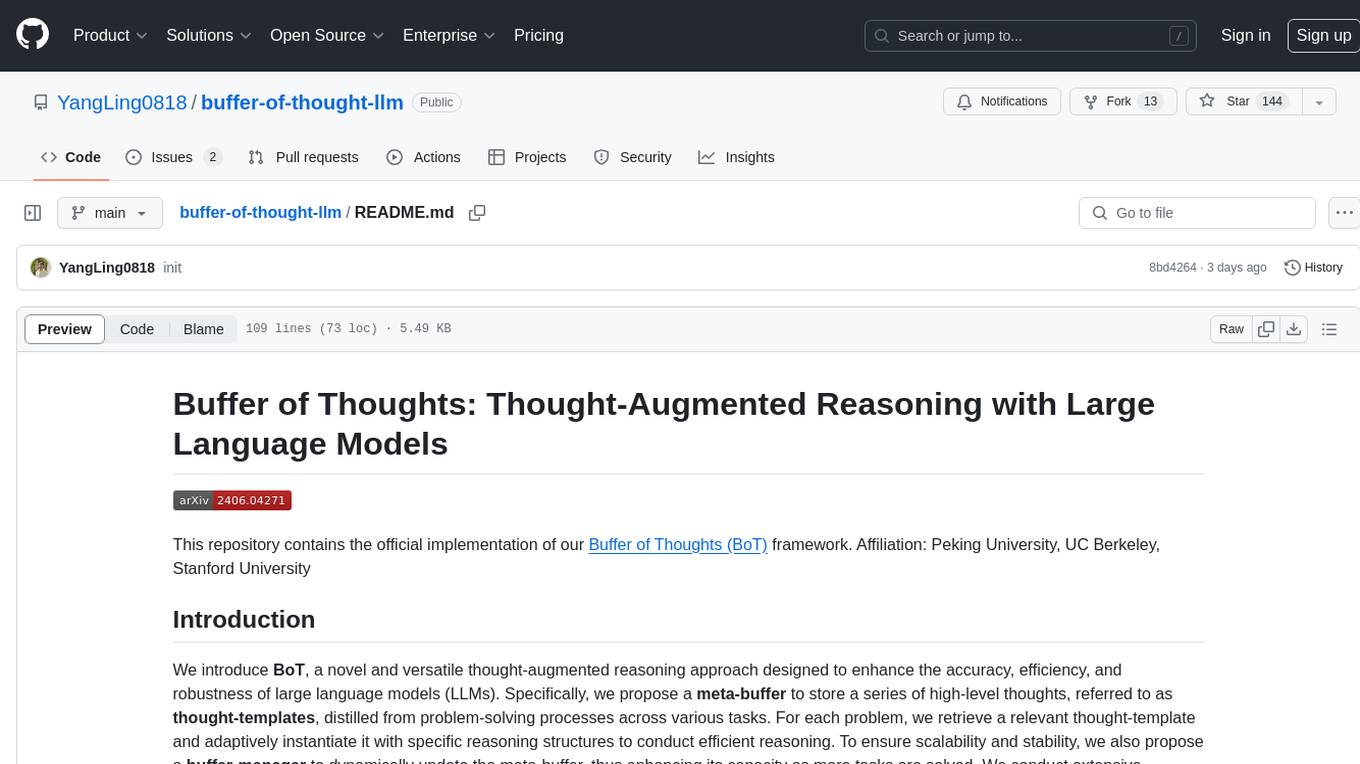
buffer-of-thought-llm
Buffer of Thoughts (BoT) is a thought-augmented reasoning framework designed to enhance the accuracy, efficiency, and robustness of large language models (LLMs). It introduces a meta-buffer to store high-level thought-templates distilled from problem-solving processes, enabling adaptive reasoning for efficient problem-solving. The framework includes a buffer-manager to dynamically update the meta-buffer, ensuring scalability and stability. BoT achieves significant performance improvements on reasoning-intensive tasks and demonstrates superior generalization ability and robustness while being cost-effective compared to other methods.
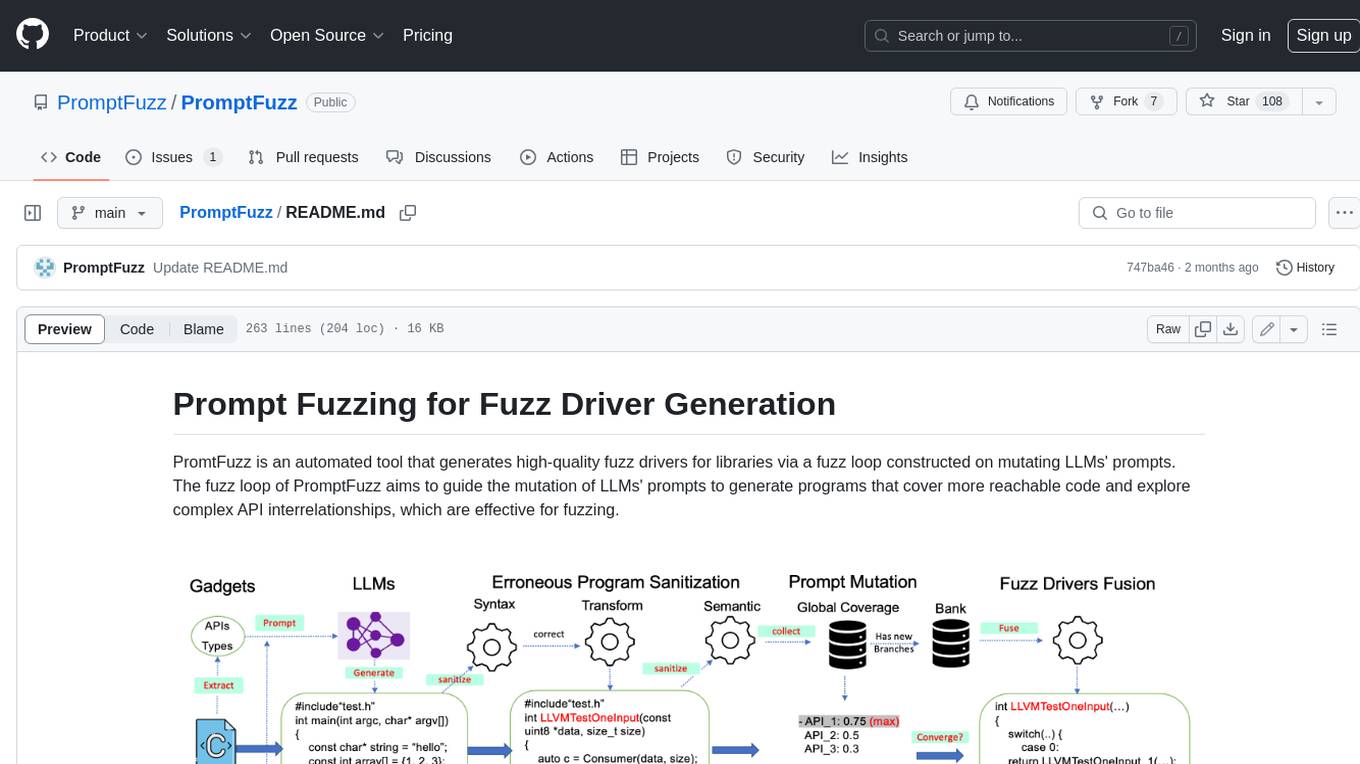
PromptFuzz
**Description:** PromptFuzz is an automated tool that generates high-quality fuzz drivers for libraries via a fuzz loop constructed on mutating LLMs' prompts. The fuzz loop of PromptFuzz aims to guide the mutation of LLMs' prompts to generate programs that cover more reachable code and explore complex API interrelationships, which are effective for fuzzing. **Features:** * **Multiply LLM support** : Supports the general LLMs: Codex, Inocder, ChatGPT, and GPT4 (Currently tested on ChatGPT). * **Context-based Prompt** : Construct LLM prompts with the automatically extracted library context. * **Powerful Sanitization** : The program's syntax, semantics, behavior, and coverage are thoroughly analyzed to sanitize the problematic programs. * **Prioritized Mutation** : Prioritizes mutating the library API combinations within LLM's prompts to explore complex interrelationships, guided by code coverage. * **Fuzz Driver Exploitation** : Infers API constraints using statistics and extends fixed API arguments to receive random bytes from fuzzers. * **Fuzz engine integration** : Integrates with grey-box fuzz engine: LibFuzzer. **Benefits:** * **High branch coverage:** The fuzz drivers generated by PromptFuzz achieved a branch coverage of 40.12% on the tested libraries, which is 1.61x greater than _OSS-Fuzz_ and 1.67x greater than _Hopper_. * **Bug detection:** PromptFuzz detected 33 valid security bugs from 49 unique crashes. * **Wide range of bugs:** The fuzz drivers generated by PromptFuzz can detect a wide range of bugs, most of which are security bugs. * **Unique bugs:** PromptFuzz detects uniquely interesting bugs that other fuzzers may miss. **Usage:** 1. Build the library using the provided build scripts. 2. Export the LLM API KEY if using ChatGPT or GPT4. 3. Generate fuzz drivers using the `fuzzer` command. 4. Run the fuzz drivers using the `harness` command. 5. Deduplicate and analyze the reported crashes. **Future Works:** * **Custom LLMs suport:** Support custom LLMs. * **Close-source libraries:** Apply PromptFuzz to close-source libraries by fine tuning LLMs on private code corpus. * **Performance** : Reduce the huge time cost required in erroneous program elimination.
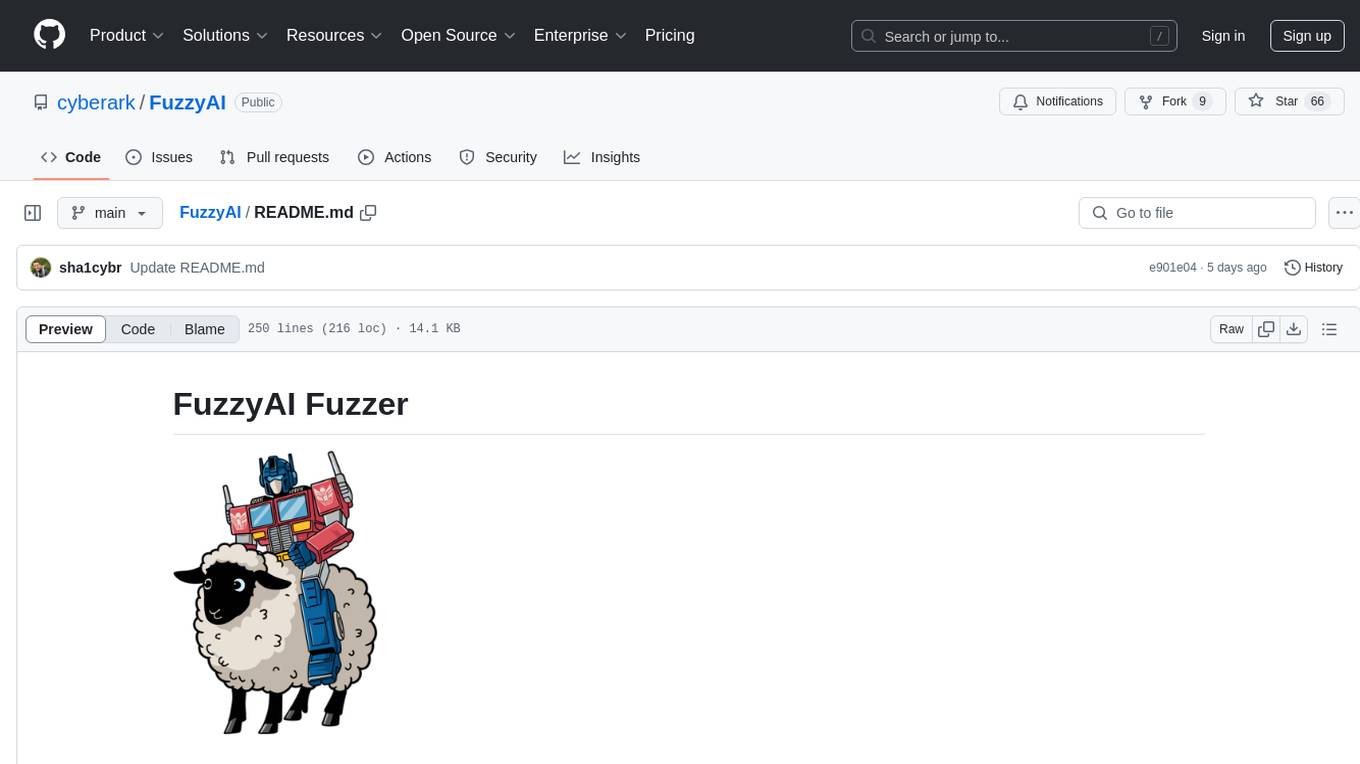
FuzzyAI
The FuzzyAI Fuzzer is a powerful tool for automated LLM fuzzing, designed to help developers and security researchers identify jailbreaks and mitigate potential security vulnerabilities in their LLM APIs. It supports various fuzzing techniques, provides input generation capabilities, can be easily integrated into existing workflows, and offers an extensible architecture for customization and extension. The tool includes attacks like ArtPrompt, Taxonomy-based paraphrasing, Many-shot jailbreaking, Genetic algorithm, Hallucinations, DAN (Do Anything Now), WordGame, Crescendo, ActorAttack, Back To The Past, Please, Thought Experiment, and Default. It supports models from providers like Anthropic, OpenAI, Gemini, Azure, Bedrock, AI21, and Ollama, with the ability to add support for newer models. The tool also supports various cloud APIs and datasets for testing and experimentation.
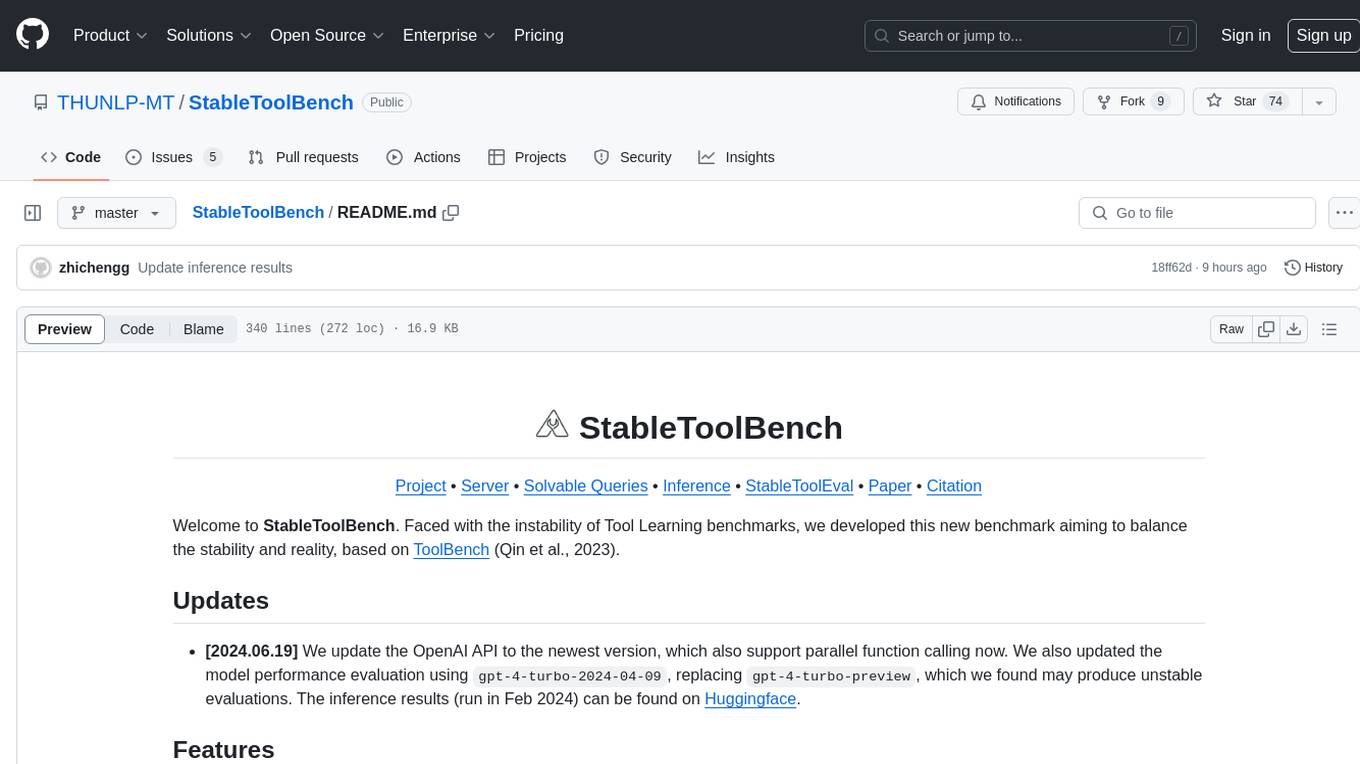
StableToolBench
StableToolBench is a new benchmark developed to address the instability of Tool Learning benchmarks. It aims to balance stability and reality by introducing features like Virtual API System, Solvable Queries, and Stable Evaluation System. The benchmark ensures consistency through a caching system and API simulators, filters queries based on solvability using LLMs, and evaluates model performance using GPT-4 with metrics like Solvable Pass Rate and Solvable Win Rate.
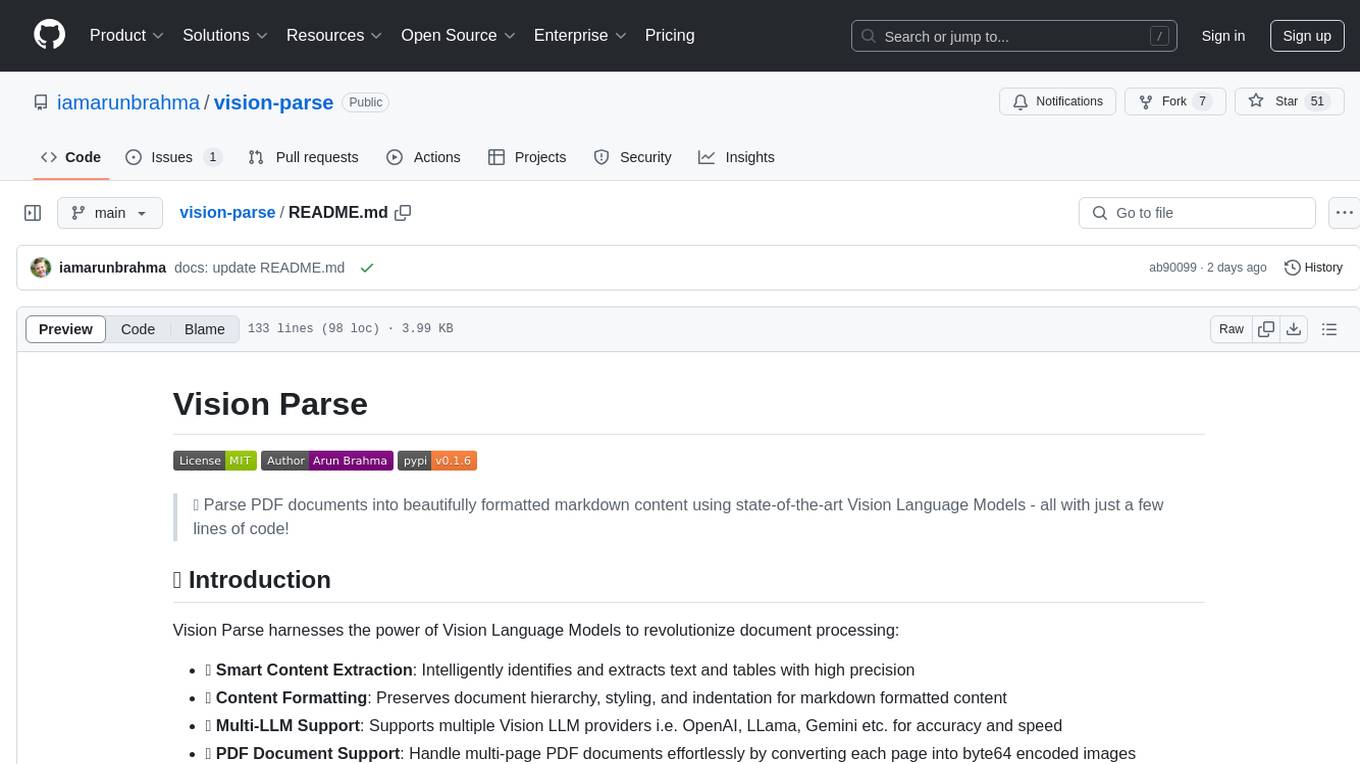
vision-parse
Vision Parse is a tool that leverages Vision Language Models to parse PDF documents into beautifully formatted markdown content. It offers smart content extraction, content formatting, multi-LLM support, PDF document support, and local model hosting using Ollama. Users can easily convert PDFs to markdown with high precision and preserve document hierarchy and styling. The tool supports multiple Vision LLM providers like OpenAI, LLama, and Gemini for accuracy and speed, making document processing efficient and effortless.
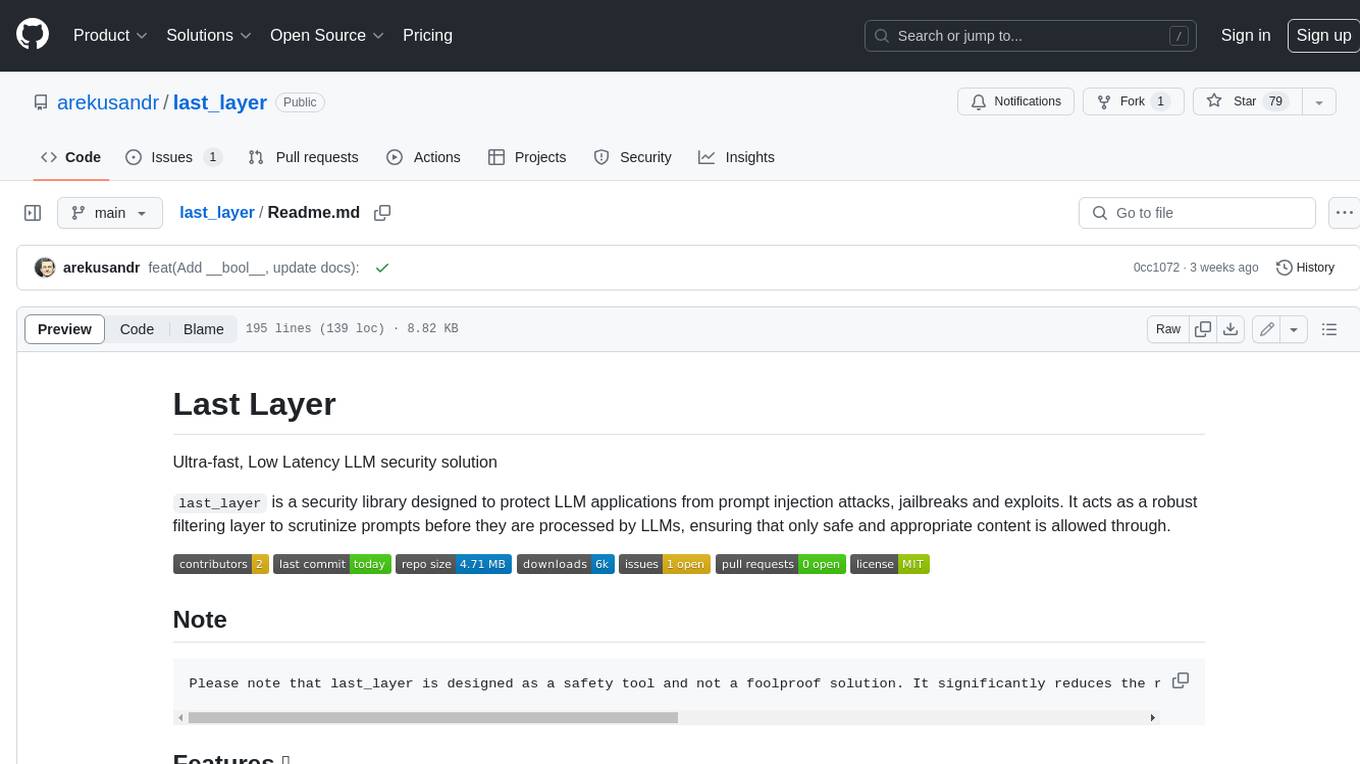
last_layer
last_layer is a security library designed to protect LLM applications from prompt injection attacks, jailbreaks, and exploits. It acts as a robust filtering layer to scrutinize prompts before they are processed by LLMs, ensuring that only safe and appropriate content is allowed through. The tool offers ultra-fast scanning with low latency, privacy-focused operation without tracking or network calls, compatibility with serverless platforms, advanced threat detection mechanisms, and regular updates to adapt to evolving security challenges. It significantly reduces the risk of prompt-based attacks and exploits but cannot guarantee complete protection against all possible threats.
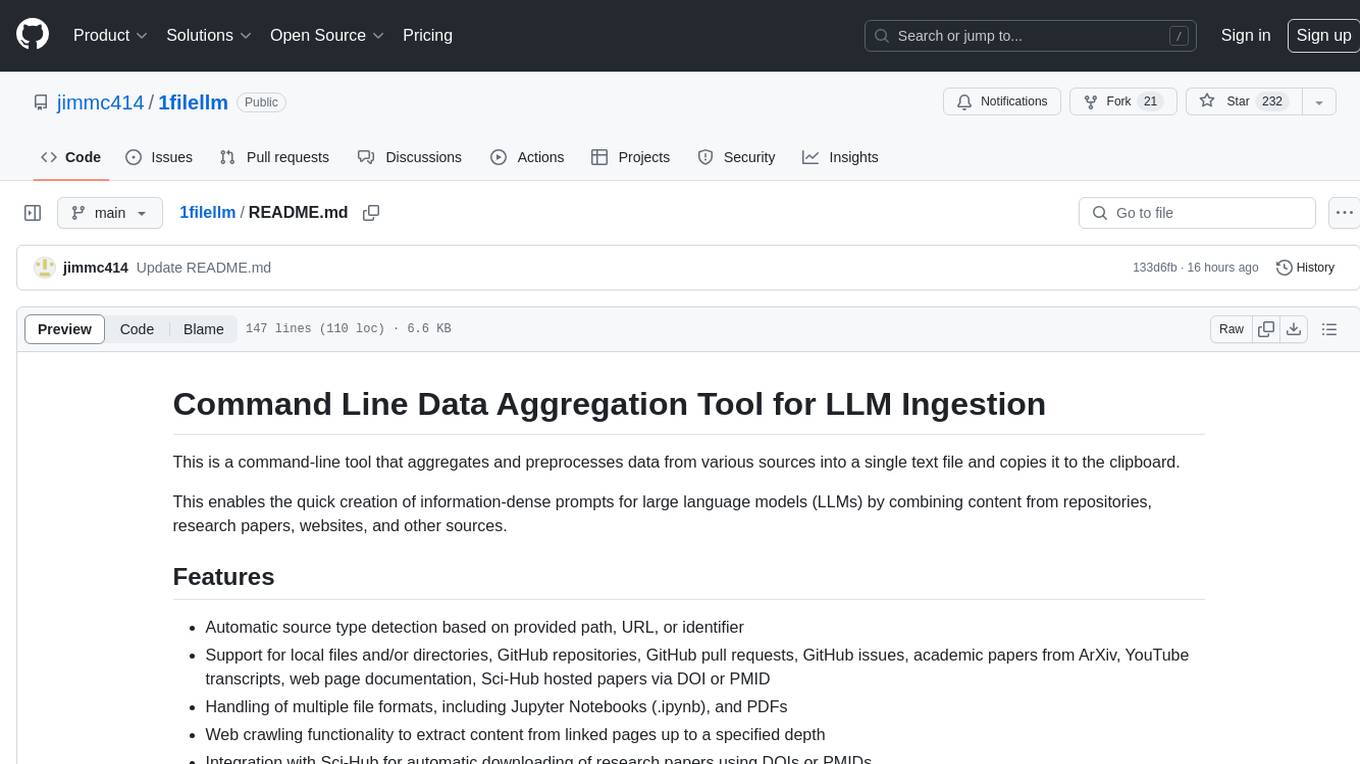
1filellm
1filellm is a command-line data aggregation tool designed for LLM ingestion. It aggregates and preprocesses data from various sources into a single text file, facilitating the creation of information-dense prompts for large language models. The tool supports automatic source type detection, handling of multiple file formats, web crawling functionality, integration with Sci-Hub for research paper downloads, text preprocessing, and token count reporting. Users can input local files, directories, GitHub repositories, pull requests, issues, ArXiv papers, YouTube transcripts, web pages, Sci-Hub papers via DOI or PMID. The tool provides uncompressed and compressed text outputs, with the uncompressed text automatically copied to the clipboard for easy pasting into LLMs.
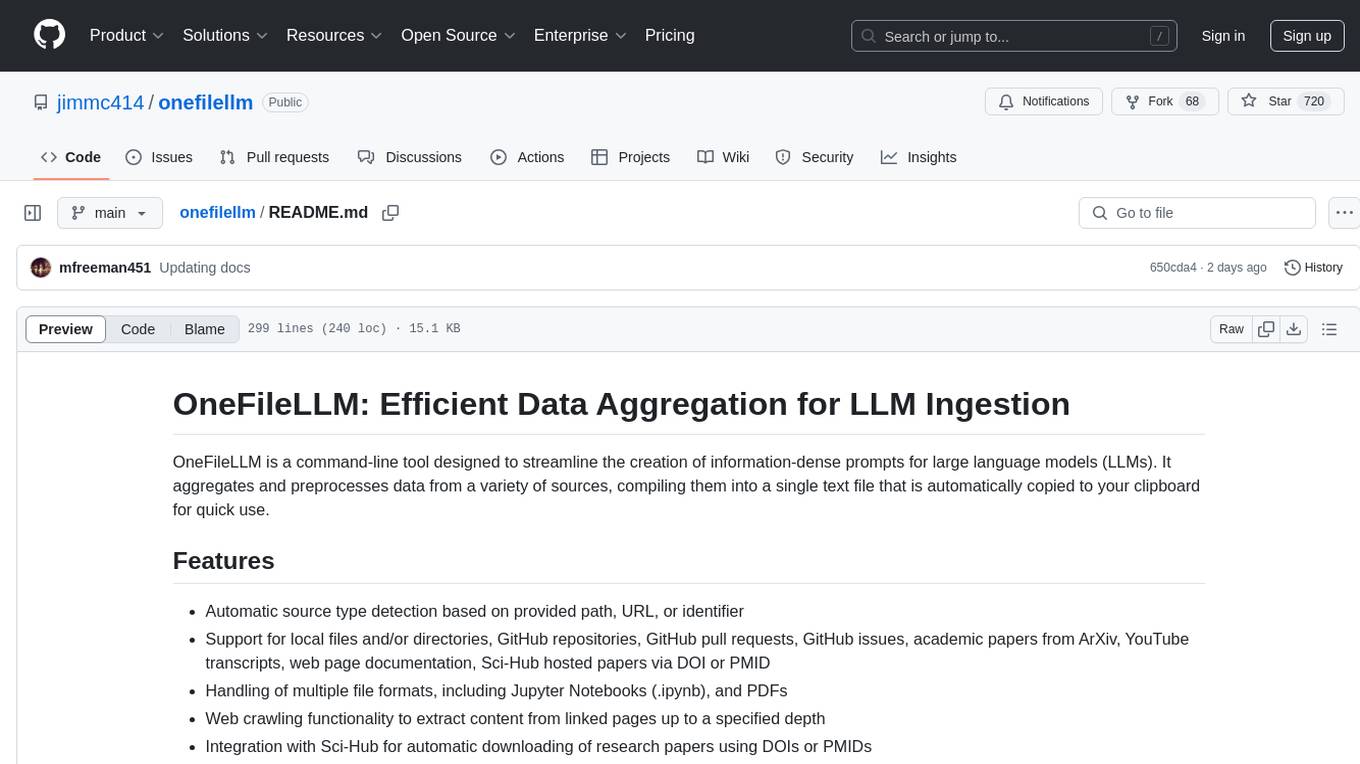
onefilellm
OneFileLLM is a command-line tool that streamlines the creation of information-dense prompts for large language models (LLMs). It aggregates and preprocesses data from various sources, compiling them into a single text file for quick use. The tool supports automatic source type detection, handling of multiple file formats, web crawling functionality, integration with Sci-Hub for research paper downloads, text preprocessing, token count reporting, and XML encapsulation of output for improved LLM performance. Users can easily access private GitHub repositories by generating a personal access token. The tool's output is encapsulated in XML tags to enhance LLM understanding and processing.
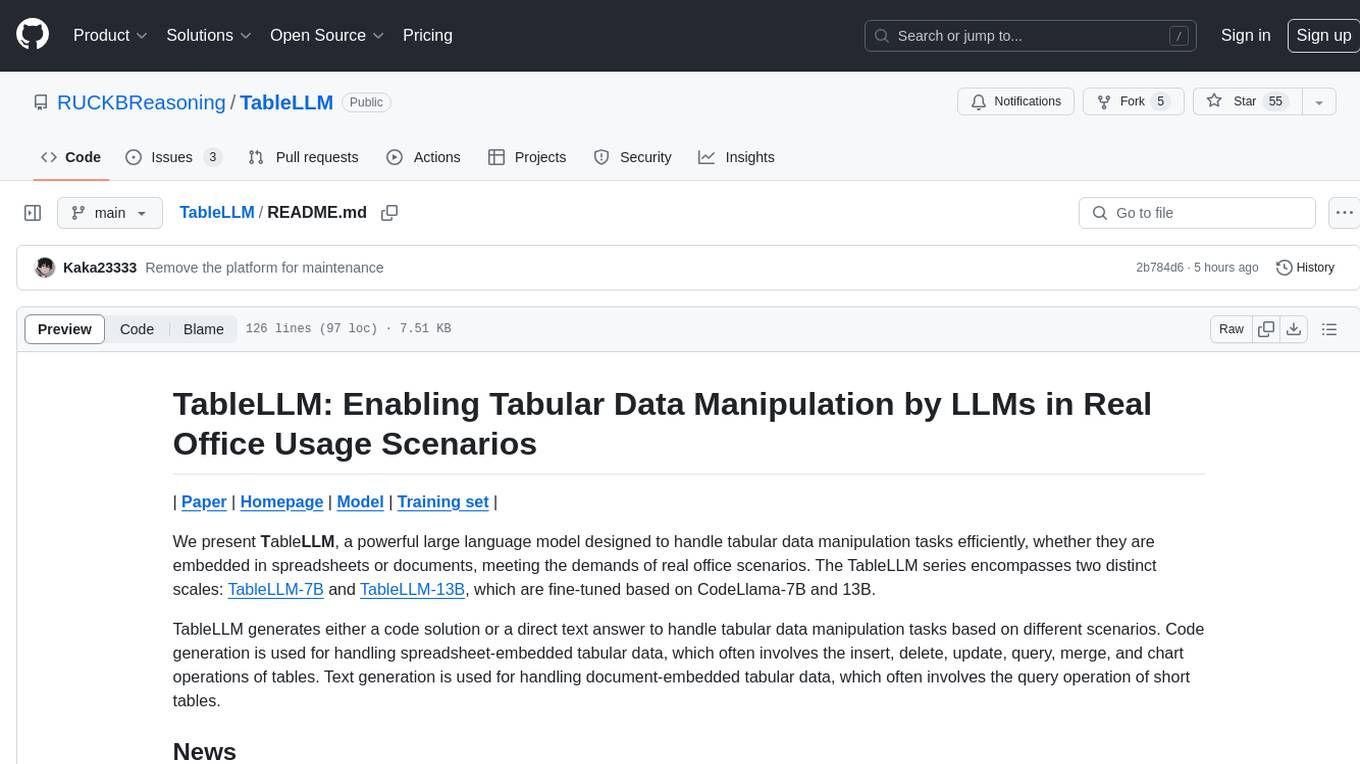
TableLLM
TableLLM is a large language model designed for efficient tabular data manipulation tasks in real office scenarios. It can generate code solutions or direct text answers for tasks like insert, delete, update, query, merge, and chart operations on tables embedded in spreadsheets or documents. The model has been fine-tuned based on CodeLlama-7B and 13B, offering two scales: TableLLM-7B and TableLLM-13B. Evaluation results show its performance on benchmarks like WikiSQL, Spider, and self-created table operation benchmark. Users can use TableLLM for code and text generation tasks on tabular data.
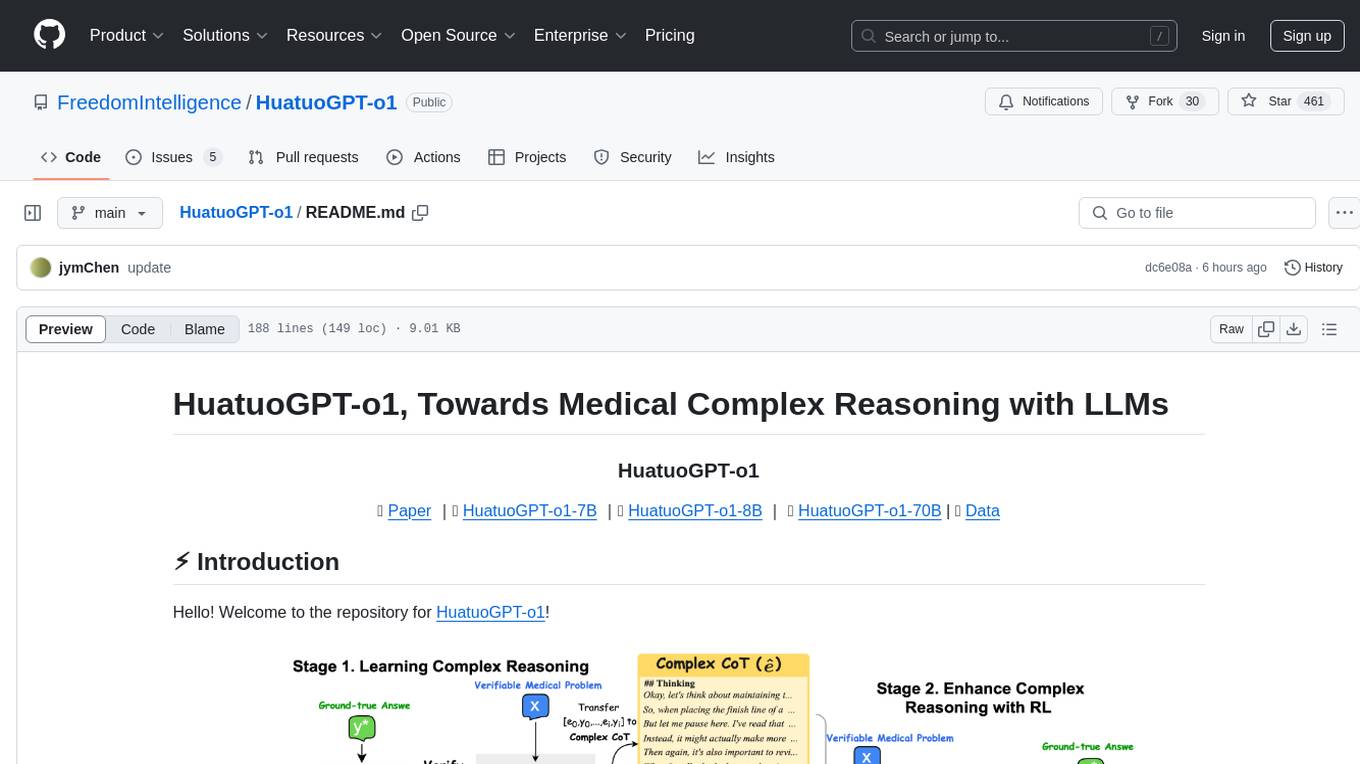
HuatuoGPT-o1
HuatuoGPT-o1 is a medical language model designed for advanced medical reasoning. It can identify mistakes, explore alternative strategies, and refine answers. The model leverages verifiable medical problems and a specialized medical verifier to guide complex reasoning trajectories and enhance reasoning through reinforcement learning. The repository provides access to models, data, and code for HuatuoGPT-o1, allowing users to deploy the model for medical reasoning tasks.
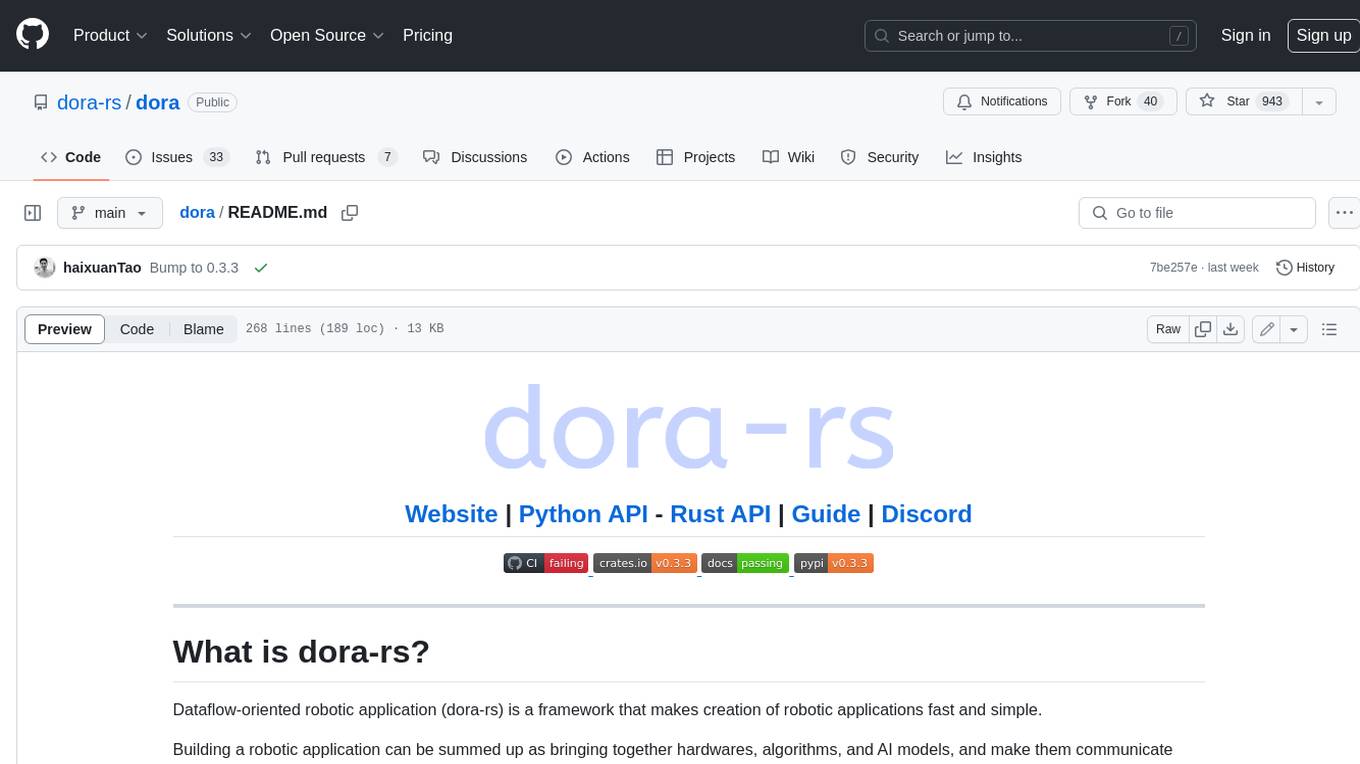
dora
Dataflow-oriented robotic application (dora-rs) is a framework that makes creation of robotic applications fast and simple. Building a robotic application can be summed up as bringing together hardwares, algorithms, and AI models, and make them communicate with each others. At dora-rs, we try to: make integration of hardware and software easy by supporting Python, C, C++, and also ROS2. make communication low latency by using zero-copy Arrow messages. dora-rs is still experimental and you might experience bugs, but we're working very hard to make it stable as possible.
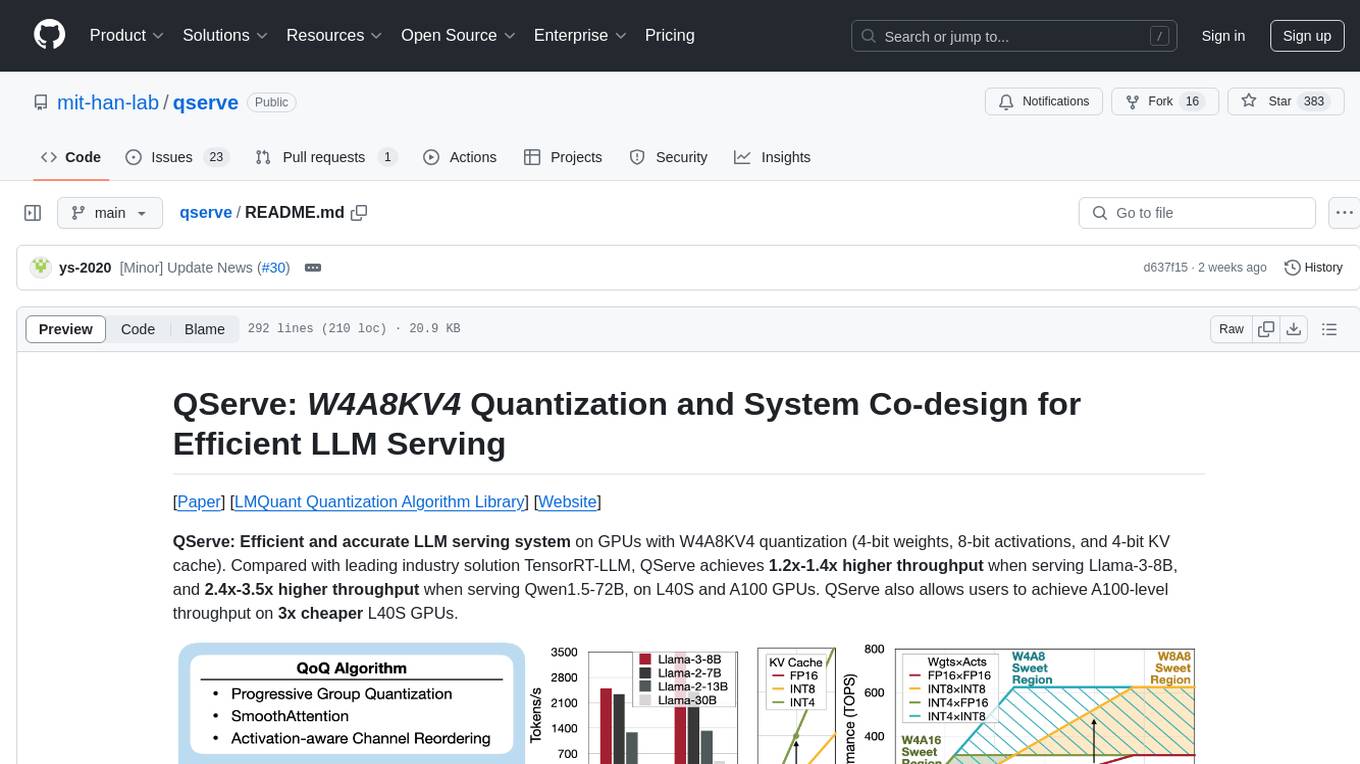
qserve
QServe is a serving system designed for efficient and accurate Large Language Models (LLM) on GPUs with W4A8KV4 quantization. It achieves higher throughput compared to leading industry solutions, allowing users to achieve A100-level throughput on cheaper L40S GPUs. The system introduces the QoQ quantization algorithm with 4-bit weight, 8-bit activation, and 4-bit KV cache, addressing runtime overhead challenges. QServe improves serving throughput for various LLM models by implementing compute-aware weight reordering, register-level parallelism, and fused attention memory-bound techniques.
For similar tasks
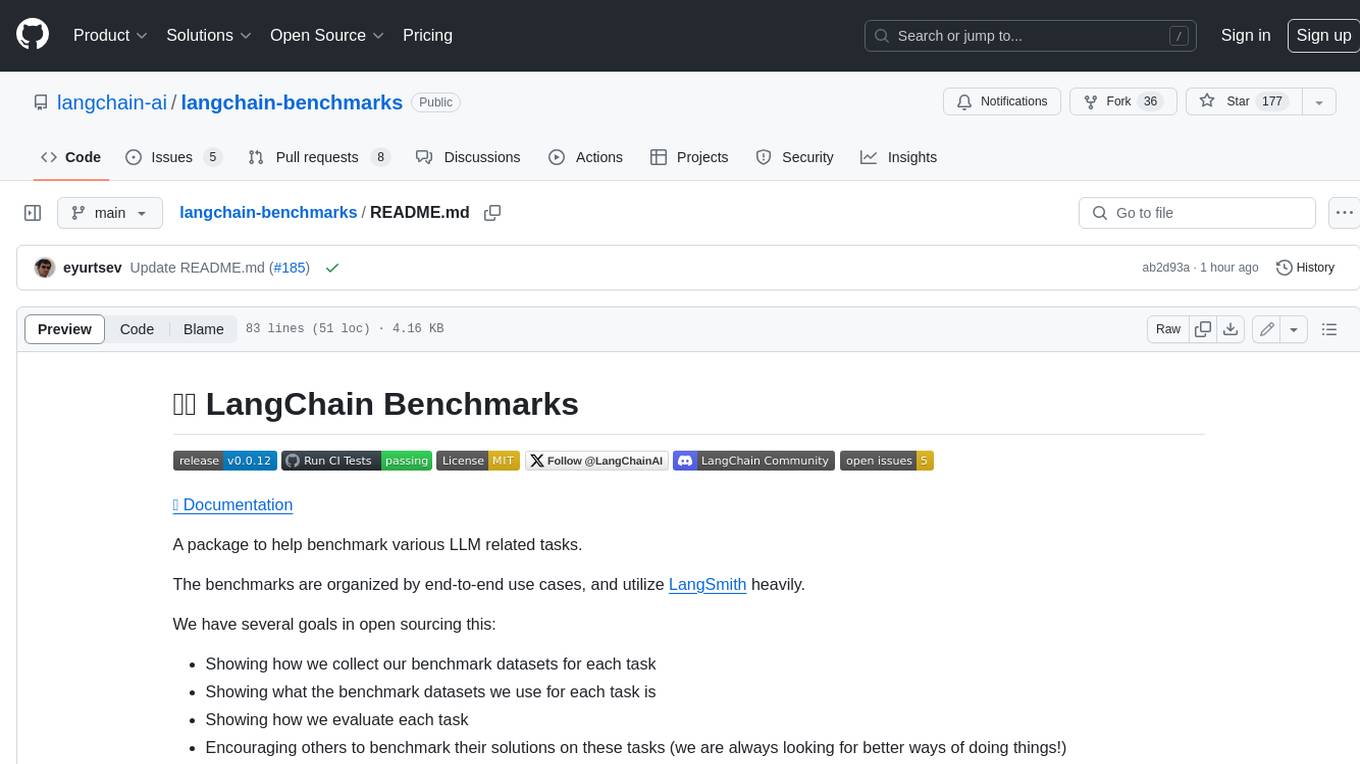
langchain-benchmarks
A package to help benchmark various LLM related tasks. The benchmarks are organized by end-to-end use cases, and utilize LangSmith heavily. We have several goals in open sourcing this: * Showing how we collect our benchmark datasets for each task * Showing what the benchmark datasets we use for each task is * Showing how we evaluate each task * Encouraging others to benchmark their solutions on these tasks (we are always looking for better ways of doing things!)
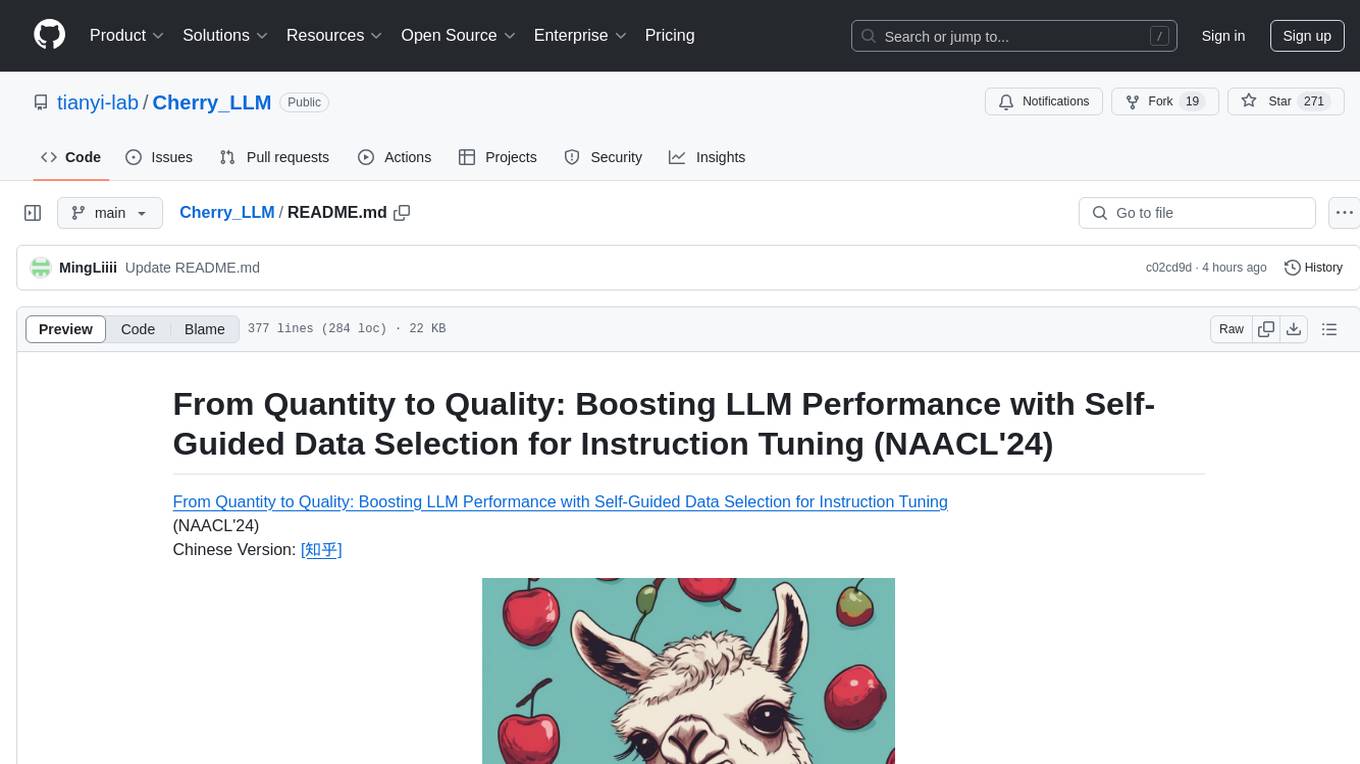
Cherry_LLM
Cherry Data Selection project introduces a self-guided methodology for LLMs to autonomously discern and select cherry samples from open-source datasets, minimizing manual curation and cost for instruction tuning. The project focuses on selecting impactful training samples ('cherry data') to enhance LLM instruction tuning by estimating instruction-following difficulty. The method involves phases like 'Learning from Brief Experience', 'Evaluating Based on Experience', and 'Retraining from Self-Guided Experience' to improve LLM performance.
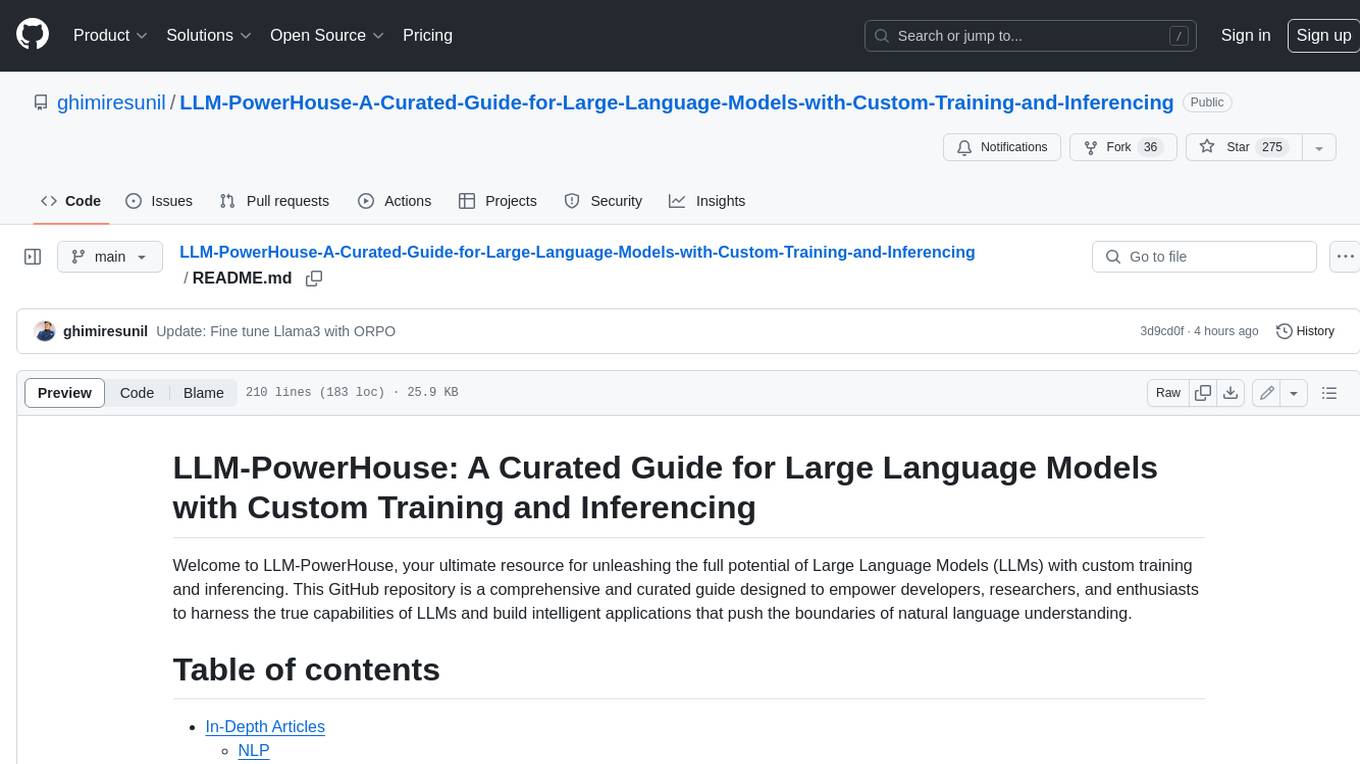
LLM-PowerHouse-A-Curated-Guide-for-Large-Language-Models-with-Custom-Training-and-Inferencing
LLM-PowerHouse is a comprehensive and curated guide designed to empower developers, researchers, and enthusiasts to harness the true capabilities of Large Language Models (LLMs) and build intelligent applications that push the boundaries of natural language understanding. This GitHub repository provides in-depth articles, codebase mastery, LLM PlayLab, and resources for cost analysis and network visualization. It covers various aspects of LLMs, including NLP, models, training, evaluation metrics, open LLMs, and more. The repository also includes a collection of code examples and tutorials to help users build and deploy LLM-based applications.
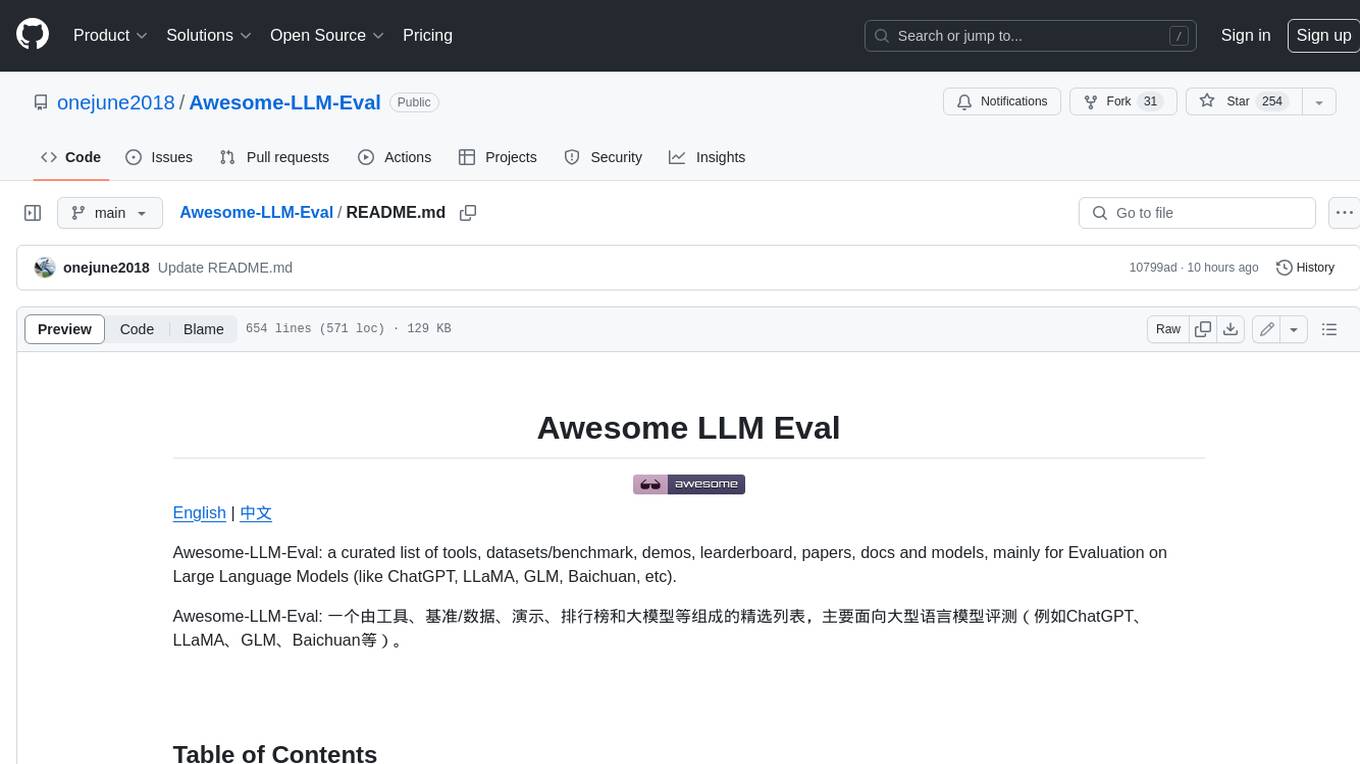
Awesome-LLM-Eval
Awesome-LLM-Eval: a curated list of tools, benchmarks, demos, papers for Large Language Models (like ChatGPT, LLaMA, GLM, Baichuan, etc) Evaluation on Language capabilities, Knowledge, Reasoning, Fairness and Safety.
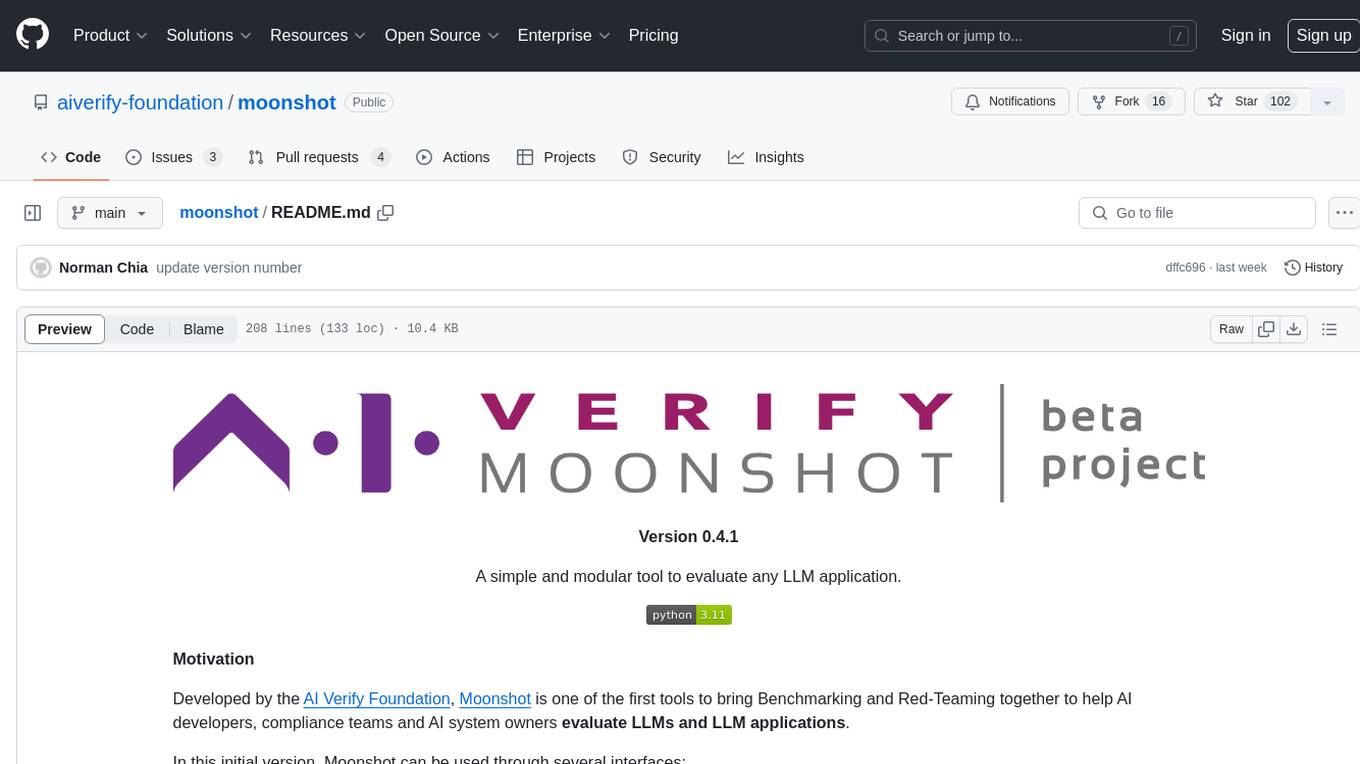
moonshot
Moonshot is a simple and modular tool developed by the AI Verify Foundation to evaluate Language Model Models (LLMs) and LLM applications. It brings Benchmarking and Red-Teaming together to assist AI developers, compliance teams, and AI system owners in assessing LLM performance. Moonshot can be accessed through various interfaces including User-friendly Web UI, Interactive Command Line Interface, and seamless integration into MLOps workflows via Library APIs or Web APIs. It offers features like benchmarking LLMs from popular model providers, running relevant tests, creating custom cookbooks and recipes, and automating Red Teaming to identify vulnerabilities in AI systems.
For similar jobs
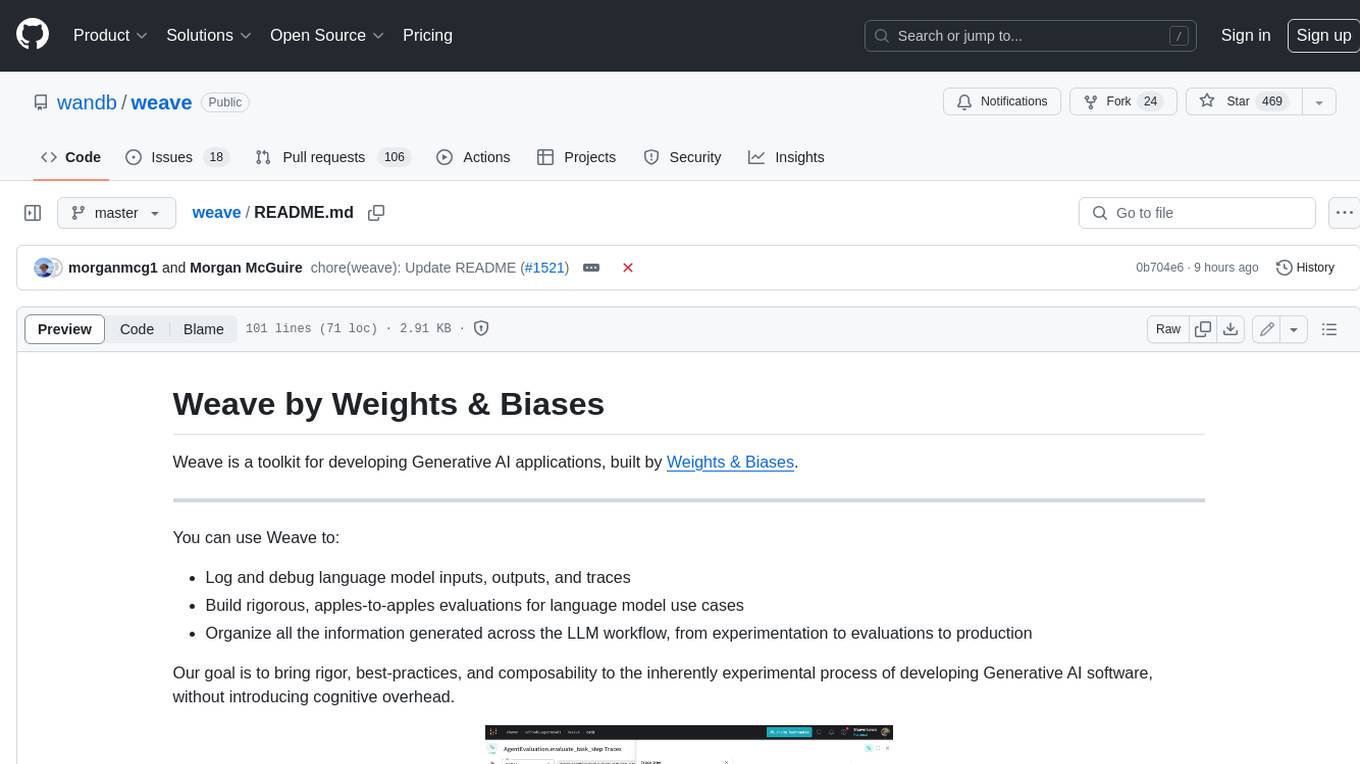
weave
Weave is a toolkit for developing Generative AI applications, built by Weights & Biases. With Weave, you can log and debug language model inputs, outputs, and traces; build rigorous, apples-to-apples evaluations for language model use cases; and organize all the information generated across the LLM workflow, from experimentation to evaluations to production. Weave aims to bring rigor, best-practices, and composability to the inherently experimental process of developing Generative AI software, without introducing cognitive overhead.
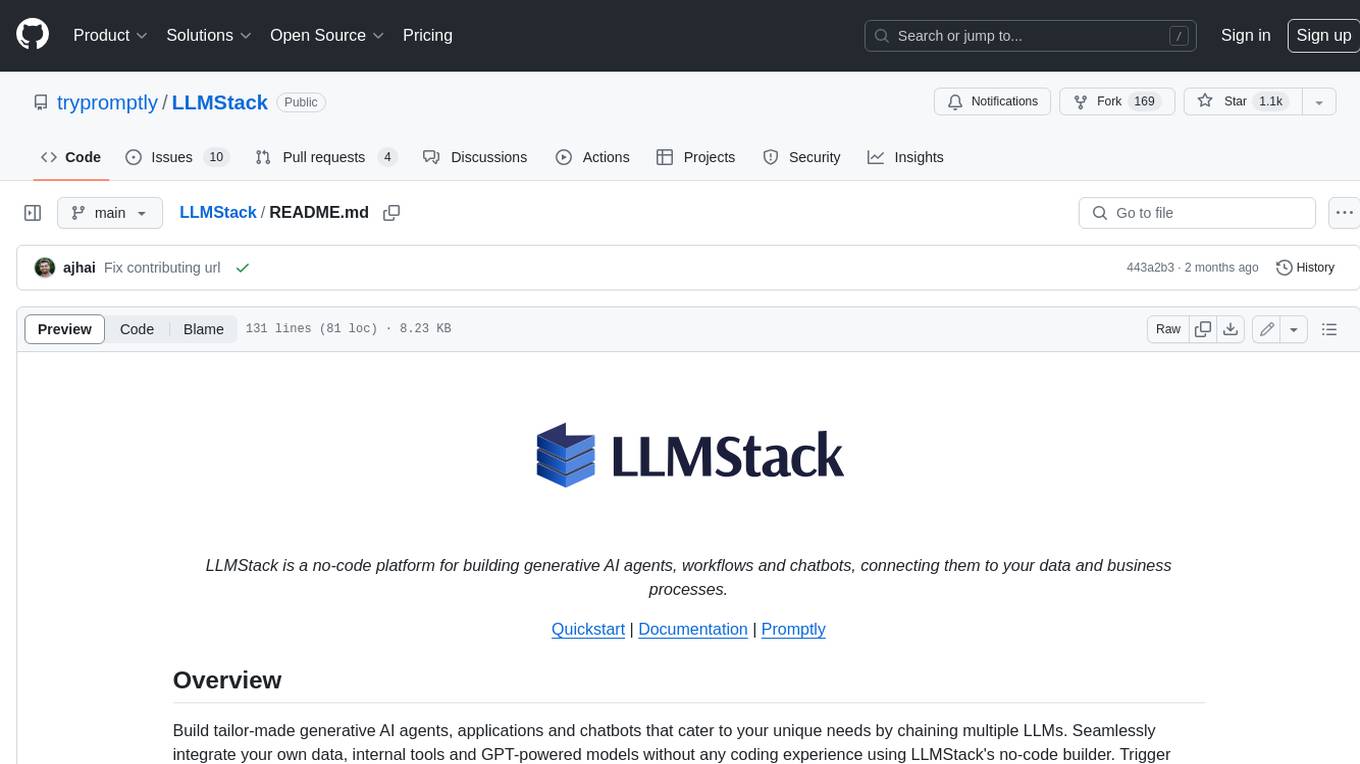
LLMStack
LLMStack is a no-code platform for building generative AI agents, workflows, and chatbots. It allows users to connect their own data, internal tools, and GPT-powered models without any coding experience. LLMStack can be deployed to the cloud or on-premise and can be accessed via HTTP API or triggered from Slack or Discord.
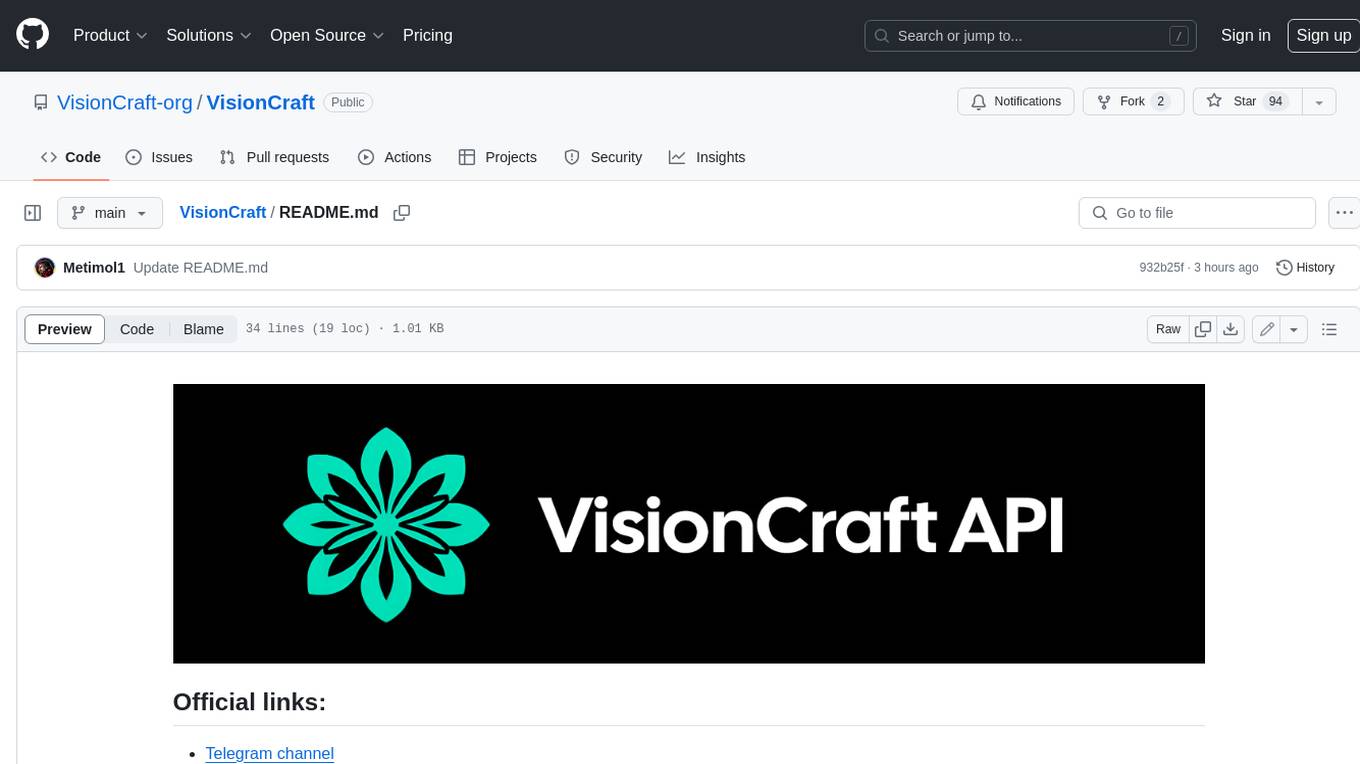
VisionCraft
The VisionCraft API is a free API for using over 100 different AI models. From images to sound.
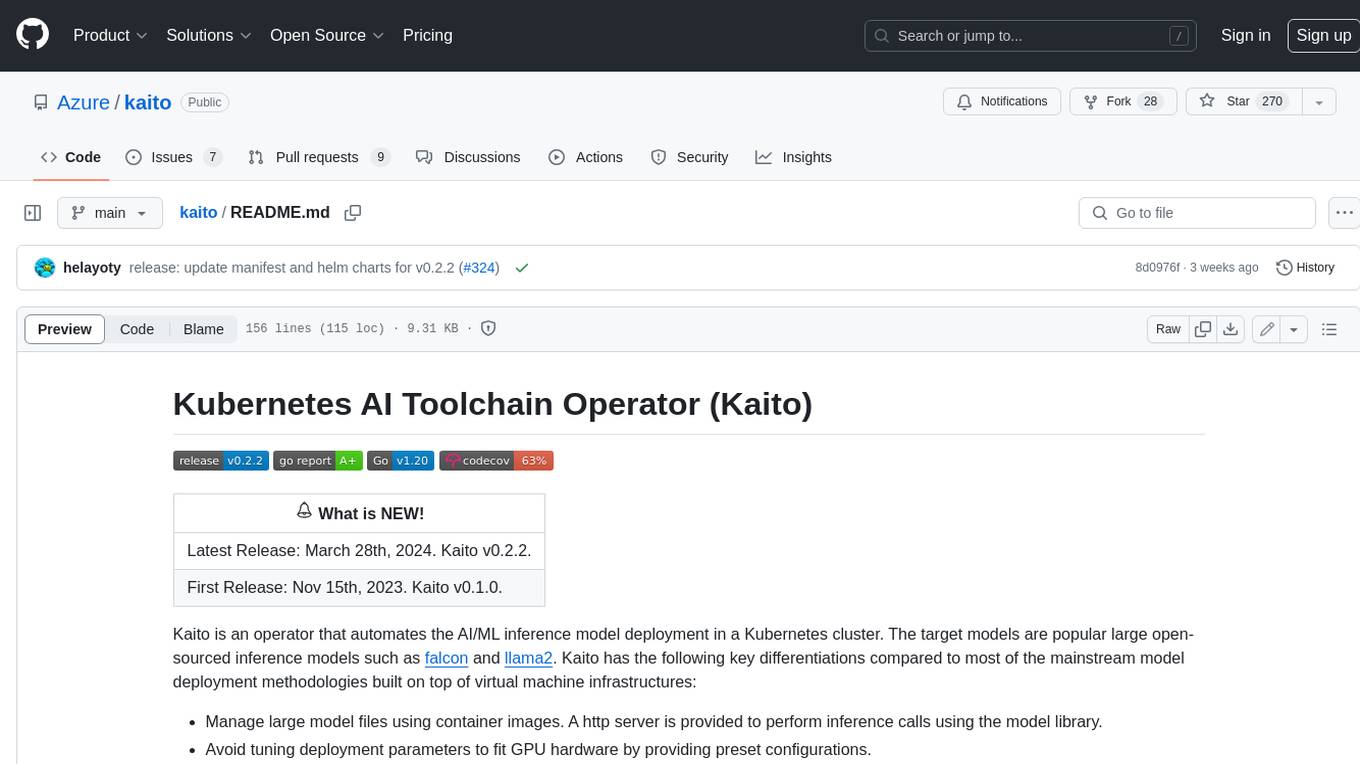
kaito
Kaito is an operator that automates the AI/ML inference model deployment in a Kubernetes cluster. It manages large model files using container images, avoids tuning deployment parameters to fit GPU hardware by providing preset configurations, auto-provisions GPU nodes based on model requirements, and hosts large model images in the public Microsoft Container Registry (MCR) if the license allows. Using Kaito, the workflow of onboarding large AI inference models in Kubernetes is largely simplified.
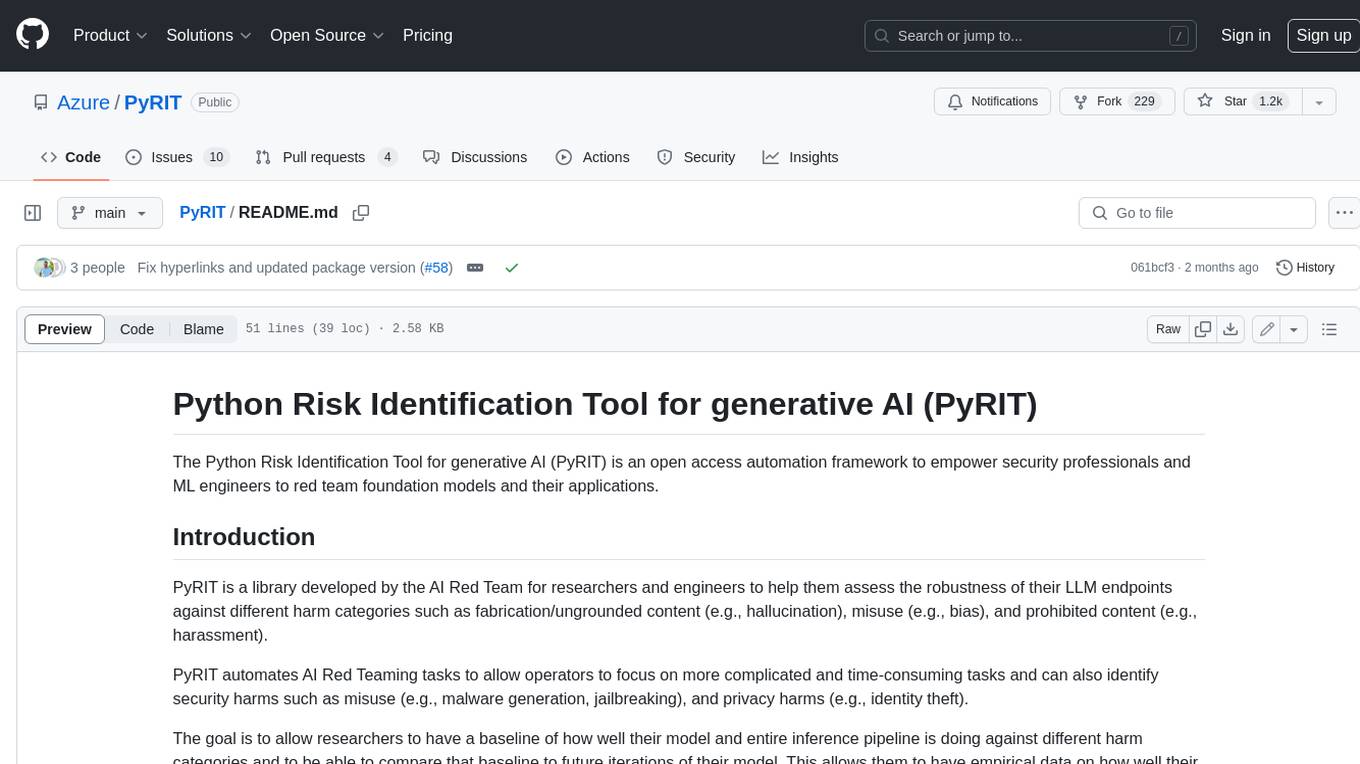
PyRIT
PyRIT is an open access automation framework designed to empower security professionals and ML engineers to red team foundation models and their applications. It automates AI Red Teaming tasks to allow operators to focus on more complicated and time-consuming tasks and can also identify security harms such as misuse (e.g., malware generation, jailbreaking), and privacy harms (e.g., identity theft). The goal is to allow researchers to have a baseline of how well their model and entire inference pipeline is doing against different harm categories and to be able to compare that baseline to future iterations of their model. This allows them to have empirical data on how well their model is doing today, and detect any degradation of performance based on future improvements.
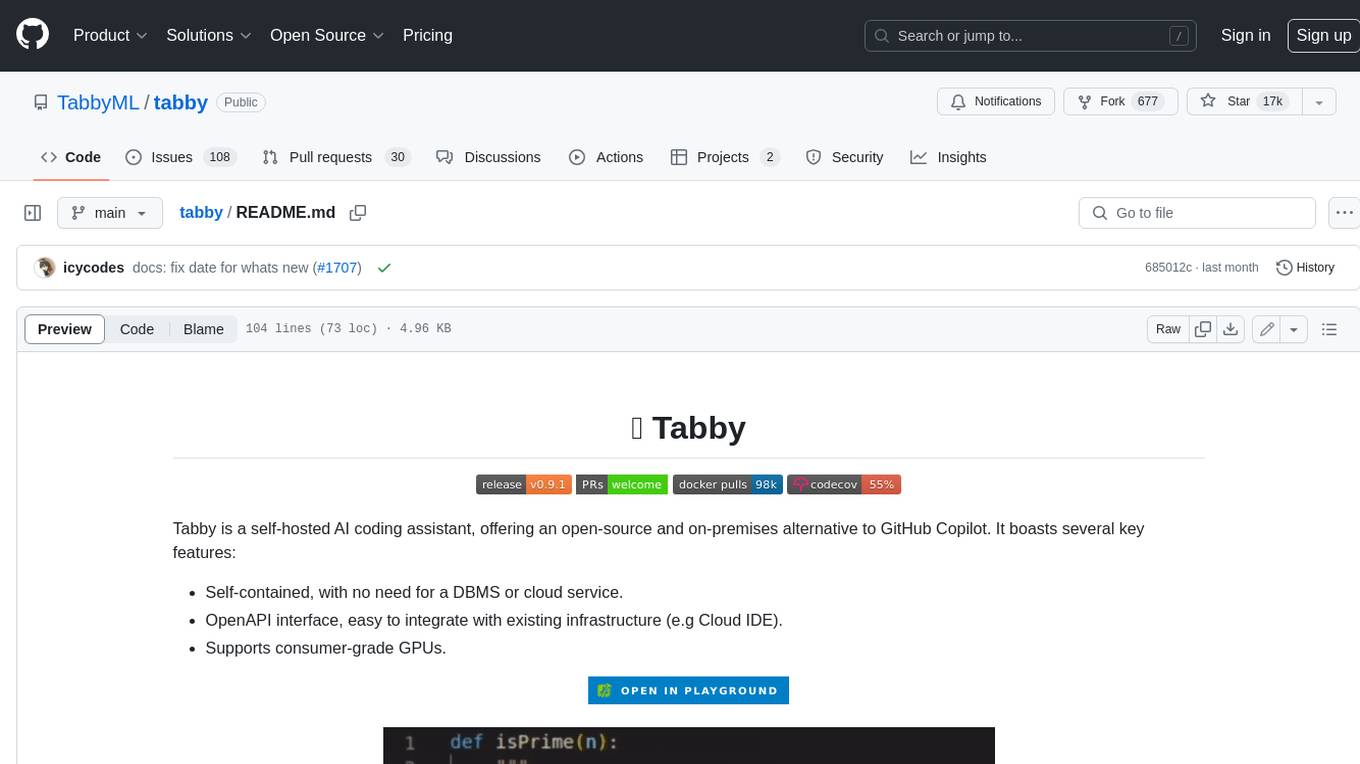
tabby
Tabby is a self-hosted AI coding assistant, offering an open-source and on-premises alternative to GitHub Copilot. It boasts several key features: * Self-contained, with no need for a DBMS or cloud service. * OpenAPI interface, easy to integrate with existing infrastructure (e.g Cloud IDE). * Supports consumer-grade GPUs.
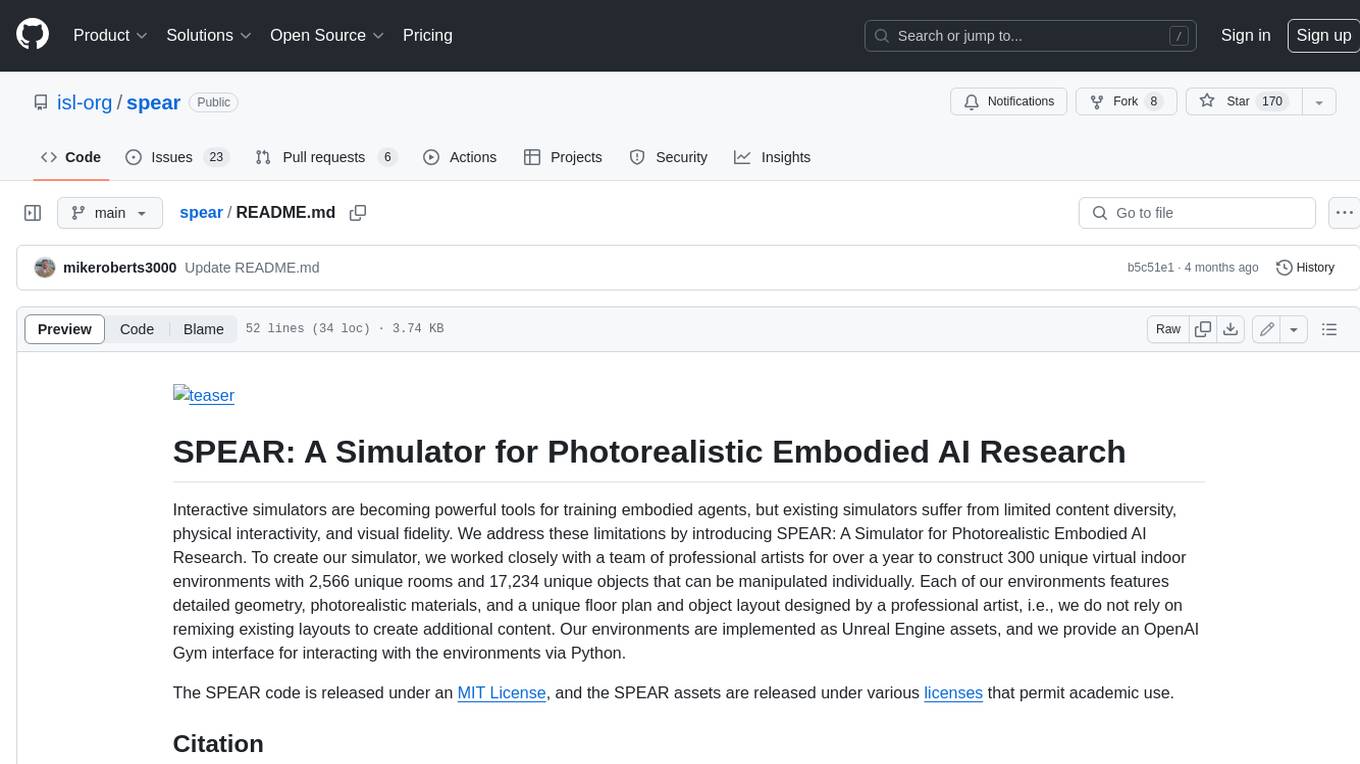
spear
SPEAR (Simulator for Photorealistic Embodied AI Research) is a powerful tool for training embodied agents. It features 300 unique virtual indoor environments with 2,566 unique rooms and 17,234 unique objects that can be manipulated individually. Each environment is designed by a professional artist and features detailed geometry, photorealistic materials, and a unique floor plan and object layout. SPEAR is implemented as Unreal Engine assets and provides an OpenAI Gym interface for interacting with the environments via Python.
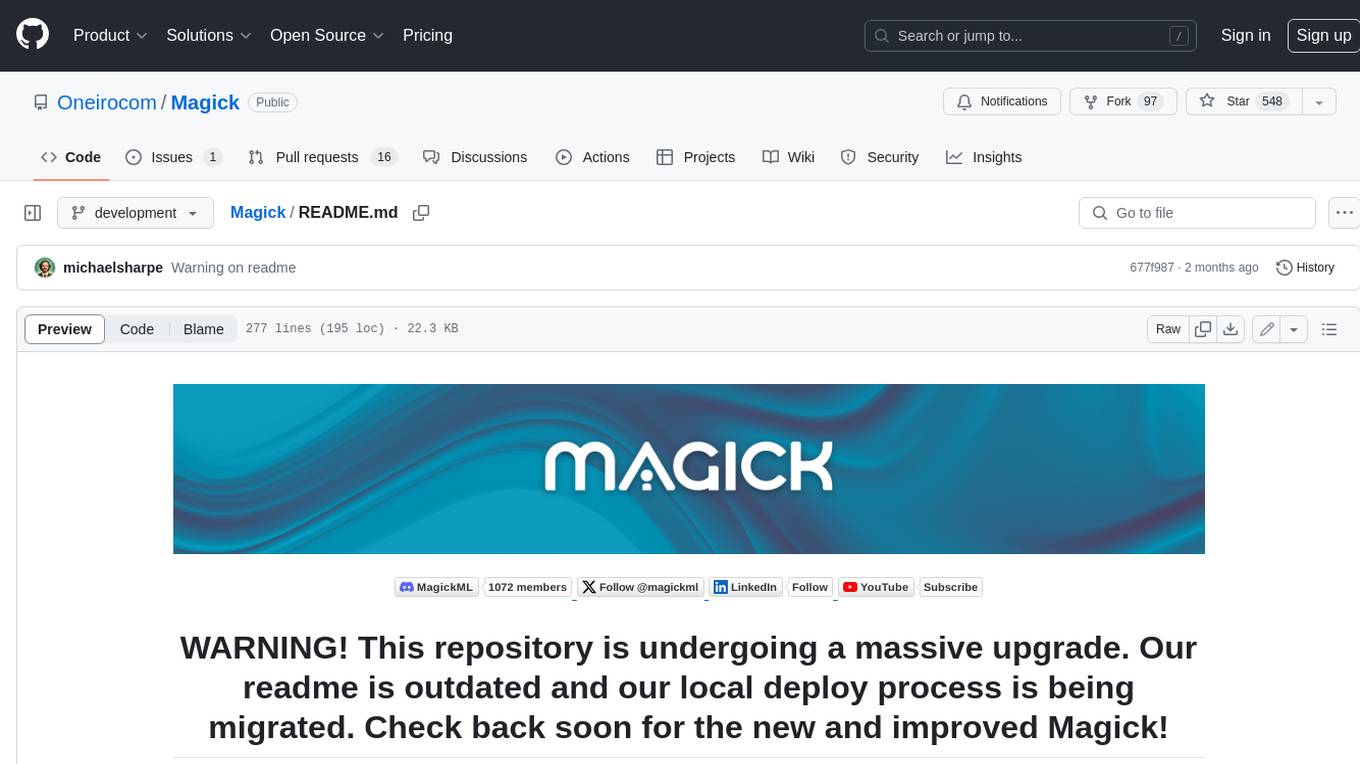
Magick
Magick is a groundbreaking visual AIDE (Artificial Intelligence Development Environment) for no-code data pipelines and multimodal agents. Magick can connect to other services and comes with nodes and templates well-suited for intelligent agents, chatbots, complex reasoning systems and realistic characters.