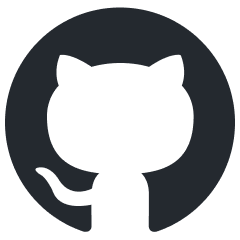
tau
Open source distributed Platform as a Service (PaaS). A self-hosted Vercel / Netlify / Cloudflare alternative.
Stars: 3815
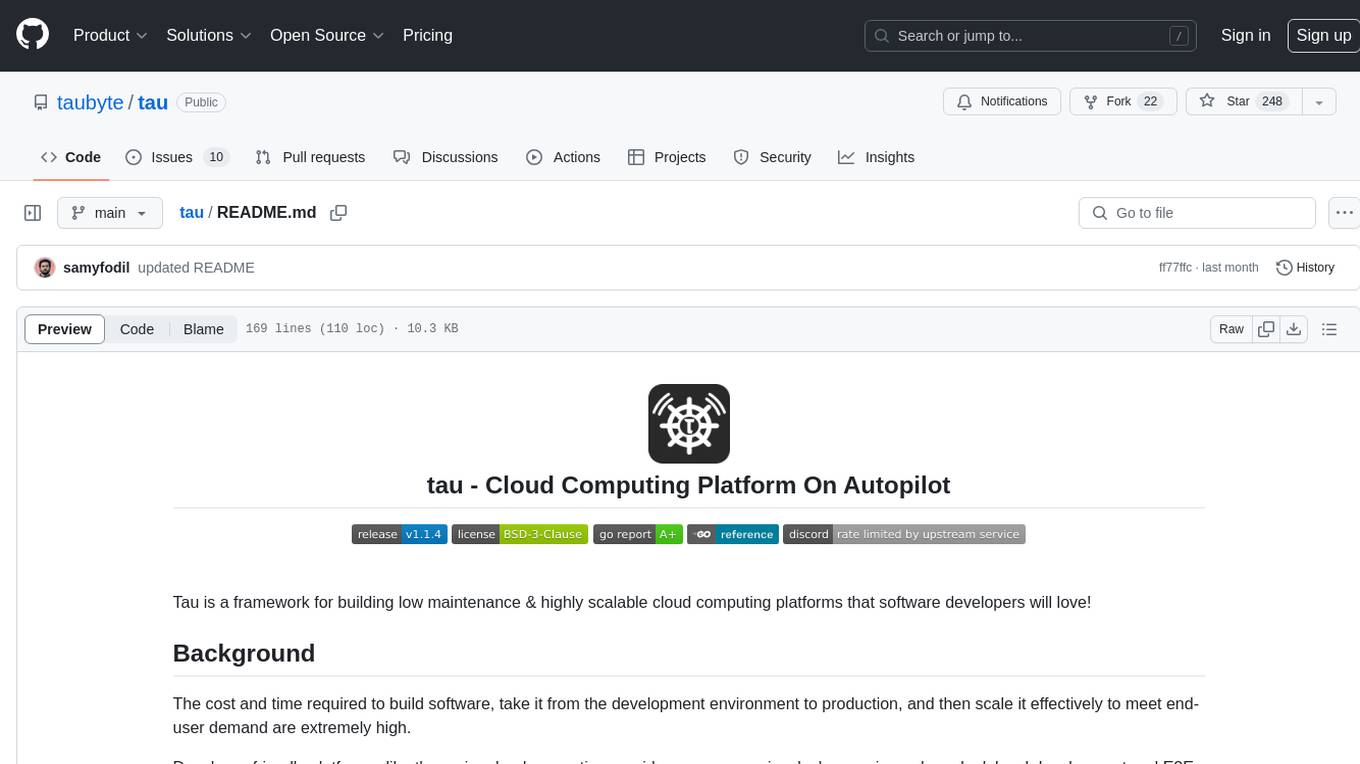
Tau is a framework for building low maintenance & highly scalable cloud computing platforms that software developers will love. It aims to solve the high cost and time required to build, deploy, and scale software by providing a developer-friendly platform that offers autonomy and flexibility. Tau simplifies the process of building and maintaining a cloud computing platform, enabling developers to achieve 'Local Coding Equals Global Production' effortlessly. With features like auto-discovery, content-addressing, and support for WebAssembly, Tau empowers users to create serverless computing environments, host frontends, manage databases, and more. The platform also supports E2E testing and can be extended using a plugin system called orbit.
README:
Explore the docs »
Quick Start Guide
·
Try our Sandbox Cloud
·
Join Our Discord
Tau is a framework for building low maintenance & highly scalable cloud computing platforms that software developers will love!
tau
is a single binary with no external dependencies except standard system libraries. On top of that, it requires minimal configuration. These are the main steps:
-
Install Tau
curl https://get.tau.link/tau | sh
-
Configure
tau config generate -n yourdomain.com -s compute --services all --ip your_public_ip --dv --swarm
-
Launch
tau start -s compute
For a complete step-by-step guide, refer to Deploy tau.
Building tau
youself is a straightforward go build
given you have Go installed.
The cost and time required to build software, take it from the development environment to production, and then scale it effectively to meet end-user demand are extremely high.
Developer-friendly platforms, like the major cloud computing providers, are expensive, lock users in, and overlook local development and E2E testing.
This is really a two-sided problem. Do you save on infrastructure cost, or do you lower development time?
If you invest in your own platform, it's a rocky road that impedes the speed of development and generally ends up costing more. We all know the Kubernetes fairy tale does not end well!
If you invest in development speed, you're limited by your provider's features and cost.
To us, solving this problem means:
- Giving you, or your very small team, the ability to build and maintain a cloud computing platform that will go head-to-head with the ones backed by thousands of engineers.
- Setting software developers free from infrastructure and operational constraints. We refer to this as "Local Coding Equals Global Production."
tau
solves for building and maintaining a cloud computing platform, and also provides the foundations for an amazing developer experience.
One of the reasons tau
requires minimal configuration is because it has built-in auto-discovery.
Just like a self-driving car gathering information through sensors, tau
will gather information and try to find the best ways to be reachable, available, etc.
That said, some configuration like bootstrap peers is necessary. Unless you're running a single-node cloud, each node will need to know at least one other peer.
A Cloud built with tau
is very dynamic; at a low level, nodes communicate assets, routes, and services, and they also exchange information about other peers. Enriched by distributed services like seer
and gateway
, the cloud can load-balance incoming requests to ensure optimal performance and reliability.
This behavior is built into cloud resources as well. For example, a service we call hoarder
ensures object storages and databases are replicated; all you need to do is enable it on a few nodes.
In your traditional setup, the platform is a complex set of templates, pipelines, and integrations that ultimately help turn configuration into API calls and code into assets. Because of that complexity, and also the fact that many components need to run inside a very complex environment of their own, it's impossible to satisfy the 'local == production' equation.
Granted, there are some solutions that either mock or reroute to dev/prod resources, enabling developers to build or debug locally. However, it's still a 3rd party service you need to integrate and manage.
In order to satisfy the equation, we decided to build tau
so it simplifies, ports, and/or sandboxes every aspect of the cloud.
Traditionally, you interface with infrastructure through API calls. This is the case for every cloud computing provider alongside orchestration solutions like Kubernetes.
A few years back, the concept of GitOps started to make waves, and that was around the time we started building, so we decided to cut the unnecessary garbage between the definition of a cloud resource, which should be stored in Git, and its instantiation.
As a result, tau
has no API calls to create a serverless function, for example. Instead, it adopts Git as the only way to alter infrastructure.
Also, git being core to tau
means that nodes in the cloud do tune to a specific branch, by default main or master. Among what it enables is an easy way to set up development environments, for example.
A specific use case is local development in which case dream-cli nodes can also be tuned to the current branch.
In addition to the nodes being on a branch, the application registry, managed by the 'tns' service, uses commit ids to version entries, allowing nodes serving the assets to detect new versions, or a roll-back for that matter.
Internally, tau
, using libp2p, builds an overlay peer-to-peer network between the nodes, enabling some pretty cool features like:
- Automatic node and service discovery & routing. If, for example, a node is down, changes its IP address/port, or the services it supports, other nodes will update the info automatically.
- Transport independent. Nodes can use any combination of TCP/IP, WebSocket, QUIC, and more.
- NAT Traversal & Circuit Relay, which allow nodes that are not public to be part of the cloud.
Unless absolutely required, which is extremely rare, no well-designed software should rely on IP addresses and ports. This is why every tau
cloud is identified with an FQDN (i.e., enterprise.starships.ws) so no absolute network reference is used in an application. Under the hood, the Cloud will transparently take care of DNS resolution and HTTP load balancing, eliminating the need to set these up.
In every other cloud computing implementation, storage means a location and generally a path. For example, https://tau.how/assets/logo-w.svg
has two main components tau.how
, which translates to an IP address and a location, and /assets/logo-w.svg
, which is a path relative to the location. This way of addressing, called "location-based addressing," is simply not portable. Why? you might ask. Well, for starters, nothing guarantees the data returned is an SVG logo in this case. The other issue is the tau.how
host we connected to might not have it.
To solve this issue, tau
uses content-addressing, a concept introduced by torrent networks and popularized by IPFS.
So when you request https://tau.how/assets/logo-w.svg
, which is actually hosted by a tau
Cloud, the host that handles the request will resolve (host=tau.how, path=/assets/logo-w.svg
) to a content address, or CID, then retrieve the content reader and then forward it through an HTTP writer to you.
A few cool facts about this approach:
- Content is chunked and then stored in a DAG, which means it's deduplicated.
- Content can be downloaded from multiple peers in parallel.
- Content can be verified as the CID is its hash.
- When content is in demand, the cloud automatically dedicates more peers to its distribution.
As of today, tau
supports WebAssembly for computing. The reason we started with it is that it's highly portable and sandboxed. We support containers for CI/CD but not for computing yet. We're working on a way to implement containers and virtual machines while abiding by our principles of portability and sandboxing.
Code, binary, images, along with any attached assets, are stored and retrieved using the same principles described in Storage, which considerably reduces provisioning time and brings computing close to data (data gravity) and/or user (edge computing).
If you're looking to create E2E tests for projects hosted on tau
, you can use dream
, a sub-package within tau
. We don't have documentation for it yet, but you can quickly learn from tests like services/seer/tests/dns_test.go.
While you can't practically run tau
on your local machine, you can do so using dream-cli, which is a CLI wrapper around dream
. It creates local cloud environments mirroring production settings. Unlike tau
, it offers an API for real-time configuration and testing.
tau
can be extended using a plugin system we call orbit. An open-source example is ollama-cloud, which demonstrates how to add LLM capabilities to your cloud.
To learn more, check:
For comprehensive documentation, visit our documentation.
Questions or need assistance? Ping us on Discord!
For Tasks:
Click tags to check more tools for each tasksFor Jobs:
Alternative AI tools for tau
Similar Open Source Tools
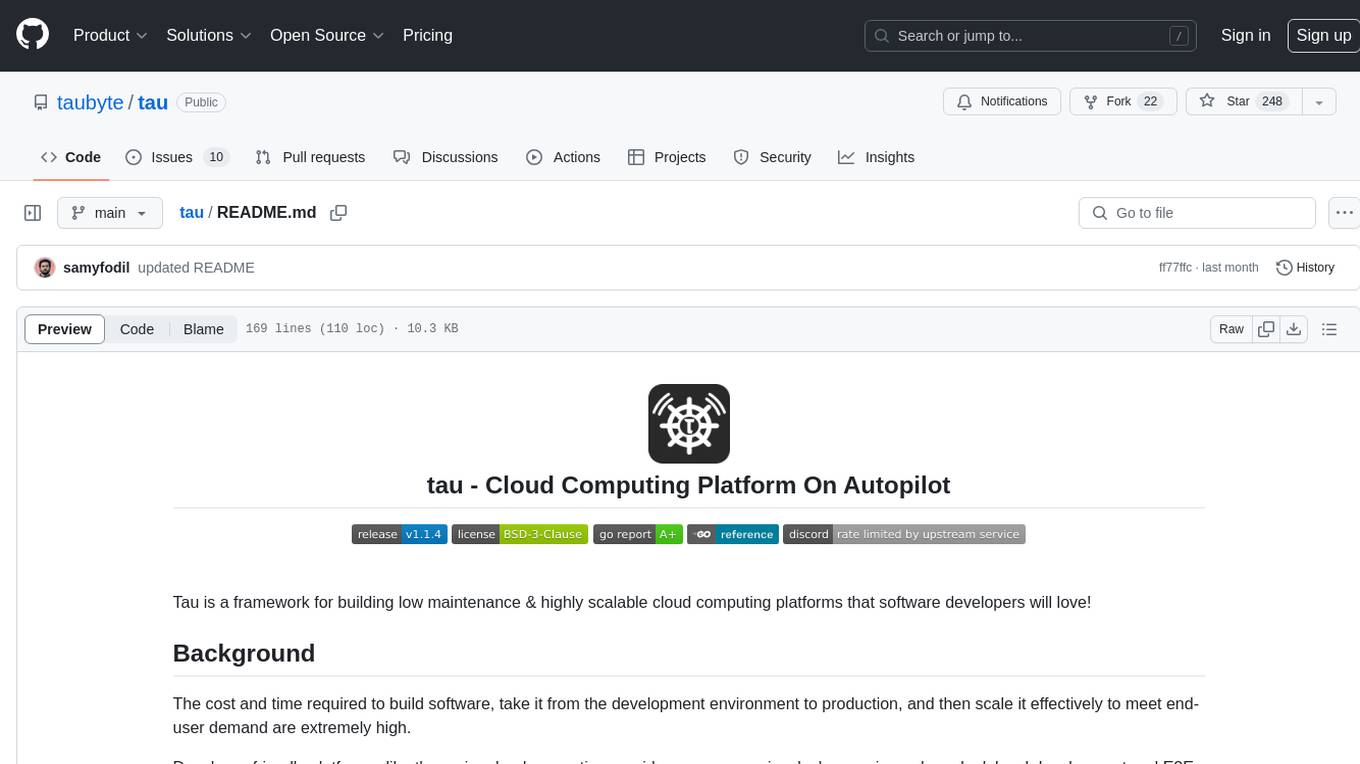
tau
Tau is a framework for building low maintenance & highly scalable cloud computing platforms that software developers will love. It aims to solve the high cost and time required to build, deploy, and scale software by providing a developer-friendly platform that offers autonomy and flexibility. Tau simplifies the process of building and maintaining a cloud computing platform, enabling developers to achieve 'Local Coding Equals Global Production' effortlessly. With features like auto-discovery, content-addressing, and support for WebAssembly, Tau empowers users to create serverless computing environments, host frontends, manage databases, and more. The platform also supports E2E testing and can be extended using a plugin system called orbit.
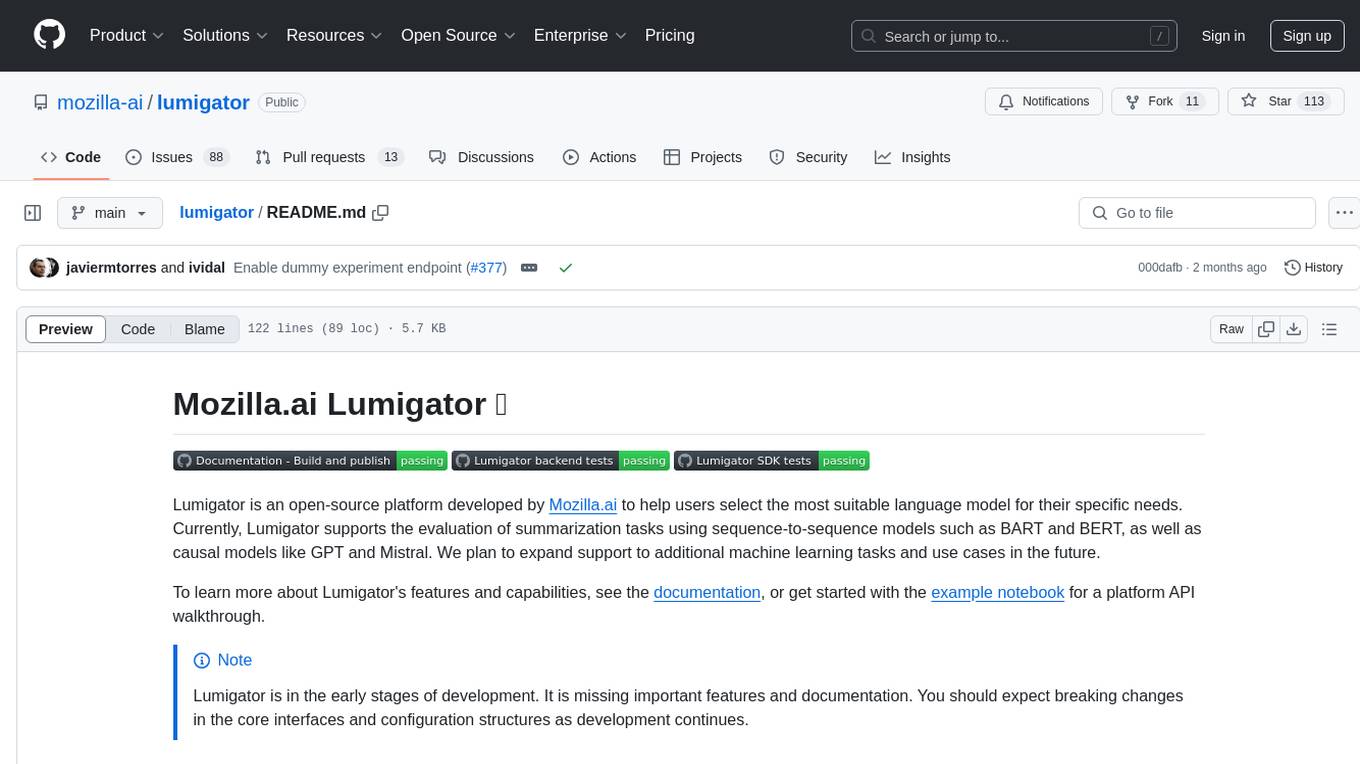
lumigator
Lumigator is an open-source platform developed by Mozilla.ai to help users select the most suitable language model for their specific needs. It supports the evaluation of summarization tasks using sequence-to-sequence models such as BART and BERT, as well as causal models like GPT and Mistral. The platform aims to make model selection transparent, efficient, and empowering by providing a framework for comparing LLMs using task-specific metrics to evaluate how well a model fits a project's needs. Lumigator is in the early stages of development and plans to expand support to additional machine learning tasks and use cases in the future.
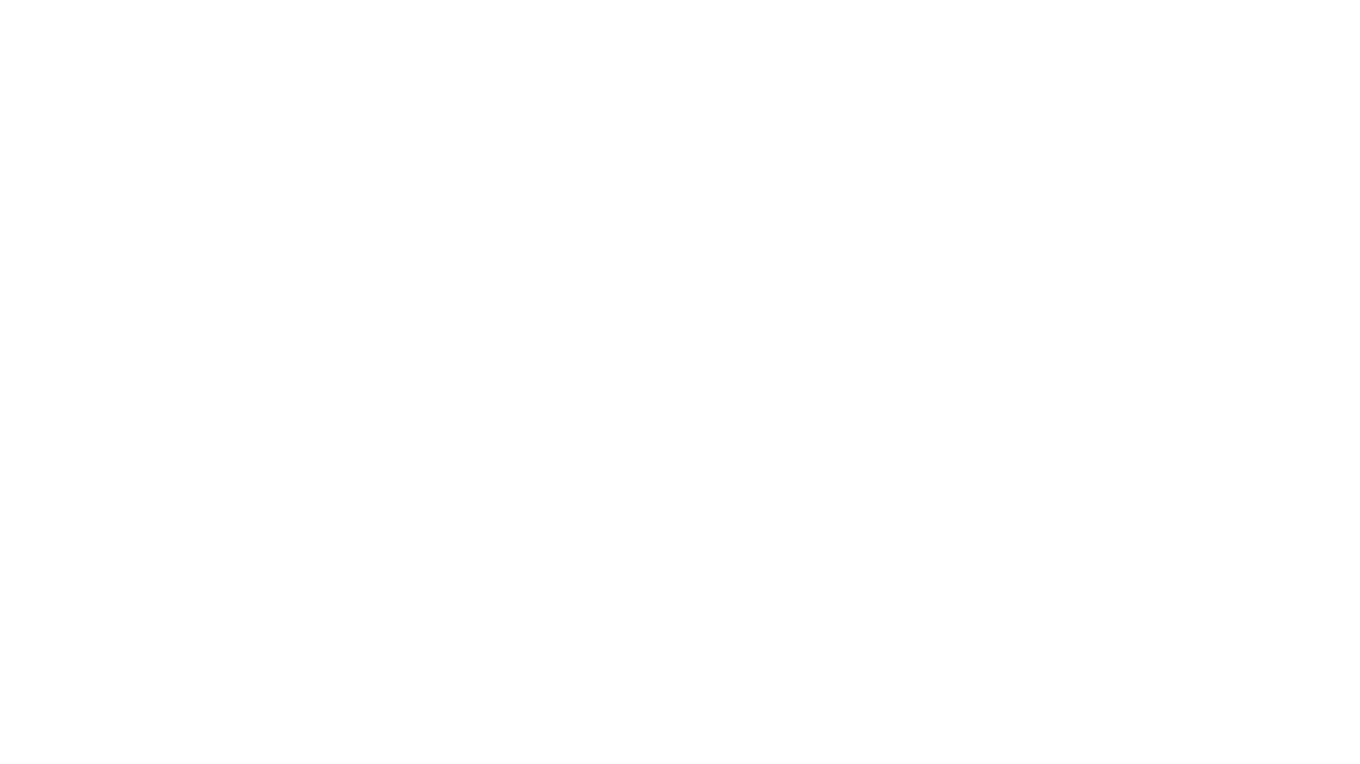
GlaDOS
This project aims to create a real-life version of GLaDOS, an aware, interactive, and embodied AI entity. It involves training a voice generator, developing a 'Personality Core,' implementing a memory system, providing vision capabilities, creating 3D-printable parts, and designing an animatronics system. The software architecture focuses on low-latency voice interactions, utilizing a circular buffer for data recording, text streaming for quick transcription, and a text-to-speech system. The project also emphasizes minimal dependencies for running on constrained hardware. The hardware system includes servo- and stepper-motors, 3D-printable parts for GLaDOS's body, animations for expression, and a vision system for tracking and interaction. Installation instructions cover setting up the TTS engine, required Python packages, compiling llama.cpp, installing an inference backend, and voice recognition setup. GLaDOS can be run using 'python glados.py' and tested using 'demo.ipynb'.
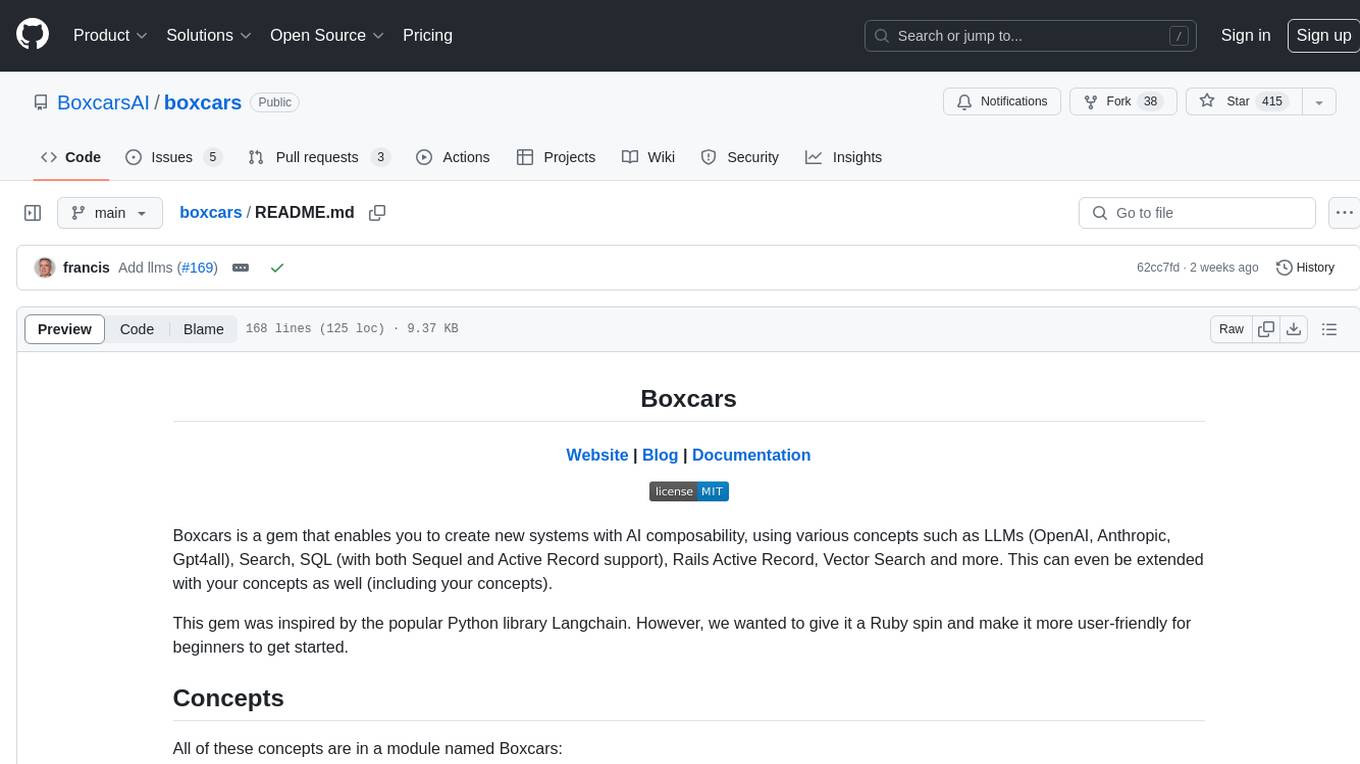
boxcars
Boxcars is a Ruby gem that enables users to create new systems with AI composability, incorporating concepts such as LLMs, Search, SQL, Rails Active Record, Vector Search, and more. It allows users to work with Boxcars, Trains, Prompts, Engines, and VectorStores to solve problems and generate text results. The gem is designed to be user-friendly for beginners and can be extended with custom concepts. Boxcars is actively seeking ways to enhance security measures to prevent malicious actions. Users can use Boxcars for tasks like running calculations, performing searches, generating Ruby code for math operations, and interacting with APIs like OpenAI, Anthropic, and Google SERP.
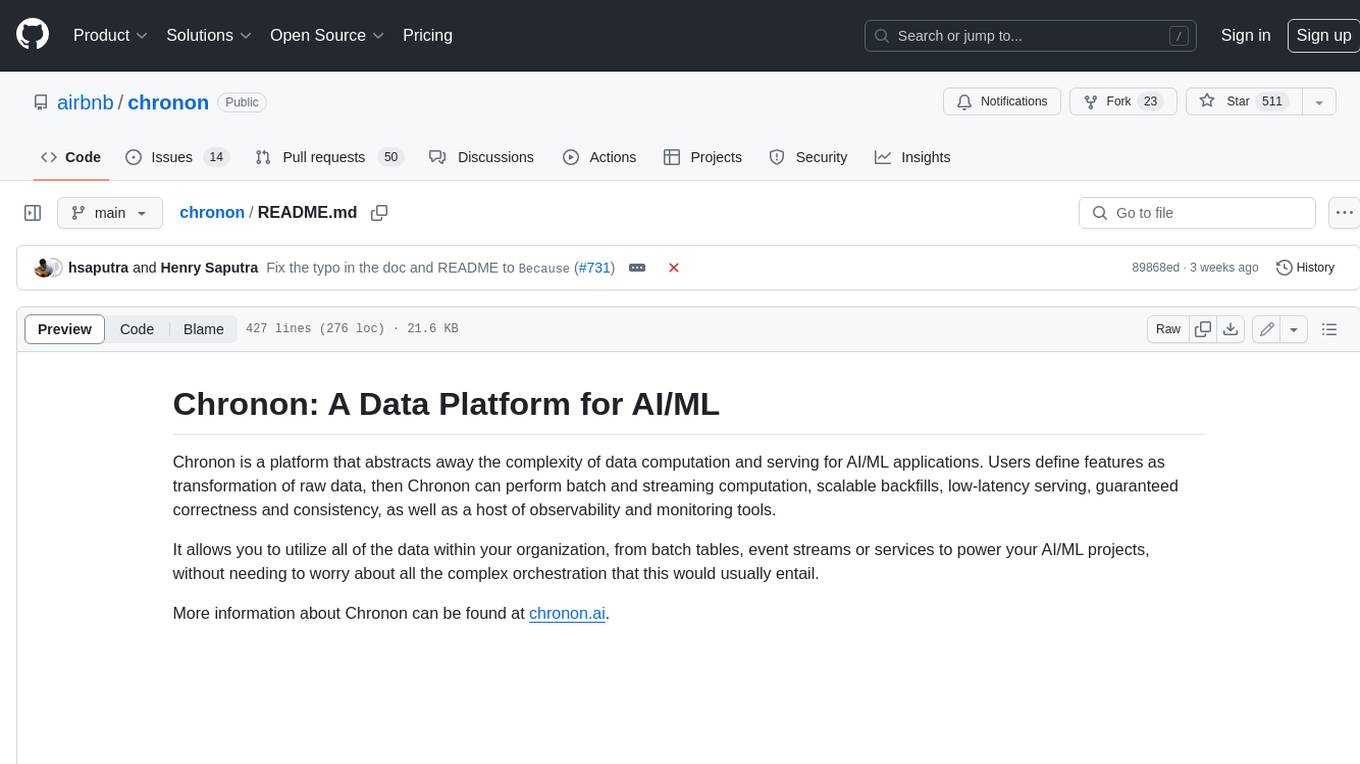
chronon
Chronon is a platform that simplifies and improves ML workflows by providing a central place to define features, ensuring point-in-time correctness for backfills, simplifying orchestration for batch and streaming pipelines, offering easy endpoints for feature fetching, and guaranteeing and measuring consistency. It offers benefits over other approaches by enabling the use of a broad set of data for training, handling large aggregations and other computationally intensive transformations, and abstracting away the infrastructure complexity of data plumbing.
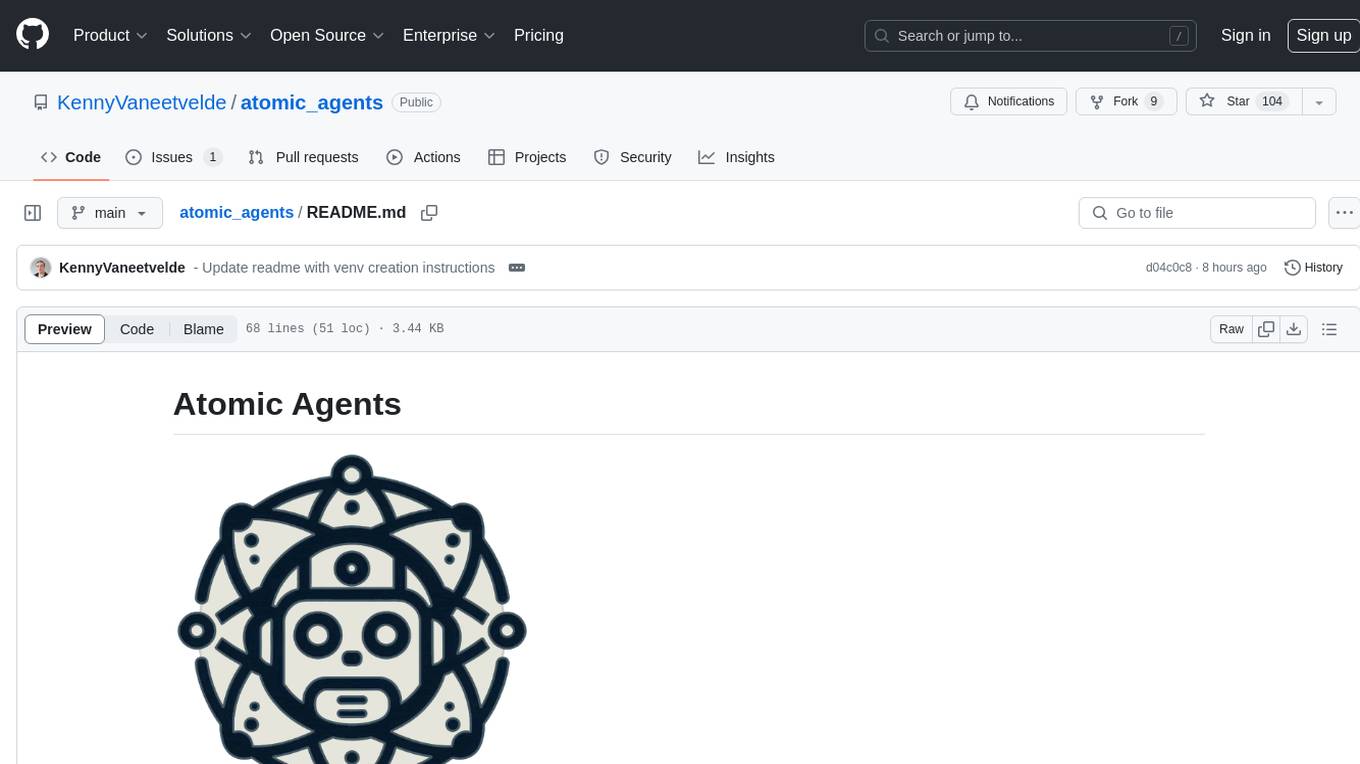
atomic_agents
Atomic Agents is a modular and extensible framework designed for creating powerful applications. It follows the principles of Atomic Design, emphasizing small and single-purpose components. Leveraging Pydantic for data validation and serialization, the framework offers a set of tools and agents that can be combined to build AI applications. It depends on the Instructor package and supports various APIs like OpenAI, Cohere, Anthropic, and Gemini. Atomic Agents is suitable for developers looking to create AI agents with a focus on modularity and flexibility.
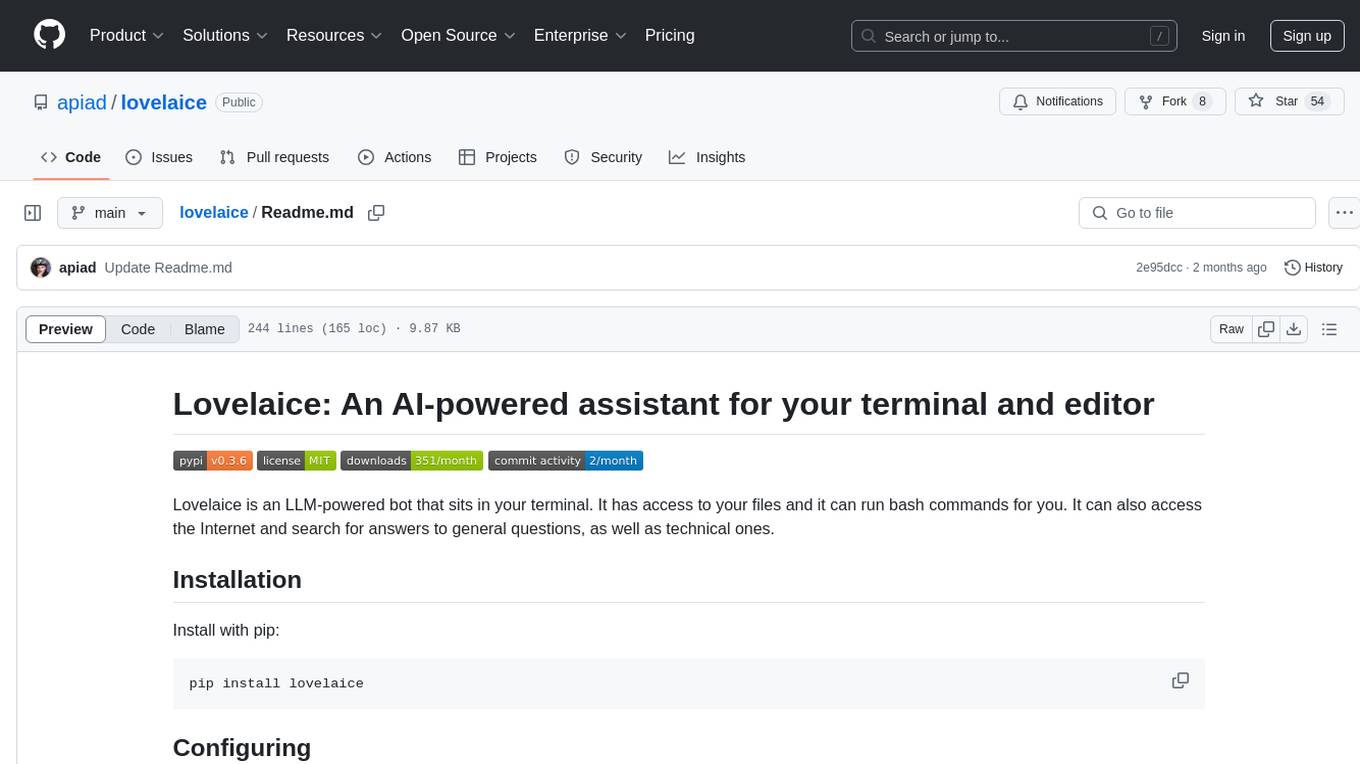
lovelaice
Lovelaice is an AI-powered assistant for your terminal and editor. It can run bash commands, search the Internet, answer general and technical questions, complete text files, chat casually, execute code in various languages, and more. Lovelaice is configurable with API keys and LLM models, and can be used for a wide range of tasks requiring bash commands or coding assistance. It is designed to be versatile, interactive, and helpful for daily tasks and projects.
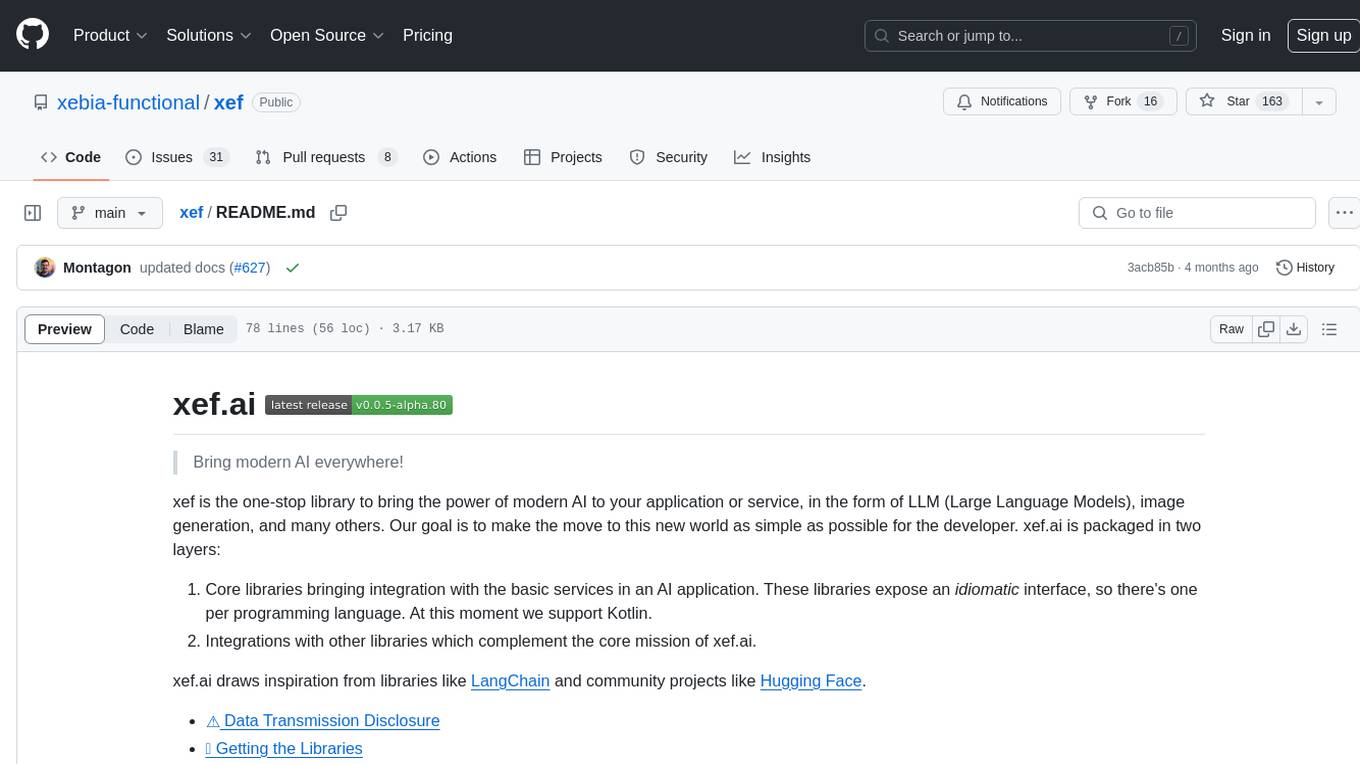
xef
xef.ai is a one-stop library designed to bring the power of modern AI to applications and services. It offers integration with Large Language Models (LLM), image generation, and other AI services. The library is packaged in two layers: core libraries for basic AI services integration and integrations with other libraries. xef.ai aims to simplify the transition to modern AI for developers by providing an idiomatic interface, currently supporting Kotlin. Inspired by LangChain and Hugging Face, xef.ai may transmit source code and user input data to third-party services, so users should review privacy policies and take precautions. Libraries are available in Maven Central under the `com.xebia` group, with `xef-core` as the core library. Developers can add these libraries to their projects and explore examples to understand usage.
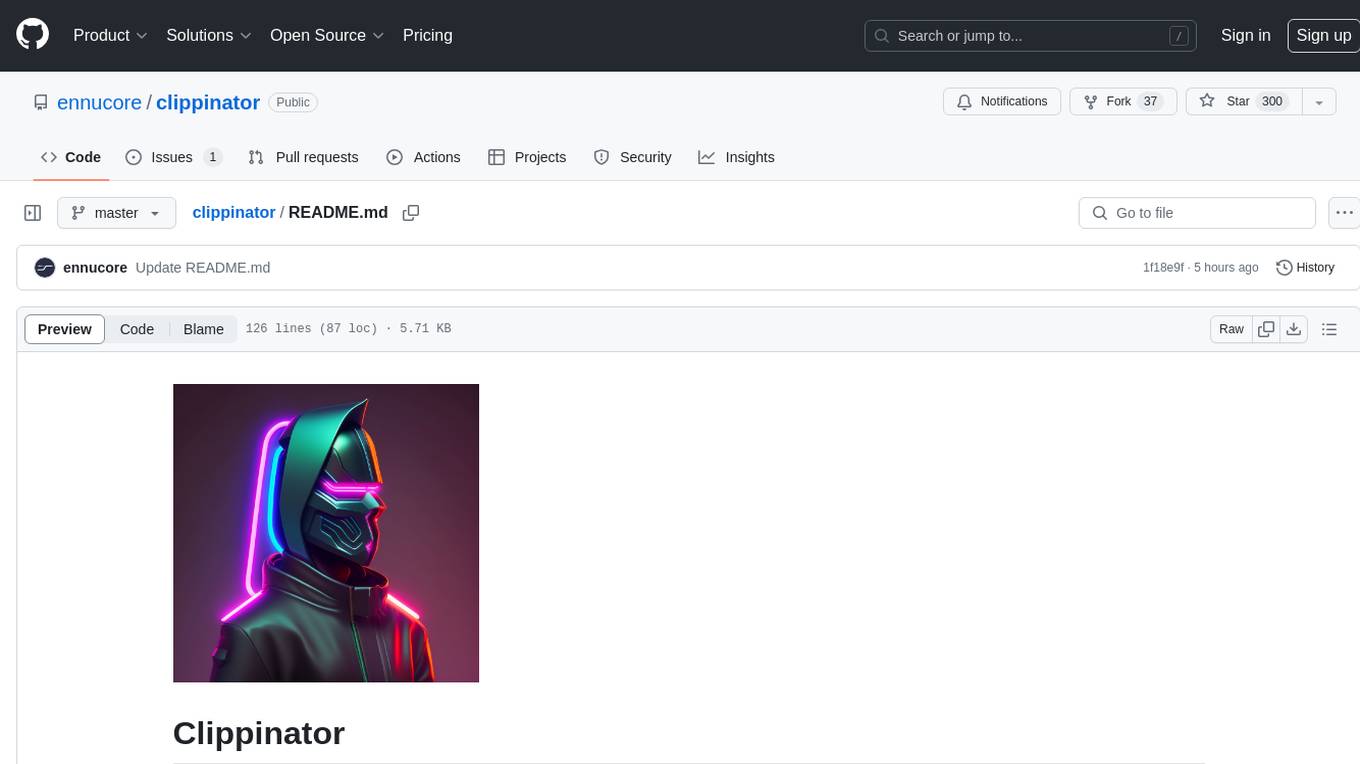
clippinator
Clippinator is a code assistant tool that helps users develop code autonomously by planning, writing, debugging, and testing projects. It consists of agents based on GPT-4 that work together to assist the user in coding tasks. The main agent, Taskmaster, delegates tasks to specialized subagents like Architect, Writer, Frontender, Editor, QA, and Devops. The tool provides project architecture, tools for file and terminal operations, browser automation with Selenium, linting capabilities, CI integration, and memory management. Users can interact with the tool to provide feedback and guide the coding process, making it a powerful tool when combined with human intervention.
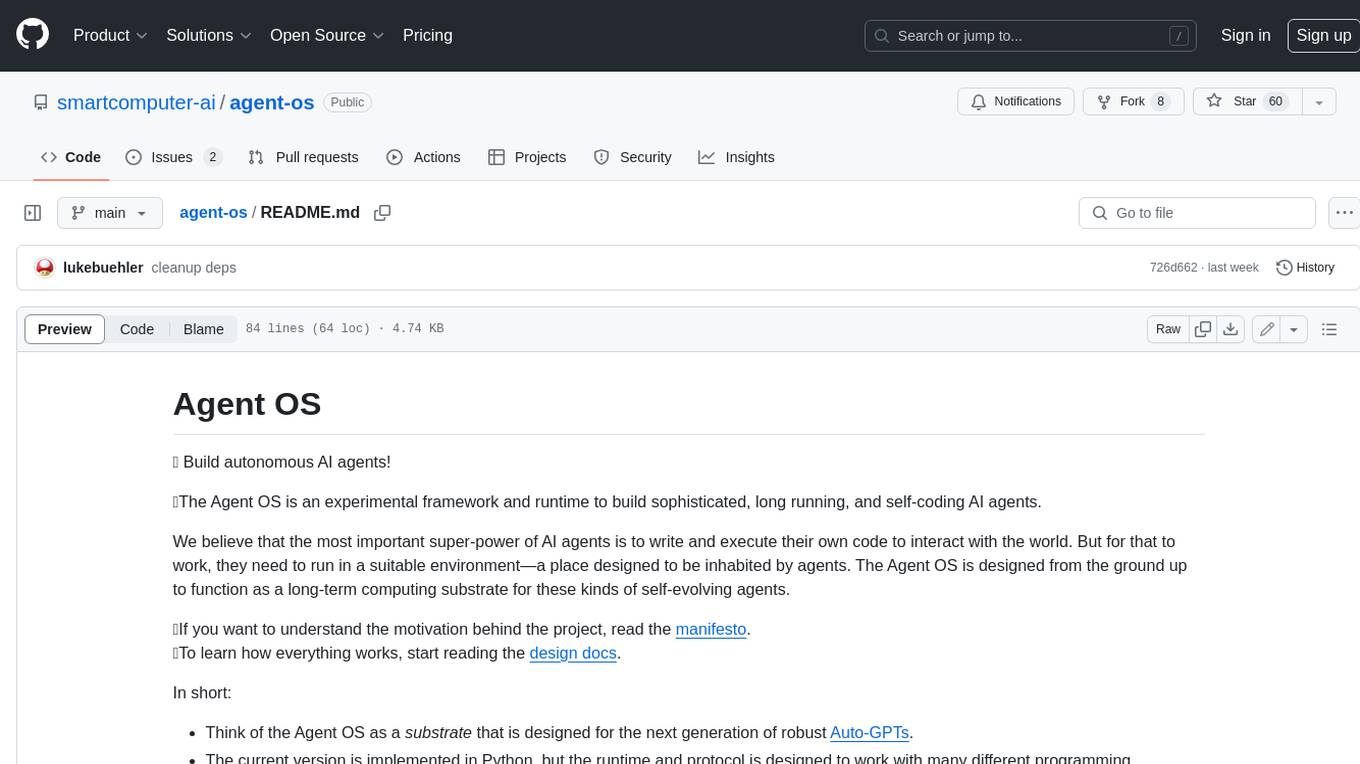
agent-os
The Agent OS is an experimental framework and runtime to build sophisticated, long running, and self-coding AI agents. We believe that the most important super-power of AI agents is to write and execute their own code to interact with the world. But for that to work, they need to run in a suitable environment—a place designed to be inhabited by agents. The Agent OS is designed from the ground up to function as a long-term computing substrate for these kinds of self-evolving agents.
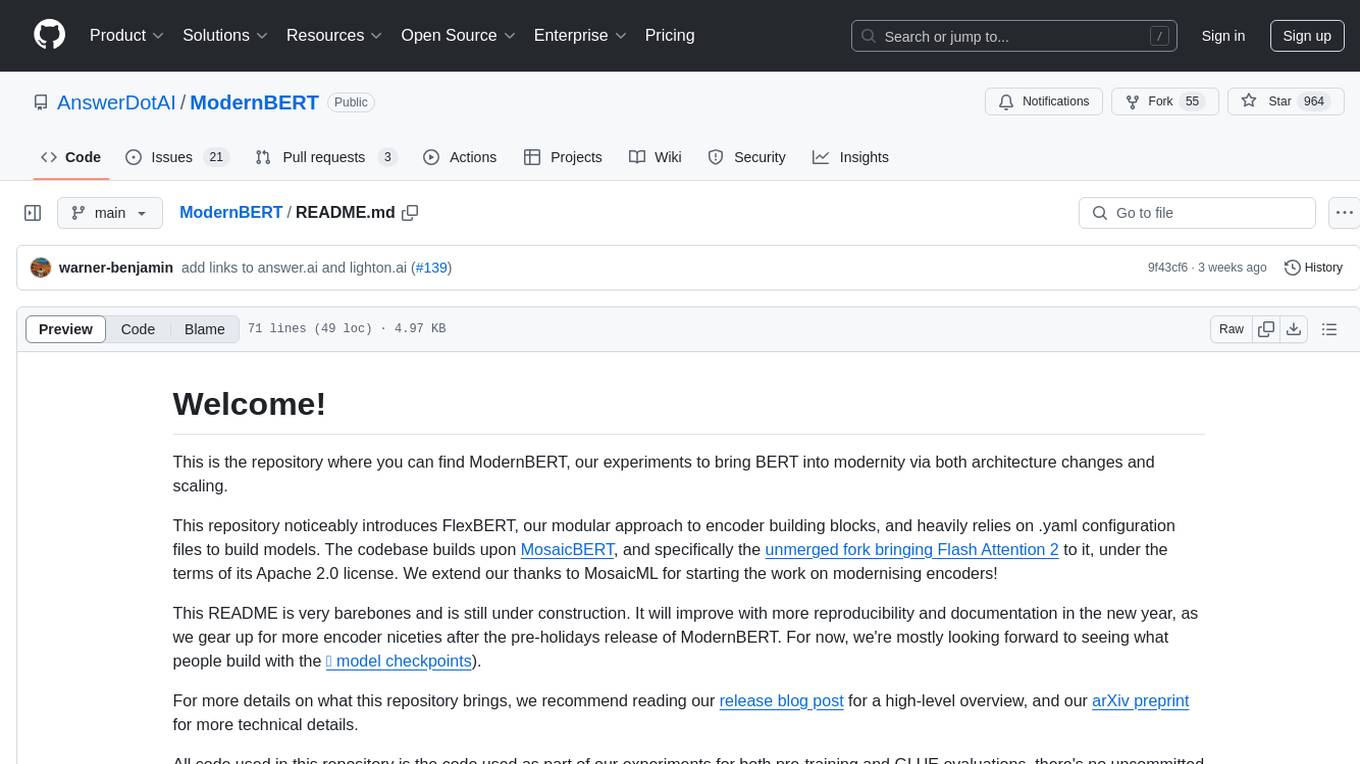
ModernBERT
ModernBERT is a repository focused on modernizing BERT through architecture changes and scaling. It introduces FlexBERT, a modular approach to encoder building blocks, and heavily relies on .yaml configuration files to build models. The codebase builds upon MosaicBERT and incorporates Flash Attention 2. The repository is used for pre-training and GLUE evaluations, with a focus on reproducibility and documentation. It provides a collaboration between Answer.AI, LightOn, and friends.
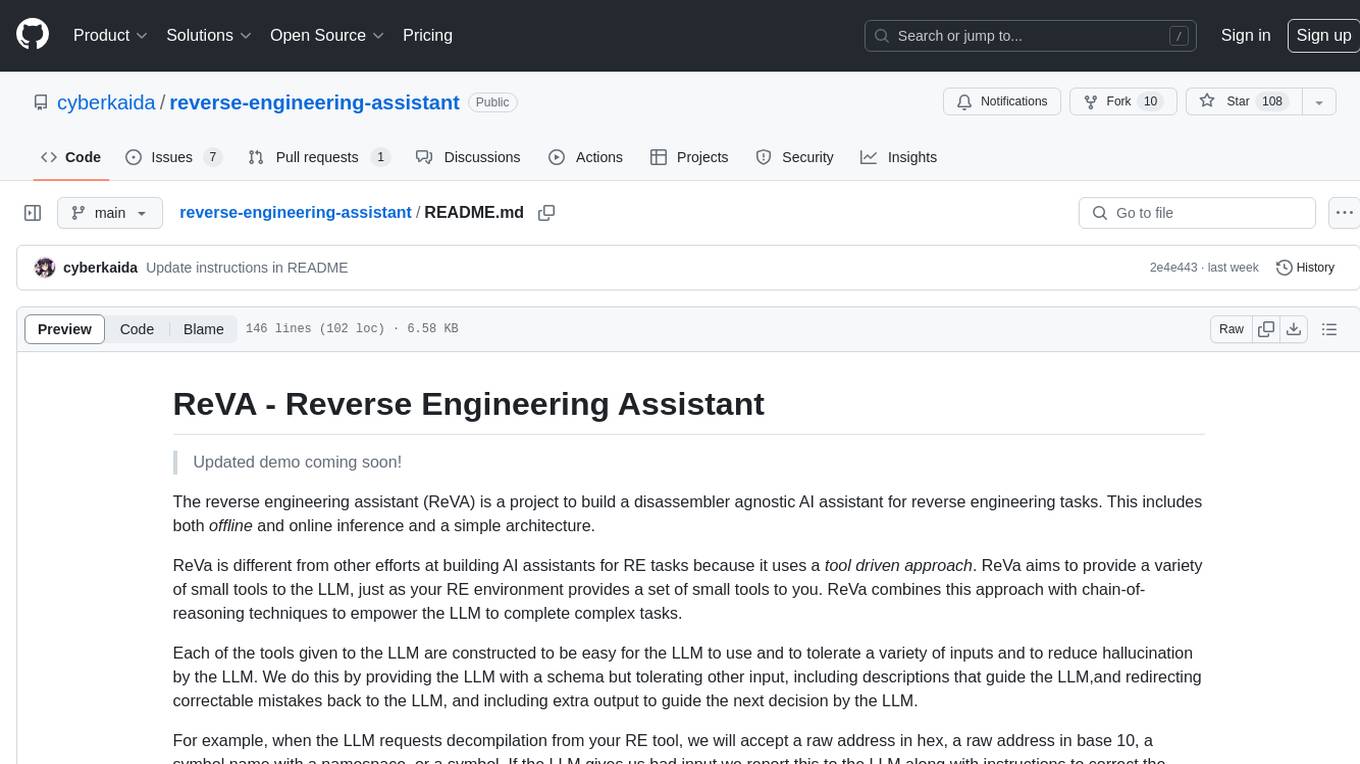
reverse-engineering-assistant
ReVA (Reverse Engineering Assistant) is a project aimed at building a disassembler agnostic AI assistant for reverse engineering tasks. It utilizes a tool-driven approach, providing small tools to the user to empower them in completing complex tasks. The assistant is designed to accept various inputs, guide the user in correcting mistakes, and provide additional context to encourage exploration. Users can ask questions, perform tasks like decompilation, class diagram generation, variable renaming, and more. ReVA supports different language models for online and local inference, with easy configuration options. The workflow involves opening the RE tool and program, then starting a chat session to interact with the assistant. Installation includes setting up the Python component, running the chat tool, and configuring the Ghidra extension for seamless integration. ReVA aims to enhance the reverse engineering process by breaking down actions into small parts, including the user's thoughts in the output, and providing support for monitoring and adjusting prompts.
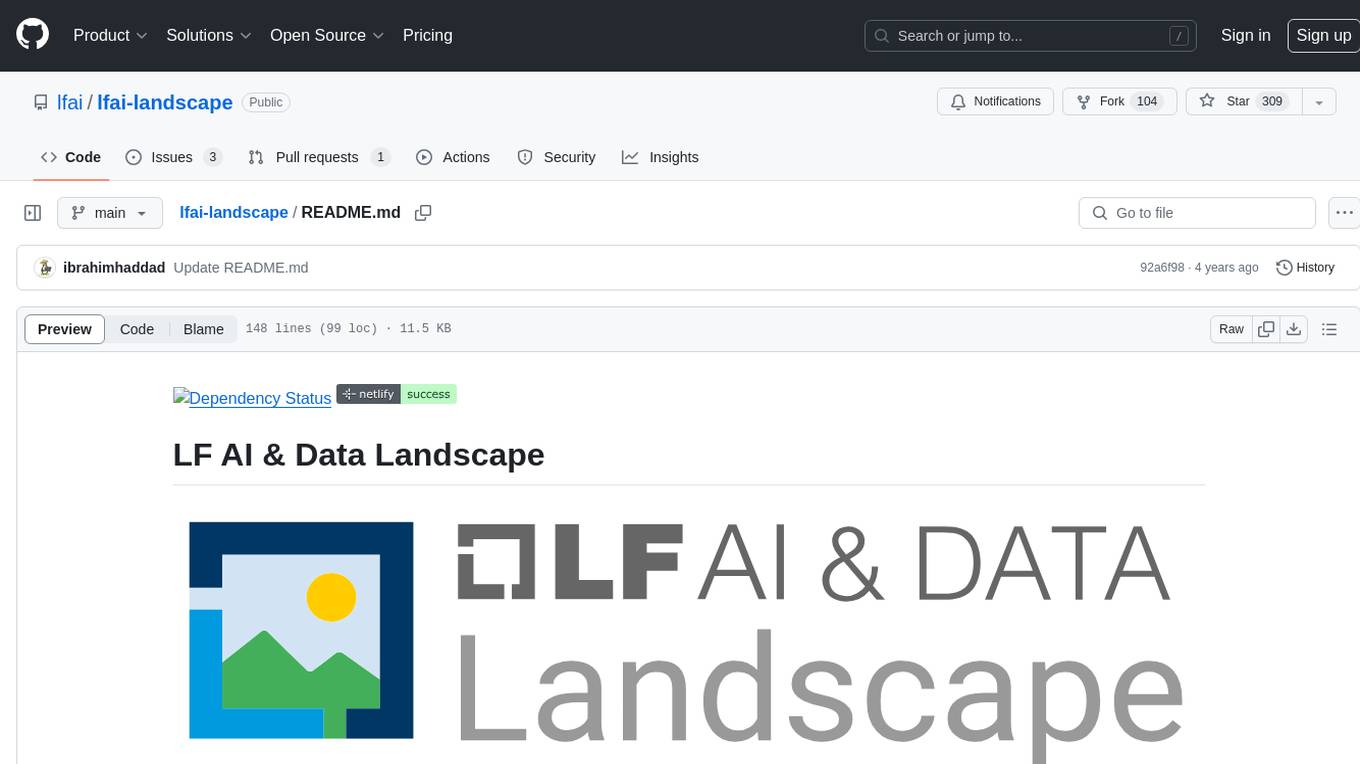
lfai-landscape
LF AI & Data Landscape is a map to explore open source projects in the AI & Data domains, highlighting companies that are members of LF AI & Data. It showcases members of the Foundation and is modelled after the Cloud Native Computing Foundation landscape. The landscape includes current version, interactive version, new entries, logos, proper SVGs, corrections, external data, best practices badge, non-updated items, license, formats, installation, vulnerability reporting, and adjusting the landscape view.
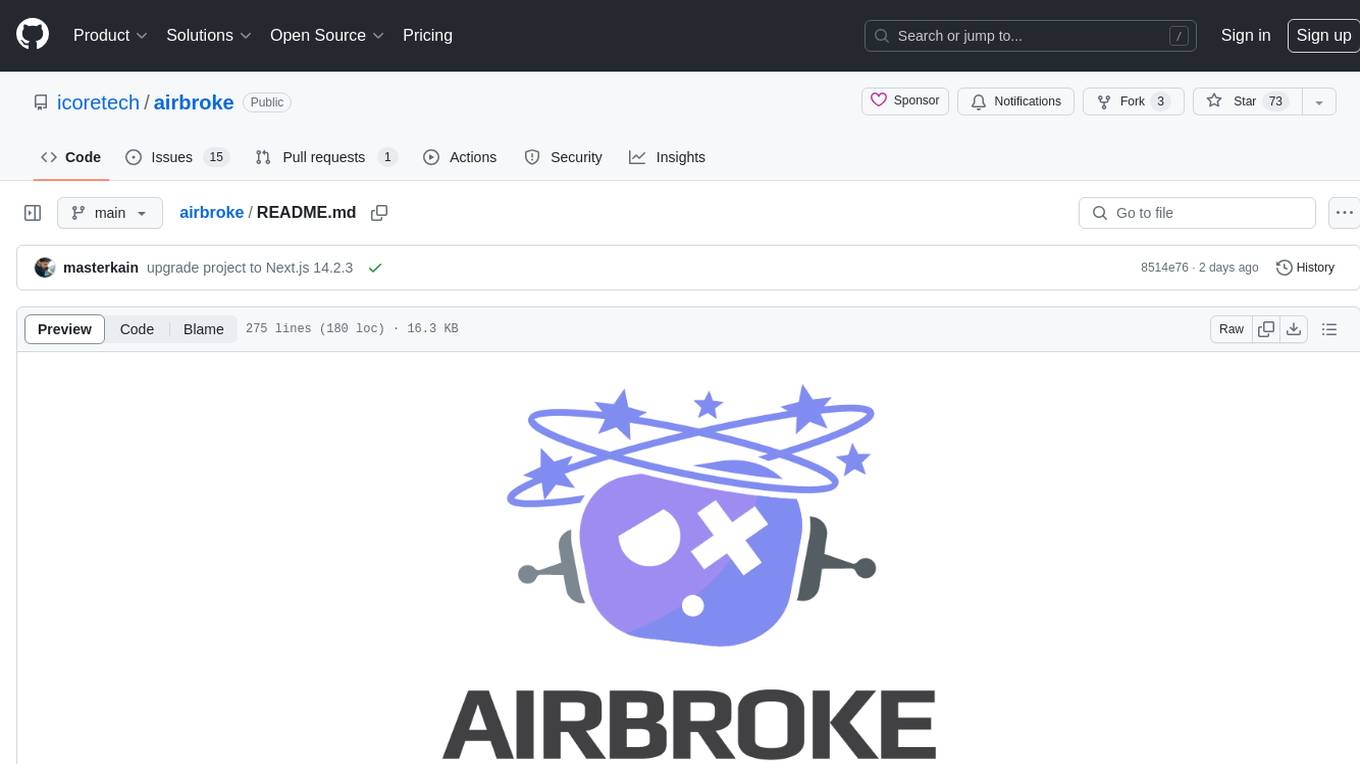
airbroke
Airbroke is an open-source error catcher tool designed for modern web applications. It provides a PostgreSQL-based backend with an Airbrake-compatible HTTP collector endpoint and a React-based frontend for error management. The tool focuses on simplicity, maintaining a small database footprint even under heavy data ingestion. Users can ask AI about issues, replay HTTP exceptions, and save/manage bookmarks for important occurrences. Airbroke supports multiple OAuth providers for secure user authentication and offers occurrence charts for better insights into error occurrences. The tool can be deployed in various ways, including building from source, using Docker images, deploying on Vercel, Render.com, Kubernetes with Helm, or Docker Compose. It requires Node.js, PostgreSQL, and specific system resources for deployment.
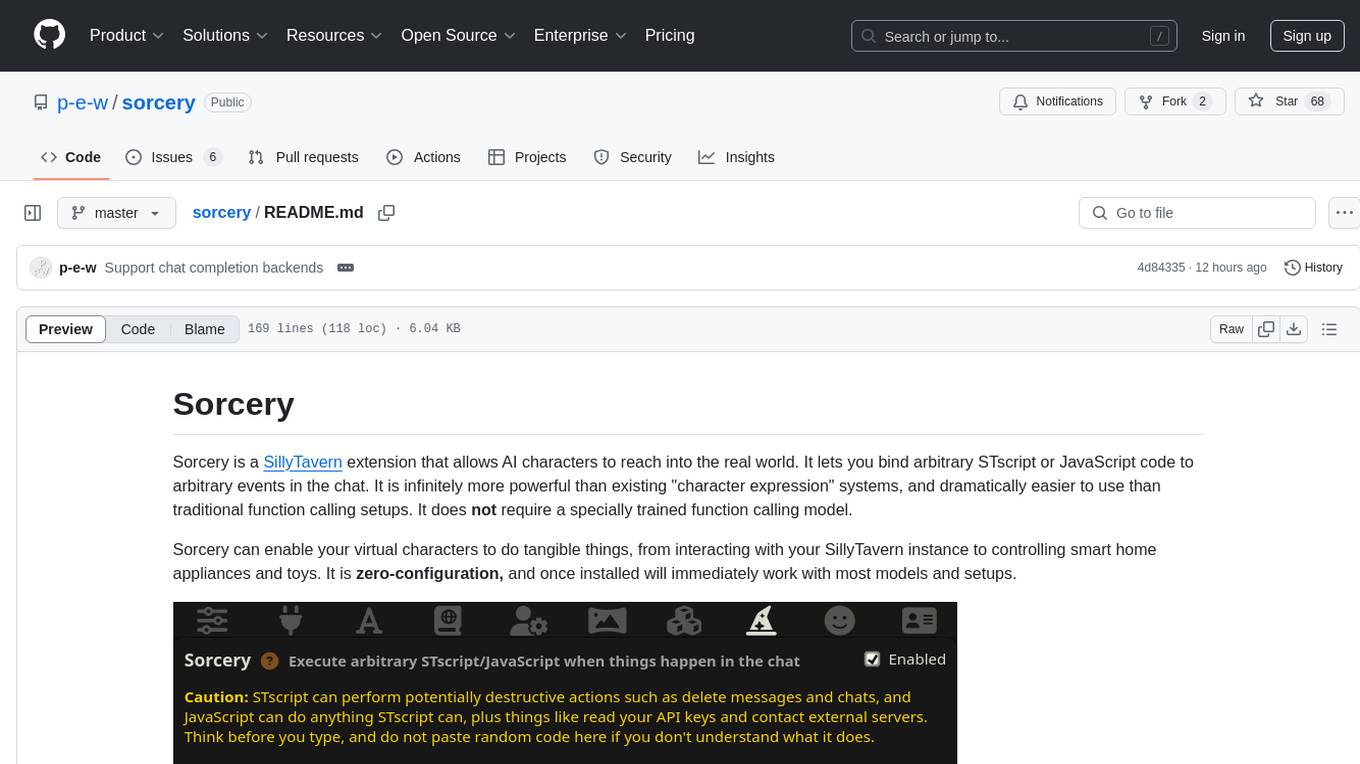
sorcery
Sorcery is a SillyTavern extension that allows AI characters to interact with the real world by executing user-defined scripts at specific events in the chat. It is easy to use and does not require a specially trained function calling model. Sorcery can be used to control smart home appliances, interact with virtual characters, and perform various tasks in the chat environment. It works by injecting instructions into the system prompt and intercepting markers to run associated scripts, providing a seamless user experience.
For similar tasks
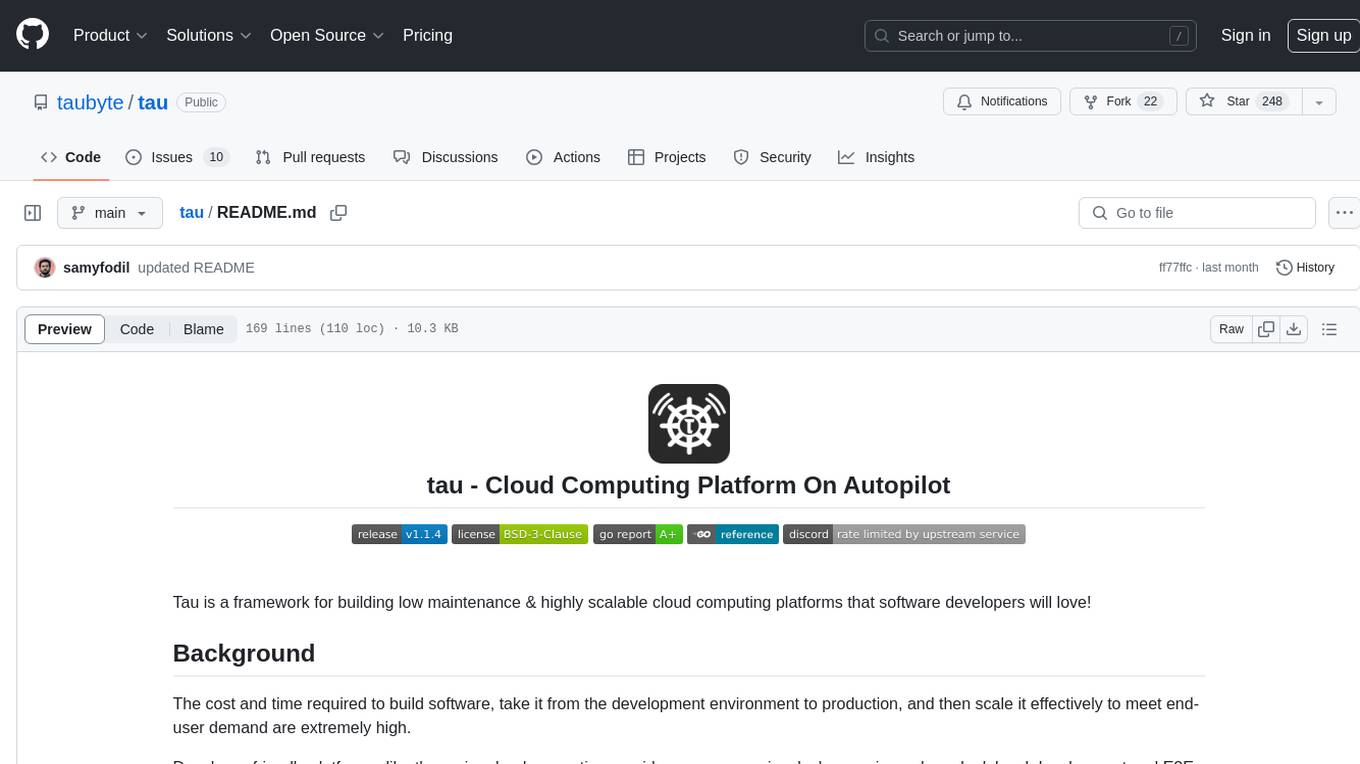
tau
Tau is a framework for building low maintenance & highly scalable cloud computing platforms that software developers will love. It aims to solve the high cost and time required to build, deploy, and scale software by providing a developer-friendly platform that offers autonomy and flexibility. Tau simplifies the process of building and maintaining a cloud computing platform, enabling developers to achieve 'Local Coding Equals Global Production' effortlessly. With features like auto-discovery, content-addressing, and support for WebAssembly, Tau empowers users to create serverless computing environments, host frontends, manage databases, and more. The platform also supports E2E testing and can be extended using a plugin system called orbit.
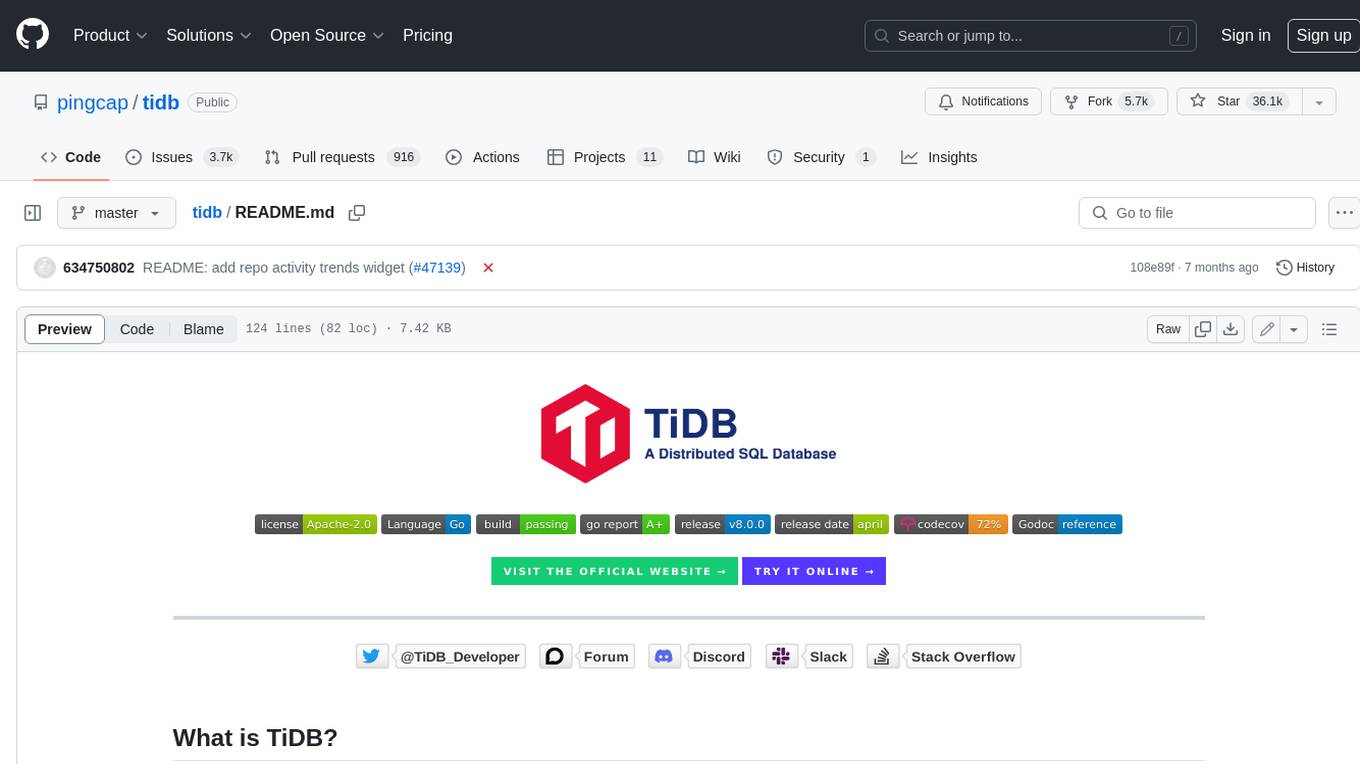
tidb
TiDB is an open-source distributed SQL database that supports Hybrid Transactional and Analytical Processing (HTAP) workloads. It is MySQL compatible and features horizontal scalability, strong consistency, and high availability.
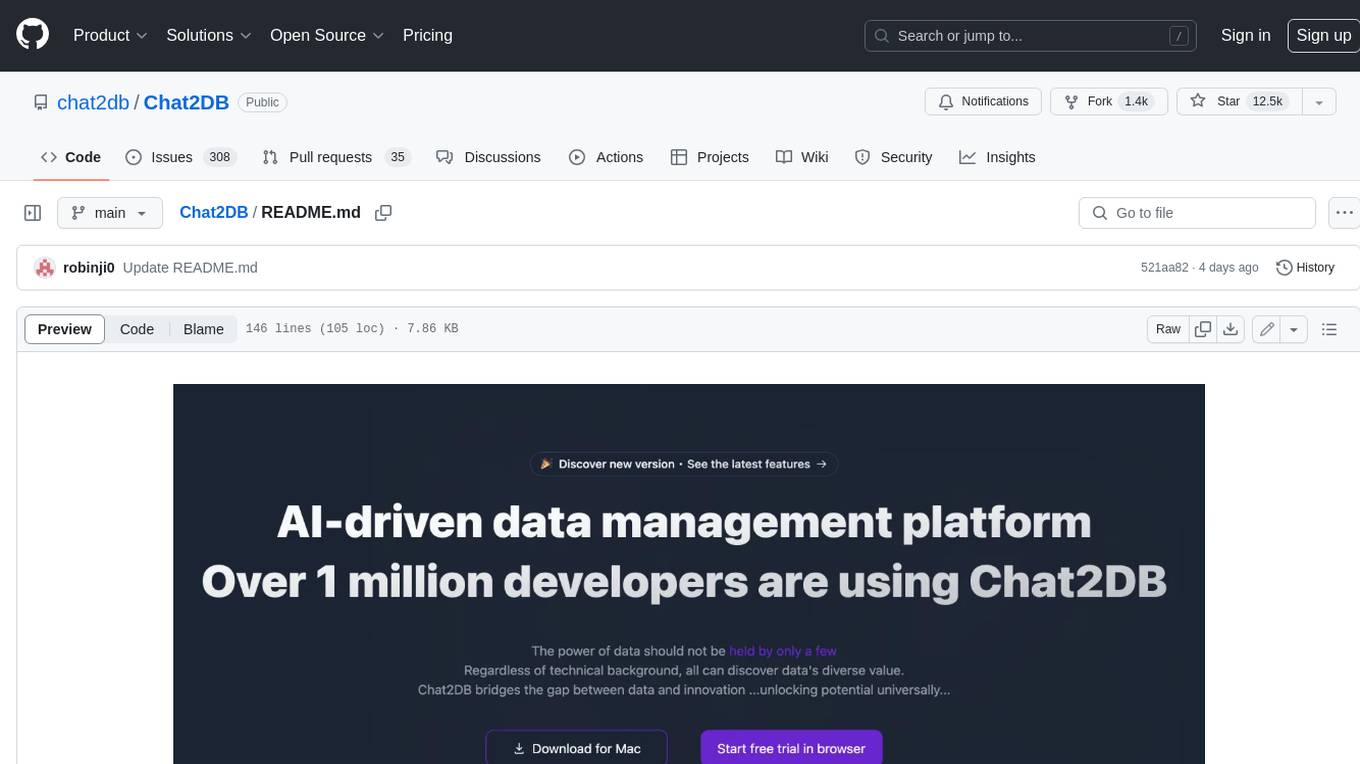
Chat2DB
Chat2DB is an AI-driven data development and analysis platform that enables users to communicate with databases using natural language. It supports a wide range of databases, including MySQL, PostgreSQL, Oracle, SQLServer, SQLite, MariaDB, ClickHouse, DM, Presto, DB2, OceanBase, Hive, KingBase, MongoDB, Redis, and Snowflake. Chat2DB provides a user-friendly interface that allows users to query databases, generate reports, and explore data using natural language commands. It also offers a variety of features to help users improve their productivity, such as auto-completion, syntax highlighting, and error checking.
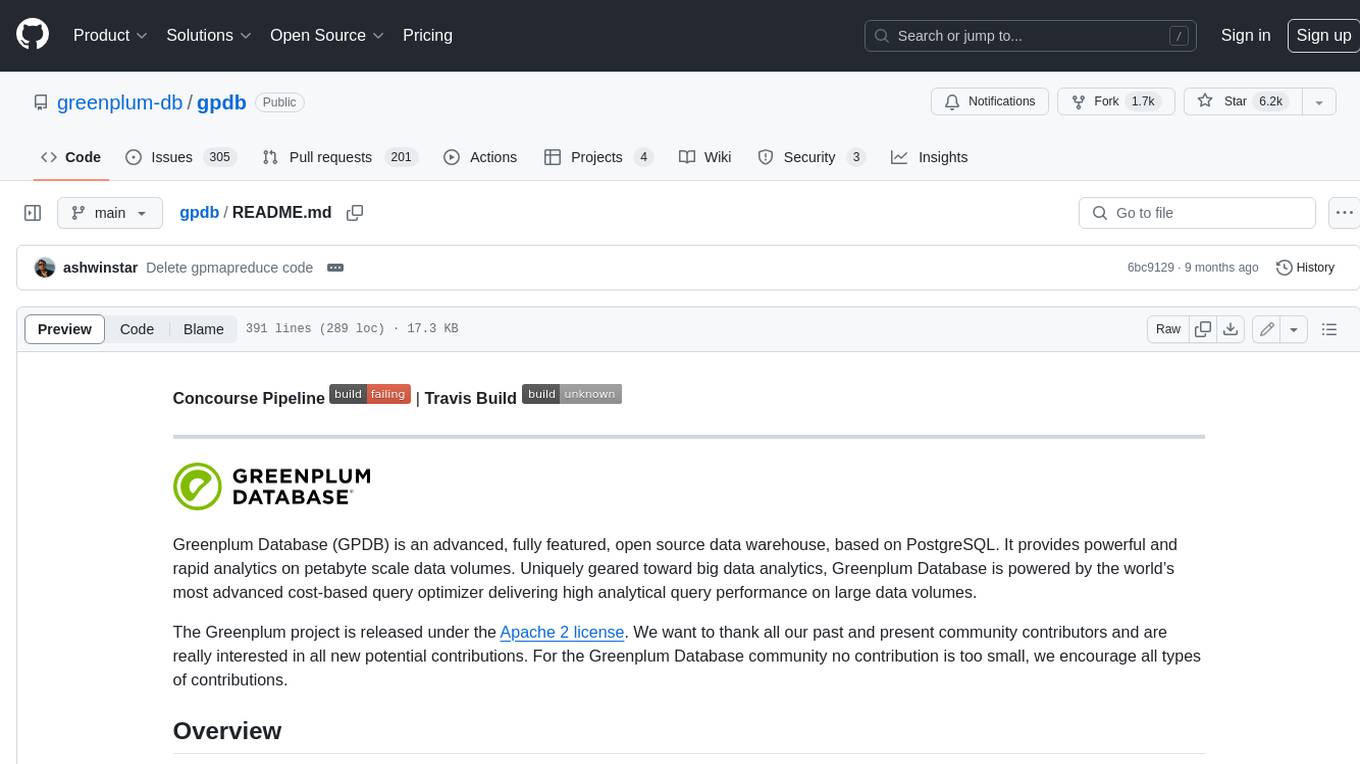
gpdb
Greenplum Database (GPDB) is an advanced, fully featured, open source data warehouse, based on PostgreSQL. It provides powerful and rapid analytics on petabyte scale data volumes. Uniquely geared toward big data analytics, Greenplum Database is powered by the world’s most advanced cost-based query optimizer delivering high analytical query performance on large data volumes.
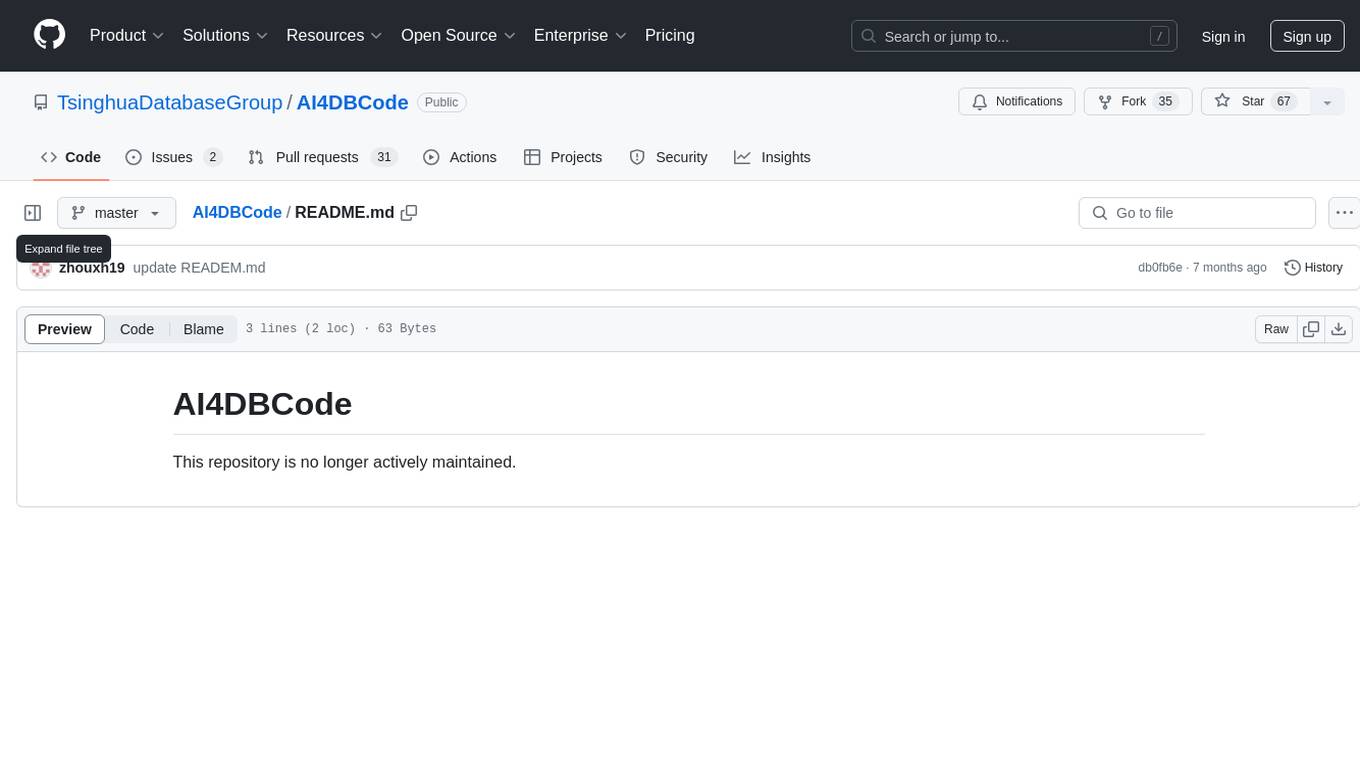
AI4DBCode
AI4DBCode is a repository that is no longer actively maintained. It likely contains code related to artificial intelligence for databases. Users can explore the existing codebase for reference or historical purposes, but should be aware that updates and support are no longer provided.
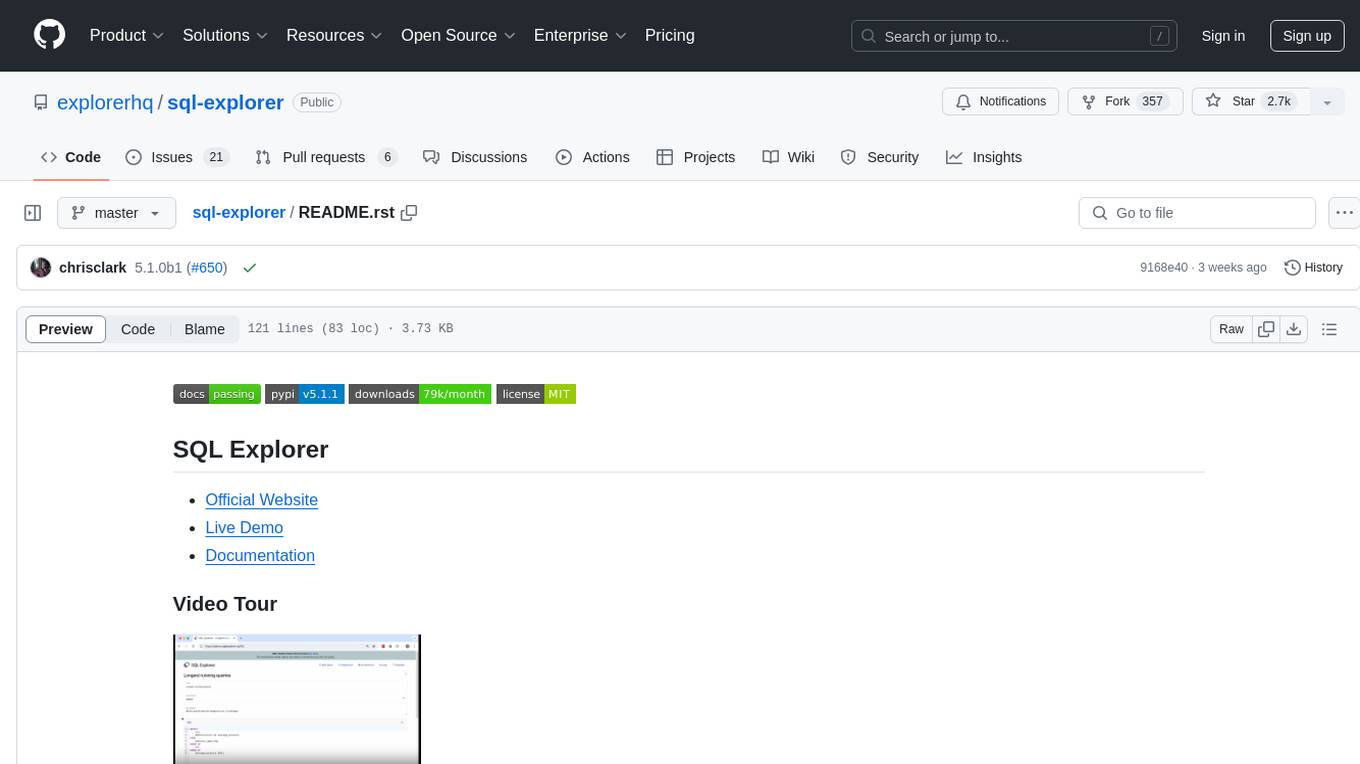
sql-explorer
SQL Explorer is a Django-based application that simplifies the flow of data between users by providing a user-friendly SQL editor to write and share queries. It supports multiple database connections, AI-powered SQL assistant, schema information access, query snapshots, in-browser statistics, parameterized queries, ad-hoc query running, email query results, and more. Users can upload and query JSON or CSV files, and the tool can connect to various SQL databases supported by Django. It aims for simplicity, stability, and ease of use, offering features like autocomplete, pivot tables, and query history logs.
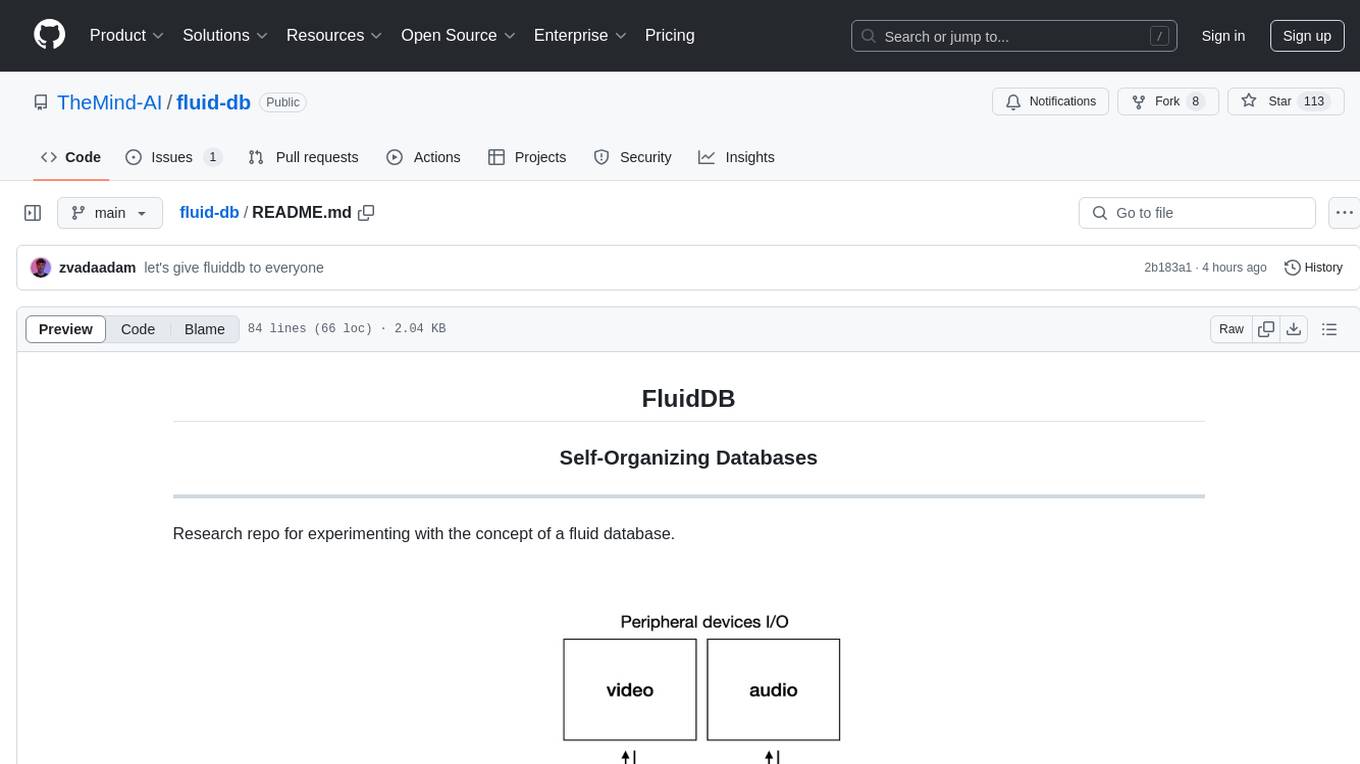
fluid-db
FluidDB is a research repository focusing on the concept of a fluid database that dynamically updates its schema based on ingested data. It enables the creation of personalized AI agents with features like adaptive schema, flexible querying, and versatile data input. The tool allows for storing unstructured data in a structured form and supports natural language queries. It aims to revolutionize database management by providing a dynamic and intuitive approach to data storage and retrieval.
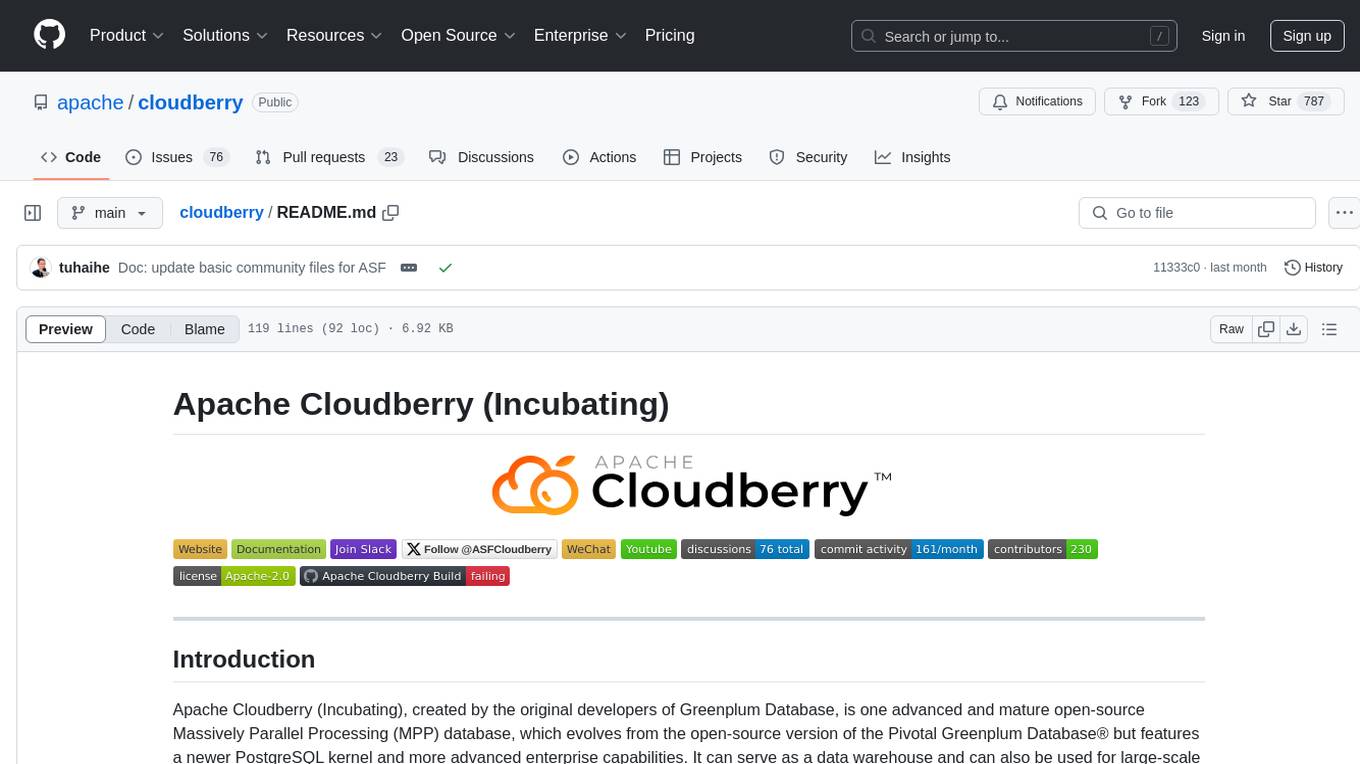
cloudberry
Apache Cloudberry (Incubating) is an advanced and mature open-source Massively Parallel Processing (MPP) database, evolving from the open-source version of the Pivotal Greenplum Database®️. It features a newer PostgreSQL kernel and advanced enterprise capabilities, serving as a data warehouse for large-scale analytics and AI/ML workloads. The main repository includes ecosystem repositories for the website, extensions, connectors, adapters, and utilities.
For similar jobs
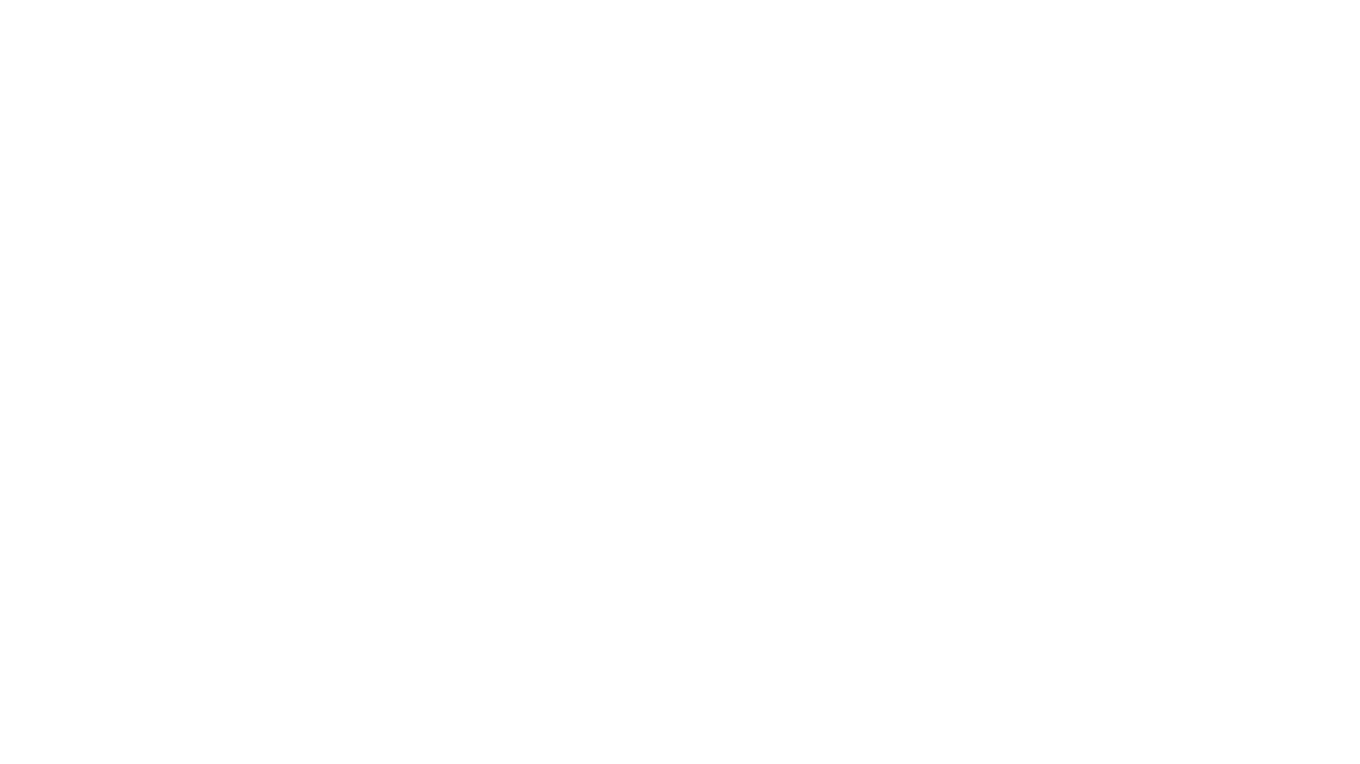
AirGo
AirGo is a front and rear end separation, multi user, multi protocol proxy service management system, simple and easy to use. It supports vless, vmess, shadowsocks, and hysteria2.
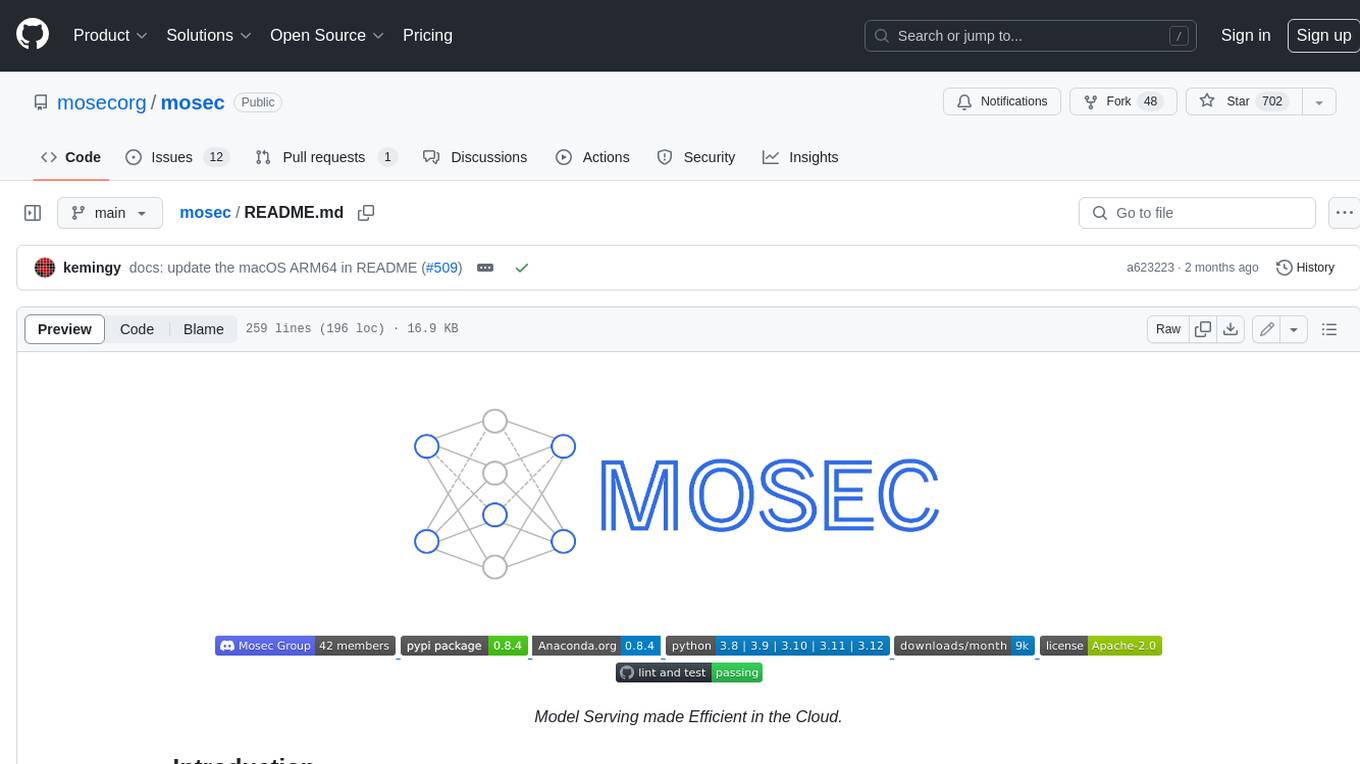
mosec
Mosec is a high-performance and flexible model serving framework for building ML model-enabled backend and microservices. It bridges the gap between any machine learning models you just trained and the efficient online service API. * **Highly performant** : web layer and task coordination built with Rust 🦀, which offers blazing speed in addition to efficient CPU utilization powered by async I/O * **Ease of use** : user interface purely in Python 🐍, by which users can serve their models in an ML framework-agnostic manner using the same code as they do for offline testing * **Dynamic batching** : aggregate requests from different users for batched inference and distribute results back * **Pipelined stages** : spawn multiple processes for pipelined stages to handle CPU/GPU/IO mixed workloads * **Cloud friendly** : designed to run in the cloud, with the model warmup, graceful shutdown, and Prometheus monitoring metrics, easily managed by Kubernetes or any container orchestration systems * **Do one thing well** : focus on the online serving part, users can pay attention to the model optimization and business logic
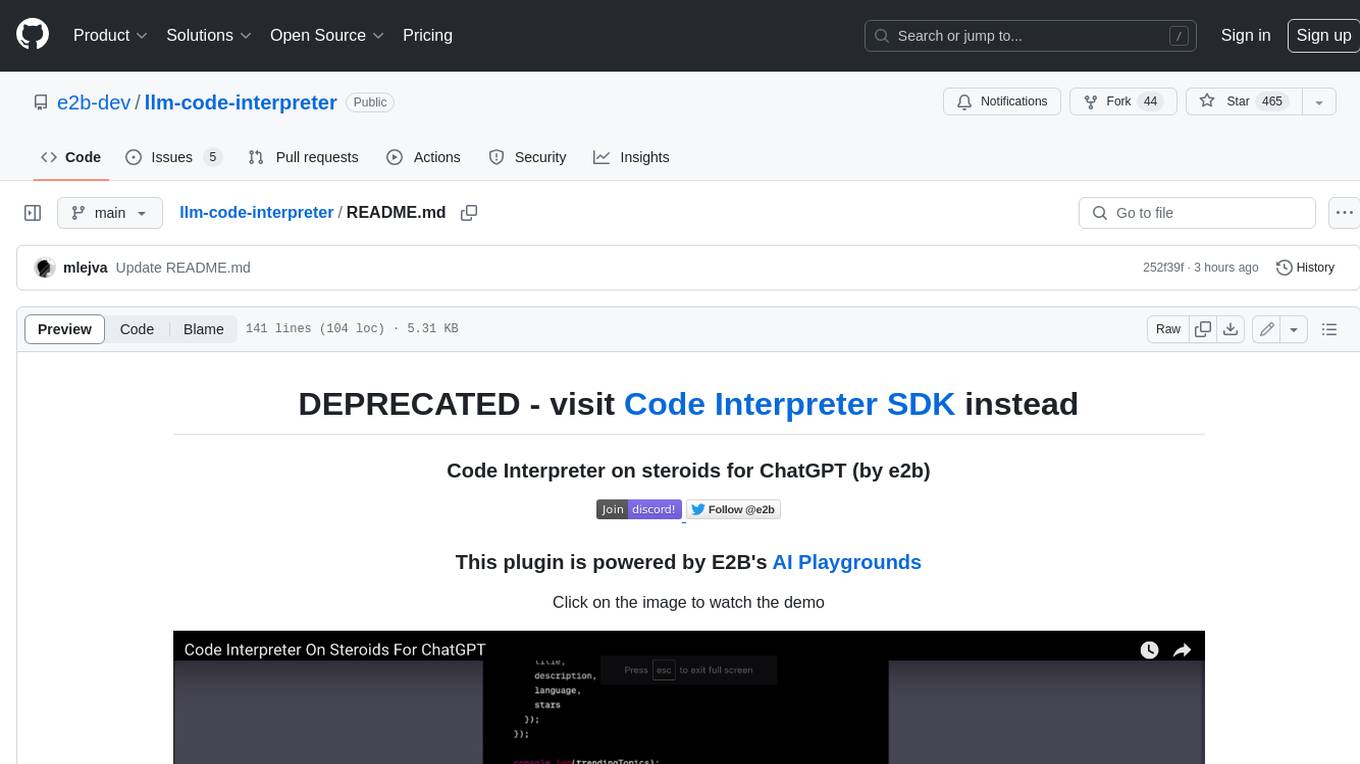
llm-code-interpreter
The 'llm-code-interpreter' repository is a deprecated plugin that provides a code interpreter on steroids for ChatGPT by E2B. It gives ChatGPT access to a sandboxed cloud environment with capabilities like running any code, accessing Linux OS, installing programs, using filesystem, running processes, and accessing the internet. The plugin exposes commands to run shell commands, read files, and write files, enabling various possibilities such as running different languages, installing programs, starting servers, deploying websites, and more. It is powered by the E2B API and is designed for agents to freely experiment within a sandboxed environment.
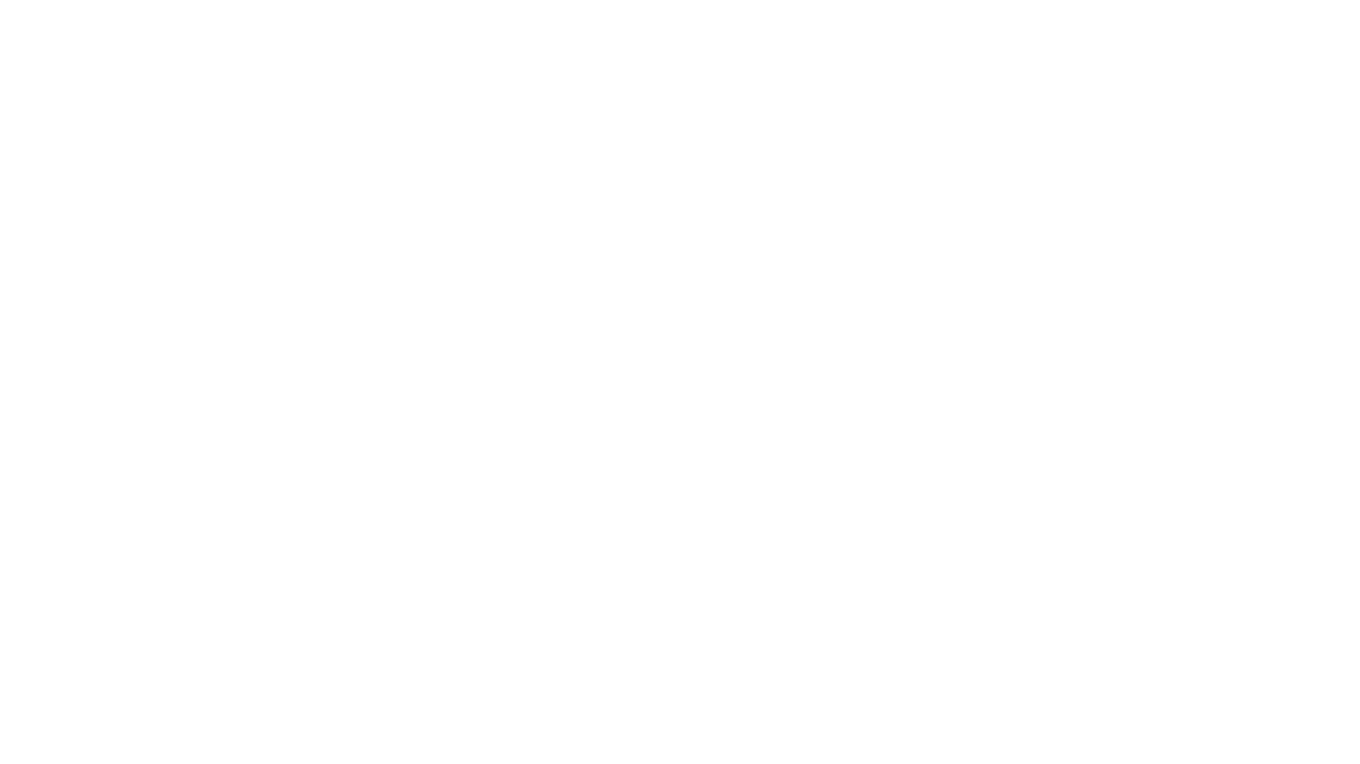
pezzo
Pezzo is a fully cloud-native and open-source LLMOps platform that allows users to observe and monitor AI operations, troubleshoot issues, save costs and latency, collaborate, manage prompts, and deliver AI changes instantly. It supports various clients for prompt management, observability, and caching. Users can run the full Pezzo stack locally using Docker Compose, with prerequisites including Node.js 18+, Docker, and a GraphQL Language Feature Support VSCode Extension. Contributions are welcome, and the source code is available under the Apache 2.0 License.
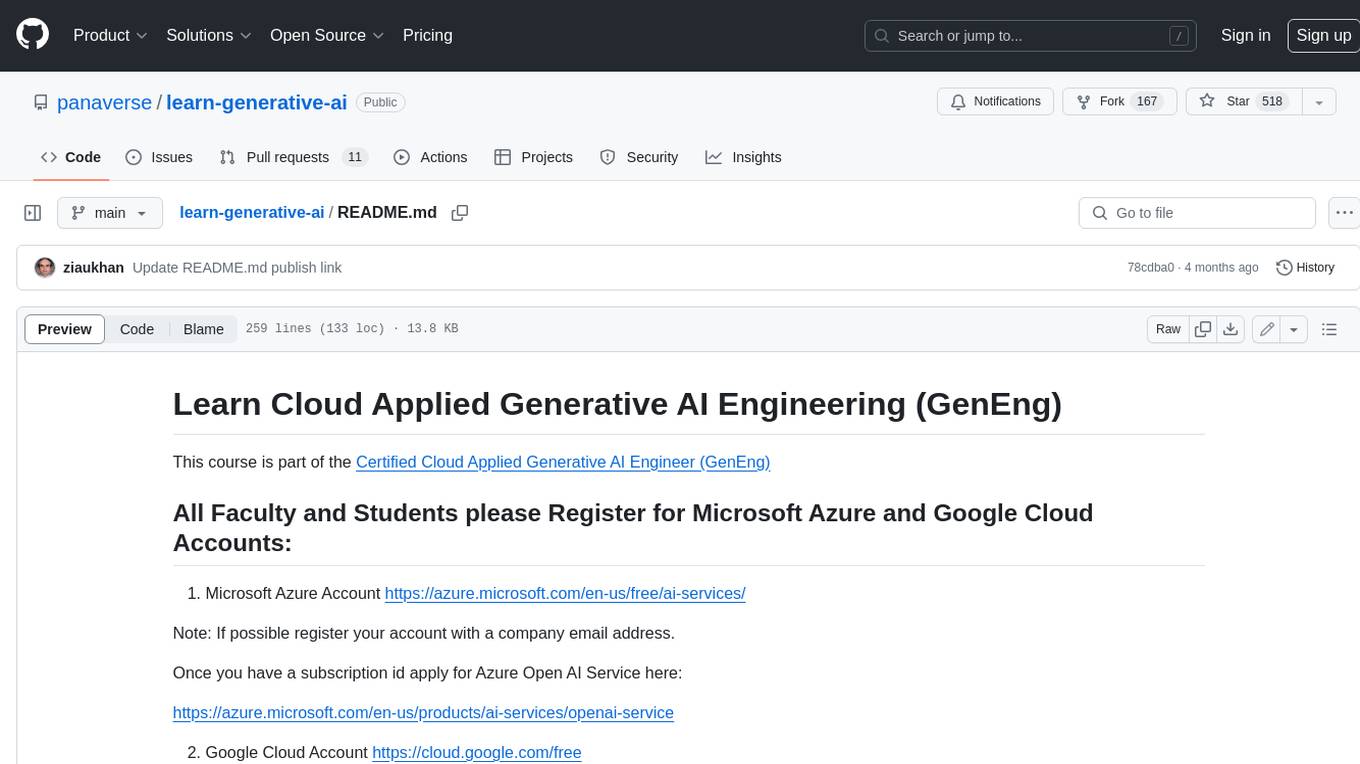
learn-generative-ai
Learn Cloud Applied Generative AI Engineering (GenEng) is a course focusing on the application of generative AI technologies in various industries. The course covers topics such as the economic impact of generative AI, the role of developers in adopting and integrating generative AI technologies, and the future trends in generative AI. Students will learn about tools like OpenAI API, LangChain, and Pinecone, and how to build and deploy Large Language Models (LLMs) for different applications. The course also explores the convergence of generative AI with Web 3.0 and its potential implications for decentralized intelligence.
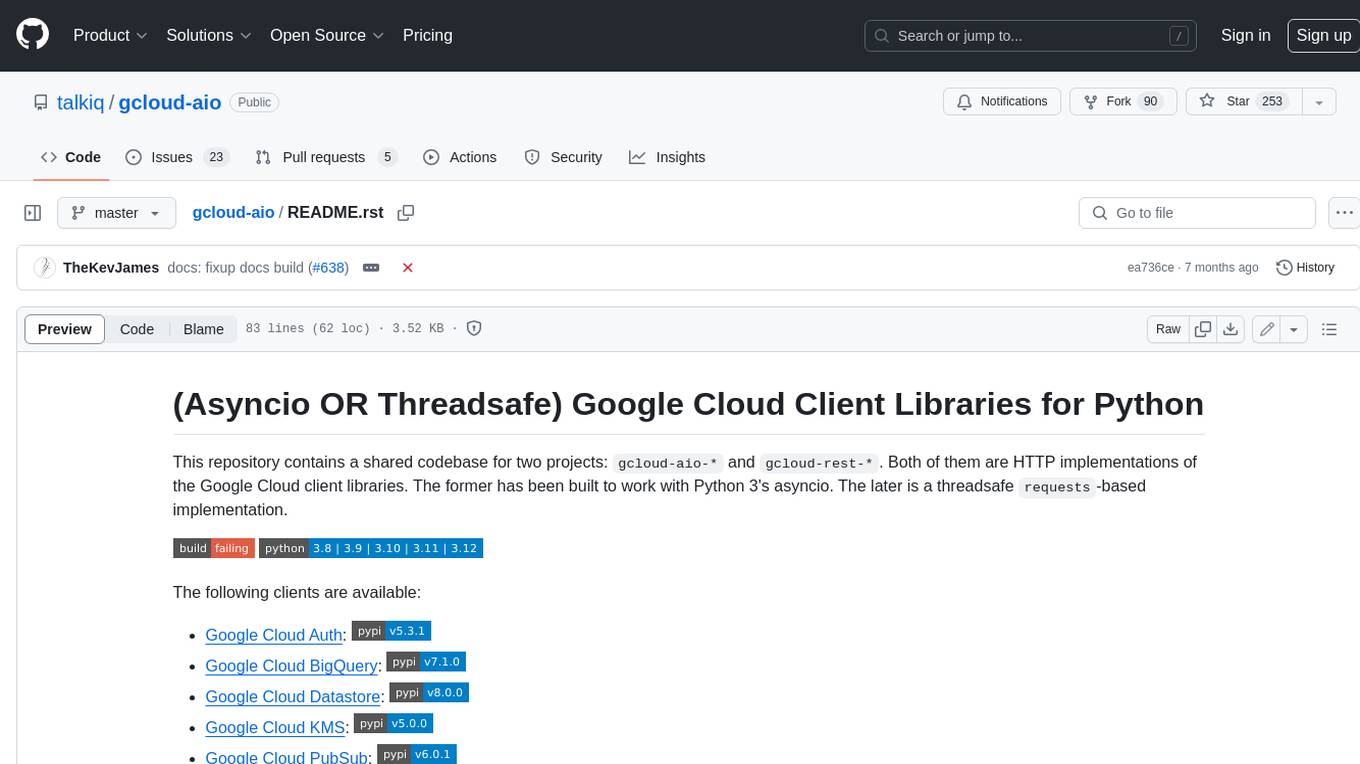
gcloud-aio
This repository contains shared codebase for two projects: gcloud-aio and gcloud-rest. gcloud-aio is built for Python 3's asyncio, while gcloud-rest is a threadsafe requests-based implementation. It provides clients for Google Cloud services like Auth, BigQuery, Datastore, KMS, PubSub, Storage, and Task Queue. Users can install the library using pip and refer to the documentation for usage details. Developers can contribute to the project by following the contribution guide.
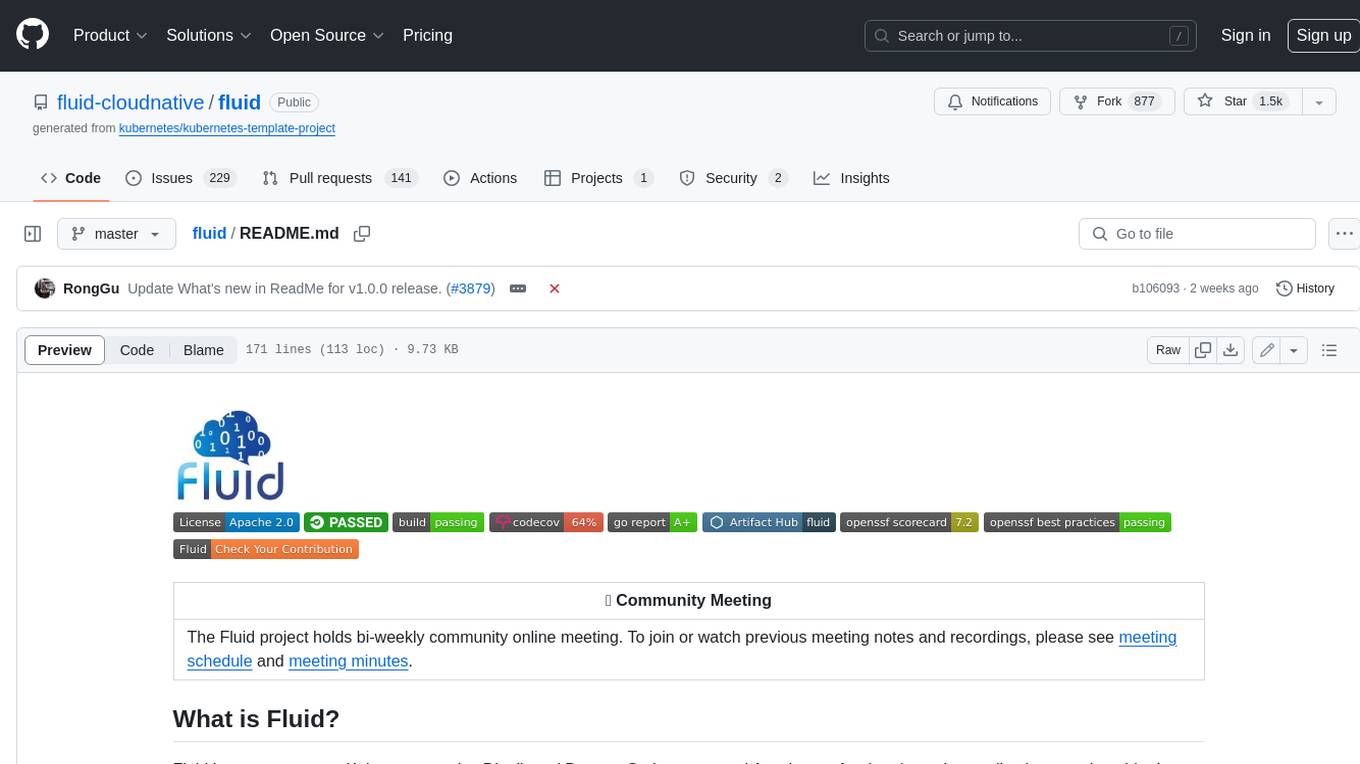
fluid
Fluid is an open source Kubernetes-native Distributed Dataset Orchestrator and Accelerator for data-intensive applications, such as big data and AI applications. It implements dataset abstraction, scalable cache runtime, automated data operations, elasticity and scheduling, and is runtime platform agnostic. Key concepts include Dataset and Runtime. Prerequisites include Kubernetes version > 1.16, Golang 1.18+, and Helm 3. The tool offers features like accelerating remote file accessing, machine learning, accelerating PVC, preloading dataset, and on-the-fly dataset cache scaling. Contributions are welcomed, and the project is under the Apache 2.0 license with a vendor-neutral approach.
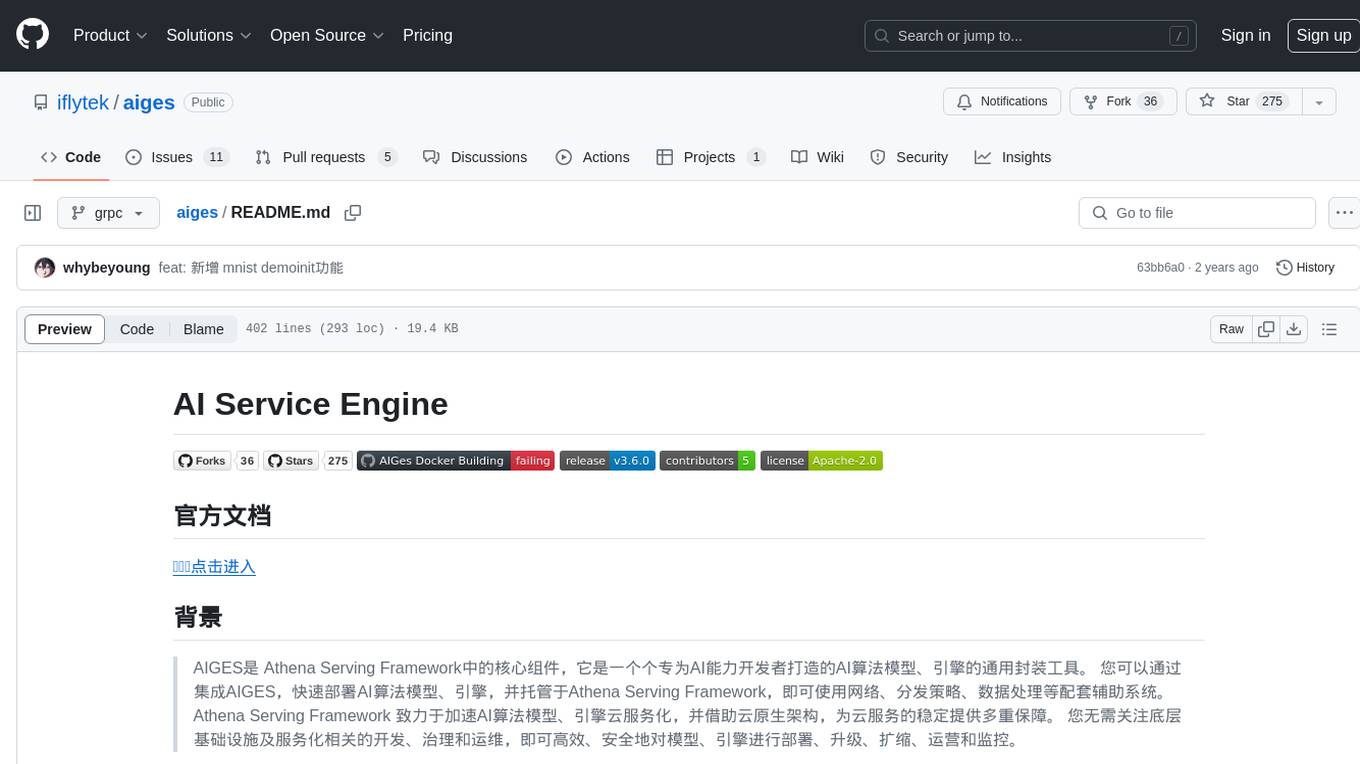
aiges
AIGES is a core component of the Athena Serving Framework, designed as a universal encapsulation tool for AI developers to deploy AI algorithm models and engines quickly. By integrating AIGES, you can deploy AI algorithm models and engines rapidly and host them on the Athena Serving Framework, utilizing supporting auxiliary systems for networking, distribution strategies, data processing, etc. The Athena Serving Framework aims to accelerate the cloud service of AI algorithm models and engines, providing multiple guarantees for cloud service stability through cloud-native architecture. You can efficiently and securely deploy, upgrade, scale, operate, and monitor models and engines without focusing on underlying infrastructure and service-related development, governance, and operations.