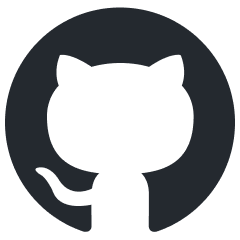
ModernBERT
Bringing BERT into modernity via both architecture changes and scaling
Stars: 963
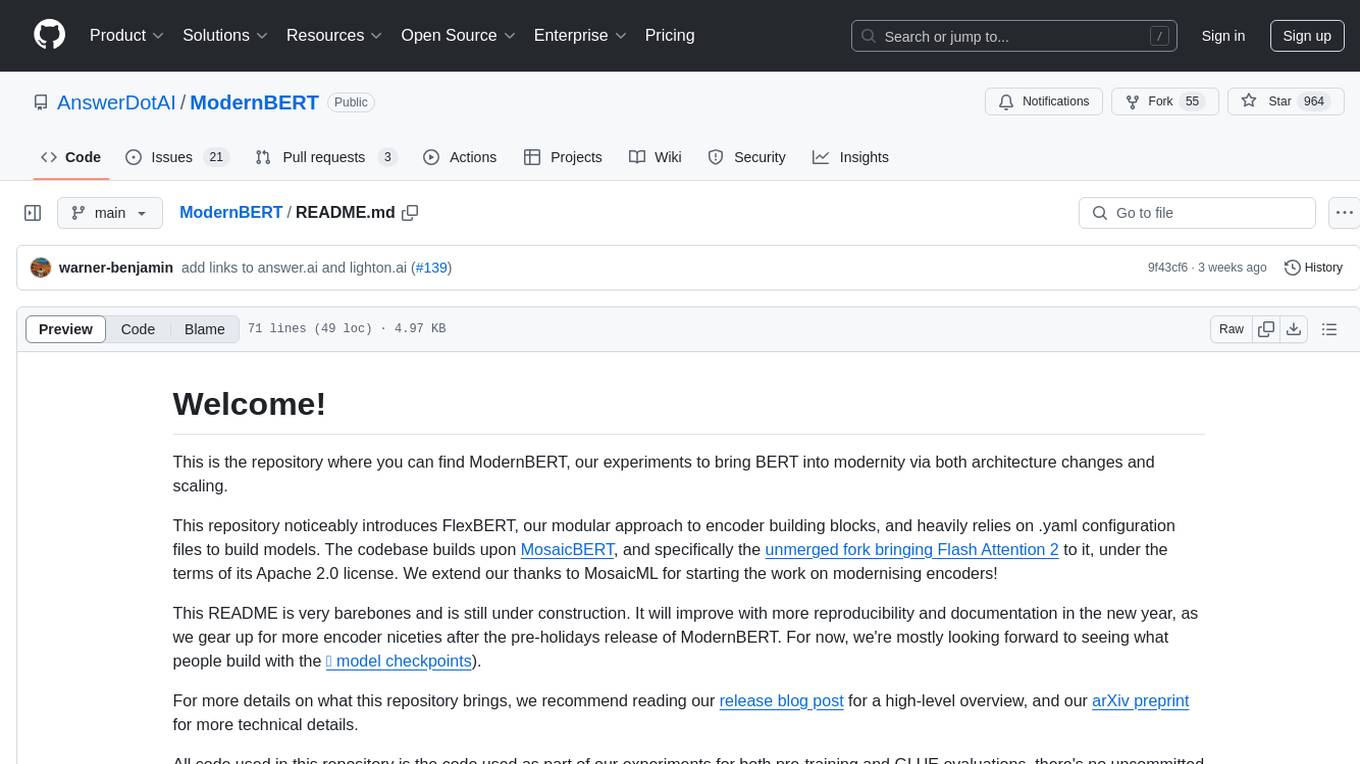
ModernBERT is a repository focused on modernizing BERT through architecture changes and scaling. It introduces FlexBERT, a modular approach to encoder building blocks, and heavily relies on .yaml configuration files to build models. The codebase builds upon MosaicBERT and incorporates Flash Attention 2. The repository is used for pre-training and GLUE evaluations, with a focus on reproducibility and documentation. It provides a collaboration between Answer.AI, LightOn, and friends.
README:
This is the repository where you can find ModernBERT, our experiments to bring BERT into modernity via both architecture changes and scaling.
This repository noticeably introduces FlexBERT, our modular approach to encoder building blocks, and heavily relies on .yaml configuration files to build models. The codebase builds upon MosaicBERT, and specifically the unmerged fork bringing Flash Attention 2 to it, under the terms of its Apache 2.0 license. We extend our thanks to MosaicML for starting the work on modernising encoders!
This README is very barebones and is still under construction. It will improve with more reproducibility and documentation in the new year, as we gear up for more encoder niceties after the pre-holidays release of ModernBERT. For now, we're mostly looking forward to seeing what people build with the 🤗 model checkpoints).
For more details on what this repository brings, we recommend reading our release blog post for a high-level overview, and our arXiv preprint for more technical details.
All code used in this repository is the code used as part of our experiments for both pre-training and GLUE evaluations, there's no uncommitted secret training sauce.
This is the research repository for ModernBERT, focused on pre-training and evaluations. If you're seeking the HuggingFace version, designed to integrate with any common pipeline, please head to the ModernBERT Collection on HuggingFace
ModernBERT is a collaboration between Answer.AI, LightOn, and friends.
We have fully documented the environment used to train ModernBERT, which can be installed on a GPU-equipped machine with the following commands:
conda env create -f environment.yaml
# if the conda environment errors out set channel priority to flexible:
# conda config --set channel_priority flexible
conda activate bert24
# if using H100s clone and build flash attention 3
# git clone https://github.com/Dao-AILab/flash-attention.git
# cd flash-attention/hopper
# python setup.py install
# install flash attention 2 (model uses FA3+FA2 or just FA2 if FA3 isn't supported)
pip install "flash_attn==2.6.3" --no-build-isolation
# or download a precompiled wheel from https://github.com/Dao-AILab/flash-attention/releases/tag/v2.6.3
# or limit the number of parallel compilation jobs
# MAX_JOBS=8 pip install "flash_attn==2.6.3" --no-build-isolation
Training heavily leverages the composer framework. All training are configured via YAML files, of which you can find examples in the yamls
folder. We highly encourage you to check out one of the example yamls, such as yamls/main/flex-bert-rope-base.yaml
, to explore the configuration options.
GLUE evaluations for a ModernBERT model trained with this repository can be ran with via run_evals.py
, by providing it with a checkpoint and a training config. To evaluate non-ModernBERT models, you should use glue.py
in conjunction with a slightly different training YAML, of which you can find examples in the yamls/finetuning
folder.
The examples
subfolder contains scripts for training retrieval models, both dense models based on Sentence Transformers and ColBERT models via the PyLate library:
-
examples/train_pylate.py
: The boilerplate code to train a ModernBERT-based ColBERT model with PyLate. -
examples/train_st.py
: The boilerplate code to train a ModernBERT-based dense retrieval model with Sentence Transformers. -
examples/evaluate_pylate.py
: The boilerplate code to evaluate a ModernBERT-based ColBERT model with PyLate. -
examples/evaluate_st.py
: The boilerplate code to evaluate a ModernBERT-based dense retrieval model with Sentence Transformers.
If you use ModernBERT in your work, be it the released models, the intermediate checkpoints (release pending) or this training repository, please cite:
@misc{modernbert,
title={Smarter, Better, Faster, Longer: A Modern Bidirectional Encoder for Fast, Memory Efficient, and Long Context Finetuning and Inference},
author={Benjamin Warner and Antoine Chaffin and Benjamin Clavié and Orion Weller and Oskar Hallström and Said Taghadouini and Alexis Gallagher and Raja Biswas and Faisal Ladhak and Tom Aarsen and Nathan Cooper and Griffin Adams and Jeremy Howard and Iacopo Poli},
year={2024},
eprint={2412.13663},
archivePrefix={arXiv},
primaryClass={cs.CL},
url={https://arxiv.org/abs/2412.13663},
}
For Tasks:
Click tags to check more tools for each tasksFor Jobs:
Alternative AI tools for ModernBERT
Similar Open Source Tools
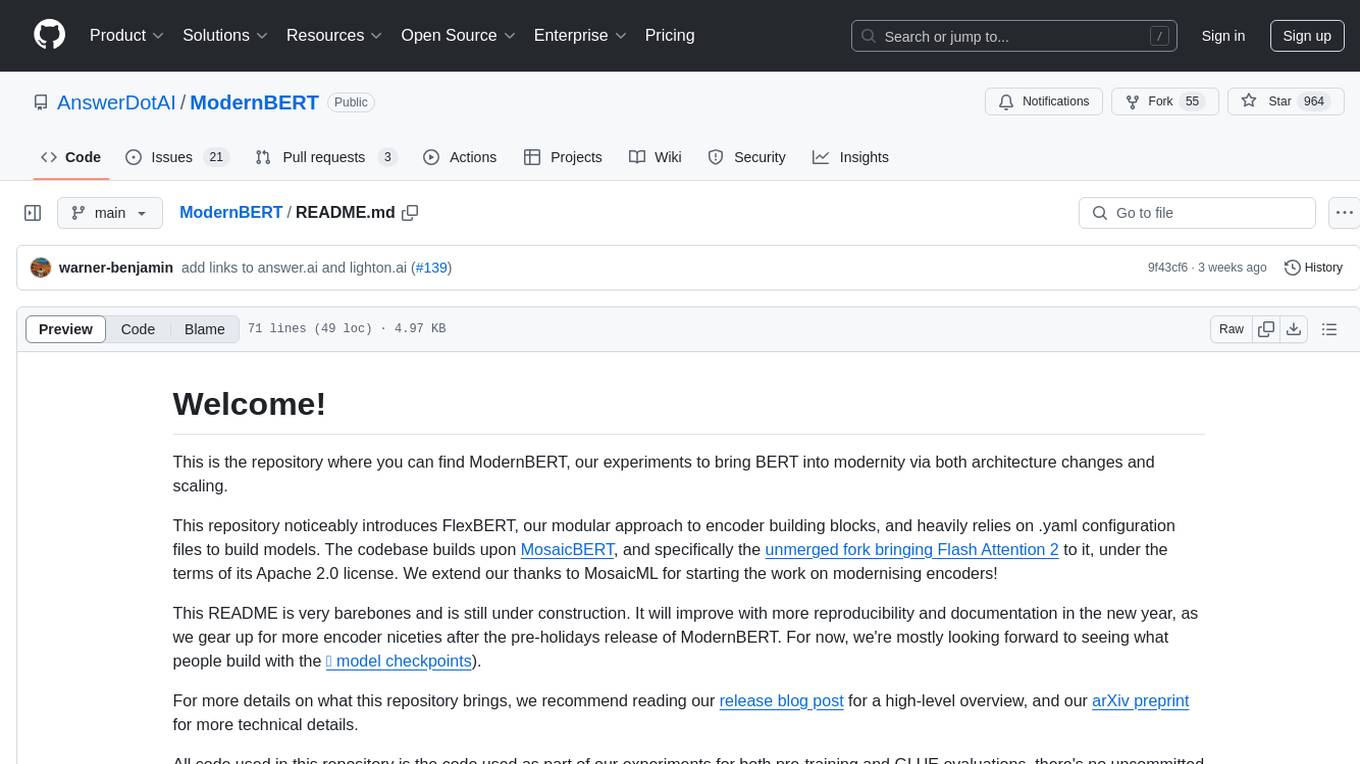
ModernBERT
ModernBERT is a repository focused on modernizing BERT through architecture changes and scaling. It introduces FlexBERT, a modular approach to encoder building blocks, and heavily relies on .yaml configuration files to build models. The codebase builds upon MosaicBERT and incorporates Flash Attention 2. The repository is used for pre-training and GLUE evaluations, with a focus on reproducibility and documentation. It provides a collaboration between Answer.AI, LightOn, and friends.
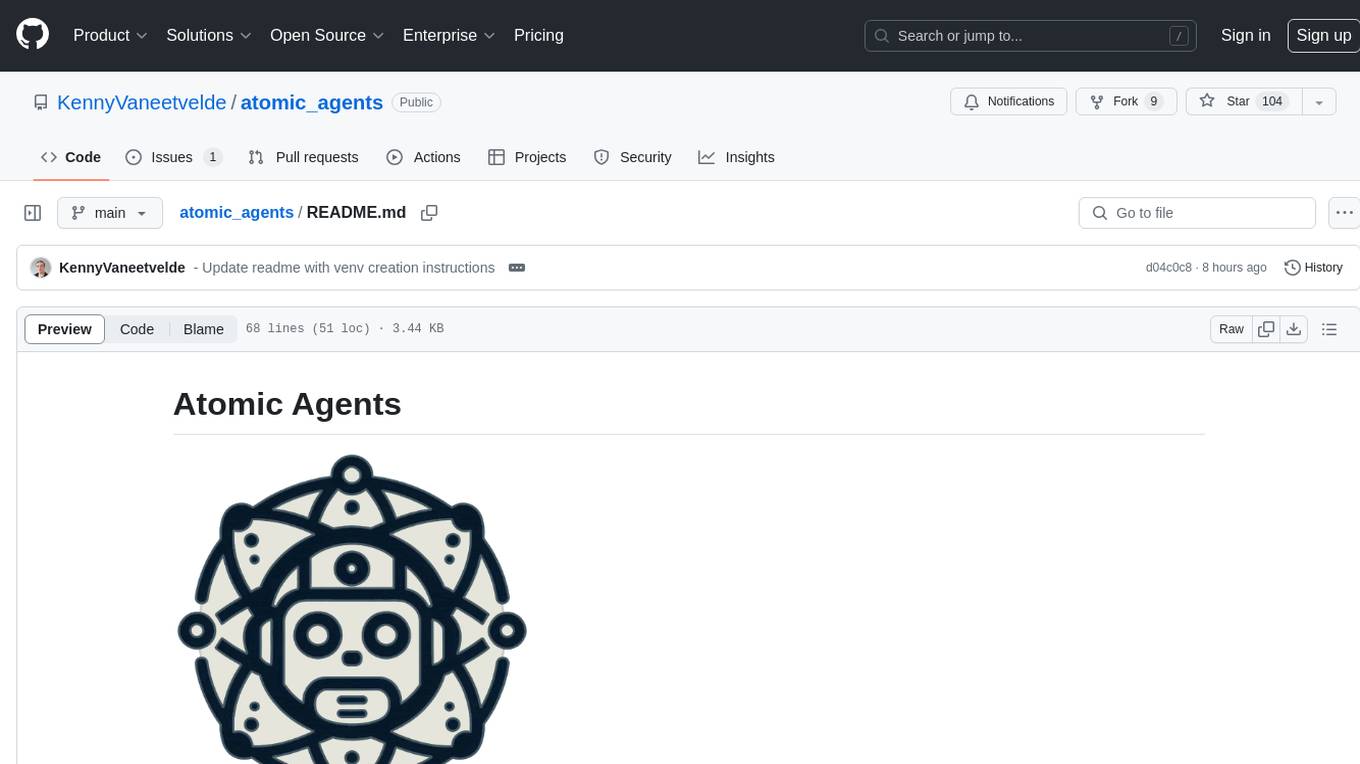
atomic_agents
Atomic Agents is a modular and extensible framework designed for creating powerful applications. It follows the principles of Atomic Design, emphasizing small and single-purpose components. Leveraging Pydantic for data validation and serialization, the framework offers a set of tools and agents that can be combined to build AI applications. It depends on the Instructor package and supports various APIs like OpenAI, Cohere, Anthropic, and Gemini. Atomic Agents is suitable for developers looking to create AI agents with a focus on modularity and flexibility.
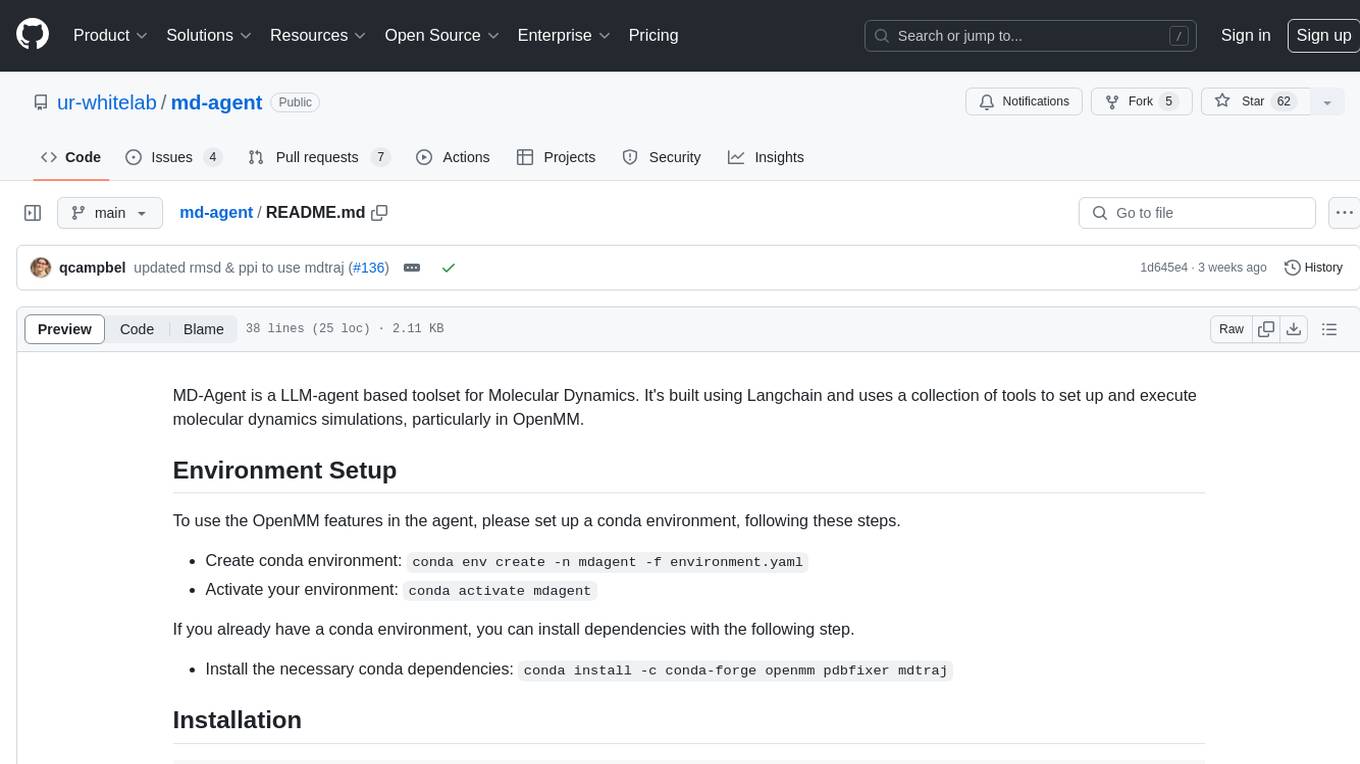
md-agent
MD-Agent is a LLM-agent based toolset for Molecular Dynamics. It uses Langchain and a collection of tools to set up and execute molecular dynamics simulations, particularly in OpenMM. The tool assists in environment setup, installation, and usage by providing detailed steps. It also requires API keys for certain functionalities, such as OpenAI and paper-qa for literature searches. Contributions to the project are welcome, with a detailed Contributor's Guide available for interested individuals.
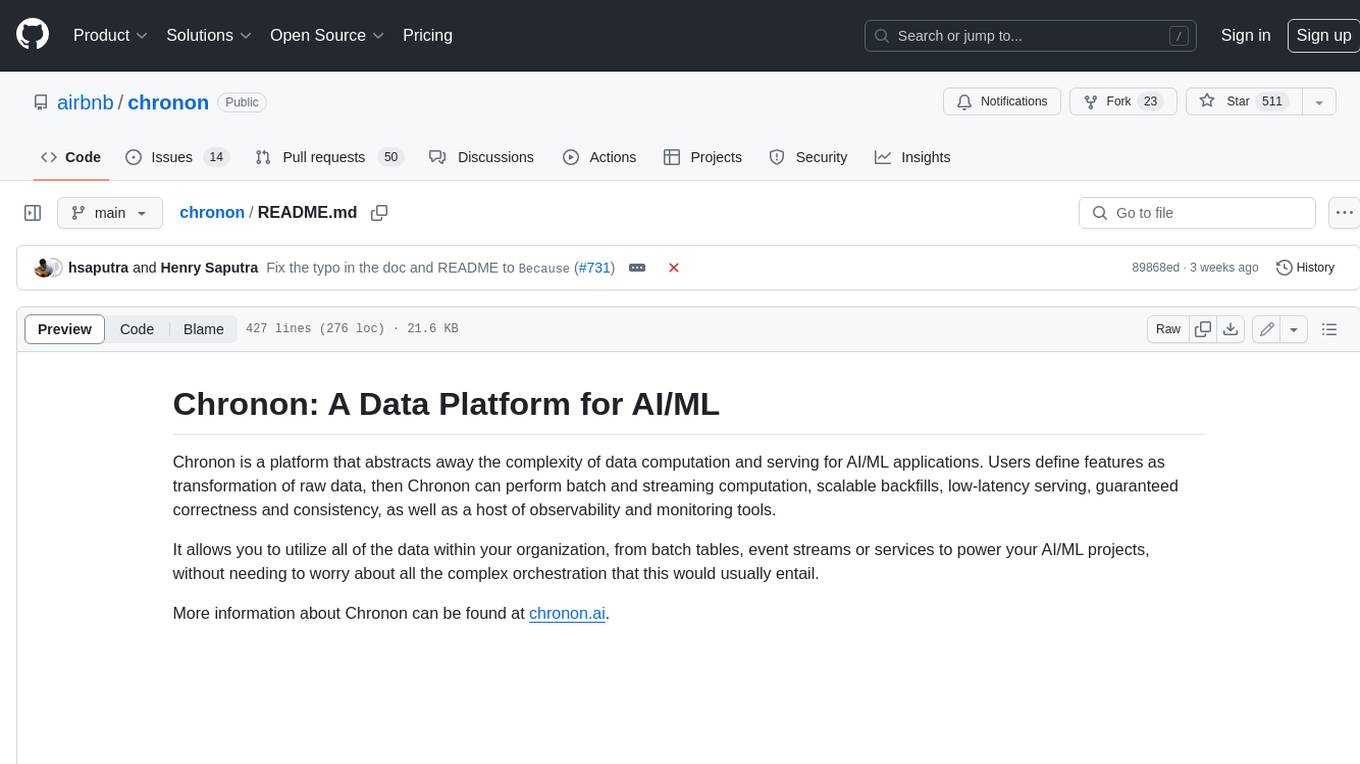
chronon
Chronon is a platform that simplifies and improves ML workflows by providing a central place to define features, ensuring point-in-time correctness for backfills, simplifying orchestration for batch and streaming pipelines, offering easy endpoints for feature fetching, and guaranteeing and measuring consistency. It offers benefits over other approaches by enabling the use of a broad set of data for training, handling large aggregations and other computationally intensive transformations, and abstracting away the infrastructure complexity of data plumbing.
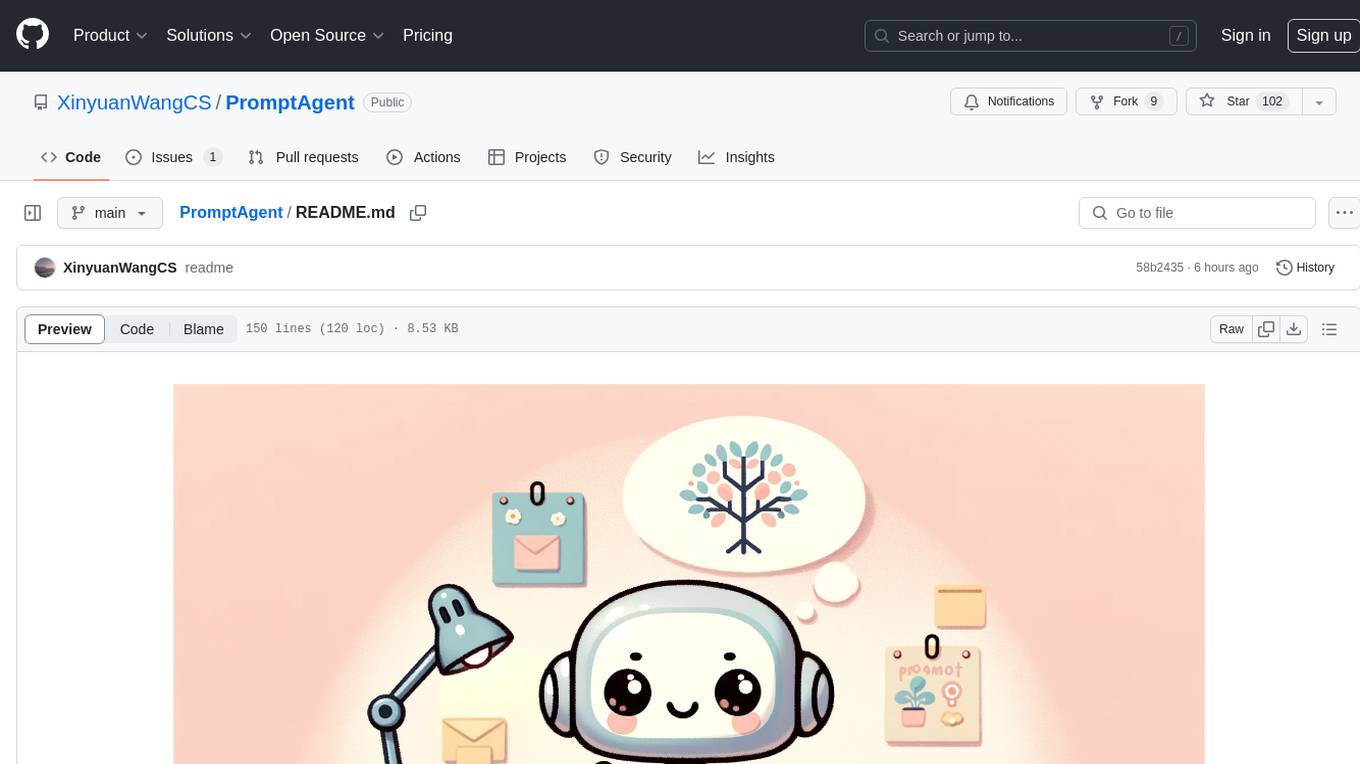
PromptAgent
PromptAgent is a repository for a novel automatic prompt optimization method that crafts expert-level prompts using language models. It provides a principled framework for prompt optimization by unifying prompt sampling and rewarding using MCTS algorithm. The tool supports different models like openai, palm, and huggingface models. Users can run PromptAgent to optimize prompts for specific tasks by strategically sampling model errors, generating error feedbacks, simulating future rewards, and searching for high-reward paths leading to expert prompts.
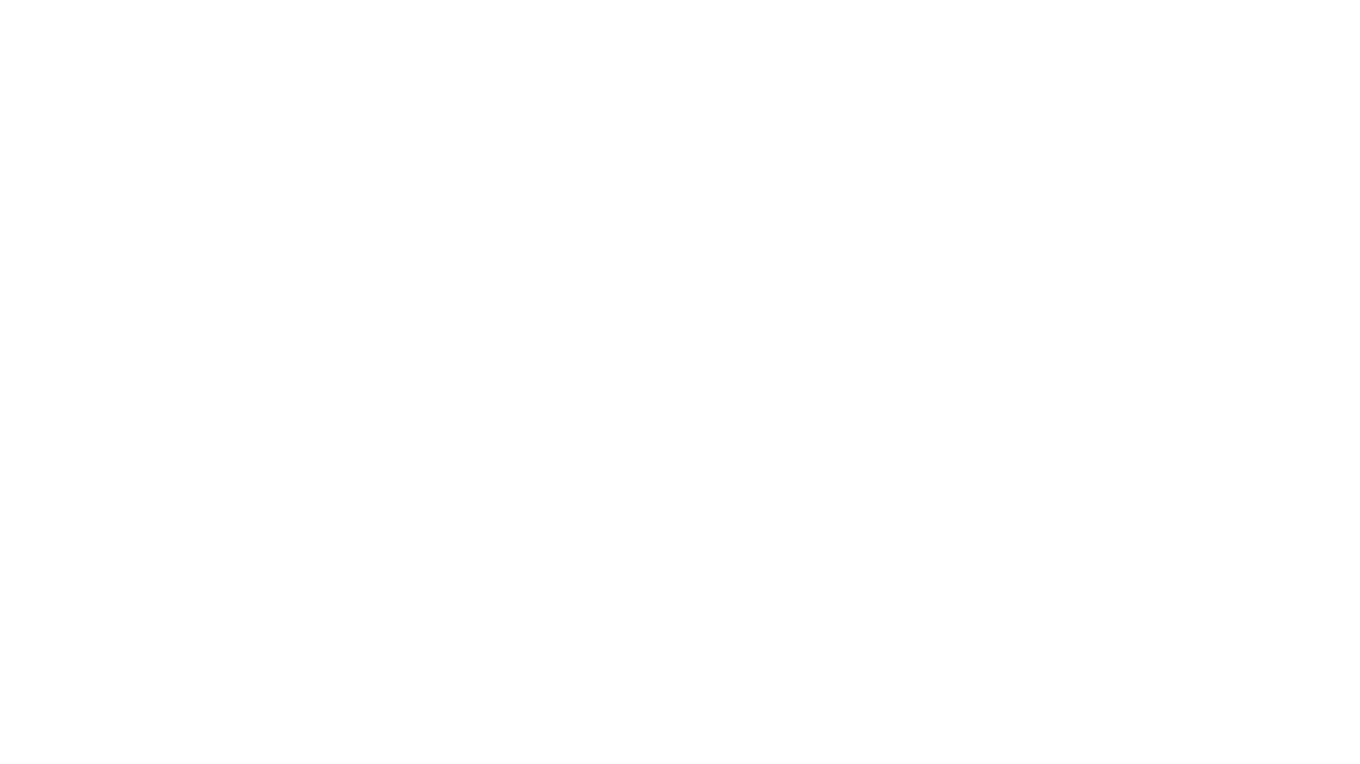
GlaDOS
This project aims to create a real-life version of GLaDOS, an aware, interactive, and embodied AI entity. It involves training a voice generator, developing a 'Personality Core,' implementing a memory system, providing vision capabilities, creating 3D-printable parts, and designing an animatronics system. The software architecture focuses on low-latency voice interactions, utilizing a circular buffer for data recording, text streaming for quick transcription, and a text-to-speech system. The project also emphasizes minimal dependencies for running on constrained hardware. The hardware system includes servo- and stepper-motors, 3D-printable parts for GLaDOS's body, animations for expression, and a vision system for tracking and interaction. Installation instructions cover setting up the TTS engine, required Python packages, compiling llama.cpp, installing an inference backend, and voice recognition setup. GLaDOS can be run using 'python glados.py' and tested using 'demo.ipynb'.
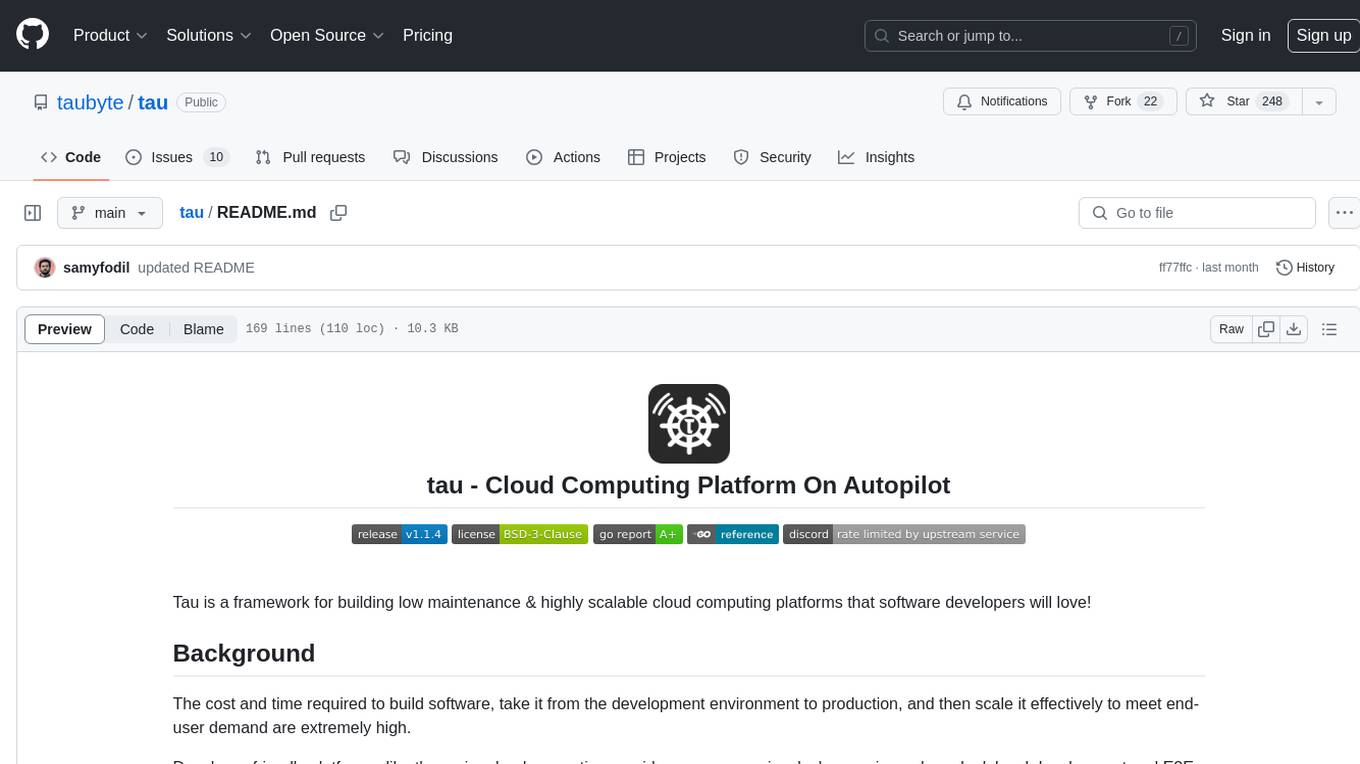
tau
Tau is a framework for building low maintenance & highly scalable cloud computing platforms that software developers will love. It aims to solve the high cost and time required to build, deploy, and scale software by providing a developer-friendly platform that offers autonomy and flexibility. Tau simplifies the process of building and maintaining a cloud computing platform, enabling developers to achieve 'Local Coding Equals Global Production' effortlessly. With features like auto-discovery, content-addressing, and support for WebAssembly, Tau empowers users to create serverless computing environments, host frontends, manage databases, and more. The platform also supports E2E testing and can be extended using a plugin system called orbit.
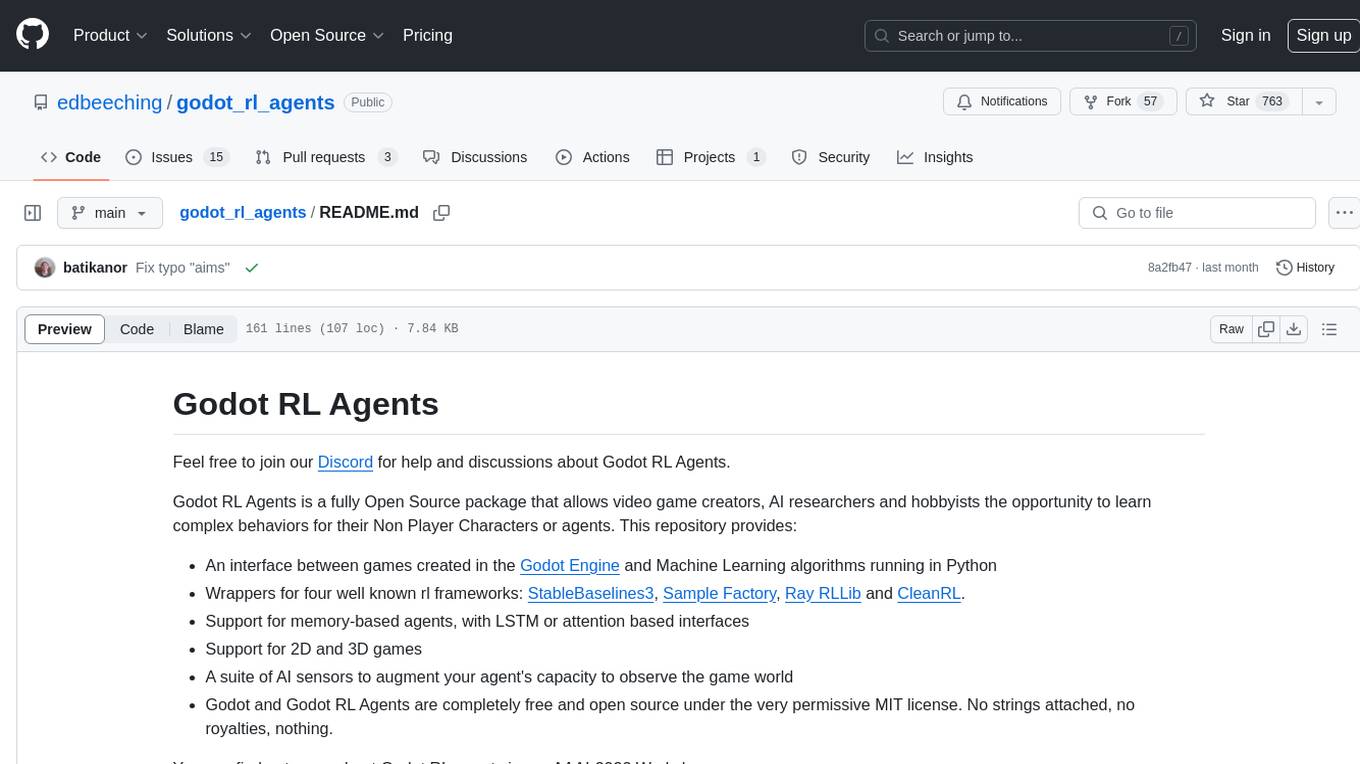
godot_rl_agents
Godot RL Agents is an open-source package that facilitates the integration of Machine Learning algorithms with games created in the Godot Engine. It provides interfaces for popular RL frameworks, support for memory-based agents, 2D and 3D games, AI sensors, and is licensed under MIT. Users can train agents in the Godot editor, create custom environments, export trained agents in ONNX format, and utilize advanced features like different RL training frameworks.
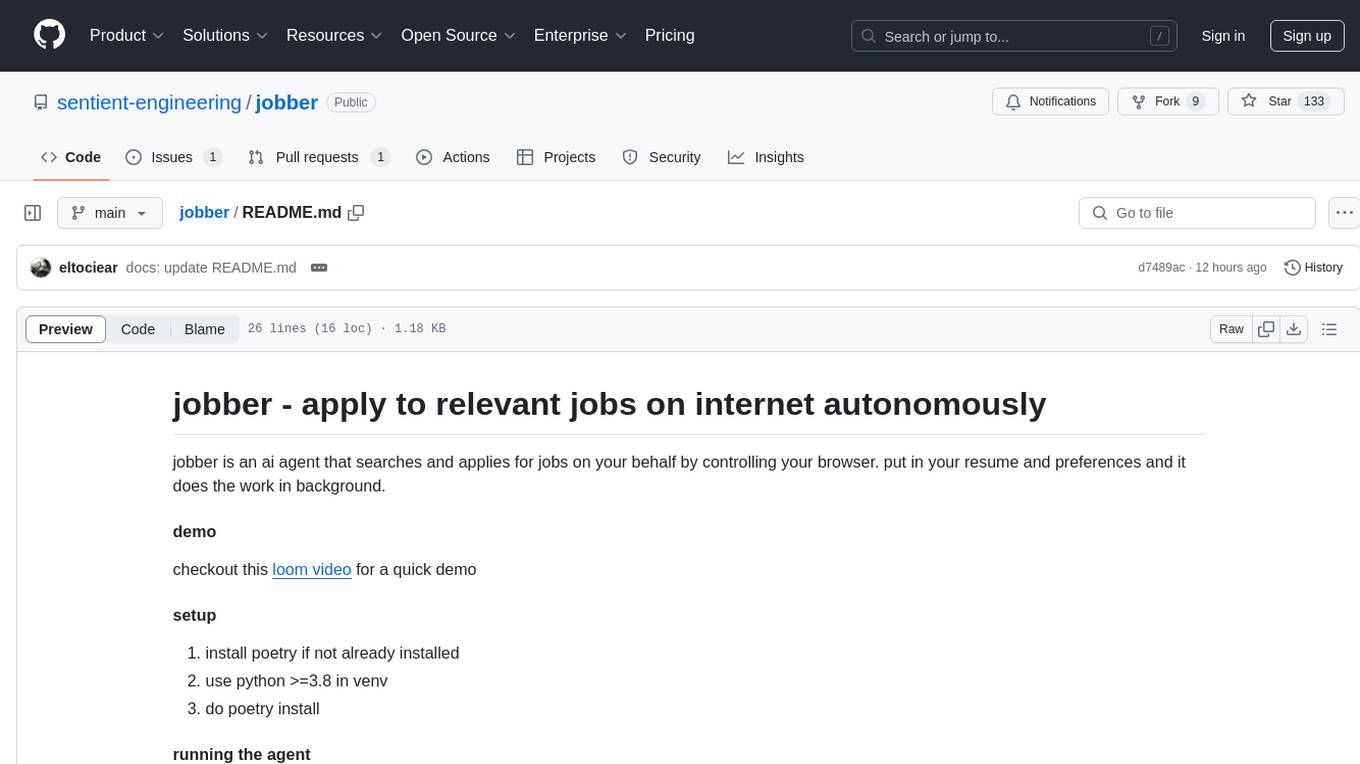
jobber
Jobber is an AI agent that autonomously searches and applies for jobs on the internet on behalf of the user. By controlling the browser, users can input their resume and preferences, allowing Jobber to work in the background. The tool streamlines the job application process by automating the repetitive task of job hunting and applying, saving users time and effort. Jobber offers a convenient solution for individuals looking to explore job opportunities without the hassle of manual job searching and application submission.
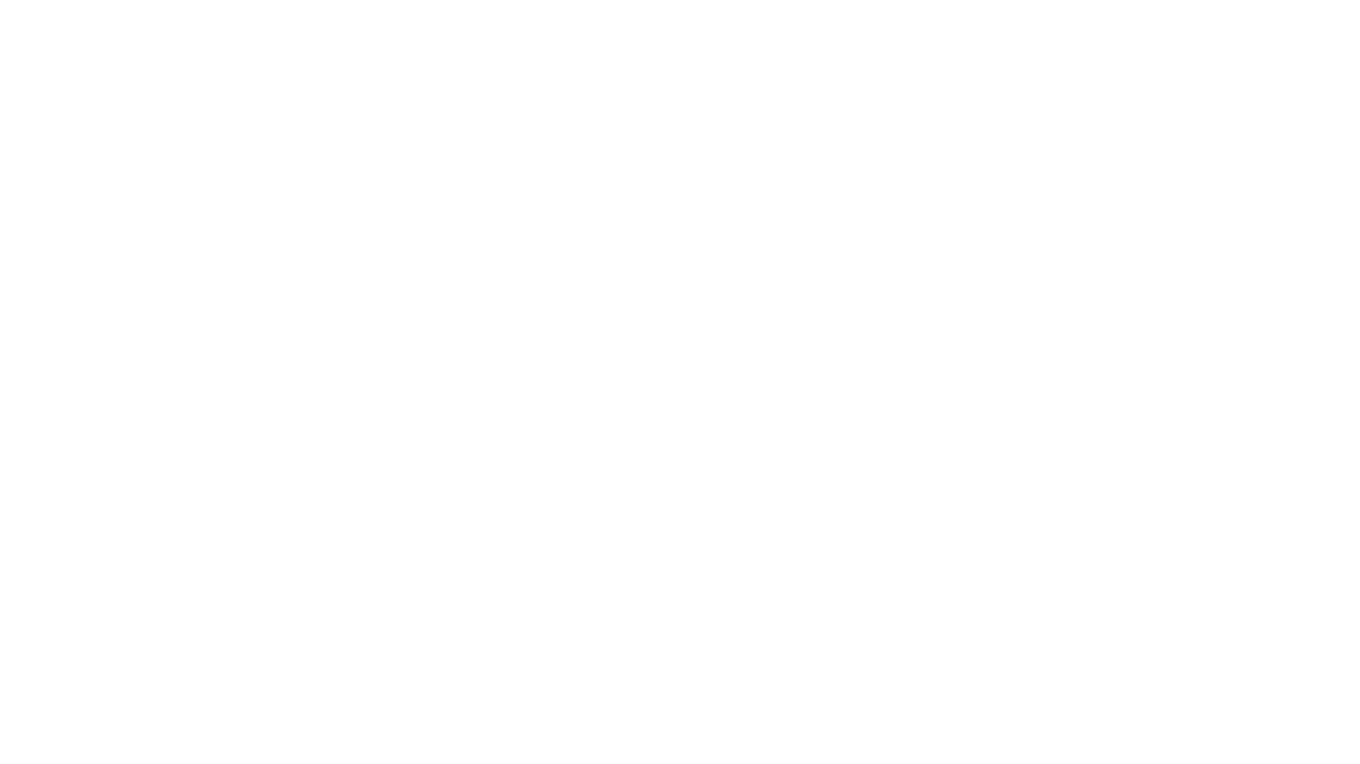
eureka-ml-insights
The Eureka ML Insights Framework is a repository containing code designed to help researchers and practitioners run reproducible evaluations of generative models efficiently. Users can define custom pipelines for data processing, inference, and evaluation, as well as utilize pre-defined evaluation pipelines for key benchmarks. The framework provides a structured approach to conducting experiments and analyzing model performance across various tasks and modalities.
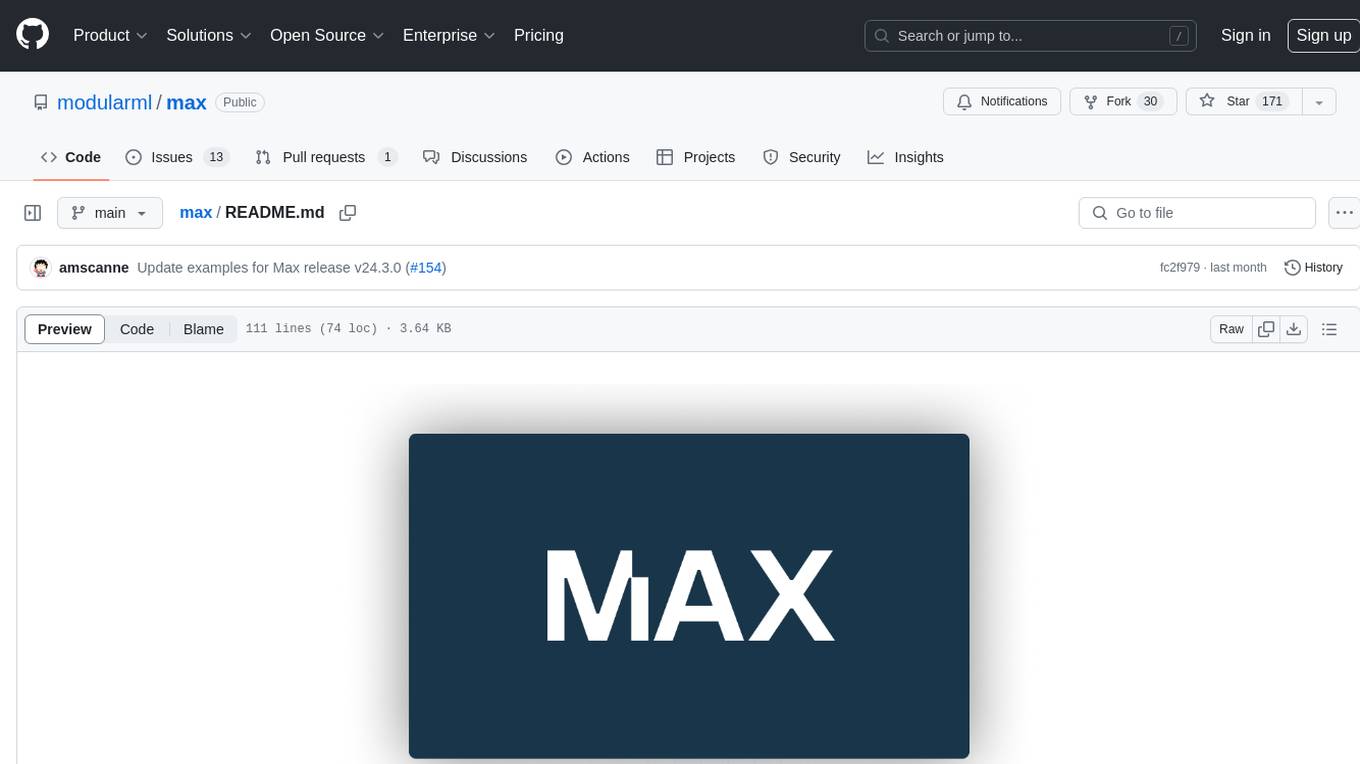
max
The Modular Accelerated Xecution (MAX) platform is an integrated suite of AI libraries, tools, and technologies that unifies commonly fragmented AI deployment workflows. MAX accelerates time to market for the latest innovations by giving AI developers a single toolchain that unlocks full programmability, unparalleled performance, and seamless hardware portability.
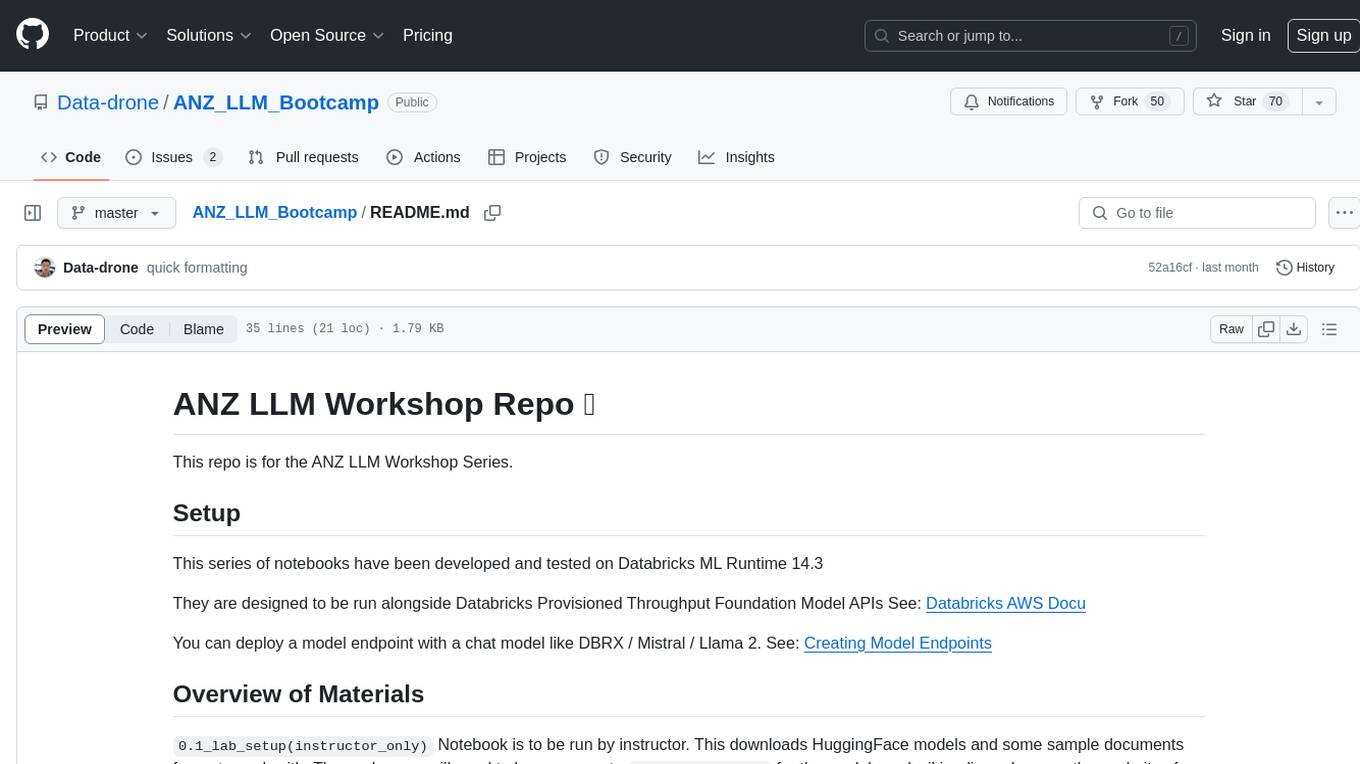
ANZ_LLM_Bootcamp
This repository is dedicated to the ANZ LLM Workshop Series, providing a series of notebooks developed and tested on Databricks ML Runtime 14.3. The notebooks cover topics such as setting up HuggingFace models, working with sample documents, constructing RAG architectures, and running applications on the driver node in Databricks. Additionally, the repository offers recordings of past webinars and further reading materials related to LLM.
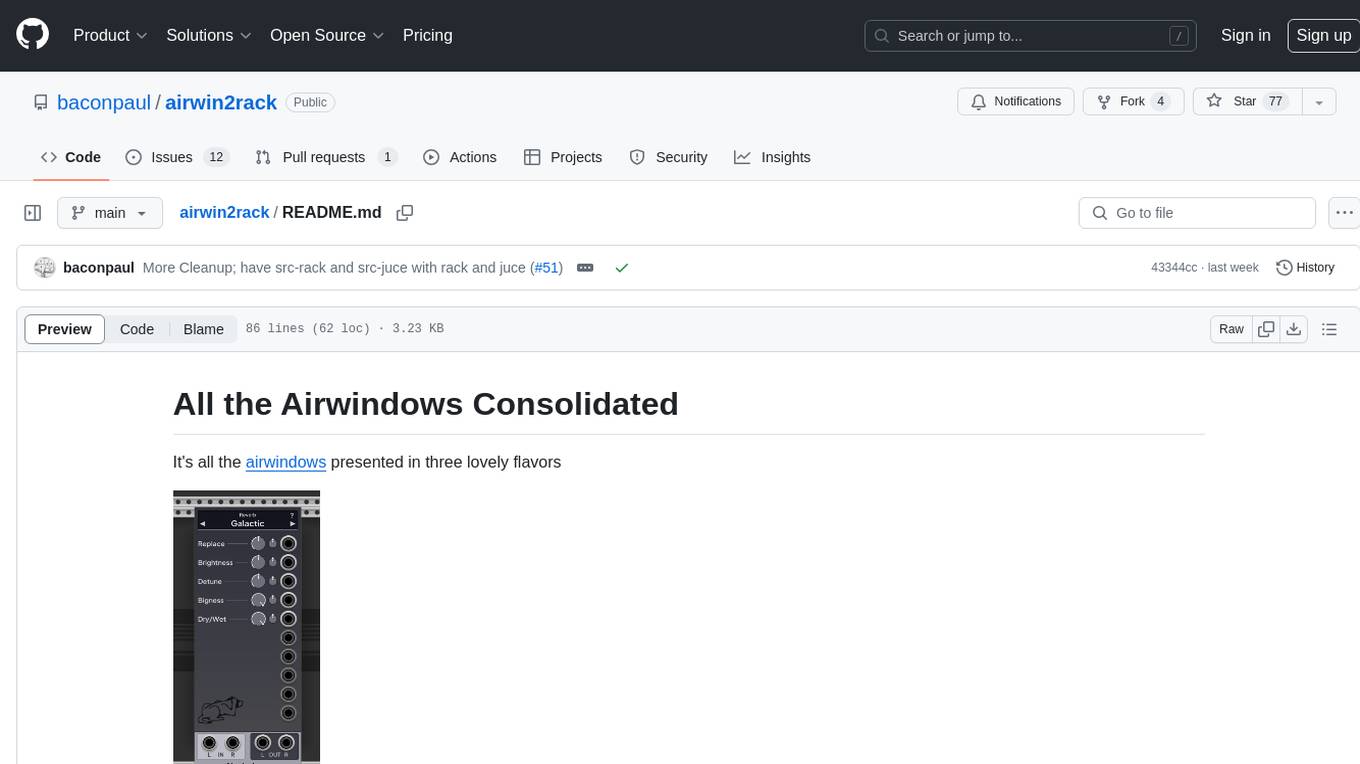
airwin2rack
The 'airwin2rack' repository is a collection of Airwindows audio plugins presented in various formats, including as a static library, a module for VCV Rack, and as CLAP/VST3/AU/LV2/Standalone plugins for DAWs. Users can access these plugins through different methods and interfaces, such as a uniform registry and access pattern, making it easy to integrate Airwindows plugins into their audio projects. The repository also provides instructions for updating the Airwindows sub-library and information on licensing, ensuring that users can utilize the plugins in both open and closed source environments.
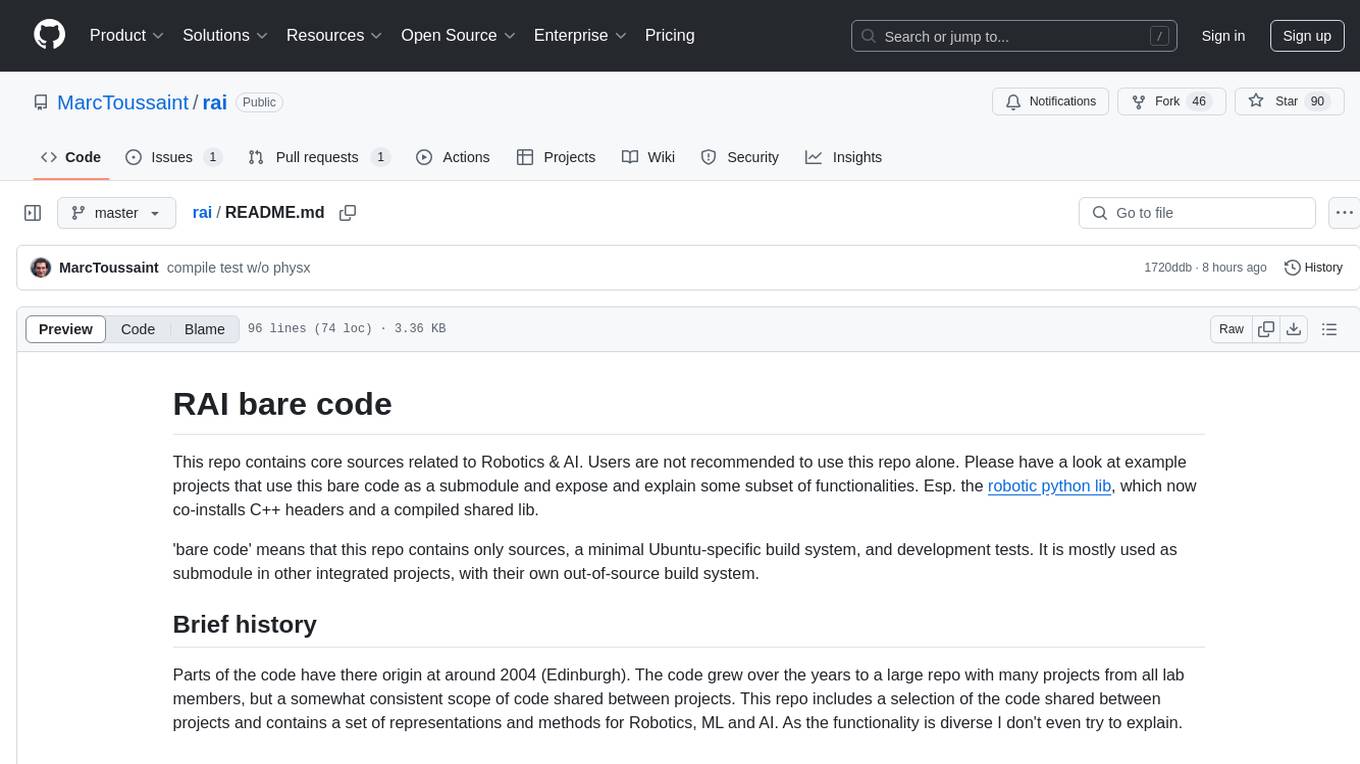
rai
This repository contains core sources related to Robotics & AI. It serves as a submodule in integrated projects, providing a minimal Ubuntu-specific build system and development tests. The code originated around 2004 in Edinburgh and has grown over the years to encompass various functionalities for Robotics, ML, and AI. Users are advised to explore example projects using this bare code for a better understanding of its capabilities.
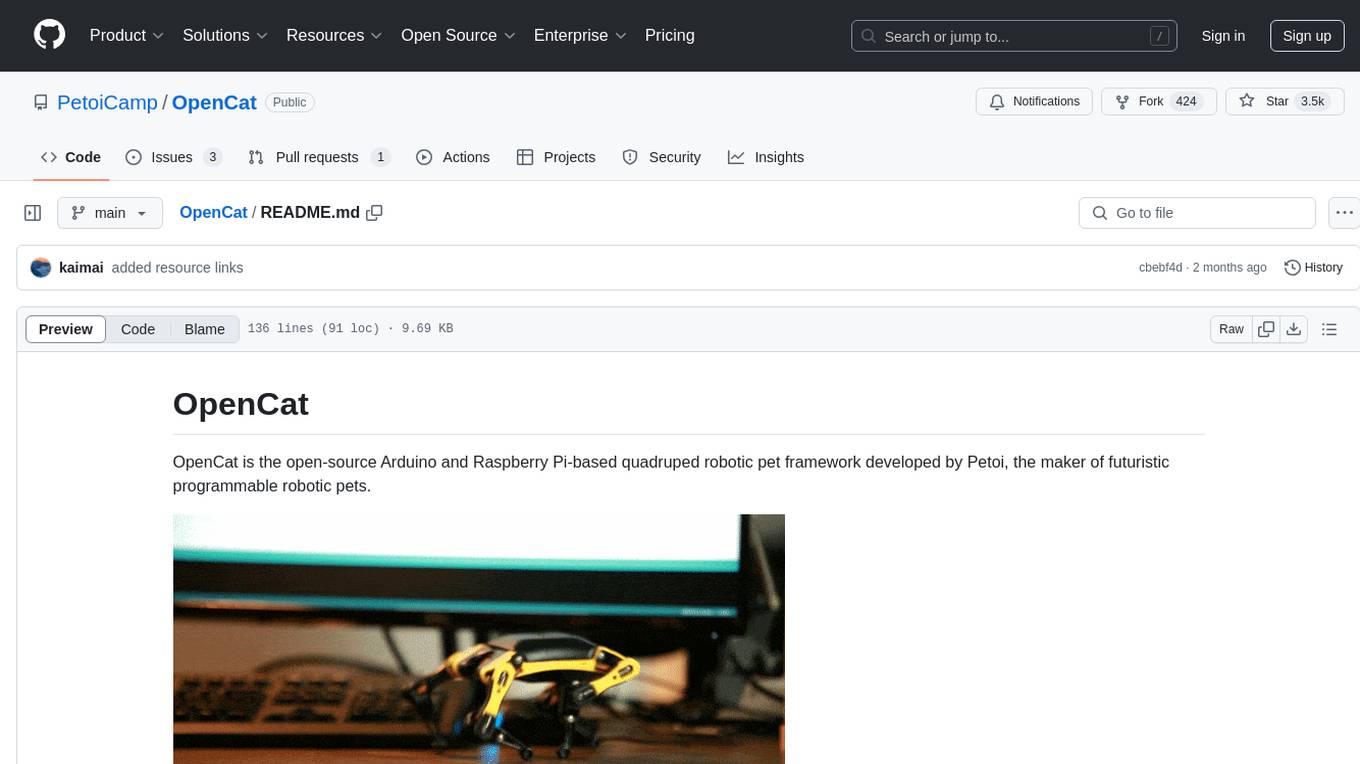
OpenCat
OpenCat is an open-source Arduino and Raspberry Pi-based quadruped robotic pet framework developed by Petoi. It aims to foster collaboration in quadruped robotics research, education, and engineering development of agile and affordable quadruped robot pets. The project provides a base open source platform for creating programmable gaits, locomotion, and deployment of inverse kinematics quadruped robots, enabling simulations to the real world via block-based coding/C/C++/Python programming languages. Users have deployed various robotics/AI/IoT applications and the project has successfully crowdfunded mini robot kits, shipped worldwide, and established a production line for affordable robotic kits and accessories.
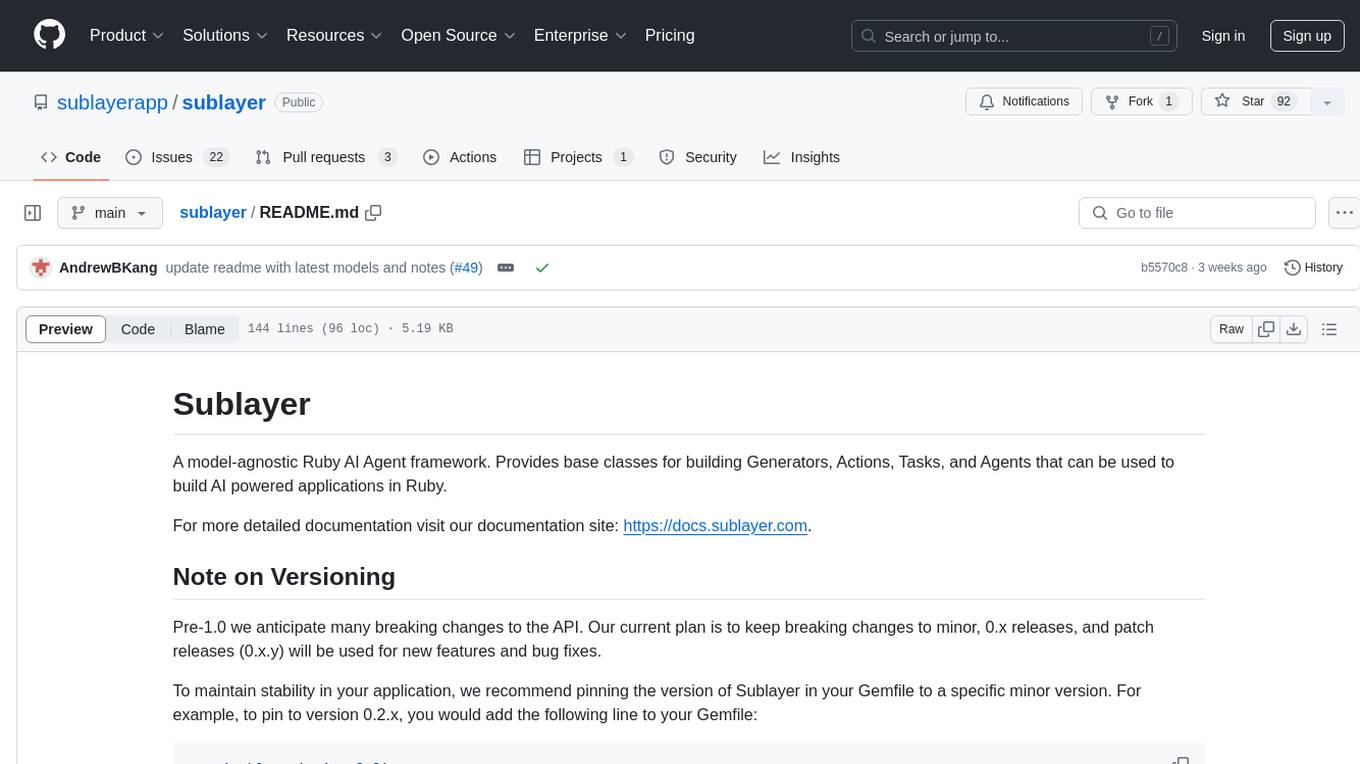
sublayer
Sublayer is a model-agnostic Ruby AI Agent framework that provides base classes for building Generators, Actions, Tasks, and Agents to create AI-powered applications in Ruby. It supports various AI models and providers, such as OpenAI, Gemini, and Claude. Generators generate specific outputs, Actions perform operations, Agents are autonomous entities for tasks or monitoring, and Triggers decide when Agents are activated. The framework offers sample Generators and usage examples for building AI applications.
For similar tasks
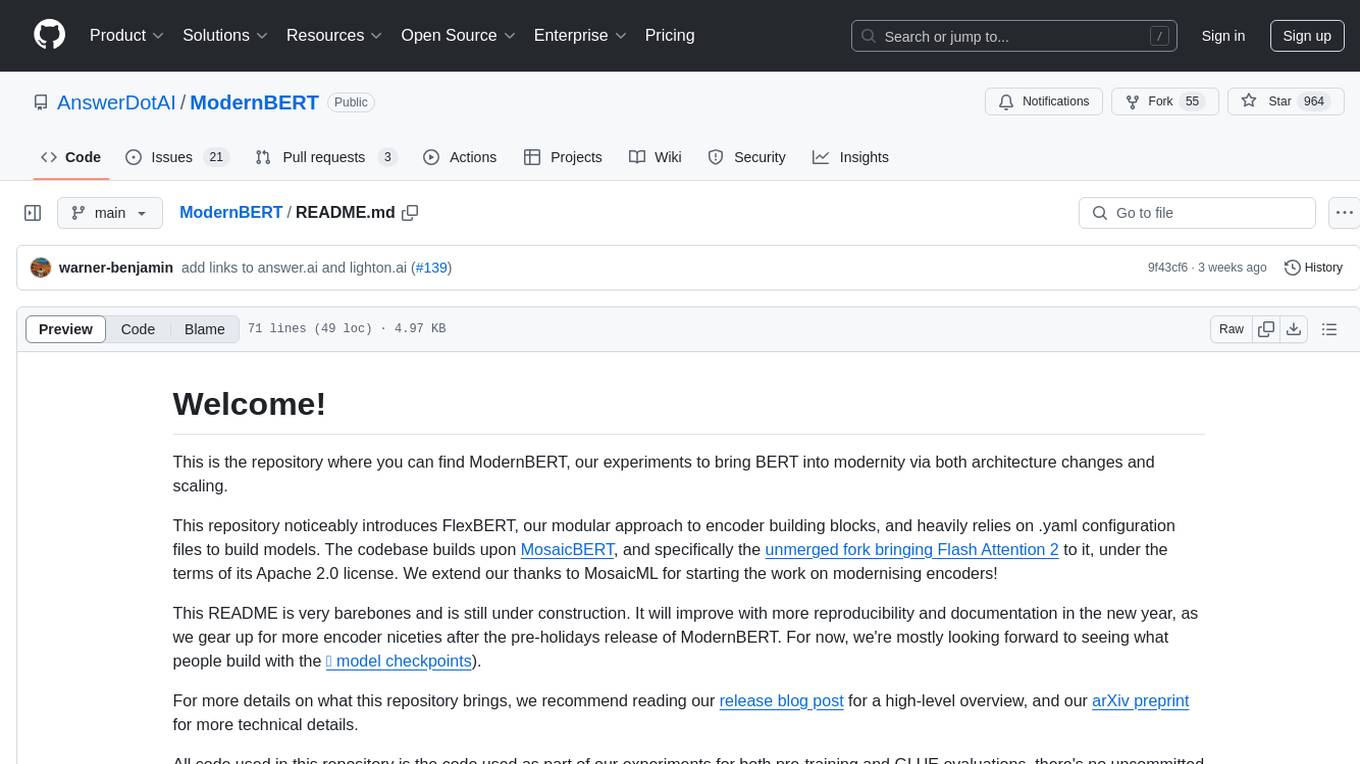
ModernBERT
ModernBERT is a repository focused on modernizing BERT through architecture changes and scaling. It introduces FlexBERT, a modular approach to encoder building blocks, and heavily relies on .yaml configuration files to build models. The codebase builds upon MosaicBERT and incorporates Flash Attention 2. The repository is used for pre-training and GLUE evaluations, with a focus on reproducibility and documentation. It provides a collaboration between Answer.AI, LightOn, and friends.
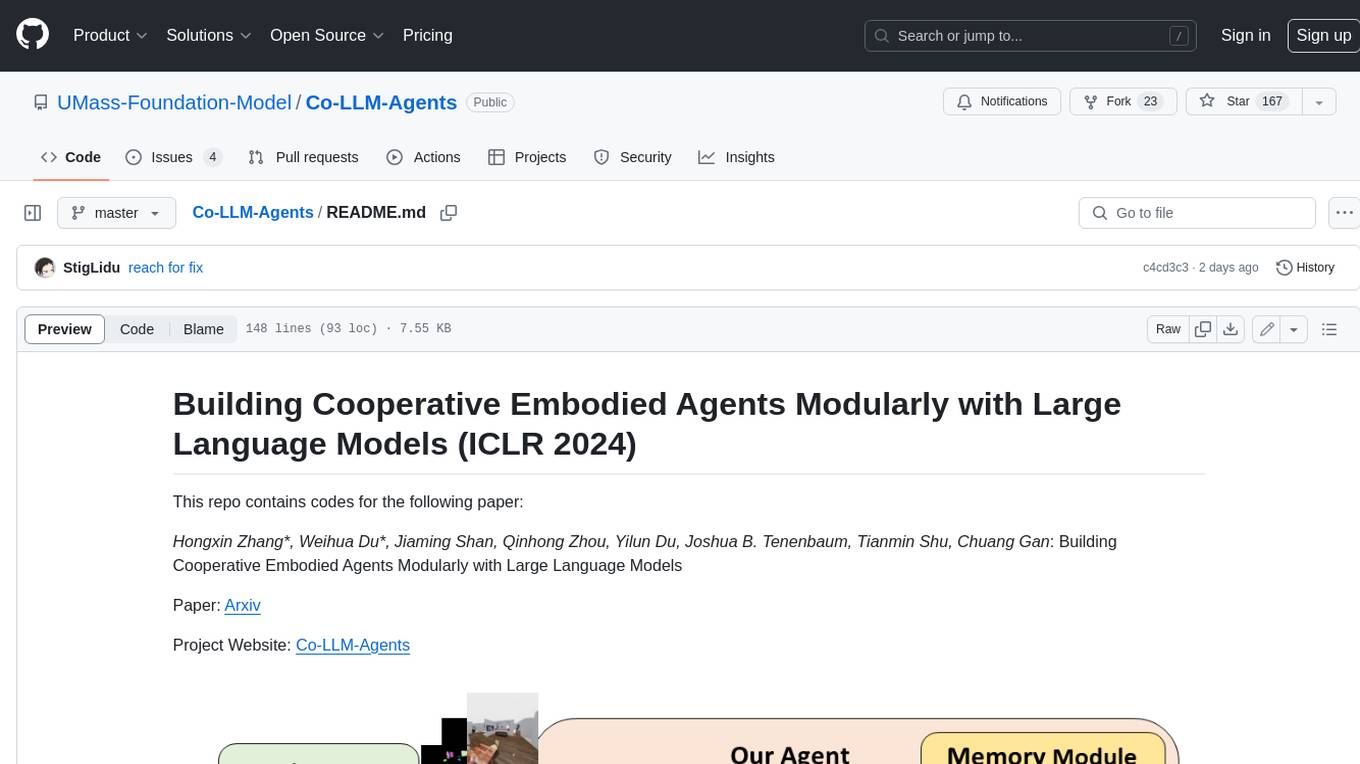
Co-LLM-Agents
This repository contains code for building cooperative embodied agents modularly with large language models. The agents are trained to perform tasks in two different environments: ThreeDWorld Multi-Agent Transport (TDW-MAT) and Communicative Watch-And-Help (C-WAH). TDW-MAT is a multi-agent environment where agents must transport objects to a goal position using containers. C-WAH is an extension of the Watch-And-Help challenge, which enables agents to send messages to each other. The code in this repository can be used to train agents to perform tasks in both of these environments.
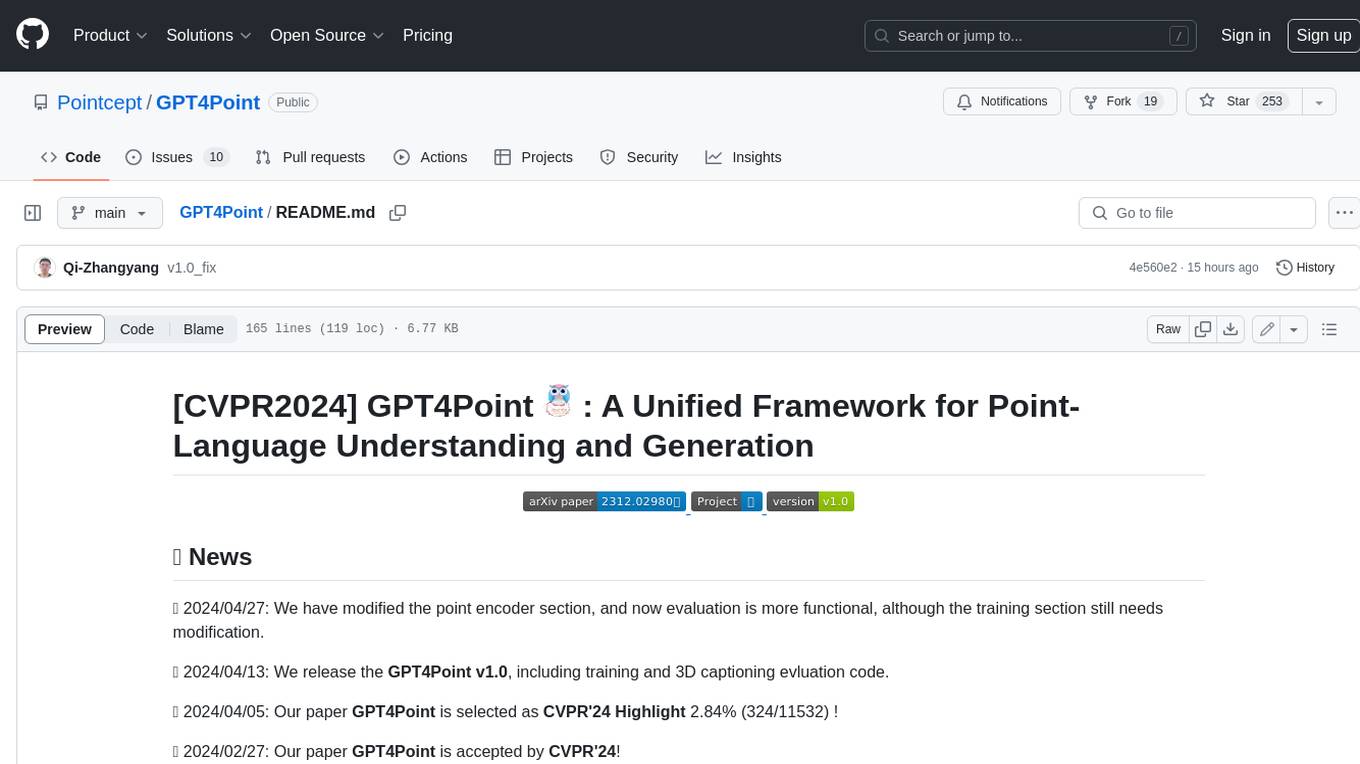
GPT4Point
GPT4Point is a unified framework for point-language understanding and generation. It aligns 3D point clouds with language, providing a comprehensive solution for tasks such as 3D captioning and controlled 3D generation. The project includes an automated point-language dataset annotation engine, a novel object-level point cloud benchmark, and a 3D multi-modality model. Users can train and evaluate models using the provided code and datasets, with a focus on improving models' understanding capabilities and facilitating the generation of 3D objects.
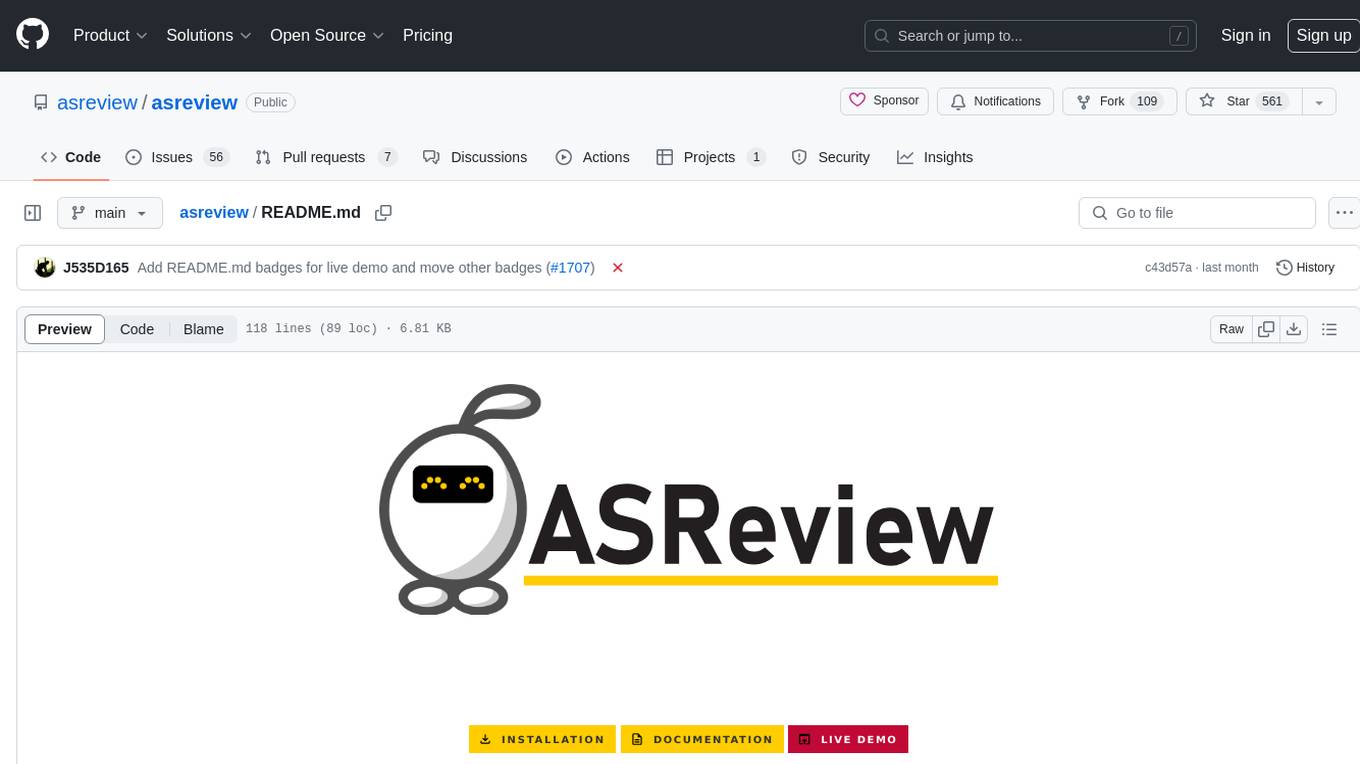
asreview
The ASReview project implements active learning for systematic reviews, utilizing AI-aided pipelines to assist in finding relevant texts for search tasks. It accelerates the screening of textual data with minimal human input, saving time and increasing output quality. The software offers three modes: Oracle for interactive screening, Exploration for teaching purposes, and Simulation for evaluating active learning models. ASReview LAB is designed to support decision-making in any discipline or industry by improving efficiency and transparency in screening large amounts of textual data.
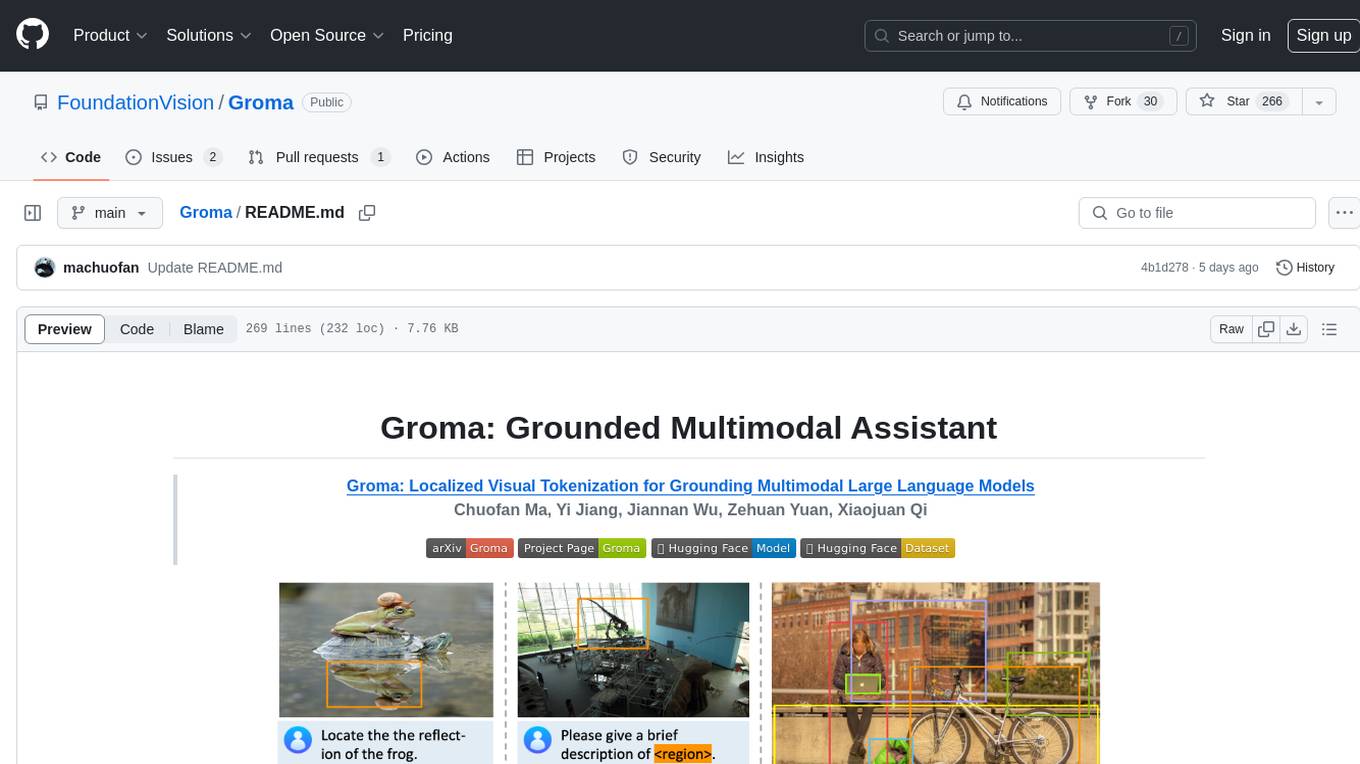
Groma
Groma is a grounded multimodal assistant that excels in region understanding and visual grounding. It can process user-defined region inputs and generate contextually grounded long-form responses. The tool presents a unique paradigm for multimodal large language models, focusing on visual tokenization for localization. Groma achieves state-of-the-art performance in referring expression comprehension benchmarks. The tool provides pretrained model weights and instructions for data preparation, training, inference, and evaluation. Users can customize training by starting from intermediate checkpoints. Groma is designed to handle tasks related to detection pretraining, alignment pretraining, instruction finetuning, instruction following, and more.
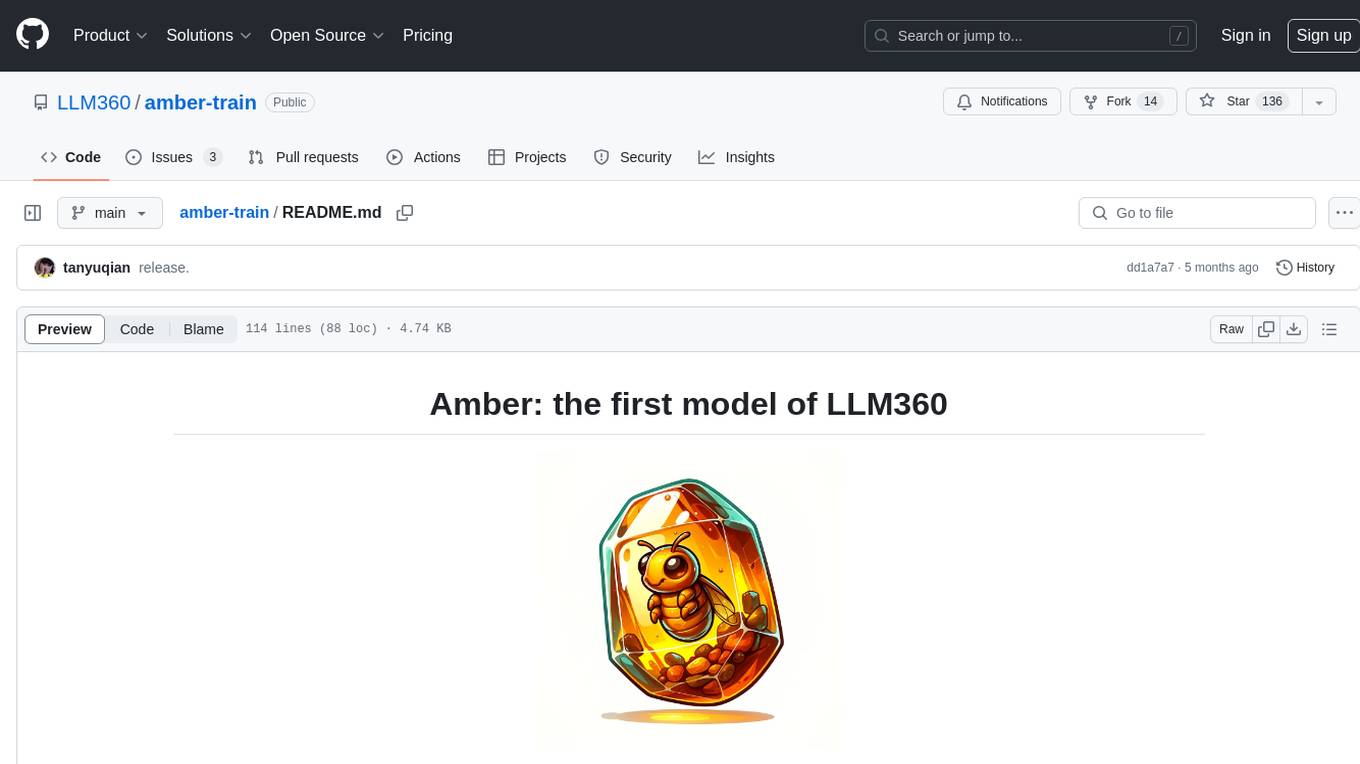
amber-train
Amber is the first model in the LLM360 family, an initiative for comprehensive and fully open-sourced LLMs. It is a 7B English language model with the LLaMA architecture. The model type is a language model with the same architecture as LLaMA-7B. It is licensed under Apache 2.0. The resources available include training code, data preparation, metrics, and fully processed Amber pretraining data. The model has been trained on various datasets like Arxiv, Book, C4, Refined-Web, StarCoder, StackExchange, and Wikipedia. The hyperparameters include a total of 6.7B parameters, hidden size of 4096, intermediate size of 11008, 32 attention heads, 32 hidden layers, RMSNorm ε of 1e^-6, max sequence length of 2048, and a vocabulary size of 32000.
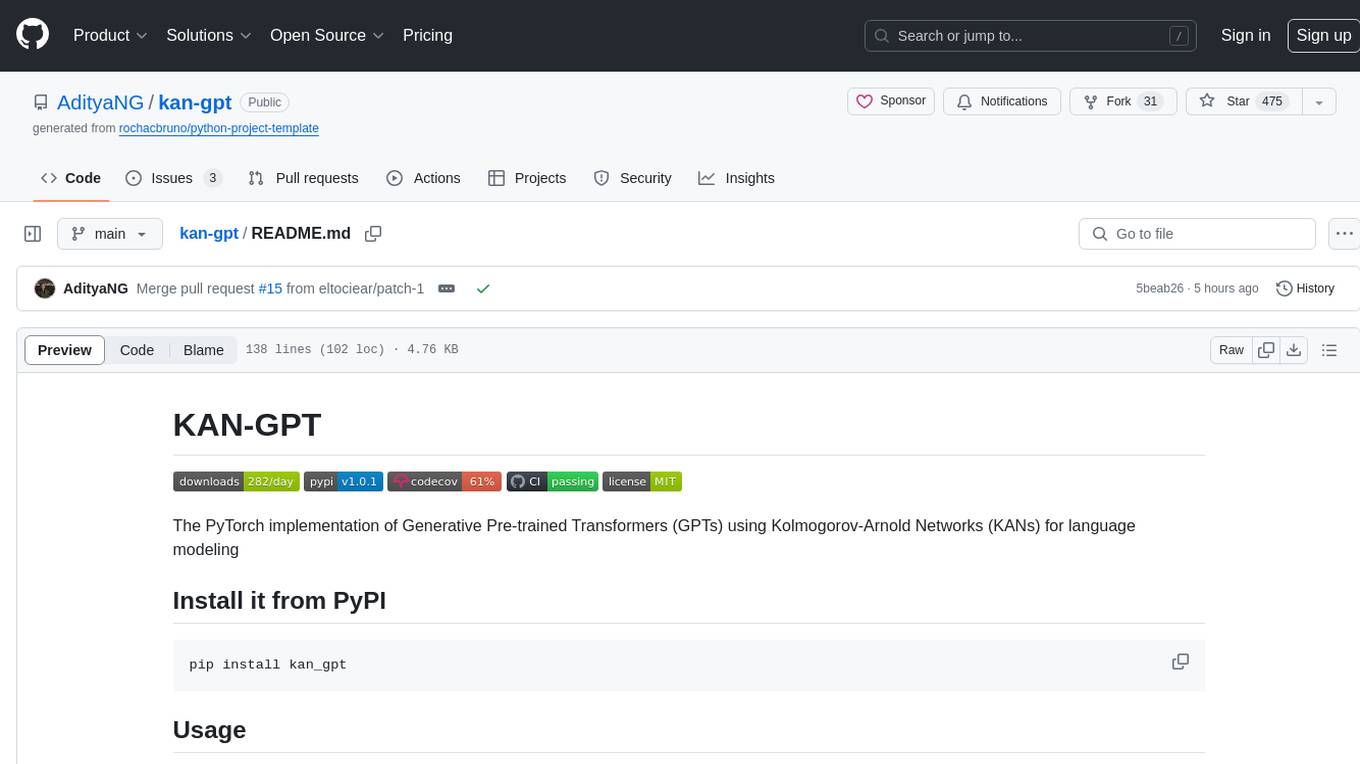
kan-gpt
The KAN-GPT repository is a PyTorch implementation of Generative Pre-trained Transformers (GPTs) using Kolmogorov-Arnold Networks (KANs) for language modeling. It provides a model for generating text based on prompts, with a focus on improving performance compared to traditional MLP-GPT models. The repository includes scripts for training the model, downloading datasets, and evaluating model performance. Development tasks include integrating with other libraries, testing, and documentation.
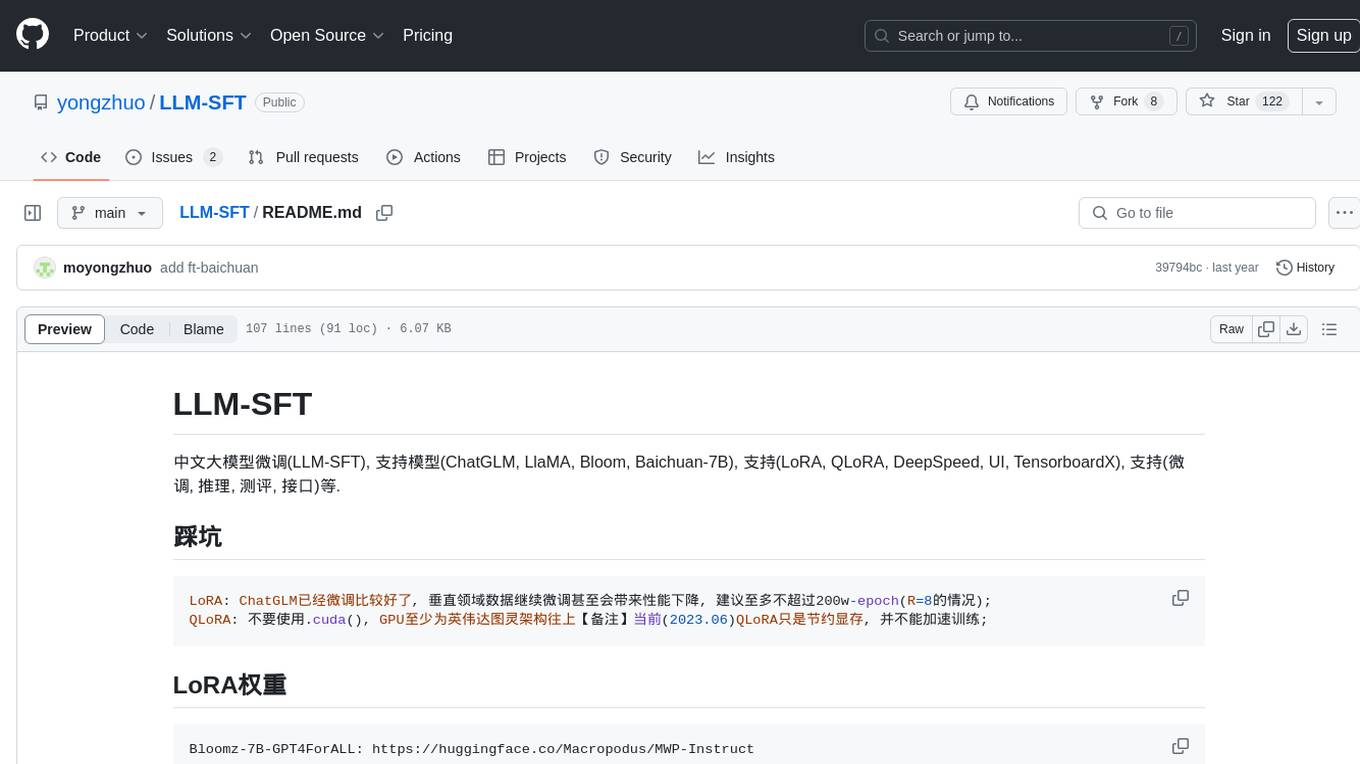
LLM-SFT
LLM-SFT is a Chinese large model fine-tuning tool that supports models such as ChatGLM, LlaMA, Bloom, Baichuan-7B, and frameworks like LoRA, QLoRA, DeepSpeed, UI, and TensorboardX. It facilitates tasks like fine-tuning, inference, evaluation, and API integration. The tool provides pre-trained weights for various models and datasets for Chinese language processing. It requires specific versions of libraries like transformers and torch for different functionalities.
For similar jobs
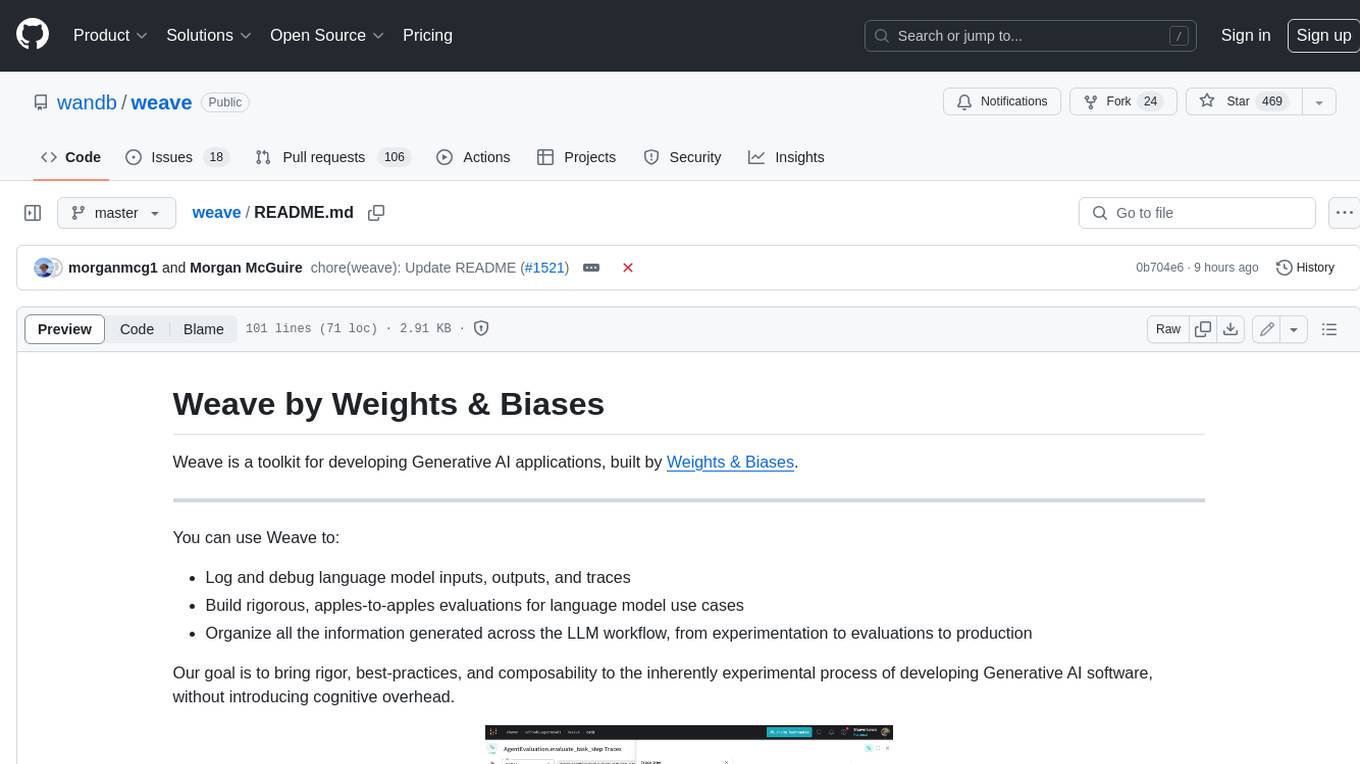
weave
Weave is a toolkit for developing Generative AI applications, built by Weights & Biases. With Weave, you can log and debug language model inputs, outputs, and traces; build rigorous, apples-to-apples evaluations for language model use cases; and organize all the information generated across the LLM workflow, from experimentation to evaluations to production. Weave aims to bring rigor, best-practices, and composability to the inherently experimental process of developing Generative AI software, without introducing cognitive overhead.
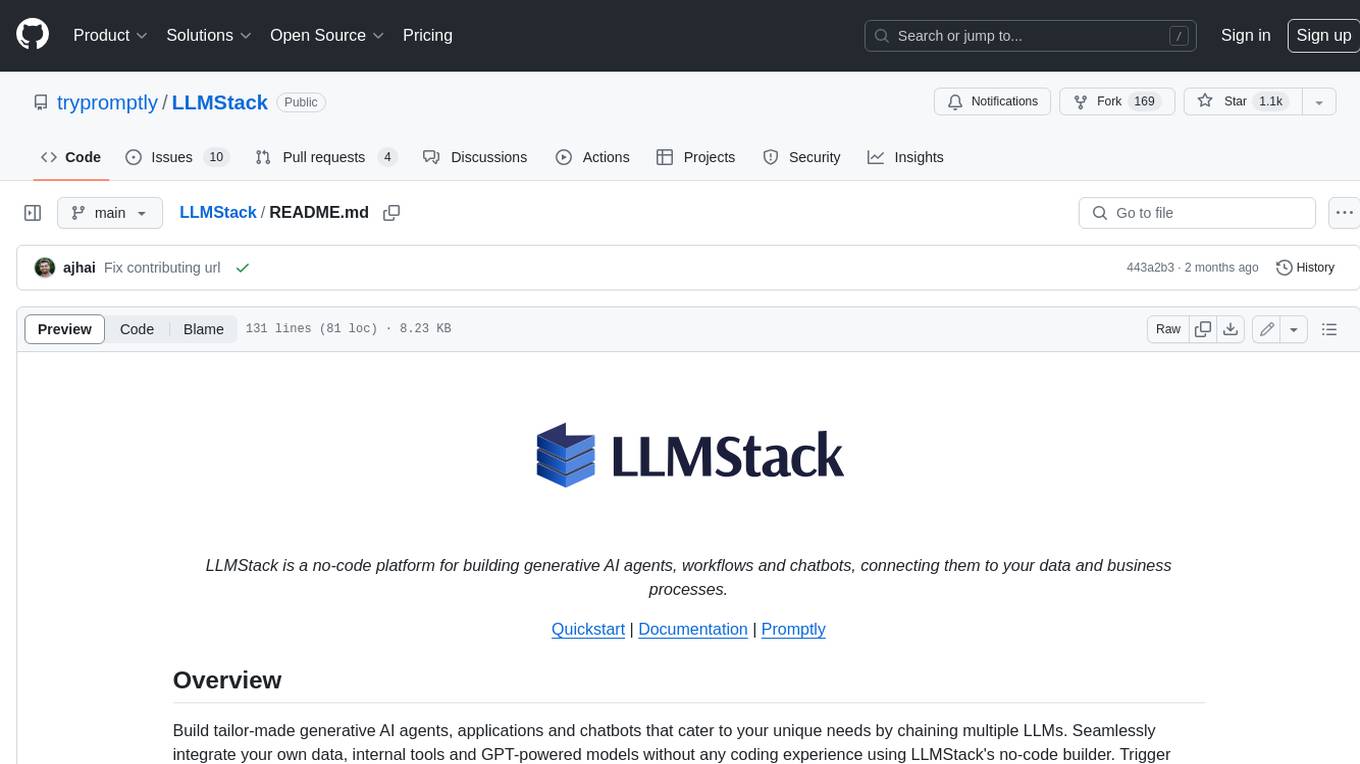
LLMStack
LLMStack is a no-code platform for building generative AI agents, workflows, and chatbots. It allows users to connect their own data, internal tools, and GPT-powered models without any coding experience. LLMStack can be deployed to the cloud or on-premise and can be accessed via HTTP API or triggered from Slack or Discord.
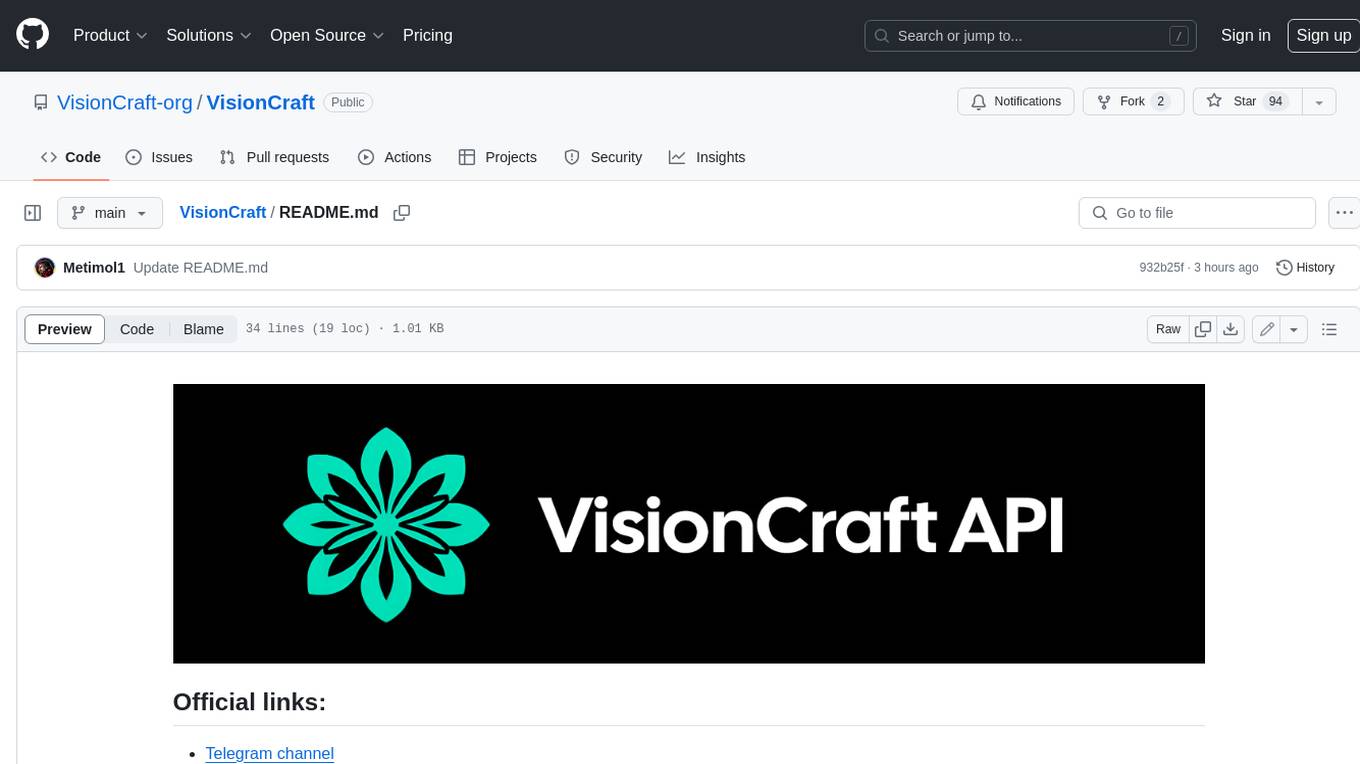
VisionCraft
The VisionCraft API is a free API for using over 100 different AI models. From images to sound.
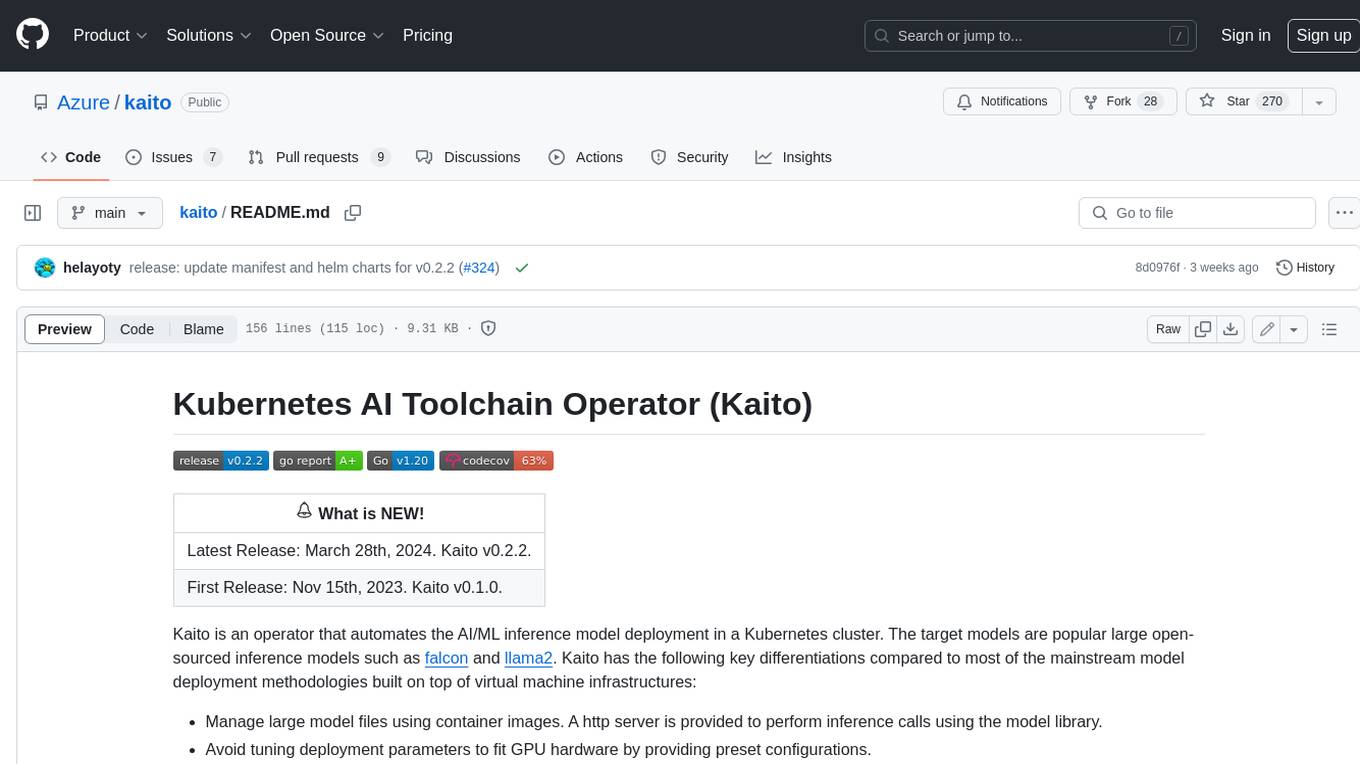
kaito
Kaito is an operator that automates the AI/ML inference model deployment in a Kubernetes cluster. It manages large model files using container images, avoids tuning deployment parameters to fit GPU hardware by providing preset configurations, auto-provisions GPU nodes based on model requirements, and hosts large model images in the public Microsoft Container Registry (MCR) if the license allows. Using Kaito, the workflow of onboarding large AI inference models in Kubernetes is largely simplified.
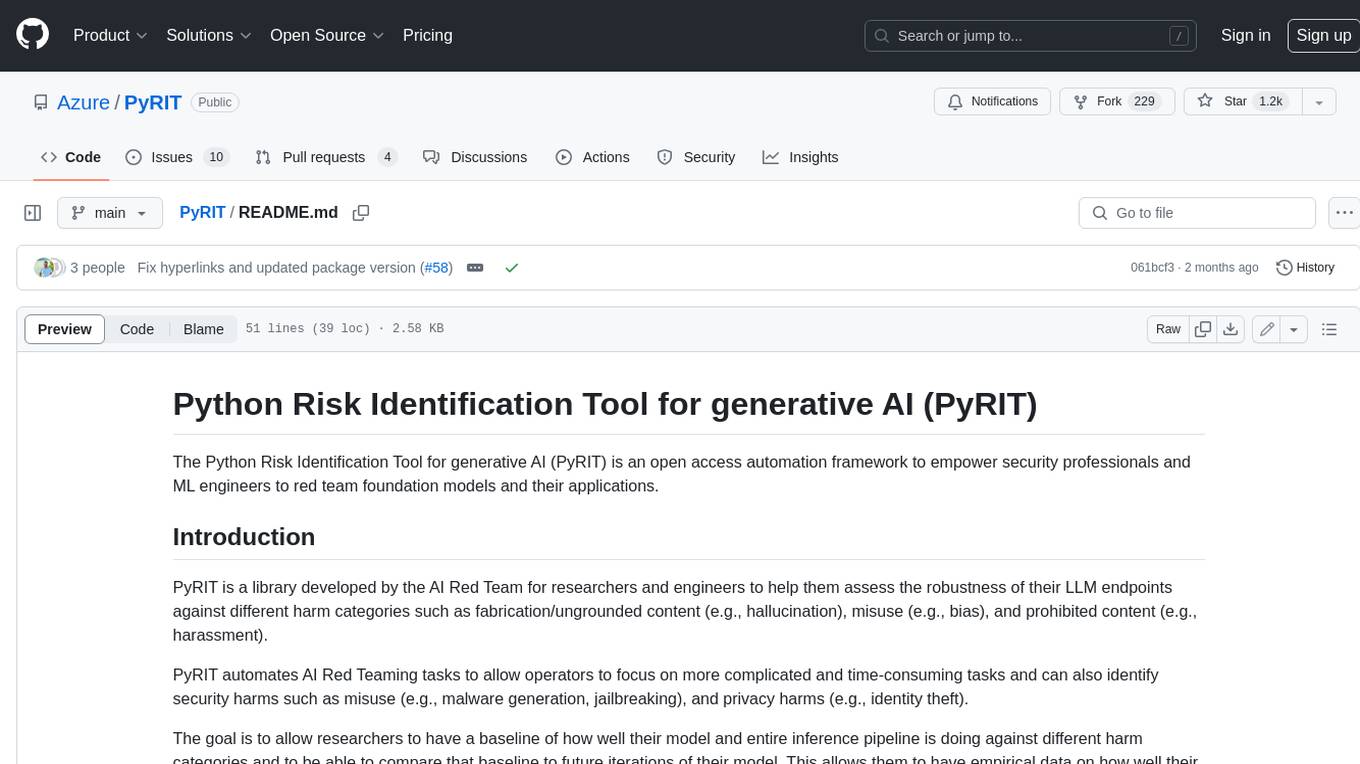
PyRIT
PyRIT is an open access automation framework designed to empower security professionals and ML engineers to red team foundation models and their applications. It automates AI Red Teaming tasks to allow operators to focus on more complicated and time-consuming tasks and can also identify security harms such as misuse (e.g., malware generation, jailbreaking), and privacy harms (e.g., identity theft). The goal is to allow researchers to have a baseline of how well their model and entire inference pipeline is doing against different harm categories and to be able to compare that baseline to future iterations of their model. This allows them to have empirical data on how well their model is doing today, and detect any degradation of performance based on future improvements.
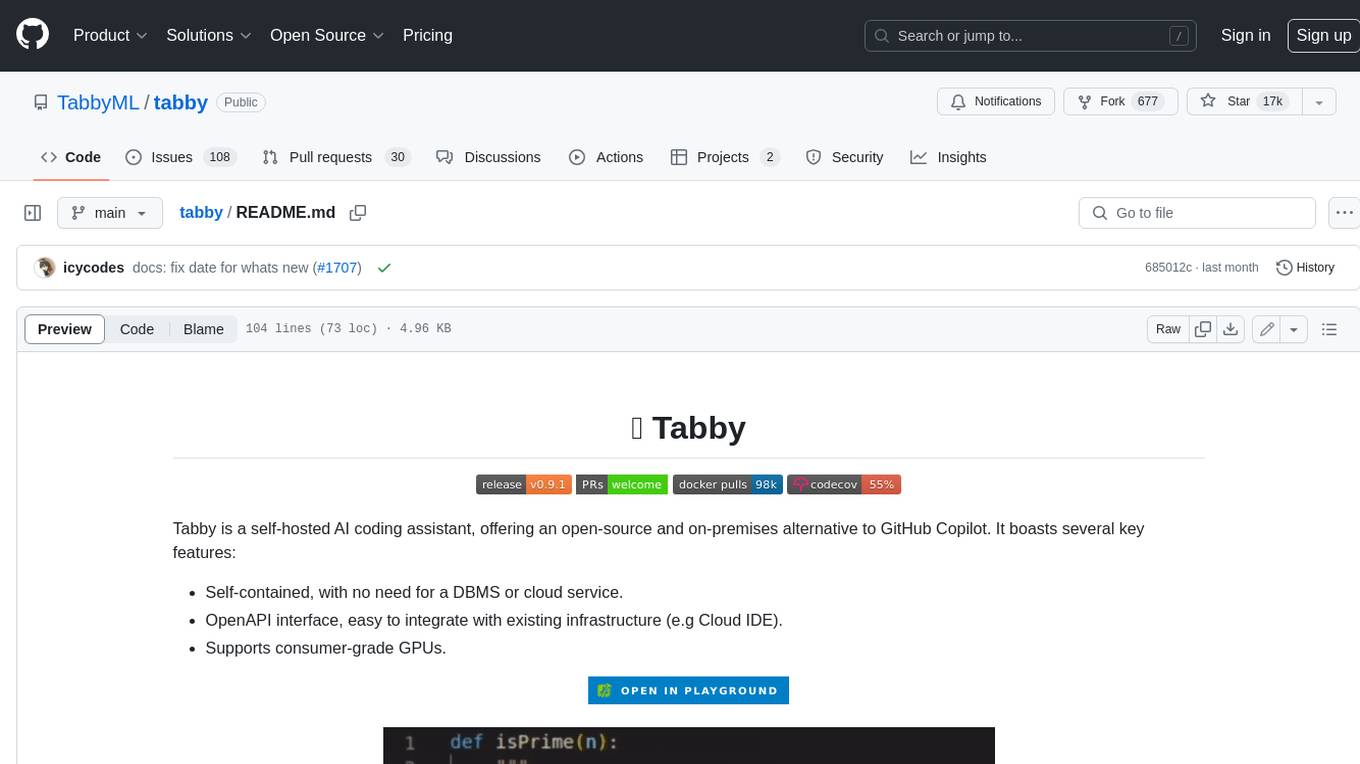
tabby
Tabby is a self-hosted AI coding assistant, offering an open-source and on-premises alternative to GitHub Copilot. It boasts several key features: * Self-contained, with no need for a DBMS or cloud service. * OpenAPI interface, easy to integrate with existing infrastructure (e.g Cloud IDE). * Supports consumer-grade GPUs.
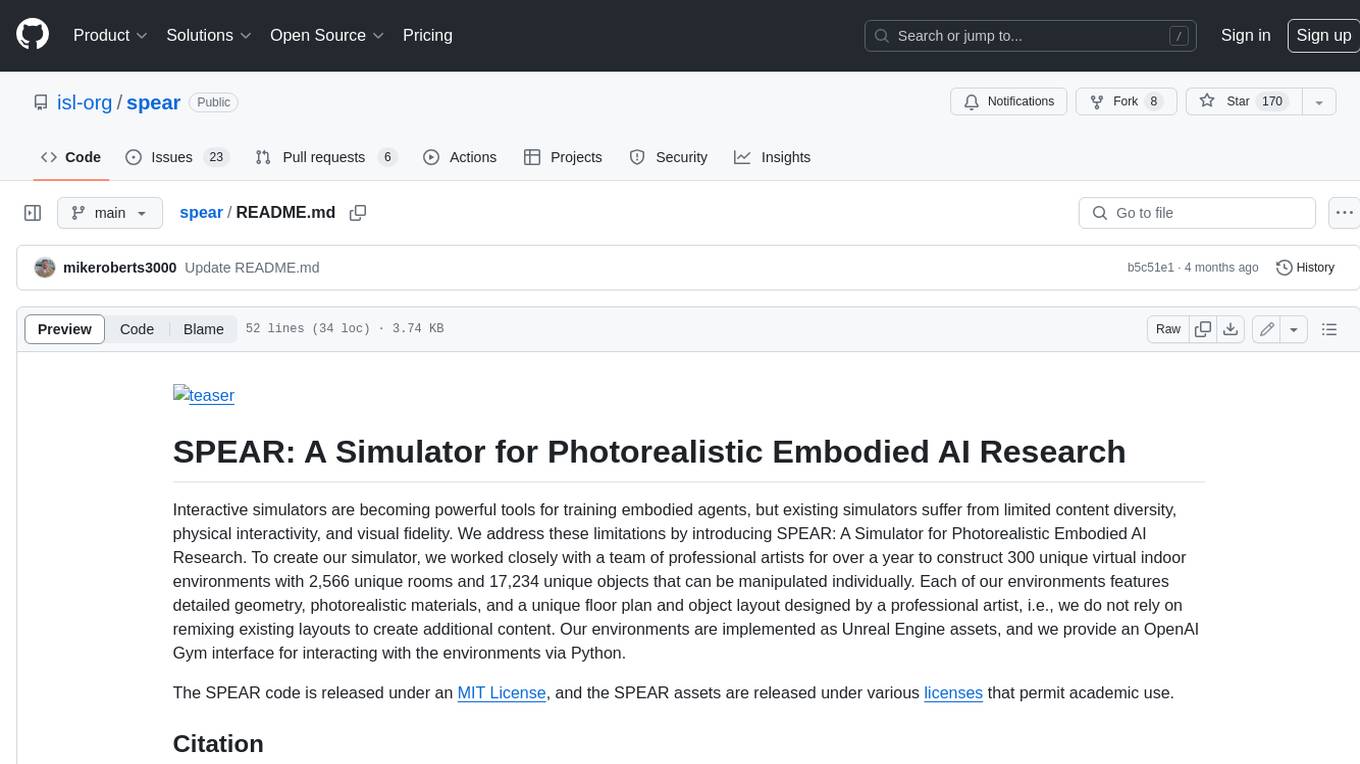
spear
SPEAR (Simulator for Photorealistic Embodied AI Research) is a powerful tool for training embodied agents. It features 300 unique virtual indoor environments with 2,566 unique rooms and 17,234 unique objects that can be manipulated individually. Each environment is designed by a professional artist and features detailed geometry, photorealistic materials, and a unique floor plan and object layout. SPEAR is implemented as Unreal Engine assets and provides an OpenAI Gym interface for interacting with the environments via Python.
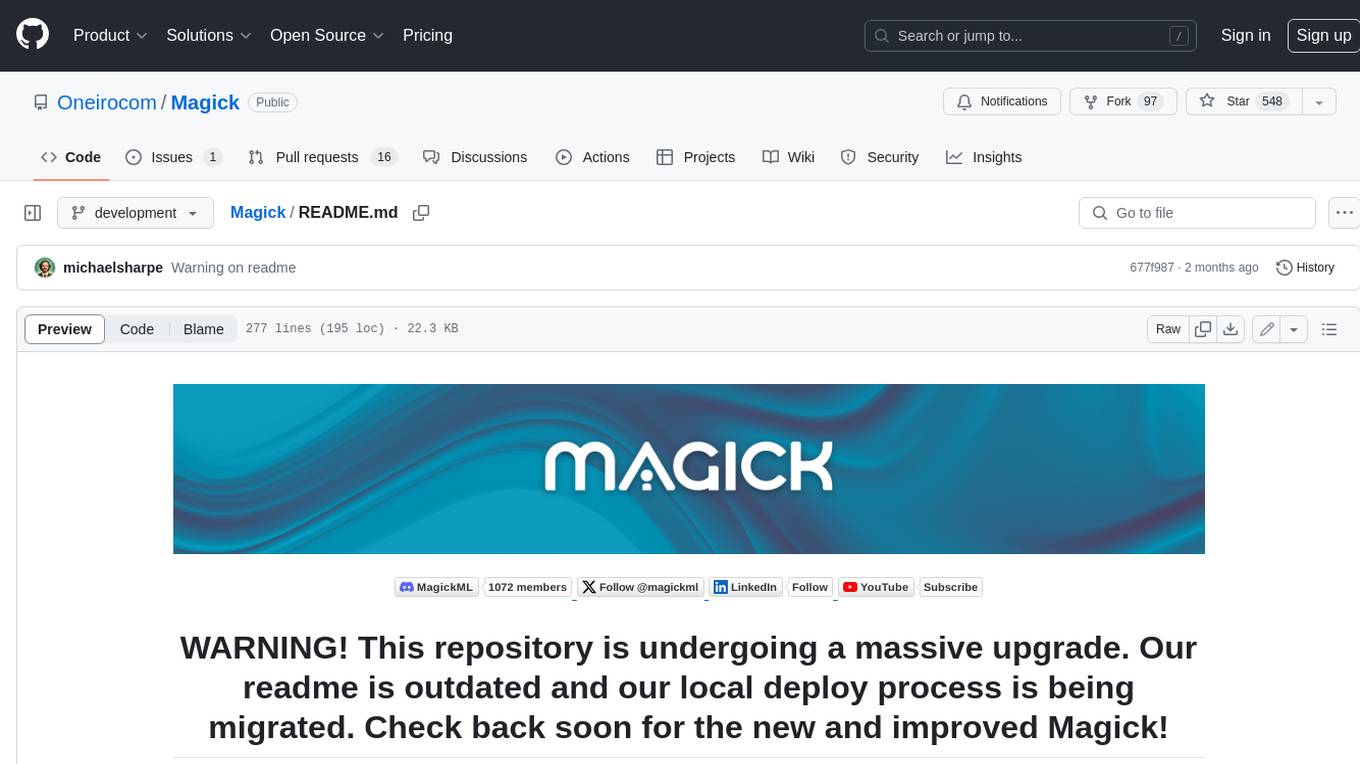
Magick
Magick is a groundbreaking visual AIDE (Artificial Intelligence Development Environment) for no-code data pipelines and multimodal agents. Magick can connect to other services and comes with nodes and templates well-suited for intelligent agents, chatbots, complex reasoning systems and realistic characters.