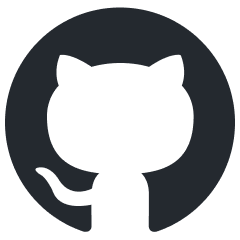
gpdb
Greenplum Database - Massively Parallel PostgreSQL for Analytics. An open-source massively parallel data platform for analytics, machine learning and AI.
Stars: 6221
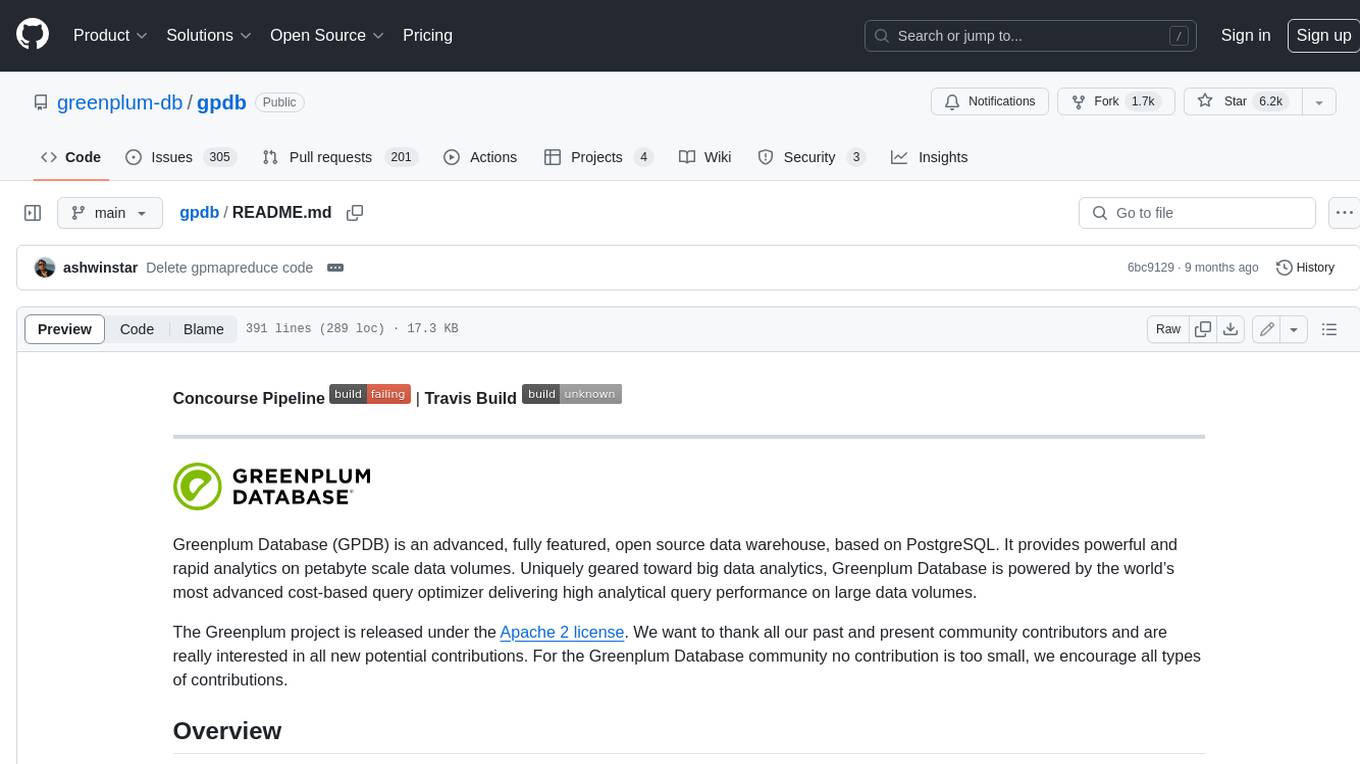
Greenplum Database (GPDB) is an advanced, fully featured, open source data warehouse, based on PostgreSQL. It provides powerful and rapid analytics on petabyte scale data volumes. Uniquely geared toward big data analytics, Greenplum Database is powered by the world’s most advanced cost-based query optimizer delivering high analytical query performance on large data volumes.
README:
Concourse Pipeline |
Travis Build
Greenplum Database (GPDB) is an advanced, fully featured, open source data warehouse, based on PostgreSQL. It provides powerful and rapid analytics on petabyte scale data volumes. Uniquely geared toward big data analytics, Greenplum Database is powered by the world’s most advanced cost-based query optimizer delivering high analytical query performance on large data volumes.
The Greenplum project is released under the Apache 2 license. We want to thank all our past and present community contributors and are really interested in all new potential contributions. For the Greenplum Database community no contribution is too small, we encourage all types of contributions.
A Greenplum cluster consists of a coordinator server, and multiple segment servers. All user data resides in the segments, the coordinator contains only metadata. The coordinator server, and all the segments, share the same schema.
Users always connect to the coordinator server, which divides up the query into fragments that are executed in the segments, and collects the results.
More information can be found on the project website.
GPORCA is a cost-based optimizer which is used by Greenplum Database in conjunction with the PostgreSQL planner. It is also known as just ORCA, and Pivotal Optimizer. The code for GPORCA resides src/backend/gporca. It is built automatically by default.
Follow these macOS steps for getting your system ready for GPDB
Follow appropriate linux steps for getting your system ready for GPDB
# Initialize and update submodules in the repository
git submodule update --init
# Configure build environment to install at /usr/local/gpdb
./configure --with-perl --with-python --with-libxml --with-gssapi --prefix=/usr/local/gpdb
# Compile and install
make -j8
make -j8 install
# Bring in greenplum environment into your running shell
source /usr/local/gpdb/greenplum_path.sh
# Start demo cluster
make create-demo-cluster
# (gpdemo-env.sh contains __PGPORT__ and __COORDINATOR_DATA_DIRECTORY__ values)
source gpAux/gpdemo/gpdemo-env.sh
The directory, the TCP ports, the number of segments, and the existence of
standbys for segments and coordinator for the demo cluster can be changed
on the fly.
Instead of make create-demo-cluster
, consider:
DATADIRS=/tmp/gpdb-cluster PORT_BASE=5555 NUM_PRIMARY_MIRROR_PAIRS=1 WITH_MIRRORS=false make create-demo-cluster
The TCP port for the regression test can be changed on the fly:
PGPORT=5555 make installcheck-world
To turn GPORCA off and use Postgres planner for query optimization:
set optimizer=off;
If you want to clean all generated files
make distclean
- The default regression tests
make installcheck-world
-
The top-level target installcheck-world will run all regression tests in GPDB against the running cluster. For testing individual parts, the respective targets can be run separately.
-
The PostgreSQL check target does not work. Setting up a Greenplum cluster is more complicated than a single-node PostgreSQL installation, and no-one's done the work to have make check create a cluster. Create a cluster manually or use gpAux/gpdemo/ (example below) and run the toplevel make installcheck-world against that. Patches are welcome!
-
The PostgreSQL installcheck target does not work either, because some tests are known to fail with Greenplum. The installcheck-good schedule in src/test/regress excludes those tests.
-
When adding a new test, please add it to one of the GPDB-specific tests, in greenplum_schedule, rather than the PostgreSQL tests inherited from the upstream. We try to keep the upstream tests identical to the upstream versions, to make merging with newer PostgreSQL releases easier.
Currently, GPDB is built with GPORCA by default. If you want to build GPDB
without GPORCA, configure requires --disable-orca
flag to be set.
# Clean environment
make distclean
# Configure build environment to install at /usr/local/gpdb
./configure --disable-orca --with-perl --with-python --with-libxml --prefix=/usr/local/gpdb
GPDB supports Python3 with plpython3u UDF
See how to enable Python3 for details.
See Building GPDB client tools on Windows for details.
There is a Vagrant-based quickstart guide for developers.
The directory layout of the repository follows the same general layout as upstream PostgreSQL. There are changes compared to PostgreSQL throughout the codebase, but a few larger additions worth noting:
-
gpMgmt/
Contains Greenplum-specific command-line tools for managing the cluster. Scripts like gpinit, gpstart, gpstop live here. They are mostly written in Python.
-
gpAux/
Contains Greenplum-specific release management scripts, and vendored dependencies. Some additional directories are submodules and will be made available over time.
-
gpcontrib/
Much like the PostgreSQL contrib/ directory, this directory contains extensions such as gpfdist which are Greenplum-specific.
-
doc/
In PostgreSQL, the user manual lives here. In Greenplum, the user manual is maintained separately and only the reference pages used to build man pages are here.
-
gpdb-doc/
Contains the Greenplum documentation in DITA XML format. Refer to
gpdb-doc/README.md
for information on how to build, and work with the documentation. -
ci/
Contains configuration files for the GPDB continuous integration system.
-
src/backend/cdb/
Contains larger Greenplum-specific backend modules. For example, communication between segments, turning plans into parallelizable plans, mirroring, distributed transaction and snapshot management, etc. cdb stands for Cluster Database - it was a workname used in the early days. That name is no longer used, but the cdb prefix remains.
-
src/backend/gpopt/
Contains the so-called translator library, for using the GPORCA optimizer with Greenplum. The translator library is written in C++ code, and contains glue code for translating plans and queries between the DXL format used by GPORCA, and the PostgreSQL internal representation.
-
src/backend/gporca/
Contains the GPORCA optimizer code and tests. This is written in C++. See README.md for more information and how to unit-test GPORCA.
-
src/backend/fts/
FTS is a process that runs in the coordinator node, and periodically polls the segments to maintain the status of each segment.
Greenplum is maintained by a core team of developers with commit rights to the main gpdb repository on GitHub. At the same time, we are very eager to receive contributions from anybody in the wider Greenplum community. This section covers all you need to know if you want to see your code or documentation changes be added to Greenplum and appear in the future releases.
Greenplum is developed on GitHub, and anybody wishing to contribute to it will have to have a GitHub account and be familiar with Git tools and workflow. It is also recommend that you follow the developer's mailing list since some of the contributions may generate more detailed discussions there.
Once you have your GitHub account, fork this repository so that you can have your private copy to start hacking on and to use as source of pull requests.
Anybody contributing to Greenplum has to be covered by either the Corporate or the Individual Contributor License Agreement. If you have not previously done so, please fill out and submit the Contributor License Agreement. Note that we do allow for really trivial changes to be contributed without a CLA if they fall under the rubric of obvious fixes. However, since our GitHub workflow checks for CLA by default you may find it easier to submit one instead of claiming an "obvious fix" exception.
If the contribution you're submitting is original work, you can assume that Pivotal will release it as part of an overall Greenplum release available to the downstream consumers under the Apache License, Version 2.0. However, in addition to that, Pivotal may also decide to release it under a different license (such as PostgreSQL License to the upstream consumers that require it. A typical example here would be Pivotal upstreaming your contribution back to PostgreSQL community (which can be done either verbatim or your contribution being upstreamed as part of the larger changeset).
If the contribution you're submitting is NOT original work you have to indicate the name of the license and also make sure that it is similar in terms to the Apache License 2.0. Apache Software Foundation maintains a list of these licenses under Category A. In addition to that, you may be required to make proper attribution in the NOTICE file similar to these examples.
Finally, keep in mind that it is NEVER a good idea to remove licensing headers from the work that is not your original one. Even if you are using parts of the file that originally had a licensing header at the top you should err on the side of preserving it. As always, if you are not quite sure about the licensing implications of your contributions, feel free to reach out to us on the developer mailing list.
Your chances of getting feedback and seeing your code merged into the project greatly depend on how granular your changes are. If you happen to have a bigger change in mind, we highly recommend engaging on the developer's mailing list first and sharing your proposal with us before you spend a lot of time writing code. Even when your proposal gets validated by the community, we still recommend doing the actual work as a series of small, self-contained commits. This makes the reviewer's job much easier and increases the timeliness of feedback.
When it comes to C and C++ parts of Greenplum, we try to follow PostgreSQL Coding Conventions. In addition to that we require that:
We recommend using git diff --color
when reviewing your changes so that you
don't have any spurious whitespace issues in the code that you submit.
All new functionality that is contributed to Greenplum should be covered by regression tests that are contributed alongside it. If you are uncertain on how to test or document your work, please raise the question on the gpdb-dev mailing list and the developer community will do its best to help you.
At the very minimum you should always be running
make installcheck-world
to make sure that you're not breaking anything.
If the change you're working on touches functionality that is common between PostgreSQL and Greenplum, you may be asked to forward-port it to PostgreSQL. This is not only so that we keep reducing the delta between the two projects, but also so that any change that is relevant to PostgreSQL can benefit from a much broader review of the upstream PostgreSQL community. In general, it is a good idea to keep both code bases handy so you can be sure whether your changes may need to be forward-ported.
To improve the odds of the right discussion of your patch or idea happening, pay attention to what the community work cycle is. For example, if you send in a brand new idea in the beta phase of a release, we may defer review or target its inclusion for a later version. Feel free to ask on the mailing list to learn more about the Greenplum release policy and timing.
Once you are ready to share your work with the Greenplum core team and the rest of the Greenplum community, you should push all the commits to a branch in your own repository forked from the official Greenplum and send us a pull request.
We welcome submissions which are work in-progress in order to get feedback early in the development process. When opening the pull request, select "Draft" in the dropdown menu when creating the PR to clearly mark the intent of the pull request. Prefixing the title with "WIP:" is also good practice.
All new features should be submitted against the main branch. Bugfixes should too be submitted against main unless they only exist in a supported back-branch. If the bug exists in both main and back-branches, explain this in the PR description.
Once you submit your pull request, you will immediately see a number of validation checks performed by our automated CI pipelines. There also will be a CLA check telling you whether your CLA was recognized. If any of these checks fails, you will need to update your pull request to take care of the issue. Pull requests with failed validation checks are very unlikely to receive any further peer review from the community members.
Keep in mind that the most common reason for a failed CLA check is a mismatch between an email on file and an email recorded in the commits submitted as part of the pull request.
If you cannot figure out why a certain validation check failed, feel free to ask on the developer's mailing list, but make sure to include a direct link to a pull request in your email.
A submitted pull request with passing validation checks is assumed to be available for peer review. Peer review is the process that ensures that contributions to Greenplum are of high quality and align well with the road map and community expectations. Every member of the Greenplum community is encouraged to review pull requests and provide feedback. Since you don't have to be a core team member to be able to do that, we recommend following a stream of pull reviews to anybody who's interested in becoming a long-term contributor to Greenplum. As Linus would say "given enough eyeballs, all bugs are shallow".
One outcome of the peer review could be a consensus that you need to modify your pull request in certain ways. GitHub allows you to push additional commits into a branch from which a pull request was sent. Those additional commits will be then visible to all of the reviewers.
A peer review converges when it receives at least one +1 and no -1s votes from the participants. At that point you should expect one of the core team members to pull your changes into the project.
Greenplum prides itself on being a collaborative, consensus-driven environment. We do not believe in vetoes and any -1 vote casted as part of the peer review has to have a detailed technical explanation of what's wrong with the change. Should a strong disagreement arise it may be advisable to take the matter onto the mailing list since it allows for a more natural flow of the conversation.
At any time during the patch review, you may experience delays based on the availability of reviewers and core team members. Please be patient. That being said, don't get discouraged either. If you're not getting expected feedback for a few days add a comment asking for updates on the pull request itself or send an email to the mailing list.
On occasion you will see core team members committing directly to the repository without going through the pull request workflow. This is reserved for small changes only and the rule of thumb we use is this: if the change touches any functionality that may result in a test failure, then it has to go through a pull request workflow. If, on the other hand, the change is in the non-functional part of the code base (such as fixing a typo inside of a comment block) core team members can decide to just commit to the repository directly.
For Greenplum Database documentation, please check the online documentation.
For further information beyond the scope of this README, please see our wiki
For Tasks:
Click tags to check more tools for each tasksFor Jobs:
Alternative AI tools for gpdb
Similar Open Source Tools
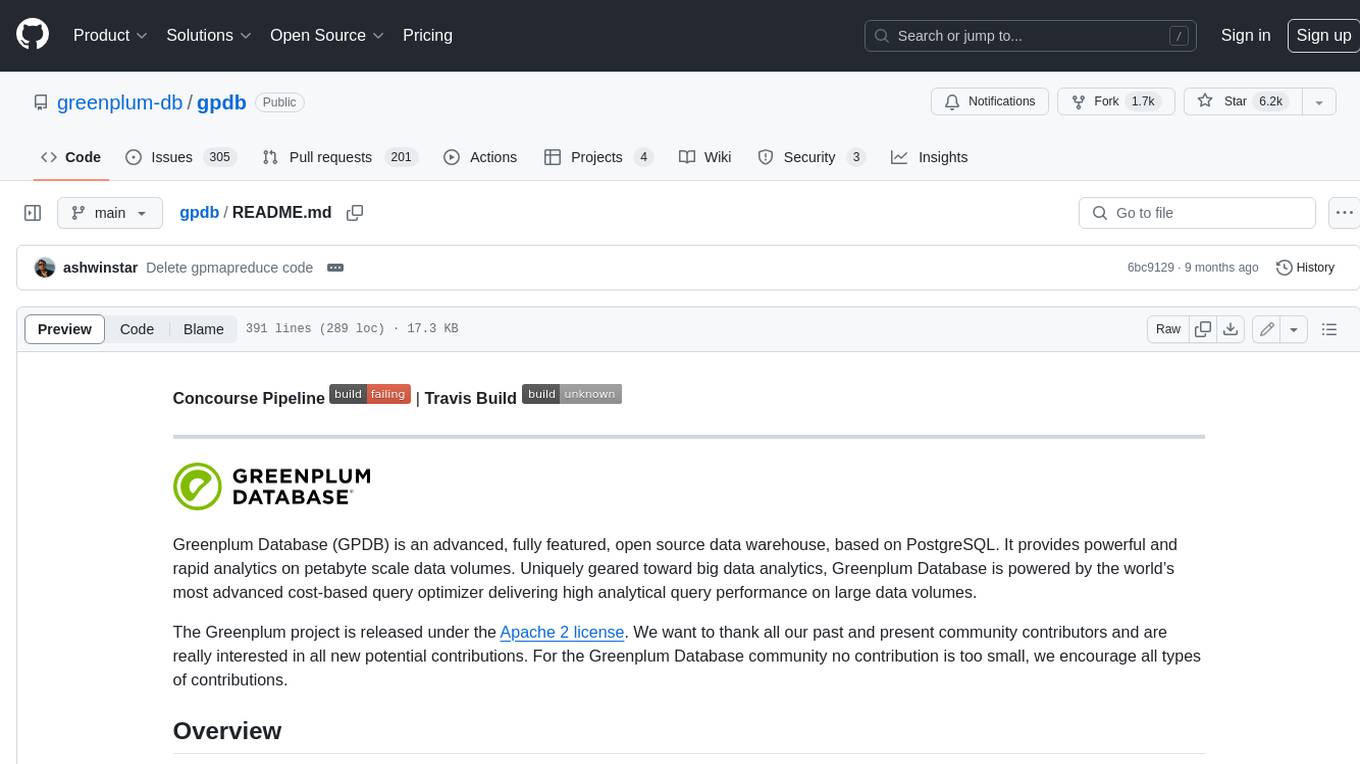
gpdb
Greenplum Database (GPDB) is an advanced, fully featured, open source data warehouse, based on PostgreSQL. It provides powerful and rapid analytics on petabyte scale data volumes. Uniquely geared toward big data analytics, Greenplum Database is powered by the world’s most advanced cost-based query optimizer delivering high analytical query performance on large data volumes.
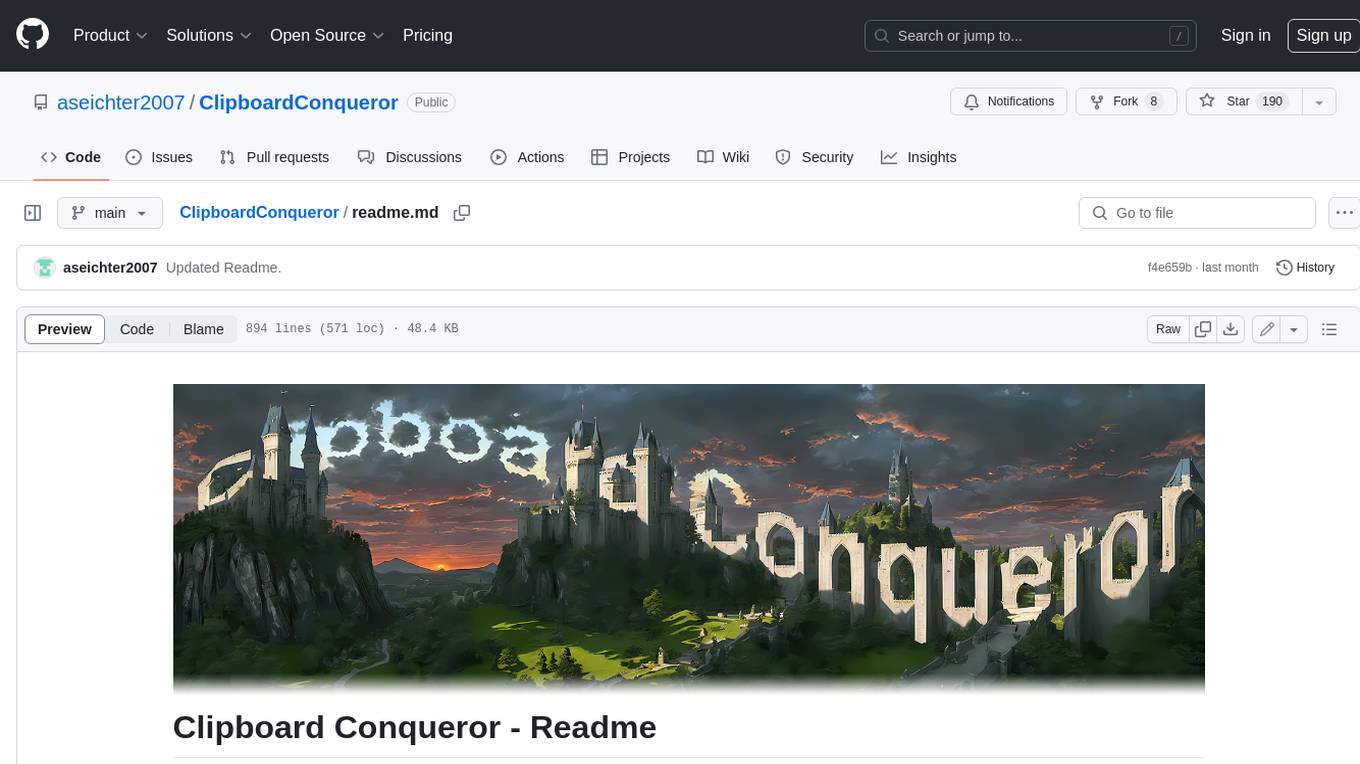
ClipboardConqueror
Clipboard Conqueror is a multi-platform omnipresent copilot alternative. Currently requiring a kobold united or openAI compatible back end, this software brings powerful LLM based tools to any text field, the universal copilot you deserve. It simply works anywhere. No need to sign in, no required key. Provided you are using local AI, CC is a data secure alternative integration provided you trust whatever backend you use. *Special thank you to the creators of KoboldAi, KoboldCPP, llamma, openAi, and the communities that made all this possible to figure out.
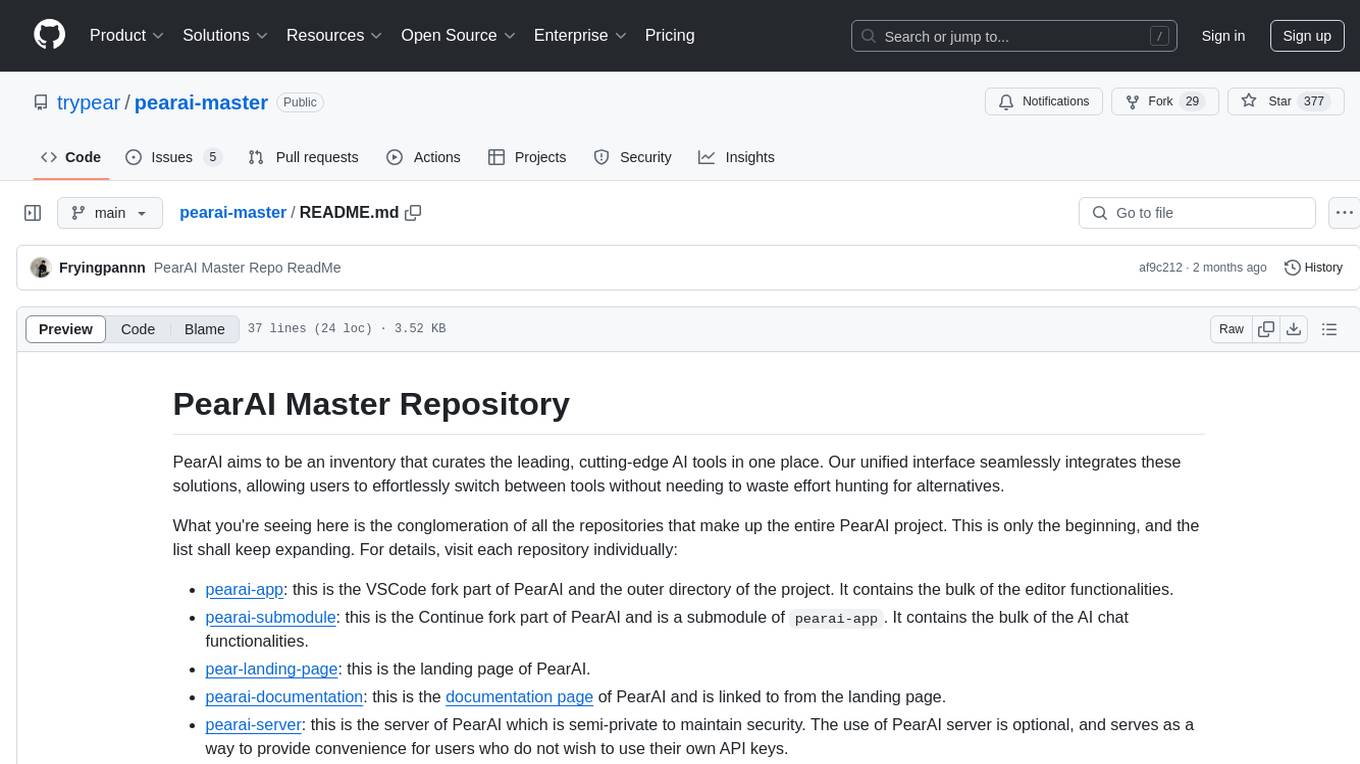
pearai-master
PearAI is an inventory that curates cutting-edge AI tools in one place, offering a unified interface for seamless tool integration. The repository serves as the conglomeration of all PearAI project repositories, including VSCode fork, AI chat functionalities, landing page, documentation, and server. Contributions are welcome through quests and issue tackling, with the project stack including TypeScript/Electron.js, Next.js/React, Python FastAPI, and Axiom for logging/telemetry.
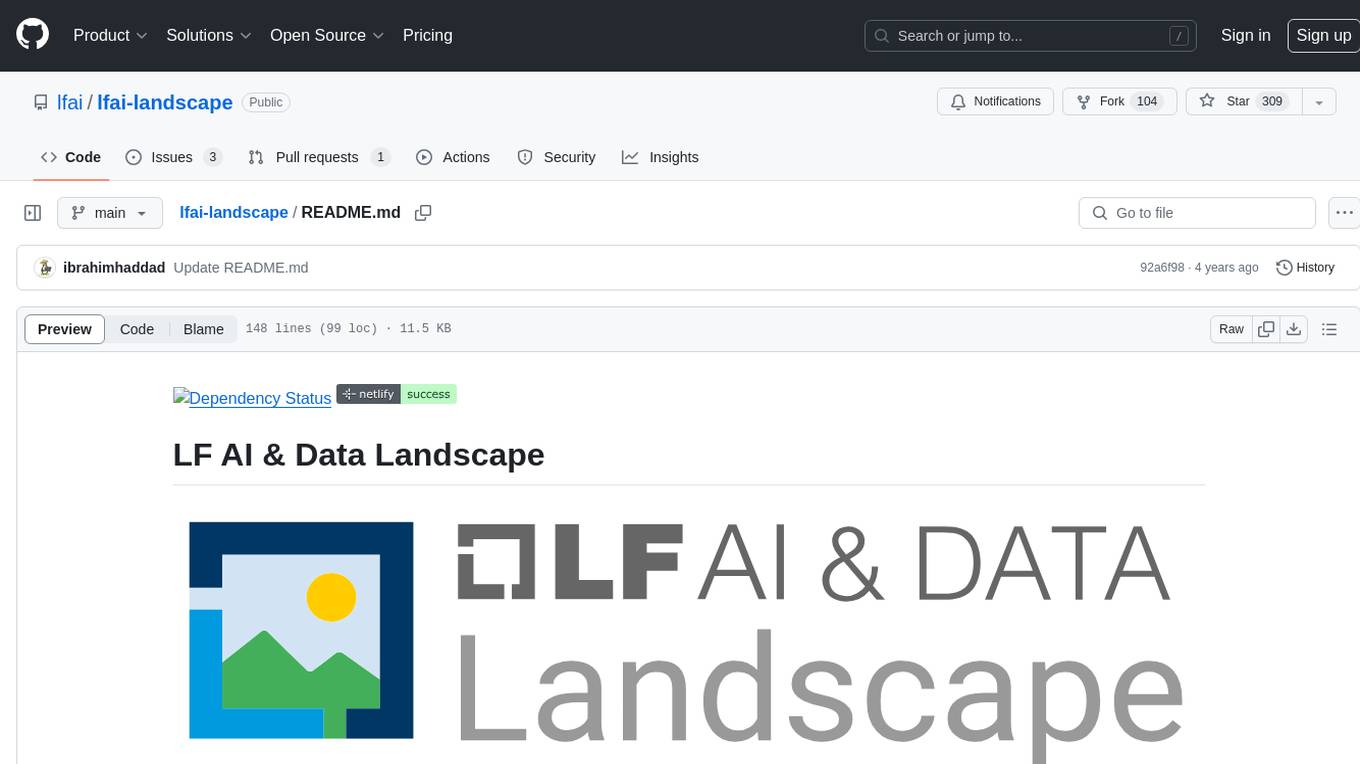
lfai-landscape
LF AI & Data Landscape is a map to explore open source projects in the AI & Data domains, highlighting companies that are members of LF AI & Data. It showcases members of the Foundation and is modelled after the Cloud Native Computing Foundation landscape. The landscape includes current version, interactive version, new entries, logos, proper SVGs, corrections, external data, best practices badge, non-updated items, license, formats, installation, vulnerability reporting, and adjusting the landscape view.
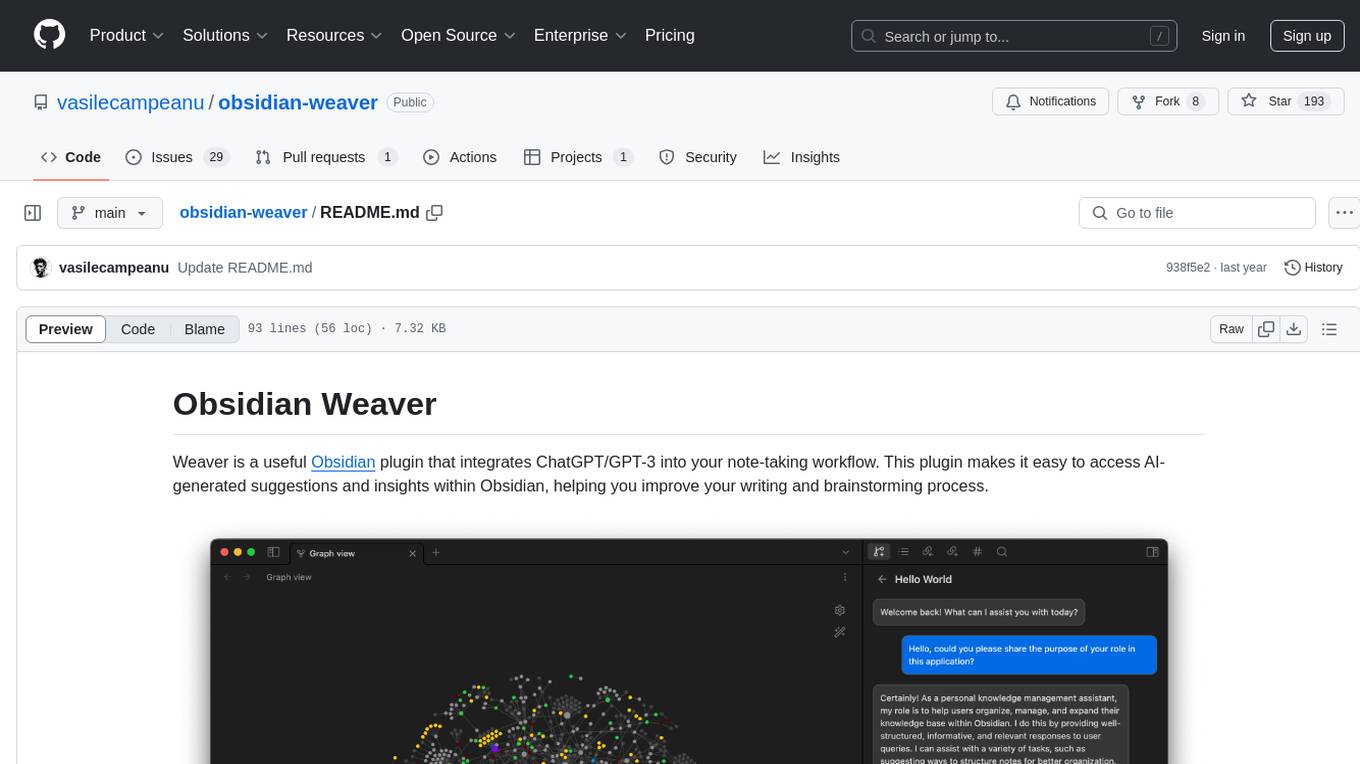
obsidian-weaver
Obsidian Weaver is a plugin that integrates ChatGPT/GPT-3 into the note-taking workflow of Obsidian. It allows users to easily access AI-generated suggestions and insights within Obsidian, enhancing the writing and brainstorming process. The plugin respects Obsidian's philosophy of storing notes locally, ensuring data security and privacy. Weaver offers features like creating new chat sessions with the AI assistant and receiving instant responses, all within the Obsidian environment. It provides a seamless integration with Obsidian's interface, making the writing process efficient and helping users stay focused. The plugin is constantly being improved with new features and updates to enhance the note-taking experience.
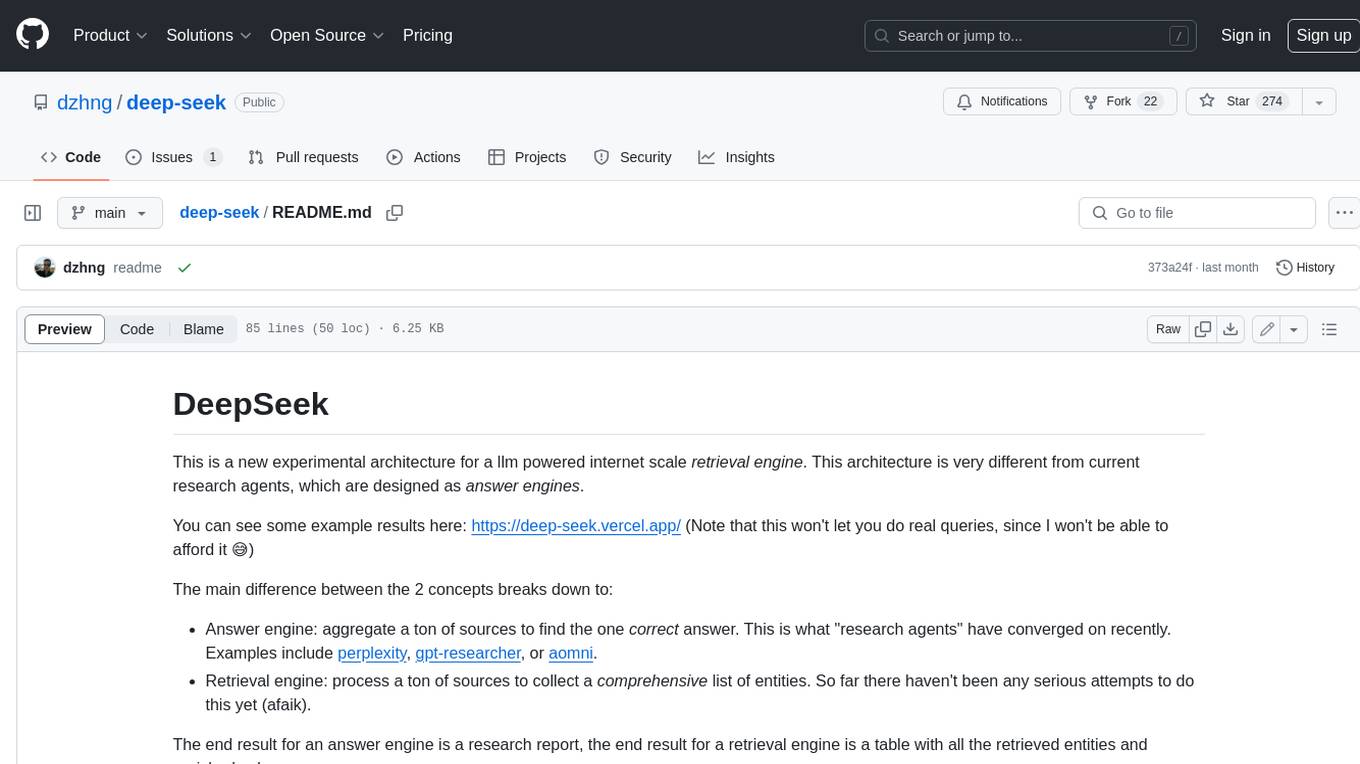
deep-seek
DeepSeek is a new experimental architecture for a large language model (LLM) powered internet-scale retrieval engine. Unlike current research agents designed as answer engines, DeepSeek aims to process a vast amount of sources to collect a comprehensive list of entities and enrich them with additional relevant data. The end result is a table with retrieved entities and enriched columns, providing a comprehensive overview of the topic. DeepSeek utilizes both standard keyword search and neural search to find relevant content, and employs an LLM to extract specific entities and their associated contents. It also includes a smaller answer agent to enrich the retrieved data, ensuring thoroughness. DeepSeek has the potential to revolutionize research and information gathering by providing a comprehensive and structured way to access information from the vastness of the internet.
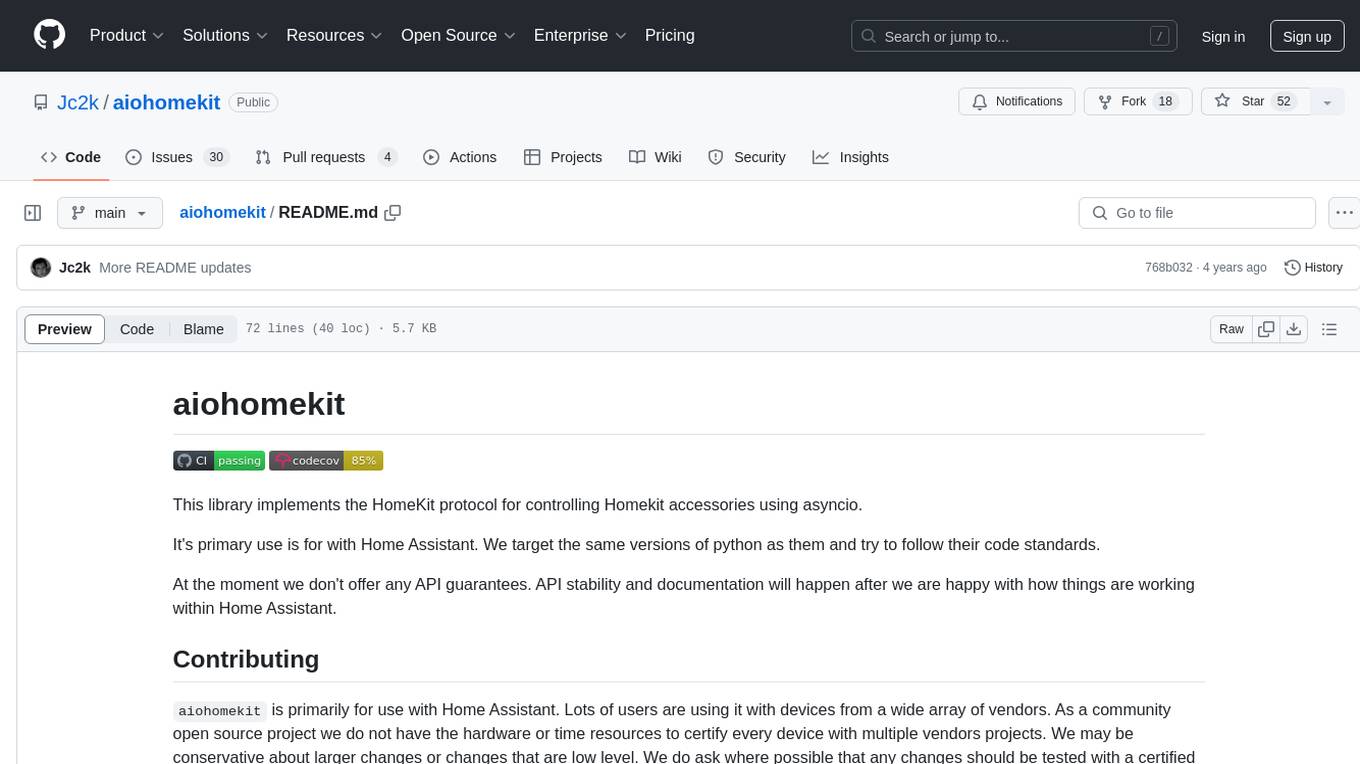
aiohomekit
aiohomekit is a Python library that implements the HomeKit protocol for controlling HomeKit accessories using asyncio. It is primarily used with Home Assistant, targeting the same versions of Python and following their code standards. The library is still under development and does not offer API guarantees yet. It aims to match the behavior of real HAP controllers, even when not strictly specified, and works around issues like JSON formatting, boolean encoding, header sensitivity, and TCP packet splitting. aiohomekit is primarily tested with Phillips Hue and Eve Extend bridges via Home Assistant, but is known to work with many more devices. It does not support BLE accessories and is intended for client-side use only.
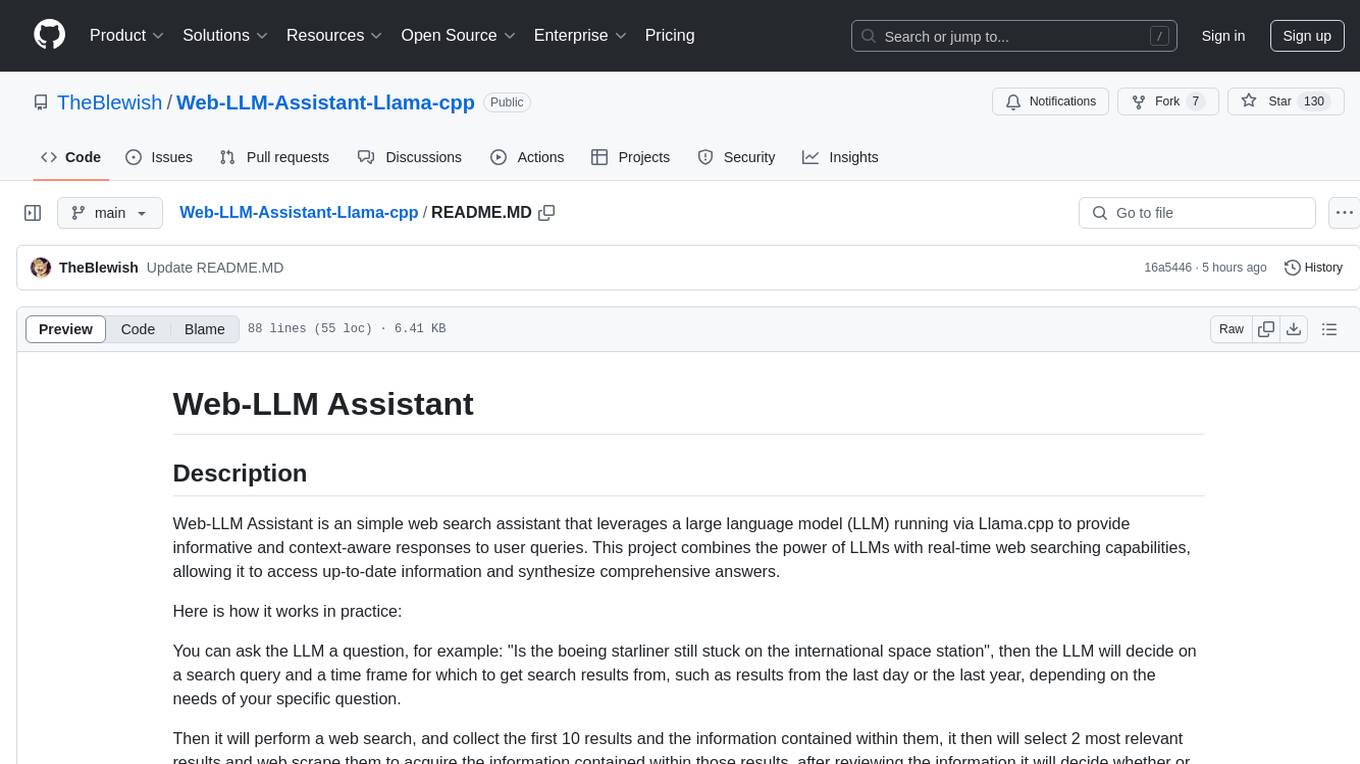
Web-LLM-Assistant-Llama-cpp
Web-LLM Assistant is a simple web search assistant that leverages a large language model (LLM) running via Llama.cpp to provide informative and context-aware responses to user queries. It combines the power of LLMs with real-time web searching capabilities, allowing it to access up-to-date information and synthesize comprehensive answers. The tool performs web searches, collects and scrapes information from search results, refines search queries, and provides answers based on the acquired information. Users can interact with the tool by asking questions or requesting web searches, making it a valuable resource for obtaining information beyond the LLM's training data.
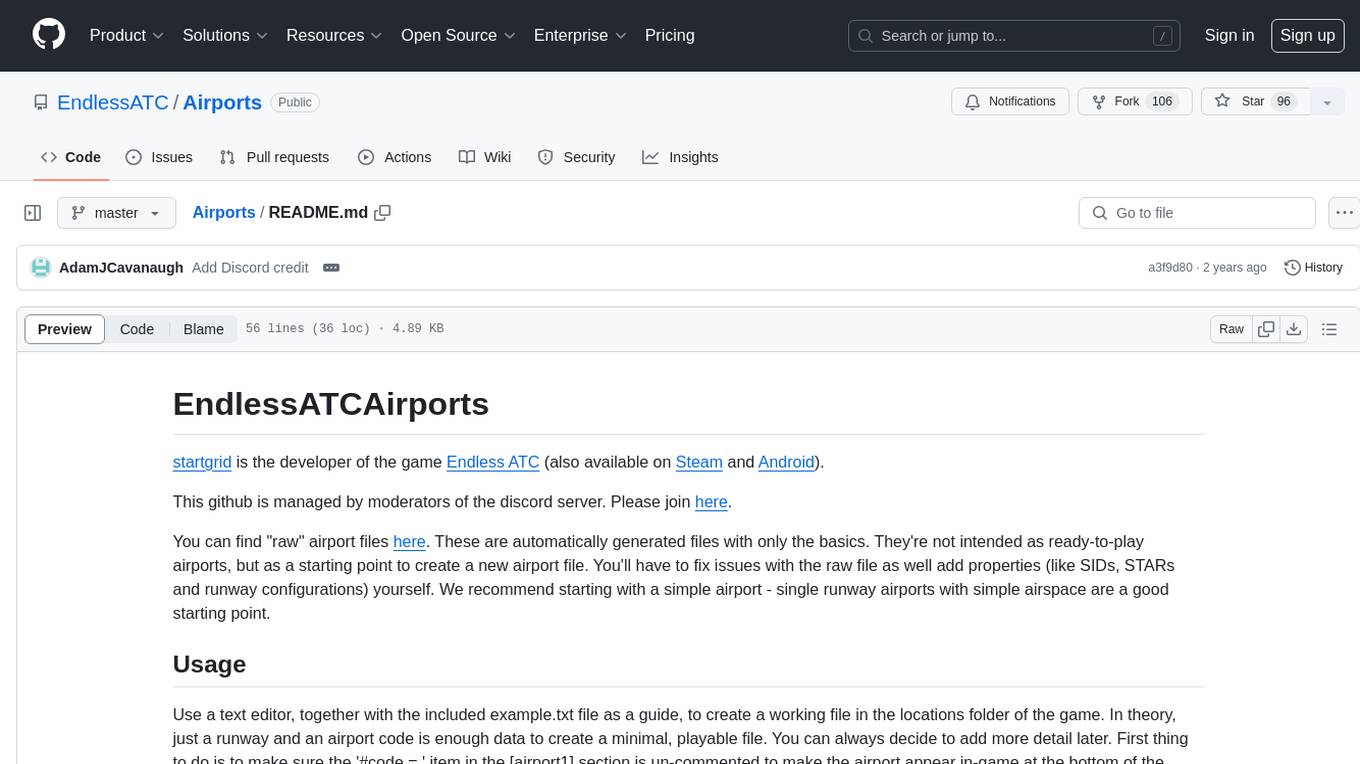
Airports
This repository contains raw airport files intended as a starting point to create new airport files for the game Endless ATC. Users can contribute by customizing airport files and submitting pull requests. The repository also welcomes markdown files with gameplay and development tips. Contributors are encouraged to join the Discord server for assistance and information.
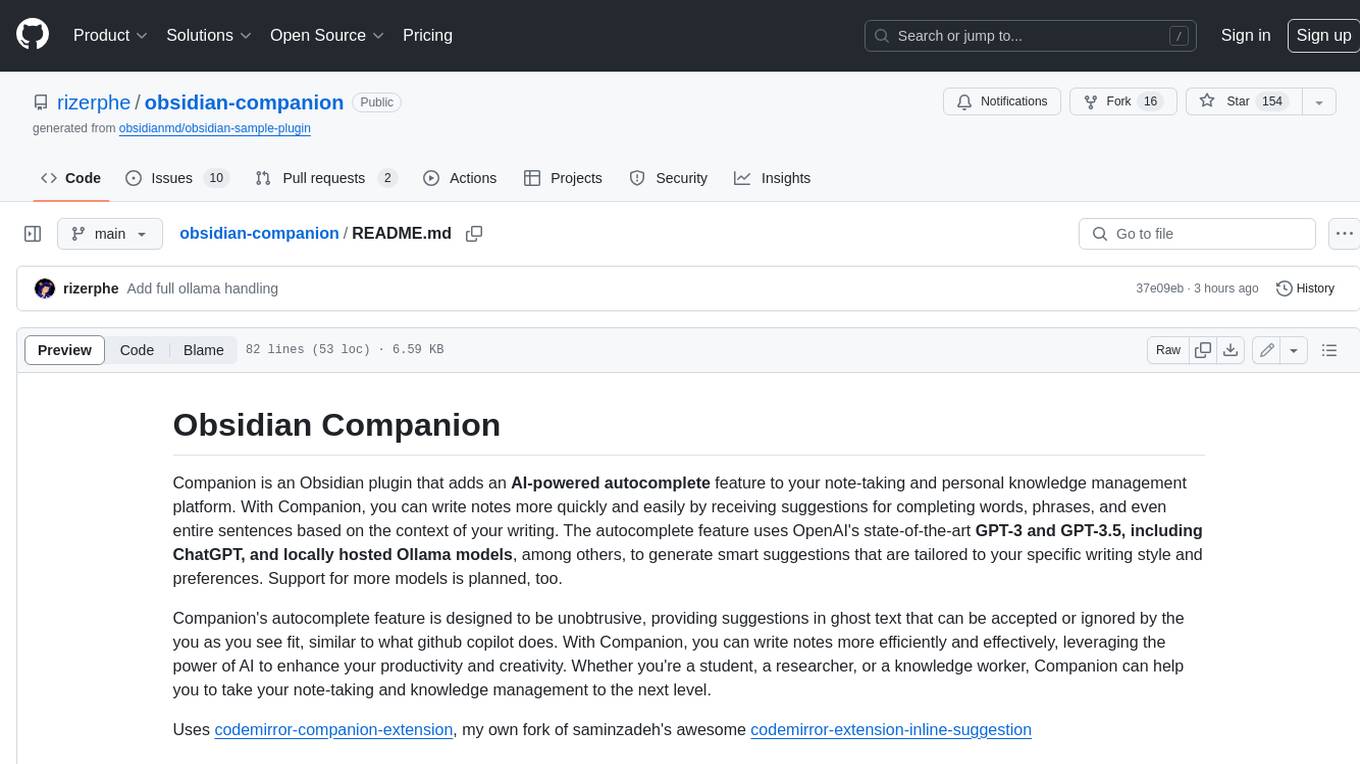
obsidian-companion
Companion is an Obsidian plugin that adds an AI-powered autocomplete feature to your note-taking and personal knowledge management platform. With Companion, you can write notes more quickly and easily by receiving suggestions for completing words, phrases, and even entire sentences based on the context of your writing. The autocomplete feature uses OpenAI's state-of-the-art GPT-3 and GPT-3.5, including ChatGPT, and locally hosted Ollama models, among others, to generate smart suggestions that are tailored to your specific writing style and preferences. Support for more models is planned, too.
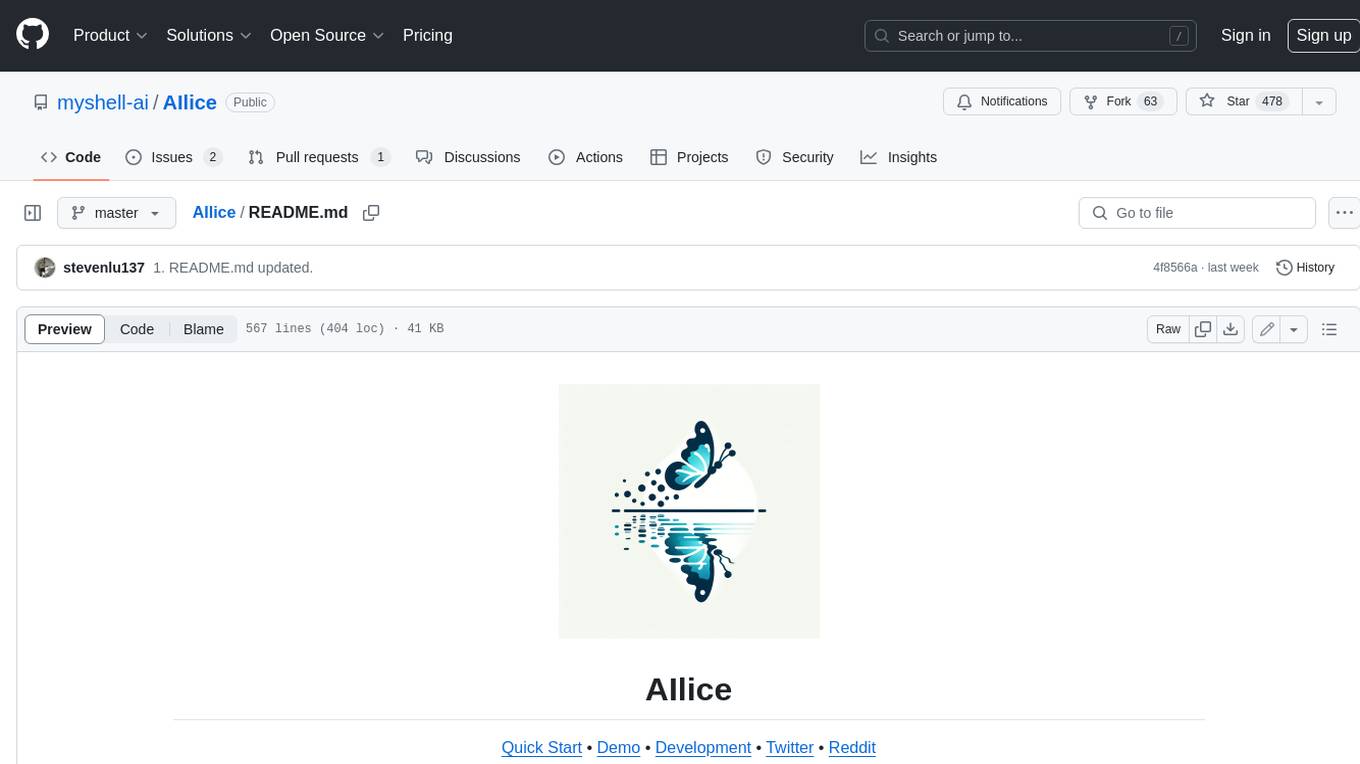
AIlice
AIlice is a fully autonomous, general-purpose AI agent that aims to create a standalone artificial intelligence assistant, similar to JARVIS, based on the open-source LLM. AIlice achieves this goal by building a "text computer" that uses a Large Language Model (LLM) as its core processor. Currently, AIlice demonstrates proficiency in a range of tasks, including thematic research, coding, system management, literature reviews, and complex hybrid tasks that go beyond these basic capabilities. AIlice has reached near-perfect performance in everyday tasks using GPT-4 and is making strides towards practical application with the latest open-source models. We will ultimately achieve self-evolution of AI agents. That is, AI agents will autonomously build their own feature expansions and new types of agents, unleashing LLM's knowledge and reasoning capabilities into the real world seamlessly.
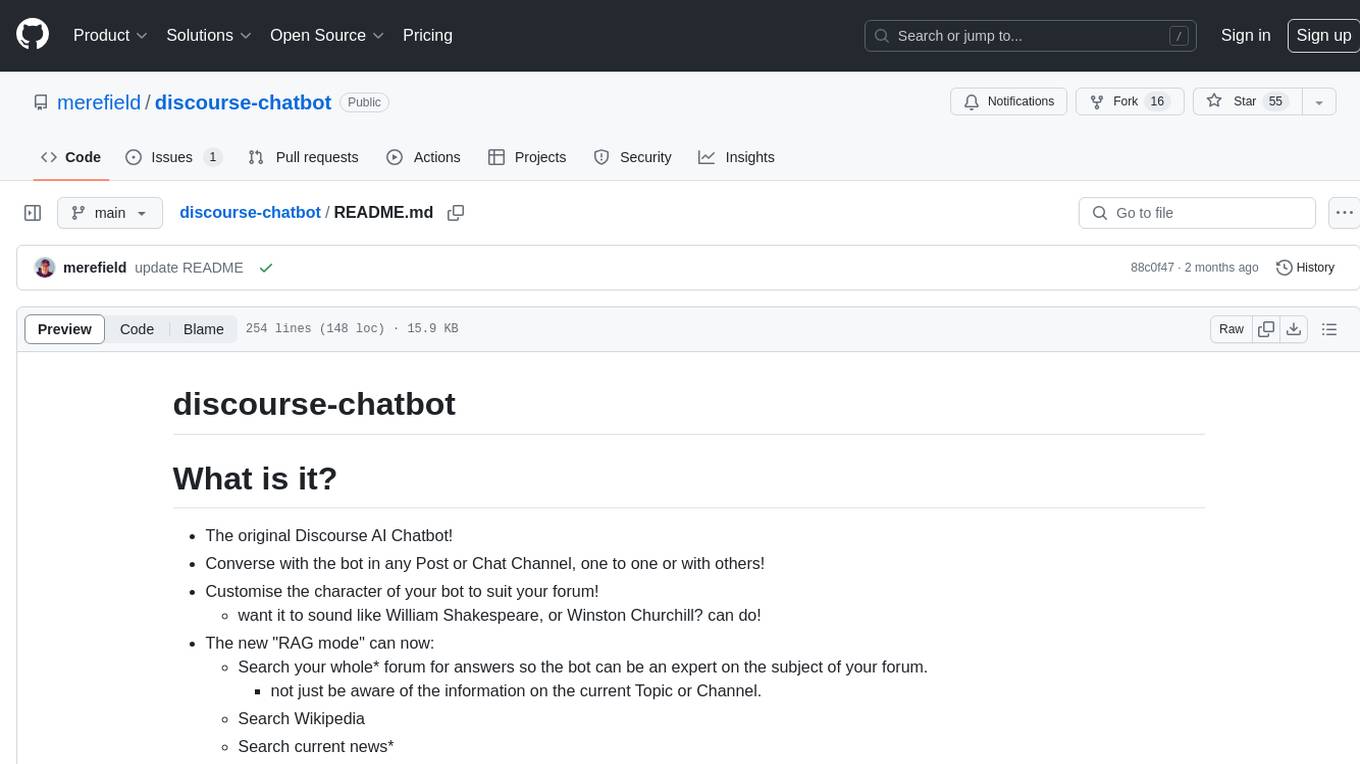
discourse-chatbot
The discourse-chatbot is an original AI chatbot for Discourse forums that allows users to converse with the bot in posts or chat channels. Users can customize the character of the bot, enable RAG mode for expert answers, search Wikipedia, news, and Google, provide market data, perform accurate math calculations, and experiment with vision support. The bot uses cutting-edge Open AI API and supports Azure and proxy server connections. It includes a quota system for access management and can be used in RAG mode or basic bot mode. The setup involves creating embeddings to make the bot aware of forum content and setting up bot access permissions based on trust levels. Users must obtain an API token from Open AI and configure group quotas to interact with the bot. The plugin is extensible to support other cloud bots and content search beyond the provided set.
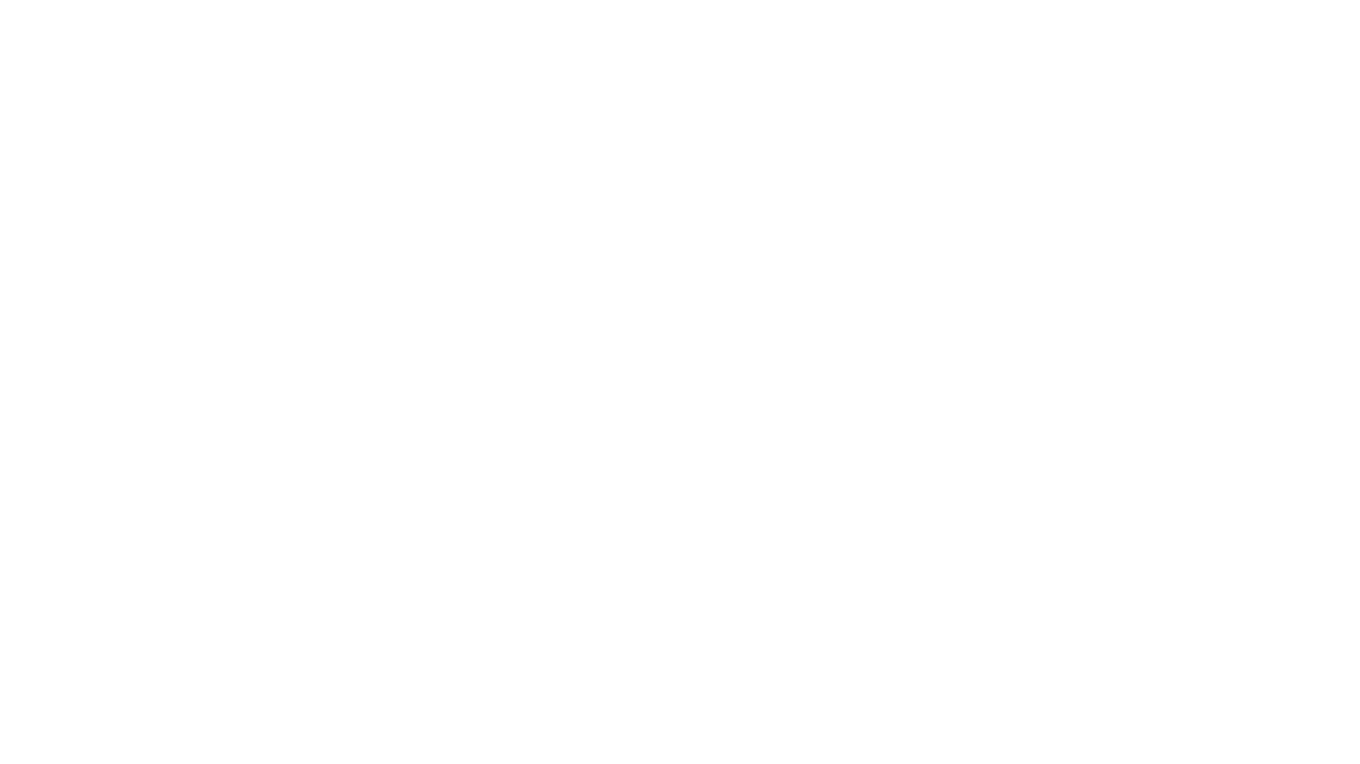
local-chat
LocalChat is a simple, easy-to-set-up, and open-source local AI chat tool that allows users to interact with generative language models on their own computers without transmitting data to a cloud server. It provides a chat-like interface for users to experience ChatGPT-like behavior locally, ensuring GDPR compliance and data privacy. Users can download LocalChat for macOS, Windows, or Linux to chat with open-weight generative language models.
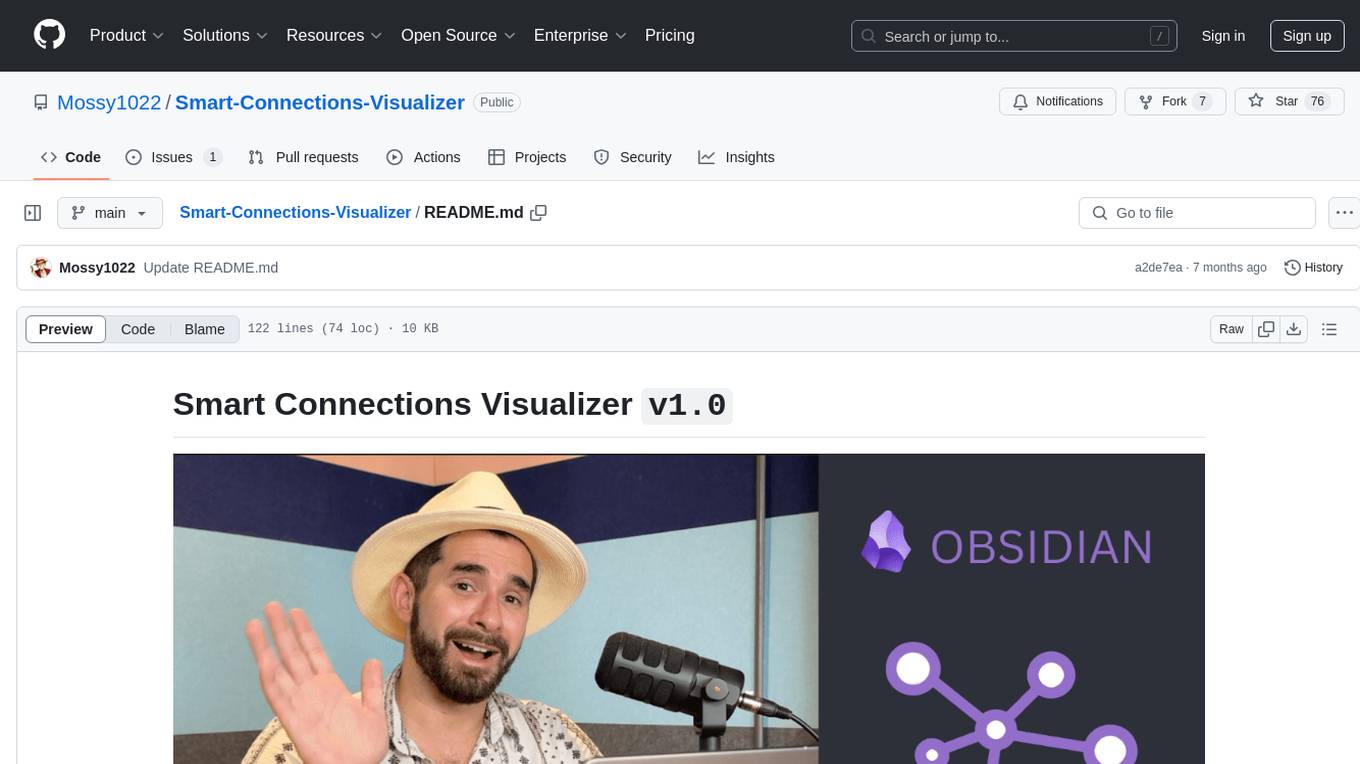
Smart-Connections-Visualizer
The Smart Connections Visualizer Plugin is a tool designed to enhance note-taking and information visualization by creating dynamic force-directed graphs that represent connections between notes or excerpts. Users can customize visualization settings, preview notes, and interact with the graph to explore relationships and insights within their notes. The plugin aims to revolutionize communication with AI and improve decision-making processes by visualizing complex information in a more intuitive and context-driven manner.
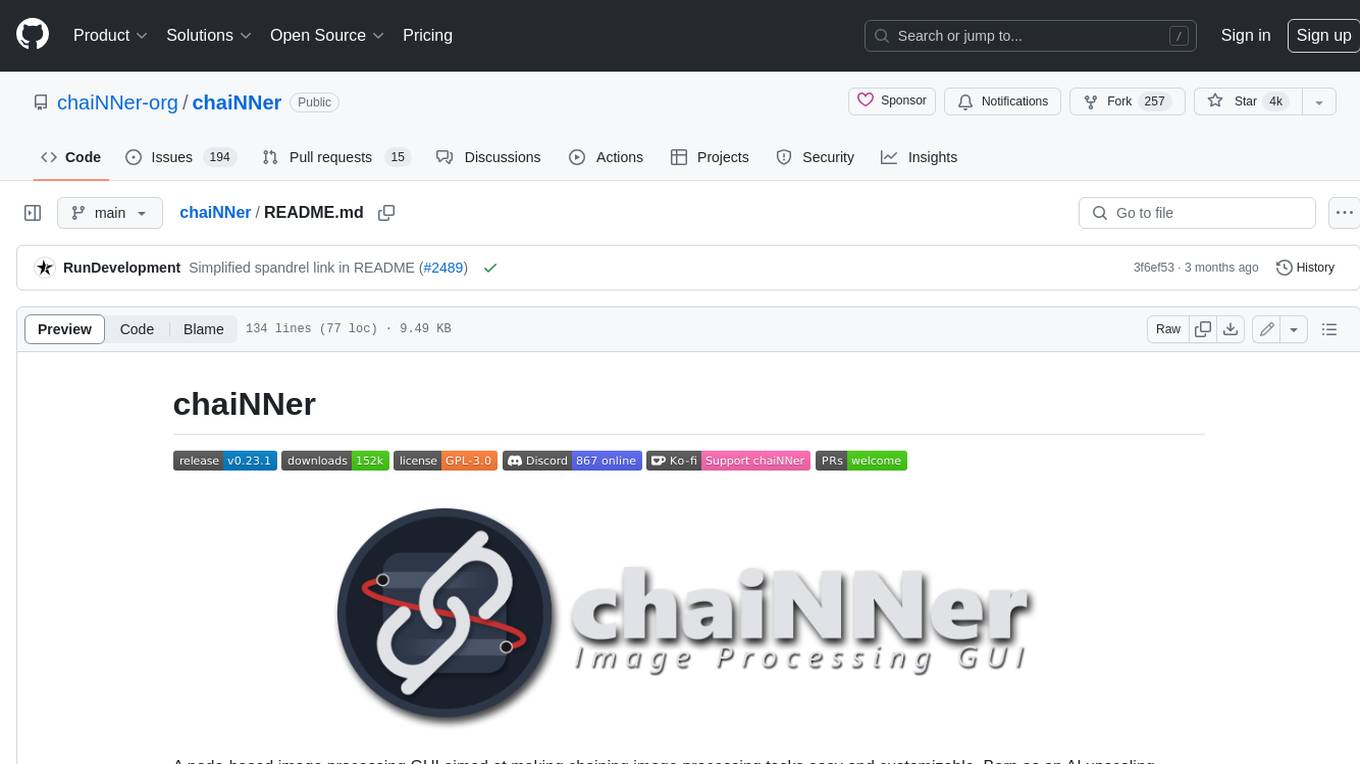
chaiNNer
ChaiNNer is a node-based image processing GUI aimed at making chaining image processing tasks easy and customizable. It gives users a high level of control over their processing pipeline and allows them to perform complex tasks by connecting nodes together. ChaiNNer is cross-platform, supporting Windows, MacOS, and Linux. It features an intuitive drag-and-drop interface, making it easy to create and modify processing chains. Additionally, ChaiNNer offers a wide range of nodes for various image processing tasks, including upscaling, denoising, sharpening, and color correction. It also supports batch processing, allowing users to process multiple images or videos at once.
For similar tasks
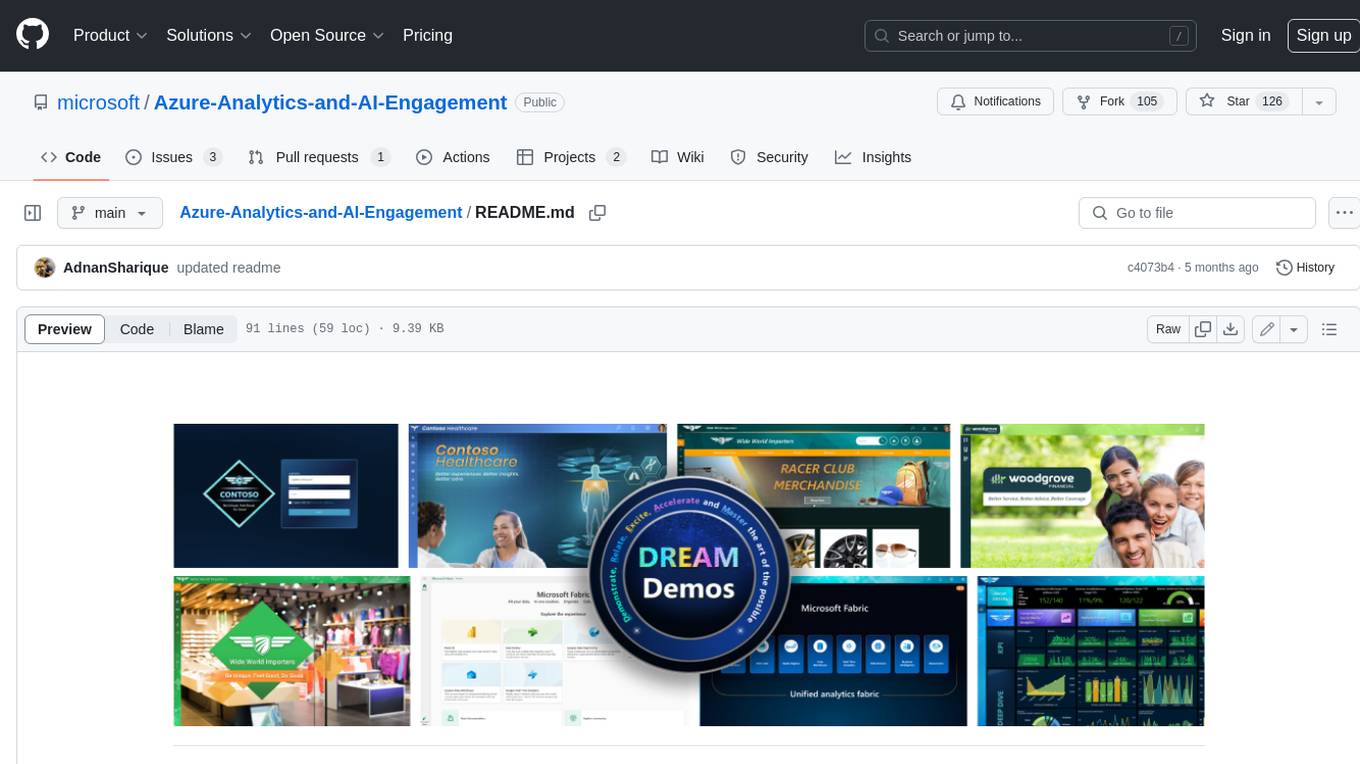
Azure-Analytics-and-AI-Engagement
The Azure-Analytics-and-AI-Engagement repository provides packaged Industry Scenario DREAM Demos with ARM templates (Containing a demo web application, Power BI reports, Synapse resources, AML Notebooks etc.) that can be deployed in a customer’s subscription using the CAPE tool within a matter of few hours. Partners can also deploy DREAM Demos in their own subscriptions using DPoC.
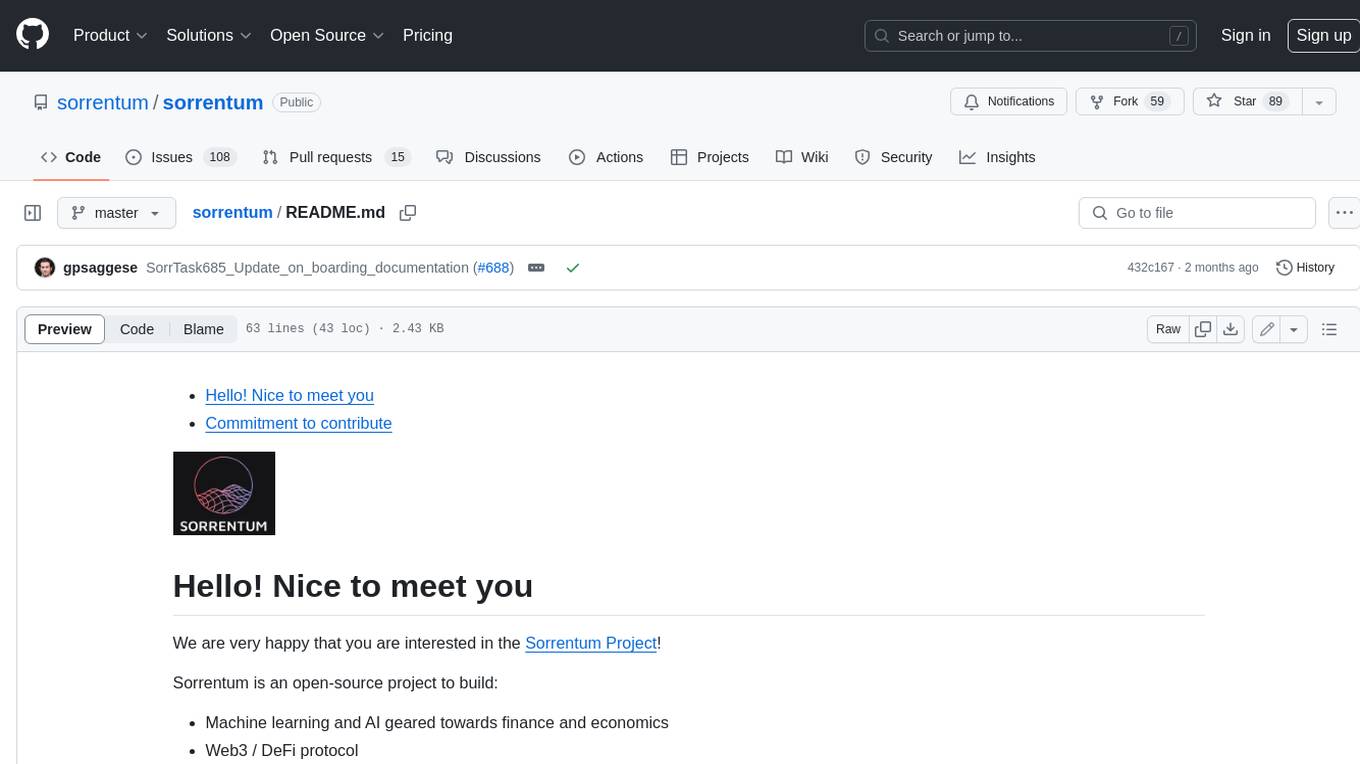
sorrentum
Sorrentum is an open-source project that aims to combine open-source development, startups, and brilliant students to build machine learning, AI, and Web3 / DeFi protocols geared towards finance and economics. The project provides opportunities for internships, research assistantships, and development grants, as well as the chance to work on cutting-edge problems, learn about startups, write academic papers, and get internships and full-time positions at companies working on Sorrentum applications.
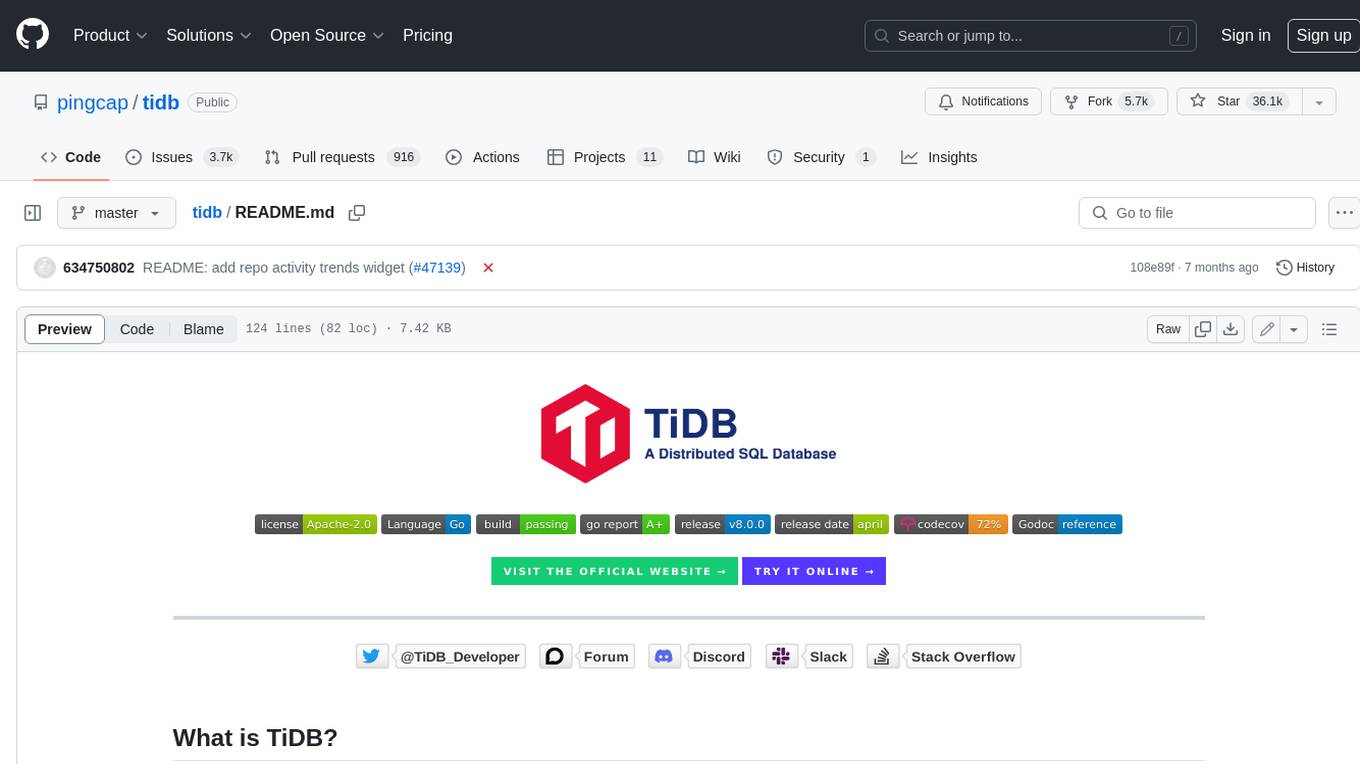
tidb
TiDB is an open-source distributed SQL database that supports Hybrid Transactional and Analytical Processing (HTAP) workloads. It is MySQL compatible and features horizontal scalability, strong consistency, and high availability.
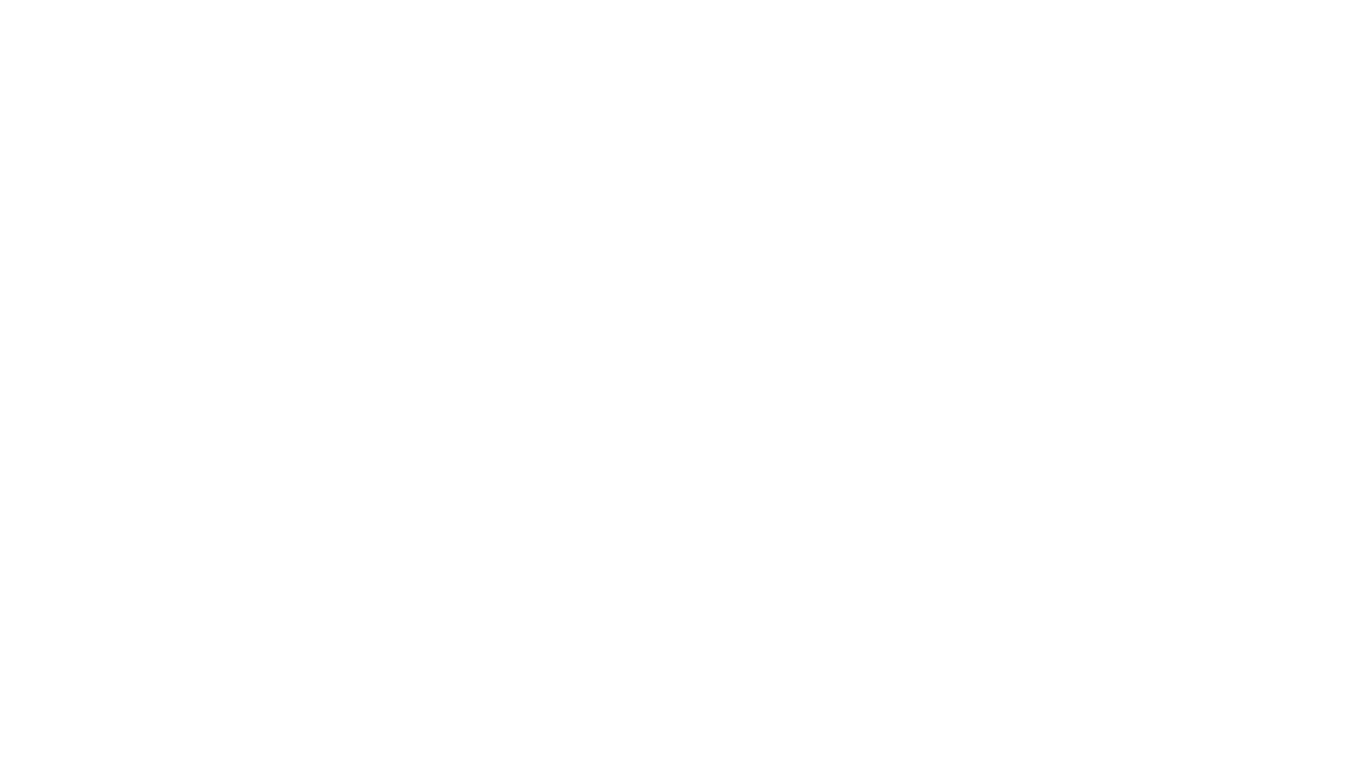
zep-python
Zep is an open-source platform for building and deploying large language model (LLM) applications. It provides a suite of tools and services that make it easy to integrate LLMs into your applications, including chat history memory, embedding, vector search, and data enrichment. Zep is designed to be scalable, reliable, and easy to use, making it a great choice for developers who want to build LLM-powered applications quickly and easily.
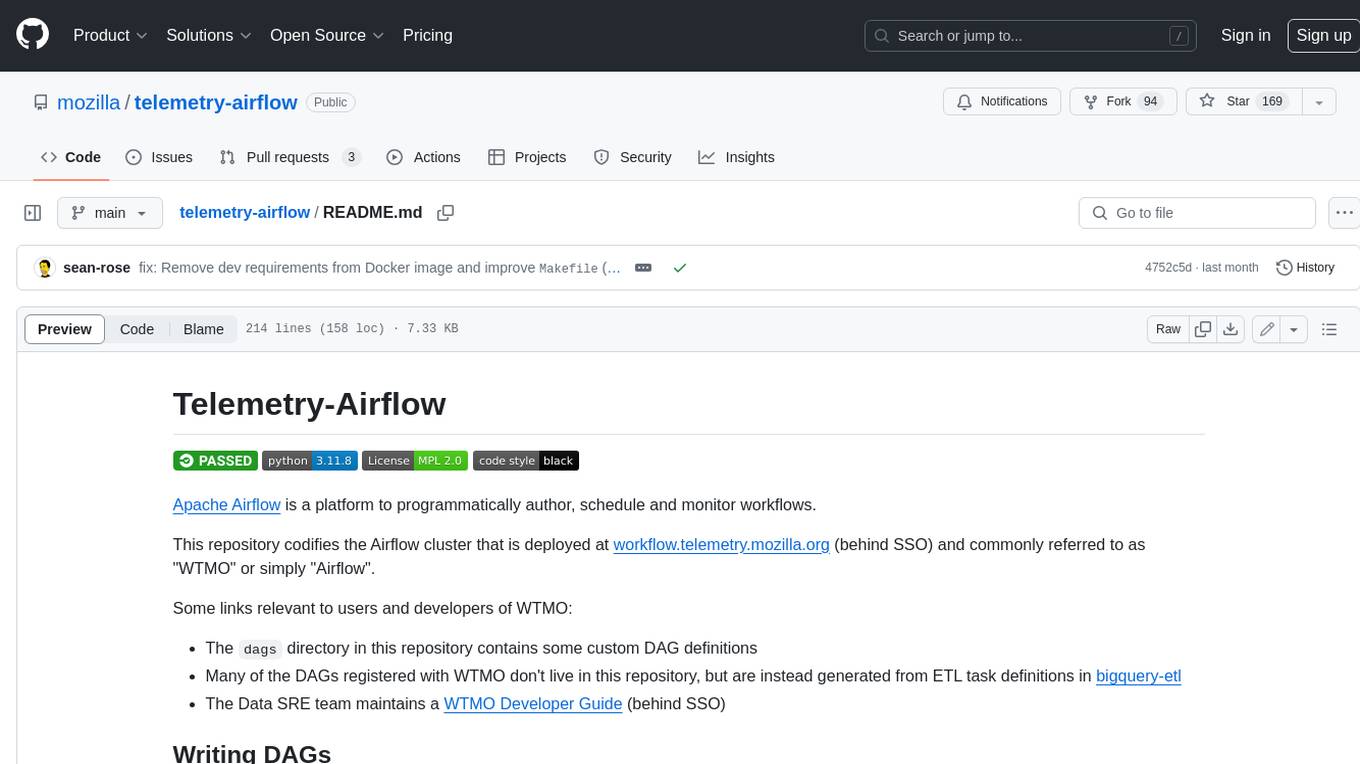
telemetry-airflow
This repository codifies the Airflow cluster that is deployed at workflow.telemetry.mozilla.org (behind SSO) and commonly referred to as "WTMO" or simply "Airflow". Some links relevant to users and developers of WTMO: * The `dags` directory in this repository contains some custom DAG definitions * Many of the DAGs registered with WTMO don't live in this repository, but are instead generated from ETL task definitions in bigquery-etl * The Data SRE team maintains a WTMO Developer Guide (behind SSO)
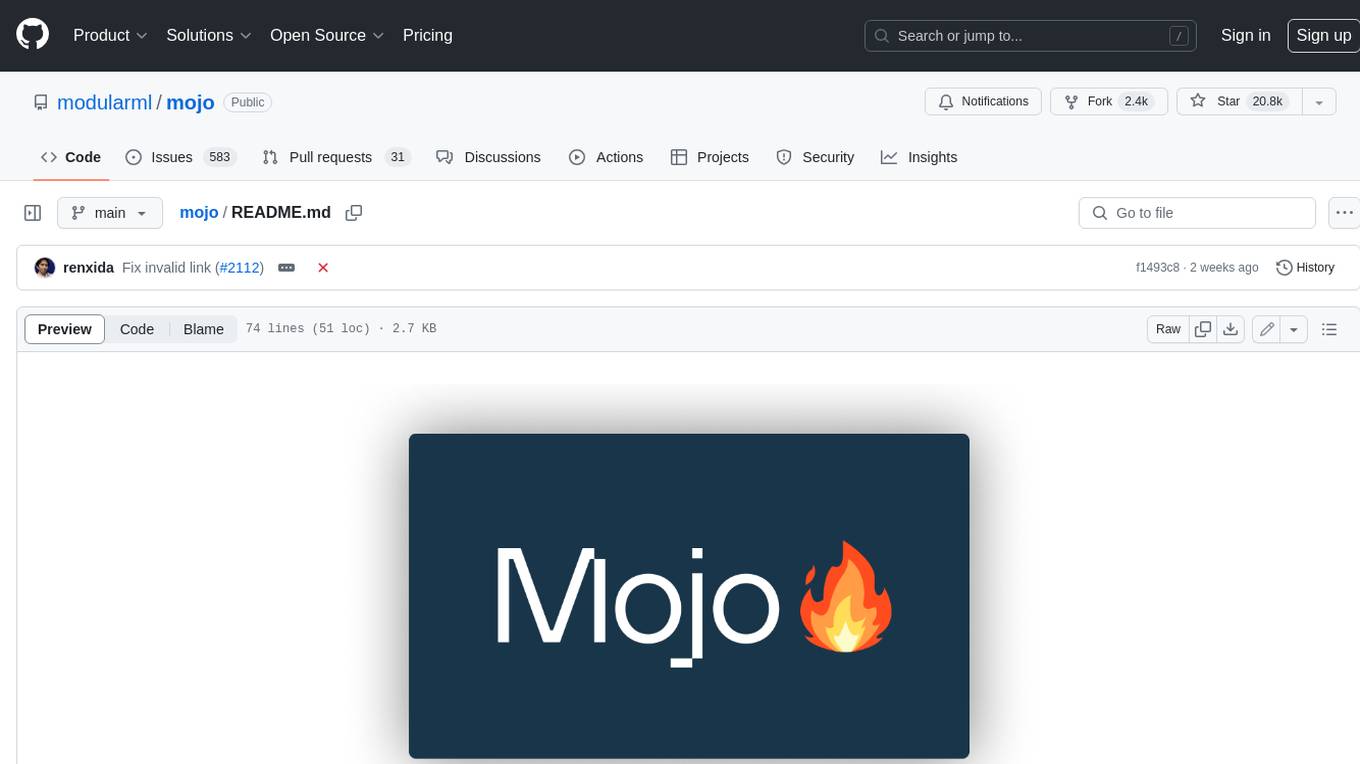
mojo
Mojo is a new programming language that bridges the gap between research and production by combining Python syntax and ecosystem with systems programming and metaprogramming features. Mojo is still young, but it is designed to become a superset of Python over time.
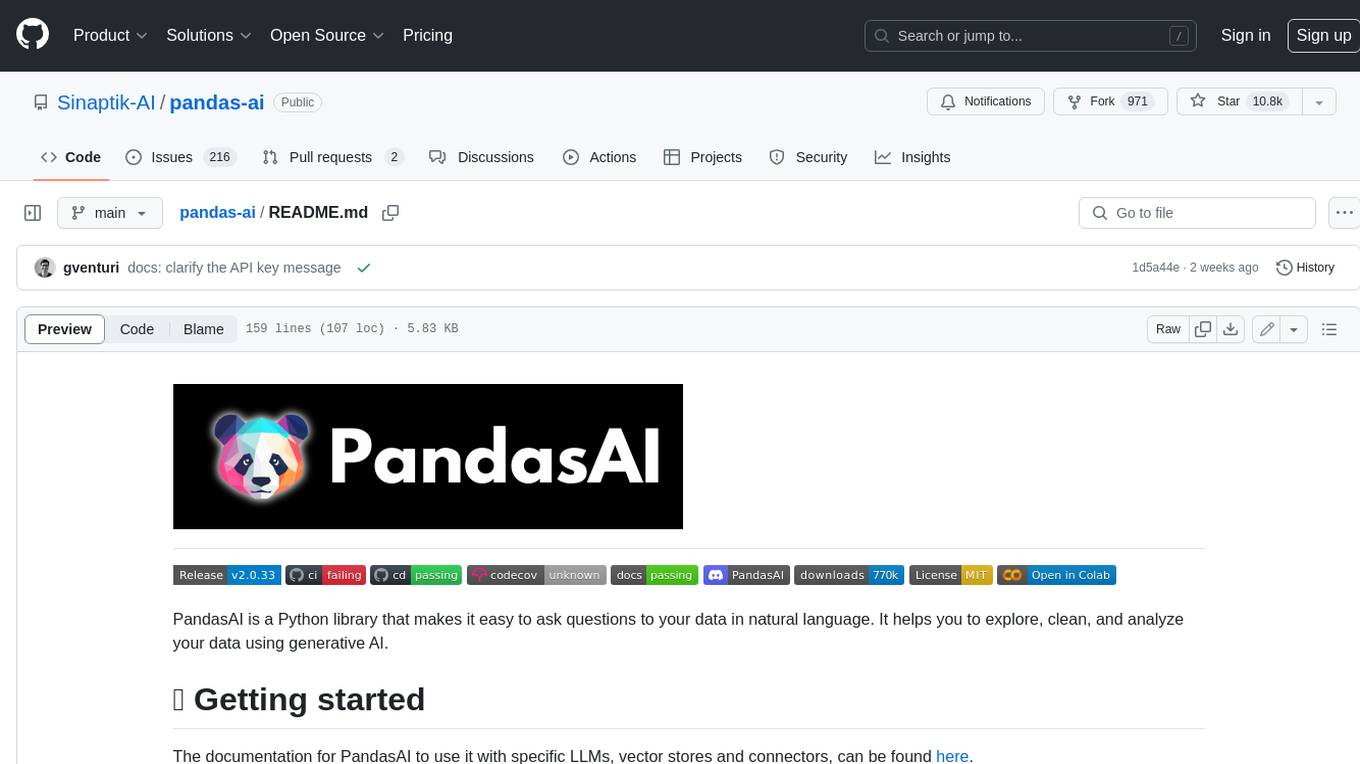
pandas-ai
PandasAI is a Python library that makes it easy to ask questions to your data in natural language. It helps you to explore, clean, and analyze your data using generative AI.
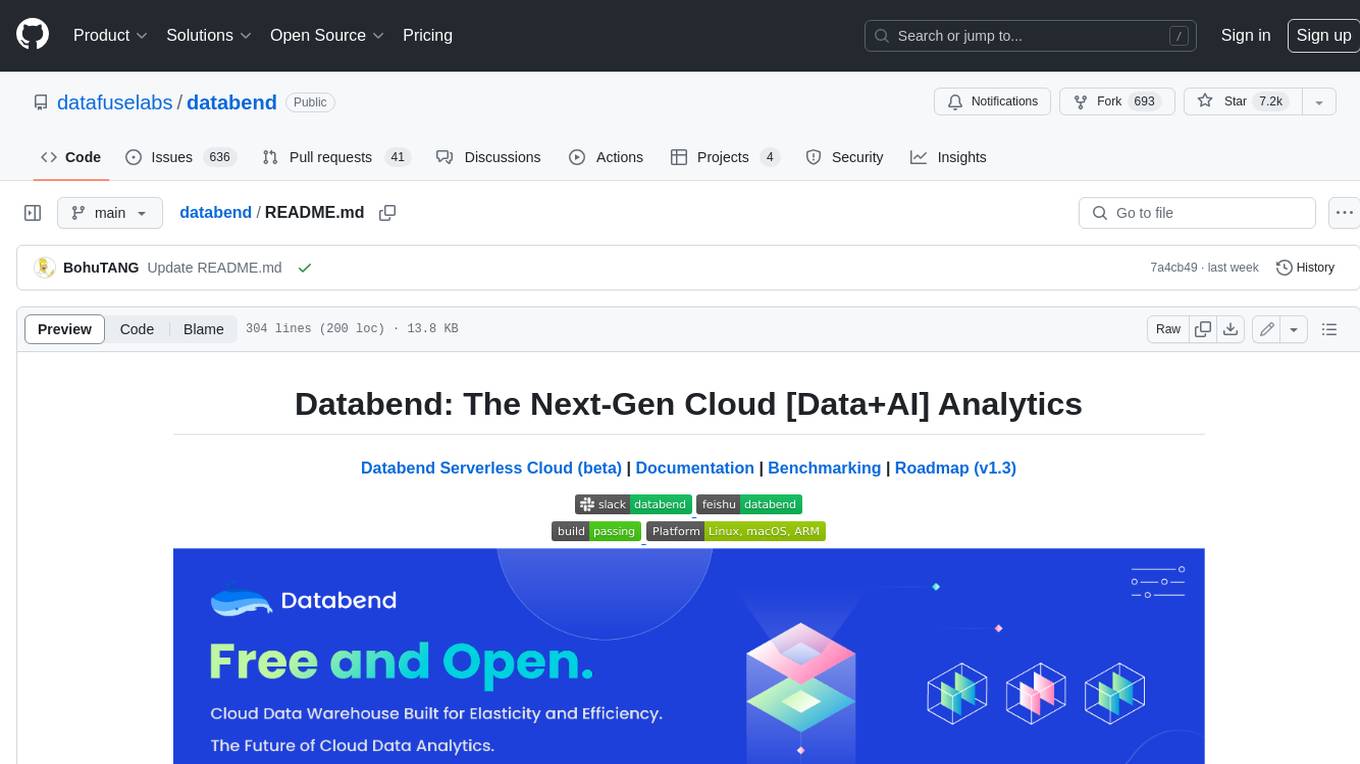
databend
Databend is an open-source cloud data warehouse that serves as a cost-effective alternative to Snowflake. With its focus on fast query execution and data ingestion, it's designed for complex analysis of the world's largest datasets.
For similar jobs
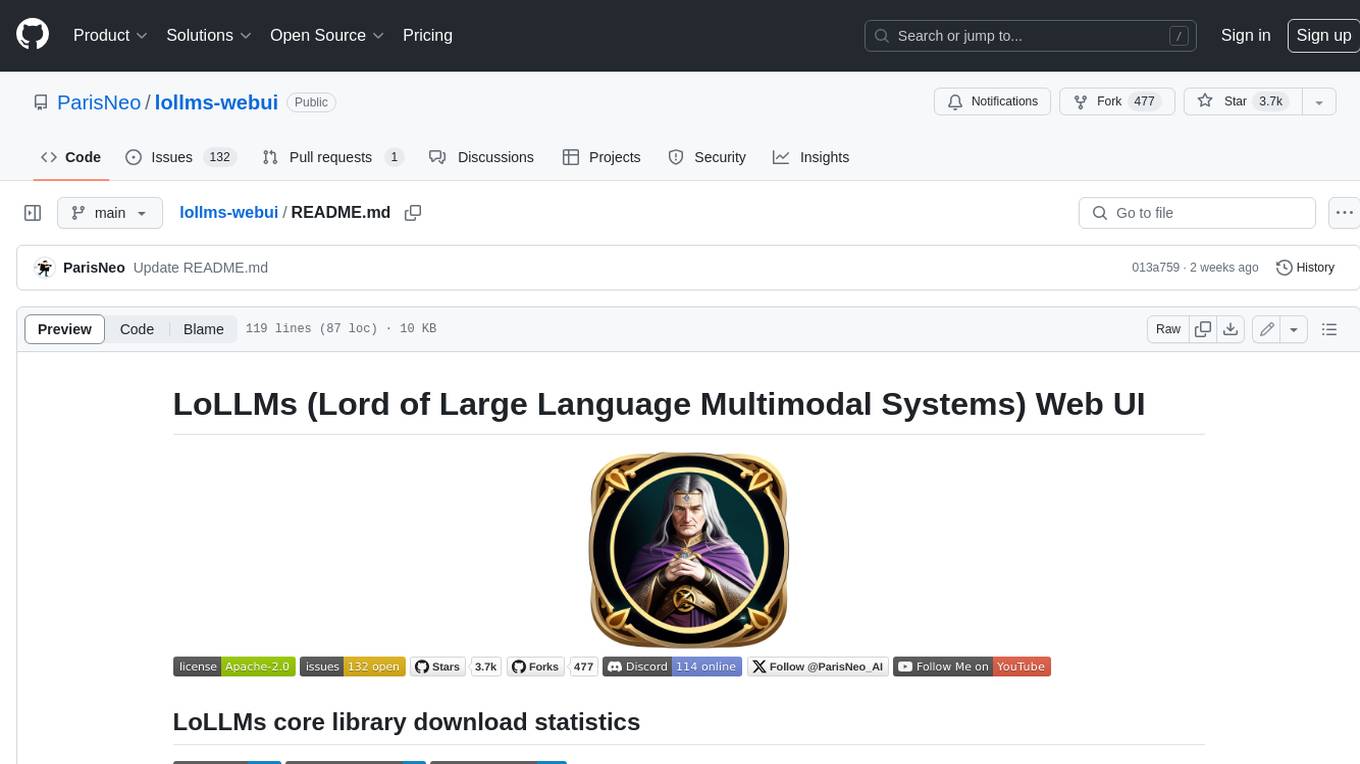
lollms-webui
LoLLMs WebUI (Lord of Large Language Multimodal Systems: One tool to rule them all) is a user-friendly interface to access and utilize various LLM (Large Language Models) and other AI models for a wide range of tasks. With over 500 AI expert conditionings across diverse domains and more than 2500 fine tuned models over multiple domains, LoLLMs WebUI provides an immediate resource for any problem, from car repair to coding assistance, legal matters, medical diagnosis, entertainment, and more. The easy-to-use UI with light and dark mode options, integration with GitHub repository, support for different personalities, and features like thumb up/down rating, copy, edit, and remove messages, local database storage, search, export, and delete multiple discussions, make LoLLMs WebUI a powerful and versatile tool.
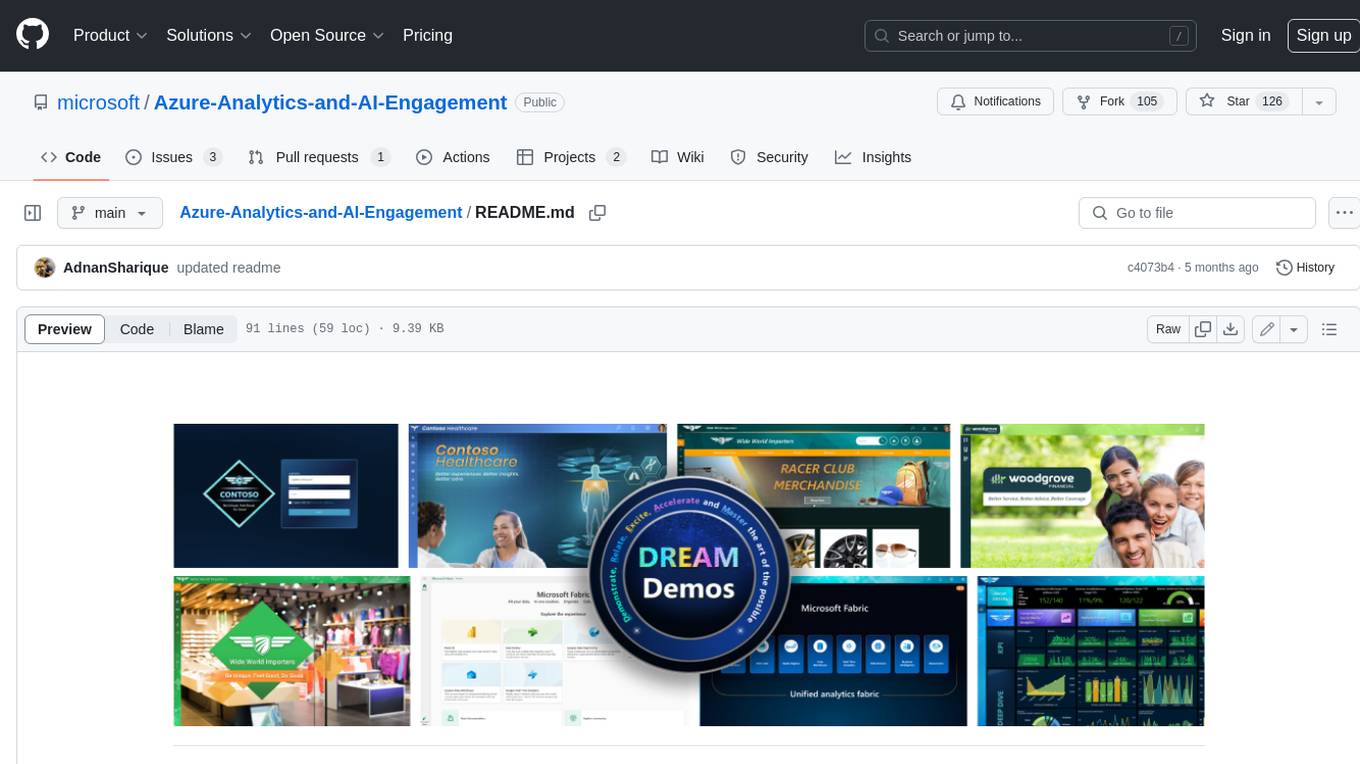
Azure-Analytics-and-AI-Engagement
The Azure-Analytics-and-AI-Engagement repository provides packaged Industry Scenario DREAM Demos with ARM templates (Containing a demo web application, Power BI reports, Synapse resources, AML Notebooks etc.) that can be deployed in a customer’s subscription using the CAPE tool within a matter of few hours. Partners can also deploy DREAM Demos in their own subscriptions using DPoC.
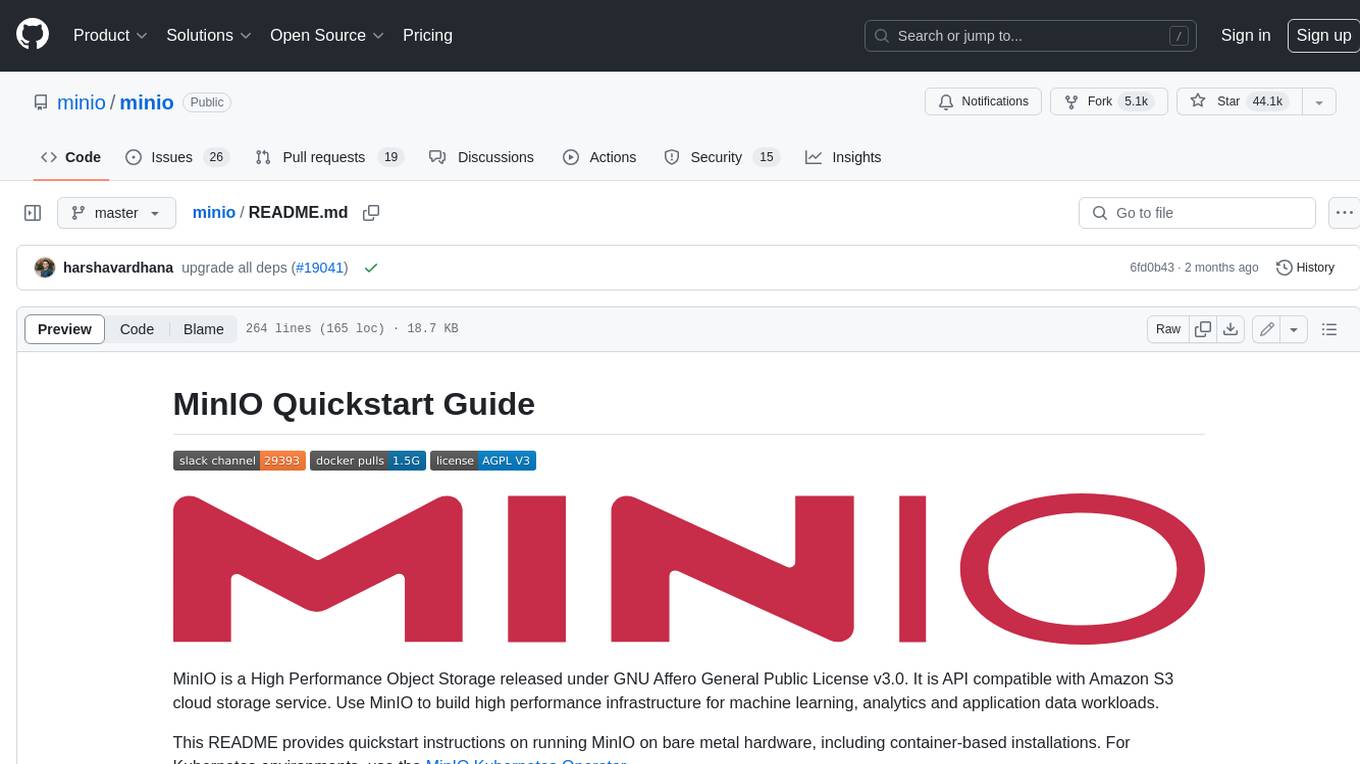
minio
MinIO is a High Performance Object Storage released under GNU Affero General Public License v3.0. It is API compatible with Amazon S3 cloud storage service. Use MinIO to build high performance infrastructure for machine learning, analytics and application data workloads.
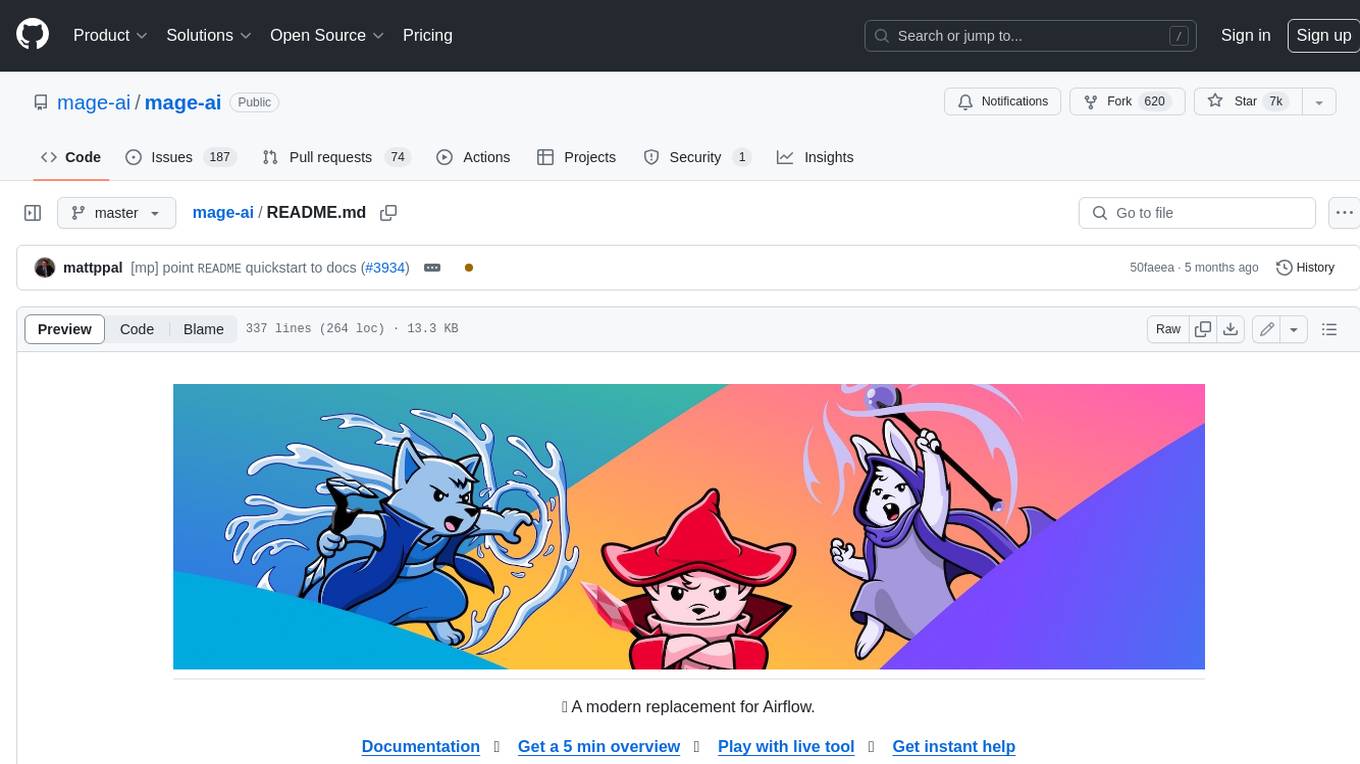
mage-ai
Mage is an open-source data pipeline tool for transforming and integrating data. It offers an easy developer experience, engineering best practices built-in, and data as a first-class citizen. Mage makes it easy to build, preview, and launch data pipelines, and provides observability and scaling capabilities. It supports data integrations, streaming pipelines, and dbt integration.
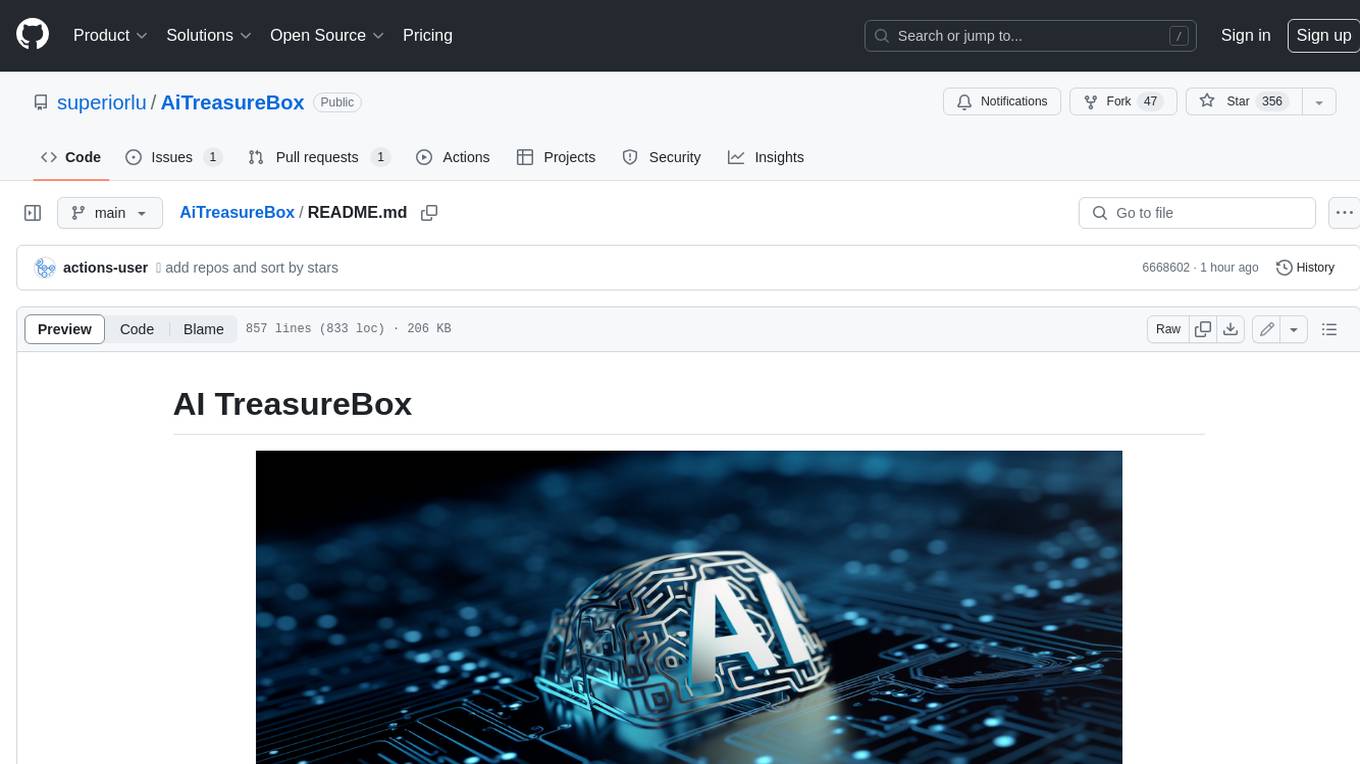
AiTreasureBox
AiTreasureBox is a versatile AI tool that provides a collection of pre-trained models and algorithms for various machine learning tasks. It simplifies the process of implementing AI solutions by offering ready-to-use components that can be easily integrated into projects. With AiTreasureBox, users can quickly prototype and deploy AI applications without the need for extensive knowledge in machine learning or deep learning. The tool covers a wide range of tasks such as image classification, text generation, sentiment analysis, object detection, and more. It is designed to be user-friendly and accessible to both beginners and experienced developers, making AI development more efficient and accessible to a wider audience.
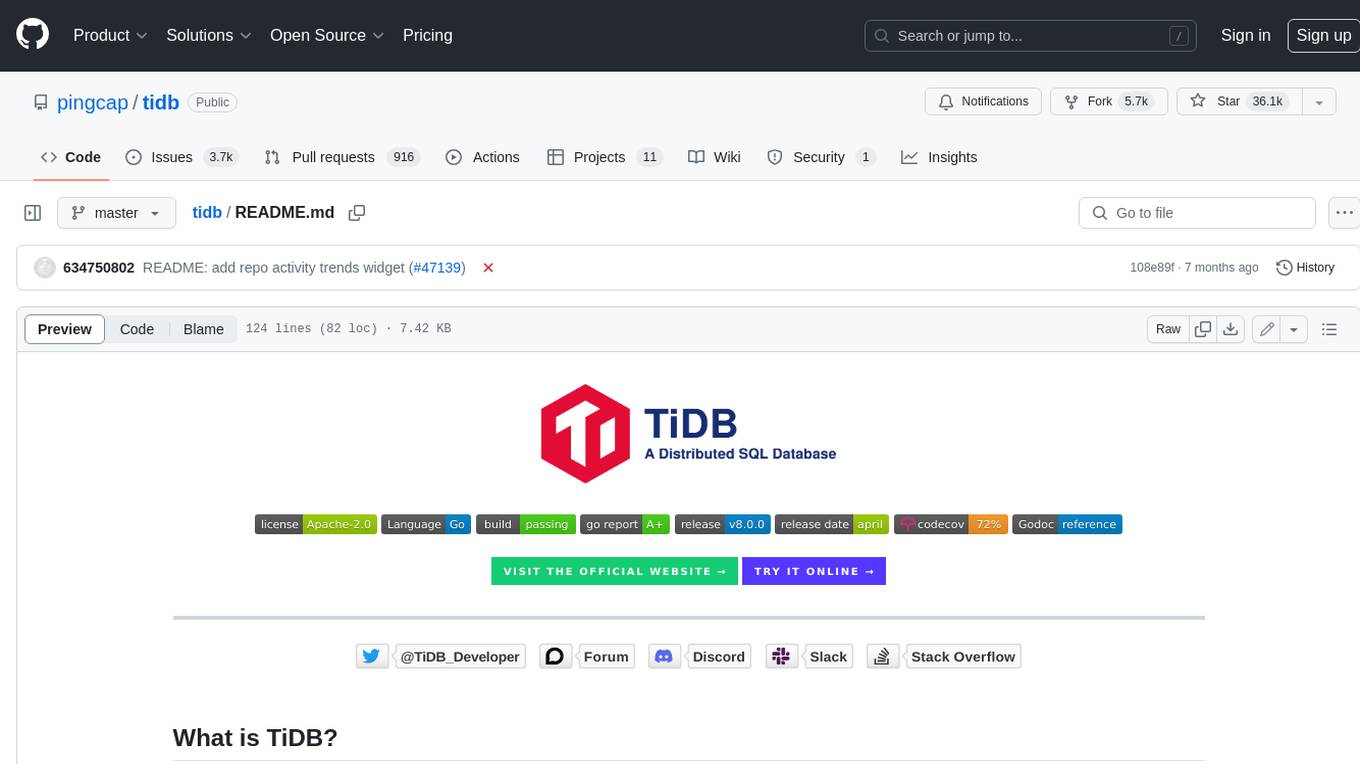
tidb
TiDB is an open-source distributed SQL database that supports Hybrid Transactional and Analytical Processing (HTAP) workloads. It is MySQL compatible and features horizontal scalability, strong consistency, and high availability.
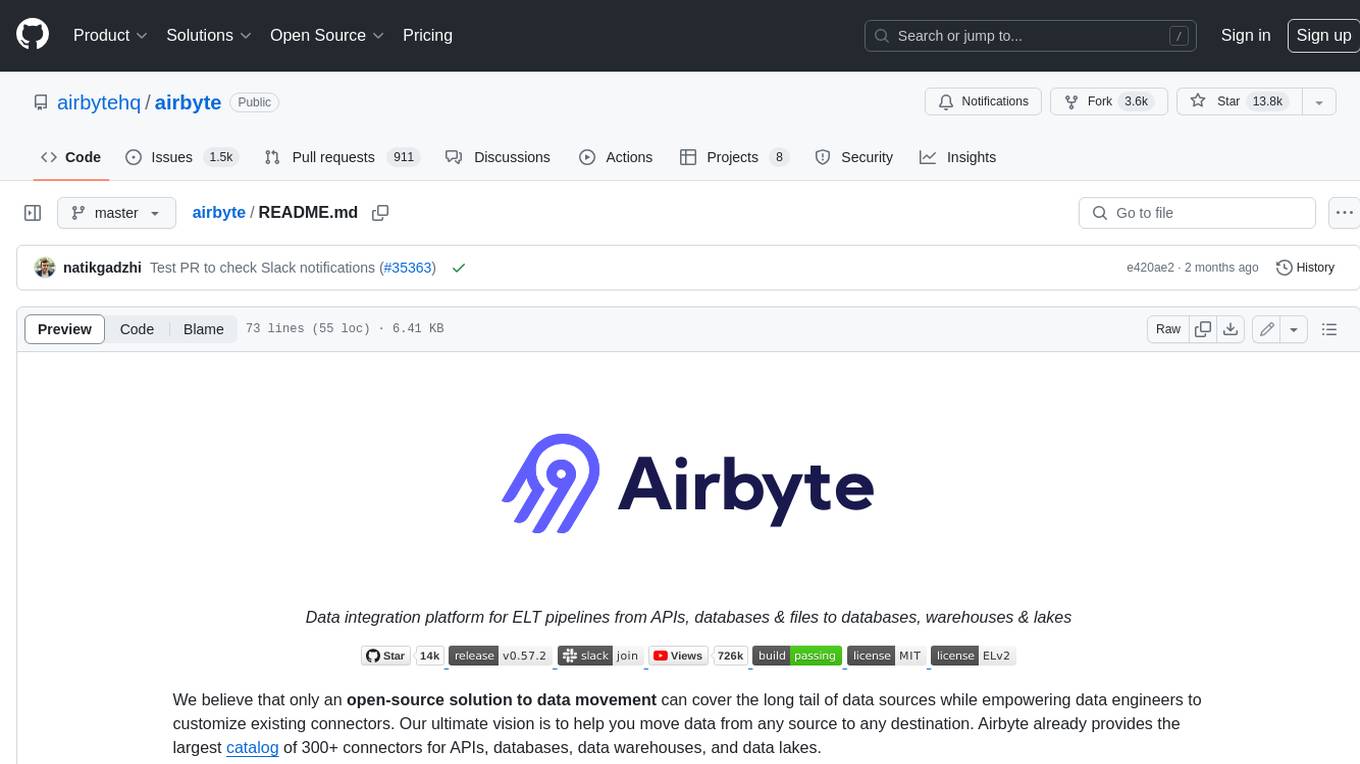
airbyte
Airbyte is an open-source data integration platform that makes it easy to move data from any source to any destination. With Airbyte, you can build and manage data pipelines without writing any code. Airbyte provides a library of pre-built connectors that make it easy to connect to popular data sources and destinations. You can also create your own connectors using Airbyte's no-code Connector Builder or low-code CDK. Airbyte is used by data engineers and analysts at companies of all sizes to build and manage their data pipelines.
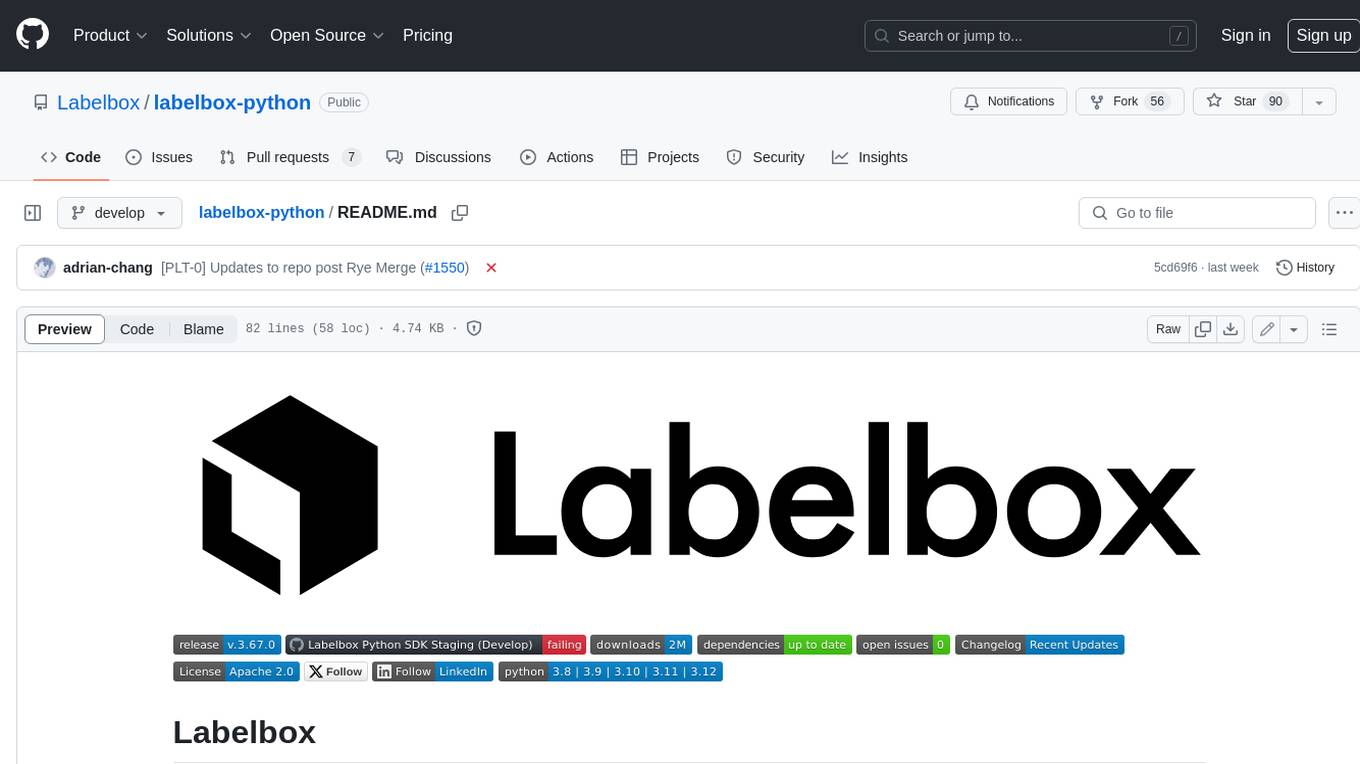
labelbox-python
Labelbox is a data-centric AI platform for enterprises to develop, optimize, and use AI to solve problems and power new products and services. Enterprises use Labelbox to curate data, generate high-quality human feedback data for computer vision and LLMs, evaluate model performance, and automate tasks by combining AI and human-centric workflows. The academic & research community uses Labelbox for cutting-edge AI research.