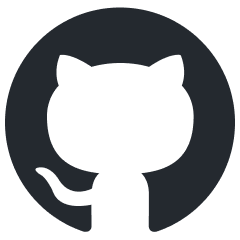
premsql
End-to-End Local-First Text-to-SQL Pipelines
Stars: 90
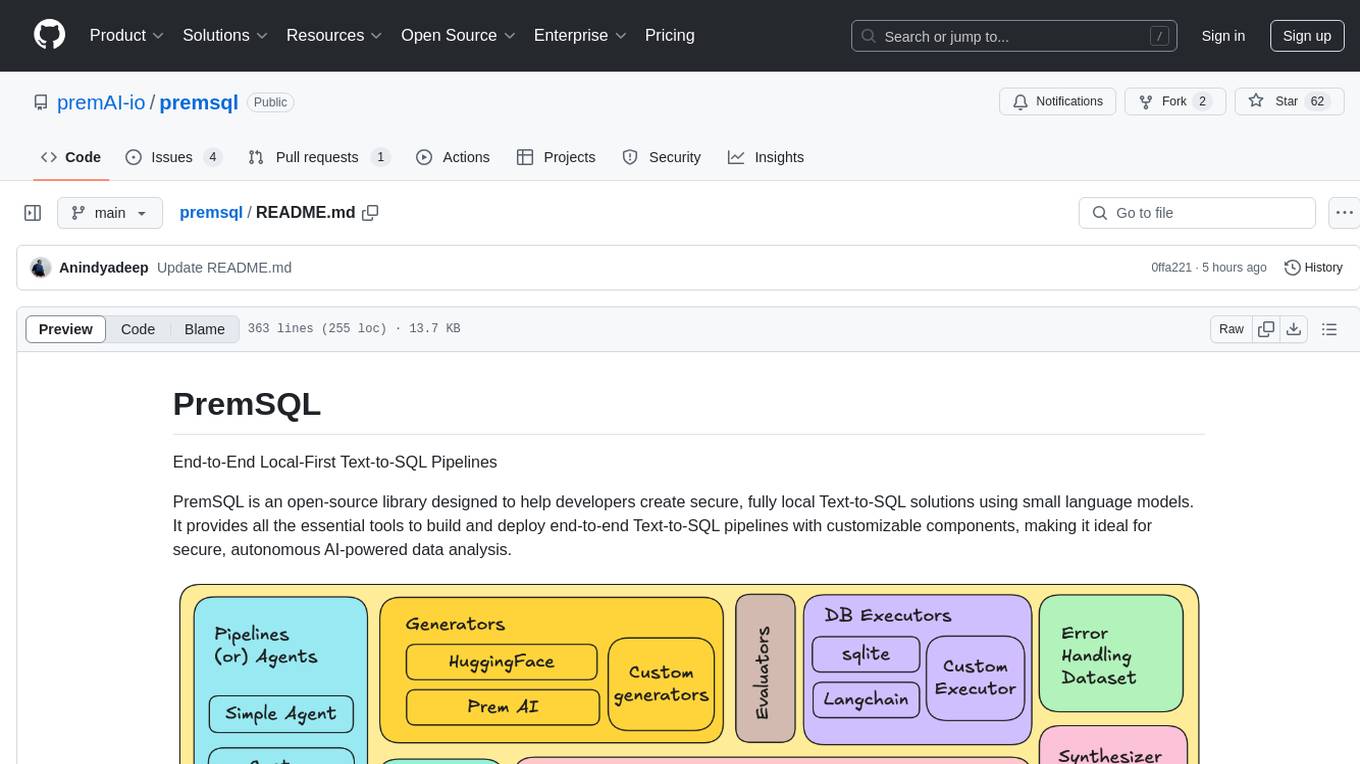
PremSQL is an open-source library designed to help developers create secure, fully local Text-to-SQL solutions using small language models. It provides essential tools for building and deploying end-to-end Text-to-SQL pipelines with customizable components, ideal for secure, autonomous AI-powered data analysis. The library offers features like Local-First approach, Customizable Datasets, Robust Executors and Evaluators, Advanced Generators, Error Handling and Self-Correction, Fine-Tuning Support, and End-to-End Pipelines. Users can fine-tune models, generate SQL queries from natural language inputs, handle errors, and evaluate model performance against predefined metrics. PremSQL is extendible for customization and private data usage.
README:
End-to-End Local-First Text-to-SQL Pipelines
PremSQL is an open-source library designed to help developers create secure, fully local Text-to-SQL solutions using small language models. It provides all the essential tools to build and deploy end-to-end Text-to-SQL pipelines with customizable components, making it ideal for secure, autonomous AI-powered data analysis.
- [Sep 20th 2024] First release of Prem-1B-SQL (51.54% on BirdBench private dataset) | Blog post
- [Sep 10th 2024] First release of PremSQL | Blog Post
- [Blog post]: Using PremSQL to evaluate different open and closed source models
- [Blog post]: State of Text to SQL 2024
- Local-First: Avoid third-party closed-source providers and keep your data secure.
- Customizable Datasets: Create, fine-tune, and evaluate models with built-in or custom datasets.
- Robust Executors and Evaluators: Easily connect to databases and assess model performance.
- Advanced Generators: Convert natural language prompts into executable SQL queries.
- Error Handling and Self-Correction: Automatically correct SQL queries during inference.
- Fine-Tuning Support: Fine-tune models with LoRA, QLoRA, or full fine-tuning strategies.
- End-to-End Pipelines: Seamlessly integrate all components for autonomous data analysis.
Last but not the least, all the features are extendible for your very own customization and private data.
PremSQL requires Python 3.8 or higher. Install the library via pip:
pip install premsql
Hereβs a quick example of how to use PremSQL to generate SQL queries from natural language inputs:
from premsql.pipelines import SimpleText2SQLAgent
from premsql.generators import Text2SQLGeneratorHF
from premsql.executors import SQLiteExecutor
# Provide a SQLite file here or see documentation for more customization
dsn_or_db_path = "./data/db/california_schools.sqlite"
agent = SimpleText2SQLAgent(
dsn_or_db_path=dsn_or_db_path,
generator=Text2SQLGeneratorHF(
model_or_name_or_path="premai-io/prem-1B-SQL",
experiment_name="simple_pipeline",
device="cuda:0",
type="test"
),
)
question = "please list the phone numbers of the direct charter-funded schools that are opened after 2000/1/1"
response = agent.query(question)
response["table"]
PremSQL provides a simple API to use various pre-processed datasets for Text-to-SQL tasks. Text-to-SQL is complex as it requires data dependencies on databases and tables. The premsql datasets help streamline this by providing easy access to datasets and enabling you to create your own datasets with private databases.
Currently, the following datasets are readily available:
Example usage:
from premsql.datasets import Text2SQLDataset
bird_dataset = Text2SQLDataset(
dataset_name='bird', split="train", force_download=False,
dataset_folder="/path/to/your/data" # change this to the path where you want to store the dataset
)
PremSQL generators are responsible for converting natural language questions into SQL queries. Think of these as modular inference APIs specific to text-to-SQL. You can integrate various third-party APIs, models, or custom pipelines.
Example:
from premsql.generators import Text2SQLGeneratorHF
from premsql.datasets import Text2SQLDataset
# Define a dataset
dataset = bird_dataset = Text2SQLDataset(
dataset_name='bird', split="train", force_download=False,
dataset_folder="/path/to/dataset"
).setup_dataset(num_rows=10, num_fewshot=3)
# Define a generator
generator = Text2SQLGeneratorHF(
model_or_name_or_path="premai-io/prem-1B-SQL",
experiment_name="test_generators",
device="cuda:0",
type="test"
)
# Generate on the full dataset
responses = generator.generate_and_save_results(
dataset=bird_dataset,
temperature=0.1,
max_new_tokens=256
)
print(responses)
Results are saved in the experiment_path as predict.json.
We also support execution guided decoding. This strategy executes the generated SQL against the DB and, if it fails, uses the error message for correction, repeating until it gets a valid result or the retries run out.
A quick glance on execution guided decoding:
from premsql.executors import SQLiteExecutor
executor = SQLiteExecutor()
response = generator.generate_and_save_results(
dataset=bird_dataset,
temperature=0.1,
max_new_tokens=256,
force=True,
executor=executor,
max_retries=5 # this is optional (default is already set to 5)
)
An executor executes the generated SQL queries against the database and fetches the results. It is a crucial component in the Text-to-SQL pipeline, as it ensures that the generated SQL queries are valid and return the expected results. PremSQL supports a native executor for SQLite databases and also supports LangChain's SQLDatabase as an executor.
Example usage
from premsql.executors import SQLiteExecutor
# Instantiate the executor
executor = SQLiteExecutor()
# Set a sample dataset path
db_path = "./data/db/california_schools.sqlite"
sql = 'SELECT movie_title FROM movies WHERE movie_release_year = 1945 ORDER BY movie_popularity DESC LIMIT 1'
# execute the SQL
result = executor.execute_sql(
sql=sql,
dsn_or_db_path=db_path
)
print(result)
This will show:
{'result': [('Brief Encounter',)], 'error': None, 'execution_time': 0.03717160224914551}
Executors connect to databases and execute SQL, while evaluators assess the performance of your models against predefined metrics like Execution Accuracy (EX) and Valid Efficiency Score (VES).
Example Usage:
from premsql.executors import SQLiteExecutor
from premsql.evaluator import Text2SQLEvaluator
# Define the executor
executor = SQLiteExecutor()
# Define the evaluator
evaluator = Text2SQLEvaluator(
executor=executor,
experiment_path=generator.experiment_path
)
# Now evaluate the models
results = evaluator.execute(
metric_name="accuracy",
model_responses=response,
filter_by="db_id",
meta_time_out=10
)
print(results)
Using the filter_by
option to filter results by db_id
allows you to see overall accuracy and its distribution across different databases. If a key like difficulty
is available, it will show performance distribution over various difficulty levels. Filtering evaluations by available keys helps in analyzing and understanding model performance empirically. Below is a visualization of model performance across different databases based on the applied filters.
Error-handling prompts are crucial for refining model performance, especially in complex tasks like Text-to-SQL generation. The prompts help the model learn how to handle errors by providing additional context and guidance based on past mistakes. By training on these prompts, the model can self-correct during inference, improving the quality of its output.
Example Error Correction Prompt:
{existing_prompt}
# Generated SQL: {sql}
## Error Message
{error_msg}
Carefully review the original question and error message, then rewrite the SQL query to address the identified issues.
To create a self-correction / error-correction dataset:
- You start with an existing training dataset
- You run an evaluation on that training dataset using an un-trained model.
- You gather the data and pass it to the error-handling prompt
- Finally, you save the results ready to be used for fine-tuning.
Here is the code to get started to make a self-correction dataset using existing datasets:
from premsql.datasets.error_dataset import ErrorDatasetGenerator
from premsql.generators.huggingface import Text2SQLGeneratorHF
from premsql.executors.from_langchain import ExecutorUsingLangChain
from premsql.datasets import BirdDataset
generator = Text2SQLGeneratorHF(
model_or_name_or_path="premai-io/prem-1B-SQL",
experiment_name="testing_error_gen",
type="train", # do not type: 'test' since this will be used during training
device="cuda:0"
)
executor = ExecutorUsingLangChain()
bird_train = BirdDataset(
split="train",
dataset_folder="/path/to/dataset"
).setup_dataset(num_rows=10)
error_dataset_gen = ErrorDatasetGenerator(generator=generator, executor=executor)
error_dataset = error_dataset_gen.generate_and_save(
datasets=bird_train,
force=True
)
premsql tuner
is a module designed to fine-tune models specifically for text-to-SQL tasks. The module offers multiple ways of fine-tuning, providing flexibility based on your project's needs.
- Full Fine-Tuning: Standard model fine-tuning with all its parameters.
- PEFT using LoRA: Parameter-efficient-fine-tuning with LoRA (Low-Rank Adaptation) for faster and more efficient training.
- PEFT using QLoRA: Another PEFT approach using Quantized LoRA, optimizing resource use during training.
In addition to these methods, you can create custom fine-tuning pipelines using the components and tools provided by premsql.
PremSQL pipelines are end-to-end solutions that connect to your database and generate SQL queries from natural language questions, providing complete control over your data analysis workflows.
Example Simple Pipeline:
from premsql.pipelines.simple import SimpleText2SQLAgent
from premsql.generators.huggingface import Text2SQLGeneratorHF
from langchain_community.utilities.sql_database import SQLDatabase
from premsql.utils import convert_sqlite_path_to_dsn
# Change it some SQLite database path or any other DB URI connection.
dsn_or_db_path = convert_sqlite_path_to_dsn(
"../data/bird/test/test_databases/california_schools/california_schools.sqlite"
)
db = SQLDatabase.from_uri(dsn_or_db_path)
agent = SimpleText2SQLAgent(
dsn_or_db_path=db,
generator=Text2SQLGeneratorHF(
model_or_name_or_path="premai-io/prem-1B-SQL",
experiment_name="test_nli",
device="cuda:0",
type="test"
),
)
response = agent.query(
question="please list the phone numbers of the direct charter-funded schools that are opened after 2000/1/1",
)
response["table"]
We welcome contributions from the community! If youβd like to contribute to PremSQL, please follow these guidelines:
- Fork the repository and clone your fork.
- Create a new branch for your feature or bug fix.
- Make your changes and ensure the code passes all tests.
- Submit a pull request with a clear description of your changes.
For detailed guidelines, please check the CONTRIBUTING.md.
PremSQL is continuously evolving, with exciting features planned for future releases:
- Synthesizer Component: A tool to generate synthetic datasets from private data, enabling fully private text-to-SQL workflows and enhancing model fine-tuning capabilities.
- Agentic Pipelines with Function-Calling Features: Advanced pipelines with graph plotting, natural language analysis, and other enhancements to provide a more versatile and powerful system.
- Training Better Small Language Models: Ongoing training and optimization of small language models specifically tailored to PremSQLβs unique requirements, ensuring efficient and effective performance in text-to-SQL tasks.
- Optimization of Generators and Executors: Improvements to enhance the robustness of existing components, including parallel processing to speed up generation and execution times.
- Standard Tests and Stability Improvements: Introduction of comprehensive tests for greater stability of the library and the planned rollout of a simple user interface to improve the overall user experience.
Stay tuned for these exciting updates! We encourage you to contribute and provide feedback to help us shape the future of PremSQL.
PremSQL is licensed under the MIT License. See the LICENSE file for more information.
For Tasks:
Click tags to check more tools for each tasksFor Jobs:
Alternative AI tools for premsql
Similar Open Source Tools
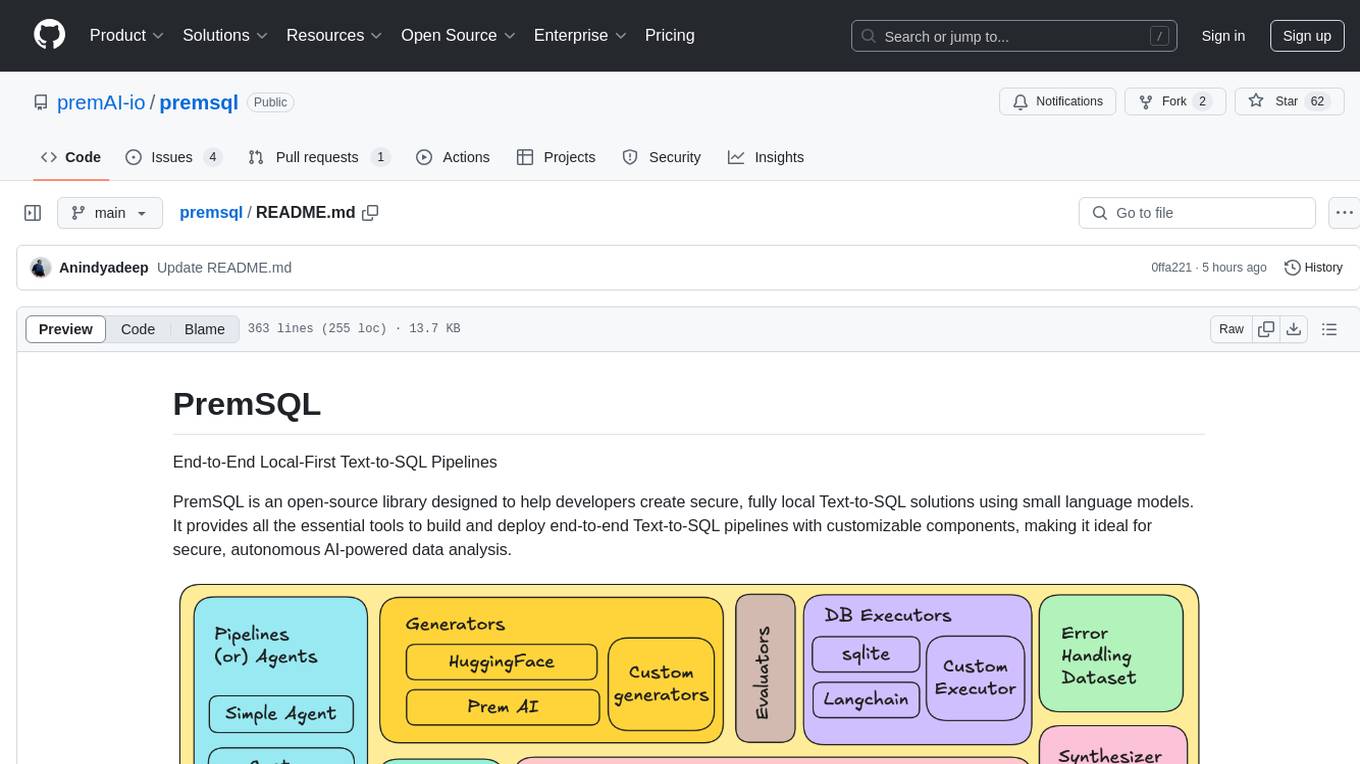
premsql
PremSQL is an open-source library designed to help developers create secure, fully local Text-to-SQL solutions using small language models. It provides essential tools for building and deploying end-to-end Text-to-SQL pipelines with customizable components, ideal for secure, autonomous AI-powered data analysis. The library offers features like Local-First approach, Customizable Datasets, Robust Executors and Evaluators, Advanced Generators, Error Handling and Self-Correction, Fine-Tuning Support, and End-to-End Pipelines. Users can fine-tune models, generate SQL queries from natural language inputs, handle errors, and evaluate model performance against predefined metrics. PremSQL is extendible for customization and private data usage.
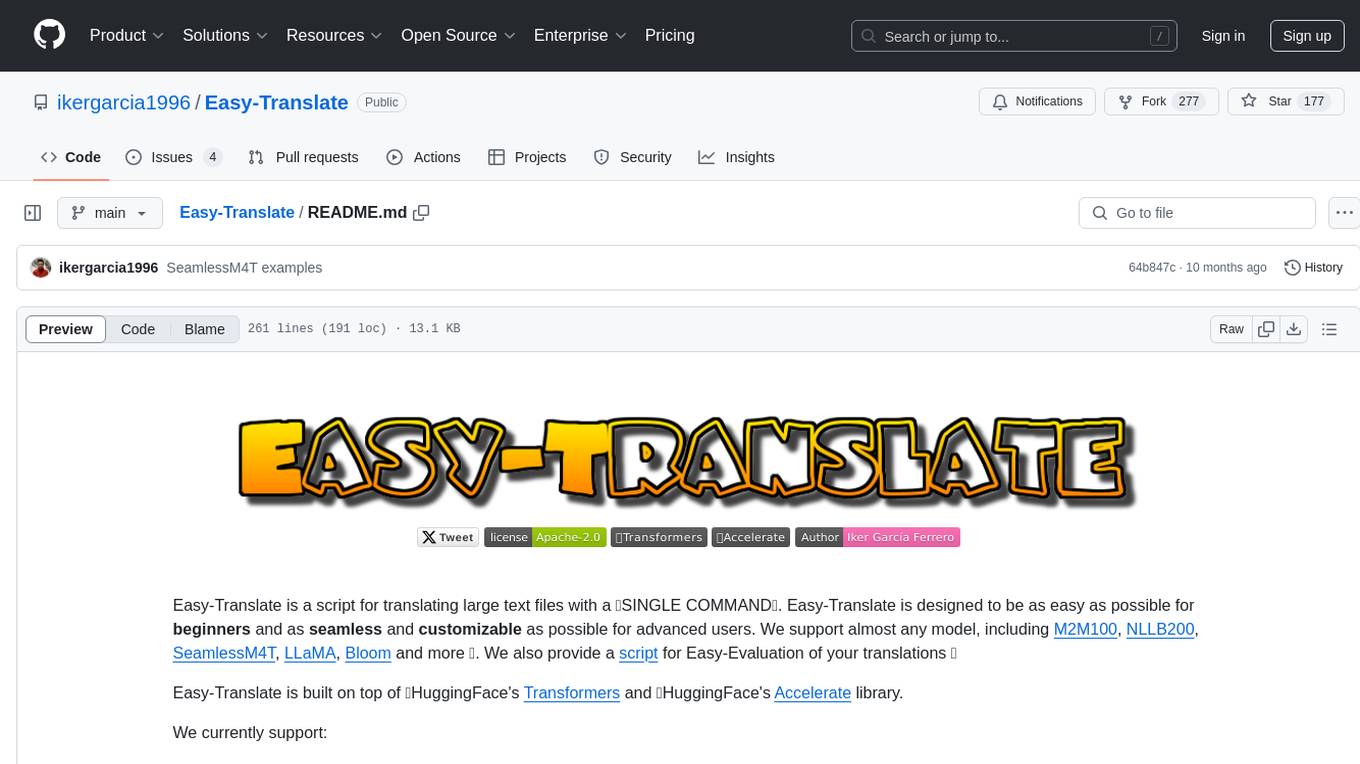
Easy-Translate
Easy-Translate is a script designed for translating large text files with a single command. It supports various models like M2M100, NLLB200, SeamlessM4T, LLaMA, and Bloom. The tool is beginner-friendly and offers seamless and customizable features for advanced users. It allows acceleration on CPU, multi-CPU, GPU, multi-GPU, and TPU, with support for different precisions and decoding strategies. Easy-Translate also provides an evaluation script for translations. Built on HuggingFace's Transformers and Accelerate library, it supports prompt usage and loading huge models efficiently.
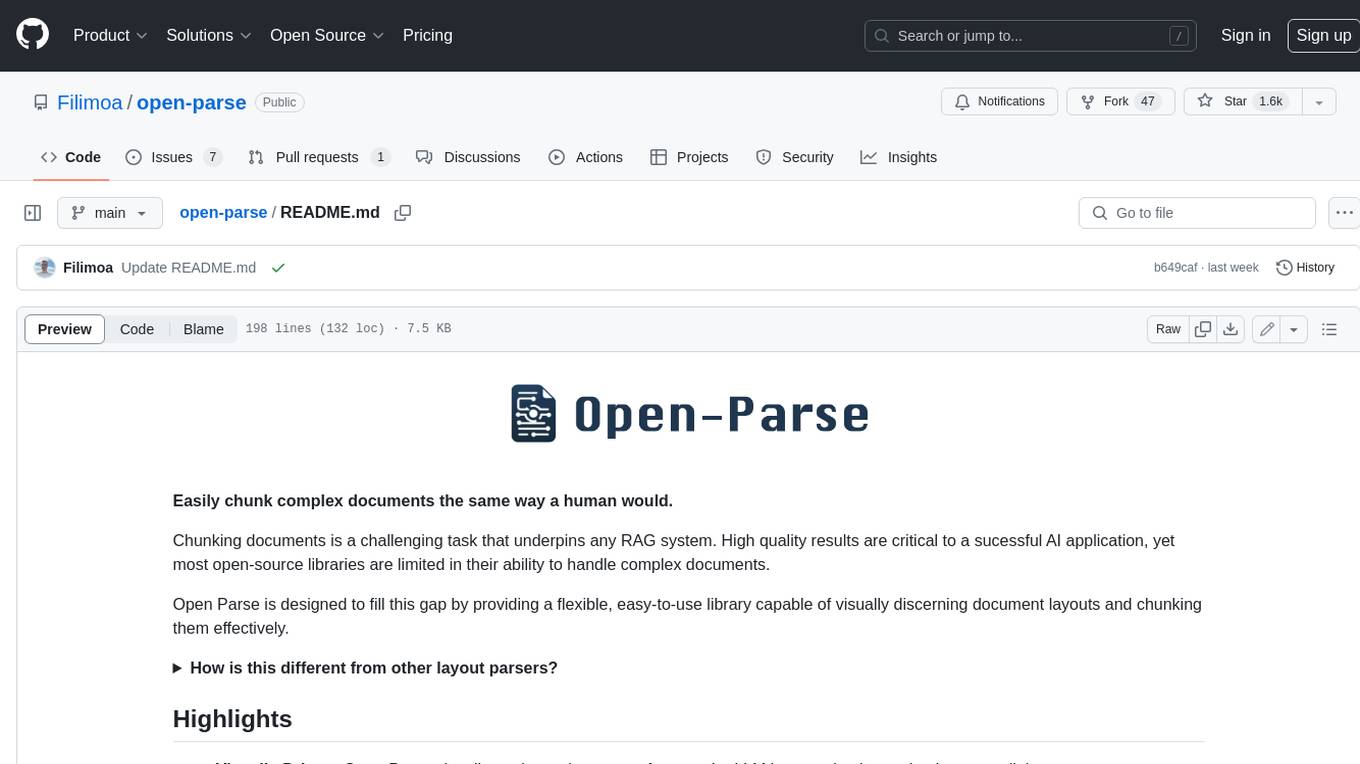
open-parse
Open Parse is a Python library for visually discerning document layouts and chunking them effectively. It is designed to fill the gap in open-source libraries for handling complex documents. Unlike text splitting, which converts a file to raw text and slices it up, Open Parse visually analyzes documents for superior LLM input. It also supports basic markdown for parsing headings, bold, and italics, and has high-precision table support, extracting tables into clean Markdown formats with accuracy that surpasses traditional tools. Open Parse is extensible, allowing users to easily implement their own post-processing steps. It is also intuitive, with great editor support and completion everywhere, making it easy to use and learn.
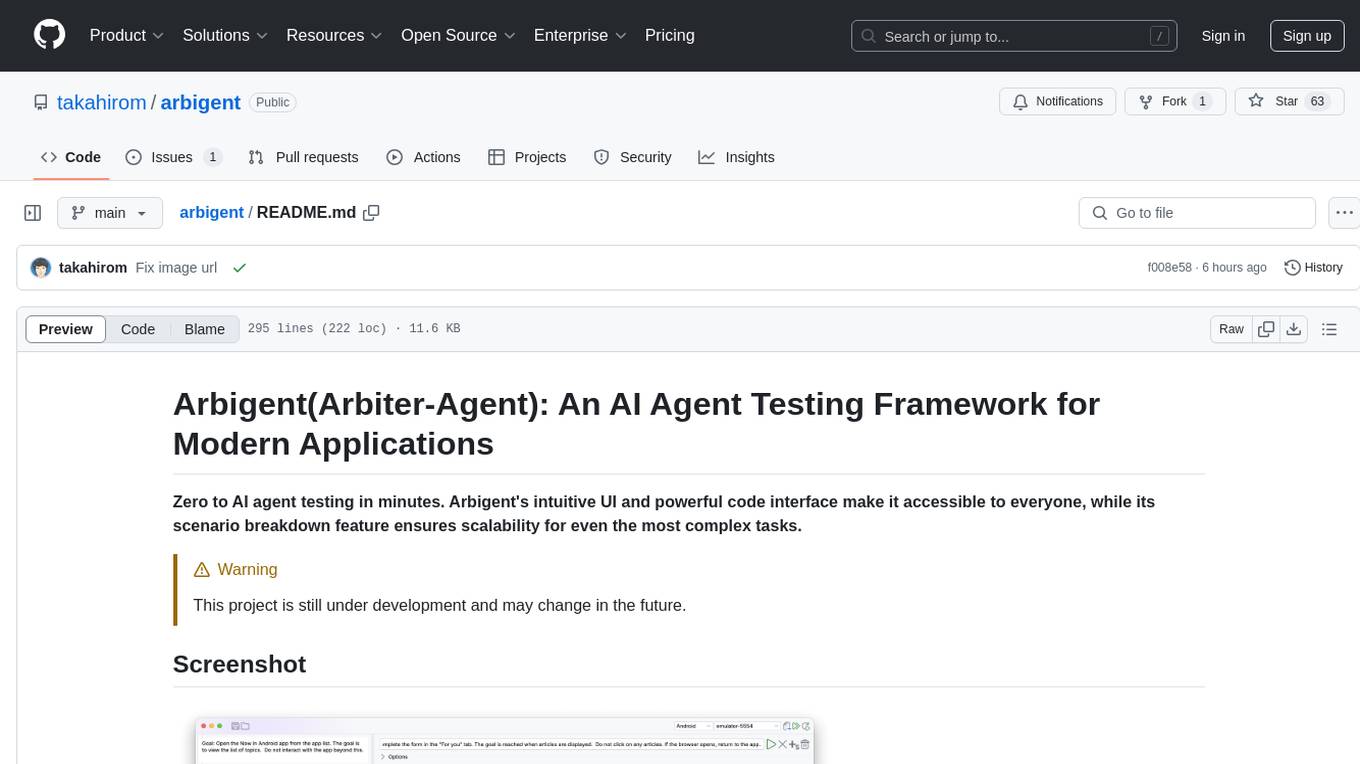
arbigent
Arbigent (Arbiter-Agent) is an AI agent testing framework designed to make AI agent testing practical for modern applications. It addresses challenges faced by traditional UI testing frameworks and AI agents by breaking down complex tasks into smaller, dependent scenarios. The framework is customizable for various AI providers, operating systems, and form factors, empowering users with extensive customization capabilities. Arbigent offers an intuitive UI for scenario creation and a powerful code interface for seamless test execution. It supports multiple form factors, optimizes UI for AI interaction, and is cost-effective by utilizing models like GPT-4o mini. With a flexible code interface and open-source nature, Arbigent aims to revolutionize AI agent testing in modern applications.
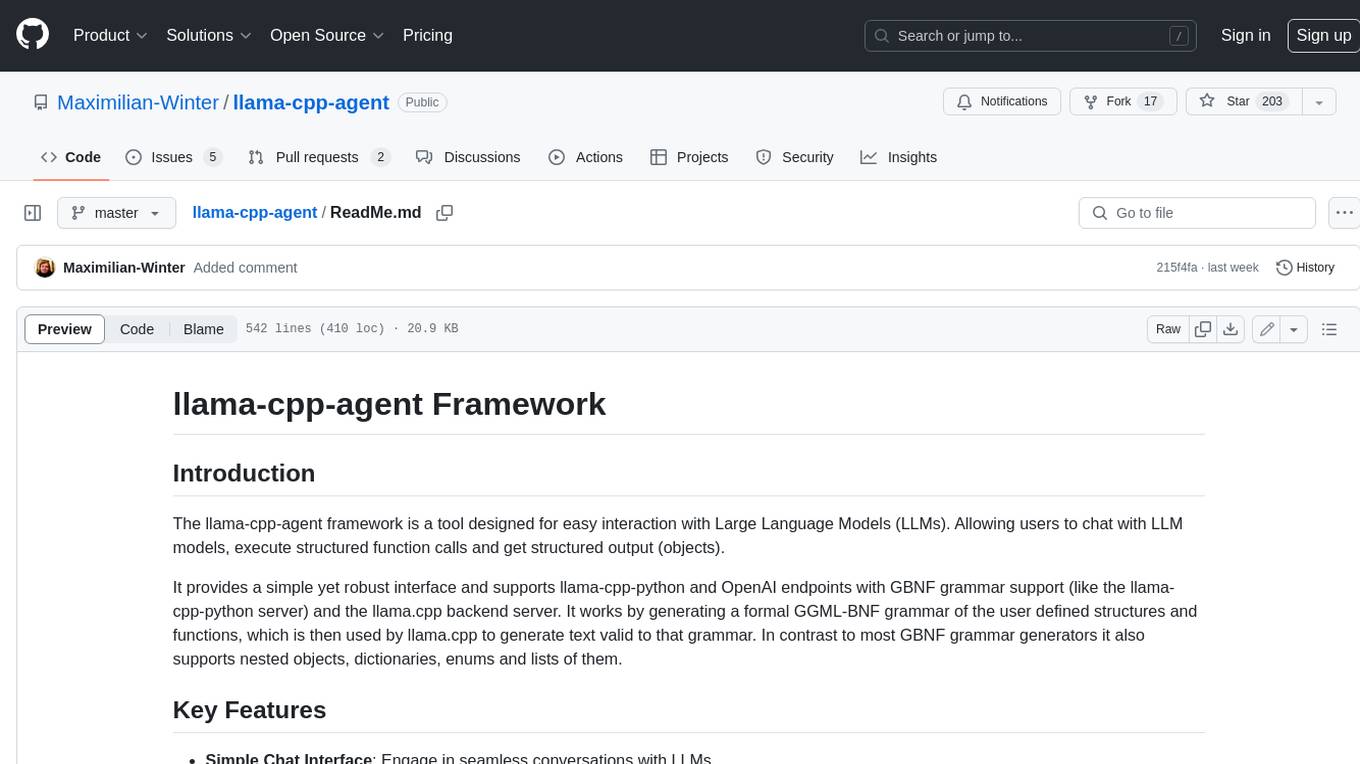
llama-cpp-agent
The llama-cpp-agent framework is a tool designed for easy interaction with Large Language Models (LLMs). Allowing users to chat with LLM models, execute structured function calls and get structured output (objects). It provides a simple yet robust interface and supports llama-cpp-python and OpenAI endpoints with GBNF grammar support (like the llama-cpp-python server) and the llama.cpp backend server. It works by generating a formal GGML-BNF grammar of the user defined structures and functions, which is then used by llama.cpp to generate text valid to that grammar. In contrast to most GBNF grammar generators it also supports nested objects, dictionaries, enums and lists of them.
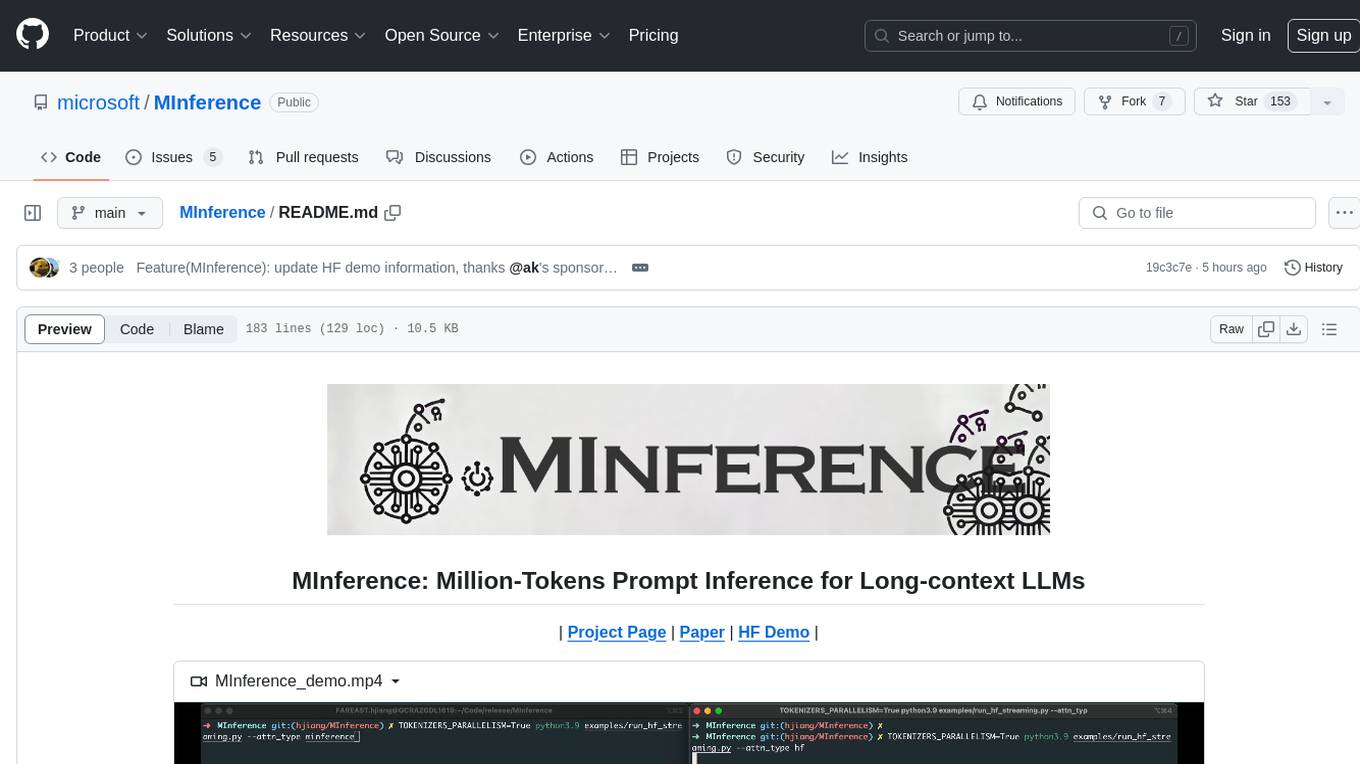
MInference
MInference is a tool designed to accelerate pre-filling for long-context Language Models (LLMs) by leveraging dynamic sparse attention. It achieves up to a 10x speedup for pre-filling on an A100 while maintaining accuracy. The tool supports various decoding LLMs, including LLaMA-style models and Phi models, and provides custom kernels for attention computation. MInference is useful for researchers and developers working with large-scale language models who aim to improve efficiency without compromising accuracy.
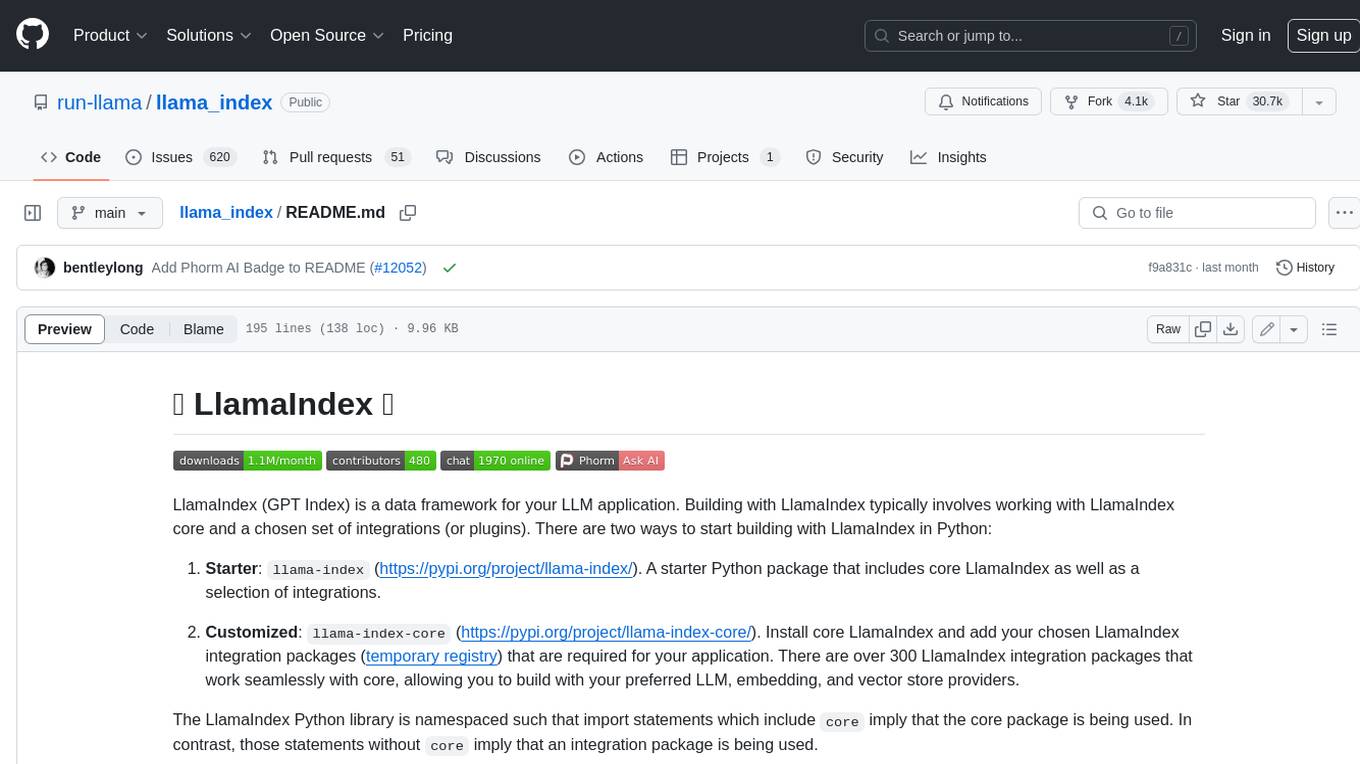
llama_index
LlamaIndex is a data framework for building LLM applications. It provides tools for ingesting, structuring, and querying data, as well as integrating with LLMs and other tools. LlamaIndex is designed to be easy to use for both beginner and advanced users, and it provides a comprehensive set of features for building LLM applications.
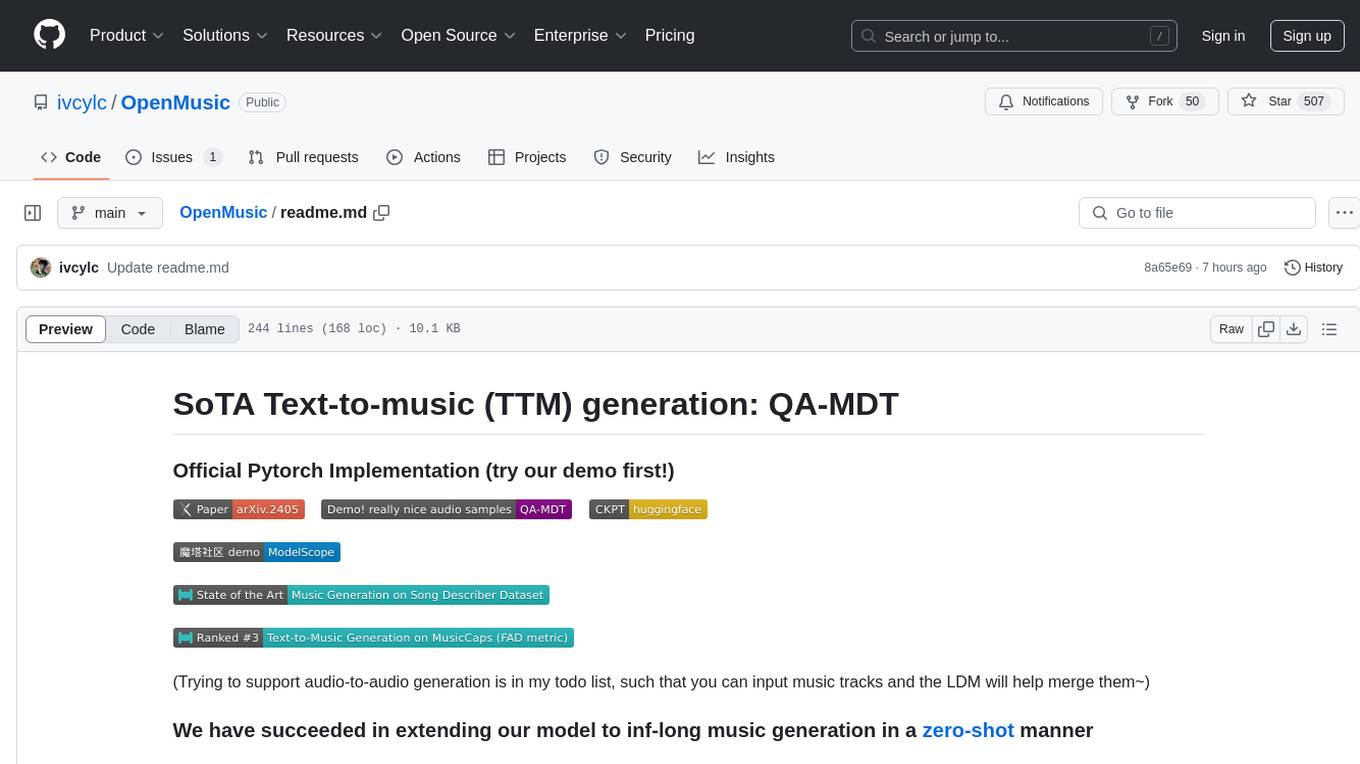
OpenMusic
OpenMusic is a repository providing an implementation of QA-MDT, a Quality-Aware Masked Diffusion Transformer for music generation. The code integrates state-of-the-art models and offers training strategies for music generation. The repository includes implementations of AudioLDM, PixArt-alpha, MDT, AudioMAE, and Open-Sora. Users can train or fine-tune the model using different strategies and datasets. The model is well-pretrained and can be used for music generation tasks. The repository also includes instructions for preparing datasets, training the model, and performing inference. Contact information is provided for any questions or suggestions regarding the project.
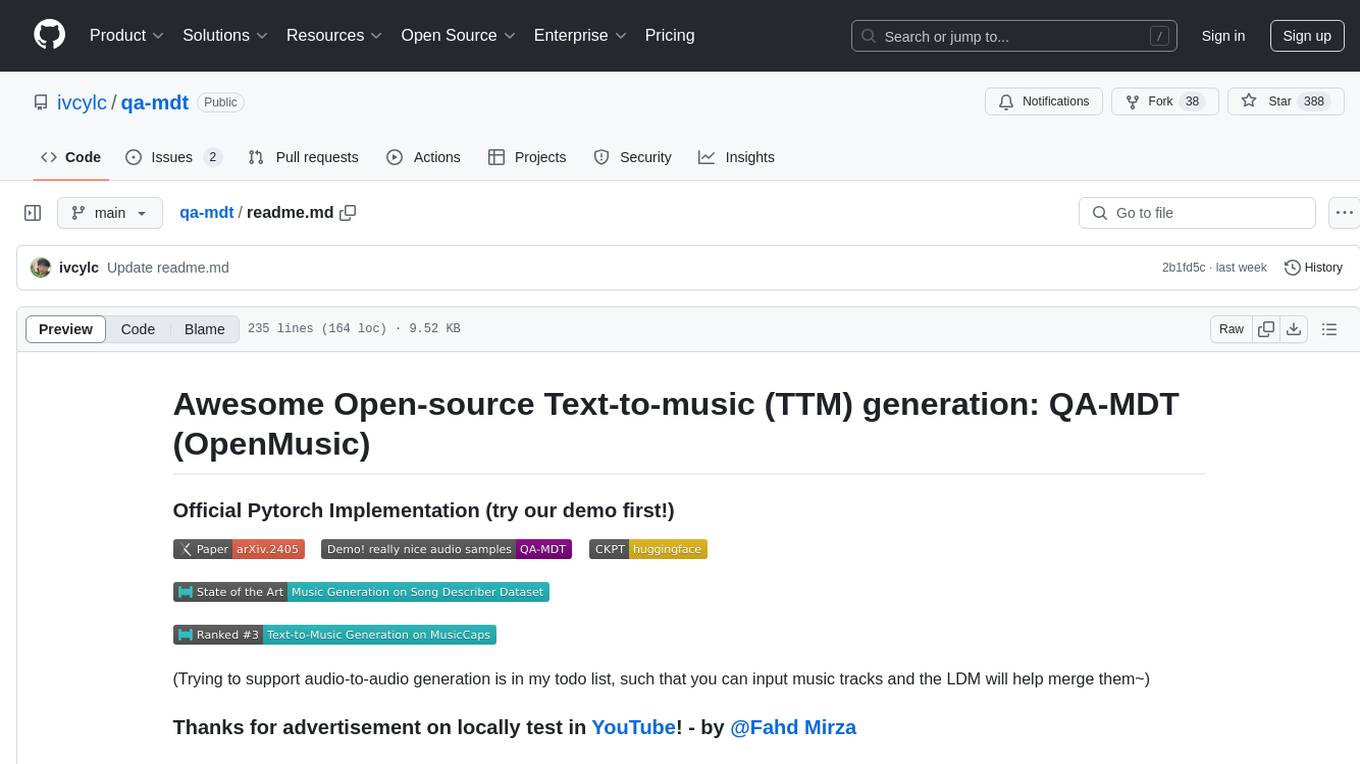
qa-mdt
This repository provides an implementation of QA-MDT, integrating state-of-the-art models for music generation. It offers a Quality-Aware Masked Diffusion Transformer for enhanced music generation. The code is based on various repositories like AudioLDM, PixArt-alpha, MDT, AudioMAE, and Open-Sora. The implementation allows for training and fine-tuning the model with different strategies and datasets. The repository also includes instructions for preparing datasets in LMDB format and provides a script for creating a toy LMDB dataset. The model can be used for music generation tasks, with a focus on quality injection to enhance the musicality of generated music.
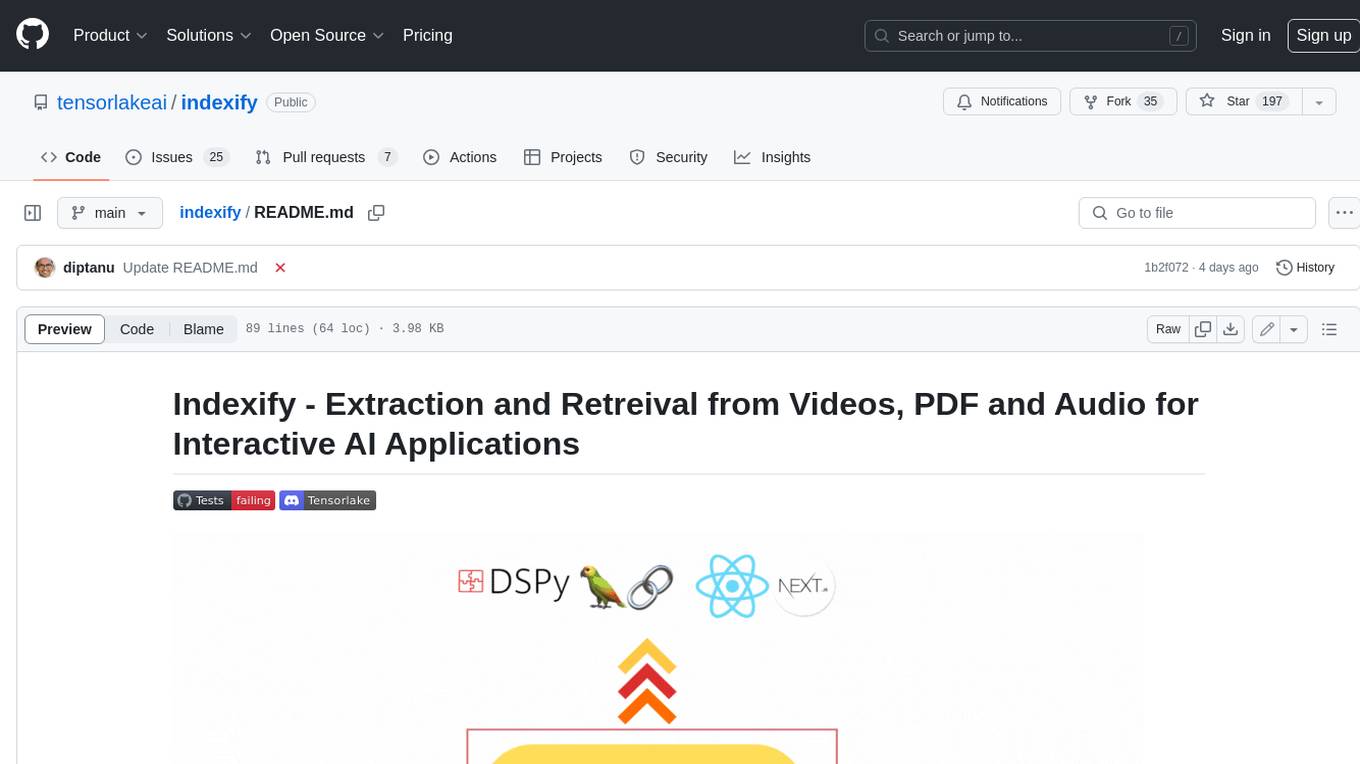
indexify
Indexify is an open-source engine for building fast data pipelines for unstructured data (video, audio, images, and documents) using reusable extractors for embedding, transformation, and feature extraction. LLM Applications can query transformed content friendly to LLMs by semantic search and SQL queries. Indexify keeps vector databases and structured databases (PostgreSQL) updated by automatically invoking the pipelines as new data is ingested into the system from external data sources. **Why use Indexify** * Makes Unstructured Data **Queryable** with **SQL** and **Semantic Search** * **Real-Time** Extraction Engine to keep indexes **automatically** updated as new data is ingested. * Create **Extraction Graph** to describe **data transformation** and extraction of **embedding** and **structured extraction**. * **Incremental Extraction** and **Selective Deletion** when content is deleted or updated. * **Extractor SDK** allows adding new extraction capabilities, and many readily available extractors for **PDF**, **Image**, and **Video** indexing and extraction. * Works with **any LLM Framework** including **Langchain**, **DSPy**, etc. * Runs on your laptop during **prototyping** and also scales to **1000s of machines** on the cloud. * Works with many **Blob Stores**, **Vector Stores**, and **Structured Databases** * We have even **Open Sourced Automation** to deploy to Kubernetes in production.
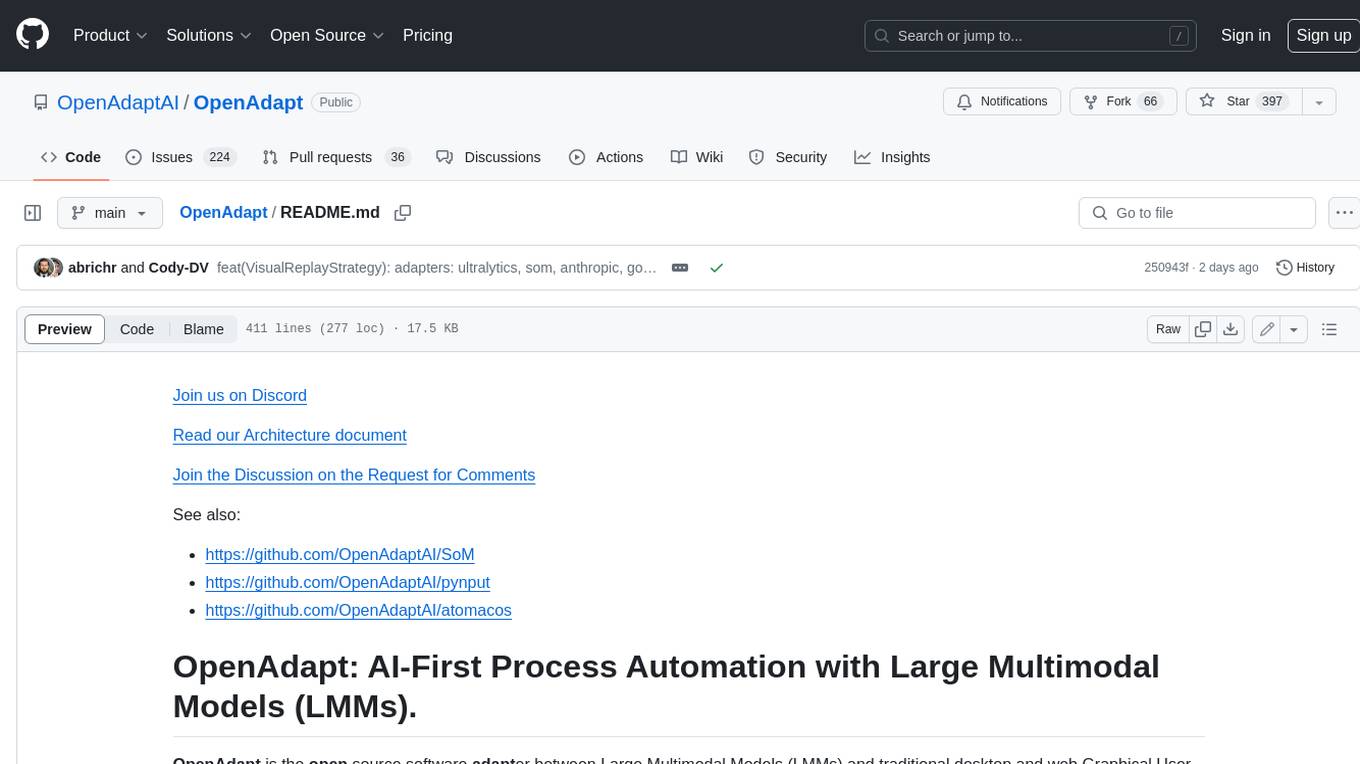
OpenAdapt
OpenAdapt is an open-source software adapter between Large Multimodal Models (LMMs) and traditional desktop and web Graphical User Interfaces (GUIs). It aims to automate repetitive GUI workflows by leveraging the power of LMMs. OpenAdapt records user input and screenshots, converts them into tokenized format, and generates synthetic input via transformer model completions. It also analyzes recordings to generate task trees and replay synthetic input to complete tasks. OpenAdapt is model agnostic and generates prompts automatically by learning from human demonstration, ensuring that agents are grounded in existing processes and mitigating hallucinations. It works with all types of desktop GUIs, including virtualized and web, and is open source under the MIT license.
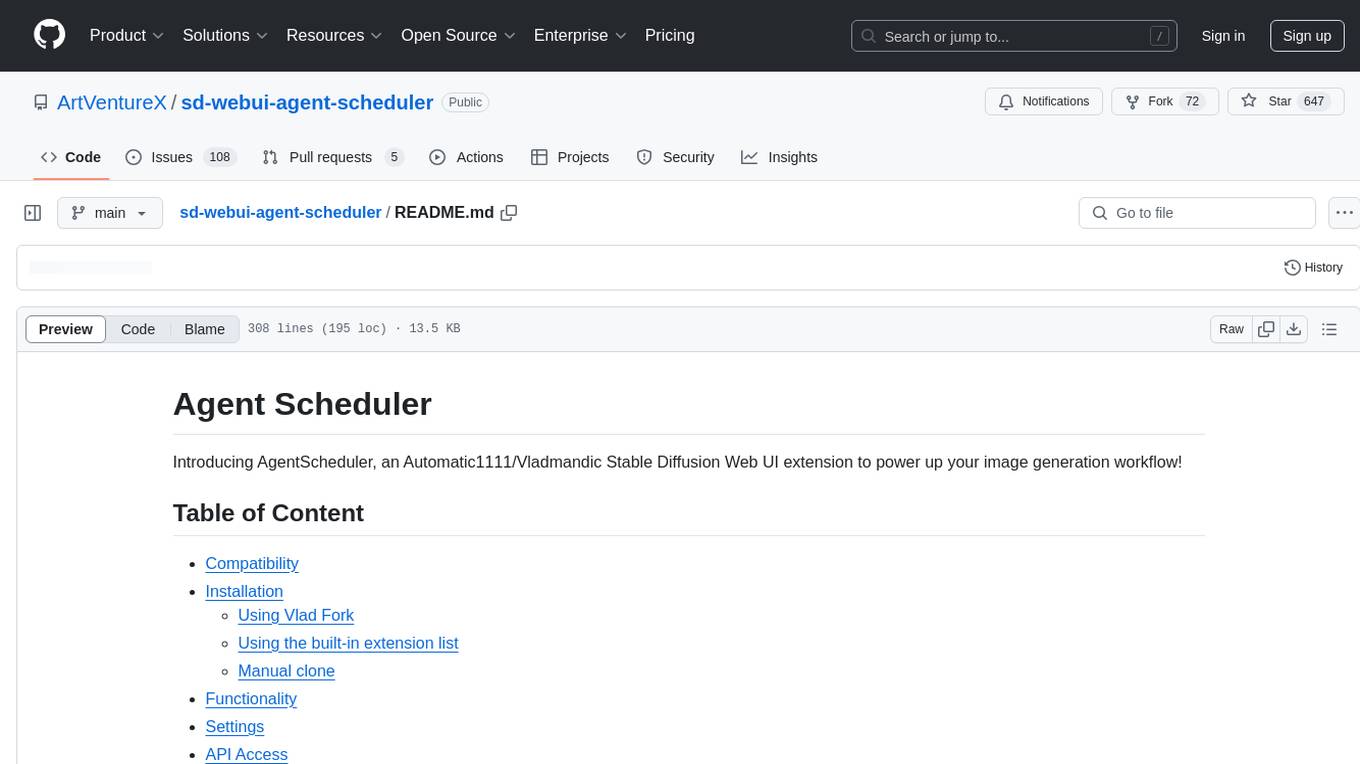
sd-webui-agent-scheduler
AgentScheduler is an Automatic/Vladmandic Stable Diffusion Web UI extension designed to enhance image generation workflows. It allows users to enqueue prompts, settings, and controlnets, manage queued tasks, prioritize, pause, resume, and delete tasks, view generation results, and more. The extension offers hidden features like queuing checkpoints, editing queued tasks, and custom checkpoint selection. Users can access the functionality through HTTP APIs and API callbacks. Troubleshooting steps are provided for common errors. The extension is compatible with latest versions of A1111 and Vladmandic. It is licensed under Apache License 2.0.
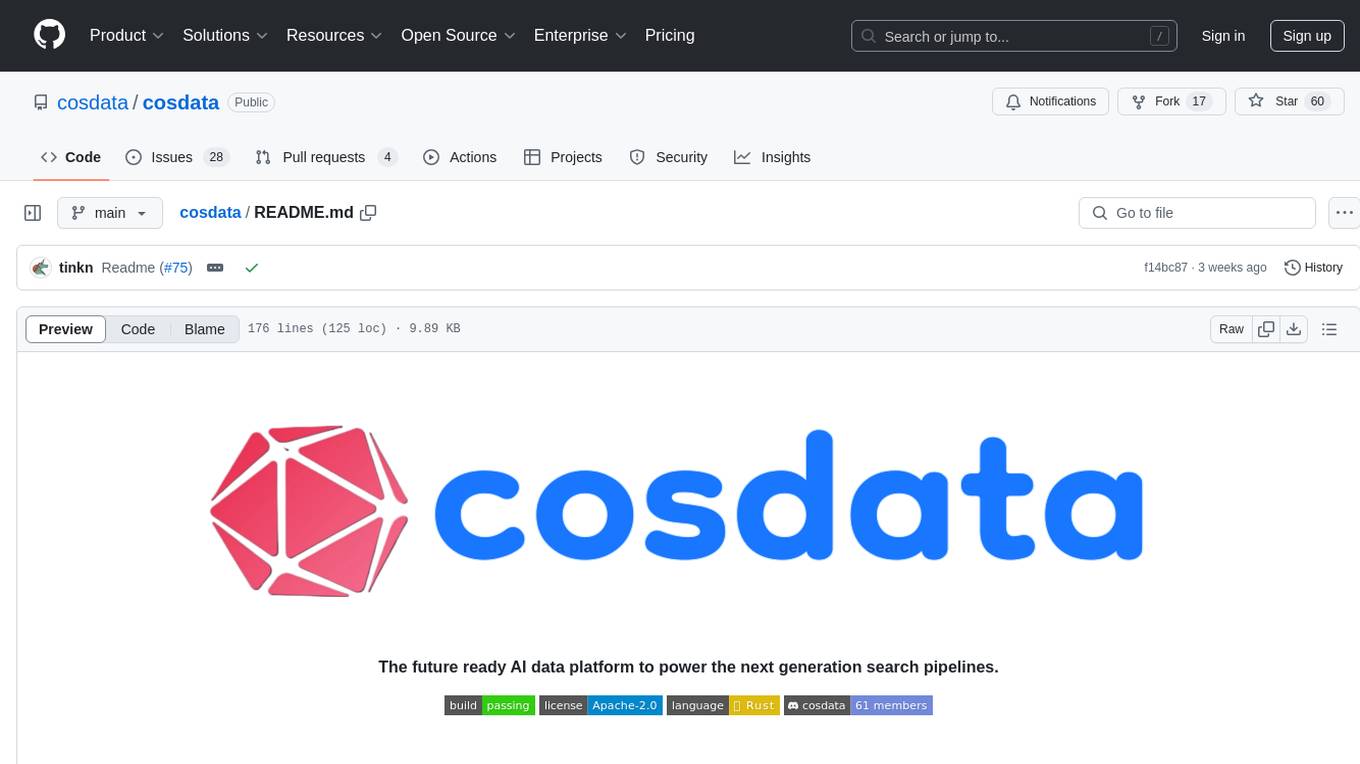
cosdata
Cosdata is a cutting-edge AI data platform designed to power the next generation search pipelines. It features immutability, version control, and excels in semantic search, structured knowledge graphs, hybrid search capabilities, real-time search at scale, and ML pipeline integration. The platform is customizable, scalable, efficient, enterprise-grade, easy to use, and can manage multi-modal data. It offers high performance, indexing, low latency, and high requests per second. Cosdata is designed to meet the demands of modern search applications, empowering businesses to harness the full potential of their data.
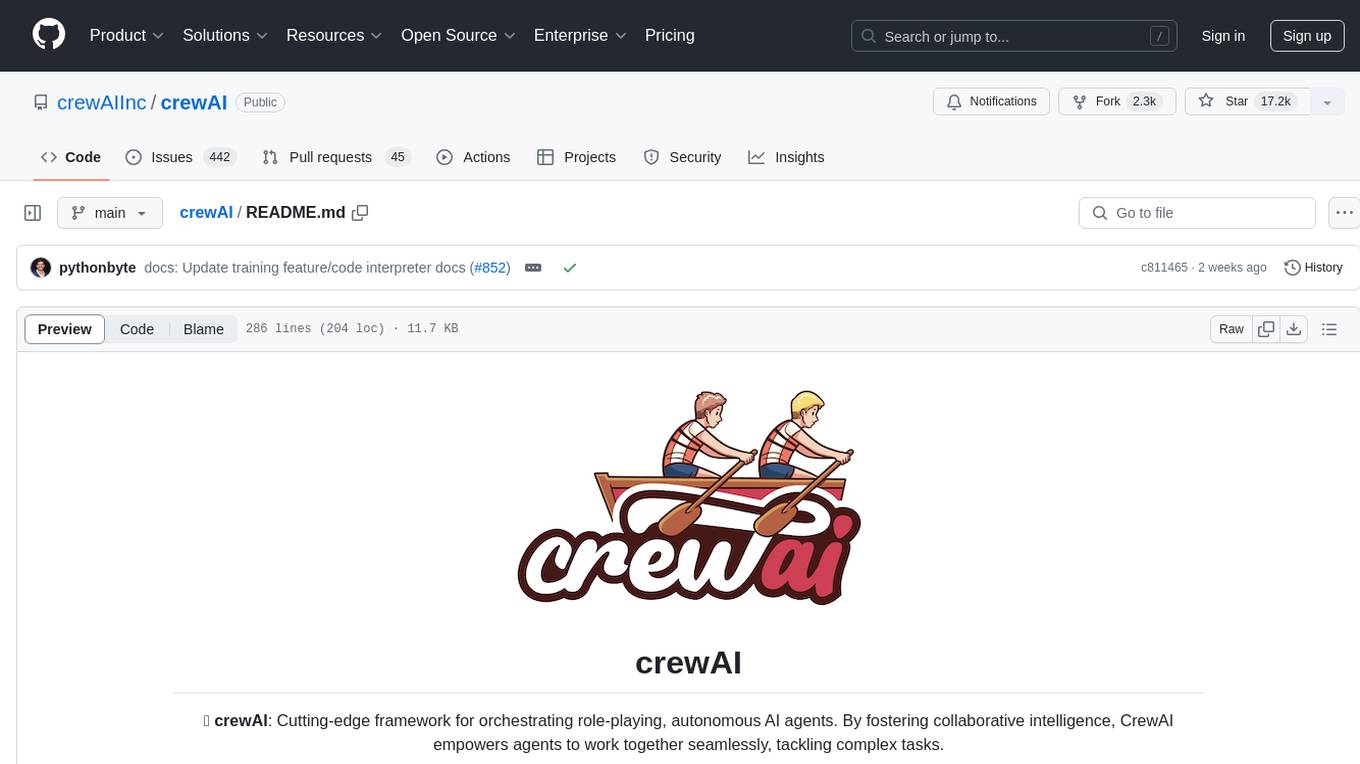
crewAI
CrewAI is a cutting-edge framework designed to orchestrate role-playing autonomous AI agents. By fostering collaborative intelligence, CrewAI empowers agents to work together seamlessly, tackling complex tasks. It enables AI agents to assume roles, share goals, and operate in a cohesive unit, much like a well-oiled crew. Whether you're building a smart assistant platform, an automated customer service ensemble, or a multi-agent research team, CrewAI provides the backbone for sophisticated multi-agent interactions. With features like role-based agent design, autonomous inter-agent delegation, flexible task management, and support for various LLMs, CrewAI offers a dynamic and adaptable solution for both development and production workflows.
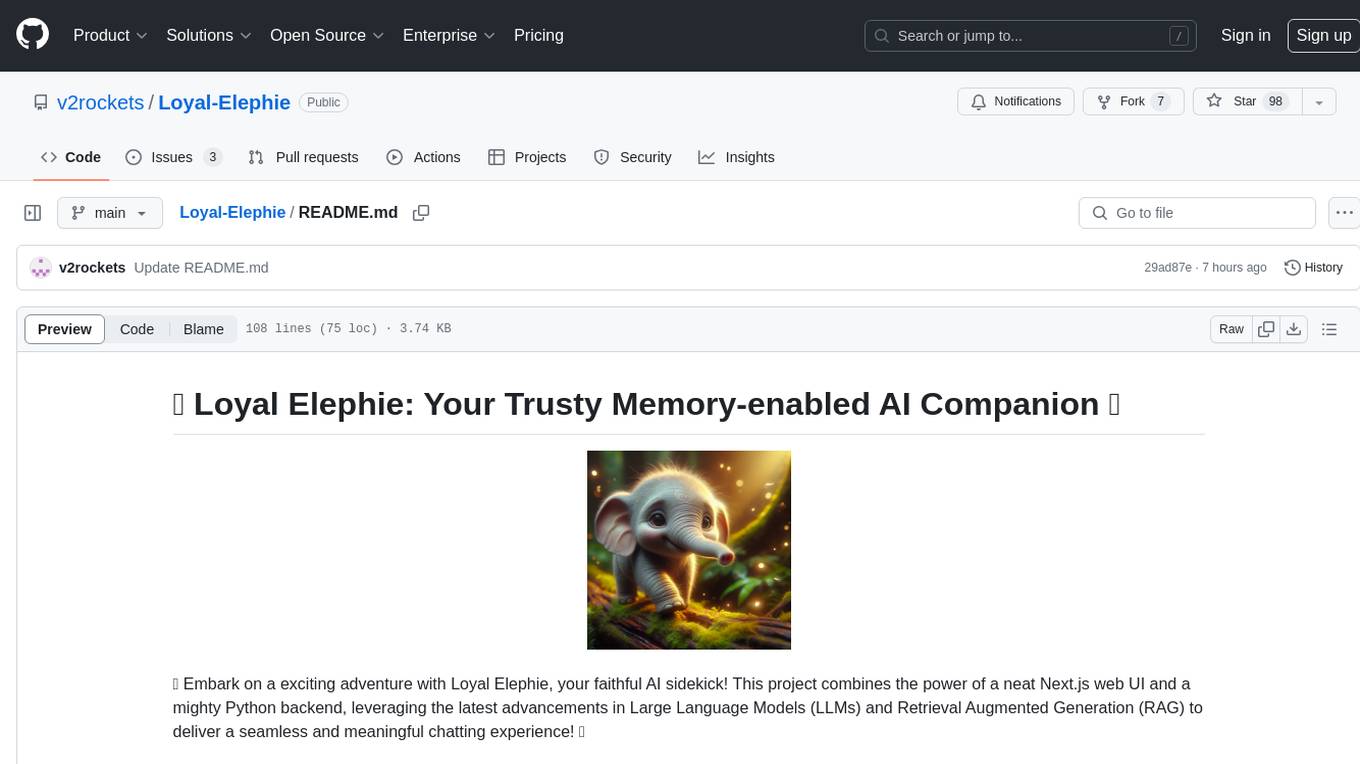
Loyal-Elephie
Embark on an exciting adventure with Loyal Elephie, your faithful AI sidekick! This project combines the power of a neat Next.js web UI and a mighty Python backend, leveraging the latest advancements in Large Language Models (LLMs) and Retrieval Augmented Generation (RAG) to deliver a seamless and meaningful chatting experience. Features include controllable memory, hybrid search, secure web access, streamlined LLM agent, and optional Markdown editor integration. Loyal Elephie supports both open and proprietary LLMs and embeddings serving as OpenAI compatible APIs.
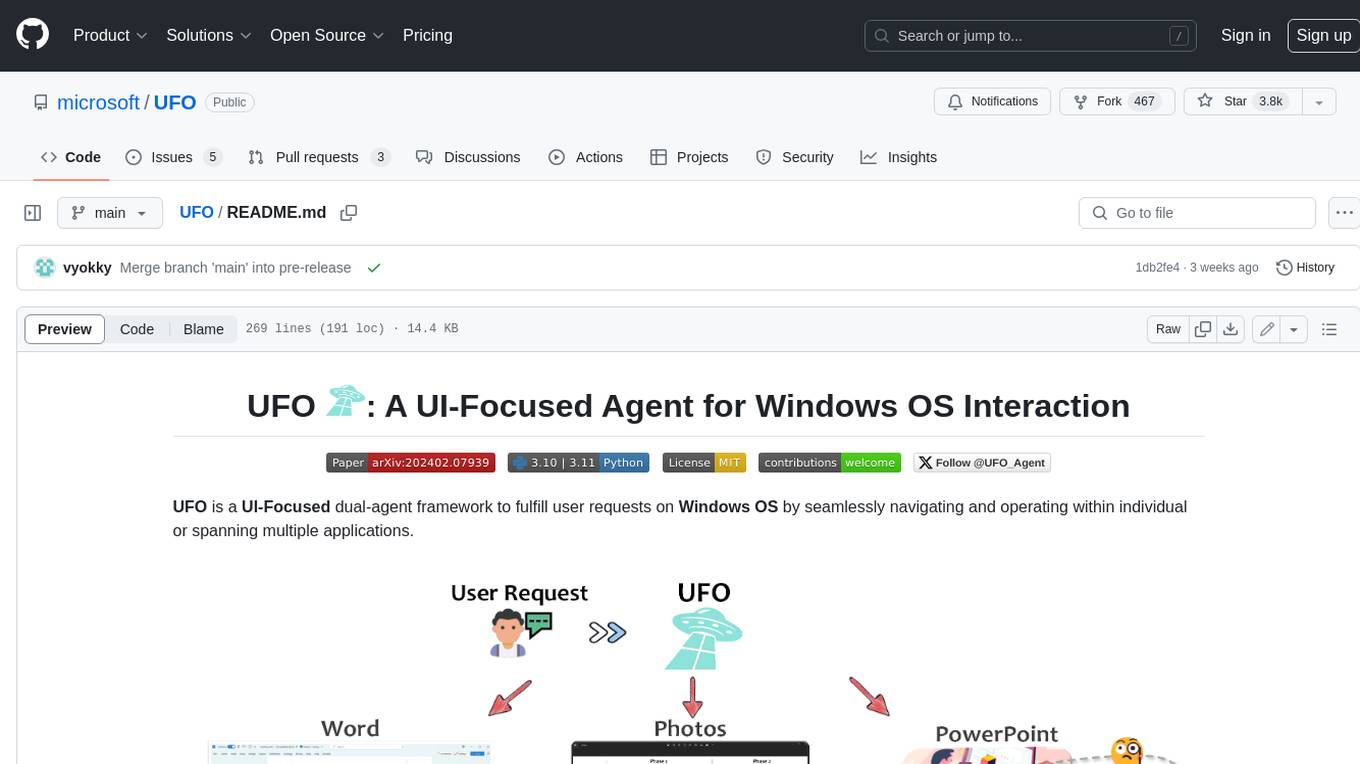
UFO
UFO is a UI-focused dual-agent framework to fulfill user requests on Windows OS by seamlessly navigating and operating within individual or spanning multiple applications.
For similar tasks
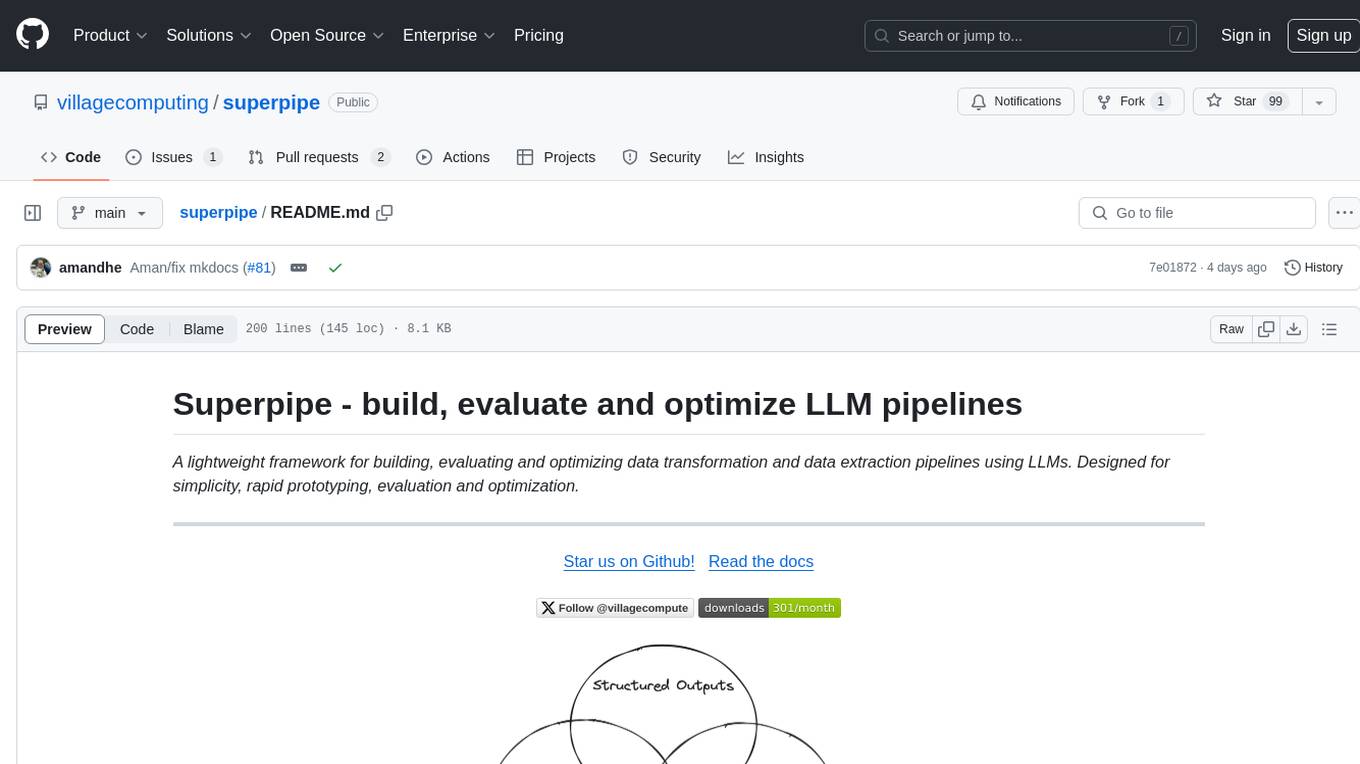
superpipe
Superpipe is a lightweight framework designed for building, evaluating, and optimizing data transformation and data extraction pipelines using LLMs. It allows users to easily combine their favorite LLM libraries with Superpipe's building blocks to create pipelines tailored to their unique data and use cases. The tool facilitates rapid prototyping, evaluation, and optimization of end-to-end pipelines for tasks such as classification and evaluation of job departments based on work history. Superpipe also provides functionalities for evaluating pipeline performance, optimizing parameters for cost, accuracy, and speed, and conducting grid searches to experiment with different models and prompts.
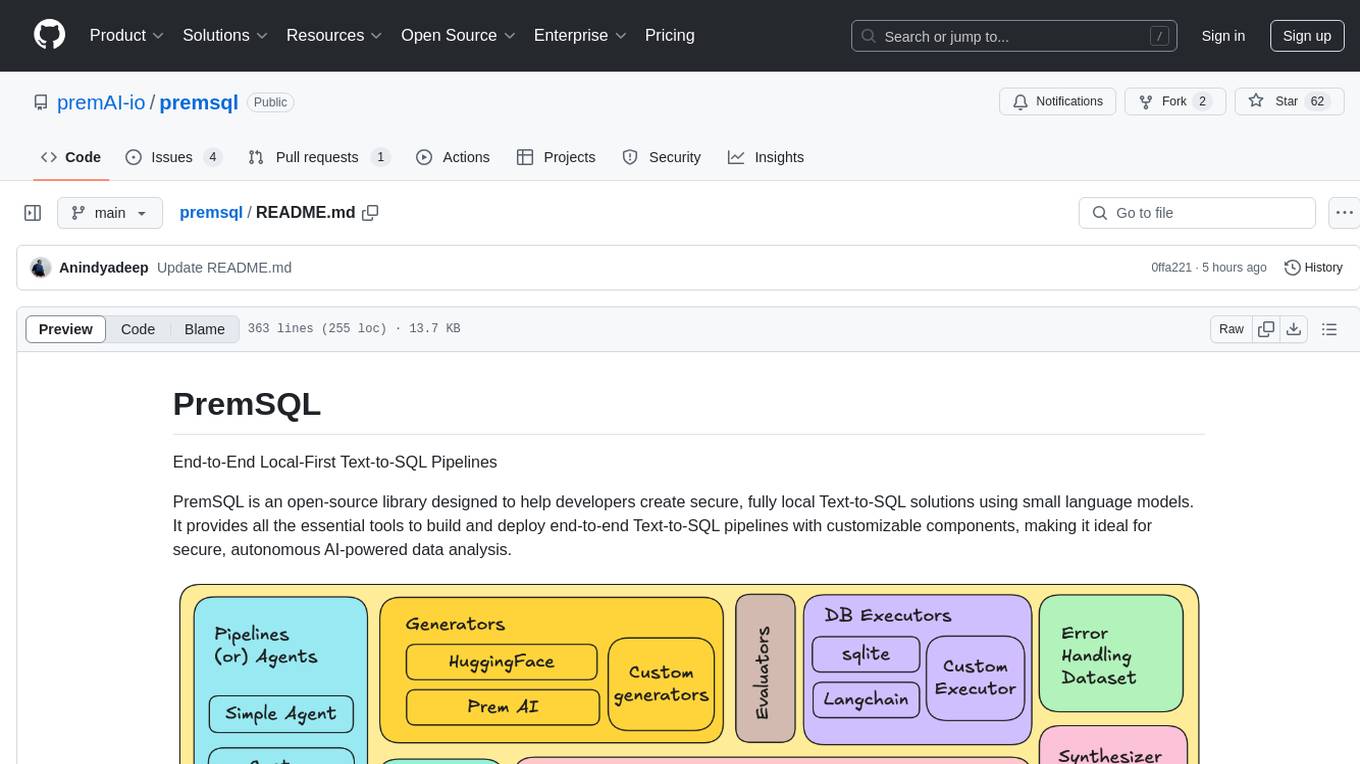
premsql
PremSQL is an open-source library designed to help developers create secure, fully local Text-to-SQL solutions using small language models. It provides essential tools for building and deploying end-to-end Text-to-SQL pipelines with customizable components, ideal for secure, autonomous AI-powered data analysis. The library offers features like Local-First approach, Customizable Datasets, Robust Executors and Evaluators, Advanced Generators, Error Handling and Self-Correction, Fine-Tuning Support, and End-to-End Pipelines. Users can fine-tune models, generate SQL queries from natural language inputs, handle errors, and evaluate model performance against predefined metrics. PremSQL is extendible for customization and private data usage.
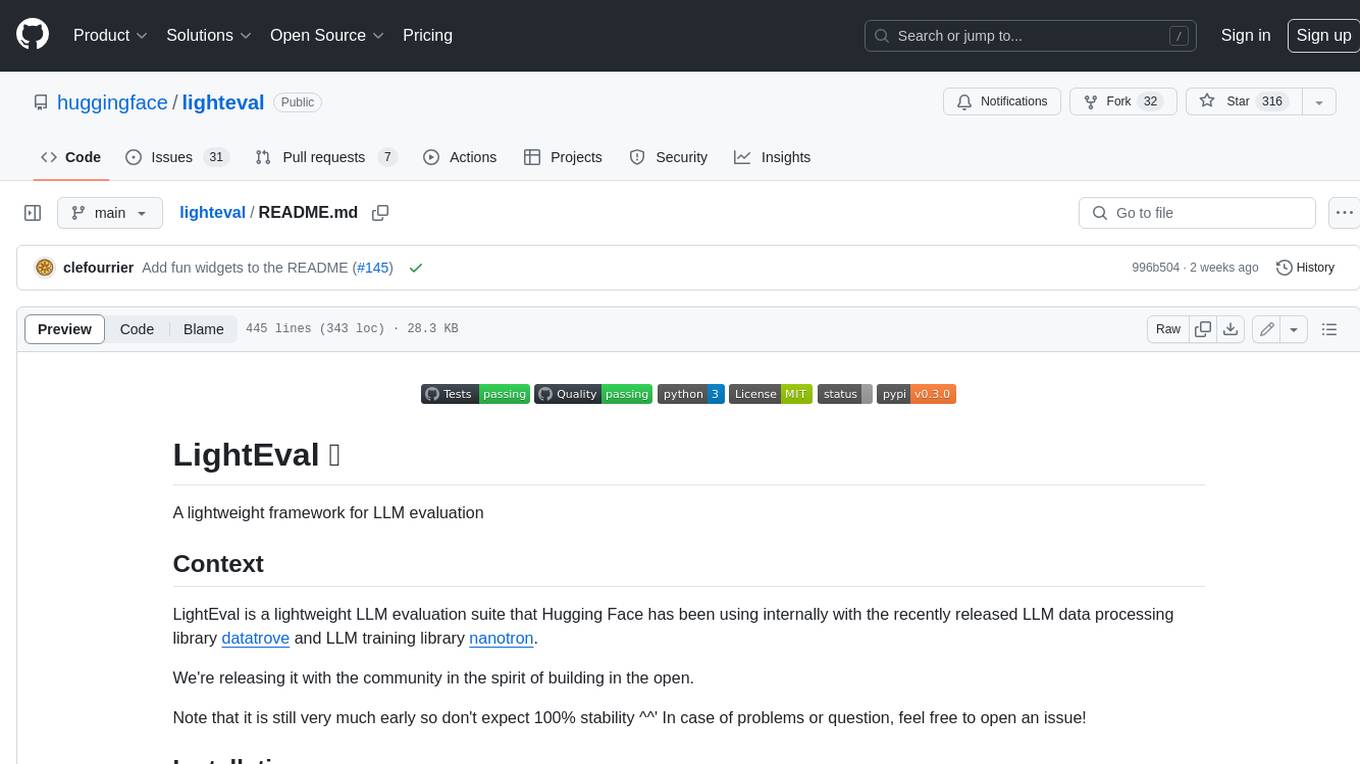
lighteval
LightEval is a lightweight LLM evaluation suite that Hugging Face has been using internally with the recently released LLM data processing library datatrove and LLM training library nanotron. We're releasing it with the community in the spirit of building in the open. Note that it is still very much early so don't expect 100% stability ^^' In case of problems or question, feel free to open an issue!
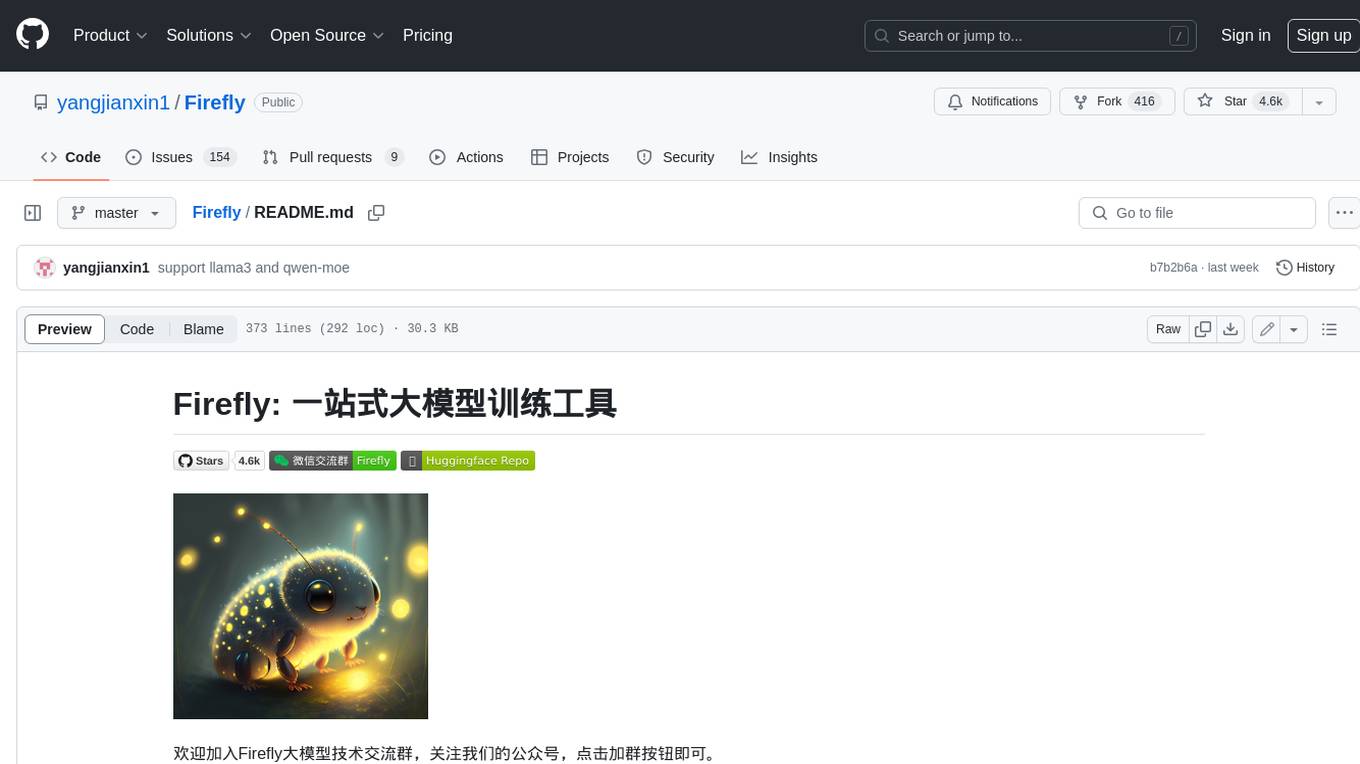
Firefly
Firefly is an open-source large model training project that supports pre-training, fine-tuning, and DPO of mainstream large models. It includes models like Llama3, Gemma, Qwen1.5, MiniCPM, Llama, InternLM, Baichuan, ChatGLM, Yi, Deepseek, Qwen, Orion, Ziya, Xverse, Mistral, Mixtral-8x7B, Zephyr, Vicuna, Bloom, etc. The project supports full-parameter training, LoRA, QLoRA efficient training, and various tasks such as pre-training, SFT, and DPO. Suitable for users with limited training resources, QLoRA is recommended for fine-tuning instructions. The project has achieved good results on the Open LLM Leaderboard with QLoRA training process validation. The latest version has significant updates and adaptations for different chat model templates.
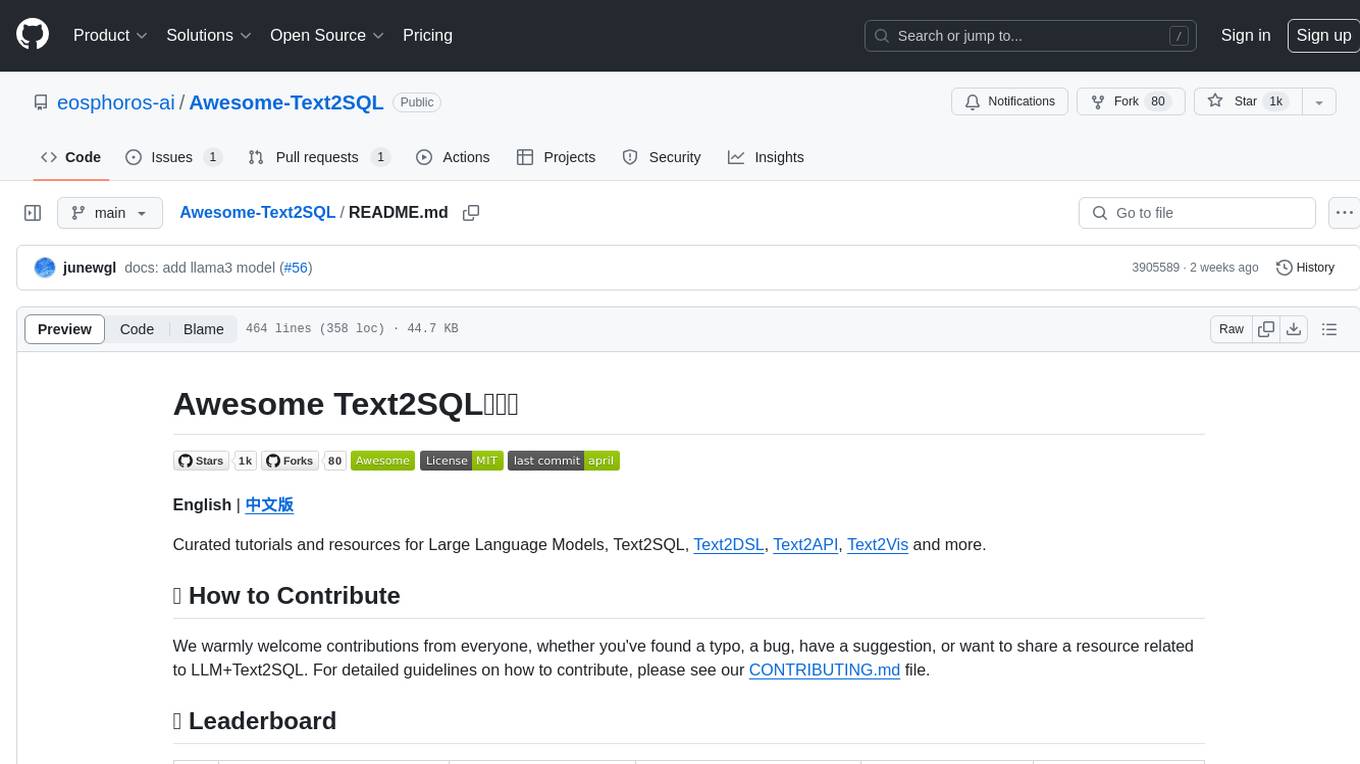
Awesome-Text2SQL
Awesome Text2SQL is a curated repository containing tutorials and resources for Large Language Models, Text2SQL, Text2DSL, Text2API, Text2Vis, and more. It provides guidelines on converting natural language questions into structured SQL queries, with a focus on NL2SQL. The repository includes information on various models, datasets, evaluation metrics, fine-tuning methods, libraries, and practice projects related to Text2SQL. It serves as a comprehensive resource for individuals interested in working with Text2SQL and related technologies.
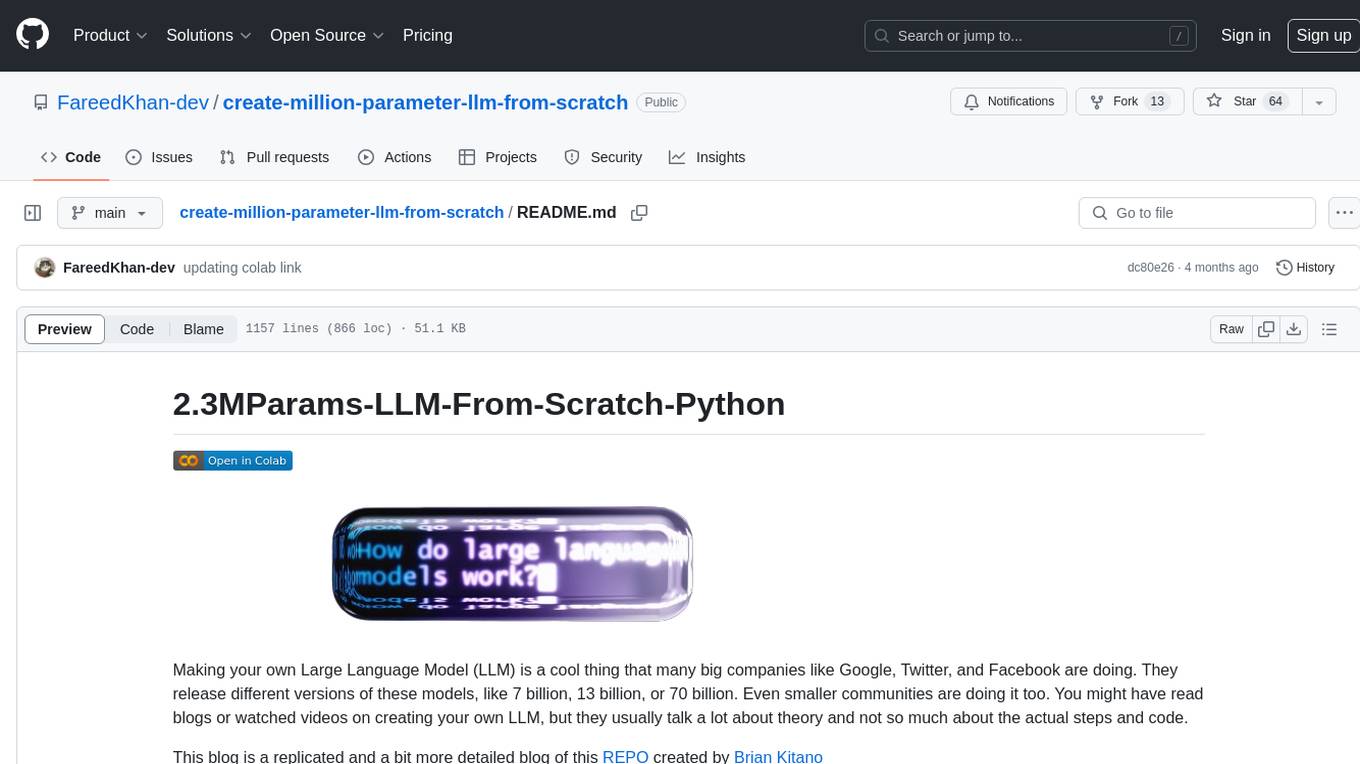
create-million-parameter-llm-from-scratch
The 'create-million-parameter-llm-from-scratch' repository provides a detailed guide on creating a Large Language Model (LLM) with 2.3 million parameters from scratch. The blog replicates the LLaMA approach, incorporating concepts like RMSNorm for pre-normalization, SwiGLU activation function, and Rotary Embeddings. The model is trained on a basic dataset to demonstrate the ease of creating a million-parameter LLM without the need for a high-end GPU.
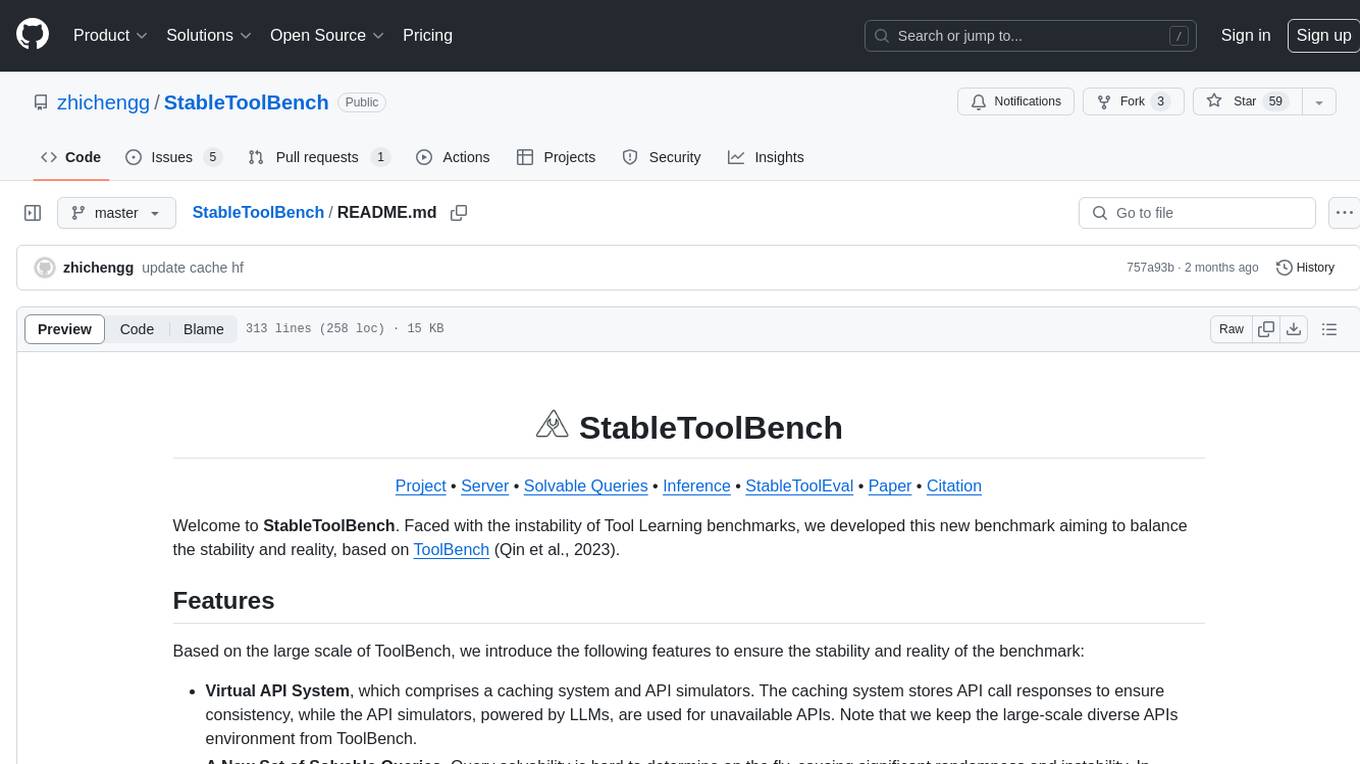
StableToolBench
StableToolBench is a new benchmark developed to address the instability of Tool Learning benchmarks. It aims to balance stability and reality by introducing features such as a Virtual API System with caching and API simulators, a new set of solvable queries determined by LLMs, and a Stable Evaluation System using GPT-4. The Virtual API Server can be set up either by building from source or using a prebuilt Docker image. Users can test the server using provided scripts and evaluate models with Solvable Pass Rate and Solvable Win Rate metrics. The tool also includes model experiments results comparing different models' performance.
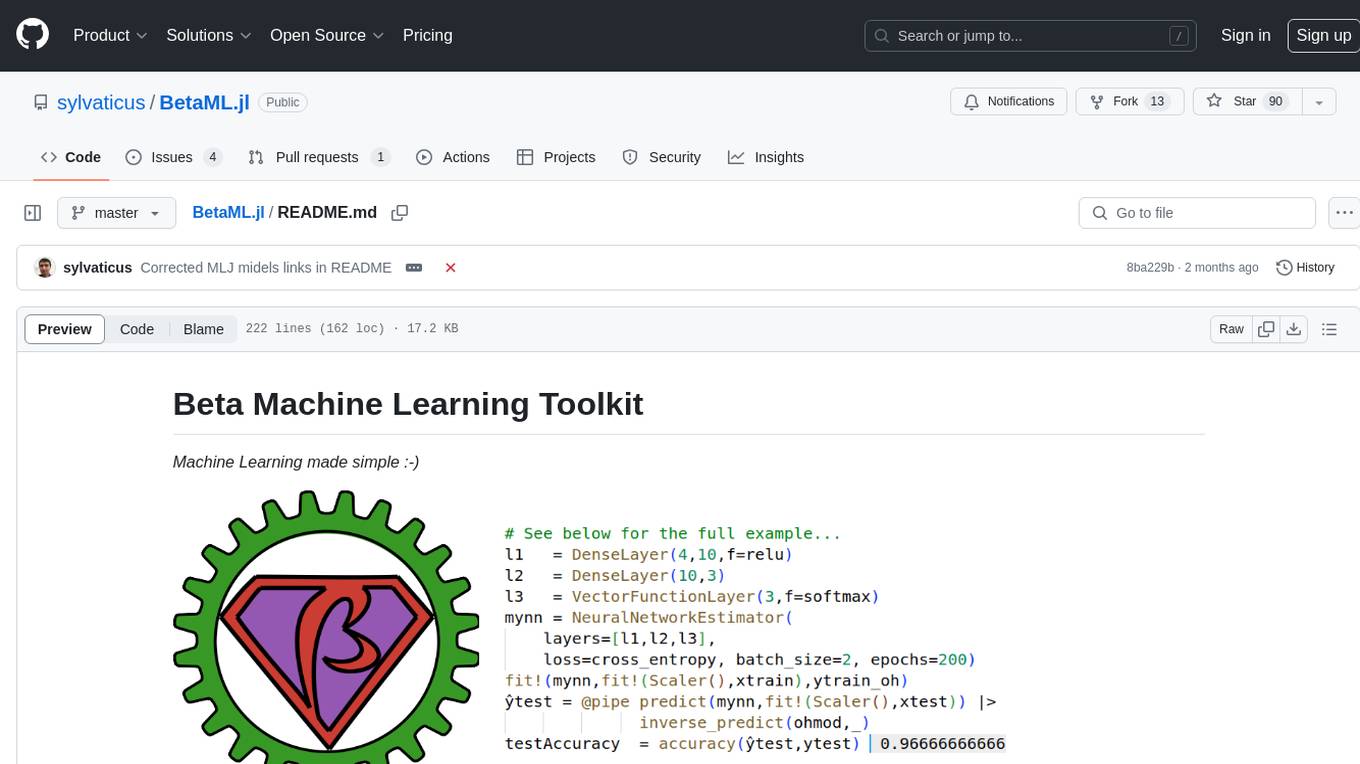
BetaML.jl
The Beta Machine Learning Toolkit is a package containing various algorithms and utilities for implementing machine learning workflows in multiple languages, including Julia, Python, and R. It offers a range of supervised and unsupervised models, data transformers, and assessment tools. The models are implemented entirely in Julia and are not wrappers for third-party models. Users can easily contribute new models or request implementations. The focus is on user-friendliness rather than computational efficiency, making it suitable for educational and research purposes.
For similar jobs
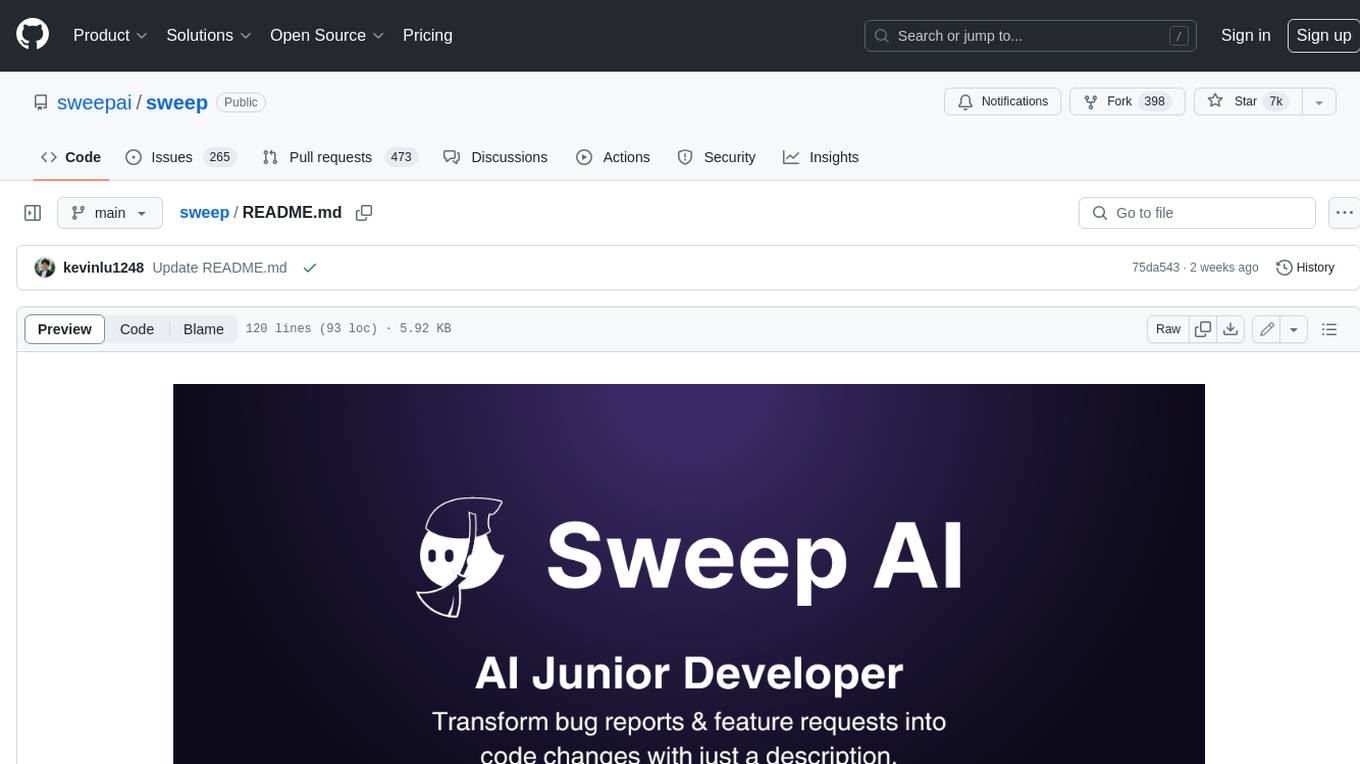
sweep
Sweep is an AI junior developer that turns bugs and feature requests into code changes. It automatically handles developer experience improvements like adding type hints and improving test coverage.
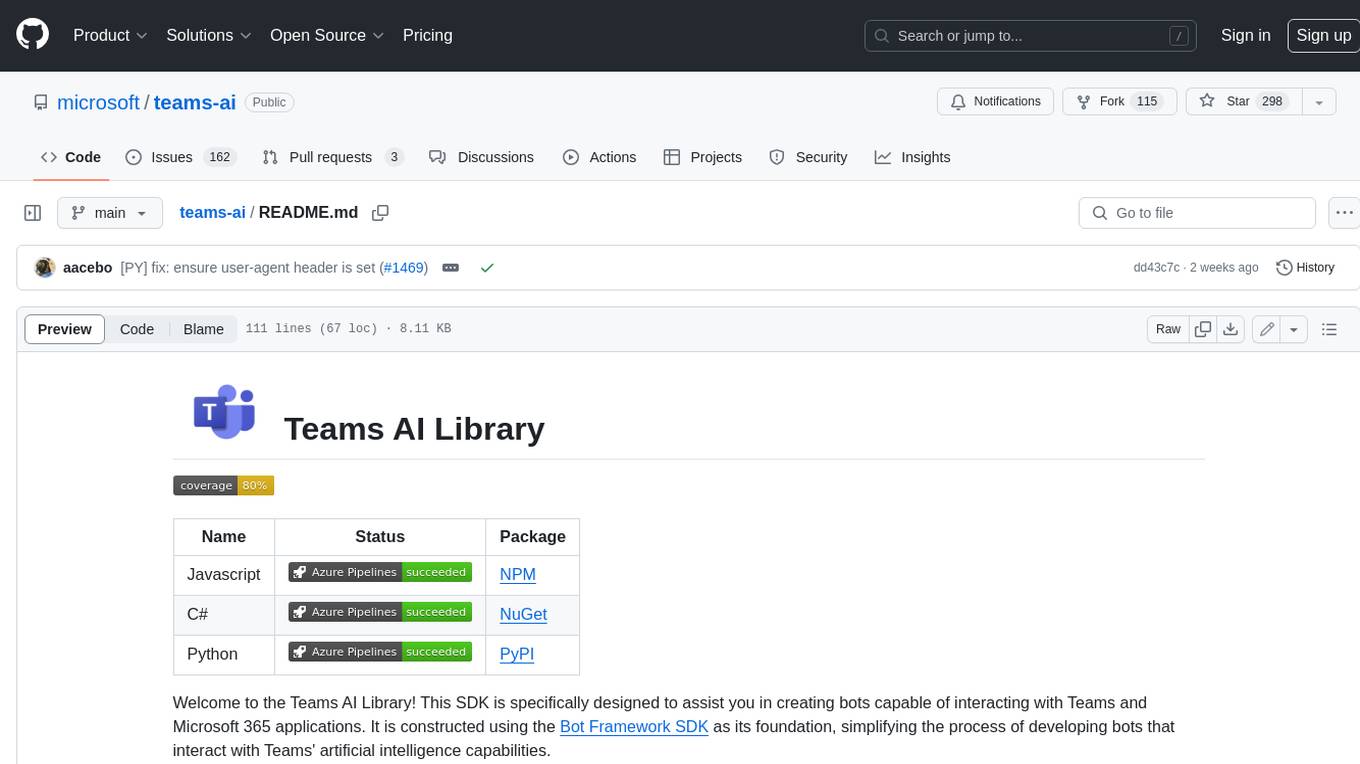
teams-ai
The Teams AI Library is a software development kit (SDK) that helps developers create bots that can interact with Teams and Microsoft 365 applications. It is built on top of the Bot Framework SDK and simplifies the process of developing bots that interact with Teams' artificial intelligence capabilities. The SDK is available for JavaScript/TypeScript, .NET, and Python.
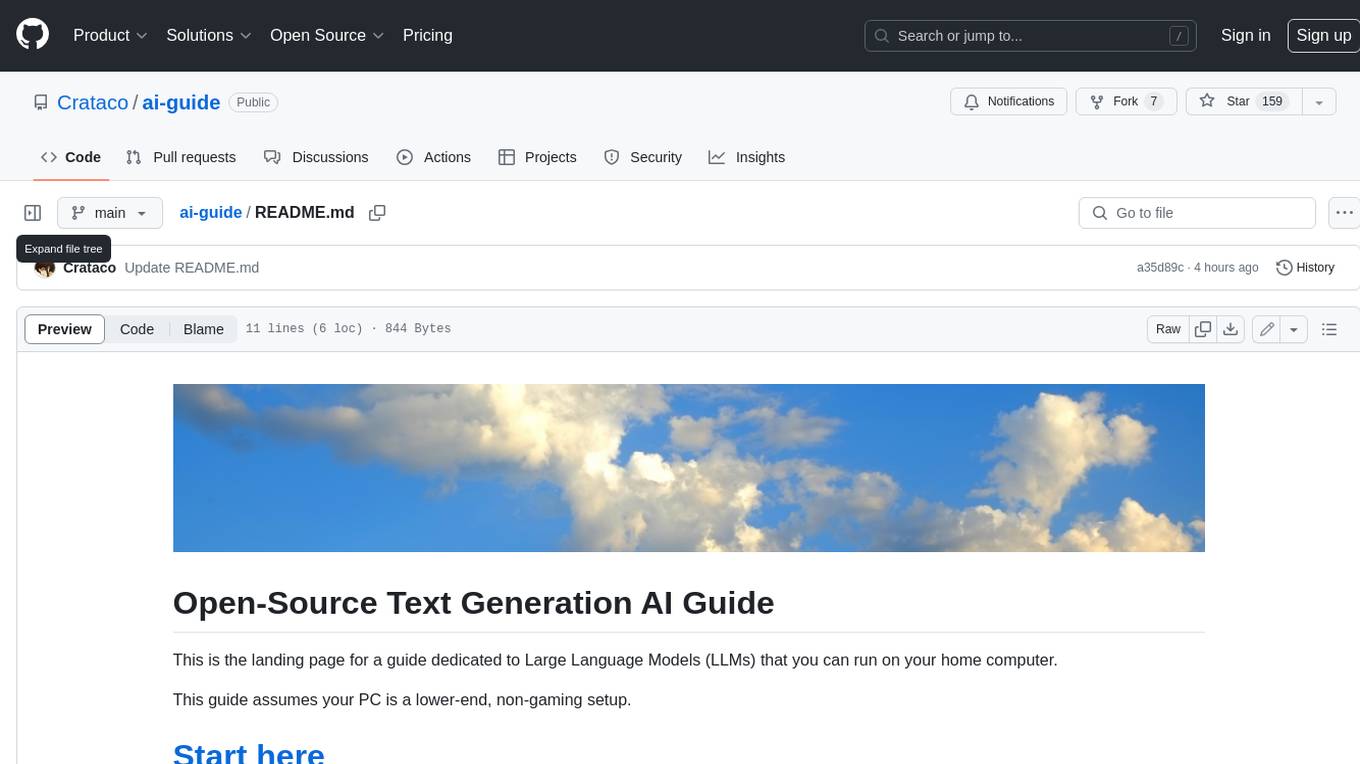
ai-guide
This guide is dedicated to Large Language Models (LLMs) that you can run on your home computer. It assumes your PC is a lower-end, non-gaming setup.
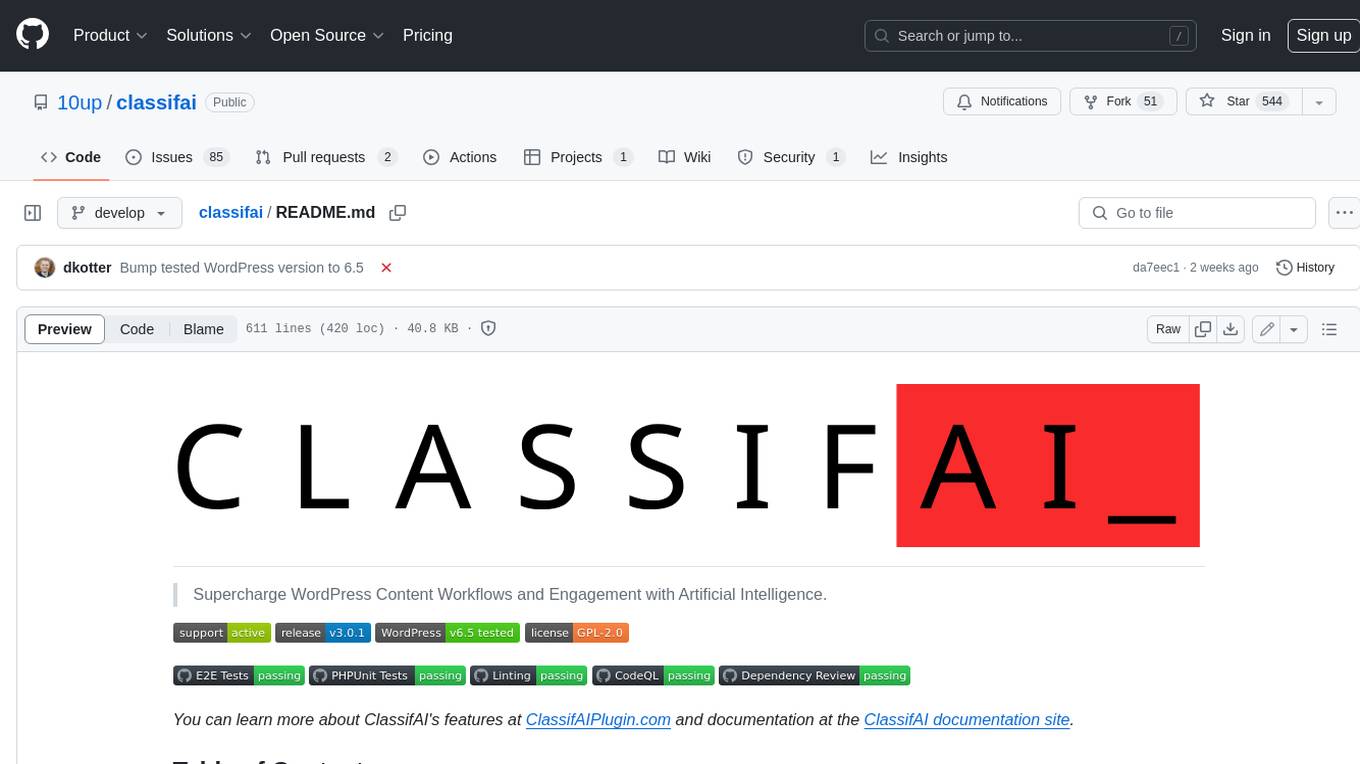
classifai
Supercharge WordPress Content Workflows and Engagement with Artificial Intelligence. Tap into leading cloud-based services like OpenAI, Microsoft Azure AI, Google Gemini and IBM Watson to augment your WordPress-powered websites. Publish content faster while improving SEO performance and increasing audience engagement. ClassifAI integrates Artificial Intelligence and Machine Learning technologies to lighten your workload and eliminate tedious tasks, giving you more time to create original content that matters.
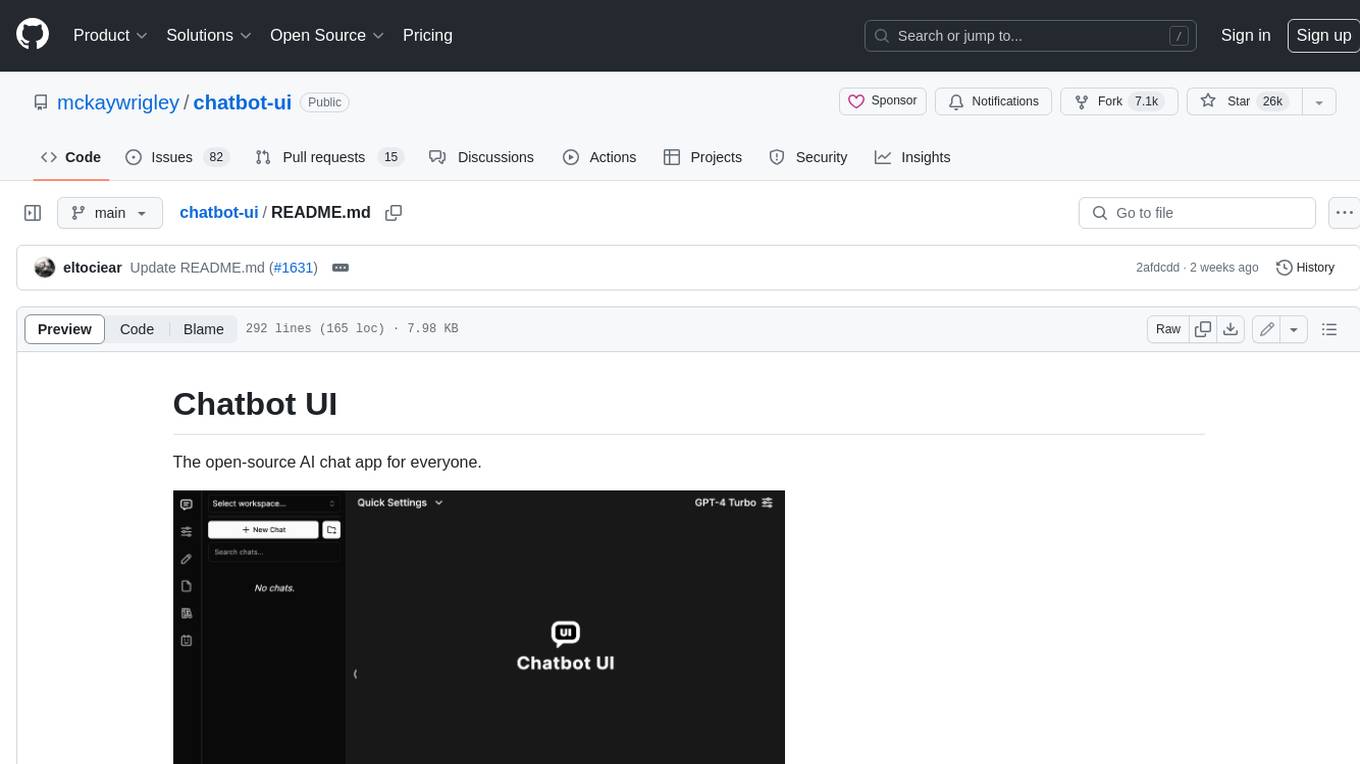
chatbot-ui
Chatbot UI is an open-source AI chat app that allows users to create and deploy their own AI chatbots. It is easy to use and can be customized to fit any need. Chatbot UI is perfect for businesses, developers, and anyone who wants to create a chatbot.
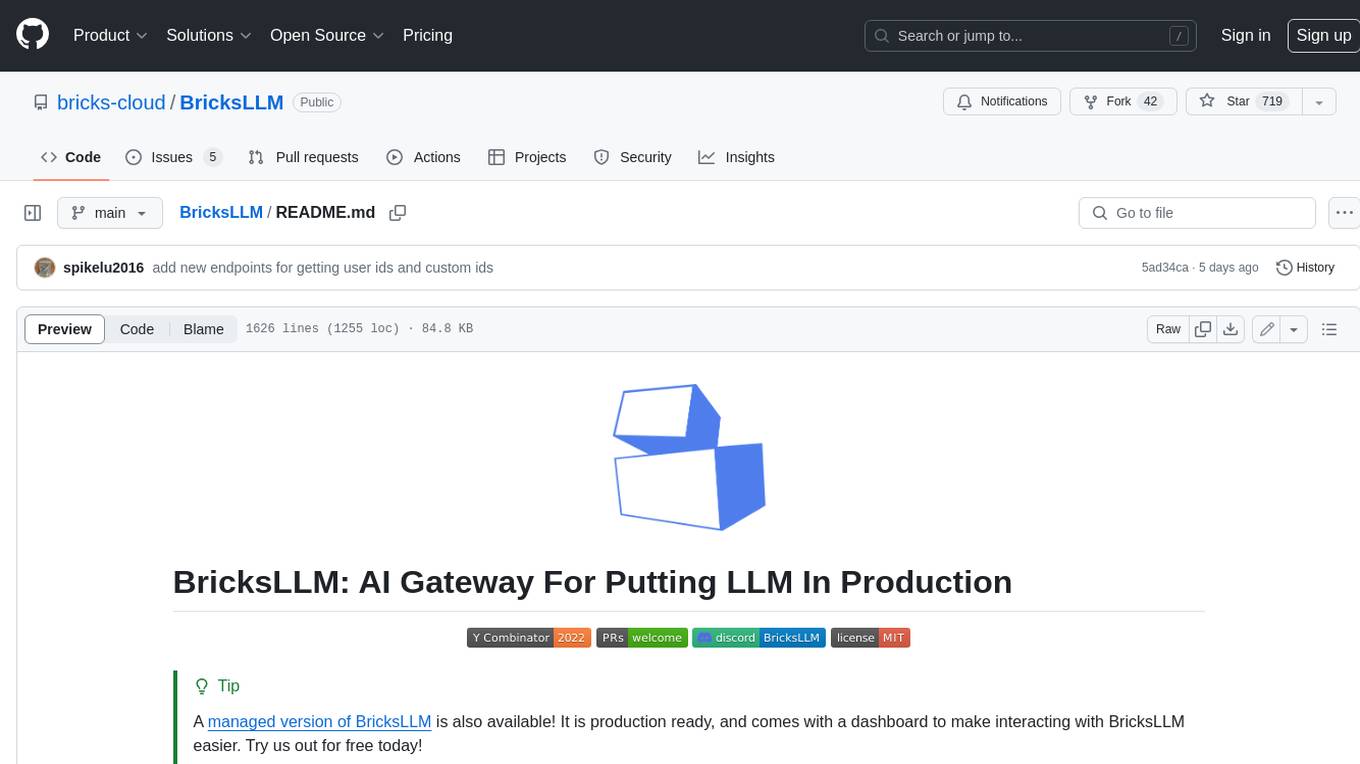
BricksLLM
BricksLLM is a cloud native AI gateway written in Go. Currently, it provides native support for OpenAI, Anthropic, Azure OpenAI and vLLM. BricksLLM aims to provide enterprise level infrastructure that can power any LLM production use cases. Here are some use cases for BricksLLM: * Set LLM usage limits for users on different pricing tiers * Track LLM usage on a per user and per organization basis * Block or redact requests containing PIIs * Improve LLM reliability with failovers, retries and caching * Distribute API keys with rate limits and cost limits for internal development/production use cases * Distribute API keys with rate limits and cost limits for students
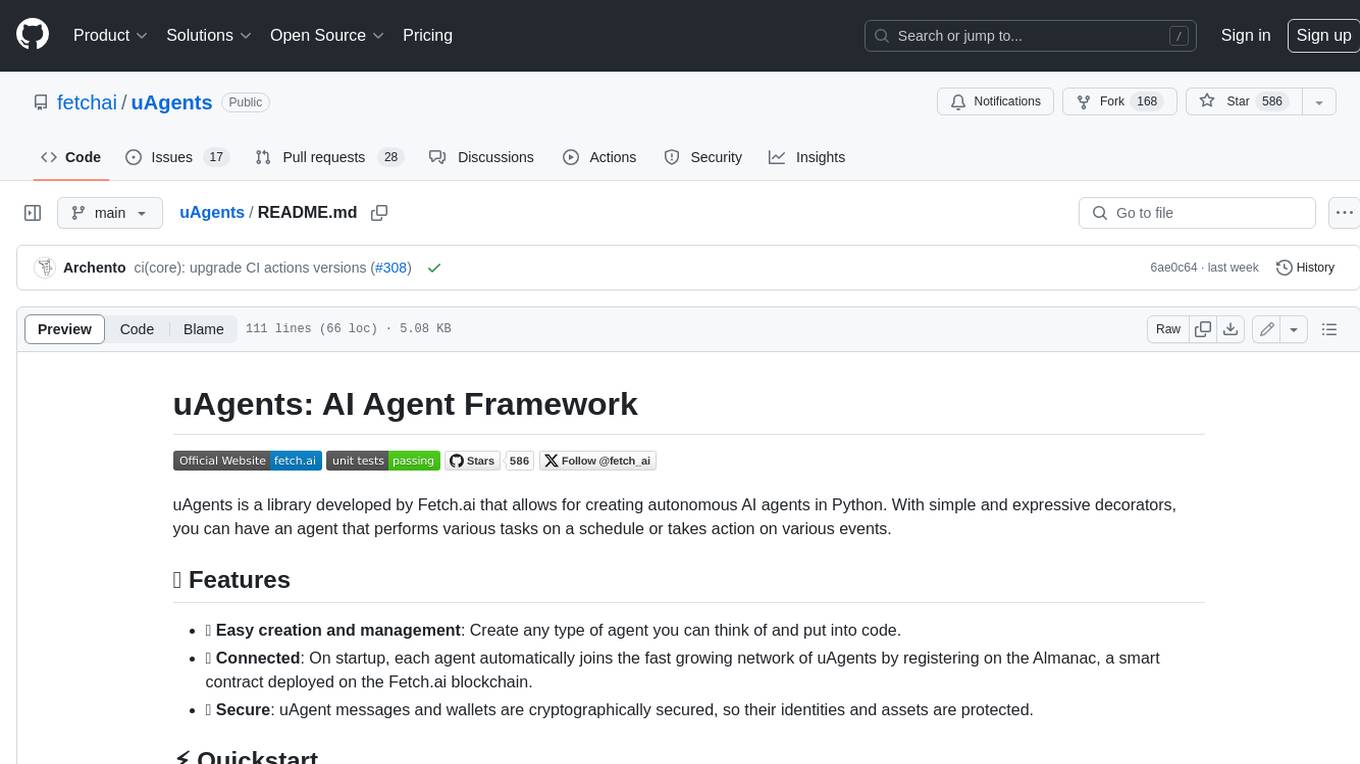
uAgents
uAgents is a Python library developed by Fetch.ai that allows for the creation of autonomous AI agents. These agents can perform various tasks on a schedule or take action on various events. uAgents are easy to create and manage, and they are connected to a fast-growing network of other uAgents. They are also secure, with cryptographically secured messages and wallets.
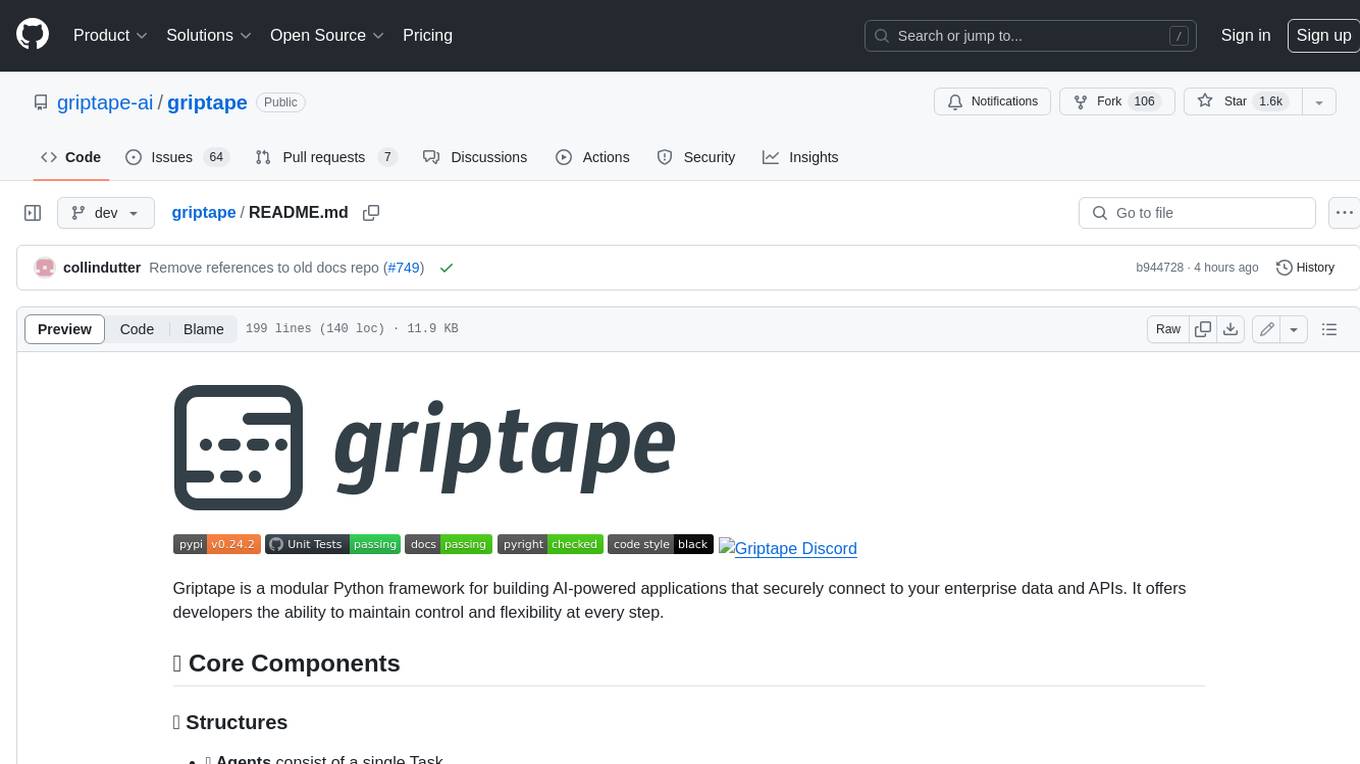
griptape
Griptape is a modular Python framework for building AI-powered applications that securely connect to your enterprise data and APIs. It offers developers the ability to maintain control and flexibility at every step. Griptape's core components include Structures (Agents, Pipelines, and Workflows), Tasks, Tools, Memory (Conversation Memory, Task Memory, and Meta Memory), Drivers (Prompt and Embedding Drivers, Vector Store Drivers, Image Generation Drivers, Image Query Drivers, SQL Drivers, Web Scraper Drivers, and Conversation Memory Drivers), Engines (Query Engines, Extraction Engines, Summary Engines, Image Generation Engines, and Image Query Engines), and additional components (Rulesets, Loaders, Artifacts, Chunkers, and Tokenizers). Griptape enables developers to create AI-powered applications with ease and efficiency.