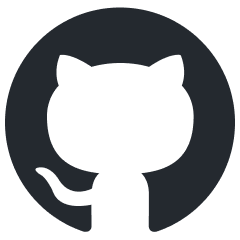
ai_all_resources
A curated list of Best Artificial Intelligence Resources
Stars: 791
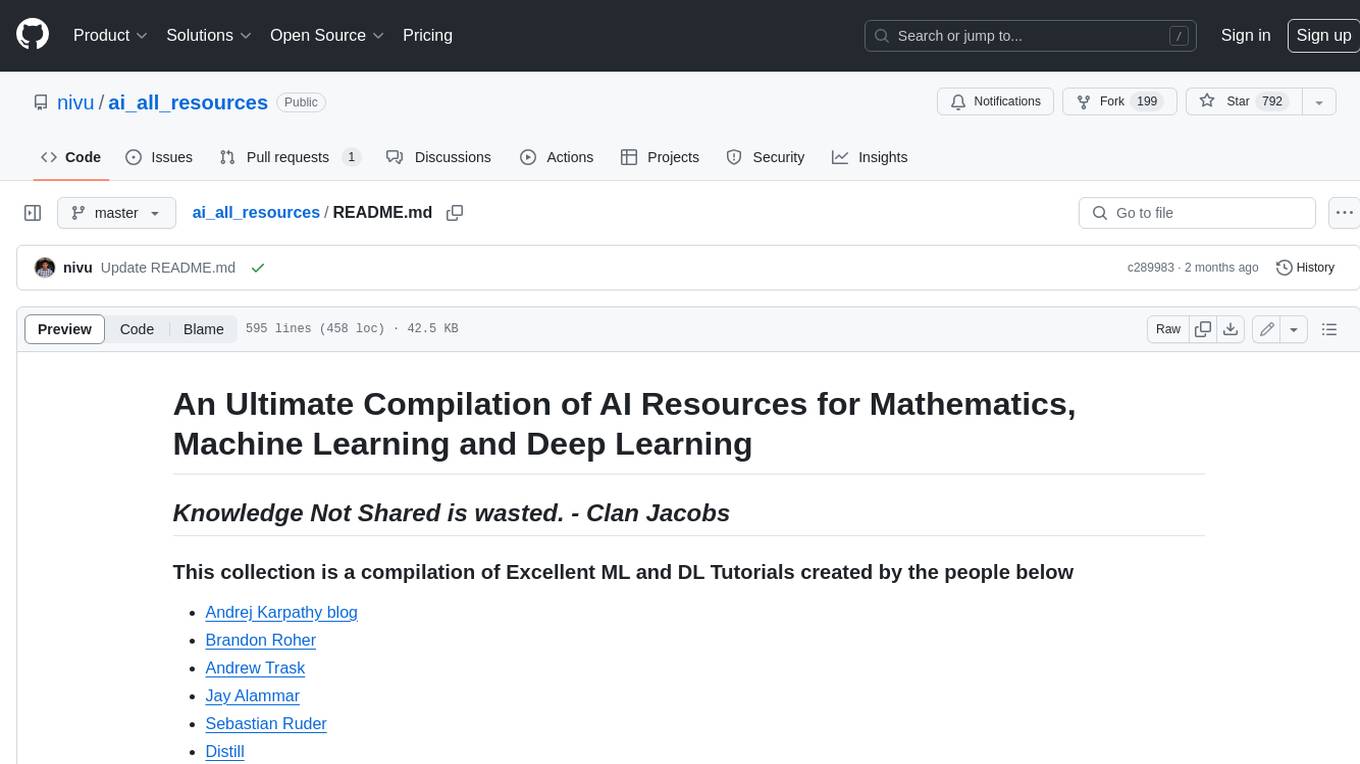
This repository is a compilation of excellent ML and DL tutorials created by various individuals and organizations. It covers a wide range of topics, including machine learning fundamentals, deep learning, computer vision, natural language processing, reinforcement learning, and more. The resources are organized into categories, making it easy to find the information you need. Whether you're a beginner or an experienced practitioner, you're sure to find something valuable in this repository.
README:
- Andrej Karpathy blog
- Brandon Roher
- Andrew Trask
- Jay Alammar
- Sebastian Ruder
- Distill
- StatQuest with Josh Starmer
- sentdex
- Lex Fridman
- 3Blue1Brown
- Alexander Amini
- The Coding Train
- Christopher Olah
- AI Coimbatore Join here🔗⬇️
- TensorFlow User Group Coimbatore
Navaneeth Malingan
- 🖥️ HOW TO GET STARTED WITH MACHINE LEARNING!
- How to Build a Meaningful Career in Data Science
- My Self-Created Artificial Intelligence Masters Degree
- PyImageSearch
- 5 Beginner Friendly Steps to Learn Machine Learning and Data Science with Python
- Luis Serrano: A Friendly Introduction to Machine Learning
- StatQuest: A Gentle Introduction to Machine Learning
- Machine Learning For Everyone Summarize's Machine Learning algorithms and their applications in simple words with real-world examples.
- Teachable Machine Train a computer to recognize your own images, sounds, & poses. A fast, easy way to create machine learning models for your sites, apps, and more – no expertise or coding required.
- Machine Learning by Andrew Ng, Stanford IMDB 10/10 LOL :P
- Datacamp : Data Engineer with Python
- Intro to Machine Learning Topics Covered Naive Bayes, SVM, Decision Trees, Regressions, Outliers, Clustering, Feature Scaling, Text Learning, Feature Selection, PCA, Validation, Evaluation Metrics
- Intro to TensorFlow for Deep Learning The Best Course for Learning TensorFlow
- End-to-End Machine Learning
- NVIDIA DEEP LEARNING INSTITUTE
- Introduction to Machine Learning for Coders!
- Practical Deep Learning for Coders, v3
- FastAI
- CS221 - Artificial Intelligence: Principles and Techniques by Percy Liang and Dorsa Sadigh
- CS229 - Machine Learning by Andrew Ng
- CS230 - Deep Learning by Andrew Ng
- CS231n - Convolutional Neural Networks for Visual Recognition by Fei-Fei Li and Andrej Karpathy
- CS224n - Natural Language Processing with Deep Learning by Christopher Manning
- CS234 - Reinforcement Learning by Emma Brunskill
- CS330 - Deep Multi-task and Meta Learning by Chelsea Finn
- CS25 - Transformers United
- CS/LTI 11-711: Advanced NLP by Graham Neubig
- CS/LTI 11-747: Neural Networks for NLP by Graham Neubig
- CS/LTI 11-737: Multilingual NLP by Graham Neubig
- CS/LTI 11-777: Multimodal Machine Learning by Louis-Philippe Morency
- CS/LTI 11-785: Introduction to Deep Learning by Bhiksha Raj and Rita Singh
- CS/LTI Low Resource NLP Bootcamp 2020 by Graham Neubig
- 6.S191 - Introduction to Deep Learning by Alexander Amini and Ava Amini
- 6.S094 - Deep Learning by Lex Fridman
- 6.S192 - Deep Learning for Art, Aesthetics, and Creativity by Ali Jahanian
- Bias and Variance
- Cross Validation
- Machine Learning Fundamentals: The Confusion Matrix
- Sensitivity and Specivicity
- ROC and AUC, Clearly Explained!
- StatQuest: R-squared explained
- Regularization Part 1: Ridge Regression
- Regularization Part 2: Lasso Regression
- Maximum Likelihood
- Covariance and Correlation Part 1: Covariance
- Statistics Fundamentals: The Mean, Variance and Standard Deviation
- Statistics Fundamentals: Population Parameters
- Glossary: Statistics
- Glossary: Machine Learning
- Looking at R-Squared
- Mathematics for Machine Learning In this post I have compiled great e-resources (MOOC, YouTube Lectures, Books) for learning Mathematics for Machine Learning.
- Mathematics for Machine Learning - Book One great book for all things math for machine learning. (free eBook)
- I highly Recommend you to go through the following resources by 3Blue1Brown
-
Gilbert Strang: Linear Algebra vs Calculus
▶️ -
Basics of Integral Calculus in Tamil
▶️ - New fast.ai course: Computational Linear Algebra
- Linear Algebra Book
-
Python Programming Tutorials by Socratica
▶️ - Python Tutorial by w3schools📙
- Learning Python Programming📙
- A Visual Intro to NumPy and Data Representation
- CS231n : Python Numpy Tutorial
- NumPy resources : part of the End-to-End Machine Learning library
- 100 numpy exercises (with solutions)
- 101 NumPy Exercises for Data Analysis (Python)
- Numpy Tutorial – Introduction to ndarray
- Sci-Py Lectures : NumPy: creating and manipulating numerical data
-
Python NumPy Tutorial for Beginners
▶️ Learn the basics of the NumPy library in this tutorial for beginners. It provides background information on how NumPy works and how it compares to Python's Built-in lists. This video goes through how to write code with NumPy. It starts with the basics of creating arrays and then gets into more advanced stuff. The video covers creating arrays, indexing, math, statistics, reshaping, and more. - Python NumPy Tutorial – Learn NumPy Arrays With Examples
- Python Numpy Array Tutorial
- NumPy Tutorial: Data analysis with Python
-
Deep Learning Prerequisites: The Numpy Stack in Python
▶️
- A Gentle Visual Intro to Data Analysis in Python Using Pandas
-
Data analysis in Python with pandas by Data School
▶️ -
Best practices with pandas by Data School
▶️ - Python Pandas Tutorial: A Complete Introduction for Beginners
-
CodeBasics: Machine Learning Tutorial Python
▶️ -
StatQuest: Machine Learning
▶️ -
sentdex: Machine Learning with Python
▶️ -
Simplilearn: Machine Learning Tutorial Videos
▶️ -
Machine Learning Tutorial in Python
▶️ -
deeplizard: Machine Learning & Deep Learning Fundamentals
▶️
Note: Below you can find the best lectures for popular Machine Learning Algorithms
-
Linear Regression: A friendly introduction by Luis Serrano
▶️ -
Statistics 101: Linear Regression, The Very Basics
▶️ - Regression Line Fitting Playground
- Regression Curve Fitting Playground
-
Linear Regression vs Logistic Regression | Data Science Training | Edureka
▶️ -
Logistic Regression and the Perceptron Algorithm: A friendly introduction by Luis Serrano
▶️
-
StatQuest: Decision Trees
▶️ -
StatQuest: Decision Trees, Part 2 - Feature Selection and Missing Data
▶️ - Decision Tree Introduction with example📙
- Decision Tree📙
- Python | Decision Tree Regression using sklearn📙
- ML | Logistic Regression v/s Decision Tree Classification📙
-
StatQuest: Random Forests Part 1 - Building, Using and Evaluating
▶️ -
StatQuest: Random Forests Part 2: Missing data and clustering
▶️ - Random Forests for Complete Beginners📙
-
Boosting Machine Learning Tutorial | Adaptive Boosting, Gradient Boosting, XGBoost | Edureka
▶️ -
AdaBoost, Clearly Explained
▶️ -
Gradient Boost Part 1: Regression Main Ideas
▶️ -
Gradient Boost Part 2: Regression Details
▶️ -
Gradient Boost Part 3: Classification
▶️ -
Gradient Boost Part 4: Classification Details
▶️ -
XGBoost Part1: XGBoost Trees for Regression
▶️ -
XGBoost Part 2: XGBoost Trees For Classification
▶️ - Ensemble methods Sci-kit learn
-
Support Vector Machines (SVMs): A friendly introduction by Luis Serrano
▶️ -
Support Vector Machines, Clearly Explained!!! by StatQuest
▶️ -
Support Vector Machines Part 2: The Polynomial Kernel by StatQuest
▶️ -
Support Vector Machines Part 3: The Radial (RBF) Kernel by StatQuest
▶️ -
How Support Vector Machines work / How to open a black box
▶️ -
Support Vector Machines - The Math of Intelligence (Week 1)
▶️ - Demystifying Support Vector Machines📙
-
Support Vector Machine (SVM) - Fun and Easy Machine Learning
▶️
-
Bayes theorem, and making probability intuitive
▶️ -
A friendly introduction to Bayes Theorem and Hidden Markov Models
▶️ -
The Bayesian Trap
▶️ -
Naive Bayes classifier: A friendly approach
▶️
-
StatQuest: K-means clustering
▶️ -
Machine Learning Tutorial Python - 13: K Means Clustering
▶️ -
K Means Clustering Algorithm - K Means Example in Python - Machine Learning Algorithms - Edureka
▶️
-
StatQuest: PCA main ideas in only 5 minutes!!!
▶️ -
StatQuest: Principal Component Analysis (PCA), Step-by-Step
▶️ -
Principal Component Analysis (PCA) by Luis Serrano
▶️
- Linear Regression using Gradient Descent📙
-
Gradient Descent, Step-by-Step
▶️ -
Stochastic Gradient Descent, Clearly Explained!!!
▶️ - How Optimization Works A short series on the fundamentals of optimization for machine learning
- Linear Regression using Gradient Descent
- Polynomial Regression
- Gradient Descent in Linear Regression - Math📙
-
Neural Network Backpropagation Basics For Dummies
▶️
-
3.4: Linear Regression with Gradient Descent - Intelligence and Learning
▶️ -
3.5: Mathematics of Gradient Descent - Intelligence and Learning
▶️ -
3.5a: Calculus: Power Rule - Intelligence and Learning
▶️ -
3.5b: Calculus: Chain Rule - Intelligence and Learning
▶️ -
3.5c: Calculus: Partial Derivative - Intelligence and Learning
▶️
- https://datascience.stackexchange.com/questions/24534/does-gradient-descent-always-converge-to-an-optimum
- https://datascience.stackexchange.com/questions/18802/does-mlp-always-find-local-minimum
- https://www.coursera.org/learn/deep-neural-network/lecture/RFANA/the-problem-of-local-optima
- An introduction to machine learning with scikit-learn📙
- Python Machine Learning: Scikit-Learn Tutorial
- DEEP BLUEBERRY BOOK This is a tiny and very focused collection of links about deep learning. If you've always wanted to learn deep learning stuff but don't know where to start, you might have stumbled upon the right place!
- 6.S191: Introduction to Deep Learning (2019)
- MIT 6.S191 Introduction to Deep Learning (2020)
- MIT 6.S191 Introduction to Deep Learning (2023) (YouTube)
- MIT Deep Learning Basics: Introduction and Overview
- MIT Deep Learning by Lex Fridman
- Deep Learning in Tamil
- The Deep Learning Textbook from Ian Goodfellow, Yoshua Bengio, and Aaron Courville
- Neural Networks And Deep Learning by Michael Nielsen
- Grokking Deep Learning by Andrew Trask
-
A friendly introduction to Deep Learning and Neural Networks
▶️ - Machine Learning for Beginners: An Introduction to Neural Networks📙 A simple explanation of how they work and how to implement one from scratch in Python.
- A Visual and Interactive Guide to the Basics of Neural Networks📙
- A Visual And Interactive Look at Basic Neural Network Math📙
-
Neural Network Architectures
▶️ -
Neural Networks Demystified by Welch Labs
▶️ -
Neural networks Series by 3Blue1Brows
▶️
- CS131 Computer Vision: Foundations and Applications Fall 2019
- CS231A: Computer Vision, From 3D Reconstruction to Recognition Winter 2018
- CS231n Convolutional Neural Networks for Visual Recognition
- Computer Vision Notebooks
- CS231n: Convolutional Neural Networks for Visual Recognition Spring 2019
- CS231n: Convolutional Neural Networks for Visual Recognition
- A friendly introduction to Convolutional Neural Networks and Image Recognition
- A Comprehensive Guide to Convolutional Neural Networks — the ELI5 way
- Tensorflow Convolutional Neural Network (CNN)
- Convolutional Networks Book
- CNNs, Part 1: An Introduction to Convolutional Neural Networks
- CS231n Winter 2016 BY Andrej Karpathy 15 Videos
- Intuitive understanding of 1D, 2D, and 3D Convolutions in Convolutional Neural Networks
- CNN ExplainerAn interactive visualization system designed to help non-experts learn about Convolutional Neural Networks (CNNs)
Deep-dive tutorial on Object Detection. Intuition lectures on topics ranging from Classical CV techniques like HOG, SIFT to Convolutional Neural Network based techniques like Overfeat, Faster RCNN etc. You will learn how the ideas have evolved from some of the earliest papers to current ones. And hence the name Evolution of Object Detection Networks.
- SIFT | Scale Invariant Feature Transform
- Hog Intuition | Histogram of Oriented Gradients
- NMS | Non Max Suppression
- Object Localization | Bounding Box Regression
- Object Detection
- RCNN
- Spatial Pyramid Matching | SPM
- SPPNet Object Detection
- Fast RCNN Network
- Faster RCNN
- Yolo v4 Object Detection - How it Works & Why it's So Amazing!
- Frameworks and Libraries
- Labeling Tools
- Code samples
- Announcing the Objectron Dataset | Google AI Blog
- MediaPipe Objectron | Objectron (3D Object Detection)
- A Friendly Introduction to Generative Adversarial Networks (GANs) by Luis Serrano
- Generative Adversarial Networks (GANs) by Ahlad Kumar
- Building our first simple GAN
- Face editing with Generative Adversarial Networks
- Variational Autoencoders
- Generative Adversarial Networks (GANs) in 50 lines of code (PyTorch)
- Generative Models
- CS224n: Natural Language Processing with Deep Learning
- NLP and The Reformer
- The Illustrated Word2vec
- Illustrated Guide to Recurrent Neural Networks: Understanding the Intuition
- Anyone Can Learn To Code an LSTM-RNN in Python (Part 1: RNN) Baby steps to your neural network's first memories.
- The Unreasonable Effectiveness of Recurrent Neural Networks
- An Introduction to Recurrent Neural Networks for Beginners A simple walkthrough of what RNNs are, how they work, and how to build one from scratch in Python.
- Attention and Augmented Recurrent Neural Networks by Distill
- Visualizing memorization in RNNs by Distill Inspecting gradient magnitudes in context can be a powerful tool to see when recurrent units use short-term or long-term contextual understanding.
- Deep Learning for NLP: ANNs, RNNs and LSTMs explained!
- Understanding LSTM Networks
- LSTM implementation explained
- A Gentle Introduction to LSTM Autoencoders
- Part 1 - Position Embeddings
- Part 2 - Multi-Head & Self Attention
- Part 3 - Decoder’s Masked Attention
- NLP Transformers Attention Playlist
- The Illustrated Transformer
- The Annotated Transformer
- Transformers Paper and Code
- Transformers from Scratch
- Transformers Notes
- Transformers, Explained: Understand the Model Behind GPT-3, BERT, and T5
- A comprehensive overview of Transformer variants.
- How to become an NLP & Transformer Model Guru
- [MASTERCLASS] Transformers | Attention Models
- Explaining BERT Simply Using Sketches
- A Visual Guide to Using BERT for the First Time
- The Illustrated BERT, ELMo, and co. (How NLP Cracked Transfer Learning)
- BERT Explained: State of the art language model for NLP
- BioBERT, a language representation model for biomedical domain, especially designed for biomedical text mining tasks such as biomedical named entity recognition, relation extraction, question answering, etc
- Deep Reinforcement Learning Course 🕹️ A Free course in Deep Reinforcement Learning from beginner to expert.
- Implementation of Reinforcement Learning Algorithms. Python, OpenAI Gym, Tensorflow. Exercises and Solutions to accompany Sutton's Book and David Silver's course.
- Unity Machine Learning Agents Toolkit
- 🖥️ WRITING MY FIRST MACHINE LEARNING GAME! (1/4)
- Deep Reinforcement Learning: Pong from Pixels by Andrej Karpathy
- A Beginner's Guide to Deep Reinforcement Learning
- An Introduction to Unity ML-Agents
- Deep Reinforcement Learning Algorithms with PyTorch
- LECTURES: Introduction to Reinforcement Learning - David Silver
- BOOK: Reinforcement Learning - An Introduction by Sutton and Barto
- BOOK: Deep Reinforcement Learning Hands On by Maxim Lapan
- Udacity : Deep Learning with PyTorch
- Deep Learning (PyTorch) : Code
- Udacity : Secure AI
- TORCHSCRIPT
- PyTorchZeroToAll (in English) Sung Kim a Series of 14 Videos
- Introduction to TensorFlow 2.0: Easier for beginners, and more powerful for experts (TF World '19)
- TensorFlow Lite: Solution for running ML on-device (TF World '19)
- Machine Learning in JavaScript (TensorFlow Dev Summit 2018)
- TensorFlow.js Quick Start
- Keras vs. tf.keras: What’s the difference in TensorFlow 2.0?
- How To Run TensorFlow Lite on Raspberry Pi for Object Detection
- How computers learn to recognize objects instantly | Joseph Redmon
- Intro to TensorFlow for Deep Learning
- TensorFlow in Practice Specialization : Coursera
- TensorFlow: Data and Deployment Specialization : Coursera
- Transfer Learning with Keras and Deep Learning
- A Comprehensive Hands-on Guide to Transfer Learning with Real-World Applications in Deep Learning
- TensorFlow Core Tutorials
- Machine Learning in 5 Minutes: How to deploy a ML model (SurveyMonkey Engineer explains)
- Deploy Machine Learning Models with Django
- MlFlow - An open source platform for the machine learning lifecycle
- TensorFlow: Data and Deployment Specialization
- Artificial Intelligence
- Machine Learning
- Deep Learning
- Machine Learning tips and tricks
- Data Science Tools
- Machine Learning with R
- CHRIS ALBON Cheat Sheets and Flash Cards
- MLOps Tooling Landscape v2 (+84 new tools) - Dec '20
- Mathematical tools
- Ordinary Differential Equations for Engineers
- 3Blue1Brown
- StatQuest with Josh Starmer
- Sentdex
- Luis Serrano
- Brandon Rohrer
- deeplizard
- Tech With Tim
- Microsoft Research
- Corey Schafer
- Data School
- Two Minute Papers
- Welch Labs
- Simplilearn
- Great Learning
- DeepLearning.TV
- TensorFlow
- Deeplearning.ai
- Code Bullet
- edureka!
- Lex Fridman
- The Artificial Intelligence Channel
- freeCodeCamp.org
- CloudxLab
- Alexander Amini
- Jeff Heaton
- Abhishek Thakur
- The Coding Train
- 🖥️ HOW TO GET STARTED WITH MACHINE LEARNING!
- My Self-Created Artificial Intelligence Masters Degree
- https://end-to-end-machine-learning.teachable.com/courses/667372/lectures/11900568
- ML Fundamentals by StatQuest
- Machine Learning with Python by sentdex
- 5 Beginner Friendly Steps to Learn Machine Learning and Data Science with Python - Daniel Bourke
- Data School
- Neural Networks and Deep Learning
- https://www.machinelearningisfun.com/
- https://medium.com/@ageitgey/machine-learning-is-fun-80ea3ec3c471
- https://medium.com/greyatom
- https://greyatom.com/glabs
- John Searle: "Consciousness in Artificial Intelligence" | Talks at Google
- https://github.com/nature-of-code/NOC-S17-2-Intelligence-Learning/tree/master/week3-classification-regression
- https://github.com/nature-of-code/NOC-S17-2-Intelligence-Learning
For Tasks:
Click tags to check more tools for each tasksFor Jobs:
Alternative AI tools for ai_all_resources
Similar Open Source Tools
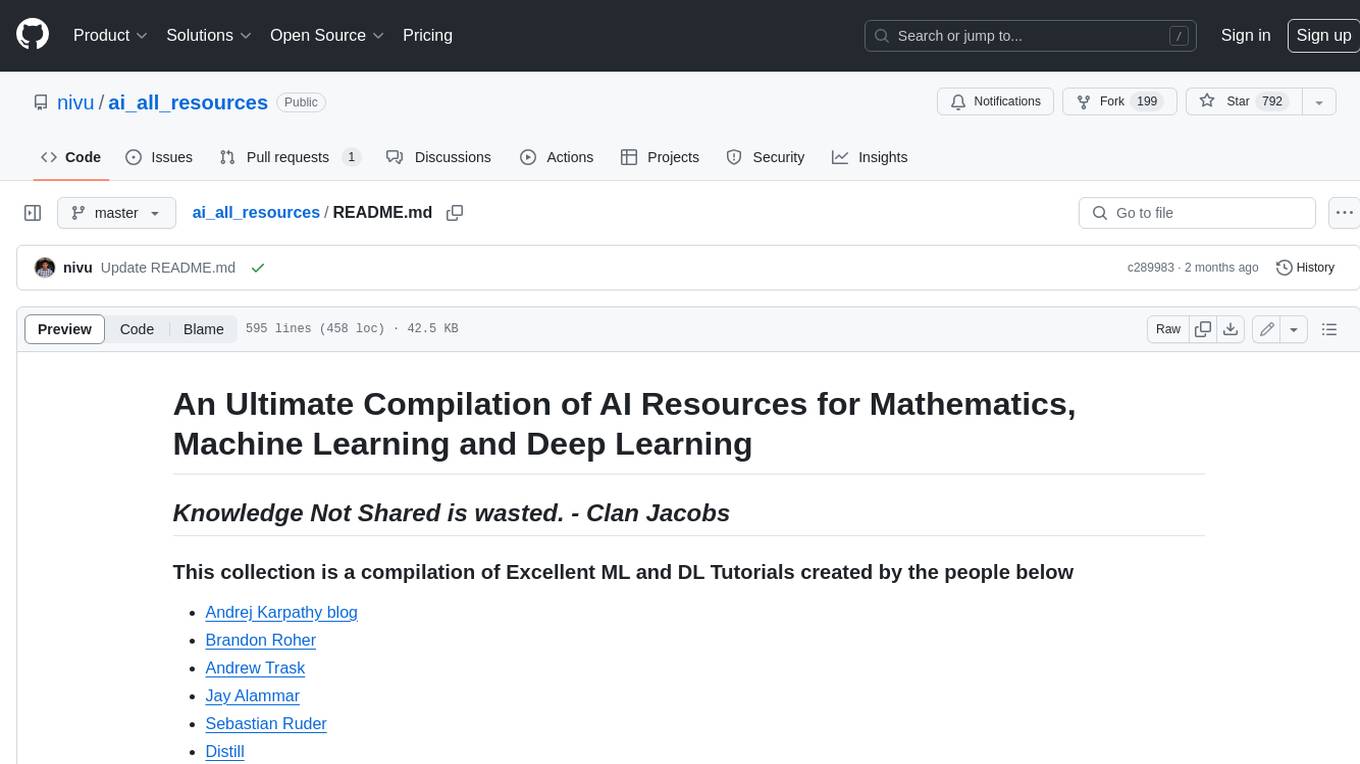
ai_all_resources
This repository is a compilation of excellent ML and DL tutorials created by various individuals and organizations. It covers a wide range of topics, including machine learning fundamentals, deep learning, computer vision, natural language processing, reinforcement learning, and more. The resources are organized into categories, making it easy to find the information you need. Whether you're a beginner or an experienced practitioner, you're sure to find something valuable in this repository.
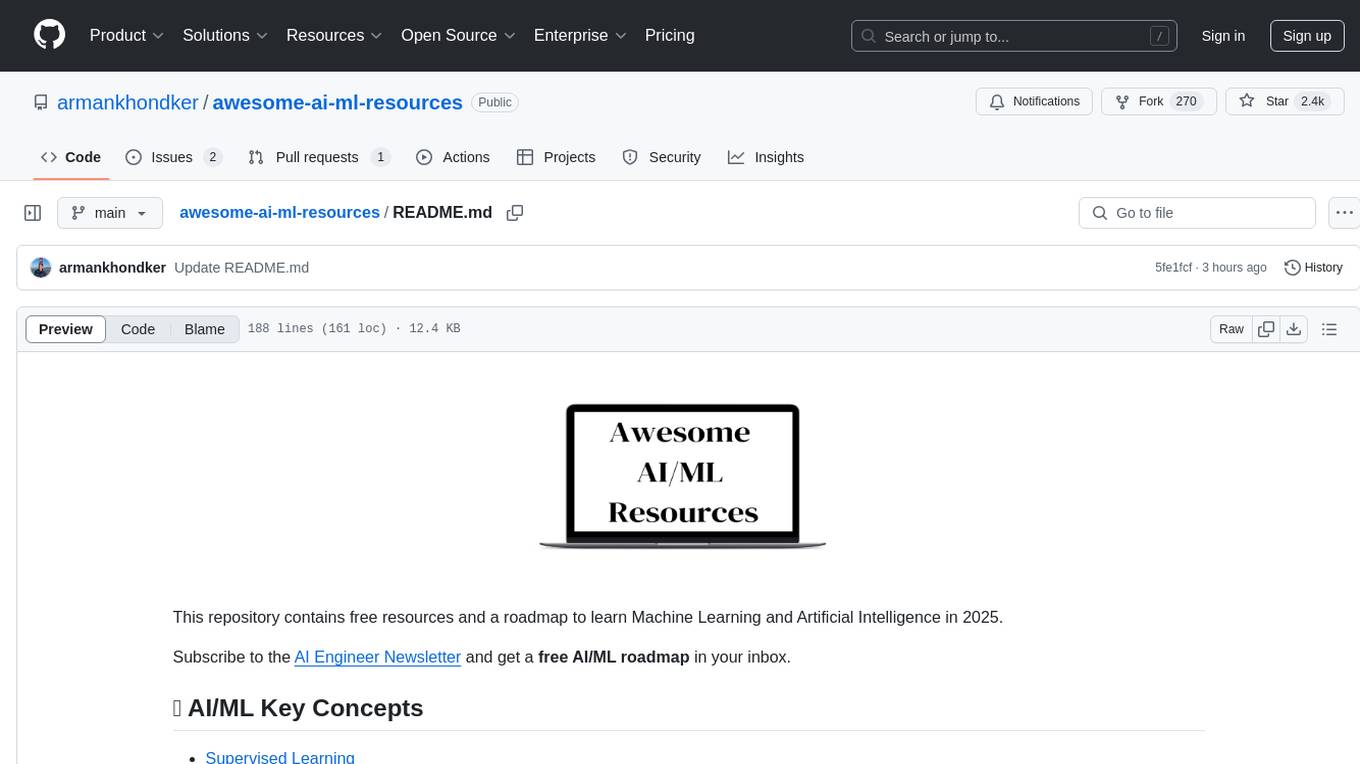
awesome-ai-ml-resources
This repository is a collection of free resources and a roadmap designed to help individuals learn Machine Learning and Artificial Intelligence concepts by providing key concepts, building blocks, roles, a learning roadmap, courses, certifications, books, tools & frameworks, research blogs, applied blogs, practice problems, communities, YouTube channels, newsletters, and must-read papers. It covers a wide range of topics from supervised learning to MLOps, offering guidance on learning paths, practical experience, and job interview preparation.
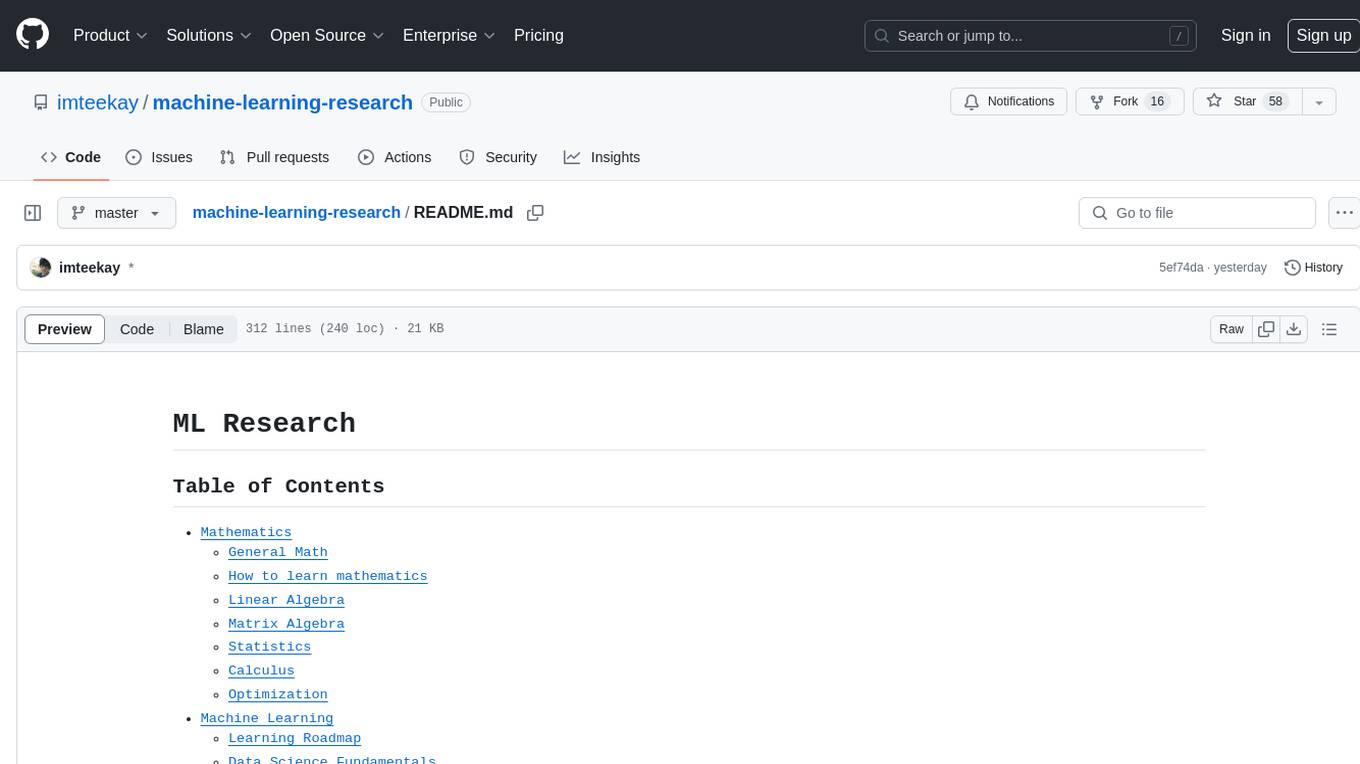
machine-learning-research
The 'machine-learning-research' repository is a comprehensive collection of resources related to mathematics, machine learning, deep learning, artificial intelligence, data science, and various scientific fields. It includes materials such as courses, tutorials, books, podcasts, communities, online courses, papers, and dissertations. The repository covers topics ranging from fundamental math skills to advanced machine learning concepts, with a focus on applications in healthcare, genetics, computational biology, precision health, and AI in science. It serves as a valuable resource for individuals interested in learning and researching in the fields of machine learning and related disciplines.
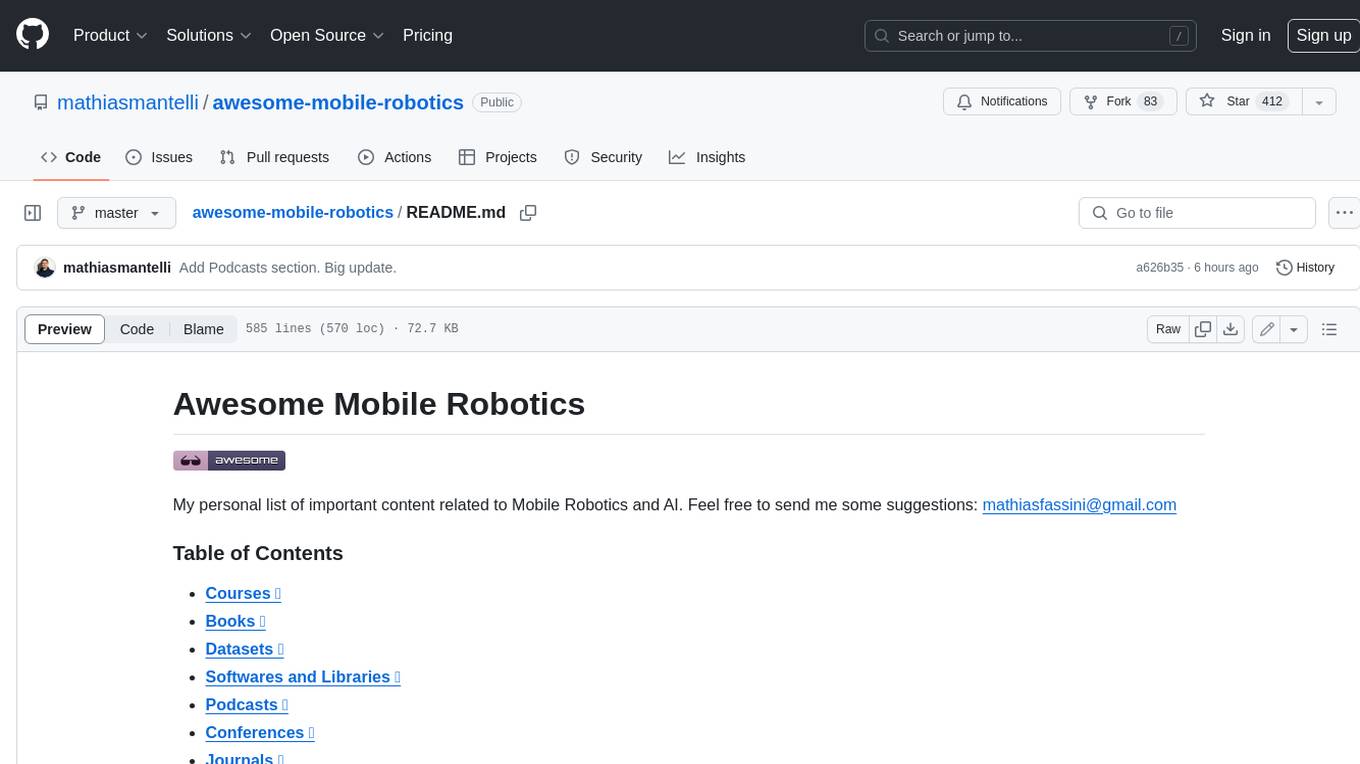
awesome-mobile-robotics
The 'awesome-mobile-robotics' repository is a curated list of important content related to Mobile Robotics and AI. It includes resources such as courses, books, datasets, software and libraries, podcasts, conferences, journals, companies and jobs, laboratories and research groups, and miscellaneous resources. The repository covers a wide range of topics in the field of Mobile Robotics and AI, providing valuable information for enthusiasts, researchers, and professionals in the domain.
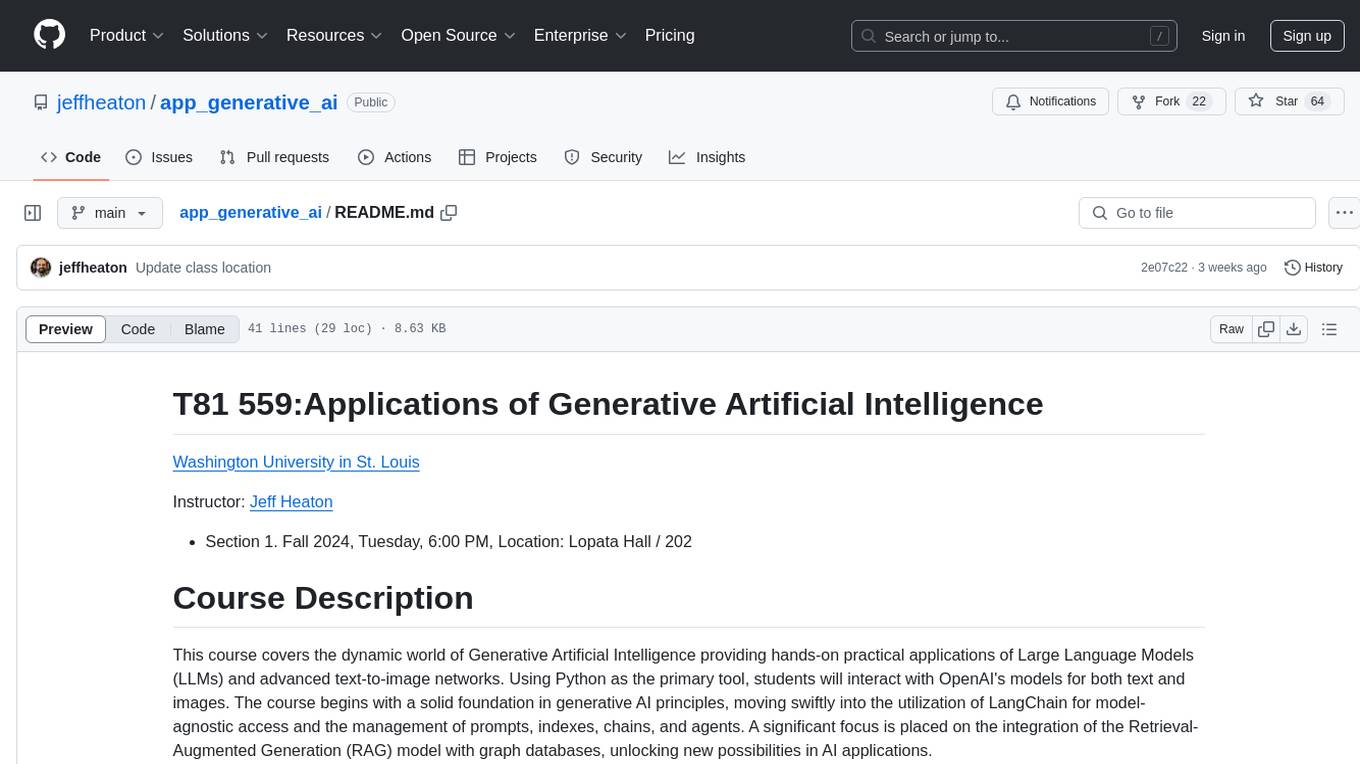
app_generative_ai
This repository contains course materials for T81 559: Applications of Generative Artificial Intelligence at Washington University in St. Louis. The course covers practical applications of Large Language Models (LLMs) and text-to-image networks using Python. Students learn about generative AI principles, LangChain, Retrieval-Augmented Generation (RAG) model, image generation techniques, fine-tuning neural networks, and prompt engineering. Ideal for students, researchers, and professionals in computer science, the course offers a transformative learning experience in the realm of Generative AI.
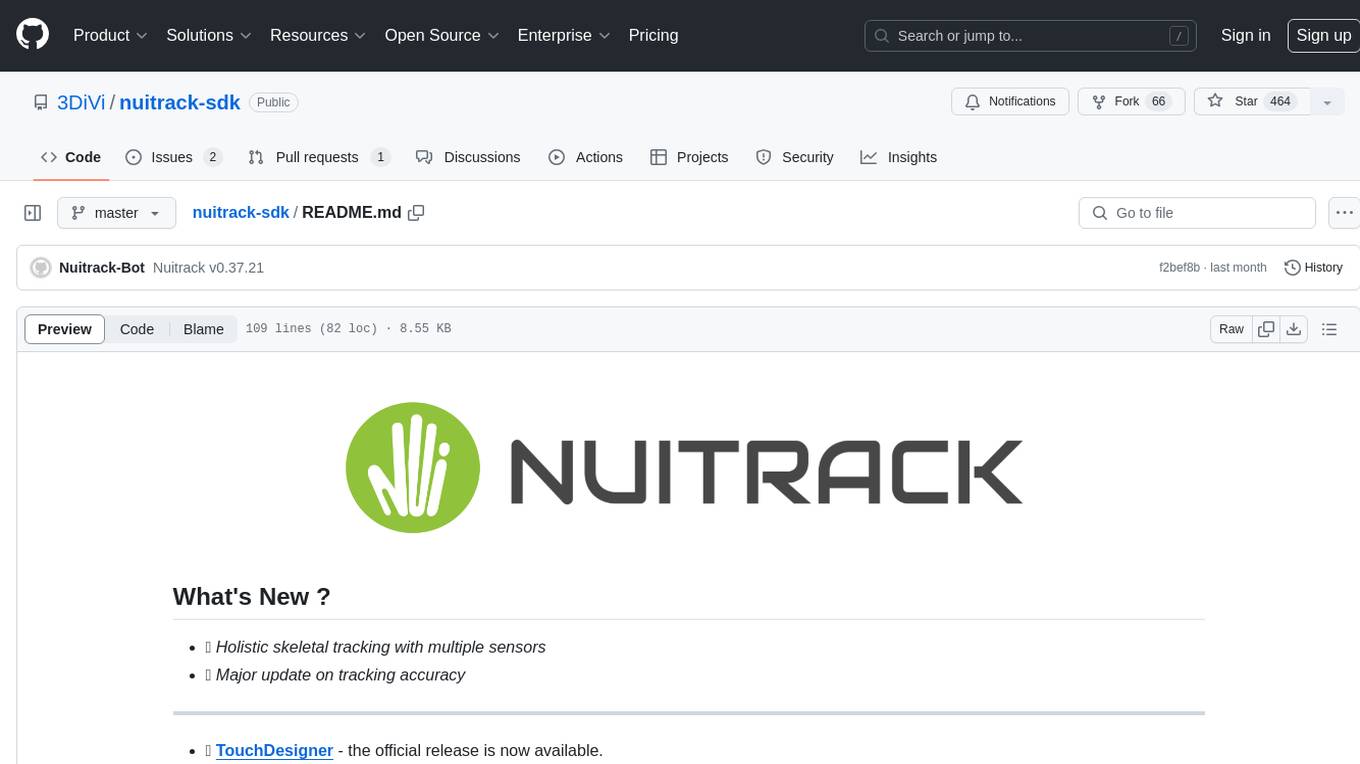
nuitrack-sdk
Nuitrack™ is an ultimate 3D body tracking solution developed by 3DiVi Inc. It enables body motion analytics applications for virtually any widespread depth sensors and hardware platforms, supporting a wide range of applications from real-time gesture recognition on embedded platforms to large-scale multisensor analytical systems. Nuitrack provides highly-sophisticated 3D skeletal tracking, basic facial analysis, hand tracking, and gesture recognition APIs for UI control. It offers two skeletal tracking engines: classical for embedded hardware and AI for complex poses, providing a human-centric spatial understanding tool for natural and intelligent user engagement.
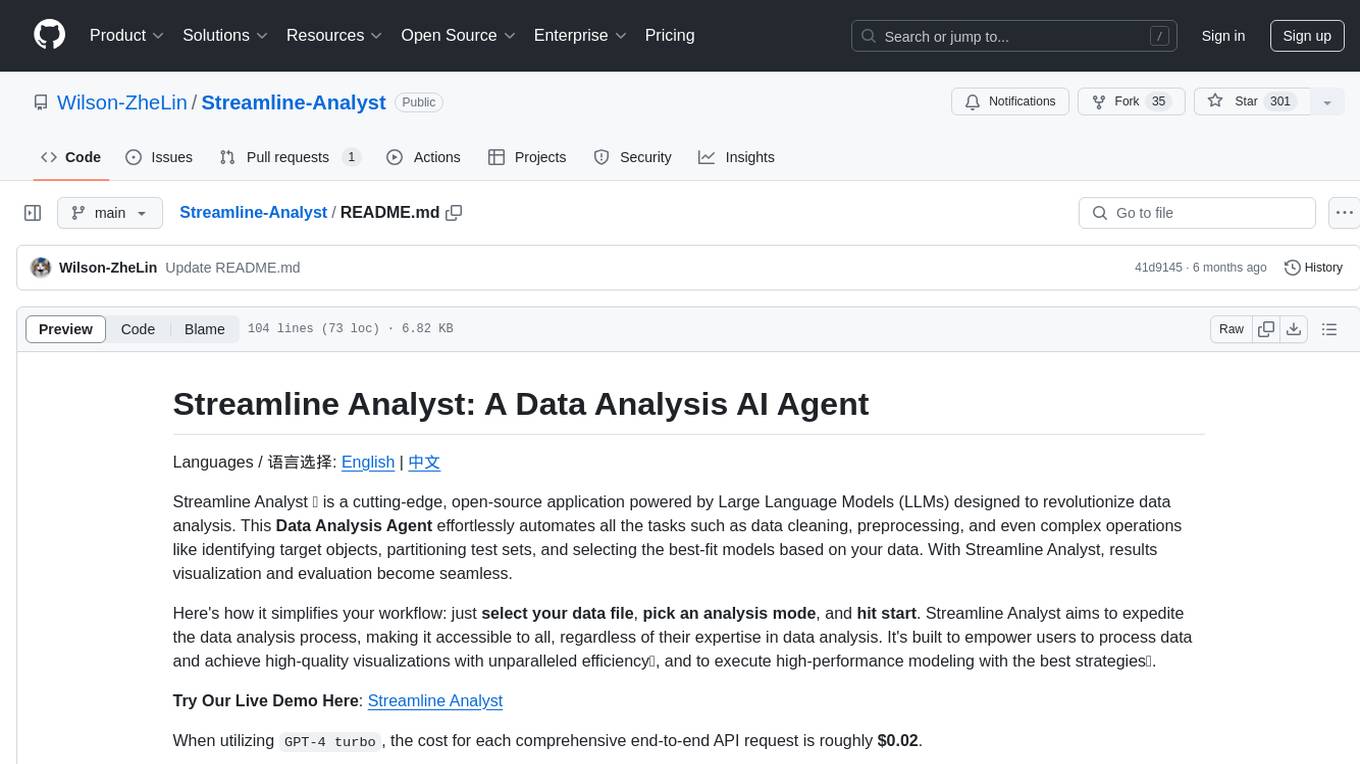
Streamline-Analyst
Streamline Analyst is a cutting-edge, open-source application powered by Large Language Models (LLMs) designed to revolutionize data analysis. This Data Analysis Agent effortlessly automates tasks such as data cleaning, preprocessing, and complex operations like identifying target objects, partitioning test sets, and selecting the best-fit models based on your data. With Streamline Analyst, results visualization and evaluation become seamless. It aims to expedite the data analysis process, making it accessible to all, regardless of their expertise in data analysis. The tool is built to empower users to process data and achieve high-quality visualizations with unparalleled efficiency, and to execute high-performance modeling with the best strategies. Future enhancements include Natural Language Processing (NLP), neural networks, and object detection utilizing YOLO, broadening its capabilities to meet diverse data analysis needs.
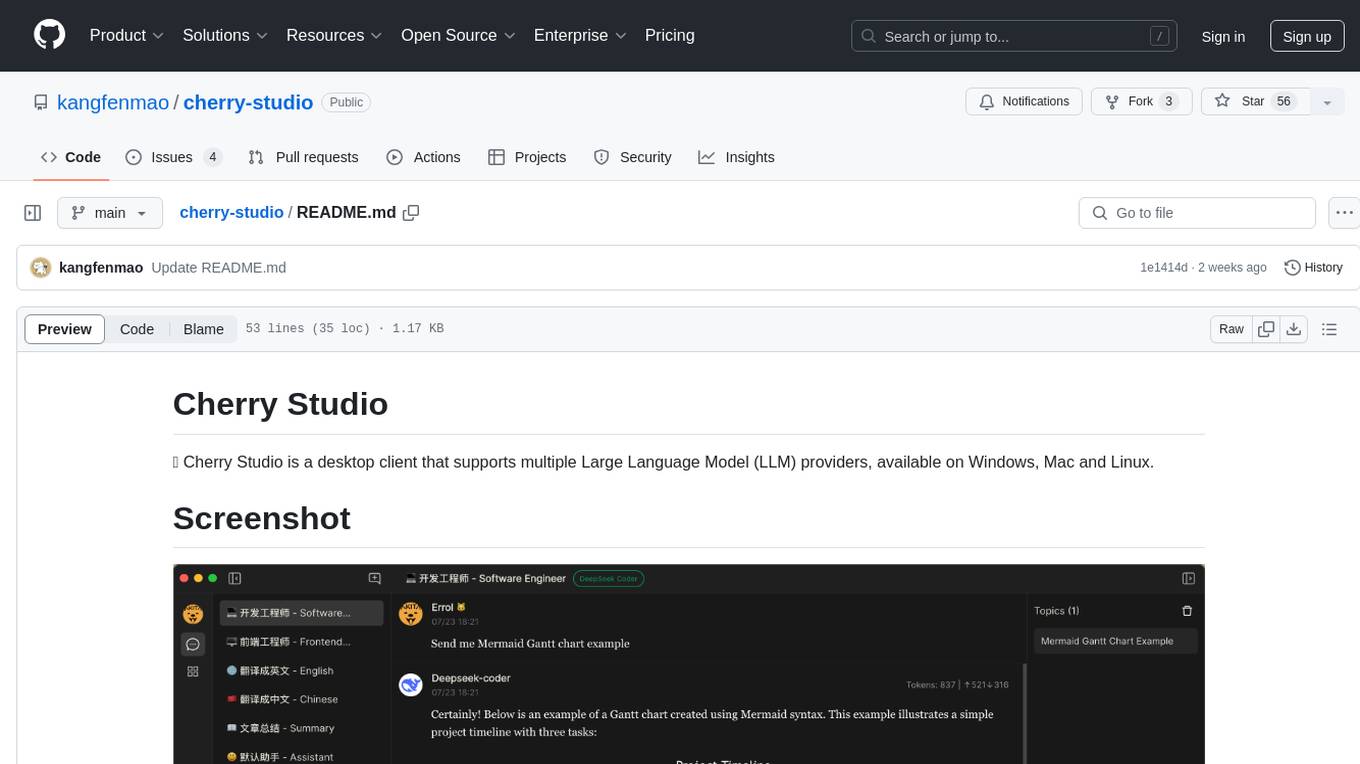
cherry-studio
Cherry Studio is a desktop client that supports multiple Large Language Model (LLM) providers, available on Windows, Mac, and Linux. It allows users to create multiple Assistants and topics, use multiple models to answer questions in the same conversation, and supports drag-and-drop sorting, code highlighting, and Mermaid chart. The tool is designed to enhance productivity and streamline the process of interacting with various language models.
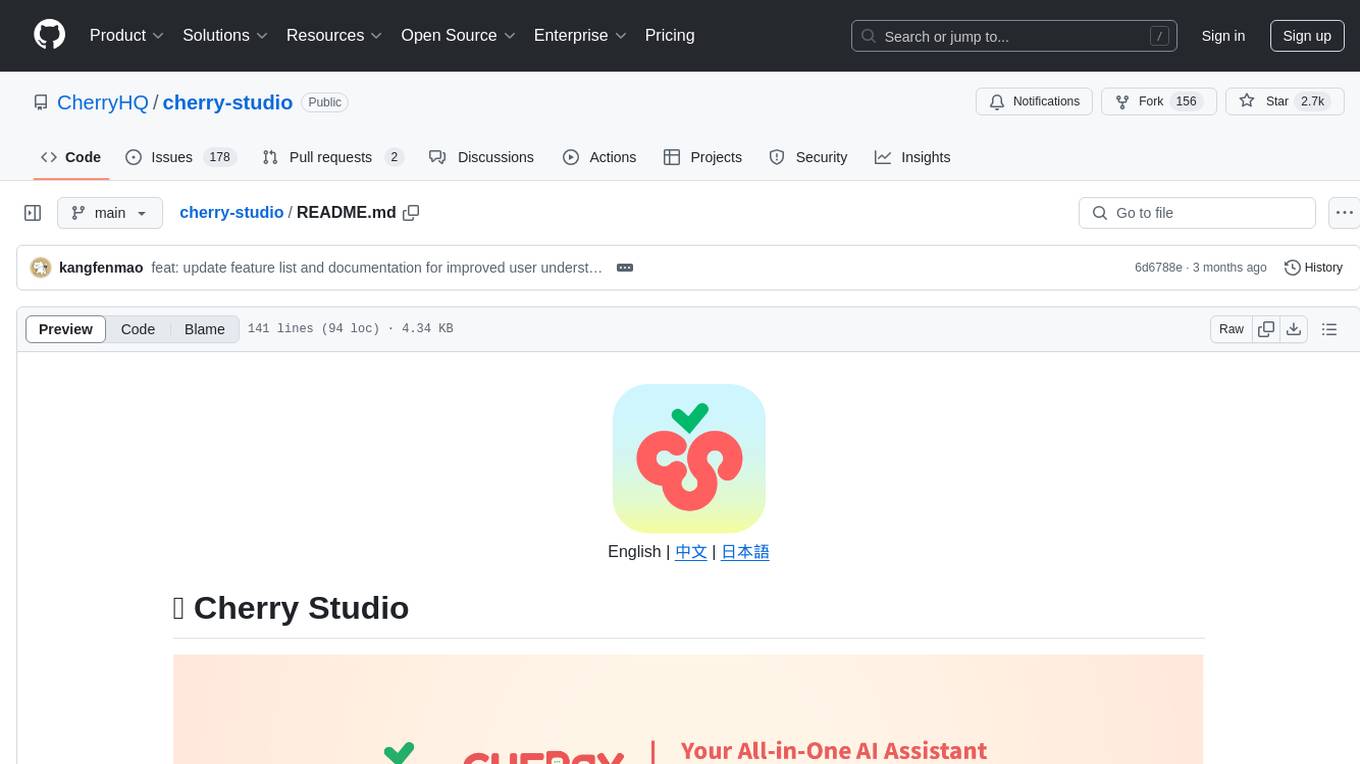
cherry-studio
Cherry Studio is a desktop client that supports multiple LLM providers on Windows, Mac, and Linux. It offers diverse LLM provider support, AI assistants & conversations, document & data processing, practical tools integration, and enhanced user experience. The tool includes features like support for major LLM cloud services, AI web service integration, local model support, pre-configured AI assistants, document processing for text, images, and more, global search functionality, topic management system, AI-powered translation, and cross-platform support with ready-to-use features and themes for a better user experience.
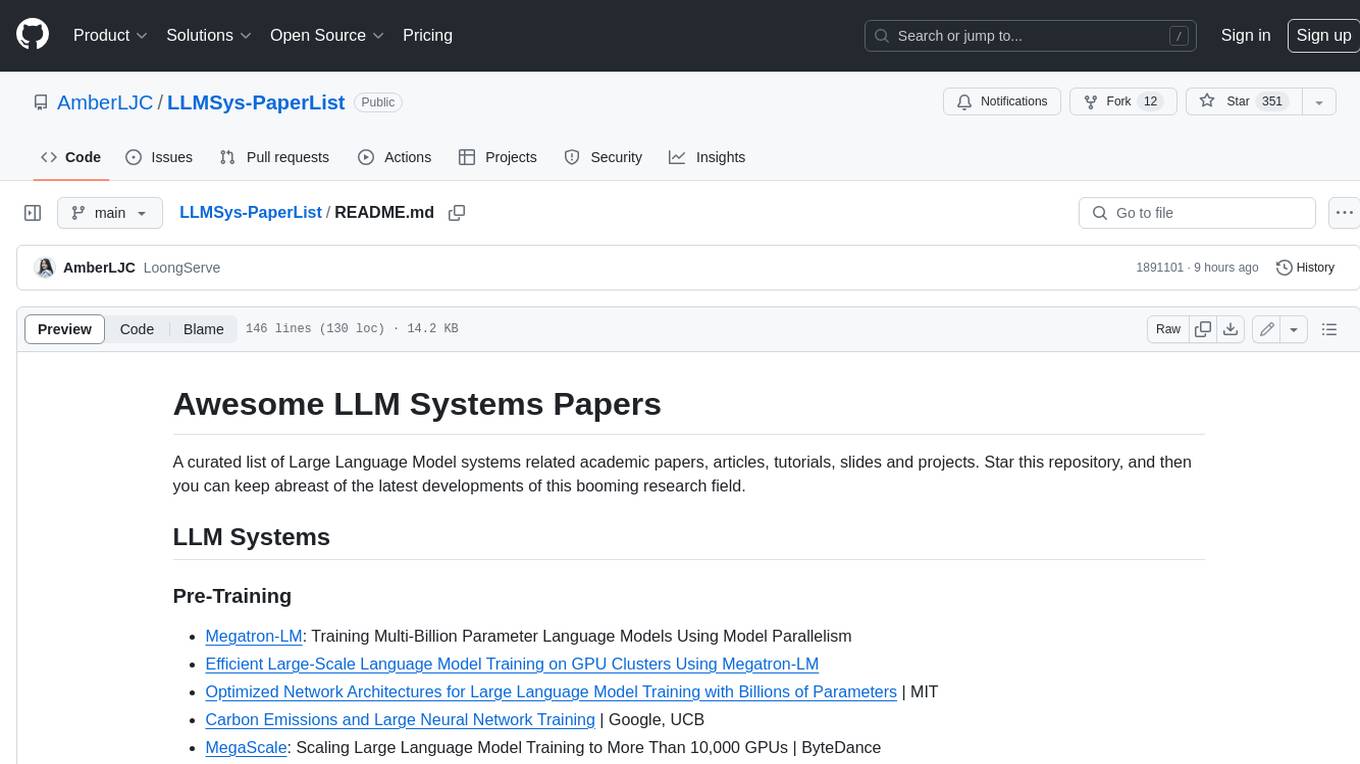
LLMSys-PaperList
This repository provides a comprehensive list of academic papers, articles, tutorials, slides, and projects related to Large Language Model (LLM) systems. It covers various aspects of LLM research, including pre-training, serving, system efficiency optimization, multi-model systems, image generation systems, LLM applications in systems, ML systems, survey papers, LLM benchmarks and leaderboards, and other relevant resources. The repository is regularly updated to include the latest developments in this rapidly evolving field, making it a valuable resource for researchers, practitioners, and anyone interested in staying abreast of the advancements in LLM technology.
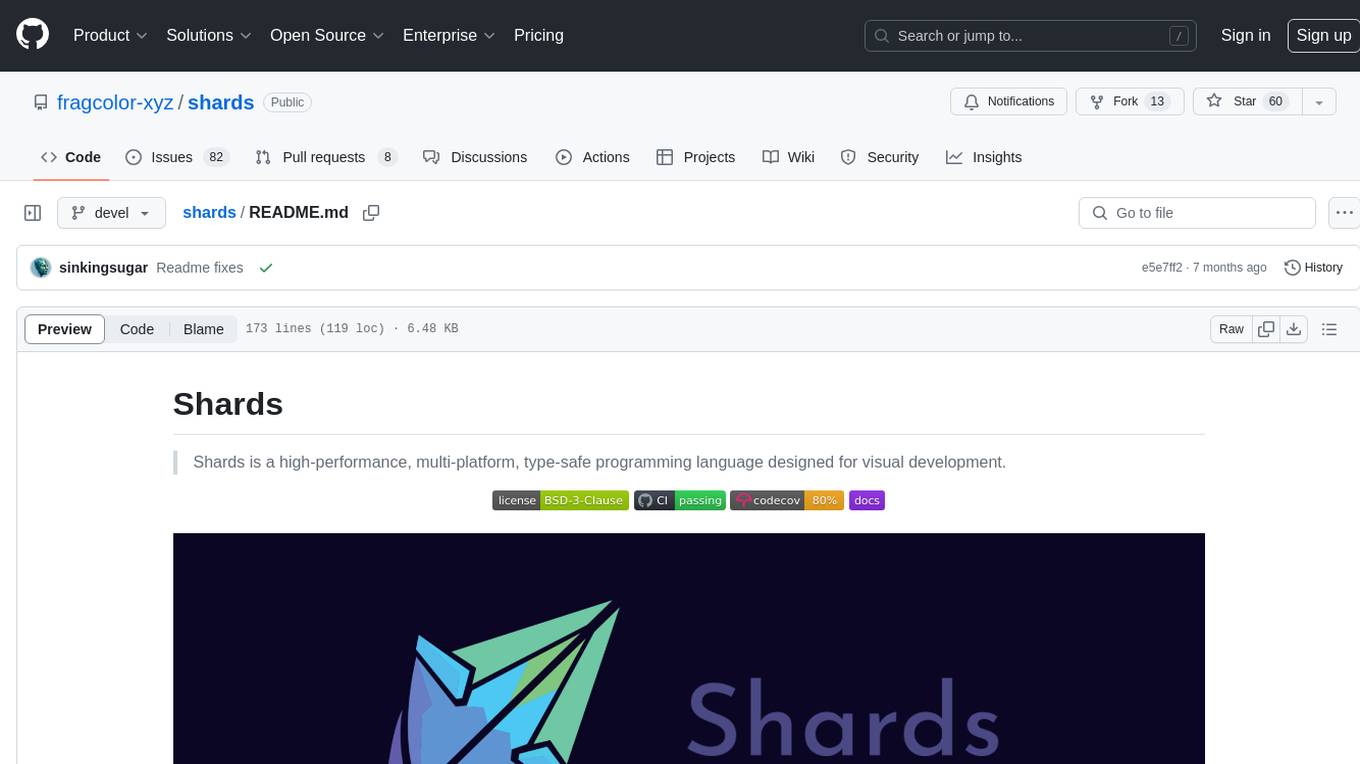
shards
Shards is a high-performance, multi-platform, type-safe programming language designed for visual development. It is a dataflow visual programming language that enables building full-fledged apps and games without traditional coding. Shards features automatic type checking, optimized shard implementations for high performance, and an intuitive visual workflow for beginners. The language allows seamless round-trip engineering between code and visual models, empowering users to create multi-platform apps easily. Shards also powers an upcoming AI-powered game creation system, enabling real-time collaboration and game development in a low to no-code environment.
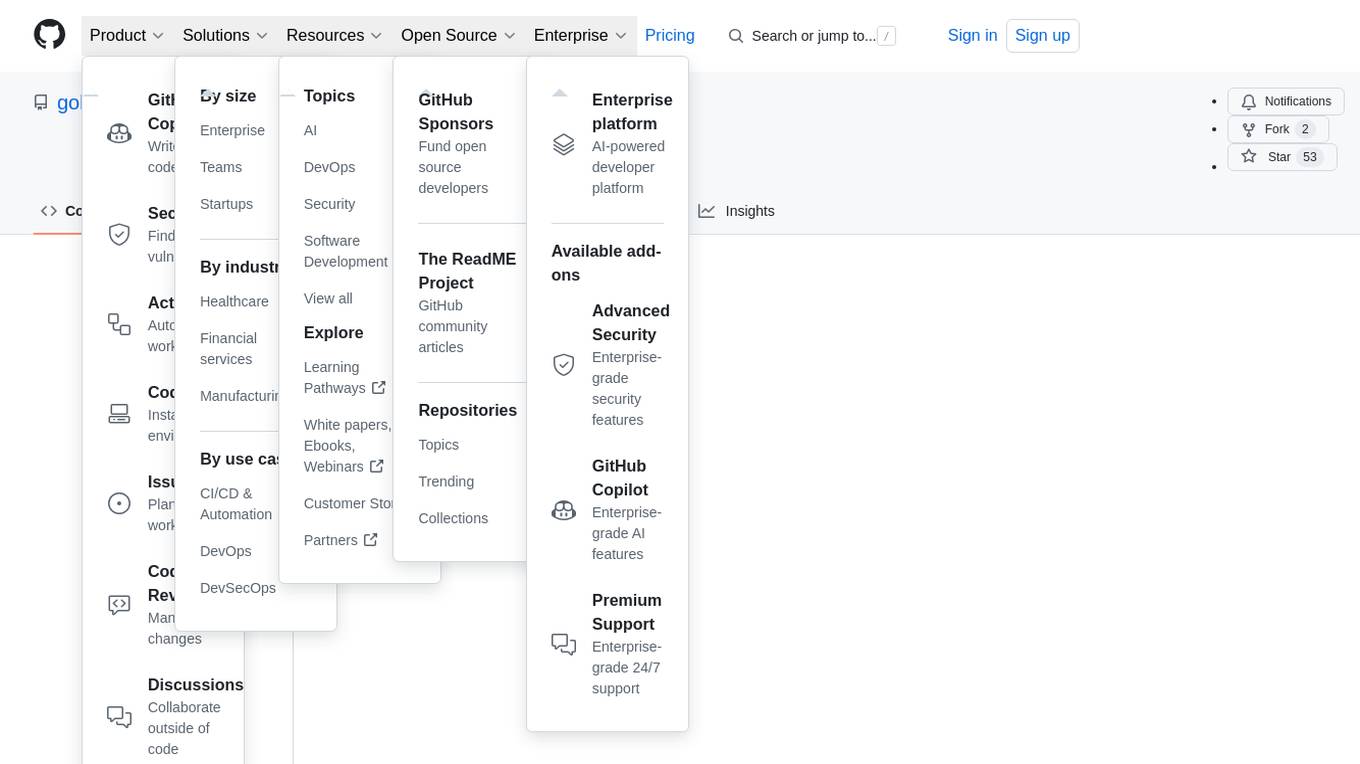
ComfyUI-fal-API
ComfyUI-fal-API is a repository containing custom nodes for using Flux models with fal API in ComfyUI. It provides nodes for image generation, video generation, language models, and vision language models. Users can easily install and configure the repository to access various nodes for different tasks such as generating images, creating videos, processing text, and understanding images. The repository also includes troubleshooting steps and is licensed under the Apache License 2.0.
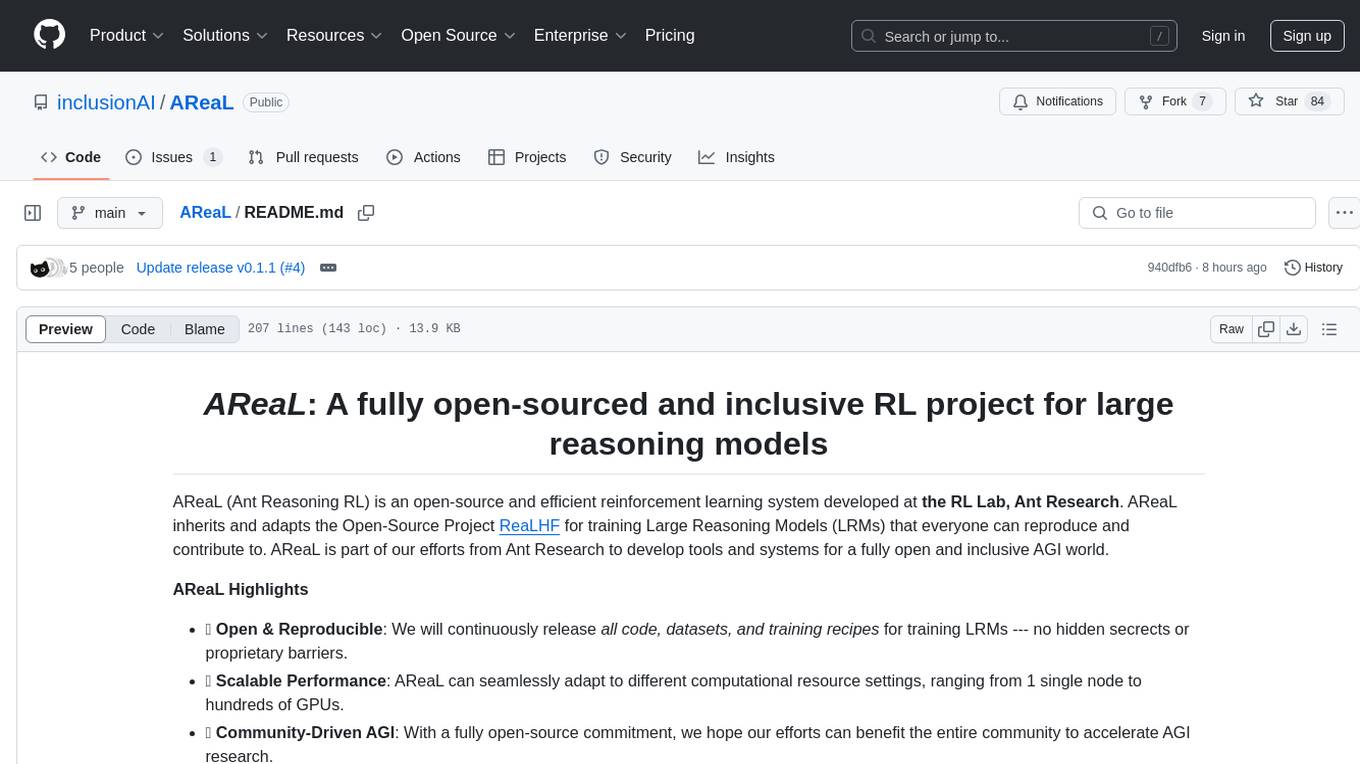
AReaL
AReaL (Ant Reasoning RL) is an open-source reinforcement learning system developed at the RL Lab, Ant Research. It is designed for training Large Reasoning Models (LRMs) in a fully open and inclusive manner. AReaL provides reproducible experiments for 1.5B and 7B LRMs, showcasing its scalability and performance across diverse computational budgets. The system follows an iterative training process to enhance model performance, with a focus on mathematical reasoning tasks. AReaL is equipped to adapt to different computational resource settings, enabling users to easily configure and launch training trials. Future plans include support for advanced models, optimizations for distributed training, and exploring research topics to enhance LRMs' reasoning capabilities.
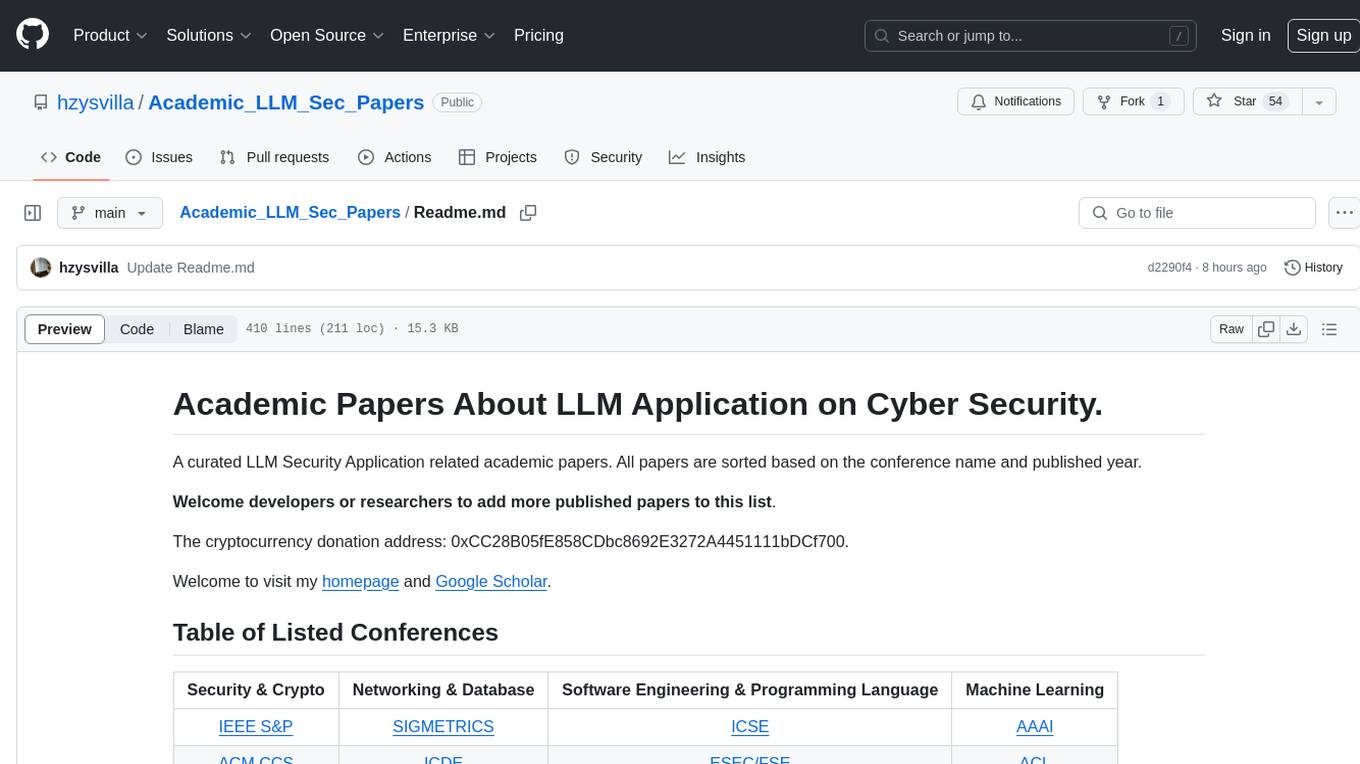
Academic_LLM_Sec_Papers
Academic_LLM_Sec_Papers is a curated collection of academic papers related to LLM Security Application. The repository includes papers sorted by conference name and published year, covering topics such as large language models for blockchain security, software engineering, machine learning, and more. Developers and researchers are welcome to contribute additional published papers to the list. The repository also provides information on listed conferences and journals related to security, networking, software engineering, and cryptography. The papers cover a wide range of topics including privacy risks, ethical concerns, vulnerabilities, threat modeling, code analysis, fuzzing, and more.
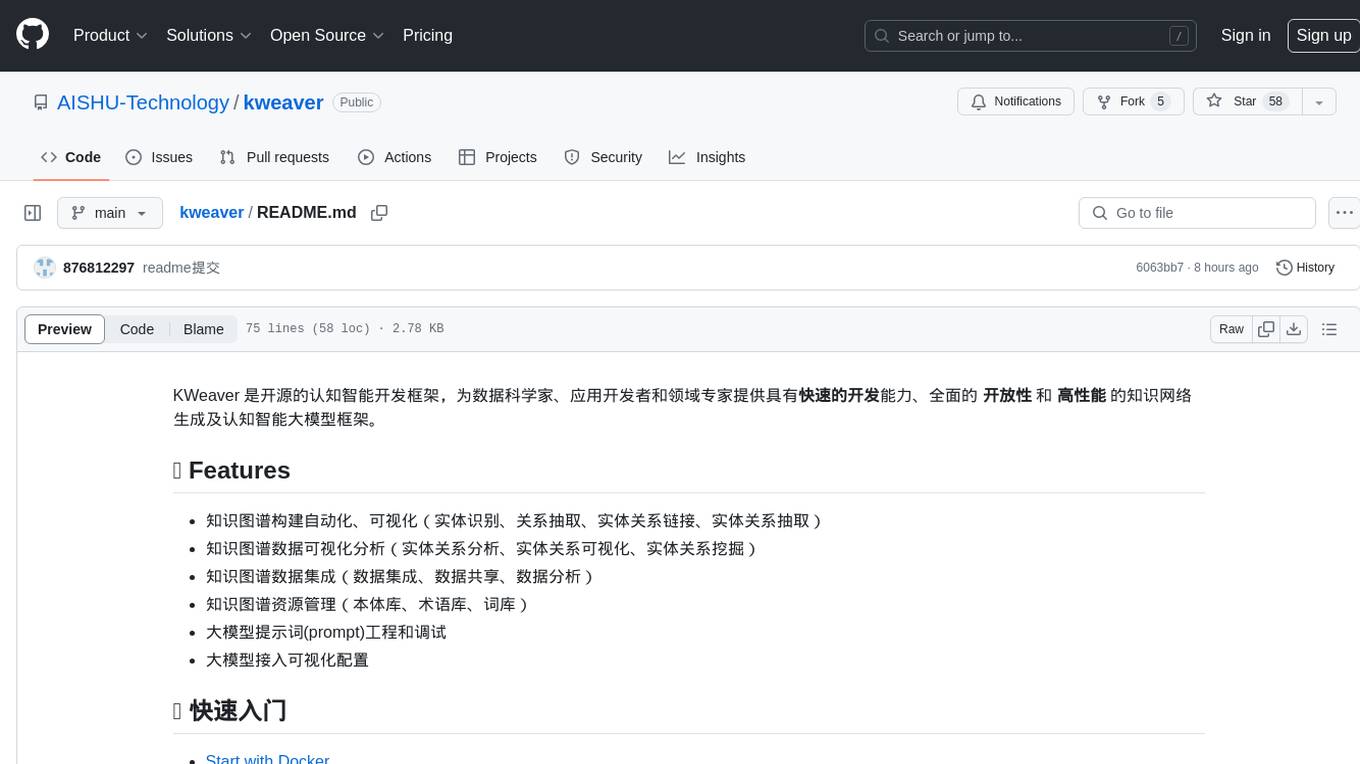
kweaver
KWeaver is an open-source cognitive intelligence development framework that provides data scientists, application developers, and domain experts with the ability for rapid development, comprehensive openness, and high-performance knowledge network generation and cognitive intelligence large model framework. It offers features such as automated and visual knowledge graph construction, visualization and analysis of knowledge graph data, knowledge graph integration, knowledge graph resource management, large model prompt engineering and debugging, and visual configuration for large model access.
For similar tasks
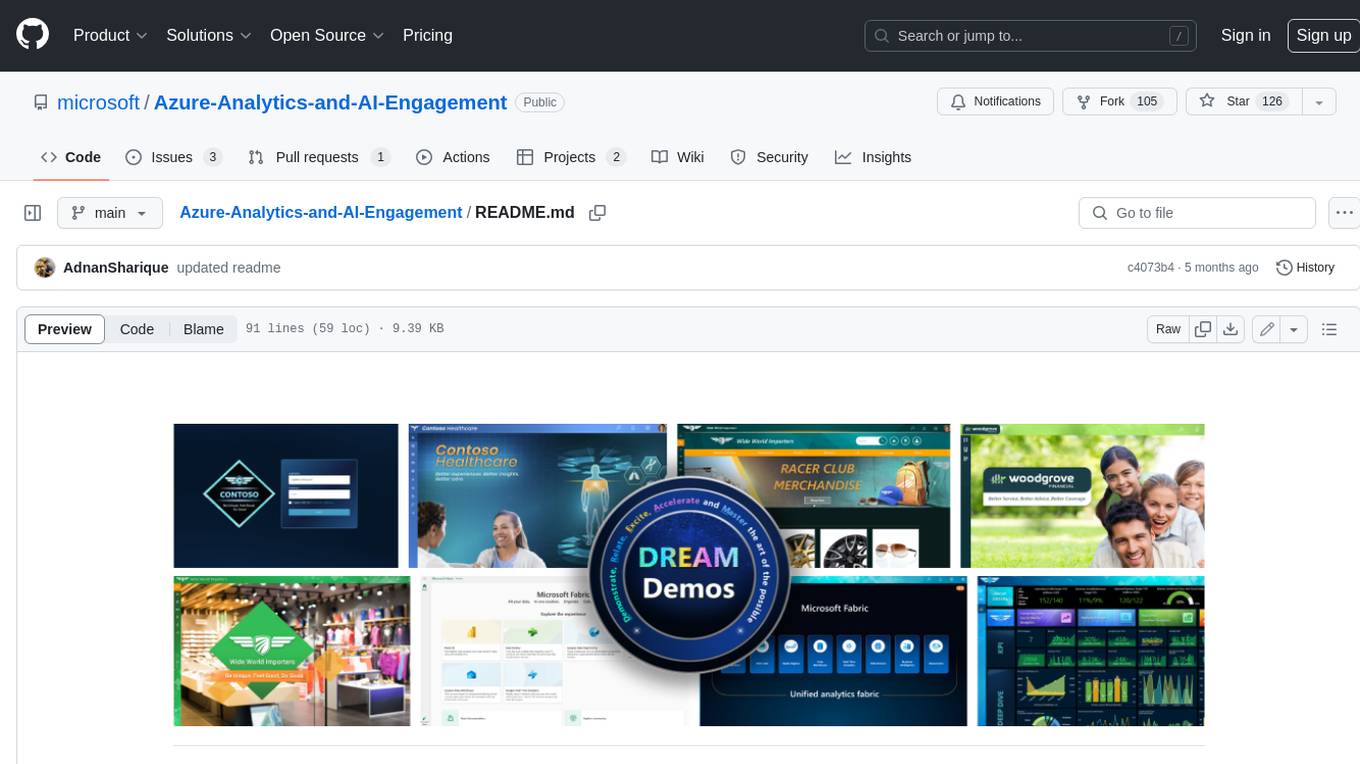
Azure-Analytics-and-AI-Engagement
The Azure-Analytics-and-AI-Engagement repository provides packaged Industry Scenario DREAM Demos with ARM templates (Containing a demo web application, Power BI reports, Synapse resources, AML Notebooks etc.) that can be deployed in a customer’s subscription using the CAPE tool within a matter of few hours. Partners can also deploy DREAM Demos in their own subscriptions using DPoC.
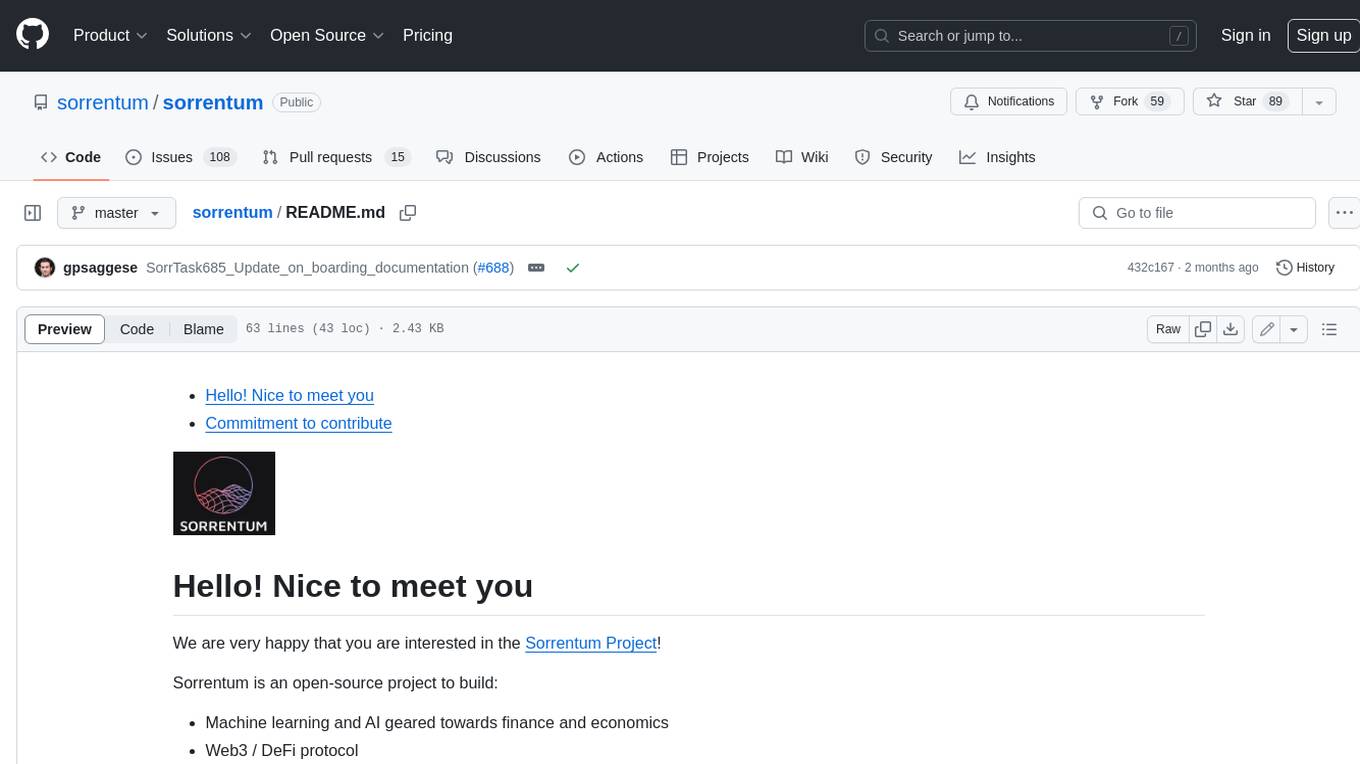
sorrentum
Sorrentum is an open-source project that aims to combine open-source development, startups, and brilliant students to build machine learning, AI, and Web3 / DeFi protocols geared towards finance and economics. The project provides opportunities for internships, research assistantships, and development grants, as well as the chance to work on cutting-edge problems, learn about startups, write academic papers, and get internships and full-time positions at companies working on Sorrentum applications.
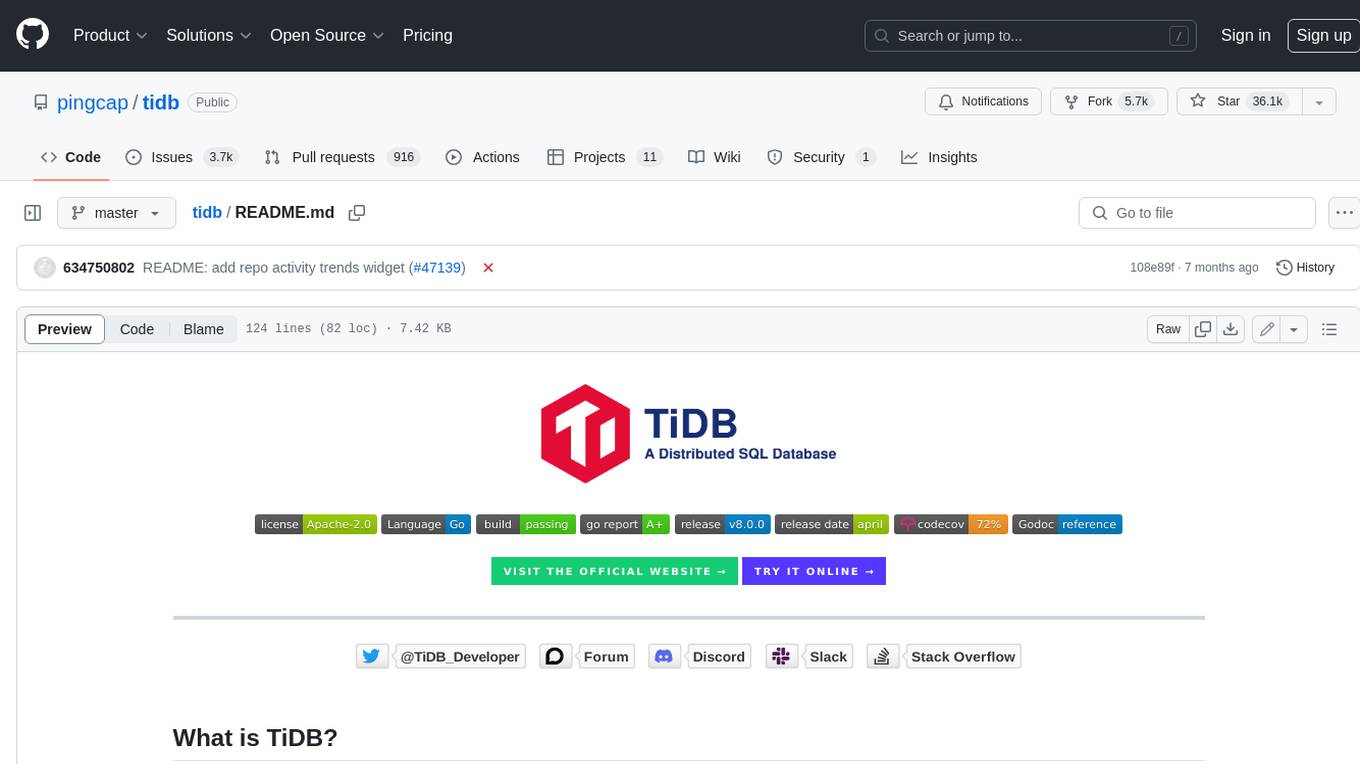
tidb
TiDB is an open-source distributed SQL database that supports Hybrid Transactional and Analytical Processing (HTAP) workloads. It is MySQL compatible and features horizontal scalability, strong consistency, and high availability.
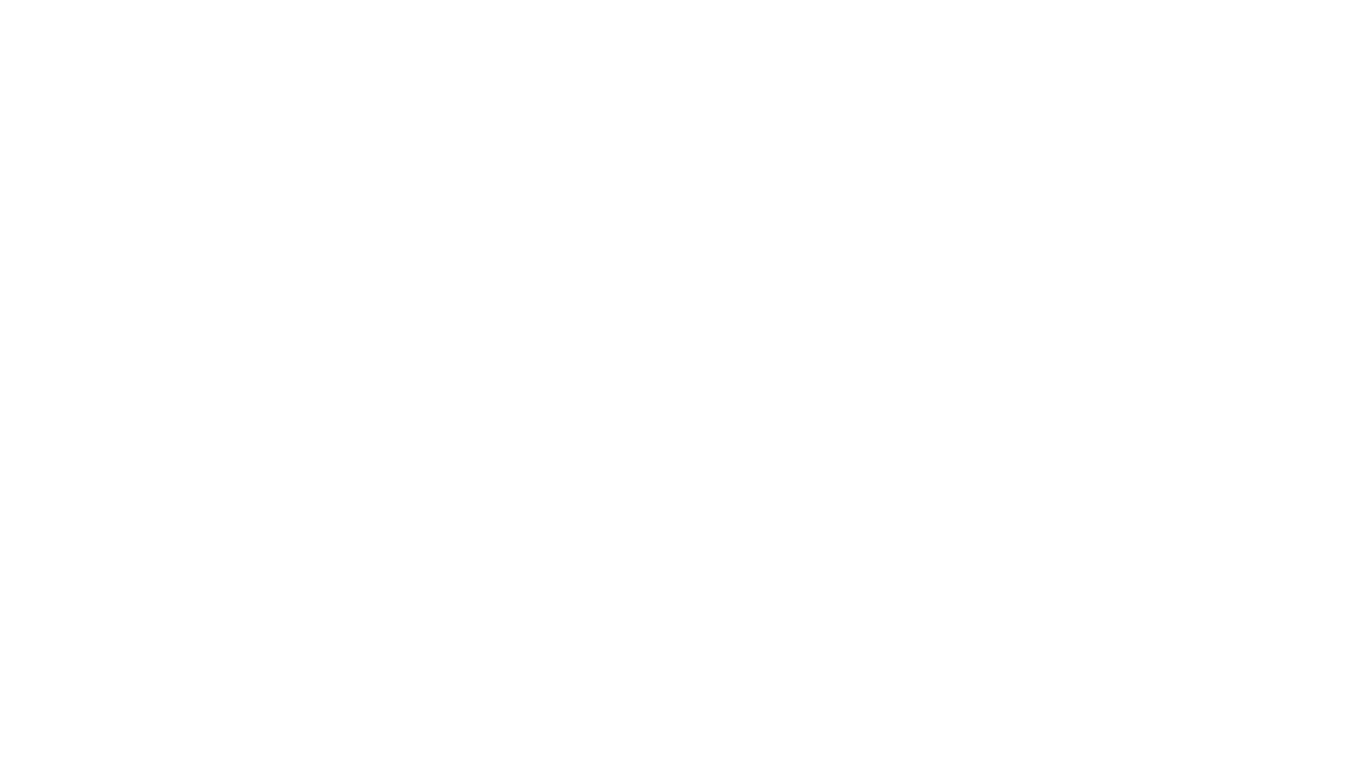
zep-python
Zep is an open-source platform for building and deploying large language model (LLM) applications. It provides a suite of tools and services that make it easy to integrate LLMs into your applications, including chat history memory, embedding, vector search, and data enrichment. Zep is designed to be scalable, reliable, and easy to use, making it a great choice for developers who want to build LLM-powered applications quickly and easily.
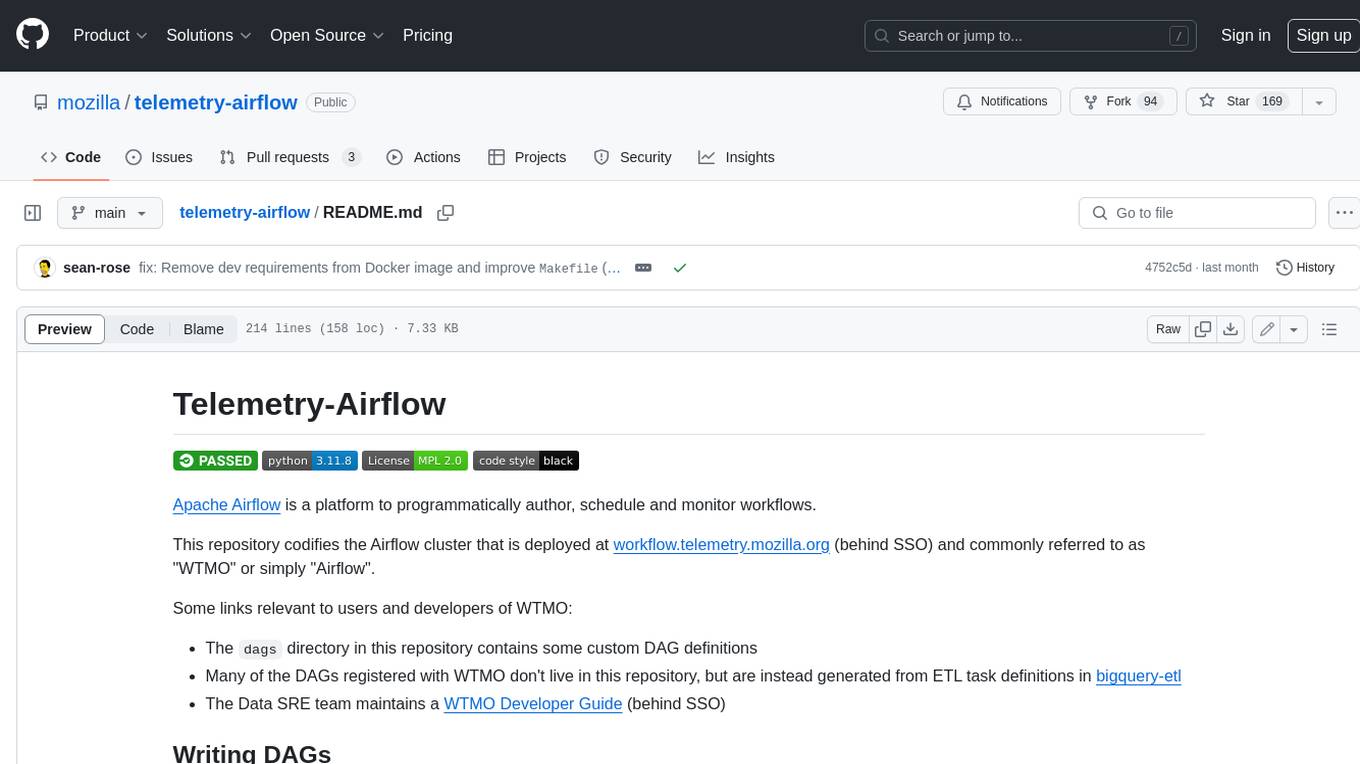
telemetry-airflow
This repository codifies the Airflow cluster that is deployed at workflow.telemetry.mozilla.org (behind SSO) and commonly referred to as "WTMO" or simply "Airflow". Some links relevant to users and developers of WTMO: * The `dags` directory in this repository contains some custom DAG definitions * Many of the DAGs registered with WTMO don't live in this repository, but are instead generated from ETL task definitions in bigquery-etl * The Data SRE team maintains a WTMO Developer Guide (behind SSO)
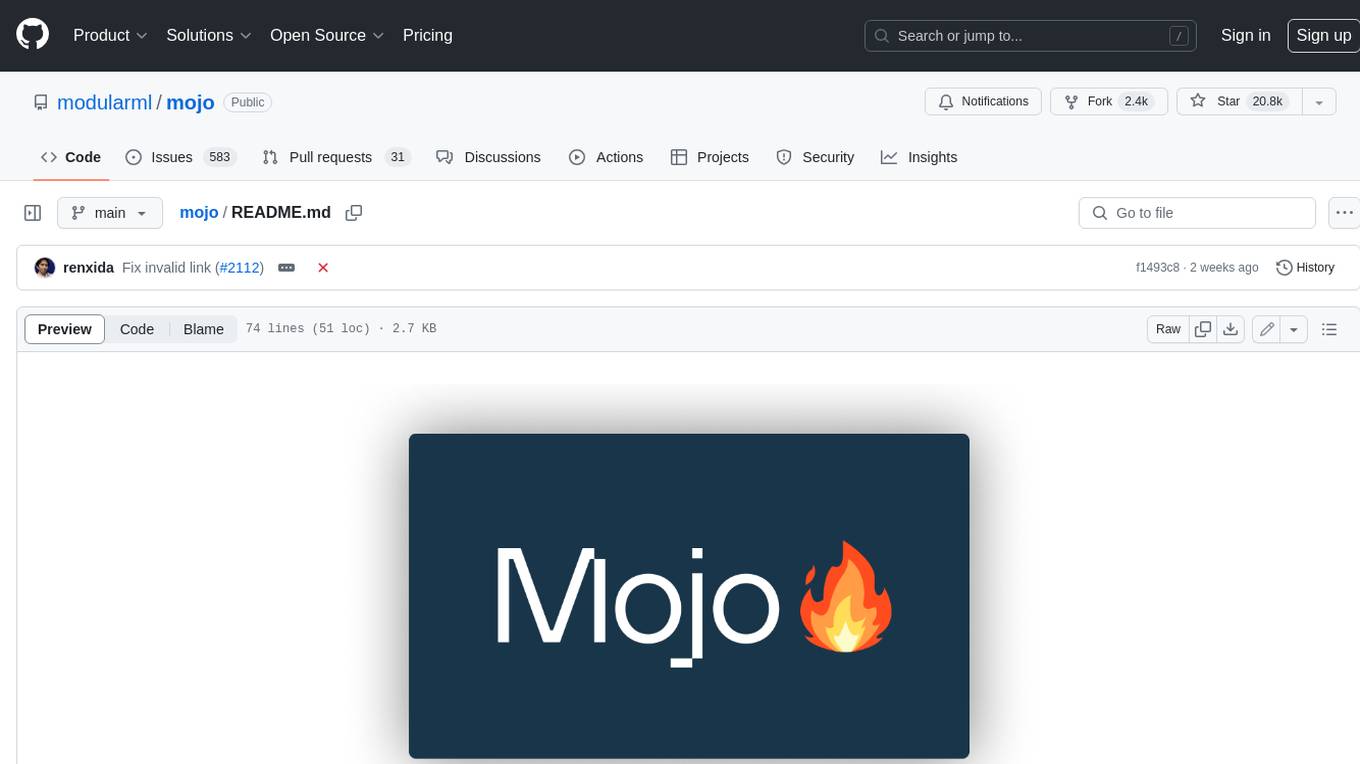
mojo
Mojo is a new programming language that bridges the gap between research and production by combining Python syntax and ecosystem with systems programming and metaprogramming features. Mojo is still young, but it is designed to become a superset of Python over time.
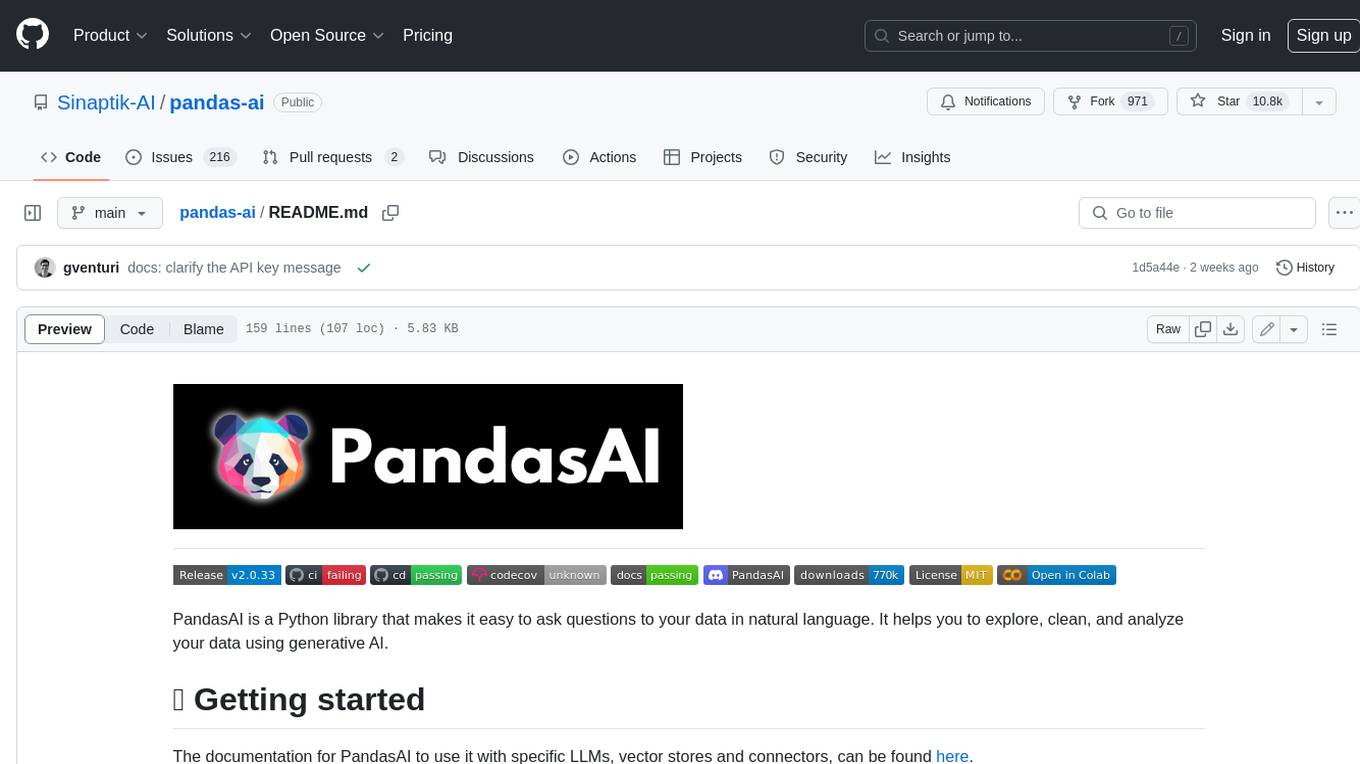
pandas-ai
PandasAI is a Python library that makes it easy to ask questions to your data in natural language. It helps you to explore, clean, and analyze your data using generative AI.
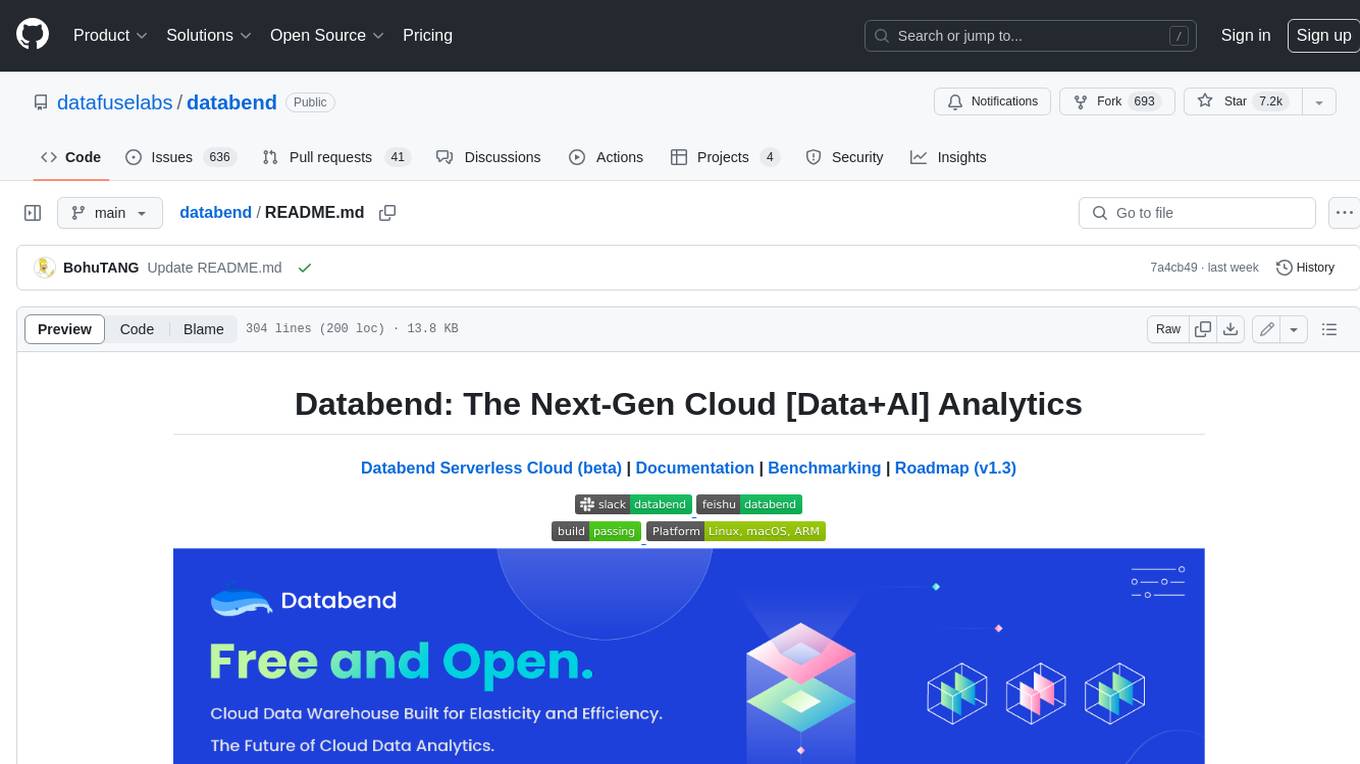
databend
Databend is an open-source cloud data warehouse that serves as a cost-effective alternative to Snowflake. With its focus on fast query execution and data ingestion, it's designed for complex analysis of the world's largest datasets.
For similar jobs
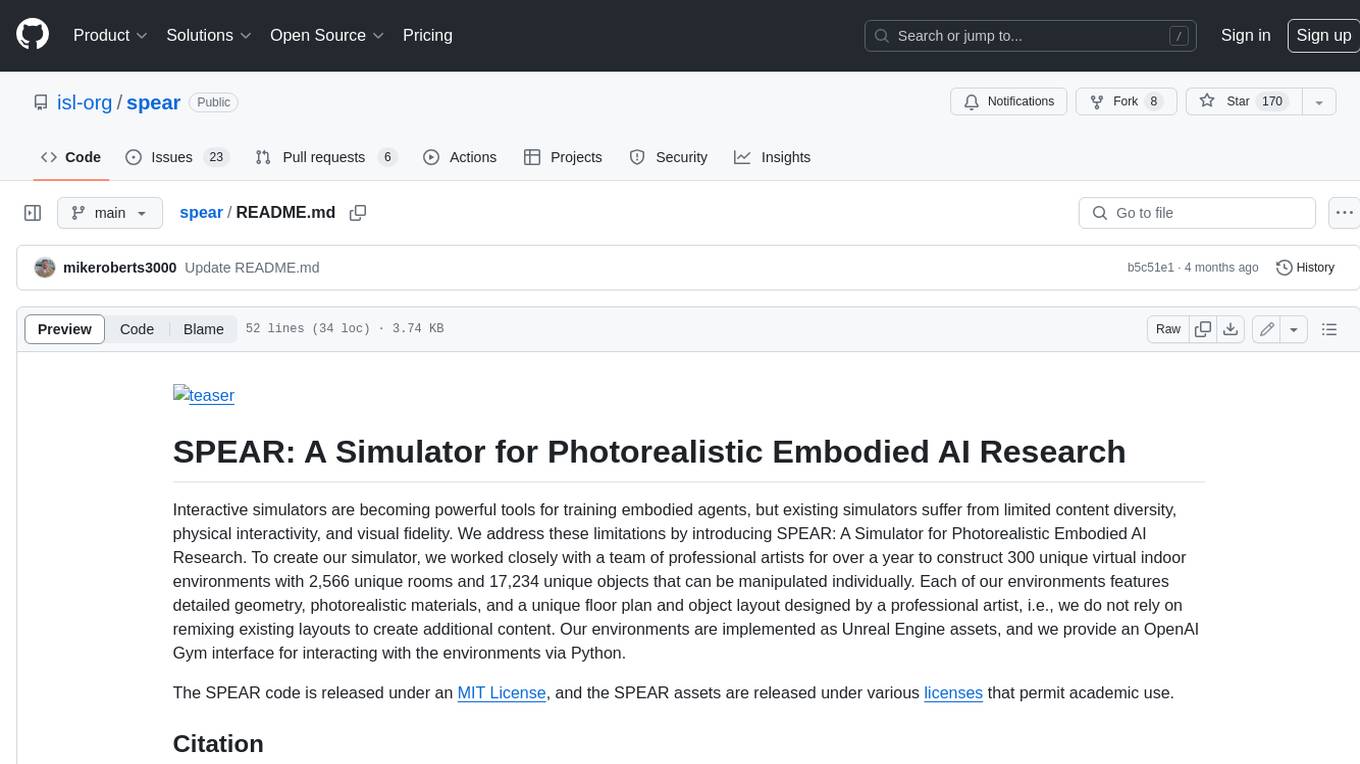
spear
SPEAR (Simulator for Photorealistic Embodied AI Research) is a powerful tool for training embodied agents. It features 300 unique virtual indoor environments with 2,566 unique rooms and 17,234 unique objects that can be manipulated individually. Each environment is designed by a professional artist and features detailed geometry, photorealistic materials, and a unique floor plan and object layout. SPEAR is implemented as Unreal Engine assets and provides an OpenAI Gym interface for interacting with the environments via Python.
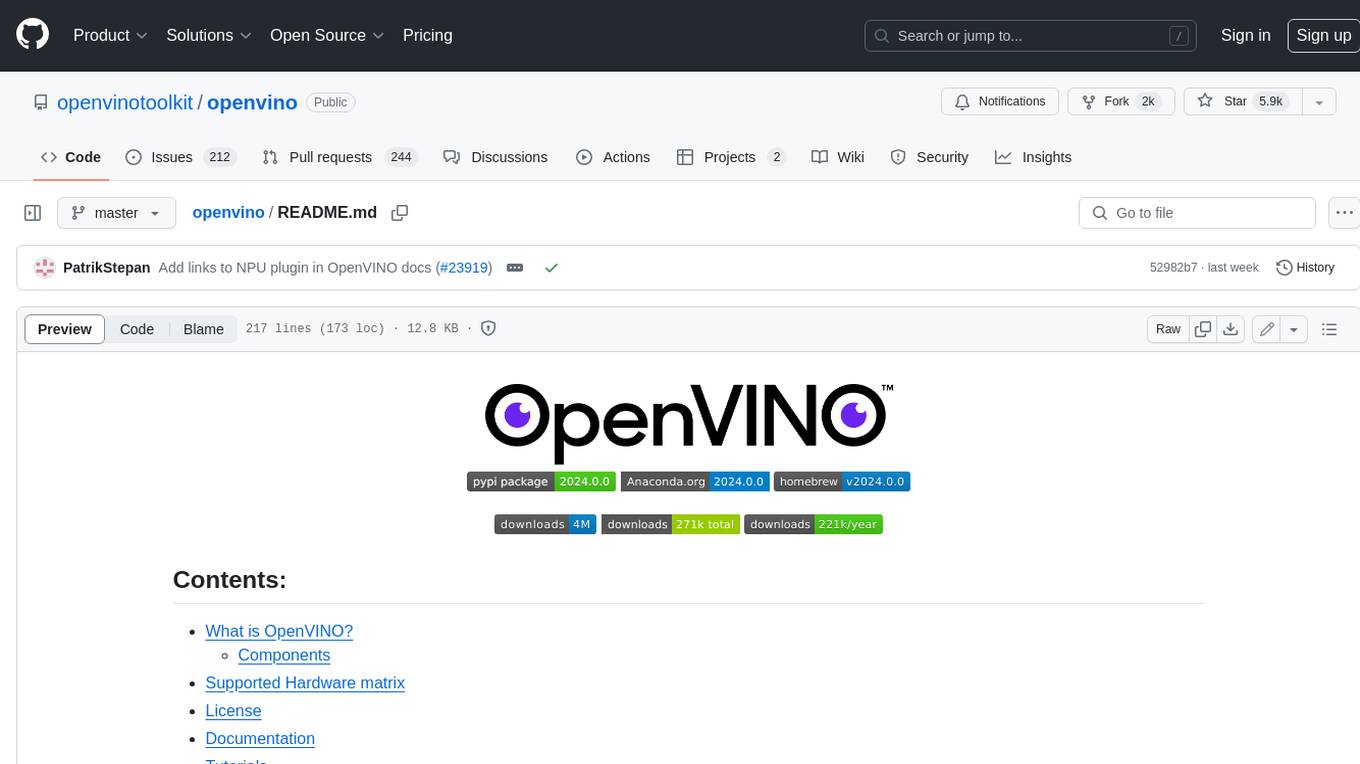
openvino
OpenVINO™ is an open-source toolkit for optimizing and deploying AI inference. It provides a common API to deliver inference solutions on various platforms, including CPU, GPU, NPU, and heterogeneous devices. OpenVINO™ supports pre-trained models from Open Model Zoo and popular frameworks like TensorFlow, PyTorch, and ONNX. Key components of OpenVINO™ include the OpenVINO™ Runtime, plugins for different hardware devices, frontends for reading models from native framework formats, and the OpenVINO Model Converter (OVC) for adjusting models for optimal execution on target devices.
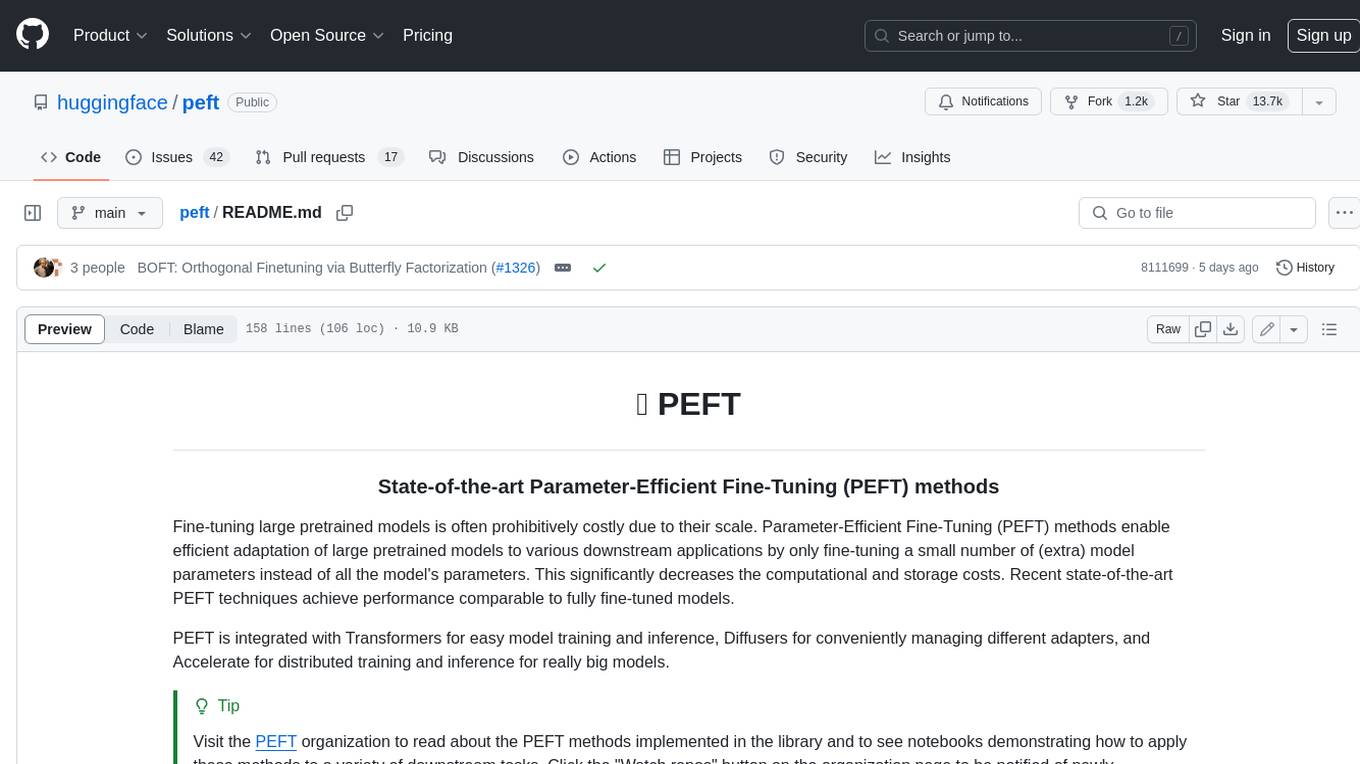
peft
PEFT (Parameter-Efficient Fine-Tuning) is a collection of state-of-the-art methods that enable efficient adaptation of large pretrained models to various downstream applications. By only fine-tuning a small number of extra model parameters instead of all the model's parameters, PEFT significantly decreases the computational and storage costs while achieving performance comparable to fully fine-tuned models.
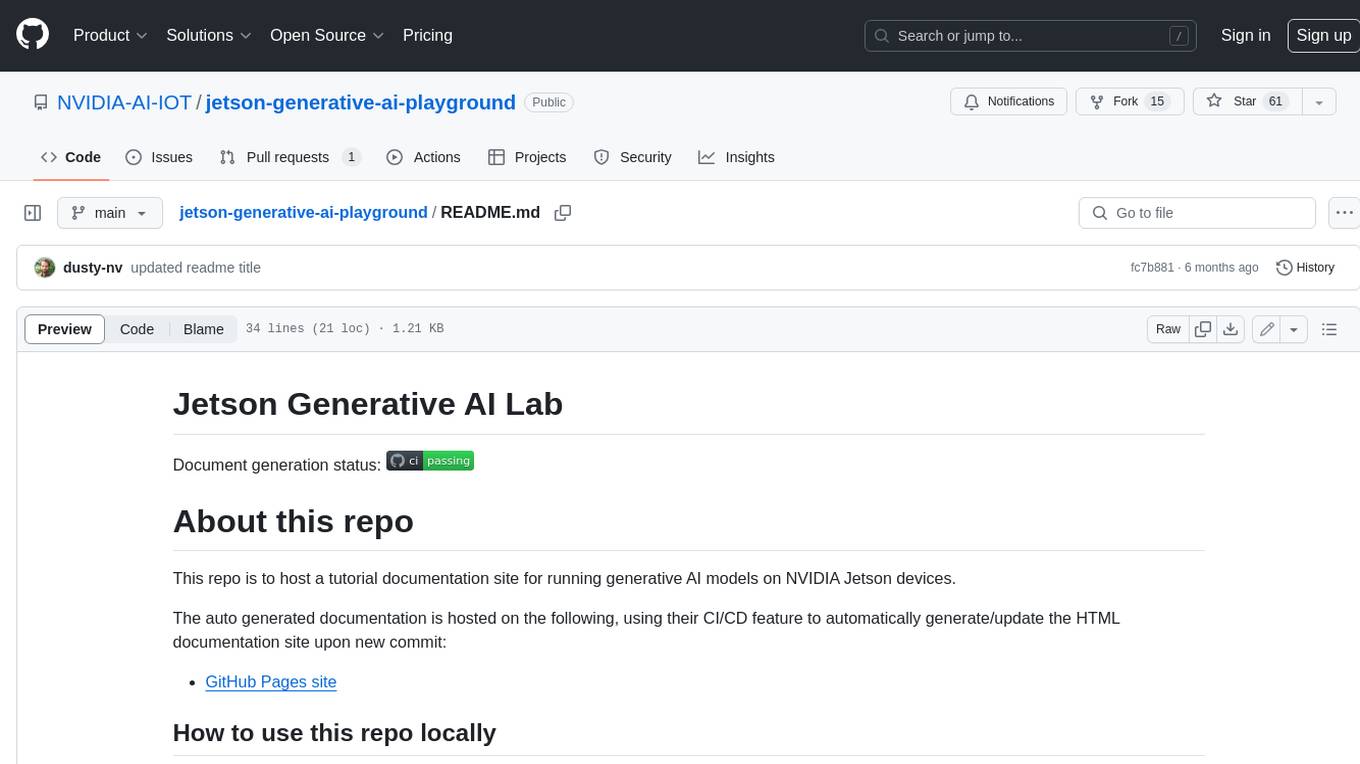
jetson-generative-ai-playground
This repo hosts tutorial documentation for running generative AI models on NVIDIA Jetson devices. The documentation is auto-generated and hosted on GitHub Pages using their CI/CD feature to automatically generate/update the HTML documentation site upon new commits.
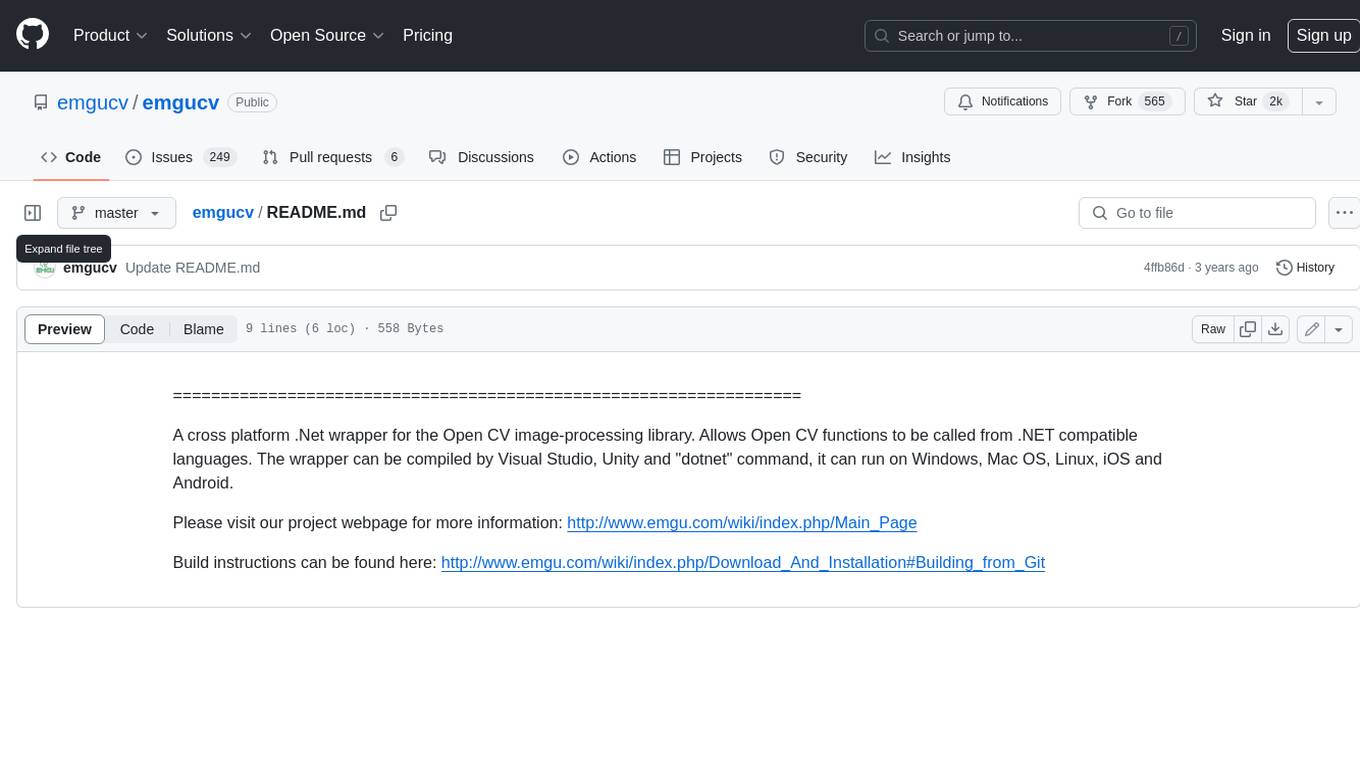
emgucv
Emgu CV is a cross-platform .Net wrapper for the OpenCV image-processing library. It allows OpenCV functions to be called from .NET compatible languages. The wrapper can be compiled by Visual Studio, Unity, and "dotnet" command, and it can run on Windows, Mac OS, Linux, iOS, and Android.
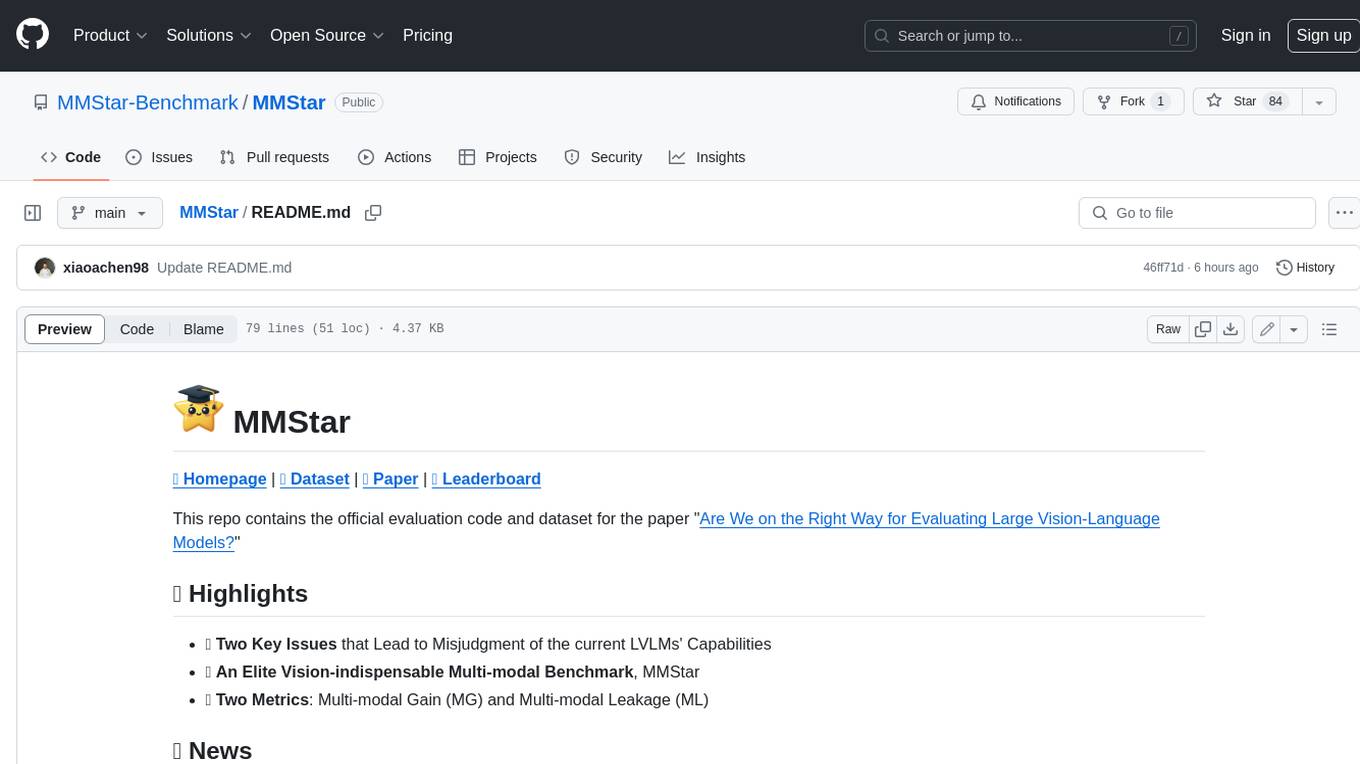
MMStar
MMStar is an elite vision-indispensable multi-modal benchmark comprising 1,500 challenge samples meticulously selected by humans. It addresses two key issues in current LLM evaluation: the unnecessary use of visual content in many samples and the existence of unintentional data leakage in LLM and LVLM training. MMStar evaluates 6 core capabilities across 18 detailed axes, ensuring a balanced distribution of samples across all dimensions.
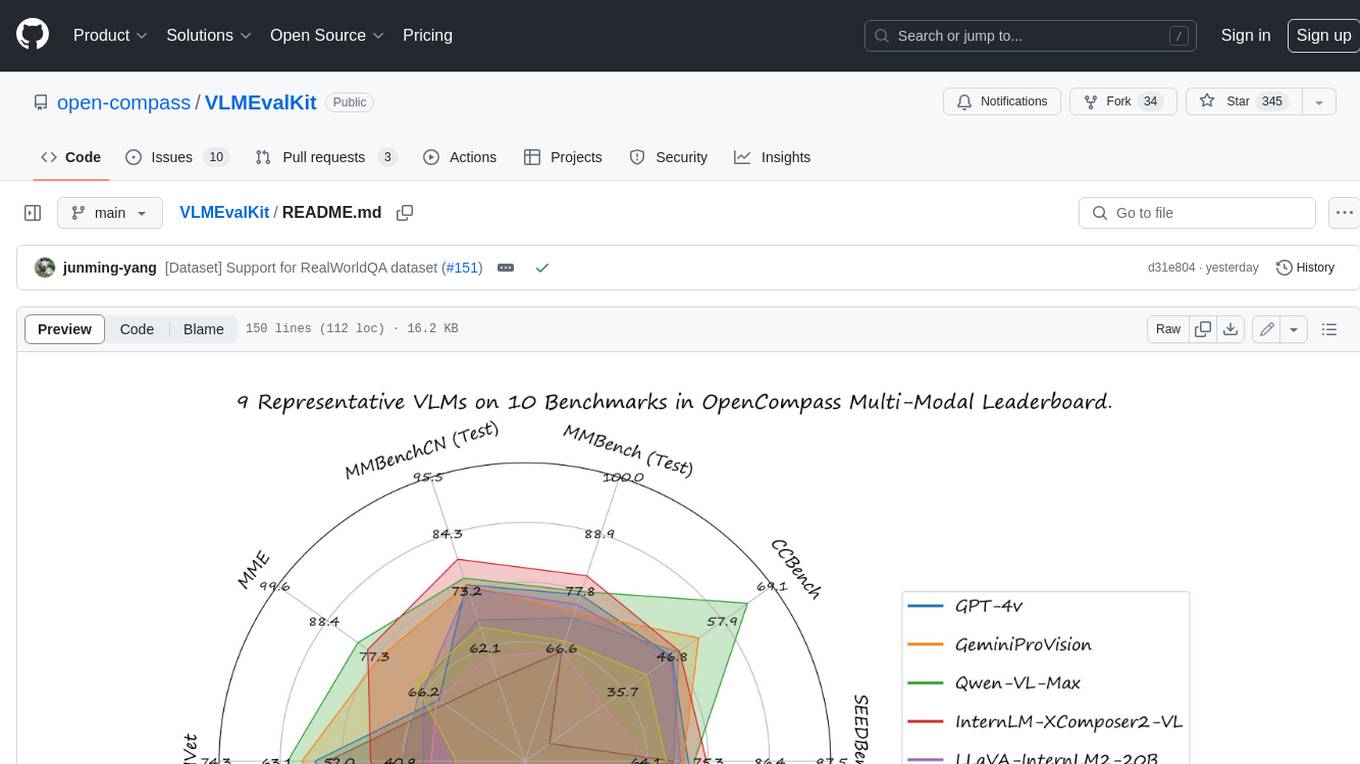
VLMEvalKit
VLMEvalKit is an open-source evaluation toolkit of large vision-language models (LVLMs). It enables one-command evaluation of LVLMs on various benchmarks, without the heavy workload of data preparation under multiple repositories. In VLMEvalKit, we adopt generation-based evaluation for all LVLMs, and provide the evaluation results obtained with both exact matching and LLM-based answer extraction.
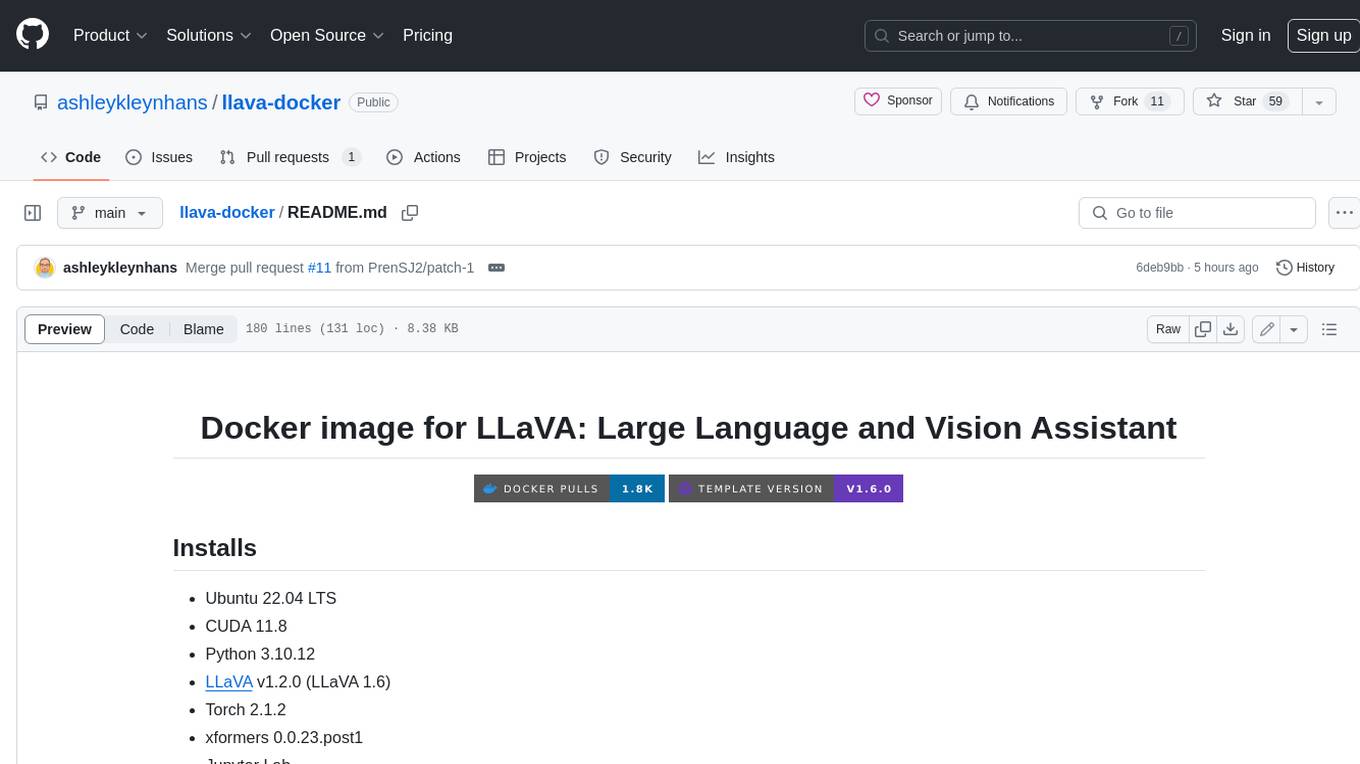
llava-docker
This Docker image for LLaVA (Large Language and Vision Assistant) provides a convenient way to run LLaVA locally or on RunPod. LLaVA is a powerful AI tool that combines natural language processing and computer vision capabilities. With this Docker image, you can easily access LLaVA's functionalities for various tasks, including image captioning, visual question answering, text summarization, and more. The image comes pre-installed with LLaVA v1.2.0, Torch 2.1.2, xformers 0.0.23.post1, and other necessary dependencies. You can customize the model used by setting the MODEL environment variable. The image also includes a Jupyter Lab environment for interactive development and exploration. Overall, this Docker image offers a comprehensive and user-friendly platform for leveraging LLaVA's capabilities.