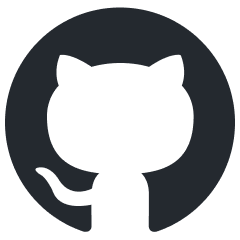
MMStar
This repo contains evaluation code for the paper "Are We on the Right Way for Evaluating Large Vision-Language Models"
Stars: 84
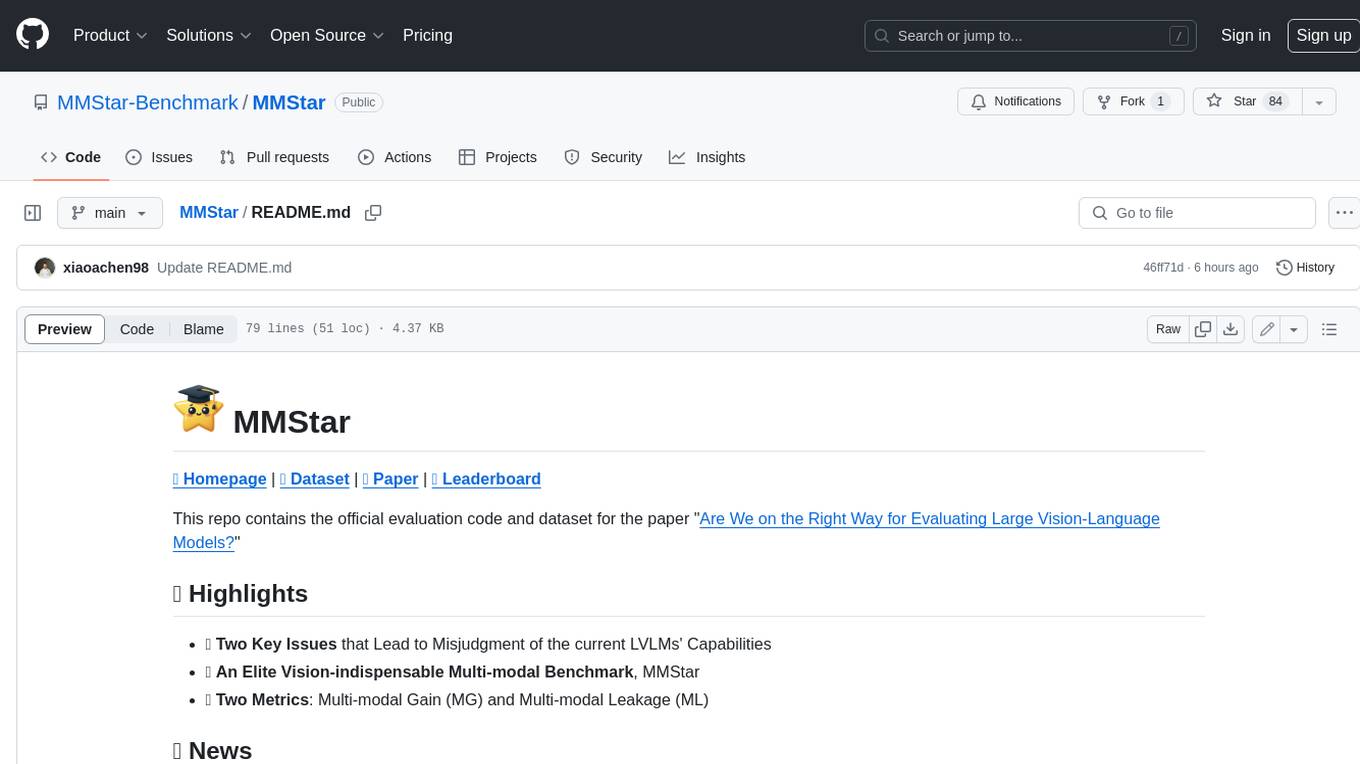
MMStar is an elite vision-indispensable multi-modal benchmark comprising 1,500 challenge samples meticulously selected by humans. It addresses two key issues in current LLM evaluation: the unnecessary use of visual content in many samples and the existence of unintentional data leakage in LLM and LVLM training. MMStar evaluates 6 core capabilities across 18 detailed axes, ensuring a balanced distribution of samples across all dimensions.
README:
π Homepage | π€ Dataset | π Paper | π Leaderboard
This repo contains the official evaluation code and dataset for the paper "Are We on the Right Way for Evaluating Large Vision-Language Models?"
- π₯ Two Key Issues that Lead to Misjudgment of the current LVLMs' Capabilities
- π₯ An Elite Vision-indispensable Multi-modal Benchmark, MMStar
- π₯ Two Metrics: Multi-modal Gain (MG) and Multi-modal Leakage (ML)
[2024.4.16] π MMStar has been supported in the VLMEvalKit repository and OpenCompass leaderboard.
[2024.4.2] π Huggingface Dataset and evaluation code are available!
[2024.4.1] π We released the ArXiv paper.
- [x] Evaluation code for MMStar
- [x] Support online Leaderboard
- [ ] Curate online test set, MMStar-test (This involves working with the existing multi-modal benchmarks containing protected test set, feel free to contact us!)
We dig into current evaluation works and identify two primary issues:
(1) Visual content is unnecessary for many samples.
(2) Unintentional data leakage exists in LLM and LVLM training.
Both problems lead to misjudgments of actual multi-modal performance gains and potentially misguide the study of LVLM. To this end, we present MMStar, an elite vision-indispensable multi-modal benchmark comprising 1,500 challenge samples meticulously selected by humans. After applying the coarse filter process and manual review, we narrow down from a total of 22,401 samples to 11,607 candidate samples and finally select 1,500 high-quality samples to construct our MMStar benchmark.
In MMStar, we display 6 core capabilities in the inner ring, with 18 detailed axes presented in the outer ring. The middle ring showcases the number of samples for each detailed dimension. Each core capability contains a meticulously balanced 250 samples. We further ensure a relatively even distribution across the 18 detailed axes.
You can evaluate any LLMs and LVLMs on our MMStar following with the evaluation guidelines.
π― The Leaderboard for MMStar is continuously being updated, welcoming the contribution of your LVLMs!
Please note that to thoroughly evaluate your own LVLM, you are required to provide us with three result files in xlsx format. These should include the results of your LVLM with visual input, the results of your LVLM without visual input, and the results of your original LLM base without visual input. We have provided a submission format in the submits
folder. After completing the aforementioned steps, please contact us via [email protected] to submit your results and to update the leaderboard.
If you find our work helpful for your research, please consider giving a star β and citation π
@article{chen2024we,
title={Are We on the Right Way for Evaluating Large Vision-Language Models?},
author={Chen, Lin and Li, Jinsong and Dong, Xiaoyi and Zhang, Pan and Zang, Yuhang and Chen, Zehui and Duan, Haodong and Wang, Jiaqi and Qiao, Yu and Lin, Dahua and others},
journal={arXiv preprint arXiv:2403.20330},
year={2024}
}
For Tasks:
Click tags to check more tools for each tasksFor Jobs:
Alternative AI tools for MMStar
Similar Open Source Tools
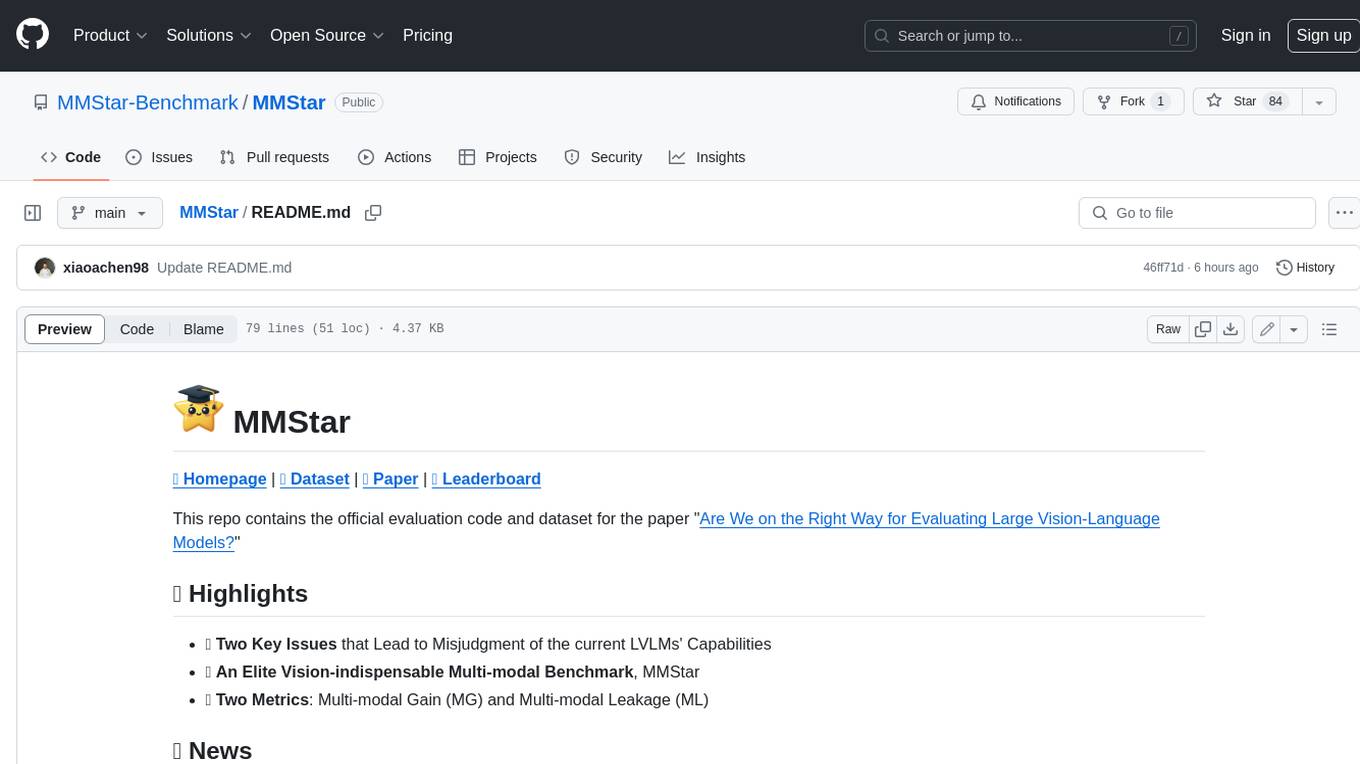
MMStar
MMStar is an elite vision-indispensable multi-modal benchmark comprising 1,500 challenge samples meticulously selected by humans. It addresses two key issues in current LLM evaluation: the unnecessary use of visual content in many samples and the existence of unintentional data leakage in LLM and LVLM training. MMStar evaluates 6 core capabilities across 18 detailed axes, ensuring a balanced distribution of samples across all dimensions.
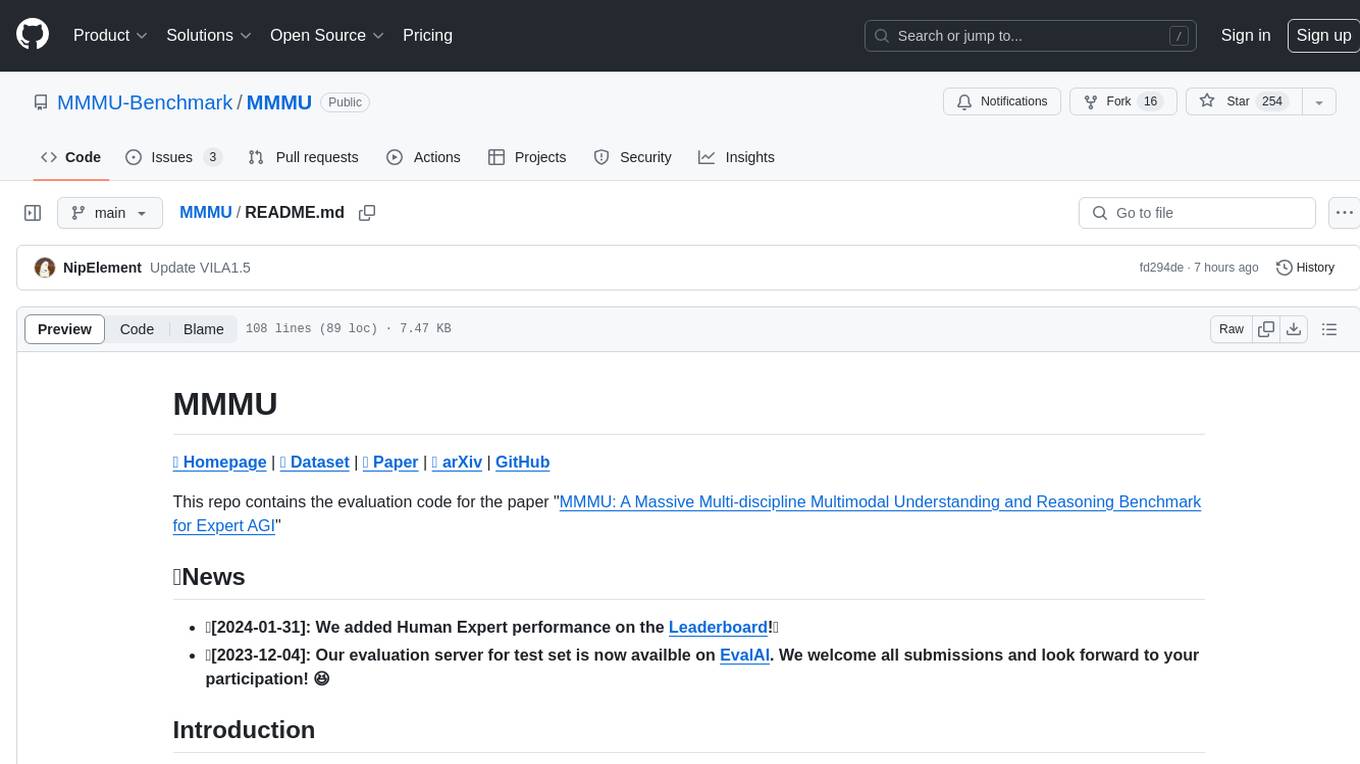
MMMU
MMMU is a benchmark designed to evaluate multimodal models on college-level subject knowledge tasks, covering 30 subjects and 183 subfields with 11.5K questions. It focuses on advanced perception and reasoning with domain-specific knowledge, challenging models to perform tasks akin to those faced by experts. The evaluation of various models highlights substantial challenges, with room for improvement to stimulate the community towards expert artificial general intelligence (AGI).
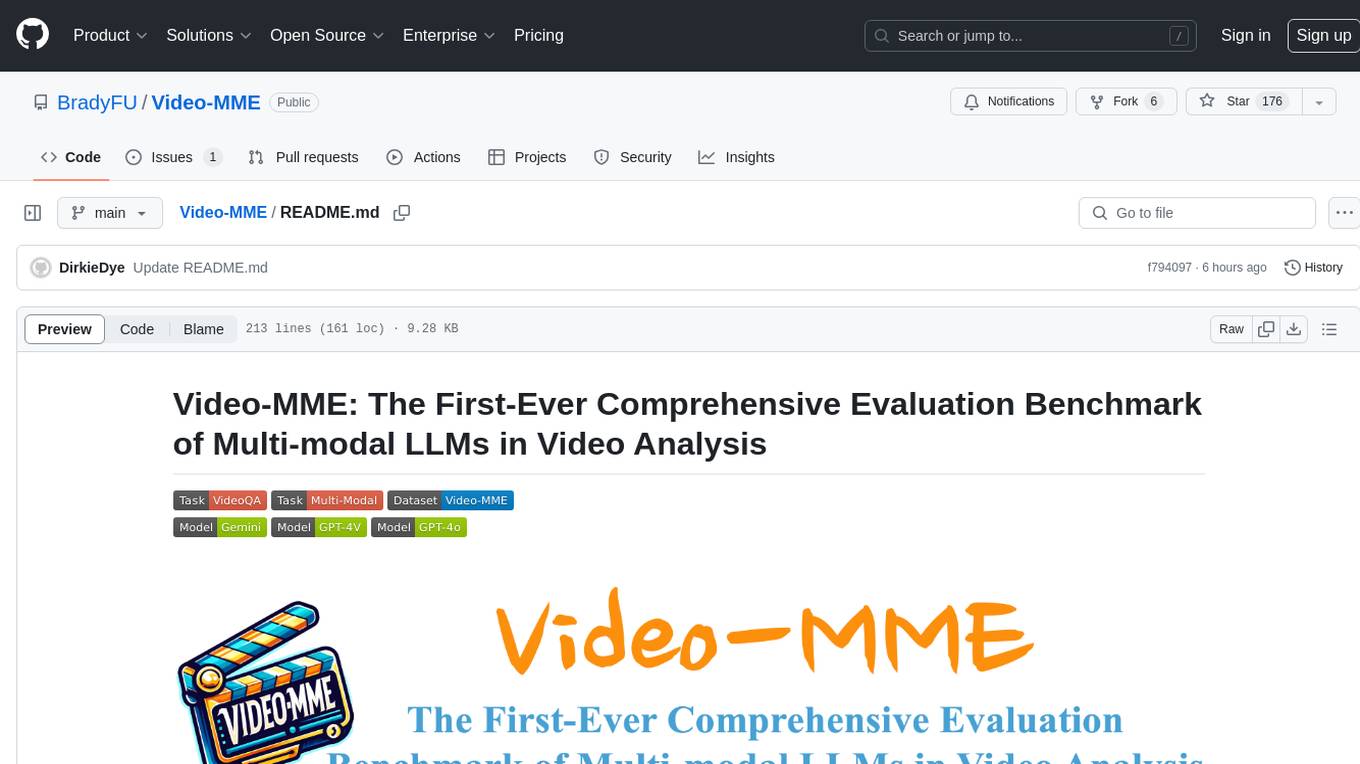
Video-MME
Video-MME is the first-ever comprehensive evaluation benchmark of Multi-modal Large Language Models (MLLMs) in Video Analysis. It assesses the capabilities of MLLMs in processing video data, covering a wide range of visual domains, temporal durations, and data modalities. The dataset comprises 900 videos with 256 hours and 2,700 human-annotated question-answer pairs. It distinguishes itself through features like duration variety, diversity in video types, breadth in data modalities, and quality in annotations.
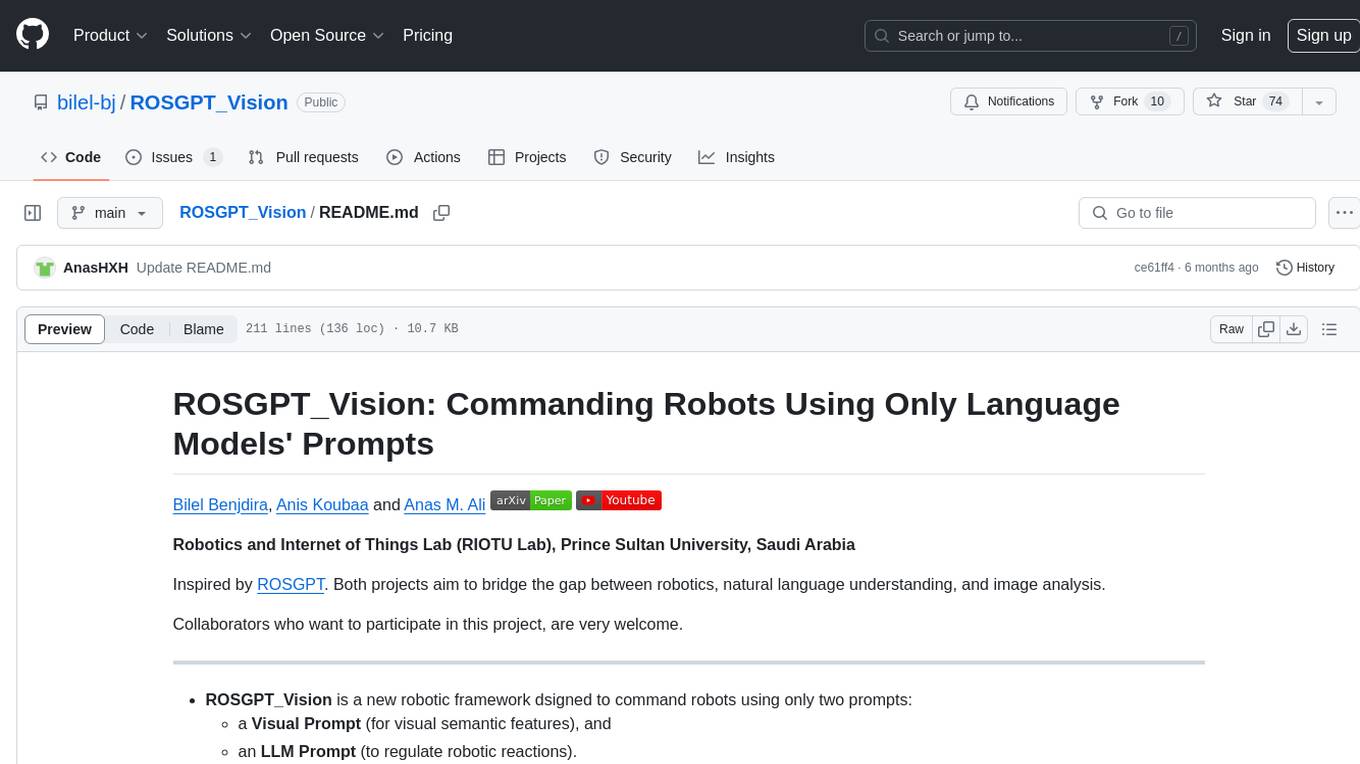
ROSGPT_Vision
ROSGPT_Vision is a new robotic framework designed to command robots using only two prompts: a Visual Prompt for visual semantic features and an LLM Prompt to regulate robotic reactions. It is based on the Prompting Robotic Modalities (PRM) design pattern and is used to develop CarMate, a robotic application for monitoring driver distractions and providing real-time vocal notifications. The framework leverages state-of-the-art language models to facilitate advanced reasoning about image data and offers a unified platform for robots to perceive, interpret, and interact with visual data through natural language. LangChain is used for easy customization of prompts, and the implementation includes the CarMate application for driver monitoring and assistance.
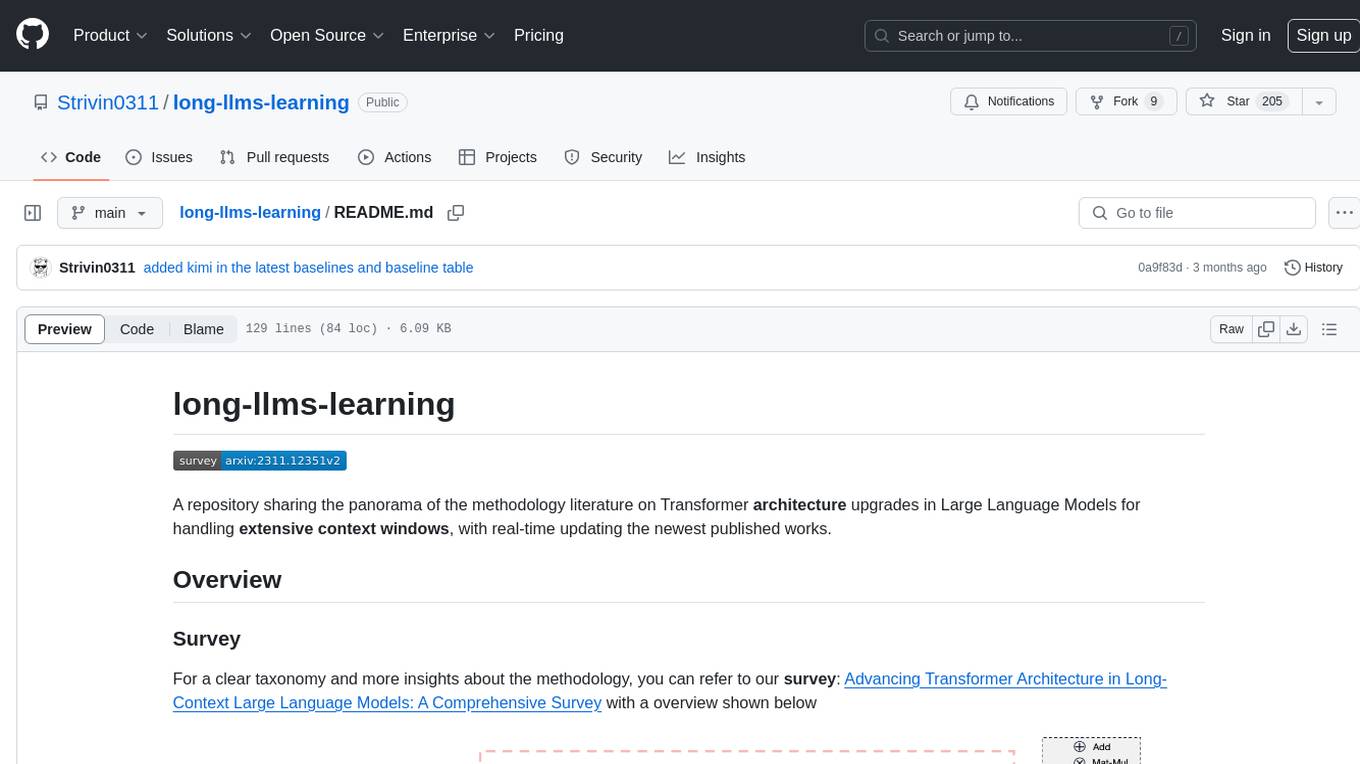
long-llms-learning
A repository sharing the panorama of the methodology literature on Transformer architecture upgrades in Large Language Models for handling extensive context windows, with real-time updating the newest published works. It includes a survey on advancing Transformer architecture in long-context large language models, flash-ReRoPE implementation, latest news on data engineering, lightning attention, Kimi AI assistant, chatglm-6b-128k, gpt-4-turbo-preview, benchmarks like InfiniteBench and LongBench, long-LLMs-evals for evaluating methods for enhancing long-context capabilities, and LLMs-learning for learning technologies and applicated tasks about Large Language Models.
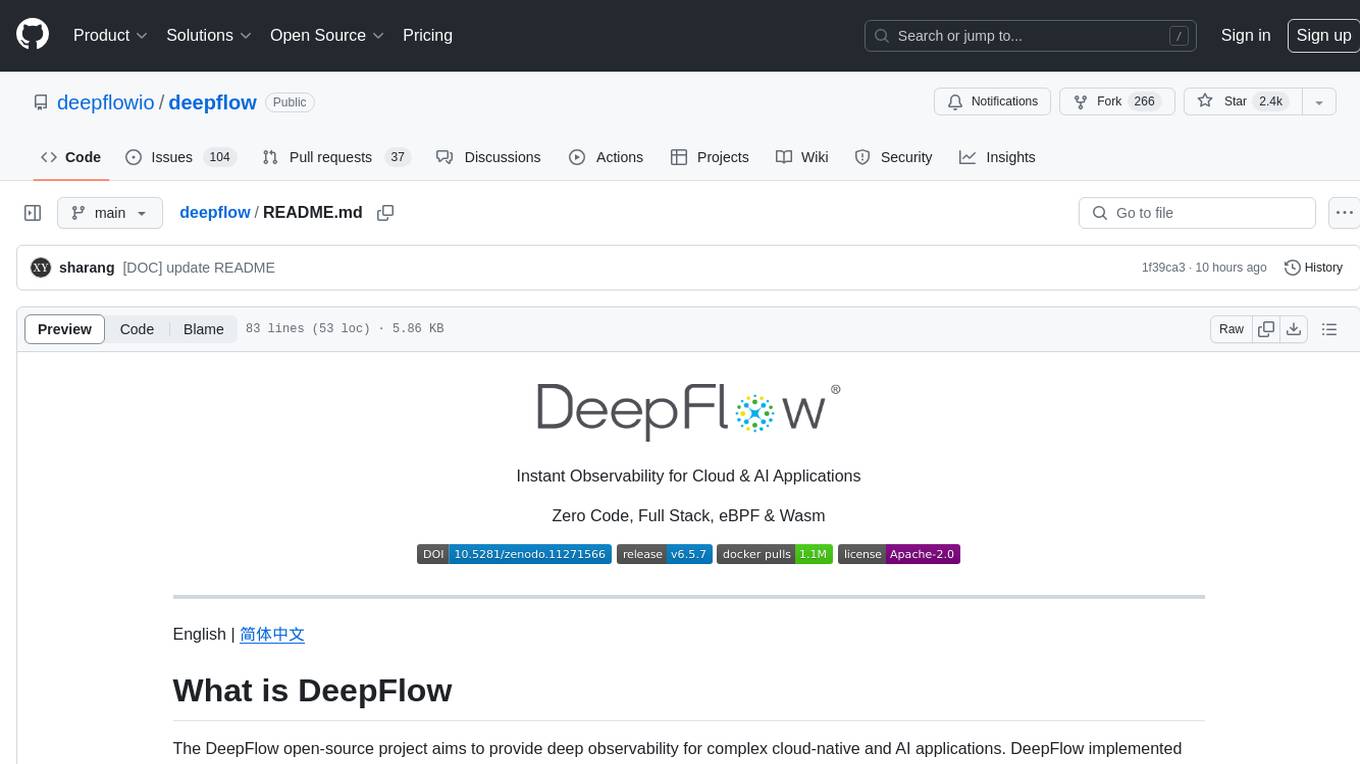
deepflow
DeepFlow is an open-source project that provides deep observability for complex cloud-native and AI applications. It offers Zero Code data collection with eBPF for metrics, distributed tracing, request logs, and function profiling. DeepFlow is integrated with SmartEncoding to achieve Full Stack correlation and efficient access to all observability data. With DeepFlow, cloud-native and AI applications automatically gain deep observability, removing the burden of developers continually instrumenting code and providing monitoring and diagnostic capabilities covering everything from code to infrastructure for DevOps/SRE teams.
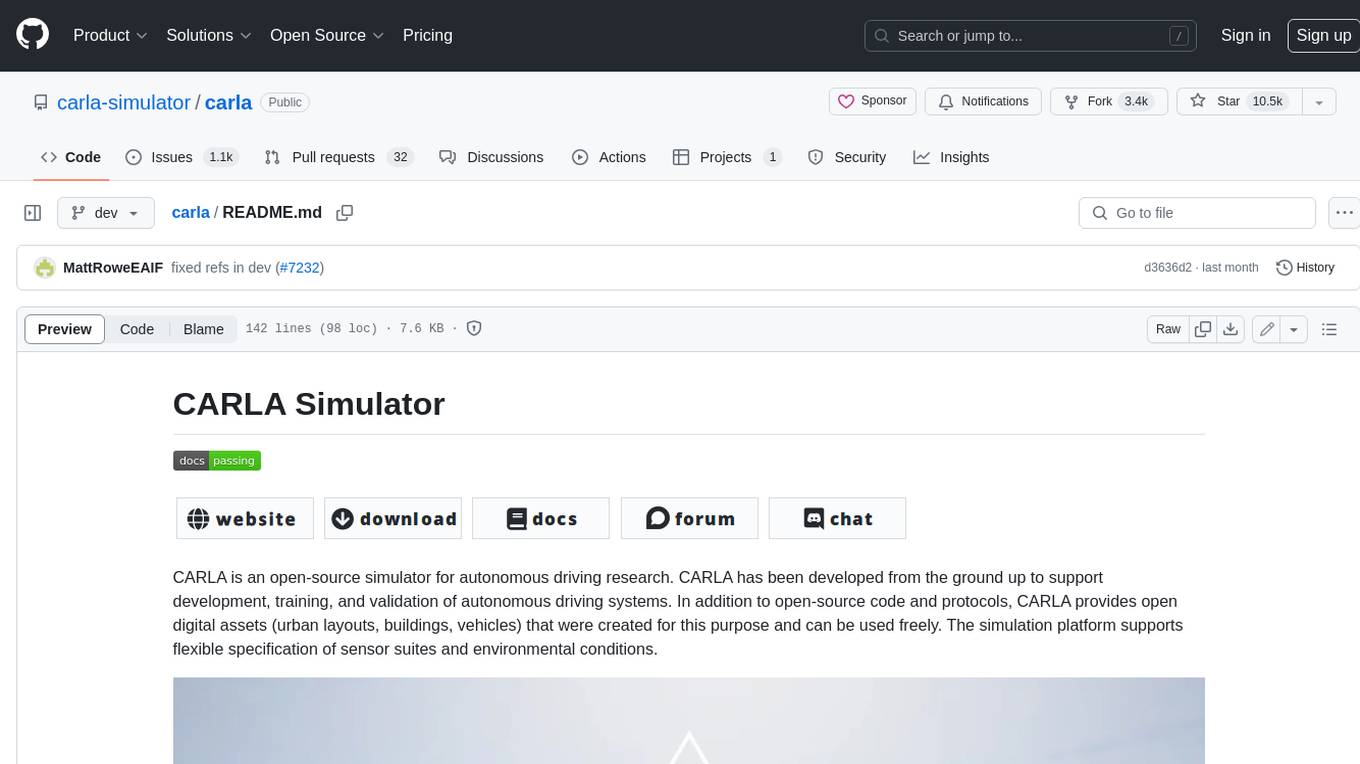
carla
CARLA is an open-source simulator for autonomous driving research. It provides open-source code, protocols, and digital assets (urban layouts, buildings, vehicles) for developing, training, and validating autonomous driving systems. CARLA supports flexible specification of sensor suites and environmental conditions.
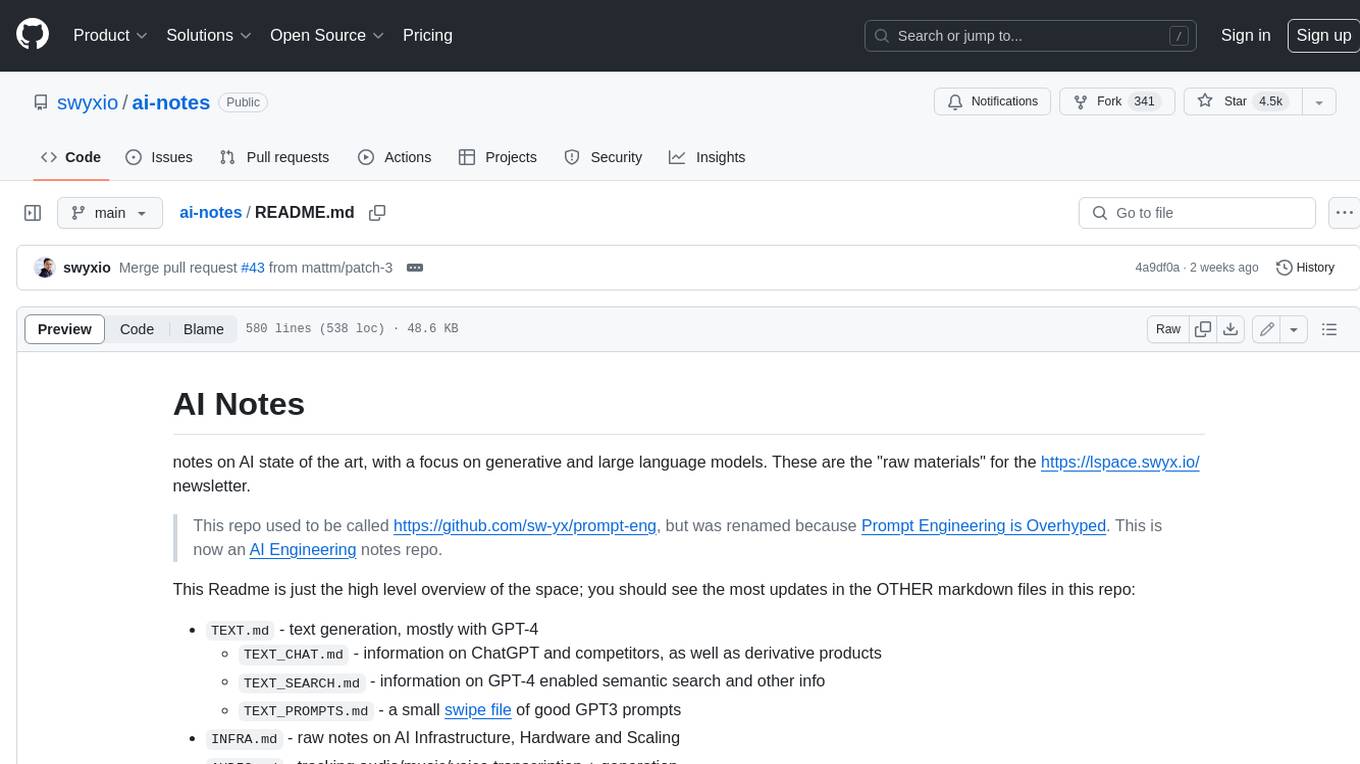
ai-notes
Notes on AI state of the art, with a focus on generative and large language models. These are the "raw materials" for the https://lspace.swyx.io/ newsletter. This repo used to be called https://github.com/sw-yx/prompt-eng, but was renamed because Prompt Engineering is Overhyped. This is now an AI Engineering notes repo.
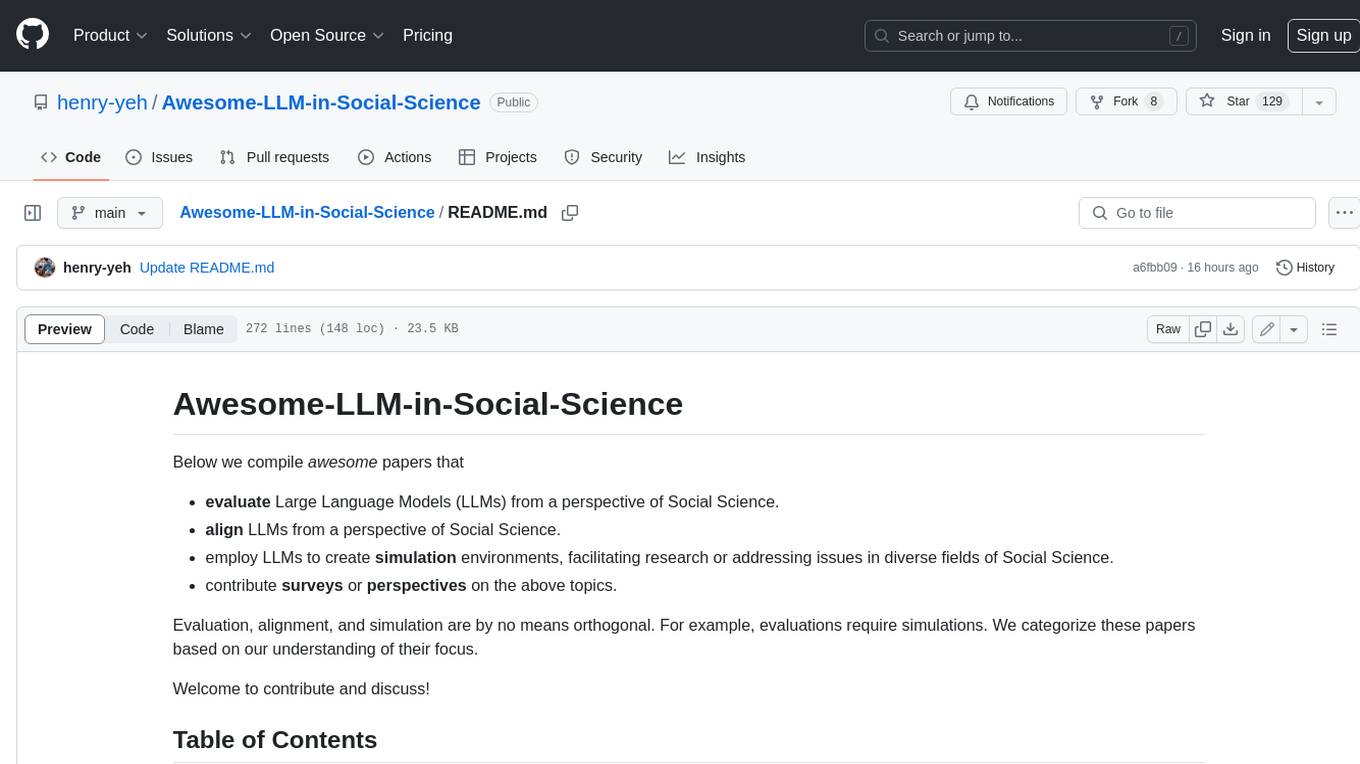
Awesome-LLM-in-Social-Science
This repository compiles a list of academic papers that evaluate, align, simulate, and provide surveys or perspectives on the use of Large Language Models (LLMs) in the field of Social Science. The papers cover various aspects of LLM research, including assessing their alignment with human values, evaluating their capabilities in tasks such as opinion formation and moral reasoning, and exploring their potential for simulating social interactions and addressing issues in diverse fields of Social Science. The repository aims to provide a comprehensive resource for researchers and practitioners interested in the intersection of LLMs and Social Science.
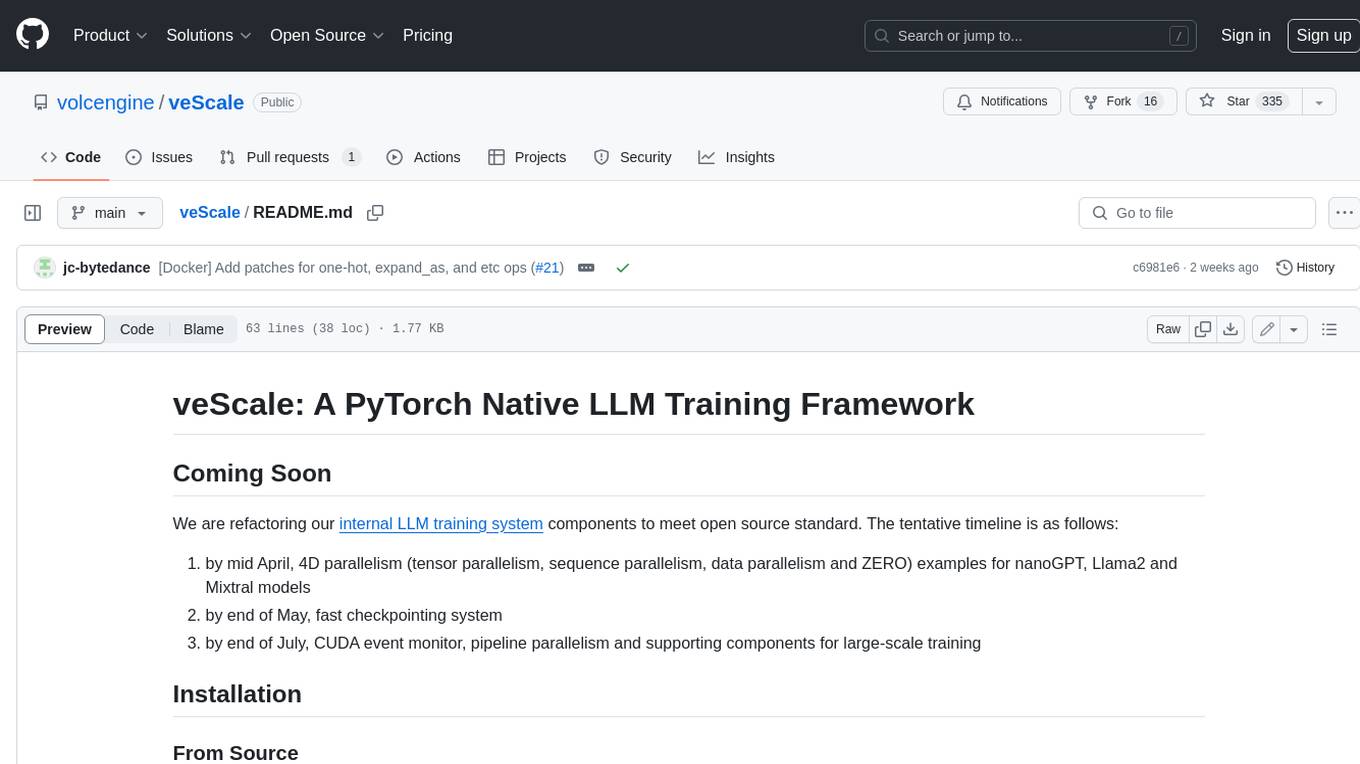
veScale
veScale is a PyTorch Native LLM Training Framework. It provides a set of tools and components to facilitate the training of large language models (LLMs) using PyTorch. veScale includes features such as 4D parallelism, fast checkpointing, and a CUDA event monitor. It is designed to be scalable and efficient, and it can be used to train LLMs on a variety of hardware platforms.
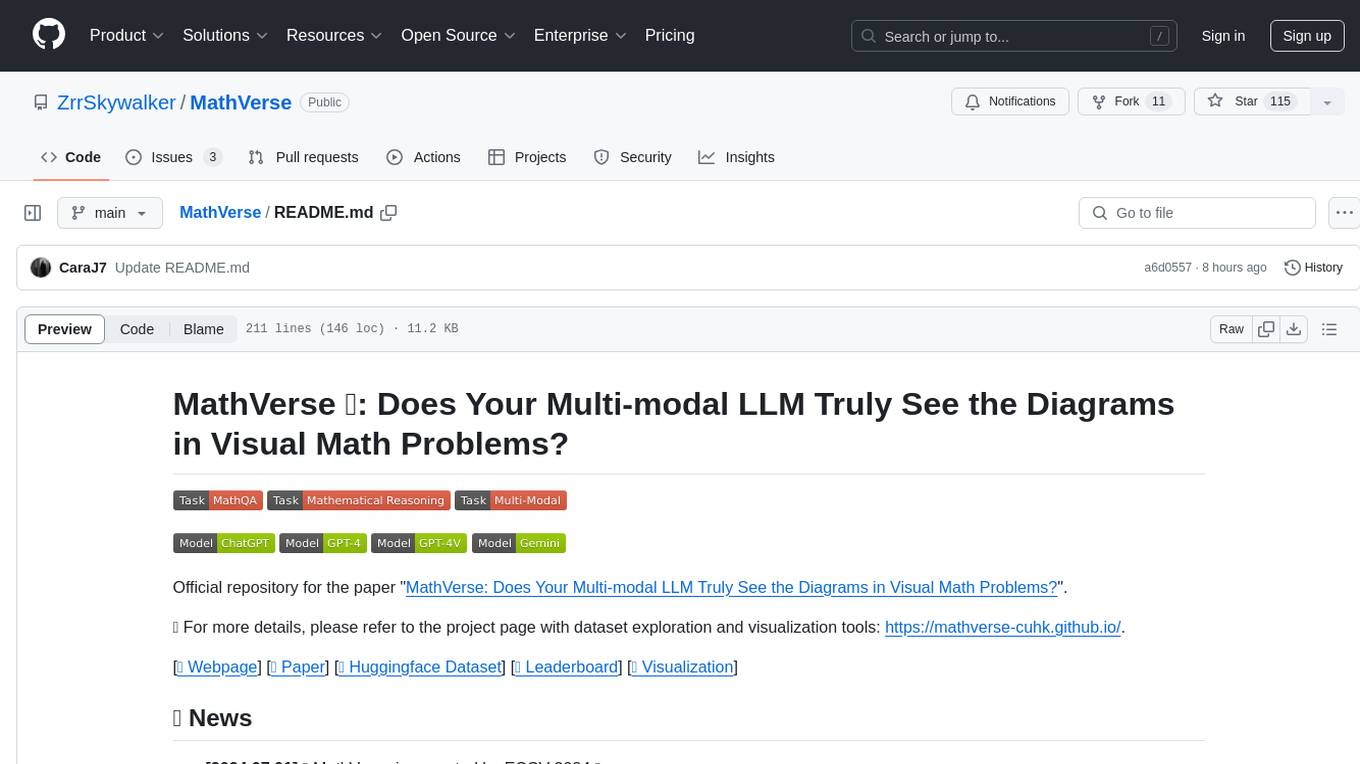
MathVerse
MathVerse is an all-around visual math benchmark designed to evaluate the capabilities of Multi-modal Large Language Models (MLLMs) in visual math problem-solving. It collects high-quality math problems with diagrams to assess how well MLLMs can understand visual diagrams for mathematical reasoning. The benchmark includes 2,612 problems transformed into six versions each, contributing to 15K test samples. It also introduces a Chain-of-Thought (CoT) Evaluation strategy for fine-grained assessment of output answers.
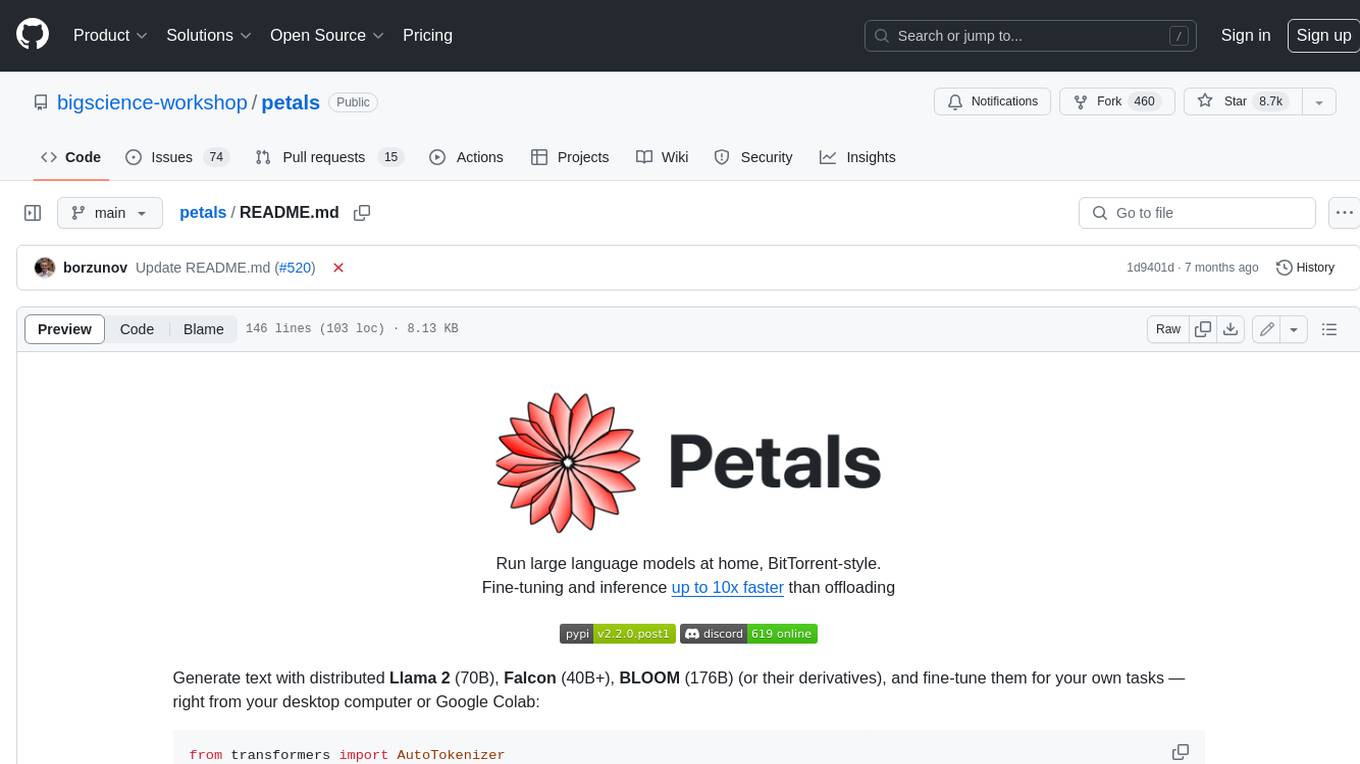
petals
Petals is a tool that allows users to run large language models at home in a BitTorrent-style manner. It enables fine-tuning and inference up to 10x faster than offloading. Users can generate text with distributed models like Llama 2, Falcon, and BLOOM, and fine-tune them for specific tasks directly from their desktop computer or Google Colab. Petals is a community-run system that relies on people sharing their GPUs to increase its capacity and offer a distributed network for hosting model layers.
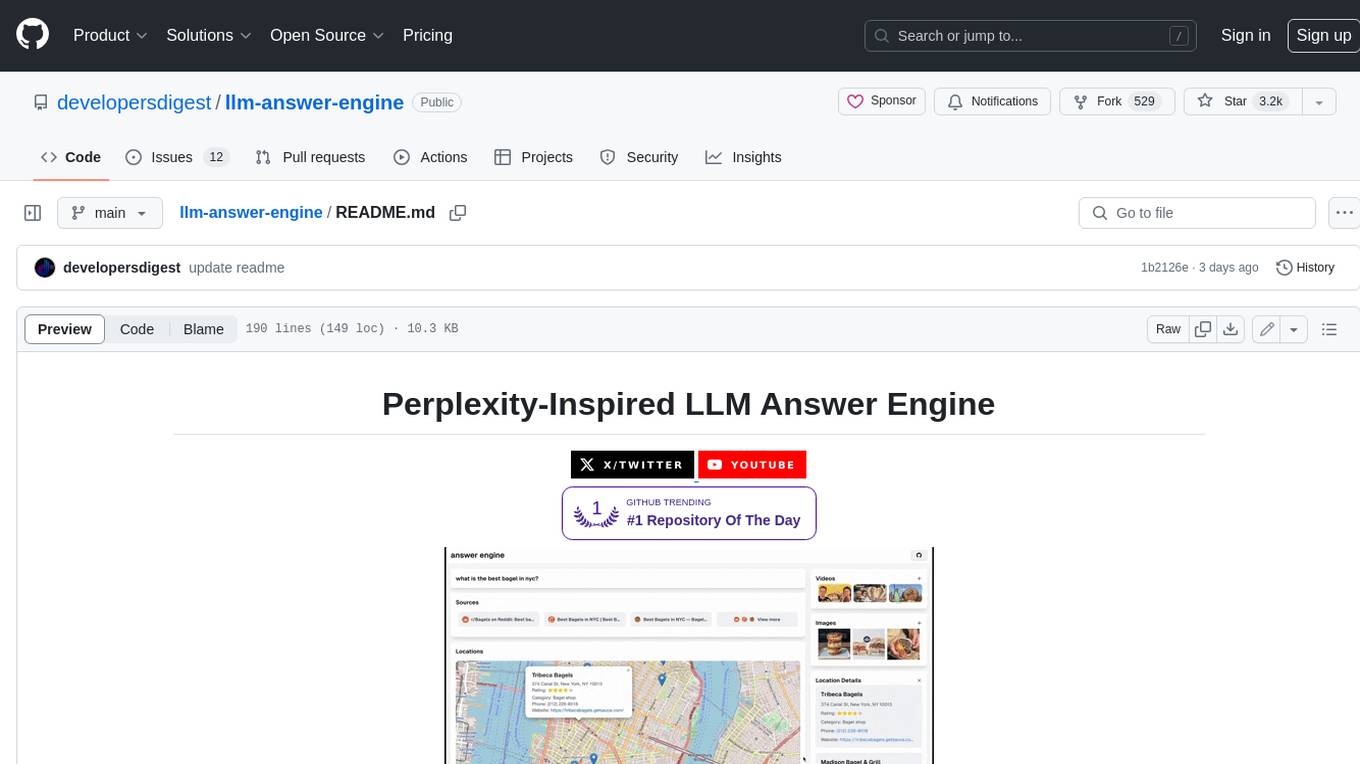
llm-answer-engine
This repository contains the code and instructions needed to build a sophisticated answer engine that leverages the capabilities of Groq, Mistral AI's Mixtral, Langchain.JS, Brave Search, Serper API, and OpenAI. Designed to efficiently return sources, answers, images, videos, and follow-up questions based on user queries, this project is an ideal starting point for developers interested in natural language processing and search technologies.
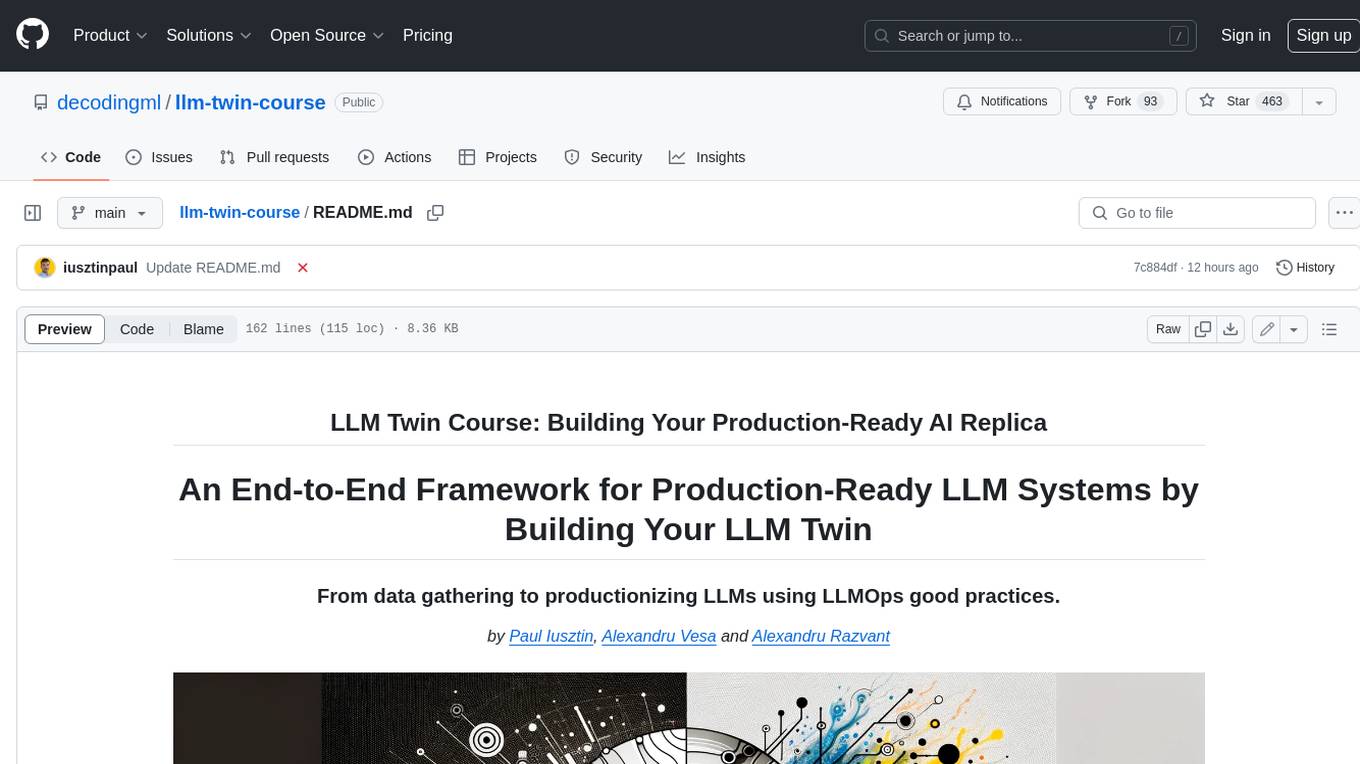
llm-twin-course
The LLM Twin Course is a free, end-to-end framework for building production-ready LLM systems. It teaches you how to design, train, and deploy a production-ready LLM twin of yourself powered by LLMs, vector DBs, and LLMOps good practices. The course is split into 11 hands-on written lessons and the open-source code you can access on GitHub. You can read everything and try out the code at your own pace.
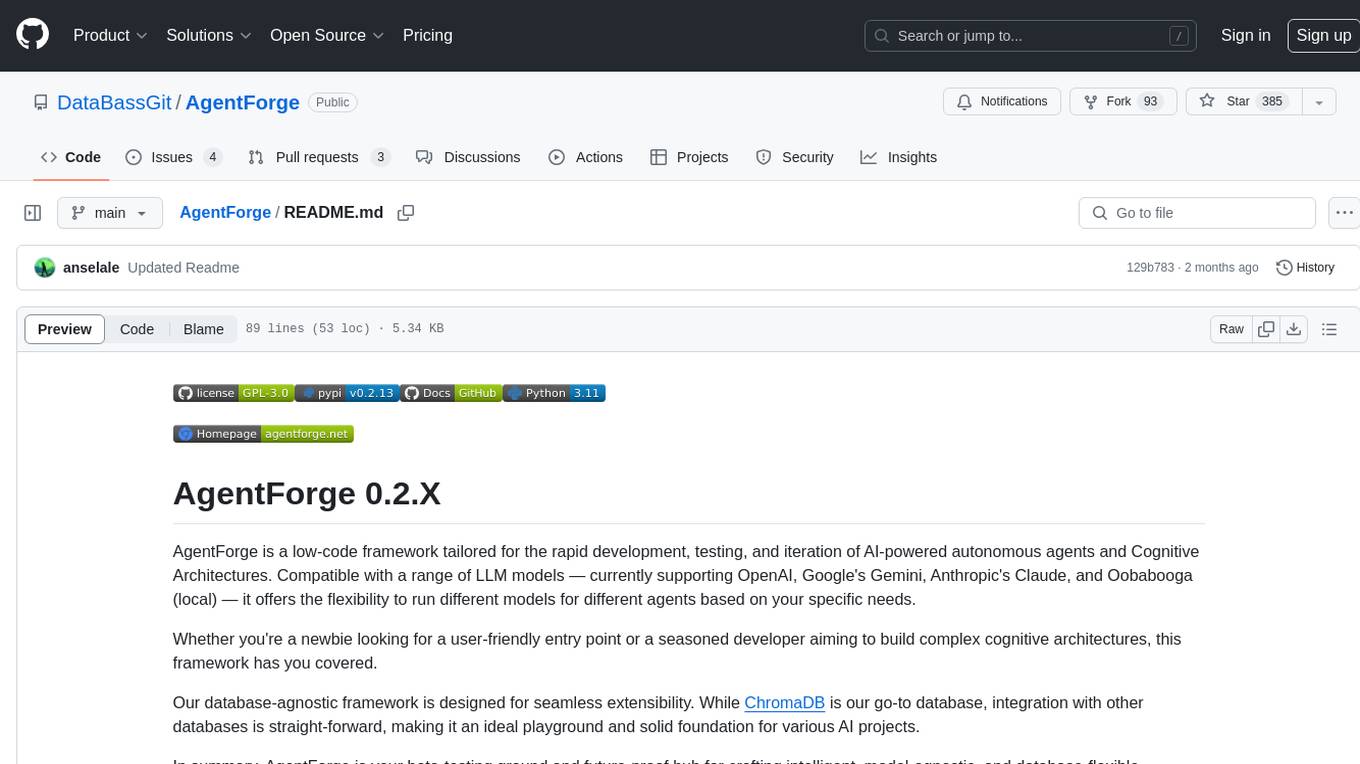
AgentForge
AgentForge is a low-code framework tailored for the rapid development, testing, and iteration of AI-powered autonomous agents and Cognitive Architectures. It is compatible with a range of LLM models and offers flexibility to run different models for different agents based on specific needs. The framework is designed for seamless extensibility and database-flexibility, making it an ideal playground for various AI projects. AgentForge is a beta-testing ground and future-proof hub for crafting intelligent, model-agnostic autonomous agents.
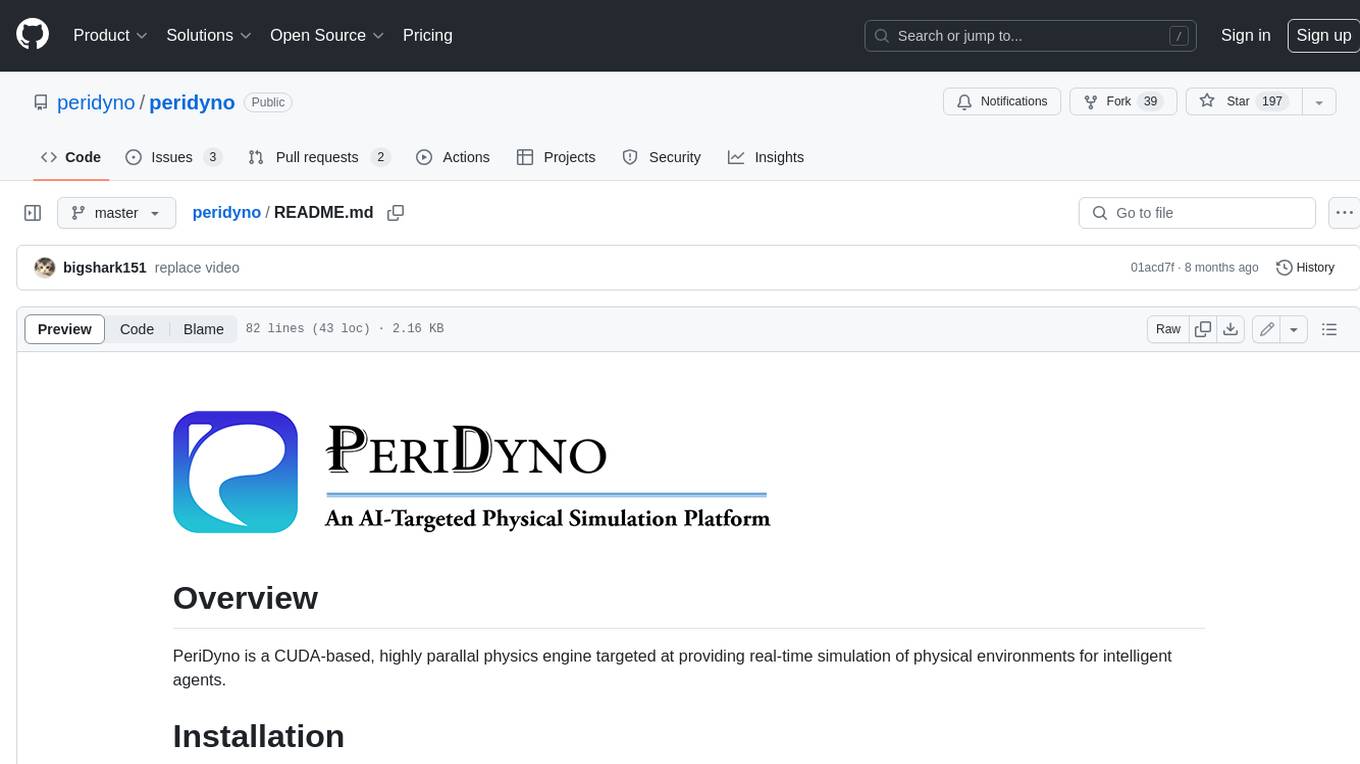
peridyno
PeriDyno is a CUDA-based, highly parallel physics engine targeted at providing real-time simulation of physical environments for intelligent agents. It is designed to be easy to use and integrate into existing projects, and it provides a wide range of features for simulating a variety of physical phenomena. PeriDyno is open source and available under the Apache 2.0 license.
For similar tasks
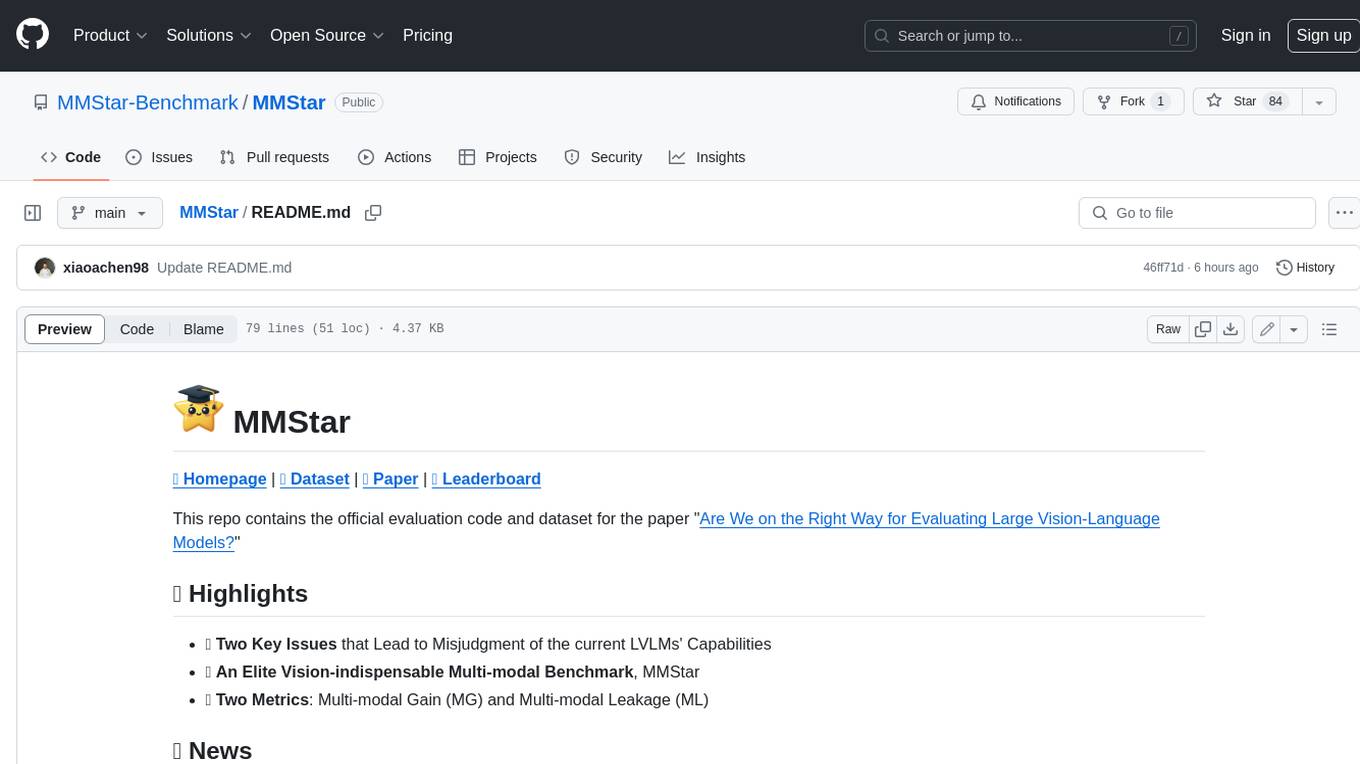
MMStar
MMStar is an elite vision-indispensable multi-modal benchmark comprising 1,500 challenge samples meticulously selected by humans. It addresses two key issues in current LLM evaluation: the unnecessary use of visual content in many samples and the existence of unintentional data leakage in LLM and LVLM training. MMStar evaluates 6 core capabilities across 18 detailed axes, ensuring a balanced distribution of samples across all dimensions.
For similar jobs
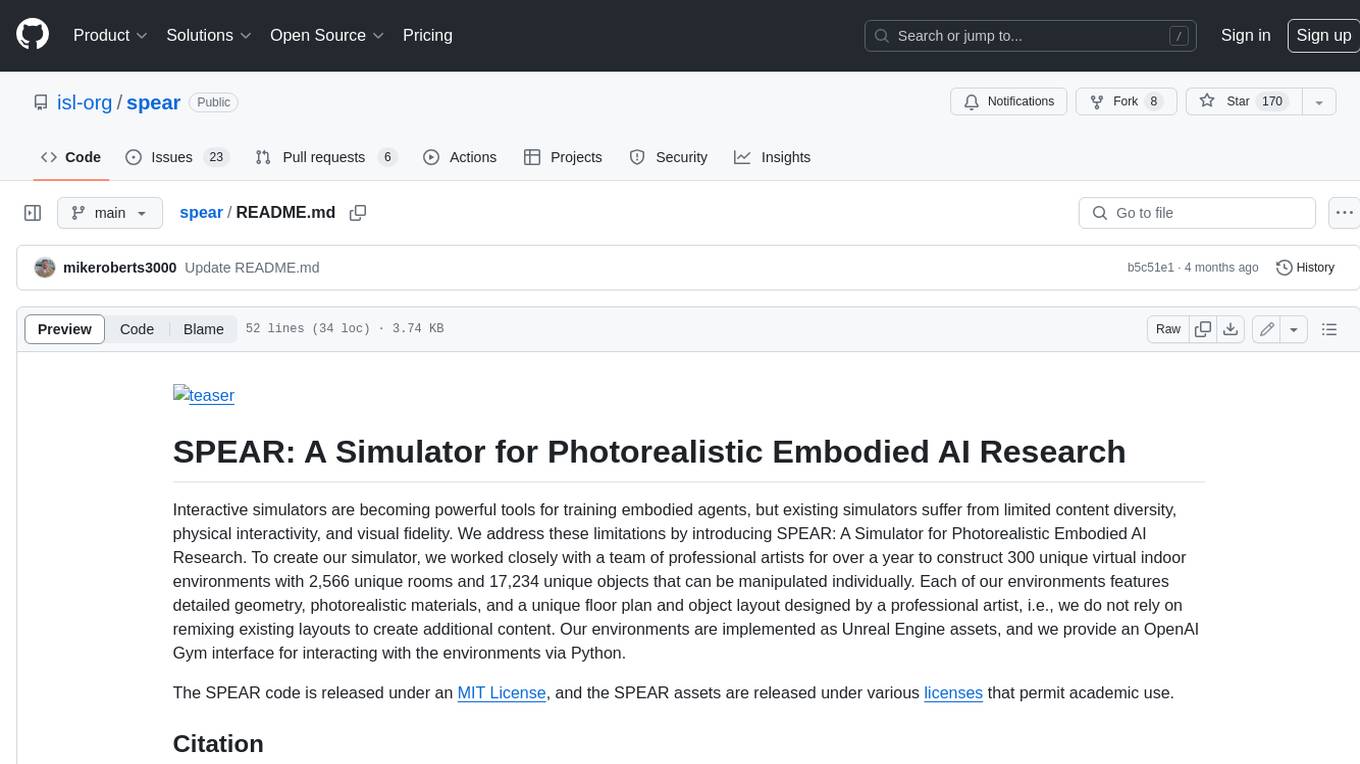
spear
SPEAR (Simulator for Photorealistic Embodied AI Research) is a powerful tool for training embodied agents. It features 300 unique virtual indoor environments with 2,566 unique rooms and 17,234 unique objects that can be manipulated individually. Each environment is designed by a professional artist and features detailed geometry, photorealistic materials, and a unique floor plan and object layout. SPEAR is implemented as Unreal Engine assets and provides an OpenAI Gym interface for interacting with the environments via Python.
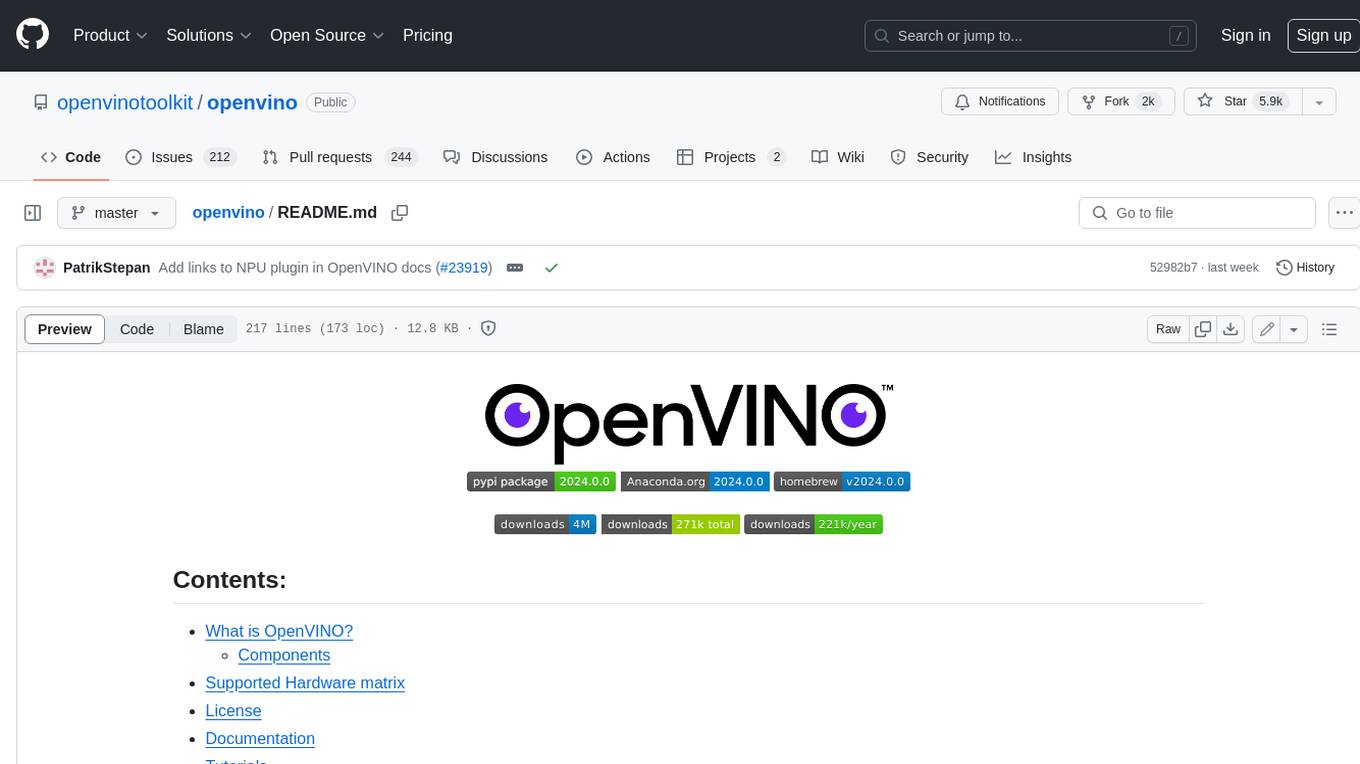
openvino
OpenVINOβ’ is an open-source toolkit for optimizing and deploying AI inference. It provides a common API to deliver inference solutions on various platforms, including CPU, GPU, NPU, and heterogeneous devices. OpenVINOβ’ supports pre-trained models from Open Model Zoo and popular frameworks like TensorFlow, PyTorch, and ONNX. Key components of OpenVINOβ’ include the OpenVINOβ’ Runtime, plugins for different hardware devices, frontends for reading models from native framework formats, and the OpenVINO Model Converter (OVC) for adjusting models for optimal execution on target devices.
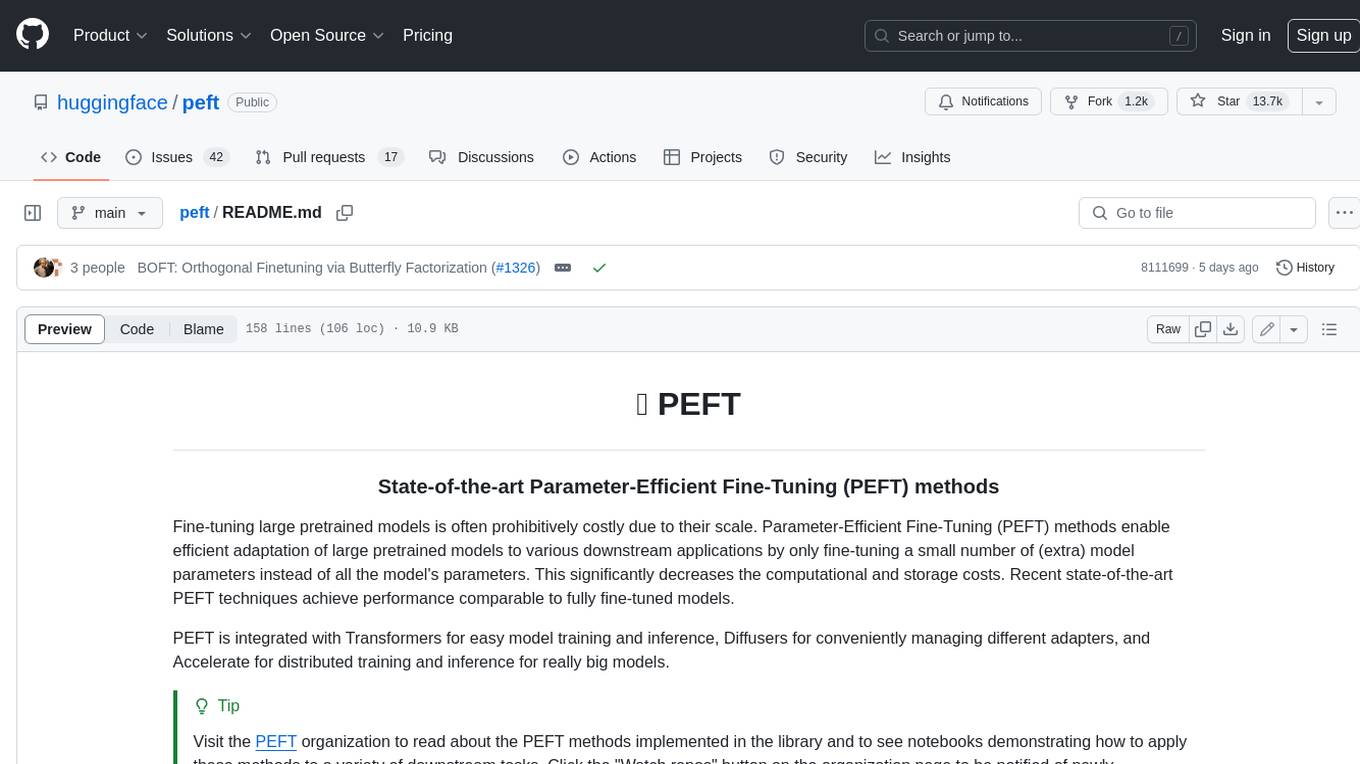
peft
PEFT (Parameter-Efficient Fine-Tuning) is a collection of state-of-the-art methods that enable efficient adaptation of large pretrained models to various downstream applications. By only fine-tuning a small number of extra model parameters instead of all the model's parameters, PEFT significantly decreases the computational and storage costs while achieving performance comparable to fully fine-tuned models.
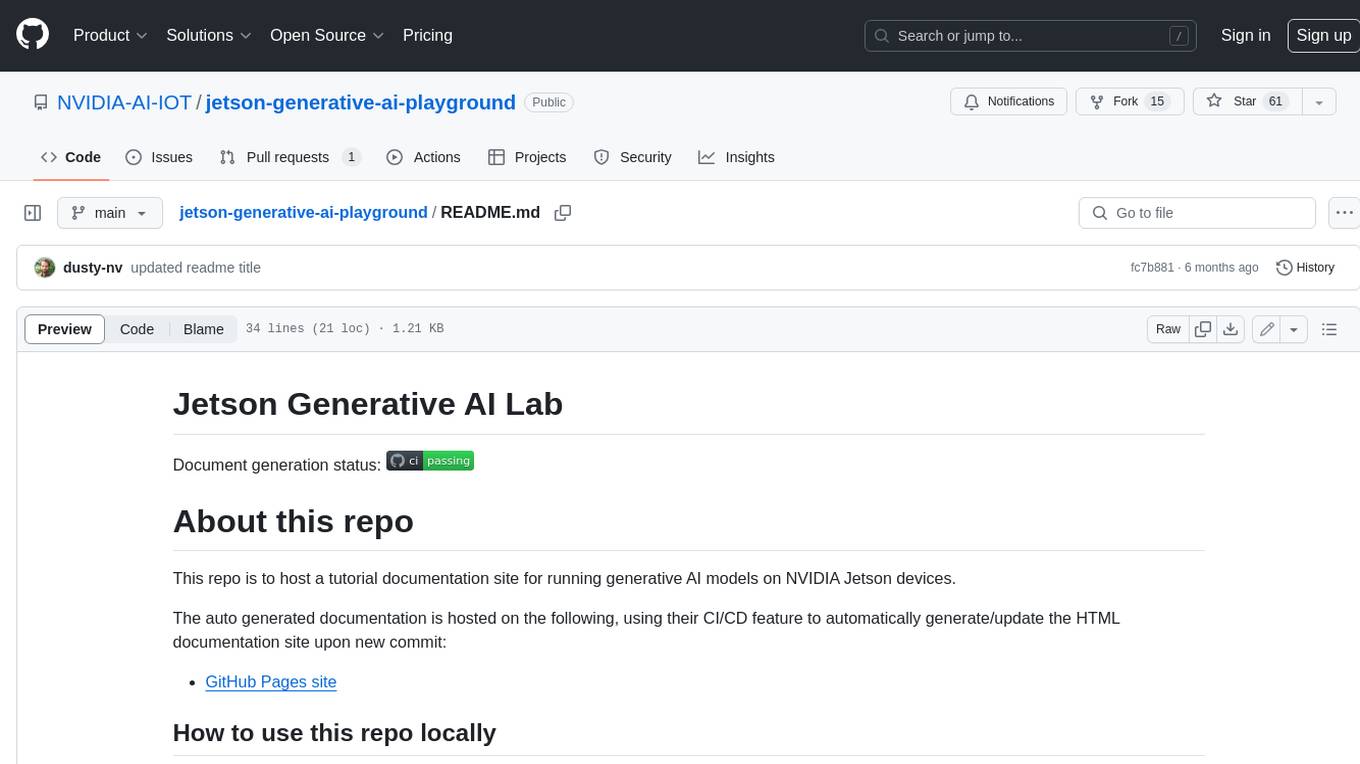
jetson-generative-ai-playground
This repo hosts tutorial documentation for running generative AI models on NVIDIA Jetson devices. The documentation is auto-generated and hosted on GitHub Pages using their CI/CD feature to automatically generate/update the HTML documentation site upon new commits.
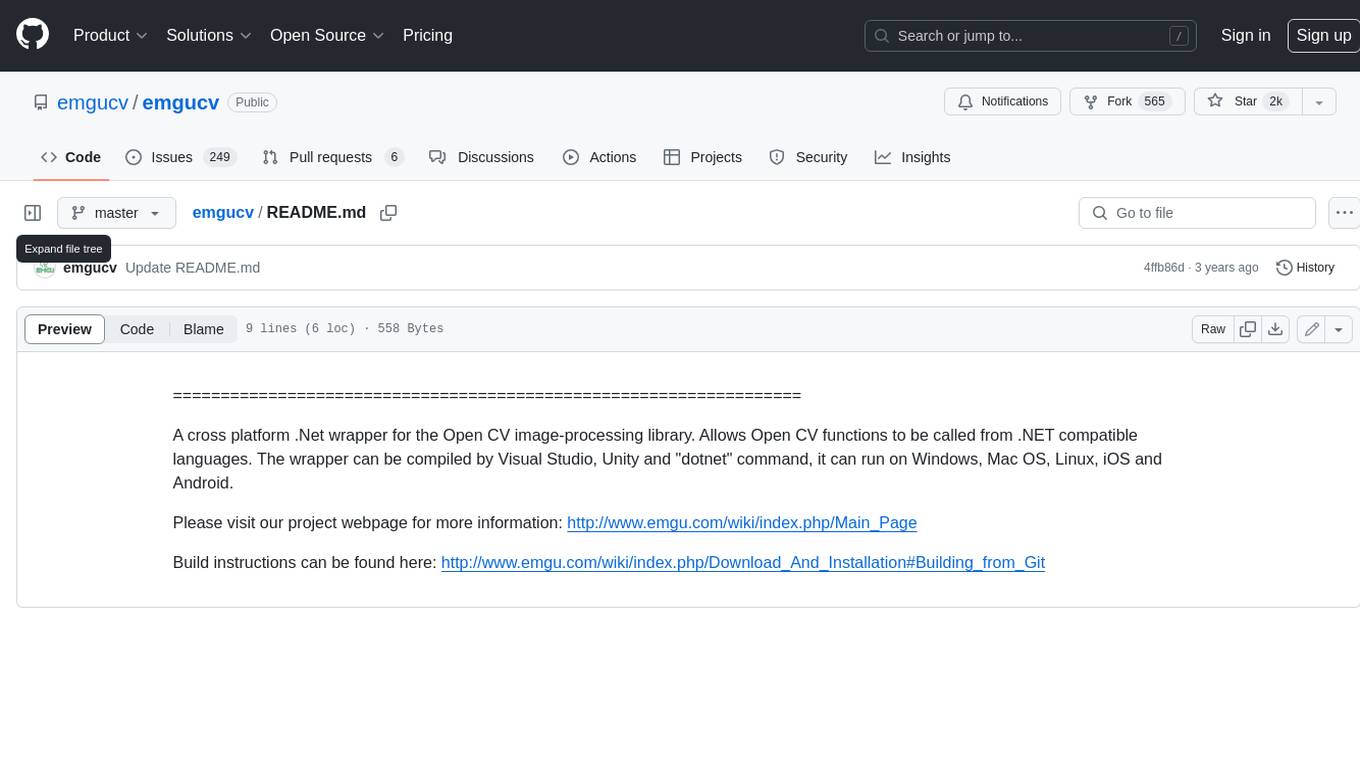
emgucv
Emgu CV is a cross-platform .Net wrapper for the OpenCV image-processing library. It allows OpenCV functions to be called from .NET compatible languages. The wrapper can be compiled by Visual Studio, Unity, and "dotnet" command, and it can run on Windows, Mac OS, Linux, iOS, and Android.
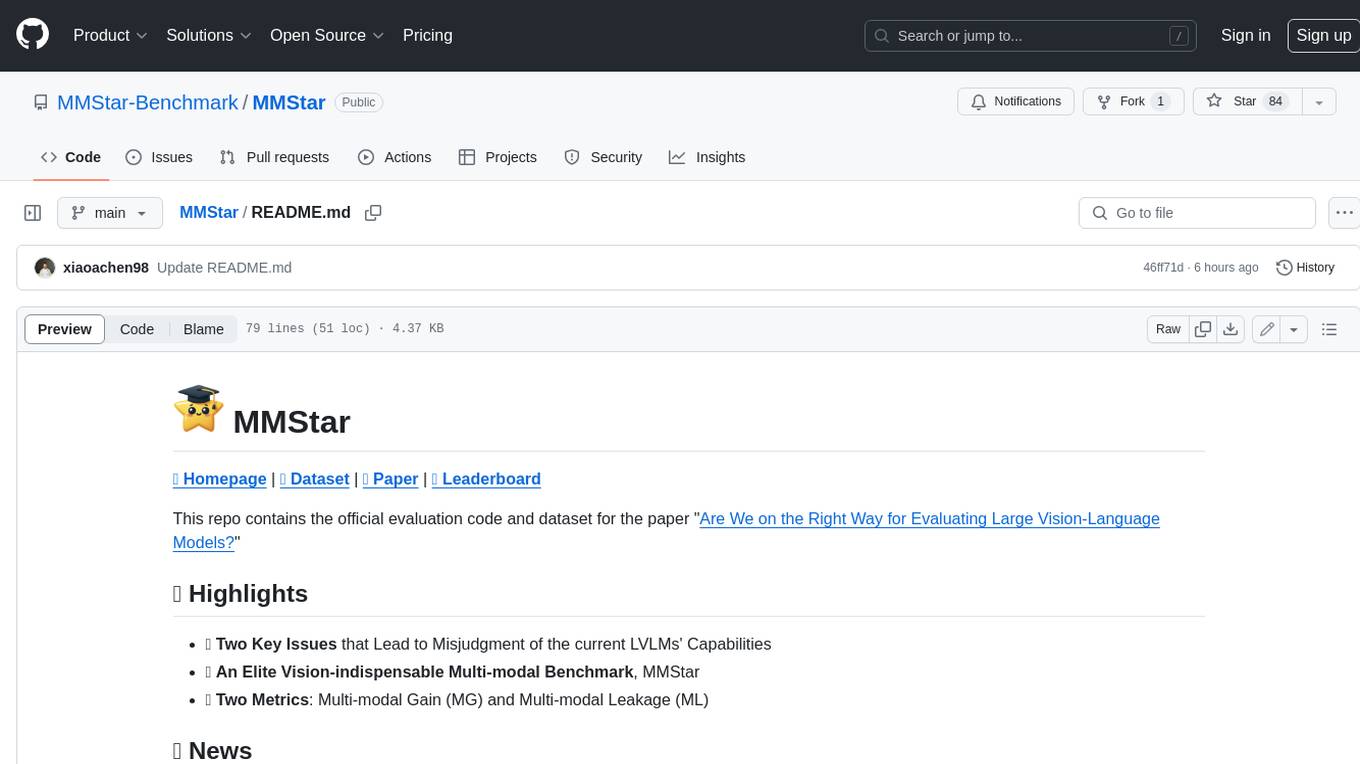
MMStar
MMStar is an elite vision-indispensable multi-modal benchmark comprising 1,500 challenge samples meticulously selected by humans. It addresses two key issues in current LLM evaluation: the unnecessary use of visual content in many samples and the existence of unintentional data leakage in LLM and LVLM training. MMStar evaluates 6 core capabilities across 18 detailed axes, ensuring a balanced distribution of samples across all dimensions.
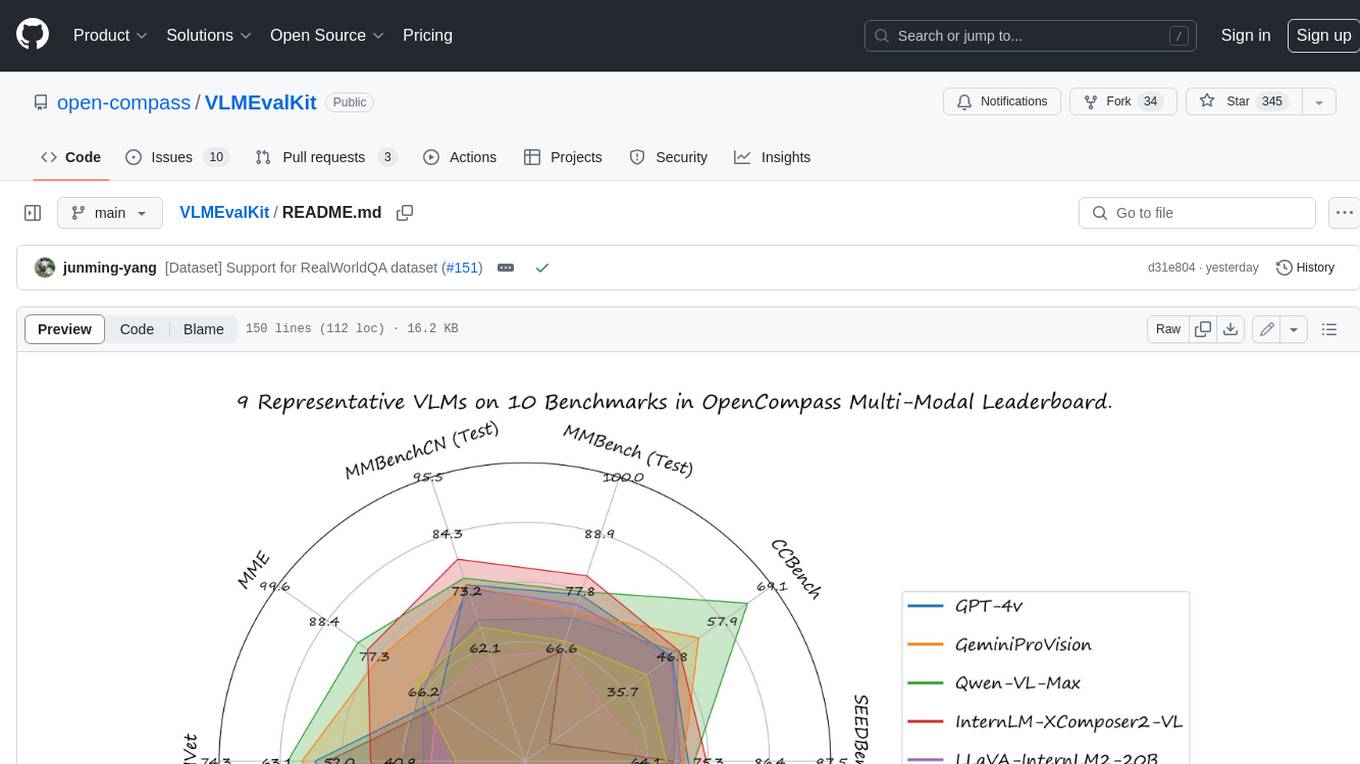
VLMEvalKit
VLMEvalKit is an open-source evaluation toolkit of large vision-language models (LVLMs). It enables one-command evaluation of LVLMs on various benchmarks, without the heavy workload of data preparation under multiple repositories. In VLMEvalKit, we adopt generation-based evaluation for all LVLMs, and provide the evaluation results obtained with both exact matching and LLM-based answer extraction.
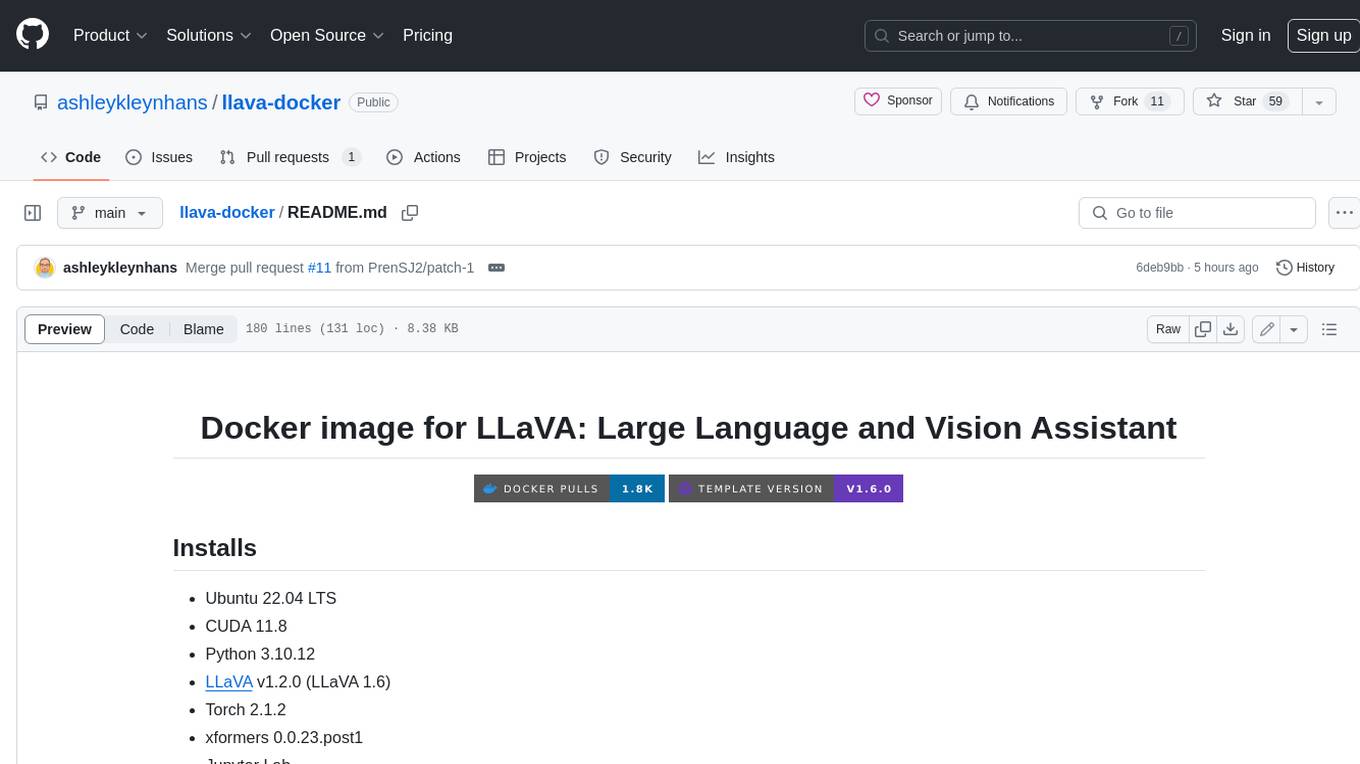
llava-docker
This Docker image for LLaVA (Large Language and Vision Assistant) provides a convenient way to run LLaVA locally or on RunPod. LLaVA is a powerful AI tool that combines natural language processing and computer vision capabilities. With this Docker image, you can easily access LLaVA's functionalities for various tasks, including image captioning, visual question answering, text summarization, and more. The image comes pre-installed with LLaVA v1.2.0, Torch 2.1.2, xformers 0.0.23.post1, and other necessary dependencies. You can customize the model used by setting the MODEL environment variable. The image also includes a Jupyter Lab environment for interactive development and exploration. Overall, this Docker image offers a comprehensive and user-friendly platform for leveraging LLaVA's capabilities.