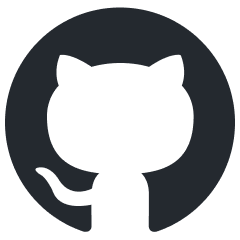
Awesome-LLM-in-Social-Science
Awesome papers involving LLMs in Social Science.
Stars: 136
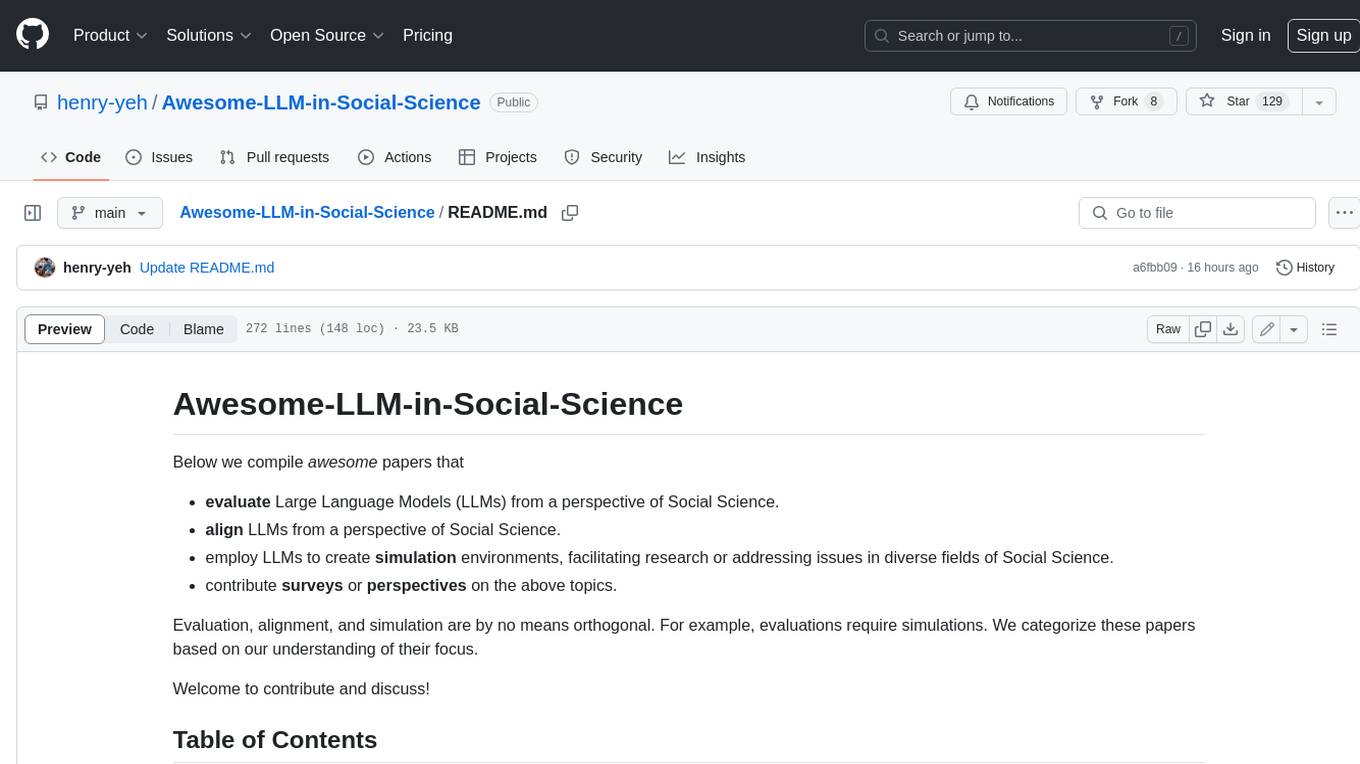
This repository compiles a list of academic papers that evaluate, align, simulate, and provide surveys or perspectives on the use of Large Language Models (LLMs) in the field of Social Science. The papers cover various aspects of LLM research, including assessing their alignment with human values, evaluating their capabilities in tasks such as opinion formation and moral reasoning, and exploring their potential for simulating social interactions and addressing issues in diverse fields of Social Science. The repository aims to provide a comprehensive resource for researchers and practitioners interested in the intersection of LLMs and Social Science.
README:
Below we compile awesome papers that
- evaluate Large Language Models (LLMs) from a perspective of Social Science.
- align LLMs from a perspective of Social Science.
- employ LLMs to create simulation environments, facilitating research or addressing issues in diverse fields of Social Science.
- contribute surveys or perspectives on the above topics.
Evaluation, alignment, and simulation are by no means orthogonal. For example, evaluations require simulations. We categorize these papers based on our understanding of their focus.
Welcome to contribute and discuss!
-
- 2.1. โค๏ธ Value
- 2.2. ๐ฉท Personality
- 2.3. ๐ Morality
- 2.4. ๐ค Opinion
- 2.5. ๐ง Ability
- Foundational Challenges in Assuring Alignment and Safety of Large Language Models, 2024.04, [paper].
- Large Language Model based Multi-Agents: A Survey of Progress and Challenges, 2024.01, [paper], [repo].
- The Rise and Potential of Large Language Model Based Agents: A Survey, 2023, [paper], [repo].
- A Survey on Large Language Model based Autonomous Agents, 2023, [paper], [repo].
- AI Alignment: A Comprehensive Survey, 2023.11, [paper], [website].
- Aligning Large Language Models with Human: A Survey, 2023, [paper], [repo].
- Large Language Model Alignment: A Survey, 2023, [paper].
- Large Language Models Empowered Agent-based Modeling and Simulation: A Survey and Perspectives, 2023.12, [paper].
- A Survey on Evaluation of Large Language Models, 2023.07, [paper], [repo].
- From Instructions to Intrinsic Human Values -- A Survey of Alignment Goals for Big Models, 2023.08, [paper], [repo].
-
Heterogeneous Value Evaluation for Large Language Models, 2023.03, [paper], [code].
TL;DR: This paper introduces the A2EHV method to assess how well these models align with a range of human values categorized under the Social Value Orientation (SVO) framework.
-
Measuring Value Understanding in Language Models through Discriminator-Critique Gap, 2023.10, [paper].
TL;DR: This paper introduces Value Understanding Measurement (VUM) framework to quantitatively assess an LLM's understanding of values. This is done by measuring the discriminator-critique gap (DCG), which evaluates both the model's knowledge of values ("know what") and the reasoning behind this knowledge ("know why").
-
Value FULCRA: Mapping Large Language Models to the Multidimensional Spectrum of Basic Human Values, 2023.11, [paper].
-
Value Kaleidoscope: Engaging AI with Pluralistic Human Values, Rights, and Duties, AAAI24, [paper], [code].
-
Who is GPT-3? An Exploration of Personality, Values and Demographics, 2022.09, [paper]
-
[BFI] Identifying and Manipulating the Personality Traits of Language Models, 2022,12, [paper]
-
[BFI] Evaluating and Inducing Personality in Pre-trained Language Models, NeurIPS 2023 (spotlight), [paper]
-
[BFI] Have Large Language Models Developed a Personality?: Applicability of Self-Assessment Tests in Measuring Personality in LLMs, 2023.05, [paper]
-
[BFI] Personality Traits in Large Language Models, 2023.07, [paper]
-
[BFI] Revisiting the Reliability of Psychological Scales on Large Language Models, 2023.05, [paper]
-
[BFI] Systematic Evaluation of GPT-3 for Zero-Shot Personality Estimation, ACL 2023 workshop, [paper]
-
[BFI] AI Psychometrics: Assessing the Psychological Profiles of Large Language Models Through Psychometric Inventories, Journal, 2024.01, [paper]
-
Does GPT-3 Demonstrate Psychopathy? Evaluating Large Language Models from a Psychological Perspective, 2022.12, [paper]
-
Does Role-Playing Chatbots Capture the Character Personalities? Assessing Personality Traits for Role-Playing Chatbots, 2023.10, [paper]
-
[MBTI] Do LLMs Possess a Personality? Making the MBTI Test an Amazing Evaluation for Large Language Models, 2023.07, [paper]
-
[MBTI] Can ChatGPT Assess Human Personalities? A General Evaluation Framework, 2023.03, EMNLP 2023, [paper], [code].
TL;DR: (1) Using LLM to evaluate MBTI of different groups of people via prompt engineering. (2) Unbiased prompts by averaging over randomly permuted options. (3) Converting the original subject of the question statements into a target subject (e.g., men, barbers). (4) Ask LLM "is it right/wrong" instead of "do you agree/disagree". (5) Metrics to evaluate consistency, robustness, and fairness.
-
[MBTI] Open Models, Closed Minds? On Agents Capabilities in Mimicking Human Personalities through Open Large Language Models, 2024.01, [paper]
-
Who is ChatGPT? Benchmarking LLMs' Psychological Portrayal Using PsychoBench, ICLR 2024, [paper], [code]
TL;DR: (1) Using 13 psychometric scales. (2) Directly prompt LLMs to generate numbers. (3) Discussing reliability and validity.
-
Aligning AI With Shared Human Values, 2020, [paper].
-
Exploring the psychology of GPT-4's Moral and Legal Reasoning, 2023.08, [paper].
TL;DR: The paper investigates GPT-4's moral and legal reasoning compared to humans across several domains, using vignette-based studies. It reveals significant parallels and differences in GPT-4's responses, offering insights into its alignment with human moral judgments.
-
Probing the Moral Development of Large Language Models through Defining Issues Test
TL;DR: Defining Issues Test (DIT) based on Kohlberg's model of moral development is used to evaluate the ethical reasoning abilities of LLMs. GPT-3 performs at random baseline level while GPT-4 achieves the highest moral development score equivalent to graduate students.
-
Moral Foundations of Large Language Models, 2023.10, [paper].
-
Moral Mimicry: Large Language Models Produce Moral Rationalizations Tailored to Political Identity, 2023.06, [paper]
-
Evaluating the Moral Beliefs Encoded in LLMs, 2023.07, [paper]
-
More human than human: measuring ChatGPT political bias, 2023, [paper].
TL;DR: This paper proposed empirical designs to measure political bias in ChatGPT, showing that ChatGPT exhibits a significant and systematic political bias towards the Democrats in the US, Lula in Brazil, and the Labour Party in the UK.
-
Towards Measuring the Representation of Subjective Global Opinions in Language Models, 2023.07, [paper], [website].
TL;DR: This study explores how to quantitatively assess the representation of subjective global opinions in LLMs. It introduces a dataset from cross-national surveys to capture diverse global perspectives, and develops a metric to measure the similarity between LLM-generated responses and human responses conditioned on nationality, revealing biases and stereotypes in the model's responses.
-
Language Models in Sociological Research: An Application to Classifying Large Administrative Data and Measuring Religiosity, 2021, [paper].
-
Can Large Language Models Transform Computational Social Science?, 2023, [paper], [code].
TL;DR: This document provides a roadmap for using LLMs as CSS tools, including prompting best practices and an evaluation pipeline. Evaluations show that LLMs can serve as zero-shot data annotators and assist with challenging creative generation tasks.
-
SOTOPIA: Interactive Evaluation for Social Intelligence in Language Agents, 2023, [paper], [code].
TL;DR: The paper introduces SOTOPIA, a novel interactive environment for evaluating social intelligence in language agents through goal-driven social interactions. Experiments using SOTOPIA reveal gaps between SOTA models and human social intelligence, despite models showing some promising capabilities.
-
Exploring Collaboration Mechanisms for LLM Agents: A Social Psychology View, 2023, [paper], [code].
TL;DR: This paper explores collaboration mechanisms among LLMs in a multi-agent system by drawing insights from social psychology. Multi-agent collaboration strategies are more important than scaling up single LLMs; fostering effective collaboration is key for more socially-aware AI.
-
Using large language models in psychology, 2023, [paper].
TL;DR: This paper explores the potential applications and concerns of using LLMs in psychological research, and recommends investments in high-quality datasets, performance benchmarks, and infrastructure to enable responsible use of LLMs.
-
Playing repeated games with Large Language Models, 2023.05, [paper].
TL;DR: This paper studies Large Language Models' (LLMs) cooperative and coordinated behavior by letting them play repeated 2-player games. The key findings are that LLMs like GPT-4 perform well in competitive games but struggle to coordinate and alternate strategies in games requiring more cooperation.
-
Machine Psychology: Investigating Emergent Capabilities and Behavior in Large Language Models Using Psychological Methods, 2023, [paper].
-
Using cognitive psychology to understand GPT-3, 2023.02, PNAS, [paper].
-
Large language models as a substitute for human experts in annotating political text, 2024.02, [paper].
-
ValueNet: A New Dataset for Human Value Driven Dialogue System, AAAI 2022, [paper], [dataset].
-
Fine-tuning language models to find agreement among humans with diverse preferences, 2022, [paper].
Keywords: consensus, fine-tuning, diverse preferences, alignment
TL;DR: This work fine-tunes LLM to generate statements that maximize the expected approval for a group of people with potentially diverse opinions, especially on moral and political issues.
-
Training Socially Aligned Language Models in Simulated Human Society, 2023, [paper], [code].
Keywords: Stable Alignment, social alignment, societal norms and values, simulated social interactions, contrastive supervised learning
TL;DR: This paper presents a training paradigm that permits LMs to learn from simulated social interactions for their social alignment. The model trained under such a paradigm better handles โjailbreaking promptsโ.
-
[Norm] Align on the Fly: Adapting Chatbot Behavior to Established Norms, 2023.12, [paper], [code].
TL;DR: Using RAG to align LLMs with dynamic, diverse human values such as social norms.
-
[MBTI] Machine Mindset: An MBTI Exploration of Large Language Models, 2023.12, [paper], [code].
TL;DR: Train LLM toward certain MBTI via instruction tuning and direct preference optimization (DPO).
-
Agent Alignment in Evolving Social Norms, 2024.01, [paper].
-
Out of One, Many: Using Language Models to Simulate Human Samples, 2022, [paper].
TL;DR: This work introduces "algorithmic fidelity" - the degree to which the relationships between ideas, attitudes, and contexts in a model mirror those in human groups. They propose 4 criteria for assessing algorithmic fidelity and demonstrate that GPT-3 exhibits a high degree of fidelity for modeling public opinion and political attitudes in the U.S.
-
Social Simulacra: Creating Populated Prototypes for Social Computing Systems, 2022, [paper].
Keywords: social computing prototypes, social simulacra, LLMs, system design refinement
TL;DR: This paper proposes Social Simulacra, a social computing prototype, to mimic authentic social interactions within a system populated by diverse community members, each with distinct behaviors such as posts, replies, and anti-social tendencies.
-
Generative Agents: Interactive Simulacra of Human Behavior, 2023, [paper], [code].
Keywords: generative agents, sandbox environment, natural language communication, emergent social behaviors, Smallville
TL;DR: This paper introduces generative agents and their architecture for memory storage, reflection, retrieval, etc. The agents produce believable individual and emergent social behaviors in an interactive sandbox environment.
-
Using Large Language Models to Simulate Multiple Humans and Replicate Human Subject Studies, 2023, [paper], [code].
TL;DR: This paper presents a methodology for simulating Turing Experiments (TEs) and applies it to replicate well-established findings from economic, psycholinguistic, and social psychology experiments. The results show that larger language models provide more faithful simulations, except for a "hyper-accuracy distortion" (being unhumanly accurate) present in some recent models.
-
Large Language Models as Simulated Economic Agents: What Can We Learn from Homo Silicus?, 2023 [paper], [code].
TL;DR: LLMs can be used like economists use homo economicus. Experiments using LLMs show qualitatively similar results to the original economic research. It is promising to use LLM to search for novel social science insights to test in the real world.
-
$S^3$: Social-network Simulation System with Large Language Model-Empowered Agents, 2023, [paper].
Keywords: social network simulation, agent-based simulation, information/attitude/emotion propagation, user behavior modeling
TL;DR: This paper introduces the Social-network Simulation System (S3) to simulate social networks via LLM-based agents. Evaluations using two real-world scenarios, namely gender discrimination and nuclear energy, display high accuracy in replicating individual attitudes, emotions, and behaviors, as well as successfully modeling the phenomena of information, attitude, and emotion propagation at the population level.
-
Rethinking the Buyerโs Inspection Paradox in Information Markets with Language Agents, 2023, [paper].
Keywords: buyerโs inspection paradox, information economics, information market, language model, agent
TL;DR: This work explores the buyer's inspection paradox in a simulated information marketplace, highlighting enhanced decision-making and answer quality when agents temporarily access information before purchase.
-
SocioDojo: Building Lifelong Analytical Agents with Real-world Text and Time Series, 2023, [paper].
Keywords: lifelong learning, human society analysis, hyperportfolio, time series investment, Analyst-Assistant-Actuator architecture, Hypothesis and Proof prompting
TL;DR: The paper introduces SocioDojo, a new environment and hyperportfolio task for training lifelong agents to analyze and make decisions about human society, along with a novel Analyst-Assistant-Actuator architecture and Hypothesis & Proof prompting technique. Experiments show the proposed method achieves over 30% higher returns compared to state-of-the-art methods in the hyperportfolio task requiring societal understanding.
-
Humanoid Agents: Platform for Simulating Human-like Generative Agents, 2023, [paper], [code].
Keywords: humanoid agents, generative agents, basic needs, emotions, relationships
TL;DR: This paper proposes Humanoid Agents, a system that guides generative agents to behave more like humans by introducing dynamic elements that affect behavior - basic needs like hunger and rest, emotions, and relationship closeness.
-
When Large Language Model based Agent Meets User Behavior Analysis: A Novel User Simulation Paradigm, 2023, [paper], [code].
Keywords: user behavior analysis, user simulation, recommender system, profiling/memory/action module
TL;DR: This work employs LLM for user simulation in recommender systems. The experiments demonstrate the superiority of RecAgent over baseline simulation systems and its ability to generate reliable user behaviors.
-
Large Language Model-Empowered Agents for Simulating Macroeconomic Activities, 2023, [paper].
Keywords: macroeconomic simulation, agent-based modeling, prompt-engineering, perception/reflection/decision-making abilities
TL;DR: This work leverages LLM-based agents for macroeconomic simulation. Experiments show that LLM-based agents make realistic decisions, reproducing classic macro phenomena better than rule-based or other AI agents.
-
Generative Agent-Based Modeling: Unveiling Social System Dynamics through Coupling Mechanistic Models with Generative Artificial Intelligence, 2023, [paper].
Keywords: Generative Agend-Based Modeling, norm diffusion, social dynamics
TL;DR: The authors demonstrate Generative Agent-Based Modeling (GABM) through a simple model of norm diffusion, where agents decide on wearing green or blue shirts based on peer influence. The results show emergence of group norms, sensitivity to agent personas, and conformity to asymmetric adoption forces.
-
Using Imperfect Surrogates for Downstream Inference: Design-based Supervised Learning for Social Science Applications of Large Language Models, 2023.06, NeurIPS 2023, [paper].
TL;DR: We present a new algorithm for using outputs from LLMs for downstream statistic analyses while guaranteeing statistical properties -- like asymptotic unbiasedness and proper uncertainty quantification -- which are fundamental to CSS research. (็จLLM็่พๅบ่ฟ่ก็คพไผ็งๅญฆ็ๆๆกฃๆ ็ญพ็ไธๆธธ็ป่ฎกๅๆ)
-
Epidemic Modeling with Generative Agents, 2023.07, [paper], [code].
Keywords: epidemic modeling, generative AI, agent-based model, human behavior, COVID-19
TL;DR: The paper presents a new epidemic modeling approach using generative AI to empower individual agents with reasoning ability. The generative agent-based model collectively flattens the epidemic curve, mimicking patterns like multiple waves, through AI-powered decision-making without imposed rules.
-
Emergent analogical reasoning in large language models, 2023.08, nature human behavior, [paper].
Keywords: GPT-3, Analogical Reasoning, Zero-Shot Learning, Cognitive Processes, Human Comparison
TL;DR: This paper investigates the emergent analogical reasoning capabilities of GPT-3, demonstrating its proficiency in various analogy tasks compared to college students. The research highlights GPT-3's potential in zero-shot learning and its similarity to human cognitive processes in problem-solving.
-
MetaAgents: Simulating Interactions of Human Behaviors for LLM-based Task-oriented Coordination via Collaborative Generative Agents, 2023.10, [paper].
Keywords: agent simulation, job fair environment, task-oriented coordination
TL;DR: The paper introduces "MetaAgents" to enhance coordination in LLMs through a novel collaborative and reasoning approach, tested in a simulated job fair environment. The study reveals both the potential and limitations of LLM-based agents in complex social coordination tasks.
-
War and Peace (WarAgent): Large Language Model-based Multi-Agent Simulation of World Wars, 2023.11, [paper], [code].
TL;DR: This paper presents WarAgent, an AI system simulating historical conflicts, revealing how historical and policy factors critically drive the inevitability and nature of wars.
-
Emergence of Social Norms in Large Language Model-based Agent Societies, 2024.03, [paper], [code].
-
A social path to human-like artificial intelligence, 2023.11, Nature Machine Intelligence, [paper].
TL;DR: This paper explores the social pathways to human intelligence, highlighting the roles of collective living, social relationships, and key evolutionary transformations in the development of intelligence.
-
The benefits, risks and bounds of personalizing the alignment of large language models to individuals, 2024.04, Nature Machine Intelligence, [paper].
For Tasks:
Click tags to check more tools for each tasksFor Jobs:
Alternative AI tools for Awesome-LLM-in-Social-Science
Similar Open Source Tools
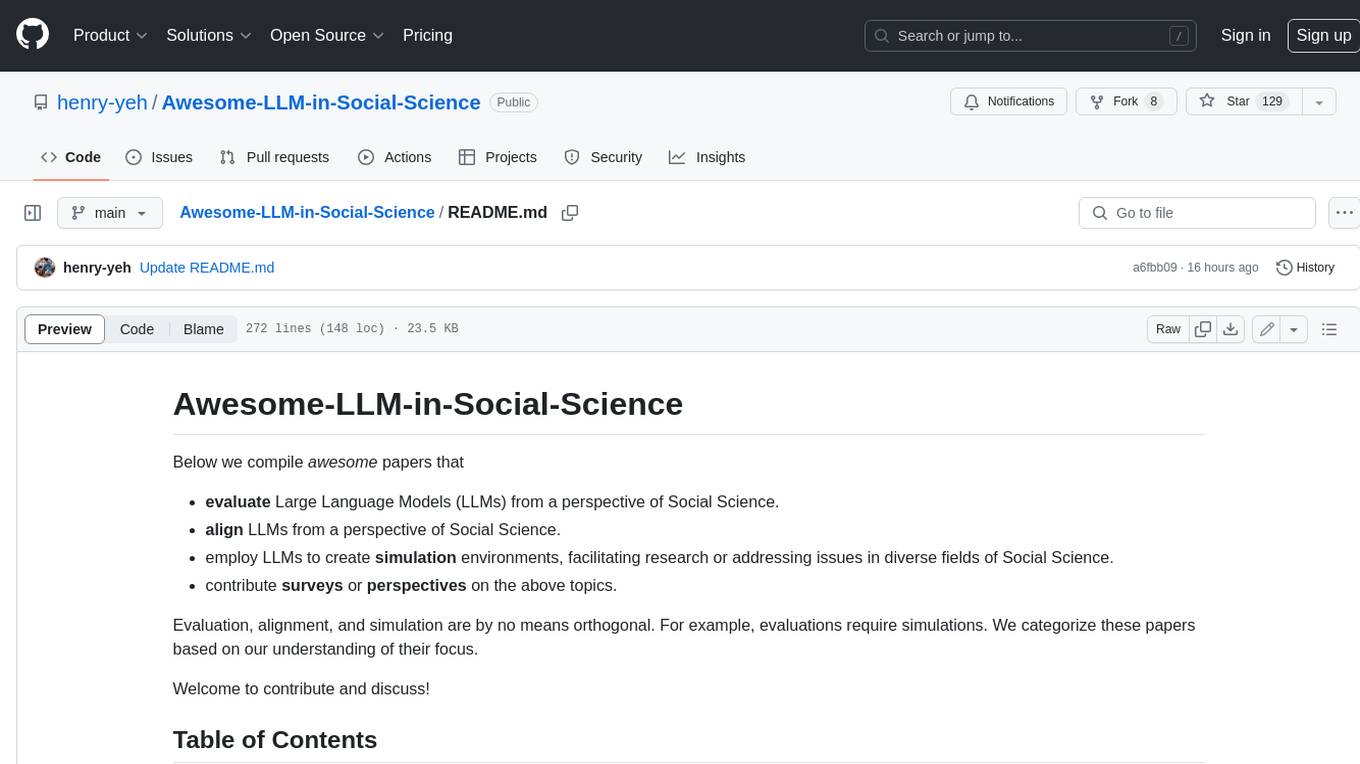
Awesome-LLM-in-Social-Science
This repository compiles a list of academic papers that evaluate, align, simulate, and provide surveys or perspectives on the use of Large Language Models (LLMs) in the field of Social Science. The papers cover various aspects of LLM research, including assessing their alignment with human values, evaluating their capabilities in tasks such as opinion formation and moral reasoning, and exploring their potential for simulating social interactions and addressing issues in diverse fields of Social Science. The repository aims to provide a comprehensive resource for researchers and practitioners interested in the intersection of LLMs and Social Science.
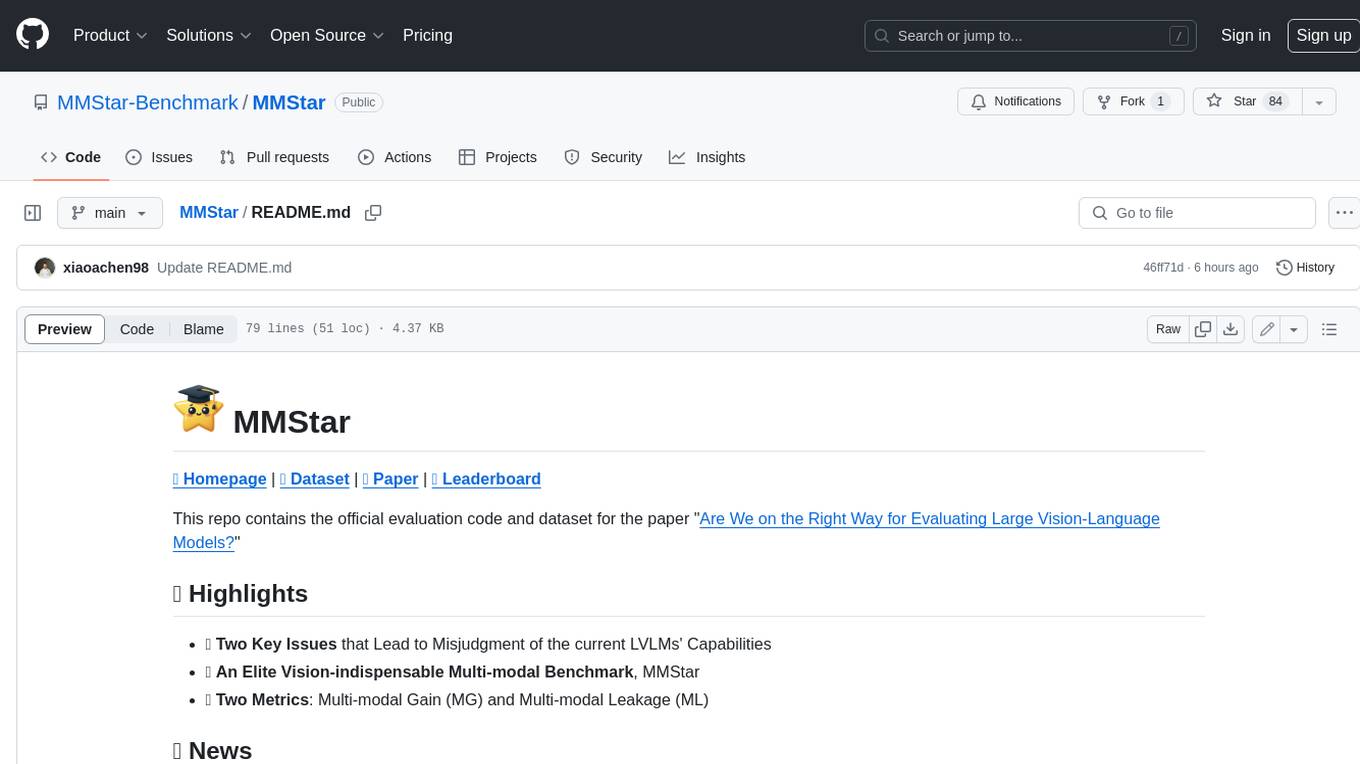
MMStar
MMStar is an elite vision-indispensable multi-modal benchmark comprising 1,500 challenge samples meticulously selected by humans. It addresses two key issues in current LLM evaluation: the unnecessary use of visual content in many samples and the existence of unintentional data leakage in LLM and LVLM training. MMStar evaluates 6 core capabilities across 18 detailed axes, ensuring a balanced distribution of samples across all dimensions.
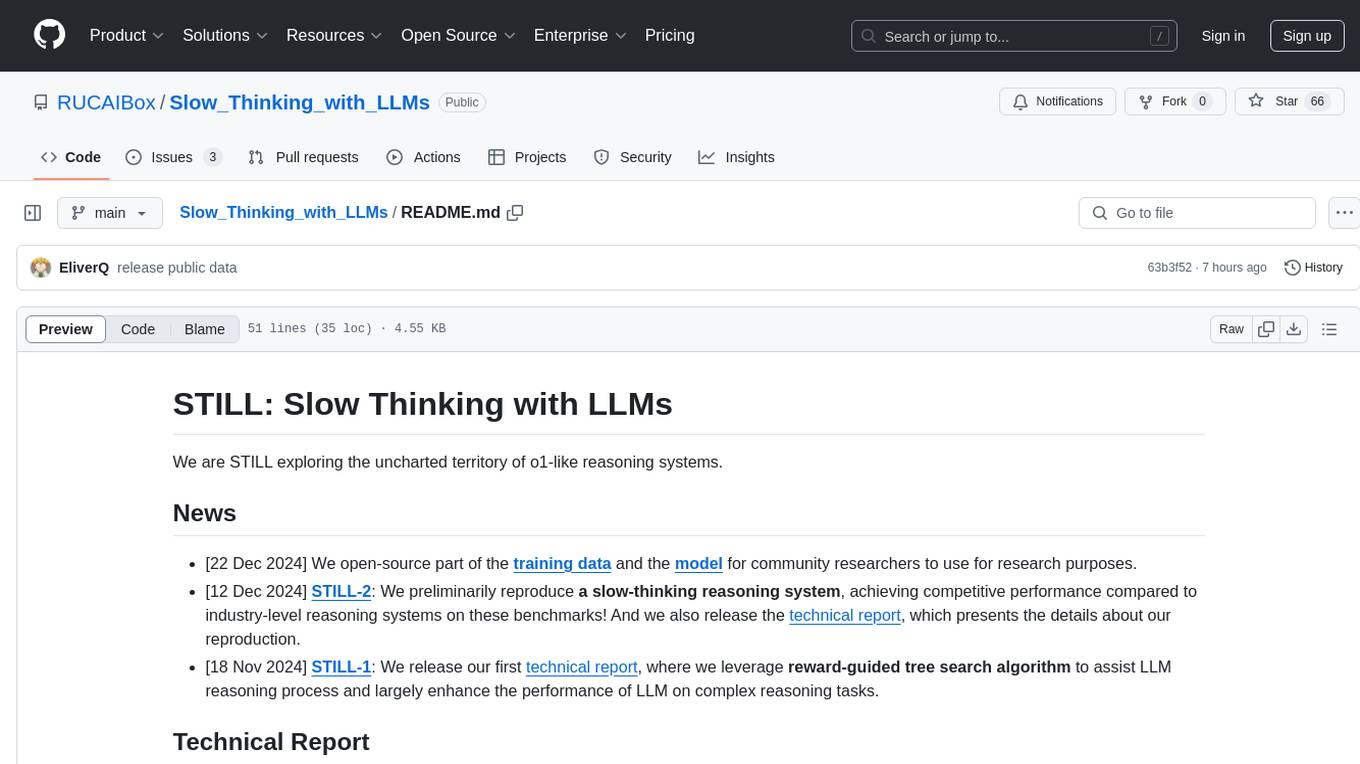
Slow_Thinking_with_LLMs
STILL is an open-source project exploring slow-thinking reasoning systems, focusing on o1-like reasoning systems. The project has released technical reports on enhancing LLM reasoning with reward-guided tree search algorithms and implementing slow-thinking reasoning systems using an imitate, explore, and self-improve framework. The project aims to replicate the capabilities of industry-level reasoning systems by fine-tuning reasoning models with long-form thought data and iteratively refining training datasets.
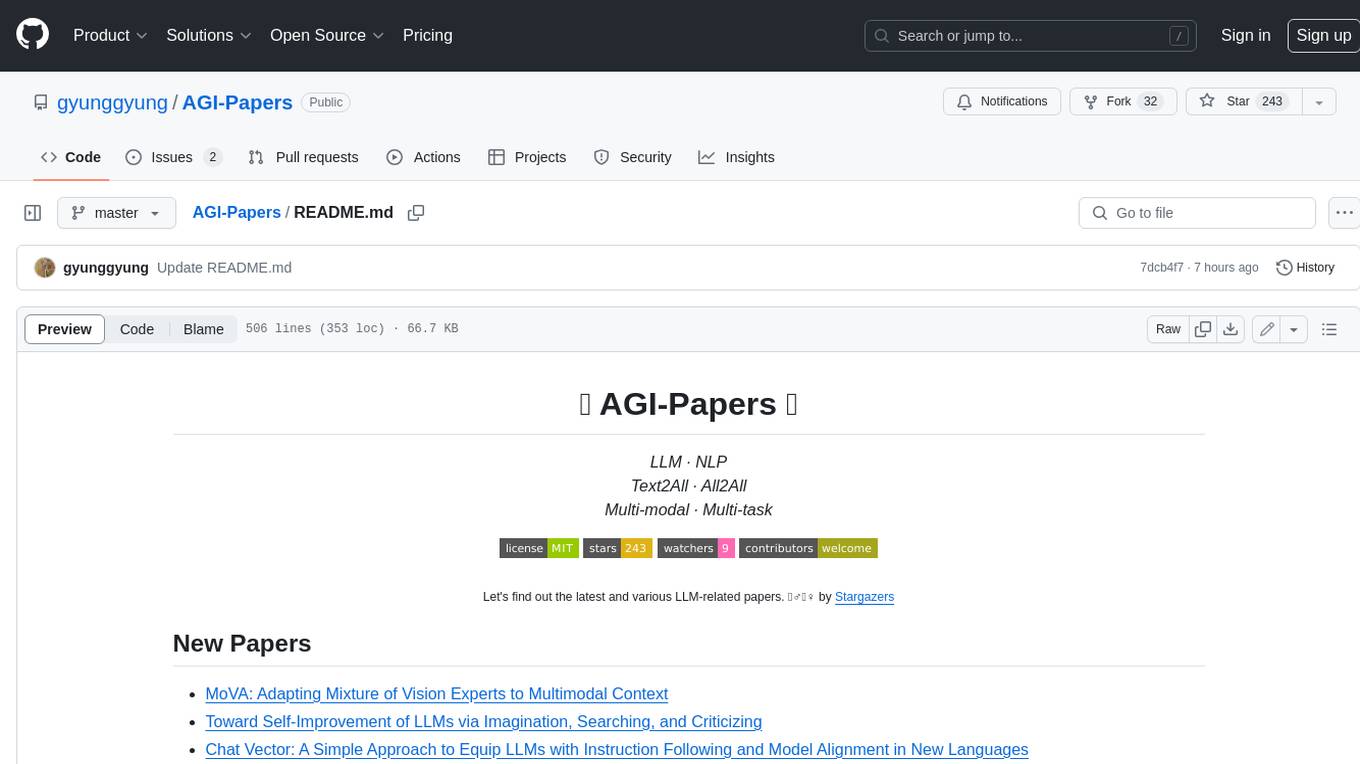
AGI-Papers
This repository contains a collection of papers and resources related to Large Language Models (LLMs), including their applications in various domains such as text generation, translation, question answering, and dialogue systems. The repository also includes discussions on the ethical and societal implications of LLMs. **Description** This repository is a collection of papers and resources related to Large Language Models (LLMs). LLMs are a type of artificial intelligence (AI) that can understand and generate human-like text. They have a wide range of applications, including text generation, translation, question answering, and dialogue systems. **For Jobs** - **Content Writer** - **Copywriter** - **Editor** - **Journalist** - **Marketer** **AI Keywords** - **Large Language Models** - **Natural Language Processing** - **Machine Learning** - **Artificial Intelligence** - **Deep Learning** **For Tasks** - **Generate text** - **Translate text** - **Answer questions** - **Engage in dialogue** - **Summarize text**
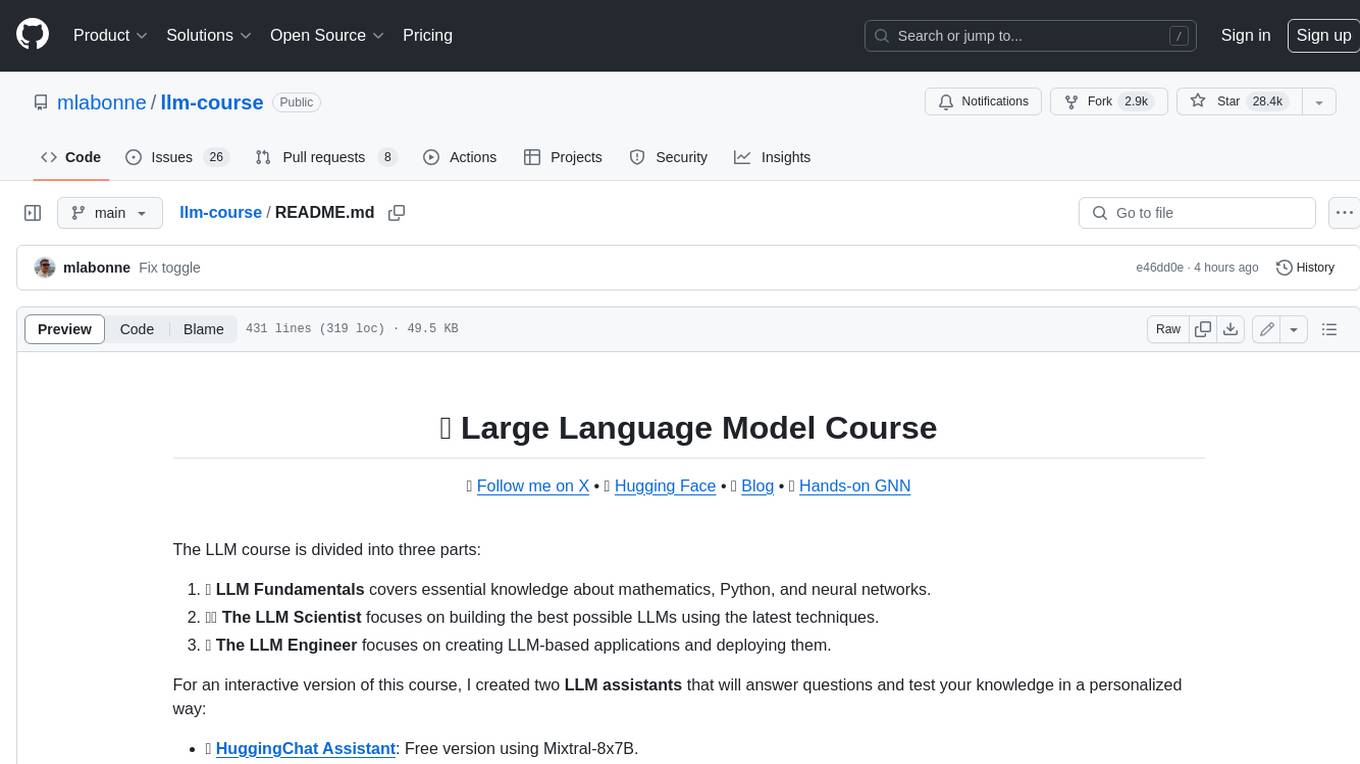
llm-course
The LLM course is divided into three parts: 1. ๐งฉ **LLM Fundamentals** covers essential knowledge about mathematics, Python, and neural networks. 2. ๐งโ๐ฌ **The LLM Scientist** focuses on building the best possible LLMs using the latest techniques. 3. ๐ท **The LLM Engineer** focuses on creating LLM-based applications and deploying them. For an interactive version of this course, I created two **LLM assistants** that will answer questions and test your knowledge in a personalized way: * ๐ค **HuggingChat Assistant**: Free version using Mixtral-8x7B. * ๐ค **ChatGPT Assistant**: Requires a premium account. ## ๐ Notebooks A list of notebooks and articles related to large language models. ### Tools | Notebook | Description | Notebook | |----------|-------------|----------| | ๐ง LLM AutoEval | Automatically evaluate your LLMs using RunPod |  | | ๐ฅฑ LazyMergekit | Easily merge models using MergeKit in one click. |  | | ๐ฆ LazyAxolotl | Fine-tune models in the cloud using Axolotl in one click. |  | | โก AutoQuant | Quantize LLMs in GGUF, GPTQ, EXL2, AWQ, and HQQ formats in one click. |  | | ๐ณ Model Family Tree | Visualize the family tree of merged models. |  | | ๐ ZeroSpace | Automatically create a Gradio chat interface using a free ZeroGPU. |  |
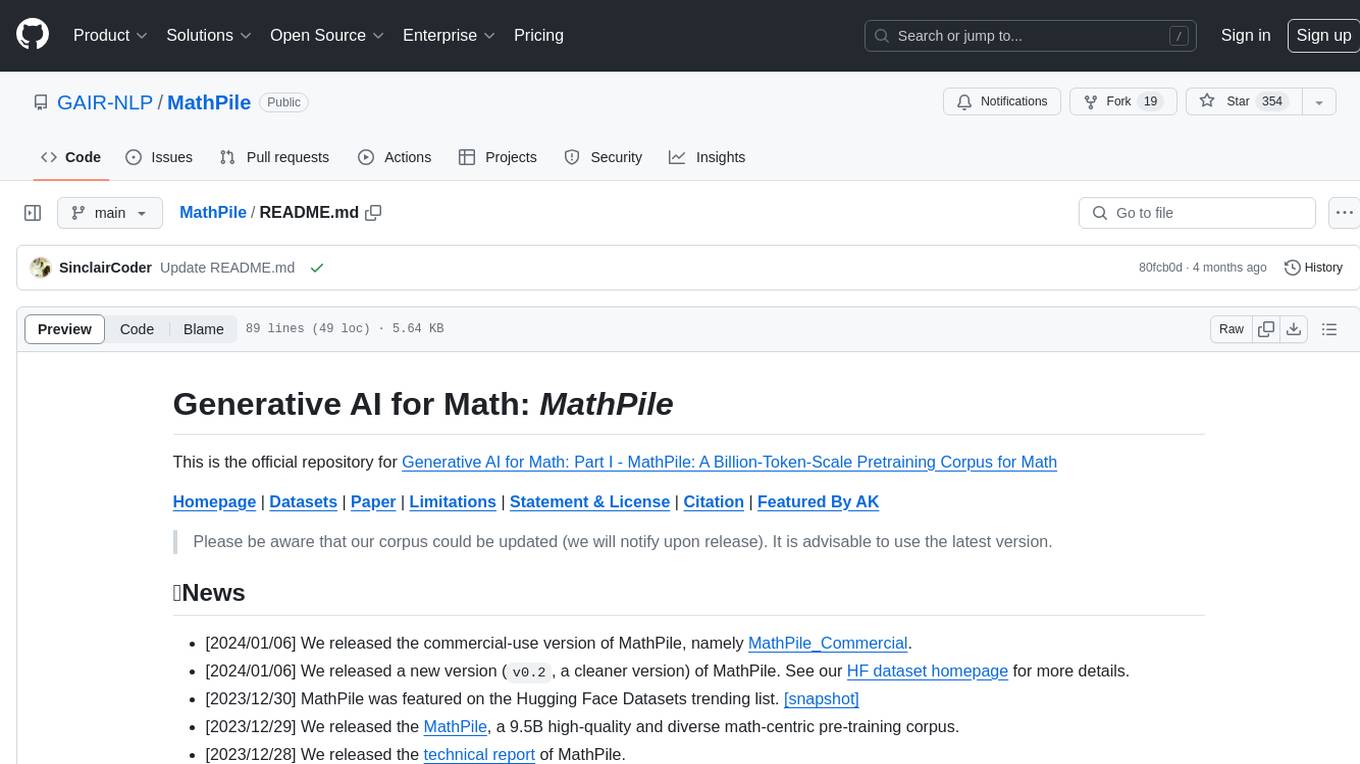
MathPile
MathPile is a generative AI tool designed for math, offering a diverse and high-quality math-centric corpus comprising about 9.5 billion tokens. It draws from various sources such as textbooks, arXiv, Wikipedia, ProofWiki, StackExchange, and web pages, catering to different educational levels and math competitions. The corpus is meticulously processed to ensure data quality, with extensive documentation and data contamination detection. MathPile aims to enhance mathematical reasoning abilities of language models.
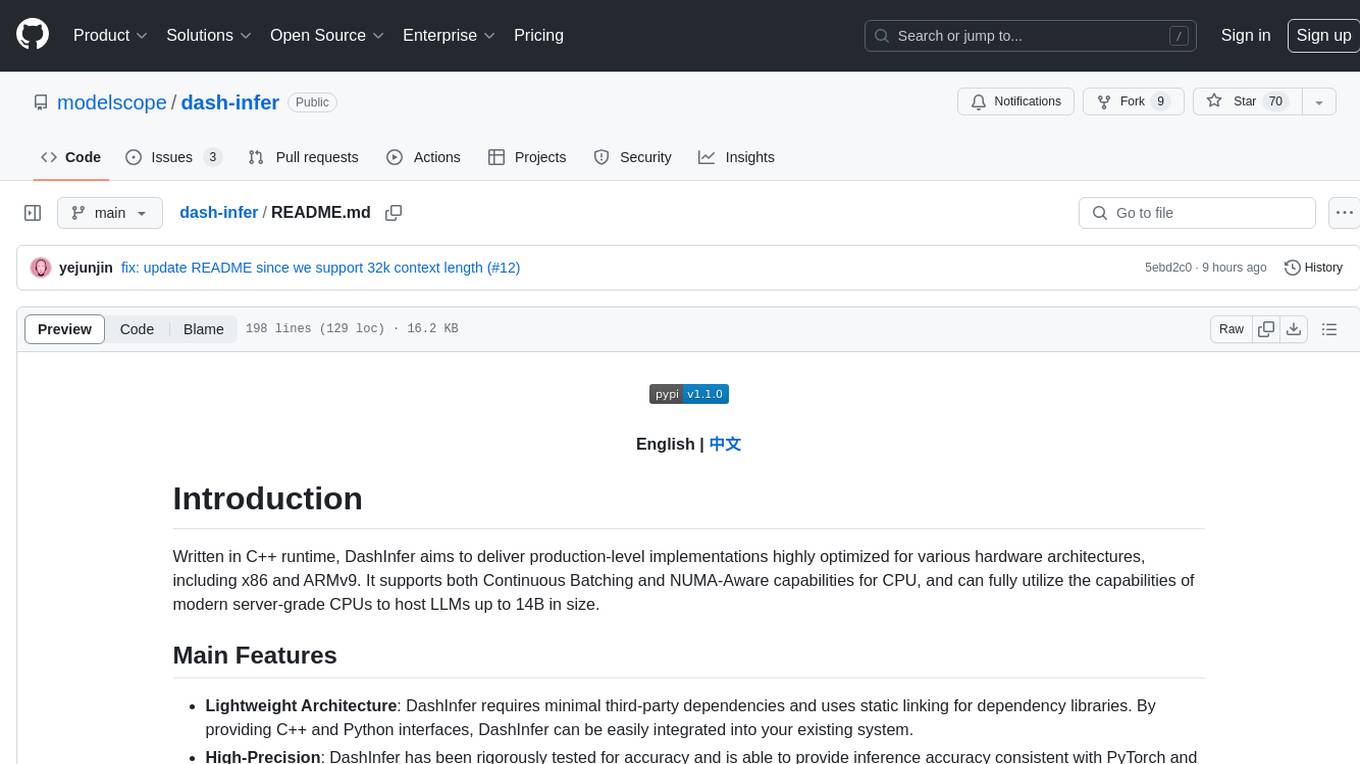
dash-infer
DashInfer is a C++ runtime tool designed to deliver production-level implementations highly optimized for various hardware architectures, including x86 and ARMv9. It supports Continuous Batching and NUMA-Aware capabilities for CPU, and can fully utilize modern server-grade CPUs to host large language models (LLMs) up to 14B in size. With lightweight architecture, high precision, support for mainstream open-source LLMs, post-training quantization, optimized computation kernels, NUMA-aware design, and multi-language API interfaces, DashInfer provides a versatile solution for efficient inference tasks. It supports x86 CPUs with AVX2 instruction set and ARMv9 CPUs with SVE instruction set, along with various data types like FP32, BF16, and InstantQuant. DashInfer also offers single-NUMA and multi-NUMA architectures for model inference, with detailed performance tests and inference accuracy evaluations available. The tool is supported on mainstream Linux server operating systems and provides documentation and examples for easy integration and usage.
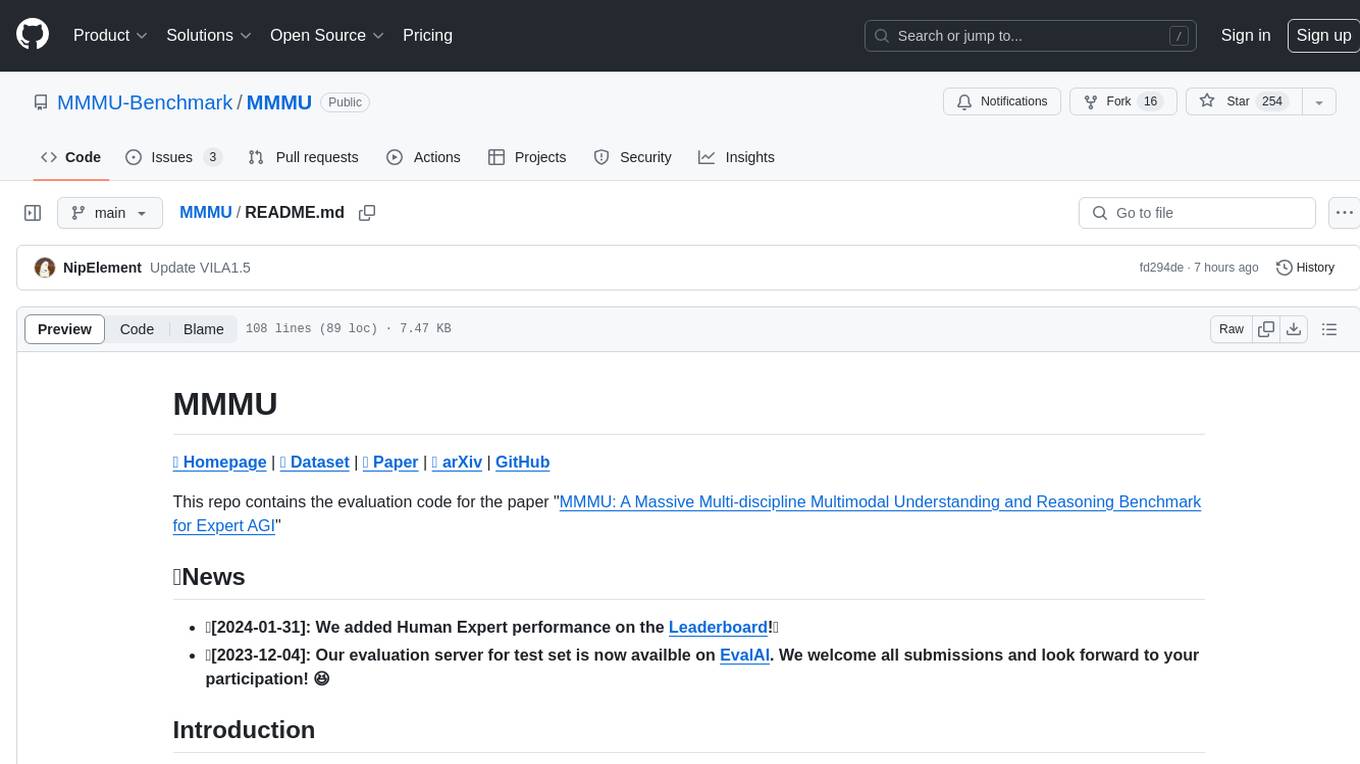
MMMU
MMMU is a benchmark designed to evaluate multimodal models on college-level subject knowledge tasks, covering 30 subjects and 183 subfields with 11.5K questions. It focuses on advanced perception and reasoning with domain-specific knowledge, challenging models to perform tasks akin to those faced by experts. The evaluation of various models highlights substantial challenges, with room for improvement to stimulate the community towards expert artificial general intelligence (AGI).
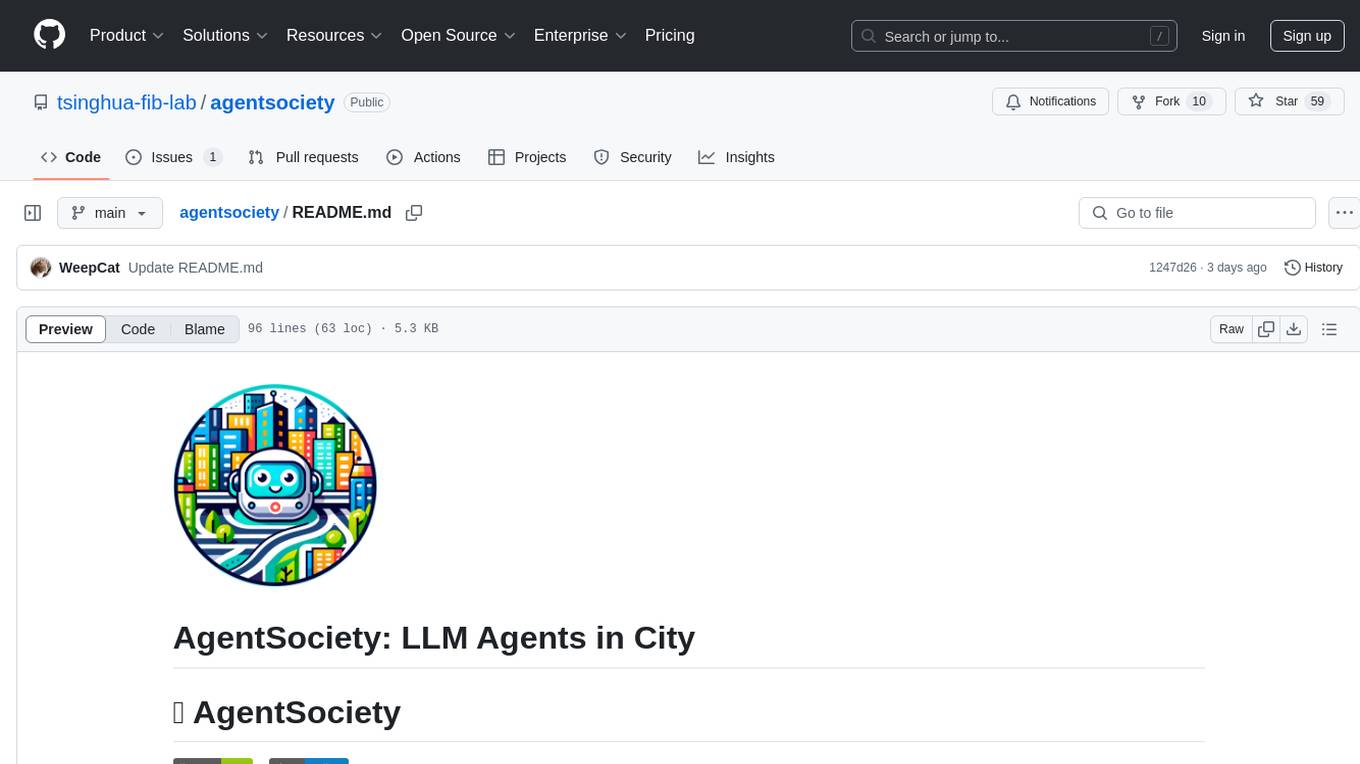
agentsociety
AgentSociety is an advanced framework designed for building agents in urban simulation environments. It integrates LLMs' planning, memory, and reasoning capabilities to generate realistic behaviors. The framework supports dataset-based, text-based, and rule-based environments with interactive visualization. It includes tools for interviews, surveys, interventions, and metric recording tailored for social experimentation.
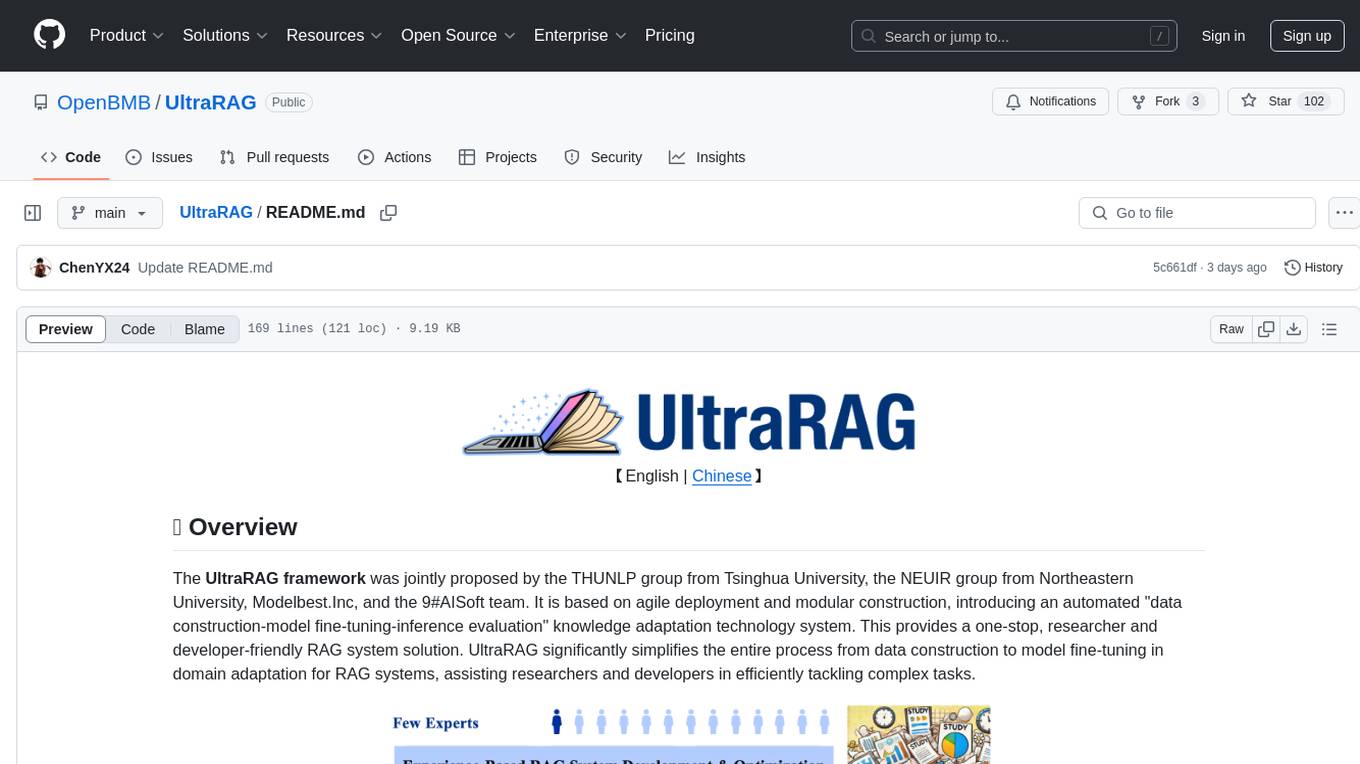
UltraRAG
The UltraRAG framework is a researcher and developer-friendly RAG system solution that simplifies the process from data construction to model fine-tuning in domain adaptation. It introduces an automated knowledge adaptation technology system, supporting no-code programming, one-click synthesis and fine-tuning, multidimensional evaluation, and research-friendly exploration work integration. The architecture consists of Frontend, Service, and Backend components, offering flexibility in customization and optimization. Performance evaluation in the legal field shows improved results compared to VanillaRAG, with specific metrics provided. The repository is licensed under Apache-2.0 and encourages citation for support.
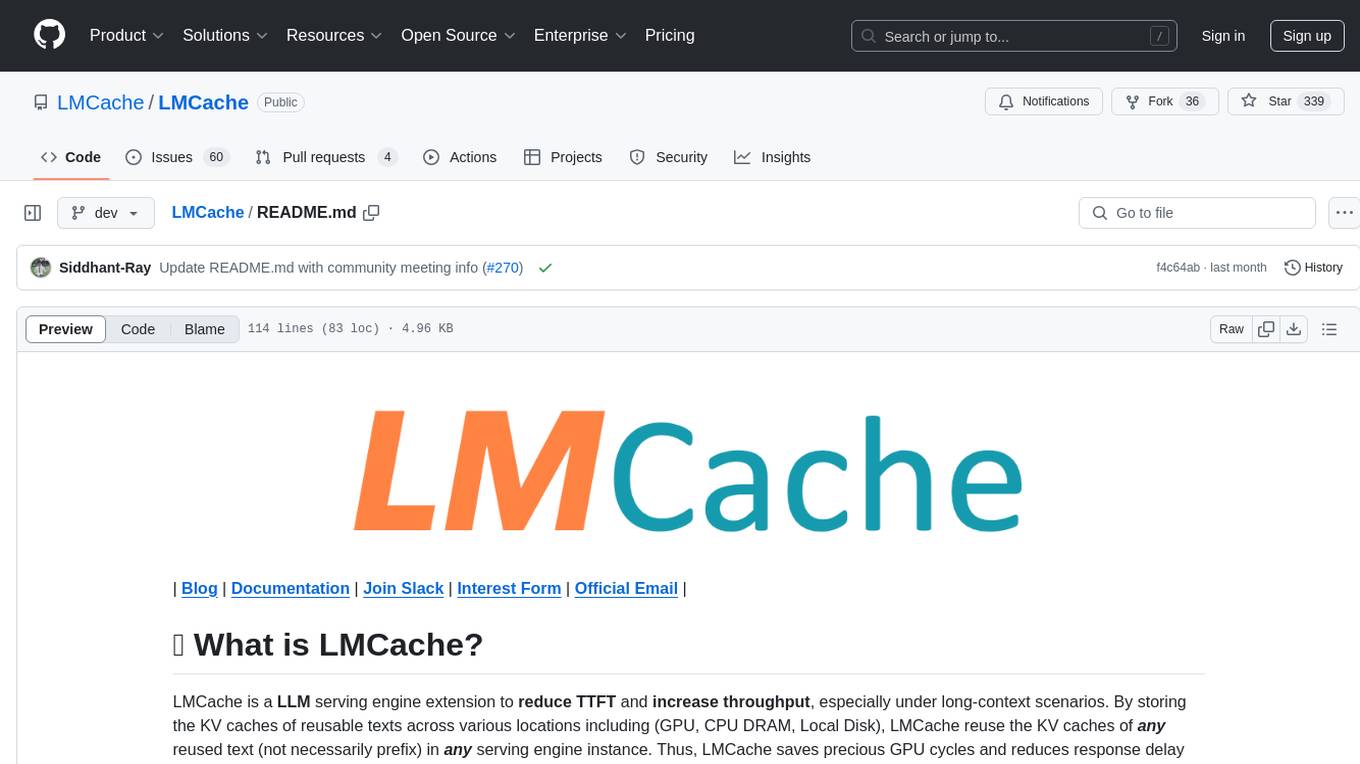
LMCache
LMCache is a serving engine extension designed to reduce time to first token (TTFT) and increase throughput, particularly in long-context scenarios. It stores key-value caches of reusable texts across different locations like GPU, CPU DRAM, and Local Disk, allowing the reuse of any text in any serving engine instance. By combining LMCache with vLLM, significant delay savings and GPU cycle reduction are achieved in various large language model (LLM) use cases, such as multi-round question answering and retrieval-augmented generation (RAG). LMCache provides integration with the latest vLLM version, offering both online serving and offline inference capabilities. It supports sharing key-value caches across multiple vLLM instances and aims to provide stable support for non-prefix key-value caches along with user and developer documentation.
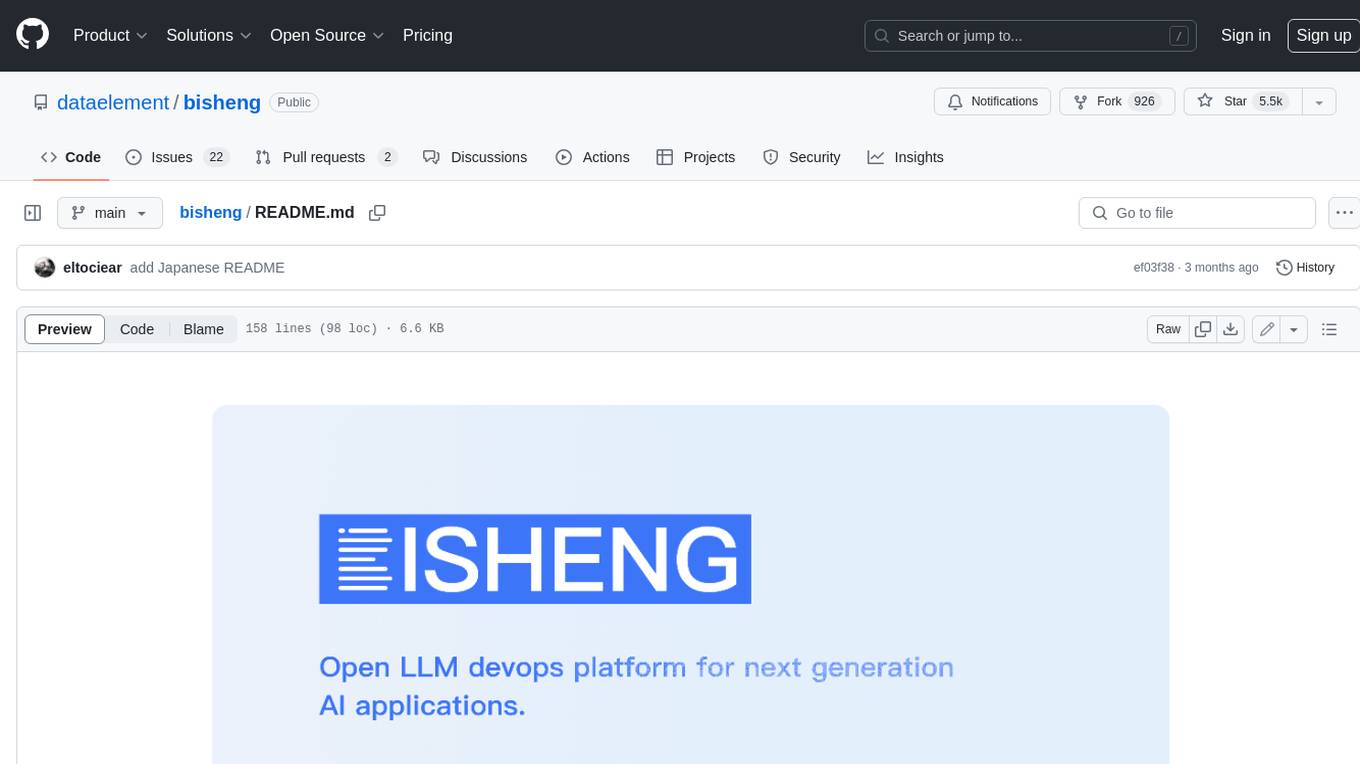
bisheng
Bisheng is a leading open-source **large model application development platform** that empowers and accelerates the development and deployment of large model applications, helping users enter the next generation of application development with the best possible experience.
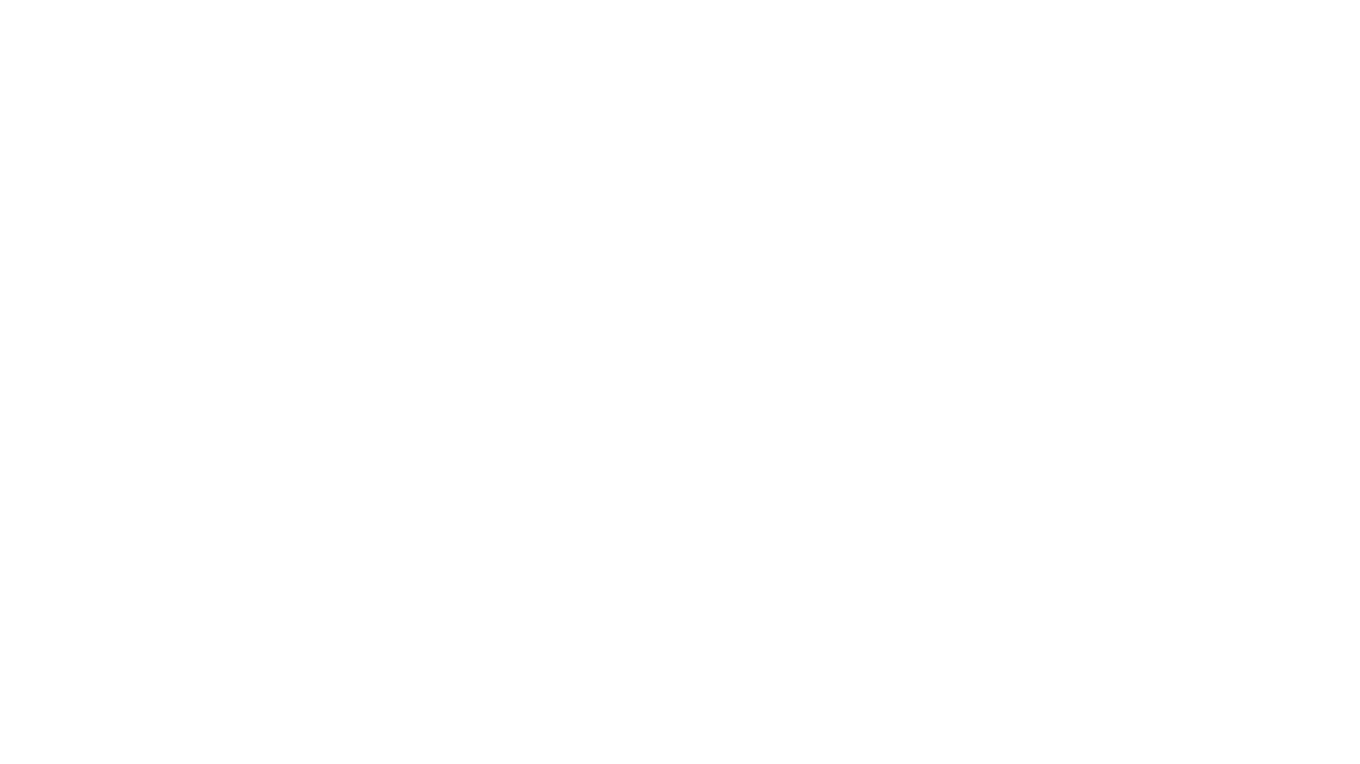
DocsGPT
DocsGPT is an open-source documentation assistant powered by GPT models. It simplifies the process of searching for information in project documentation by allowing developers to ask questions and receive accurate answers. With DocsGPT, users can say goodbye to manual searches and quickly find the information they need. The tool aims to revolutionize project documentation experiences and offers features like live previews, Discord community, guides, and contribution opportunities. It consists of a Flask app, Chrome extension, similarity search index creation script, and a frontend built with Vite and React. Users can quickly get started with DocsGPT by following the provided setup instructions and can contribute to its development by following the guidelines in the CONTRIBUTING.md file. The project follows a Code of Conduct to ensure a harassment-free community environment for all participants. DocsGPT is licensed under MIT and is built with LangChain.
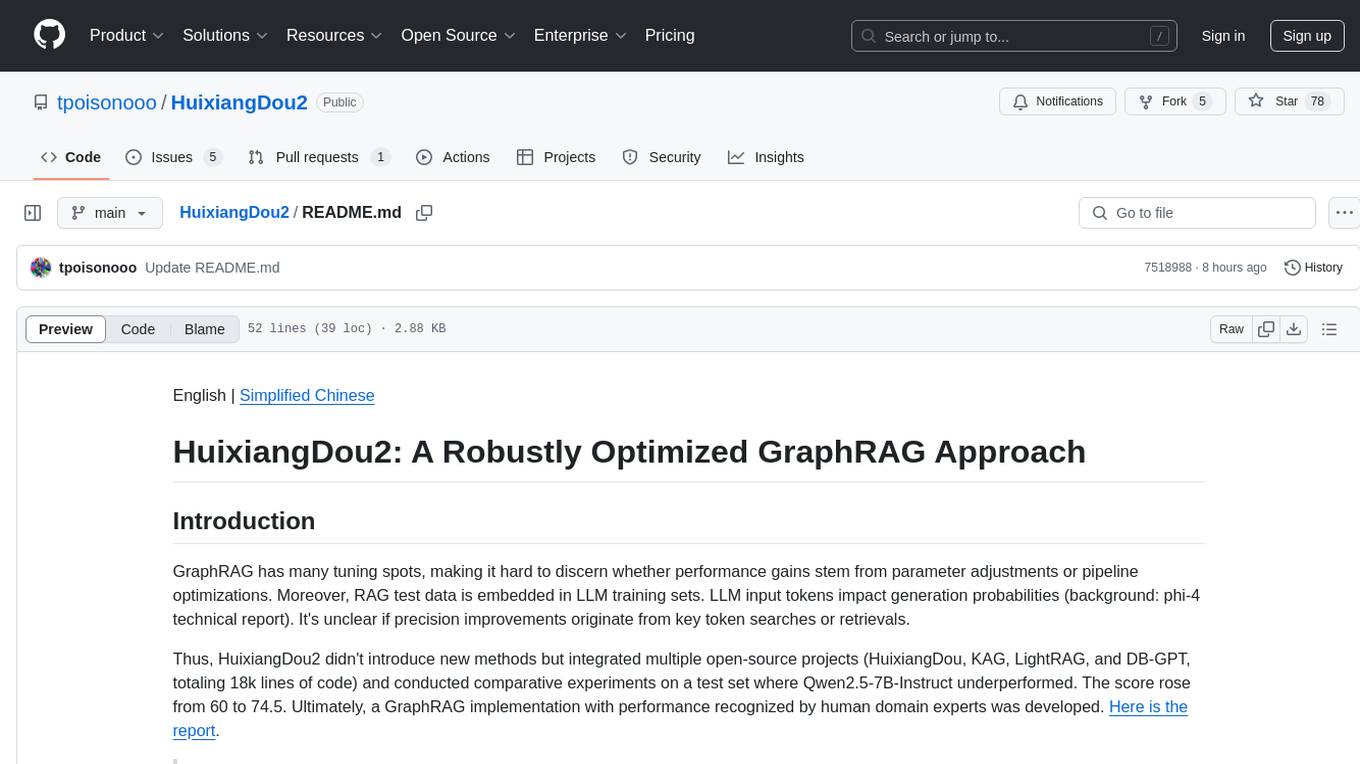
HuixiangDou2
HuixiangDou2 is a robustly optimized GraphRAG approach that integrates multiple open-source projects to improve performance in graph-based augmented generation. It conducts comparative experiments and achieves a significant score increase, leading to a GraphRAG implementation with recognized performance. The repository provides code improvements, dense retrieval for querying entities and relationships, real domain knowledge testing, and impact analysis on accuracy.
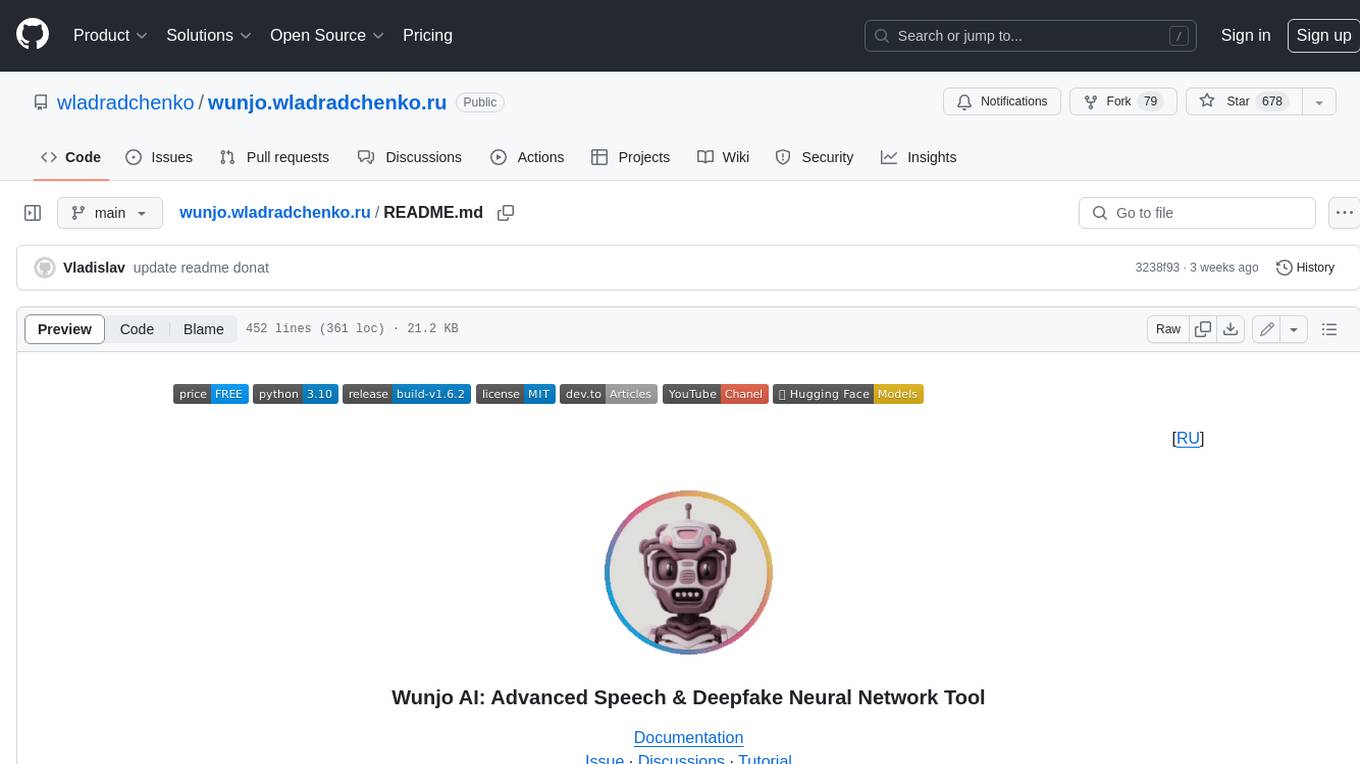
wunjo.wladradchenko.ru
Wunjo AI is a comprehensive tool that empowers users to explore the realm of speech synthesis, deepfake animations, video-to-video transformations, and more. Its user-friendly interface and privacy-first approach make it accessible to both beginners and professionals alike. With Wunjo AI, you can effortlessly convert text into human-like speech, clone voices from audio files, create multi-dialogues with distinct voice profiles, and perform real-time speech recognition. Additionally, you can animate faces using just one photo combined with audio, swap faces in videos, GIFs, and photos, and even remove unwanted objects or enhance the quality of your deepfakes using the AI Retouch Tool. Wunjo AI is an all-in-one solution for your voice and visual AI needs, offering endless possibilities for creativity and expression.
For similar tasks
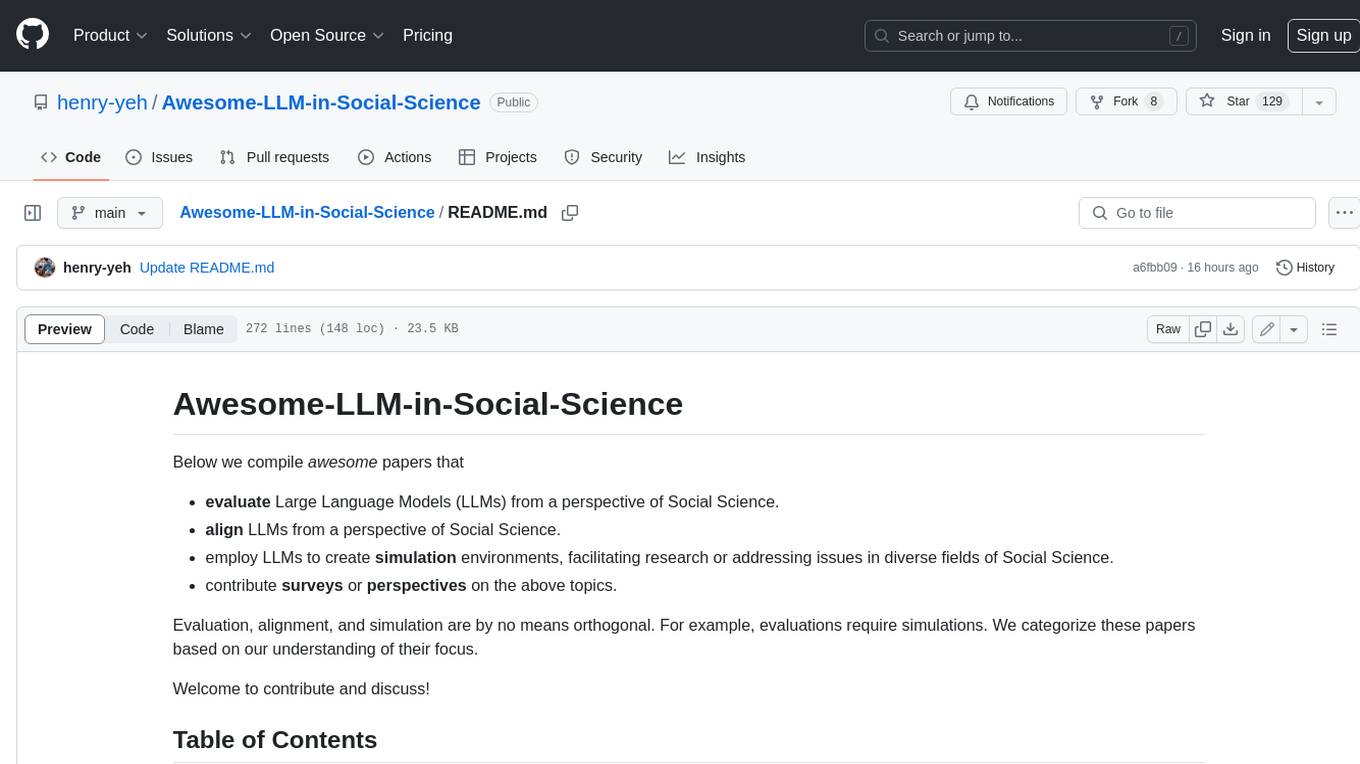
Awesome-LLM-in-Social-Science
This repository compiles a list of academic papers that evaluate, align, simulate, and provide surveys or perspectives on the use of Large Language Models (LLMs) in the field of Social Science. The papers cover various aspects of LLM research, including assessing their alignment with human values, evaluating their capabilities in tasks such as opinion formation and moral reasoning, and exploring their potential for simulating social interactions and addressing issues in diverse fields of Social Science. The repository aims to provide a comprehensive resource for researchers and practitioners interested in the intersection of LLMs and Social Science.
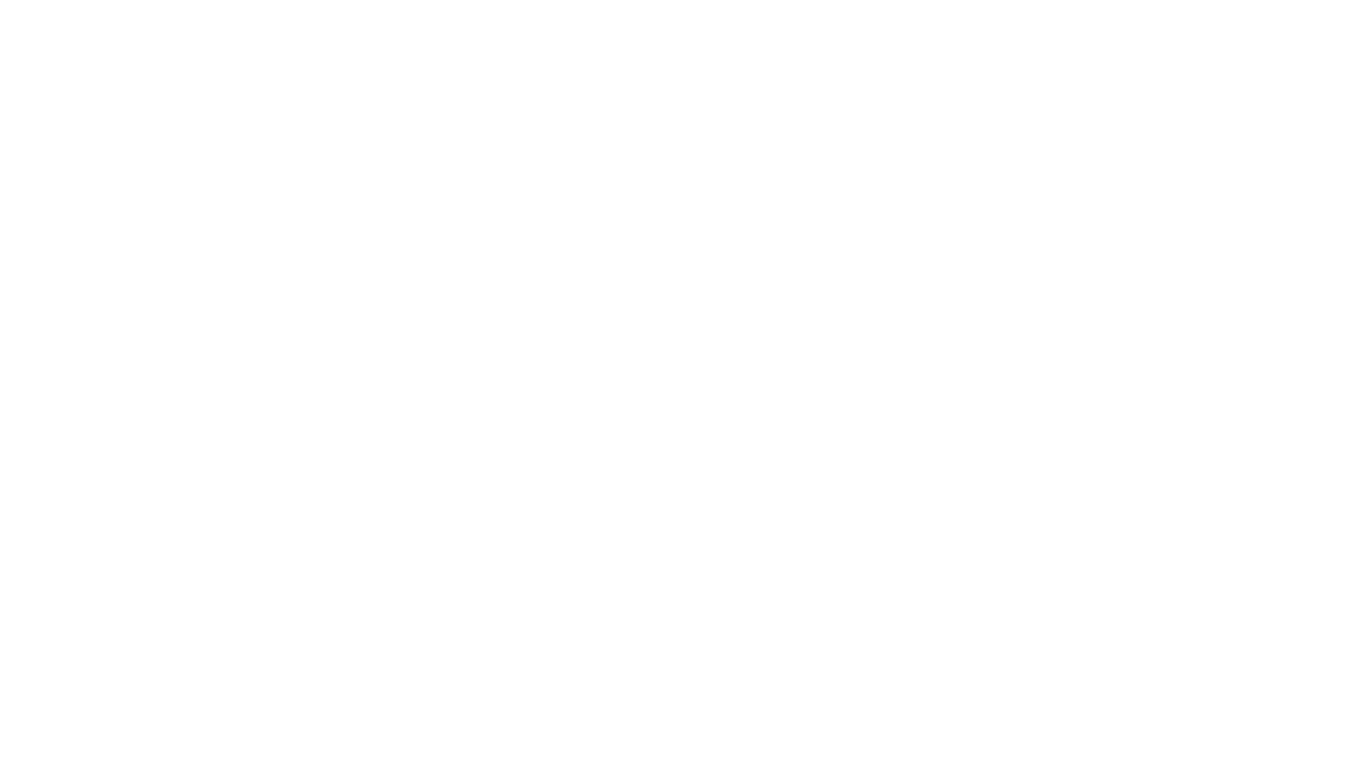
LMOps
LMOps is a research initiative focusing on fundamental research and technology for building AI products with foundation models, particularly enabling AI capabilities with Large Language Models (LLMs) and Generative AI models. The project explores various aspects such as prompt optimization, longer context handling, LLM alignment, acceleration of LLMs, LLM customization, and understanding in-context learning. It also includes tools like Promptist for automatic prompt optimization, Structured Prompting for efficient long-sequence prompts consumption, and X-Prompt for extensible prompts beyond natural language. Additionally, LLMA accelerators are developed to speed up LLM inference by referencing and copying text spans from documents. The project aims to advance technologies that facilitate prompting language models and enhance the performance of LLMs in various scenarios.
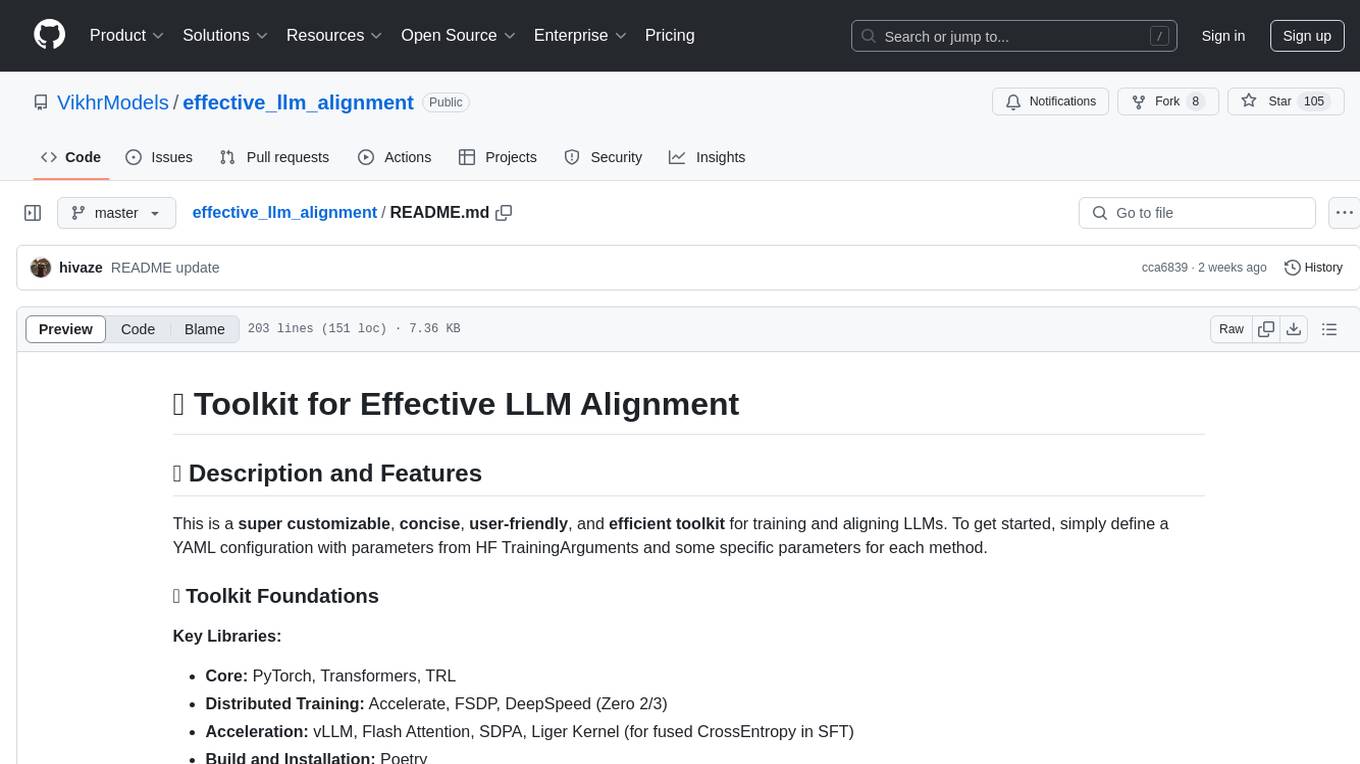
effective_llm_alignment
This is a super customizable, concise, user-friendly, and efficient toolkit for training and aligning LLMs. It provides support for various methods such as SFT, Distillation, DPO, ORPO, CPO, SimPO, SMPO, Non-pair Reward Modeling, Special prompts basket format, Rejection Sampling, Scoring using RM, Effective FAISS Map-Reduce Deduplication, LLM scoring using RM, NER, CLIP, Classification, and STS. The toolkit offers key libraries like PyTorch, Transformers, TRL, Accelerate, FSDP, DeepSpeed, and tools for result logging with wandb or clearml. It allows mixing datasets, generation and logging in wandb/clearml, vLLM batched generation, and aligns models using the SMPO method.
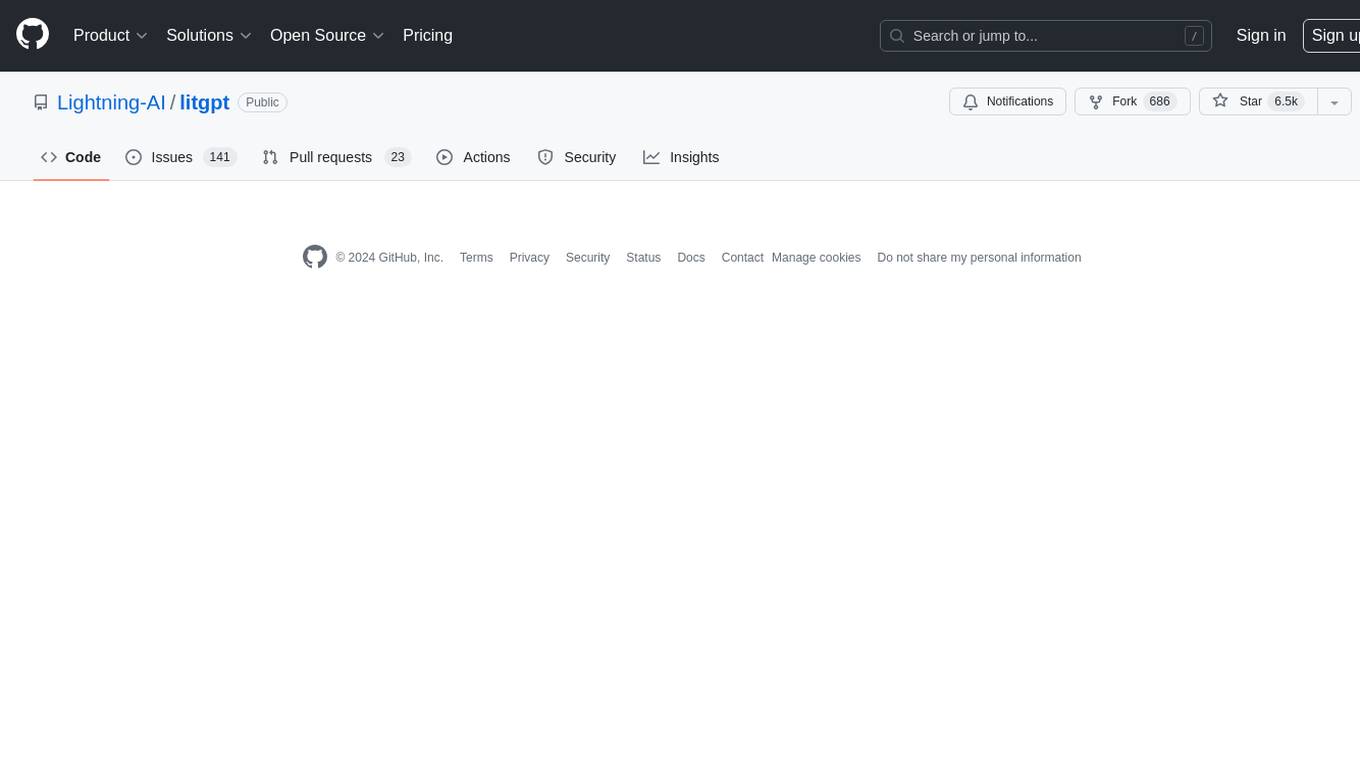
litgpt
LitGPT is a command-line tool designed to easily finetune, pretrain, evaluate, and deploy 20+ LLMs **on your own data**. It features highly-optimized training recipes for the world's most powerful open-source large-language-models (LLMs).
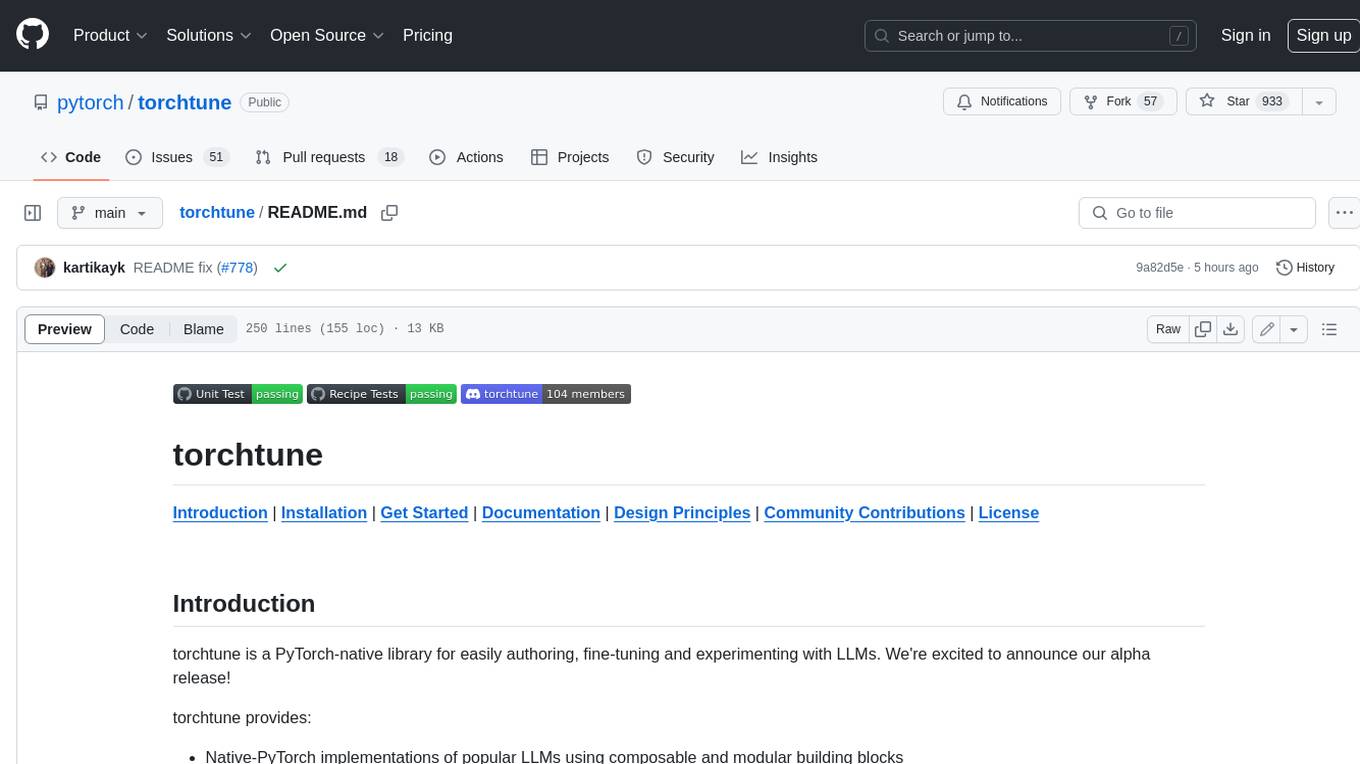
torchtune
Torchtune is a PyTorch-native library for easily authoring, fine-tuning, and experimenting with LLMs. It provides native-PyTorch implementations of popular LLMs using composable and modular building blocks, easy-to-use and hackable training recipes for popular fine-tuning techniques, YAML configs for easily configuring training, evaluation, quantization, or inference recipes, and built-in support for many popular dataset formats and prompt templates to help you quickly get started with training.
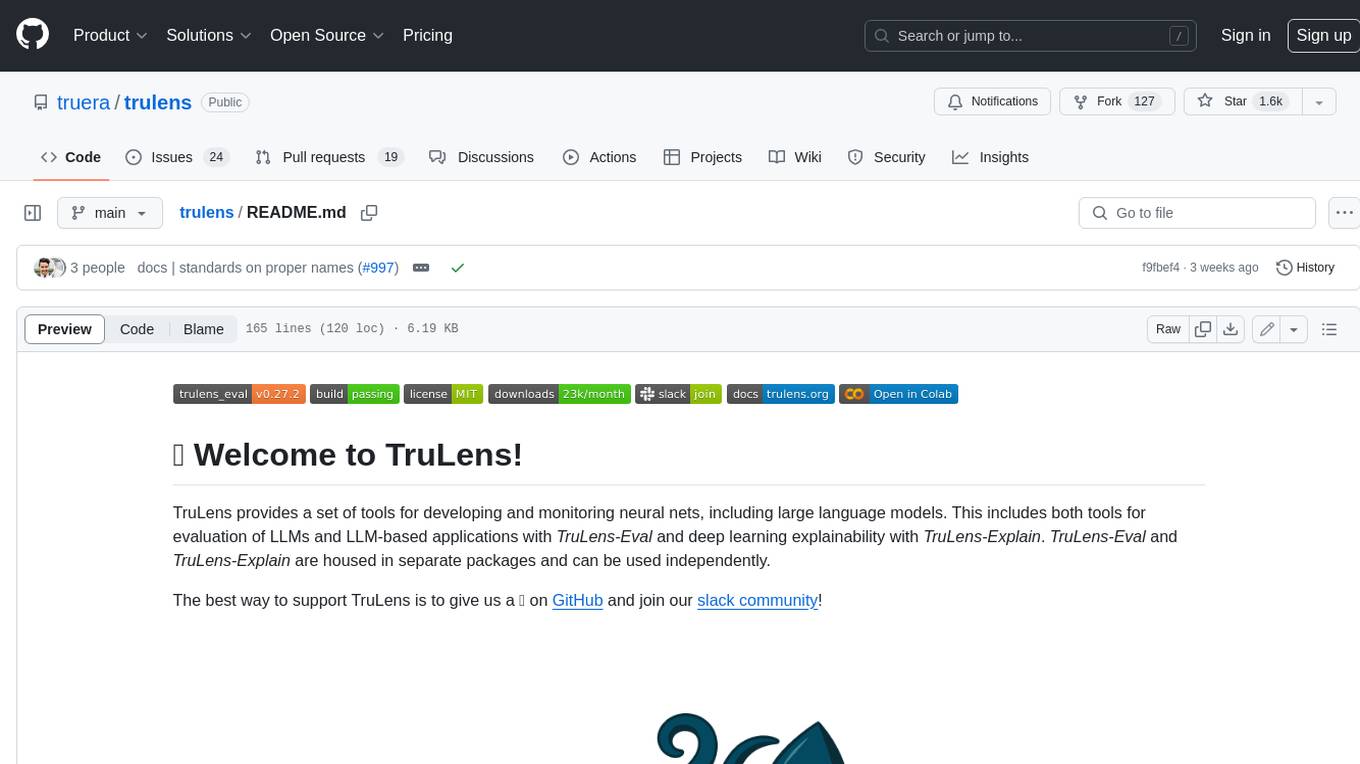
trulens
TruLens provides a set of tools for developing and monitoring neural nets, including large language models. This includes both tools for evaluation of LLMs and LLM-based applications with _TruLens-Eval_ and deep learning explainability with _TruLens-Explain_. _TruLens-Eval_ and _TruLens-Explain_ are housed in separate packages and can be used independently.
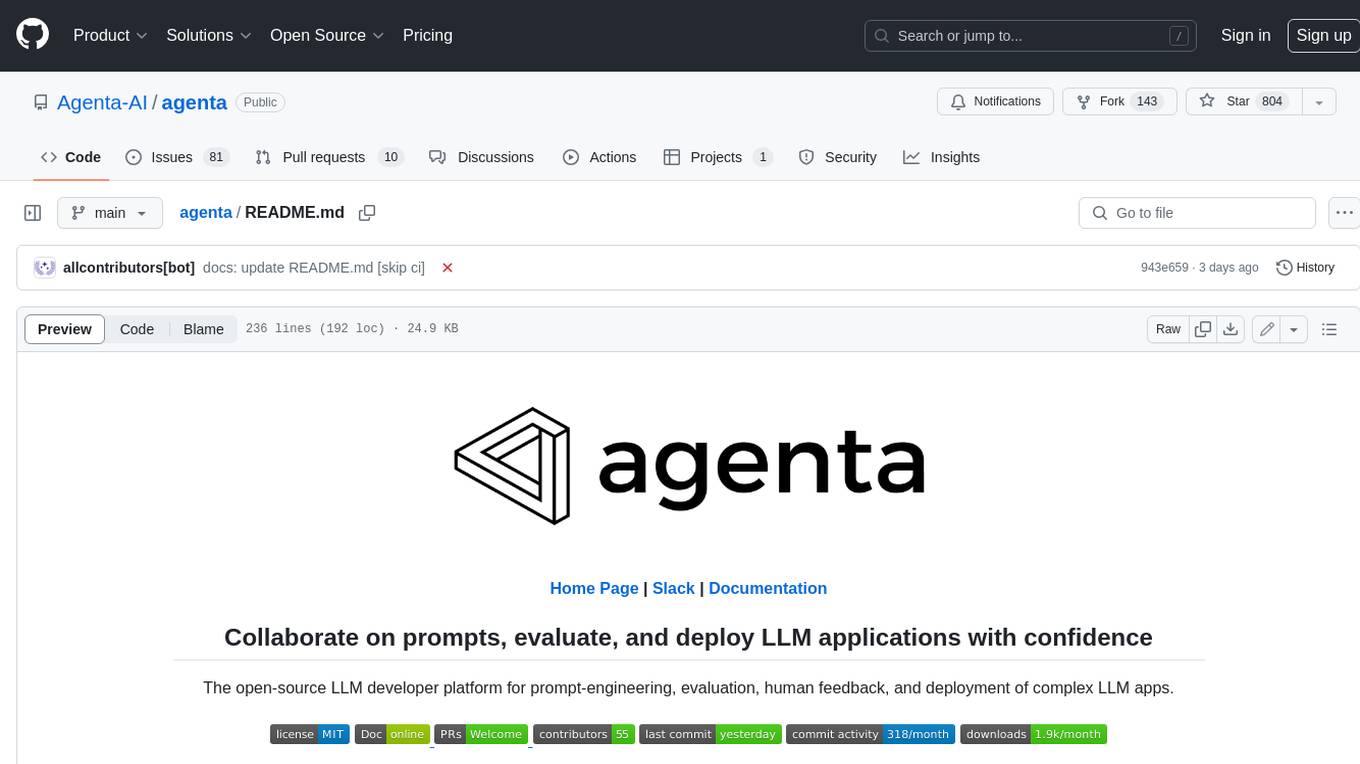
agenta
Agenta is an open-source LLM developer platform for prompt engineering, evaluation, human feedback, and deployment of complex LLM applications. It provides tools for prompt engineering and management, evaluation, human annotation, and deployment, all without imposing any restrictions on your choice of framework, library, or model. Agenta allows developers and product teams to collaborate in building production-grade LLM-powered applications in less time.
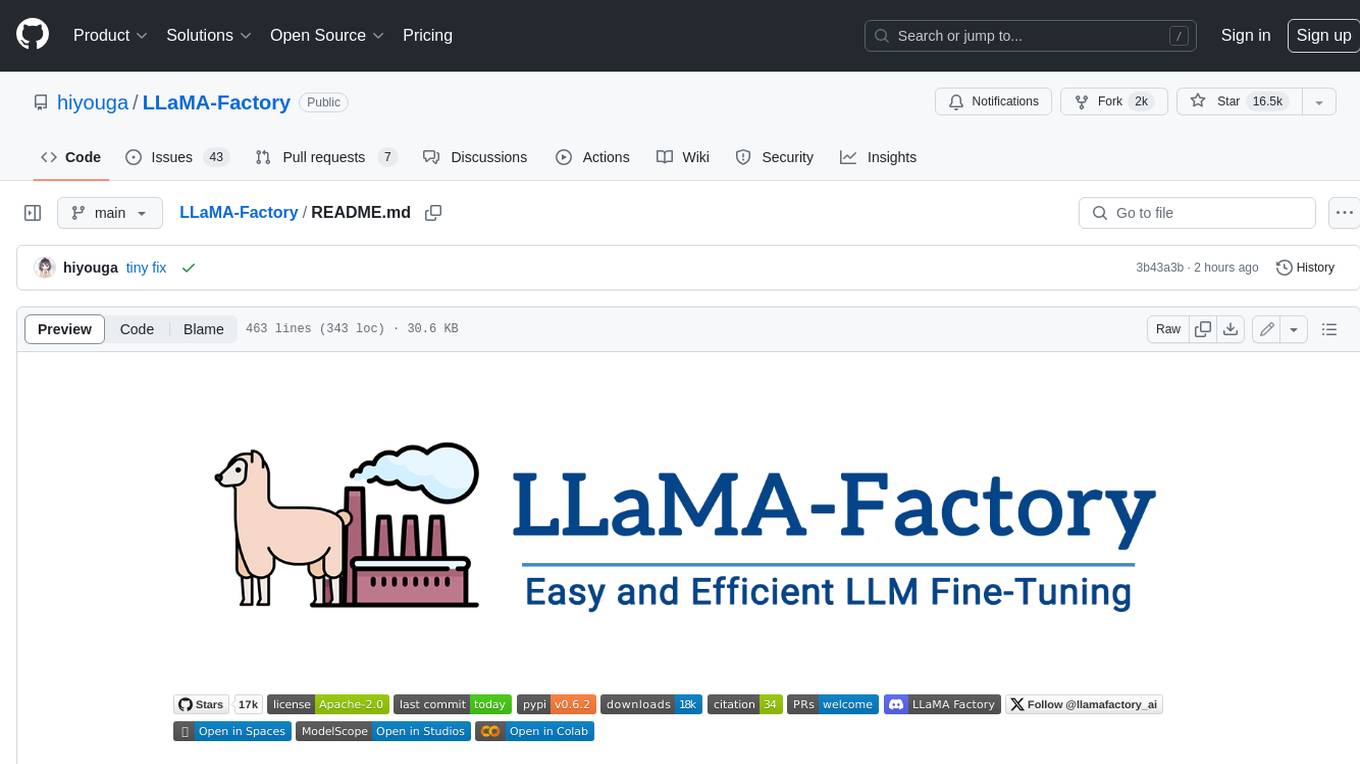
LLaMA-Factory
LLaMA Factory is a unified framework for fine-tuning 100+ large language models (LLMs) with various methods, including pre-training, supervised fine-tuning, reward modeling, PPO, DPO and ORPO. It features integrated algorithms like GaLore, BAdam, DoRA, LongLoRA, LLaMA Pro, LoRA+, LoftQ and Agent tuning, as well as practical tricks like FlashAttention-2, Unsloth, RoPE scaling, NEFTune and rsLoRA. LLaMA Factory provides experiment monitors like LlamaBoard, TensorBoard, Wandb, MLflow, etc., and supports faster inference with OpenAI-style API, Gradio UI and CLI with vLLM worker. Compared to ChatGLM's P-Tuning, LLaMA Factory's LoRA tuning offers up to 3.7 times faster training speed with a better Rouge score on the advertising text generation task. By leveraging 4-bit quantization technique, LLaMA Factory's QLoRA further improves the efficiency regarding the GPU memory.
For similar jobs
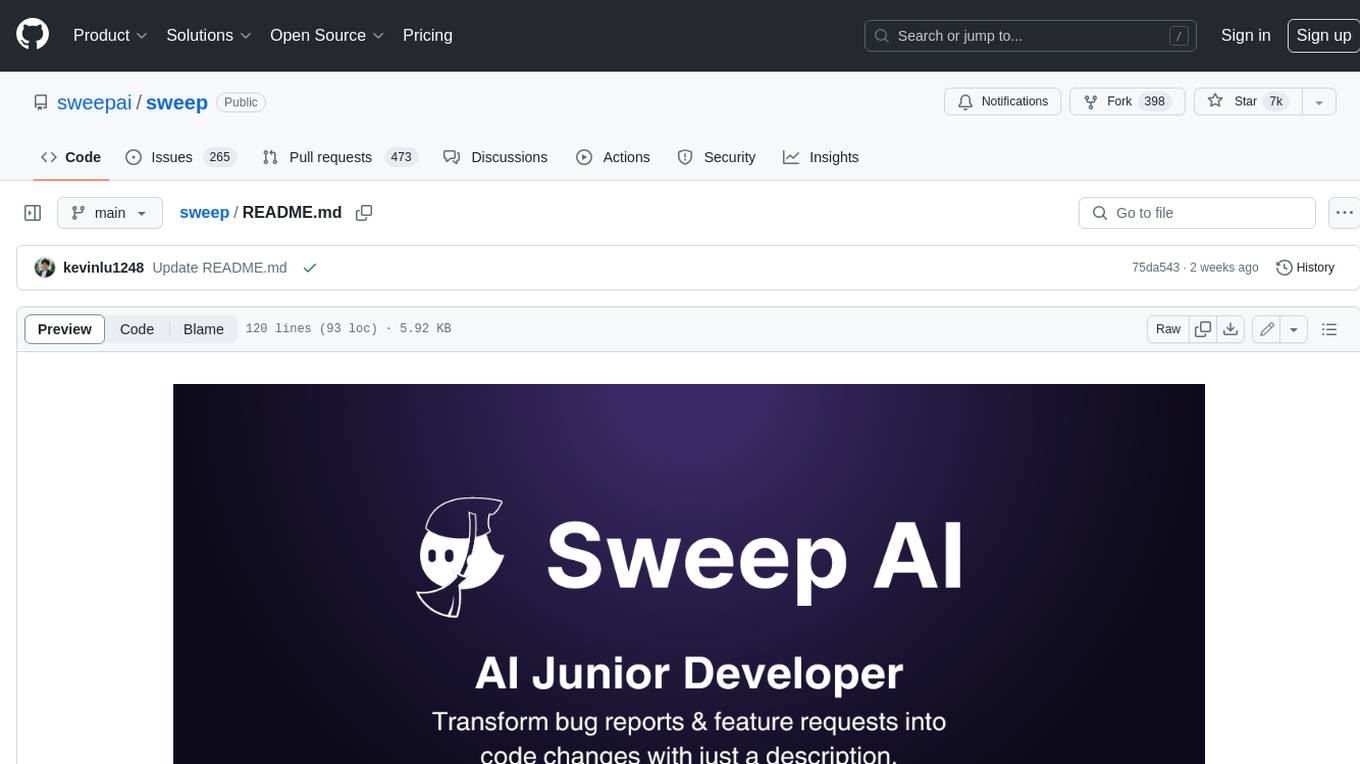
sweep
Sweep is an AI junior developer that turns bugs and feature requests into code changes. It automatically handles developer experience improvements like adding type hints and improving test coverage.
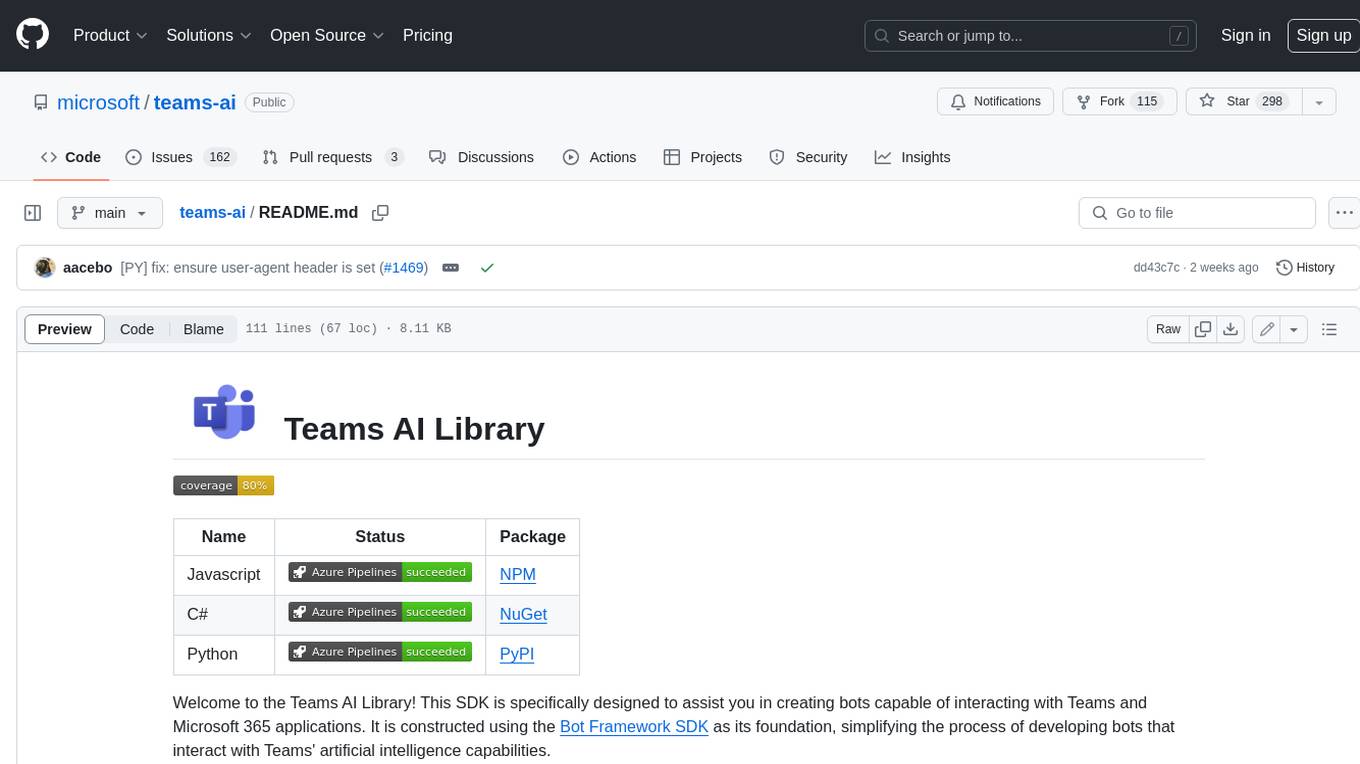
teams-ai
The Teams AI Library is a software development kit (SDK) that helps developers create bots that can interact with Teams and Microsoft 365 applications. It is built on top of the Bot Framework SDK and simplifies the process of developing bots that interact with Teams' artificial intelligence capabilities. The SDK is available for JavaScript/TypeScript, .NET, and Python.
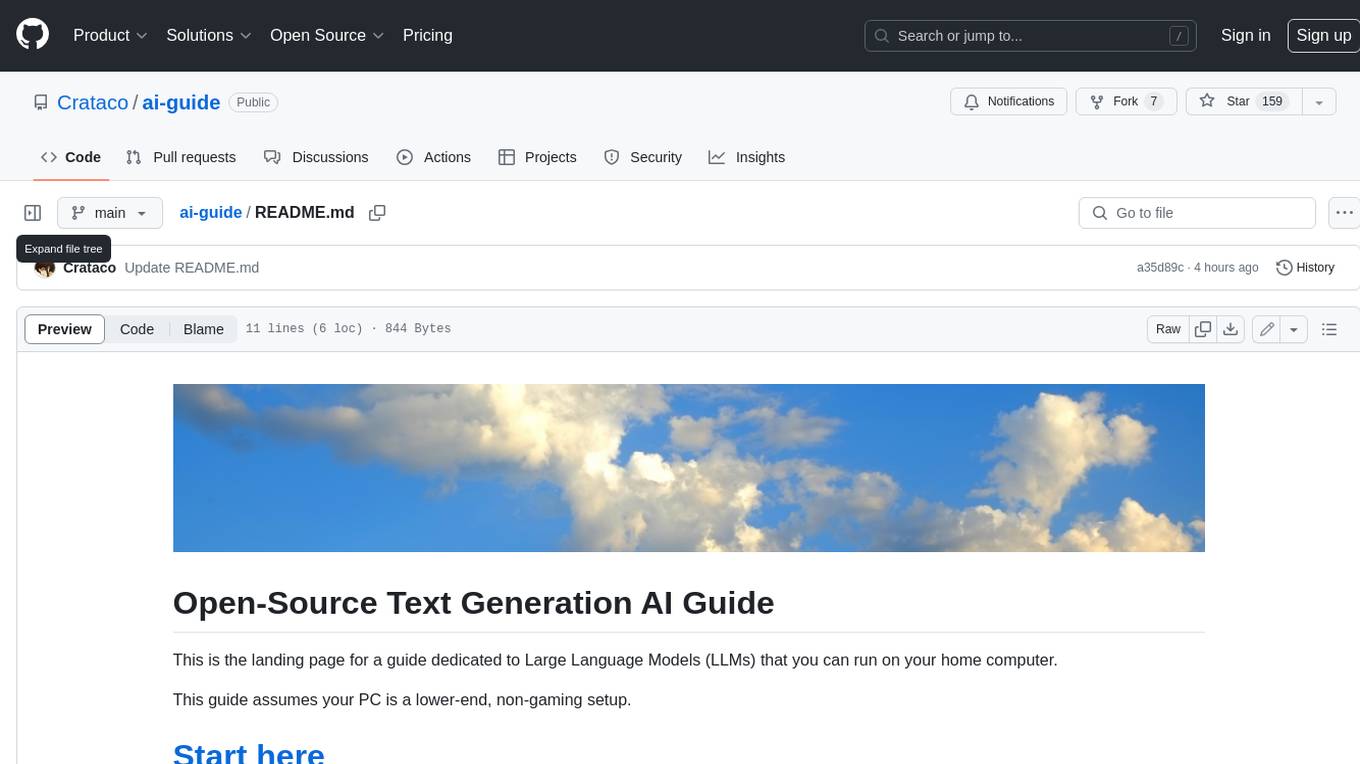
ai-guide
This guide is dedicated to Large Language Models (LLMs) that you can run on your home computer. It assumes your PC is a lower-end, non-gaming setup.
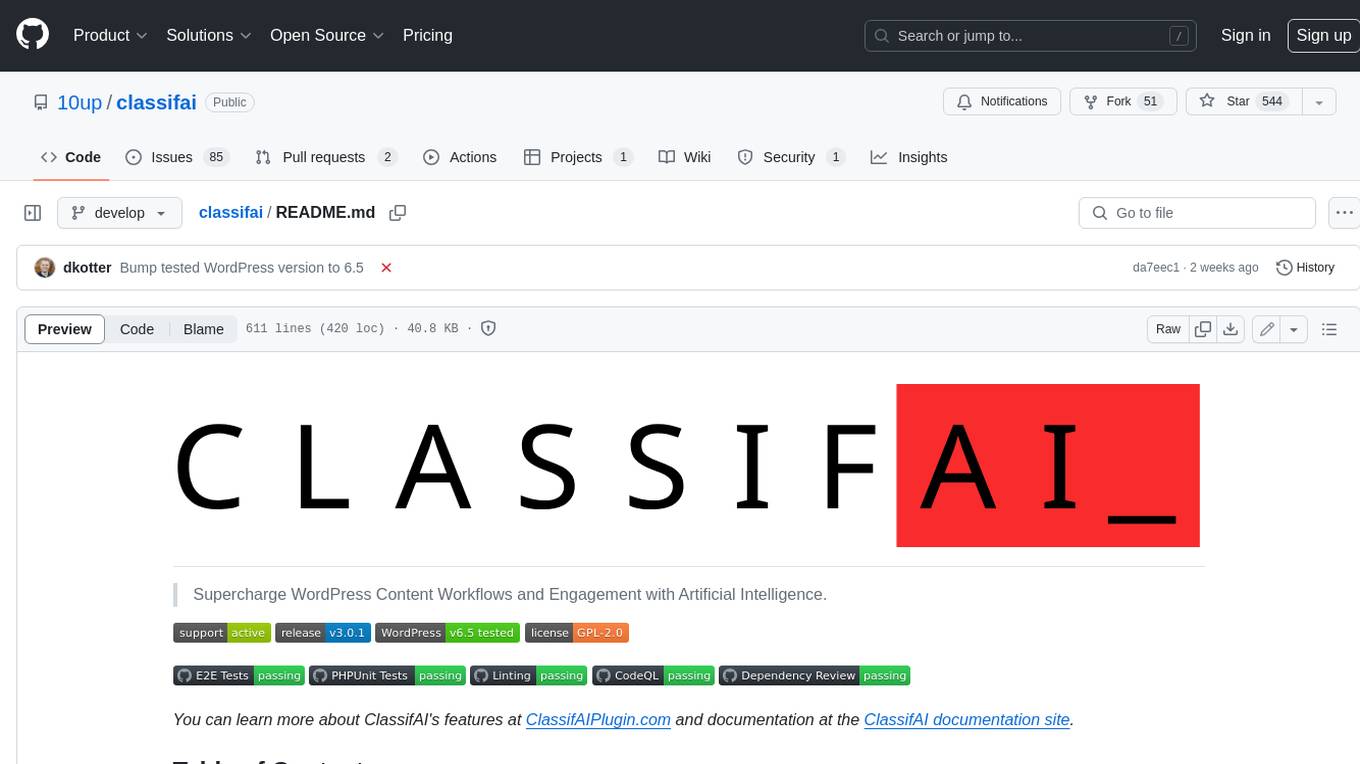
classifai
Supercharge WordPress Content Workflows and Engagement with Artificial Intelligence. Tap into leading cloud-based services like OpenAI, Microsoft Azure AI, Google Gemini and IBM Watson to augment your WordPress-powered websites. Publish content faster while improving SEO performance and increasing audience engagement. ClassifAI integrates Artificial Intelligence and Machine Learning technologies to lighten your workload and eliminate tedious tasks, giving you more time to create original content that matters.
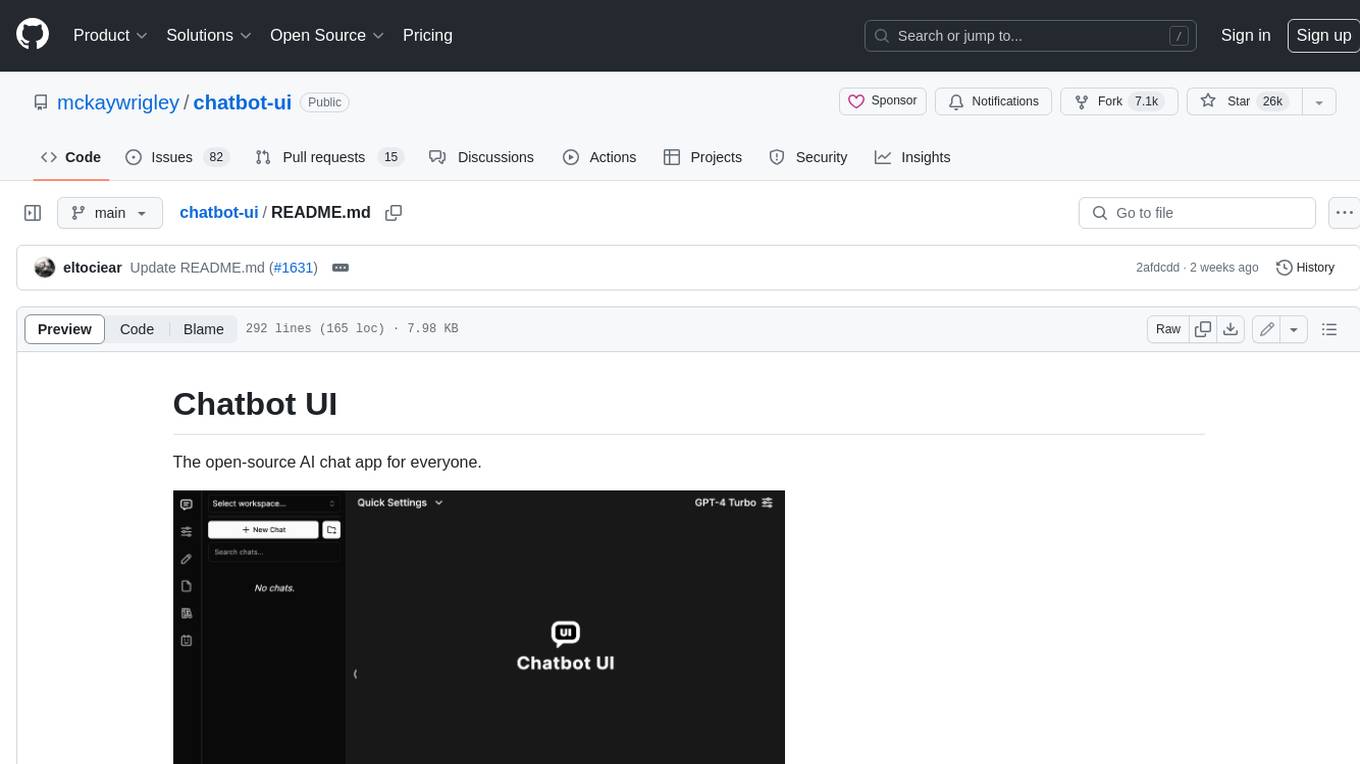
chatbot-ui
Chatbot UI is an open-source AI chat app that allows users to create and deploy their own AI chatbots. It is easy to use and can be customized to fit any need. Chatbot UI is perfect for businesses, developers, and anyone who wants to create a chatbot.
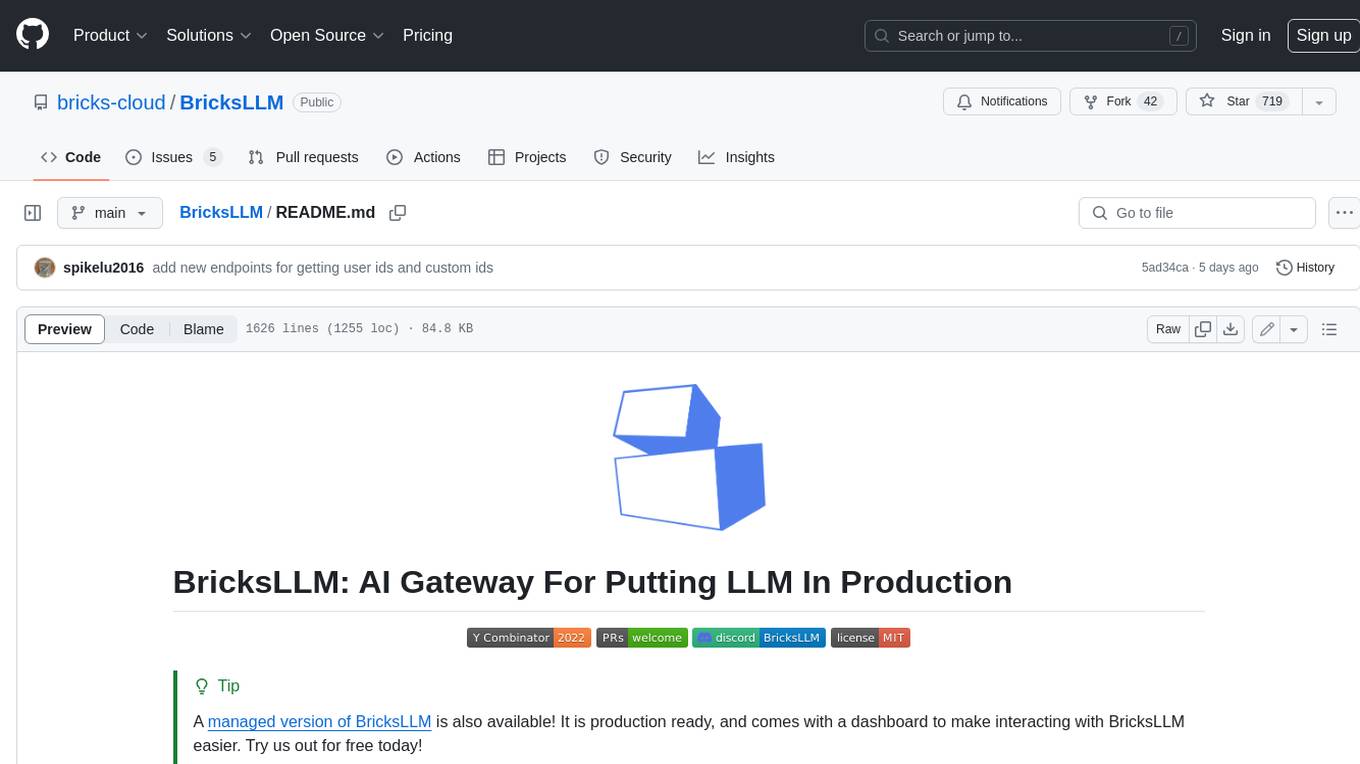
BricksLLM
BricksLLM is a cloud native AI gateway written in Go. Currently, it provides native support for OpenAI, Anthropic, Azure OpenAI and vLLM. BricksLLM aims to provide enterprise level infrastructure that can power any LLM production use cases. Here are some use cases for BricksLLM: * Set LLM usage limits for users on different pricing tiers * Track LLM usage on a per user and per organization basis * Block or redact requests containing PIIs * Improve LLM reliability with failovers, retries and caching * Distribute API keys with rate limits and cost limits for internal development/production use cases * Distribute API keys with rate limits and cost limits for students
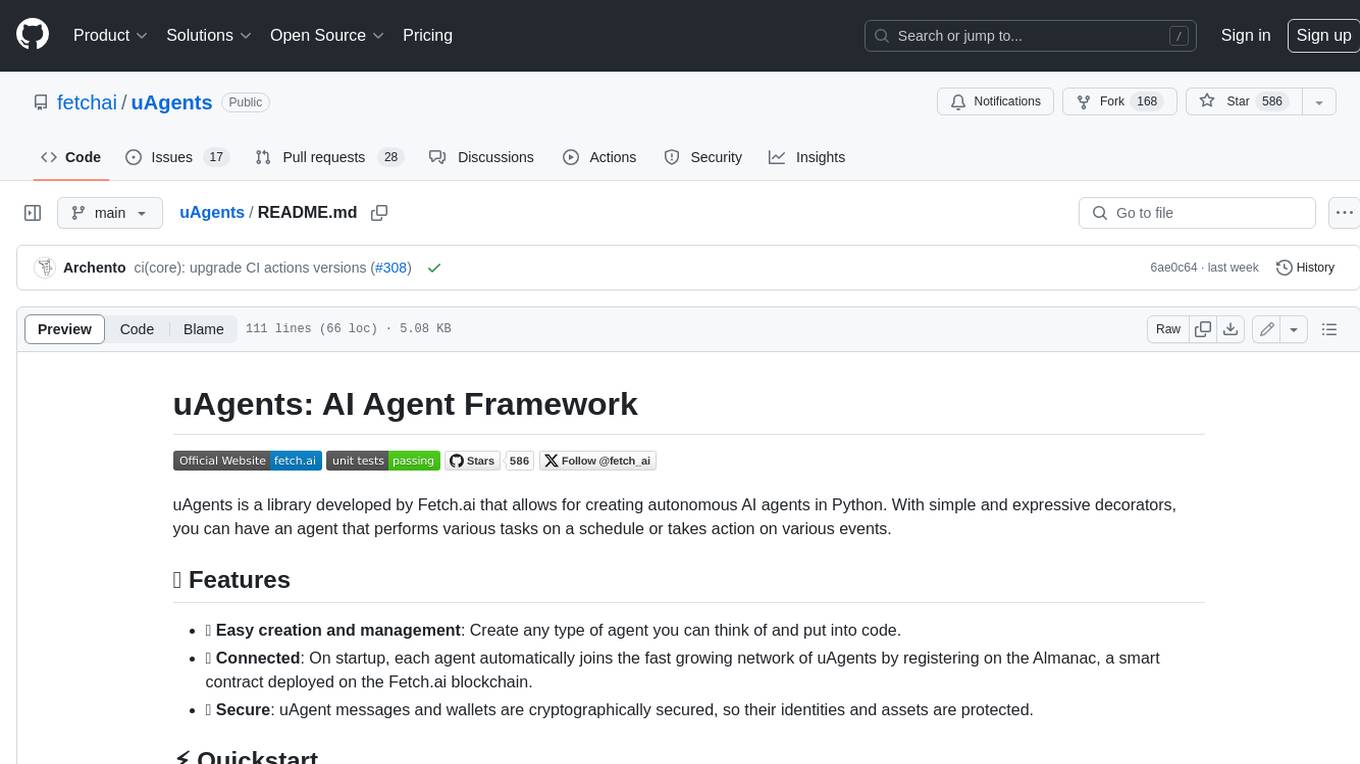
uAgents
uAgents is a Python library developed by Fetch.ai that allows for the creation of autonomous AI agents. These agents can perform various tasks on a schedule or take action on various events. uAgents are easy to create and manage, and they are connected to a fast-growing network of other uAgents. They are also secure, with cryptographically secured messages and wallets.
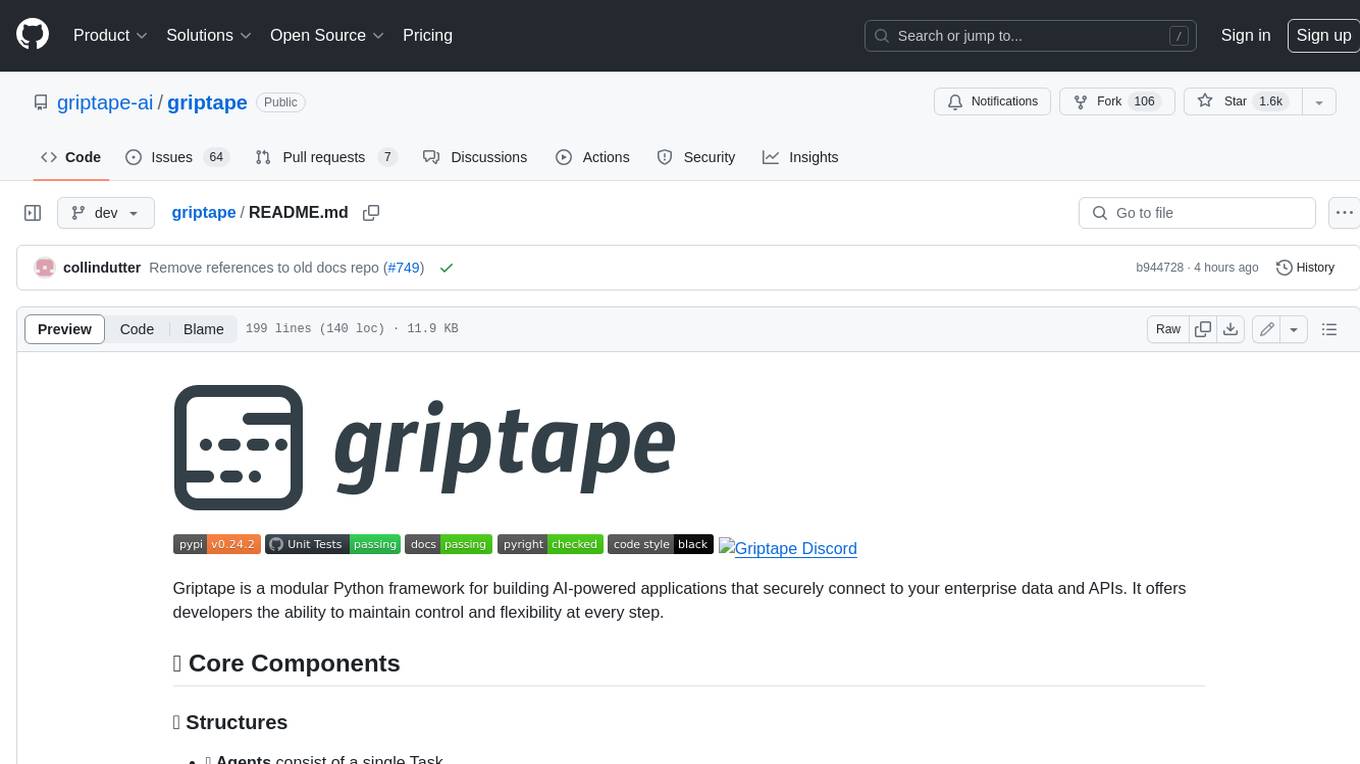
griptape
Griptape is a modular Python framework for building AI-powered applications that securely connect to your enterprise data and APIs. It offers developers the ability to maintain control and flexibility at every step. Griptape's core components include Structures (Agents, Pipelines, and Workflows), Tasks, Tools, Memory (Conversation Memory, Task Memory, and Meta Memory), Drivers (Prompt and Embedding Drivers, Vector Store Drivers, Image Generation Drivers, Image Query Drivers, SQL Drivers, Web Scraper Drivers, and Conversation Memory Drivers), Engines (Query Engines, Extraction Engines, Summary Engines, Image Generation Engines, and Image Query Engines), and additional components (Rulesets, Loaders, Artifacts, Chunkers, and Tokenizers). Griptape enables developers to create AI-powered applications with ease and efficiency.