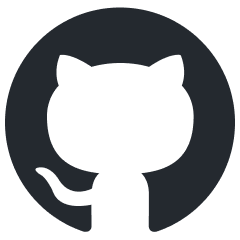
LMCache
10x Faster Long-Context LLM By Smart KV Cache Optimizations
Stars: 515
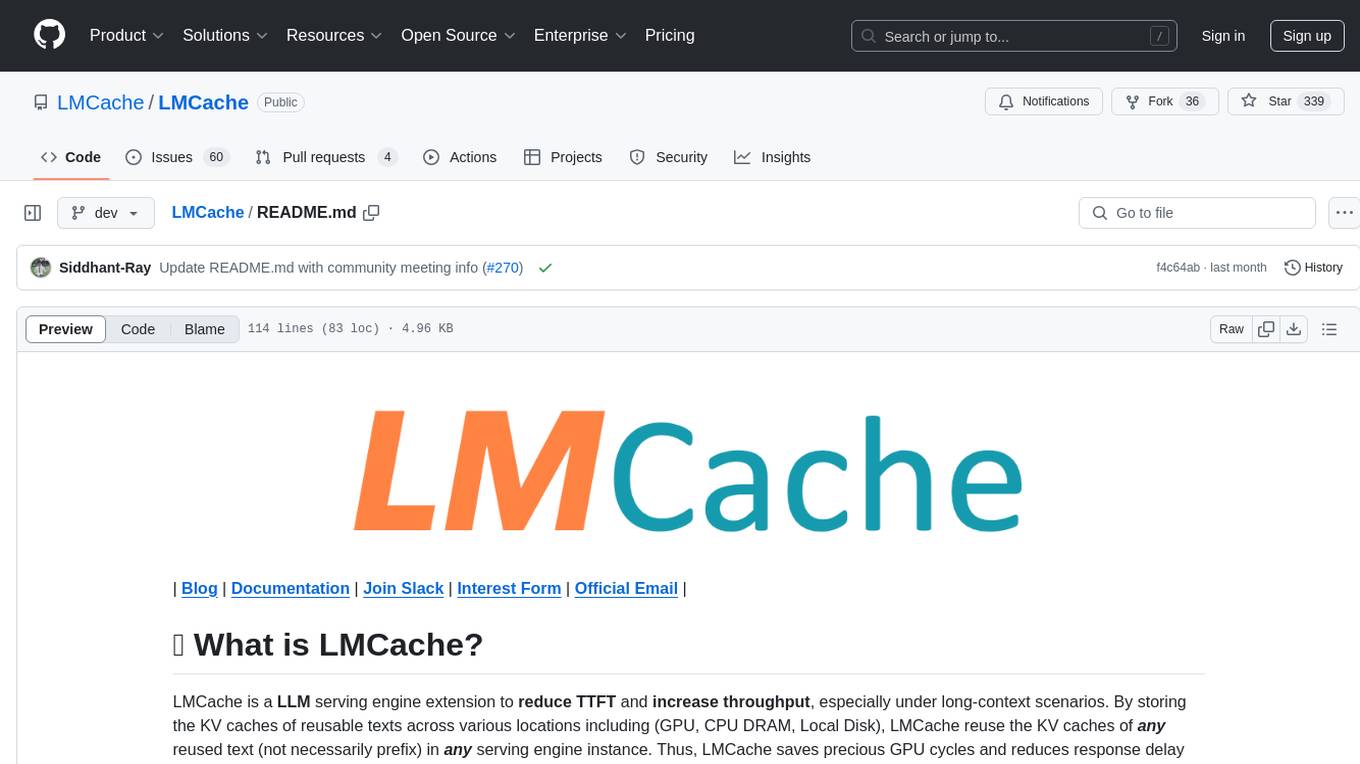
LMCache is a serving engine extension designed to reduce time to first token (TTFT) and increase throughput, particularly in long-context scenarios. It stores key-value caches of reusable texts across different locations like GPU, CPU DRAM, and Local Disk, allowing the reuse of any text in any serving engine instance. By combining LMCache with vLLM, significant delay savings and GPU cycle reduction are achieved in various large language model (LLM) use cases, such as multi-round question answering and retrieval-augmented generation (RAG). LMCache provides integration with the latest vLLM version, offering both online serving and offline inference capabilities. It supports sharing key-value caches across multiple vLLM instances and aims to provide stable support for non-prefix key-value caches along with user and developer documentation.
README:
| Blog | Documentation | Join Slack | Interest Form | Official Email |
LMCache is a LLM serving engine extension to reduce TTFT and increase throughput, especially under long-context scenarios. By storing the KV caches of reusable texts across various locations including (GPU, CPU DRAM, Local Disk), LMCache reuse the KV caches of any reused text (not necessarily prefix) in any serving engine instance. Thus, LMCache saves precious GPU cycles and reduces response delay for users.
By combining LMCache with vLLM, LMCaches achieves 3-10x delay savings and GPU cycle reduction in many LLM use cases, including multi-round QA and RAG.
Try LMCache with pre-built vllm docker images here.
LMCache provides the integration to the latest vLLM (0.6.2). To install LMCache, use the following command:
# requires python >= 3.10 and nvcc >= 12.1
pip install lmcache lmcache_vllm
LMCache has the same interface as vLLM (both online serving and offline inference). To use the online serving, you can start an OpenAI API-compatible vLLM server with LMCache via:
lmcache_vllm serve lmsys/longchat-7b-16k --gpu-memory-utilization 0.8
To use vLLM's offline inference with LMCache, just simply add lmcache_vllm
before the import to vLLM components. For example
import lmcache_vllm.vllm as vllm
from lmcache_vllm.vllm import LLM
More detailed documentation will be available soon.
LMCache supports sharing KV across different vLLM instances by the lmcache.server
module. Here is a quick guide:
# Start lmcache server
lmcache_server localhost 65432
Then, start two vLLM instances with the LMCache config file
wget https://raw.githubusercontent.com/LMCache/LMCache/refs/heads/dev/examples/example.yaml
# start the first vLLM instance
LMCACHE_CONFIG_FILE=example.yaml CUDA_VISIBLE_DEVICES=0 lmcache_vllm serve lmsys/longchat-7b-16k --gpu-memory-utilization 0.8 --port 8000
# start the second vLLM instance
LMCACHE_CONFIG_FILE=example.yaml CUDA_VISIBLE_DEVICES=1 lmcache_vllm serve lmsys/longchat-7b-16k --gpu-memory-utilization 0.8 --port 8001
We also provide multiple docker-based demos at πLMCache-demos repo. The demos cover the following use cases:
- Share KV caches across multiple serving engines (πlink)
- Loading non-prefix KV caches for RAG (πlink)
Fill out the interest form and our team will reach out to you! https://forms.gle/mQfQDUXbKfp2St1z7
- [x] First release of LMCache
- [x] Support installation through pip install and integrate with latest vLLM
- [ ] Stable support for non-prefix KV caches
- [ ] User and developer documentation
Our blog posts and documentations are available online
- π Meeting link - https://uchicago.zoom.us/j/91454186439?pwd=Qu3IMJH7c83Qbg9hHsXZ3BxzLaEFoF.1
- π Community Meeting Document - https://docs.google.com/document/d/1SnCKnB2UFBUyPhIpL9zzdZsn_hGp50spoZue-2SoxJY/edit?usp=sharing
- ποΈ Calendar - https://calendar.app.google/rsu7Xgq4y4y5YuDj7
If you use LMCache for your research, please cite our papers:
@inproceedings{liu2024cachegen,
title={Cachegen: Kv cache compression and streaming for fast large language model serving},
author={Liu, Yuhan and Li, Hanchen and Cheng, Yihua and Ray, Siddhant and Huang, Yuyang and Zhang, Qizheng and Du, Kuntai and Yao, Jiayi and Lu, Shan and Ananthanarayanan, Ganesh and others},
booktitle={Proceedings of the ACM SIGCOMM 2024 Conference},
pages={38--56},
year={2024}
}
@article{cheng2024large,
title={Do Large Language Models Need a Content Delivery Network?},
author={Cheng, Yihua and Du, Kuntai and Yao, Jiayi and Jiang, Junchen},
journal={arXiv preprint arXiv:2409.13761},
year={2024}
}
@article{yao2024cacheblend,
title={CacheBlend: Fast Large Language Model Serving with Cached Knowledge Fusion},
author={Yao, Jiayi and Li, Hanchen and Liu, Yuhan and Ray, Siddhant and Cheng, Yihua and Zhang, Qizheng and Du, Kuntai and Lu, Shan and Jiang, Junchen},
journal={arXiv preprint arXiv:2405.16444},
year={2024}
}
For Tasks:
Click tags to check more tools for each tasksFor Jobs:
Alternative AI tools for LMCache
Similar Open Source Tools
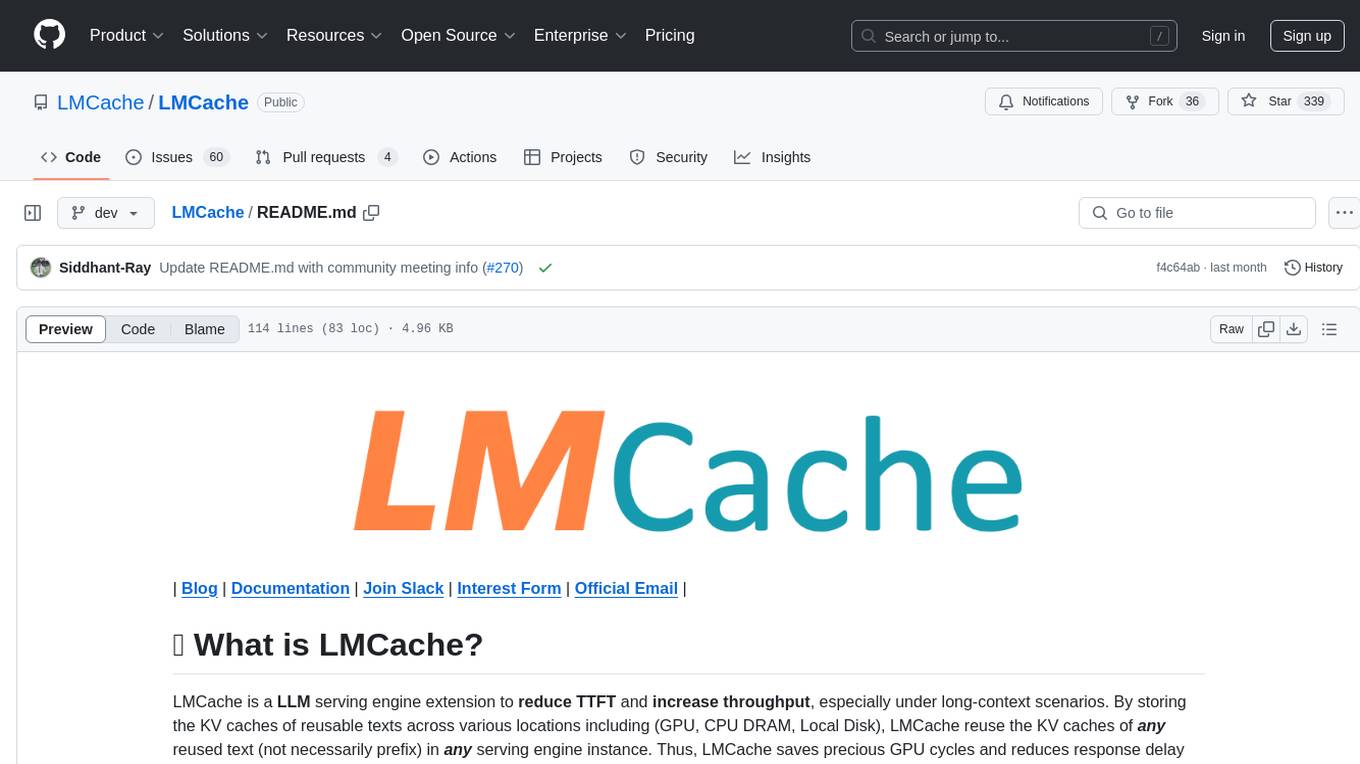
LMCache
LMCache is a serving engine extension designed to reduce time to first token (TTFT) and increase throughput, particularly in long-context scenarios. It stores key-value caches of reusable texts across different locations like GPU, CPU DRAM, and Local Disk, allowing the reuse of any text in any serving engine instance. By combining LMCache with vLLM, significant delay savings and GPU cycle reduction are achieved in various large language model (LLM) use cases, such as multi-round question answering and retrieval-augmented generation (RAG). LMCache provides integration with the latest vLLM version, offering both online serving and offline inference capabilities. It supports sharing key-value caches across multiple vLLM instances and aims to provide stable support for non-prefix key-value caches along with user and developer documentation.
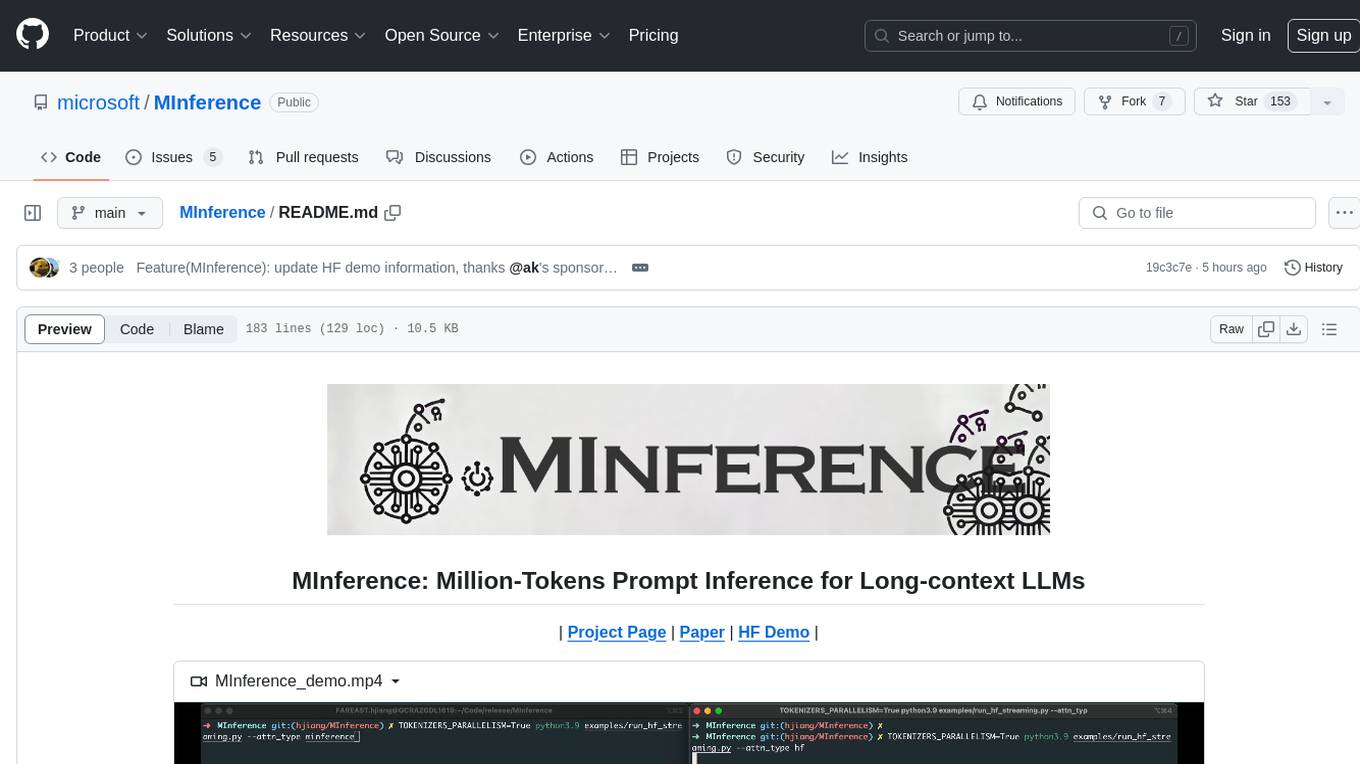
MInference
MInference is a tool designed to accelerate pre-filling for long-context Language Models (LLMs) by leveraging dynamic sparse attention. It achieves up to a 10x speedup for pre-filling on an A100 while maintaining accuracy. The tool supports various decoding LLMs, including LLaMA-style models and Phi models, and provides custom kernels for attention computation. MInference is useful for researchers and developers working with large-scale language models who aim to improve efficiency without compromising accuracy.
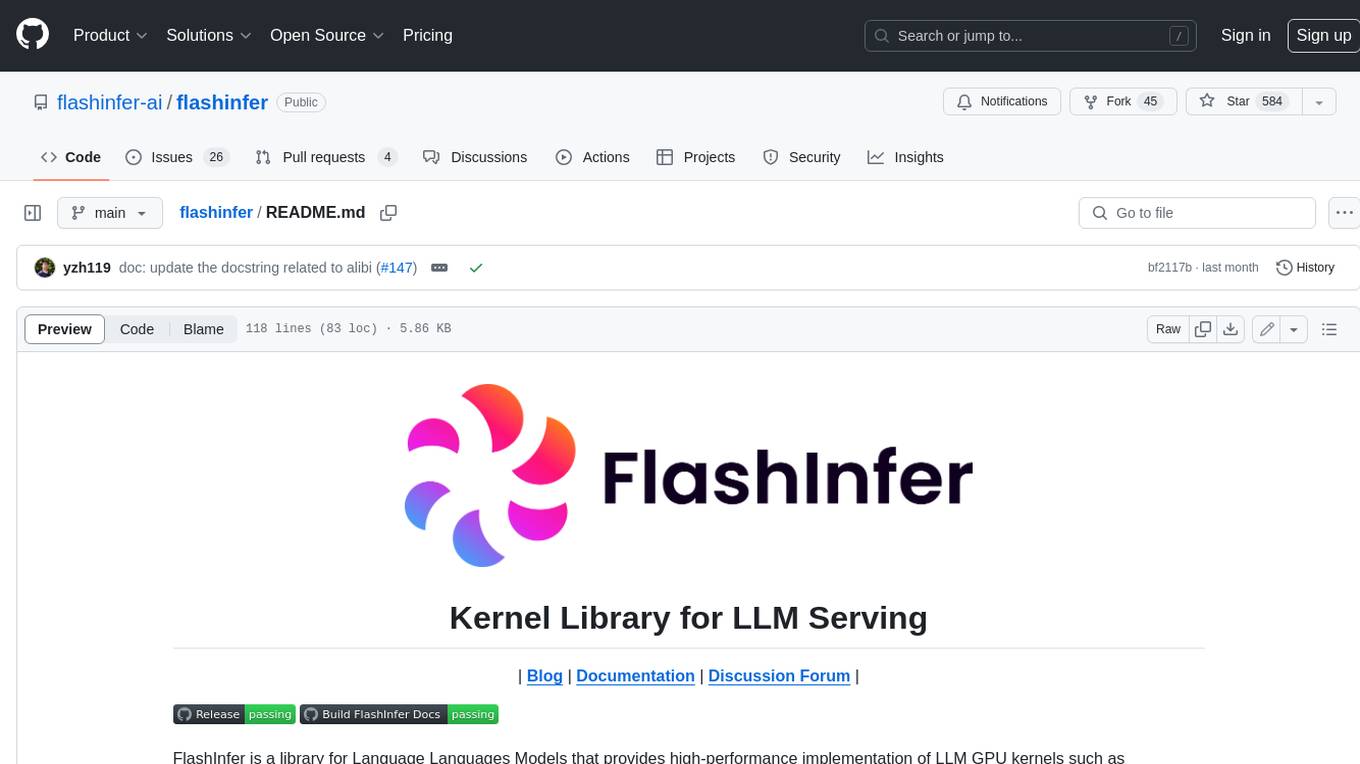
flashinfer
FlashInfer is a library for Language Languages Models that provides high-performance implementation of LLM GPU kernels such as FlashAttention, PageAttention and LoRA. FlashInfer focus on LLM serving and inference, and delivers state-the-art performance across diverse scenarios.
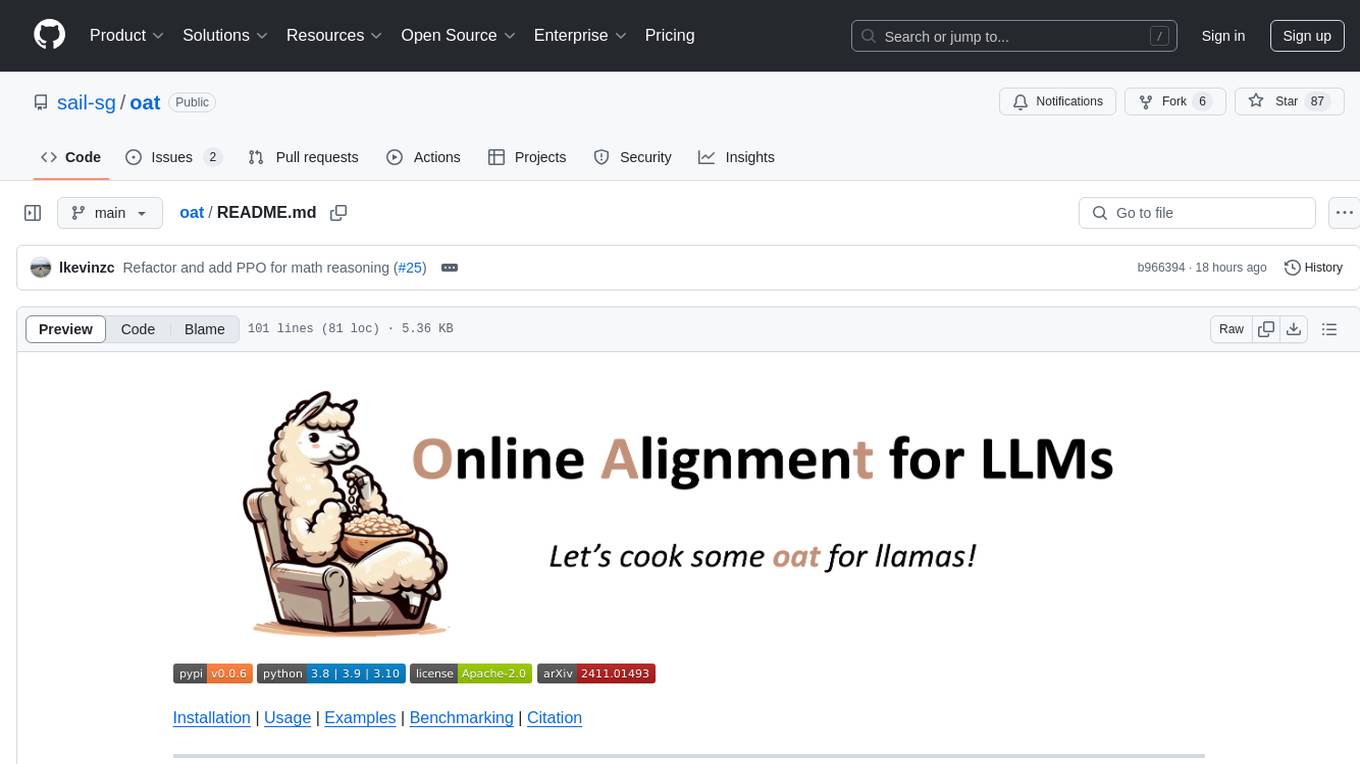
oat
Oat is a simple and efficient framework for running online LLM alignment algorithms. It implements a distributed Actor-Learner-Oracle architecture, with components optimized using state-of-the-art tools. Oat simplifies the experimental pipeline of LLM alignment by serving an Oracle online for preference data labeling and model evaluation. It provides a variety of oracles for simulating feedback and supports verifiable rewards. Oat's modular structure allows for easy inheritance and modification of classes, enabling rapid prototyping and experimentation with new algorithms. The framework implements cutting-edge online algorithms like PPO for math reasoning and various online exploration algorithms.
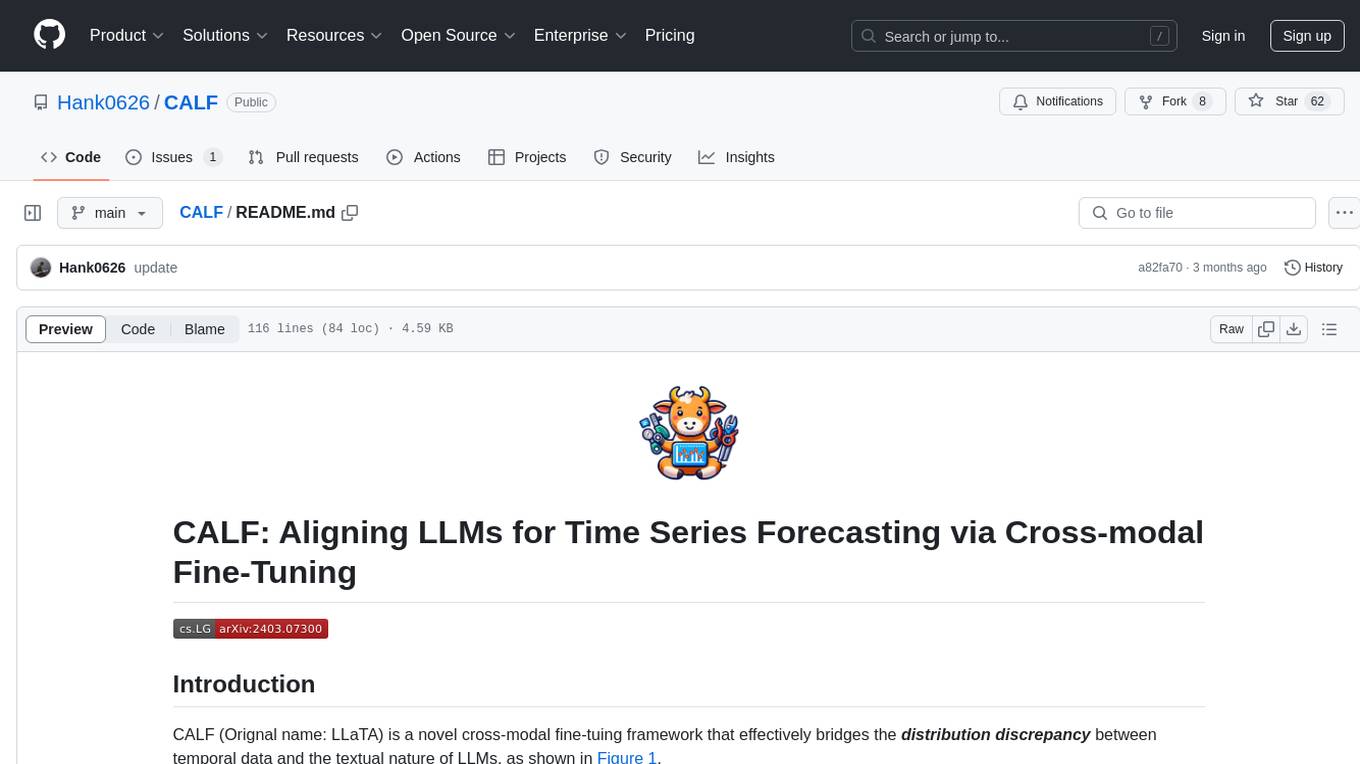
CALF
CALF (LLaTA) is a cross-modal fine-tuning framework that bridges the distribution discrepancy between temporal data and the textual nature of LLMs. It introduces three cross-modal fine-tuning techniques: Cross-Modal Match Module, Feature Regularization Loss, and Output Consistency Loss. The framework aligns time series and textual inputs, ensures effective weight updates, and maintains consistent semantic context for time series data. CALF provides scripts for long-term and short-term forecasting, requires Python 3.9, and utilizes word token embeddings for model training.
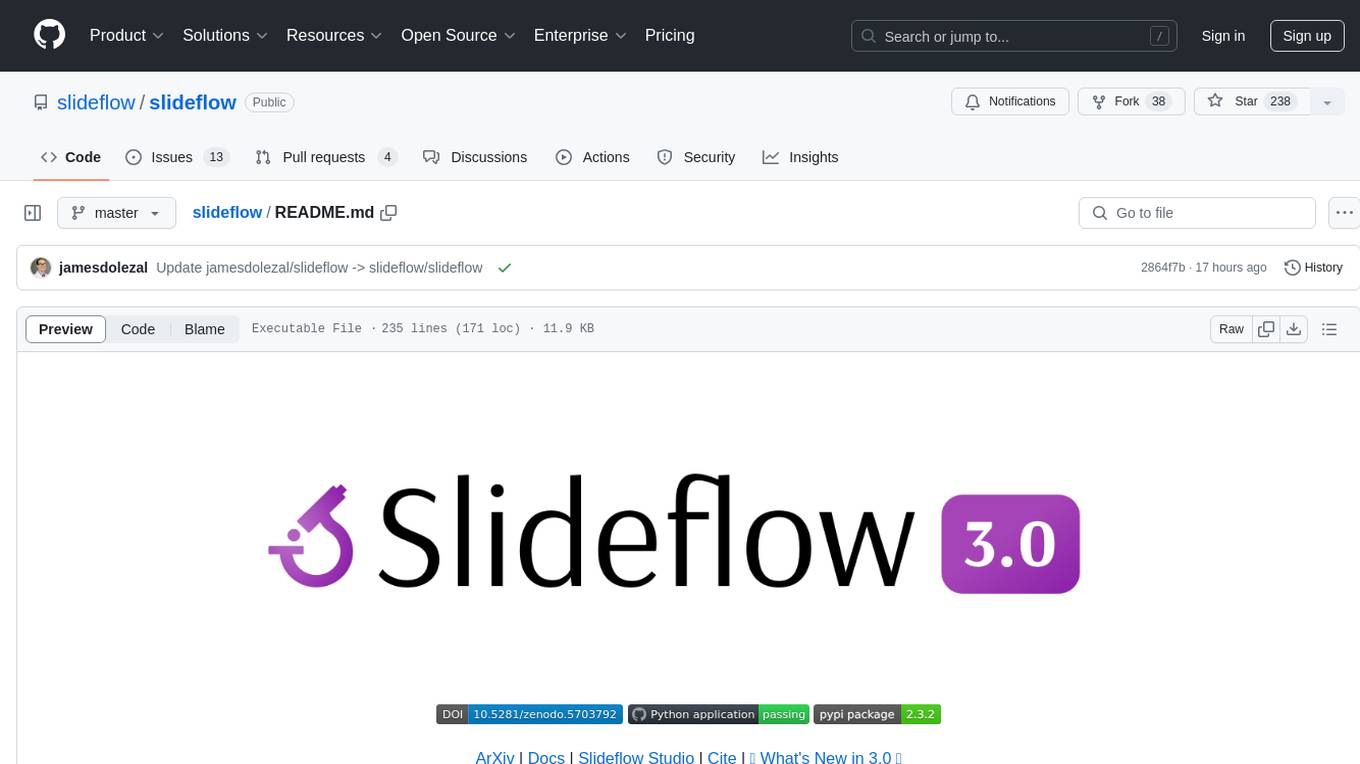
slideflow
Slideflow is a deep learning library for digital pathology, offering a user-friendly interface for model development. It is designed for medical researchers and AI enthusiasts, providing an accessible platform for developing state-of-the-art pathology models. Slideflow offers customizable training pipelines, robust slide processing and stain normalization toolkit, support for weakly-supervised or strongly-supervised labels, built-in foundation models, multiple-instance learning, self-supervised learning, generative adversarial networks, explainability tools, layer activation analysis tools, uncertainty quantification, interactive user interface for model deployment, and more. It supports both PyTorch and Tensorflow, with optional support for Libvips for slide reading. Slideflow can be installed via pip, Docker container, or from source, and includes non-commercial add-ons for additional tools and pretrained models. It allows users to create projects, extract tiles from slides, train models, and provides evaluation tools like heatmaps and mosaic maps.
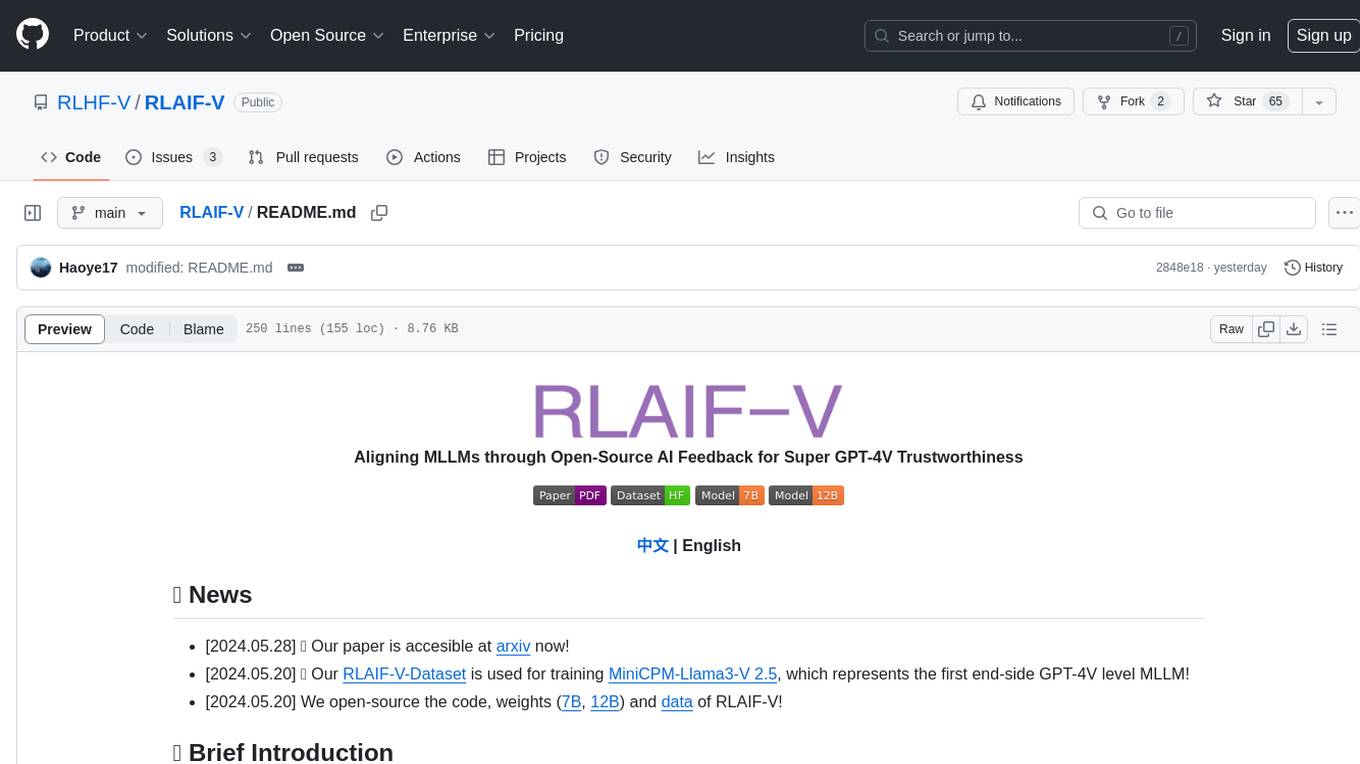
RLAIF-V
RLAIF-V is a novel framework that aligns MLLMs in a fully open-source paradigm for super GPT-4V trustworthiness. It maximally exploits open-source feedback from high-quality feedback data and online feedback learning algorithm. Notable features include achieving super GPT-4V trustworthiness in both generative and discriminative tasks, using high-quality generalizable feedback data to reduce hallucination of different MLLMs, and exhibiting better learning efficiency and higher performance through iterative alignment.
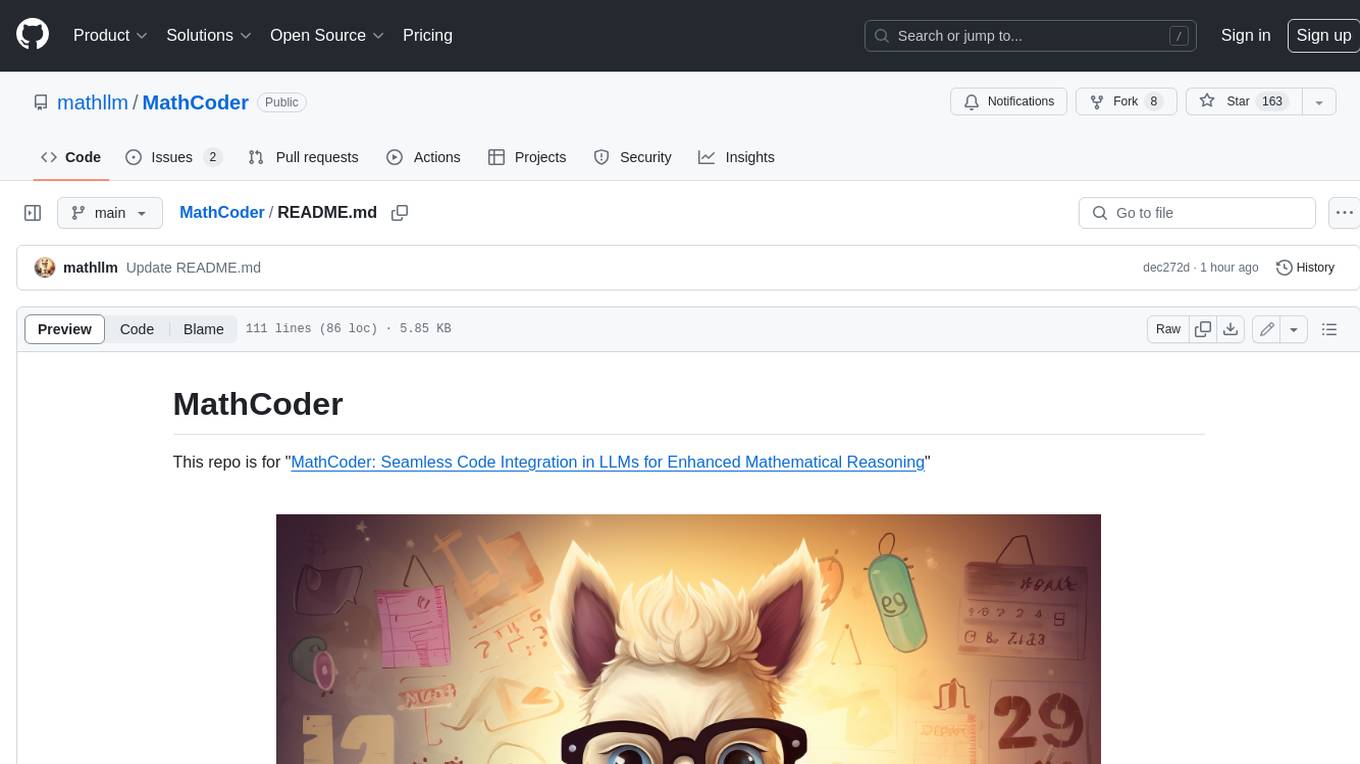
MathCoder
MathCoder is a repository focused on enhancing mathematical reasoning by fine-tuning open-source language models to use code for modeling and deriving math equations. It introduces MathCodeInstruct dataset with solutions interleaving natural language, code, and execution results. The repository provides MathCoder models capable of generating code-based solutions for challenging math problems, achieving state-of-the-art scores on MATH and GSM8K datasets. It offers tools for model deployment, inference, and evaluation, along with a citation for referencing the work.
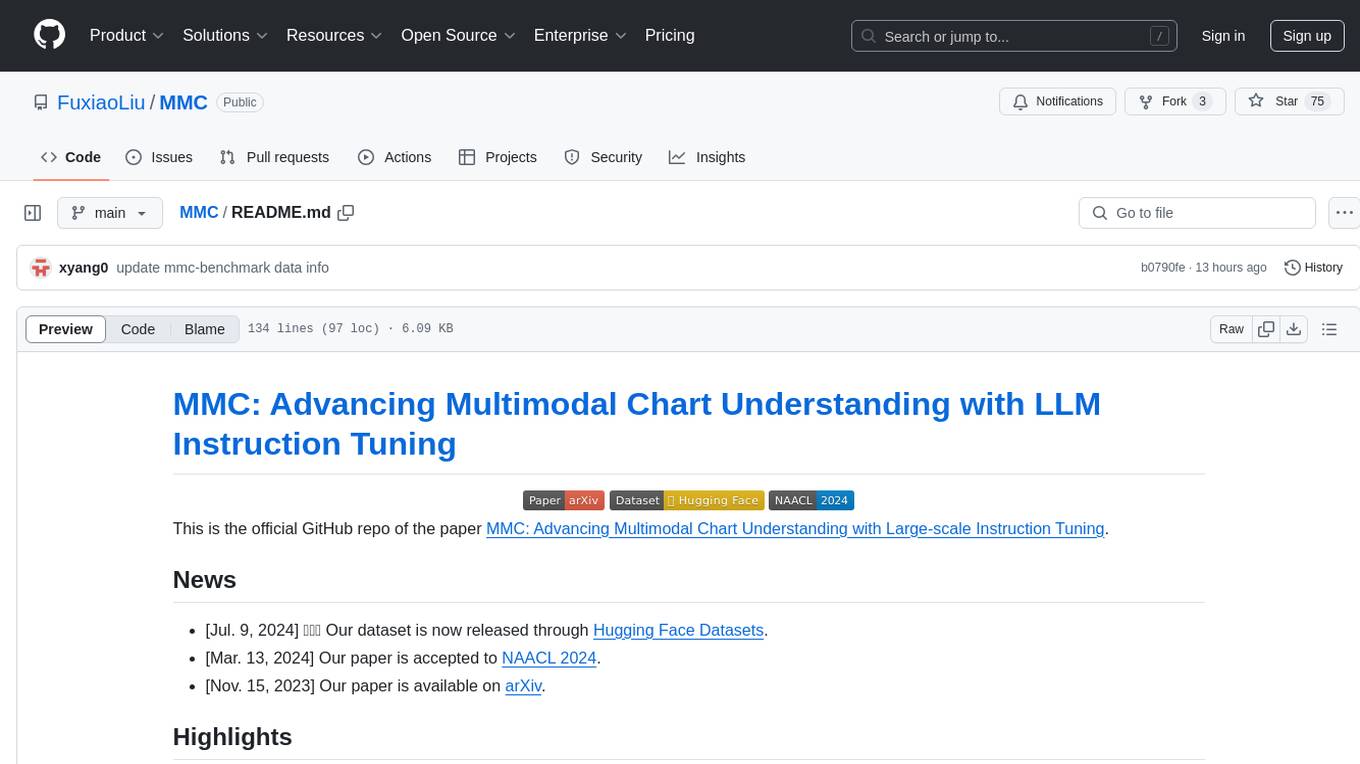
MMC
This repository, MMC, focuses on advancing multimodal chart understanding through large-scale instruction tuning. It introduces a dataset supporting various tasks and chart types, a benchmark for evaluating reasoning capabilities over charts, and an assistant achieving state-of-the-art performance on chart QA benchmarks. The repository provides data for chart-text alignment, benchmarking, and instruction tuning, along with existing datasets used in experiments. Additionally, it offers a Gradio demo for the MMCA model.
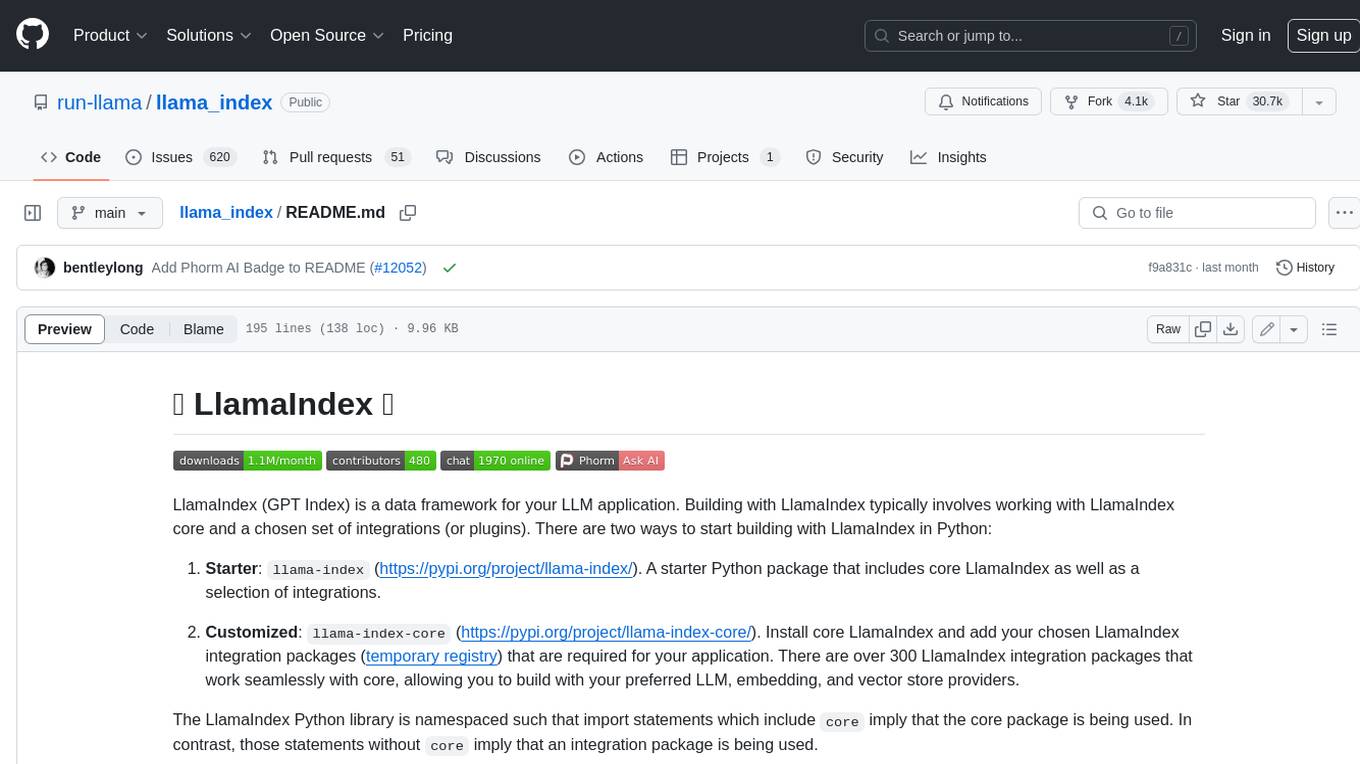
llama_index
LlamaIndex is a data framework for building LLM applications. It provides tools for ingesting, structuring, and querying data, as well as integrating with LLMs and other tools. LlamaIndex is designed to be easy to use for both beginner and advanced users, and it provides a comprehensive set of features for building LLM applications.
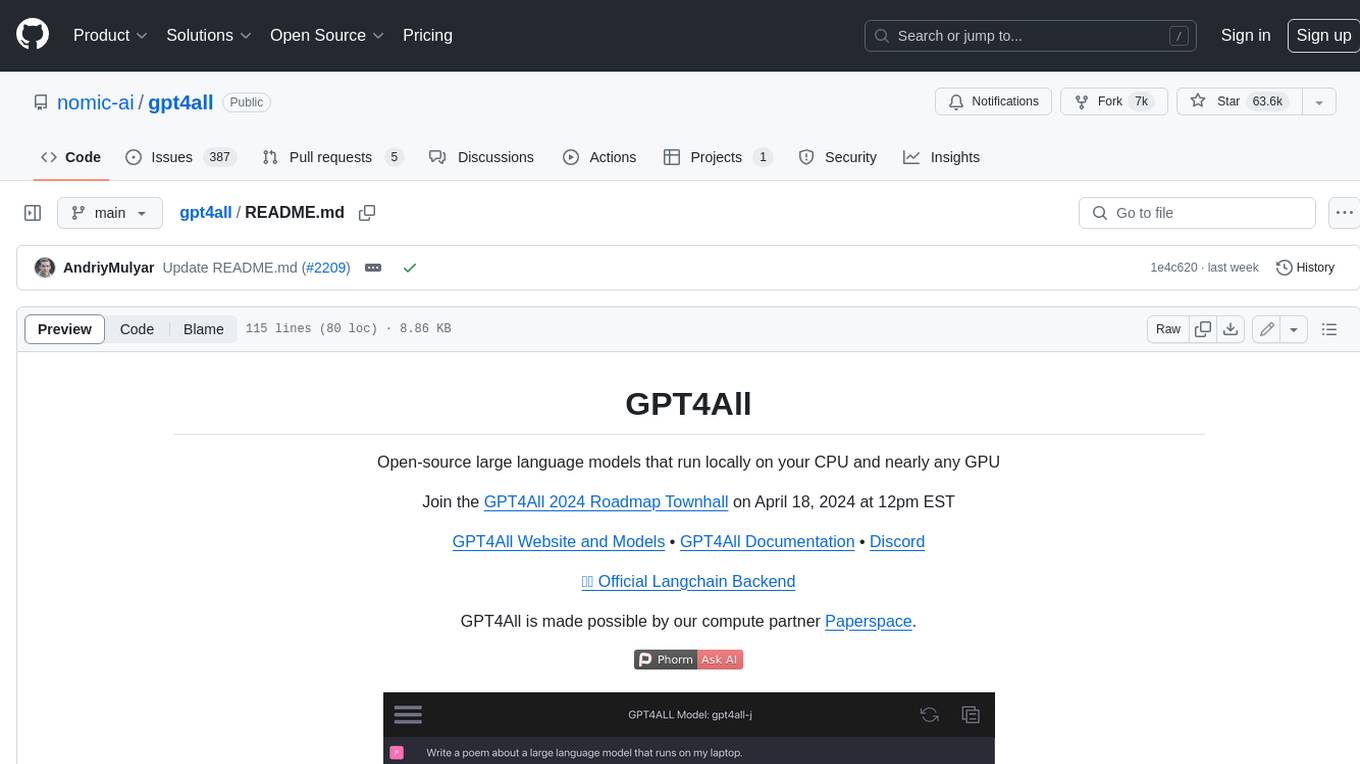
gpt4all
GPT4All is an ecosystem to run powerful and customized large language models that work locally on consumer grade CPUs and any GPU. Note that your CPU needs to support AVX or AVX2 instructions. Learn more in the documentation. A GPT4All model is a 3GB - 8GB file that you can download and plug into the GPT4All open-source ecosystem software. Nomic AI supports and maintains this software ecosystem to enforce quality and security alongside spearheading the effort to allow any person or enterprise to easily train and deploy their own on-edge large language models.
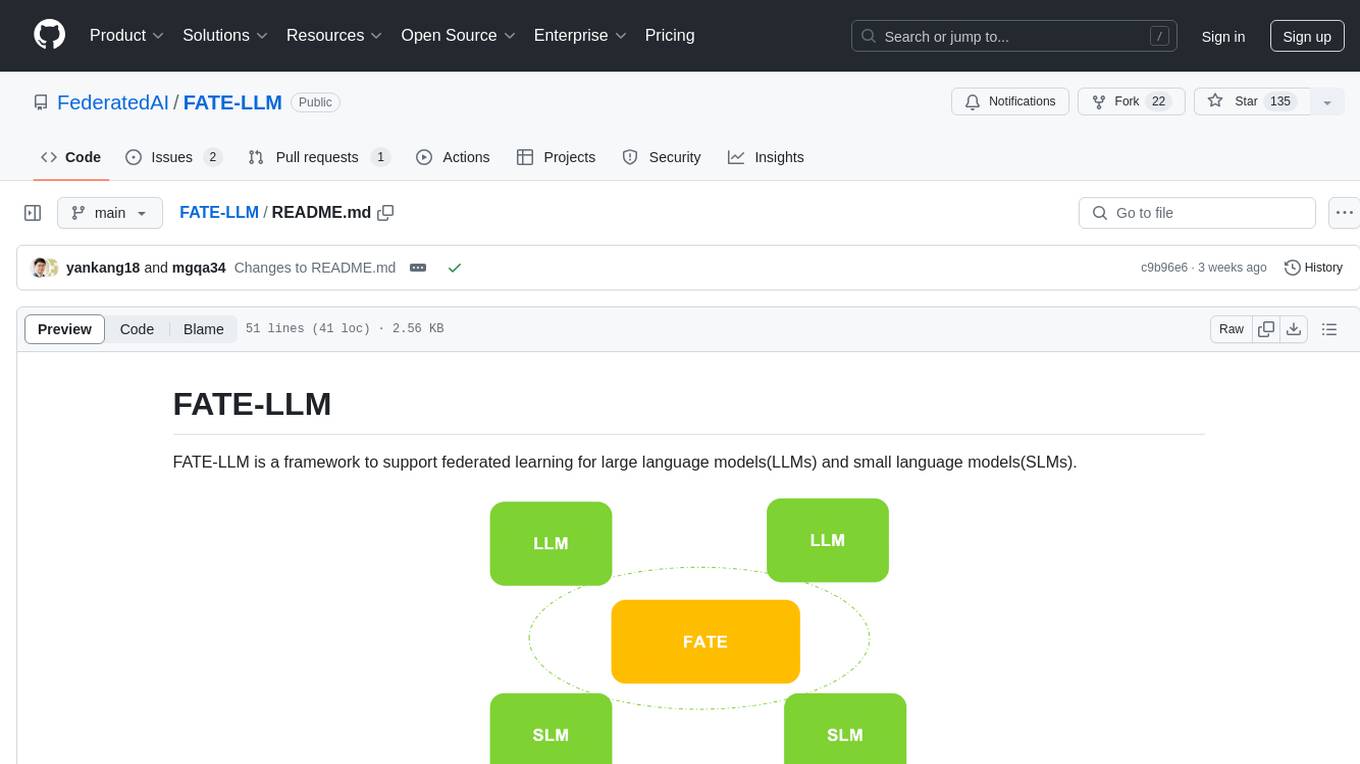
FATE-LLM
FATE-LLM is a framework supporting federated learning for large and small language models. It promotes training efficiency of federated LLMs using Parameter-Efficient methods, protects the IP of LLMs using FedIPR, and ensures data privacy during training and inference through privacy-preserving mechanisms.
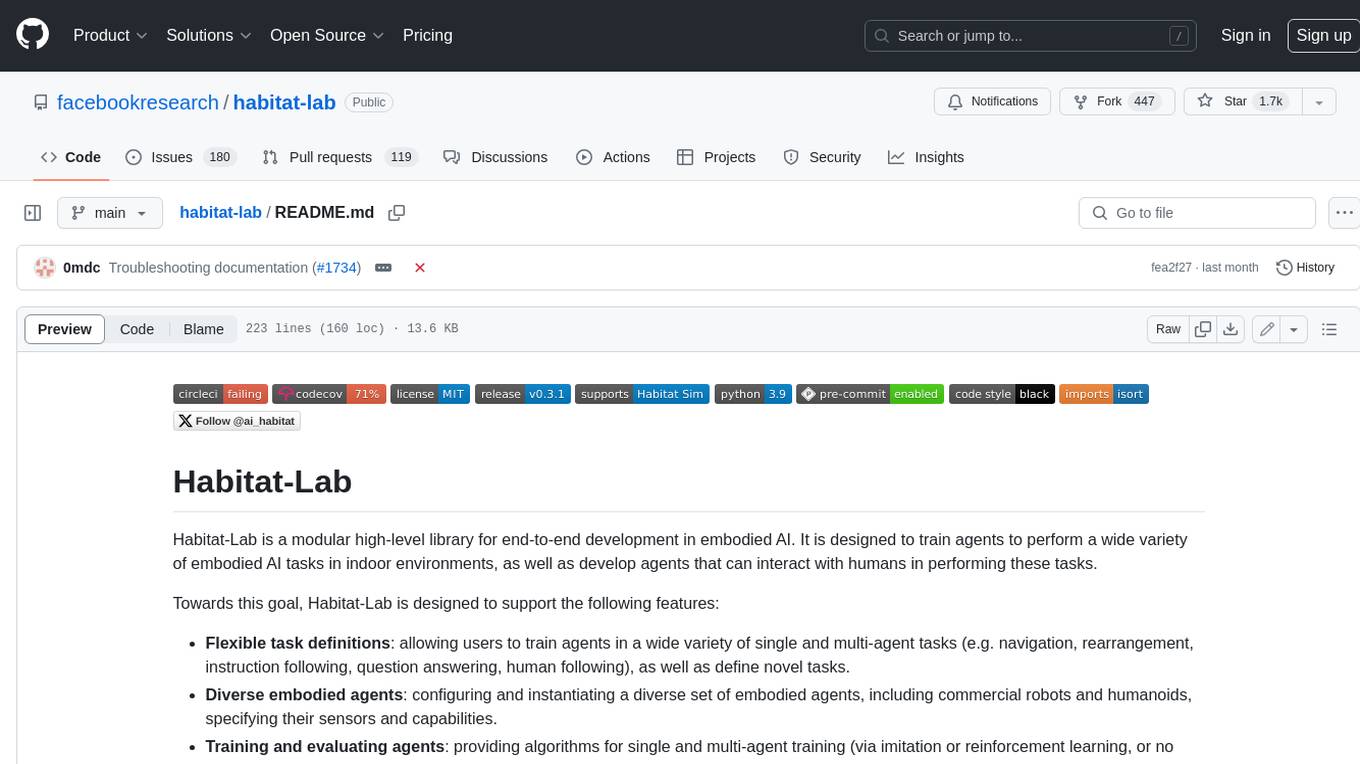
habitat-lab
Habitat-Lab is a modular high-level library for end-to-end development in embodied AI. It is designed to train agents to perform a wide variety of embodied AI tasks in indoor environments, as well as develop agents that can interact with humans in performing these tasks.
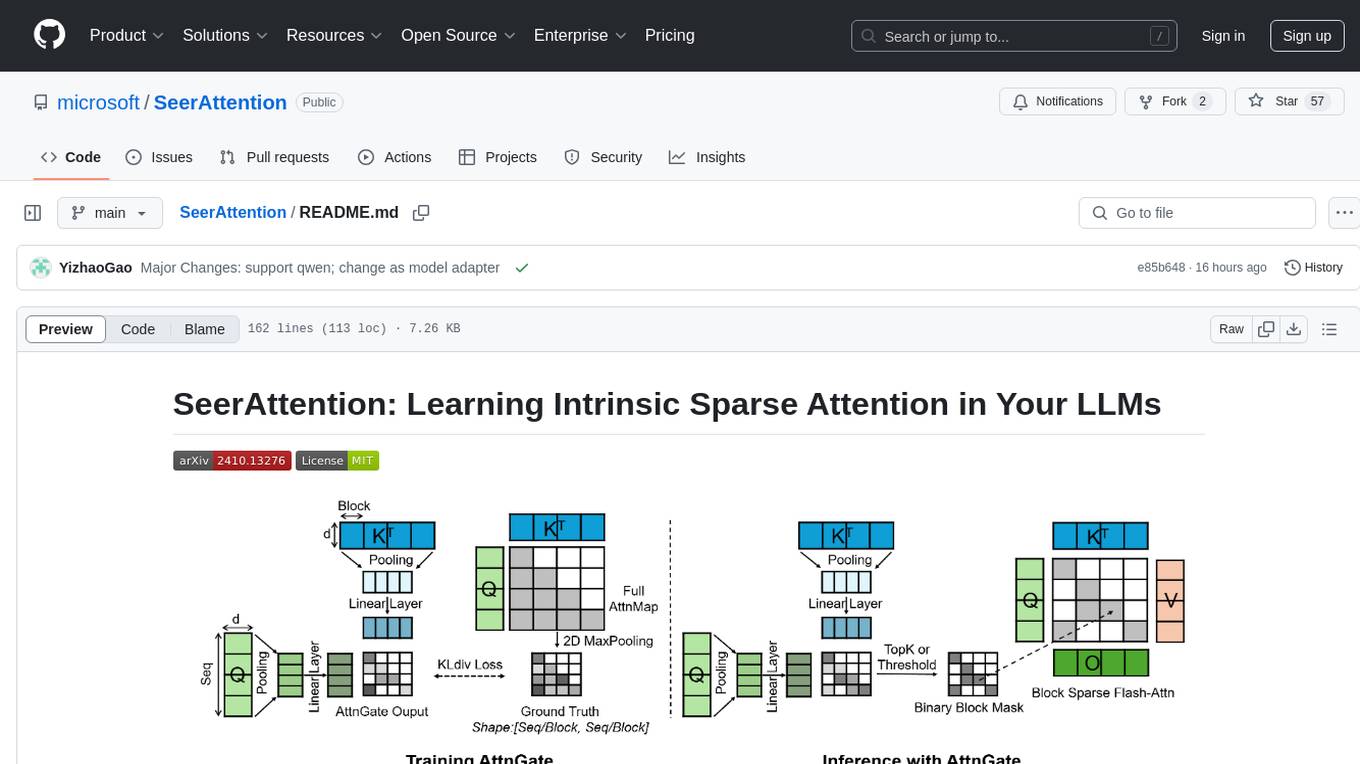
SeerAttention
SeerAttention is a novel trainable sparse attention mechanism that learns intrinsic sparsity patterns directly from LLMs through self-distillation at post-training time. It achieves faster inference while maintaining accuracy for long-context prefilling. The tool offers features such as trainable sparse attention, block-level sparsity, self-distillation, efficient kernel, and easy integration with existing transformer architectures. Users can quickly start using SeerAttention for inference with AttnGate Adapter and training attention gates with self-distillation. The tool provides efficient evaluation methods and encourages contributions from the community.
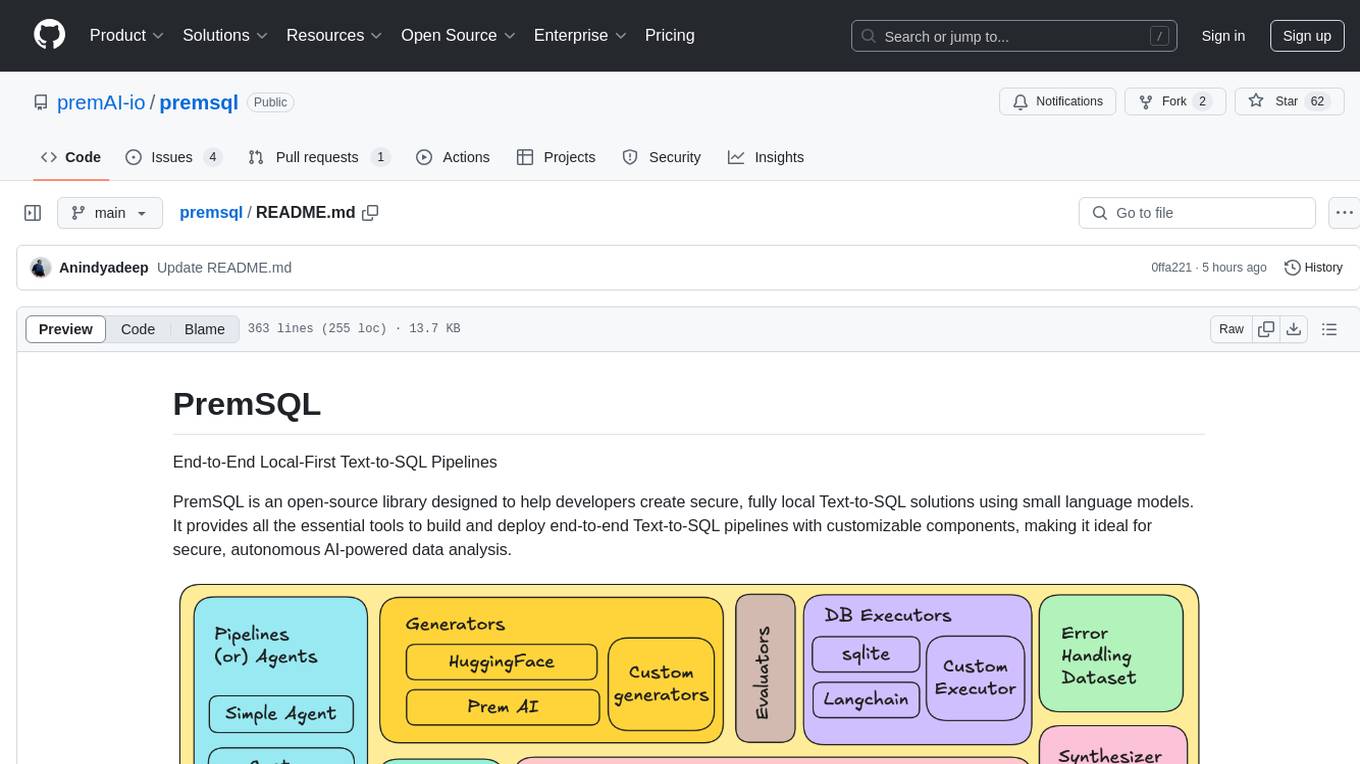
premsql
PremSQL is an open-source library designed to help developers create secure, fully local Text-to-SQL solutions using small language models. It provides essential tools for building and deploying end-to-end Text-to-SQL pipelines with customizable components, ideal for secure, autonomous AI-powered data analysis. The library offers features like Local-First approach, Customizable Datasets, Robust Executors and Evaluators, Advanced Generators, Error Handling and Self-Correction, Fine-Tuning Support, and End-to-End Pipelines. Users can fine-tune models, generate SQL queries from natural language inputs, handle errors, and evaluate model performance against predefined metrics. PremSQL is extendible for customization and private data usage.
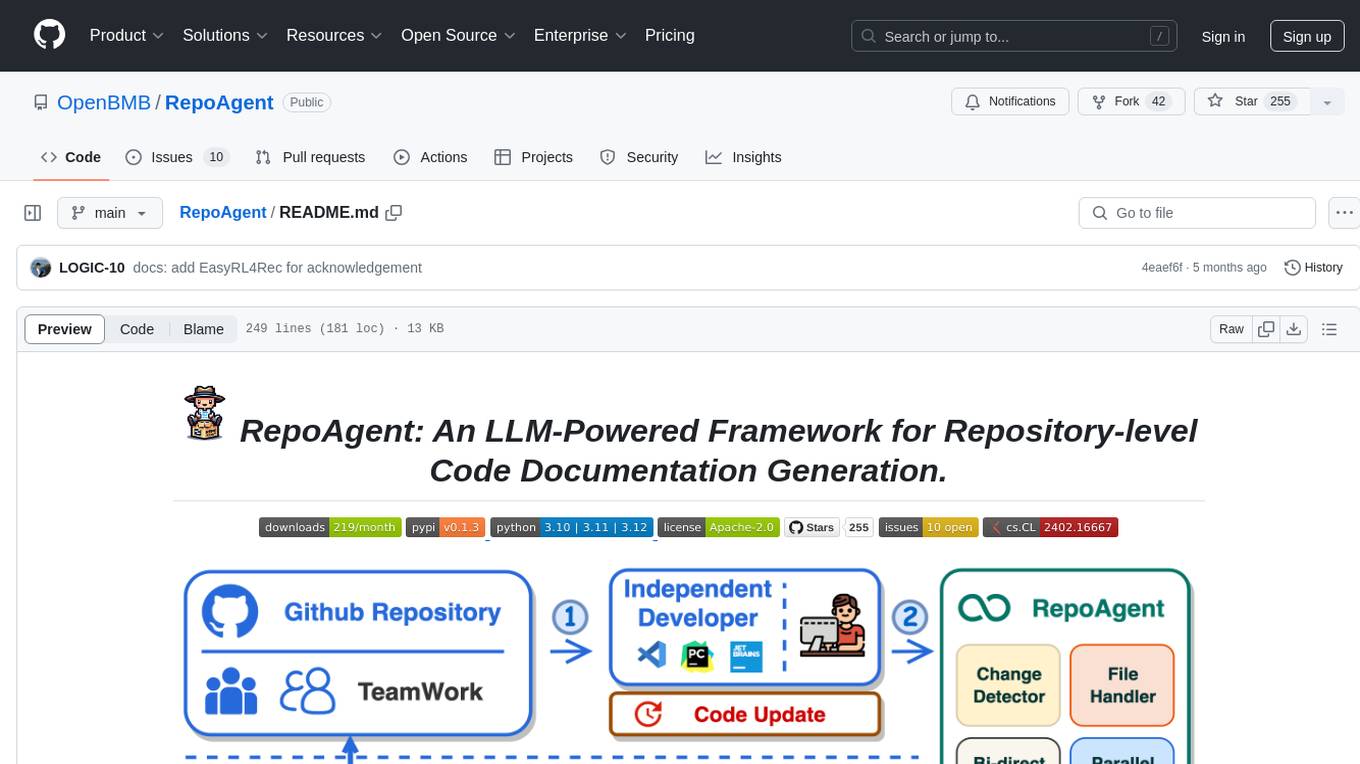
RepoAgent
RepoAgent is an LLM-powered framework designed for repository-level code documentation generation. It automates the process of detecting changes in Git repositories, analyzing code structure through AST, identifying inter-object relationships, replacing Markdown content, and executing multi-threaded operations. The tool aims to assist developers in understanding and maintaining codebases by providing comprehensive documentation, ultimately improving efficiency and saving time.
For similar tasks
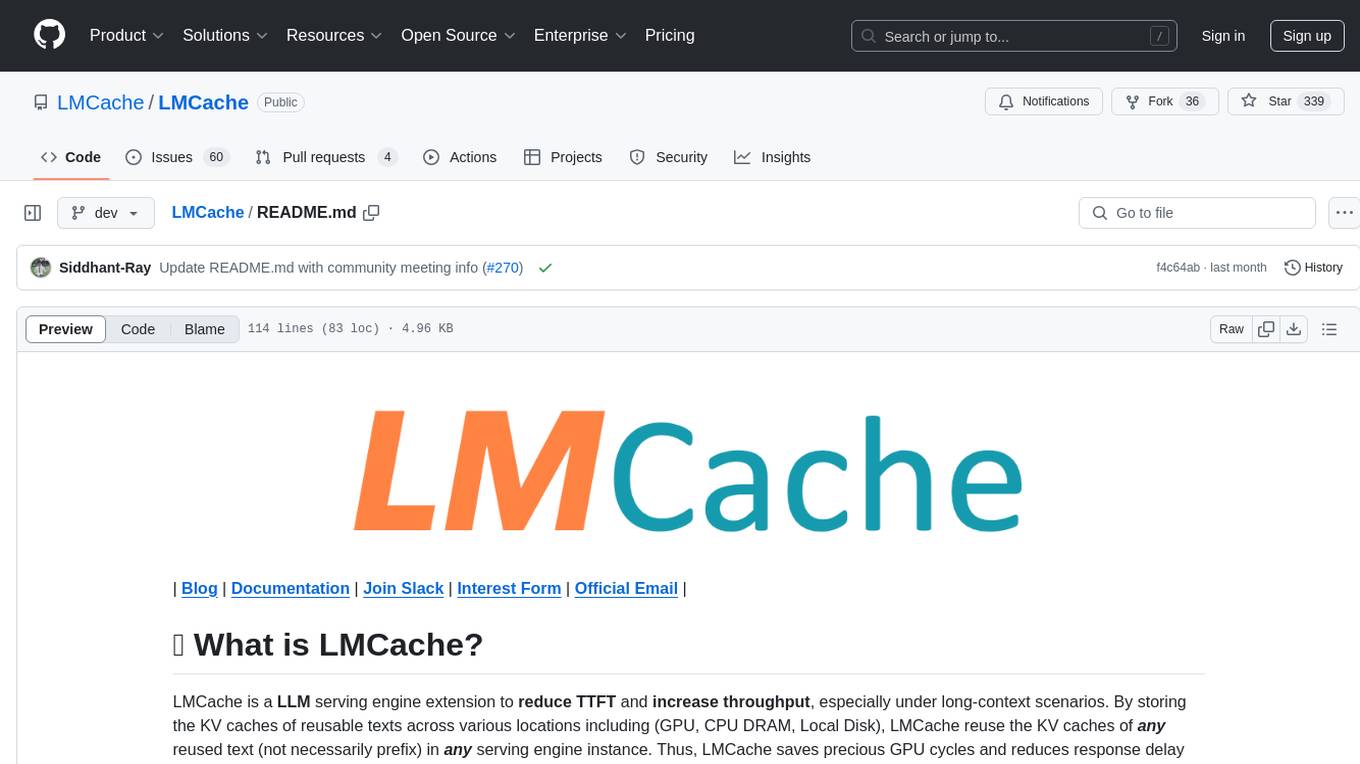
LMCache
LMCache is a serving engine extension designed to reduce time to first token (TTFT) and increase throughput, particularly in long-context scenarios. It stores key-value caches of reusable texts across different locations like GPU, CPU DRAM, and Local Disk, allowing the reuse of any text in any serving engine instance. By combining LMCache with vLLM, significant delay savings and GPU cycle reduction are achieved in various large language model (LLM) use cases, such as multi-round question answering and retrieval-augmented generation (RAG). LMCache provides integration with the latest vLLM version, offering both online serving and offline inference capabilities. It supports sharing key-value caches across multiple vLLM instances and aims to provide stable support for non-prefix key-value caches along with user and developer documentation.
For similar jobs
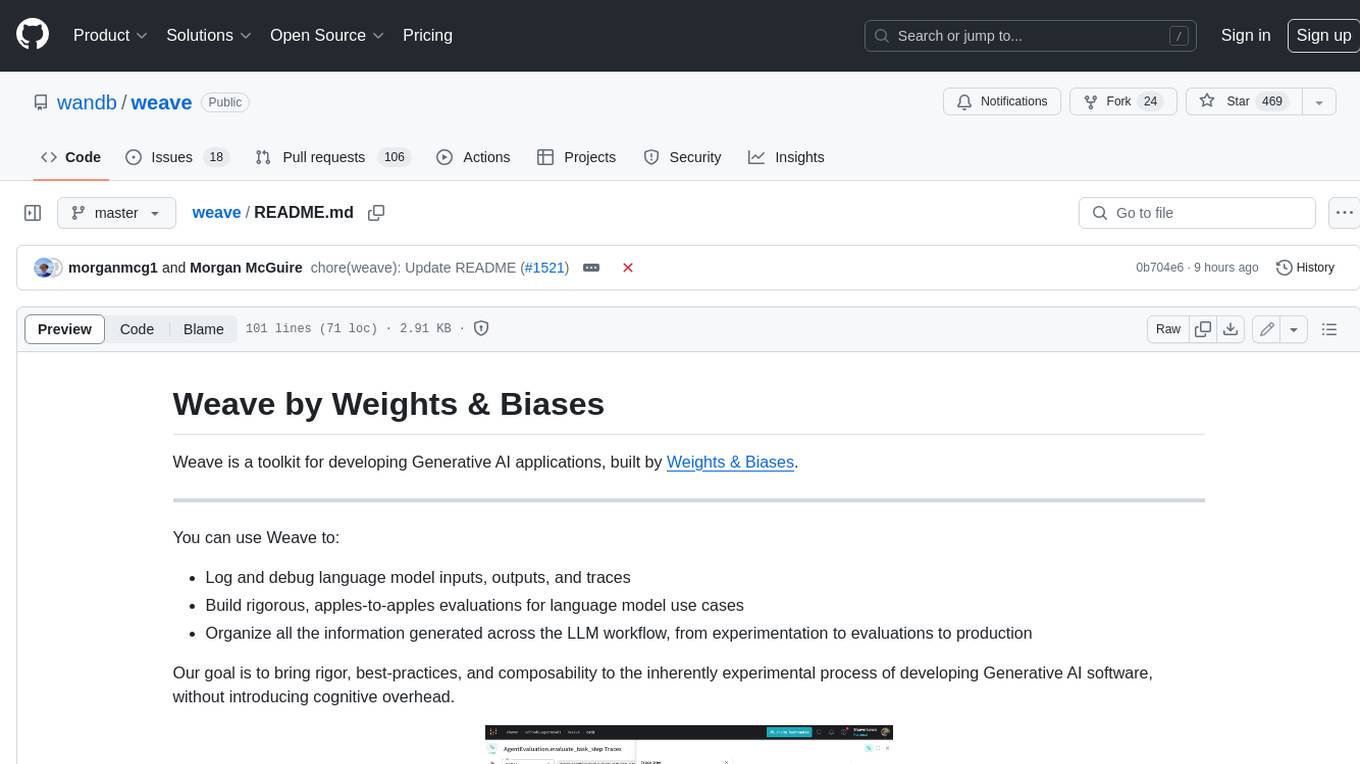
weave
Weave is a toolkit for developing Generative AI applications, built by Weights & Biases. With Weave, you can log and debug language model inputs, outputs, and traces; build rigorous, apples-to-apples evaluations for language model use cases; and organize all the information generated across the LLM workflow, from experimentation to evaluations to production. Weave aims to bring rigor, best-practices, and composability to the inherently experimental process of developing Generative AI software, without introducing cognitive overhead.
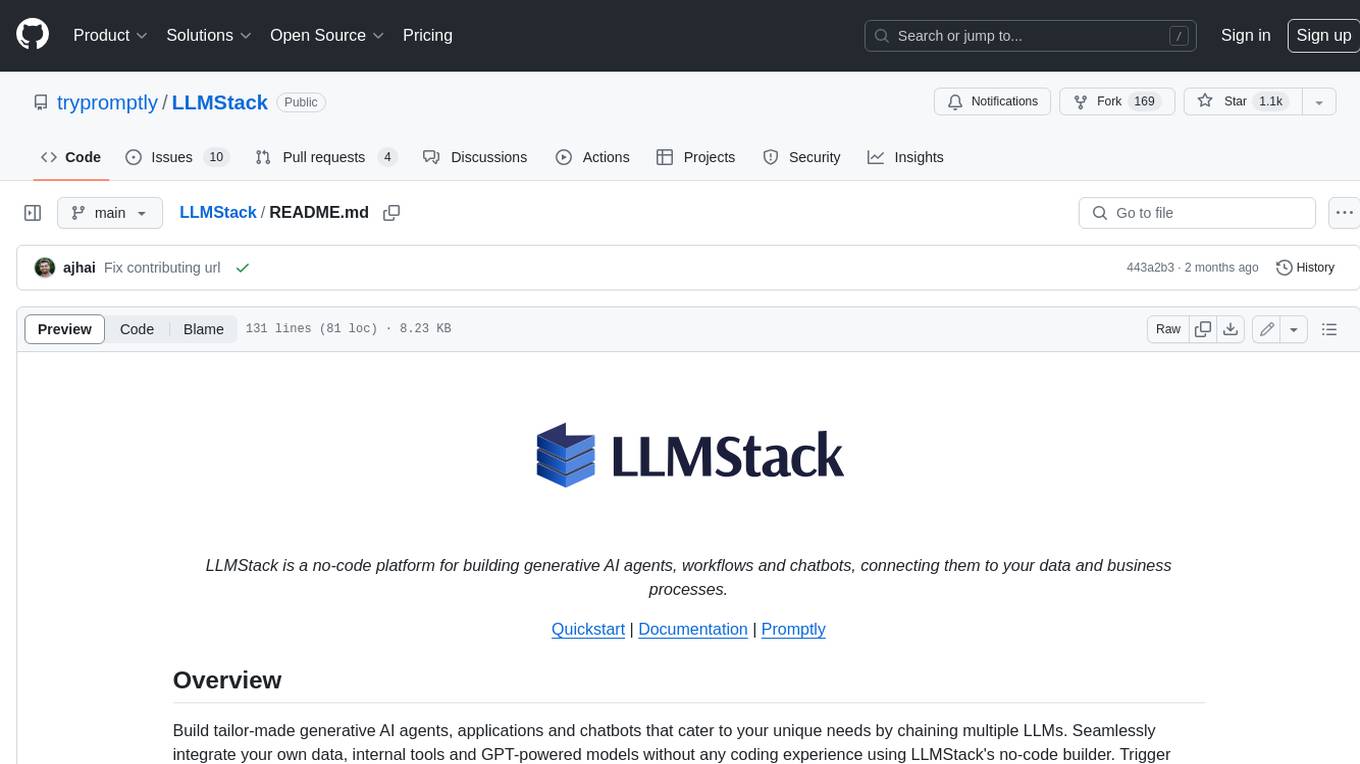
LLMStack
LLMStack is a no-code platform for building generative AI agents, workflows, and chatbots. It allows users to connect their own data, internal tools, and GPT-powered models without any coding experience. LLMStack can be deployed to the cloud or on-premise and can be accessed via HTTP API or triggered from Slack or Discord.
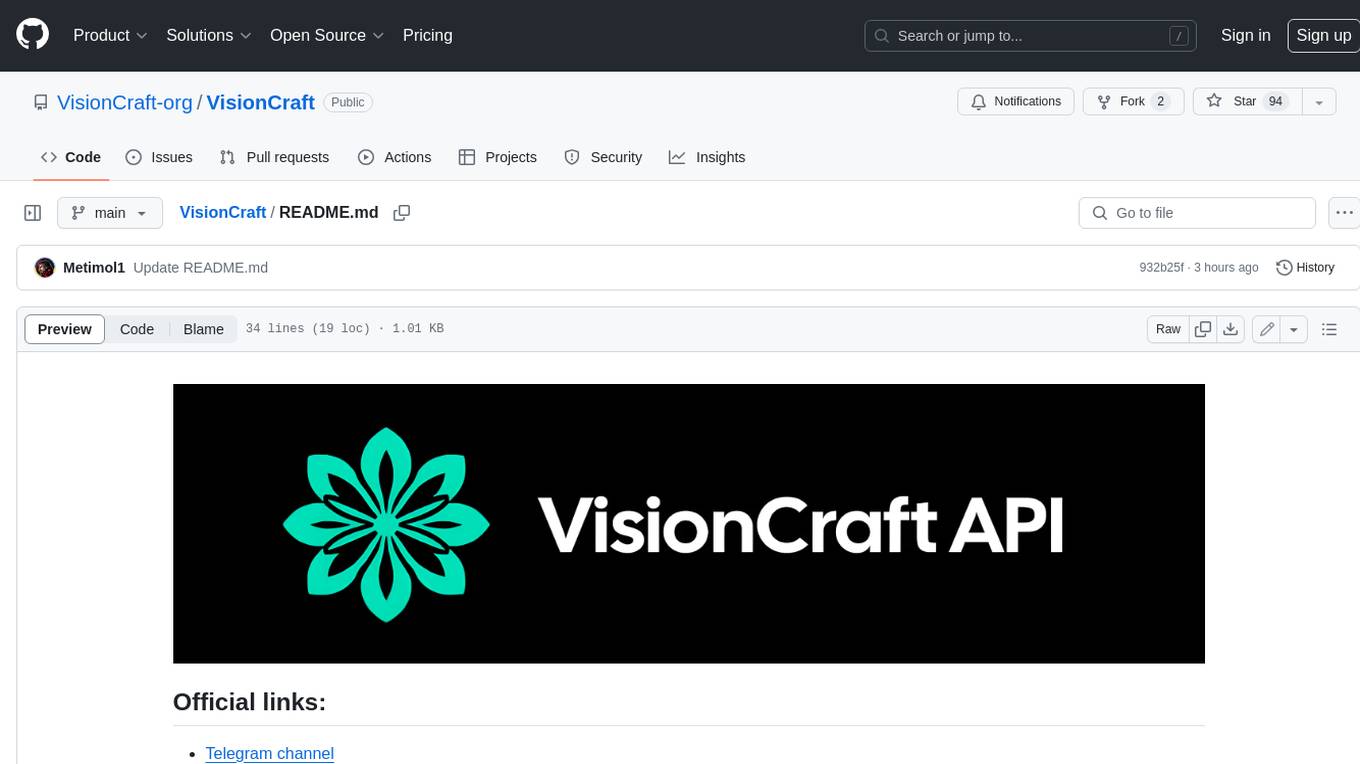
VisionCraft
The VisionCraft API is a free API for using over 100 different AI models. From images to sound.
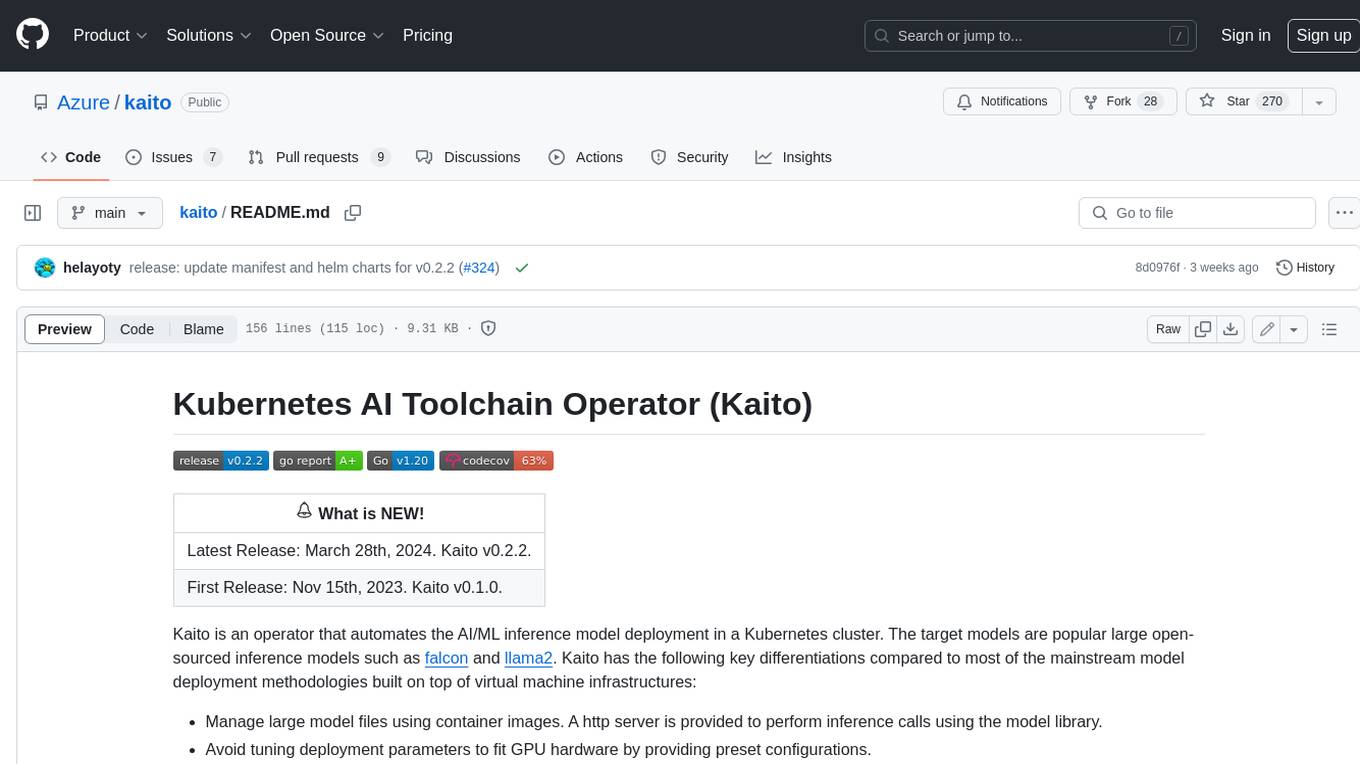
kaito
Kaito is an operator that automates the AI/ML inference model deployment in a Kubernetes cluster. It manages large model files using container images, avoids tuning deployment parameters to fit GPU hardware by providing preset configurations, auto-provisions GPU nodes based on model requirements, and hosts large model images in the public Microsoft Container Registry (MCR) if the license allows. Using Kaito, the workflow of onboarding large AI inference models in Kubernetes is largely simplified.
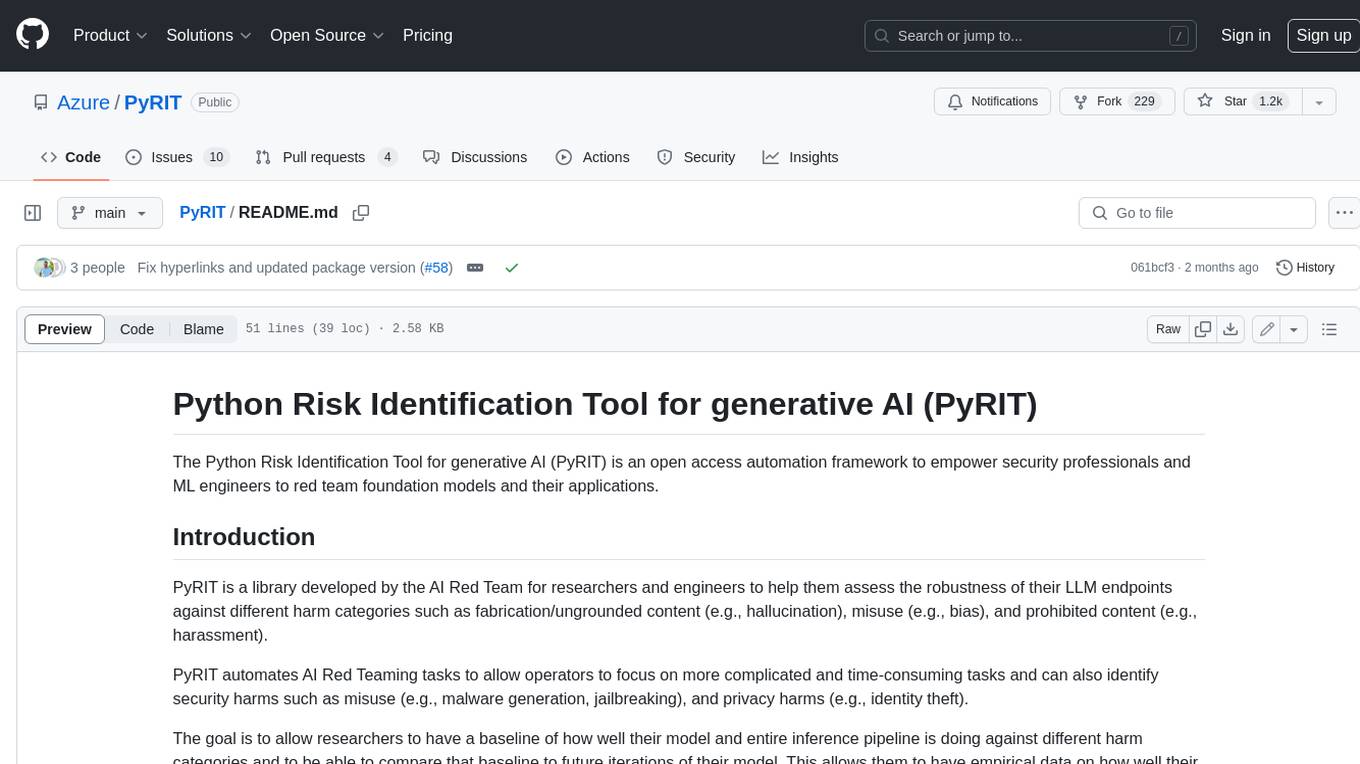
PyRIT
PyRIT is an open access automation framework designed to empower security professionals and ML engineers to red team foundation models and their applications. It automates AI Red Teaming tasks to allow operators to focus on more complicated and time-consuming tasks and can also identify security harms such as misuse (e.g., malware generation, jailbreaking), and privacy harms (e.g., identity theft). The goal is to allow researchers to have a baseline of how well their model and entire inference pipeline is doing against different harm categories and to be able to compare that baseline to future iterations of their model. This allows them to have empirical data on how well their model is doing today, and detect any degradation of performance based on future improvements.
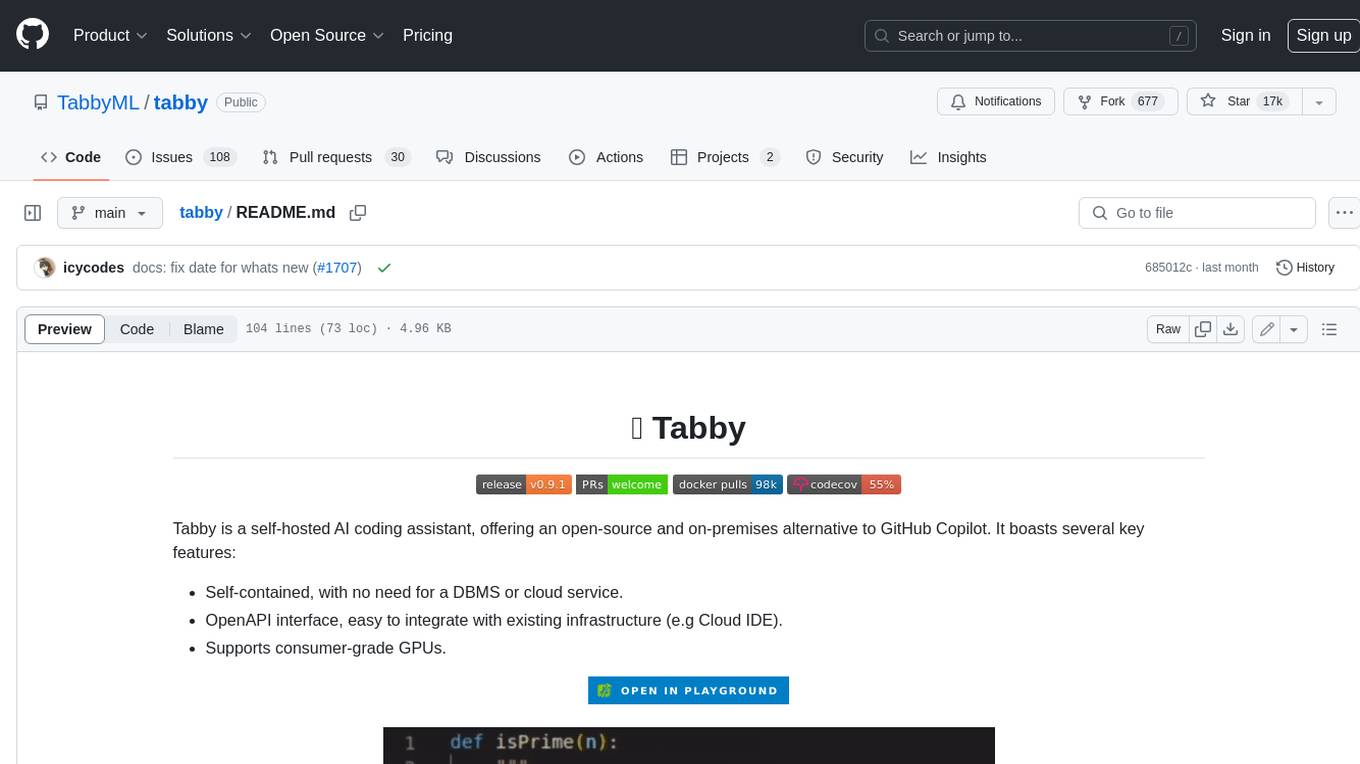
tabby
Tabby is a self-hosted AI coding assistant, offering an open-source and on-premises alternative to GitHub Copilot. It boasts several key features: * Self-contained, with no need for a DBMS or cloud service. * OpenAPI interface, easy to integrate with existing infrastructure (e.g Cloud IDE). * Supports consumer-grade GPUs.
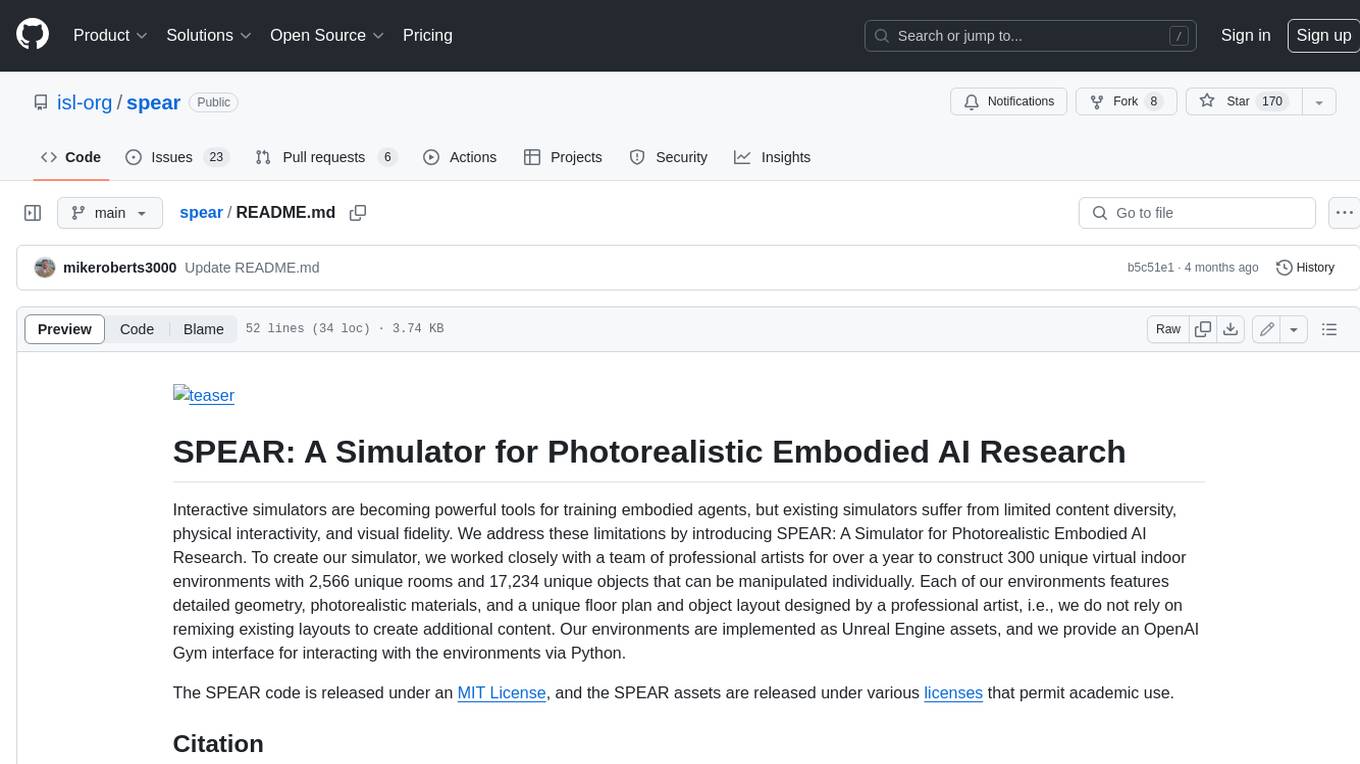
spear
SPEAR (Simulator for Photorealistic Embodied AI Research) is a powerful tool for training embodied agents. It features 300 unique virtual indoor environments with 2,566 unique rooms and 17,234 unique objects that can be manipulated individually. Each environment is designed by a professional artist and features detailed geometry, photorealistic materials, and a unique floor plan and object layout. SPEAR is implemented as Unreal Engine assets and provides an OpenAI Gym interface for interacting with the environments via Python.
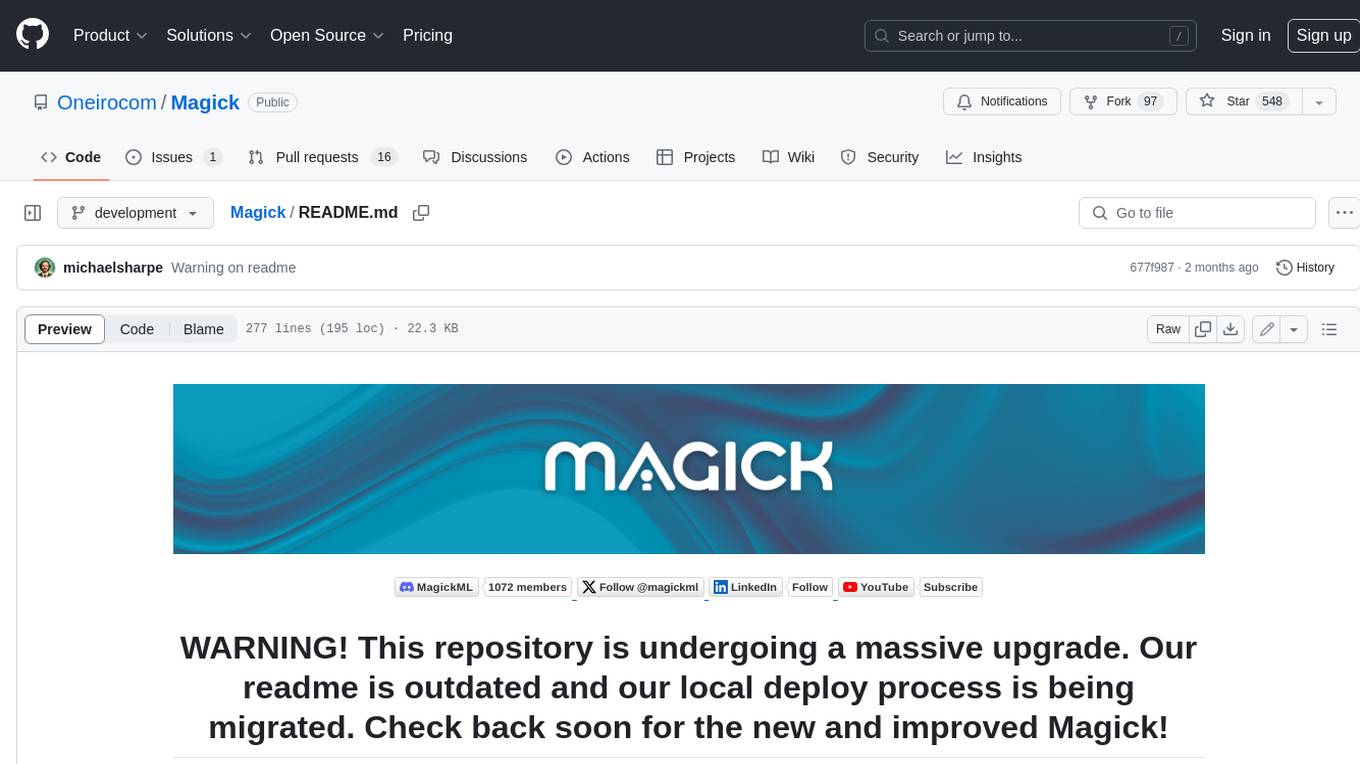
Magick
Magick is a groundbreaking visual AIDE (Artificial Intelligence Development Environment) for no-code data pipelines and multimodal agents. Magick can connect to other services and comes with nodes and templates well-suited for intelligent agents, chatbots, complex reasoning systems and realistic characters.