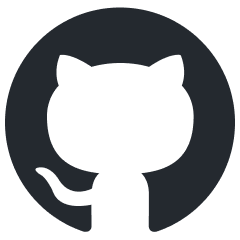
VLMEvalKit
Open-source evaluation toolkit of large multi-modality models (LMMs), support 220+ LMMs, 80+ benchmarks
Stars: 2104
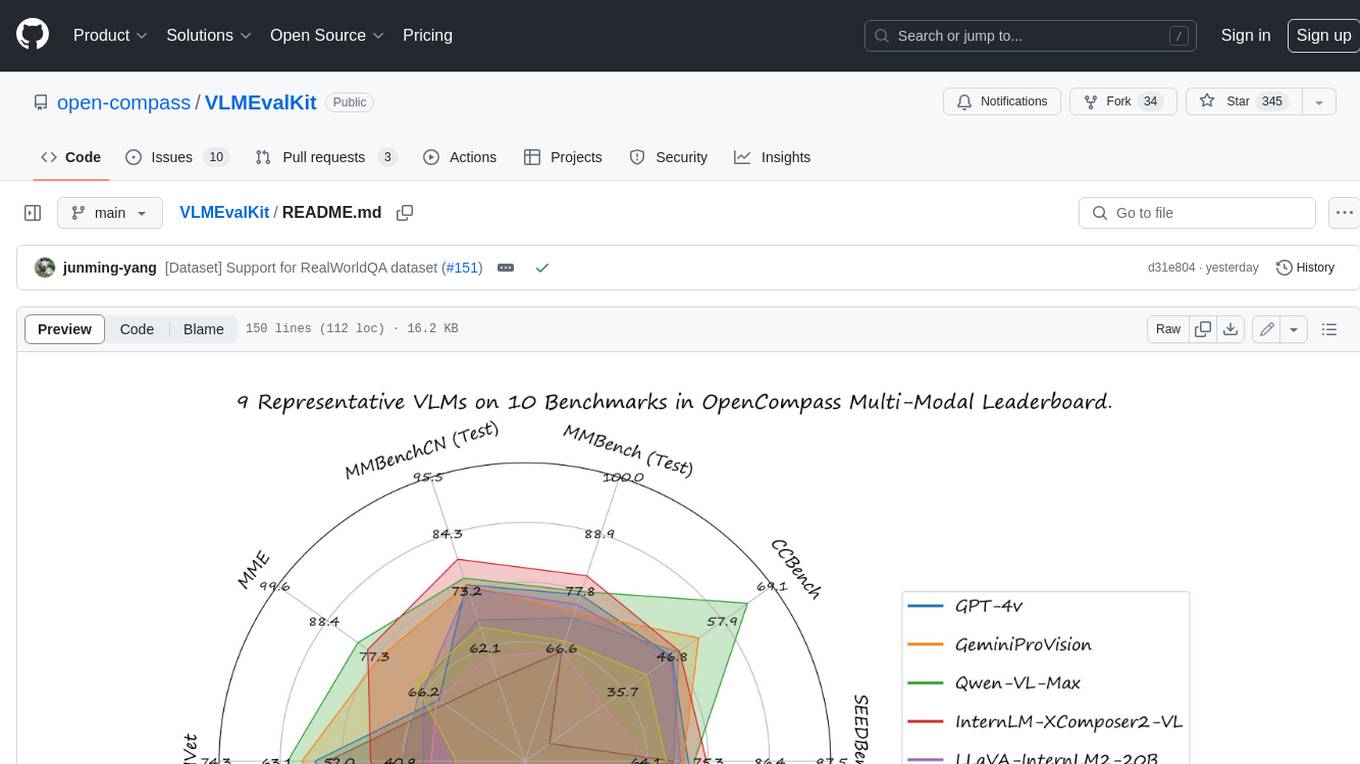
VLMEvalKit is an open-source evaluation toolkit of large vision-language models (LVLMs). It enables one-command evaluation of LVLMs on various benchmarks, without the heavy workload of data preparation under multiple repositories. In VLMEvalKit, we adopt generation-based evaluation for all LVLMs, and provide the evaluation results obtained with both exact matching and LLM-based answer extraction.
README:
A Toolkit for Evaluating Large Vision-Language Models.
🏆 OC Learderboard • 🏗️Quickstart • 📊Datasets & Models • 🛠️Development
🤗 HF Leaderboard • 🤗 Evaluation Records • 🤗 HF Video Leaderboard •
🔊 Discord • 📝 Report • 🎯Goal • 🖊️Citation
VLMEvalKit (the python package name is vlmeval) is an open-source evaluation toolkit of large vision-language models (LVLMs). It enables one-command evaluation of LVLMs on various benchmarks, without the heavy workload of data preparation under multiple repositories. In VLMEvalKit, we adopt generation-based evaluation for all LVLMs, and provide the evaluation results obtained with both exact matching and LLM-based answer extraction.
We have presented a comprehensive survey on the evaluation of large multi-modality models, jointly with MME Team and LMMs-Lab 🔥🔥🔥
- [2025-02-20] Supported Models: InternVL2.5 series, QwenVL2.5 series, QVQ-72B, Doubao-VL, Janus-Pro-7B, MiniCPM-o-2.6, InternVL2-MPO, LLaVA-CoT, Hunyuan-Standard-Vision, Ovis2, Valley, SAIL-VL, Ross, Long-VITA, EMU3, SmolVLM. Supported Benchmarks: MMMU-Pro, WeMath, 3DSRBench, LogicVista, VL-RewardBench, CC-OCR, CG-Bench, CMMMU, WorldSense. Please refer to VLMEvalKit Features for more details. Thanks to all contributors 🔥🔥🔥
- [2024-12-11] Supported NaturalBench, a vision-centric VQA benchmark (NeurIPS'24) that challenges vision-language models with simple questions about natural imagery.
- [2024-12-02] Supported VisOnlyQA, a benchmark for evaluating the visual perception capabilities 🔥🔥🔥
- [2024-11-26] Supported Ovis1.6-Gemma2-27B, thanks to runninglsy 🔥🔥🔥
-
[2024-11-25] Create a new flag
VLMEVALKIT_USE_MODELSCOPE
. By setting this environment variable, you can download the video benchmarks supported from modelscope 🔥🔥🔥 - [2024-11-25] Supported VizWiz benchmark 🔥🔥🔥
- [2024-11-22] Supported the inference of MMGenBench, thanks lerogo 🔥🔥🔥
- [2024-11-22] Supported Dynamath, a multimodal math benchmark comprising of 501 SEED problems and 10 variants generated based on random seeds. The benchmark can be used to measure the robustness of MLLMs in multi-modal math solving 🔥🔥🔥
-
[2024-11-21] Integrated a new config system to enable more flexible evaluation settings. Check the Document or run
python run.py --help
for more details 🔥🔥🔥 - [2024-11-21] Supported QSpatial, a multimodal benchmark for Quantitative Spatial Reasoning (determine the size / distance, e.g.), thanks andrewliao11 for providing the official support 🔥🔥🔥
- [2024-11-21] Supported MM-Math, a new multimodal math benchmark comprising of ~6K middle school multi-modal reasoning math problems. GPT-4o-20240806 achieces 22.5% accuracy on this benchmark 🔥🔥🔥
See [QuickStart | 快速开始] for a quick start guide.
The performance numbers on our official multi-modal leaderboards can be downloaded from here!
OpenVLM Leaderboard: Download All DETAILED Results.
Check Supported Benchmarks Tab in VLMEvalKit Features to view all supported image & video benchmarks (70+).
Check Supported LMMs Tab in VLMEvalKit Features to view all supported LMMs, including commercial APIs, open-source models, and more (200+).
Transformers Version Recommendation:
Note that some VLMs may not be able to run under certain transformer versions, we recommend the following settings to evaluate each VLM:
-
Please use
transformers==4.33.0
for:Qwen series
,Monkey series
,InternLM-XComposer Series
,mPLUG-Owl2
,OpenFlamingo v2
,IDEFICS series
,VisualGLM
,MMAlaya
,ShareCaptioner
,MiniGPT-4 series
,InstructBLIP series
,PandaGPT
,VXVERSE
. -
Please use
transformers==4.36.2
for:Moondream1
. -
Please use
transformers==4.37.0
for:LLaVA series
,ShareGPT4V series
,TransCore-M
,LLaVA (XTuner)
,CogVLM Series
,EMU2 Series
,Yi-VL Series
,MiniCPM-[V1/V2]
,OmniLMM-12B
,DeepSeek-VL series
,InternVL series
,Cambrian Series
,VILA Series
,Llama-3-MixSenseV1_1
,Parrot-7B
,PLLaVA Series
. -
Please use
transformers==4.40.0
for:IDEFICS2
,Bunny-Llama3
,MiniCPM-Llama3-V2.5
,360VL-70B
,Phi-3-Vision
,WeMM
. -
Please use
transformers==4.42.0
for:AKI
. -
Please use
transformers==4.44.0
for:Moondream2
,H2OVL series
. -
Please use
transformers==4.45.0
for:Aria
. -
Please use
transformers==latest
for:LLaVA-Next series
,PaliGemma-3B
,Chameleon series
,Video-LLaVA-7B-HF
,Ovis series
,Mantis series
,MiniCPM-V2.6
,OmChat-v2.0-13B-sinlge-beta
,Idefics-3
,GLM-4v-9B
,VideoChat2-HD
,RBDash_72b
,Llama-3.2 series
,Kosmos series
.
Torchvision Version Recommendation:
Note that some VLMs may not be able to run under certain torchvision versions, we recommend the following settings to evaluate each VLM:
-
Please use
torchvision>=0.16
for:Moondream series
andAria
Flash-attn Version Recommendation:
Note that some VLMs may not be able to run under certain flash-attention versions, we recommend the following settings to evaluate each VLM:
-
Please use
pip install flash-attn --no-build-isolation
for:Aria
# Demo
from vlmeval.config import supported_VLM
model = supported_VLM['idefics_9b_instruct']()
# Forward Single Image
ret = model.generate(['assets/apple.jpg', 'What is in this image?'])
print(ret) # The image features a red apple with a leaf on it.
# Forward Multiple Images
ret = model.generate(['assets/apple.jpg', 'assets/apple.jpg', 'How many apples are there in the provided images? '])
print(ret) # There are two apples in the provided images.
To develop custom benchmarks, VLMs, or simply contribute other codes to VLMEvalKit, please refer to [Development_Guide | 开发指南].
Call for contributions
To promote the contribution from the community and share the corresponding credit (in the next report update):
- All Contributions will be acknowledged in the report.
- Contributors with 3 or more major contributions (implementing an MLLM, benchmark, or major feature) can join the author list of VLMEvalKit Technical Report on ArXiv. Eligible contributors can create an issue or dm kennyutc in VLMEvalKit Discord Channel.
Here is a contributor list we curated based on the records.
The codebase is designed to:
- Provide an easy-to-use, opensource evaluation toolkit to make it convenient for researchers & developers to evaluate existing LVLMs and make evaluation results easy to reproduce.
- Make it easy for VLM developers to evaluate their own models. To evaluate the VLM on multiple supported benchmarks, one just need to implement a single
generate_inner()
function, all other workloads (data downloading, data preprocessing, prediction inference, metric calculation) are handled by the codebase.
The codebase is not designed to:
- Reproduce the exact accuracy number reported in the original papers of all 3rd party benchmarks. The reason can be two-fold:
- VLMEvalKit uses generation-based evaluation for all VLMs (and optionally with LLM-based answer extraction). Meanwhile, some benchmarks may use different approaches (SEEDBench uses PPL-based evaluation, eg.). For those benchmarks, we compare both scores in the corresponding result. We encourage developers to support other evaluation paradigms in the codebase.
- By default, we use the same prompt template for all VLMs to evaluate on a benchmark. Meanwhile, some VLMs may have their specific prompt templates (some may not covered by the codebase at this time). We encourage VLM developers to implement their own prompt template in VLMEvalKit, if that is not covered currently. That will help to improve the reproducibility.
If you find this work helpful, please consider to star🌟 this repo. Thanks for your support!
If you use VLMEvalKit in your research or wish to refer to published OpenSource evaluation results, please use the following BibTeX entry and the BibTex entry corresponding to the specific VLM / benchmark you used.
@inproceedings{duan2024vlmevalkit,
title={Vlmevalkit: An open-source toolkit for evaluating large multi-modality models},
author={Duan, Haodong and Yang, Junming and Qiao, Yuxuan and Fang, Xinyu and Chen, Lin and Liu, Yuan and Dong, Xiaoyi and Zang, Yuhang and Zhang, Pan and Wang, Jiaqi and others},
booktitle={Proceedings of the 32nd ACM International Conference on Multimedia},
pages={11198--11201},
year={2024}
}
For Tasks:
Click tags to check more tools for each tasksFor Jobs:
Alternative AI tools for VLMEvalKit
Similar Open Source Tools
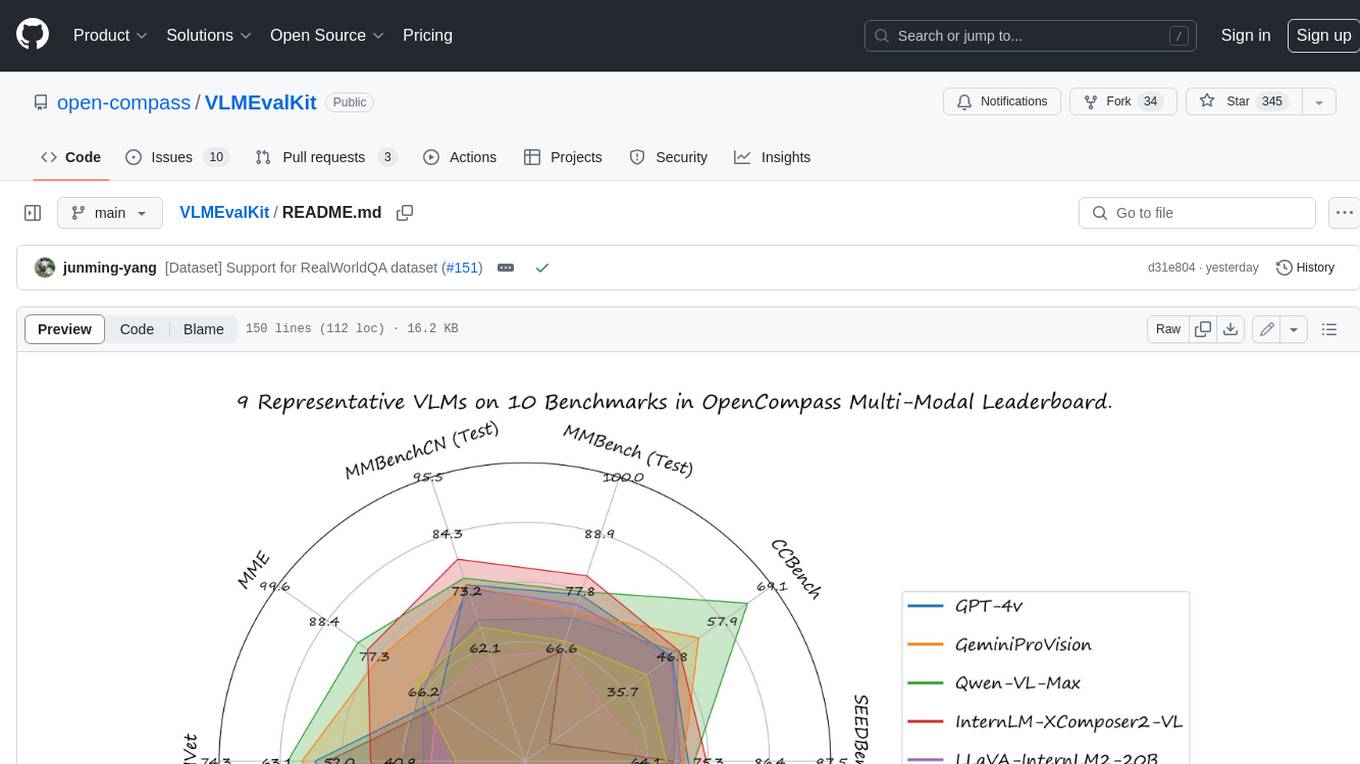
VLMEvalKit
VLMEvalKit is an open-source evaluation toolkit of large vision-language models (LVLMs). It enables one-command evaluation of LVLMs on various benchmarks, without the heavy workload of data preparation under multiple repositories. In VLMEvalKit, we adopt generation-based evaluation for all LVLMs, and provide the evaluation results obtained with both exact matching and LLM-based answer extraction.
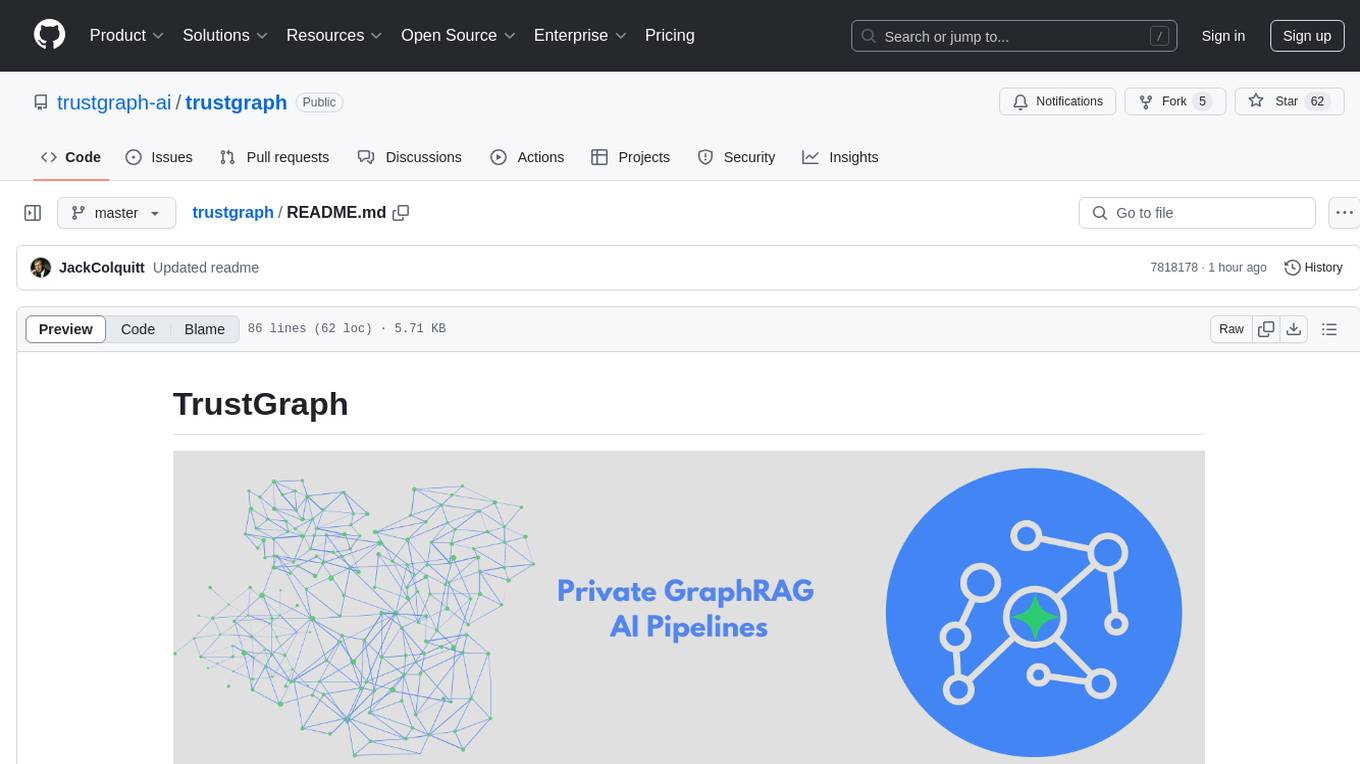
trustgraph
TrustGraph is a tool that deploys private GraphRAG pipelines to build a RDF style knowledge graph from data, enabling accurate and secure `RAG` requests compatible with cloud LLMs and open-source SLMs. It showcases the reliability and efficiencies of GraphRAG algorithms, capturing contextual language flags missed in conventional RAG approaches. The tool offers features like PDF decoding, text chunking, inference of various LMs, RDF-aligned Knowledge Graph extraction, and more. TrustGraph is designed to be modular, supporting multiple Language Models and environments, with a plug'n'play architecture for easy customization.
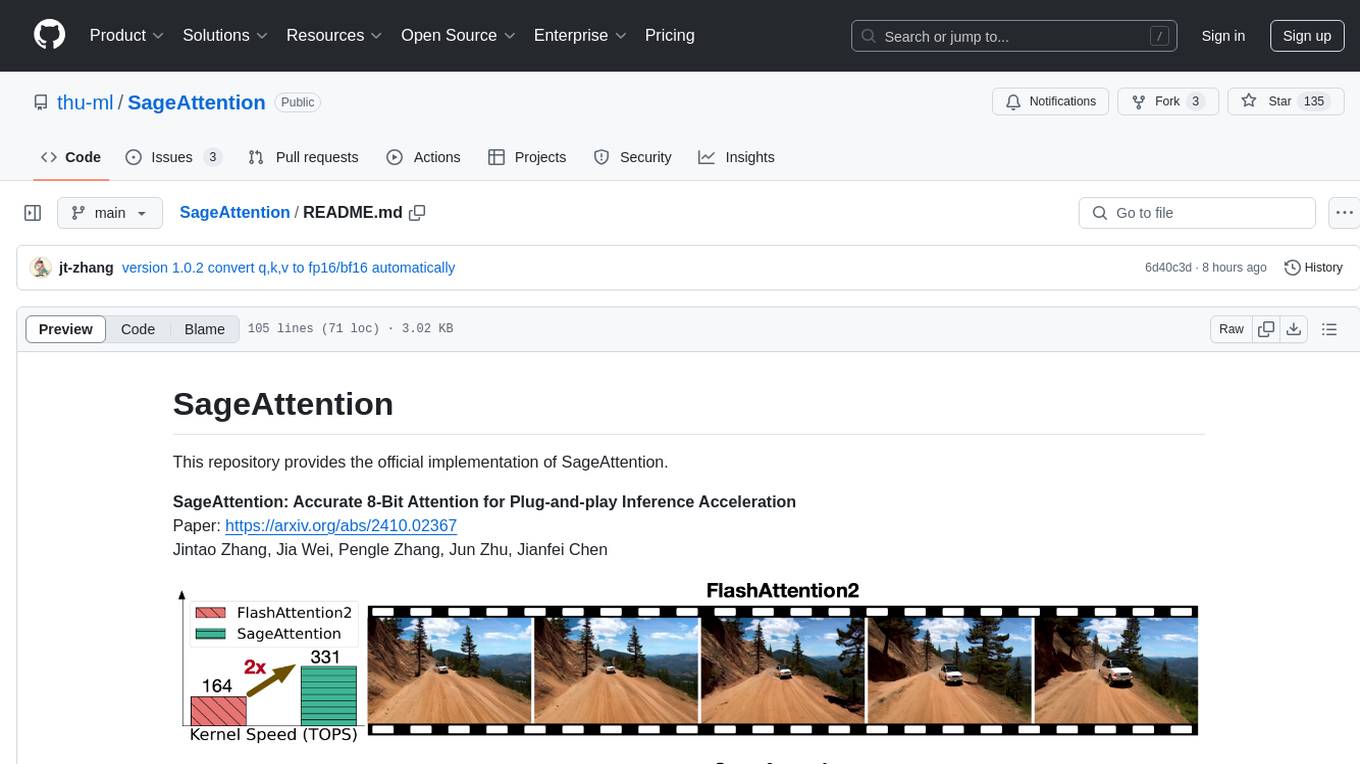
SageAttention
SageAttention is an official implementation of an accurate 8-bit attention mechanism for plug-and-play inference acceleration. It is optimized for RTX4090 and RTX3090 GPUs, providing performance improvements for specific GPU architectures. The tool offers a technique called 'smooth_k' to ensure accuracy in processing FP16/BF16 data. Users can easily replace 'scaled_dot_product_attention' with SageAttention for faster video processing.
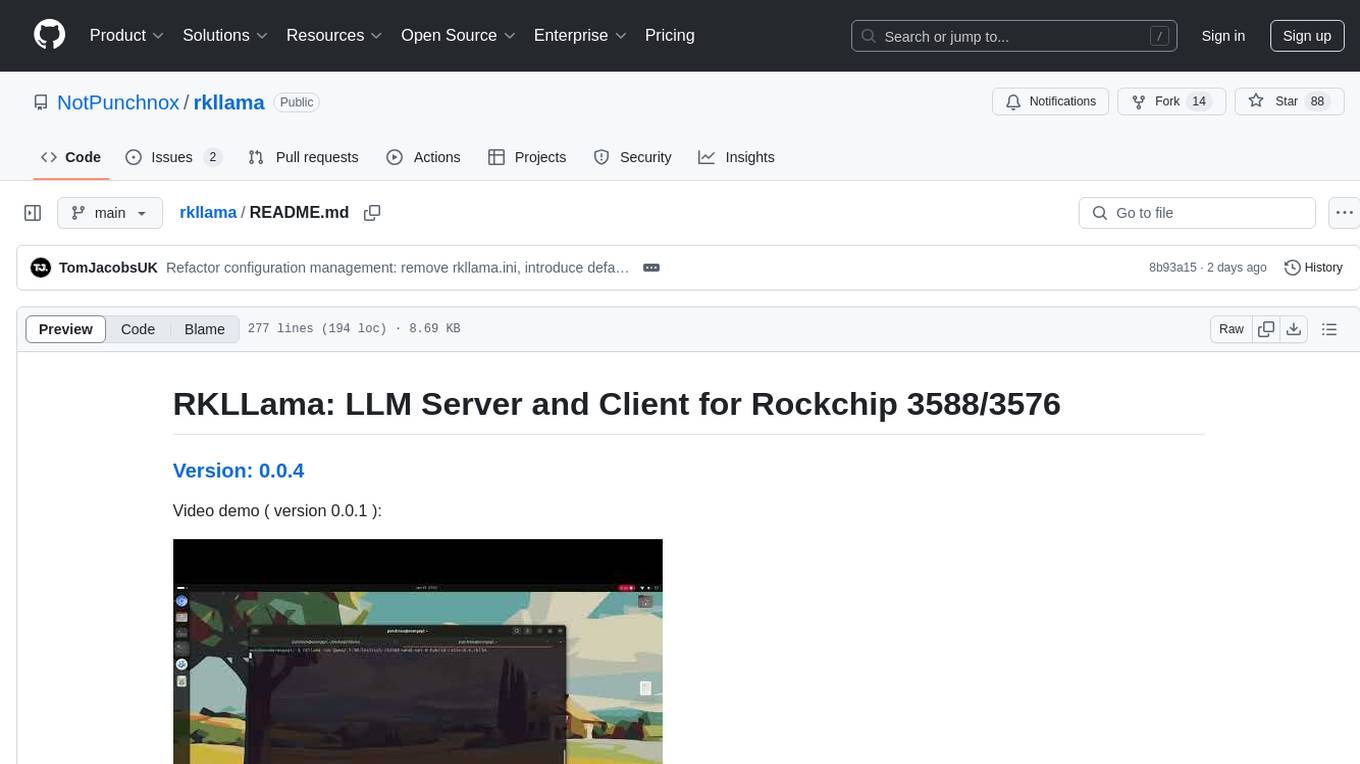
rkllama
RKLLama is a server and client tool designed for running and interacting with LLM models optimized for Rockchip RK3588(S) and RK3576 platforms. It allows models to run on the NPU, with features such as running models on NPU, partial Ollama API compatibility, pulling models from Huggingface, API REST with documentation, dynamic loading/unloading of models, inference requests with streaming modes, simplified model naming, CPU model auto-detection, and optional debug mode. The tool supports Python 3.8 to 3.12 and has been tested on Orange Pi 5 Pro and Orange Pi 5 Plus with specific OS versions.
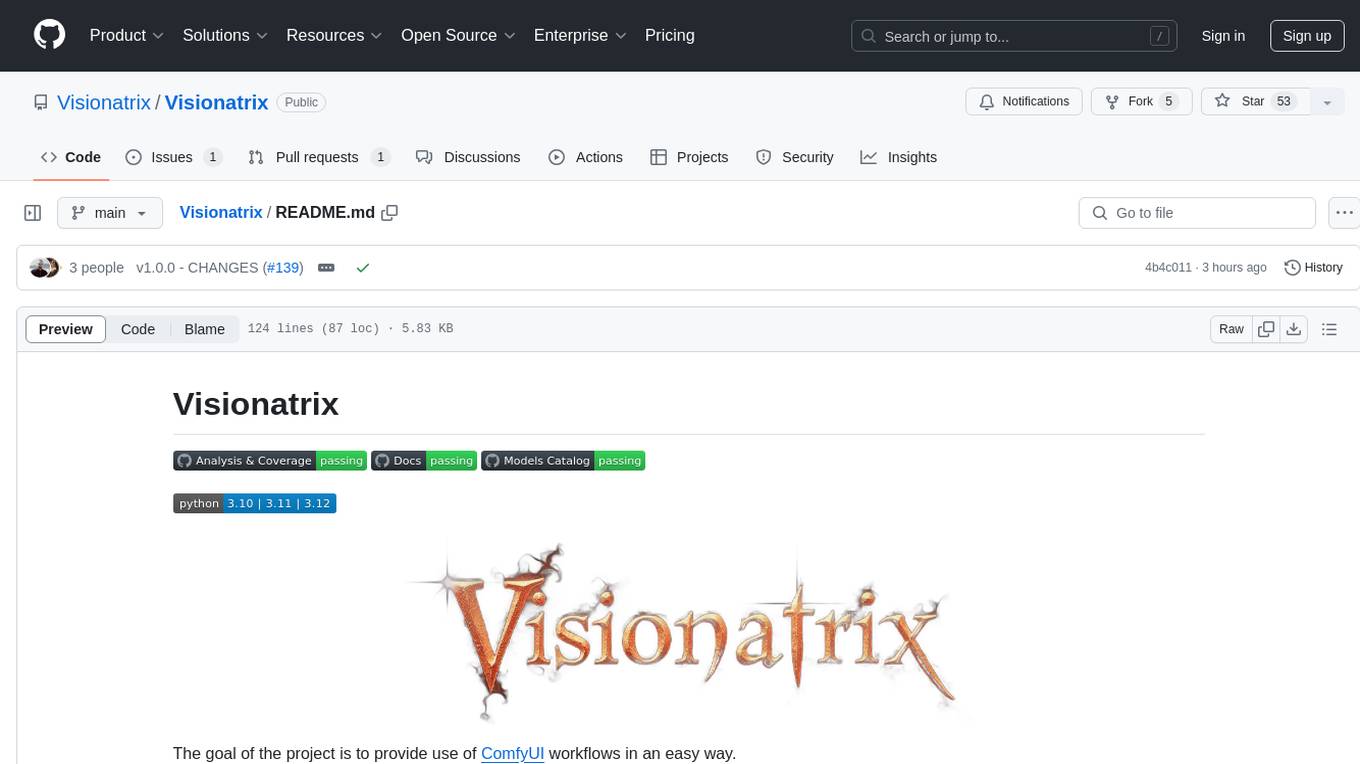
Visionatrix
Visionatrix is a project aimed at providing easy use of ComfyUI workflows. It offers simplified setup and update processes, a minimalistic UI for daily workflow use, stable workflows with versioning and update support, scalability for multiple instances and task workers, multiple user support with integration of different user backends, LLM power for integration with Ollama/Gemini, and seamless integration as a service with backend endpoints and webhook support. The project is approaching version 1.0 release and welcomes new ideas for further implementation.
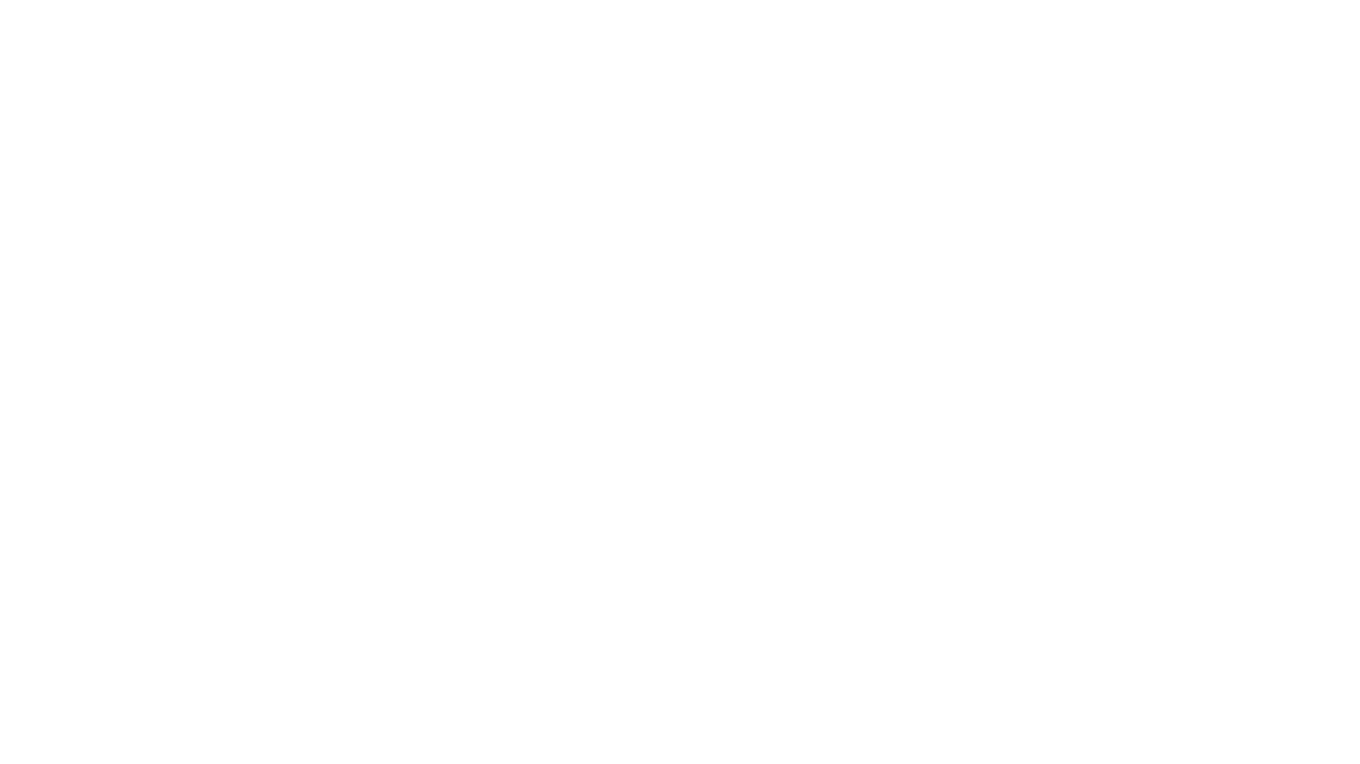
ebook2audiobook
ebook2audiobook is a CPU/GPU converter tool that converts eBooks to audiobooks with chapters and metadata using tools like Calibre, ffmpeg, XTTSv2, and Fairseq. It supports voice cloning and a wide range of languages. The tool is designed to run on 4GB RAM and provides a new v2.0 Web GUI interface for user-friendly interaction. Users can convert eBooks to text format, split eBooks into chapters, and utilize high-quality text-to-speech functionalities. Supported languages include Arabic, Chinese, English, French, German, Hindi, and many more. The tool can be used for legal, non-DRM eBooks only and should be used responsibly in compliance with applicable laws.
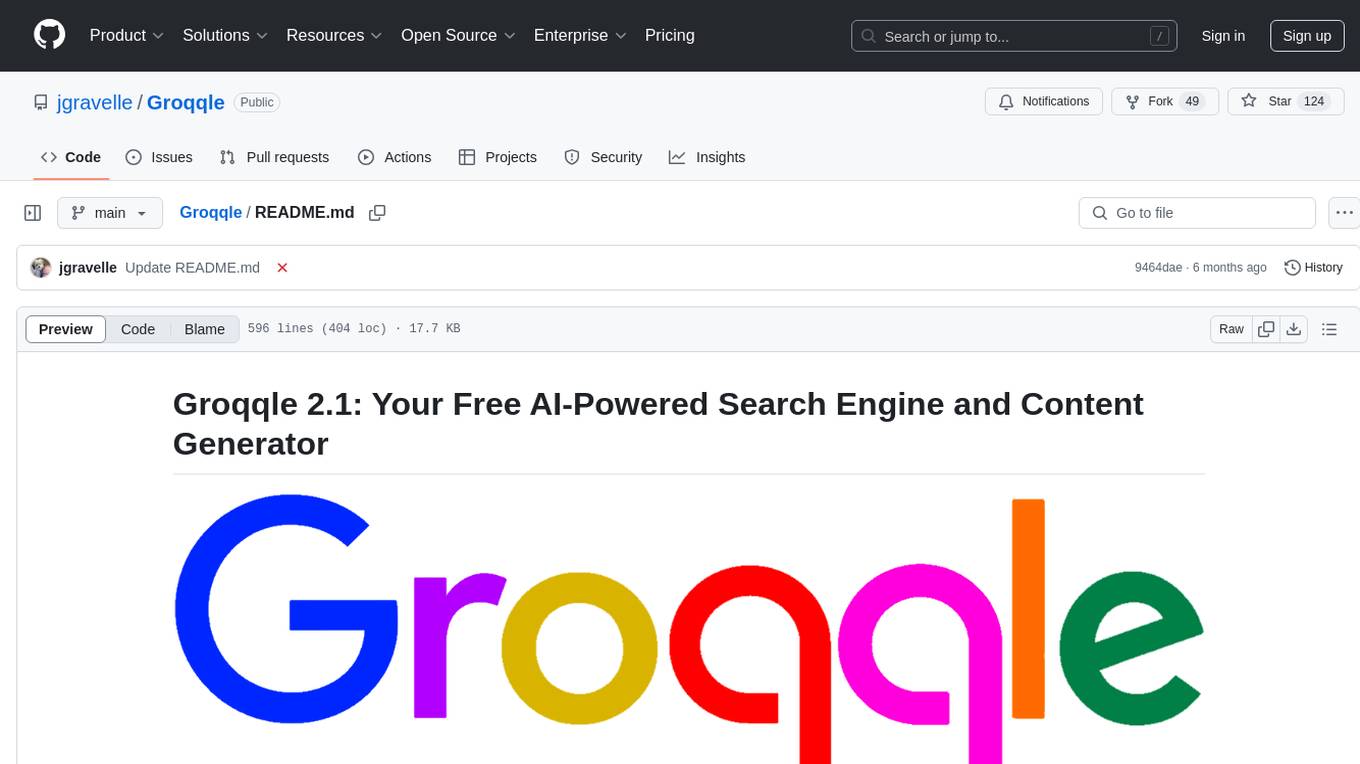
Groqqle
Groqqle 2.1 is a revolutionary, free AI web search and API that instantly returns ORIGINAL content derived from source articles, websites, videos, and even foreign language sources, for ANY target market of ANY reading comprehension level! It combines the power of large language models with advanced web and news search capabilities, offering a user-friendly web interface, a robust API, and now a powerful Groqqle_web_tool for seamless integration into your projects. Developers can instantly incorporate Groqqle into their applications, providing a powerful tool for content generation, research, and analysis across various domains and languages.
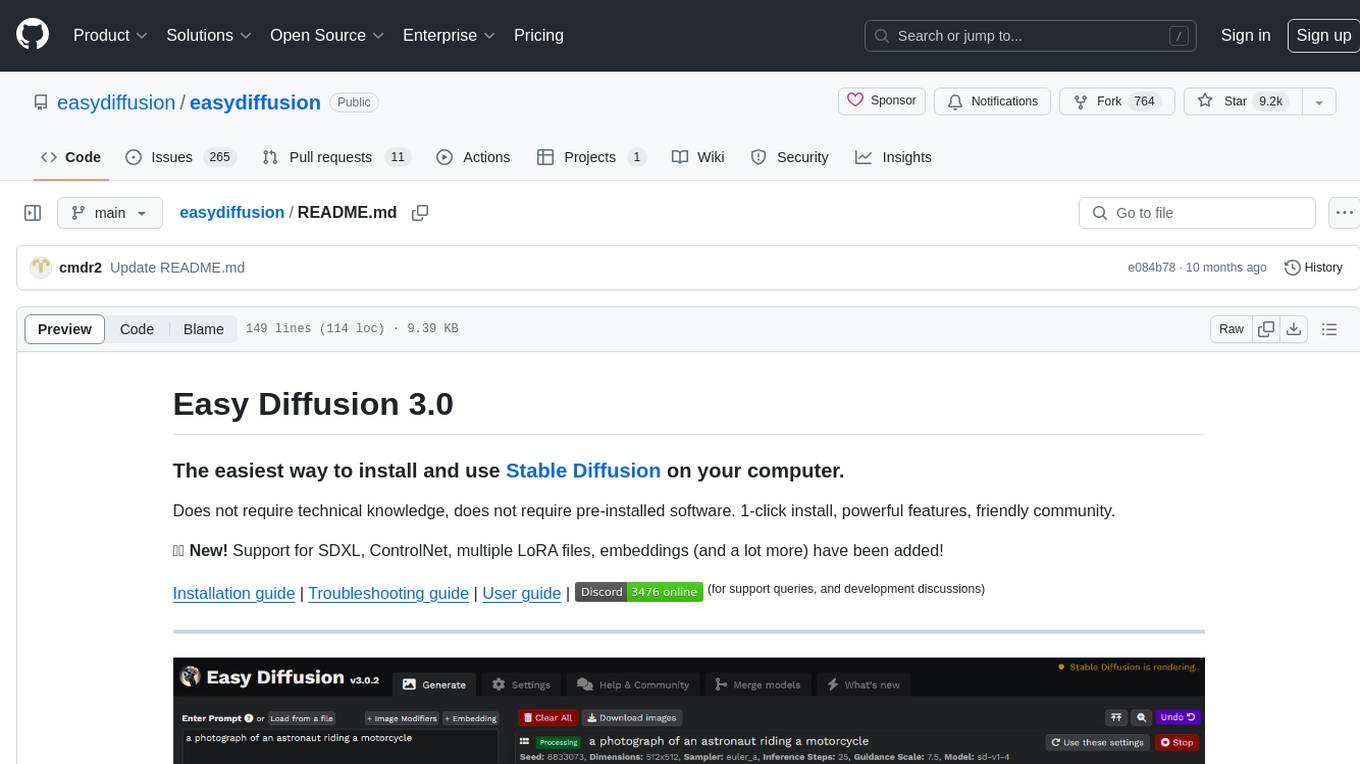
easydiffusion
Easy Diffusion 3.0 is a user-friendly tool for installing and using Stable Diffusion on your computer. It offers hassle-free installation, clutter-free UI, task queue, intelligent model detection, live preview, image modifiers, multiple prompts file, saving generated images, UI themes, searchable models dropdown, and supports various image generation tasks like 'Text to Image', 'Image to Image', and 'InPainting'. The tool also provides advanced features such as custom models, merge models, custom VAE models, multi-GPU support, auto-updater, developer console, and more. It is designed for both new users and advanced users looking for powerful AI image generation capabilities.
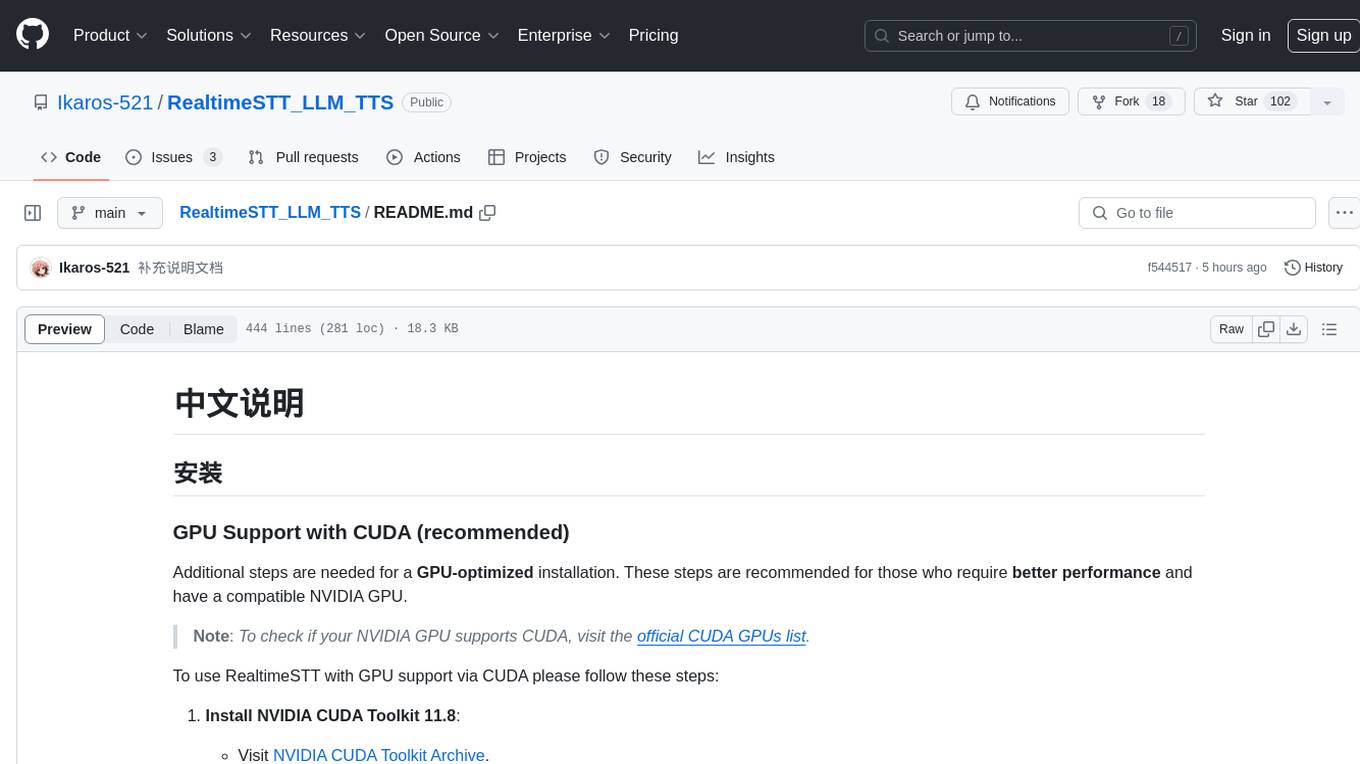
RealtimeSTT_LLM_TTS
RealtimeSTT is an easy-to-use, low-latency speech-to-text library for realtime applications. It listens to the microphone and transcribes voice into text, making it ideal for voice assistants and applications requiring fast and precise speech-to-text conversion. The library utilizes Voice Activity Detection, Realtime Transcription, and Wake Word Activation features. It supports GPU-accelerated transcription using PyTorch with CUDA support. RealtimeSTT offers various customization options for different parameters to enhance user experience and performance. The library is designed to provide a seamless experience for developers integrating speech-to-text functionality into their applications.
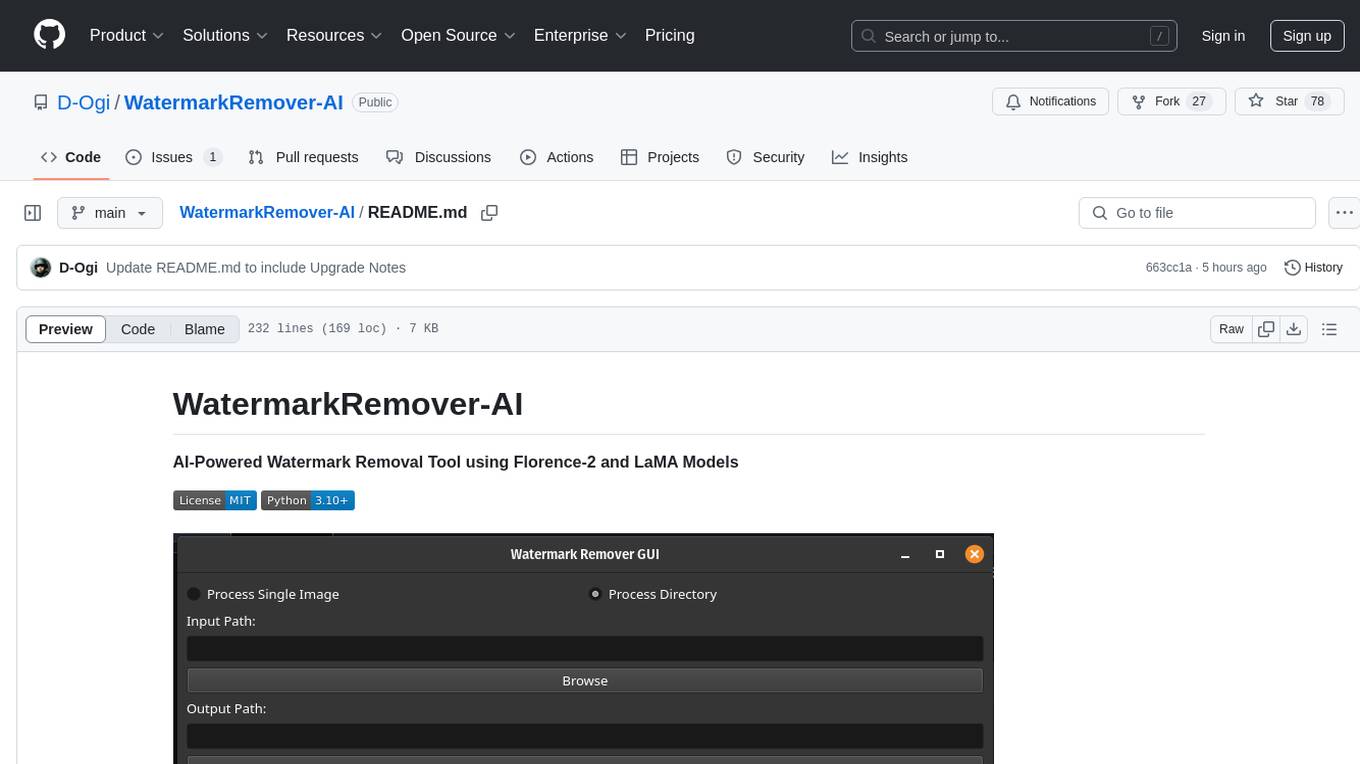
WatermarkRemover-AI
WatermarkRemover-AI is an advanced application that utilizes AI models for precise watermark detection and seamless removal. It leverages Florence-2 for watermark identification and LaMA for inpainting. The tool offers both a command-line interface (CLI) and a PyQt6-based graphical user interface (GUI), making it accessible to users of all levels. It supports dual modes for processing images, advanced watermark detection, seamless inpainting, customizable output settings, real-time progress tracking, dark mode support, and efficient GPU acceleration using CUDA.
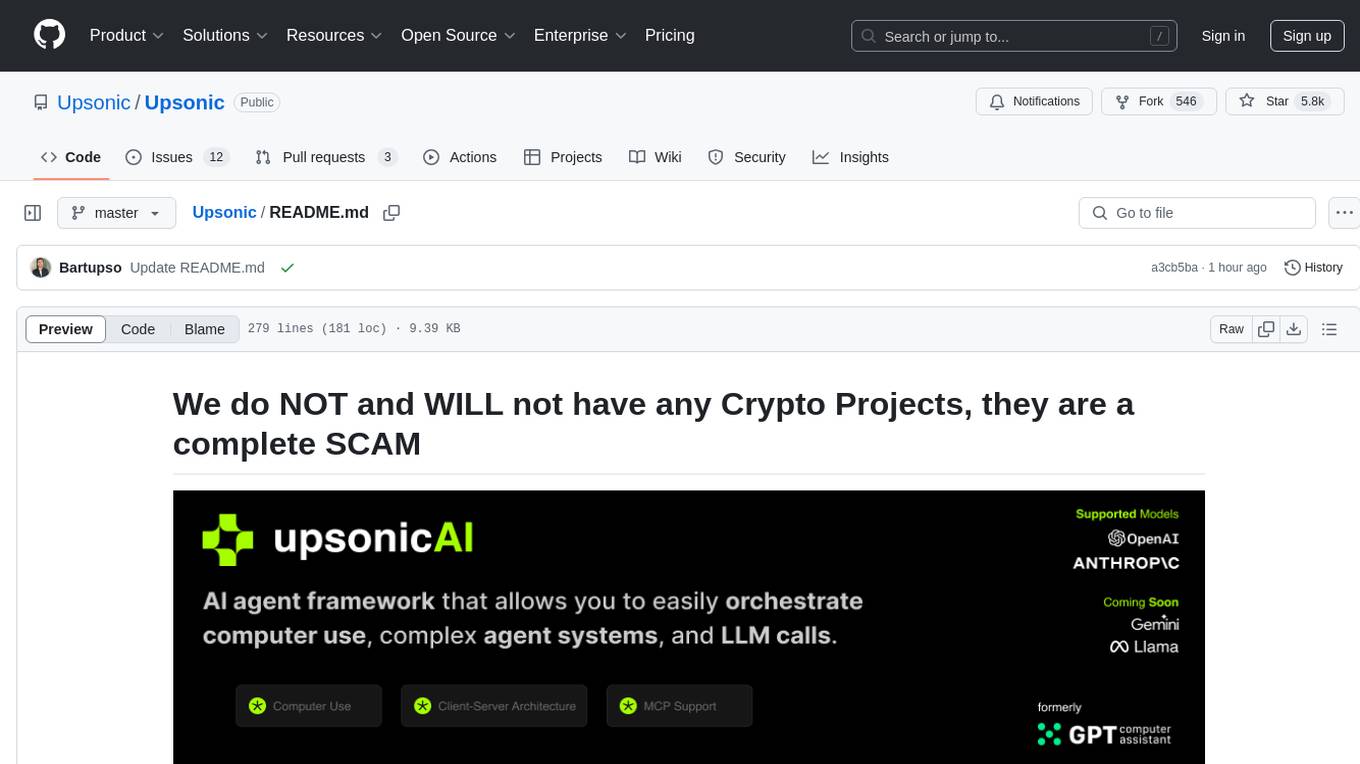
gpt-computer-assistant
GPT Computer Assistant (GCA) is an open-source framework designed to build vertical AI agents that can automate tasks on Windows, macOS, and Ubuntu systems. It leverages the Model Context Protocol (MCP) and its own modules to mimic human-like actions and achieve advanced capabilities. With GCA, users can empower themselves to accomplish more in less time by automating tasks like updating dependencies, analyzing databases, and configuring cloud security settings.
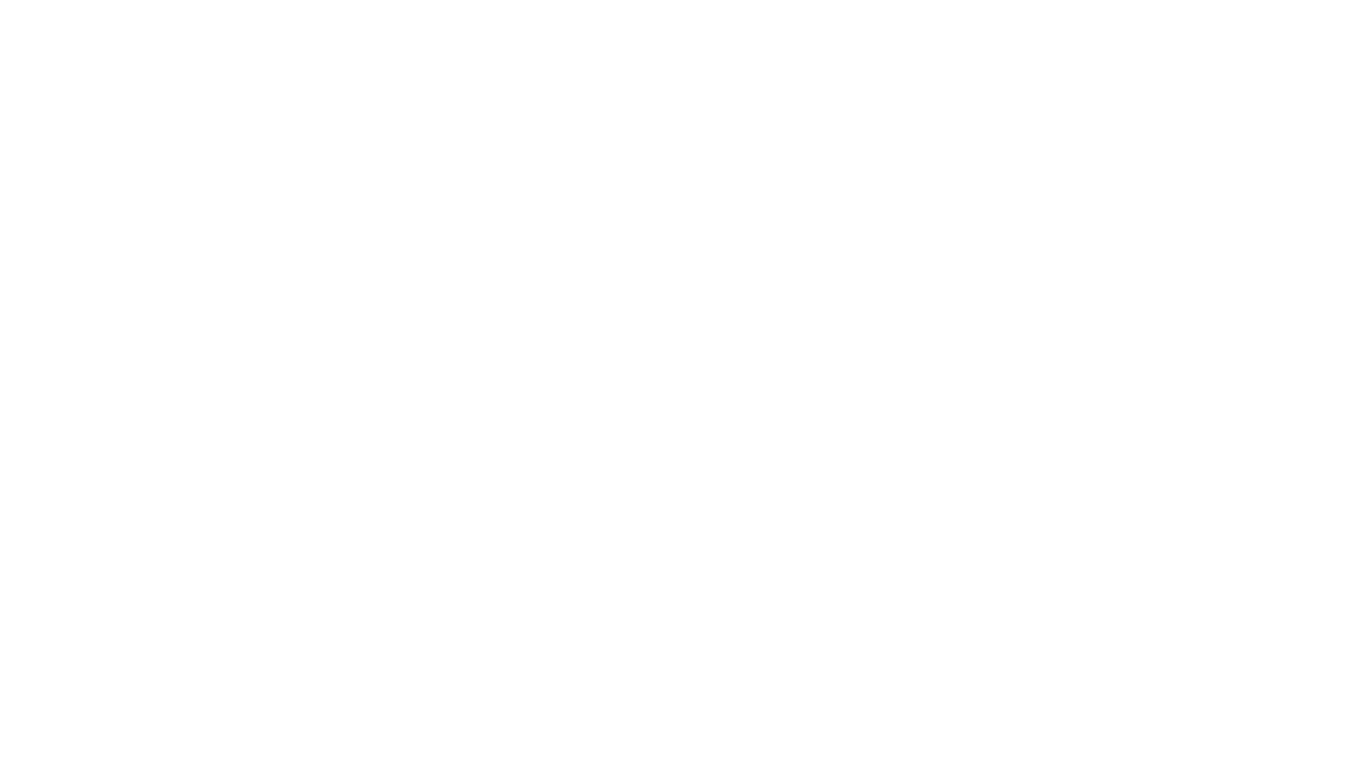
Zero
Zero is an open-source AI email solution that allows users to self-host their email app while integrating external services like Gmail. It aims to modernize and enhance emails through AI agents, offering features like open-source transparency, AI-driven enhancements, data privacy, self-hosting freedom, unified inbox, customizable UI, and developer-friendly extensibility. Built with modern technologies, Zero provides a reliable tech stack including Next.js, React, TypeScript, TailwindCSS, Node.js, Drizzle ORM, and PostgreSQL. Users can set up Zero using standard setup or Dev Container setup for VS Code users, with detailed environment setup instructions for Better Auth, Google OAuth, and optional GitHub OAuth. Database setup involves starting a local PostgreSQL instance, setting up database connection, and executing database commands for dependencies, tables, migrations, and content viewing.
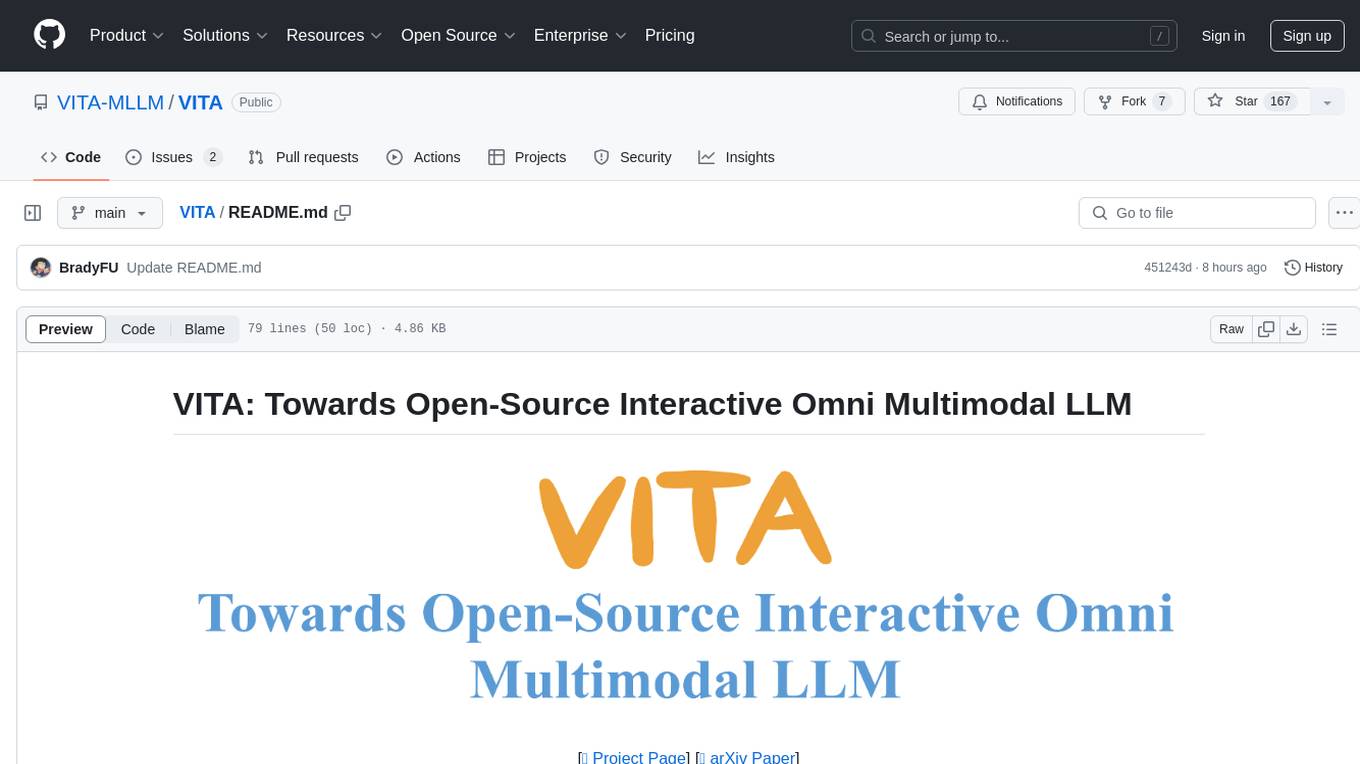
VITA
VITA is an open-source interactive omni multimodal Large Language Model (LLM) capable of processing video, image, text, and audio inputs simultaneously. It stands out with features like Omni Multimodal Understanding, Non-awakening Interaction, and Audio Interrupt Interaction. VITA can respond to user queries without a wake-up word, track and filter external queries in real-time, and handle various query inputs effectively. The model utilizes state tokens and a duplex scheme to enhance the multimodal interactive experience.
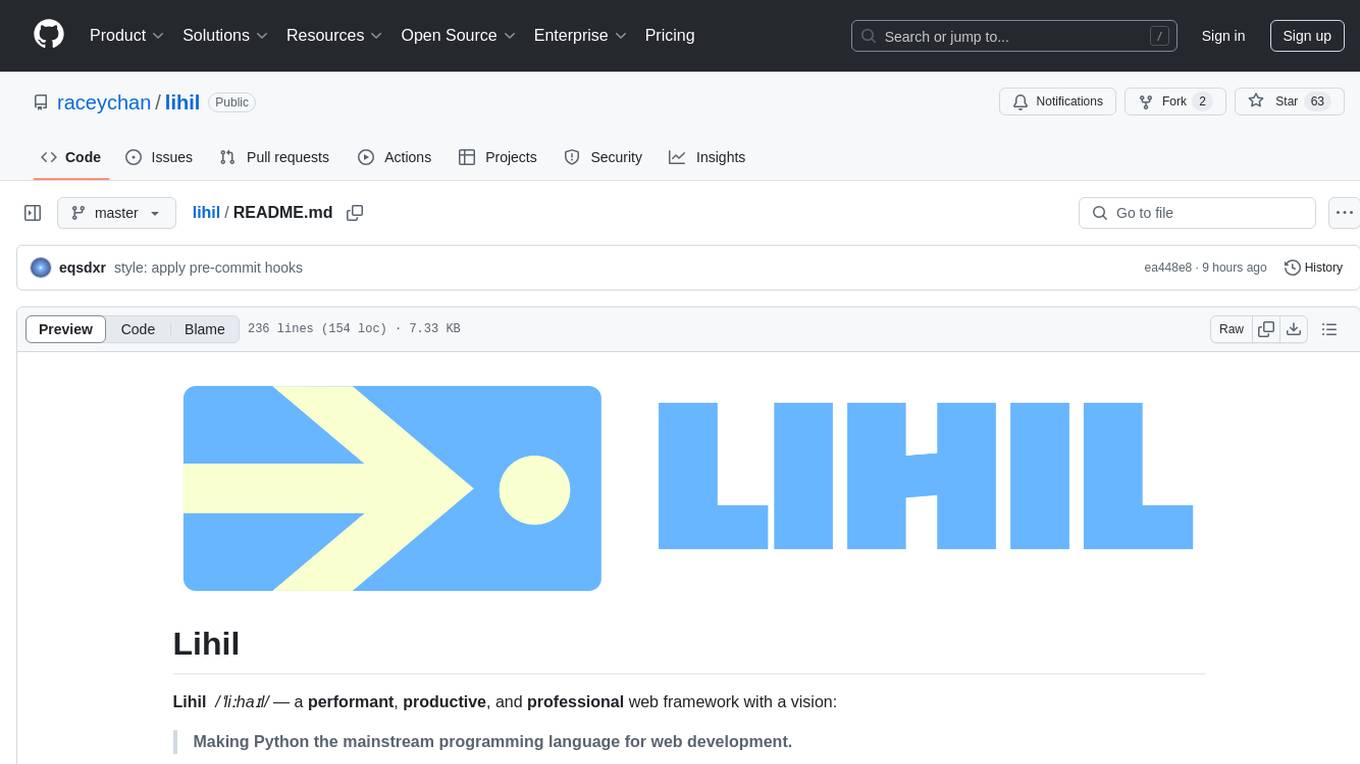
lihil
Lihil is a performant, productive, and professional web framework designed to make Python the mainstream programming language for web development. It is 100% test covered and strictly typed, offering fast performance, ergonomic API, and built-in solutions for common problems. Lihil is suitable for enterprise web development, delivering robust and scalable solutions with best practices in microservice architecture and related patterns. It features dependency injection, OpenAPI docs generation, error response generation, data validation, message system, testability, and strong support for AI features. Lihil is ASGI compatible and uses starlette as its ASGI toolkit, ensuring compatibility with starlette classes and middlewares. The framework follows semantic versioning and has a roadmap for future enhancements and features.
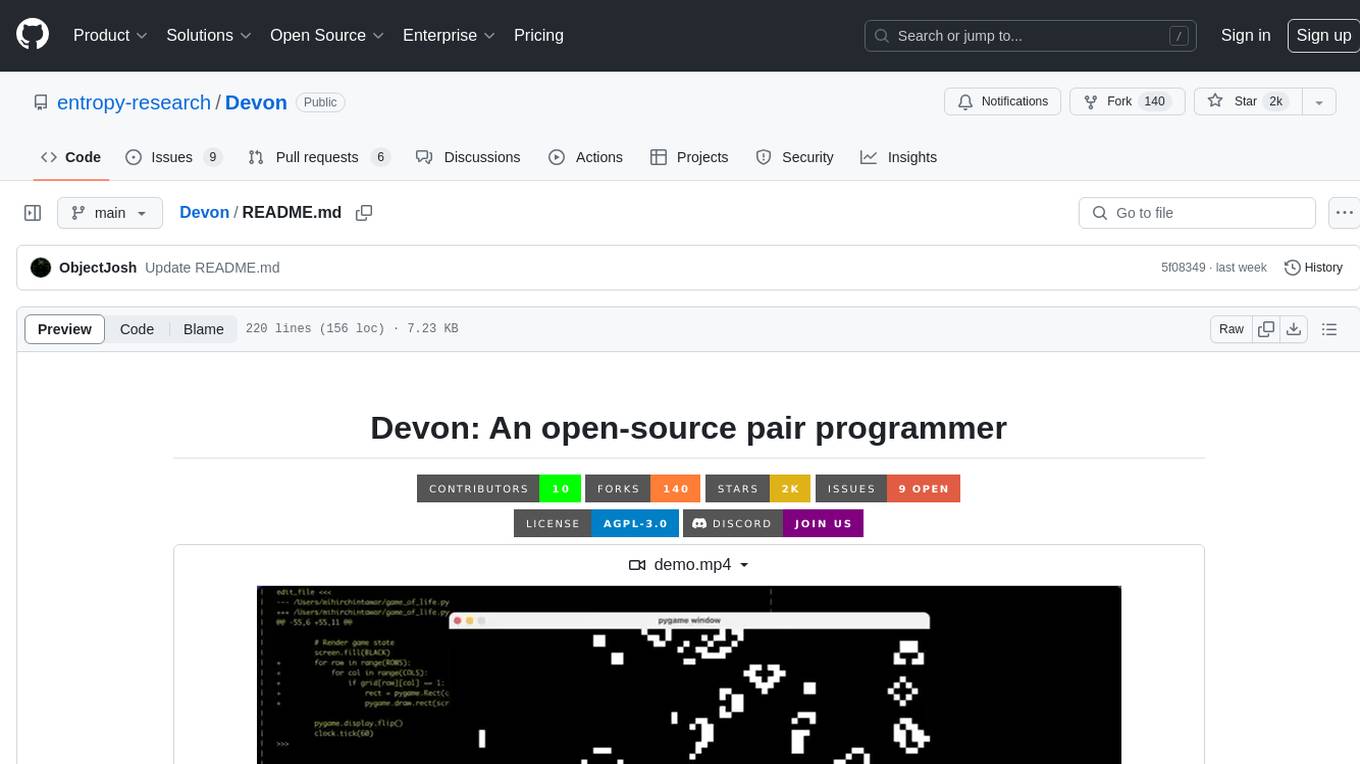
Devon
Devon is an open-source pair programmer tool designed to facilitate collaborative coding sessions. It provides features such as multi-file editing, codebase exploration, test writing, bug fixing, and architecture exploration. The tool supports Anthropic, OpenAI, and Groq APIs, with plans to add more models in the future. Devon is community-driven, with ongoing development goals including multi-model support, plugin system for tool builders, self-hostable Electron app, and setting SOTA on SWE-bench Lite. Users can contribute to the project by developing core functionality, conducting research on agent performance, providing feedback, and testing the tool.
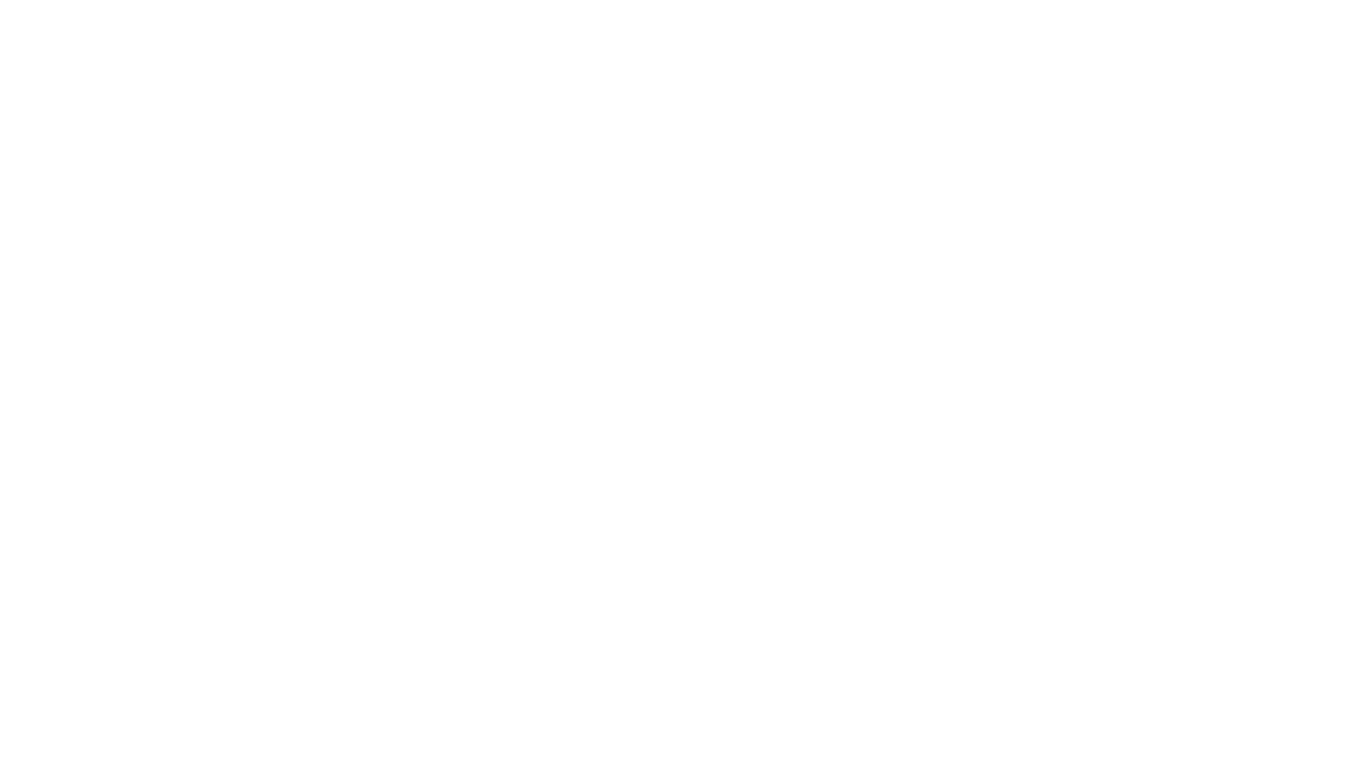
minefield
BitBom Minefield is a tool that uses roaring bit maps to graph Software Bill of Materials (SBOMs) with a focus on speed, air-gapped operation, scalability, and customizability. It is optimized for rapid data processing, operates securely in isolated environments, supports millions of nodes effortlessly, and allows users to extend the project without relying on upstream changes. The tool enables users to manage and explore software dependencies within isolated environments by offline processing and analyzing SBOMs.
For similar tasks
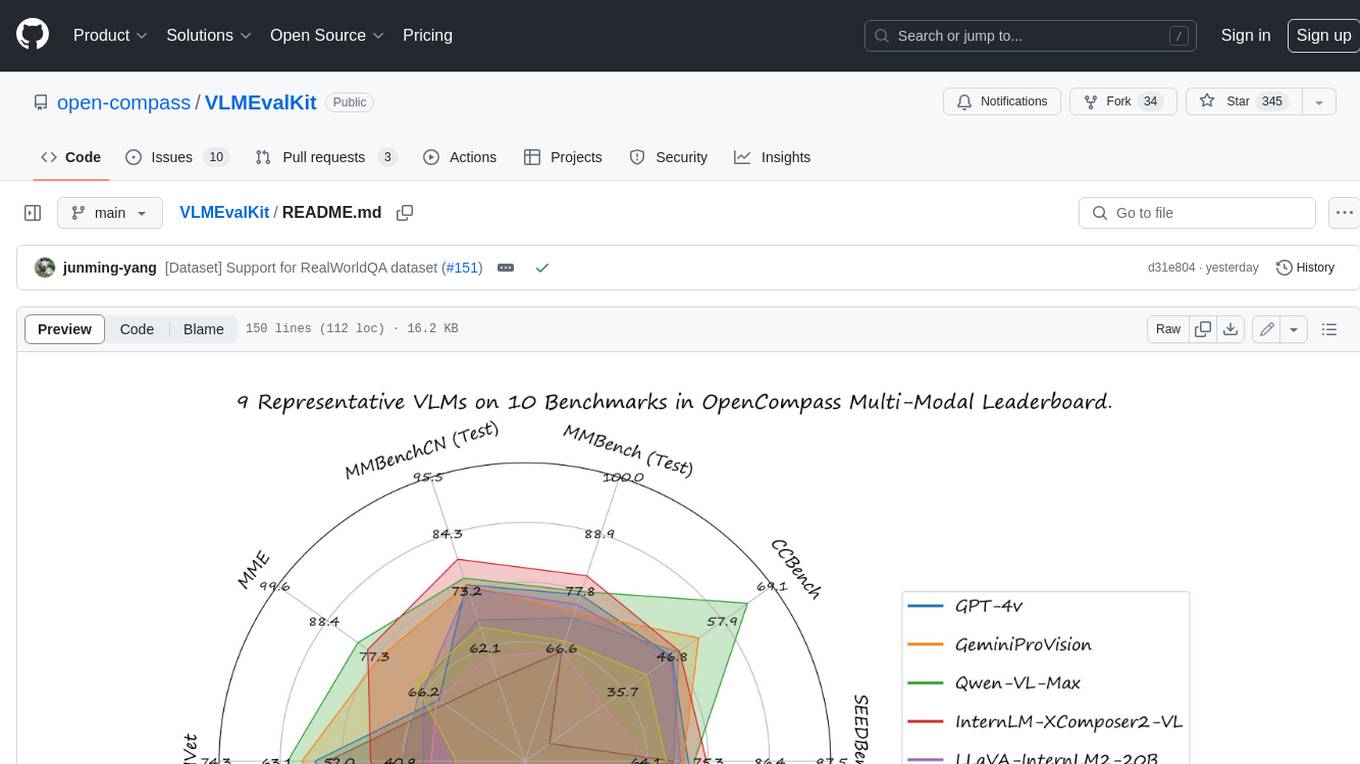
VLMEvalKit
VLMEvalKit is an open-source evaluation toolkit of large vision-language models (LVLMs). It enables one-command evaluation of LVLMs on various benchmarks, without the heavy workload of data preparation under multiple repositories. In VLMEvalKit, we adopt generation-based evaluation for all LVLMs, and provide the evaluation results obtained with both exact matching and LLM-based answer extraction.
For similar jobs
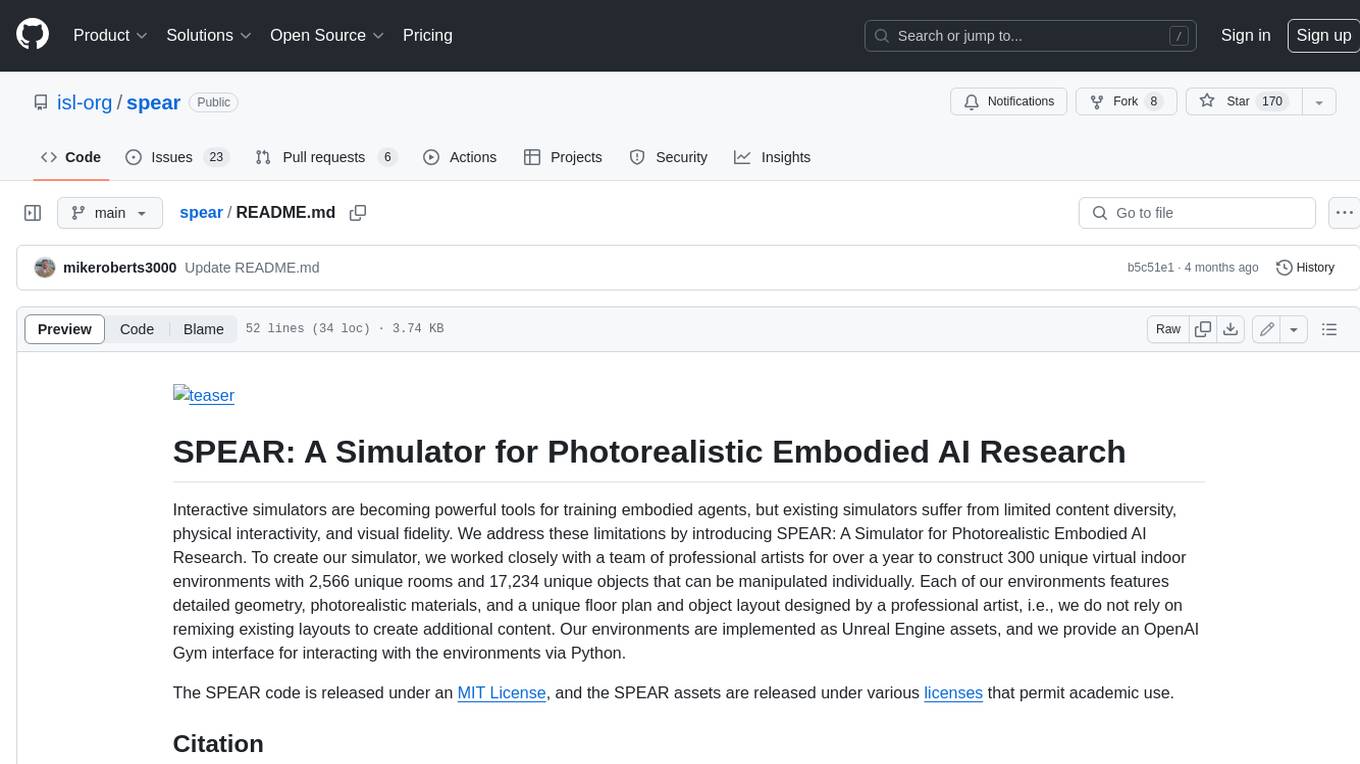
spear
SPEAR (Simulator for Photorealistic Embodied AI Research) is a powerful tool for training embodied agents. It features 300 unique virtual indoor environments with 2,566 unique rooms and 17,234 unique objects that can be manipulated individually. Each environment is designed by a professional artist and features detailed geometry, photorealistic materials, and a unique floor plan and object layout. SPEAR is implemented as Unreal Engine assets and provides an OpenAI Gym interface for interacting with the environments via Python.
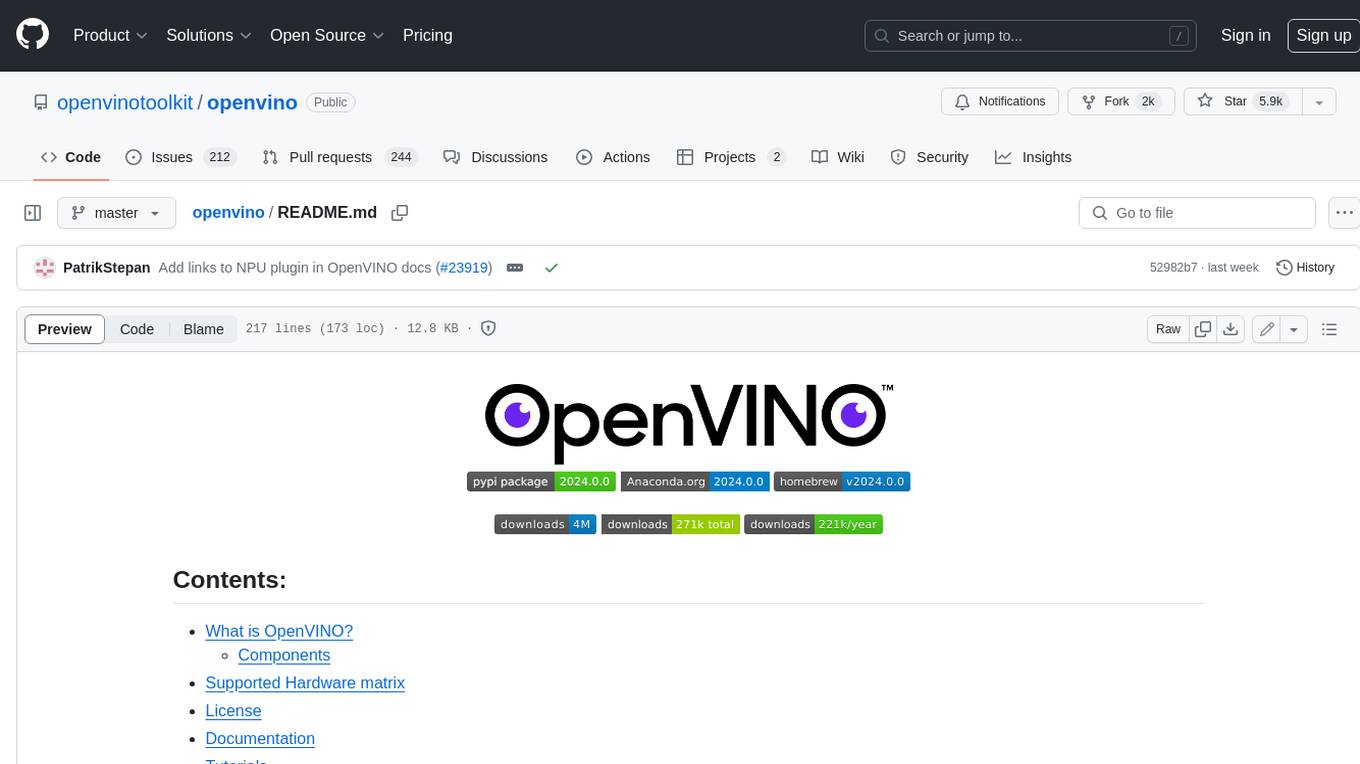
openvino
OpenVINO™ is an open-source toolkit for optimizing and deploying AI inference. It provides a common API to deliver inference solutions on various platforms, including CPU, GPU, NPU, and heterogeneous devices. OpenVINO™ supports pre-trained models from Open Model Zoo and popular frameworks like TensorFlow, PyTorch, and ONNX. Key components of OpenVINO™ include the OpenVINO™ Runtime, plugins for different hardware devices, frontends for reading models from native framework formats, and the OpenVINO Model Converter (OVC) for adjusting models for optimal execution on target devices.
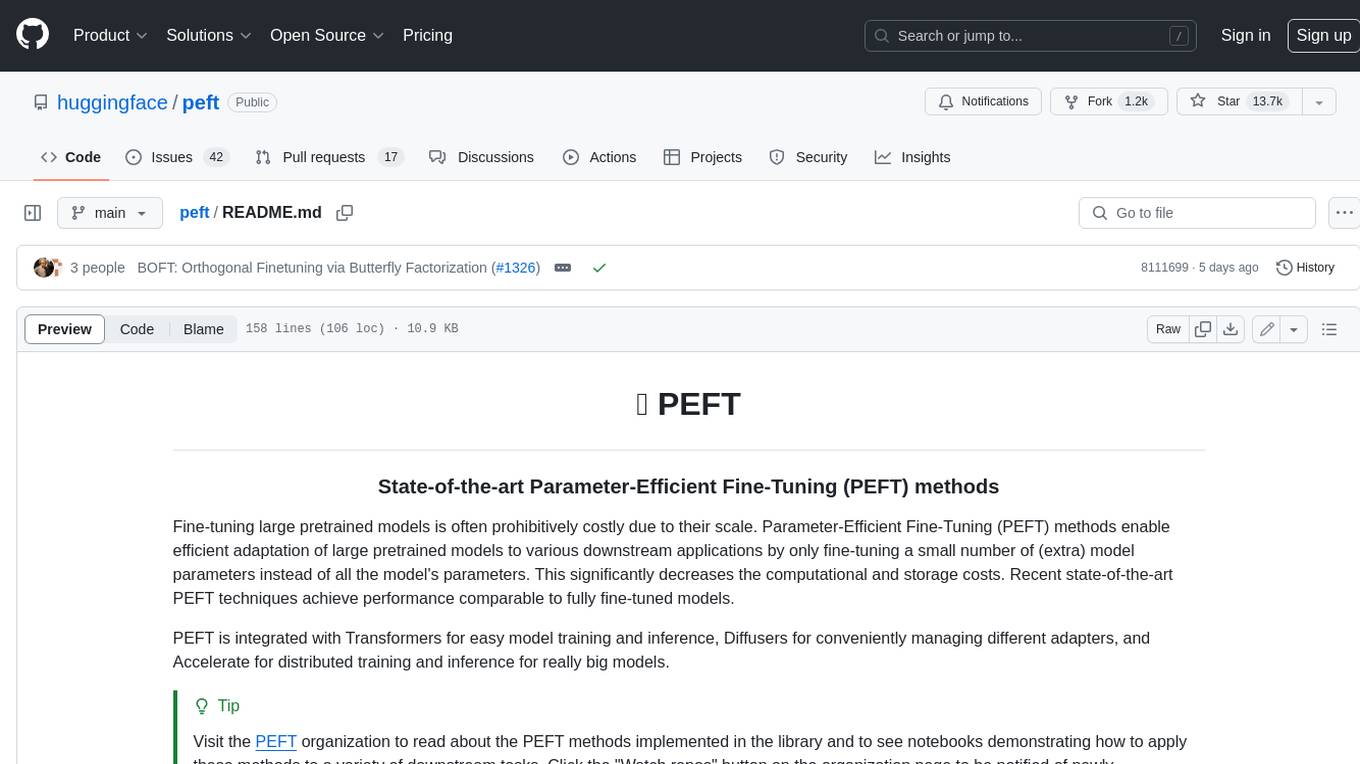
peft
PEFT (Parameter-Efficient Fine-Tuning) is a collection of state-of-the-art methods that enable efficient adaptation of large pretrained models to various downstream applications. By only fine-tuning a small number of extra model parameters instead of all the model's parameters, PEFT significantly decreases the computational and storage costs while achieving performance comparable to fully fine-tuned models.
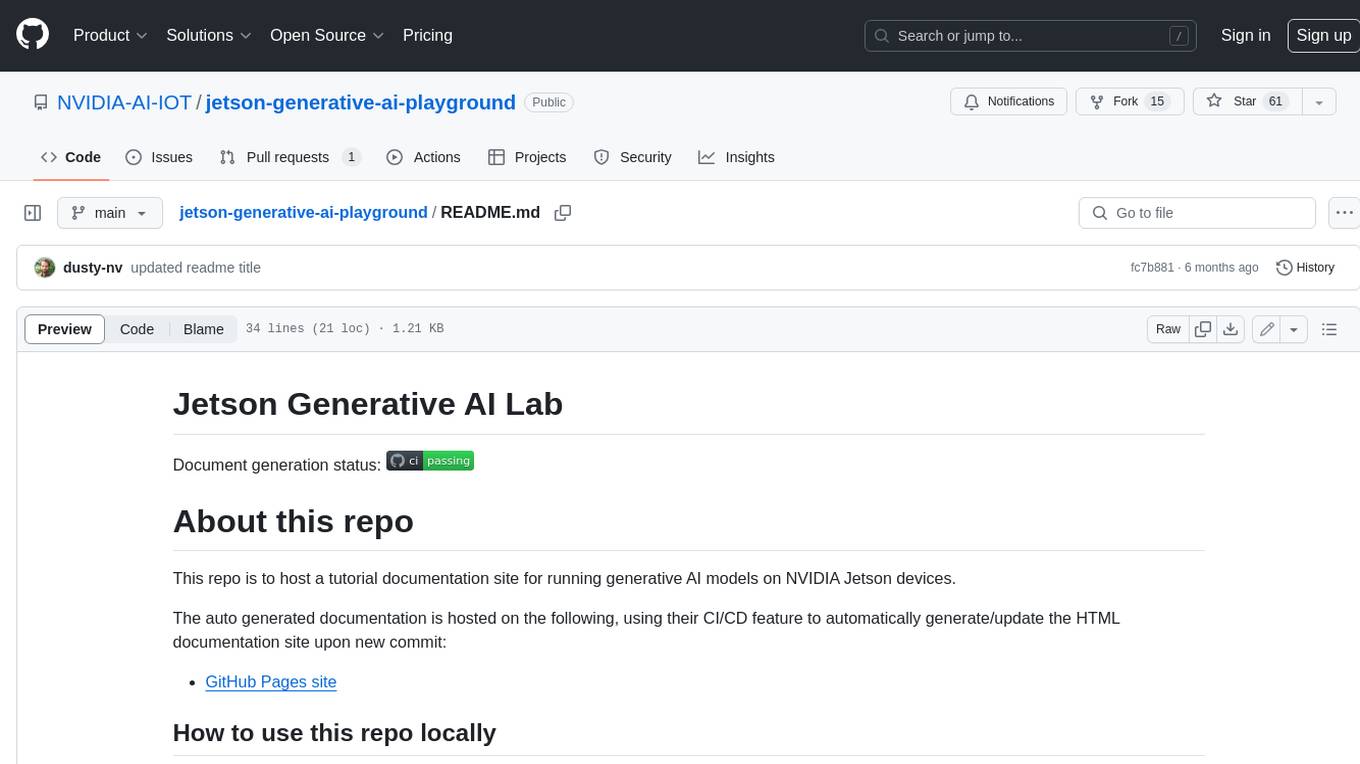
jetson-generative-ai-playground
This repo hosts tutorial documentation for running generative AI models on NVIDIA Jetson devices. The documentation is auto-generated and hosted on GitHub Pages using their CI/CD feature to automatically generate/update the HTML documentation site upon new commits.
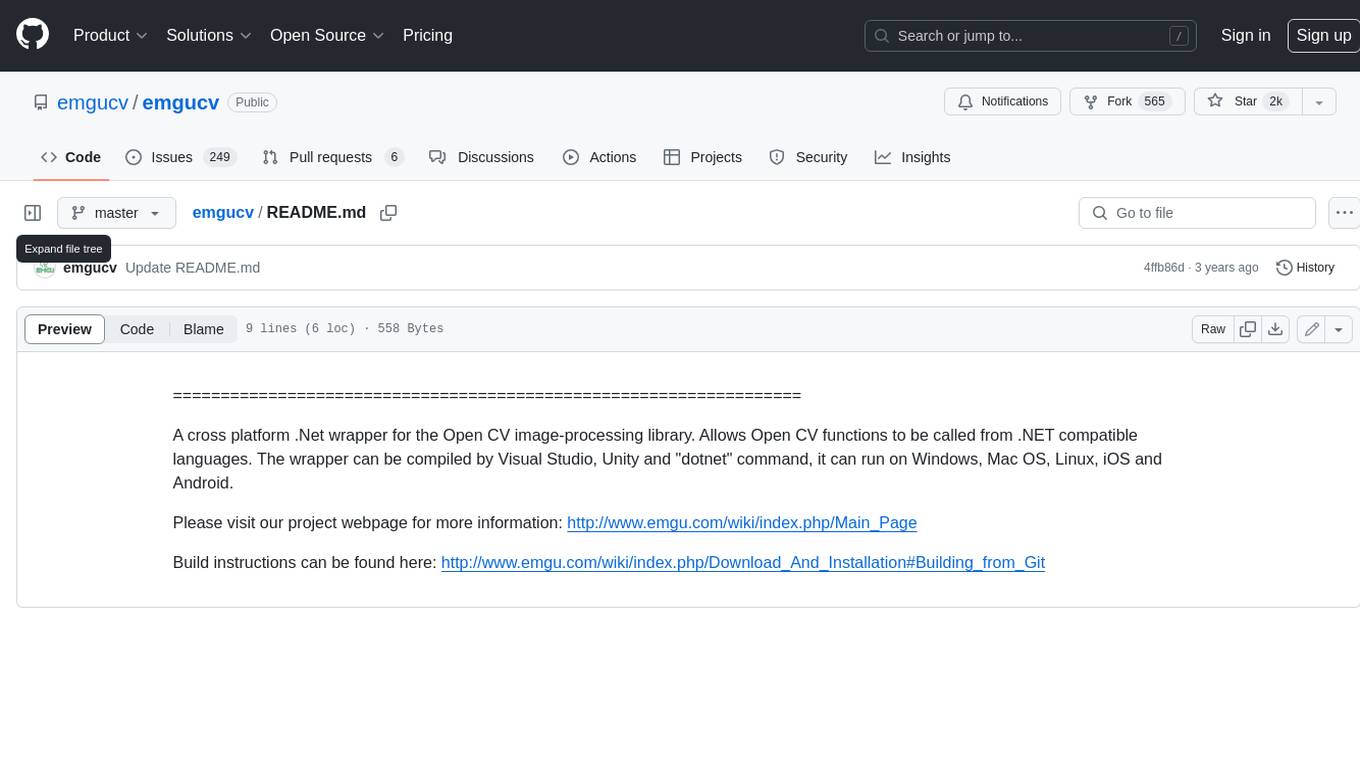
emgucv
Emgu CV is a cross-platform .Net wrapper for the OpenCV image-processing library. It allows OpenCV functions to be called from .NET compatible languages. The wrapper can be compiled by Visual Studio, Unity, and "dotnet" command, and it can run on Windows, Mac OS, Linux, iOS, and Android.
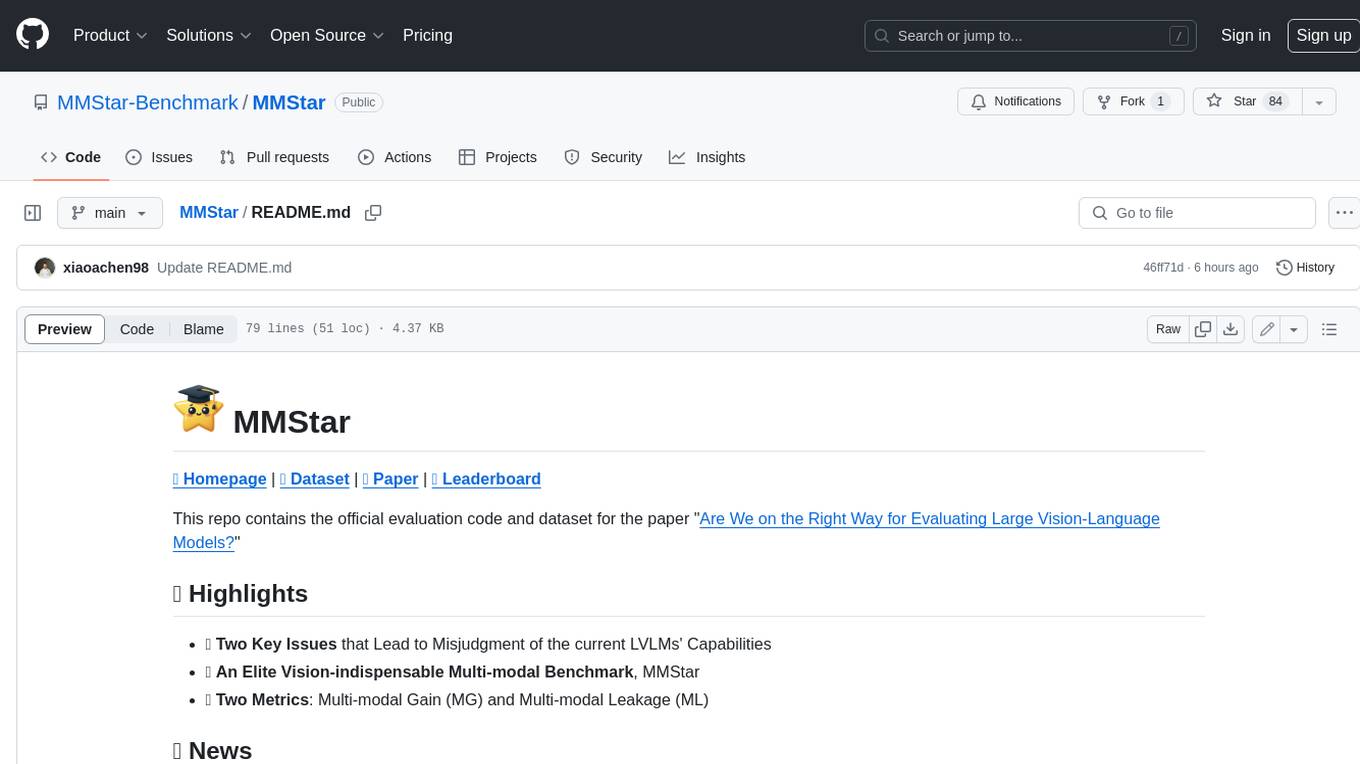
MMStar
MMStar is an elite vision-indispensable multi-modal benchmark comprising 1,500 challenge samples meticulously selected by humans. It addresses two key issues in current LLM evaluation: the unnecessary use of visual content in many samples and the existence of unintentional data leakage in LLM and LVLM training. MMStar evaluates 6 core capabilities across 18 detailed axes, ensuring a balanced distribution of samples across all dimensions.
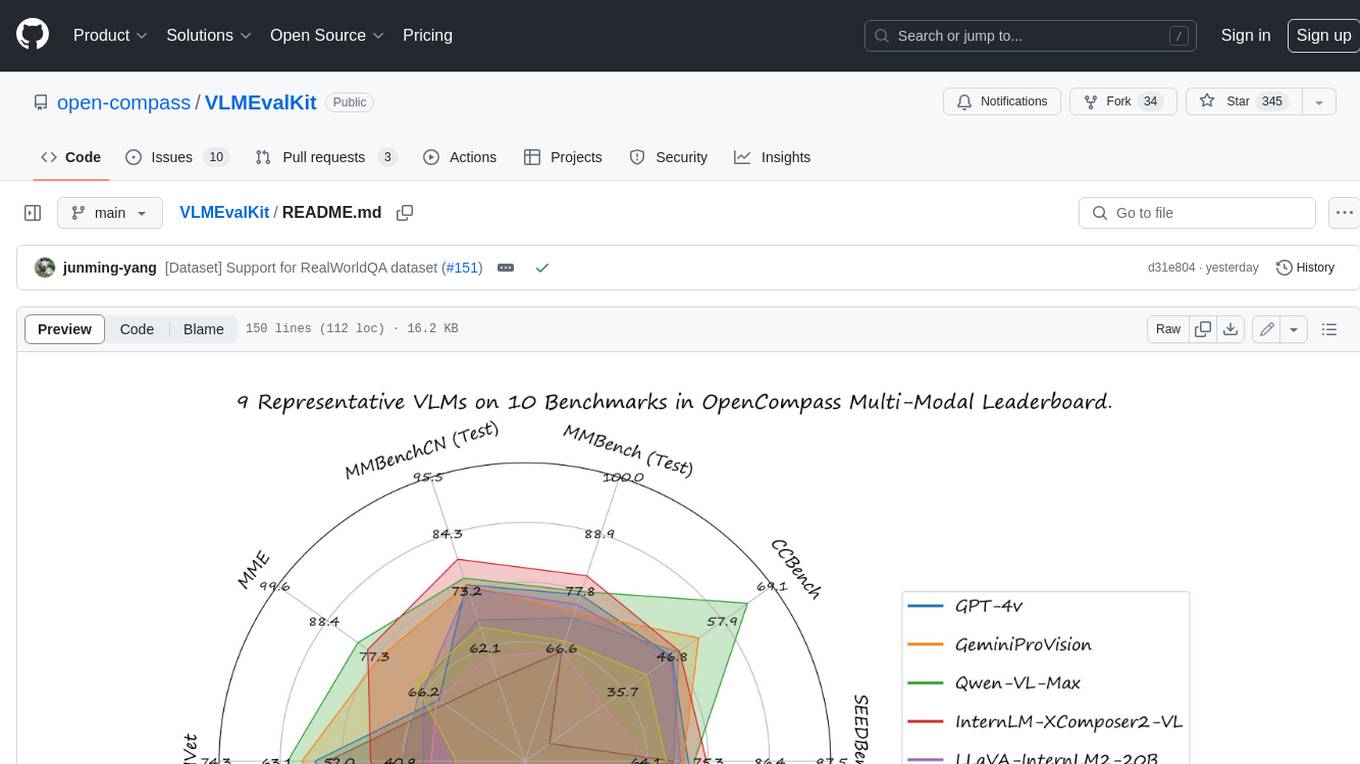
VLMEvalKit
VLMEvalKit is an open-source evaluation toolkit of large vision-language models (LVLMs). It enables one-command evaluation of LVLMs on various benchmarks, without the heavy workload of data preparation under multiple repositories. In VLMEvalKit, we adopt generation-based evaluation for all LVLMs, and provide the evaluation results obtained with both exact matching and LLM-based answer extraction.
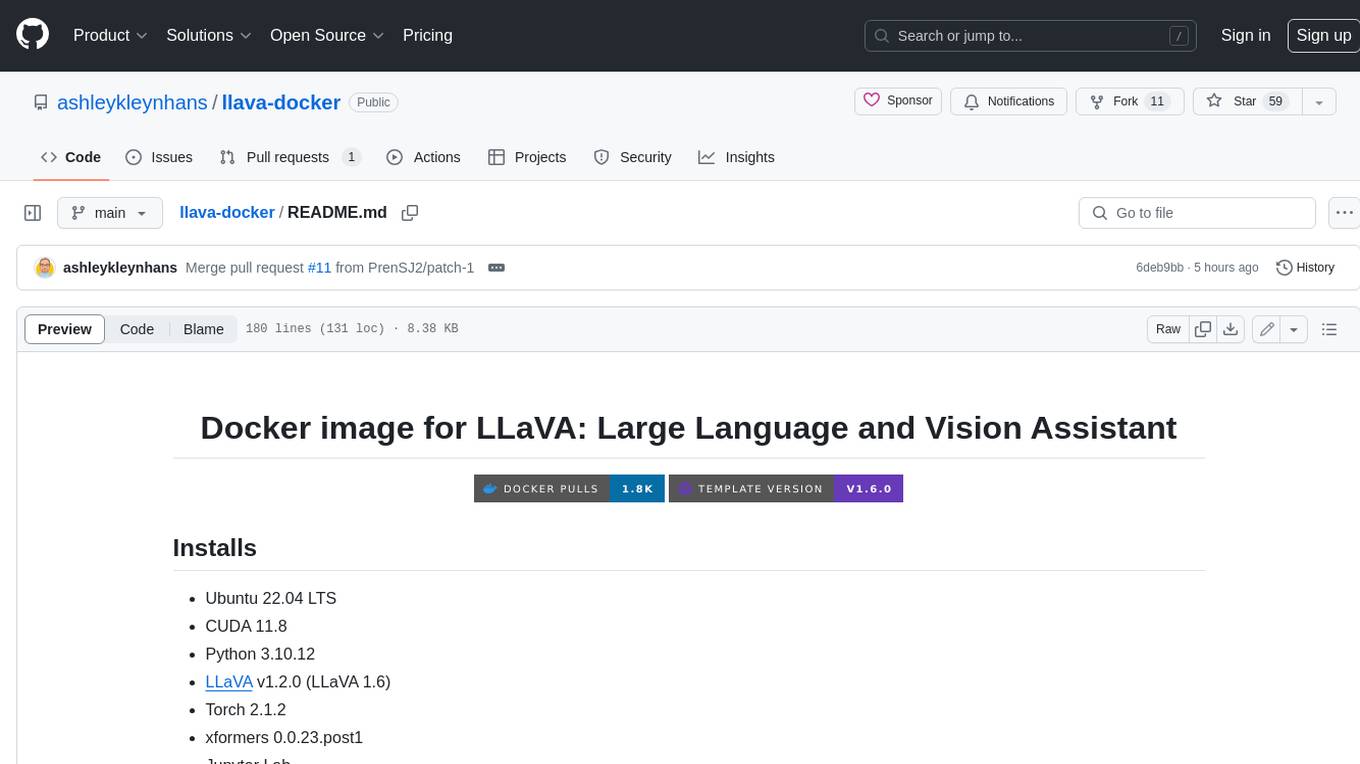
llava-docker
This Docker image for LLaVA (Large Language and Vision Assistant) provides a convenient way to run LLaVA locally or on RunPod. LLaVA is a powerful AI tool that combines natural language processing and computer vision capabilities. With this Docker image, you can easily access LLaVA's functionalities for various tasks, including image captioning, visual question answering, text summarization, and more. The image comes pre-installed with LLaVA v1.2.0, Torch 2.1.2, xformers 0.0.23.post1, and other necessary dependencies. You can customize the model used by setting the MODEL environment variable. The image also includes a Jupyter Lab environment for interactive development and exploration. Overall, this Docker image offers a comprehensive and user-friendly platform for leveraging LLaVA's capabilities.