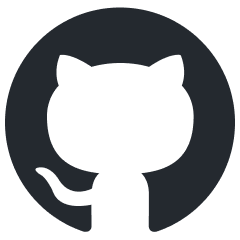
mistreevous
A tool to build and execute behaviour trees in JavaScript and TypeScript
Stars: 82
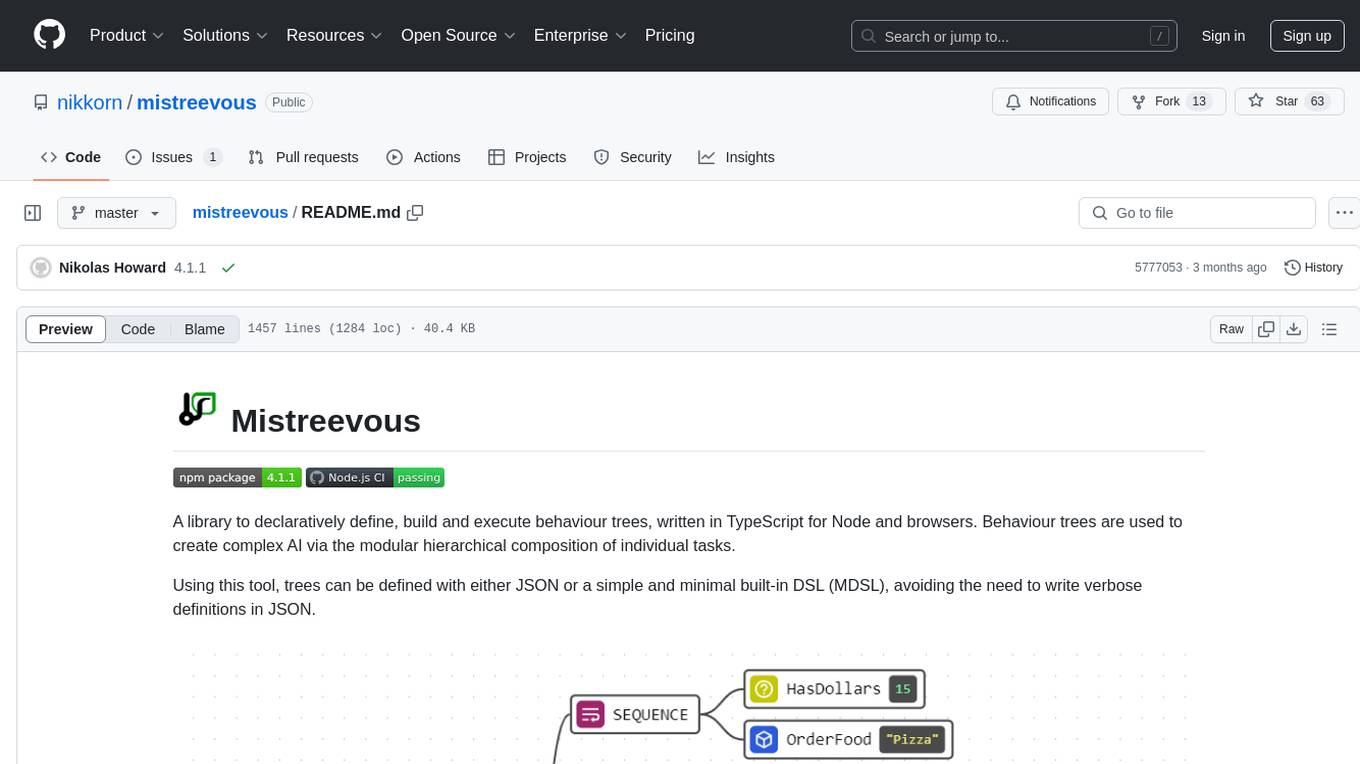
Mistreevous is a library written in TypeScript for Node and browsers, used to declaratively define, build, and execute behaviour trees for creating complex AI. It allows defining trees with JSON or a minimal DSL, providing in-browser editor and visualizer. The tool offers methods for tree state, stepping, resetting, and getting node details, along with various composite, decorator, leaf nodes, callbacks, guards, and global functions/subtrees. Version history includes updates for node types, callbacks, global functions, and TypeScript conversion.
README:
A library to declaratively define, build and execute behaviour trees, written in TypeScript for Node and browsers. Behaviour trees are used to create complex AI via the modular hierarchical composition of individual tasks.
Using this tool, trees can be defined with either JSON or a simple and minimal built-in DSL (MDSL), avoiding the need to write verbose definitions in JSON.
There is an in-browser editor and tree visualiser that you can try HERE
$ npm install --save mistreevous
This package is built using esbuild to target both Node and browsers. If you would like to use this package in a browser you can just use dist/mistreevous.js
or dist/mistreevous.min.js
.
import { State, BehaviourTree } from "mistreevous";
/** Define some behaviour for an agent. */
const definition = `root {
sequence {
action [Walk]
action [Fall]
action [Laugh]
}
}`;
/** Create an agent that we will be modelling the behaviour for. */
const agent = {
Walk: () => {
console.log("walking!");
return State.SUCCEEDED;
},
Fall: () => {
console.log("falling!");
return State.SUCCEEDED;
},
Laugh: () => {
console.log("laughing!");
return State.SUCCEEDED;
},
};
/** Create the behaviour tree, passing our tree definition and the agent that we are modelling behaviour for. */
const behaviourTree = new BehaviourTree(definition, agent);
/** Step the tree. */
behaviourTree.step();
// 'walking!'
// 'falling!
// 'laughing!'
Returns true
if the tree is in the RUNNING
state, otherwise false
.
Gets the current tree state of SUCCEEDED
, FAILED
or RUNNING
.
Carries out a node update that traverses the tree from the root node outwards to any child nodes, skipping those that are already in a resolved state of SUCCEEDED
or FAILED
. After being updated, leaf nodes will have a state of SUCCEEDED
, FAILED
or RUNNING
. Leaf nodes that are left in the RUNNING
state as part of a tree step will be revisited each subsequent step until they move into a resolved state of either SUCCEEDED
or FAILED
, after which execution will move through the tree to the next node with a state of READY
.
Calling this method when the tree is already in a resolved state of SUCCEEDED
or FAILED
will cause it to be reset before tree traversal begins.
Resets the tree from the root node outwards to each nested node, giving each a state of READY
.
Gets the details of every node in the tree, starting from the root. This will include the current state of each node, which is useful for debugging a running tree instance.
The BehaviourTree
constructor can take an options object as an argument, the properties of which are shown below.
Option | Type | Description |
---|---|---|
getDeltaTime | () => number | A function returning a delta time in seconds that is used to calculate the elapsed duration of any wait nodes. If this function is not defined then Date.prototype.getTime() is used instead by default. |
random | () => number | A function returning a floating-point number between 0 (inclusive) and 1 (exclusive). If defined, this function is used to source a pseudo-random number to use in operations such as the selection of active children for any lotto nodes as well as the selection of durations for wait nodes, iterations for repeat nodes and attempts for retry nodes when minimum and maximum bounds are defined. If not defined then Math.random() will be used instead by default. This function can be useful in seeding all random numbers used in the running of a tree instance to make any behaviour completely deterministic. |
onNodeStateChange | (change: NodeStateChange) => void | An event handler that is called whenever the state of a node changes. The change object will contain details of the updated node and will include the previous state and current state. |
Behaviour tree nodes can be in one of the following states:
-
READY A node is in the
READY
state when it has not been visited yet in the execution of the tree. -
RUNNING A node is in the
RUNNING
state when it is still being processed, these nodes will usually represent or encompass a long-running action. -
SUCCEEDED A node is in a
SUCCEEDED
state when it is no longer being processed and has succeeded. -
FAILED A node is in the
FAILED
state when it is no longer being processed but has failed.
Composite nodes wrap one or more child nodes, each of which will be processed in a sequence determined by the type of the composite node. A composite node will remain in the RUNNING
state until it is finished processing the child nodes, after which the state of the composite node will reflect the success or failure of the child nodes.
This composite node will update each child node in sequence. It will move to the SUCCEEDED
state if all of its children have moved to the SUCCEEDED
state and will move to the FAILED
state if any of its children move to the FAILED
state. This node will remain in the RUNNING
state if one of its children remains in the RUNNING
state.
Example
MDSL
root {
sequence {
action [Walk]
action [Fall]
action [Laugh]
}
}
JSON
{
"type": "root",
"child": {
"type": "sequence",
"children": [
{
"type": "action",
"call": "Walk"
},
{
"type": "action",
"call": "Fall"
},
{
"type": "action",
"call": "Laugh"
}
]
}
}
This composite node will update each child node in sequence. It will move to the FAILED
state if all of its children have moved to the FAILED
state and will move to the SUCCEEDED
state if any of its children move to the SUCCEEDED
state. This node will remain in the RUNNING
state if one of its children is in the RUNNING
state.
Example
MDSL
root {
selector {
action [TryThis]
action [ThenTryThis]
action [TryThisLast]
}
}
JSON
{
"type": "root",
"child": {
"type": "selector",
"children": [
{
"type": "action",
"call": "TryThis"
},
{
"type": "action",
"call": "ThenTryThis"
},
{
"type": "action",
"call": "TryThisLast"
}
]
}
}
This composite node will update each child node concurrently. It will move to the SUCCEEDED
state if all of its children have moved to the SUCCEEDED
state and will move to the FAILED
state if any of its children move to the FAILED
state, otherwise it will remain in the RUNNING
state.
Example
MDSL
root {
parallel {
action [RubBelly]
action [PatHead]
}
}
JSON
{
"type": "root",
"child": {
"type": "parallel",
"children": [
{
"type": "action",
"call": "RubBelly"
},
{
"type": "action",
"call": "PatHead"
}
]
}
}
This composite node will update each child node concurrently. It will move to the SUCCEEDED
state if any of its children have moved to the SUCCEEDED
state and will move to the FAILED
state if all of its children move to the FAILED
state, otherwise it will remain in the RUNNING
state.
Example
MDSL
root {
race {
action [UnlockDoor]
action [FindAlternativePath]
}
}
JSON
{
"type": "root",
"child": {
"type": "race",
"children": [
{
"type": "action",
"call": "UnlockDoor"
},
{
"type": "action",
"call": "FindAlternativePath"
}
]
}
}
This composite node will update each child node concurrently. It will stay in the RUNNING
state until all of its children have moved to either the SUCCEEDED
or FAILED
state, after which this node will move to the SUCCEEDED
state if any of its children have moved to the SUCCEEDED
state, otherwise it will move to the FAILED
state.
Example
MDSL
root {
all {
action [Reload]
action [MoveToCover]
}
}
JSON
{
"type": "root",
"child": {
"type": "all",
"children": [
{
"type": "action",
"call": "Reload"
},
{
"type": "action",
"call": "MoveToCover"
}
]
}
}
This composite node will select a single child at random to run as the active running node. The state of this node will reflect the state of the active child. Example
MDSL
root {
lotto {
action [MoveLeft]
action [MoveRight]
}
}
JSON
{
"type": "root",
"child": {
"type": "lotto",
"children": [
{
"type": "action",
"call": "MoveLeft"
},
{
"type": "action",
"call": "MoveRight"
}
]
}
}
A probability weight can be defined for each child node as an optional integer node argument in MDSL, influencing the likelihood that a particular child will be picked. In JSON this would be done by setting a value of an array containing the integer weight values for the weights
property.
Example
MDSL
root {
lotto [10,5,3,1] {
action [CommonAction]
action [UncommonAction]
action [RareAction]
action [VeryRareAction]
}
}
JSON
{
"type": "root",
"child": {
"type": "lotto",
"children": [
{
"type": "action",
"call": "CommonAction"
},
{
"type": "action",
"call": "UncommonAction"
},
{
"type": "action",
"call": "RareAction"
},
{
"type": "action",
"call": "VeryRareAction"
}
],
"weights": [10, 5, 3, 1]
}
}
A decorator node is similar to a composite node, but it can only have a single child node. The state of a decorator node is usually some transformation of the state of the child node. Decorator nodes are also used to repeat or terminate the execution of a particular node.
This decorator node represents the root of a behaviour tree and cannot be the child of another composite node.
The state of a root node will reflect the state of its child node.
MDSL
root {
action [Dance]
}
JSON
{
"type": "root",
"child": {
"type": "action",
"call": "Dance"
}
}
Additional named root nodes can be defined and reused throughout a definition. Other root nodes can be referenced via the branch node. Exactly one root node must be left unnamed, this root node will be used as the main root node for the entire tree.
MDSL
root {
branch [SomeOtherTree]
}
root [SomeOtherTree] {
action [Dance]
}
JSON
[
{
"type": "root",
"child": {
"type": "branch",
"ref": "SomeOtherTree"
}
},
{
"type": "root",
"id": "SomeOtherTree",
"child": {
"type": "action",
"call": "Dance",
"args": []
}
}
]
This decorator node will repeat the execution of its child node if the child moves to the SUCCEEDED
state. It will do this until either the child moves to the FAILED
state, at which point the repeat node will move to the FAILED
state, or the maximum number of iterations is reached, which moves the repeat node to the SUCCEEDED
state. This node will be in the RUNNING
state if its child is also in the RUNNING
state, or if further iterations need to be made.
Example
The maximum number of iterations can be defined as a single integer iteration argument in MDSL, or by setting the iterations
property in JSON. In the example below, we would be repeating the action SomeAction 5 times.
MDSL
root {
repeat [5] {
action [SomeAction]
}
}
JSON
{
"type": "root",
"child": {
"type": "repeat",
"iterations": 5,
"child": {
"type": "action",
"call": "SomeAction"
}
}
}
The number of iterations to make can be selected at random within a lower and upper bound if these are defined as two integer arguments in MDSL, or by setting a value of an array containing two integer values for the iterations
property in JSON. In the example below, we would be repeating the action SomeAction between 1 and 5 times.
MDSL
root {
repeat [1,5] {
action [SomeAction]
}
}
JSON
{
"type": "root",
"child": {
"type": "repeat",
"iterations": [1, 5],
"child": {
"type": "action",
"call": "SomeAction"
}
}
}
The maximum number of iterations to make can be omitted. This would result in the child node being run infinitely, as can be seen in the example below.
MDSL
root {
repeat {
action [SomeAction]
}
}
JSON
{
"type": "root",
"child": {
"type": "repeat",
"child": {
"type": "action",
"call": "SomeAction"
}
}
}
This decorator node will repeat the execution of its child node if the child moves to the FAILED
state. It will do this until either the child moves to the SUCCEEDED
state, at which point the retry node will move to the SUCCEEDED
state or the maximum number of attempts is reached, which moves the retry node to the FAILED
state. This node will be in a RUNNING
state if its child is also in the RUNNING
state, or if further attempts need to be made.
The maximum number of attempts can be defined as a single integer attempt argument in MDSL, or by setting the attempts
property in JSON. In the example below, we would be retrying the action SomeAction 5 times.
MDSL
root {
retry [5] {
action [SomeAction]
}
}
JSON
{
"type": "root",
"child": {
"type": "retry",
"attempts": 5,
"child": {
"type": "action",
"call": "SomeAction"
}
}
}
The number of attempts to make can be selected at random within a lower and upper bound if these are defined as two integer arguments in MDSL, or by setting a value of an array containing two integer values for the attempts
property in JSON. In the example below, we would be retrying the action SomeAction between 1 and 5 times.
MDSL
root {
retry [1,5] {
action [SomeAction]
}
}
JSON
{
"type": "root",
"child": {
"type": "retry",
"attempts": [1, 5],
"child": {
"type": "action",
"call": "SomeAction"
}
}
}
The maximum number of attempts to make can be omitted. This would result in the child node being run infinitely until it moves to the SUCCEEDED
state, as can be seen in the example below.
MDSL
root {
retry {
action [SomeAction]
}
}
JSON
{
"type": "root",
"child": {
"type": "retry",
"child": {
"type": "action",
"call": "SomeAction"
}
}
}
This decorator node will move to the SUCCEEDED
state when its child moves to the FAILED
state, and it will move to the FAILED
if its child moves to the SUCCEEDED
state. This node will remain in the RUNNING
state if its child is in the RUNNING
state.
Example
MDSL
root {
flip {
action [SomeAction]
}
}
JSON
{
"type": "root",
"child": {
"type": "flip",
"child": {
"type": "action",
"call": "SomeAction"
}
}
}
This decorator node will move to the SUCCEEDED
state when its child moves to either the FAILED
state or the SUCCEEDED
state. This node will remain in the RUNNING
state if its child is in the RUNNING
state.
Example
MDSL
root {
succeed {
action [SomeAction]
}
}
JSON
{
"type": "root",
"child": {
"type": "succeed",
"child": {
"type": "action",
"call": "SomeAction"
}
}
}
This decorator node will move to the FAILED
state when its child moves to either the FAILED
state or the SUCCEEDED
state. This node will remain in the RUNNING
state if its child is in the RUNNING
state.
Example
MDSL
root {
fail {
action [SomeAction]
}
}
JSON
{
"type": "root",
"child": {
"type": "fail",
"child": {
"type": "action",
"call": "SomeAction"
}
}
}
Leaf nodes are the lowest-level node type and cannot be the parent of other child nodes.
An action node represents an action that can be completed immediately as part of a single tree step, or ongoing behaviour that can take a prolonged amount of time and may take multiple tree steps to complete. Each action node will correspond to some action that can be carried out by the agent, where the first action node argument (if using MDSL, or the call
property if using JSON) will be an identifier matching the name of the corresponding agent action function or globally registered function.
Example
An agent action function can optionally return a value of State.SUCCEEDED, State.FAILED or State.RUNNING. If the State.SUCCEEDED or State.FAILED state is returned, then the action will move to that state.
MDSL
root {
action [Attack]
}
JSON
{
"type": "root",
"child": {
"type": "action",
"call": "Attack"
}
}
const agent = {
//...
Attack: () => {
// If we do not have a weapon then we cannot attack.
if (!this.isHoldingWeapon()) {
// We have failed to carry out an attack!
return Mistreevous.State.FAILED;
}
// ... Attack with swiftness and precision ...
// We have carried out our attack.
return Mistreevous.State.SUCCEEDED;
}
// ...
};
If no value or a value of State.RUNNING is returned from the action function the action node will move into the RUNNING
state and no following nodes will be processed as part of the current tree step. In the example below, any action node that references WalkToPosition will remain in the RUNNING
state until the target position is reached.
const agent = {
//...
WalkToPosition: () => {
// ... Walk towards the position we are trying to reach ...
// Check whether we have finally reached the target position.
if (this.isAtTargetPosition()) {
// We have finally reached the target position!
return Mistreevous.State.SUCCEEDED;
}
// We have not reached the target position yet.
return Mistreevous.State.RUNNING;
}
// ...
};
Further steps of the tree will resume processing from leaf nodes that were left in the RUNNING
state until those nodes move to either the SUCCEEDED
or FAILED
state or processing of the running branch is aborted via a guard.
As well as returning a finished action state from an action function, you can also return a promise that should eventually resolve with a finished state of State.SUCCEEDED or State.FAILED as its value. The action will remain in the RUNNING
state until the promise is fulfilled, and any following tree steps will not call the action function again.
Example
const agent = {
//...
SomeAsyncAction: () => {
return new Promise(function(resolve, reject) {
setTimeout(function() {
resolve(Mistreevous.State.SUCCEEDED);
}, 5000);
});
}
// ...
};
Arguments can optionally be passed to agent action functions. In MDSL these optional arguments must be defined after the action name identifier argument and can be a number
, string
, boolean
or null
. If using JSON then these arguments are defined in an args
array and these arguments can be any valid JSON.
Example
MDSL
root {
action [Say, "hello world", 5, true]
}
JSON
{
"type": "root",
"child": {
"type": "action",
"call": "Say",
"args": ["hello world", 5, true]
}
}
const agent = {
//...
Say: (dialog, times = 1, sayLoudly = false) =>
{
for (var index = 0; index < times; index++) {
showDialog(sayLoudly ? dialog.toUpperCase() + "!!!" : dialog);
}
return Mistreevous.State.SUCCEEDED;
}
// ...
};
A Condition node will immediately move into either a SUCCEEDED
or FAILED
state based on the boolean result of calling either an agent function or globally registered function. The first condition node argument will be an identifier matching the name of the corresponding agent condition function or globally registered function (if using MDSL, or the call
property if using JSON).
Example
MDSL
root {
sequence {
condition [HasWeapon]
action [Attack]
}
}
JSON
{
"type": "root",
"child": {
"type": "sequence",
"children": [
{
"type": "condition",
"call": "HasWeapon"
},
{
"type": "action",
"call": "Attack"
}
]
}
}
const agent = {
//...
HasWeapon: () => this.isHoldingWeapon(),
//...
Attack: () => this.attackPlayer(),
// ...
};
Arguments can optionally be passed to agent condition functions in the same way as action nodes. In MDSL these optional arguments must be defined after the condition name identifier argument, and can be a number
, string
, boolean
or null
, or can be any valid JSON when using a JSON definition.
Example
MDSL
root {
sequence {
condition [HasItem, "potion"]
}
}
JSON
{
"type": "root",
"child": {
"type": "sequence",
"children": [
{
"type": "condition",
"call": "HasItem",
"args": ["potion"]
}
]
}
}
const agent = {
//...
HasItem: (itemName) => this.inventory.includes(itemName),
// ...
};
A wait node will remain in a RUNNING
state for a specified duration, after which it will move into the SUCCEEDED
state. The duration in milliseconds can be defined as an optional single integer node argument in MDSL, or by setting a value for the duration
property in JSON.
Example
MDSL
root {
repeat {
sequence {
action [FireWeapon]
wait [2000]
}
}
}
JSON
{
"type": "root",
"child": {
"type": "repeat",
"child": {
"type": "sequence",
"children": [
{
"type": "action",
"call": "FireWeapon"
},
{
"type": "wait",
"duration": 2000
}
]
}
}
}
In the above example, we are using a wait node to wait 2 seconds between each run of the FireWeapon action.
The duration to wait in milliseconds can also be selected at random within a lower and upper bound if these are defined as two integer node arguments in MDSL, or by setting a value of an array containing two integer values for the duration
property in JSON. In the example below, we would run the PickUpProjectile action and then wait for 2 to 8 seconds before running the ThrowProjectile action.
Example
MDSL
root {
sequence {
action [PickUpProjectile]
wait [2000, 8000]
action [ThrowProjectile]
}
}
JSON
{
"type": "root",
"child": {
"type": "sequence",
"children": [
{
"type": "action",
"call": "PickUpProjectile"
},
{
"type": "wait",
"duration": [2000, 8000]
},
{
"type": "action",
"call": "ThrowProjectile"
}
]
}
}
If no duration is defined then the wait node will remain in the RUNNING
state indefinitely until it is aborted.
Example
MDSL
root {
wait
}
JSON
{
"type": "root",
"child": {
"type": "wait"
}
}
Named root nodes can be referenced using the branch node. This node acts as a placeholder that will be replaced by the child node of the referenced root node. All definitions below are synonymous. Example
MDSL
root {
branch [SomeOtherTree]
}
root [SomeOtherTree] {
action [Dance]
}
root {
action [Dance]
}
JSON
[
{
"type": "root",
"child": {
"type": "branch",
"ref": "SomeOtherTree"
}
},
{
"type": "root",
"id": "SomeOtherTree",
"child": {
"type": "action",
"call": "Dance"
}
}
]
{
"type": "root",
"child": {
"type": "action",
"call": "Dance"
}
}
Callbacks can be defined for tree nodes and will be invoked as the node is processed during a tree step. Any number of callbacks can be attached to a node as long as there are not multiple callbacks of the same type. Example
Optional arguments can be defined for callback functions in the same way as action and condition functions.
An entry callback defines an agent function or globally registered function to call whenever the associated node moves into the RUNNING
state when it is first visited.
MDSL
root {
sequence entry(StartWalkingAnimation) {
action [WalkNorthOneSpace]
action [WalkEastOneSpace]
action [WalkSouthOneSpace]
action [WalkWestOneSpace]
}
}
JSON
{
"type": "root",
"child": {
"type": "sequence",
"entry": {
"call": "StartWalkingAnimation"
},
"children": [
{
"type": "action",
"call": "WalkNorthOneSpace"
},
{
"type": "action",
"call": "WalkEastOneSpace"
},
{
"type": "action",
"call": "WalkSouthOneSpace"
},
{
"type": "action",
"call": "WalkWestOneSpace"
}
]
}
}
An exit callback defines an agent function or globally registered function to call whenever the associated node moves to a state of SUCCEEDED
or FAILED
or is aborted. A results object is passed to the referenced function containing the succeeded and aborted boolean properties.
MDSL
root {
sequence entry(StartWalkingAnimation) exit(StopWalkingAnimation) {
action [WalkNorthOneSpace]
action [WalkEastOneSpace]
action [WalkSouthOneSpace]
action [WalkWestOneSpace]
}
}
JSON
{
"type": "root",
"child": {
"type": "sequence",
"entry": {
"call": "StartWalkingAnimation"
},
"exit": {
"call": "StopWalkingAnimation"
},
"children": [
{
"type": "action",
"call": "WalkNorthOneSpace"
},
{
"type": "action",
"call": "WalkEastOneSpace"
},
{
"type": "action",
"call": "WalkSouthOneSpace"
},
{
"type": "action",
"call": "WalkWestOneSpace"
}
]
}
}
A step callback defines an agent function or globally registered function to call whenever the associated node is updated as part of a tree step.
MDSL
root {
sequence step(OnMoving) {
action [WalkNorthOneSpace]
action [WalkEastOneSpace]
action [WalkSouthOneSpace]
action [WalkWestOneSpace]
}
}
JSON
{
"type": "root",
"child": {
"type": "sequence",
"step": {
"call": "OnMoving"
},
"children": [
{
"type": "action",
"call": "WalkNorthOneSpace"
},
{
"type": "action",
"call": "WalkEastOneSpace"
},
{
"type": "action",
"call": "WalkSouthOneSpace"
},
{
"type": "action",
"call": "WalkWestOneSpace"
}
]
}
}
Arguments can optionally be passed to agent callback functions and can be a number
, string
, boolean
or null
if using MDSL, or any valid JSON when using a JSON definition.
MDSL
root {
action [Walk] entry(OnMovementStart, "walking")
}
JSON
{
"type": "root",
"child": {
"type": "action",
"call": "Walk",
"entry": {
"call": "OnMovementStart",
"args": [
"walking"
]
}
}
}
A guard defines a condition that must be met in order for the associated node to remain active. Any nodes in the RUNNING
state will have their guard condition evaluated for each leaf node update and will move to the FAILED
state if the guard condition is not met.
Example
This functionality is useful as a means of aborting long-running actions or branches that span across multiple steps of the tree.
MDSL
root {
wait while(CanWait)
}
JSON
{
"type": "root",
"child": {
"type": "wait",
"while": {
"call": "CanWait"
}
}
}
In the above example, we have a wait node that waits indefinitely. We are using a while guard to give up on waiting if the guard function CanWait returns false during a tree step.
Arguments can optionally be passed to agent guard functions and can be a number
, string
, boolean
or null
if using MDSL, or any valid JSON when using a JSON definition.
MDSL
root {
action [Run] while(HasItemEquipped, "running-shoes")
}
root {
action [Gamble] until(HasGold, 1000)
}
JSON
{
"type": "root",
"child": {
"type": "action",
"call": "Run",
"while": {
"call": "HasItemEquipped",
"args": [
"running-shoes"
]
}
}
}
{
"type": "root",
"child": {
"type": "action",
"call": "Gamble",
"until": {
"call": "HasGold",
"args": [
1000
]
}
}
}
A while guard will be satisfied as long as its condition evaluates to true.
MDSL
root {
sequence while(IsWandering) {
action [Whistle]
wait [5000]
action [Yawn]
wait [5000]
}
}
JSON
{
"type": "root",
"child": {
"type": "sequence",
"while": {
"call": "IsWandering"
},
"children": [
{
"type": "action",
"call": "Whistle"
},
{
"type": "wait",
"duration": 5000
},
{
"type": "action",
"call": "Yawn"
},
{
"type": "wait",
"duration": 5000
}
]
}
}
An until guard will be satisfied as long as its condition evaluates to false.
MDSL
root {
sequence until(CanSeePlayer) {
action [LookLeft]
wait [5000]
action [LookRight]
wait [5000]
}
}
JSON
{
"type": "root",
"child": {
"type": "sequence",
"until": {
"call": "CanSeePlayer"
},
"children": [
{
"type": "action",
"call": "LookLeft"
},
{
"type": "wait",
"duration": 5000
},
{
"type": "action",
"call": "LookRight"
},
{
"type": "wait",
"duration": 5000
}
]
}
}
When dealing with multiple agents, each with its own behaviour tree instance, it can often be useful to have functions and subtrees that can be registered globally once and referenced by any instance.
We can globally register a subtree that can be referenced from any behaviour tree via a branch node. Example
/** Register the global subtree for some celebratory behaviour. We can also pass a JSON defintion here. */
BehaviourTree.register("Celebrate", `root {
sequence {
action [Jump]
action [Say, "Yay!"]
action [Jump]
action [Say, "We did it!"]
}
}`);
/** Define some behaviour for an agent that references our registered 'Celebrate' subtree. */
const definition = `root {
sequence {
action [AttemptDifficultTask]
branch [Celebrate]
}
}`;
/** Create our agent behaviour tree. */
const agentBehaviourTree = new BehaviourTree(definition, agent);
We can globally register functions to be invoked in place of any agent instance functions, these functions can be referenced throughout a tree definition anywhere that we can reference an agent instance function. The primary difference between these globally registered functions and any agent instance functions is that all global functions that are invoked will take the invoking agent object as the first argument, followed by any optional arguments. Example
In a situation where a tree will reference a function by name that is defined on both the agent instance as well as a function that is registered globally, the agent instance function will take precedence.
/** Register the 'speak' global function that any agent tree can invoke for an action. */
BehaviourTree.register("Speak", (agent, text) => {
showInfoToast(`${agent.getName()}: ${text}`);
return State.SUCCEEDED;
});
/** Register the 'IsSimulationRunning' global function that any agent tree can invoke for a condition. */
BehaviourTree.register("IsSimulationRunning", (agent) => {
return simulation.isRunning();
});
/** Define some behaviour for an agent that references our registered functions. */
const definition = `root {
sequence {
condition [IsSimulationRunning]
action [Speak, "I still have work to do"]
}
}`;
/** Create our agent behaviour tree. */
const agentBehaviourTree = new BehaviourTree(definition, agent);
Behavior trees for AI: How they work A great overview of behaviour trees, tackling the basic concepts.
Designing AI Agents’ Behaviors with Behavior Trees A practical look at behaviour trees and a good example of modelling behaviour for agents in a game of Pacman.
Version | Notes |
---|---|
4.2.0 | Added support for single and multi line comments in MDSL using /* ... */ syntax |
4.1.1 | Added linter and updated dependencies |
4.1.0 | Added Race and All node types |
Added onNodeStateChange callback to behaviour tree options | |
Added getTreeNodeDetails method to BehaviourTree | |
4.0.0 | Added support for JSON tree definitions |
Added validateDefinition function to use in validating JSON/MDSL definitions | |
Added convertMDSLToJSON function to convert existing MDSL definitions to JSON | |
Tidied up error handling for agent and registered function invocation | |
Action functions can now explictly return a value of State.RUNNING instead of having to return undefined | |
Fixed issue where rejected action function promises were not handled correctly | |
Fixed issue where registered functions were called with incorrect arguments | |
Fixed some typings | |
Added a BUNCH of tests | |
3.2.0 | The 'random' function option is used for iteration and attempt selection for repeat and retry nodes respectively when minimum and maximum bounds are defined |
3.1.0 | Added 'random' function option to allow users to provide psuedo-random numbers for use in operations such as lotto node child selection and wait node duration selection when a minimum and maximum duration are defined. Wait nodes will now remain in the running state indefinitely until they are aborted if no duration is defined for them |
3.0.0 | Converted to Typescript |
2.3.0 | Added Global Functions and Subtrees |
2.2.0 | Added Succeed, Fail and Retry decorators |
2.1.0 | Added optional arguments for actions, conditions and decorators |
2.0.1 | Fixed isses with inconsistent guard condition evaluation for composite nodes |
2.0.0 | Fixed broken typings |
1.1.0 | Added parallel composite node |
1.0.0 | Calls to action, condition and guard agent functions are now bound to the agent instance |
0.0.6 | Added promisey actions |
For Tasks:
Click tags to check more tools for each tasksFor Jobs:
Alternative AI tools for mistreevous
Similar Open Source Tools
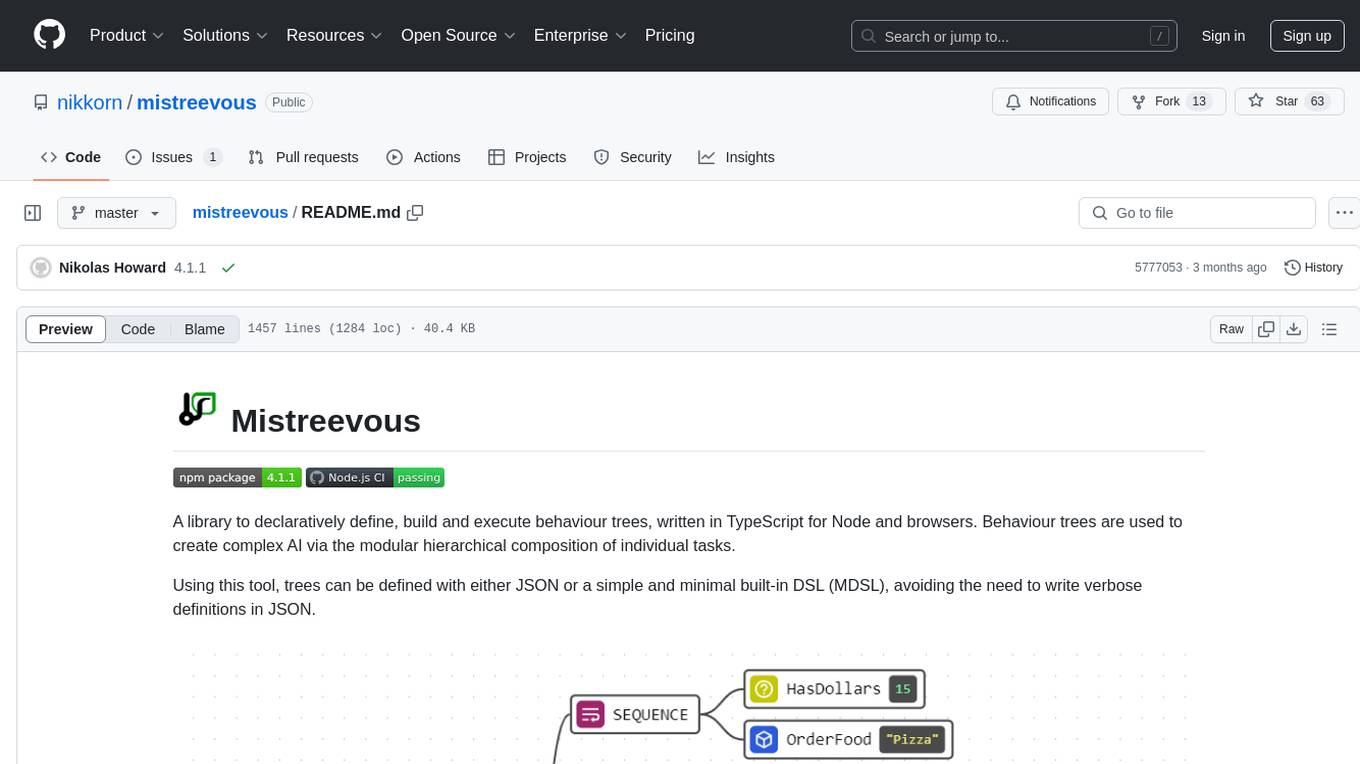
mistreevous
Mistreevous is a library written in TypeScript for Node and browsers, used to declaratively define, build, and execute behaviour trees for creating complex AI. It allows defining trees with JSON or a minimal DSL, providing in-browser editor and visualizer. The tool offers methods for tree state, stepping, resetting, and getting node details, along with various composite, decorator, leaf nodes, callbacks, guards, and global functions/subtrees. Version history includes updates for node types, callbacks, global functions, and TypeScript conversion.
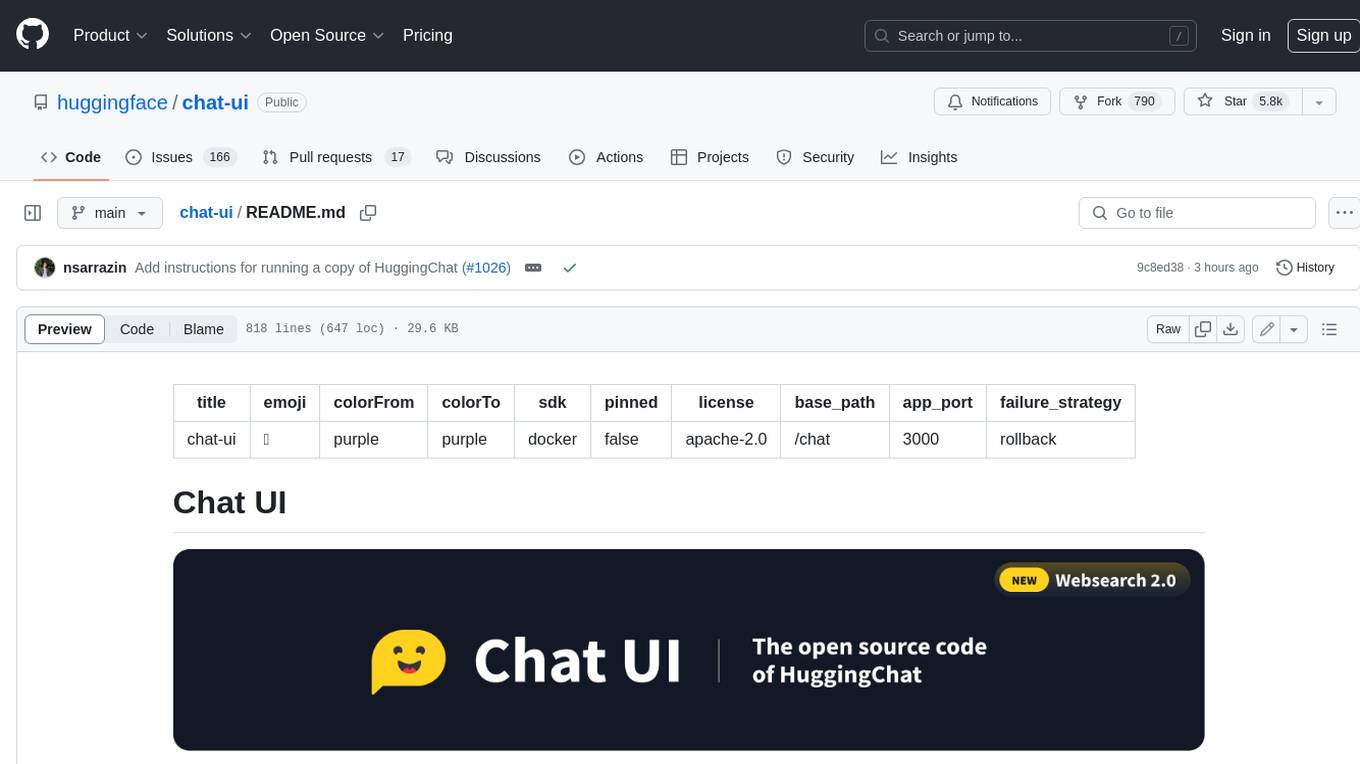
chat-ui
A chat interface using open source models, eg OpenAssistant or Llama. It is a SvelteKit app and it powers the HuggingChat app on hf.co/chat.
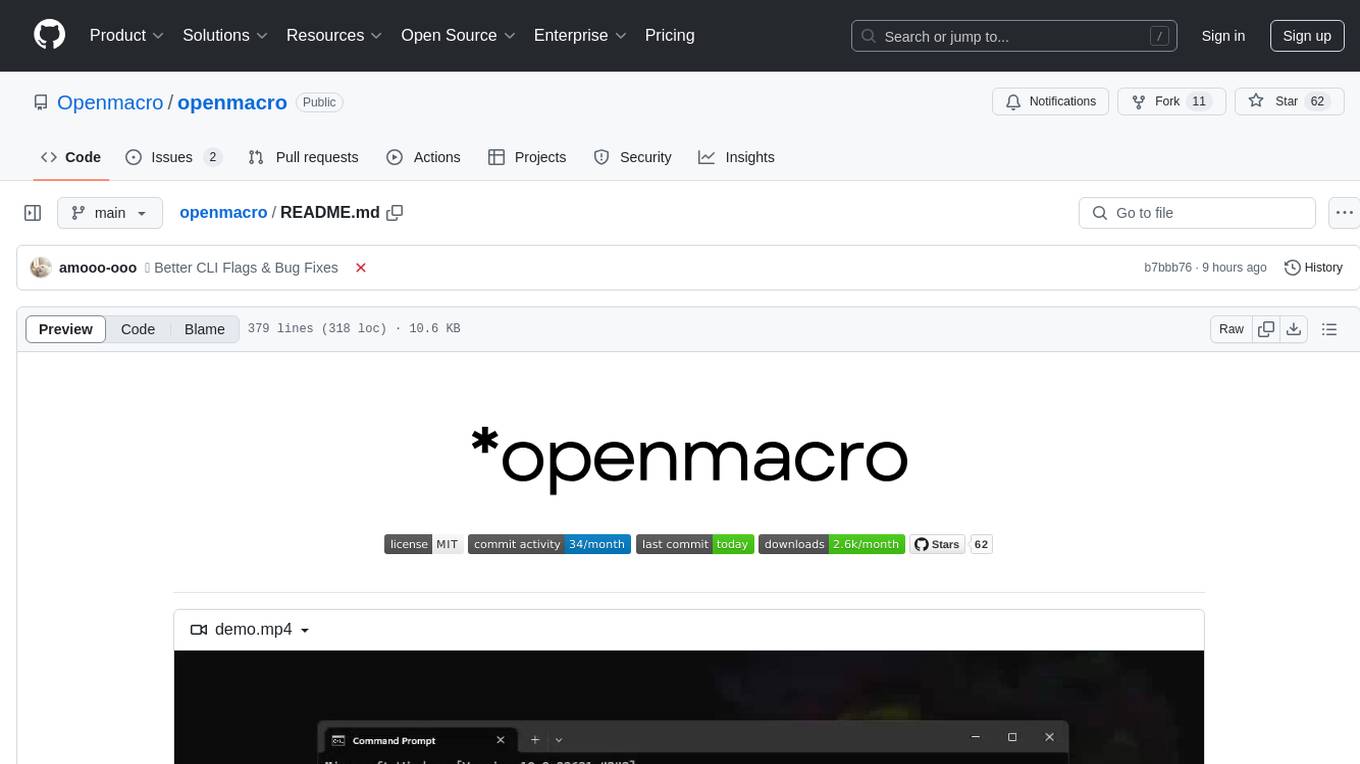
openmacro
Openmacro is a multimodal personal agent that allows users to run code locally. It acts as a personal agent capable of completing and automating tasks autonomously via self-prompting. The tool provides a CLI natural-language interface for completing and automating tasks, analyzing and plotting data, browsing the web, and manipulating files. Currently, it supports API keys for models powered by SambaNova, with plans to add support for other hosts like OpenAI and Anthropic in future versions.
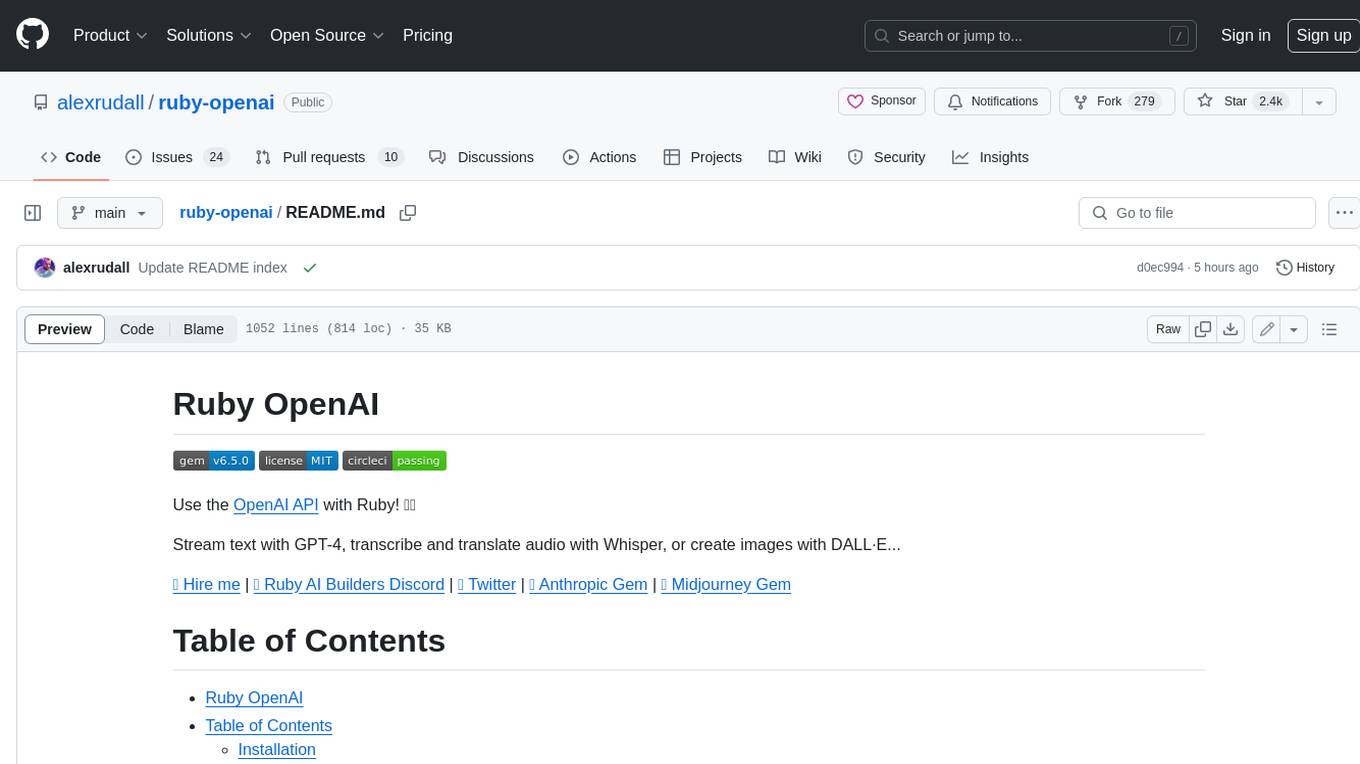
ruby-openai
Use the OpenAI API with Ruby! 🤖🩵 Stream text with GPT-4, transcribe and translate audio with Whisper, or create images with DALL·E... Hire me | 🎮 Ruby AI Builders Discord | 🐦 Twitter | 🧠 Anthropic Gem | 🚂 Midjourney Gem ## Table of Contents * Ruby OpenAI * Table of Contents * Installation * Bundler * Gem install * Usage * Quickstart * With Config * Custom timeout or base URI * Extra Headers per Client * Logging * Errors * Faraday middleware * Azure * Ollama * Counting Tokens * Models * Examples * Chat * Streaming Chat * Vision * JSON Mode * Functions * Edits * Embeddings * Batches * Files * Finetunes * Assistants * Threads and Messages * Runs * Runs involving function tools * Image Generation * DALL·E 2 * DALL·E 3 * Image Edit * Image Variations * Moderations * Whisper * Translate * Transcribe * Speech * Errors * Development * Release * Contributing * License * Code of Conduct
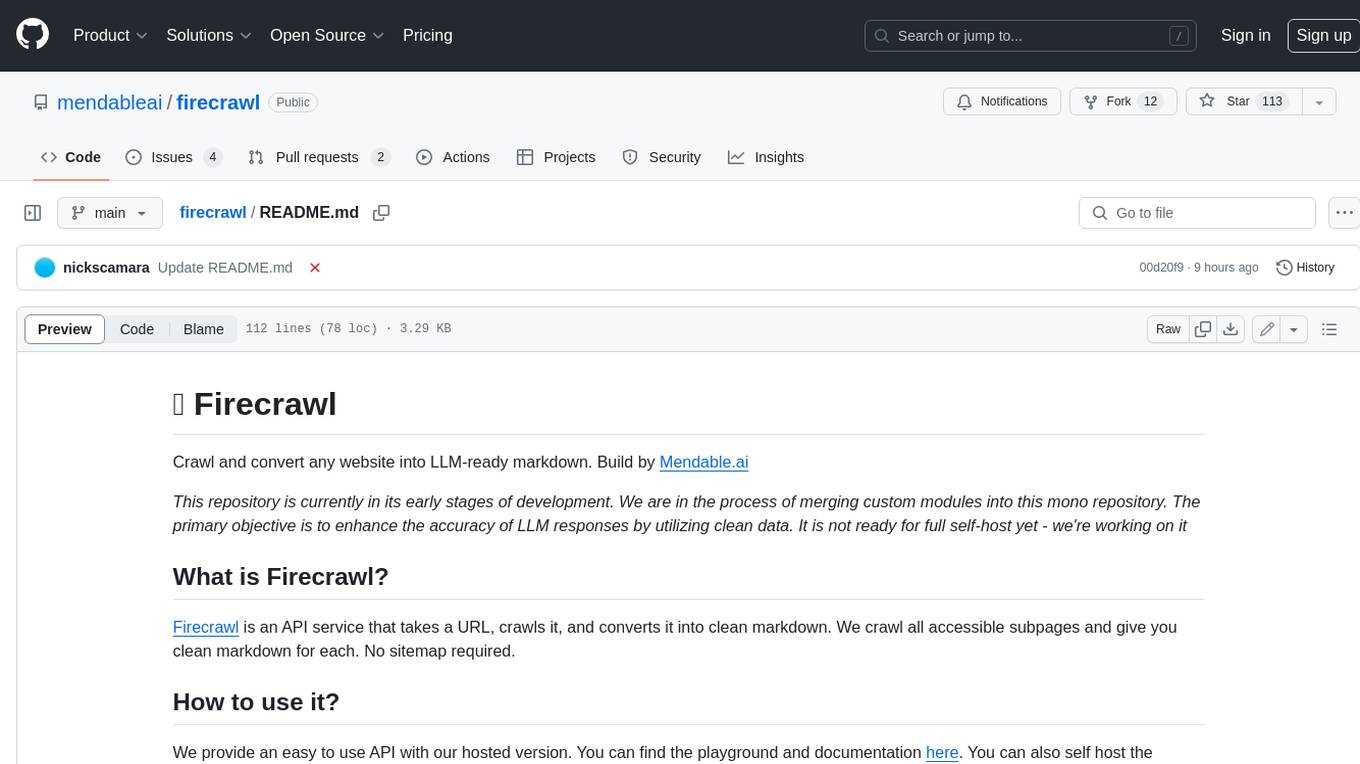
firecrawl
Firecrawl is an API service that takes a URL, crawls it, and converts it into clean markdown. It crawls all accessible subpages and provides clean markdown for each, without requiring a sitemap. The API is easy to use and can be self-hosted. It also integrates with Langchain and Llama Index. The Python SDK makes it easy to crawl and scrape websites in Python code.
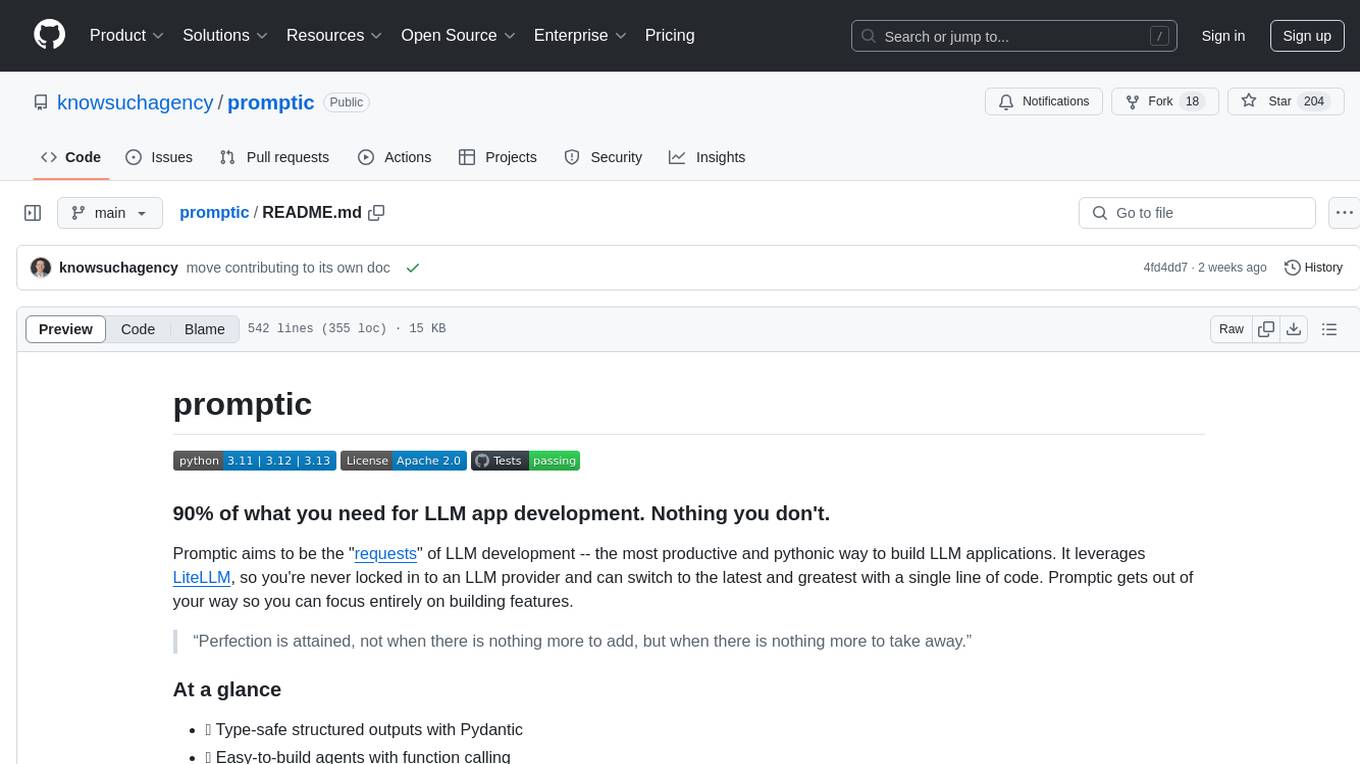
promptic
Promptic is a tool designed for LLM app development, providing a productive and pythonic way to build LLM applications. It leverages LiteLLM, allowing flexibility to switch LLM providers easily. Promptic focuses on building features by providing type-safe structured outputs, easy-to-build agents, streaming support, automatic prompt caching, and built-in conversation memory.
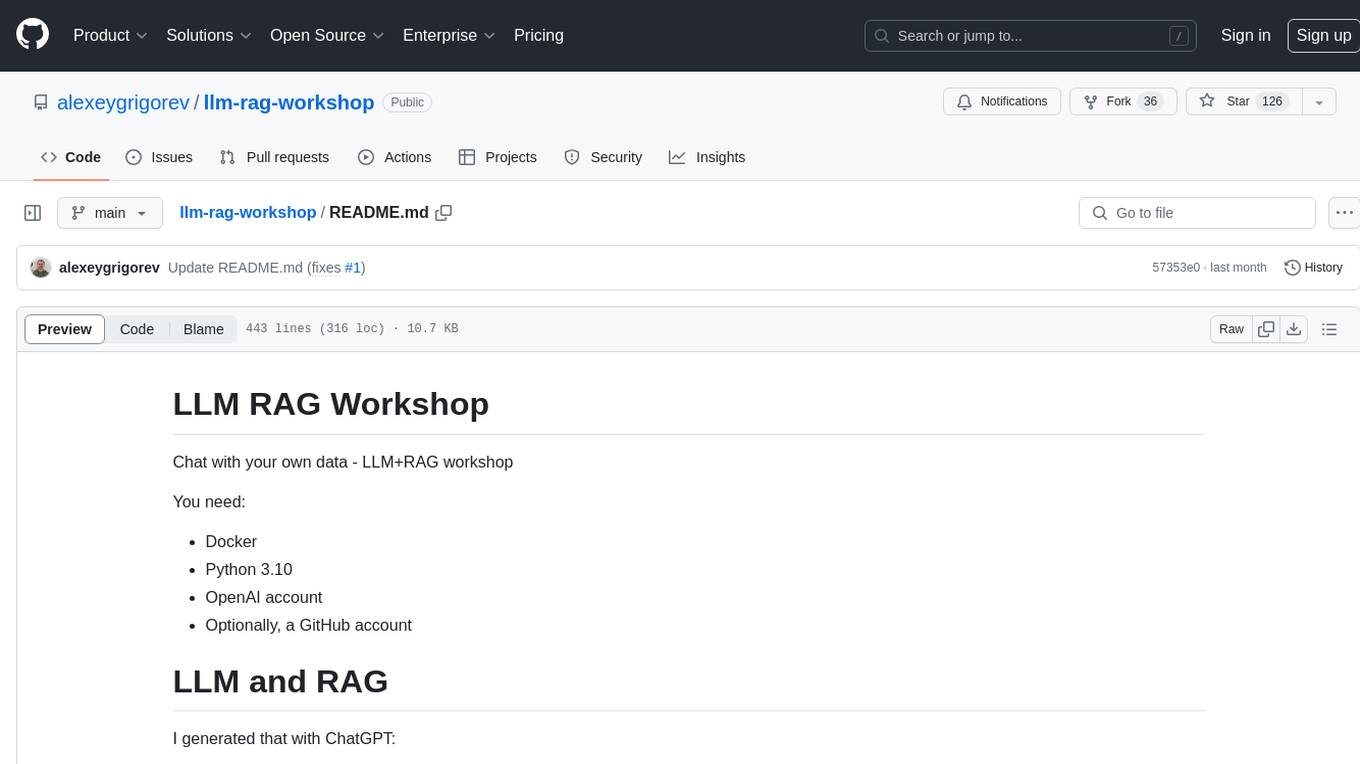
llm-rag-workshop
The LLM RAG Workshop repository provides a workshop on using Large Language Models (LLMs) and Retrieval-Augmented Generation (RAG) to generate and understand text in a human-like manner. It includes instructions on setting up the environment, indexing Zoomcamp FAQ documents, creating a Q&A system, and using OpenAI for generation based on retrieved information. The repository focuses on enhancing language model responses with retrieved information from external sources, such as document databases or search engines, to improve factual accuracy and relevance of generated text.
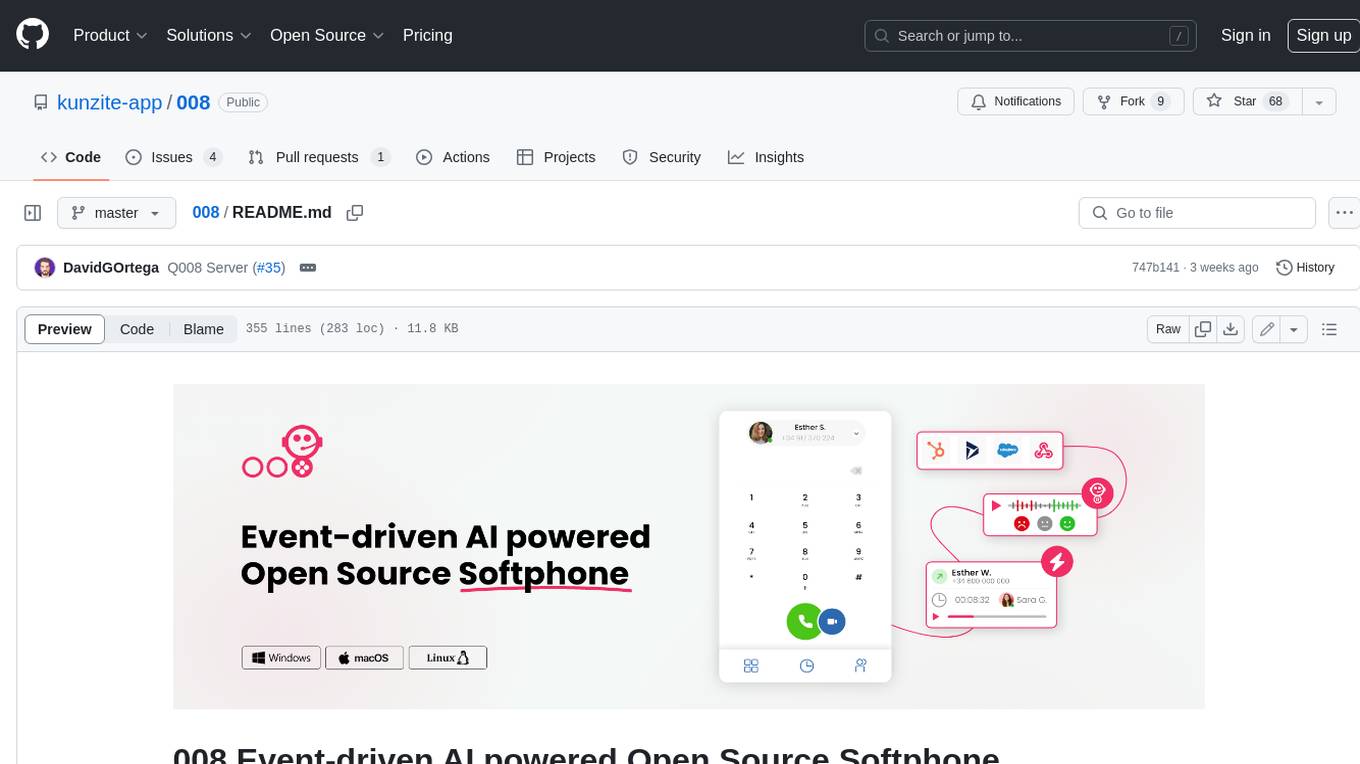
008
008 is an open-source event-driven AI powered WebRTC Softphone compatible with macOS, Windows, and Linux. It is also accessible on the web. The name '008' or 'agent 008' reflects our ambition: beyond crafting the premier Open Source Softphone, we aim to introduce a programmable, event-driven AI agent. This agent utilizes embedded artificial intelligence models operating directly on the softphone, ensuring efficiency and reduced operational costs.
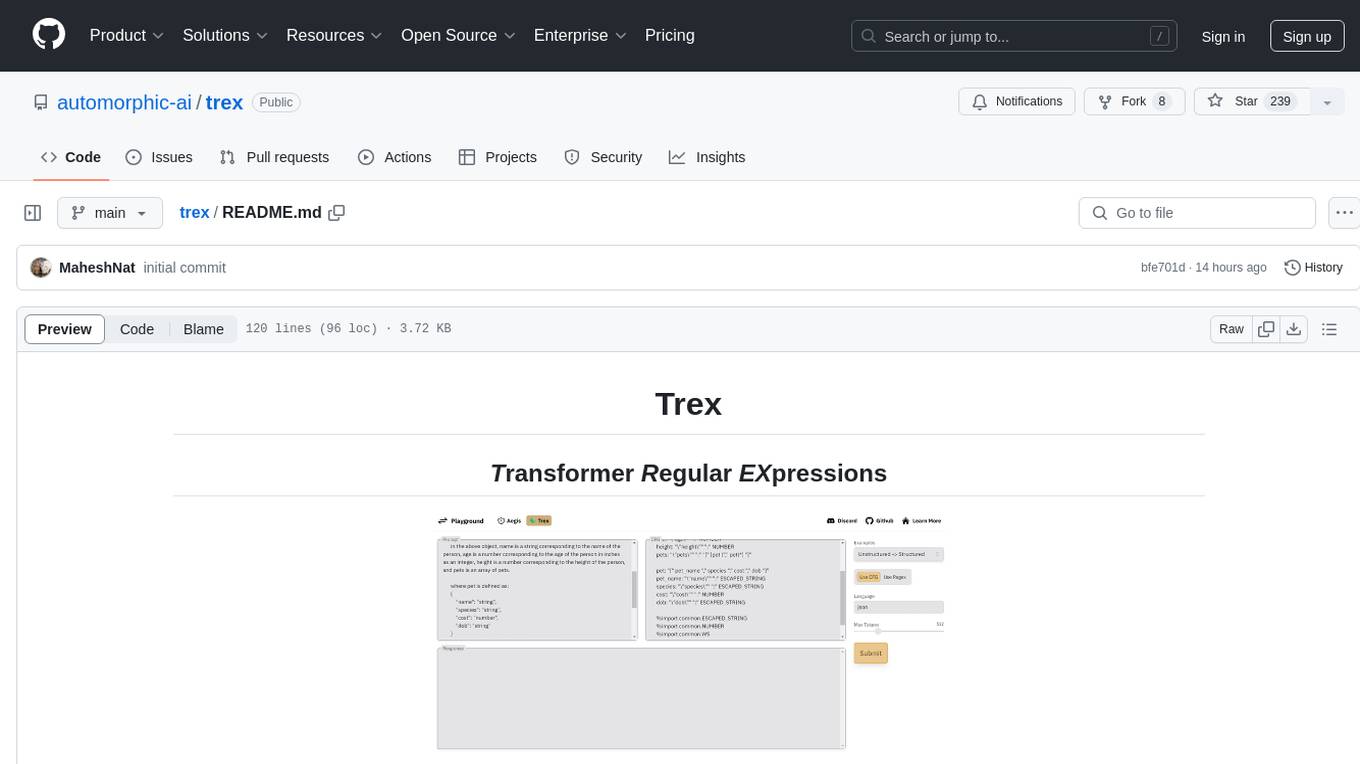
trex
Trex is a tool that transforms unstructured data into structured data by specifying a regex or context-free grammar. It intelligently restructures data to conform to the defined schema. It offers a Python client for installation and requires an API key obtained by signing up at automorphic.ai. The tool supports generating structured JSON objects based on user-defined schemas and prompts. Trex aims to provide significant speed improvements, structured custom CFG and regex generation, and generation from JSON schema. Future plans include auto-prompt generation for unstructured ETL and more intelligent models.
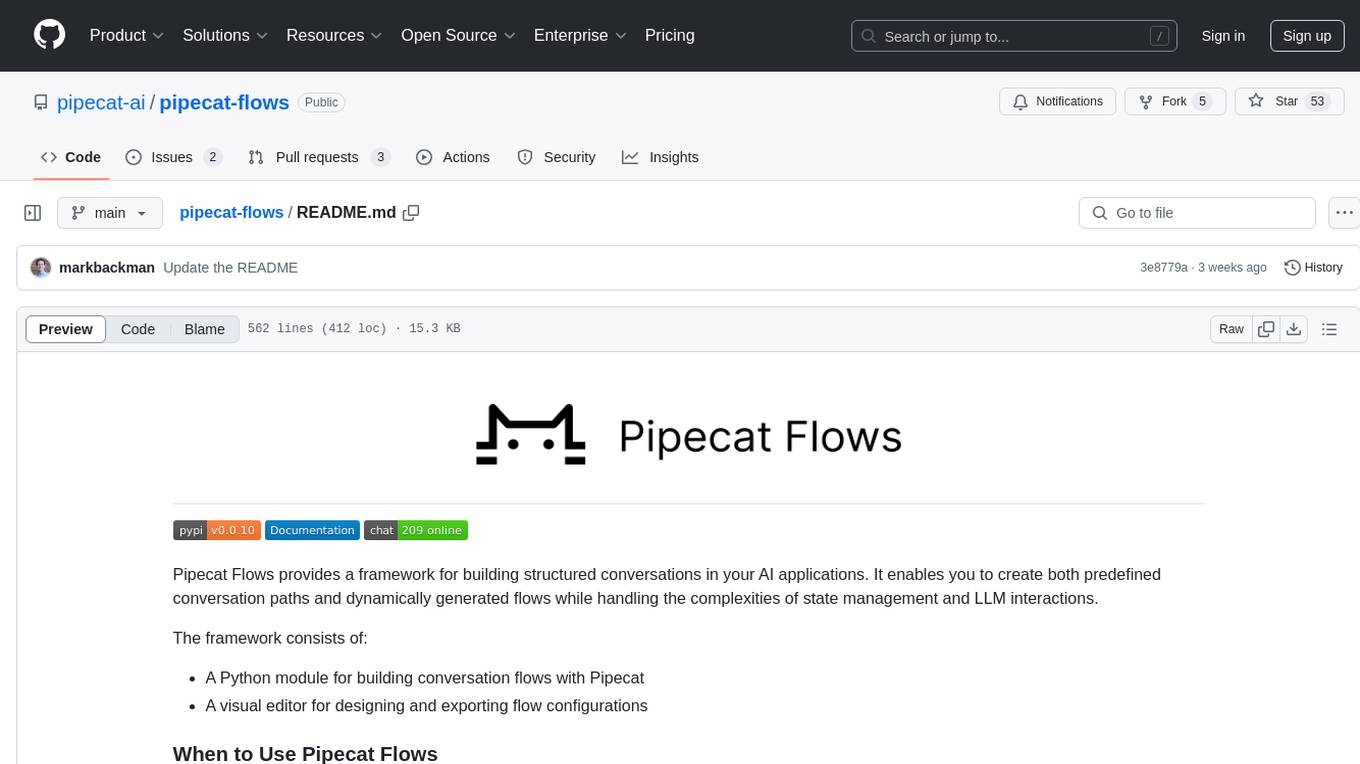
pipecat-flows
Pipecat Flows is a framework designed for building structured conversations in AI applications. It allows users to create both predefined conversation paths and dynamically generated flows, handling state management and LLM interactions. The framework includes a Python module for building conversation flows and a visual editor for designing and exporting flow configurations. Pipecat Flows is suitable for scenarios such as customer service scripts, intake forms, personalized experiences, and complex decision trees.
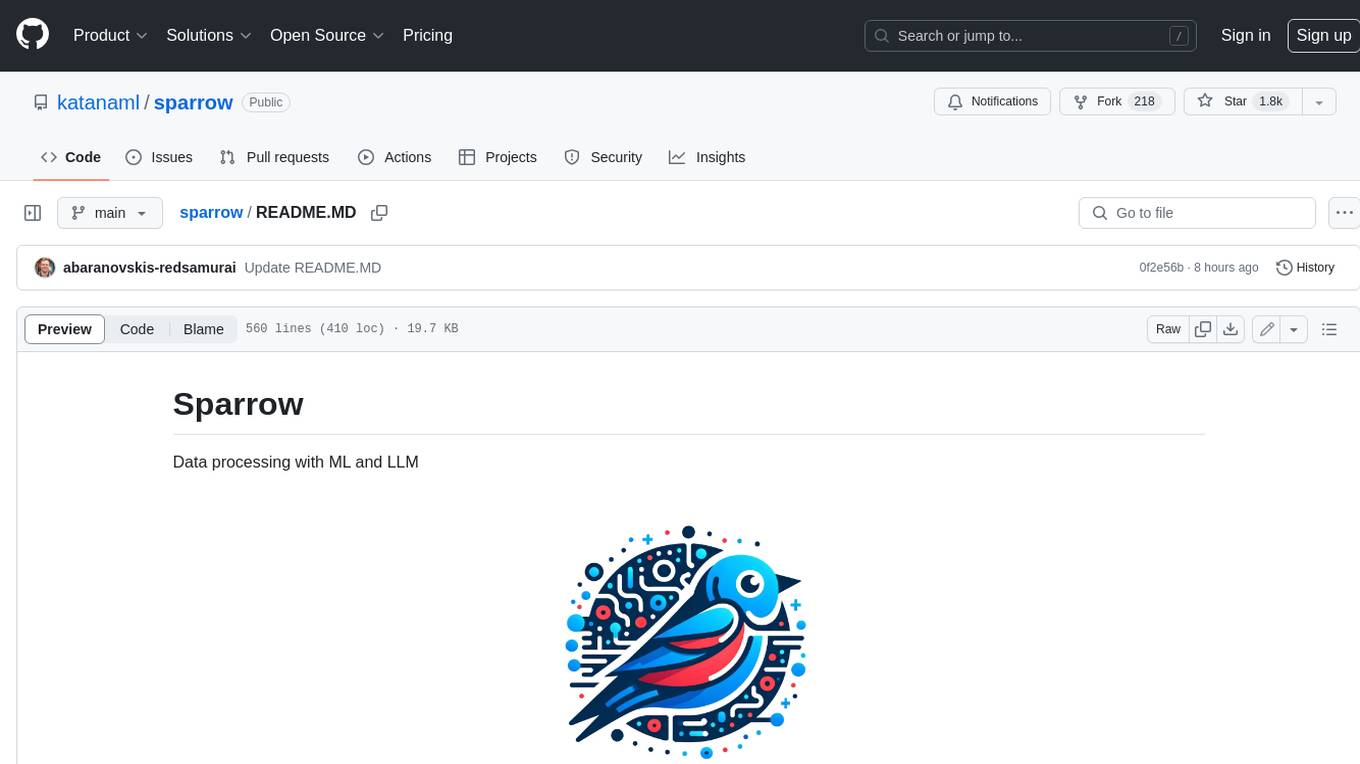
sparrow
Sparrow is an innovative open-source solution for efficient data extraction and processing from various documents and images. It seamlessly handles forms, invoices, receipts, and other unstructured data sources. Sparrow stands out with its modular architecture, offering independent services and pipelines all optimized for robust performance. One of the critical functionalities of Sparrow - pluggable architecture. You can easily integrate and run data extraction pipelines using tools and frameworks like LlamaIndex, Haystack, or Unstructured. Sparrow enables local LLM data extraction pipelines through Ollama or Apple MLX. With Sparrow solution you get API, which helps to process and transform your data into structured output, ready to be integrated with custom workflows. Sparrow Agents - with Sparrow you can build independent LLM agents, and use API to invoke them from your system. **List of available agents:** * **llamaindex** - RAG pipeline with LlamaIndex for PDF processing * **vllamaindex** - RAG pipeline with LLamaIndex multimodal for image processing * **vprocessor** - RAG pipeline with OCR and LlamaIndex for image processing * **haystack** - RAG pipeline with Haystack for PDF processing * **fcall** - Function call pipeline * **unstructured-light** - RAG pipeline with Unstructured and LangChain, supports PDF and image processing * **unstructured** - RAG pipeline with Weaviate vector DB query, Unstructured and LangChain, supports PDF and image processing * **instructor** - RAG pipeline with Unstructured and Instructor libraries, supports PDF and image processing. Works great for JSON response generation
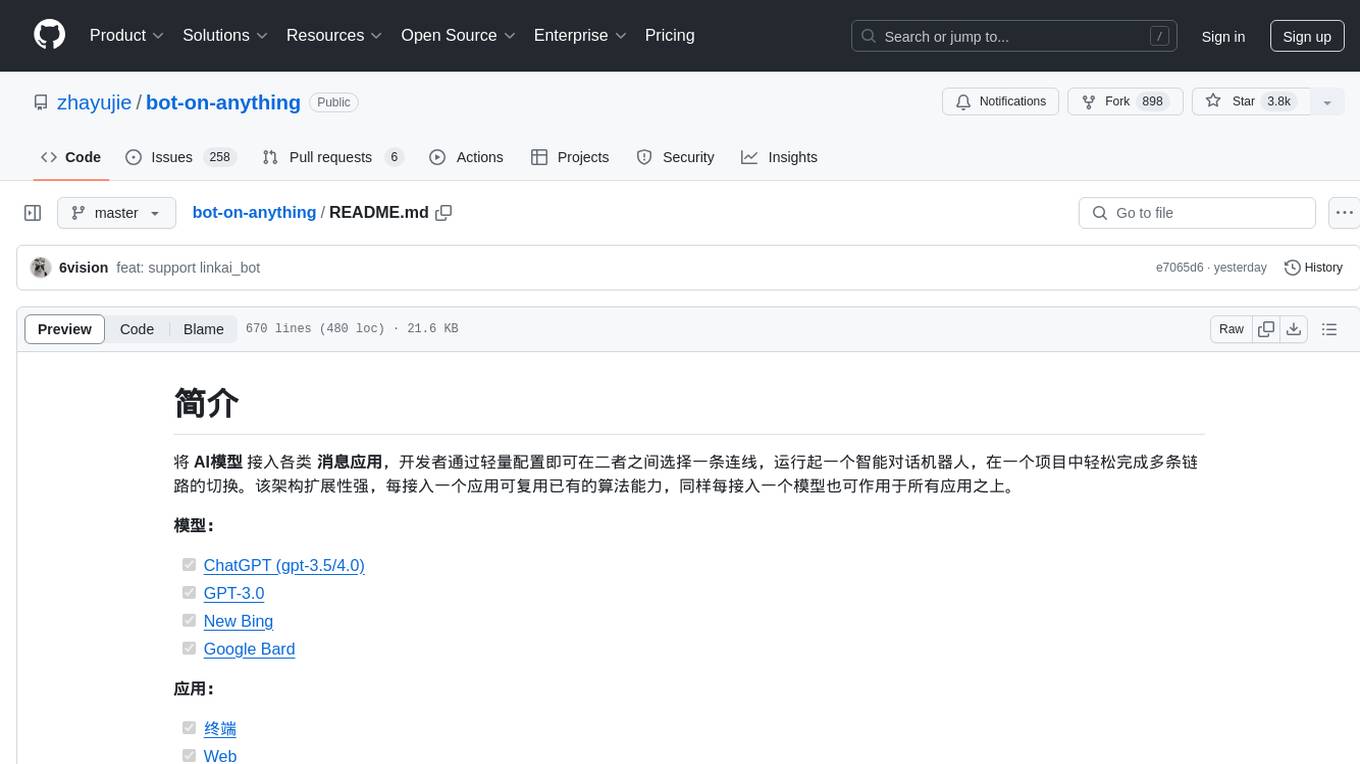
bot-on-anything
The 'bot-on-anything' repository allows developers to integrate various AI models into messaging applications, enabling the creation of intelligent chatbots. By configuring the connections between models and applications, developers can easily switch between multiple channels within a project. The architecture is highly scalable, allowing the reuse of algorithmic capabilities for each new application and model integration. Supported models include ChatGPT, GPT-3.0, New Bing, and Google Bard, while supported applications range from terminals and web platforms to messaging apps like WeChat, Telegram, QQ, and more. The repository provides detailed instructions for setting up the environment, configuring the models and channels, and running the chatbot for various tasks across different messaging platforms.
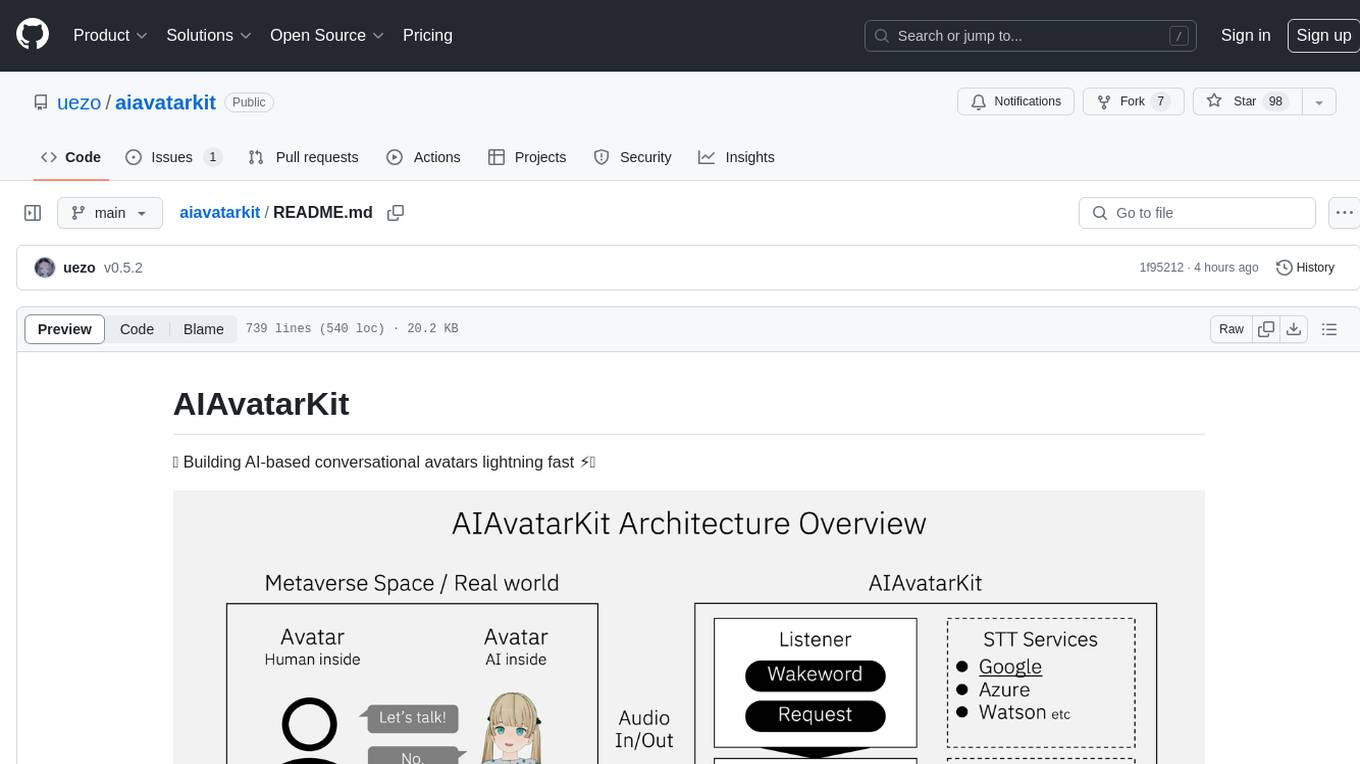
aiavatarkit
AIAvatarKit is a tool for building AI-based conversational avatars quickly. It supports various platforms like VRChat and cluster, along with real-world devices. The tool is extensible, allowing unlimited capabilities based on user needs. It requires VOICEVOX API, Google or Azure Speech Services API keys, and Python 3.10. Users can start conversations out of the box and enjoy seamless interactions with the avatars.
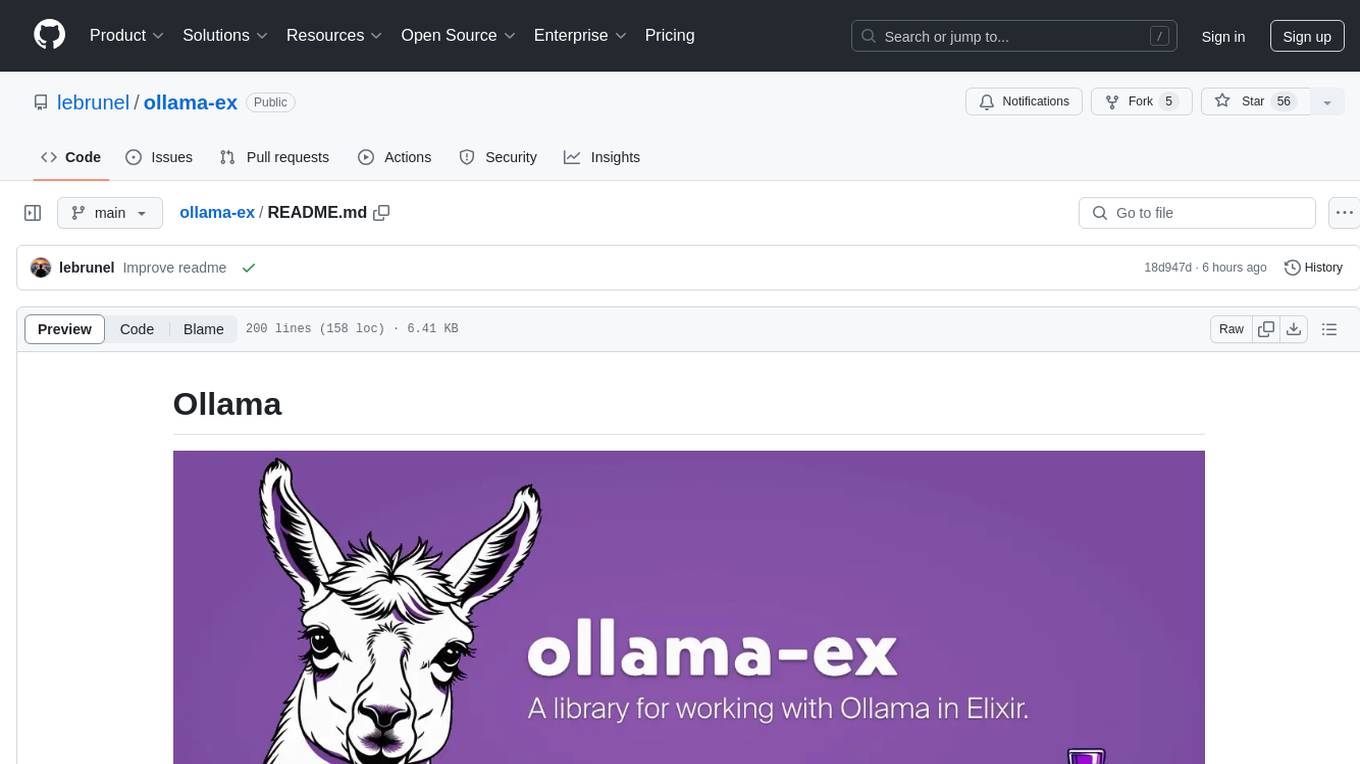
ollama-ex
Ollama is a powerful tool for running large language models locally or on your own infrastructure. It provides a full implementation of the Ollama API, support for streaming requests, and tool use capability. Users can interact with Ollama in Elixir to generate completions, chat messages, and perform streaming requests. The tool also supports function calling on compatible models, allowing users to define tools with clear descriptions and arguments. Ollama is designed to facilitate natural language processing tasks and enhance user interactions with language models.
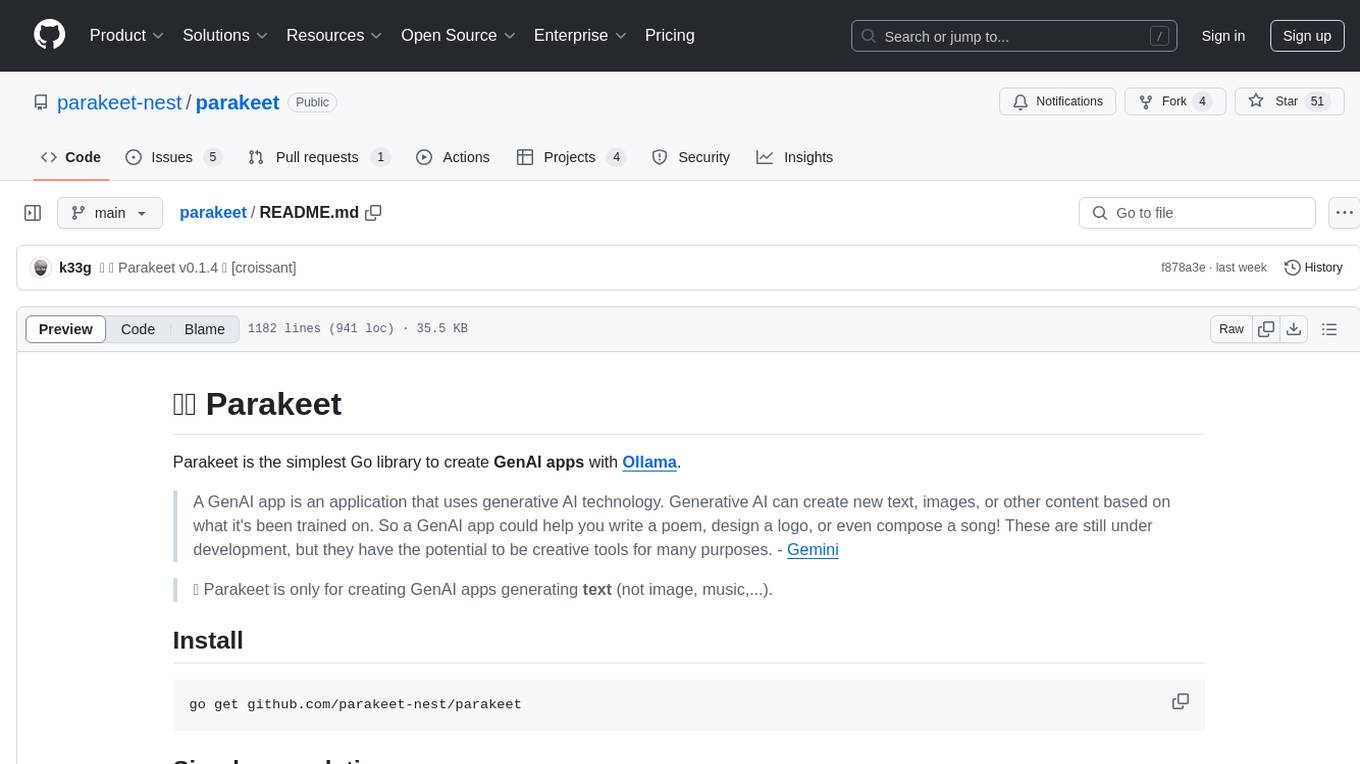
parakeet
Parakeet is a Go library for creating GenAI apps with Ollama. It enables the creation of generative AI applications that can generate text-based content. The library provides tools for simple completion, completion with context, chat completion, and more. It also supports function calling with tools and Wasm plugins. Parakeet allows users to interact with language models and create AI-powered applications easily.
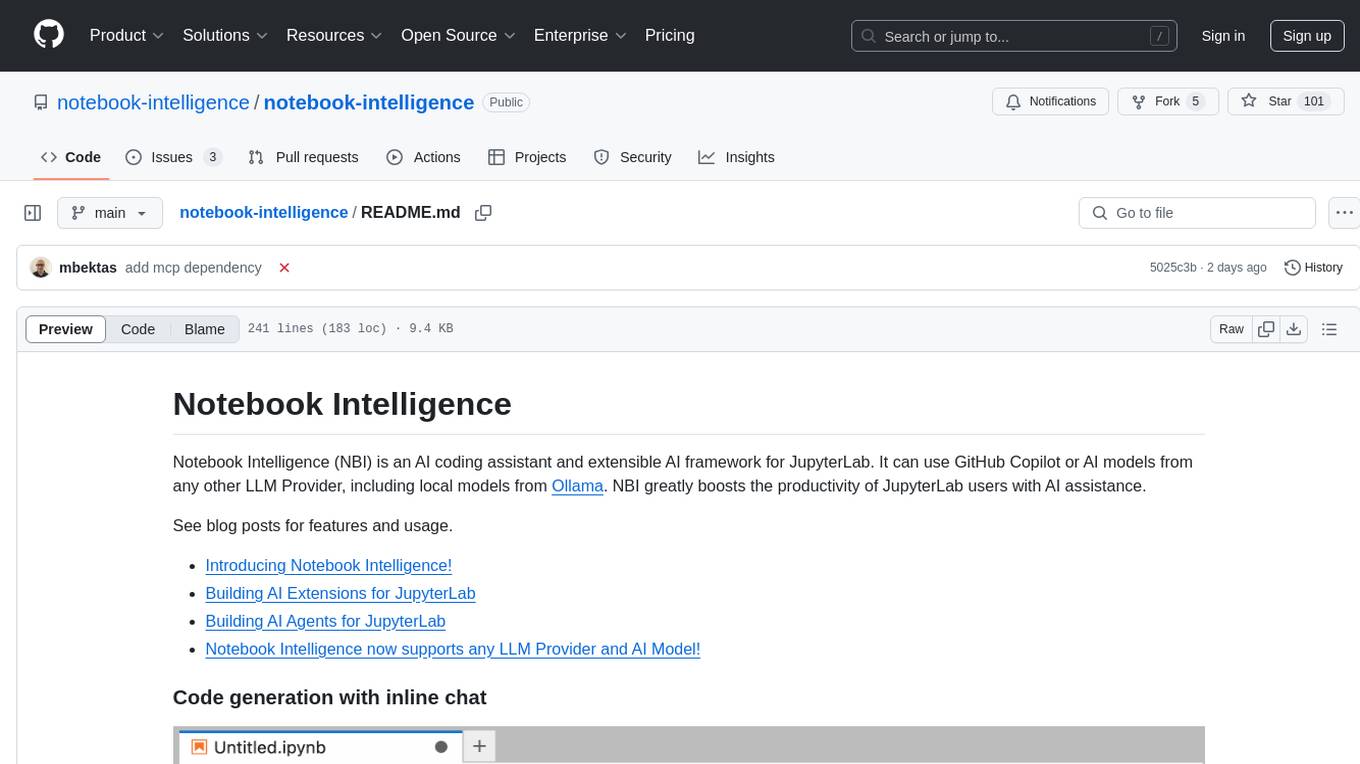
notebook-intelligence
Notebook Intelligence (NBI) is an AI coding assistant and extensible AI framework for JupyterLab. It greatly boosts the productivity of JupyterLab users with AI assistance by providing features such as code generation with inline chat, auto-complete, and chat interface. NBI supports various LLM Providers and AI Models, including local models from Ollama. Users can configure model provider and model options, remember GitHub Copilot login, and save configuration files. NBI seamlessly integrates with Model Context Protocol (MCP) servers, supporting both Standard Input/Output (stdio) and Server-Sent Events (SSE) transports. Users can easily add MCP servers to NBI, auto-approve tools, set environment variables, and group servers based on functionality. Additionally, NBI allows access to built-in tools from an MCP participant, enhancing the user experience and productivity.
For similar tasks
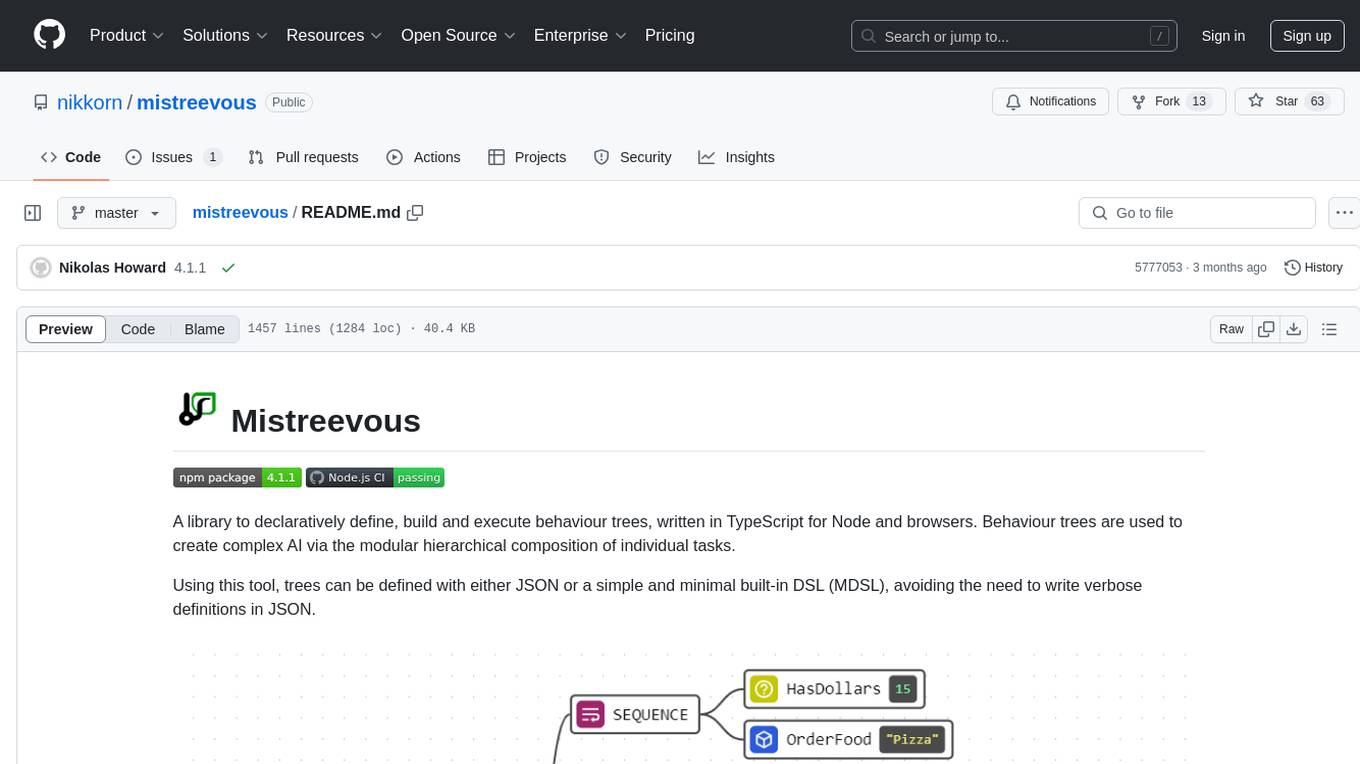
mistreevous
Mistreevous is a library written in TypeScript for Node and browsers, used to declaratively define, build, and execute behaviour trees for creating complex AI. It allows defining trees with JSON or a minimal DSL, providing in-browser editor and visualizer. The tool offers methods for tree state, stepping, resetting, and getting node details, along with various composite, decorator, leaf nodes, callbacks, guards, and global functions/subtrees. Version history includes updates for node types, callbacks, global functions, and TypeScript conversion.
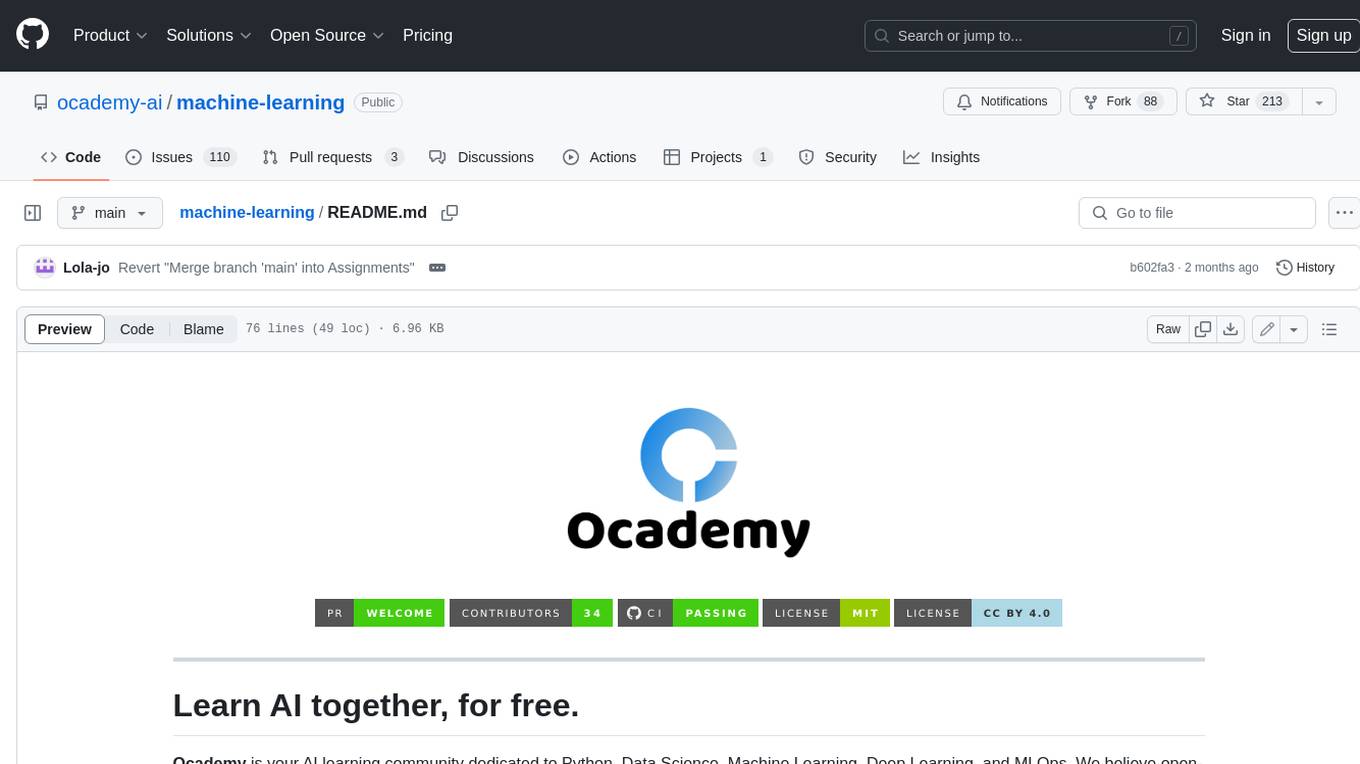
machine-learning
Ocademy is an AI learning community dedicated to Python, Data Science, Machine Learning, Deep Learning, and MLOps. They promote equal opportunities for everyone to access AI through open-source educational resources. The repository contains curated AI courses, tutorials, books, tools, and resources for learning and creating Generative AI. It also offers an interactive book to help adults transition into AI. Contributors are welcome to join and contribute to the community by following guidelines. The project follows a code of conduct to ensure inclusivity and welcomes contributions from those passionate about Data Science and AI.
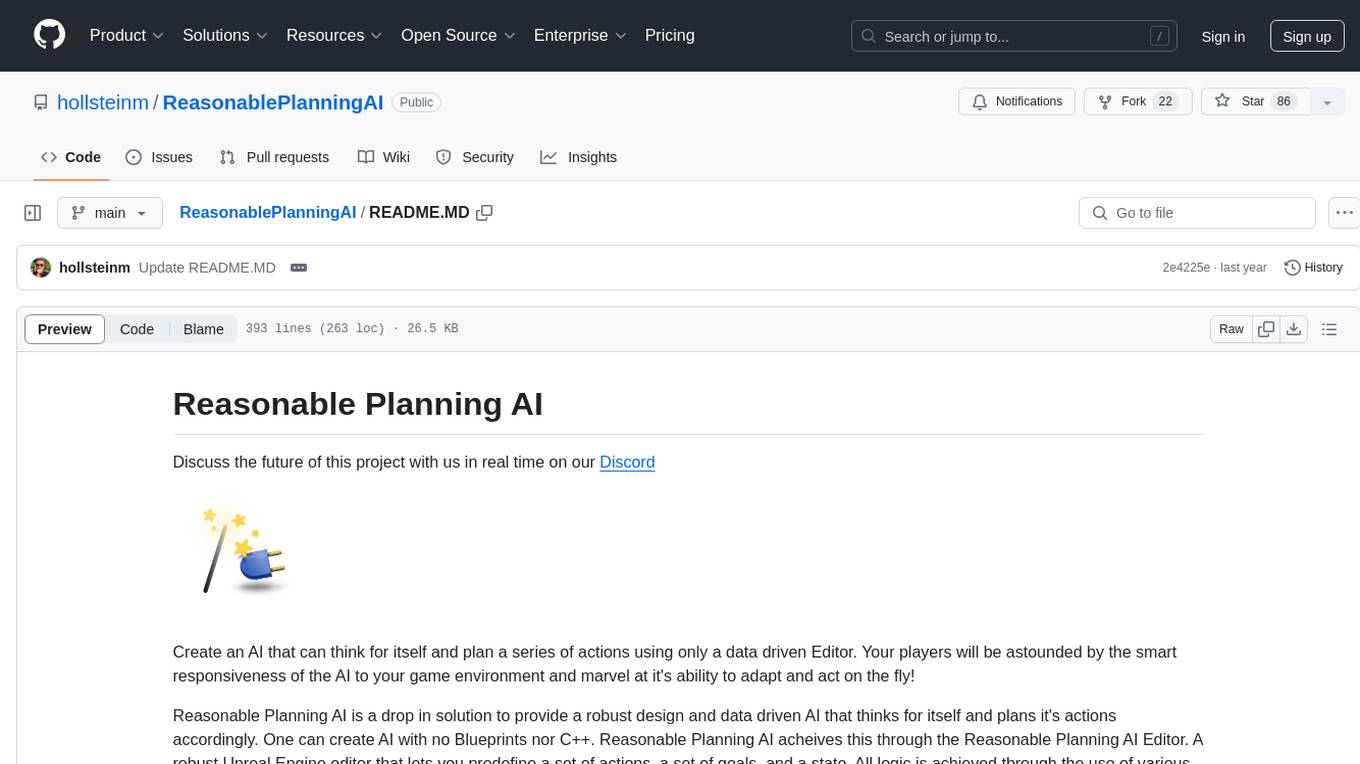
ReasonablePlanningAI
Reasonable Planning AI is a robust design and data-driven AI solution for game developers. It provides an AI Editor that allows creating AI without Blueprints or C++. The AI can think for itself, plan actions, adapt to the game environment, and act dynamically. It consists of Core components like RpaiGoalBase, RpaiActionBase, RpaiPlannerBase, RpaiReasonerBase, and RpaiBrainComponent, as well as Composer components for easier integration by Game Designers. The tool is extensible, cross-compatible with Behavior Trees, and offers debugging features like visual logging and heuristics testing. It follows a simple path of execution and supports versioning for stability and compatibility with Unreal Engine versions.
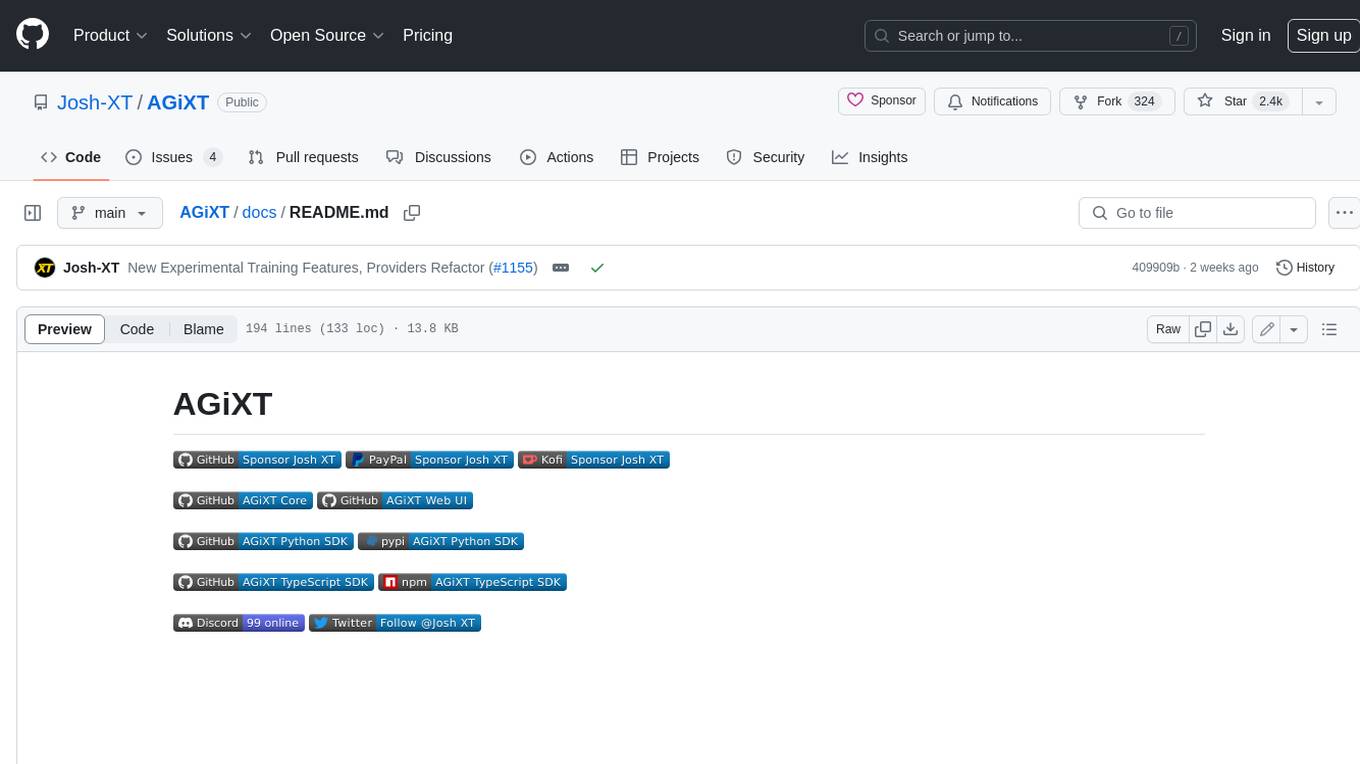
AGiXT
AGiXT is a dynamic Artificial Intelligence Automation Platform engineered to orchestrate efficient AI instruction management and task execution across a multitude of providers. Our solution infuses adaptive memory handling with a broad spectrum of commands to enhance AI's understanding and responsiveness, leading to improved task completion. The platform's smart features, like Smart Instruct and Smart Chat, seamlessly integrate web search, planning strategies, and conversation continuity, transforming the interaction between users and AI. By leveraging a powerful plugin system that includes web browsing and command execution, AGiXT stands as a versatile bridge between AI models and users. With an expanding roster of AI providers, code evaluation capabilities, comprehensive chain management, and platform interoperability, AGiXT is consistently evolving to drive a multitude of applications, affirming its place at the forefront of AI technology.
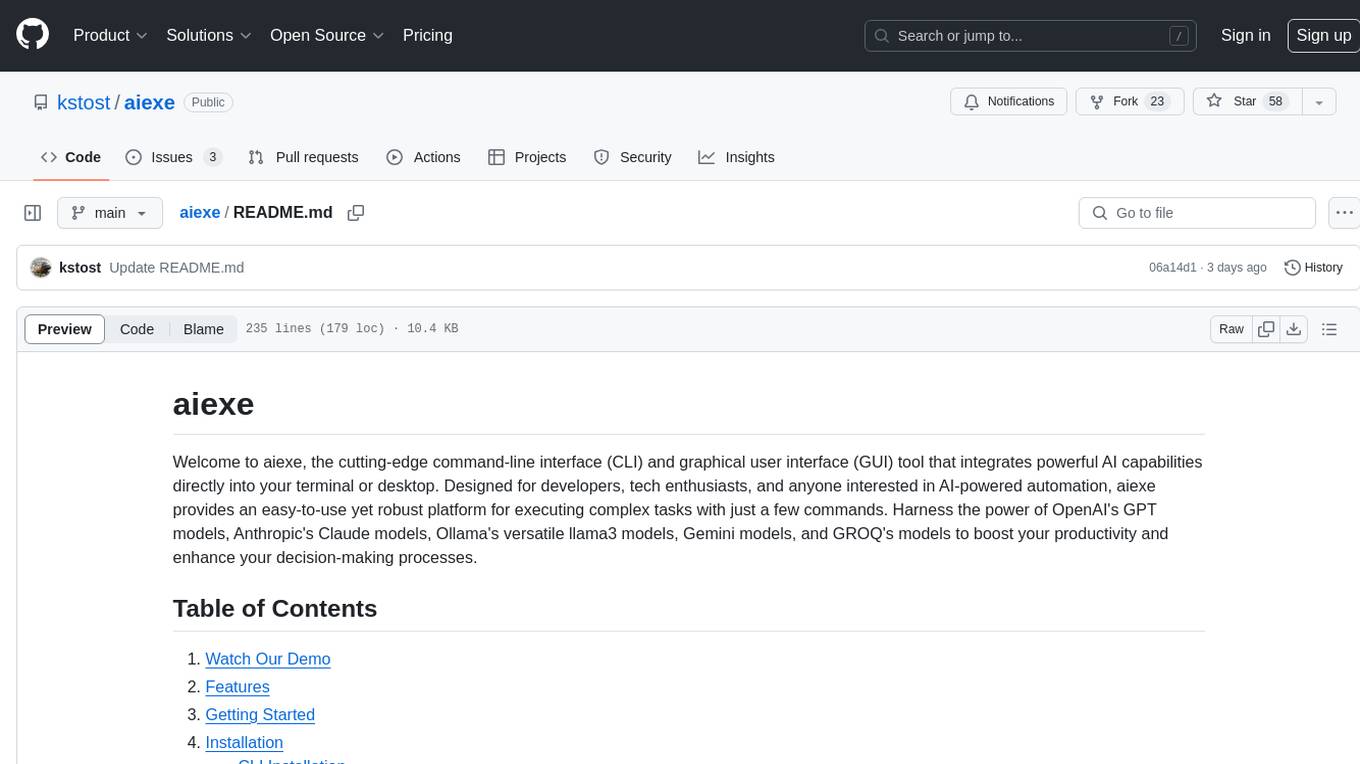
aiexe
aiexe is a cutting-edge command-line interface (CLI) and graphical user interface (GUI) tool that integrates powerful AI capabilities directly into your terminal or desktop. It is designed for developers, tech enthusiasts, and anyone interested in AI-powered automation. aiexe provides an easy-to-use yet robust platform for executing complex tasks with just a few commands. Users can harness the power of various AI models from OpenAI, Anthropic, Ollama, Gemini, and GROQ to boost productivity and enhance decision-making processes.
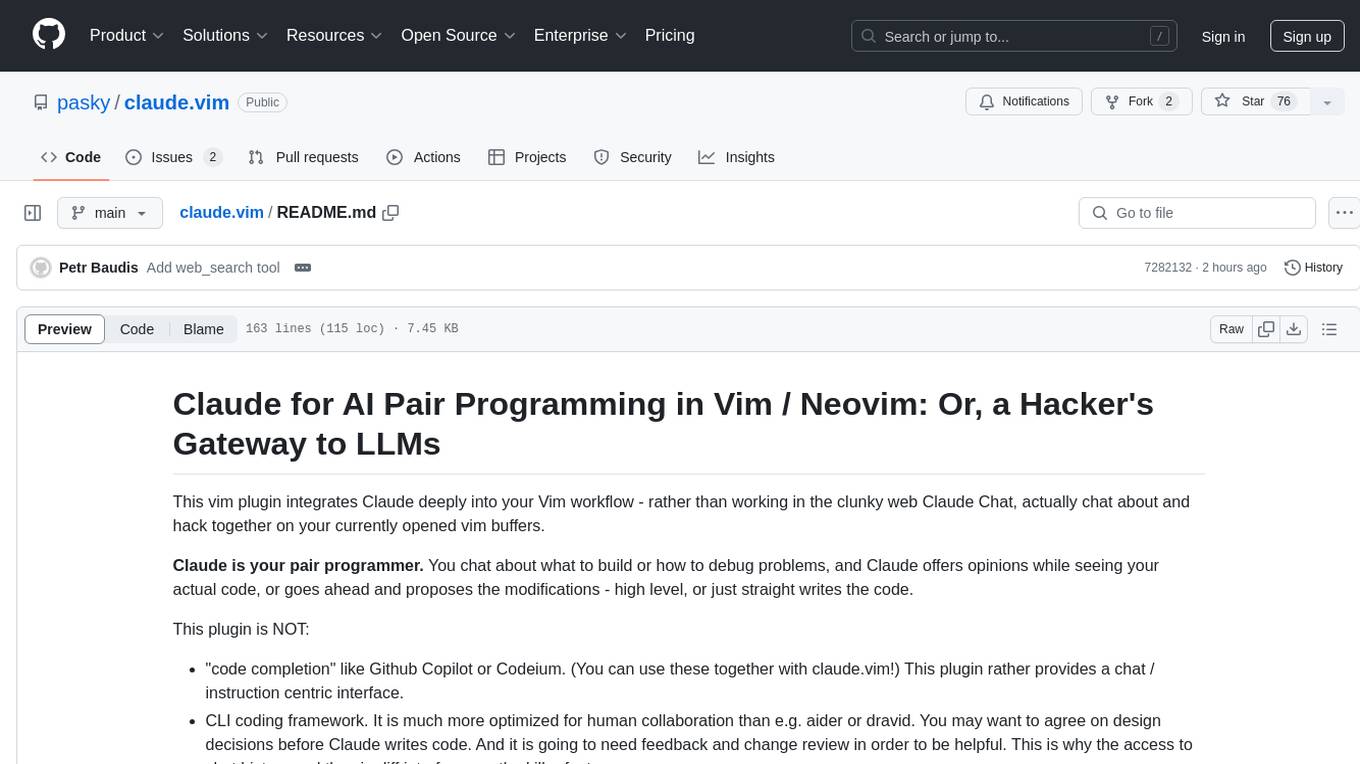
claude.vim
Claude.vim is a Vim plugin that integrates Claude, an AI pair programmer, into your Vim workflow. It allows you to chat with Claude about what to build or how to debug problems, and Claude offers opinions, proposes modifications, or even writes code. The plugin provides a chat/instruction-centric interface optimized for human collaboration, with killer features like access to chat history and vimdiff interface. It can refactor code, modify or extend selected pieces of code, execute complex tasks by reading documentation, cloning git repositories, and more. Note that it is early alpha software and expected to rapidly evolve.
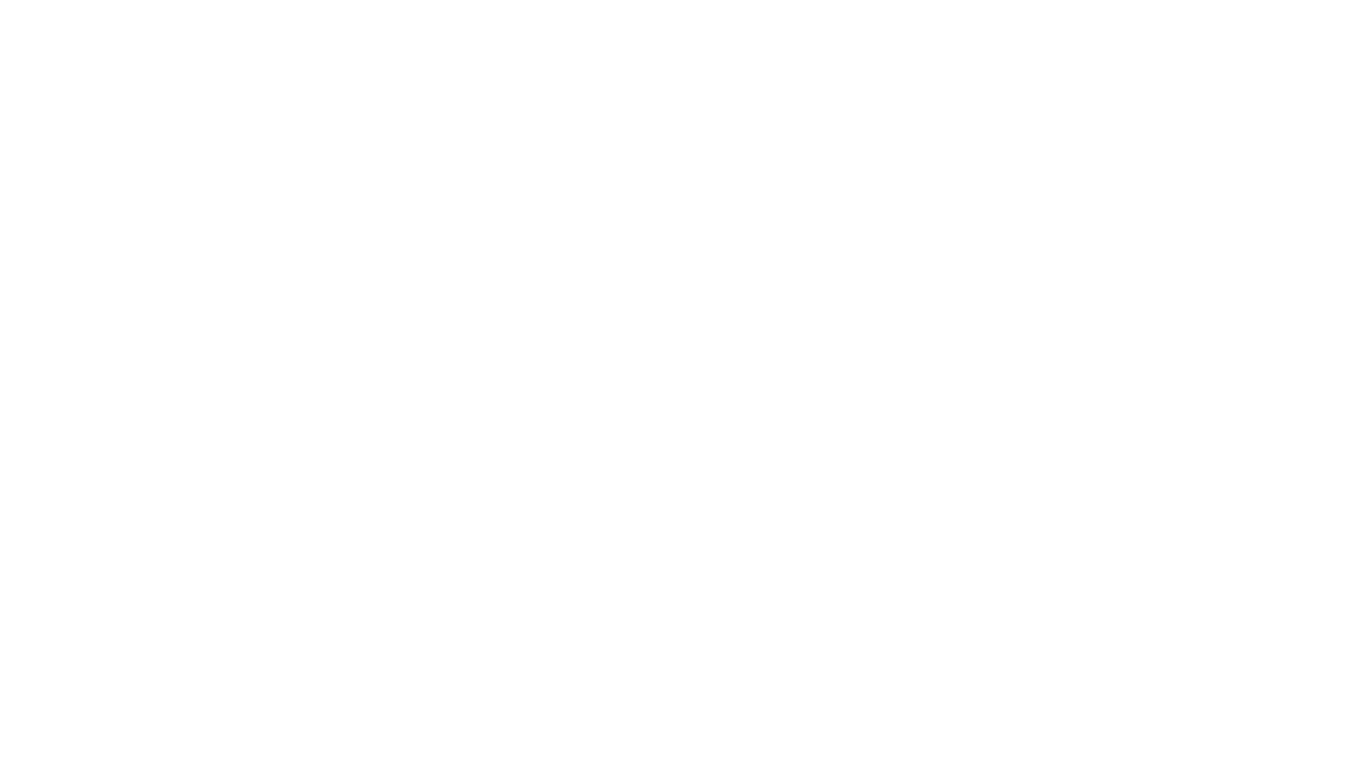
project_alice
Alice is an agentic workflow framework that integrates task execution and intelligent chat capabilities. It provides a flexible environment for creating, managing, and deploying AI agents for various purposes, leveraging a microservices architecture with MongoDB for data persistence. The framework consists of components like APIs, agents, tasks, and chats that interact to produce outputs through files, messages, task results, and URL references. Users can create, test, and deploy agentic solutions in a human-language framework, making it easy to engage with by both users and agents. The tool offers an open-source option, user management, flexible model deployment, and programmatic access to tasks and chats.
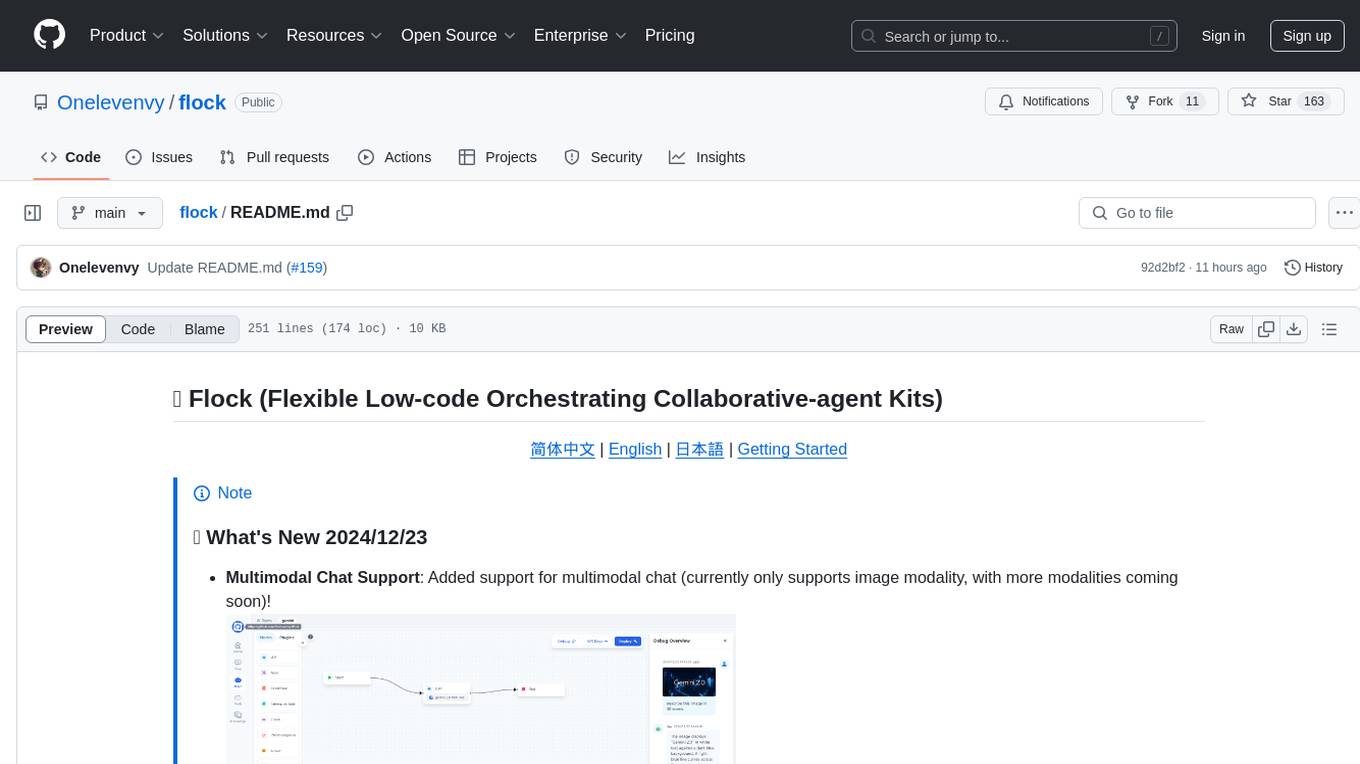
flock
Flock is a workflow-based low-code platform that enables rapid development of chatbots, RAG applications, and coordination of multi-agent teams. It offers a flexible, low-code solution for orchestrating collaborative agents, supporting various node types for specific tasks, such as input processing, text generation, knowledge retrieval, tool execution, intent recognition, answer generation, and more. Flock integrates LangChain and LangGraph to provide offline operation capabilities and supports future nodes like Conditional Branch, File Upload, and Parameter Extraction for creating complex workflows. Inspired by StreetLamb, Lobe-chat, Dify, and fastgpt projects, Flock introduces new features and directions while leveraging open-source models and multi-tenancy support.
For similar jobs
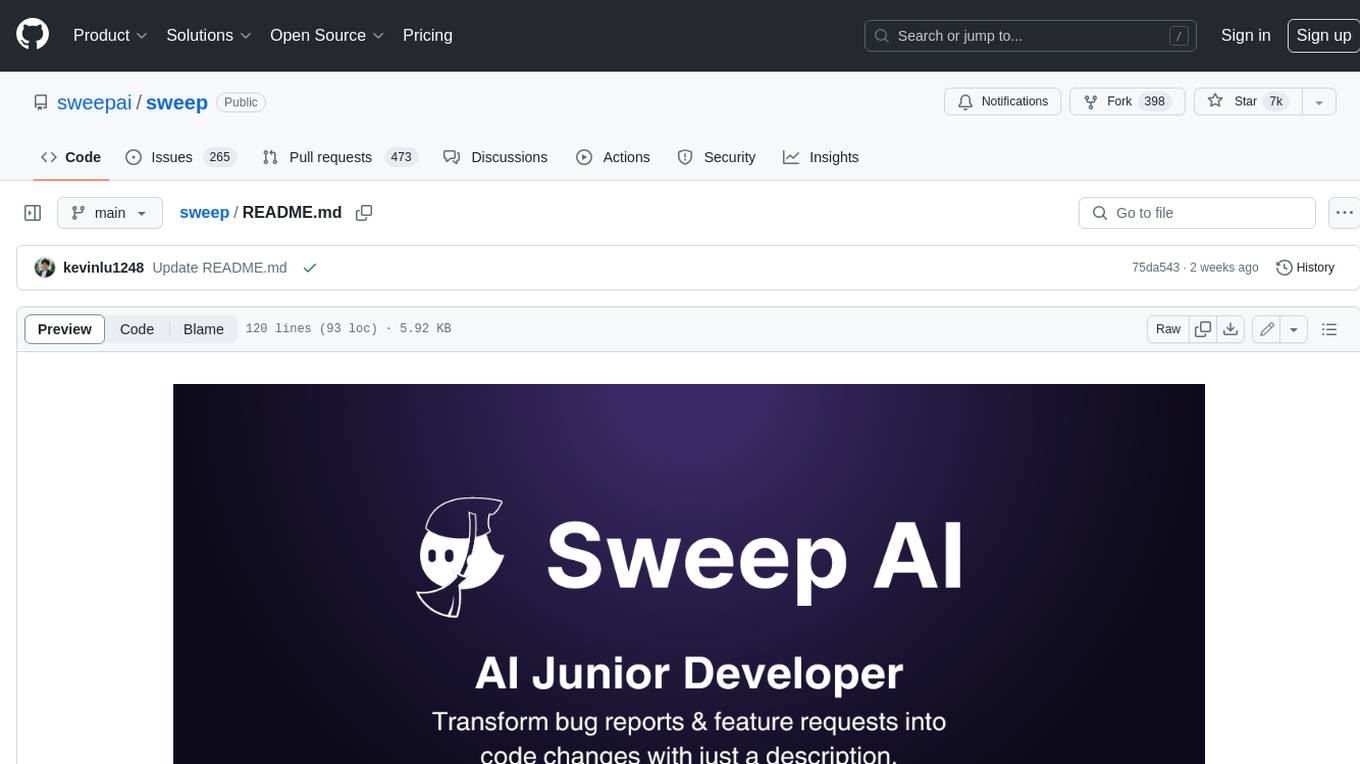
sweep
Sweep is an AI junior developer that turns bugs and feature requests into code changes. It automatically handles developer experience improvements like adding type hints and improving test coverage.
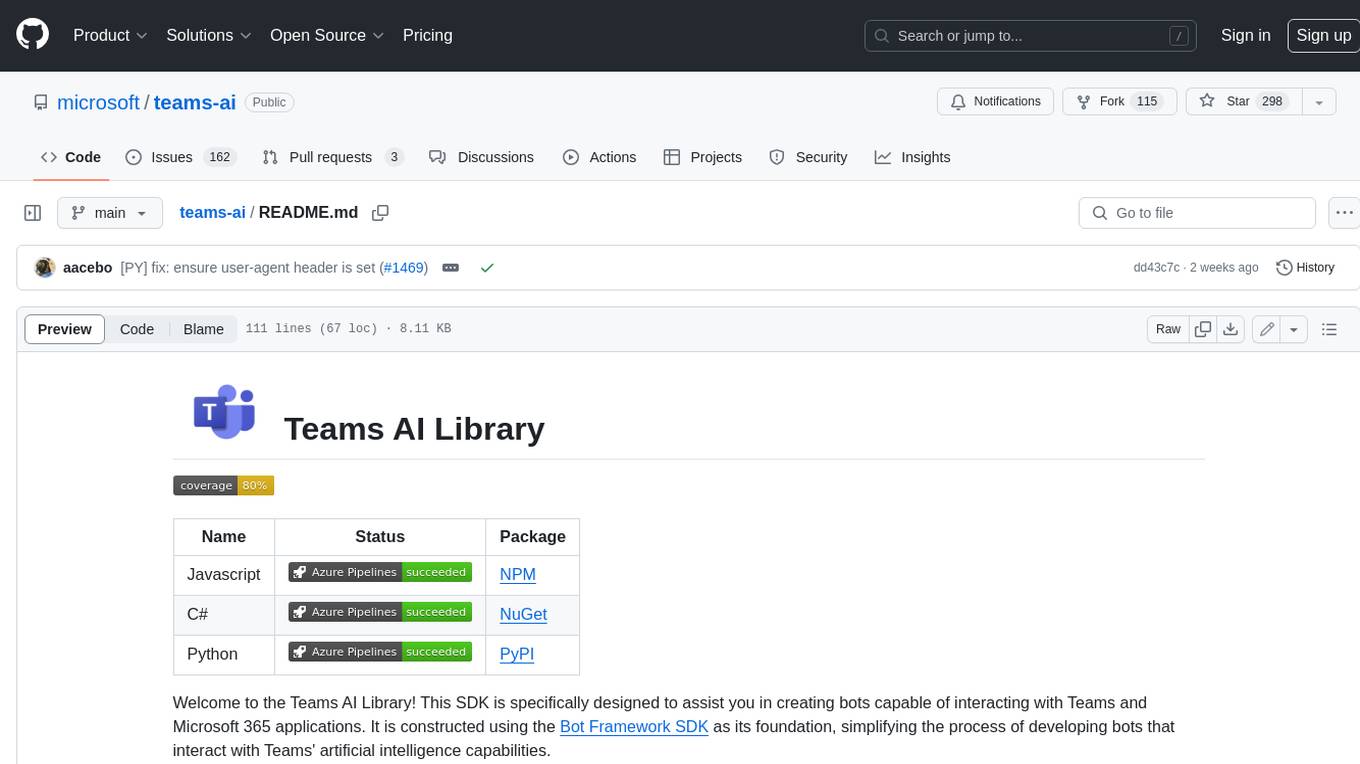
teams-ai
The Teams AI Library is a software development kit (SDK) that helps developers create bots that can interact with Teams and Microsoft 365 applications. It is built on top of the Bot Framework SDK and simplifies the process of developing bots that interact with Teams' artificial intelligence capabilities. The SDK is available for JavaScript/TypeScript, .NET, and Python.
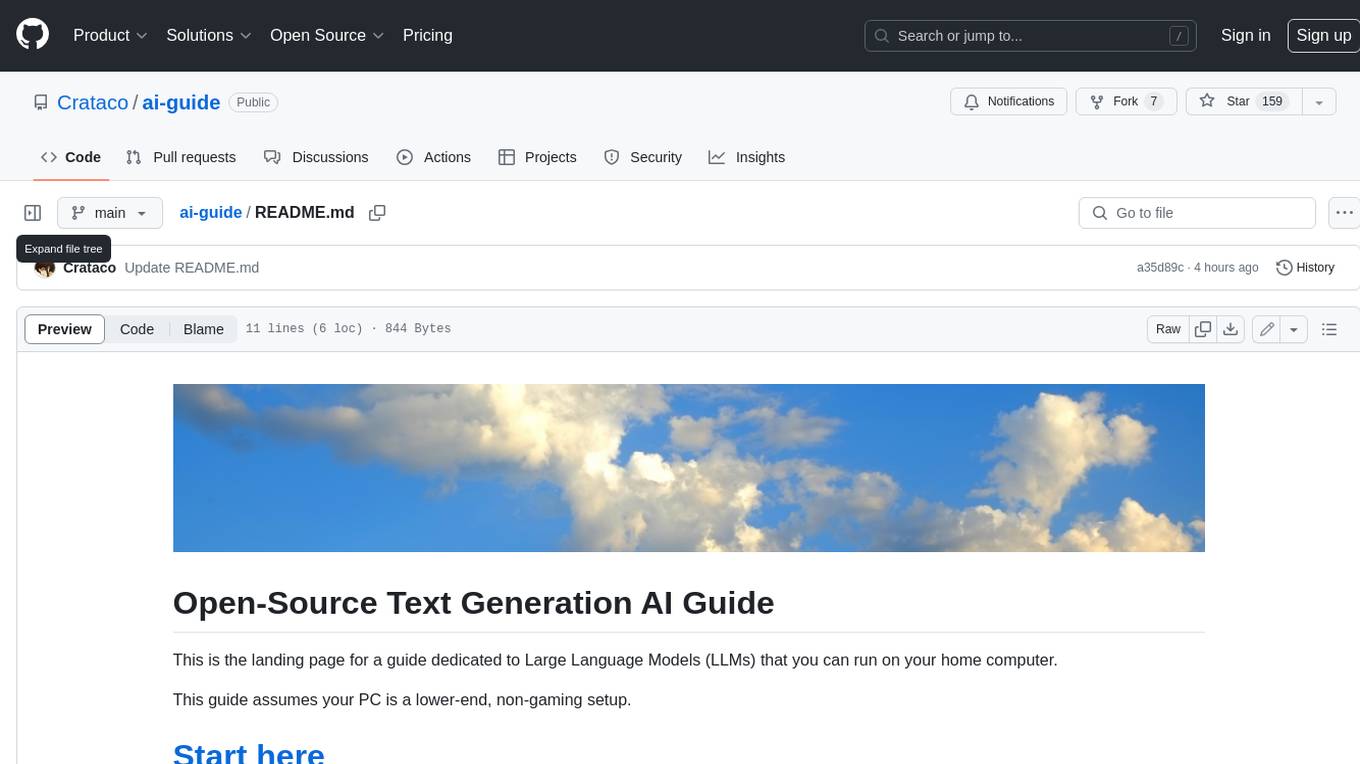
ai-guide
This guide is dedicated to Large Language Models (LLMs) that you can run on your home computer. It assumes your PC is a lower-end, non-gaming setup.
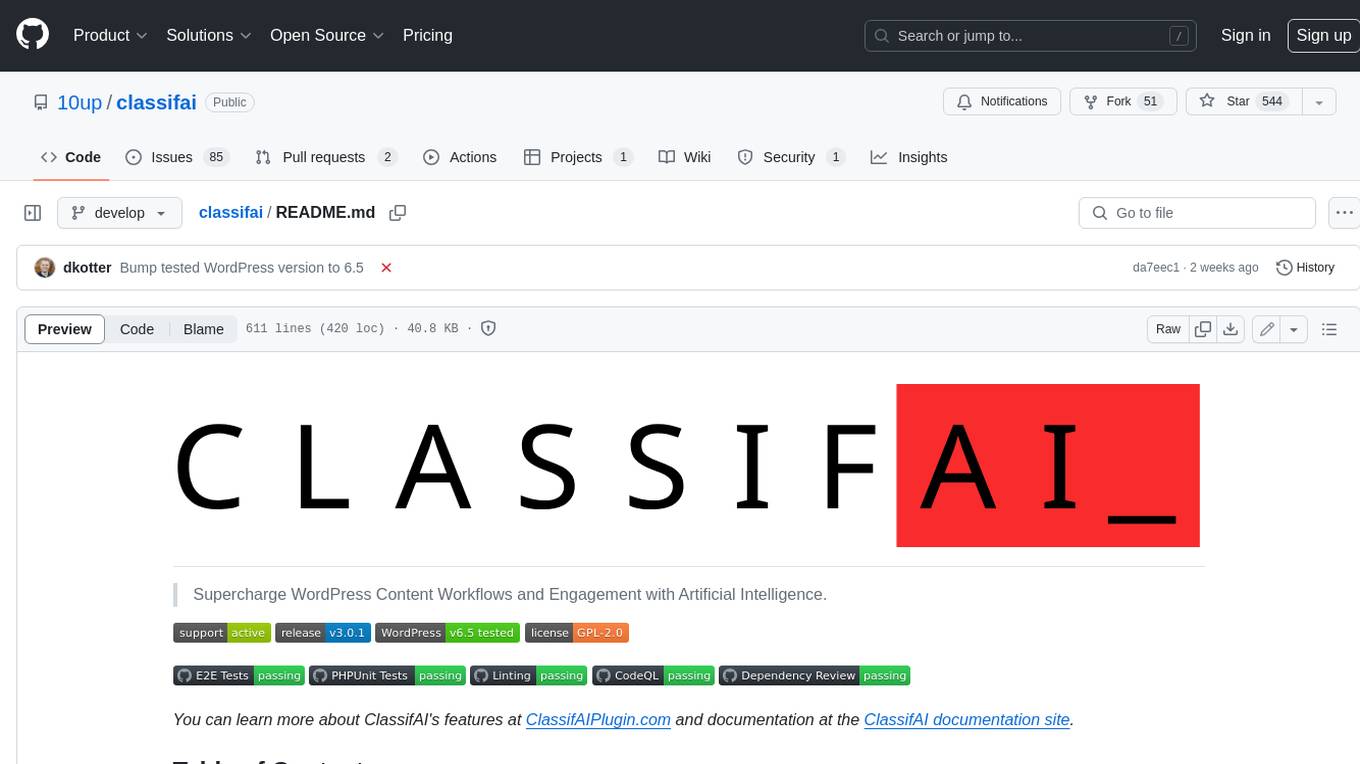
classifai
Supercharge WordPress Content Workflows and Engagement with Artificial Intelligence. Tap into leading cloud-based services like OpenAI, Microsoft Azure AI, Google Gemini and IBM Watson to augment your WordPress-powered websites. Publish content faster while improving SEO performance and increasing audience engagement. ClassifAI integrates Artificial Intelligence and Machine Learning technologies to lighten your workload and eliminate tedious tasks, giving you more time to create original content that matters.
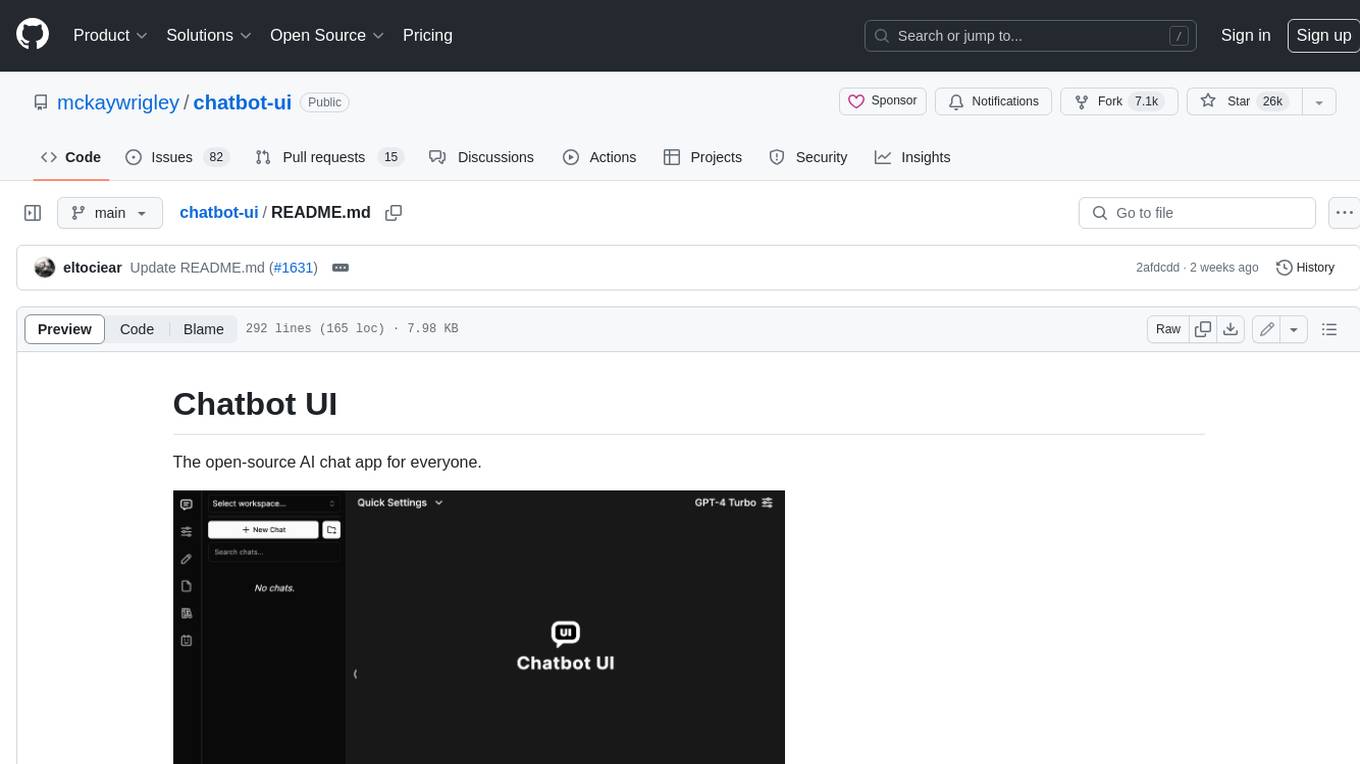
chatbot-ui
Chatbot UI is an open-source AI chat app that allows users to create and deploy their own AI chatbots. It is easy to use and can be customized to fit any need. Chatbot UI is perfect for businesses, developers, and anyone who wants to create a chatbot.
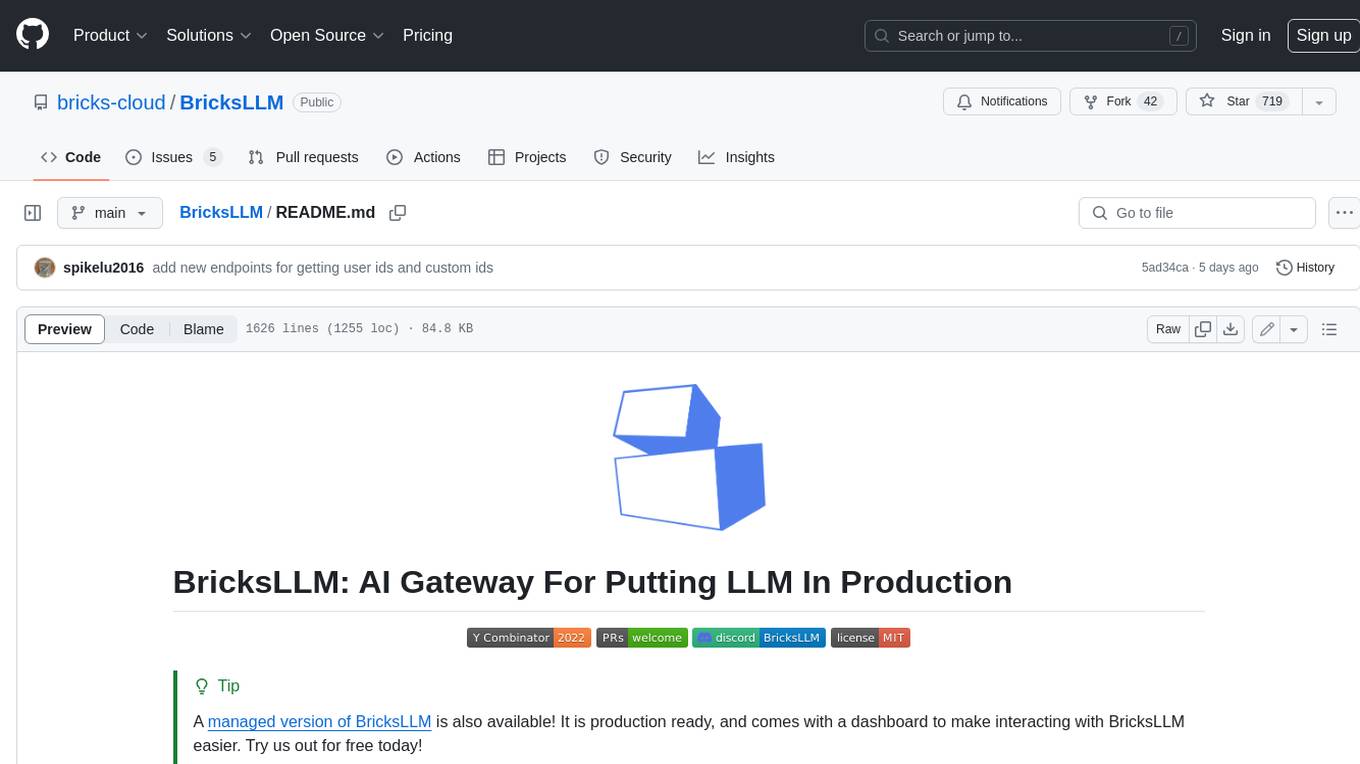
BricksLLM
BricksLLM is a cloud native AI gateway written in Go. Currently, it provides native support for OpenAI, Anthropic, Azure OpenAI and vLLM. BricksLLM aims to provide enterprise level infrastructure that can power any LLM production use cases. Here are some use cases for BricksLLM: * Set LLM usage limits for users on different pricing tiers * Track LLM usage on a per user and per organization basis * Block or redact requests containing PIIs * Improve LLM reliability with failovers, retries and caching * Distribute API keys with rate limits and cost limits for internal development/production use cases * Distribute API keys with rate limits and cost limits for students
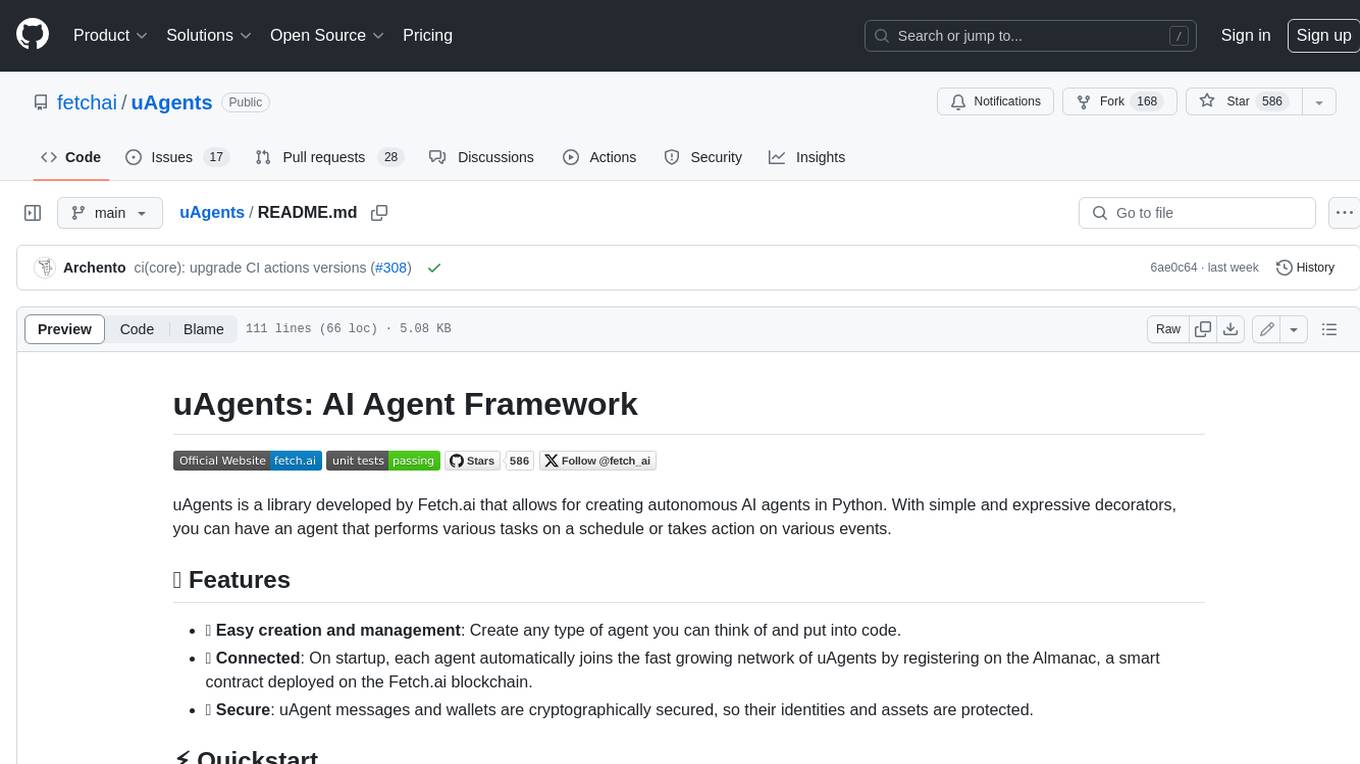
uAgents
uAgents is a Python library developed by Fetch.ai that allows for the creation of autonomous AI agents. These agents can perform various tasks on a schedule or take action on various events. uAgents are easy to create and manage, and they are connected to a fast-growing network of other uAgents. They are also secure, with cryptographically secured messages and wallets.
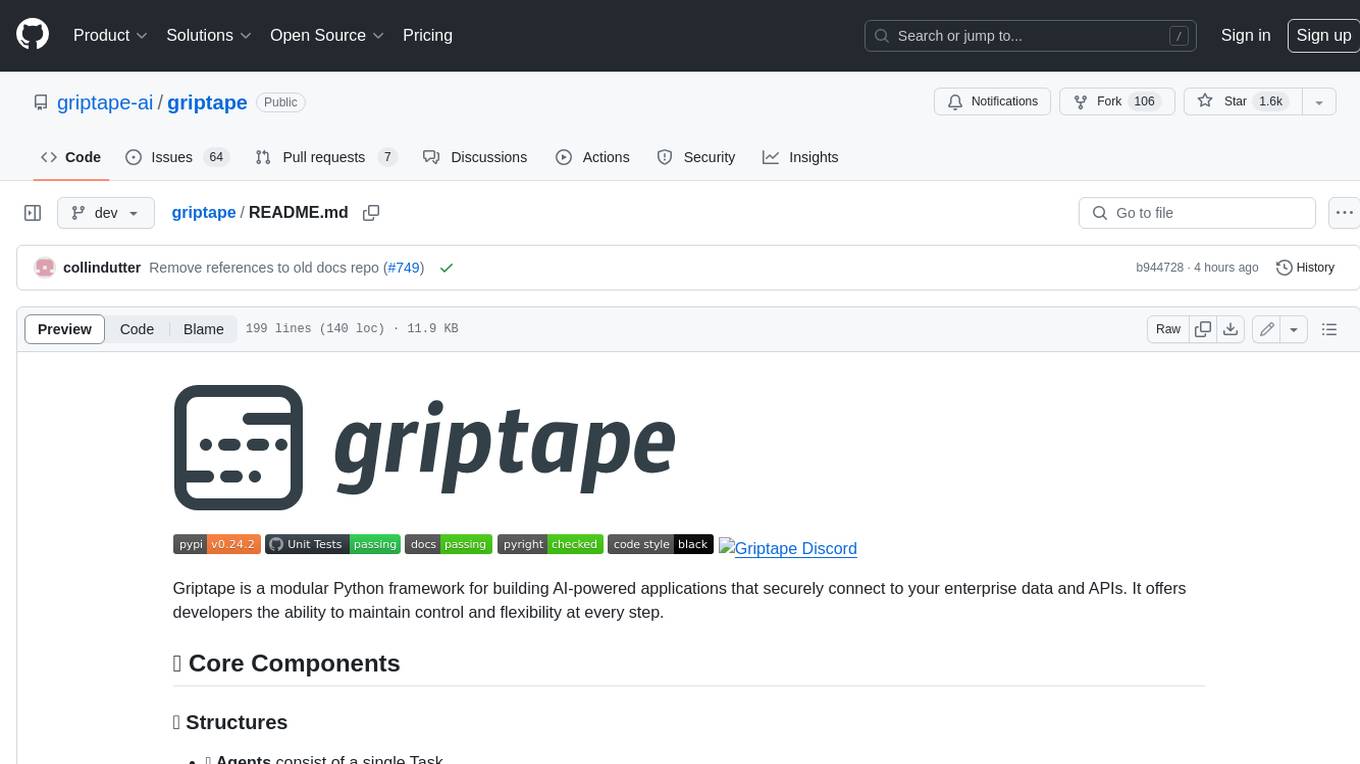
griptape
Griptape is a modular Python framework for building AI-powered applications that securely connect to your enterprise data and APIs. It offers developers the ability to maintain control and flexibility at every step. Griptape's core components include Structures (Agents, Pipelines, and Workflows), Tasks, Tools, Memory (Conversation Memory, Task Memory, and Meta Memory), Drivers (Prompt and Embedding Drivers, Vector Store Drivers, Image Generation Drivers, Image Query Drivers, SQL Drivers, Web Scraper Drivers, and Conversation Memory Drivers), Engines (Query Engines, Extraction Engines, Summary Engines, Image Generation Engines, and Image Query Engines), and additional components (Rulesets, Loaders, Artifacts, Chunkers, and Tokenizers). Griptape enables developers to create AI-powered applications with ease and efficiency.