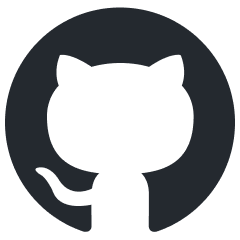
ollama-ex
A nifty little library for working with Ollama in Elixir.
Stars: 83
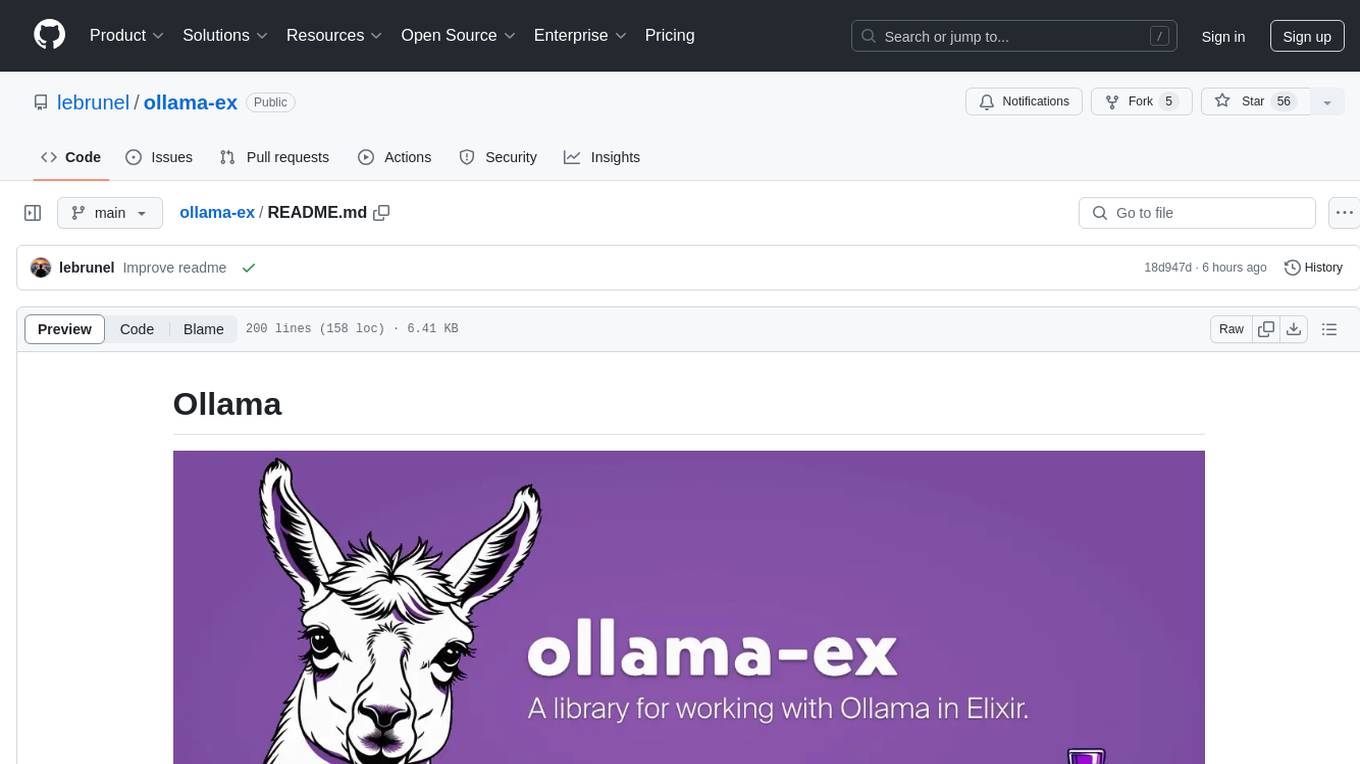
Ollama is a powerful tool for running large language models locally or on your own infrastructure. It provides a full implementation of the Ollama API, support for streaming requests, and tool use capability. Users can interact with Ollama in Elixir to generate completions, chat messages, and perform streaming requests. The tool also supports function calling on compatible models, allowing users to define tools with clear descriptions and arguments. Ollama is designed to facilitate natural language processing tasks and enhance user interactions with language models.
README:
Ollama is a powerful tool for running large language models locally or on your own infrastructure. This library provides an interface for working with Ollama in Elixir.
- 🦙 Full implementation of the Ollama API
- 🧰 Tool use (function calling)
- 🧱 Structured outputs
- 🛜 Streaming requests
- Stream to an Enumerable
- Or stream messages to any Elixir process
The package can be installed by adding ollama
to your list of dependencies in mix.exs
.
def deps do
[
{:ollama, "~> 0.8"}
]
end
For more examples, refer to the Ollama documentation.
client = Ollama.init()
Ollama.completion(client, [
model: "llama2",
prompt: "Why is the sky blue?",
])
# {:ok, %{"response" => "The sky is blue because it is the color of the sky.", ...}}
Ollama.chat(client, [
model: "llama2",
messages: [
%{role: "system", content: "You are a helpful assistant."},
%{role: "user", content: "Why is the sky blue?"},
%{role: "assistant", content: "Due to rayleigh scattering."},
%{role: "user", content: "How is that different than mie scattering?"},
]
])
# {:ok, %{"message" => %{
# "role" => "assistant",
# "content" => "Mie scattering affects all wavelengths similarly, while Rayleigh favors shorter ones."
# }, ...}}
The :format
option can be used with both completion/2
and chat/2
.
Ollama.completion(client, [
model: "llama3.1",
prompt: "Tell me about Canada",
format: %{
type: "object",
properties: %{
name: %{type: "string"},
capital: %{type: "string"},
languages: %{type: "array", items: %{type: "string"}},
},
required: ["name", "capital", "languages"]
}
])
# {:ok, %{"response" => "{ \"name\": \"Canada\" ,\"capital\": \"Ottawa\" ,\"languages\": [\"English\", \"French\"] }", ...}}
Streaming is supported on certain endpoints by setting the :stream
option to true
or a t:pid/0
.
When :stream
is set to true
, a lazy t:Enumerable.t/0
is returned, which can be used with any Stream
functions.
{:ok, stream} = Ollama.completion(client, [
model: "llama2",
prompt: "Why is the sky blue?",
stream: true,
])
stream
|> Stream.each(& Process.send(pid, &1, [])
|> Stream.run()
# :ok
This approach above builds the t:Enumerable.t/0
by calling receive
, which may cause issues in GenServer
callbacks. As an alternative, you can set the :stream
option to a t:pid/0
. This returns a t:Task.t/0
that sends messages to the specified process.
The following example demonstrates a streaming request in a LiveView event, sending each streaming message back to the same LiveView process:
defmodule MyApp.ChatLive do
use Phoenix.LiveView
# When the client invokes the "prompt" event, create a streaming request and
# asynchronously send messages back to self.
def handle_event("prompt", %{"message" => prompt}, socket) do
{:ok, task} = Ollama.completion(Ollama.init(), [
model: "llama2",
prompt: prompt,
stream: self(),
])
{:noreply, assign(socket, current_request: task)}
end
# The streaming request sends messages back to the LiveView process.
def handle_info({_request_pid, {:data, _data}} = message, socket) do
pid = socket.assigns.current_request.pid
case message do
{^pid, {:data, %{"done" => false} = data}} ->
# handle each streaming chunk
{^pid, {:data, %{"done" => true} = data}} ->
# handle the final streaming chunk
{_pid, _data} ->
# this message was not expected!
end
end
# Tidy up when the request is finished
def handle_info({ref, {:ok, %Req.Response{status: 200}}}, socket) do
Process.demonitor(ref, [:flush])
{:noreply, assign(socket, current_request: nil)}
end
end
Regardless of the streaming approach used, each streaming message is a plain t:map/0
. For the message schema, refer to the Ollama API docs.
Ollama 0.3 and later versions support tool use and function calling on compatible models. Note that Ollama currently doesn't support tool use with streaming requests, so avoid setting :stream
to true
.
Using tools typically involves at least two round-trip requests to the model. Begin by defining one or more tools using a schema similar to ChatGPT's. Provide clear and concise descriptions for the tool and each argument.
stock_price_tool = %{
type: "function",
function: %{
name: "get_stock_price",
description: "Fetches the live stock price for the given ticker.",
parameters: %{
type: "object",
properties: %{
ticker: %{
type: "string",
description: "The ticker symbol of a specific stock."
}
},
required: ["ticker"]
}
}
}
The first round-trip involves sending a prompt in a chat with the tool definitions. The model should respond with a message containing a list of tool calls.
Ollama.chat(client, [
model: "mistral-nemo",
messages: [
%{role: "user", content: "What is the current stock price for Apple?"}
],
tools: [stock_price_tool],
])
# {:ok, %{"message" => %{
# "role" => "assistant",
# "content" => "",
# "tool_calls" => [
# %{"function" => %{
# "name" => "get_stock_price",
# "arguments" => %{"ticker" => "AAPL"}
# }}
# ]
# }, ...}}
Your implementation must intercept these tool calls and execute a corresponding function in your codebase with the specified arguments. The next round-trip involves passing the function's result back to the model as a message with a :role
of "tool"
.
Ollama.chat(client, [
model: "mistral-nemo",
messages: [
%{role: "user", content: "What is the current stock price for Apple?"},
%{role: "assistant", content: "", tool_calls: [%{"function" => %{"name" => "get_stock_price", "arguments" => %{"ticker" => "AAPL"}}}]},
%{role: "tool", content: "$217.96"},
],
tools: [stock_price_tool],
])
# {:ok, %{"message" => %{
# "role" => "assistant",
# "content" => "The current stock price for Apple (AAPL) is approximately $217.96.",
# }, ...}}
After receiving the function tool's value, the model will respond to the user's original prompt, incorporating the function result into its response.
This package is open source and released under the Apache-2 License.
© Copyright 2024 Push Code Ltd.
For Tasks:
Click tags to check more tools for each tasksFor Jobs:
Alternative AI tools for ollama-ex
Similar Open Source Tools
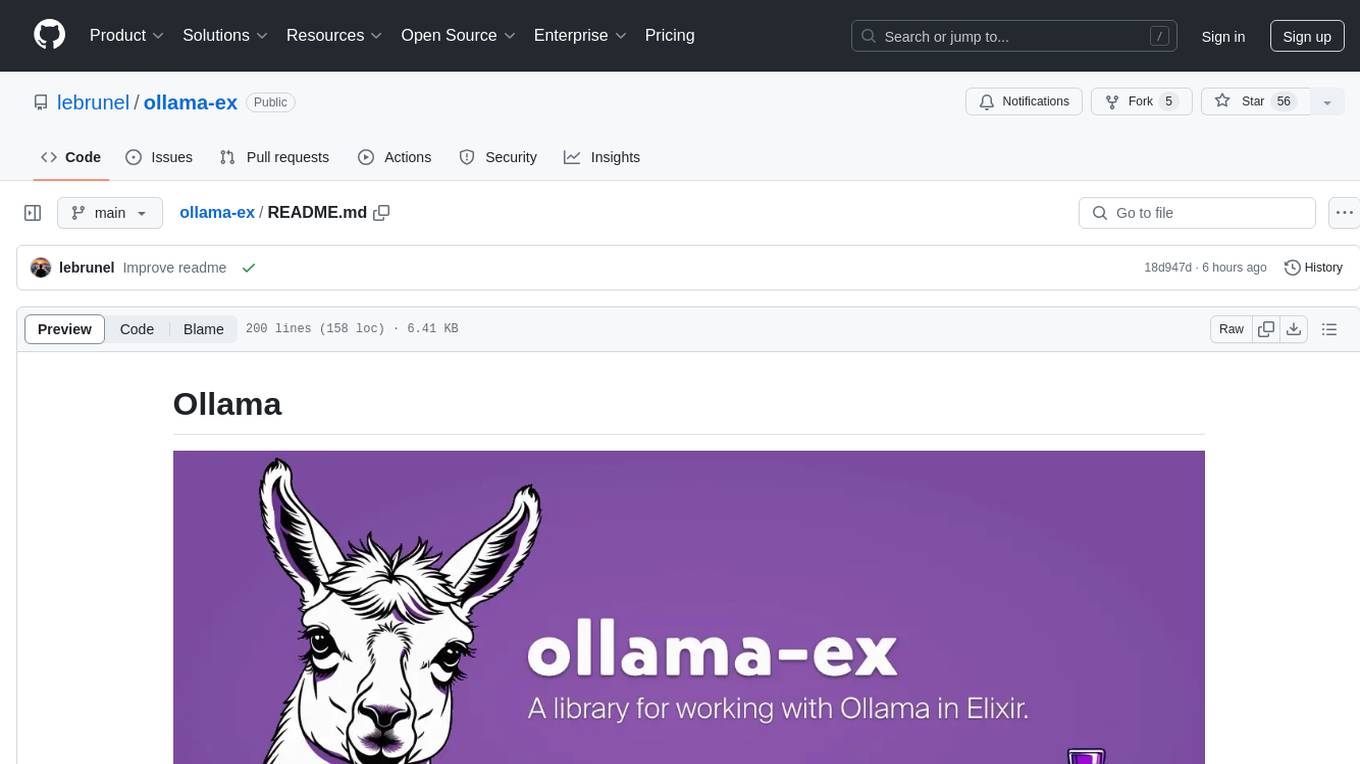
ollama-ex
Ollama is a powerful tool for running large language models locally or on your own infrastructure. It provides a full implementation of the Ollama API, support for streaming requests, and tool use capability. Users can interact with Ollama in Elixir to generate completions, chat messages, and perform streaming requests. The tool also supports function calling on compatible models, allowing users to define tools with clear descriptions and arguments. Ollama is designed to facilitate natural language processing tasks and enhance user interactions with language models.
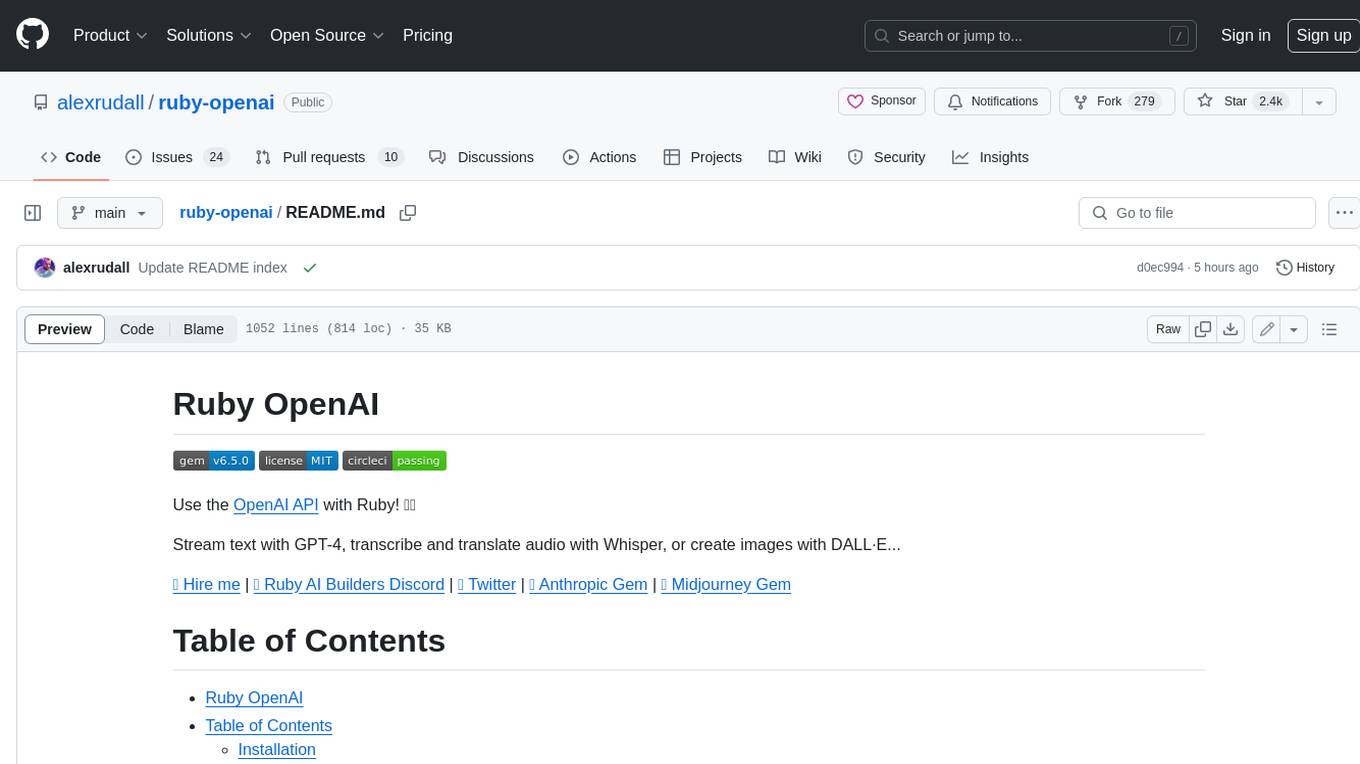
ruby-openai
Use the OpenAI API with Ruby! 🤖🩵 Stream text with GPT-4, transcribe and translate audio with Whisper, or create images with DALL·E... Hire me | 🎮 Ruby AI Builders Discord | 🐦 Twitter | 🧠 Anthropic Gem | 🚂 Midjourney Gem ## Table of Contents * Ruby OpenAI * Table of Contents * Installation * Bundler * Gem install * Usage * Quickstart * With Config * Custom timeout or base URI * Extra Headers per Client * Logging * Errors * Faraday middleware * Azure * Ollama * Counting Tokens * Models * Examples * Chat * Streaming Chat * Vision * JSON Mode * Functions * Edits * Embeddings * Batches * Files * Finetunes * Assistants * Threads and Messages * Runs * Runs involving function tools * Image Generation * DALL·E 2 * DALL·E 3 * Image Edit * Image Variations * Moderations * Whisper * Translate * Transcribe * Speech * Errors * Development * Release * Contributing * License * Code of Conduct
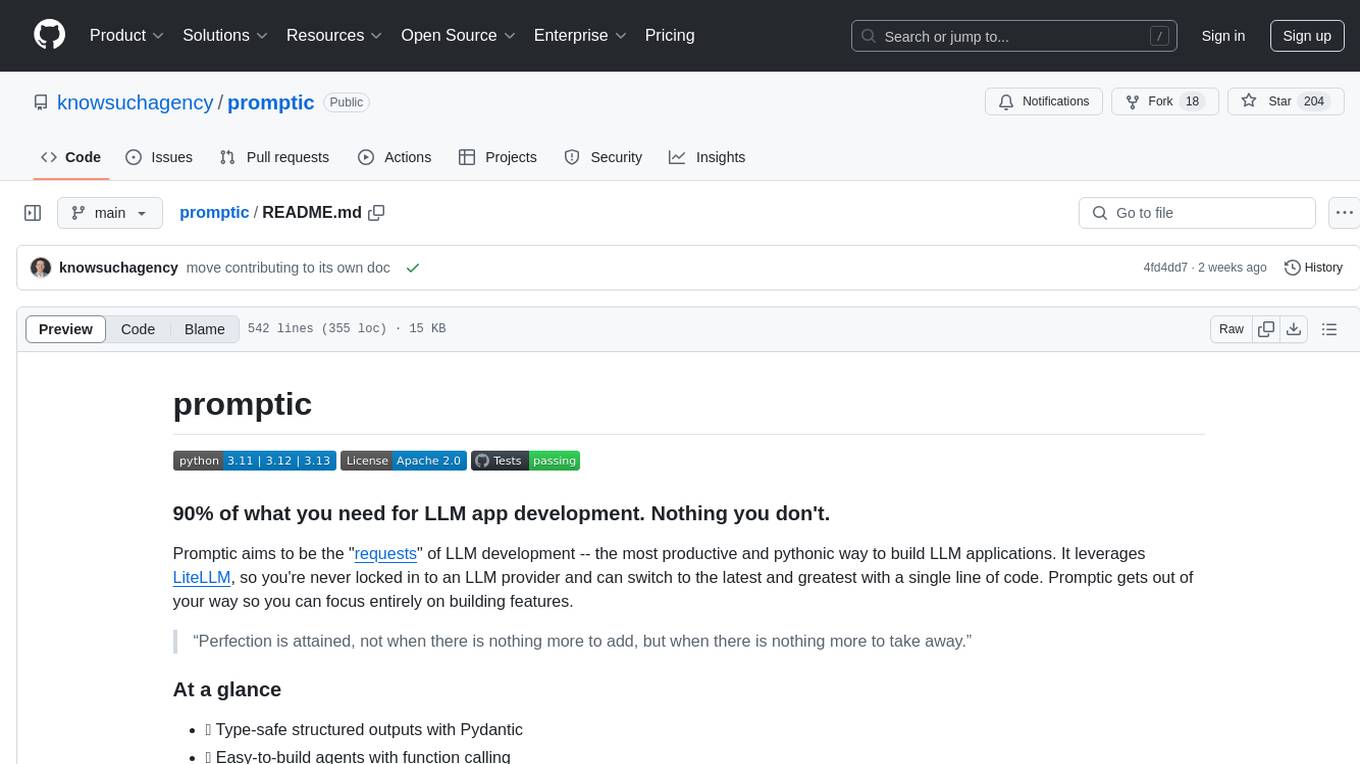
promptic
Promptic is a tool designed for LLM app development, providing a productive and pythonic way to build LLM applications. It leverages LiteLLM, allowing flexibility to switch LLM providers easily. Promptic focuses on building features by providing type-safe structured outputs, easy-to-build agents, streaming support, automatic prompt caching, and built-in conversation memory.
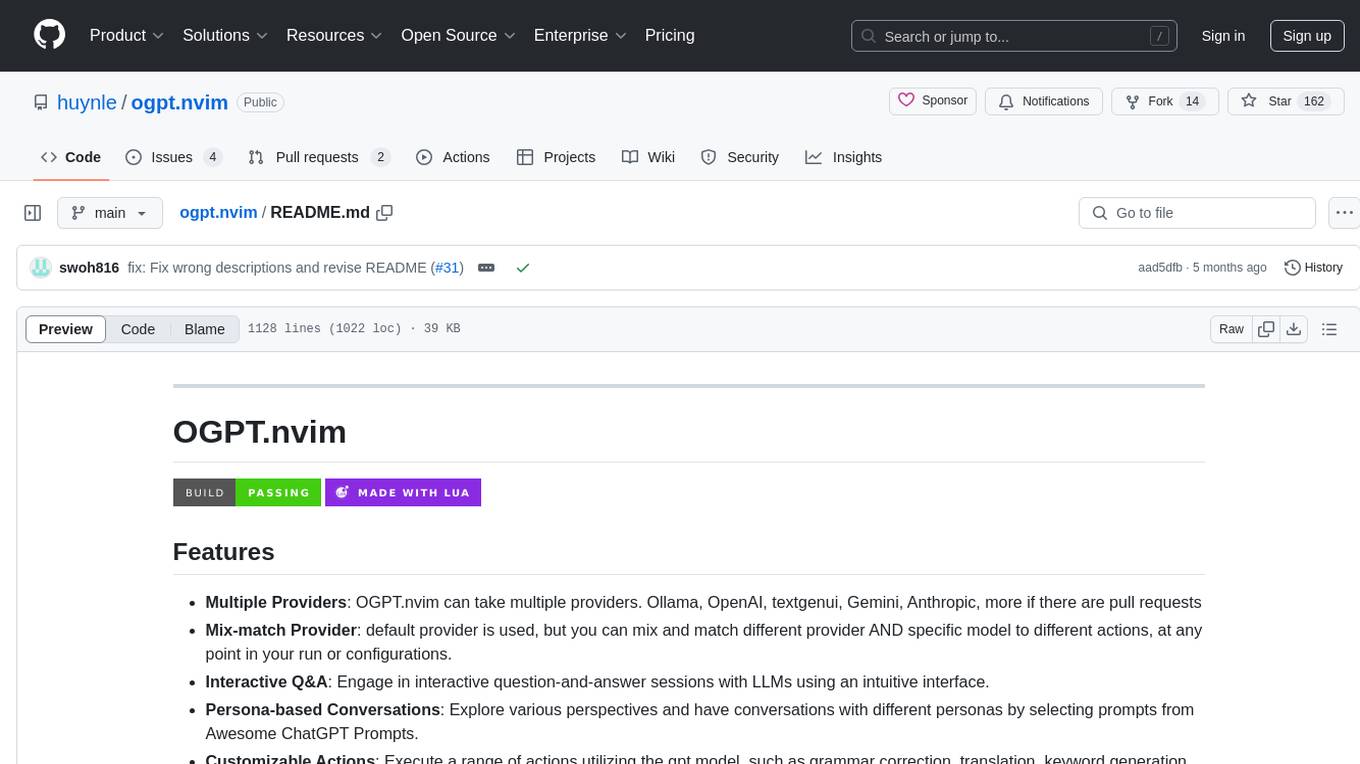
ogpt.nvim
OGPT.nvim is a Neovim plugin that enables users to interact with various language models (LLMs) such as Ollama, OpenAI, TextGenUI, and more. Users can engage in interactive question-and-answer sessions, have persona-based conversations, and execute customizable actions like grammar correction, translation, keyword generation, docstring creation, test addition, code optimization, summarization, bug fixing, code explanation, and code readability analysis. The plugin allows users to define custom actions using a JSON file or plugin configurations.
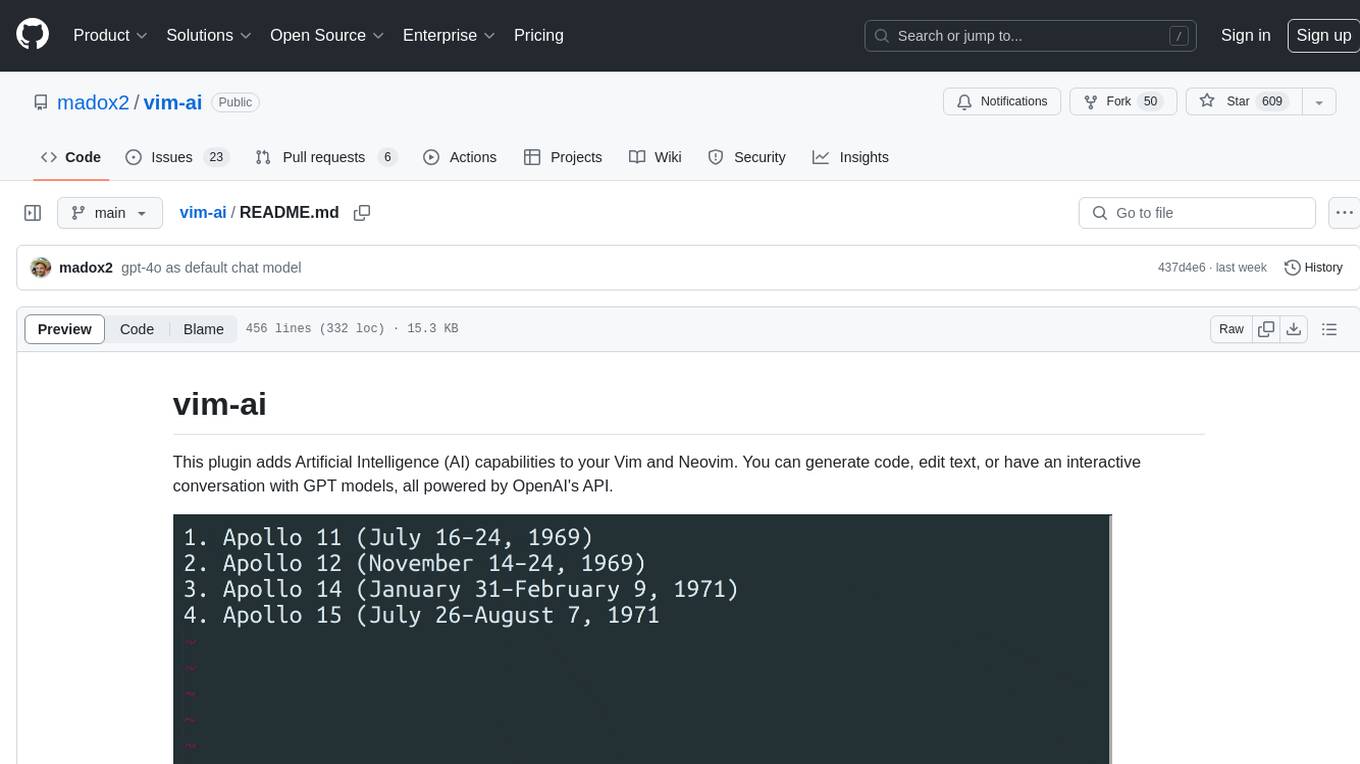
vim-ai
vim-ai is a plugin that adds Artificial Intelligence (AI) capabilities to Vim and Neovim. It allows users to generate code, edit text, and have interactive conversations with GPT models powered by OpenAI's API. The plugin uses OpenAI's API to generate responses, requiring users to set up an account and obtain an API key. It supports various commands for text generation, editing, and chat interactions, providing a seamless integration of AI features into the Vim text editor environment.
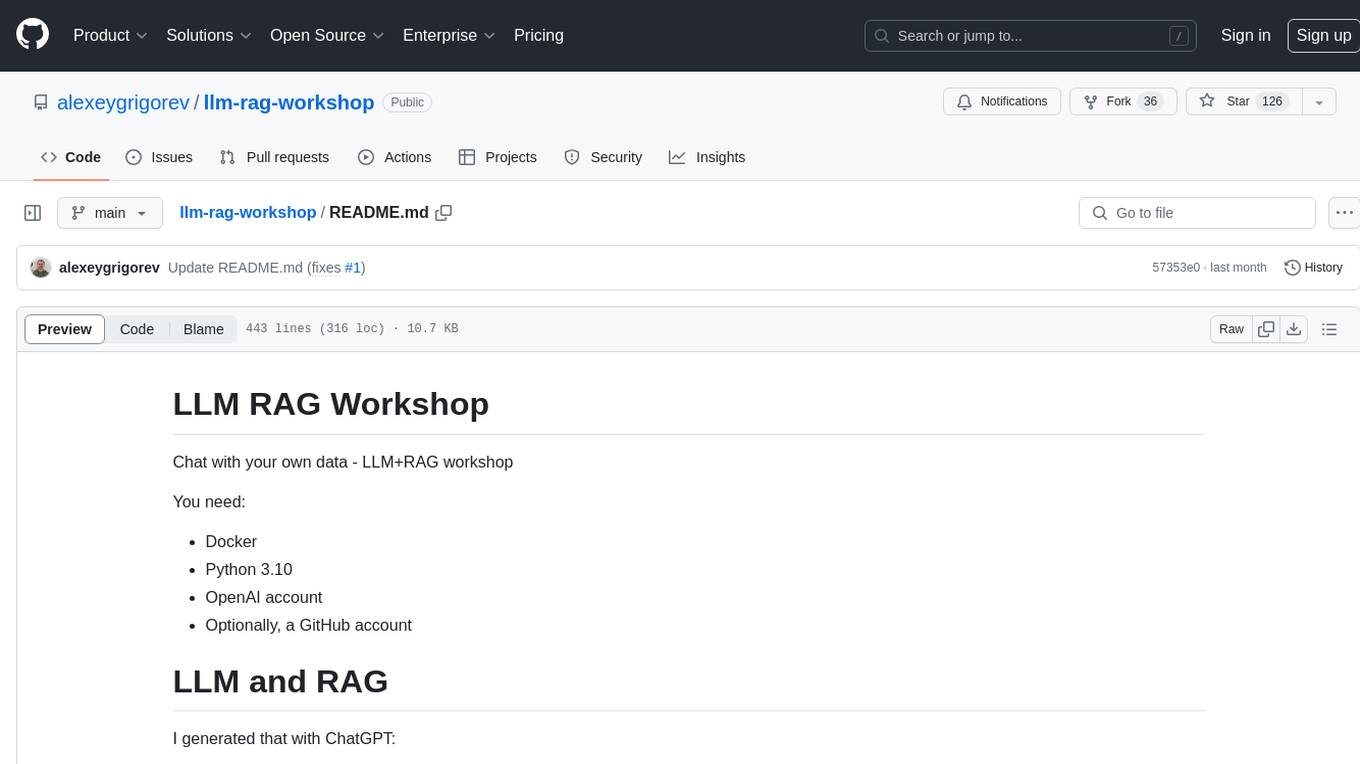
llm-rag-workshop
The LLM RAG Workshop repository provides a workshop on using Large Language Models (LLMs) and Retrieval-Augmented Generation (RAG) to generate and understand text in a human-like manner. It includes instructions on setting up the environment, indexing Zoomcamp FAQ documents, creating a Q&A system, and using OpenAI for generation based on retrieved information. The repository focuses on enhancing language model responses with retrieved information from external sources, such as document databases or search engines, to improve factual accuracy and relevance of generated text.
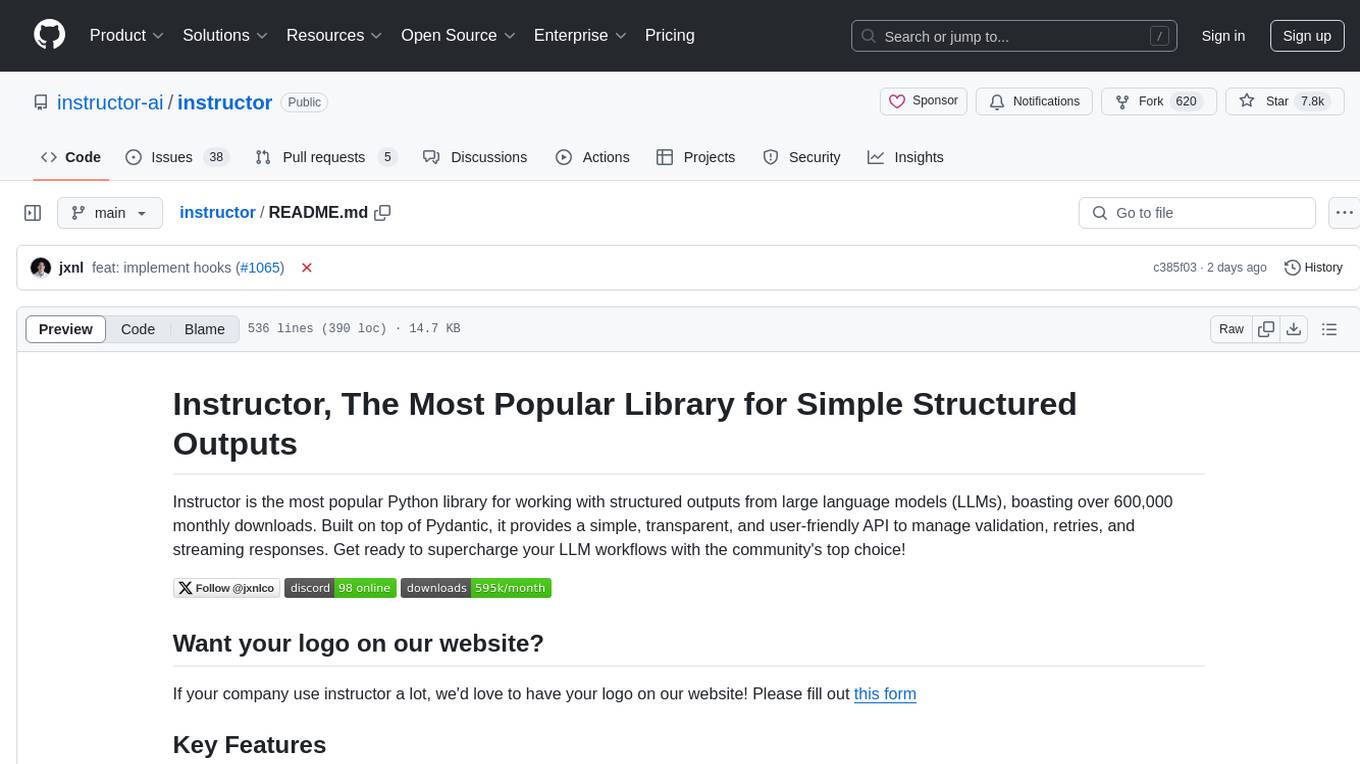
instructor
Instructor is a popular Python library for managing structured outputs from large language models (LLMs). It offers a user-friendly API for validation, retries, and streaming responses. With support for various LLM providers and multiple languages, Instructor simplifies working with LLM outputs. The library includes features like response models, retry management, validation, streaming support, and flexible backends. It also provides hooks for logging and monitoring LLM interactions, and supports integration with Anthropic, Cohere, Gemini, Litellm, and Google AI models. Instructor facilitates tasks such as extracting user data from natural language, creating fine-tuned models, managing uploaded files, and monitoring usage of OpenAI models.
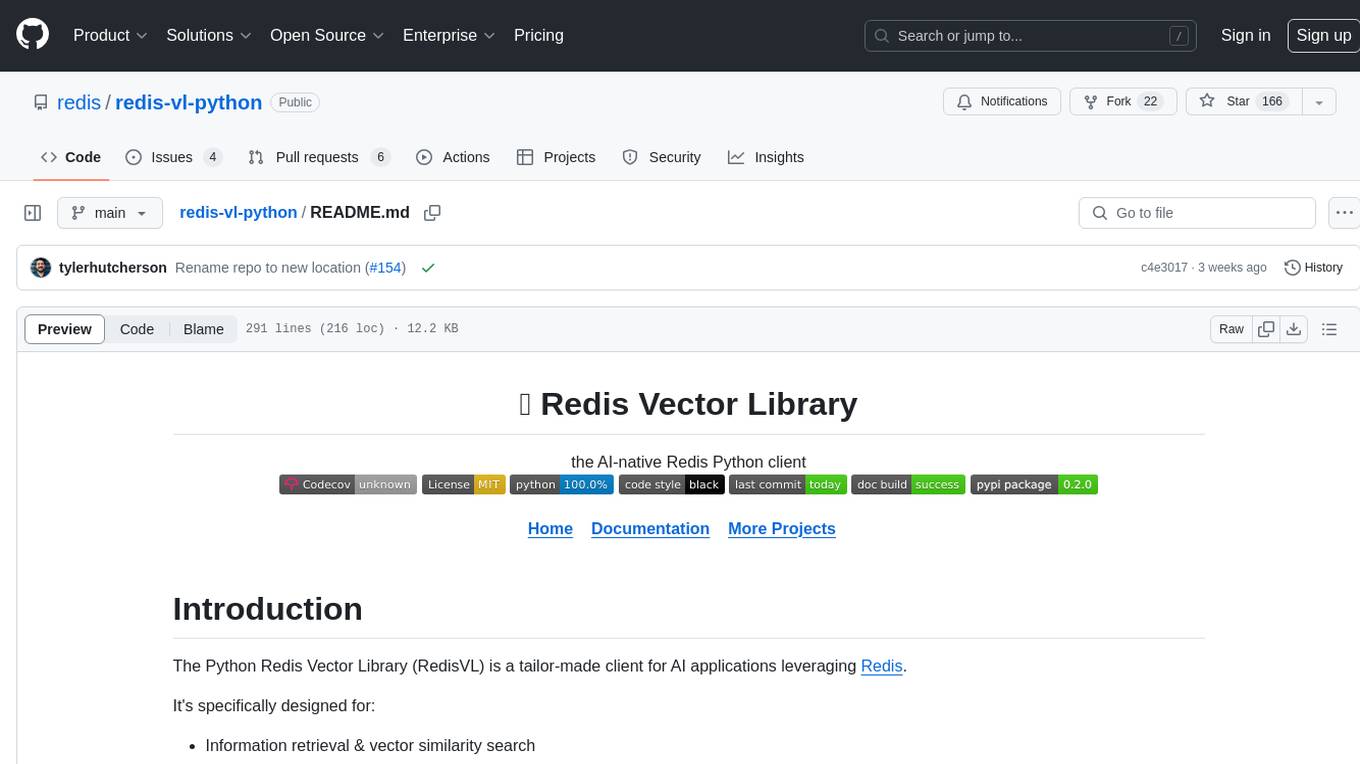
redis-vl-python
The Python Redis Vector Library (RedisVL) is a tailor-made client for AI applications leveraging Redis. It enhances applications with Redis' speed, flexibility, and reliability, incorporating capabilities like vector-based semantic search, full-text search, and geo-spatial search. The library bridges the gap between the emerging AI-native developer ecosystem and the capabilities of Redis by providing a lightweight, elegant, and intuitive interface. It abstracts the features of Redis into a grammar that is more aligned to the needs of today's AI/ML Engineers or Data Scientists.
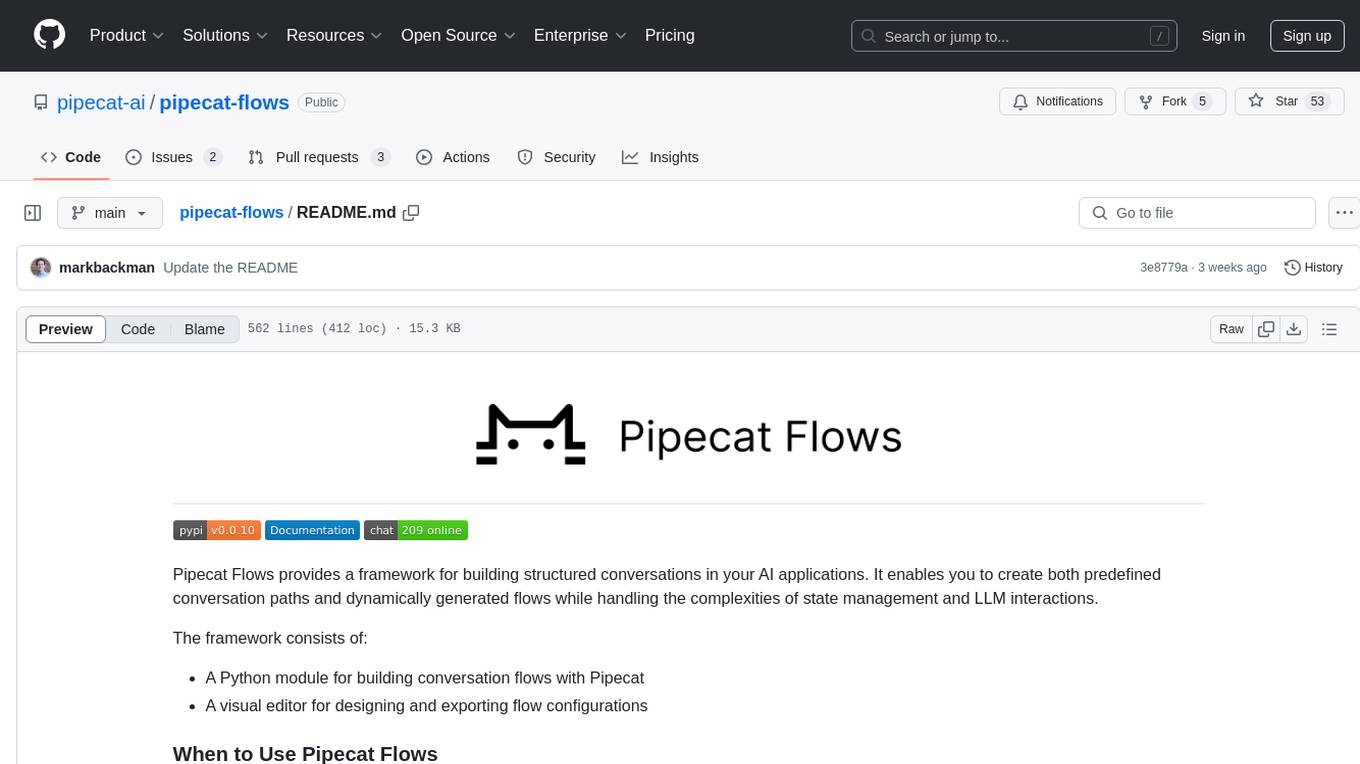
pipecat-flows
Pipecat Flows is a framework designed for building structured conversations in AI applications. It allows users to create both predefined conversation paths and dynamically generated flows, handling state management and LLM interactions. The framework includes a Python module for building conversation flows and a visual editor for designing and exporting flow configurations. Pipecat Flows is suitable for scenarios such as customer service scripts, intake forms, personalized experiences, and complex decision trees.
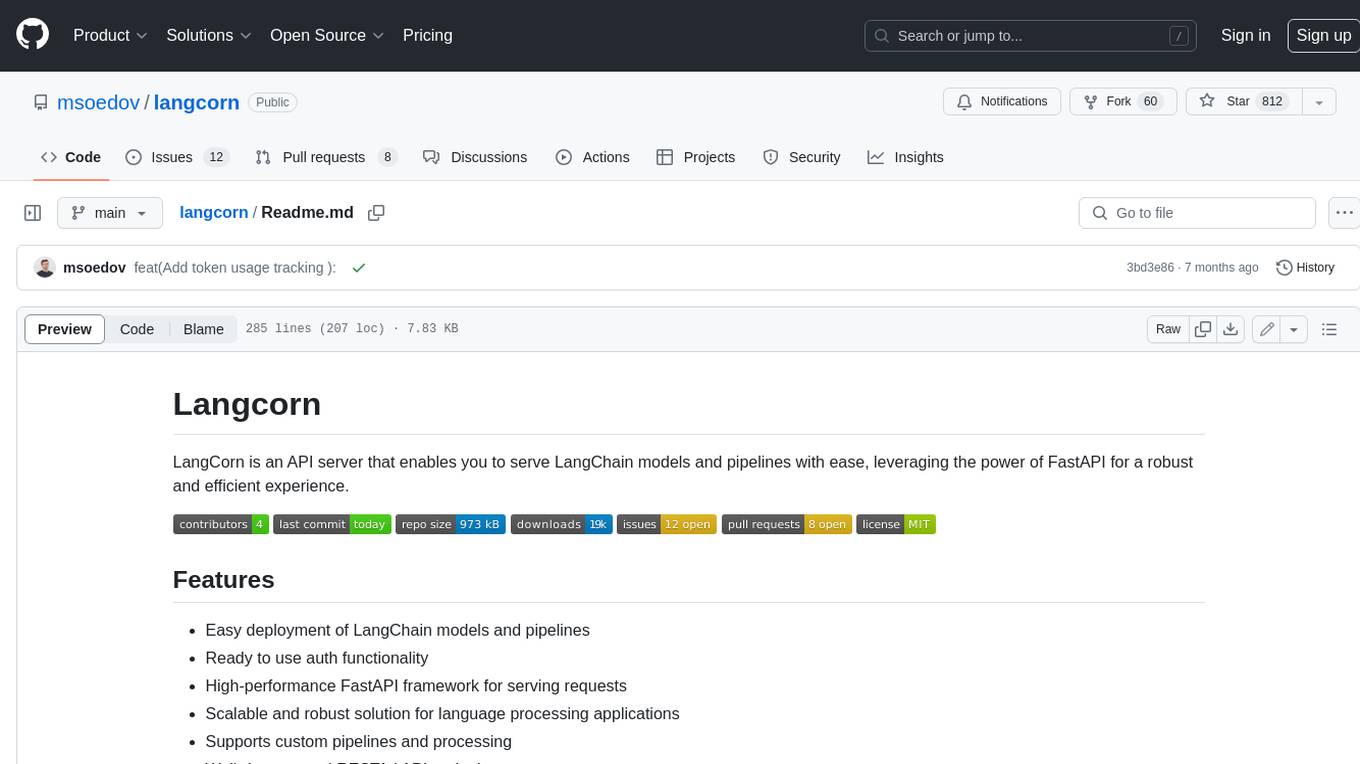
langcorn
LangCorn is an API server that enables you to serve LangChain models and pipelines with ease, leveraging the power of FastAPI for a robust and efficient experience. It offers features such as easy deployment of LangChain models and pipelines, ready-to-use authentication functionality, high-performance FastAPI framework for serving requests, scalability and robustness for language processing applications, support for custom pipelines and processing, well-documented RESTful API endpoints, and asynchronous processing for faster response times.
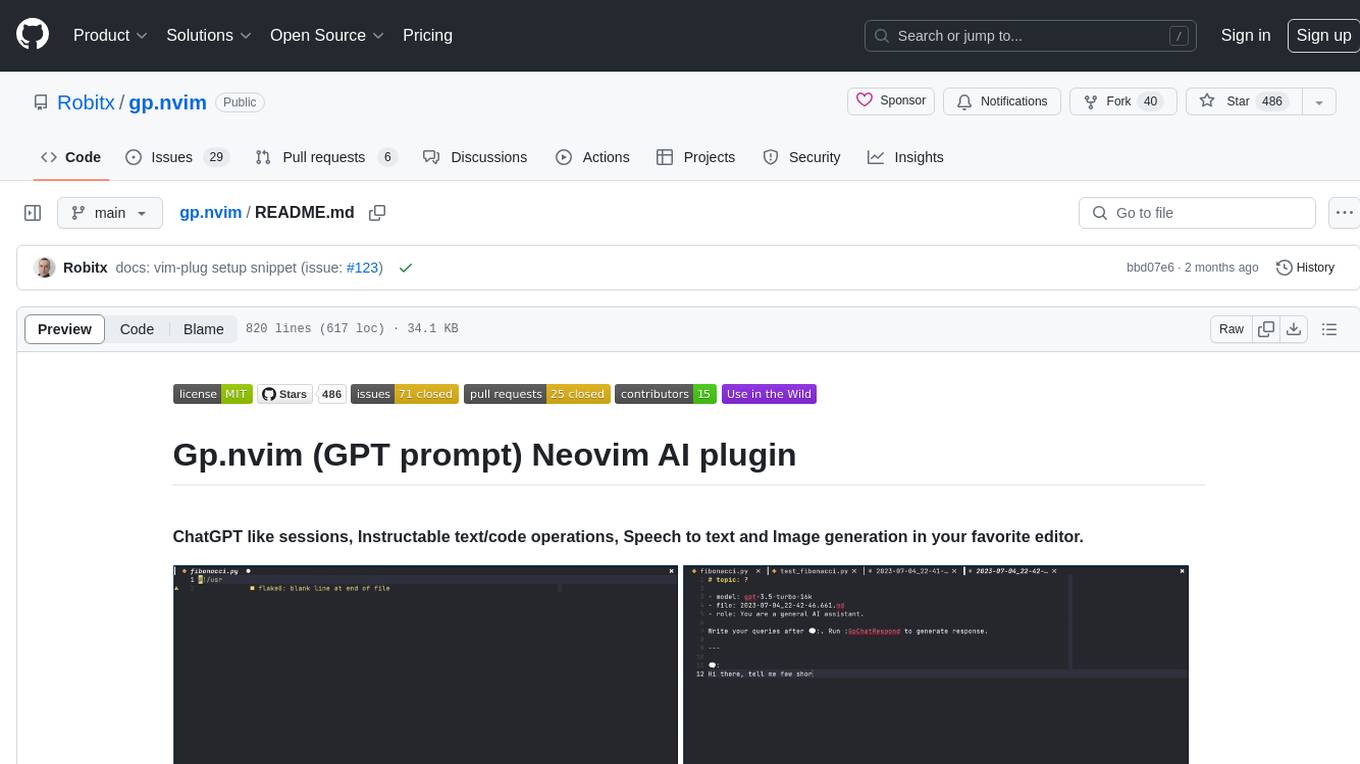
gp.nvim
Gp.nvim (GPT prompt) Neovim AI plugin provides a seamless integration of GPT models into Neovim, offering features like streaming responses, extensibility via hook functions, minimal dependencies, ChatGPT-like sessions, instructable text/code operations, speech-to-text support, and image generation directly within Neovim. The plugin aims to enhance the Neovim experience by leveraging the power of AI models in a user-friendly and native way.
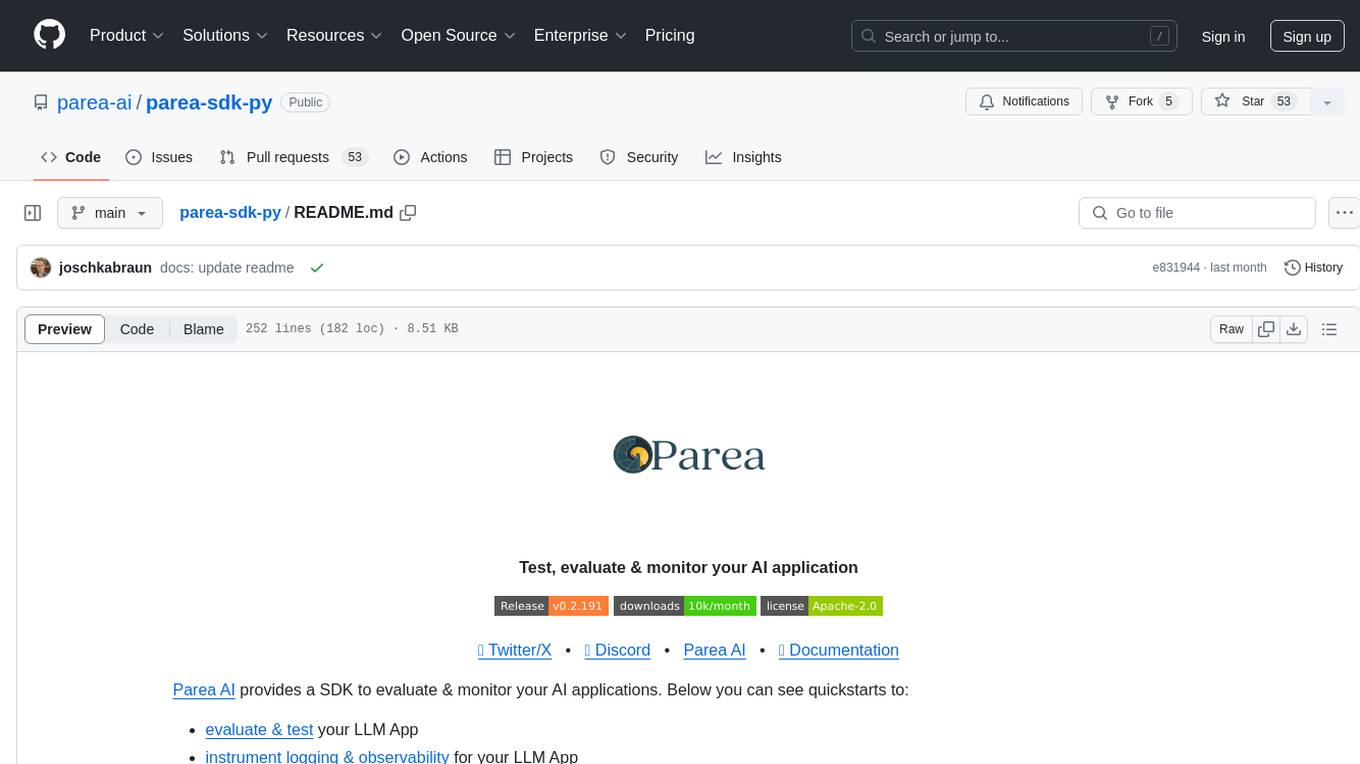
parea-sdk-py
Parea AI provides a SDK to evaluate & monitor AI applications. It allows users to test, evaluate, and monitor their AI models by defining and running experiments. The SDK also enables logging and observability for AI applications, as well as deploying prompts to facilitate collaboration between engineers and subject-matter experts. Users can automatically log calls to OpenAI and Anthropic, create hierarchical traces of their applications, and deploy prompts for integration into their applications.
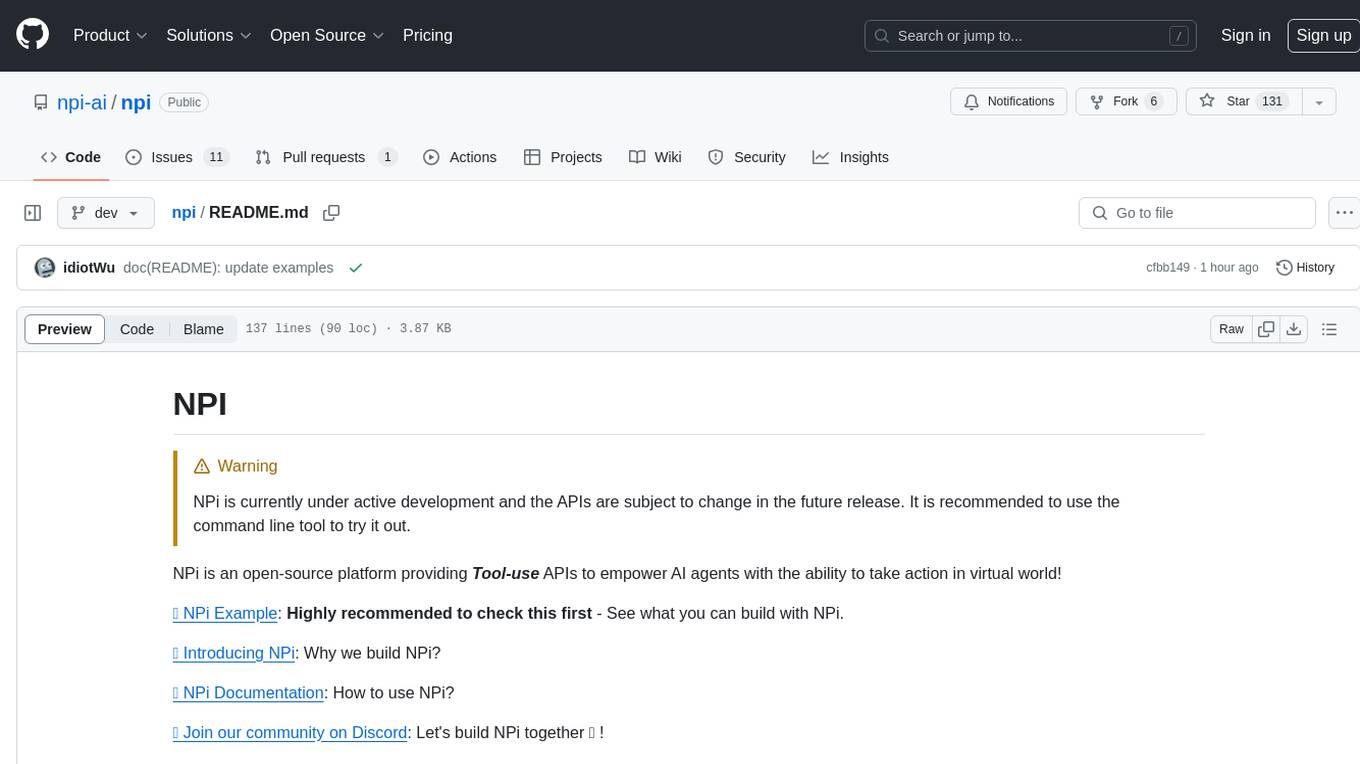
npi
NPi is an open-source platform providing Tool-use APIs to empower AI agents with the ability to take action in the virtual world. It is currently under active development, and the APIs are subject to change in future releases. NPi offers a command line tool for installation and setup, along with a GitHub app for easy access to repositories. The platform also includes a Python SDK and examples like Calendar Negotiator and Twitter Crawler. Join the NPi community on Discord to contribute to the development and explore the roadmap for future enhancements.
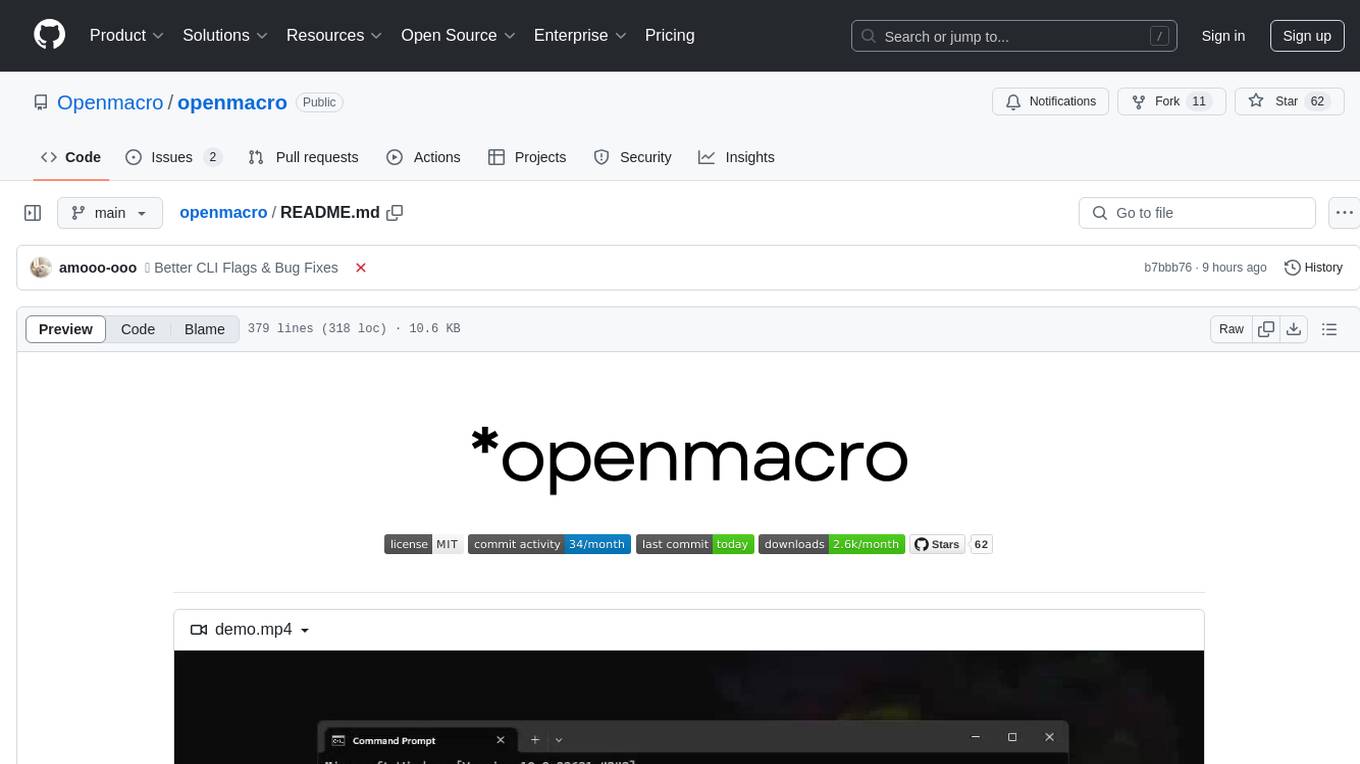
openmacro
Openmacro is a multimodal personal agent that allows users to run code locally. It acts as a personal agent capable of completing and automating tasks autonomously via self-prompting. The tool provides a CLI natural-language interface for completing and automating tasks, analyzing and plotting data, browsing the web, and manipulating files. Currently, it supports API keys for models powered by SambaNova, with plans to add support for other hosts like OpenAI and Anthropic in future versions.
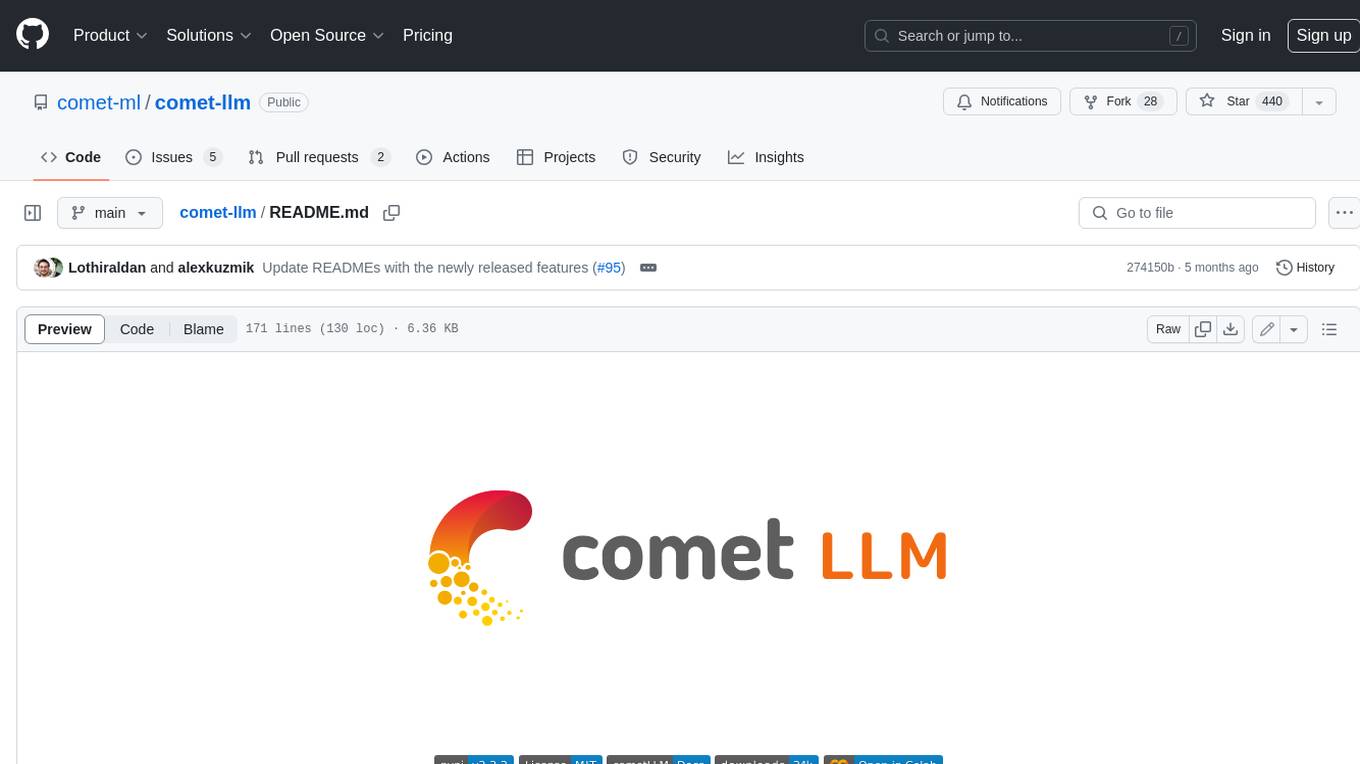
comet-llm
CometLLM is a tool to log and visualize your LLM prompts and chains. Use CometLLM to identify effective prompt strategies, streamline your troubleshooting, and ensure reproducible workflows!
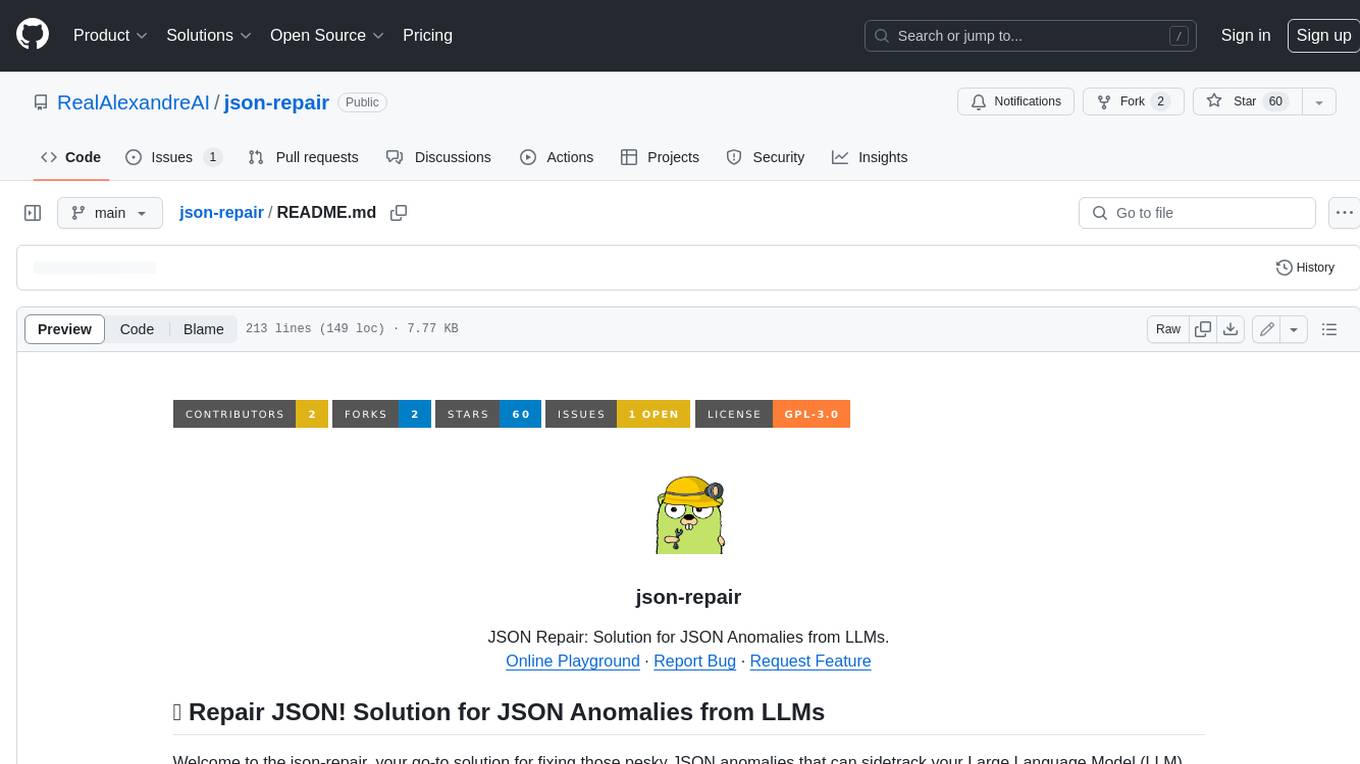
json-repair
JSON Repair is a toolkit designed to address JSON anomalies that can arise from Large Language Models (LLMs). It offers a comprehensive solution for repairing JSON strings, ensuring accuracy and reliability in your data processing. With its user-friendly interface and extensive capabilities, JSON Repair empowers developers to seamlessly integrate JSON repair into their workflows.
For similar tasks
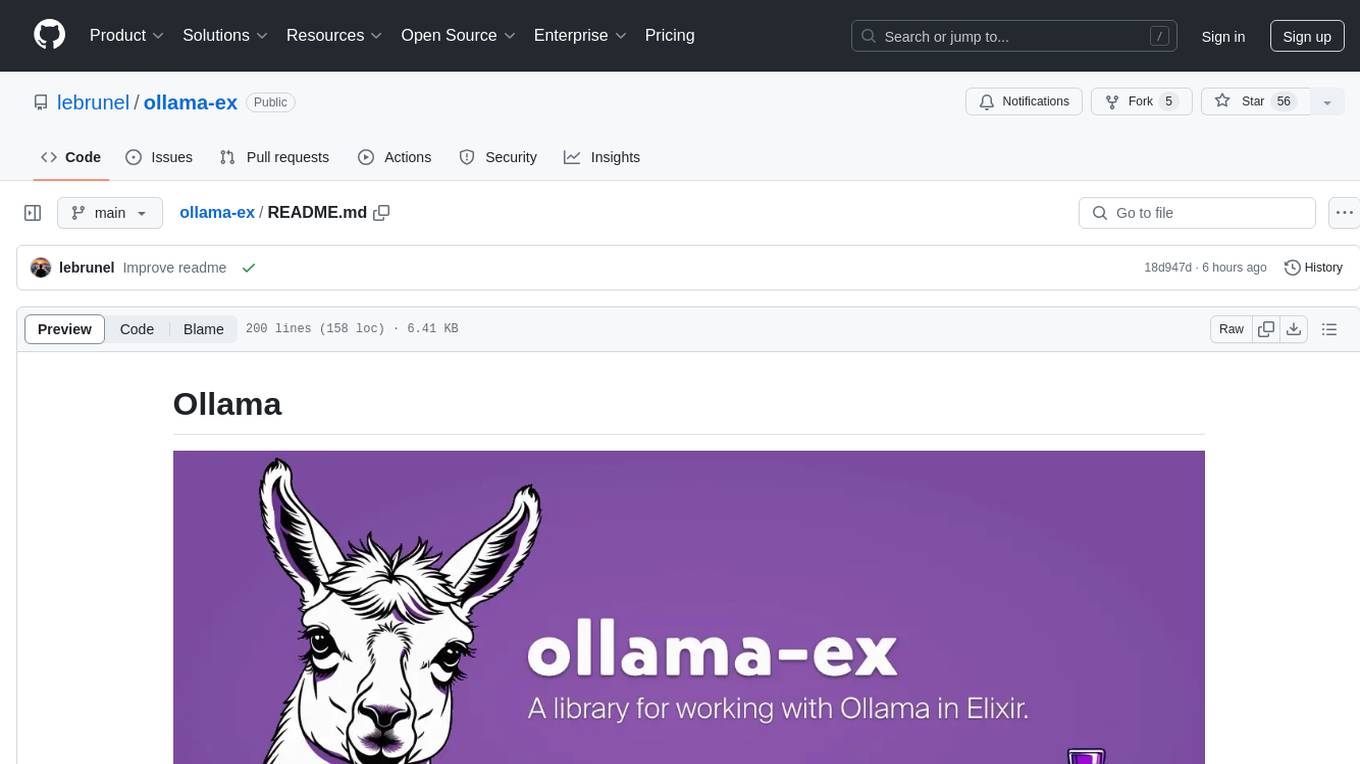
ollama-ex
Ollama is a powerful tool for running large language models locally or on your own infrastructure. It provides a full implementation of the Ollama API, support for streaming requests, and tool use capability. Users can interact with Ollama in Elixir to generate completions, chat messages, and perform streaming requests. The tool also supports function calling on compatible models, allowing users to define tools with clear descriptions and arguments. Ollama is designed to facilitate natural language processing tasks and enhance user interactions with language models.
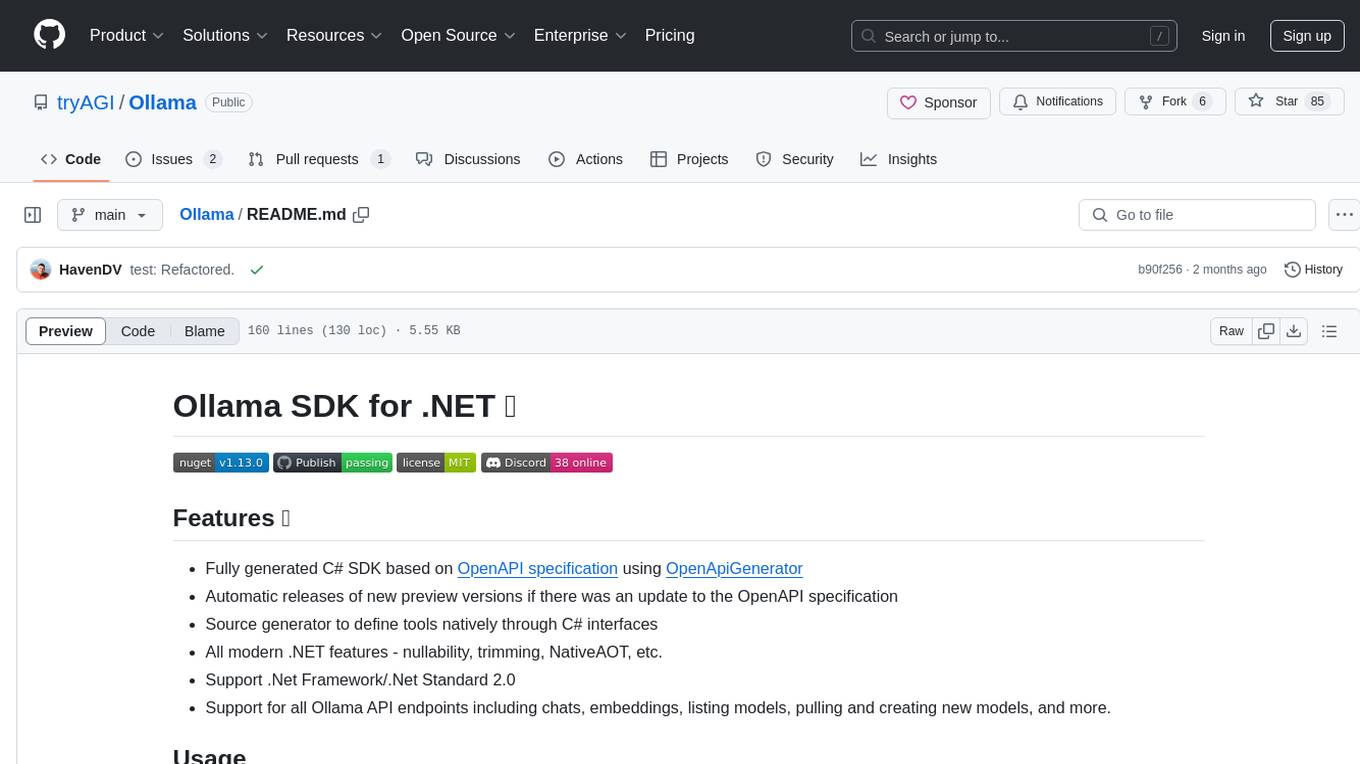
Ollama
Ollama SDK for .NET is a fully generated C# SDK based on OpenAPI specification using OpenApiGenerator. It supports automatic releases of new preview versions, source generator for defining tools natively through C# interfaces, and all modern .NET features. The SDK provides support for all Ollama API endpoints including chats, embeddings, listing models, pulling and creating new models, and more. It also offers tools for interacting with weather data and providing weather-related information to users.
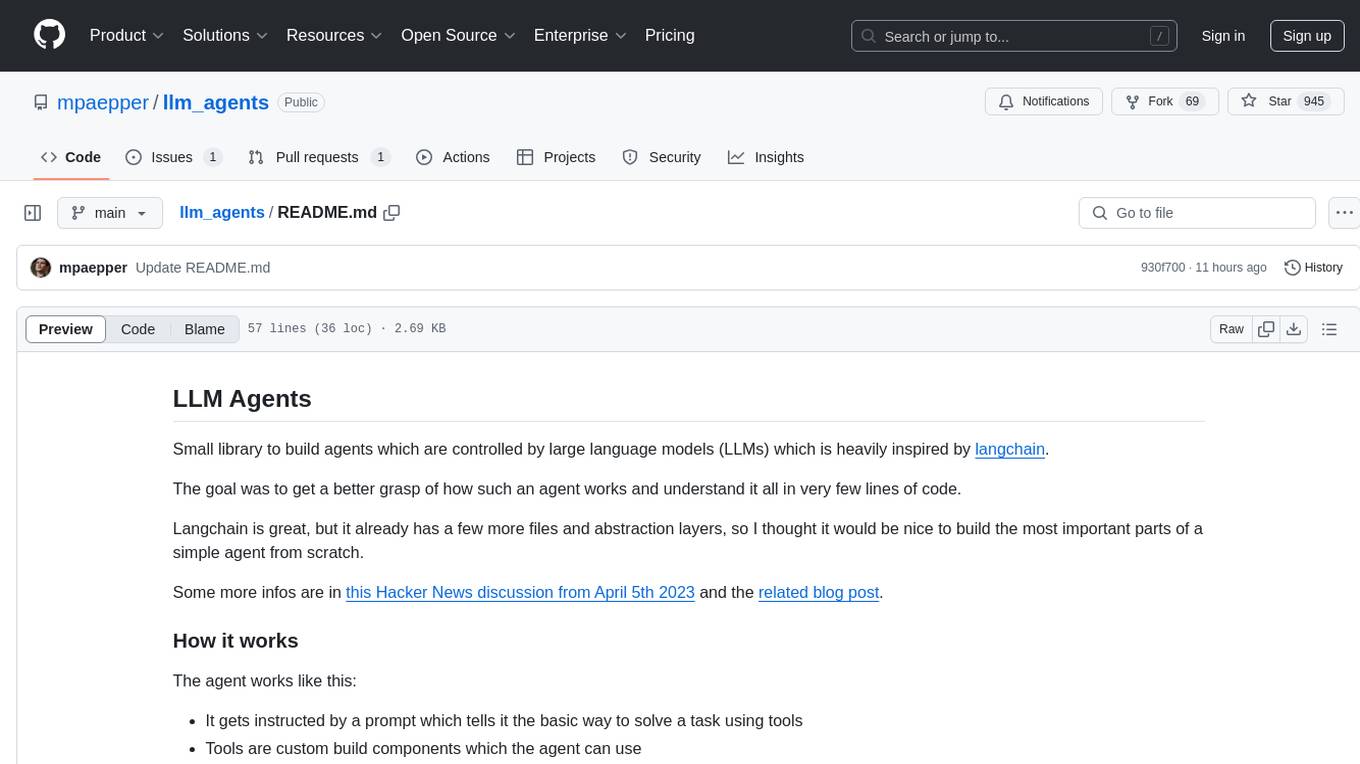
llm_agents
LLM Agents is a small library designed to build agents controlled by large language models. It aims to provide a better understanding of how such agents work in a concise manner. The library allows agents to be instructed by prompts, use custom-built components as tools, and run in a loop of Thought, Action, Observation. The agents leverage language models to generate Thought and Action, while tools like Python REPL, Google search, and Hacker News search provide Observations. The library requires setting up environment variables for OpenAI API and SERPAPI API keys. Users can create their own agents by importing the library and defining tools accordingly.
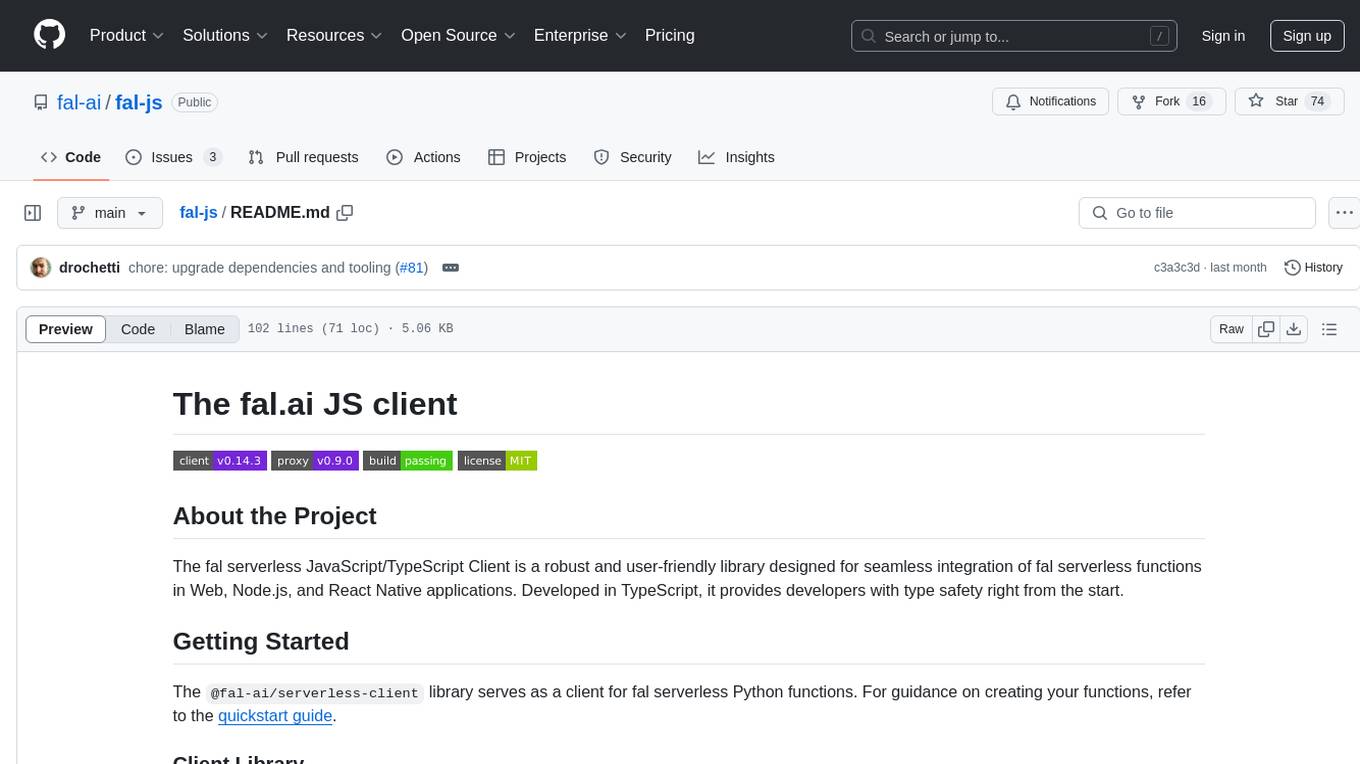
fal-js
The fal.ai JS client is a robust and user-friendly library for seamless integration of fal serverless functions in Web, Node.js, and React Native applications. Developed in TypeScript, it provides developers with type safety right from the start. The client library is crafted as a lightweight layer atop platform standards like `fetch`, ensuring hassle-free integration into existing codebases and flawless operation across various JavaScript runtimes. The client proxy feature allows secure handling of credentials by using a server proxy for serverless APIs. The repository also includes example Next.js applications for demonstration and integration.
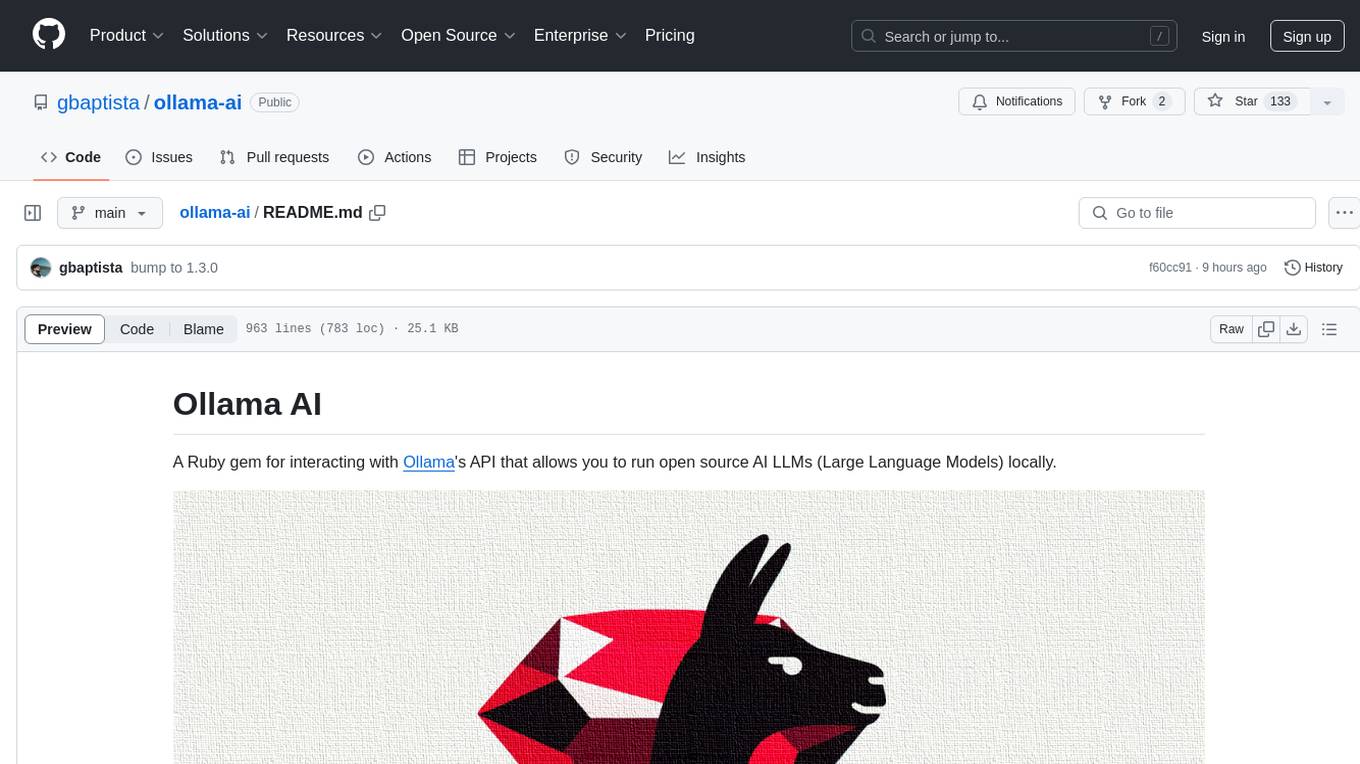
ollama-ai
Ollama AI is a Ruby gem designed to interact with Ollama's API, allowing users to run open source AI LLMs (Large Language Models) locally. The gem provides low-level access to Ollama, enabling users to build abstractions on top of it. It offers methods for generating completions, chat interactions, embeddings, creating and managing models, and more. Users can also work with text and image data, utilize Server-Sent Events for streaming capabilities, and handle errors effectively. Ollama AI is not an official Ollama project and is distributed under the MIT License.
For similar jobs
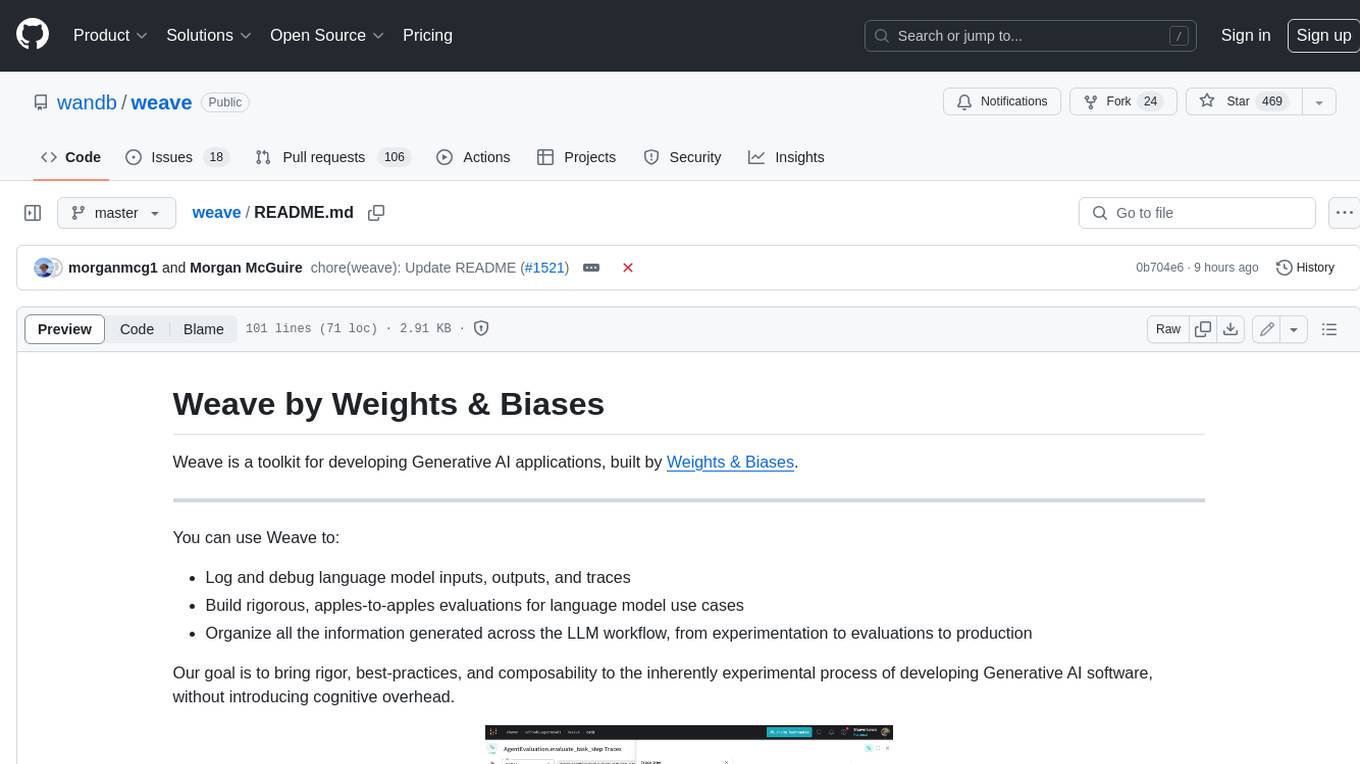
weave
Weave is a toolkit for developing Generative AI applications, built by Weights & Biases. With Weave, you can log and debug language model inputs, outputs, and traces; build rigorous, apples-to-apples evaluations for language model use cases; and organize all the information generated across the LLM workflow, from experimentation to evaluations to production. Weave aims to bring rigor, best-practices, and composability to the inherently experimental process of developing Generative AI software, without introducing cognitive overhead.
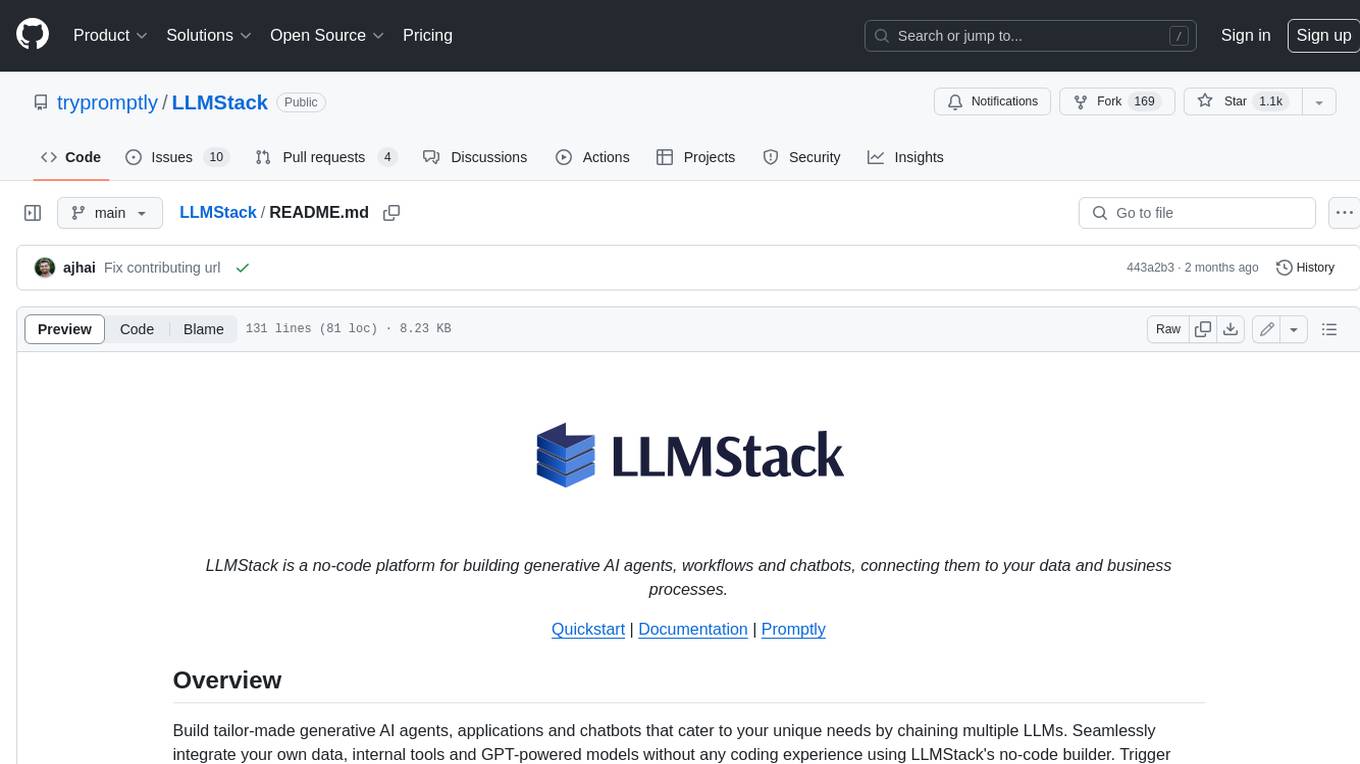
LLMStack
LLMStack is a no-code platform for building generative AI agents, workflows, and chatbots. It allows users to connect their own data, internal tools, and GPT-powered models without any coding experience. LLMStack can be deployed to the cloud or on-premise and can be accessed via HTTP API or triggered from Slack or Discord.
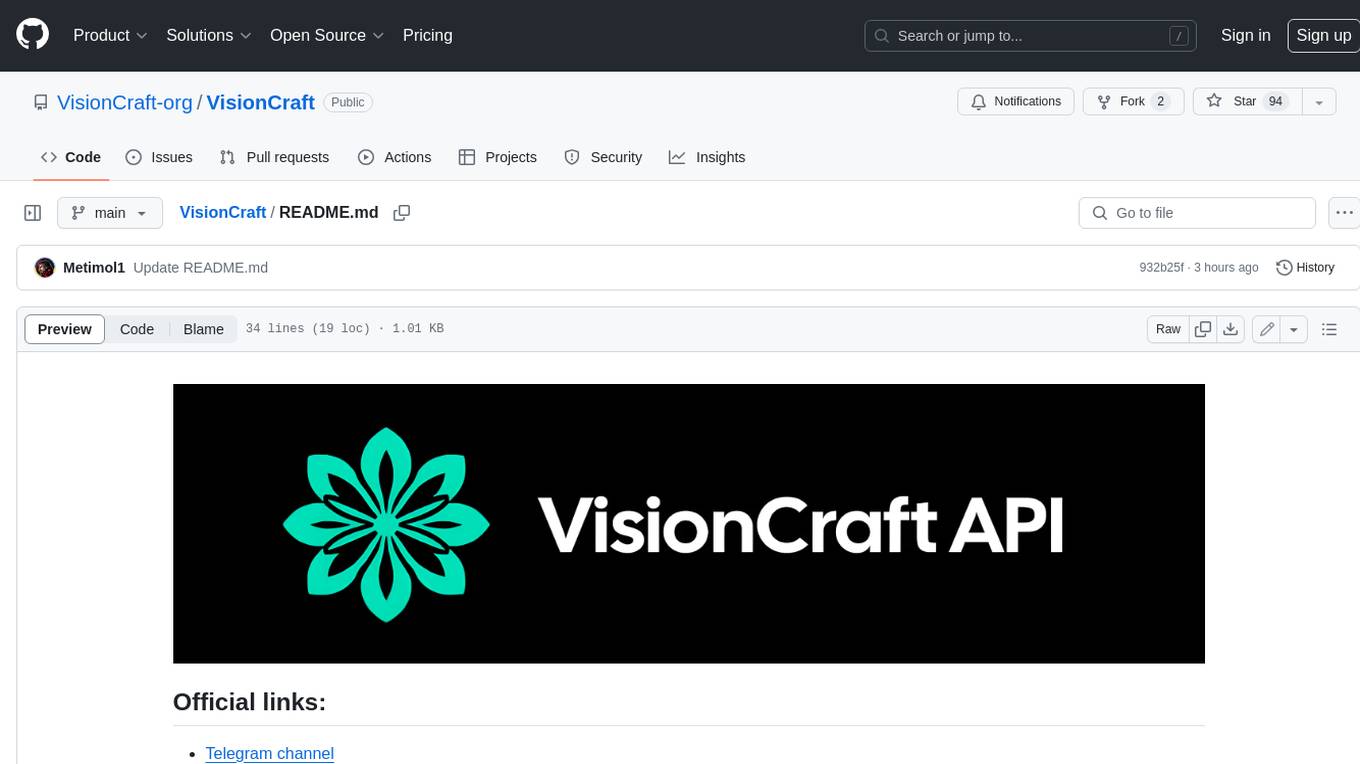
VisionCraft
The VisionCraft API is a free API for using over 100 different AI models. From images to sound.
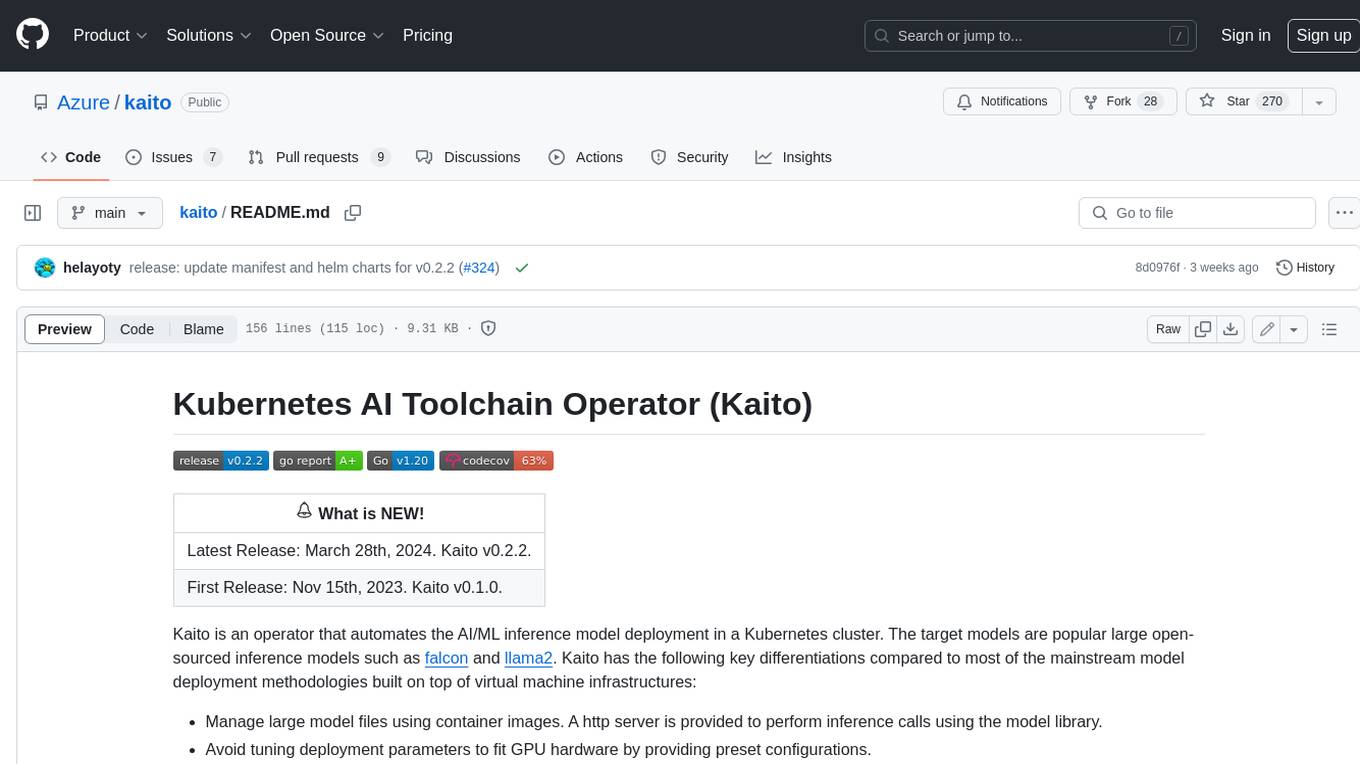
kaito
Kaito is an operator that automates the AI/ML inference model deployment in a Kubernetes cluster. It manages large model files using container images, avoids tuning deployment parameters to fit GPU hardware by providing preset configurations, auto-provisions GPU nodes based on model requirements, and hosts large model images in the public Microsoft Container Registry (MCR) if the license allows. Using Kaito, the workflow of onboarding large AI inference models in Kubernetes is largely simplified.
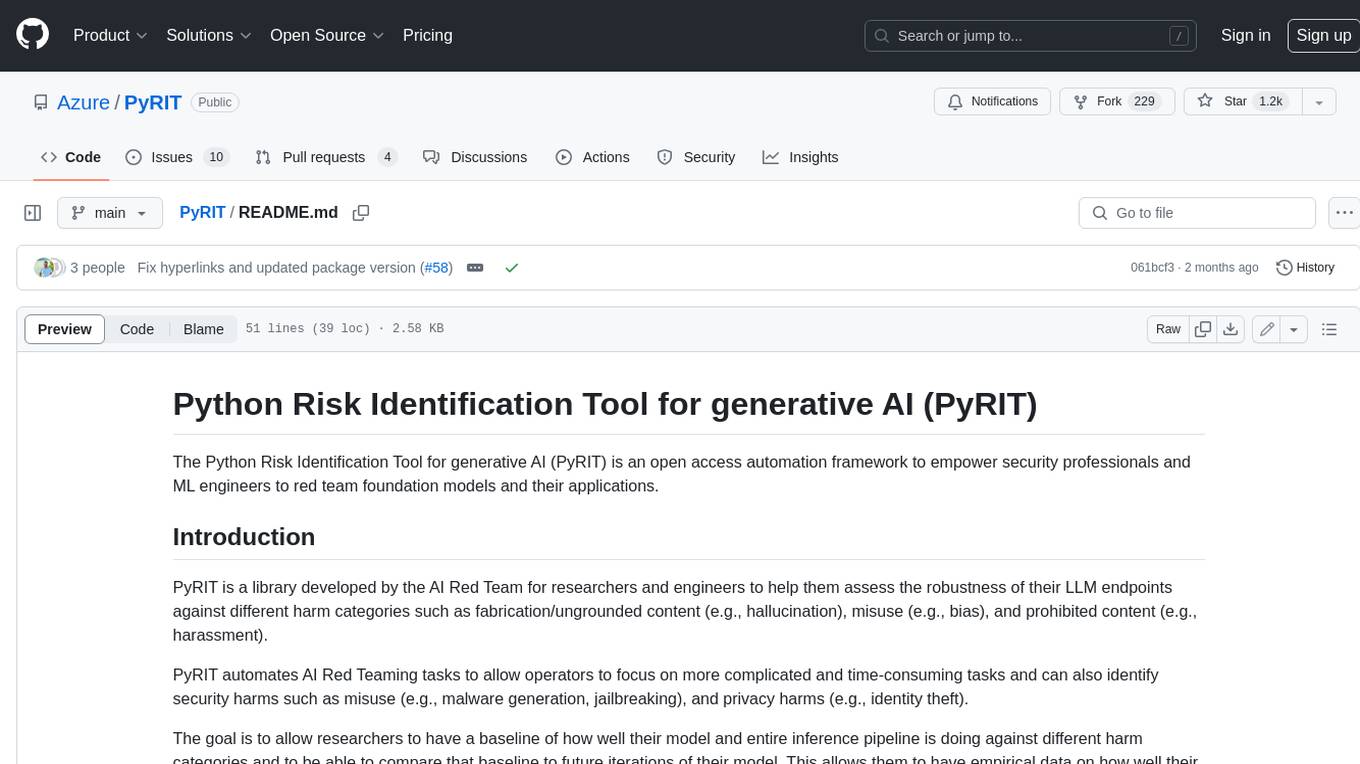
PyRIT
PyRIT is an open access automation framework designed to empower security professionals and ML engineers to red team foundation models and their applications. It automates AI Red Teaming tasks to allow operators to focus on more complicated and time-consuming tasks and can also identify security harms such as misuse (e.g., malware generation, jailbreaking), and privacy harms (e.g., identity theft). The goal is to allow researchers to have a baseline of how well their model and entire inference pipeline is doing against different harm categories and to be able to compare that baseline to future iterations of their model. This allows them to have empirical data on how well their model is doing today, and detect any degradation of performance based on future improvements.
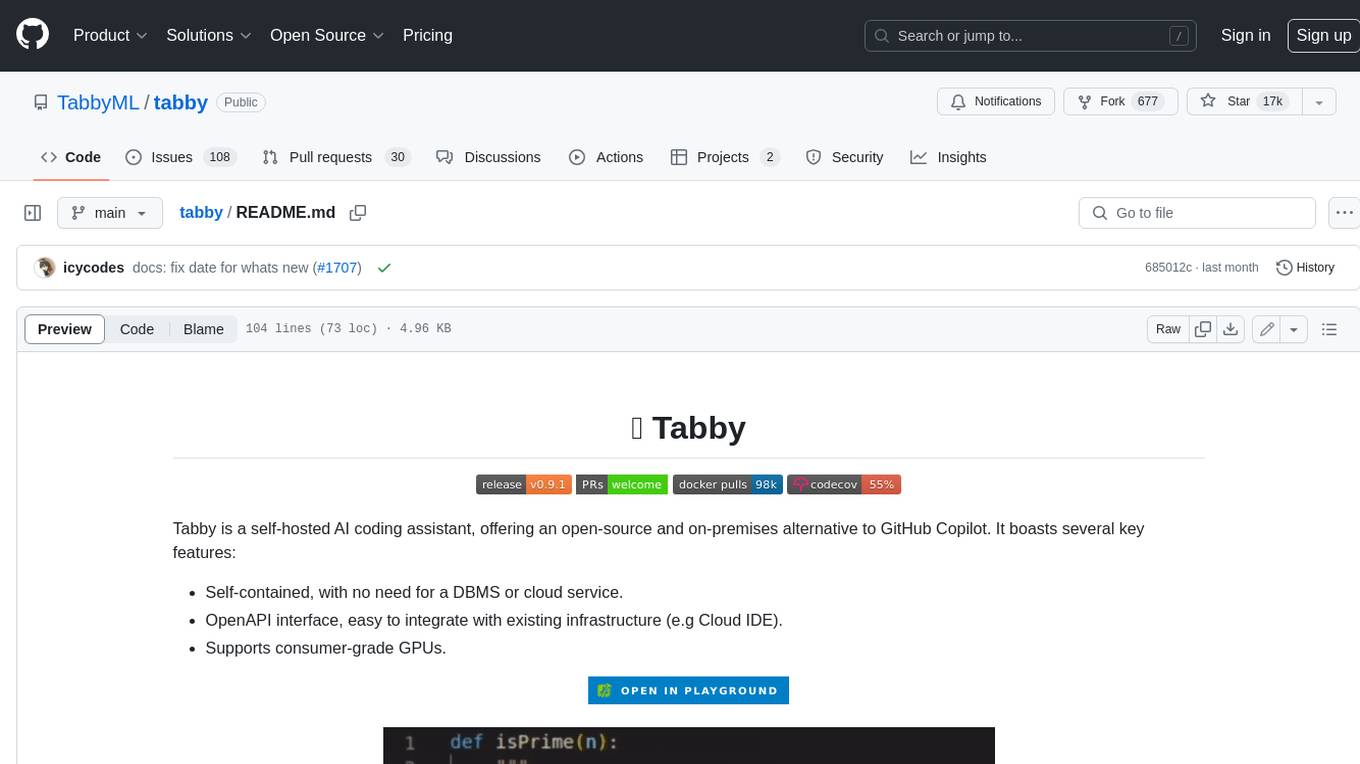
tabby
Tabby is a self-hosted AI coding assistant, offering an open-source and on-premises alternative to GitHub Copilot. It boasts several key features: * Self-contained, with no need for a DBMS or cloud service. * OpenAPI interface, easy to integrate with existing infrastructure (e.g Cloud IDE). * Supports consumer-grade GPUs.
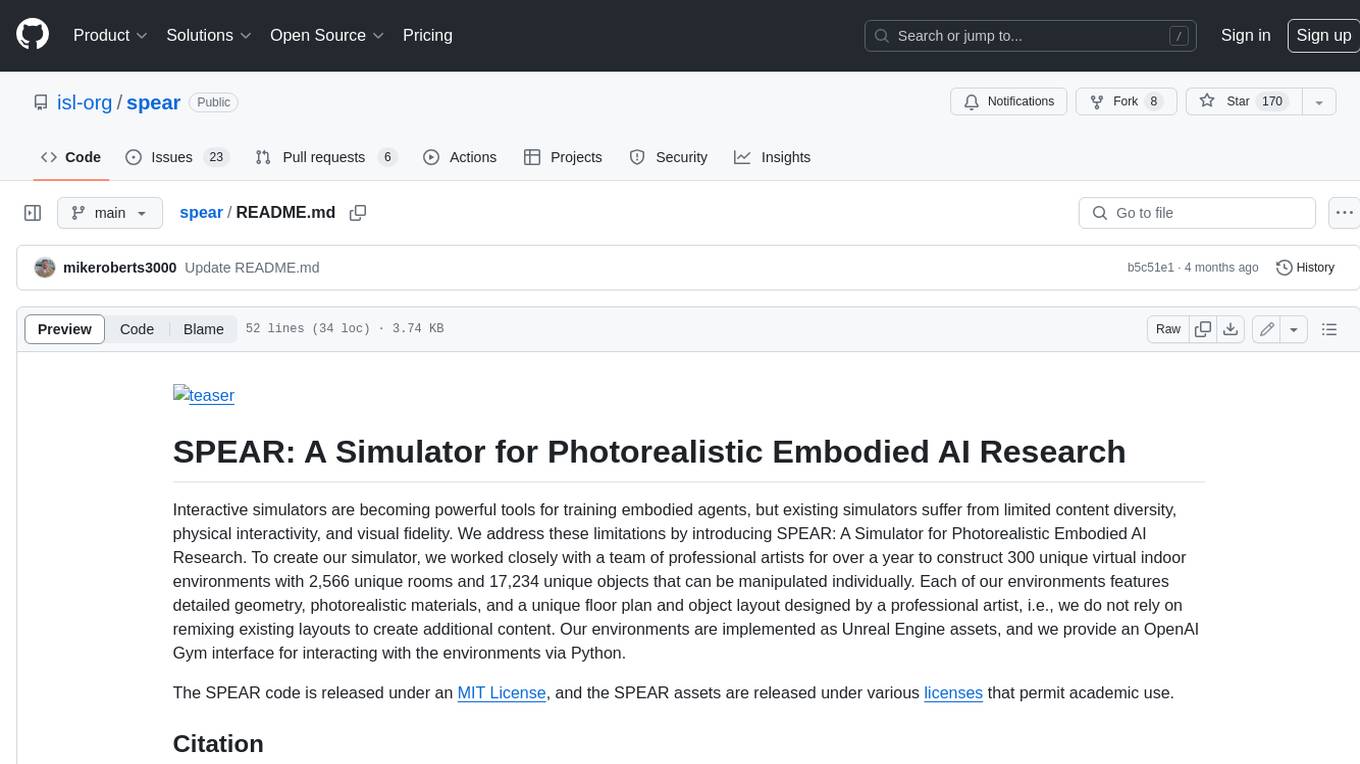
spear
SPEAR (Simulator for Photorealistic Embodied AI Research) is a powerful tool for training embodied agents. It features 300 unique virtual indoor environments with 2,566 unique rooms and 17,234 unique objects that can be manipulated individually. Each environment is designed by a professional artist and features detailed geometry, photorealistic materials, and a unique floor plan and object layout. SPEAR is implemented as Unreal Engine assets and provides an OpenAI Gym interface for interacting with the environments via Python.
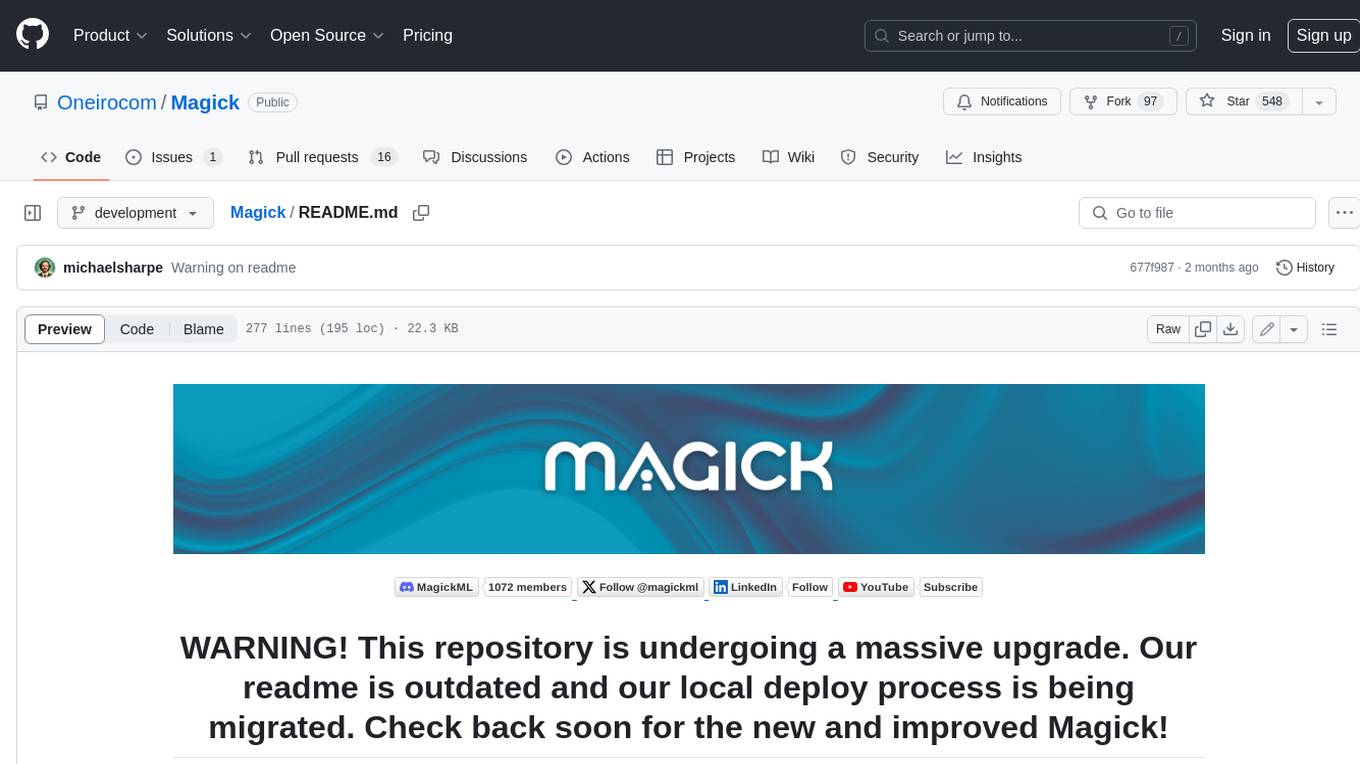
Magick
Magick is a groundbreaking visual AIDE (Artificial Intelligence Development Environment) for no-code data pipelines and multimodal agents. Magick can connect to other services and comes with nodes and templates well-suited for intelligent agents, chatbots, complex reasoning systems and realistic characters.