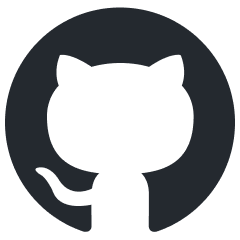
ling
The LLMs' framework optimized for ultra-fast response times.
Stars: 64
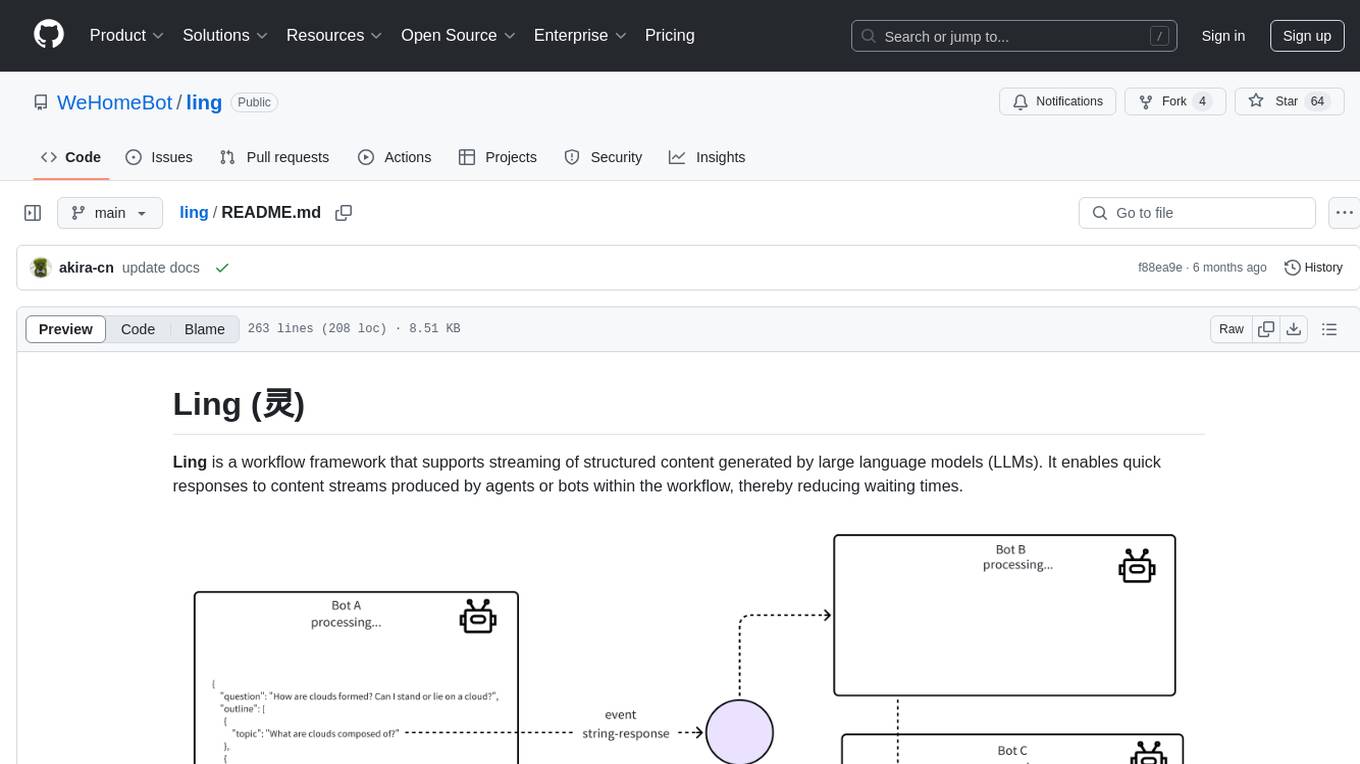
README:
Ling is a workflow framework that supports streaming of structured content generated by large language models (LLMs). It enables quick responses to content streams produced by agents or bots within the workflow, thereby reducing waiting times.
- [x] Supports data stream output via JSONL protocol.
- [x] Automatic correction of token errors in JSON output.
- [x] Supports complex asynchronous workflows.
- [x] Supports status messages during streaming output.
- [x] Supports Server-Sent Events.
- [ ] Provides Client SDK.
Complex AI workflows, such as those found in Bearbobo Learning Companion, require multiple agents/bots to process structured data collaboratively. However, considering real-time responses, utilizing structured data outputs is not conducive to enhancing timeliness through a streaming interface.
The commonly used JSON data format, although flexible, has structural integrity, meaning it is difficult to parse correctly until all the content is completely outputted. Of course, other structured data formats like YAML can be adopted, but they are not as powerful and convenient as JSON. Ling is a streaming framework created to address this issue. Its core is a real-time converter that can parse incoming JSON data streams character by character, outputting content in the form of jsonuri.
For example, consider the following JSON format:
{
"outline": [
{
"topic": "What are clouds made of?"
},
{
"topic": "Why do clouds look soft?"
}
]
// ...
}
During streaming input, the content may be converted in real-time into the following data outputs (using Server-sent Events):
data: {"uri": "outline/0/topic", "delta": "clo"}
data: {"uri": "outline/0/topic", "delta": "uds"}
data: {"uri": "outline/0/topic", "delta": "are"}
data: {"uri": "outline/0/topic", "delta": "mad"}
data: {"uri": "outline/0/topic", "delta": "e"}
data: {"uri": "outline/0/topic", "delta": "of"}
data: {"uri": "outline/0/topic", "delta": "?"}
data: {"uri": "outline/1/topic", "delta": "Why"}
data: {"uri": "outline/1/topic", "delta": "do"}
data: {"uri": "outline/1/topic", "delta": "clo"}
data: {"uri": "outline/1/topic", "delta": "uds"}
data: {"uri": "outline/1/topic", "delta": "loo"}
data: {"uri": "outline/1/topic", "delta": "k"}
data: {"uri": "outline/1/topic", "delta": "sof"}
data: {"uri": "outline/1/topic", "delta": "t"}
data: {"uri": "outline/1/topic", "delta": "?"}
...
This method of real-time data transmission facilitates immediate front-end processing.
Server
import 'dotenv/config';
import express from 'express';
import bodyParser from 'body-parser';
import cors from 'cors';
import { Ling } from "@bearbobo/ling";
import type { ChatConfig } from "@bearbobo/ling/types";
import { pipeline } from 'node:stream/promises';
const apiKey = process.env.API_KEY as string;
const model_name = process.env.MODEL_NAME as string;
const endpoint = process.env.ENDPOINT as string;
const app = express();
app.use(cors());
app.use(bodyParser.json());
const port = 3000;
app.get('/', (req, res) => {
res.send('Hello World!');
});
app.post('/api', async (req, res) => {
const question = req.body.question;
const config: ChatConfig = {
model_name,
api_key: apiKey,
endpoint: endpoint,
};
// ------- The work flow start --------
const ling = new Ling(config);
const bot = ling.createBot(/*'bearbobo'*/);
bot.addPrompt('Respond to me in JSON format, starting with {.\n[Example]\n{"answer": "My response"}');
bot.chat(question);
bot.on('string-response', ({uri, delta}) => {
// Infer the content of the string in the JSON, and send the content of the 'answer' field to the second bot.
console.log('bot string-response', uri, delta);
const bot2 = ling.createBot(/*'bearbobo'*/);
bot2.addPrompt(`Expand the content I gave you into more detailed content, answer me in JSON format, place the detailed answer text in the 'details' field, and place 2-3 related knowledge points in the 'related_question' field.\n[Example]\n{"details": "My detailed answer", "related_question": [...]}`);
bot2.chat(delta);
bot2.on('response', (content) => {
// Stream data push completed.
console.log('bot2 response finished', content);
});
const bot3 = ling.createBot();
bot3.addPrompt('Expand the content I gave you into more detailed content, using Chinese. answer me in JSON format, place the detailed answer in Chinese in the 'details' field.\n[Example]\n{"details_cn": "my answer..."}');
bot3.chat(delta);
bot3.on('response', (content) => {
// Stream data push completed.
console.log('bot3 response finished', content);
});
});
ling.close(); // It can be directly closed, and when closing, it checks whether the status of all bots has been finished.
// ------- The work flow end --------
// setting below headers for Streaming the data
res.writeHead(200, {
'Content-Type': "text/event-stream",
'Cache-Control': "no-cache",
'Connection': "keep-alive"
});
console.log(ling.stream);
pipeline((ling.stream as any), res);
});
app.listen(port, () => {
console.log(`Example app listening at http://localhost:${port}`);
});
Client
<script setup>
import { onMounted, ref } from 'vue';
import { set, get } from 'jsonuri';
const response = ref({
answer: 'Brief:',
details: 'Details:',
details_eng: 'Translation:',
related_question: [
'?',
'?',
'?'
],
});
onMounted(async () => {
const res = await fetch('http://localhost:3000/api', {
method: 'POST',
headers: {
'Content-Type': 'application/json'
},
body: JSON.stringify({
question: 'Can I laid on the cloud?'
}),
});
const reader = res.body.getReader();
const decoder = new TextDecoder();
let done = false;
const data = {
answer: 'Brief:',
details: 'Details:',
related_question: [],
};
while (!done) {
const { value, done: doneReading } = await reader.read();
done = doneReading;
if(!done) {
const content = decoder.decode(value);
const lines = content.trim().split('\n');
for(const line of lines) {
const input = JSON.parse(line);
if(input.uri) {
const content = get(data, input.uri);
set(data, input.uri, (content || '') + input.delta);
response.value = {...data};
}
}
}
}
});
</script>
<template>
<h1>Hello~</h1>
<p>{{ response.answer }}</p>
<p>{{ response.details }}</p>
<p>{{ response.details_eng }}</p>
<p v-for="item in response.related_question" :key="item.id"> >>> {{ item }}</p>
</template>
This event is triggered when a string field in the JSON output by the AI is completed, returning a jsonuri object.
This event is triggered when the AI has completed its current inference, returning the complete output content. At this point, streaming output may not have ended, and data continues to be sent to the front end.
This event is triggered when all data generated by the AI during this session has been sent to the front end.
Note: Typically, the
string-response
event occurs beforeinference-done
, which in turn occurs beforeresponse
.
Sometimes, we might want to send custom events to the front end to update its status. On the server, you can use ling.sendEvent({event, data})
to push messages to the front end. The front end can then receive and process JSON objects {event, data}
from the stream.
bot.on('inference-done', () => {
bot.sendEvent({event: 'inference-done', state: 'Outline generated!'});
});
Alternatively, you can also directly push jsonuri status updates, making it easier for the front end to set directly.
bot.on('inference-done', () => {
bot.sendEvent({uri: 'state/outline', delta: true});
});
You can force ling to response the Server-Sent Events data format by using ling.setSSE(true)
. This allows the front end to handle the data using the EventSource API.
const es = new EventSource('http://localhost:3000/?question=Can I laid on the cloud?');
es.onmessage = (e) => {
console.log(e.data);
}
es.onopen = () => {
console.log('Connecting');
}
es.onerror = (e) => {
console.log(e);
}
For Tasks:
Click tags to check more tools for each tasksFor Jobs:
Alternative AI tools for ling
Similar Open Source Tools
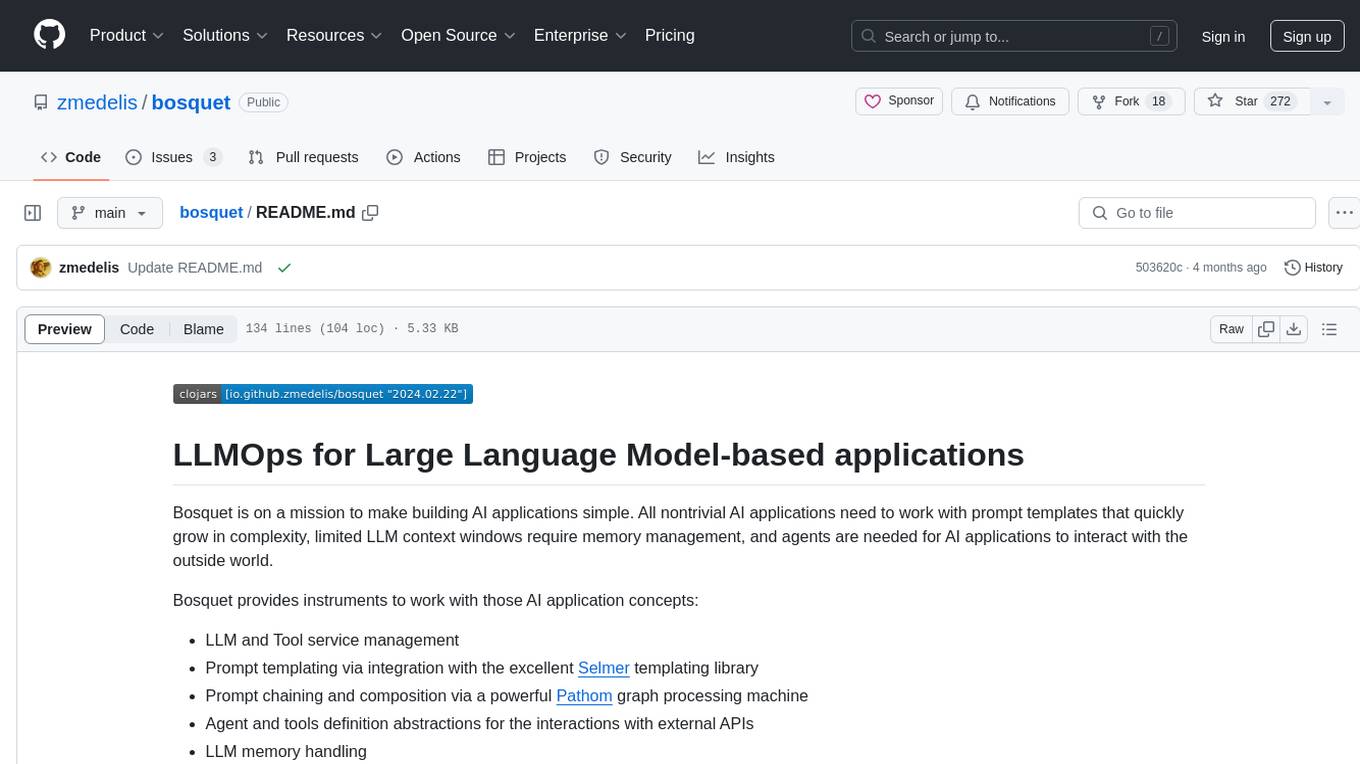
bosquet
Bosquet is a tool designed for LLMOps in large language model-based applications. It simplifies building AI applications by managing LLM and tool services, integrating with Selmer templating library for prompt templating, enabling prompt chaining and composition with Pathom graph processing, defining agents and tools for external API interactions, handling LLM memory, and providing features like call response caching. The tool aims to streamline the development process for AI applications that require complex prompt templates, memory management, and interaction with external systems.
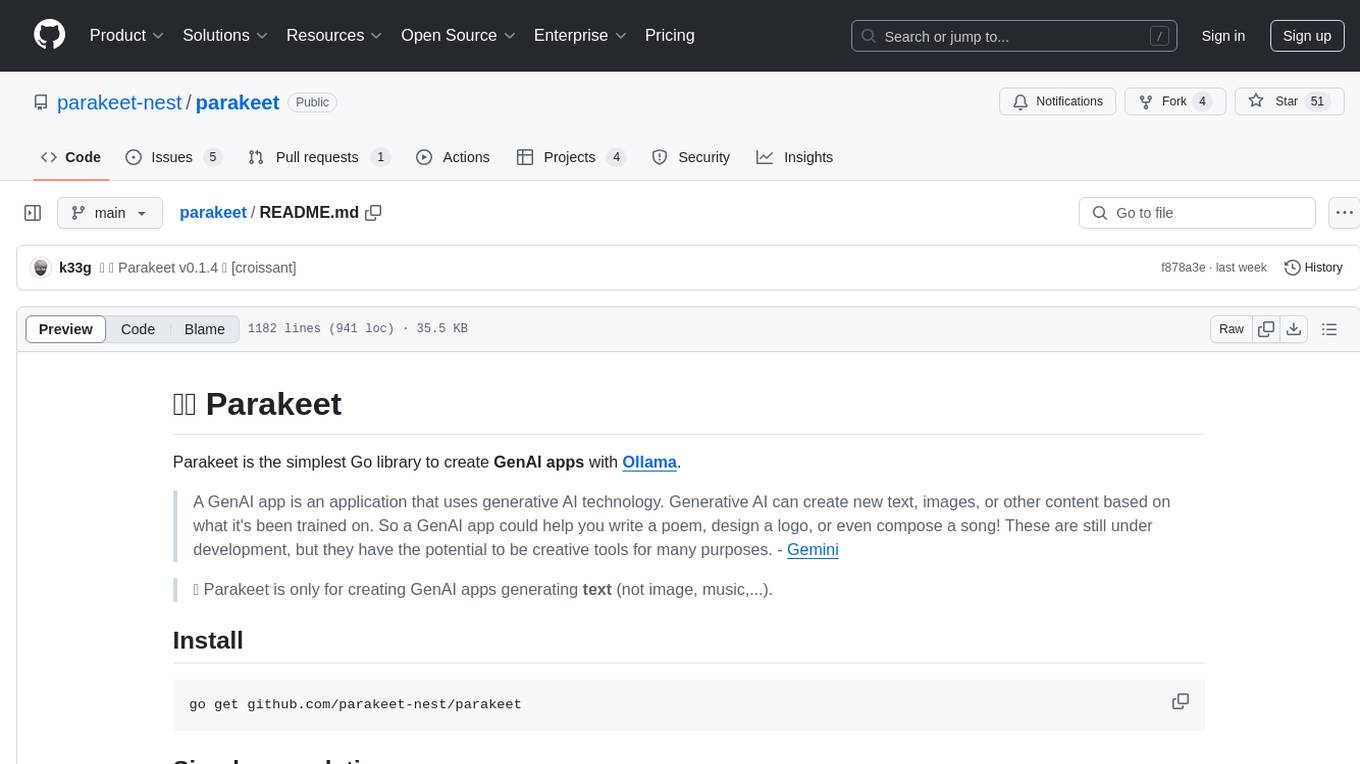
parakeet
Parakeet is a Go library for creating GenAI apps with Ollama. It enables the creation of generative AI applications that can generate text-based content. The library provides tools for simple completion, completion with context, chat completion, and more. It also supports function calling with tools and Wasm plugins. Parakeet allows users to interact with language models and create AI-powered applications easily.
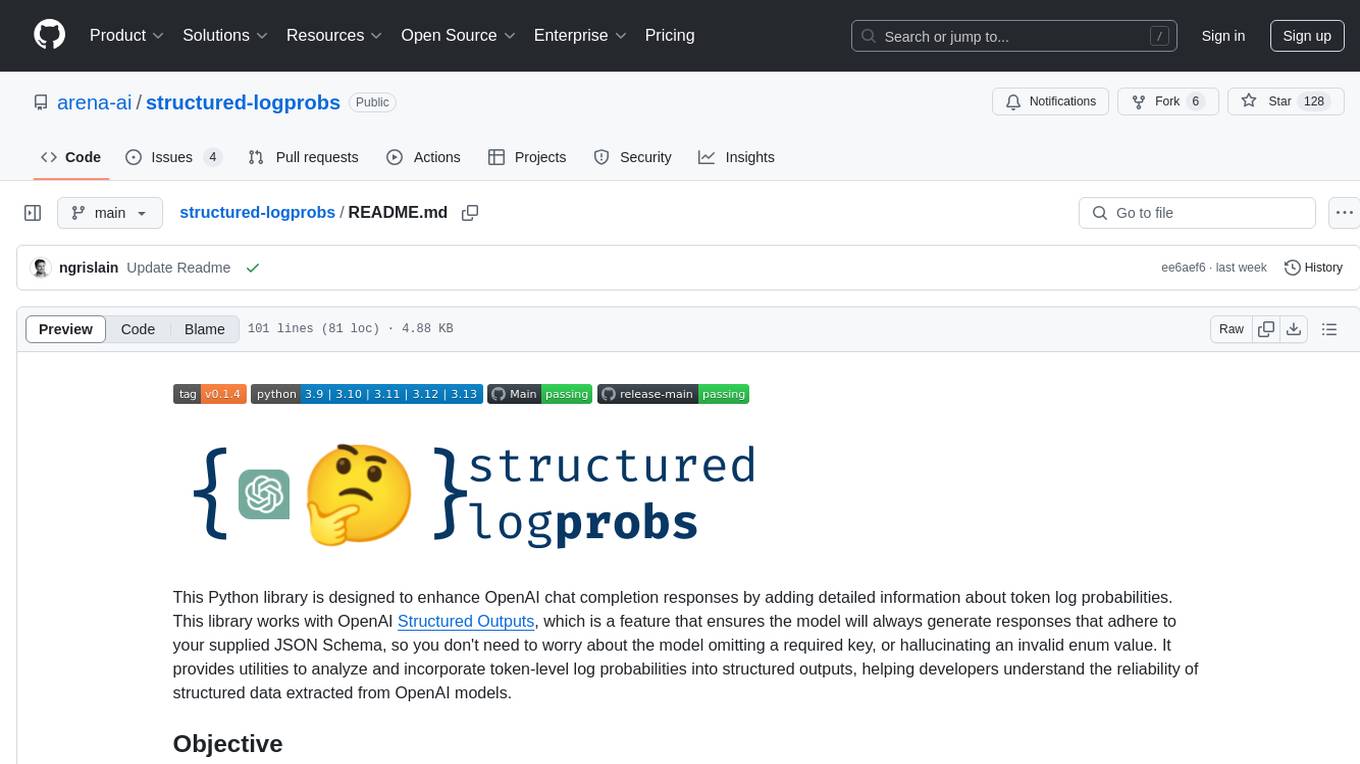
structured-logprobs
This Python library enhances OpenAI chat completion responses by providing detailed information about token log probabilities. It works with OpenAI Structured Outputs to ensure model-generated responses adhere to a JSON Schema. Developers can analyze and incorporate token-level log probabilities to understand the reliability of structured data extracted from OpenAI models.
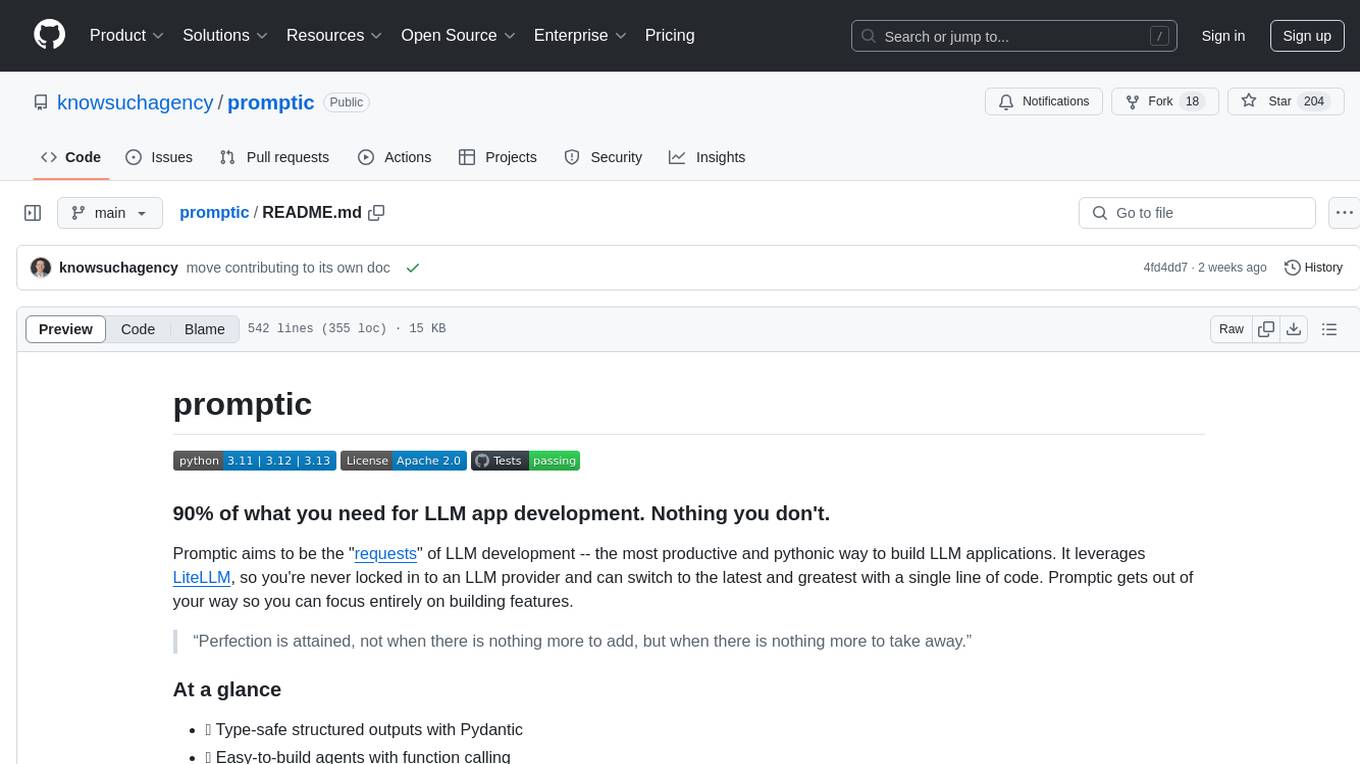
promptic
Promptic is a tool designed for LLM app development, providing a productive and pythonic way to build LLM applications. It leverages LiteLLM, allowing flexibility to switch LLM providers easily. Promptic focuses on building features by providing type-safe structured outputs, easy-to-build agents, streaming support, automatic prompt caching, and built-in conversation memory.
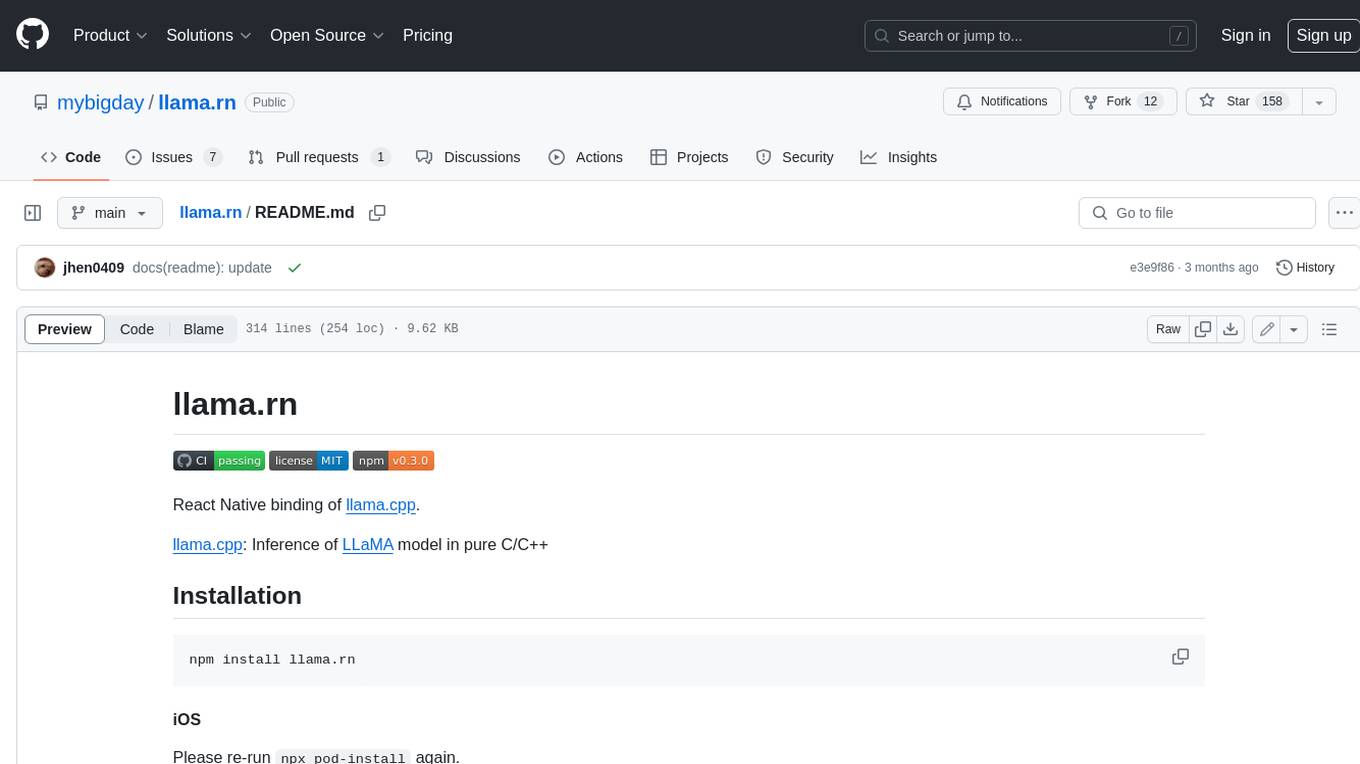
llama.rn
React Native binding of llama.cpp, which is an inference of LLaMA model in pure C/C++. This tool allows you to use the LLaMA model in your React Native applications for various tasks such as text completion, tokenization, detokenization, and embedding. It provides a convenient interface to interact with the LLaMA model and supports features like grammar sampling and mocking for testing purposes.
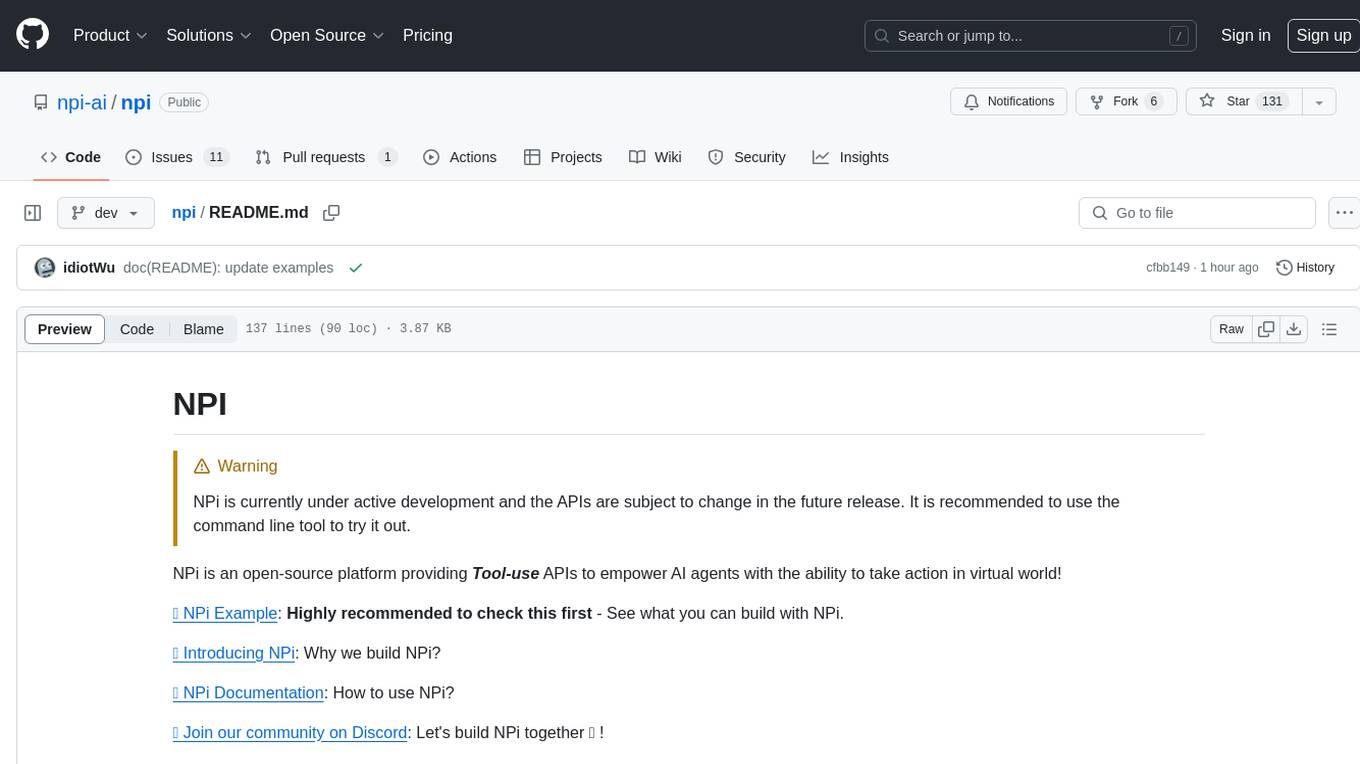
npi
NPi is an open-source platform providing Tool-use APIs to empower AI agents with the ability to take action in the virtual world. It is currently under active development, and the APIs are subject to change in future releases. NPi offers a command line tool for installation and setup, along with a GitHub app for easy access to repositories. The platform also includes a Python SDK and examples like Calendar Negotiator and Twitter Crawler. Join the NPi community on Discord to contribute to the development and explore the roadmap for future enhancements.
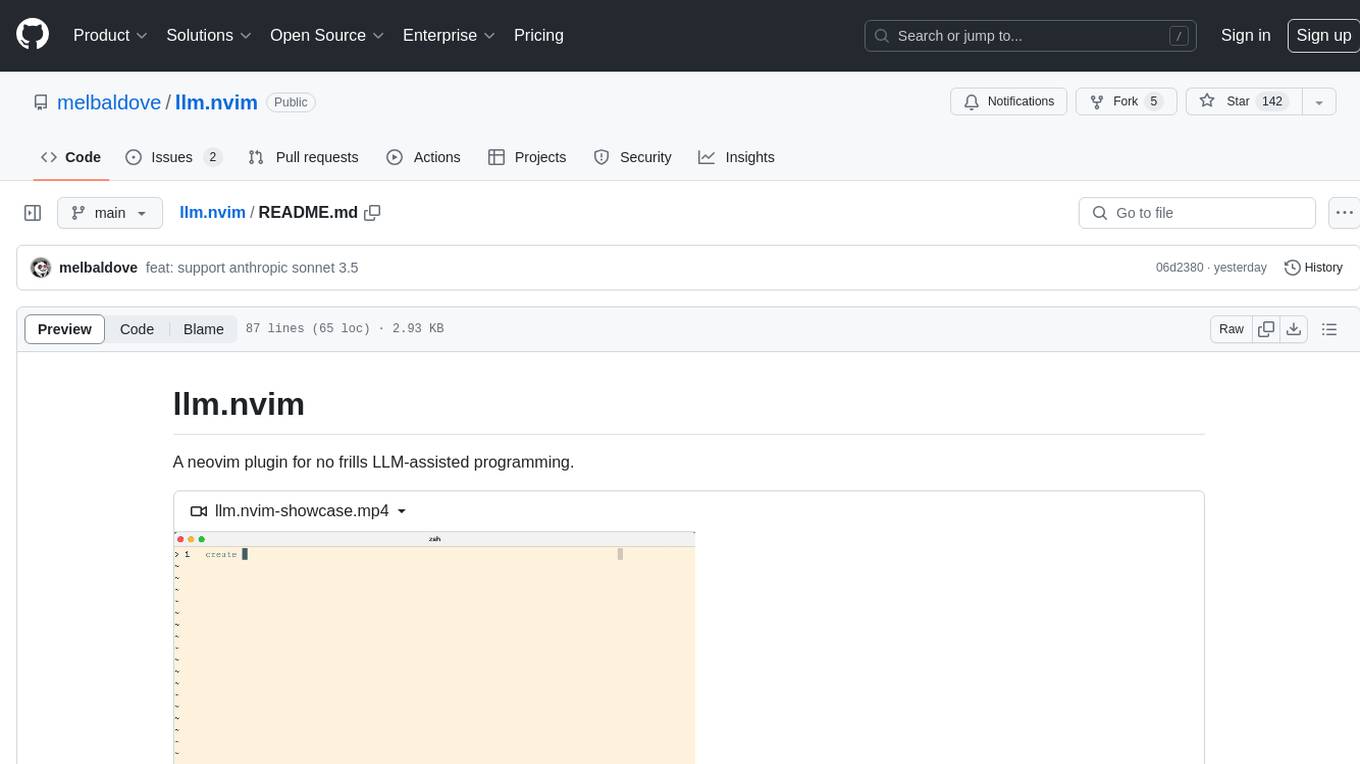
llm.nvim
llm.nvim is a neovim plugin designed for LLM-assisted programming. It provides a no-frills approach to integrating language model assistance into the coding workflow. Users can configure the plugin to interact with various AI services such as GROQ, OpenAI, and Anthropics. The plugin offers functions to trigger the LLM assistant, create new prompt files, and customize key bindings for seamless interaction. With a focus on simplicity and efficiency, llm.nvim aims to enhance the coding experience by leveraging AI capabilities within the neovim environment.
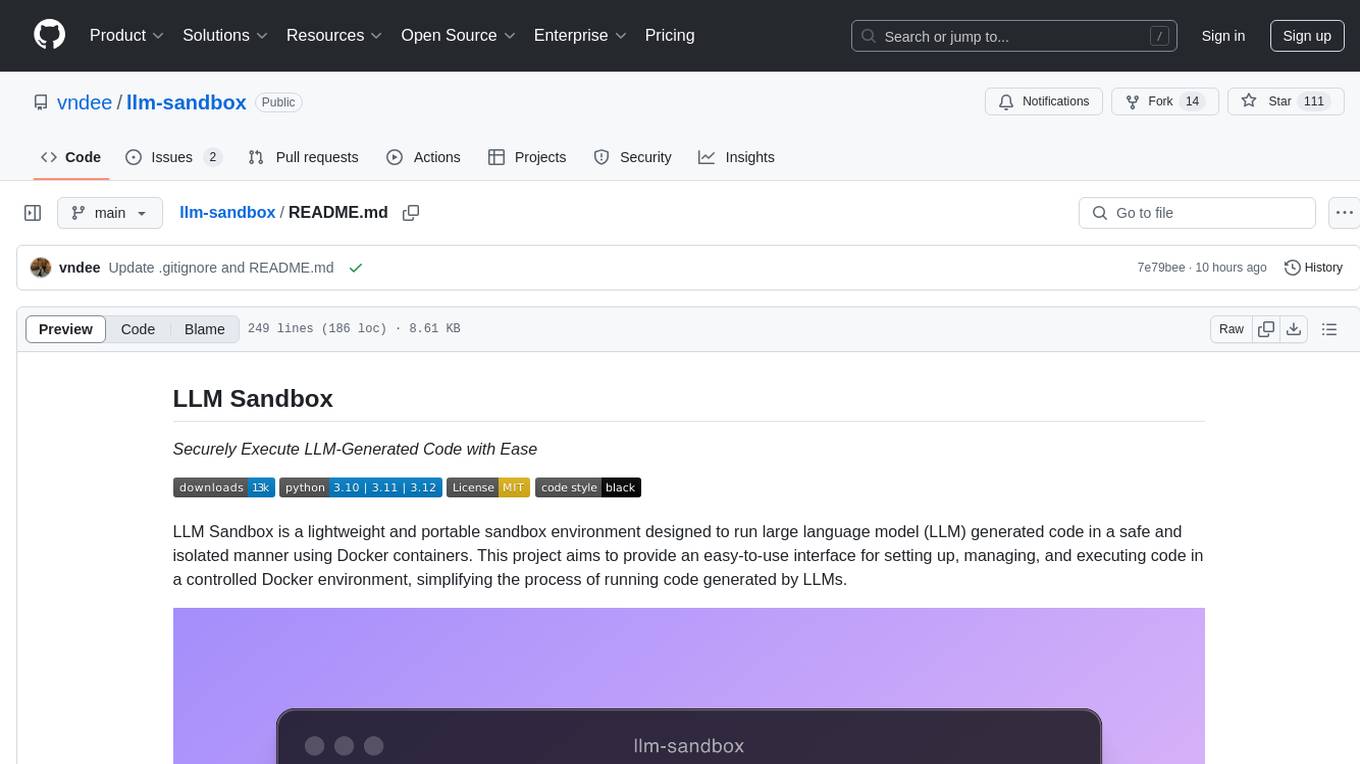
llm-sandbox
LLM Sandbox is a lightweight and portable sandbox environment designed to securely execute large language model (LLM) generated code in a safe and isolated manner using Docker containers. It provides an easy-to-use interface for setting up, managing, and executing code in a controlled Docker environment, simplifying the process of running code generated by LLMs. The tool supports multiple programming languages, offers flexibility with predefined Docker images or custom Dockerfiles, and allows scalability with support for Kubernetes and remote Docker hosts.
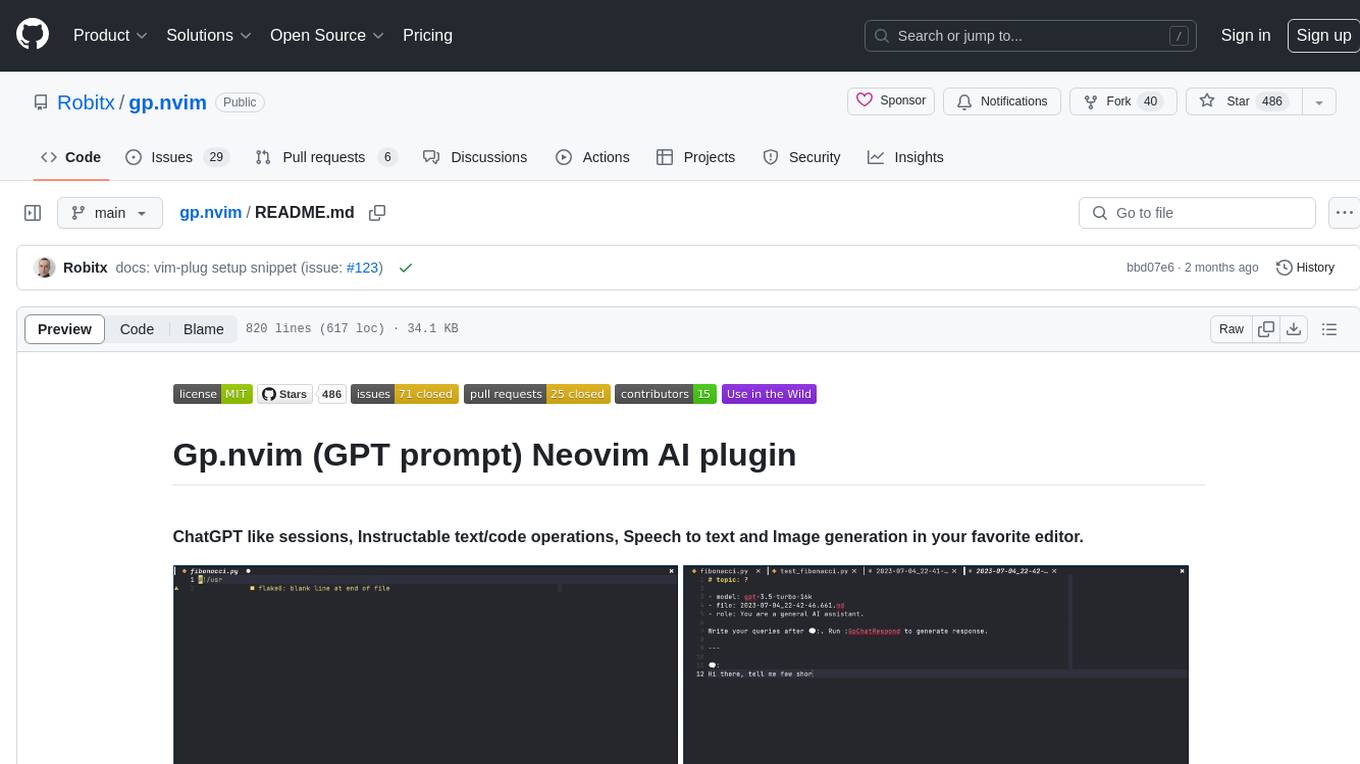
gp.nvim
Gp.nvim (GPT prompt) Neovim AI plugin provides a seamless integration of GPT models into Neovim, offering features like streaming responses, extensibility via hook functions, minimal dependencies, ChatGPT-like sessions, instructable text/code operations, speech-to-text support, and image generation directly within Neovim. The plugin aims to enhance the Neovim experience by leveraging the power of AI models in a user-friendly and native way.
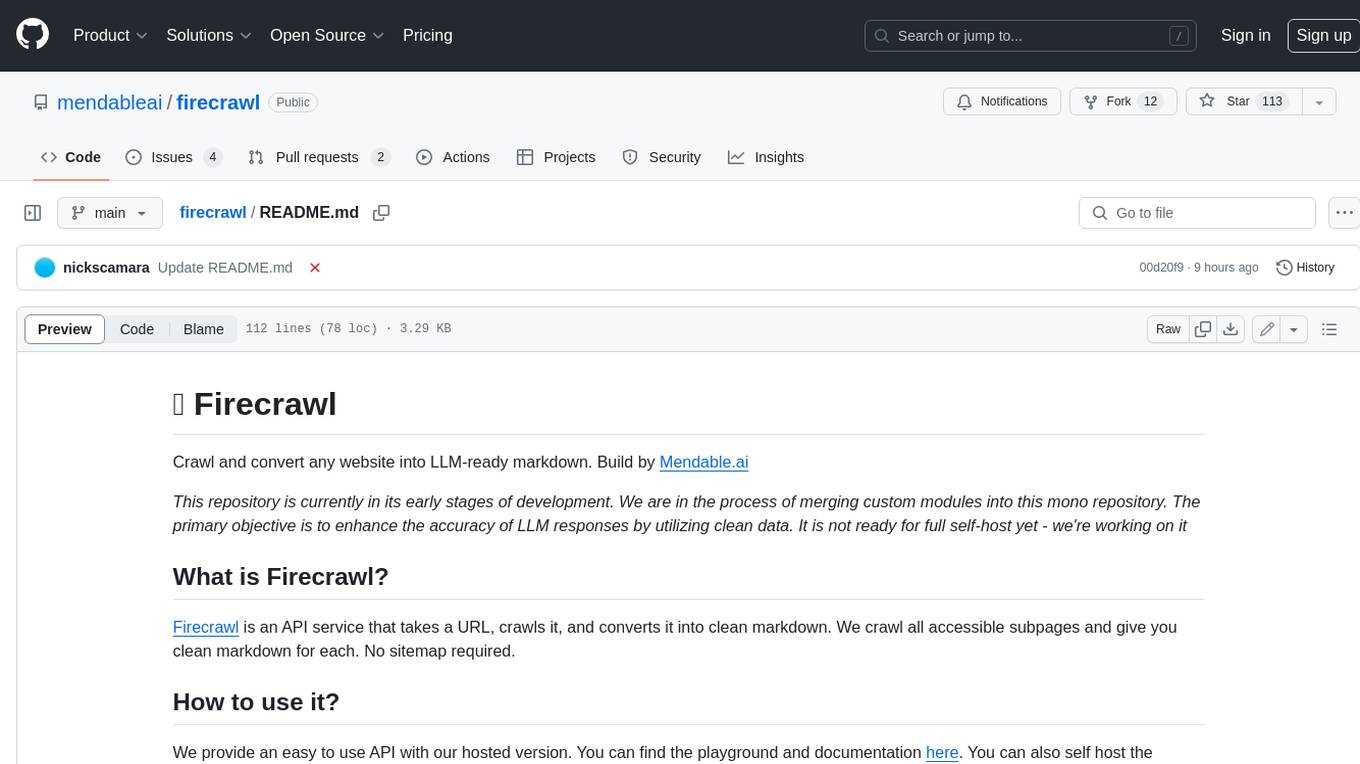
firecrawl
Firecrawl is an API service that takes a URL, crawls it, and converts it into clean markdown. It crawls all accessible subpages and provides clean markdown for each, without requiring a sitemap. The API is easy to use and can be self-hosted. It also integrates with Langchain and Llama Index. The Python SDK makes it easy to crawl and scrape websites in Python code.
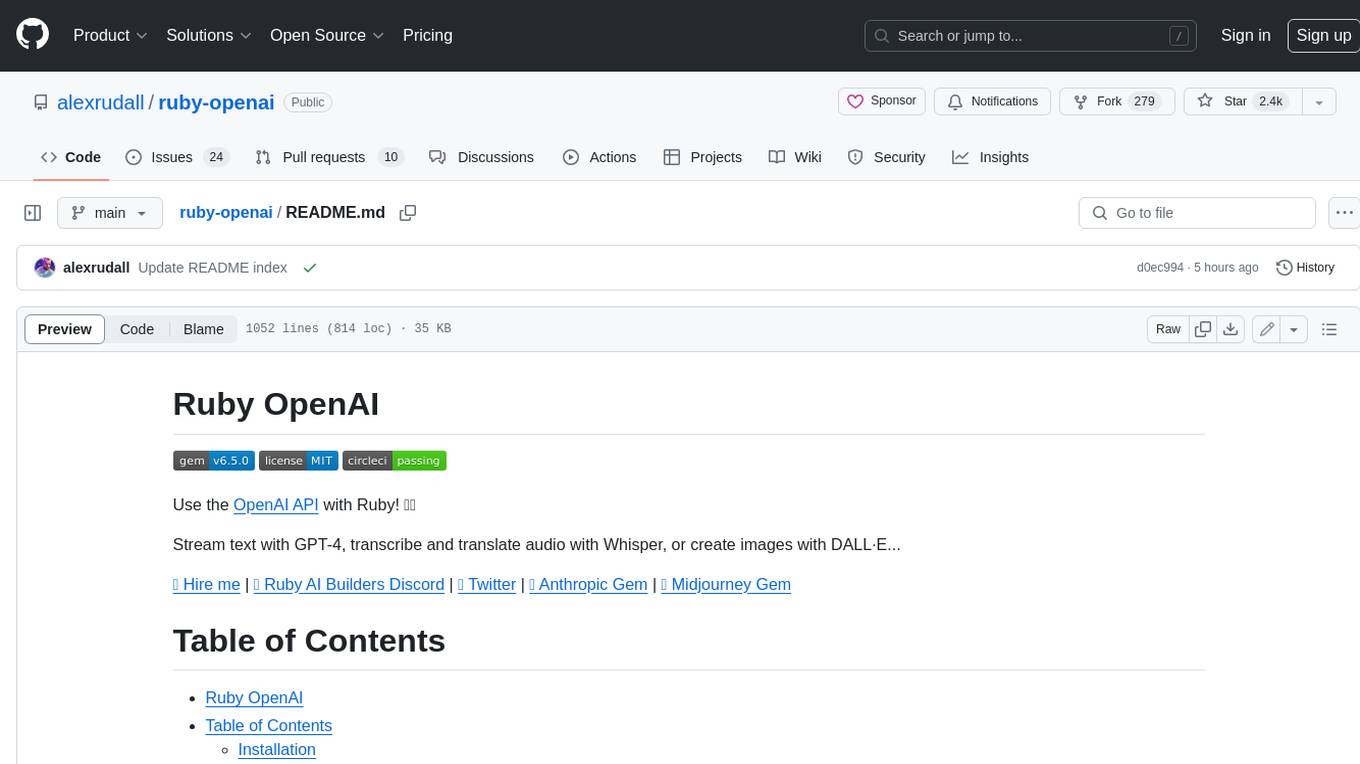
ruby-openai
Use the OpenAI API with Ruby! 🤖🩵 Stream text with GPT-4, transcribe and translate audio with Whisper, or create images with DALL·E... Hire me | 🎮 Ruby AI Builders Discord | 🐦 Twitter | 🧠 Anthropic Gem | 🚂 Midjourney Gem ## Table of Contents * Ruby OpenAI * Table of Contents * Installation * Bundler * Gem install * Usage * Quickstart * With Config * Custom timeout or base URI * Extra Headers per Client * Logging * Errors * Faraday middleware * Azure * Ollama * Counting Tokens * Models * Examples * Chat * Streaming Chat * Vision * JSON Mode * Functions * Edits * Embeddings * Batches * Files * Finetunes * Assistants * Threads and Messages * Runs * Runs involving function tools * Image Generation * DALL·E 2 * DALL·E 3 * Image Edit * Image Variations * Moderations * Whisper * Translate * Transcribe * Speech * Errors * Development * Release * Contributing * License * Code of Conduct
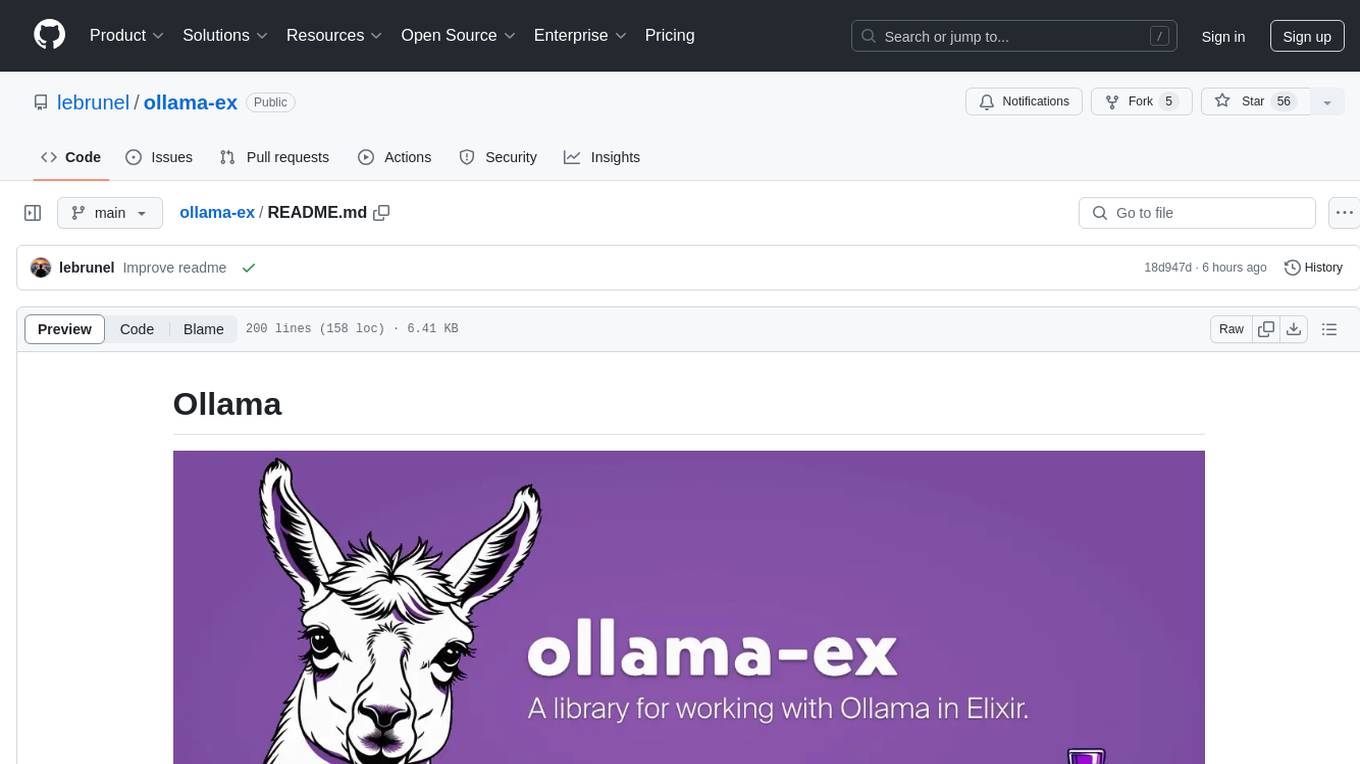
ollama-ex
Ollama is a powerful tool for running large language models locally or on your own infrastructure. It provides a full implementation of the Ollama API, support for streaming requests, and tool use capability. Users can interact with Ollama in Elixir to generate completions, chat messages, and perform streaming requests. The tool also supports function calling on compatible models, allowing users to define tools with clear descriptions and arguments. Ollama is designed to facilitate natural language processing tasks and enhance user interactions with language models.
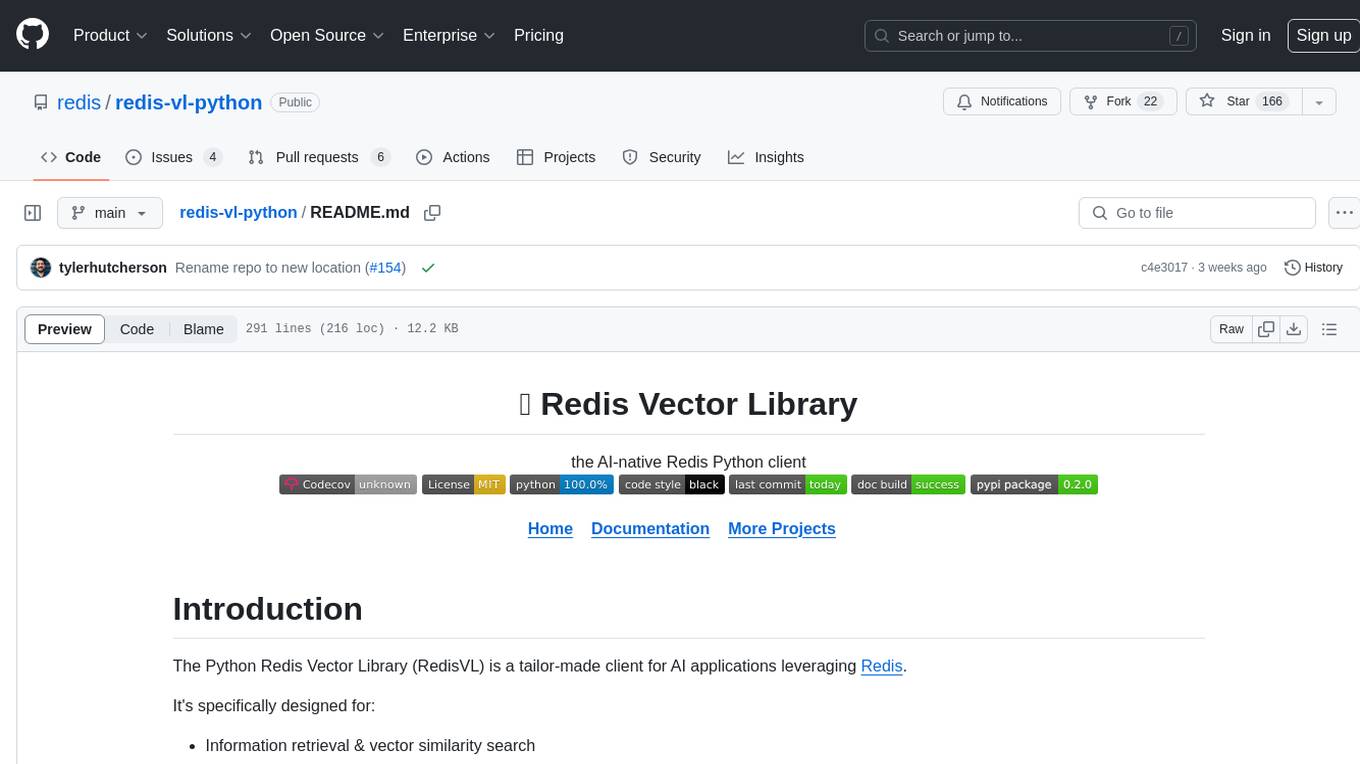
redis-vl-python
The Python Redis Vector Library (RedisVL) is a tailor-made client for AI applications leveraging Redis. It enhances applications with Redis' speed, flexibility, and reliability, incorporating capabilities like vector-based semantic search, full-text search, and geo-spatial search. The library bridges the gap between the emerging AI-native developer ecosystem and the capabilities of Redis by providing a lightweight, elegant, and intuitive interface. It abstracts the features of Redis into a grammar that is more aligned to the needs of today's AI/ML Engineers or Data Scientists.