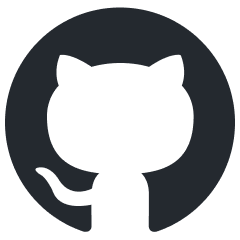
Pandrator
Turn PDFs and EPUBs into audiobooks, subtitles or videos into dubbed videos (including translation), and more. For free. Pandrator uses local models, notably XTTS, including voice-cloning (instant, RVC-enhanced, XTTS fine-tuning) and LLM processing. It aspires to be a user-friendly app with a GUI, an installer and all-in-one packages.
Stars: 429
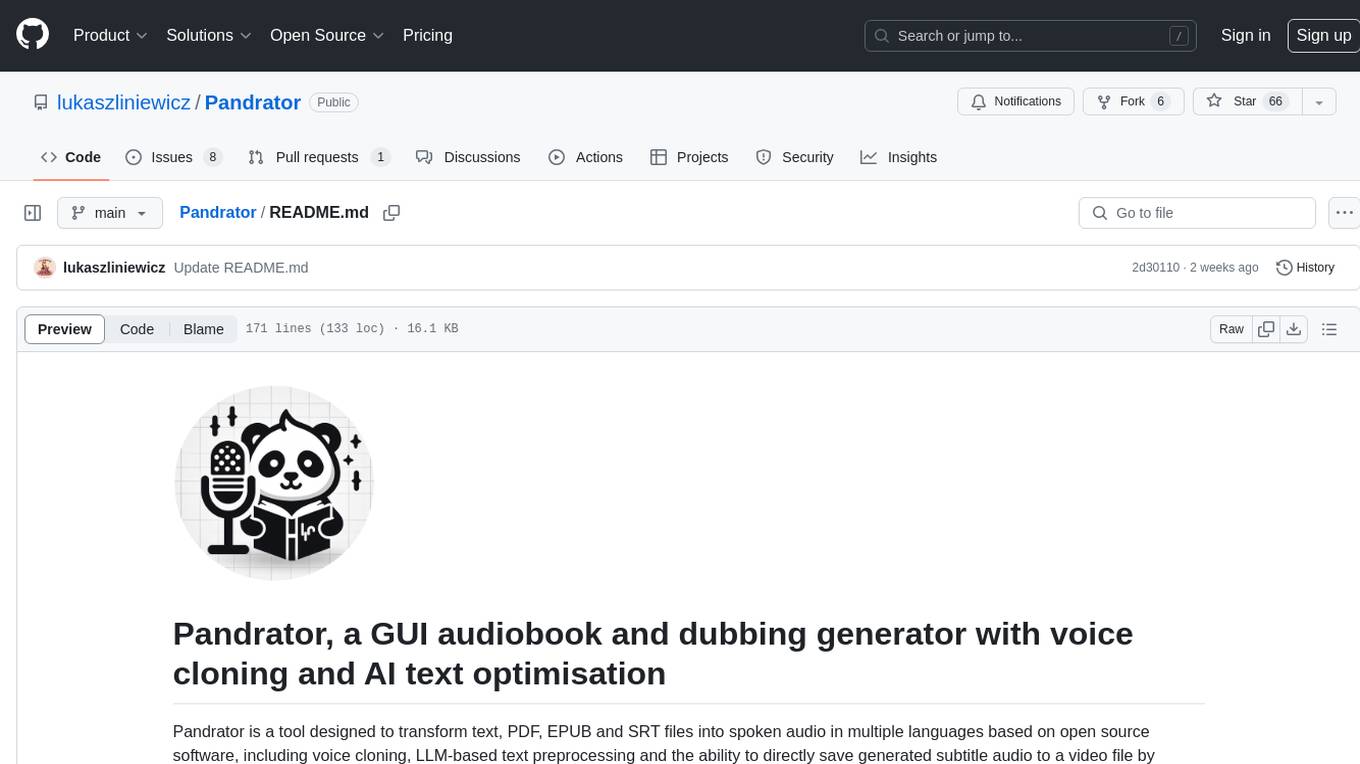
Pandrator is a GUI tool for generating audiobooks and dubbing using voice cloning and AI. It transforms text, PDF, EPUB, and SRT files into spoken audio in multiple languages. It leverages XTTS, Silero, and VoiceCraft models for text-to-speech conversion and voice cloning, with additional features like LLM-based text preprocessing and NISQA for audio quality evaluation. The tool aims to be user-friendly with a one-click installer and a graphical interface.
README:
Pandrator: a multilingual GUI audiobook, subtitle and dubbing generator with voice cloning and translation
[!TIP] TL;DR:
- Pandrator is not an AI model itself, but a GUI framework for Text-to-Speech, subtitle generation and translation projects. It can generate audiobooks and subtitles/dubbing by leveraging several AI tools, custom workflows and algorithms. It works on Windows out of the box. It does work on Linux, but you have to perform a manual installation at the moment.
- The easiest way to use it is to download one of the precompiled archives - simply unpack them and use the included launcher. See this table for their contents and sizes.
- You can talk to me or share tips/workflows/ideas on the Discord server.
This video shows the process of launching Pandrator, selecting a source file, starting generation, stopping it and previewing the saved file. It has not been sped up as it's intended to illustrate the real performance (you may skip the first 35s when the XTTS server is launching, and please remember to turn on the sound).
https://github.com/user-attachments/assets/7cab141a-e043-4057-8166-72cb29281c50
And here you can see the dubbing workflow - from a YT video, through transcription, translation, speech generation to synchronisation.
https://github.com/user-attachments/assets/dfd4b6e8-3eda-49e4-bff4-f1683ec4cf21
Pandrator aspires to be easy to use and install - it has a one-click installer and a graphical user interface. It is a tool designed to perform two tasks:
- transform text, PDF (including see-through cropping), EPUB and SRT files into spoken audio in multiple languages based chiefly on open source software run locally, including preprocessing to make the generated speech sound as natural as possible by, among other things, splitting the text into paragraphs, sentences and smaller logical text blocks (clauses), which the TTS models can process with minimal artifacts. Each sentence can be regenerated if the first attempt is not satisfacory, including marking for regeneration using mouse or keyboard actions when listening back to the generation. Voice cloning is possible for models that support it, and text can be additionally preprocessed using LLMs (to remove OCR artifacts or spell out things that the TTS models struggle with, like Roman numerals and abbreviations, for example),
- generate dubbing either directly from a video file, including transcription (using WhisperX), or from an .srt file. It includes a complete workflow from a video file to a dubbed video file with subtitles - including translation using a variety of APIs and techniques to improve the quality of translation. Subdub, a companion app developed for this purpose, can also be used on its own. You can also correct or translate subtitles without generating audio.
At the moment, it leverages XTTS for its exceptional multilingual capabilities, good quality and easy fine-tuning, and Silero for text-to-speech conversion and voice cloning, enhanced by RVC_CLI for quality improvement and better voice cloning results, and NISQA for audio quality evaluation. Additionally, it incorporates Text Generation Webui's API for local LLM-based text pre-processing, enabling a wide range of text manipulations before audio generation.
-
XTTS supports English (en), Spanish (es), French (fr), German (de), Italian (it), Portuguese (pt), Polish (pl), Turkish (tr), Russian (ru), Dutch (nl), Czech (cs), Arabic (ar), Chinese (zh-cn), Japanese (ja), Hungarian (hu) and Korean (ko).
-
Silero supports English, German, Russian, Spanish, French, Hindi, Russian, Tatar, Ukrainian, Uzbek and Kalmyk.
[!NOTE] Please note that Pandrator is still in an alpha stage and I'm not an experienced developer (I'm a noob, in fact), so the code is far from perfect in terms of optimisation, features and reliability. Please keep this in mind and contribute, if you want to help me make it better.
The samples were generated using the minimal settings - no LLM text processing, RVC or TTS evaluation, and no sentences were regenerated. Both XTTS and Silero generations were faster than playback speed, and Silero used only one CPU core.
https://github.com/user-attachments/assets/1c763c94-c66b-4c22-a698-6c4bcf3e875d
https://github.com/lukaszliniewicz/Pandrator/assets/75737665/118f5b9c-641b-4edd-8ef6-178dd924a883
Dubbing sample, including translation (video source):
https://github.com/user-attachments/assets/1ba8068d-986e-4dec-a162-3b7cc49052f4
TTS Model | CPU Requirements | GPU Requirements |
---|---|---|
XTTS | A reasonably modern CPU with 4+ cores (for CPU-only generation) | NVIDIA GPU with 4GB+ of VRAM for good performance |
Silero | Performs well on most CPUs regardless of core count | N/A |
This project relies on several APIs and services (running locally) and libraries, notably:
- XTTS API Server by daswer123 for Text-to-Speech (TTS) generation using Coqui XTTSv2 OR Silero API Server by ouoertheo for TTS generaton using the Silero models.
- FFmpeg for audio encoding.
-
Sentence Splitter by mediacloud for splitting
.txt
files into sentences, customtkinter by TomSchimansky, num2words by savoirfairelinux, and many others. For a full list, seerequirements.txt
.
- Subdub, a command line app that transcribes video files, translates subtitles and synchronises the generated speech with the video, made specially for Pandrator.
- WhisperX by m-bain, an enhanced implementation of OpenAI's Whisper model with improved alignment, used for dubbing and XTTS training.
- Easy XTTS Trainer, a command line app that enables XTTS fine-tuning using one or more audio files, made specially for Pandrator.
- RVC Python by daswer123 for enhancing voice quality and cloning results with Retrieval Based Voice Conversion.
- Text Generation Webui API by oobabooga for LLM-based text pre-processing.
- NISQA by gabrielmittag for evaluating TTS generations (using the FastAPI implementation).
I've prepared packages (archives) that you can simply unpack - everything is preinstalled in its own portable conda environment. You can download them from here.
You can use the launcher to start Pandrator, update it and install new features.
Package | Contents | Unpacked Size |
---|---|---|
1 | Pandrator and Silero | 4GB |
2 | Pandrator and XTTS | 14GB |
3 | Pandrator, XTTS, RVC, WhisperX (for dubbing) and XTTS fine-tuning | 36GB |
Run pandrator_installer_launcher.exe
with administrator priviliges. You will find it under Releases. The executable was created using pyinstaller from pandrator_installer_launcher.py
in the repository.
The file may be flagged as a threat by antivirus software, so you may have to add it as an exception; if you're not comfortable doing that, install C++ Build Tools and Calibre manually or perform a fully manual installation
You can choose which TTS engines to install and whether to install the software that enables RVC voice cloning (RVC Python), dubbing (WhisperX) and XTTS fine-tuning (Easy XTTS Trainer). You may install more components later.
The Installer/Launcher performs the following tasks:
- Creates the Pandrator folder
- Installs necessary tools if not already present:
- C++ Build Tools
- Calibre
- Installs Miniconda (locally, not system-wide)
- Clones the following repositories:
- Pandrator
- Subdub
- PyPDFCropper
- XTTS API Server (if selected)
- Silero API Server (if selected)
- Creates conda environments (pandrator_installer, xtta_api_server_installer, whisperx_installer, easy_xtts_training_installer). If you want to perform some actions inside the environments, for example for debugging, troubleshooting or customization, please go the the Pandrator folder and run:
conda/Scripts/conda.exe -p conda/envs/env_name run no-capture-output python [command]
- Installs all necessary dependencies
Note: You can use the Installer/Launcher to launch Pandrator and all the tools at any moment.
If you want to perform the setup again, remove the Pandrator folder it created. Please allow at least a couple of minutes for the initial setup process to download models and install dependencies. Depending on the options you've chosen, it may take up to 30 minutes.
For additional functionality not yet included in the installer:
- Install Text Generation Webui and remember to enable the API (add
--api
toCMD_FLAGS.txt
in the main directory of the Webui before starting it). - Set up NISQA API for automatic evaluation of generations.
Please refer to the repositories linked under Dependencies for detailed installation instructions. Remember that the API servers (XTTS, Silero) must be running to make use of the functionalities they offer.
- Git
- Miniconda or Anaconda
- Microsoft Visual C++ Build Tools
- Calibre
-
Install dependencies:
- Calibre: Download and install from https://calibre-ebook.com/download_windows
- Microsoft Visual C++ Build Tools:
winget install --id Microsoft.VisualStudio.2022.BuildTools --override "--quiet --wait --add Microsoft.VisualStudio.Workload.VCTools --includeRecommended" --accept-package-agreements --accept-source-agreements
-
Clone the repositories:
mkdir Pandrator cd Pandrator git clone https://github.com/lukaszliniewicz/Pandrator.git git clone https://github.com/lukaszliniewicz/Subdub.git
-
Create and activate a conda environment:
conda create -n pandrator_installer python=3.10 -y conda activate pandrator_installer
-
Install Pandrator and Subdub requirements:
cd Pandrator pip install -r requirements.txt cd ../Subdub pip install -r requirements.txt cd ..
-
(Optional) Install XTTS:
git clone https://github.com/daswer123/xtts-api-server.git conda create -n xtts_api_server_installer python=3.10 -y conda activate xtts_api_server_installer pip install torch==2.1.1+cu118 torchaudio==2.1.1+cu118 --extra-index-url https://download.pytorch.org/whl/cu118 pip install xtts-api-server
-
(Optional) Install Silero:
conda create -n silero_api_server_installer python=3.10 -y conda activate silero_api_server_installer pip install silero-api-server
-
(Optional) Install RVC (Retrieval-based Voice Conversion):
conda activate pandrator_installer pip install pip==24 pip install rvc-python pip install torch==2.1.1+cu118 torchaudio==2.1.1+cu118 --index-url https://download.pytorch.org/whl/cu118
-
(Optional) Install WhisperX:
conda create -n whisperx_installer python=3.10 -y conda activate whisperx_installer conda install git -c conda-forge -y pip install torch==2.0.1 torchvision==0.15.2 torchaudio==2.0.2 --index-url https://download.pytorch.org/whl/cu118 conda install cudnn=8.9.7.29 -c conda-forge -y conda install ffmpeg -c conda-forge -y pip install git+https://github.com/m-bain/whisperx.git
-
(Optional) Install XTTS Fine-tuning:
git clone https://github.com/lukaszliniewicz/easy_xtts_trainer.git conda create -n easy_xtts_trainer python=3.10 -y conda activate easy_xtts_trainer cd easy_xtts_trainer pip install -r requirements.txt pip install torch==2.1.1+cu118 torchaudio==2.1.1+cu118 --index-url https://download.pytorch.org/whl/cu118 cd ..
-
Run Pandrator:
conda activate pandrator_installer cd Pandrator python pandrator.py
-
Run XTTS API Server (if installed):
conda activate xtts_api_server_installer python -m xtts_api_server
Additional options:
- For CPU only: Add
--device cpu
- For low VRAM: Add
--lowvram
(for 4GB or less) - To use DeepSpeed: Add
--deepspeed
- For CPU only: Add
-
Run Silero API Server (if installed):
conda activate silero_api_server_installer python -m silero_api_server
After installation, your folder structure should look like this:
Pandrator/
├── Pandrator/
├── Subdub/
├── xtts-api-server/ (if XTTS is installed)
├── easy_xtts_trainer/ (if XTTS Fine-tuning is installed)
For more detailed information on using specific components or troubleshooting, please refer to the documentation of each individual repository.
If you don't want to use the additional features like RVC, you have everything you need in the Session tab.
Either create a new session or load an existing one (select a folder in Outputs
to do that).
Choose a .txt
, .srt
, .pdf
, .epub
, .mobi
or .docx
file. If you choose a PDF or EPUB file, a preview window will open with the extracted text. For PDFs, you will be able to crop the document (with translucent pages) ro remove headers and footers or selected pages. You may edit the extracted text (OCRed books often have poorly recognized text from the title page, for example) and check/add paragraphs and Chapter markers (they will be created automatically for EPUB files). Files that contain a lot of text, regardless of format, can take a moment to finish preprocessing before generation begins. The GUI will freeze, but as long as there is processor activity, it's simply working.
- Select the TTS server you want to use - XTTS or Silero - and the language from the dropdown. XTTS is the recommended option.
- Choose the voice you want to use.
-
XTTS, voices are short, 6-12s
.wav
files (22050hz sample rate, mono) stored in thetts_voices
directory (Pandrator/Pandrator/tts_voices
). You can upload and select them via the GUI. The XTTS model uses the audio to clone the voice. It doesn't matter what language the sample is in, you will be able to generate speech in all supported languages, but the quality will be best if you provide a sample in your target language. You may use the sample one in the repository or upload your own. Please make sure that the audio is between 6 and 12s, mono, and the sample rate is 22050hz. You may use a tool like Audacity to prepare the files. The less noise, the better. You may use a tool like Resemble AI for denoising and/or enhancement of your samples on Hugging Face. You may put several samples in a folder insidetts_voices
and the model will use all of them at once (generally up to 4). It can improve the quality. - Silero offers a number of voices for each language it supports. It doesn't support voice cloning. Simply select a voice from the dropdown after choosing the language.
-
XTTS, voices are short, 6-12s
The default output format is .m4b. You can also select opus, mp3 or wav, choose a cover image and provide metadata.
Click on "Start Generation" to begin. You may stop and resume it later, or close the programme and load the session later.
You can play back the generated sentences, also as a playlist, edit them (the text that will be used for regeneration), regenerate or remove individual ones. You can also mark them for regeneration. This is useful when you don't want to stop listening but work on all problematic sentences later. You can use the "m" key to mark the sentence that is currently playing or the right mouse button to mark both the current and the previous sentence (this can be useful if you're listening to the output and not looking at the screen). "Save Output" concatenates the sentences generated so far an encodes them as one file.
Pandrator offers a comprehensive workflow for generating dubbed videos from video files or existing subtitles. This includes transcription, translation, speech generation, and synchronization:
-
Select a Video or SRT File:
- Video File: Choose a video file. The audio will be extracted automatically, and transcription will be performed using WhisperX.
- SRT File: Select an existing SRT subtitle file. In this case, you also need to specify the corresponding video file (unless you only want to translate the subtitles).
-
Transcription (if using a video file):
- Language: Select the language spoken in the original video.
-
Model: Choose a WhisperX model for transcription. Smaller models are faster, while larger ones provide higher accuracy. The
large-v3
model provides the best results. - Pandrator will automatically run WhisperX to generate an SRT file containing the transcription.
-
Translation (optional):
- Enable Translation: Toggle this option to translate the subtitles.
- Original and Target Languages: Select the original language of the subtitles and the language you want to translate them into.
-
Translation Model: Choose a translation model (e.g.,
haiku
,sonnet
,sonnet thinking
,gemini-flash
,gemini-flash-thinking
,gpt-4o-mini
,gpt-4o
,deepl
,local
). With the exception of the local option, you have to set an API key in the API Keys tab. Sonnet provides the best results, but is the most expensive. Gemini-flash-thinking is decent and free (you need to obtain an API key from Google AI Studio). You can translate 500,000 characters for free with DeepL. For local translation, you need to have Text Generation Webui set up and running with the model you want to use loaded. - Chain-of-thought (optional): Enable this option to use chain-of-thought prompting, which may improve quality for non-thinking models - don't use with thinking models (available only for LLMs, not DeepL).
- In order to generate speech, click on Generate Dubbing Audio. You will be able to edit/regenerate the sentences as in the Audiobook workflow. You can also choose to only transcribe the chosen video file or only translate a subtitle file.
- Synchronization: When you're happy with the generated audio, click on Add Dubbing to Video. The dubbing will be synchronised with the video, producing a dubbed video file with embedded subtitles.
- You can change the lenght of silence appended to the end of sentences and paragraphs.
- You can enable a fade-in and -out effect and set the duration.
- You can enable RVC. For this to work, you have to install RVC_Python. You can do this in the Installer/Launcher at any time. You need to select a model - an RVC model consists of two files. A
.pth
and an.index
file. They need to have the same name (e.g. voicex.pth and voicex.index). For best results, use the same voice for XTTS. You can also fine tune the RVC options such as pitch.
- You can disable/enable splitting long sentences and set the max lenght a text fragment sent for TTS generation may have (enabled by default; it tries to split sentences whose lenght exceeds the max lenght value; it looks for punctuation marks (, ; : -) and chooses the one closest to the midpoint of the sentence; if there are no punctuation marks, it looks for conjunctions like "and"; it performs this operation twice as some sentence fragments may still be too long after just one split.
- You can disable/enable appending short sentences (to preceding or following sentences; disabled by default, may perhaps improve the flow as the lenght of text fragments sent to the model is more uniform).
- Remove diacritics (useful when generating a text that contains many foreign words or transliterations from foreign alphabets, e.g. Japanese). Do not enable this if you generate in a language that needs diacritics, like German or Polish! The pronounciation will be wrong then.
- Enable LLM processing to use language models for preprocessing the text before sending it to the TTS API. For example, you may ask the LLM to remove OCR artifacts, spell out abbreviations, correct punctuation etc.
- You can define up to three prompts for text optimization. Each prompt is sent to the LLM API separately, and the output of the last prompt is used for TTS generation.
- For each prompt, you can enable/disable it, set the prompt text, choose the LLM model to use, and enable/disable evaluation (if enabled, the LLM API will be called twice for each prompt, and then again for the model to choose the better result).
- Load the available LLM models using the "Load LLM Models" button in the Session tab.
- Enable RVC to enhance the generated audio quality and apply voice cloning.
- Select the RVC model file (.pth) and the corresponding index file using the "Select RVC Model" and "Select RVC Index" buttons in the Audio Processing tab.
- When RVC is enabled, the generated audio will be processed using the selected RVC model and index before being saved.
- Enable TTS evaluation to assess the quality of the generated audio using the NISQA (Non-Intrusive Speech Quality Assessment) model.
- Set the target MOS (Mean Opinion Score) value and the maximum number of attempts for each sentence.
- When TTS evaluation is enabled, the generated audio will be evaluated using the NISQA model, and the best audio (based on the MOS score) will be chosen for each sentence.
- If the target MOS value is not reached within the maximum number of attempts, the best audio generated so far will be used.
Contributions, suggestions for improvements, and bug reports are most welcome!
- You can find a collection of voice sample for example here. They are intended for use with ElevenLabs, so you will need to pick an 8-12s fragment and save it as 22050khz mono
.wav
usuing Audacity, for instance. - You can find a collection of RVC models for example here.
- [ ] Add support for Surya for PDF OCR, layout and redeaing order detection, plus preprocessing of chapters, headers, footers, footnotes and tables.
- [ ] Add support for StyleTTS2
- [ ] Add importing/exporting settings.
- [ ] Add support for proprietary APIs for text pre-processing and TTS generation.
- [ ] Include OCR for PDFs.
- [ ] Add support for a higher quality local TTS model, Tortoise.
- [ ] Add option to record a voice sample and use it for TTS to the GUI.
- [x] Add support for chapter segmentation
- [x] Add all API servers to the setup script.
- [x] Add support for custom XTTS models
- [x] Add workflow to create dubbing from
.srt
subtitle files. - [x] Include support for PDF files.
- [x] Integrate editing capabilities for processed sentences within the UI.
- [x] Add support for a lower quality but faster local TTS model that can easily run on CPU, e.g. Silero or Piper.
- [x] Add support for EPUB.
For Tasks:
Click tags to check more tools for each tasksFor Jobs:
Alternative AI tools for Pandrator
Similar Open Source Tools
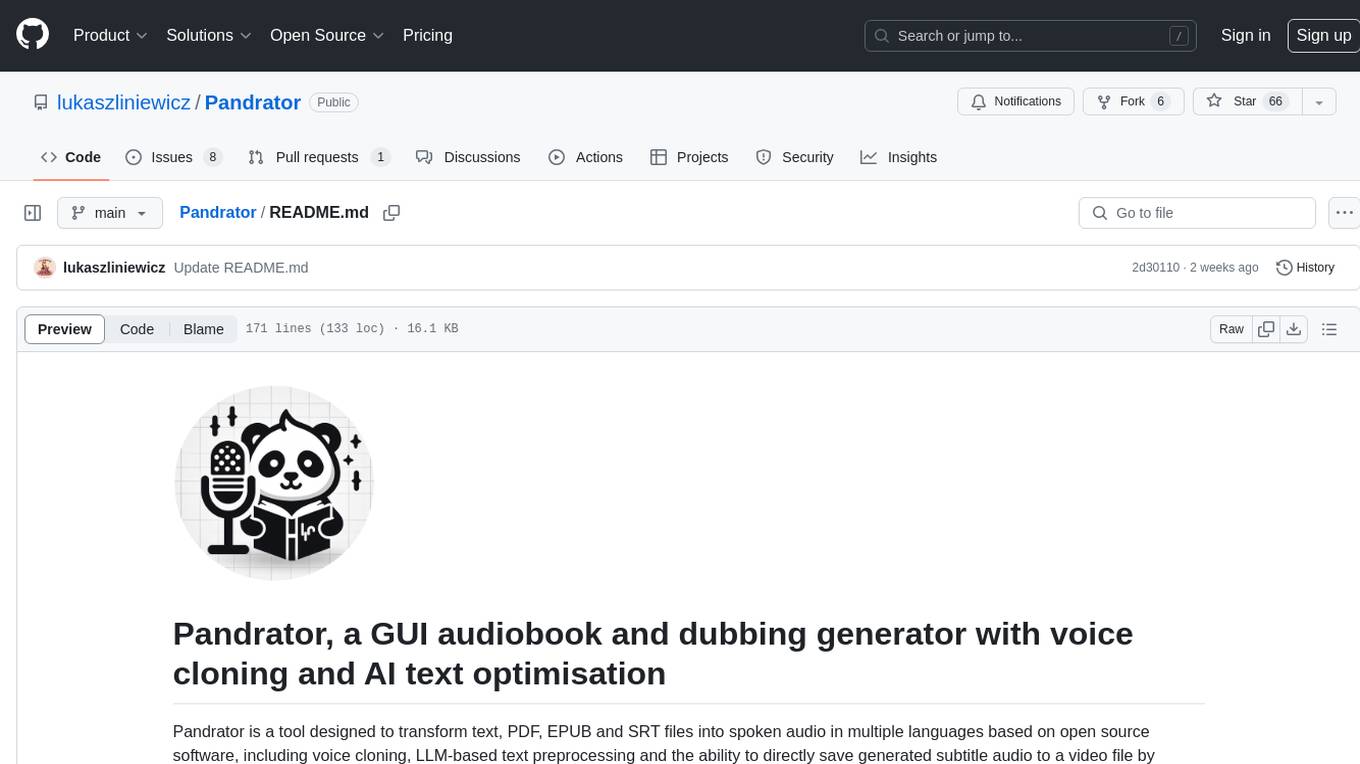
Pandrator
Pandrator is a GUI tool for generating audiobooks and dubbing using voice cloning and AI. It transforms text, PDF, EPUB, and SRT files into spoken audio in multiple languages. It leverages XTTS, Silero, and VoiceCraft models for text-to-speech conversion and voice cloning, with additional features like LLM-based text preprocessing and NISQA for audio quality evaluation. The tool aims to be user-friendly with a one-click installer and a graphical interface.
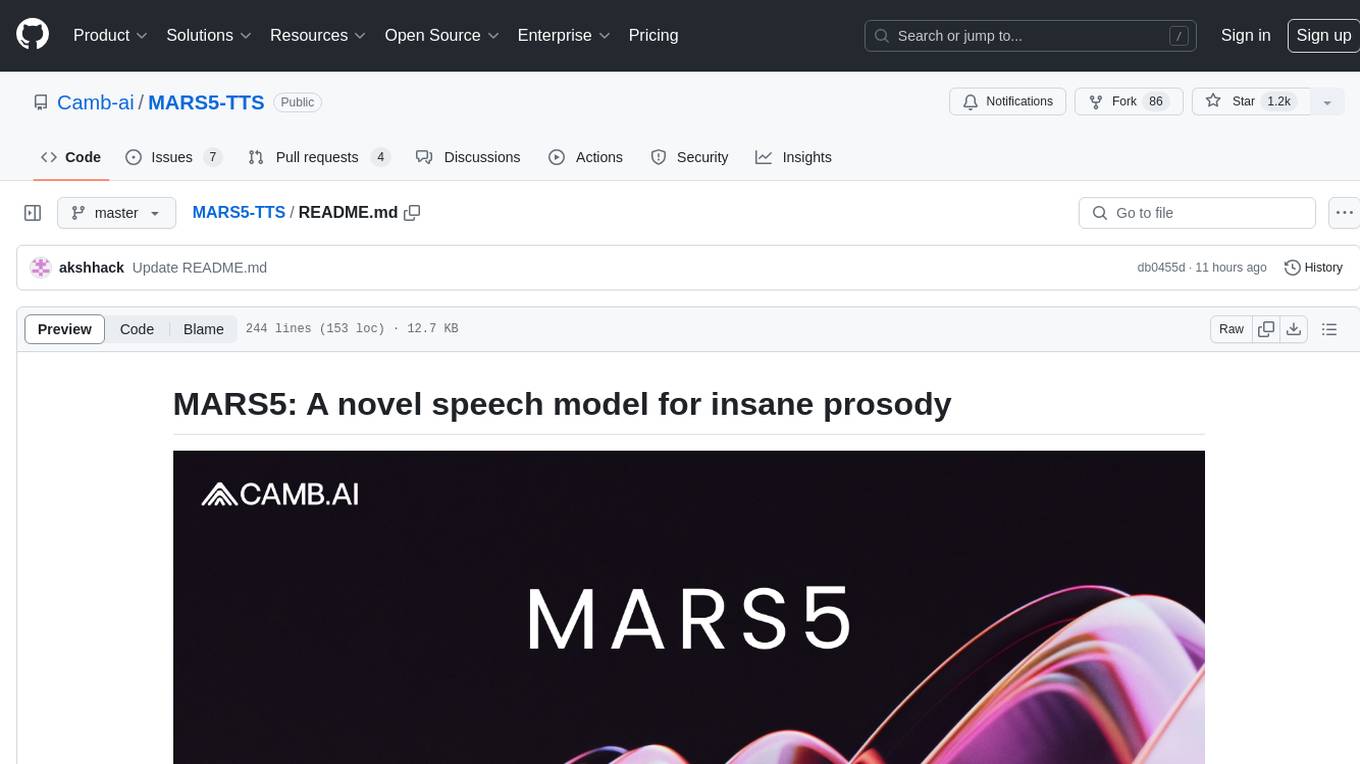
MARS5-TTS
MARS5 is a novel English speech model (TTS) developed by CAMB.AI, featuring a two-stage AR-NAR pipeline with a unique NAR component. The model can generate speech for various scenarios like sports commentary and anime with just 5 seconds of audio and a text snippet. It allows steering prosody using punctuation and capitalization in the transcript. Speaker identity is specified using an audio reference file, enabling 'deep clone' for improved quality. The model can be used via torch.hub or HuggingFace, supporting both shallow and deep cloning for inference. Checkpoints are provided for AR and NAR models, with hardware requirements of 750M+450M params on GPU. Contributions to improve model stability, performance, and reference audio selection are welcome.
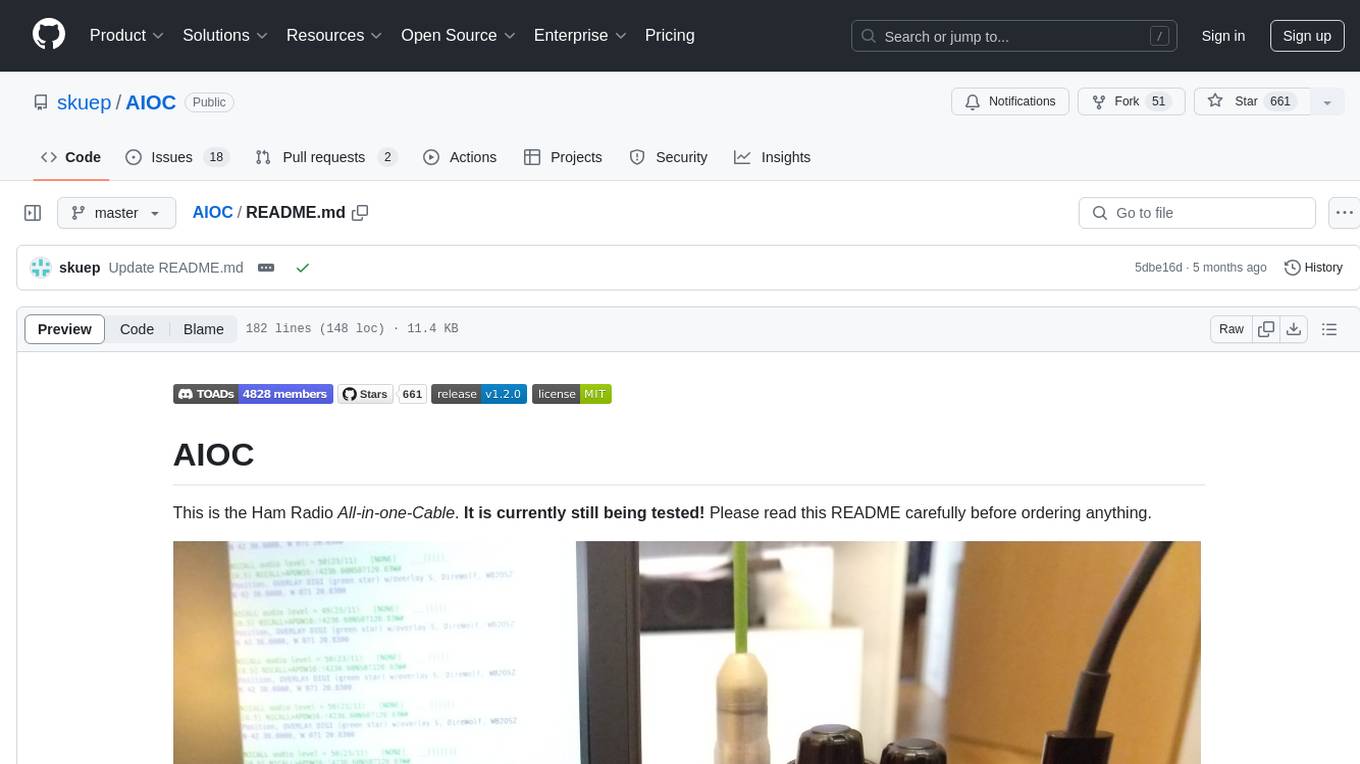
AIOC
AIOC is an All-in-one-Cable for Ham Radio enthusiasts, providing a cheap and hackable digital mode USB interface with features like sound-card, virtual tty, and CM108 compatible HID endpoint. It supports various software and tested radios for functions like programming, APRS, and Dual-PTT HTs. Users can fabricate and assemble the AIOC using specific instructions, and program it using STM32CubeIDE. The tool can be used for tasks like programming radios, asserting PTT, and accessing audio data channels. Future work includes configurable AIOC settings, virtual-PTT, and virtual-COS features.
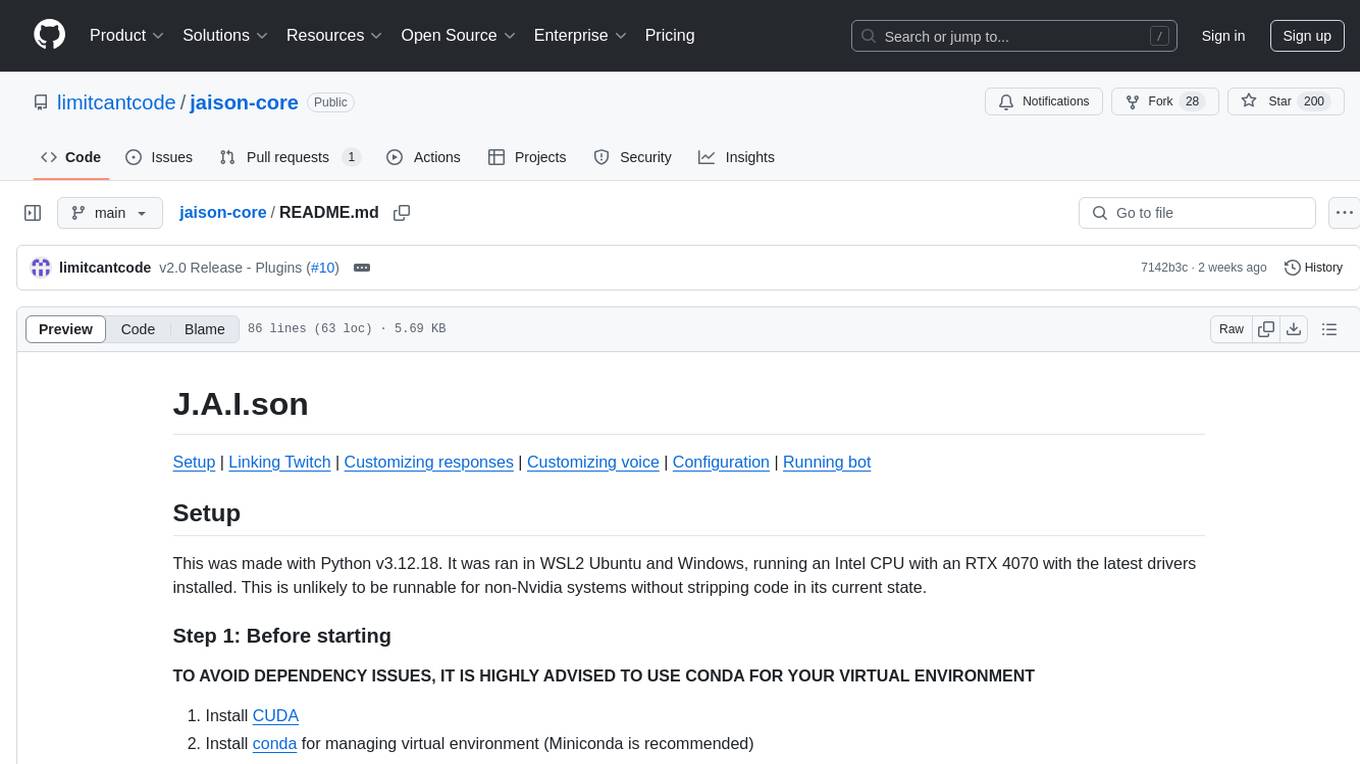
jaison-core
J.A.I.son is a Python project designed for generating responses using various components and applications. It requires specific plugins like STT, T2T, TTSG, and TTSC to function properly. Users can customize responses, voice, and configurations. The project provides a Discord bot, Twitch events and chat integration, and VTube Studio Animation Hotkeyer. It also offers features for managing conversation history, training AI models, and monitoring conversations.
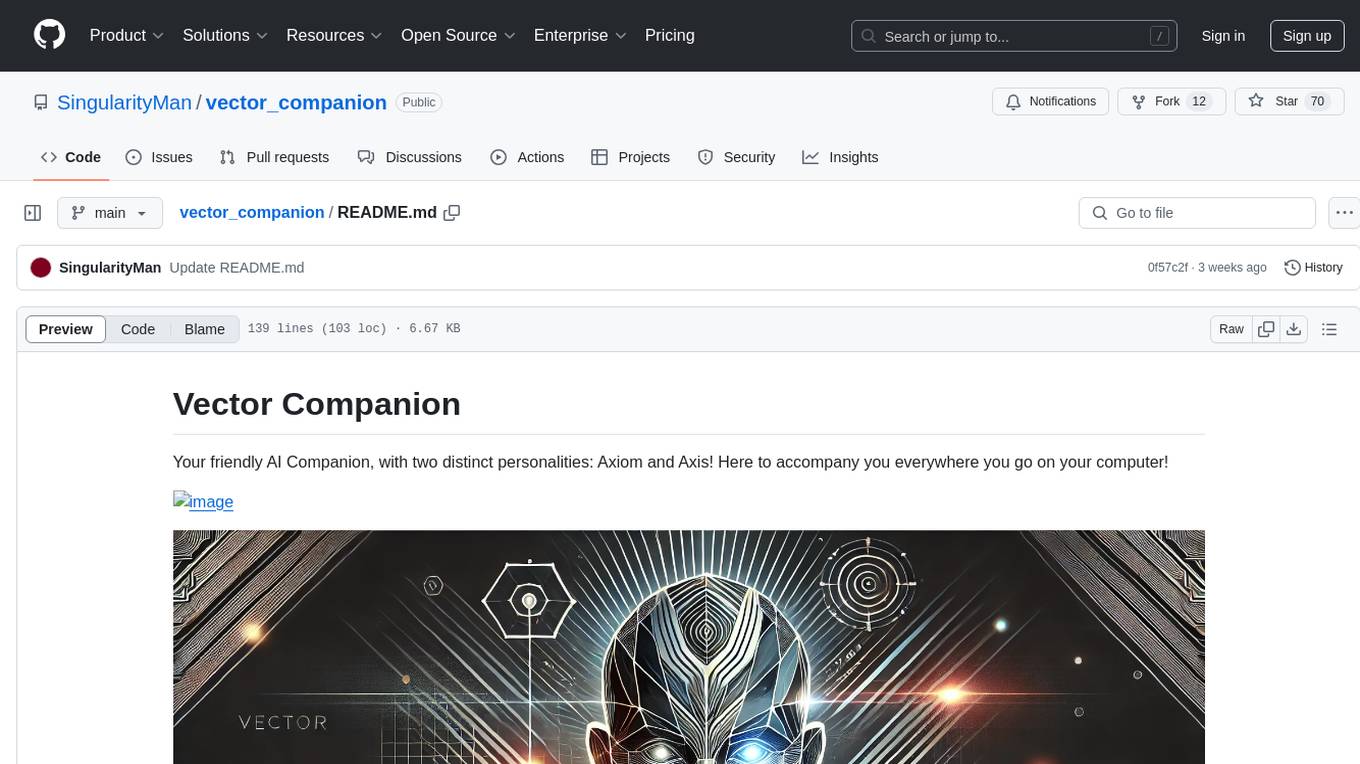
vector_companion
Vector Companion is an AI tool designed to act as a virtual companion on your computer. It consists of two personalities, Axiom and Axis, who can engage in conversations based on what is happening on the screen. The tool can transcribe audio output and user microphone input, take screenshots, and read text via OCR to create lifelike interactions. It requires specific prerequisites to run on Windows and uses VB Cable to capture audio. Users can interact with Axiom and Axis by running the main script after installation and configuration.
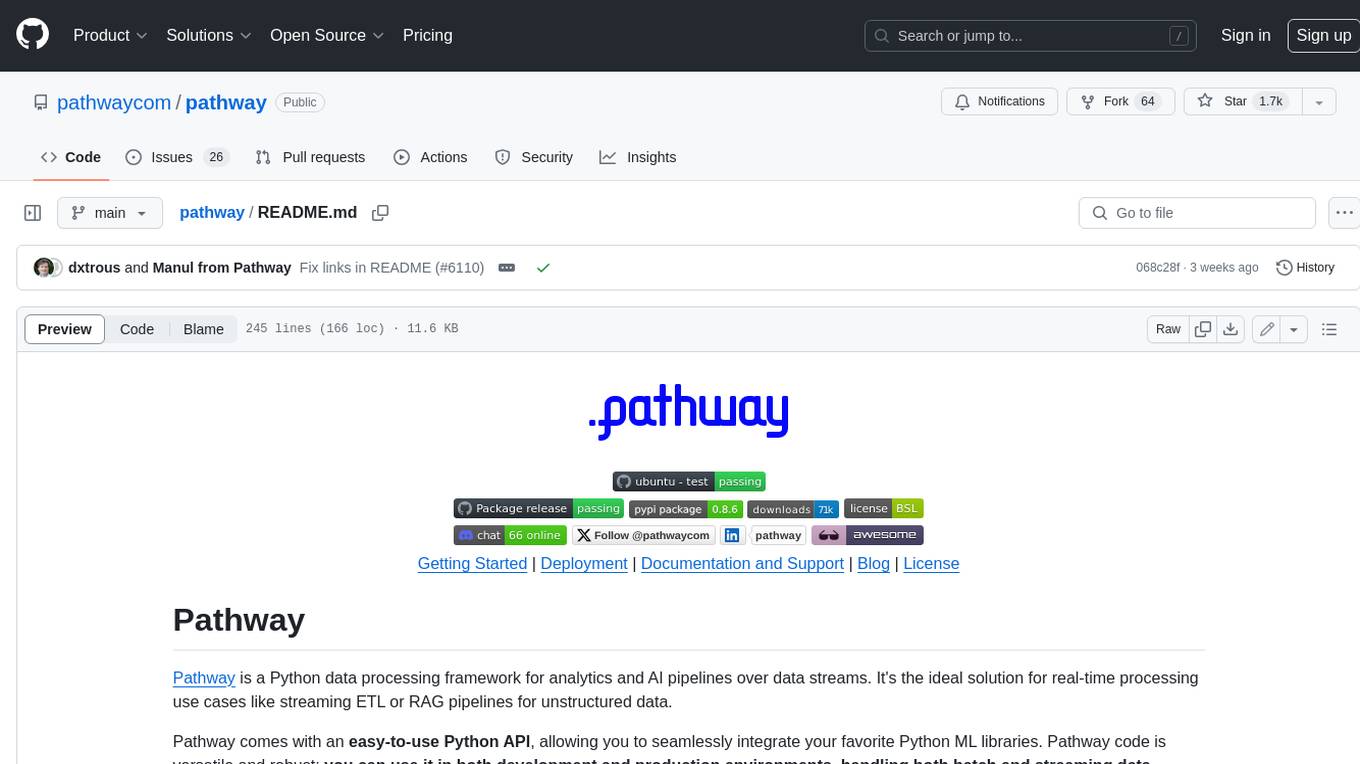
pathway
Pathway is a Python data processing framework for analytics and AI pipelines over data streams. It's the ideal solution for real-time processing use cases like streaming ETL or RAG pipelines for unstructured data. Pathway comes with an **easy-to-use Python API** , allowing you to seamlessly integrate your favorite Python ML libraries. Pathway code is versatile and robust: **you can use it in both development and production environments, handling both batch and streaming data effectively**. The same code can be used for local development, CI/CD tests, running batch jobs, handling stream replays, and processing data streams. Pathway is powered by a **scalable Rust engine** based on Differential Dataflow and performs incremental computation. Your Pathway code, despite being written in Python, is run by the Rust engine, enabling multithreading, multiprocessing, and distributed computations. All the pipeline is kept in memory and can be easily deployed with **Docker and Kubernetes**. You can install Pathway with pip: `pip install -U pathway` For any questions, you will find the community and team behind the project on Discord.
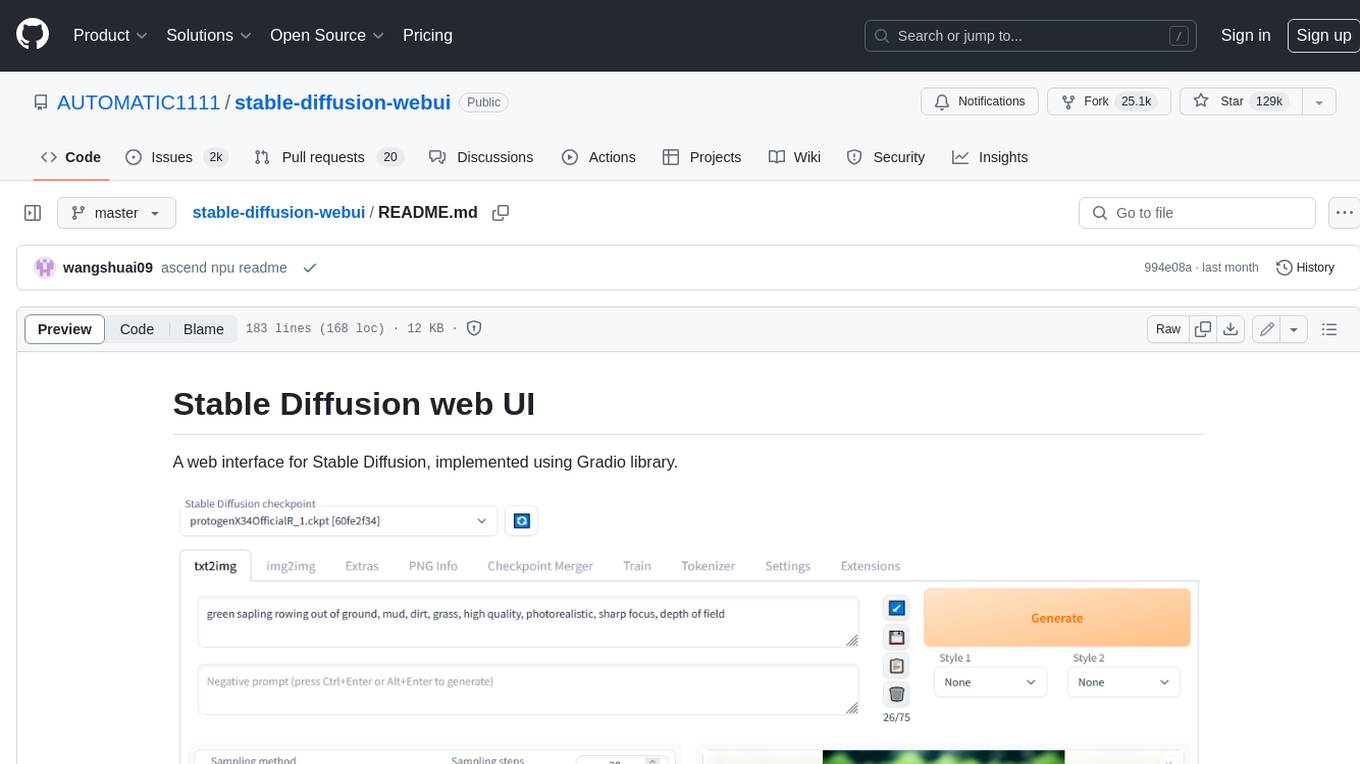
stable-diffusion-webui
Stable Diffusion web UI is a web interface for Stable Diffusion, implemented using Gradio library. It provides a user-friendly interface to access the powerful image generation capabilities of Stable Diffusion. With Stable Diffusion web UI, users can easily generate images from text prompts, edit and refine images using inpainting and outpainting, and explore different artistic styles and techniques. The web UI also includes a range of advanced features such as textual inversion, hypernetworks, and embeddings, allowing users to customize and fine-tune the image generation process. Whether you're an artist, designer, or simply curious about the possibilities of AI-generated art, Stable Diffusion web UI is a valuable tool that empowers you to create stunning and unique images.
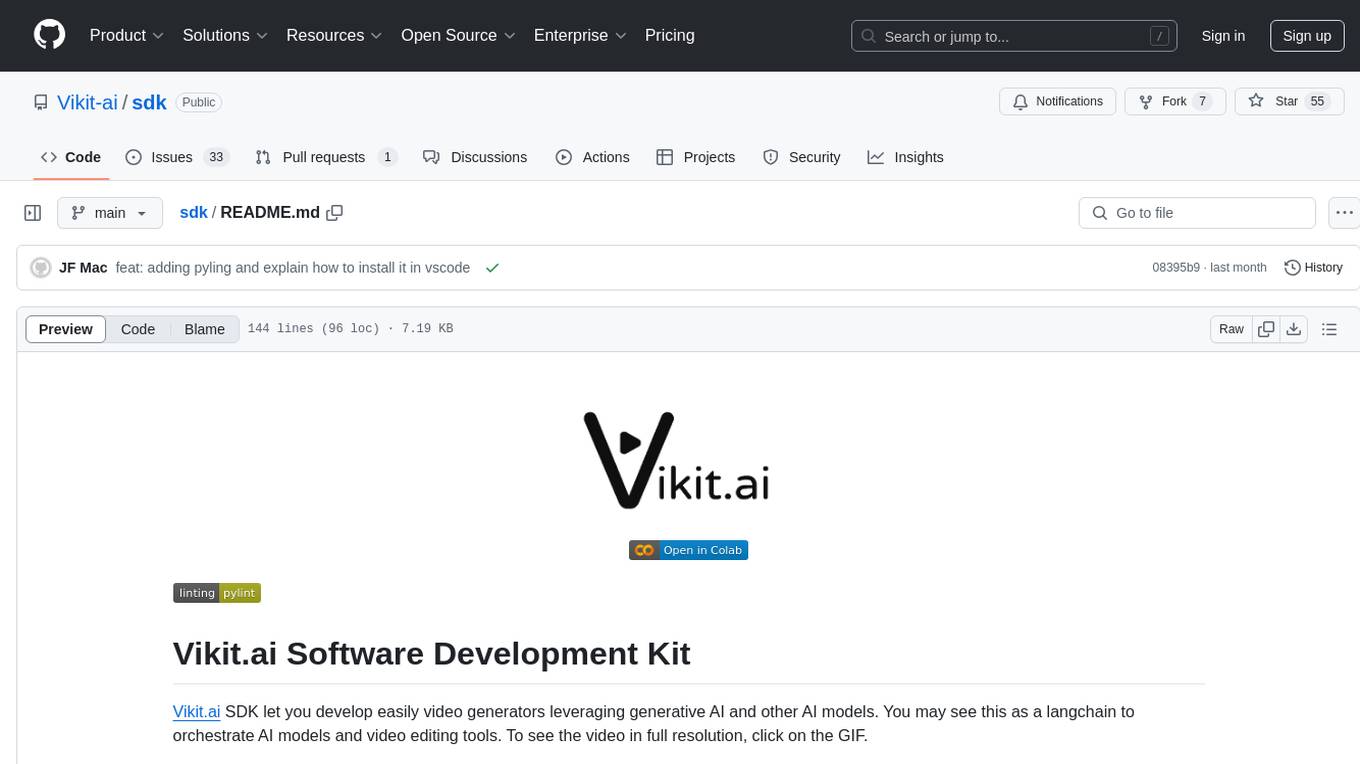
sdk
Vikit.ai SDK is a software development kit that enables easy development of video generators using generative AI and other AI models. It serves as a langchain to orchestrate AI models and video editing tools. The SDK allows users to create videos from text prompts with background music and voice-over narration. It also supports generating composite videos from multiple text prompts. The tool requires Python 3.8+, specific dependencies, and tools like FFMPEG and ImageMagick for certain functionalities. Users can contribute to the project by following the contribution guidelines and standards provided.
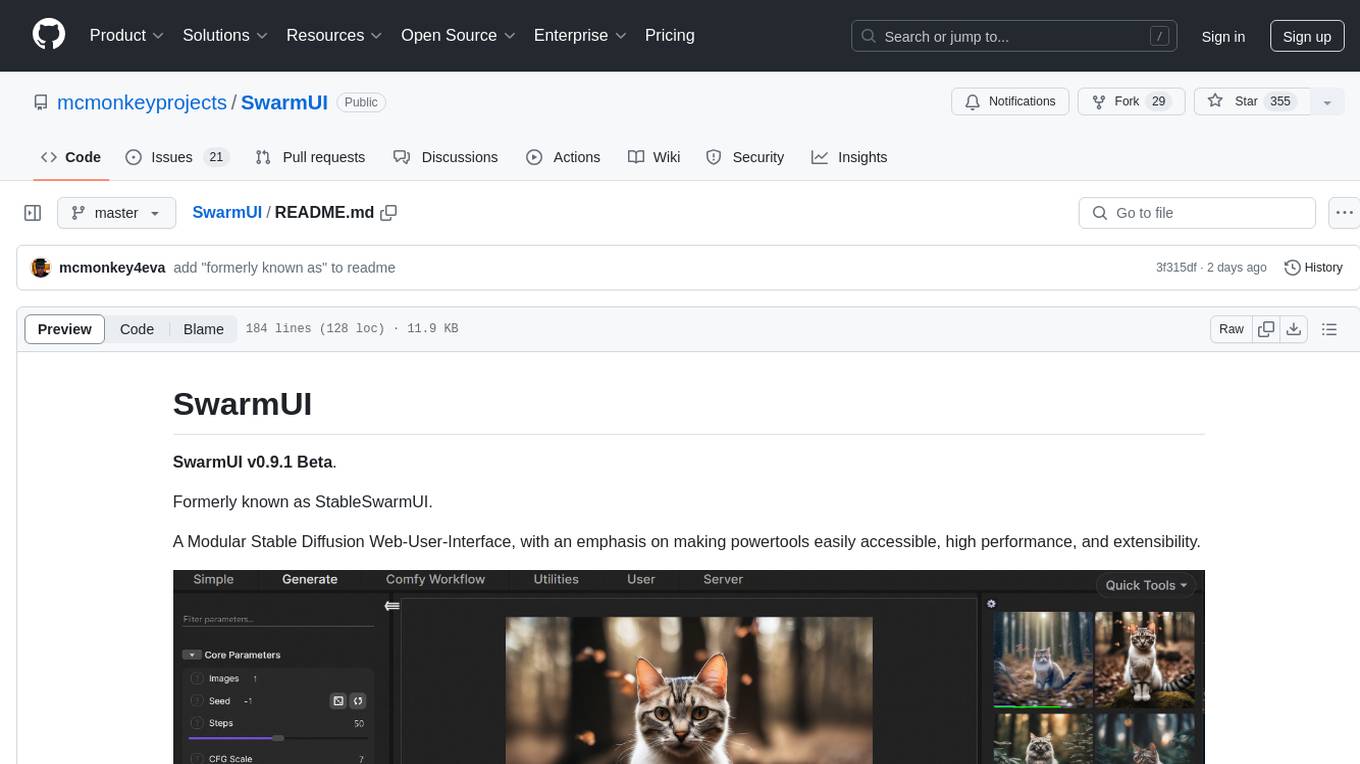
SwarmUI
SwarmUI is a modular stable diffusion web-user-interface designed to make powertools easily accessible, high performance, and extensible. It is in Beta status, offering a primary Generate tab for beginners and a Comfy Workflow tab for advanced users. The tool aims to become a full-featured one-stop-shop for all things Stable Diffusion, with plans for better mobile browser support, detailed 'Current Model' display, dynamic tab shifting, LLM-assisted prompting, and convenient direct distribution as an Electron app.
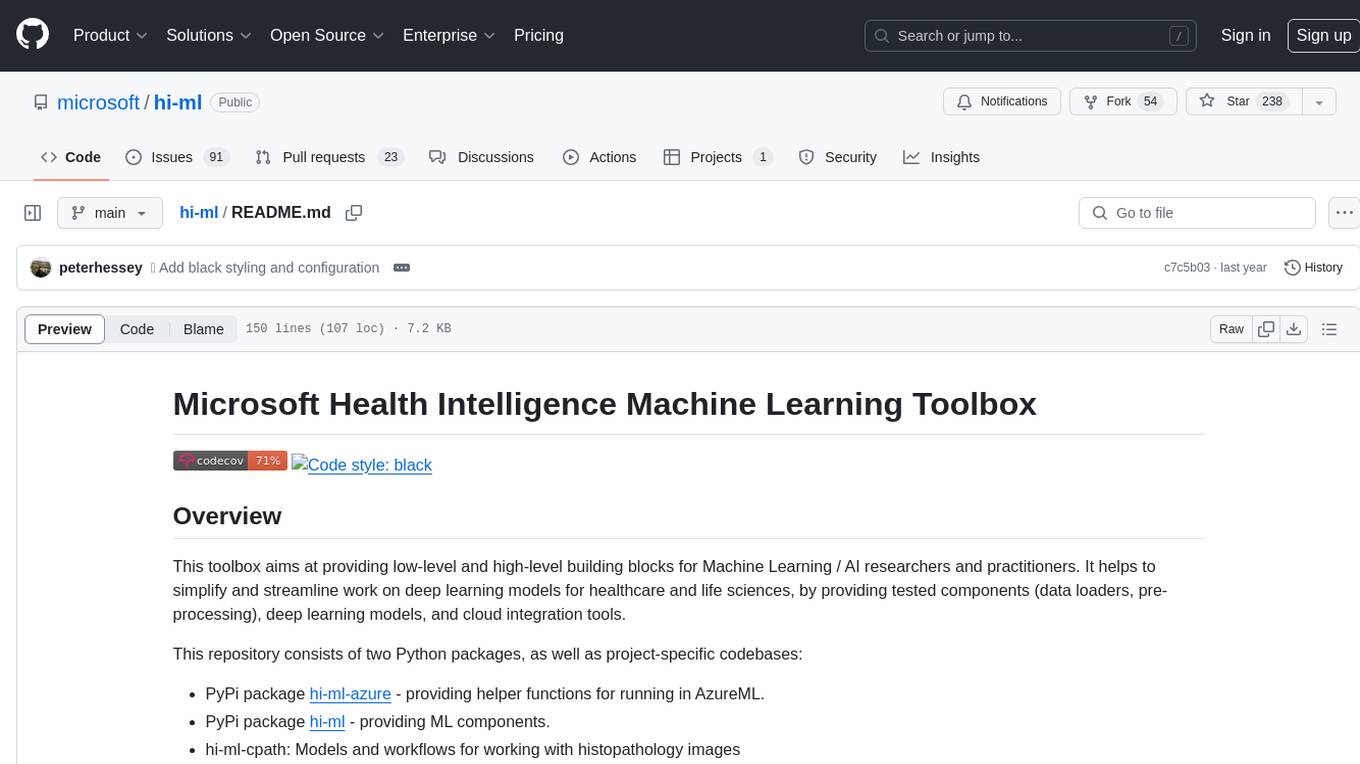
hi-ml
The Microsoft Health Intelligence Machine Learning Toolbox is a repository that provides low-level and high-level building blocks for Machine Learning / AI researchers and practitioners. It simplifies and streamlines work on deep learning models for healthcare and life sciences by offering tested components such as data loaders, pre-processing tools, deep learning models, and cloud integration utilities. The repository includes two Python packages, 'hi-ml-azure' for helper functions in AzureML, 'hi-ml' for ML components, and 'hi-ml-cpath' for models and workflows related to histopathology images.
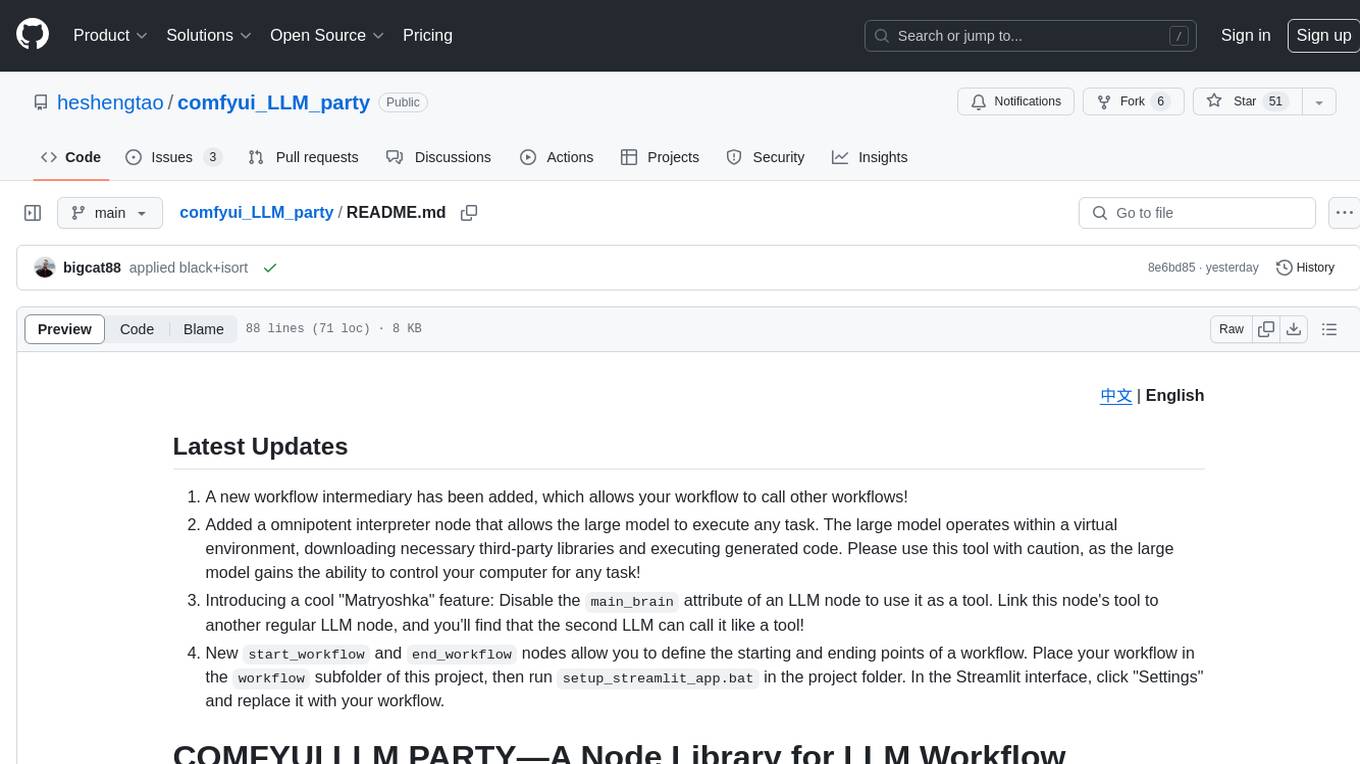
comfyui_LLM_party
COMFYUI LLM PARTY is a node library designed for LLM workflow development in ComfyUI, an extremely minimalist UI interface primarily used for AI drawing and SD model-based workflows. The project aims to provide a complete set of nodes for constructing LLM workflows, enabling users to easily integrate them into existing SD workflows. It features various functionalities such as API integration, local large model integration, RAG support, code interpreters, online queries, conditional statements, looping links for large models, persona mask attachment, and tool invocations for weather lookup, time lookup, knowledge base, code execution, web search, and single-page search. Users can rapidly develop web applications using API + Streamlit and utilize LLM as a tool node. Additionally, the project includes an omnipotent interpreter node that allows the large model to perform any task, with recommendations to use the 'show_text' node for display output.
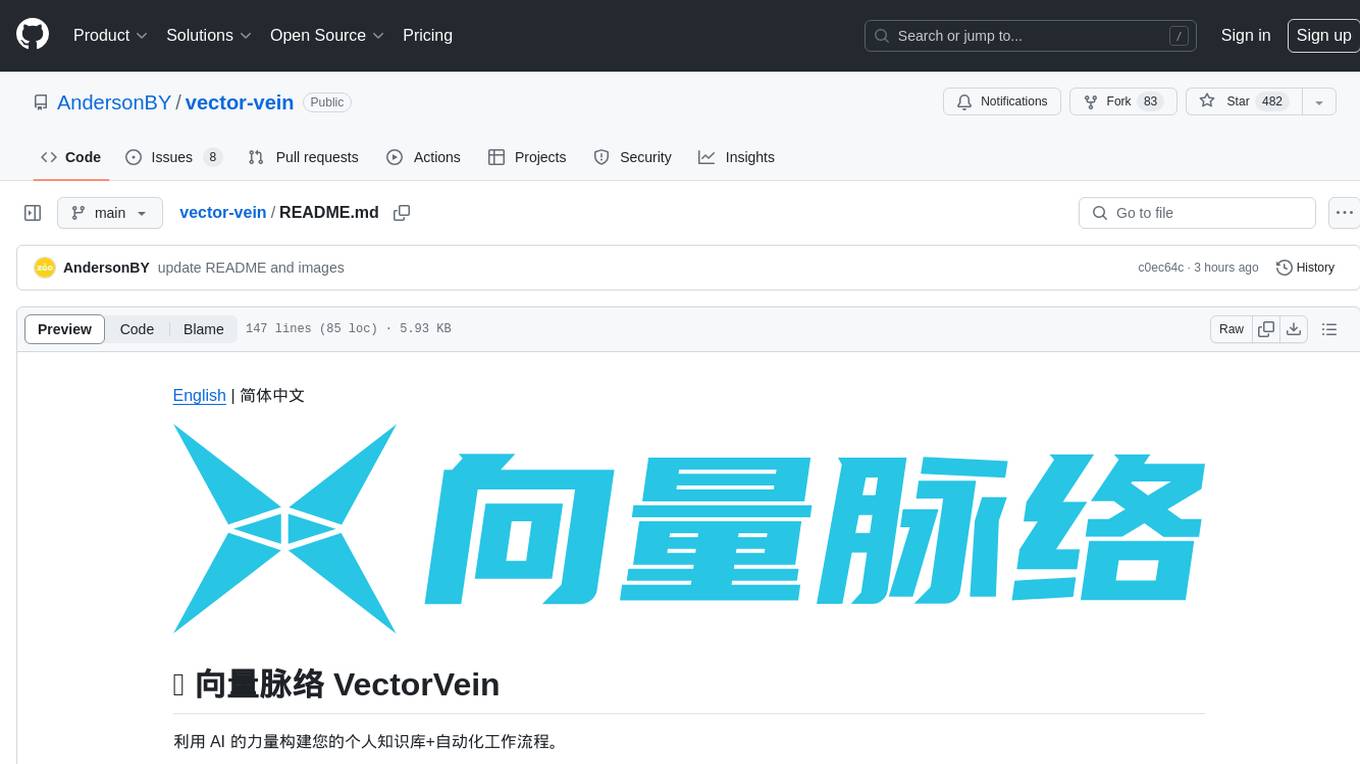
vector-vein
VectorVein is a no-code AI workflow software inspired by LangChain and langflow, aiming to combine the powerful capabilities of large language models and enable users to achieve intelligent and automated daily workflows through simple drag-and-drop actions. Users can create powerful workflows without the need for programming, automating all tasks with ease. The software allows users to define inputs, outputs, and processing methods to create customized workflow processes for various tasks such as translation, mind mapping, summarizing web articles, and automatic categorization of customer reviews.
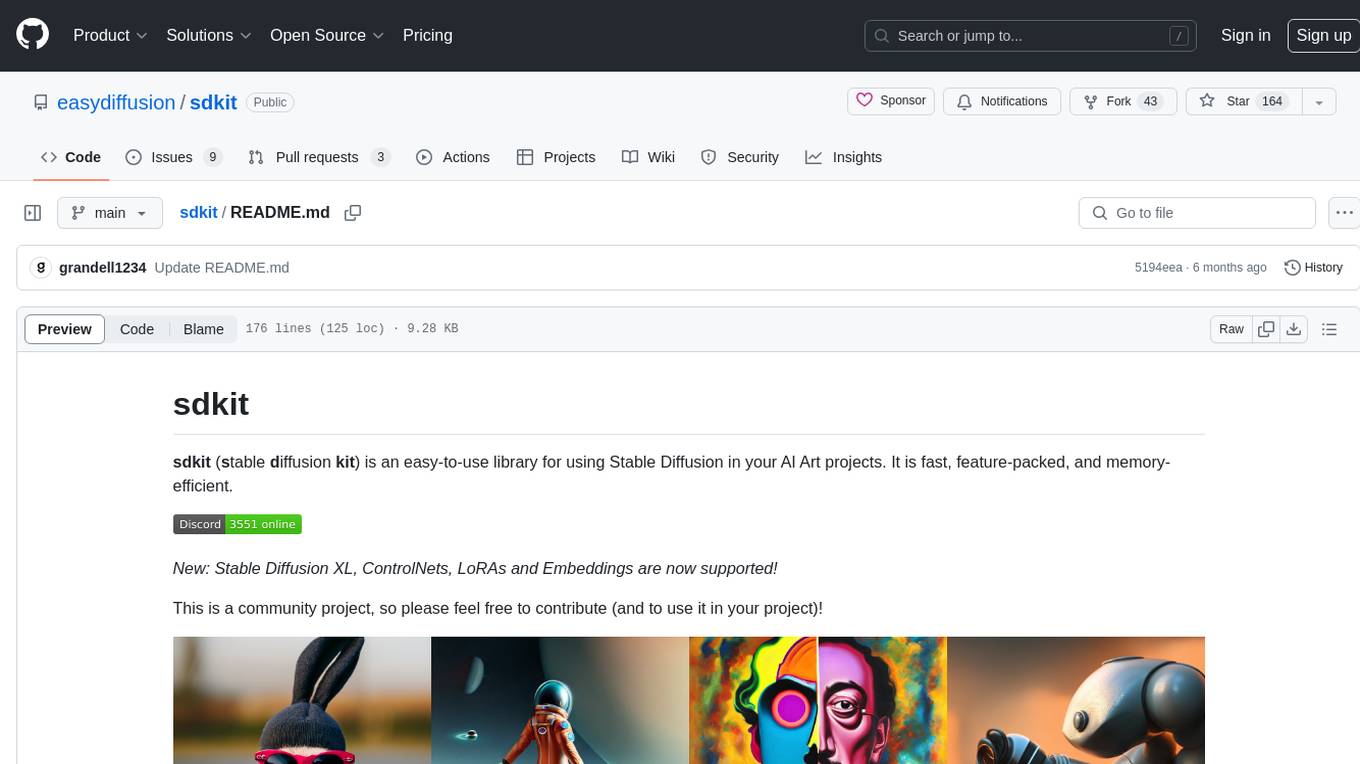
sdkit
sdkit (stable diffusion kit) is an easy-to-use library for utilizing Stable Diffusion in AI Art projects. It includes features like ControlNets, LoRAs, Textual Inversion Embeddings, GFPGAN, CodeFormer for face restoration, RealESRGAN for upscaling, k-samplers, support for custom VAEs, NSFW filter, model-downloader, parallel GPU support, and more. It offers a model database, auto-scanning for malicious models, and various optimizations. The API consists of modules for loading models, generating images, filters, model merging, and utilities, all managed through the sdkit.Context object.
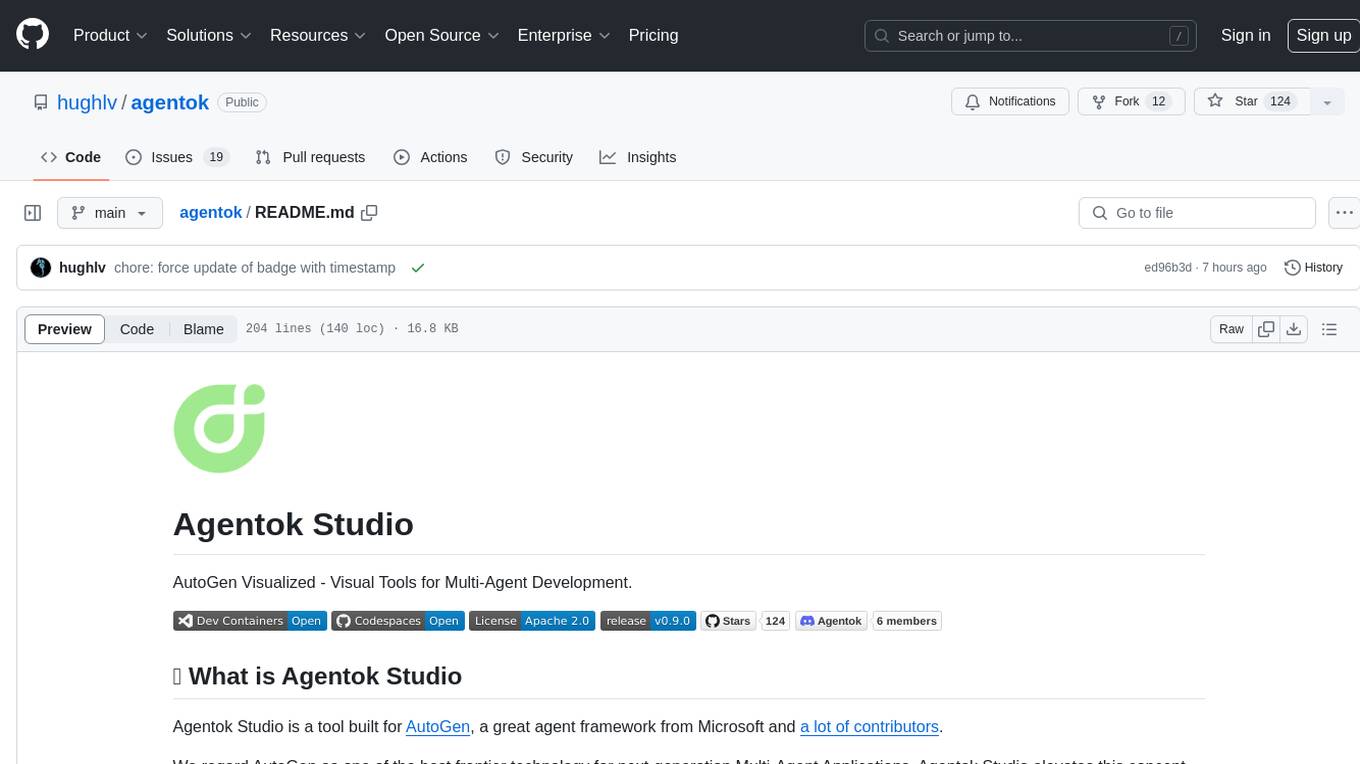
agentok
Agentok Studio is a visual tool built for AutoGen, a cutting-edge agent framework from Microsoft and various contributors. It offers intuitive visual tools to simplify the construction and management of complex agent-based workflows. Users can create workflows visually as graphs, chat with agents, and share flow templates. The tool is designed to streamline the development process for creators and developers working on next-generation Multi-Agent Applications.
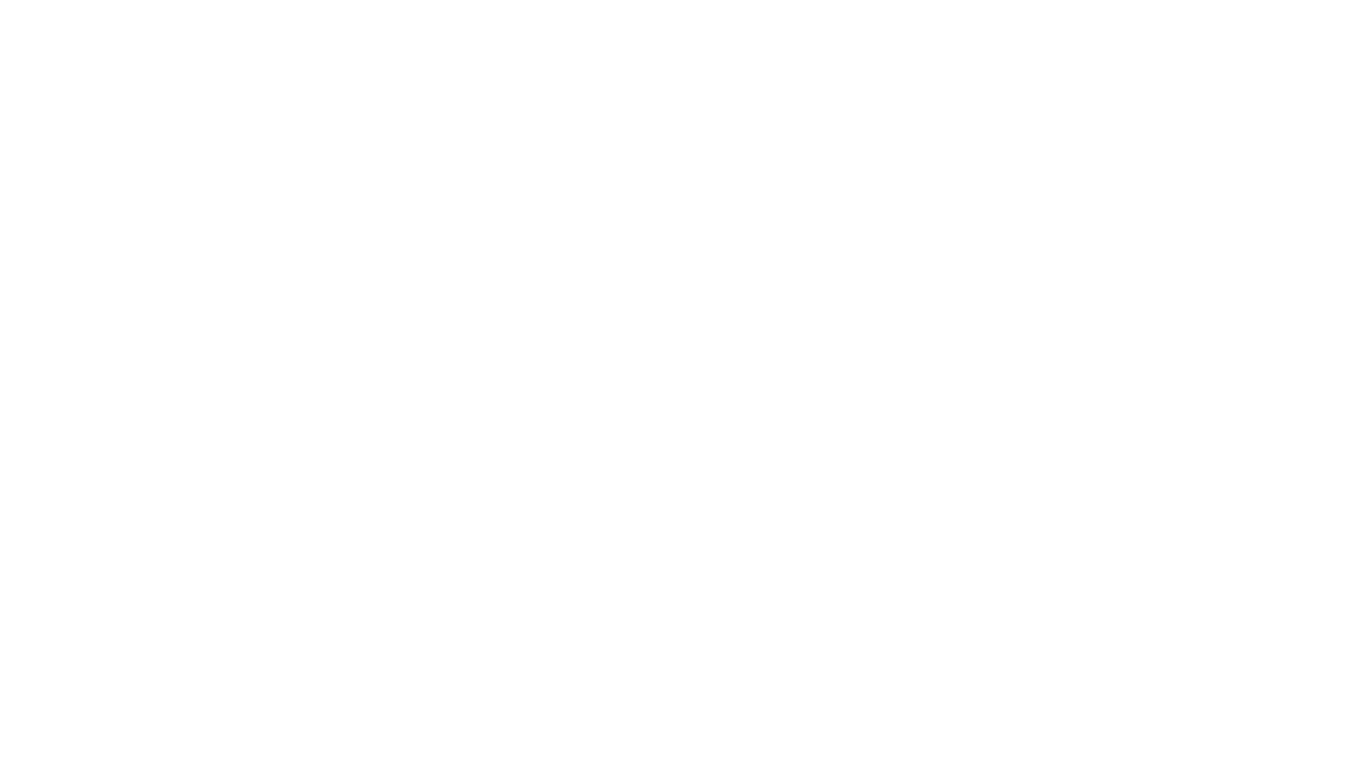
project_alice
Alice is an agentic workflow framework that integrates task execution and intelligent chat capabilities. It provides a flexible environment for creating, managing, and deploying AI agents for various purposes, leveraging a microservices architecture with MongoDB for data persistence. The framework consists of components like APIs, agents, tasks, and chats that interact to produce outputs through files, messages, task results, and URL references. Users can create, test, and deploy agentic solutions in a human-language framework, making it easy to engage with by both users and agents. The tool offers an open-source option, user management, flexible model deployment, and programmatic access to tasks and chats.
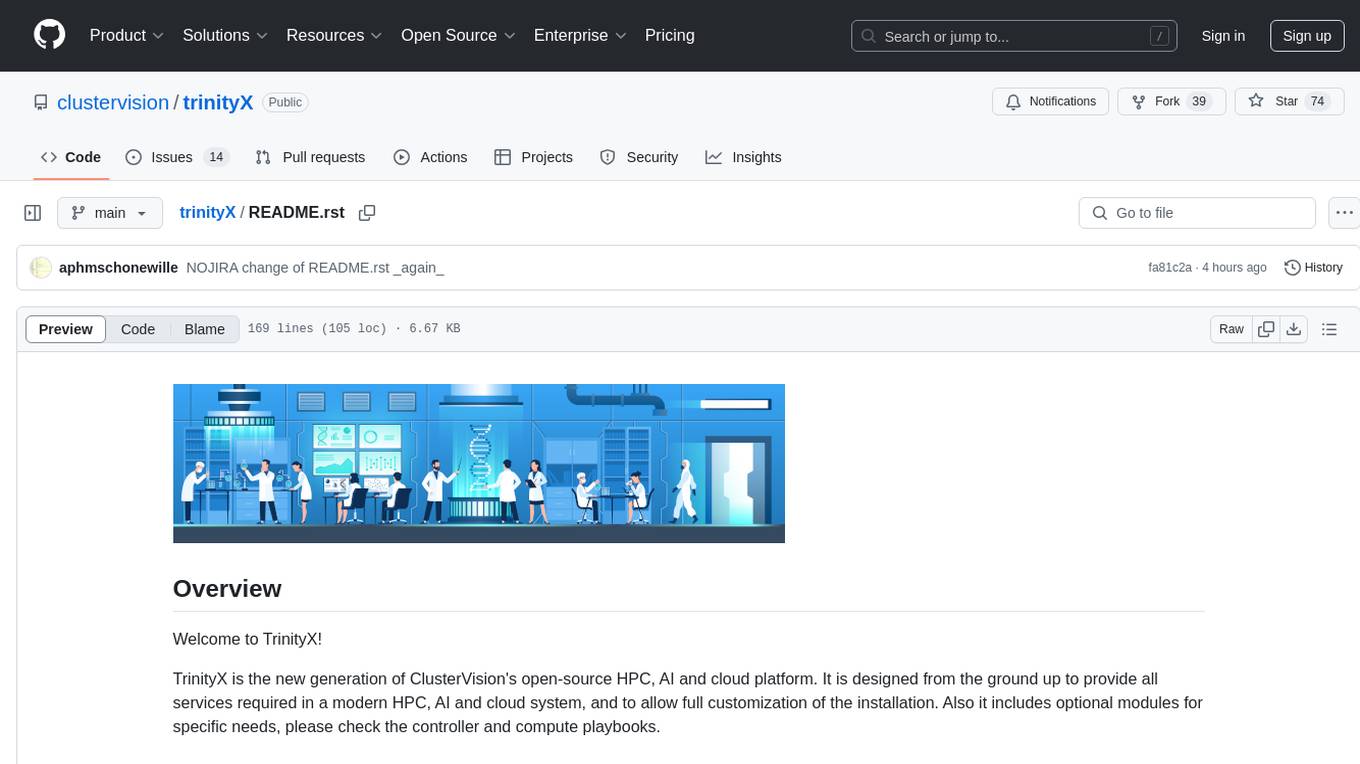
trinityX
TrinityX is an open-source HPC, AI, and cloud platform designed to provide all services required in a modern system, with full customization options. It includes default services like Luna node provisioner, OpenLDAP, SLURM or OpenPBS, Prometheus, Grafana, OpenOndemand, and more. TrinityX also sets up NFS-shared directories, OpenHPC applications, environment modules, HA, and more. Users can install TrinityX on Enterprise Linux, configure network interfaces, set up passwordless authentication, and customize the installation using Ansible playbooks. The platform supports HA, OpenHPC integration, and provides detailed documentation for users to contribute to the project.
For similar tasks
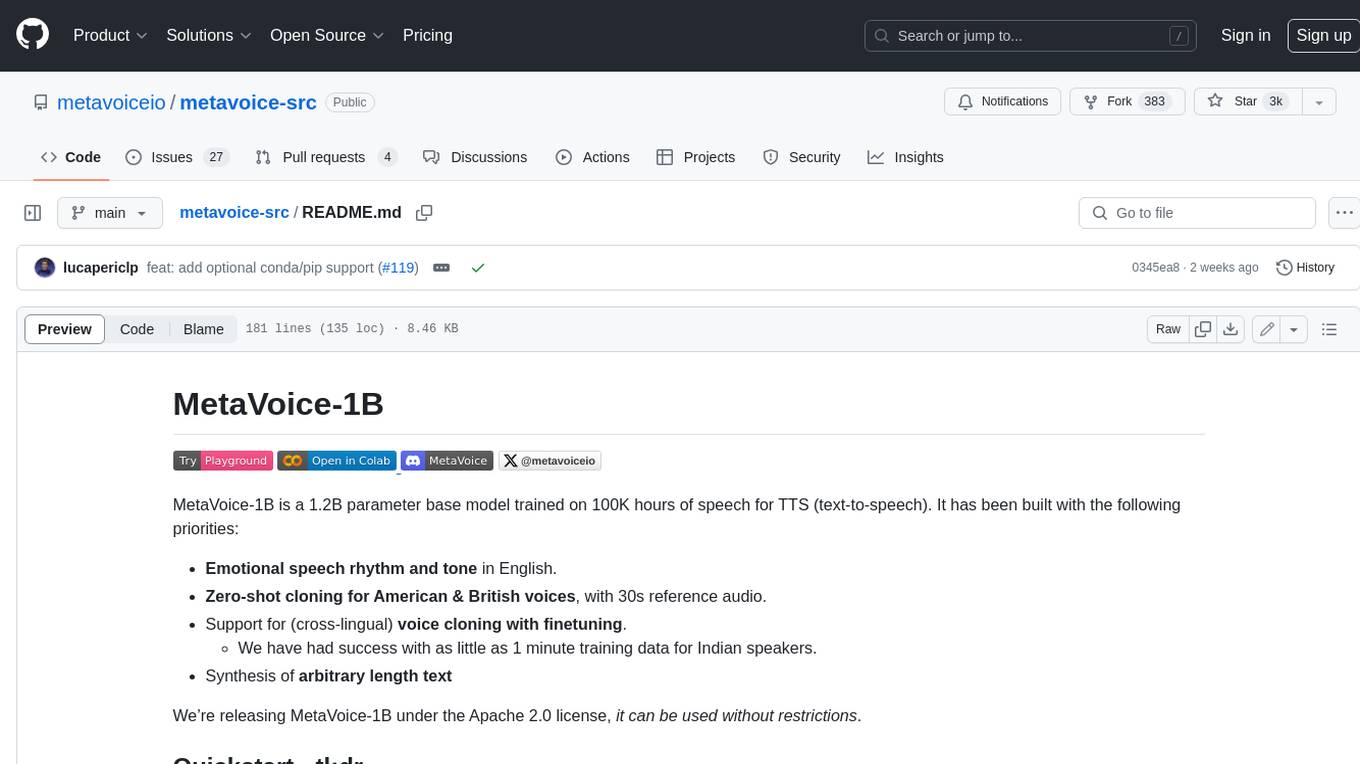
metavoice-src
MetaVoice-1B is a 1.2B parameter base model trained on 100K hours of speech for TTS (text-to-speech). It has been built with the following priorities: * Emotional speech rhythm and tone in English. * Zero-shot cloning for American & British voices, with 30s reference audio. * Support for (cross-lingual) voice cloning with finetuning. * We have had success with as little as 1 minute training data for Indian speakers. * Synthesis of arbitrary length text
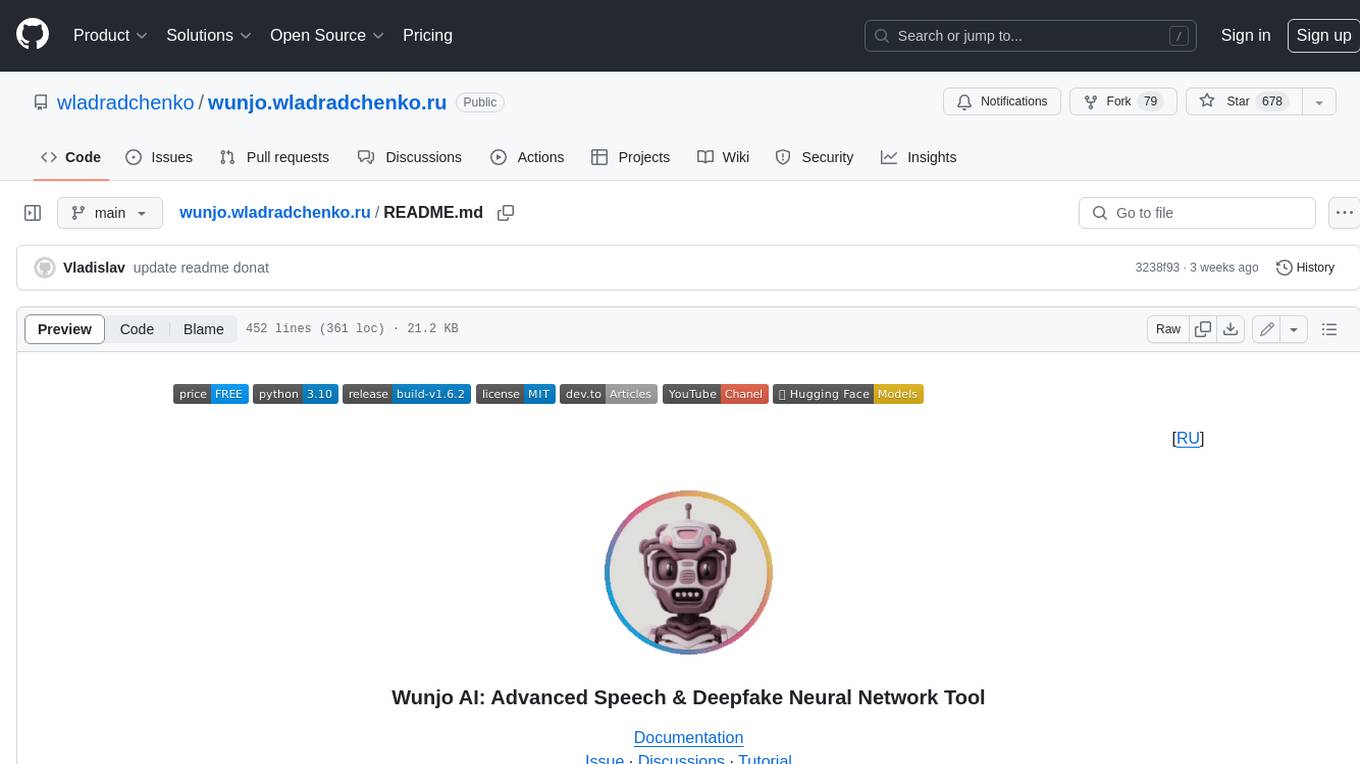
wunjo.wladradchenko.ru
Wunjo AI is a comprehensive tool that empowers users to explore the realm of speech synthesis, deepfake animations, video-to-video transformations, and more. Its user-friendly interface and privacy-first approach make it accessible to both beginners and professionals alike. With Wunjo AI, you can effortlessly convert text into human-like speech, clone voices from audio files, create multi-dialogues with distinct voice profiles, and perform real-time speech recognition. Additionally, you can animate faces using just one photo combined with audio, swap faces in videos, GIFs, and photos, and even remove unwanted objects or enhance the quality of your deepfakes using the AI Retouch Tool. Wunjo AI is an all-in-one solution for your voice and visual AI needs, offering endless possibilities for creativity and expression.
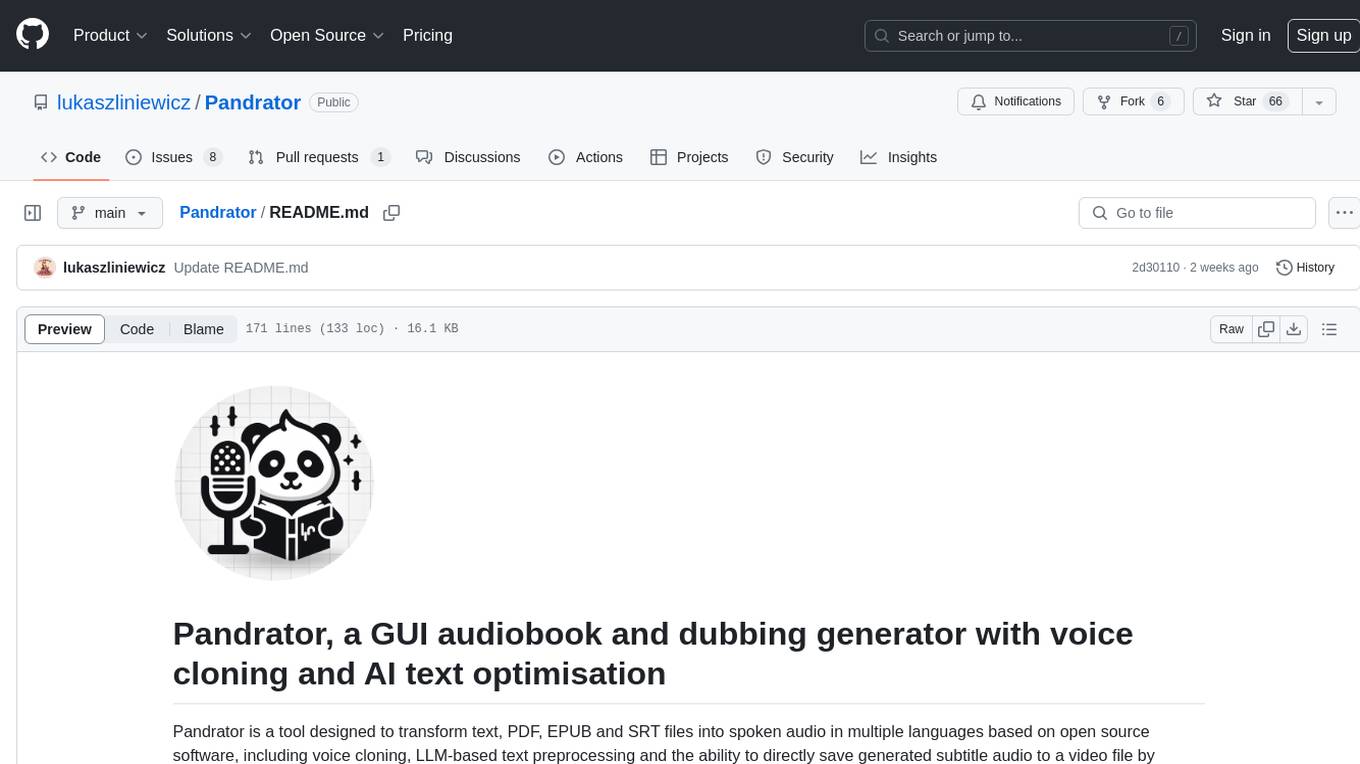
Pandrator
Pandrator is a GUI tool for generating audiobooks and dubbing using voice cloning and AI. It transforms text, PDF, EPUB, and SRT files into spoken audio in multiple languages. It leverages XTTS, Silero, and VoiceCraft models for text-to-speech conversion and voice cloning, with additional features like LLM-based text preprocessing and NISQA for audio quality evaluation. The tool aims to be user-friendly with a one-click installer and a graphical interface.
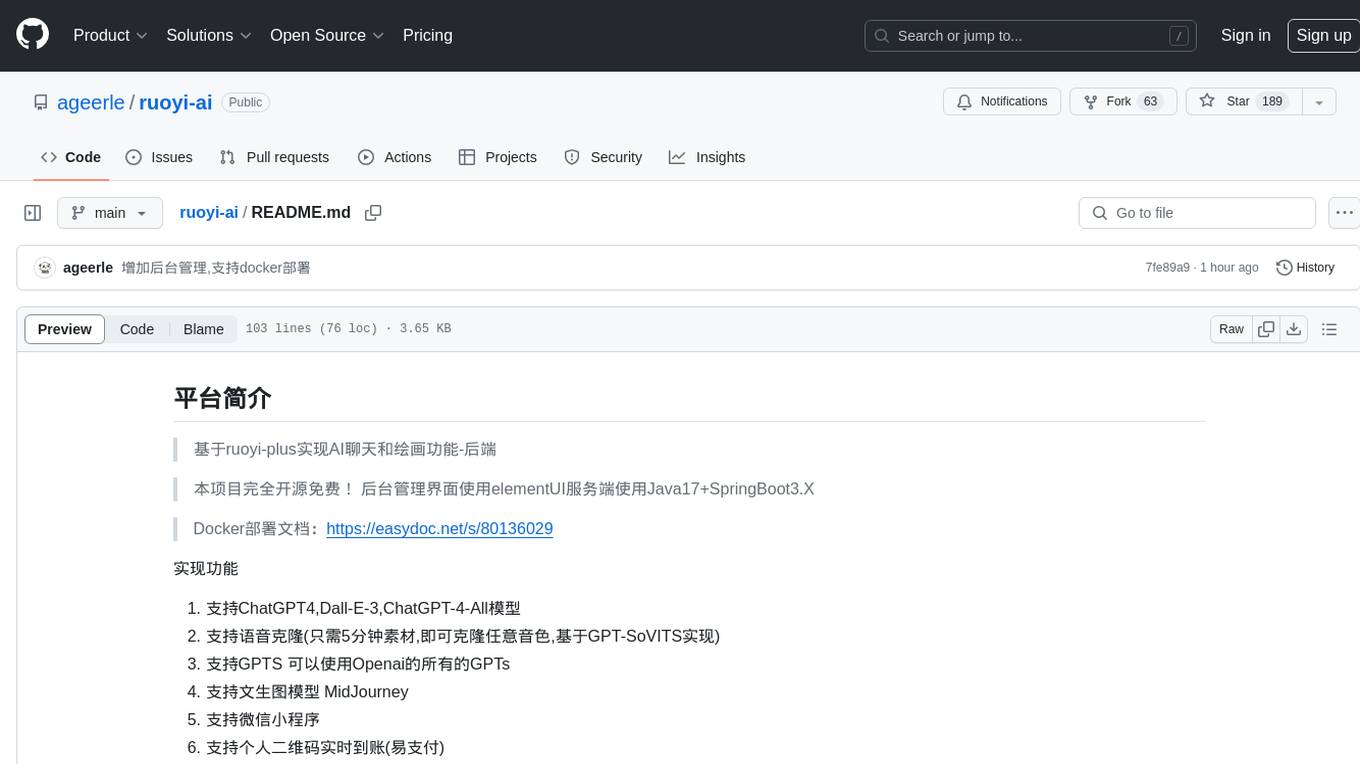
ruoyi-ai
ruoyi-ai is a platform built on top of ruoyi-plus to implement AI chat and drawing functionalities on the backend. The project is completely open source and free. The backend management interface uses elementUI, while the server side is built using Java 17 and SpringBoot 3.X. It supports various AI models such as ChatGPT4, Dall-E-3, ChatGPT-4-All, voice cloning based on GPT-SoVITS, GPTS, and MidJourney. Additionally, it supports WeChat mini programs, personal QR code real-time payments, monitoring and AI auto-reply in live streaming rooms like Douyu and Bilibili, and personal WeChat integration with ChatGPT. The platform also includes features like private knowledge base management and provides various demo interfaces for different platforms such as mobile, web, and PC.
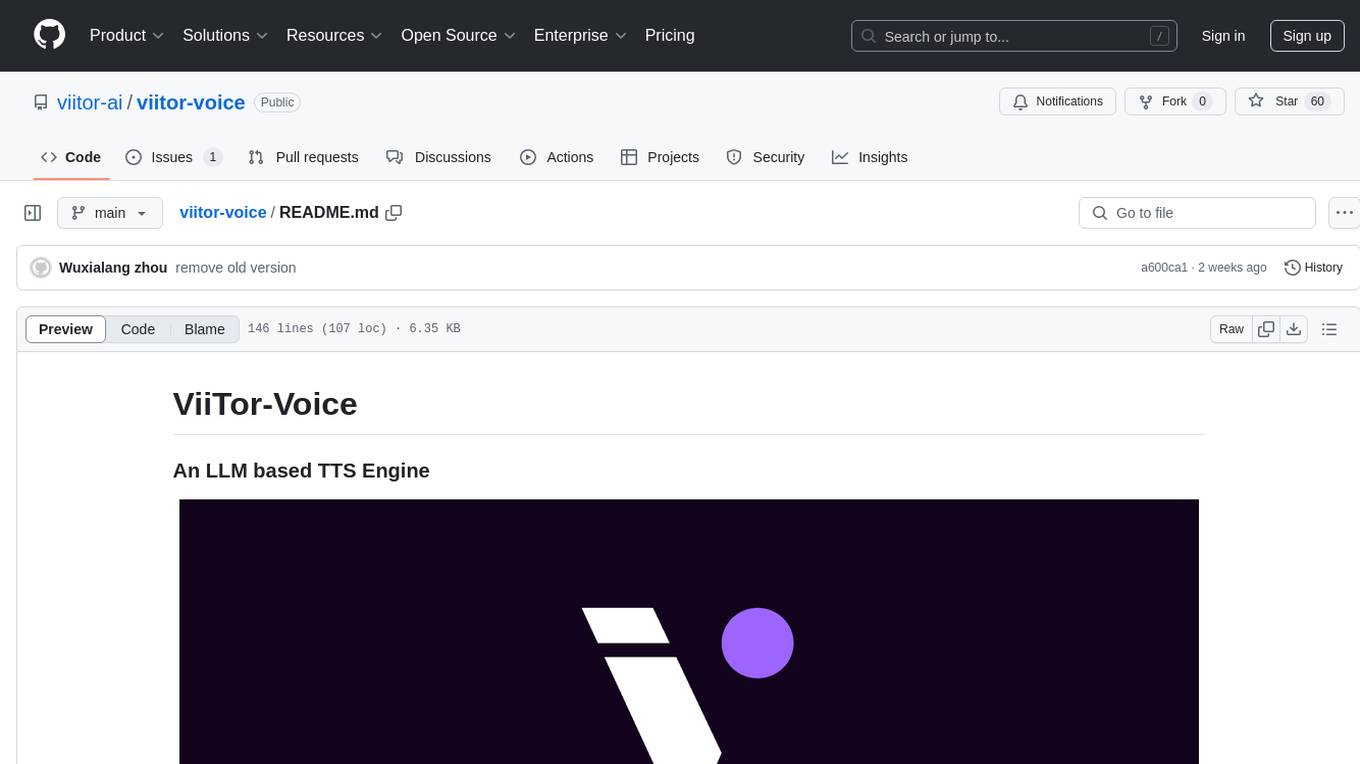
viitor-voice
ViiTor-Voice is an LLM based TTS Engine that offers a lightweight design with 0.5B parameters for efficient deployment on various platforms. It provides real-time streaming output with low latency experience, a rich voice library with over 300 voice options, flexible speech rate adjustment, and zero-shot voice cloning capabilities. The tool supports both Chinese and English languages and is suitable for applications requiring quick response and natural speech fluency.
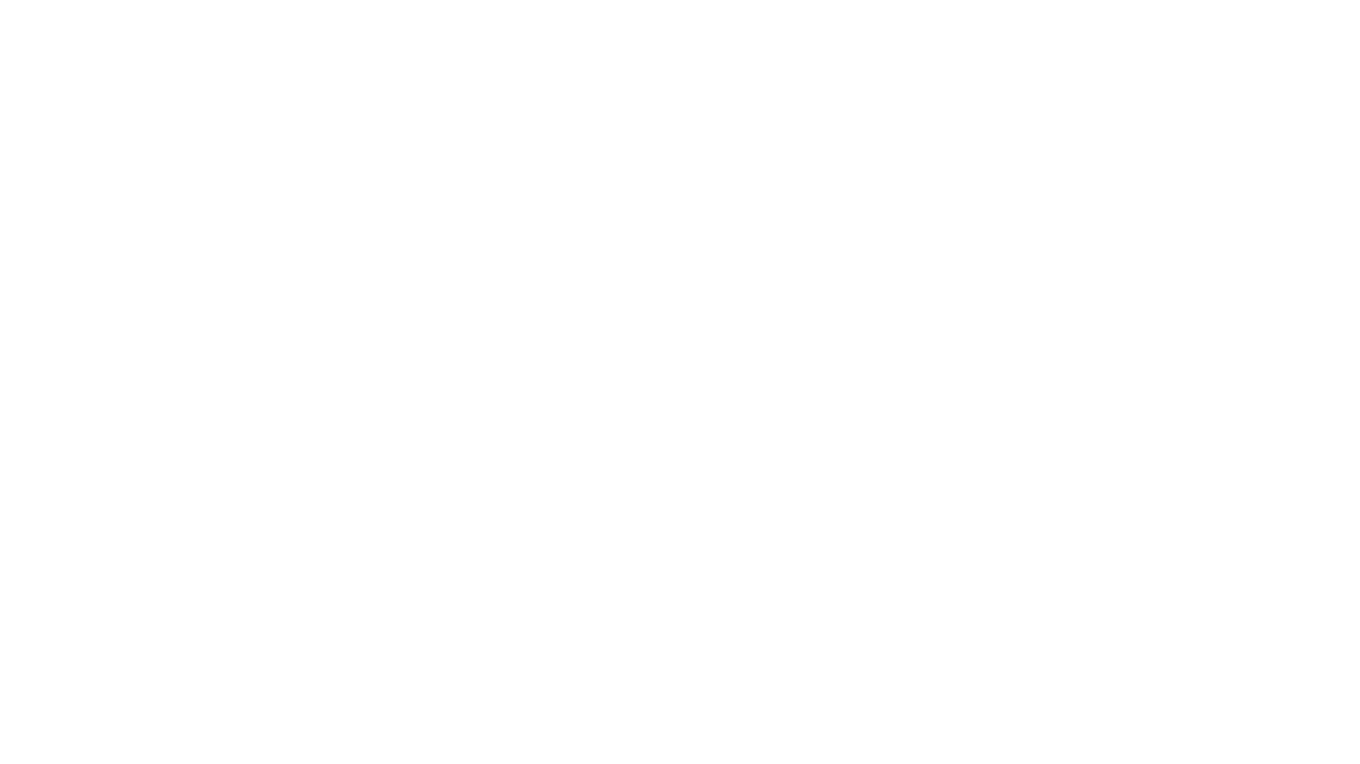
ebook2audiobook
ebook2audiobook is a CPU/GPU converter tool that converts eBooks to audiobooks with chapters and metadata using tools like Calibre, ffmpeg, XTTSv2, and Fairseq. It supports voice cloning and a wide range of languages. The tool is designed to run on 4GB RAM and provides a new v2.0 Web GUI interface for user-friendly interaction. Users can convert eBooks to text format, split eBooks into chapters, and utilize high-quality text-to-speech functionalities. Supported languages include Arabic, Chinese, English, French, German, Hindi, and many more. The tool can be used for legal, non-DRM eBooks only and should be used responsibly in compliance with applicable laws.
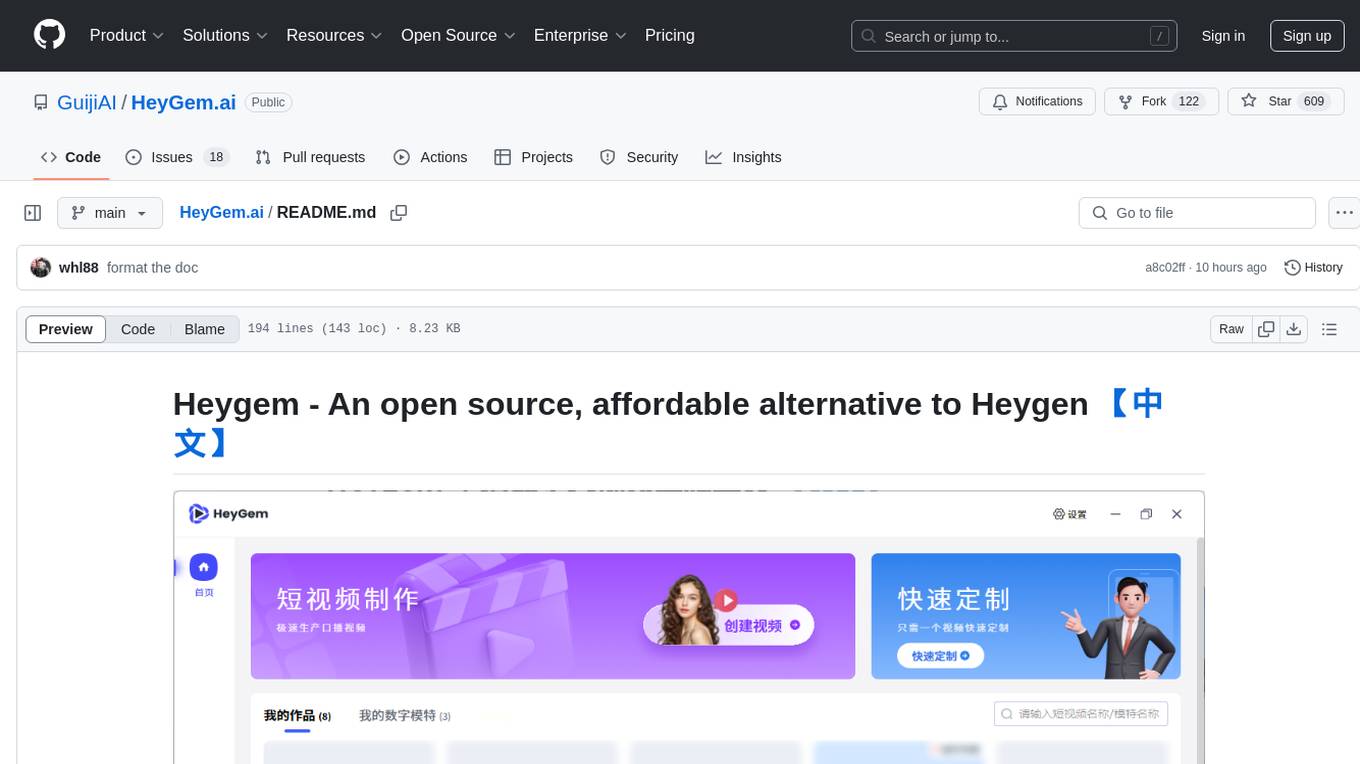
HeyGem.ai
Heygem is an open-source, affordable alternative to Heygen, offering a fully offline video synthesis tool for Windows systems. It enables precise appearance and voice cloning, allowing users to digitalize their image and drive virtual avatars through text and voice for video production. With core features like efficient video synthesis and multi-language support, Heygem ensures a user-friendly experience with fully offline operation and support for multiple models. The tool leverages advanced AI algorithms for voice cloning, automatic speech recognition, and computer vision technology to enhance the virtual avatar's performance and synchronization.
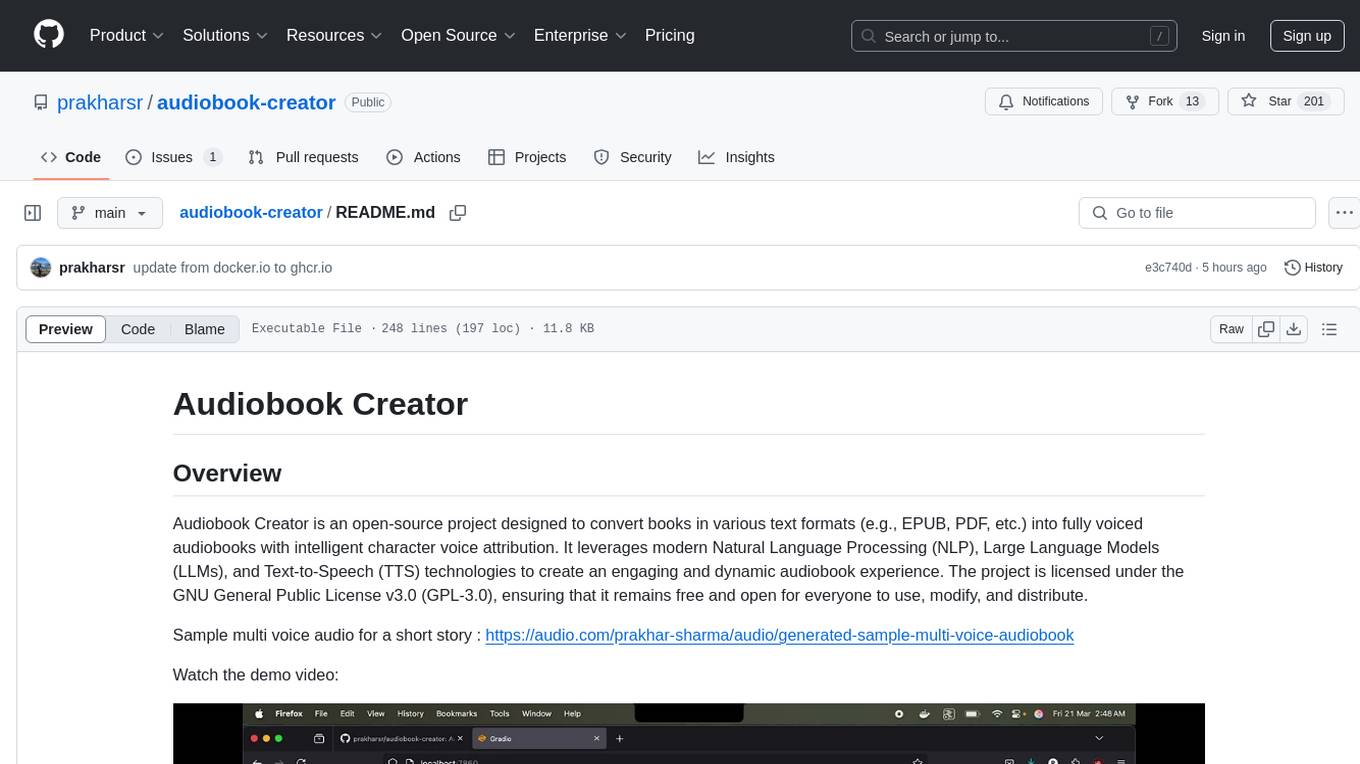
audiobook-creator
Audiobook Creator is an open-source tool that converts books in various text formats into fully voiced audiobooks with intelligent character voice attribution. It utilizes NLP, LLMs, and TTS technologies to provide an engaging audiobook experience. The project includes components for text cleaning and formatting, character identification, and audiobook generation. Key features include a Gradio UI app, M4B audiobook creation, multi-format support, Docker compatibility, customizable narration, progress tracking, and open-source licensing.
For similar jobs
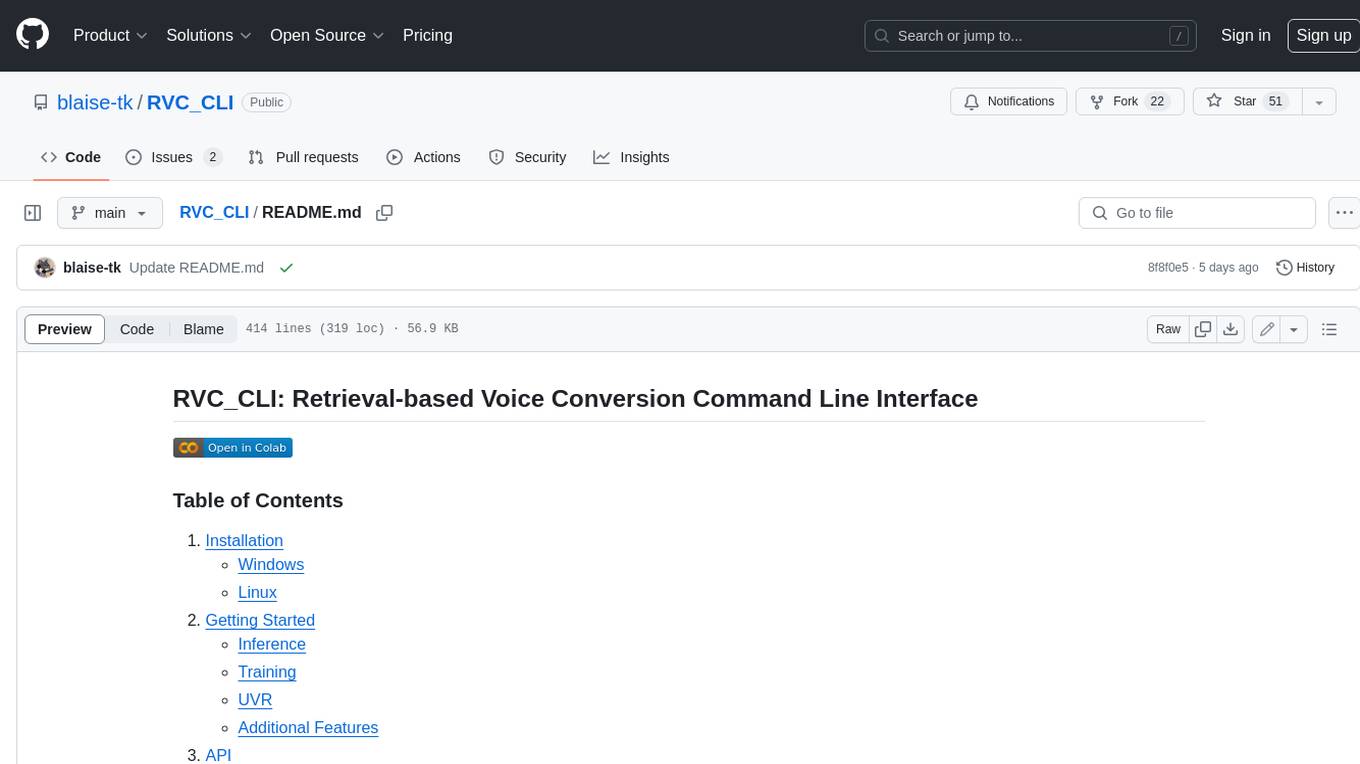
RVC_CLI
**RVC_CLI: Retrieval-based Voice Conversion Command Line Interface** This command-line interface (CLI) provides a comprehensive set of tools for voice conversion, enabling you to modify the pitch, timbre, and other characteristics of audio recordings. It leverages advanced machine learning models to achieve realistic and high-quality voice conversions. **Key Features:** * **Inference:** Convert the pitch and timbre of audio in real-time or process audio files in batch mode. * **TTS Inference:** Synthesize speech from text using a variety of voices and apply voice conversion techniques. * **Training:** Train custom voice conversion models to meet specific requirements. * **Model Management:** Extract, blend, and analyze models to fine-tune and optimize performance. * **Audio Analysis:** Inspect audio files to gain insights into their characteristics. * **API:** Integrate the CLI's functionality into your own applications or workflows. **Applications:** The RVC_CLI finds applications in various domains, including: * **Music Production:** Create unique vocal effects, harmonies, and backing vocals. * **Voiceovers:** Generate voiceovers with different accents, emotions, and styles. * **Audio Editing:** Enhance or modify audio recordings for podcasts, audiobooks, and other content. * **Research and Development:** Explore and advance the field of voice conversion technology. **For Jobs:** * Audio Engineer * Music Producer * Voiceover Artist * Audio Editor * Machine Learning Engineer **AI Keywords:** * Voice Conversion * Pitch Shifting * Timbre Modification * Machine Learning * Audio Processing **For Tasks:** * Convert Pitch * Change Timbre * Synthesize Speech * Train Model * Analyze Audio
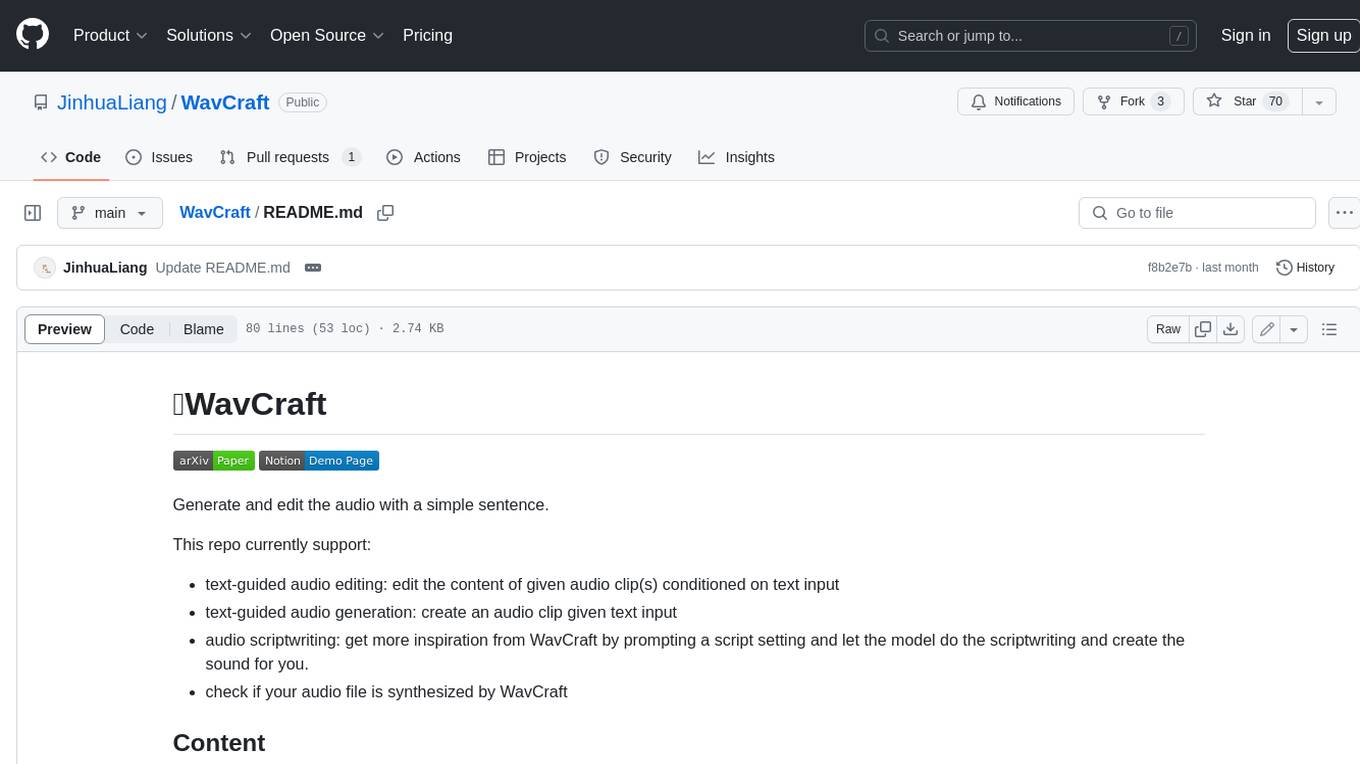
WavCraft
WavCraft is an LLM-driven agent for audio content creation and editing. It applies LLM to connect various audio expert models and DSP function together. With WavCraft, users can edit the content of given audio clip(s) conditioned on text input, create an audio clip given text input, get more inspiration from WavCraft by prompting a script setting and let the model do the scriptwriting and create the sound, and check if your audio file is synthesized by WavCraft.
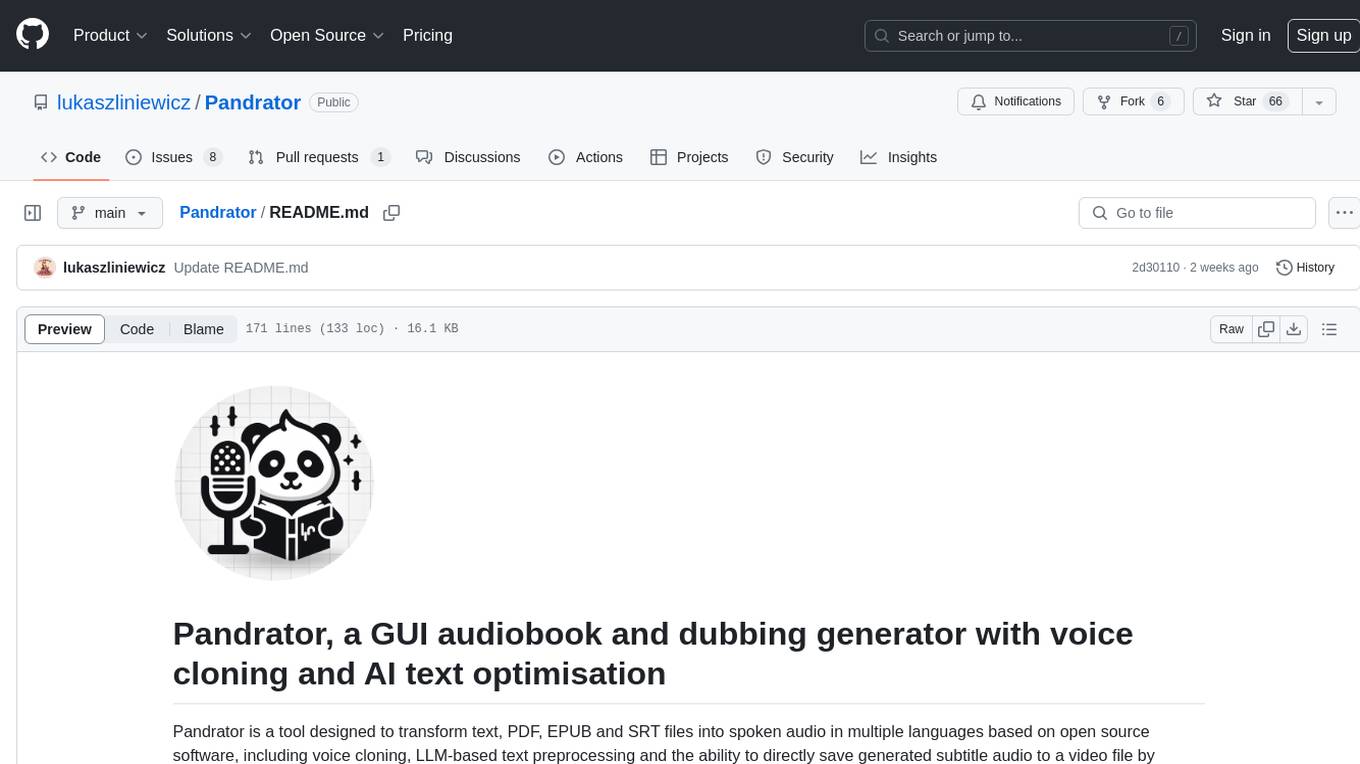
Pandrator
Pandrator is a GUI tool for generating audiobooks and dubbing using voice cloning and AI. It transforms text, PDF, EPUB, and SRT files into spoken audio in multiple languages. It leverages XTTS, Silero, and VoiceCraft models for text-to-speech conversion and voice cloning, with additional features like LLM-based text preprocessing and NISQA for audio quality evaluation. The tool aims to be user-friendly with a one-click installer and a graphical interface.
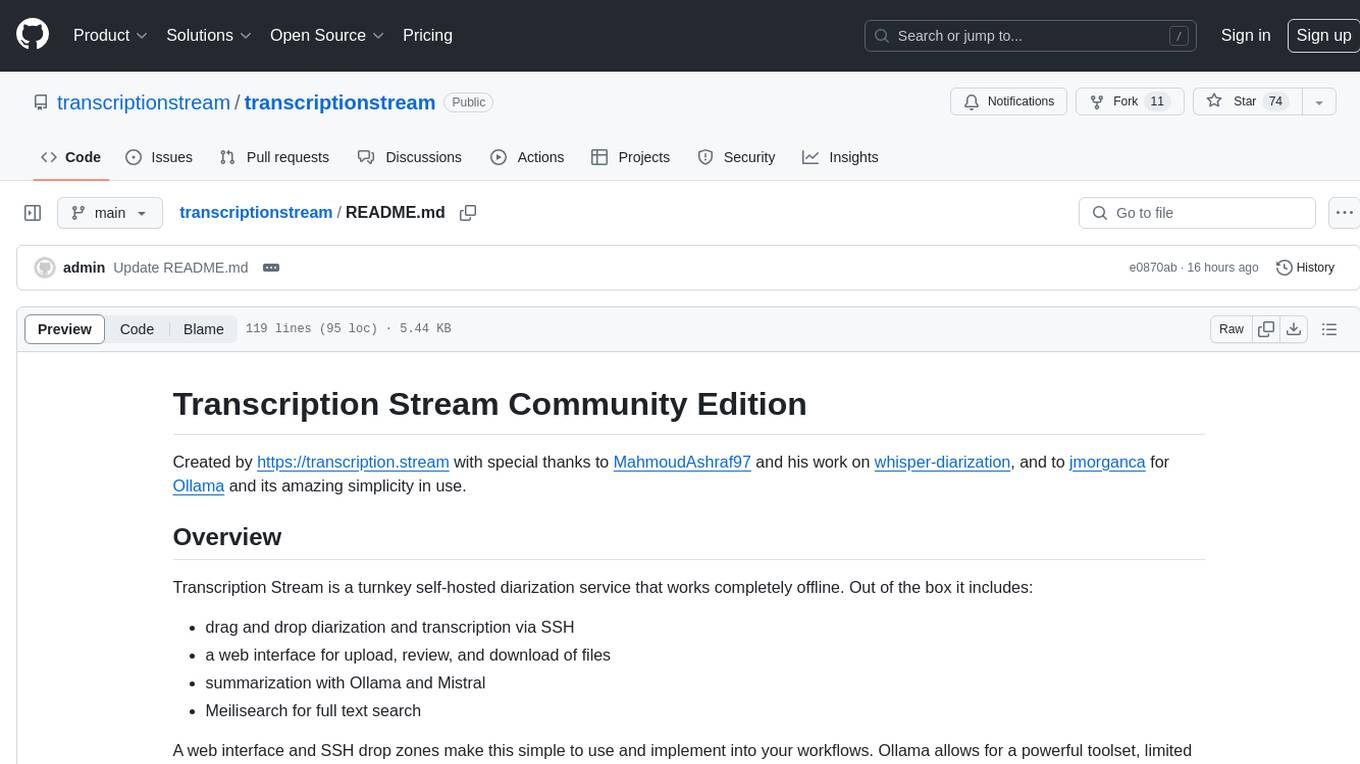
transcriptionstream
Transcription Stream is a self-hosted diarization service that works offline, allowing users to easily transcribe and summarize audio files. It includes a web interface for file management, Ollama for complex operations on transcriptions, and Meilisearch for fast full-text search. Users can upload files via SSH or web interface, with output stored in named folders. The tool requires a NVIDIA GPU and provides various scripts for installation and running. Ports for SSH, HTTP, Ollama, and Meilisearch are specified, along with access details for SSH server and web interface. Customization options and troubleshooting tips are provided in the documentation.
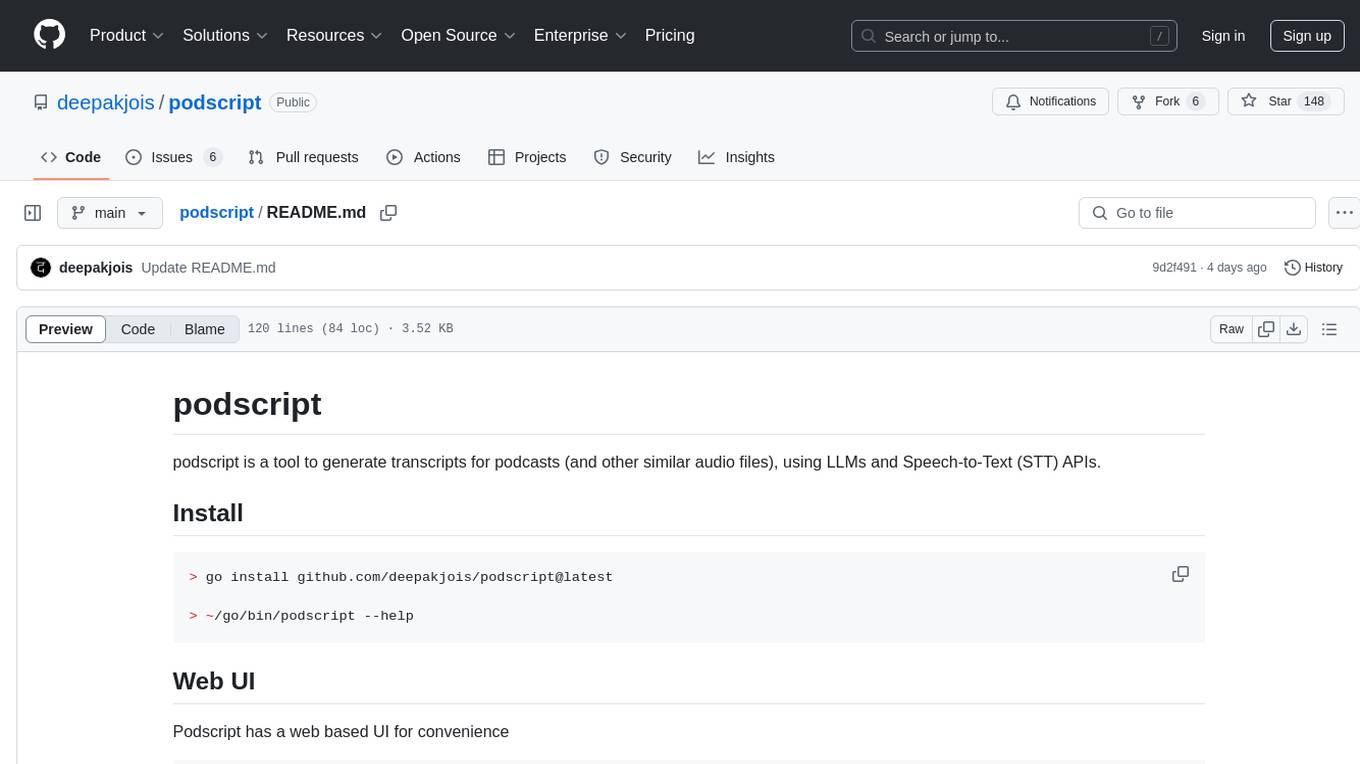
podscript
Podscript is a tool designed to generate transcripts for podcasts and similar audio files using Language Model Models (LLMs) and Speech-to-Text (STT) APIs. It provides a command-line interface (CLI) for transcribing audio from various sources, including YouTube videos and audio files, using different speech-to-text services like Deepgram, Assembly AI, and Groq. Additionally, Podscript offers a web-based user interface for convenience. Users can configure keys for supported services, transcribe audio, and customize the transcription models. The tool aims to simplify the process of creating accurate transcripts for audio content.
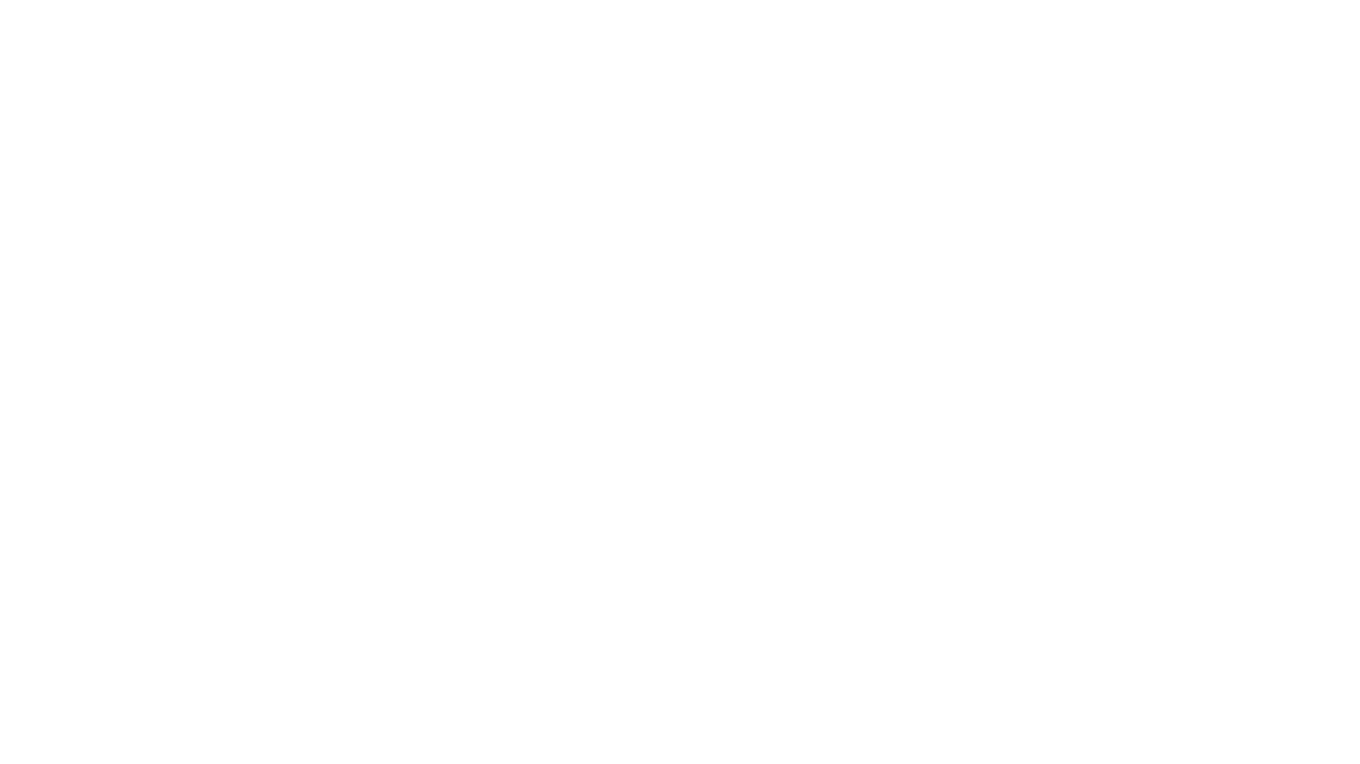
ebook2audiobook
ebook2audiobook is a CPU/GPU converter tool that converts eBooks to audiobooks with chapters and metadata using tools like Calibre, ffmpeg, XTTSv2, and Fairseq. It supports voice cloning and a wide range of languages. The tool is designed to run on 4GB RAM and provides a new v2.0 Web GUI interface for user-friendly interaction. Users can convert eBooks to text format, split eBooks into chapters, and utilize high-quality text-to-speech functionalities. Supported languages include Arabic, Chinese, English, French, German, Hindi, and many more. The tool can be used for legal, non-DRM eBooks only and should be used responsibly in compliance with applicable laws.
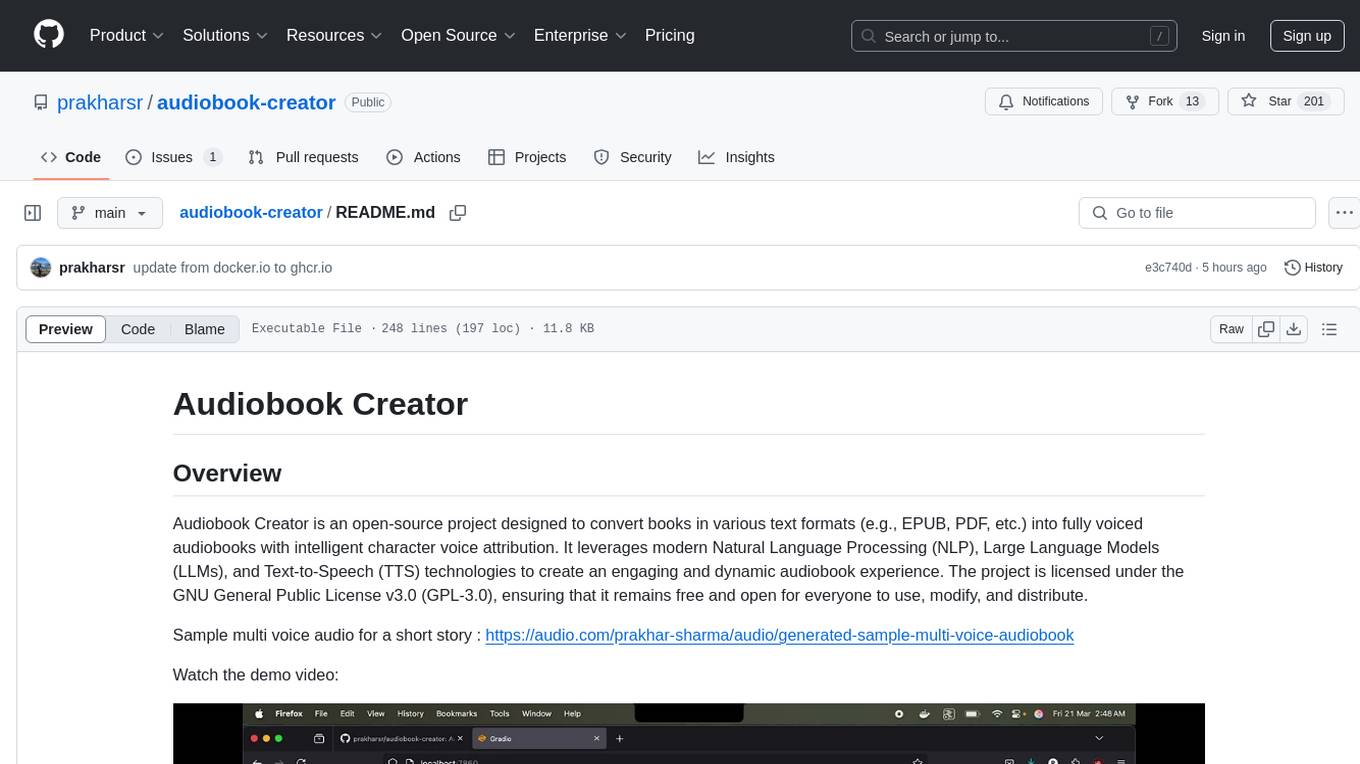
audiobook-creator
Audiobook Creator is an open-source tool that converts books in various text formats into fully voiced audiobooks with intelligent character voice attribution. It utilizes NLP, LLMs, and TTS technologies to provide an engaging audiobook experience. The project includes components for text cleaning and formatting, character identification, and audiobook generation. Key features include a Gradio UI app, M4B audiobook creation, multi-format support, Docker compatibility, customizable narration, progress tracking, and open-source licensing.
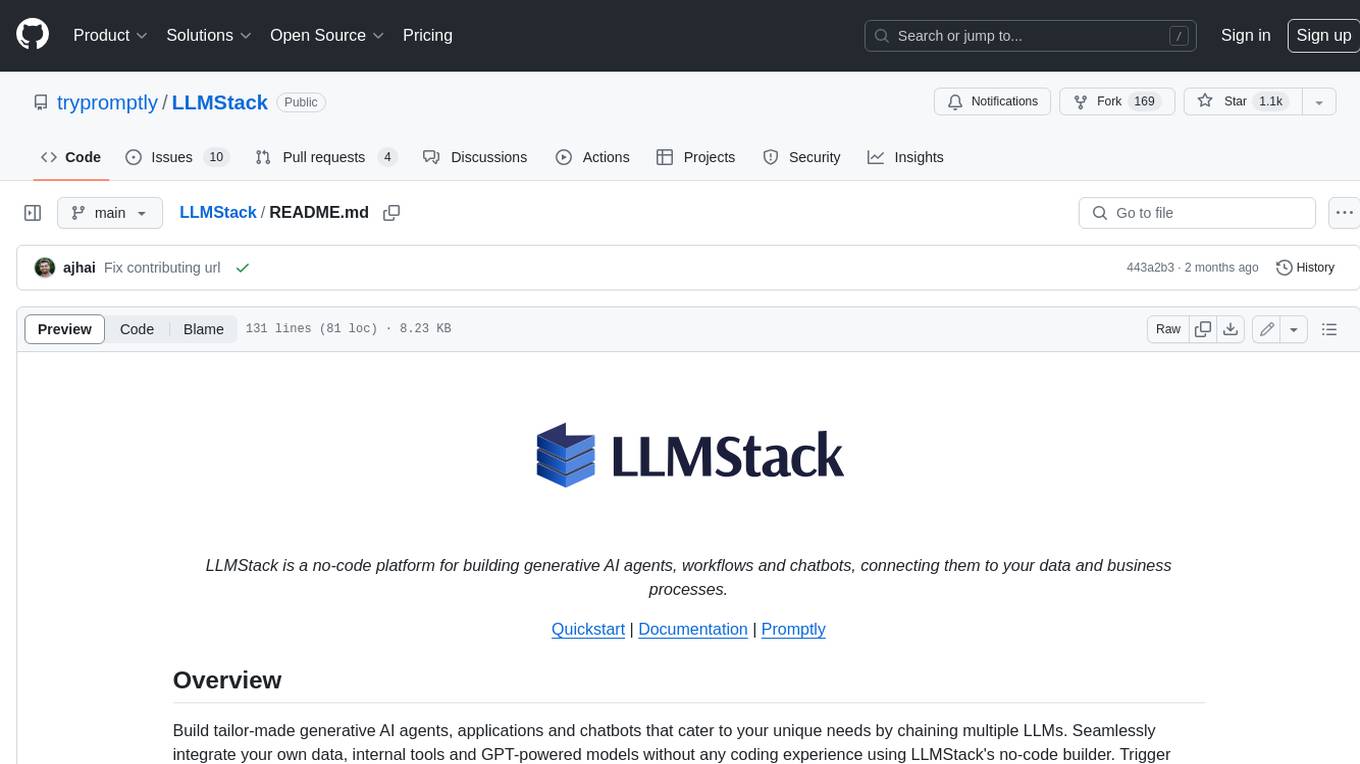
LLMStack
LLMStack is a no-code platform for building generative AI agents, workflows, and chatbots. It allows users to connect their own data, internal tools, and GPT-powered models without any coding experience. LLMStack can be deployed to the cloud or on-premise and can be accessed via HTTP API or triggered from Slack or Discord.