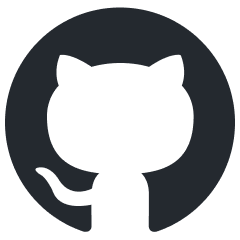
vasttools
My swiftsknife for vast.ai service
Stars: 124
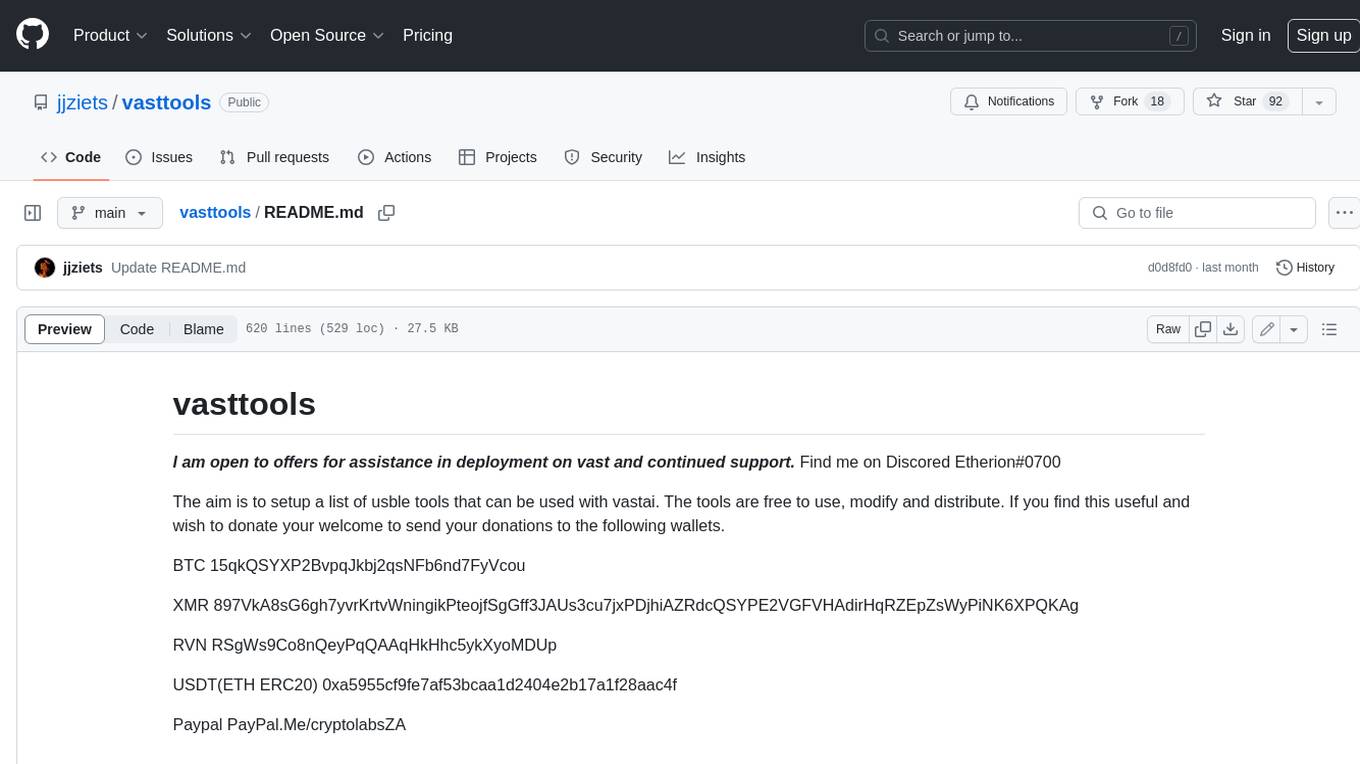
This repository contains a collection of tools that can be used with vastai. The tools are free to use, modify and distribute. If you find this useful and wish to donate your welcome to send your donations to the following wallets. BTC 15qkQSYXP2BvpqJkbj2qsNFb6nd7FyVcou XMR 897VkA8sG6gh7yvrKrtvWningikPteojfSgGff3JAUs3cu7jxPDjhiAZRdcQSYPE2VGFVHAdirHqRZEpZsWyPiNK6XPQKAg RVN RSgWs9Co8nQeyPqQAAqHkHhc5ykXyoMDUp USDT(ETH ERC20) 0xa5955cf9fe7af53bcaa1d2404e2b17a1f28aac4f Paypal PayPal.Me/cryptolabsZA
README:
The aim is to set up a list of tools that can be used with Vastai. The tools are free to use, modify and distribute. If you find this helpful and would like to donate, you can send your donations to the following wallets.
BTC 15qkQSYXP2BvpqJkbj2qsNFb6nd7FyVcou
XMR 897VkA8sG6gh7yvrKrtvWningikPteojfSgGff3JAUs3cu7jxPDjhiAZRdcQSYPE2VGFVHAdirHqRZEpZsWyPiNK6XPQKAg
RVN RSgWs9Co8nQeyPqQAAqHkHhc5ykXyoMDUp
USDT(ETH ERC20) 0xa5955cf9fe7af53bcaa1d2404e2b17a1f28aac4f
Paypal PayPal.Me/cryptolabsZA
- Host install guide for vast
- Self-verification test
- Speedtest-cli fix for vast
- Analytics dashboard
- Monitor your Nvidia 3000/4000 Core, GPU Hotspot and Vram temps
- nvml-error-when-using-ubuntu-22-and-24
- Remove Pressitent red error messages
- Memory oc
- OC monitor
- Stress testing GPUs on vast with Python benchmark of RTX3090's
- Telegram-Vast-Uptime-Bot
- Auto update the price for host listing based on mining profits
- Background job or idle job for vast
- Setting fan speeds if you have a headless system
- Remove unattended-upgrades package
- How to update a host
- How to move your vast docker driver to another drive
- Backup varlibdocker to another machine on your network
- Connecting to running instance with VNC to see applications GUI
- Setting up 3D accelerated desktop in web browser on vastai
- Useful commands
- How to set up a docker registry for the systems on your network
#Start with a clean install of ubuntu 22.04.x HWE Kernal server. Just add openssh.
sudo apt update && sudo apt upgrade -y && sudo apt dist-upgrade -y && sudo apt install update-manager-core -y
#if you did not install HWE kernal do the following
sudo apt install --install-recommends linux-generic-hwe-22.04 -y
sudo reboot
#install the drivers.
sudo apt install build-essential -y
sudo add-apt-repository ppa:graphics-drivers/ppa -y
sudo apt update
# to search for available NVIDIA drivers: use this command
sudo apt search nvidia-driver | grep nvidia-driver | sort -r
sudo apt install nvidia-driver-560 -y # assuming the latest is 560
#Remove unattended-upgrades Package so that the dirver don't upgrade when you have clients
sudo apt purge --auto-remove unattended-upgrades -y
sudo systemctl disable apt-daily-upgrade.timer
sudo systemctl mask apt-daily-upgrade.service
sudo systemctl disable apt-daily.timer
sudo systemctl mask apt-daily.service
# This is needed to remove xserver and genome if you started with ubunut desktop. clients can't run a desktop gui in an continer wothout if you have a xserver.
bash -c 'sudo apt-get update; sudo apt-get -y upgrade; sudo apt-get install -y libgtk-3-0; sudo apt-get install -y xinit; sudo apt-get install -y xserver-xorg-core; sudo apt-get remove -y gnome-shell; sudo update-grub; sudo nvidia-xconfig -a --cool-bits=28 --allow-empty-initial-configuration --enable-all-gpus'
#if Ubuntu is installed to a SSD and you plan to have the vast client data stored on a nvme follow the below instructions.
#WARRNING IF YOUR OS IS ON /dev/nvme0n1 IT WILL BE WIPED. CHECK TWICE change this device to the intended device name that you pan to use.
# this is one command that will create the xfs partion and write it to the disk /dev/nvme0n1.
echo -e "n\n\n\n\n\n\nw\n" | sudo cfdisk /dev/nvme0n1 && sudo mkfs.xfs /dev/nvme0n1p1
sudo mkdir /var/lib/docker
#I added discard so that the ssd is trimeds by ubunut and nofail if there is some problem with the drive the system will still boot.
sudo bash -c 'uuid=$(sudo xfs_admin -lu /dev/nvme0n1p1 | sed -n "2p" | awk "{print \$NF}"); echo "UUID=$uuid /var/lib/docker/ xfs rw,auto,pquota,discard,nofail 0 0" >> /etc/fstab'
sudo mount -a
# check that /dev/nvme0n1p1 is mounted to /var/lib/docker/
df -h
#this will enable Persistence mode on reboot so that the gpus can go to idle power when not used
sudo bash -c '(crontab -l; echo "@reboot nvidia-smi -pm 1" ) | crontab -'
#run the install command for vast
sudo apt install python3 -y
sudo wget https://console.vast.ai/install -O install; sudo python3 install YourKey; history -d $((HISTCMD-1));
nano /etc/default/grub # find the GRUB_CMDLINE_LINUX="" and ensure it looks like this.
GRUB_CMDLINE_LINUX="amd_iommu=on nvidia_drm.modeset=0 systemd.unified_cgroup_hierarchy=false"
#only run this command if you plan to support VM's on your machines. read vast guide to understand more https://vast.ai/docs/hosting/vms
sudo bash -c 'sed -i "/^GRUB_CMDLINE_LINUX=\"\"/s/\"\"/\"amd_iommu=on nvidia_drm.modeset=0\"/" /etc/default/grub && update-grub'
update-grub
#if you get nvml error then run this
sudo wget https://raw.githubusercontent.com/jjziets/vasttools/main/nvml_fix.py
sudo python3 nvml_fix.py
sudo reboot
#follow the Configure Networking instructions as per https://console.vast.ai/host/setup
#test the ports with running sudo nc -l -p port on the host machine and use https://portchecker.co to verify
sudo bash -c 'echo "40000-40019" > /var/lib/vastai_kaalia/host_port_range'
sudo reboot
#After reboot, check that the drive is mounted to /var/lib/docker and that your systems show up on the vast dashboard.
df -h # look for /var/lib/docker mount
sudo systemctl status vastai
sudo systemctl status docker
You can run the following test to ensure your new machine will be on the shortlist for verification testing. If you pass, there is a high chance that your machine will be eligible for verification. Take not that your router need to allow loopback if you run this from a machine on the same network as the machine you want to test. If you do not know how to enable loopback it will be better to run this on a VM from a cloud provider or with mobile connection to your pc.
The autoverify_machineid.sh
script is part of a suite of tools designed to automate the testing of machines on the Vast.ai marketplace. This script specifically tests a single machine to determine if it meets the minimum requirements necessary for further verification.
Before you start using ./autoverify_machineid.sh
, ensure you have the following:
- Vast.ai Command Line Interface (vastcli): This tool is used to interact with the Vast.ai platform.
- Vast.ai Listing: The machine should be listed on the vast marketplace.
- Ubuntu OS: The scripts are designed to run on Ubununt 20.04 or newer.
-
Download and Setup
vastcli
:-
Download the Vast.ai CLI tool using the following command:
wget https://raw.githubusercontent.com/vast-ai/vast-python/master/vast.py -O vast chmod +x vast
-
Set your Vast.ai API key:
./vast set api-key 6189d1be9f15ad2dced0ac4e3dfd1f648aeb484d592e83d13aaf50aee2d24c07
-
-
Download autoverify_machineid.sh:
- Use wget to download autoverify_machineid.sh to your local machine:
wget https://github.com/jjziets/VastVerification/releases/download/0.4-beta/autoverify_machineid.sh
- Use wget to download autoverify_machineid.sh to your local machine:
-
Make Scripts Executable:
- Change the permissions of the main scripts to make them executable:
chmod +x autoverify_machineid.sh
- Change the permissions of the main scripts to make them executable:
-
Dependencies
- Run the the following to install the required packages
apt update apt install bc jq
-
Check Machine Requirements:
- The
./autoverify_machineid.sh
script is designed to test if a single machine meets the minimum requirements for verification. This is useful for hosts who want to verify their own machines. - To test a specific machine by its
machine_id
, use the following command:Replace./autoverify_machineid.sh <machine_id>
<machine_id>
with the actual ID of the machine you want to test.
- The
-
To Ignore Requirements Check:
./autoverify_machineid.sh --ignore-requirements <machine_id>
This command runs the tests for the machine, regardless of whether it meets the minimum requirements.
-
Progress and Results Logging:
- The script logs the progress and results of the tests.
- Successful results and machines that pass the requirements will be logged in
Pass_testresults.log
. - Machines that do not meet the requirements or encounter errors during testing will be logged in
Error_testresults.log
.
-
Understanding the Logs:
-
Pass_testresults.log
: This file contains entries for machines that successfully passed all tests. -
Error_testresults.log
: This file contains entries for machines that failed to meet the minimum requirements or encountered errors during testing.
-
Here’s how you can run the autoverify_machineid.sh
script to test a machine with machine_id
10921:
./autoverify_machineid.sh 10921
-
API Key Issues: Ensure your API key is correctly set using
./vast set api-key <your-api-key>
. -
Permission Denied: If you encounter permission issues, make sure the script files have executable permissions (
chmod +x <script_name>
). - Connection Issues: Verify your network connection and ensure the Vast.ai CLI can communicate with the Vast.ai servers.
By following this guide, you will be able to use the ./autoverify_machineid.sh
script to test individual machines on the Vast.ai marketplace. This process helps ensure that machines meet the required specifications for GPU and system performance, making them candidates for further verification and use in the marketplace.
If you are having problems with your machine not showing its upload and download speed correctly.
first check if there is a problem by forcing the speedtest to run
cd /var/lib/vastai_kaalia
./send_mach_info.py --speedtest
output should look like this
2024-10-03 08:50:04.587469
os version
running df
checking errors
nvidia-smi
560035003
/usr/bin/fio
checking speedtest
/usr/bin/speedtest
speedtest
running speedtest on random server id 19897
{"type":"result","timestamp":"2024-10-03T08:50:24Z","ping":{"jitter":0.243,"latency":21.723,"low":21.526,"high":22.047},"download":{"bandwidth":116386091,"bytes":1010581968,"elapsed":8806,"latency":{"iqm":22.562,"low":20.999,"high":296.975,"jitter":3.976}},"upload":{"bandwidth":116439919,"bytes":980885877,"elapsed":8508,"latency":{"iqm":36.457,"low":6.852,"high":349.495,"jitter":34.704}},"packetLoss":0,"isp":"Vox Telecom","interface":{"internalIp":"192.168.1.101","name":"bond0","macAddr":"F2:6A:67:0C:85:8B","isVpn":false,"externalIp":"41.193.204.66"},"server":{"id":19897,"host":"speedtest.wibernet.co.za","port":8080,"name":"Wibernet","location":"Cape Town","country":"South Africa","ip":"102.165.64.110"},"result":{"id":"18bb02e4-466d-43dd-b1fc-3f106319a9f6","url":"https://www.speedtest.net/result/c/18bb02e4-466d-43dd-b1fc-3f106319a9f6","persisted":true}}
....
If the above speedtest does not work, you can try to install an alternative newer one. Due to the newer speed test output not having the same format, a script will translate it so that vast can use the new speed test. All the commands combined
bash -c "sudo apt-get install curl -y && sudo curl -s https://packagecloud.io/install/repositories/ookla/speedtest-cli/script.deb.sh | sudo bash && sudo apt-get install speedtest -y && sudo apt install python3 -y && cd /var/lib/vastai_kaalia/latest && sudo mv speedtest-cli speedtest-cli.old && sudo wget -O speedtest-cli https://raw.githubusercontent.com/jjziets/vasttools/main/speedtest-cli.py && sudo chmod +x speedtest-cli"
or step by step
sudo apt-get install curl
sudo curl -s https://packagecloud.io/install/repositories/ookla/speedtest-cli/script.deb.sh | sudo bash
sudo apt-get install speedtest -y
sudo apt install python3 -y
cd /var/lib/vastai_kaalia/latest
sudo mv speedtest-cli speedtest-cli.old
sudo wget -O speedtest-cli https://raw.githubusercontent.com/jjziets/vasttools/main/speedtest-cli.py
sudo chmod +x speedtest-cli
This updated your speed test to the newer one and translated the output so that Vast Demon can use it. If you now get slower speeds, follow this
## If migrating from prior bintray install instructions please first...
# sudo rm /etc/apt/sources.list.d/speedtest.list
# sudo apt-get update
# sudo apt-get remove speedtest -y
## Other non-official binaries will conflict with Speedtest CLI
# Example how to remove using apt-get
# sudo apt-get remove speedtest-cli
sudo apt-get install curl
curl -s https://packagecloud.io/install/repositories/ookla/speedtest-cli/script.deb.sh | sudo bash
sudo apt-get install speedtest
Prometheus Grafana monitoring systems, send alerts and track all metrics regarding your equipment and also track earnings and rentals. https://github.com/jjziets/DCMontoring
run the script below if you have a problem with vast installer on 22,24 and nvml error this script is based on Bo26fhmC5M so credit go to him
sudo wget https://raw.githubusercontent.com/jjziets/vasttools/main/nvml_fix.py
sudo python nvml_fix.py
if you have a red error message on your machine that you have confirmed has been addressed. It might help to delete /var/lib/vastai_kaalia/kaalia.log and reboot
sudo rm /var/lib/vastai_kaalia/kaalia.log
sudo systemctl restart vastai
If you do not want to setup the Analytics dashboard and you just want to see all the Temps on your gpu then you can use the below tool
sudo wget https://github.com/jjziets/gddr6_temps/raw/master/nvml_direct_access
sudo chmod +x nvml_direct_access
sudo ./nvml_direct_access
set the OC of the RTX 3090 It requires the following
on the host run the following command:
sudo apt-get install libgtk-3-0 && sudo apt-get install xinit && sudo apt-get install xserver-xorg-core && sudo update-grub && sudo nvidia-xconfig -a --cool-bits=28 --allow-empty-initial-configuration --enable-all-gpus
wget https://raw.githubusercontent.com/jjziets/vasttools/main/set_mem.sh
sudo chmod +x set_mem.sh
sudo ./set_mem.sh 2000 # this will set the memory OC to +1000mhs on all the gpus. You can use 3000 on some gpu's which will give 1500mhs OC.
setup the monitoring program that will change the memory oc based on what programe is running. it designed for RTX3090's and targets ethminer at this stage. It requires both set_mem.sh and ocmonitor.sh to run in the root.
wget https://raw.githubusercontent.com/jjziets/vasttools/main/ocminitor.sh
sudo chmod +x ocminitor.sh
sudo ./ocminitor.sh # I suggest running this in tmux or screen so that when you close the ssh connetion. It looks for ethminer and if it finds it it will set the oc based on your choice. you can also set powerlimits with nvidia-smi -pl 350
To load at reboot use the crontab below
sudo (crontab -l; echo "@reboot screen -dmS ocmonitor /home/jzietsman/ocminitor.sh") | crontab - #replace the user with your user
Mining does not stress your system the same as python work loads do, so this is a good test to run as well.
First, set a maintenance window, and then once you have no clients running, you can do the stress testing.
https://github.com/jjziets/pytorch-benchmark-volta
a full suite of stress tests can be found docker image jjziets/vastai-benchmarks:latest in folder /app/
stress-ng - CPU stress
stress-ng - Drive stress
stress-ng - Memory stress
sysbench - Memory latency and speed benchmark
dd - Drive speed benchmark
Hashcat - Benchmark
bandwithTest - GPU bandwith benchmark
pytorch - Pytorch DL benchmark
#test or bash interface
sudo docker run --shm-size 1G --rm -it --gpus all jjziets/vastai-benchmarks /bin/bash
apt update && apt upgrade -y
./benchmark.sh
#Run using default settings Results are saved to ./output.
sudo docker run -v ${PWD}/output:/app/output --shm-size 1G --rm -it --gpus all jjziets/vastai-benchmarks
Run with params SLEEP_TIME/BENCH_TIME
sudo docker run -v ${PWD}/output:/app/output --shm-size 1G --rm -it -e SLEEP_TIME=2 -e BENCH_TIME=2 --gpus all jjziets/vastai-benchmarks
You can also do a GPU burn test.
sudo docker run --gpus all --rm oguzpastirmaci/gpu-burn <test duration in seconds>
If you want to run it for one GPU, run the command below, replacing the x with the GPU number starting at 0.
sudo docker run --gpus '"device=x"' --rm oguzpastirmaci/gpu-burn <test duration in seconds>
*based on leona / vast.ai-tools
This is a set of scripts for monitoring machine crashes. Run the client on your vast machine and the server on a remote one. You get notifications on Telegram if no heartbeats are sent within the timeout (default 12 seconds). https://github.com/jjziets/Telegram-Vast-Uptime-Bot
based on RTX 3090 120Mhs for eth. it sets the price of my 2 host. it works with a custom Vast-cli which can be found here https://github.com/jjziets/vast-python/blob/master/vast.py The manager is here https://github.com/jjziets/vasttools/blob/main/setprice.sh
This should be run on a vps not on a host. do not expose your Vast API keys by using it on the host.
wget https://github.com/jjziets/vast-python/blob/master/vast.py
sudo chmod +x vast.py
./vast.py set api-key UseYourVasset
wget https://github.com/jjziets/vasttools/blob/main/setprice.sh
sudo chmod +x setprice.sh
The best way to manage your idle job is via the vast cli. To my knowledge, the GUI set job is broken. So to set an idle job follow the following steps. You will need to download the vast cli and run the following commands. The idea is to rent yourself as an interruptible job. The vast cli allows you to set one idle job for all the GPUs or one GPU per instance. You can also set the SSH connection method or any other method. Go to https://cloud.vast.ai/cli/ and install your cli flavour.
setup your account key so that you can use the vast cli. you get this key from your account page.
./vast set api-key API_KEY
You can use my SetIdleJob.py scrip to setup your idle job based on the minimum price set on your machines.
wget https://raw.githubusercontent.com/jjziets/vasttools/main/SetIdleJob.py
Here is an example of how I mine to nicehash
python3 SetIdleJob.py --args 'env | grep _ >> /etc/environment; echo "starting up"; apt -y update; apt -y install wget; apt -y install libjansson4; apt -y install xz-utils; wget https://github.com/develsoftware/GMinerRelease/releases/download/3.44/gminer_3_44_linux64.tar.xz; tar -xvf gminer_3_44_linux64.tar.xz; while true; do ./miner --algo kawpow --server stratum+tcp://kawpow.auto.nicehash.com:9200 --user 3LNHVWvUEufL1AYcKaohxZK2P58iBHdbVH.${VAST_CONTAINERLABEL:2}; done'
Or the full command if you don't want to use the defaults
python3 SetIdleJob.py --image nvidia/cuda:12.4.1-runtime-ubuntu22.04 --disk 16 --args 'env | grep _ >> /etc/environment; echo "starting up"; apt -y update; apt -y install wget; apt -y install libjansson4; apt -y install xz-utils; wget https://github.com/develsoftware/GMinerRelease/releases/download/3.44/gminer_3_44_linux64.tar.xz; tar -xvf gminer_3_44_linux64.tar.xz; while true; do ./miner --algo kawpow --server stratum+tcp://kawpow.auto.nicehash.com:9200 --user 3LNHVWvUEufL1AYcKaohxZK2P58iBHdbVH.${VAST_CONTAINERLABEL:2}; done' --api-key b149b011a1481cd852b7a1cf1ccc9248a5182431b23f9410c1537fca063a68b1
Trouble shoot your bash -c command by using the logs on the instance page
Alternatively, you can rent yourself with the following command and then log in and load what you want to run. Make sure to add your process to onstart.sh to rent your self first find your machine with he machine id
./vast search offers "machine_id=14109 verified=any gpu_frac=1 " # gpu_frac=1 will give you the instance with all the gpus.
or
./vast search offers -i "machine_id=14109 verified=any min_bid>0.1 num_gpus=1" # it will give you the instance with one GPU
Once you have the offe_id. and in this case, the search with a -i switch will give you an interruptible instance_id
Let's assume you want to mine with lolminer
./vast create instance 9554646 --price 0.2 --image nvidia/cuda:12.0.1-devel-ubuntu20.04 --env '-p 22:22' --onstart-cmd 'bash -c "apt -y update; apt -y install wget; apt -y install libjansson4; apt -y install xz-utils; wget https://github.com/Lolliedieb/lolMiner-releases/releases/download/1.77b/lolMiner_v1.77b_Lin64.tar.gz; tar -xf lolMiner_v1.77b_Lin64.tar.gz -C ./; cd 1.77b; ./lolMiner --algo ETCHASH --pool etc.2miners.com:1010 --user 0xYour_Wallet_Goes_Here.VASTtest"' --ssh --direct --disk 100
it will start the instance on price 0.2.
./vast show instances # will give you the list of instance
./vast change bid 9554646 --price 0.3 # This will change the price to 0.3 for the instance
Here is a repo with two programs and a few scripts that you can use to manage your fans https://github.com/jjziets/GPU_FAN_OC_Manager/tree/main
bash -c "wget https://github.com/jjziets/GPU_FAN_OC_Manager/raw/main/set_fan_curve; chmod +x set_fan_curve; CURRENT_PATH=\$(pwd); nohup bash -c \"while true; do \$CURRENT_PATH/set_fan_curve 65; sleep 1; done\" > output.txt & (crontab -l; echo \"@reboot screen -dmS gpuManger bash -c 'while true; do \$CURRENT_PATH/set_fan_curve 65; sleep 1; done'\") | crontab -"
If your system updates while vast is running or even worse when a client is renting you then you might get de-verified or banned. It's advised to only update when the system is unrented and delisted. best would be to set an end date of your listing and conduct updates and upgrades at that stage. to stop unattended-upgrades run the following commands.
sudo apt purge --auto-remove unattended-upgrades -y
sudo systemctl disable apt-daily-upgrade.timer
sudo systemctl mask apt-daily-upgrade.service
sudo systemctl disable apt-daily.timer
sudo systemctl mask apt-daily.service
When the system is idle and delisted run the following commands. vast demon and docker services are stopped. It is also a good idea to upgrade Nvidia drivers like this. If you don't and the upgrades brakes a package you might get de-verifyed or even banned from vast.
bash -c ' sudo systemctl stop vastai; sudo systemctl stop docker.socket; sudo systemctl stop docker; sudo apt update; sudo apt upgrade -y; sudo systemctl start docker.socket ; sudo systemctl start docker; sudo systemctl start vastai'
This guide illustrates how to back up vastai Docker data from an existing drive and transfer it to a new drive . in this case a raid driver /dev/md0
- No clients are running and that you are un listed from the vast market.
- Docker data exists on the current drive.
-
Install required tools:
sudo apt install pv pixz
-
Stop and disable relevant services:
sudo systemctl stop vastai docker.socket docker sudo systemctl disable vastai docker.socket docker
-
Backup the Docker directory:
Create a compressed backup of the
/var/lib/docker
directory. Ensure there's enough space on the OS drive for this backup. Or move the data to backup server. see https://github.com/jjziets/vasttools/blob/main/README.md#backup-varlibdocker-to-another-machine-on-your-network
Note:sudo tar -c -I 'pixz -k -1' -f ./docker.tar.pixz /var/lib/docker | pv #you can change ./ to a destination directory
pixz
utilizes multiple cores for faster compression. -
Unmount the Docker directory:
If you're planning to shut down and install a new drive:
sudo umount /var/lib/docker
-
Update
/etc/fstab
: Disable auto-mounting of the current Docker directory at startup to prevent boot issues:
Comment out the line associated withsudo nano /etc/fstab
/var/lib/docker
by adding a#
at the start of the line. -
Partition the New Drive:
(Adjust the device name based on your system. The guide uses
/dev/md0
for RAID and/dev/nvme0n1
for NVMe drives as examples.)sudo cfdisk /dev/md0
-
Format the new partition with XFS:
sudo mkfs.xfs -f /dev/md0p1
-
Retrieve the UUID:
You'll need the UUID for updating
/etc/fstab
.sudo xfs_admin -lu /dev/md0p1
-
Update
/etc/fstab
with the New Drive:
Add the following line (replace the UUID with the one you retrieved):sudo nano /etc/fstab
UUID="YOUR_UUID_HERE" /var/lib/docker xfs rw,auto,pquota,discard,nofail 0 0
-
Mount the new partition:
Confirm the mount:sudo mount -a
Ensuredf -h
/dev/md0p1
(or the appropriate device name) is mounted to/var/lib/docker
. -
Restore the Docker data:
Navigate to the root directory:
Decompress and restore: Ensure to change the user to the relevent name
cd /
sudo cat /home/user/docker.tar.pixz | pv | sudo tar -x -I 'pixz -d -k'
-
Enable services:
sudo systemctl enable vastai docker.socket docker
-
Reboot:
sudo reboot
Check if the desired drive is mounted to /var/lib/docker
and ensure vastai
is operational.
If you're looking to migrate your Docker setup to another machine, whether for replacing the drive or setting up a RAID, follow this guide. For this example, we'll assume the backup server's IP address is 192.168.1.100
.
-
Temporarily Enable Root SSH Login:
It's essential to ensure uninterrupted SSH communication during the backup process, especially when transferring large files like compressed Docker data.
a. Open the SSH configuration:
b. Locate and change the line:sudo nano /etc/ssh/sshd_config
to:PermitRootLogin no
c. Reload the SSH configuration:PermitRootLogin yes
sudo systemctl restart sshd
-
Generate an SSH Key and Transfer it to the Backup Server:
a. Create the SSH key:
b. Copy the SSH key to the backup server:sudo ssh-keygen
sudo ssh-copy-id -i ~/.ssh/id_rsa [email protected]
-
Disable Root Password Authentication:
Ensure only the SSH key can be used for root login, enhancing security.
a. Modify the SSH configuration:
b. Change the line to:sudo nano /etc/ssh/sshd_config
c. Reload the SSH configuration:PermitRootLogin prohibit-password
sudo systemctl restart sshd
-
Preparation for Backup:
Before backing up, ensure relevant services are halted:
sudo systemctl stop docker.socket sudo systemctl stop docker sudo systemctl stop vastai sudo systemctl disable vastai sudo systemctl disable docker.socket sudo systemctl disable docker
-
Backup Procedure:
This procedure compresses the
/var/lib/docker
directory and transfers it to the backup server. a. Switch to the root user and install necessary tools:
It mght be a good idea to run the backup command in tmux or screen so that if you lose ssh connecton the process will finish. b. Perform the backup:sudo su apt install pixz apt install pv
tar -c -I 'pixz -k -0' -f - /var/lib/docker | pv | ssh [email protected] "cat > /mnt/backup/machine/docker.tar.pixz"
-
Restoring the Backup:
Make sure your new drive is mounted at
/var/lib/docker
. a. Switch to the root user:
b. Restore from the backup:sudo su
cd / ssh [email protected] "cat /mnt/backup/machine/docker.tar.pixz" | pv | sudo tar -x -I 'pixz -d -k'
-
Reactivate Services:
sudo systemctl enable vastai sudo systemctl enable docker.socket sudo systemctl enable docker sudo reboot
Post-reboot: Ensure your target drive is mounted to /var/lib/docker
and that vastai
is operational.
Using a instance with open ports If display is color depth is 16 not 16bit try another vnc viewer. TightVNC worked for me on windows
first tell vast to allow a port to be assinged. use the -p 8081:8081 and tick the direct command.
find a host with open ports and then rent it. preferbly on demand. go to the client instances page and wait for the connect button
use ssh to connect to the instances.
run the below commands. the second part can be placed in the onstart.sh to run on restart
bash -c 'apt-get update; apt-get -y upgrade; apt-get install -y x11vnc; apt-get install -y xvfb; apt-get install -y firefox;apt-get install -y xfce4;apt-get install -y xfce4-goodies'
export DISPLAY=:20
Xvfb :20 -screen 0 1920x1080x16 &
x11vnc -passwd TestVNC -display :20 -N -forever -rfbport 8081 &
startxfce4
To connect use the ip of the host and the port that was provided. In this case it is 400010
then enjoy the destkop. sadly this is not hardware accelarted. so no games will work
We will be using ghcr.io/ehfd/nvidia-glx-desktop:latest
use this env paramters
-e TZ=UTC -e SIZEW=1920 -e SIZEH=1080 -e REFRESH=60 -e DPI=96 -e CDEPTH=24 -e VIDEO_PORT=DFP -e PASSWD=mypasswd -e WEBRTC_ENCODER=nvh264enc -e BASIC_AUTH_PASSWORD=mypasswd -p 8080:8080
find a system that has open ports
username is user and password is what you set mypasswd in this case
3D accelerated desktop environment in a web browser
This will reduce the number of pull requests from your public IP. Docker is restricted to 100 pulls per 6h for unanonymous login, and it can speed up the startup time for your rentals. This guide provides instructions on how to set up a Docker registry server using Docker Compose, as well as configuring Docker clients to use this registry. Prerequisites Docker and Docker Compose are installed on the server that has a lot of fast storage on your local LAN. Docker is installed on all client machines.
Setting Up the Docker Registry Server install docker-compose if you have not already.
sudo su
curl -L "https://github.com/docker/compose/releases/download/v2.24.4/docker-compose-$(uname -s)-$(uname -m)" -o /usr/local/bin/docker-compose
chmod +x /usr/local/bin/docker-compose
ln -s /usr/local/bin/docker-compose /usr/bin/docker-compose
apt-get update && sudo apt-get install -y gettext-base
Create a docker-compose.yml file: Create a file named docker-compose.yml on your server with the following content:
version: '3'
services:
registry:
restart: unless-stopped
image: registry:2
ports:
- 5000:5000
environment:
- REGISTRY_PROXY_REMOTEURL=https://registry-1.docker.io
- REGISTRY_STORAGE_DELETE_ENABLED="true"
volumes:
- data:/var/lib/registry
volumes:
data:
This configuration sets up a Docker registry server running on port 5000 and uses a volume named data for storage. Start the Docker Registry:
Run the following command in the directory where your docker-compose.yml file is located:
sudo docker-compose up -d
This command will start the Docker registry in detached mode.
To configure Docker clients to use the registry, follow these steps on each client machine: Edit the Docker Daemon Configuration: Run the following command to add your Docker registry as a mirror in the Docker daemon configuration:
echo '{
"runtimes": {
"nvidia": {
"path": "nvidia-container-runtime",
"runtimeArgs": []
}
},
"registry-mirrors": ["http://192.168.100.7:5000"]
}' | sudo tee /etc/docker/daemon.json
If space is limisted you can run this cleanup task as a cron job
wget https://github.com/jjziets/vasttools/raw/main/cleanup-registry.sh
chmod +x cleanup-registry.sh
add this like to your corntab -e
0 * * * * /path/to/cleanup-registry.sh
replace /path/to/ with where the file is saved.
Replace 192.168.100.7:5000 with the IP address and port of your Docker registry server. Restart Docker Daemon:
sudo systemctl restart docker
Verifying the Setup To verify that the Docker registry is set up correctly, you can try pulling an image from the registry:
docker pull 192.168.100.7:5000/your-image
Replace 192.168.100.7:5000/your-image with the appropriate registry URL and image name.
"If you set up the vast CLI, you can enter this
./vast show machines | grep "current_rentals_running_on_demand"
if returns 0, then it's an interruptable rent.
Command on a host that provides logs of the deamon running
tail /var/lib/vastai_kaalia/kaalia.log -f
uninstall vast
wget https://s3.amazonaws.com/vast.ai/uninstall.py
sudo python uninstall.py
For Tasks:
Click tags to check more tools for each tasksFor Jobs:
Alternative AI tools for vasttools
Similar Open Source Tools
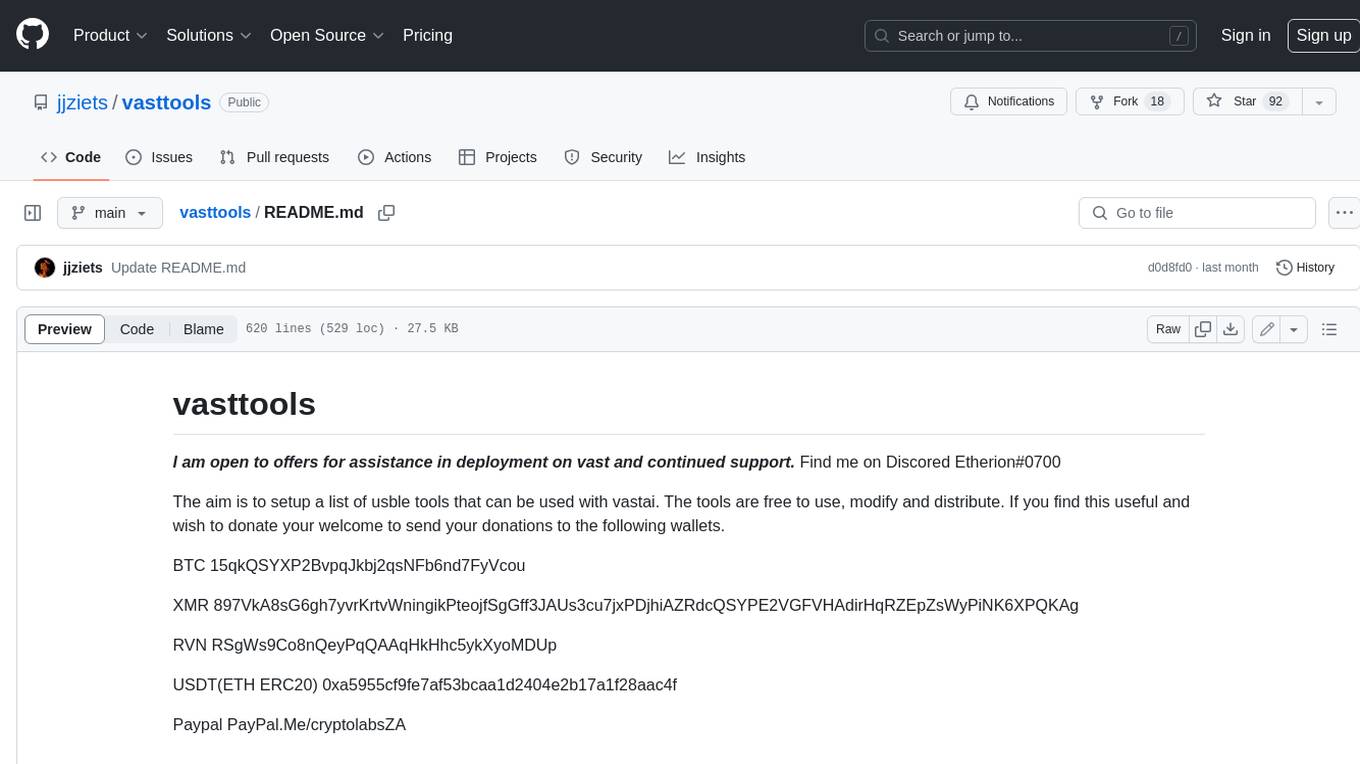
vasttools
This repository contains a collection of tools that can be used with vastai. The tools are free to use, modify and distribute. If you find this useful and wish to donate your welcome to send your donations to the following wallets. BTC 15qkQSYXP2BvpqJkbj2qsNFb6nd7FyVcou XMR 897VkA8sG6gh7yvrKrtvWningikPteojfSgGff3JAUs3cu7jxPDjhiAZRdcQSYPE2VGFVHAdirHqRZEpZsWyPiNK6XPQKAg RVN RSgWs9Co8nQeyPqQAAqHkHhc5ykXyoMDUp USDT(ETH ERC20) 0xa5955cf9fe7af53bcaa1d2404e2b17a1f28aac4f Paypal PayPal.Me/cryptolabsZA
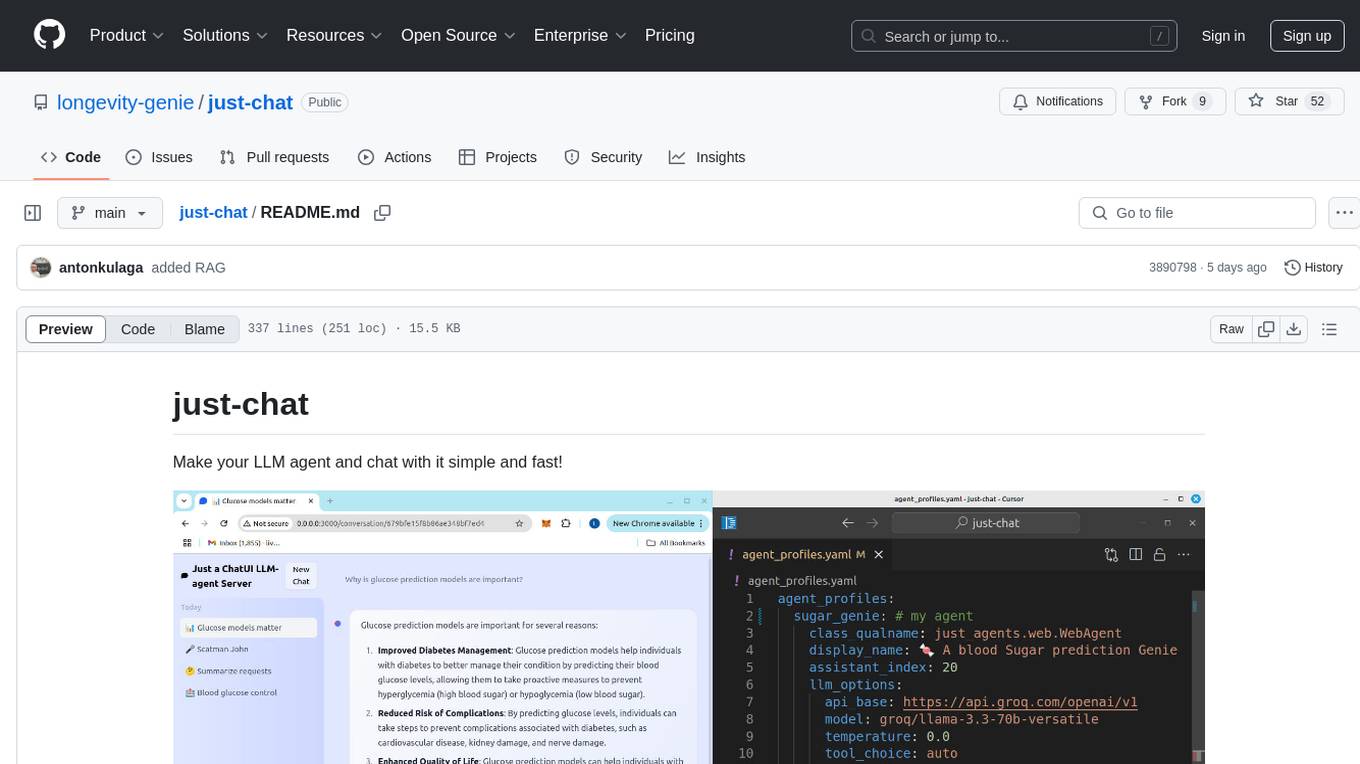
just-chat
Just-Chat is a containerized application that allows users to easily set up and chat with their AI agent. Users can customize their AI assistant using a YAML file, add new capabilities with Python tools, and interact with the agent through a chat web interface. The tool supports various modern models like DeepSeek Reasoner, ChatGPT, LLAMA3.3, etc. Users can also use semantic search capabilities with MeiliSearch to find and reference relevant information based on meaning. Just-Chat requires Docker or Podman for operation and provides detailed installation instructions for both Linux and Windows users.
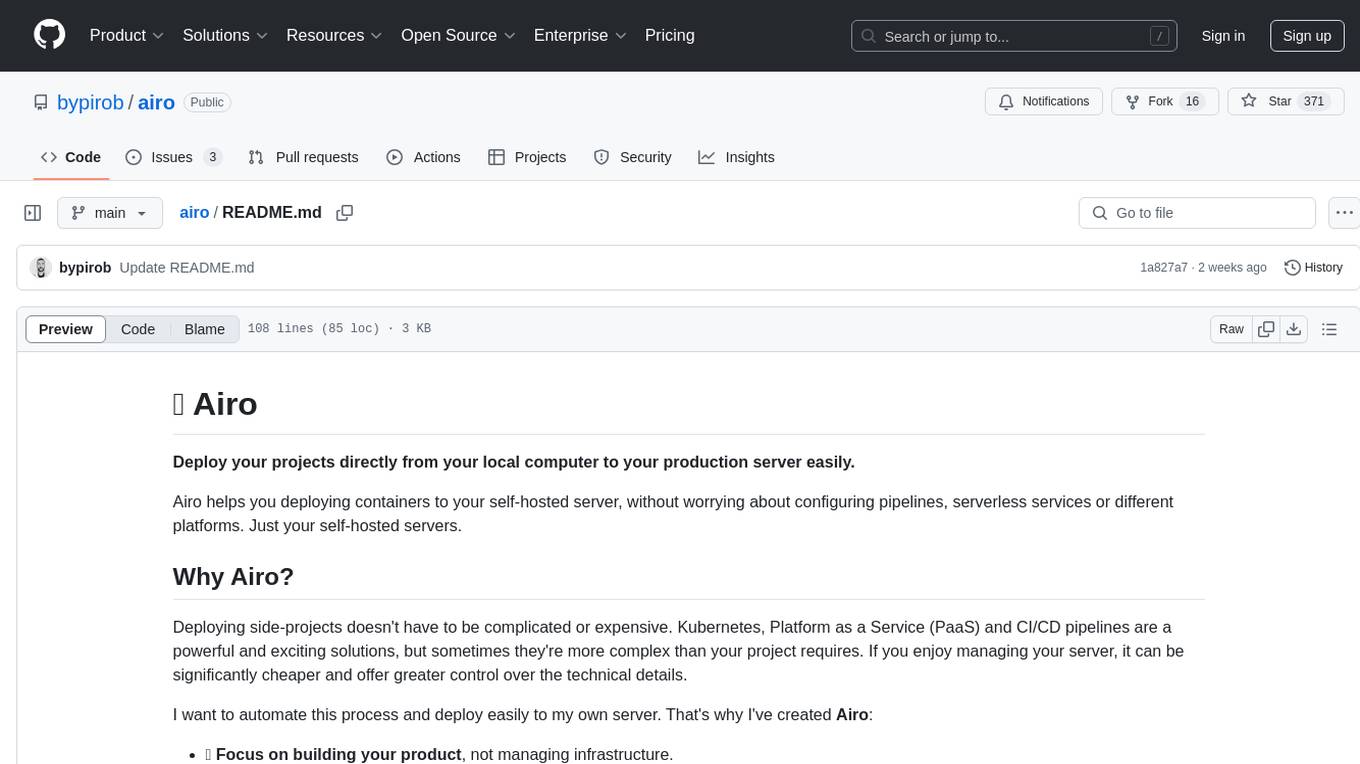
airo
Airo is a tool designed to simplify the process of deploying containers to self-hosted servers. It allows users to focus on building their products without the complexity of Kubernetes or CI/CD pipelines. With Airo, users can easily build and push Docker images, deploy instantly with a single command, update configurations securely using SSH, and set up HTTPS and reverse proxy automatically using Caddy.
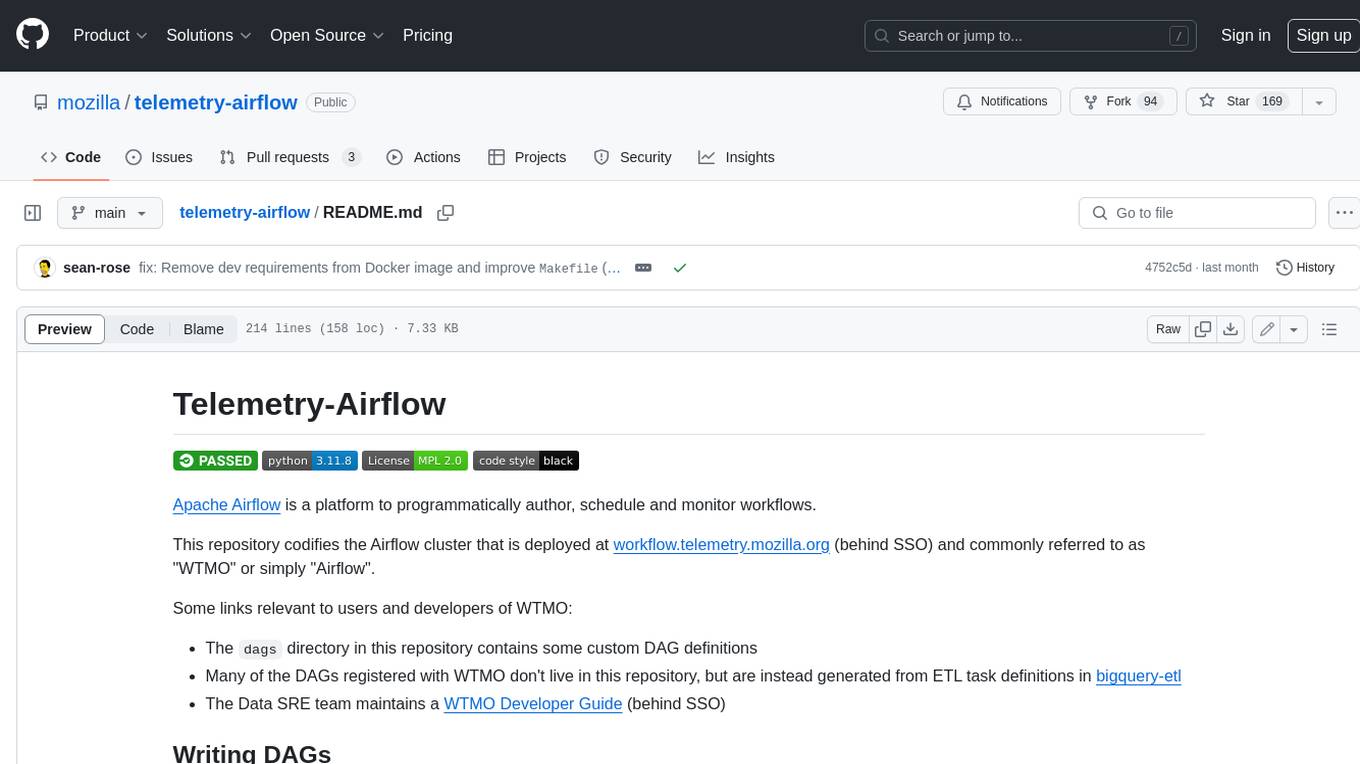
telemetry-airflow
This repository codifies the Airflow cluster that is deployed at workflow.telemetry.mozilla.org (behind SSO) and commonly referred to as "WTMO" or simply "Airflow". Some links relevant to users and developers of WTMO: * The `dags` directory in this repository contains some custom DAG definitions * Many of the DAGs registered with WTMO don't live in this repository, but are instead generated from ETL task definitions in bigquery-etl * The Data SRE team maintains a WTMO Developer Guide (behind SSO)
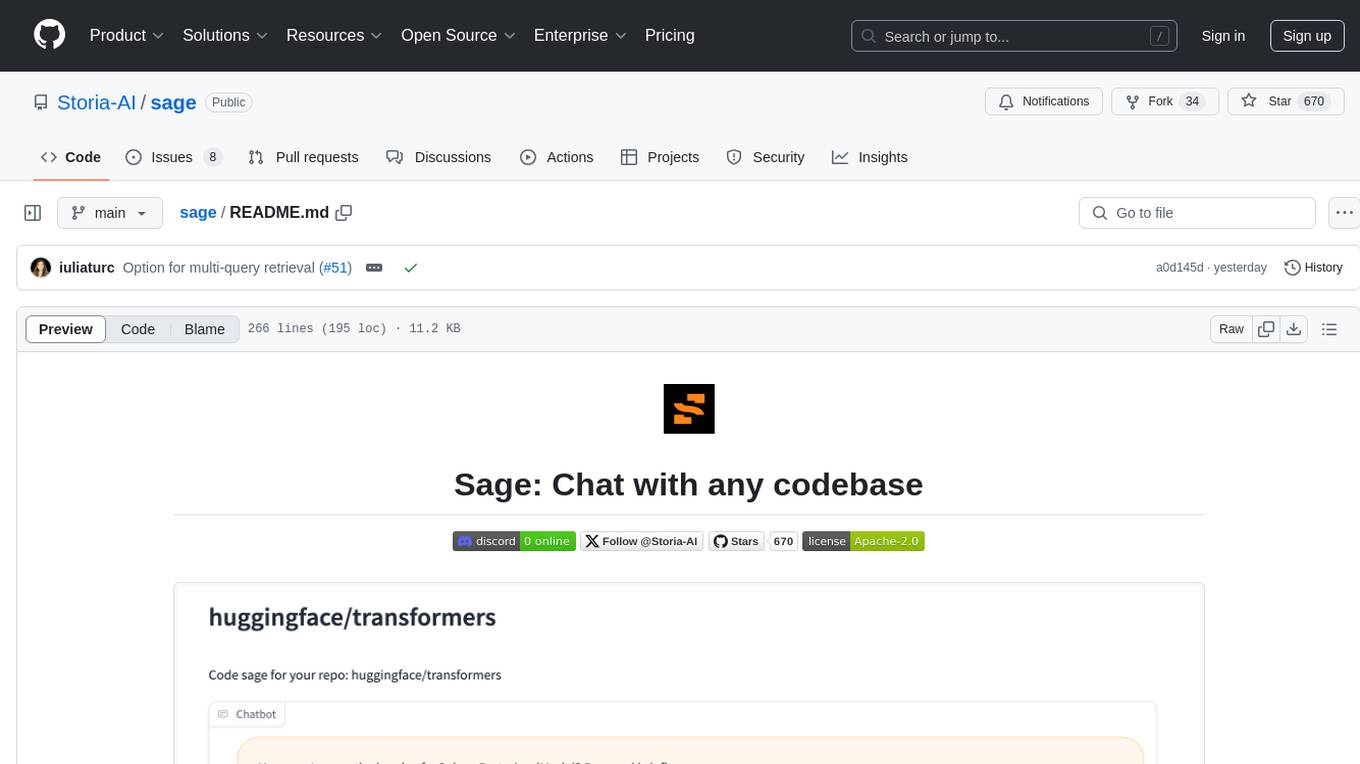
sage
Sage is a tool that allows users to chat with any codebase, providing a chat interface for code understanding and integration. It simplifies the process of learning how a codebase works by offering heavily documented answers sourced directly from the code. Users can set up Sage locally or on the cloud with minimal effort. The tool is designed to be easily customizable, allowing users to swap components of the pipeline and improve the algorithms powering code understanding and generation.
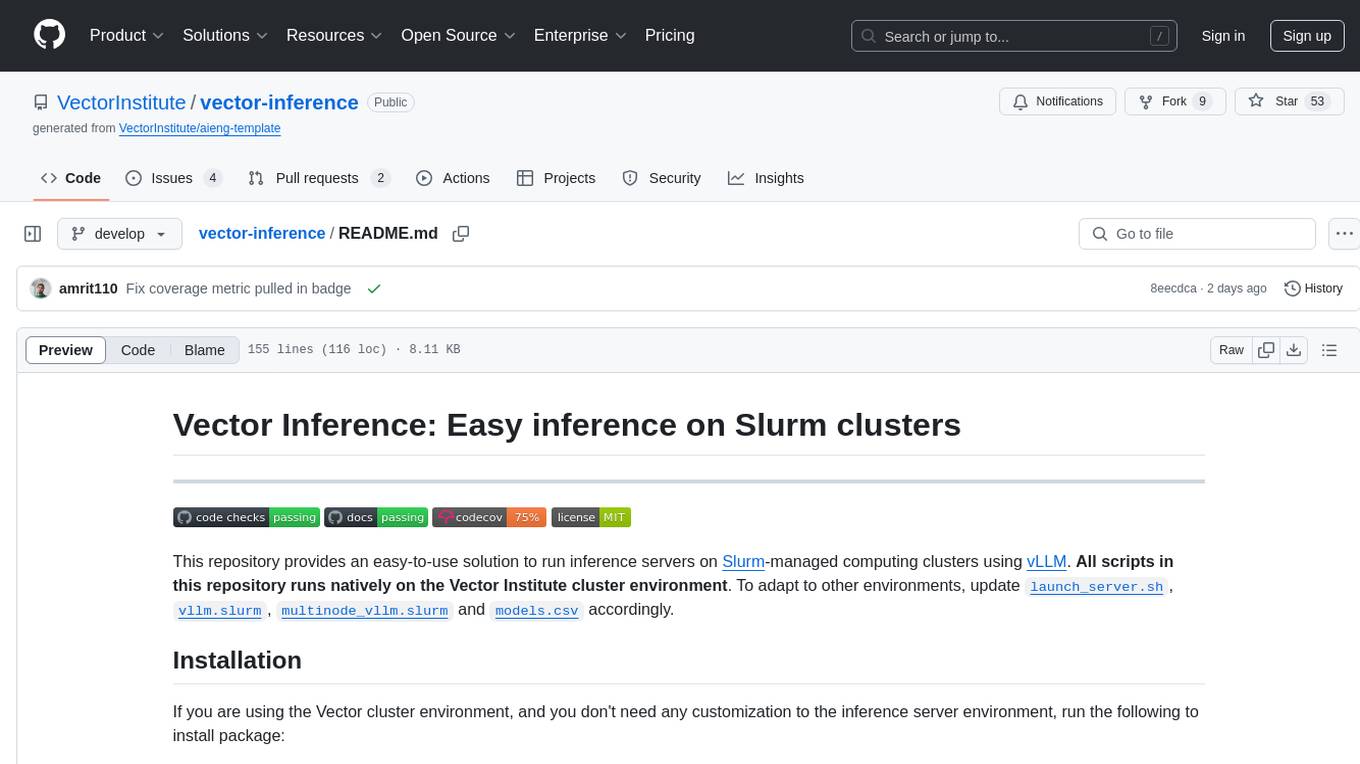
vector-inference
This repository provides an easy-to-use solution for running inference servers on Slurm-managed computing clusters using vLLM. All scripts in this repository run natively on the Vector Institute cluster environment. Users can deploy models as Slurm jobs, check server status and performance metrics, and shut down models. The repository also supports launching custom models with specific configurations. Additionally, users can send inference requests and set up an SSH tunnel to run inference from a local device.
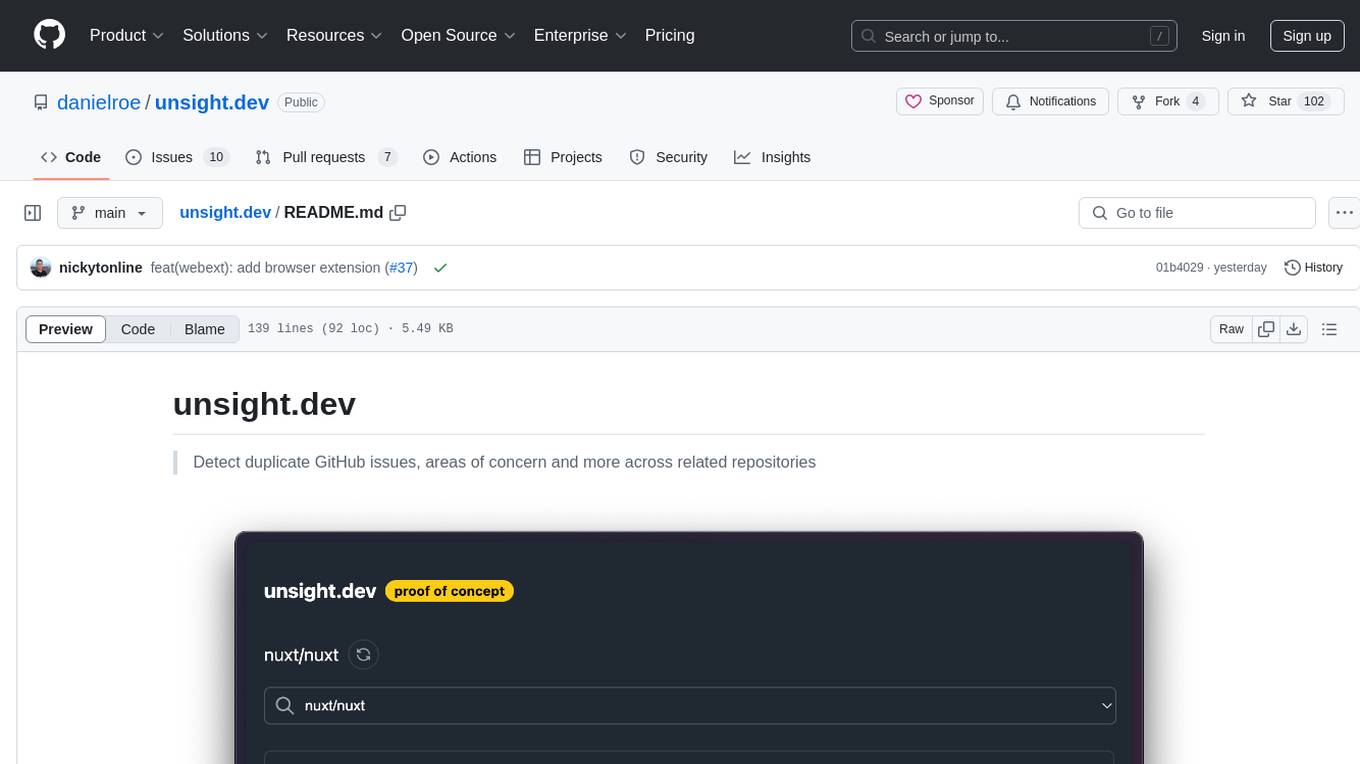
unsight.dev
unsight.dev is a tool built on Nuxt that helps detect duplicate GitHub issues and areas of concern across related repositories. It utilizes Nitro server API routes, GitHub API, and a GitHub App, along with UnoCSS. The tool is deployed on Cloudflare with NuxtHub, using Workers AI, Workers KV, and Vectorize. It also offers a browser extension soon to be released. Users can try the app locally for tweaking the UI and setting up a full development environment as a GitHub App.
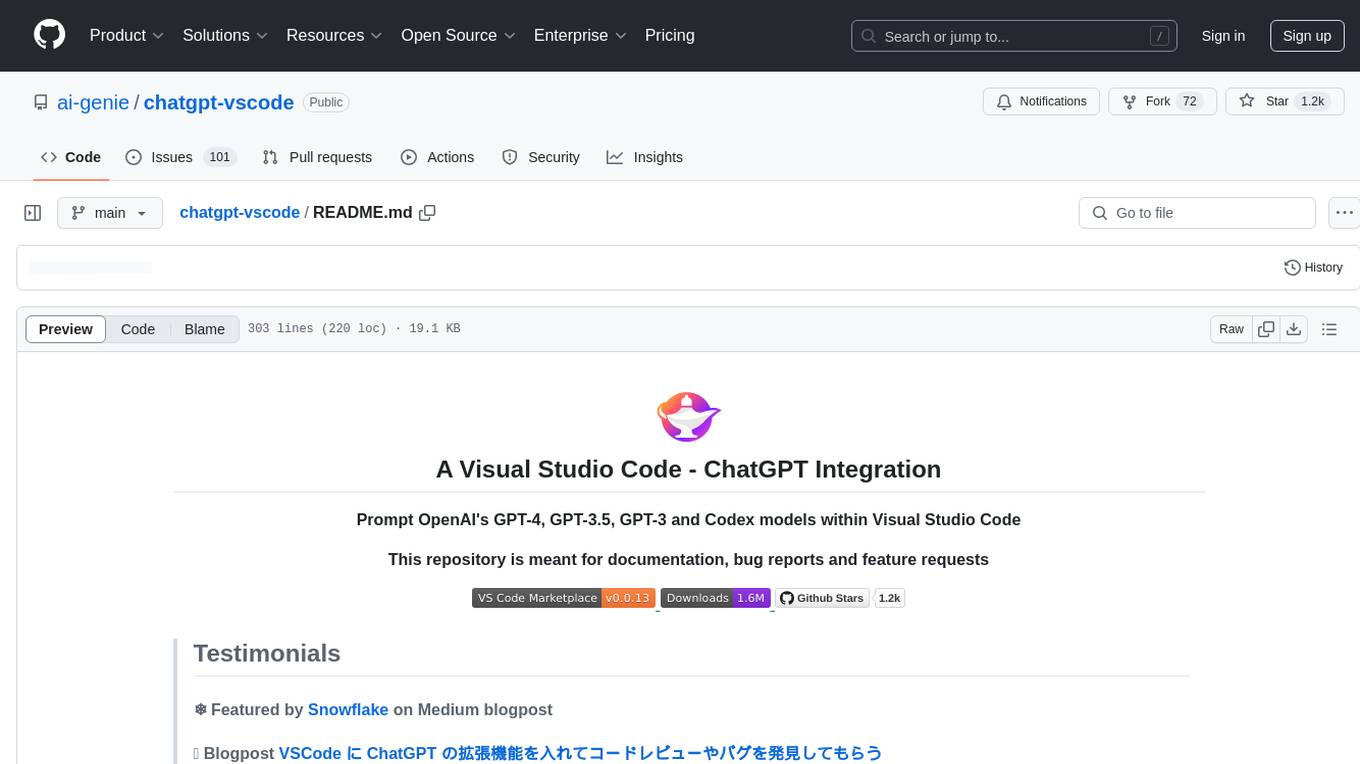
chatgpt-vscode
ChatGPT-VSCode is a Visual Studio Code integration that allows users to prompt OpenAI's GPT-4, GPT-3.5, GPT-3, and Codex models within the editor. It offers features like using improved models via OpenAI API Key, Azure OpenAI Service deployments, generating commit messages, storing conversation history, explaining and suggesting fixes for compile-time errors, viewing code differences, and more. Users can customize prompts, quick fix problems, save conversations, and export conversation history. The extension is designed to enhance developer experience by providing AI-powered assistance directly within VS Code.
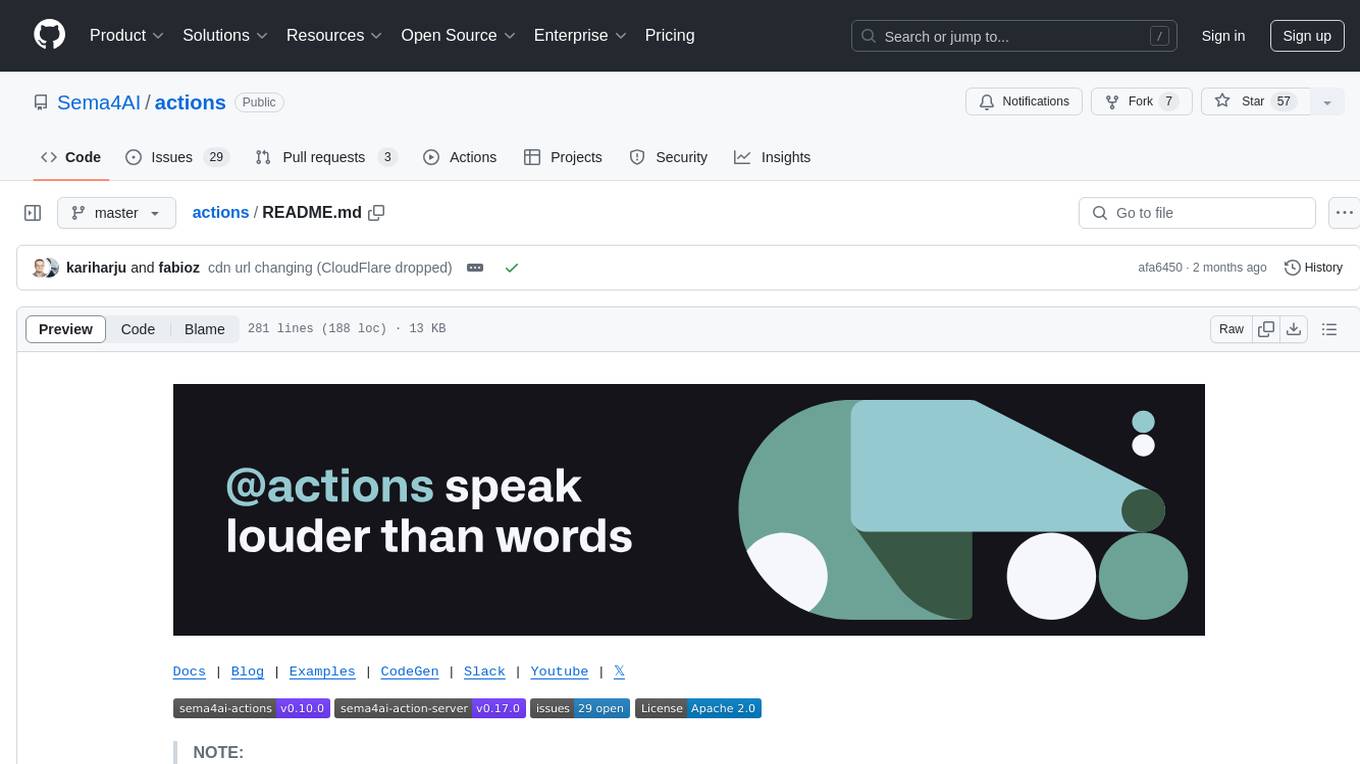
actions
Sema4.ai Action Server is a tool that allows users to build semantic actions in Python to connect AI agents with real-world applications. It enables users to create custom actions, skills, loaders, and plugins that securely connect any AI Assistant platform to data and applications. The tool automatically creates and exposes an API based on function declaration, type hints, and docstrings by adding '@action' to Python scripts. It provides an end-to-end stack supporting various connections between AI and user's apps and data, offering ease of use, security, and scalability.
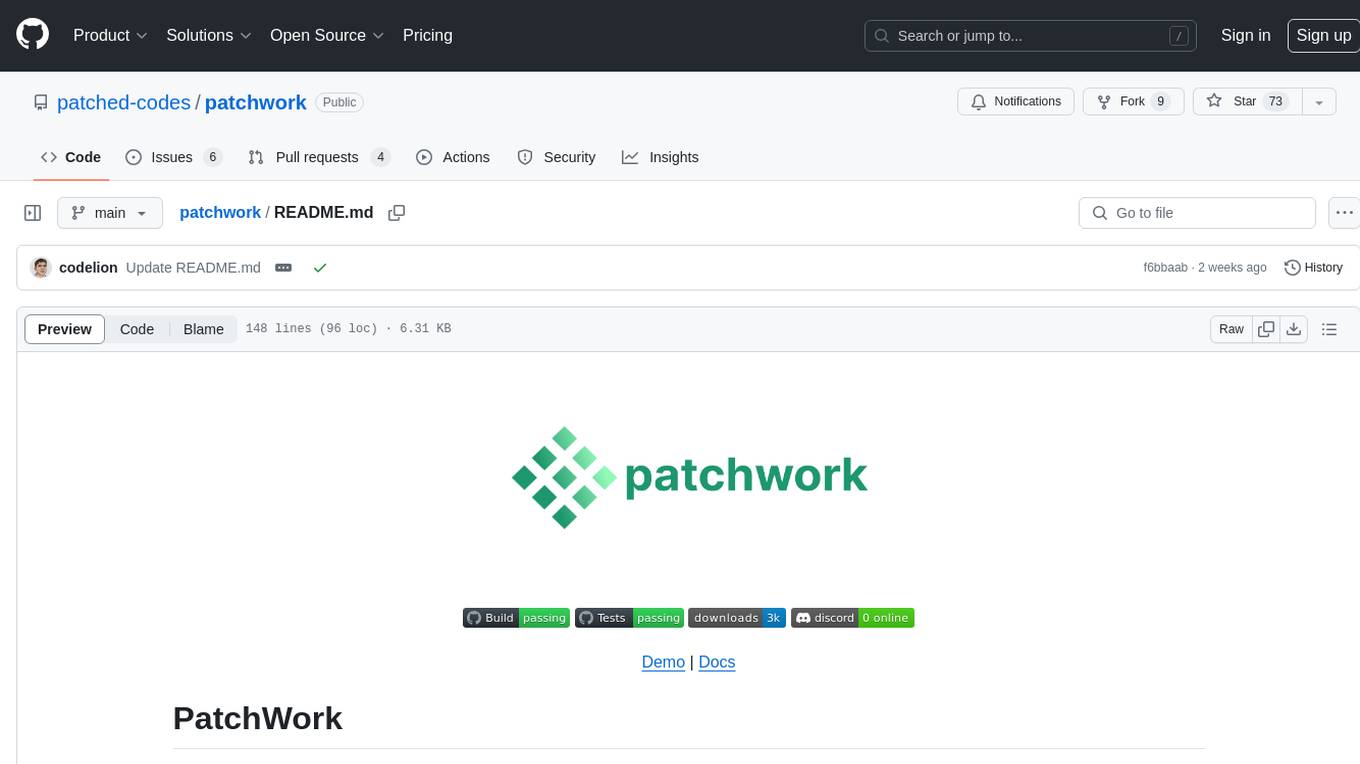
patchwork
PatchWork is an open-source framework designed for automating development tasks using large language models. It enables users to automate workflows such as PR reviews, bug fixing, security patching, and more through a self-hosted CLI agent and preferred LLMs. The framework consists of reusable atomic actions called Steps, customizable LLM prompts known as Prompt Templates, and LLM-assisted automations called Patchflows. Users can run Patchflows locally in their CLI/IDE or as part of CI/CD pipelines. PatchWork offers predefined patchflows like AutoFix, PRReview, GenerateREADME, DependencyUpgrade, and ResolveIssue, with the flexibility to create custom patchflows. Prompt templates are used to pass queries to LLMs and can be customized. Contributions to new patchflows, steps, and the core framework are encouraged, with chat assistants available to aid in the process. The roadmap includes expanding the patchflow library, introducing a debugger and validation module, supporting large-scale code embeddings, parallelization, fine-tuned models, and an open-source GUI. PatchWork is licensed under AGPL-3.0 terms, while custom patchflows and steps can be shared using the Apache-2.0 licensed patchwork template repository.
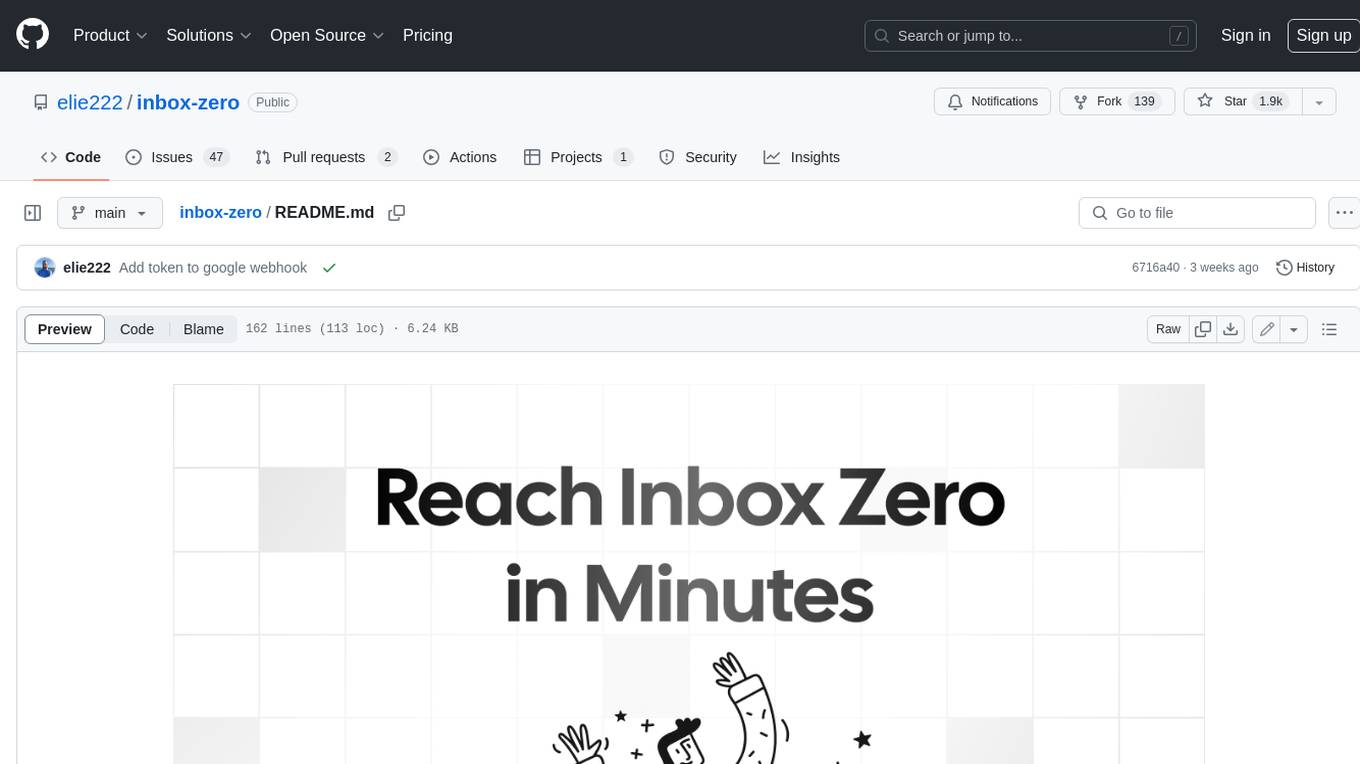
inbox-zero
Inbox Zero is an open-source email app that helps you reach inbox zero fast with AI assistance. It offers various features such as a newsletter cleaner, AI assistant for auto-responding, archiving, labeling, and forwarding emails, a cold email blocker, email analytics, tracking of new senders and unreplied emails, and a large email finder to free up space. Inbox Zero is built with Next.js, Tailwind CSS, Prisma, Tinybird, Upstash, and Turbo.
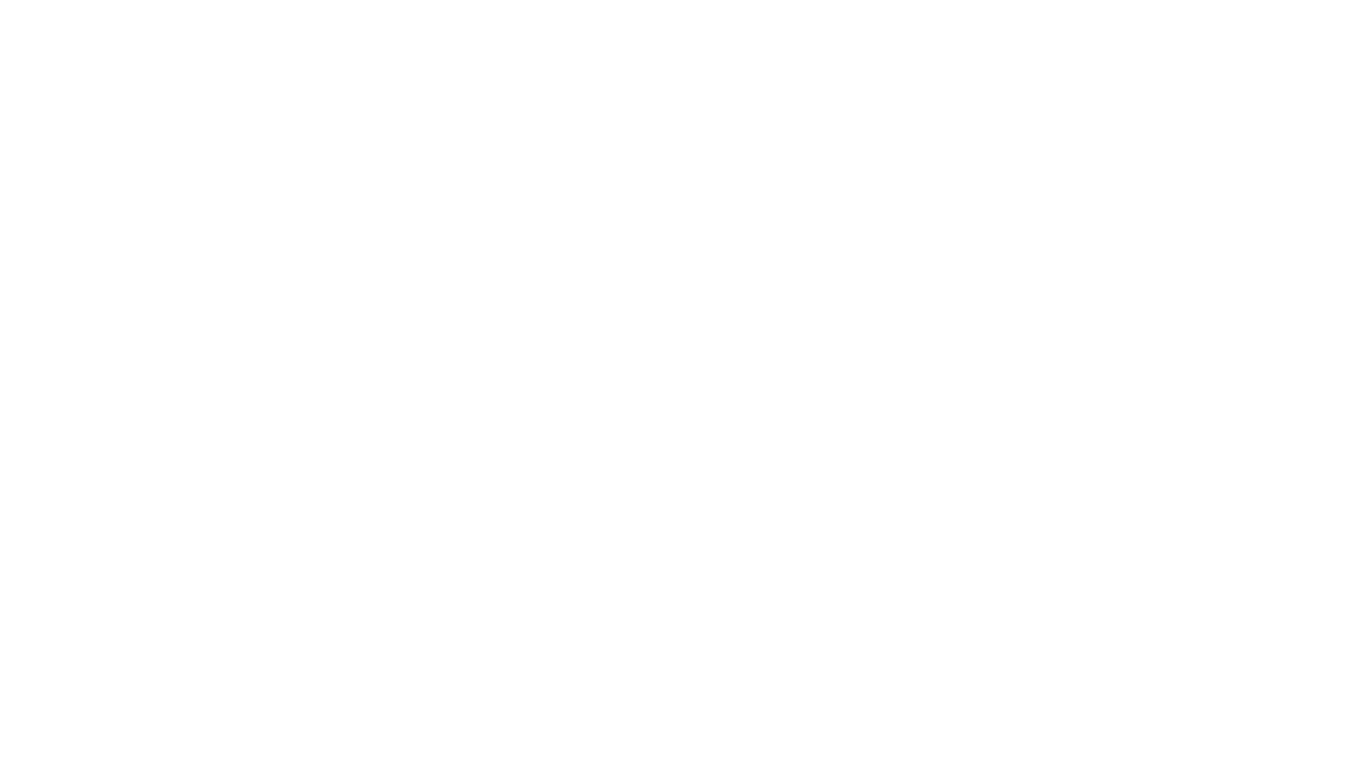
cognita
Cognita is an open-source framework to organize your RAG codebase along with a frontend to play around with different RAG customizations. It provides a simple way to organize your codebase so that it becomes easy to test it locally while also being able to deploy it in a production ready environment. The key issues that arise while productionizing RAG system from a Jupyter Notebook are: 1. **Chunking and Embedding Job** : The chunking and embedding code usually needs to be abstracted out and deployed as a job. Sometimes the job will need to run on a schedule or be trigerred via an event to keep the data updated. 2. **Query Service** : The code that generates the answer from the query needs to be wrapped up in a api server like FastAPI and should be deployed as a service. This service should be able to handle multiple queries at the same time and also autoscale with higher traffic. 3. **LLM / Embedding Model Deployment** : Often times, if we are using open-source models, we load the model in the Jupyter notebook. This will need to be hosted as a separate service in production and model will need to be called as an API. 4. **Vector DB deployment** : Most testing happens on vector DBs in memory or on disk. However, in production, the DBs need to be deployed in a more scalable and reliable way. Cognita makes it really easy to customize and experiment everything about a RAG system and still be able to deploy it in a good way. It also ships with a UI that makes it easier to try out different RAG configurations and see the results in real time. You can use it locally or with/without using any Truefoundry components. However, using Truefoundry components makes it easier to test different models and deploy the system in a scalable way. Cognita allows you to host multiple RAG systems using one app. ### Advantages of using Cognita are: 1. A central reusable repository of parsers, loaders, embedders and retrievers. 2. Ability for non-technical users to play with UI - Upload documents and perform QnA using modules built by the development team. 3. Fully API driven - which allows integration with other systems. > If you use Cognita with Truefoundry AI Gateway, you can get logging, metrics and feedback mechanism for your user queries. ### Features: 1. Support for multiple document retrievers that use `Similarity Search`, `Query Decompostion`, `Document Reranking`, etc 2. Support for SOTA OpenSource embeddings and reranking from `mixedbread-ai` 3. Support for using LLMs using `Ollama` 4. Support for incremental indexing that ingests entire documents in batches (reduces compute burden), keeps track of already indexed documents and prevents re-indexing of those docs.
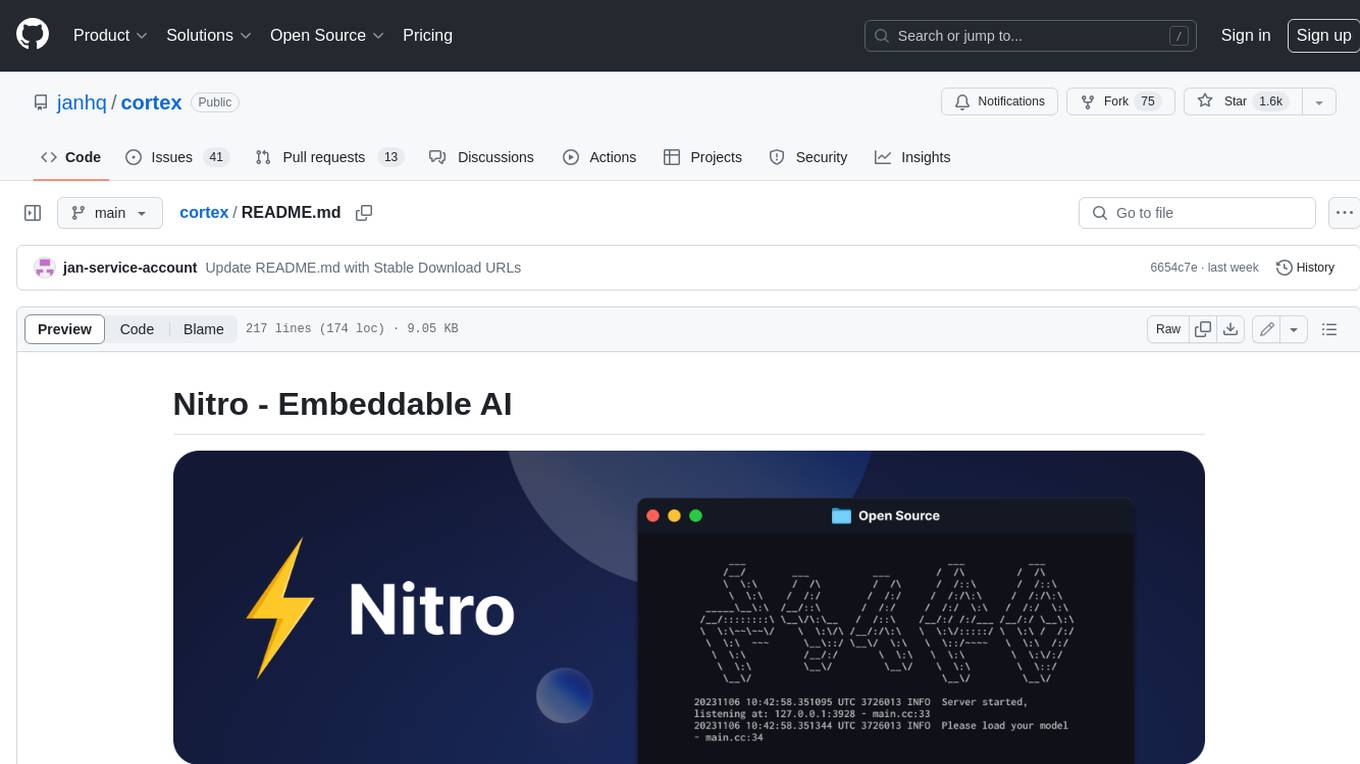
cortex
Nitro is a high-efficiency C++ inference engine for edge computing, powering Jan. It is lightweight and embeddable, ideal for product integration. The binary of nitro after zipped is only ~3mb in size with none to minimal dependencies (if you use a GPU need CUDA for example) make it desirable for any edge/server deployment.
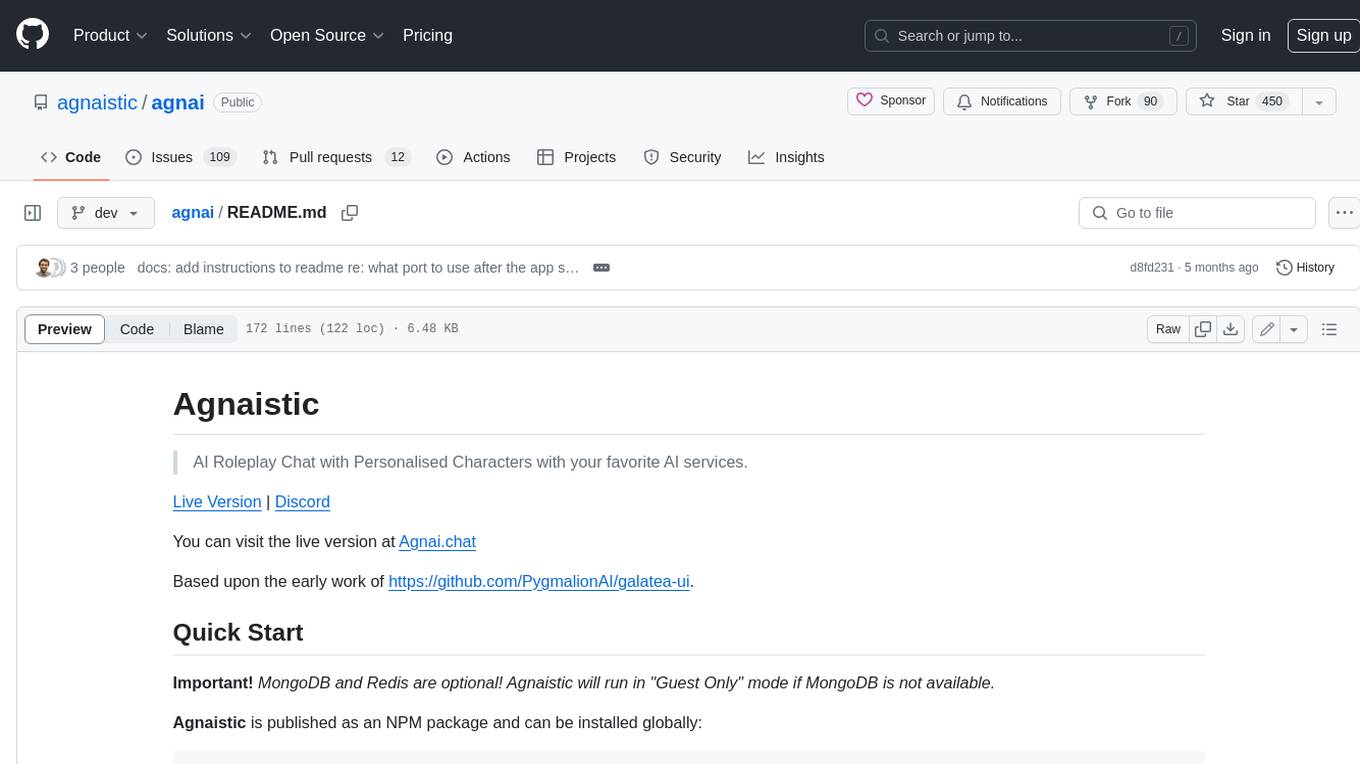
agnai
Agnaistic is an AI roleplay chat tool that allows users to interact with personalized characters using their favorite AI services. It supports multiple AI services, persona schema formats, and features such as group conversations, user authentication, and memory/lore books. Agnaistic can be self-hosted or run using Docker, and it provides a range of customization options through its settings.json file. The tool is designed to be user-friendly and accessible, making it suitable for both casual users and developers.
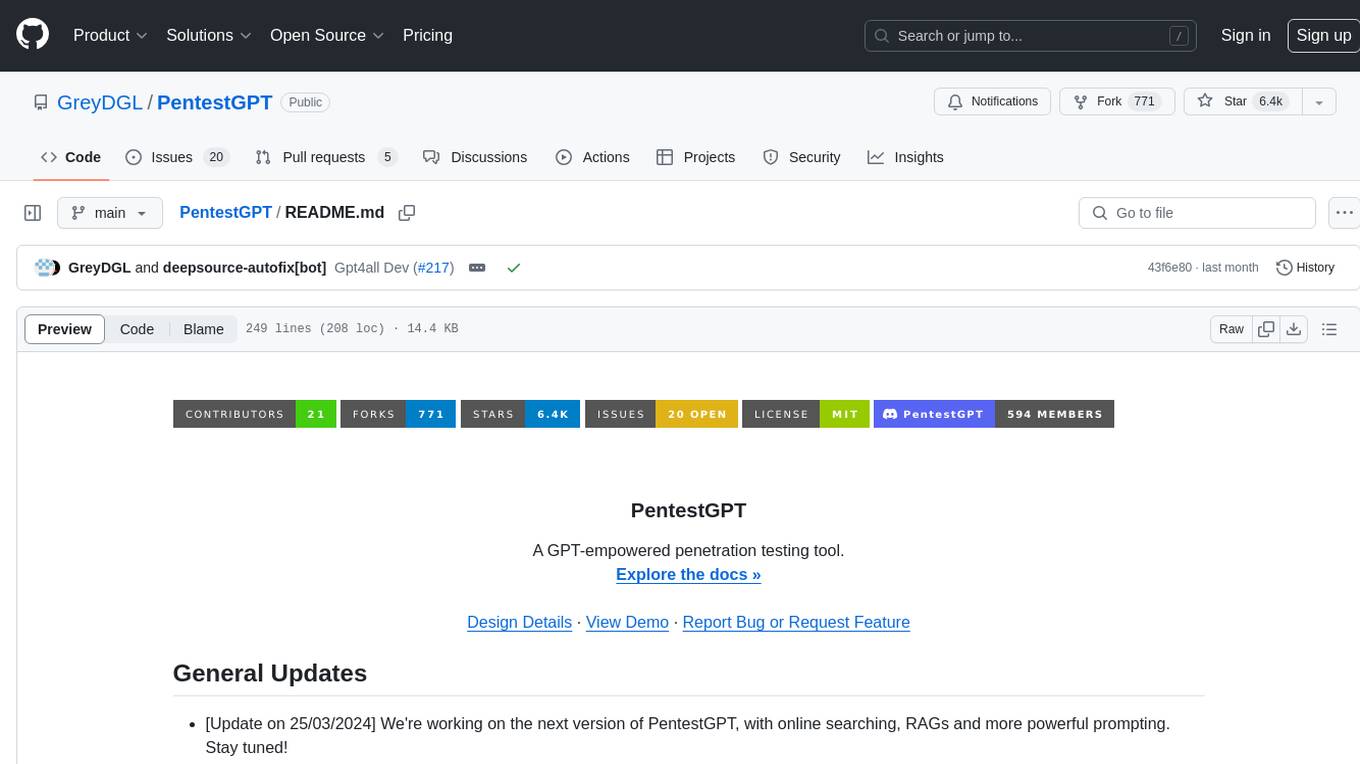
PentestGPT
PentestGPT is a penetration testing tool empowered by ChatGPT, designed to automate the penetration testing process. It operates interactively to guide penetration testers in overall progress and specific operations. The tool supports solving easy to medium HackTheBox machines and other CTF challenges. Users can use PentestGPT to perform tasks like testing connections, using different reasoning models, discussing with the tool, searching on Google, and generating reports. It also supports local LLMs with custom parsers for advanced users.
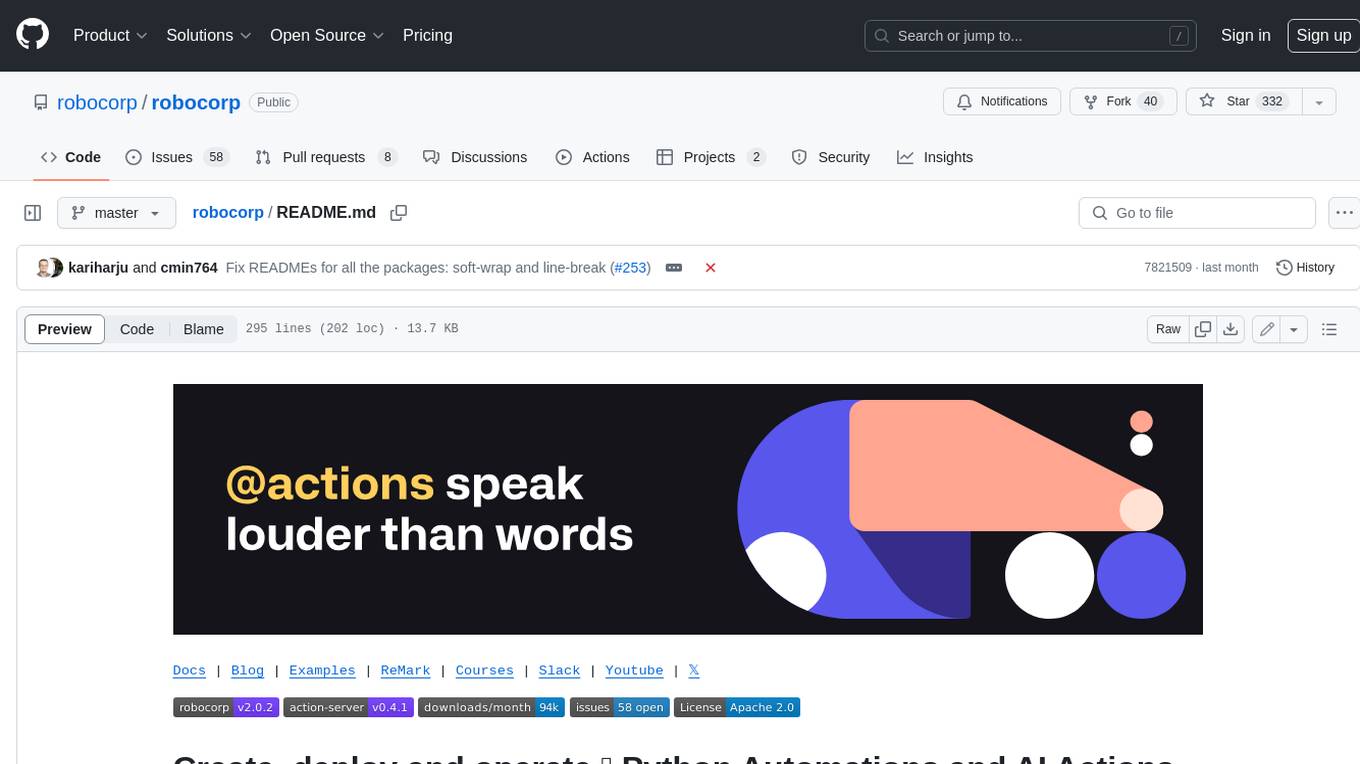
robocorp
Robocorp is a platform that allows users to create, deploy, and operate Python automations and AI actions. It provides an easy way to extend the capabilities of AI agents, assistants, and copilots with custom actions written in Python. Users can create and deploy tools, skills, loaders, and plugins that securely connect any AI Assistant platform to their data and applications. The Robocorp Action Server makes Python scripts compatible with ChatGPT and LangChain by automatically creating and exposing an API based on function declaration, type hints, and docstrings. It simplifies the process of developing and deploying AI actions, enabling users to interact with AI frameworks effortlessly.
For similar tasks
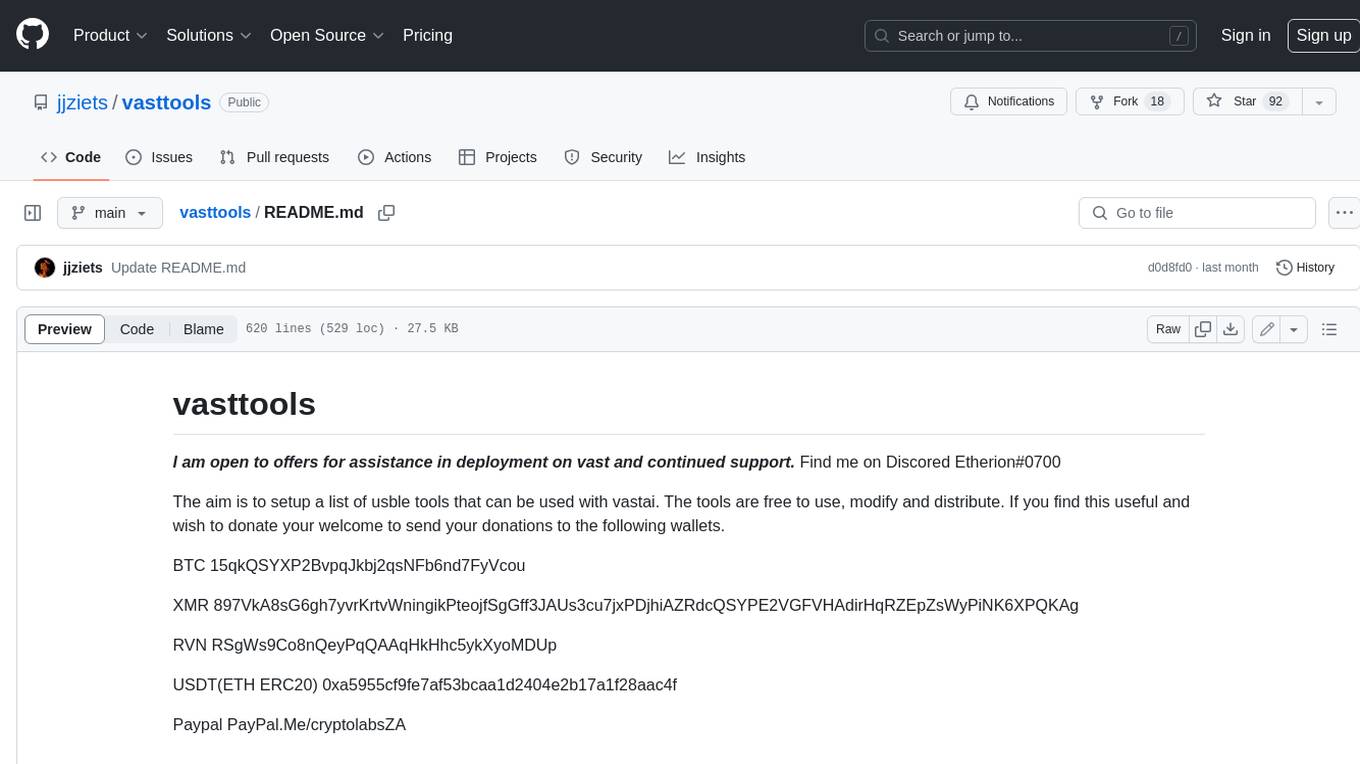
vasttools
This repository contains a collection of tools that can be used with vastai. The tools are free to use, modify and distribute. If you find this useful and wish to donate your welcome to send your donations to the following wallets. BTC 15qkQSYXP2BvpqJkbj2qsNFb6nd7FyVcou XMR 897VkA8sG6gh7yvrKrtvWningikPteojfSgGff3JAUs3cu7jxPDjhiAZRdcQSYPE2VGFVHAdirHqRZEpZsWyPiNK6XPQKAg RVN RSgWs9Co8nQeyPqQAAqHkHhc5ykXyoMDUp USDT(ETH ERC20) 0xa5955cf9fe7af53bcaa1d2404e2b17a1f28aac4f Paypal PayPal.Me/cryptolabsZA
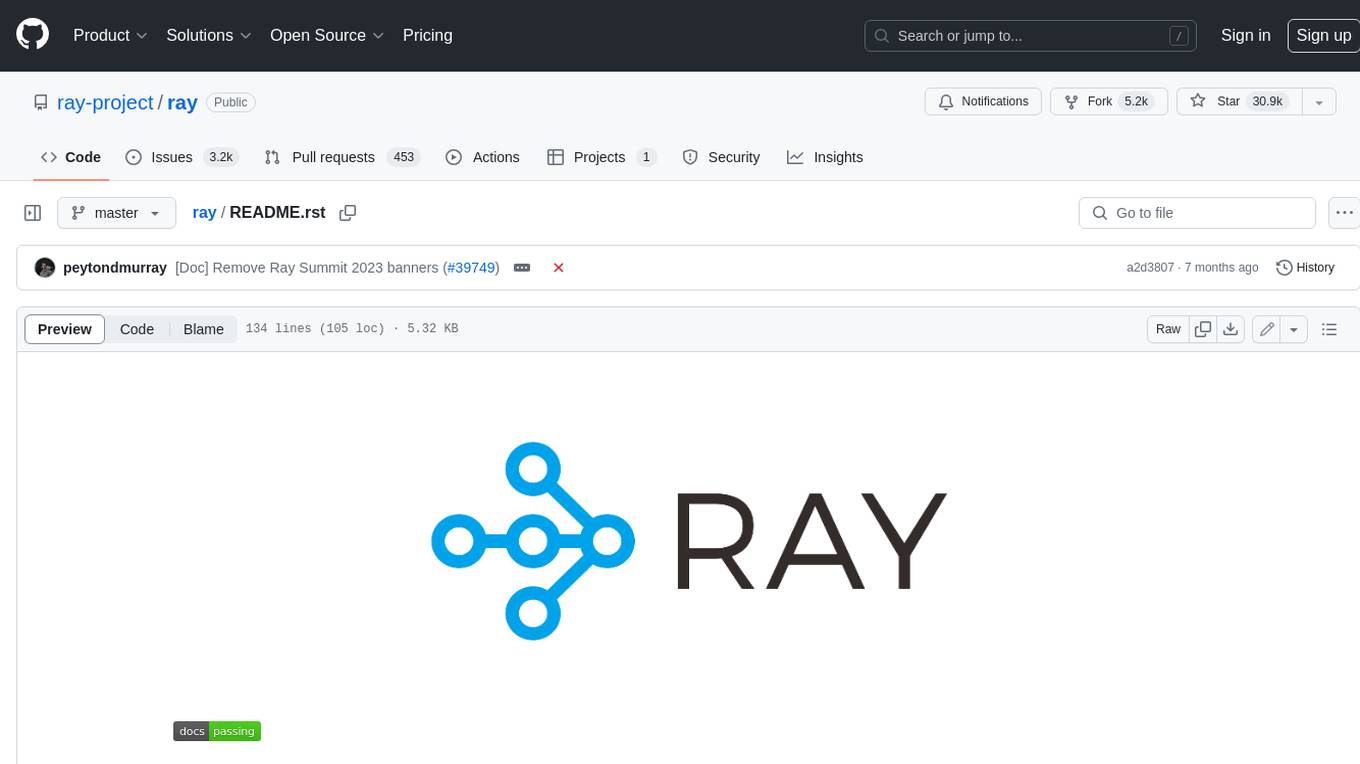
ray
Ray is a unified framework for scaling AI and Python applications. It consists of a core distributed runtime and a set of AI libraries for simplifying ML compute, including Data, Train, Tune, RLlib, and Serve. Ray runs on any machine, cluster, cloud provider, and Kubernetes, and features a growing ecosystem of community integrations. With Ray, you can seamlessly scale the same code from a laptop to a cluster, making it easy to meet the compute-intensive demands of modern ML workloads.
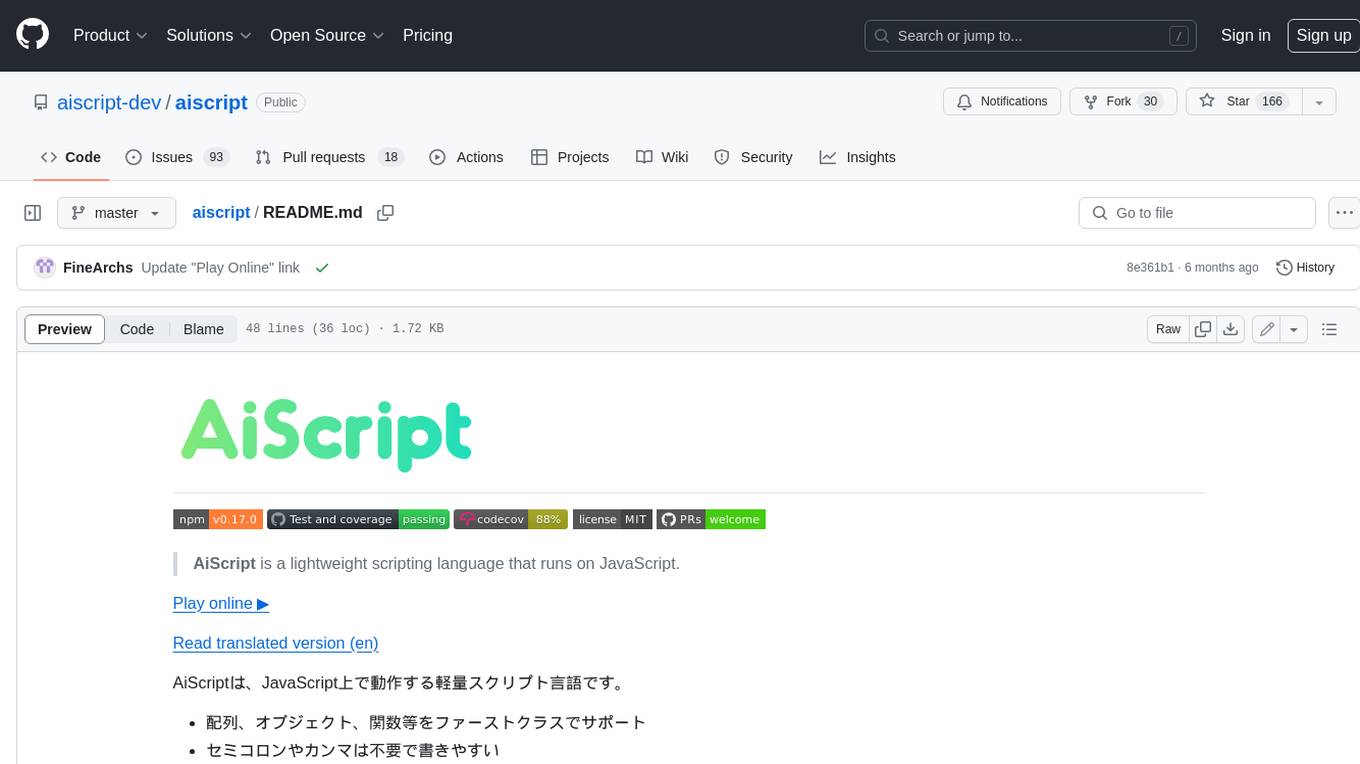
aiscript
AiScript is a lightweight scripting language that runs on JavaScript. It supports arrays, objects, and functions as first-class citizens, and is easy to write without the need for semicolons or commas. AiScript runs in a secure sandbox environment, preventing infinite loops from freezing the host. It also allows for easy provision of variables and functions from the host.
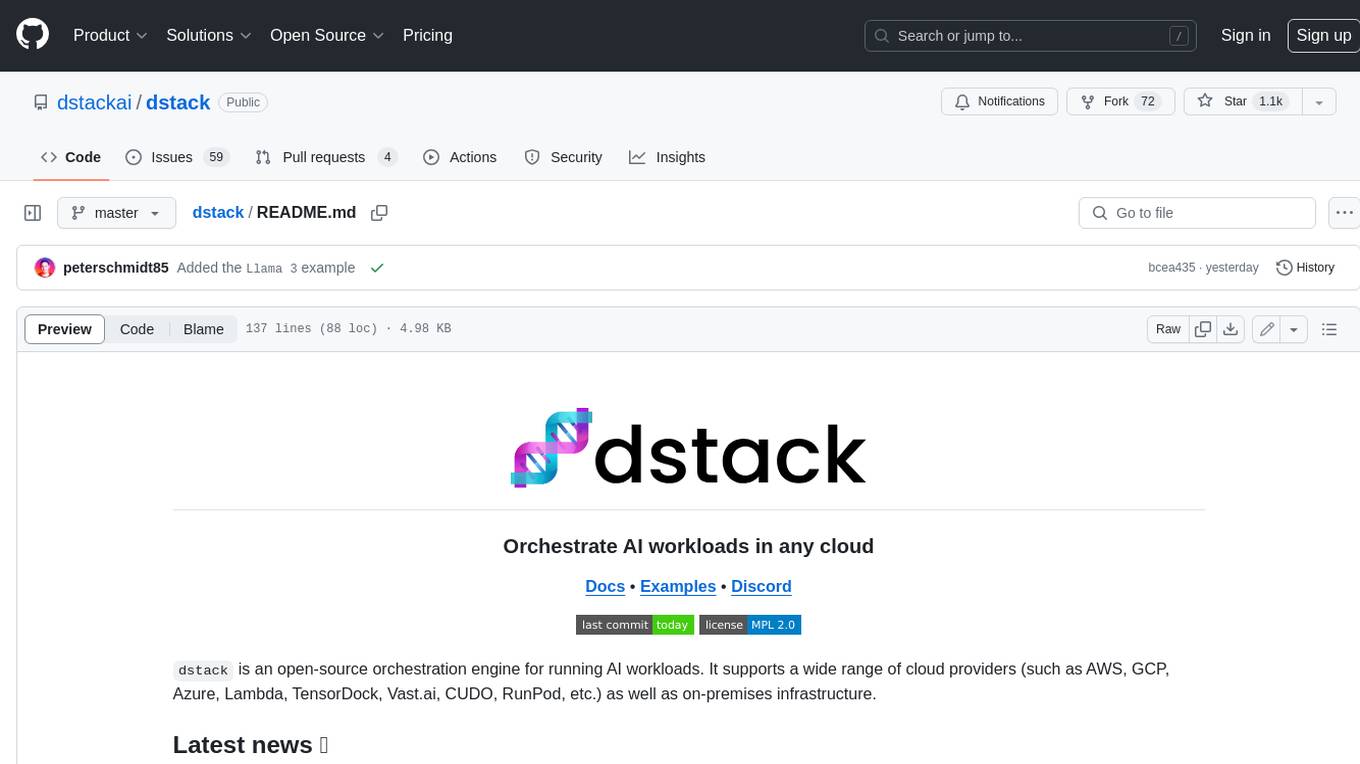
dstack
Dstack is an open-source orchestration engine for running AI workloads in any cloud. It supports a wide range of cloud providers (such as AWS, GCP, Azure, Lambda, TensorDock, Vast.ai, CUDO, RunPod, etc.) as well as on-premises infrastructure. With Dstack, you can easily set up and manage dev environments, tasks, services, and pools for your AI workloads.
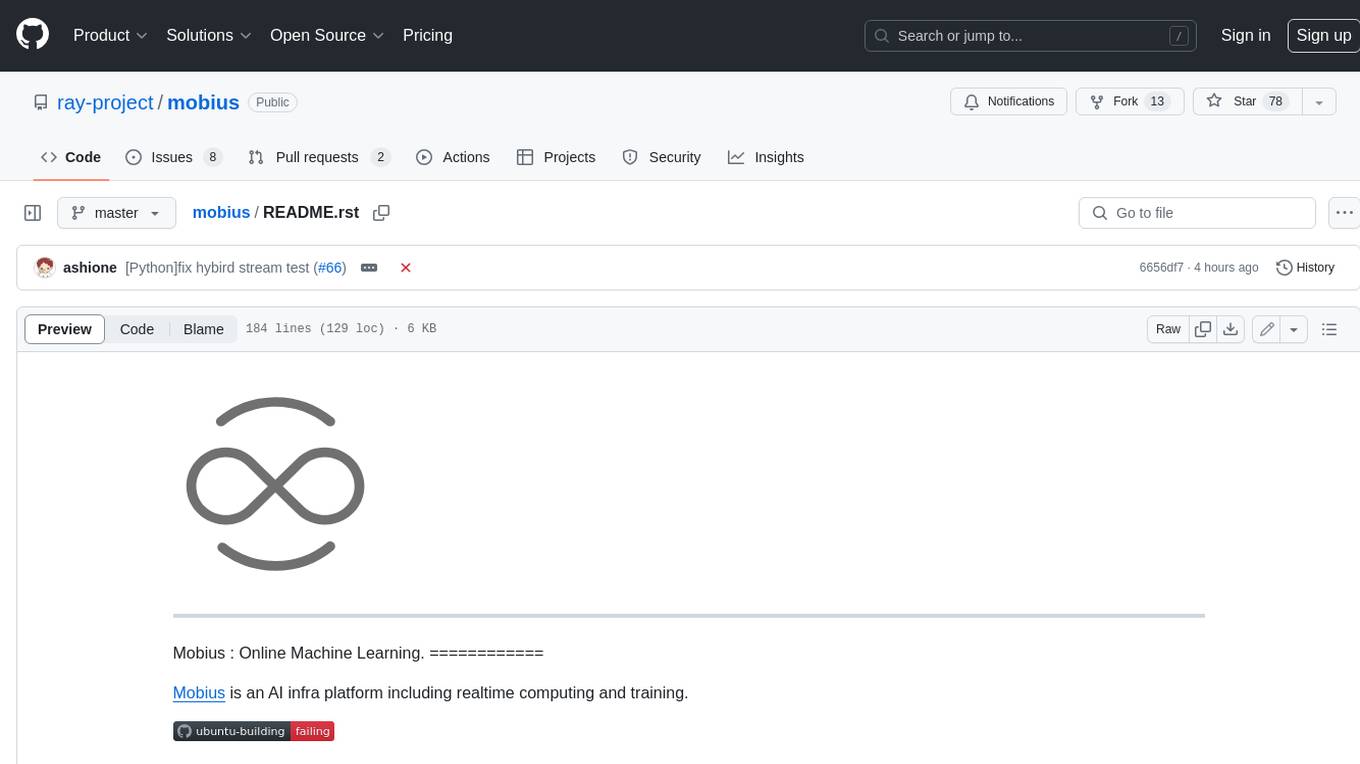
mobius
Mobius is an AI infra platform including realtime computing and training. It is built on Ray, a distributed computing framework, and provides a number of features that make it well-suited for online machine learning tasks. These features include: * **Cross Language**: Mobius can run in multiple languages (only Python and Java are supported currently) with high efficiency. You can implement your operator in different languages and run them in one job. * **Single Node Failover**: Mobius has a special failover mechanism that only needs to rollback the failed node itself, in most cases, to recover the job. This is a huge benefit if your job is sensitive about failure recovery time. * **AutoScaling**: Mobius can generate a new graph with different configurations in runtime without stopping the job. * **Fusion Training**: Mobius can combine TensorFlow/Pytorch and streaming, then building an e2e online machine learning pipeline. Mobius is still under development, but it has already been used to power a number of real-world applications, including: * A real-time recommendation system for a major e-commerce company * A fraud detection system for a large financial institution * A personalized news feed for a major news organization If you are interested in using Mobius for your own online machine learning projects, you can find more information in the documentation.
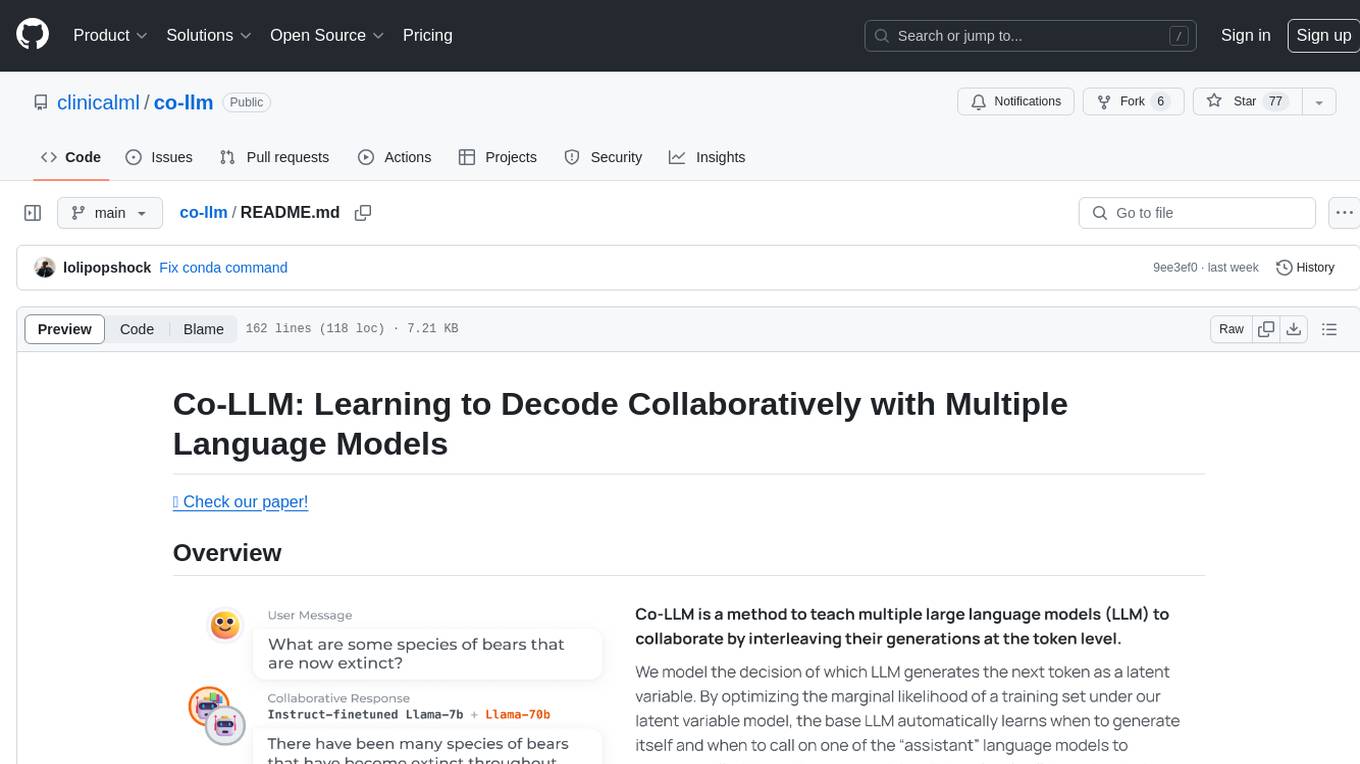
co-llm
Co-LLM (Collaborative Language Models) is a tool for learning to decode collaboratively with multiple language models. It provides a method for data processing, training, and inference using a collaborative approach. The tool involves steps such as formatting/tokenization, scoring logits, initializing Z vector, deferral training, and generating results using multiple models. Co-LLM supports training with different collaboration pairs and provides baseline training scripts for various models. In inference, it uses 'vllm' services to orchestrate models and generate results through API-like services. The tool is inspired by allenai/open-instruct and aims to improve decoding performance through collaborative learning.
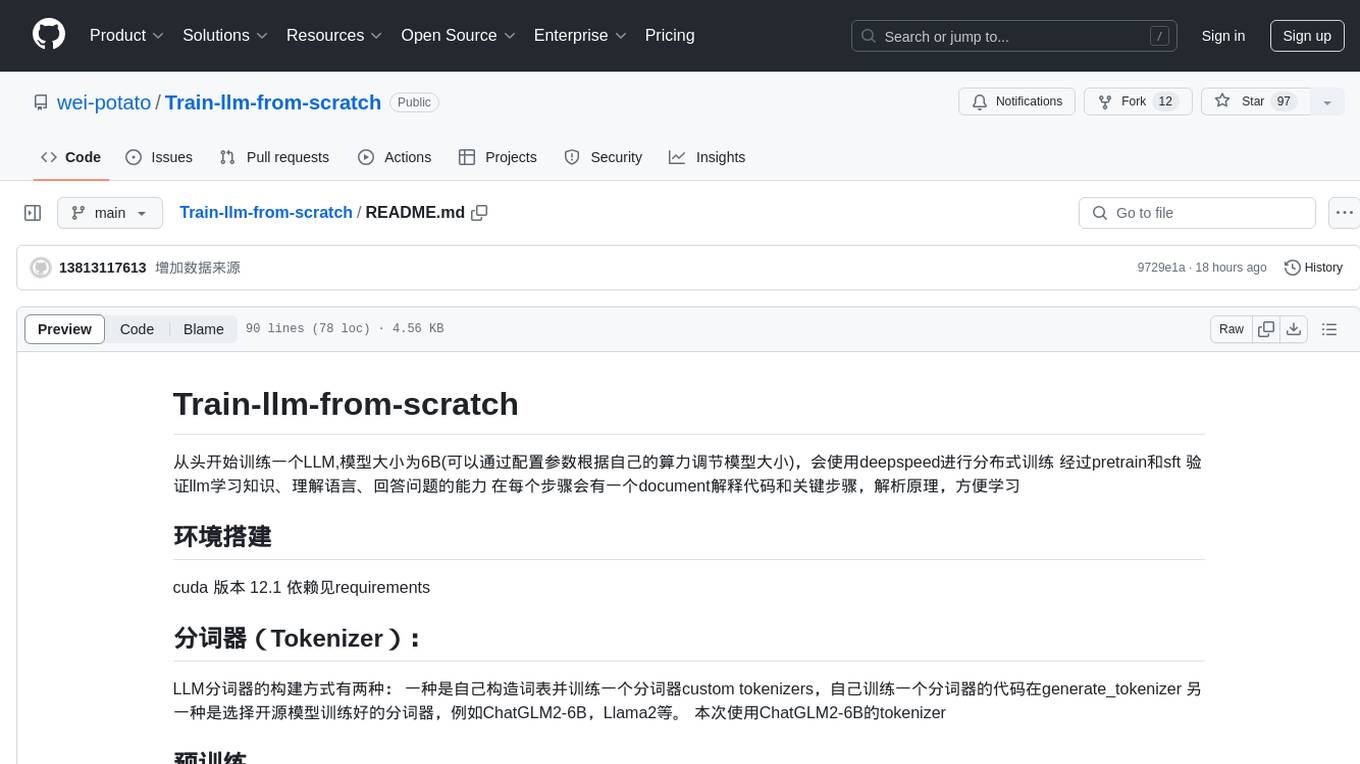
Train-llm-from-scratch
Train-llm-from-scratch is a repository that guides users through training a Large Language Model (LLM) from scratch. The model size can be adjusted based on available computing power. The repository utilizes deepspeed for distributed training and includes detailed explanations of the code and key steps at each stage to facilitate learning. Users can train their own tokenizer or use pre-trained tokenizers like ChatGLM2-6B. The repository provides information on preparing pre-training data, processing training data, and recommended SFT data for fine-tuning. It also references other projects and books related to LLM training.
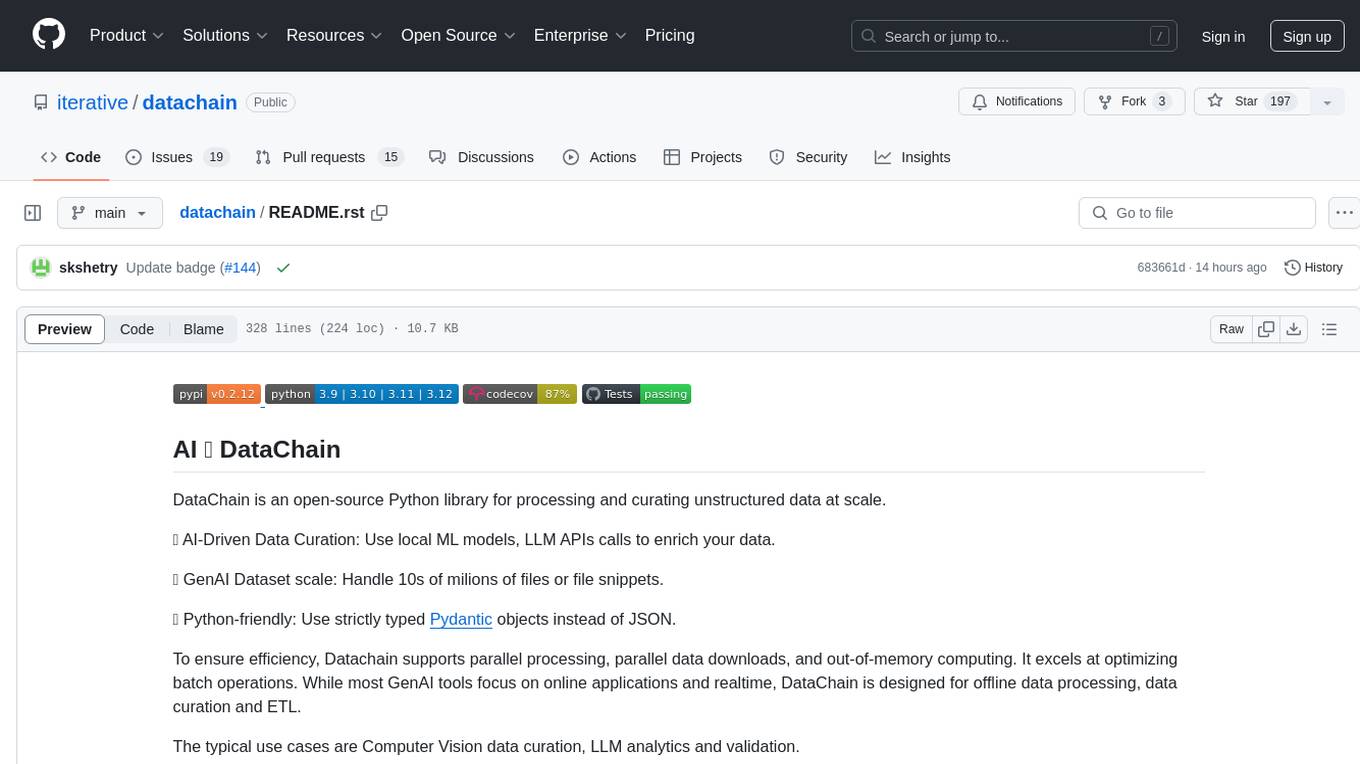
datachain
DataChain is an open-source Python library for processing and curating unstructured data at scale. It supports AI-driven data curation using local ML models and LLM APIs, handles large datasets, and is Python-friendly with Pydantic objects. It excels at optimizing batch operations and is designed for offline data processing, curation, and ETL. Typical use cases include Computer Vision data curation, LLM analytics, and validation.
For similar jobs
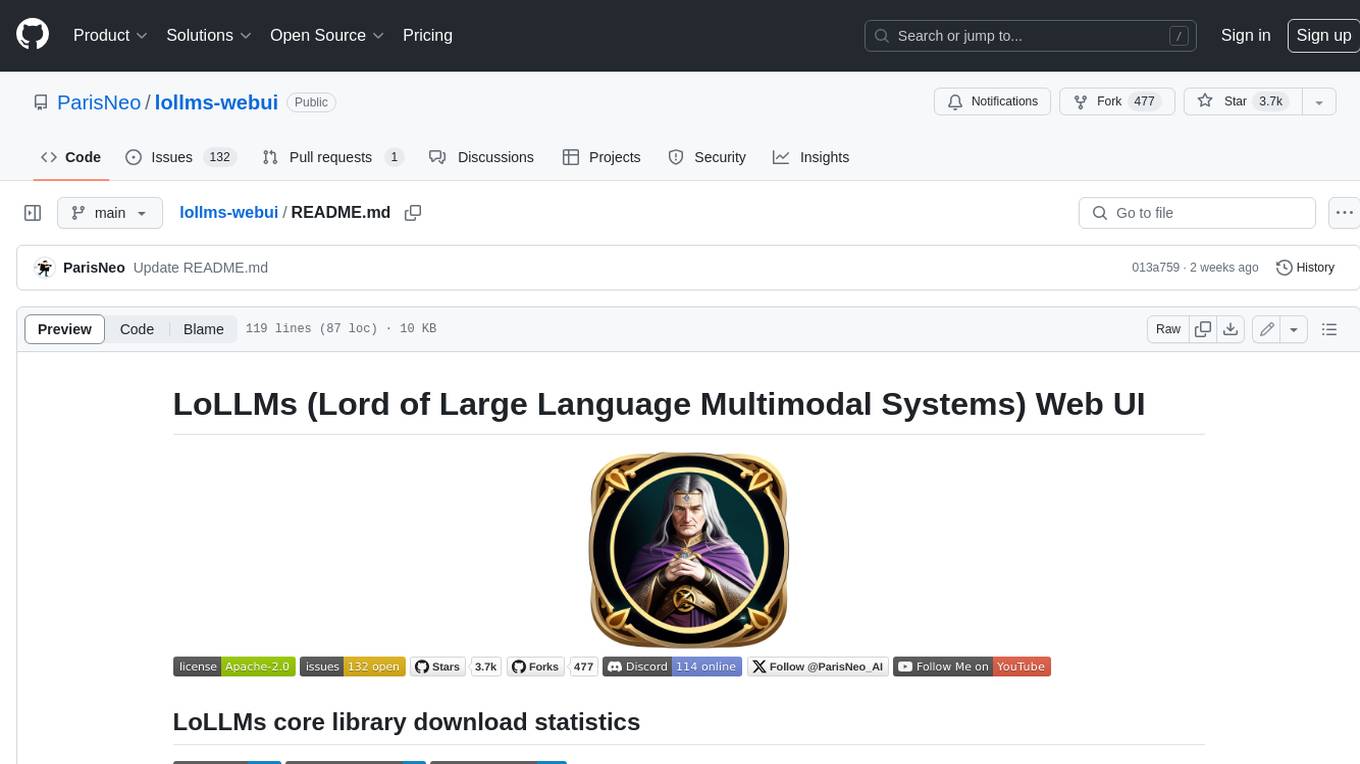
lollms-webui
LoLLMs WebUI (Lord of Large Language Multimodal Systems: One tool to rule them all) is a user-friendly interface to access and utilize various LLM (Large Language Models) and other AI models for a wide range of tasks. With over 500 AI expert conditionings across diverse domains and more than 2500 fine tuned models over multiple domains, LoLLMs WebUI provides an immediate resource for any problem, from car repair to coding assistance, legal matters, medical diagnosis, entertainment, and more. The easy-to-use UI with light and dark mode options, integration with GitHub repository, support for different personalities, and features like thumb up/down rating, copy, edit, and remove messages, local database storage, search, export, and delete multiple discussions, make LoLLMs WebUI a powerful and versatile tool.
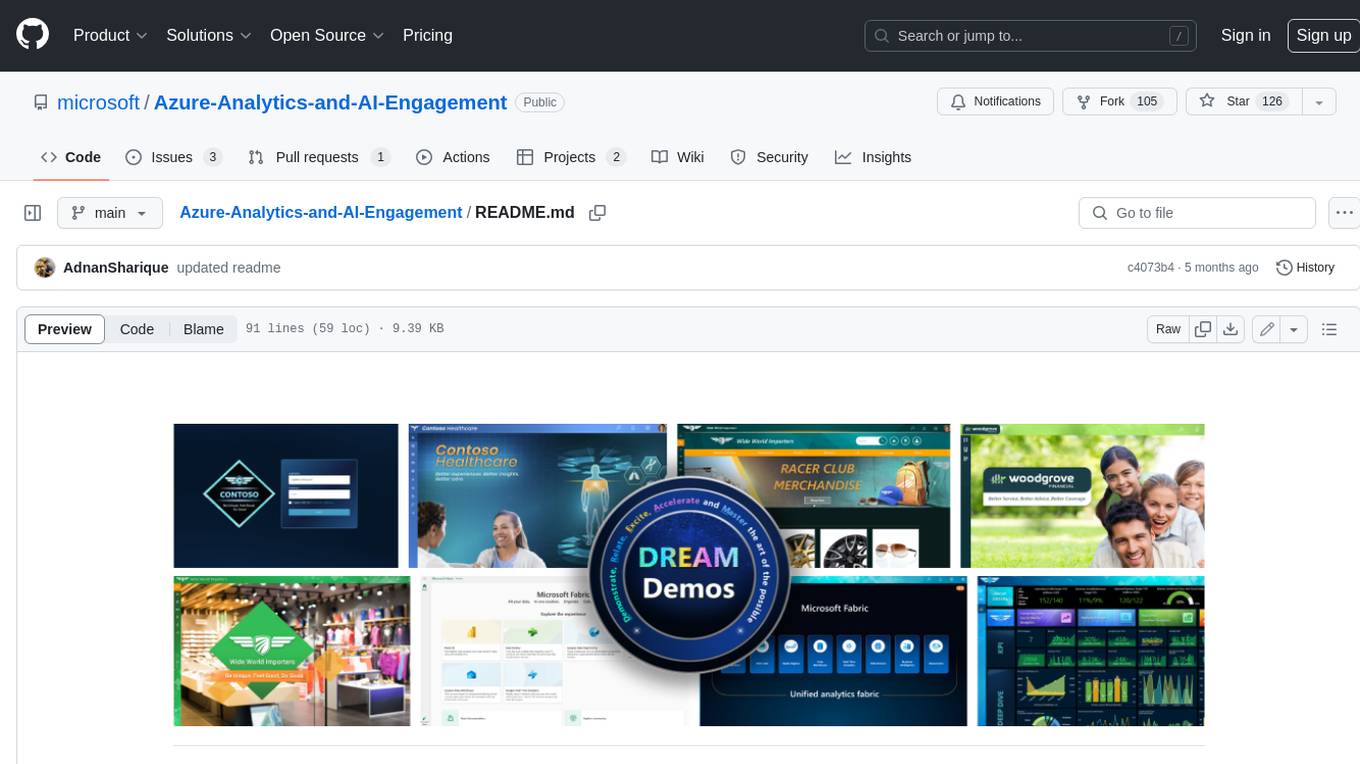
Azure-Analytics-and-AI-Engagement
The Azure-Analytics-and-AI-Engagement repository provides packaged Industry Scenario DREAM Demos with ARM templates (Containing a demo web application, Power BI reports, Synapse resources, AML Notebooks etc.) that can be deployed in a customer’s subscription using the CAPE tool within a matter of few hours. Partners can also deploy DREAM Demos in their own subscriptions using DPoC.
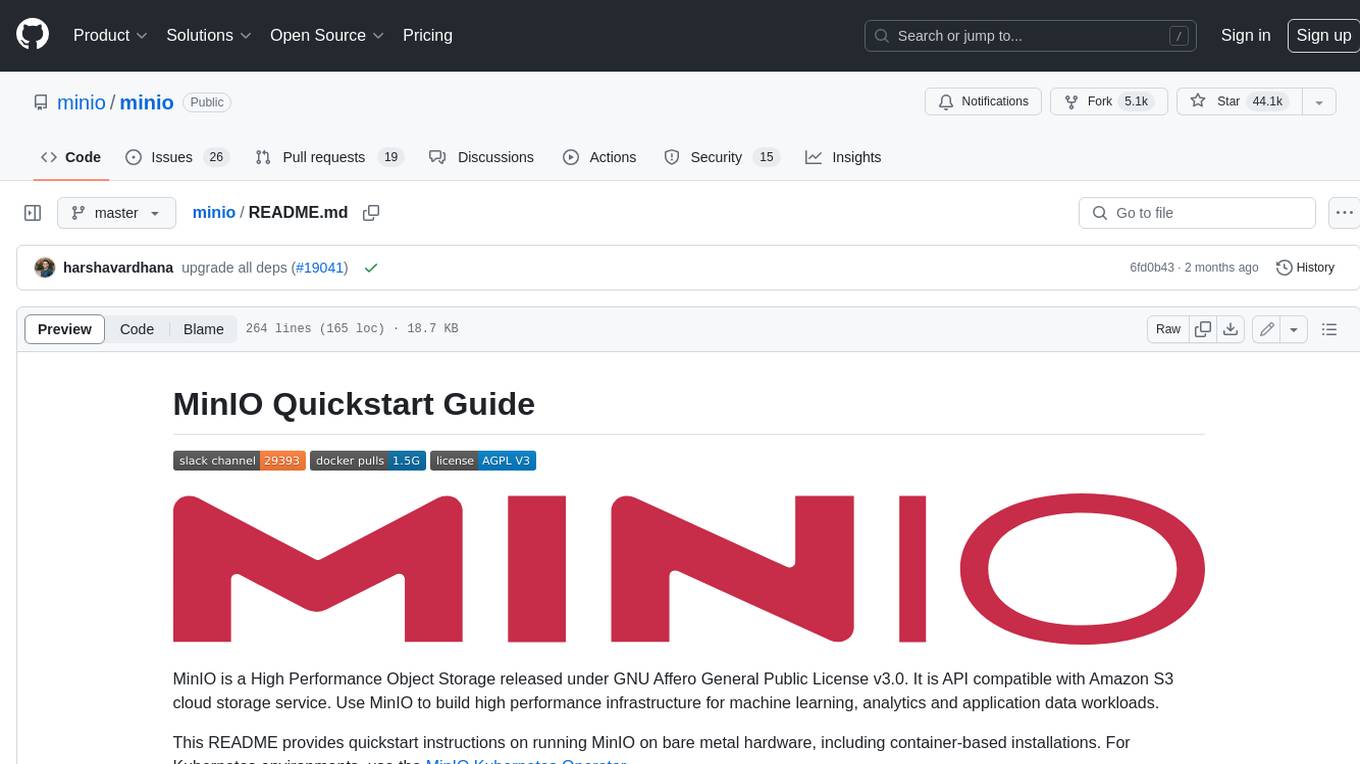
minio
MinIO is a High Performance Object Storage released under GNU Affero General Public License v3.0. It is API compatible with Amazon S3 cloud storage service. Use MinIO to build high performance infrastructure for machine learning, analytics and application data workloads.
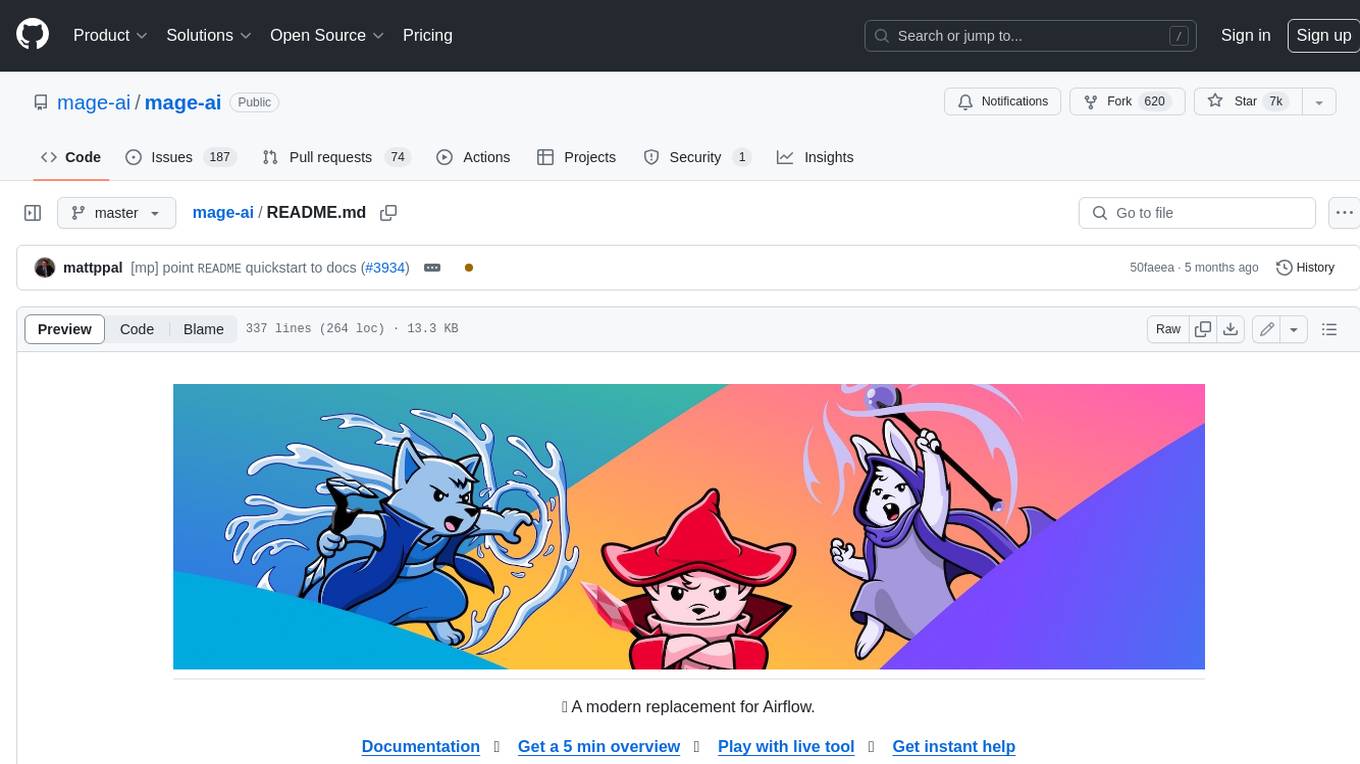
mage-ai
Mage is an open-source data pipeline tool for transforming and integrating data. It offers an easy developer experience, engineering best practices built-in, and data as a first-class citizen. Mage makes it easy to build, preview, and launch data pipelines, and provides observability and scaling capabilities. It supports data integrations, streaming pipelines, and dbt integration.
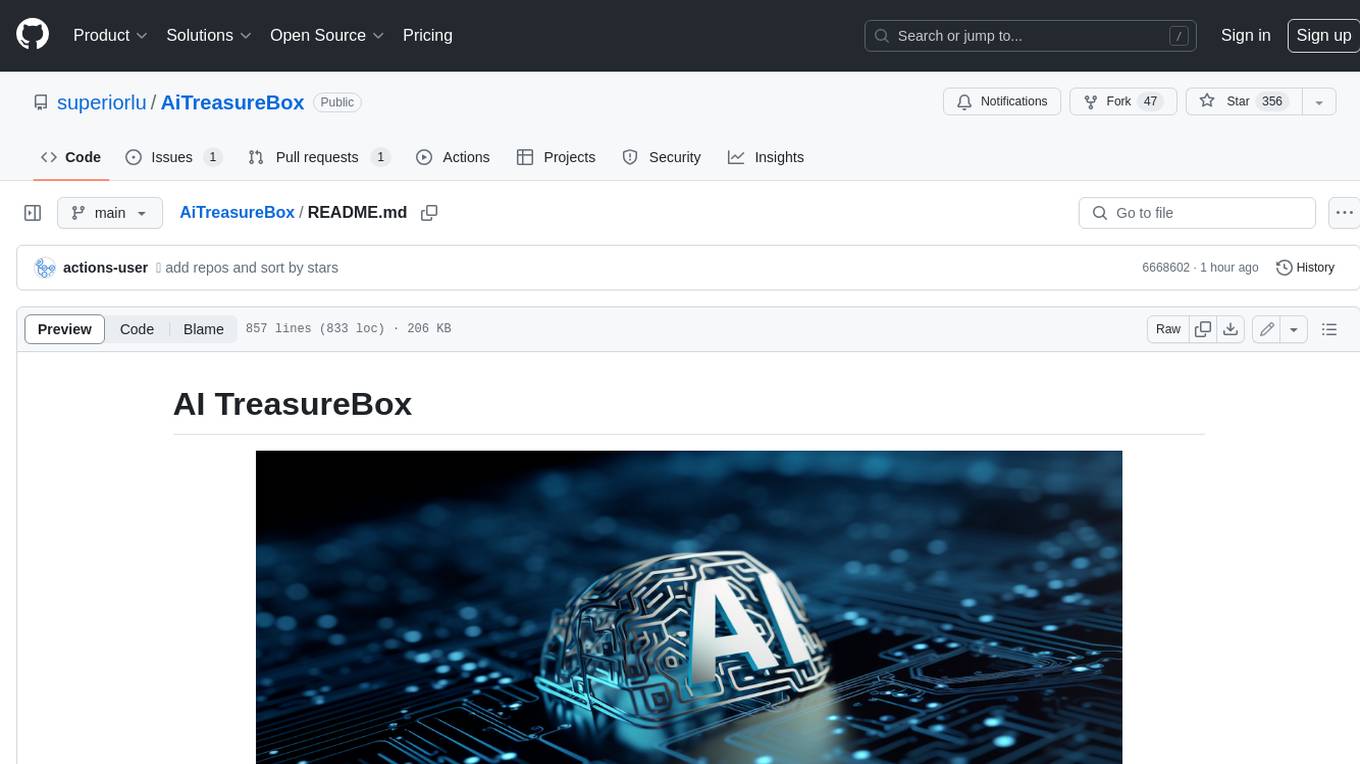
AiTreasureBox
AiTreasureBox is a versatile AI tool that provides a collection of pre-trained models and algorithms for various machine learning tasks. It simplifies the process of implementing AI solutions by offering ready-to-use components that can be easily integrated into projects. With AiTreasureBox, users can quickly prototype and deploy AI applications without the need for extensive knowledge in machine learning or deep learning. The tool covers a wide range of tasks such as image classification, text generation, sentiment analysis, object detection, and more. It is designed to be user-friendly and accessible to both beginners and experienced developers, making AI development more efficient and accessible to a wider audience.
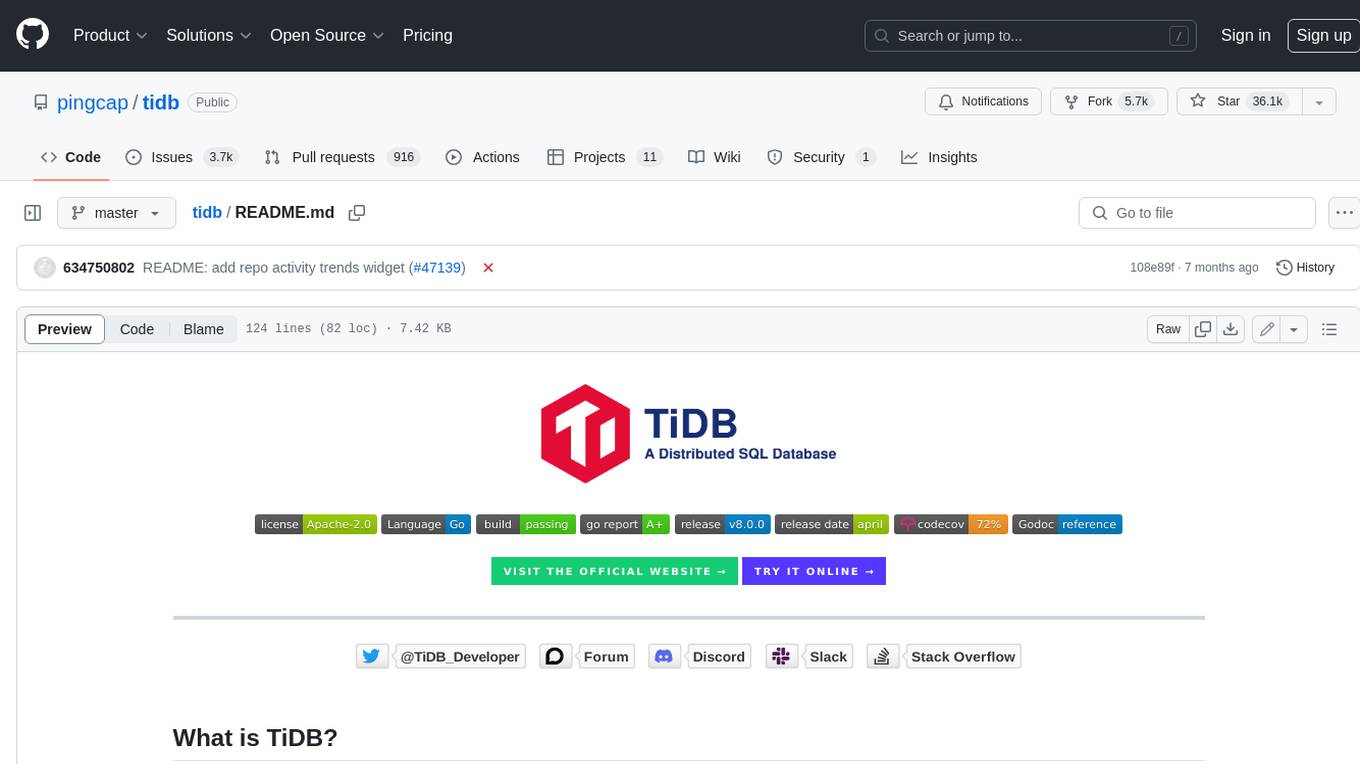
tidb
TiDB is an open-source distributed SQL database that supports Hybrid Transactional and Analytical Processing (HTAP) workloads. It is MySQL compatible and features horizontal scalability, strong consistency, and high availability.
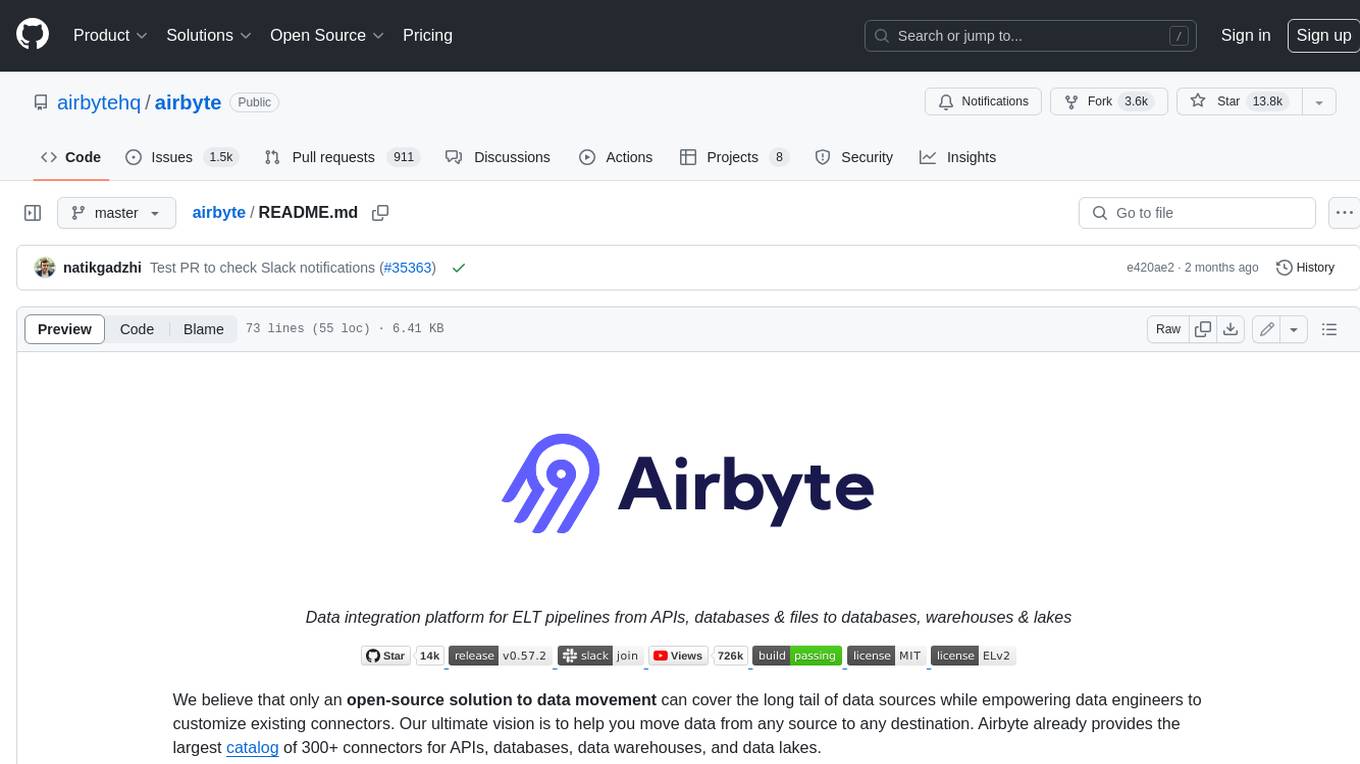
airbyte
Airbyte is an open-source data integration platform that makes it easy to move data from any source to any destination. With Airbyte, you can build and manage data pipelines without writing any code. Airbyte provides a library of pre-built connectors that make it easy to connect to popular data sources and destinations. You can also create your own connectors using Airbyte's no-code Connector Builder or low-code CDK. Airbyte is used by data engineers and analysts at companies of all sizes to build and manage their data pipelines.
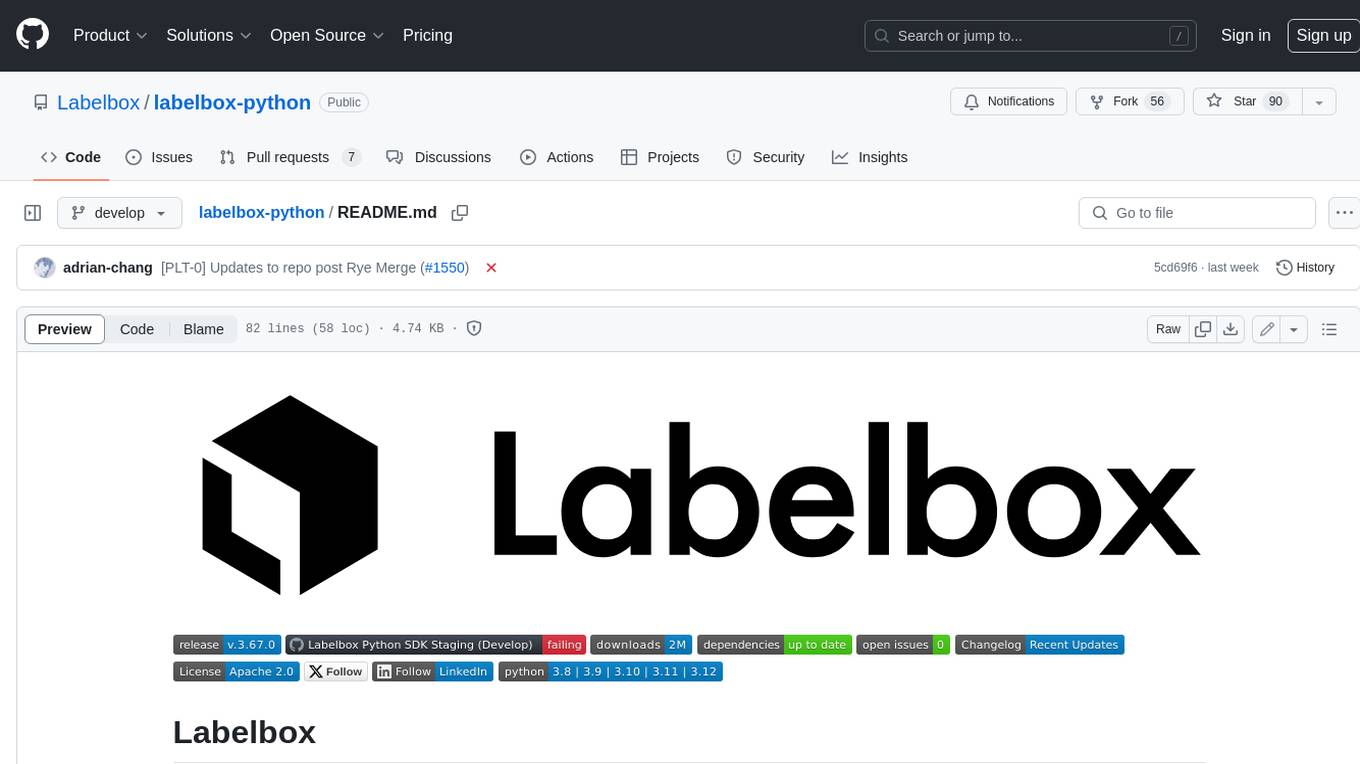
labelbox-python
Labelbox is a data-centric AI platform for enterprises to develop, optimize, and use AI to solve problems and power new products and services. Enterprises use Labelbox to curate data, generate high-quality human feedback data for computer vision and LLMs, evaluate model performance, and automate tasks by combining AI and human-centric workflows. The academic & research community uses Labelbox for cutting-edge AI research.