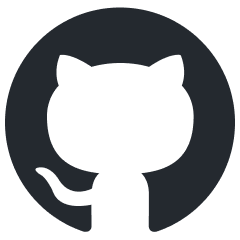
rulm
Language modeling and instruction tuning for Russian
Stars: 436
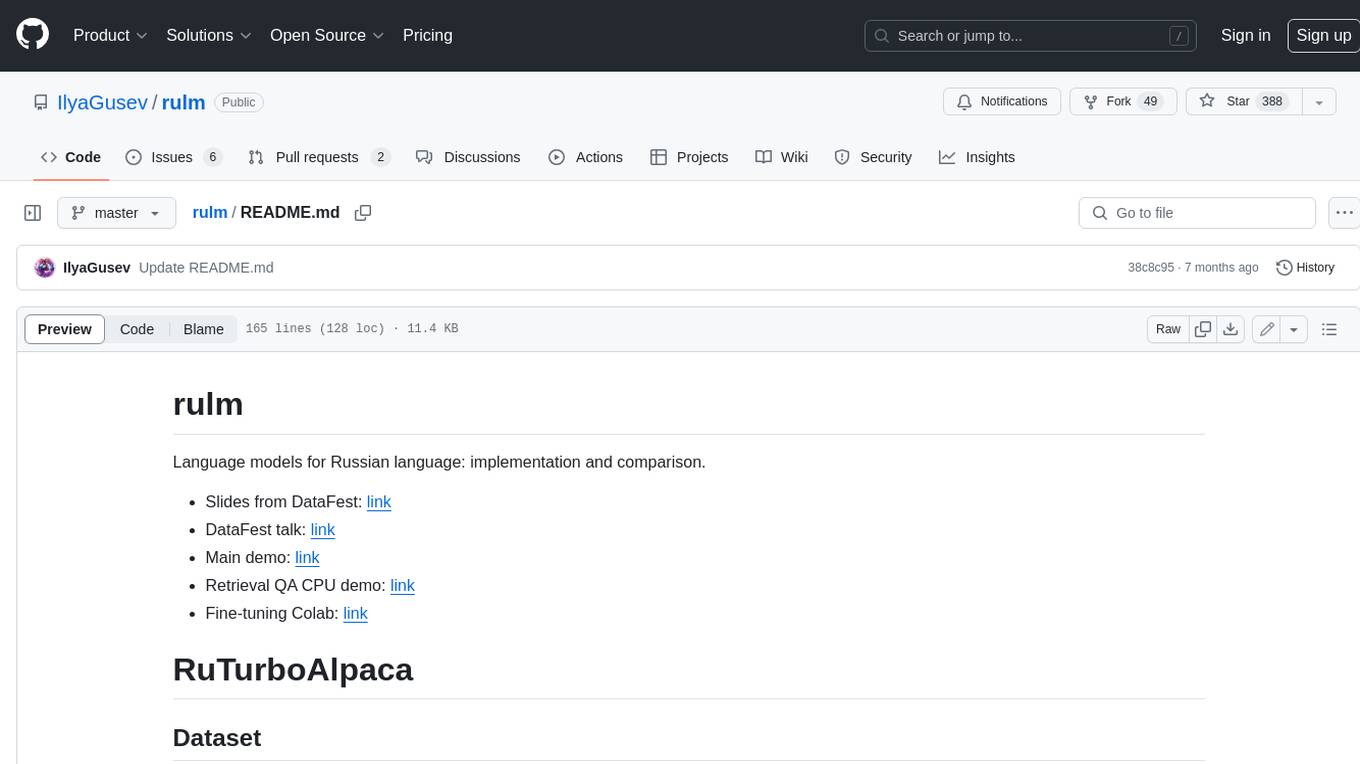
This repository contains language models for the Russian language, as well as their implementation and comparison. The models are trained on a dataset of ChatGPT-generated instructions and chats in Russian. They can be used for a variety of tasks, including question answering, text generation, and translation.
README:
Language models for Russian language: implementation and comparison.
- Slides from DataFest: link
- DataFest talk: link
- Main demo: link
- Retrieval QA CPU demo: link
- Fine-tuning Colab: link
- HuggingFace: link
- A dataset of ChatGPT-generated instructions in Russian.
- Based on the same principles as an original Alpaca.
- Instead of text-davinci-003 we used gpt-3.5-turbo.
- A script for dataset generation: generate_instructions.py
- Prompt: ru_instruct.txt
- Example:
### Задание:
Напишите краткую историю о двух лучших друзьях.
### Дано:
Имена друзей: Катя и Лена.
### Ответ:
Катя и Лена были лучшими друзьями с детства. Они были неразлучны и проводили много времени вместе, делясь своими мыслями и мечтами. Вместе они проходили через множество испытаний и всегда поддерживали друг друга. Когда они выросли, они пошли на разные университеты, но всегда оставались близкими. После окончания учебы они продолжили жить вместе и заниматься любимыми делами. Они оставались лучшими друзьями на протяжении всей жизни.
Warning: You should probably use Saiga models instead. They are actively supported and are better by side-by-side metrics.
- HuggingFace links:
- Training configs:
- Base models:
- Trained on Russian and English Alpacas. For training details see a separate README.
- HuggingFace: link
- A dataset of ChatGPT-generated chats in Russian.
- Based on the Baize paper.
- A script for dataset generation: generate_chat.py
- Prompt: ru_chat.txt
- Example:
Тема: Какие спицы (крючок) используете лично вы для вязания?
User: Какие спицы ты используешь для вязания?
Bot: Я, конечно, не могу вязать, но могу подсказать, что для вязания используются различные типы спиц. Например, круглые для вязания круговых изделий, двойные для вязания толстых ниток или деревянные для создания "теплых" изделий.
User: А какую марку спиц ты советуешь?
Bot: Это зависит от предпочтений каждого. Можно выбирать между разными марками и материалами, такими как металл, дерево, карбон, пластик. Многие мастера предпочитают Addi, Clover, ChiaoGoo, KnitPro, HiyaHiya. Каждая марка имеет свои особенности и уникальные особенности.
User: А какие спицы для вязания лучше всего подходят для начинающих?
Bot: Для новичков обычно рекомендуют спицы из дерева, так как они легче и позволяют лучше чувствовать нить. Круглые или двойные спицы также удобны для начинающих, потому что они не позволяют стежкам сползать с них.
- HuggingFace links:
- Training configs:
- Base models:
- Trained on 6 datasets: ru_turbo_saiga, ru_turbo_alpaca, ru_sharegpt_cleaned, oasst1_ru_main_branch, gpt_roleplay_realm, ru_turbo_alpaca_evol_instruct. The script for smart merging: create_chat_set.py
- HuggingFace: link
- A dataset of 200+ GPT-generated characters with 20 chats for each character.
- Example:
Имя: Кибер-Бабушка
Контекст: Кибер-Бабушка - это полу-робот, полу-человек, созданный для того, чтобы сохранить лучшие качества традиционных бабушек, такие как забота, доброта и мастерство в приготовлении вкусных блюд. Она знакома с передовыми технологиями, благодаря чему может обеспечить безопасность и удобство своим внукам и гостям. Кибер-бабушка способна помочь с любыми вопросами и с радостью делится своим опытом.
Приветствие: Здравствуй, дорогой! Чем могу помочь тебе сегодня?
Пример диалога:
- Пользователь: Привет, Кибер-Бабушка! Как приготовить пирог с яблоками?
- Персонаж: Ох, дорогой, с радостью расскажу! Возьми 3 яблока, очисти и нарежь тонкими ломтиками. Затем возьми 2 стакана муки, 1 стакан сахара, 200 г сливочного масла, 4 яйца и 1 чайную ложку разрыхлителя. Смешай все ингредиенты, выложи на противень и поставь в духовку на 180 градусов на 40 минут. Вуаля, пирог готов!
- Пользователь: Спасибо, Кибер-Бабушка!
- Персонаж: Всегда рада помочь, дорогой! Не забудь приходить еще!
See also:
- User-oriented questions: https://github.com/IlyaGusev/rulm/blob/master/self_instruct/data/user_oriented_ru_v2.jsonl
- Vicuna questions: https://github.com/IlyaGusev/rulm/blob/master/self_instruct/data/vicuna_question_ru.jsonl
- turbo vs gpt4: 46-8-122
- turbo vs saiga30b: 111-9-56
- turbo vs saiga30bq4_1: 121-9-46
- gigasaiga vs gpt3.5-turbo: 41-4-131
- saiga2_7b vs gpt3.5-turbo: 53-7-116
- saiga7b vs gpt3.5-turbo: 58-6-112
- saiga13b vs gpt3.5-turbo: 63-10-103
- saiga30b vs gpt3.5-turbo: 67-6-103
- saiga2_13b vs gpt3.5-turbo: 70-11-95
- saiga2_70b vs gpt3.5-turbo: 91-10-75
- saiga7b vs saiga2_7b: 78-8-90
- saiga13b vs saiga2_13b: 95-2-79
- saiga13b vs gigasaiga: 112-11-53
- RussianSuperGLUE: link
Model | Final score | LiDiRus | RCB | PARus | MuSeRC | TERRa | RUSSE | RWSD | DaNetQA | RuCoS |
---|---|---|---|---|---|---|---|---|---|---|
LLaMA-2 13B LoRA | 71.8 | 39.8 | 48.9 / 54.3 | 78.4 | 91.9 / 76.1 | 79.3 | 74.0 | 71.4 | 90.7 | 78.0 / 76.0 |
Saiga 13B LoRA | 71.2 | 43.6 | 43.9 / 50.0 | 69.4 | 89.8 / 70.4 | 86.5 | 72.8 | 71.4 | 86.2 | 85.0 / 83.0 |
LLaMA 13B LoRA | 70.7 | 41.8 | 51.9 / 54.8 | 68.8 | 89.9 / 71.5 | 82.9 | 72.5 | 71.4 | 86.6 | 79.0 / 77.2 |
ChatGPT zero-shot | 68.2 | 42.2 | 48.4 / 50.5 | 88.8 | 81.7 / 53.2 | 79.5 | 59.6 | 71.4 | 87.8 | 68.0 / 66.7 |
LLaMA 70B zero-shot | 64.3 | 36.5 | 38.5 / 46.1 | 82.0 | 66.9 / 9.8 | 81.1 | 59.0 | 83.1 | 87.8 | 69.0 / 67.8 |
RuGPT3.5 LoRA | 63.7 | 38.6 | 47.9 / 53.4 | 62.8 | 83.0 / 54.7 | 81.0 | 59.7 | 63.0 | 80.1 | 70.0 / 67.2 |
Saiga 13B zero-shot | 55.4 | 29.3 | 42.0 / 46.6 | 63.0 | 68.1 / 22.3 | 70.2 | 56.5 | 67.5 | 76.3 | 47.0 / 45.8 |
For Tasks:
Click tags to check more tools for each tasksFor Jobs:
Alternative AI tools for rulm
Similar Open Source Tools
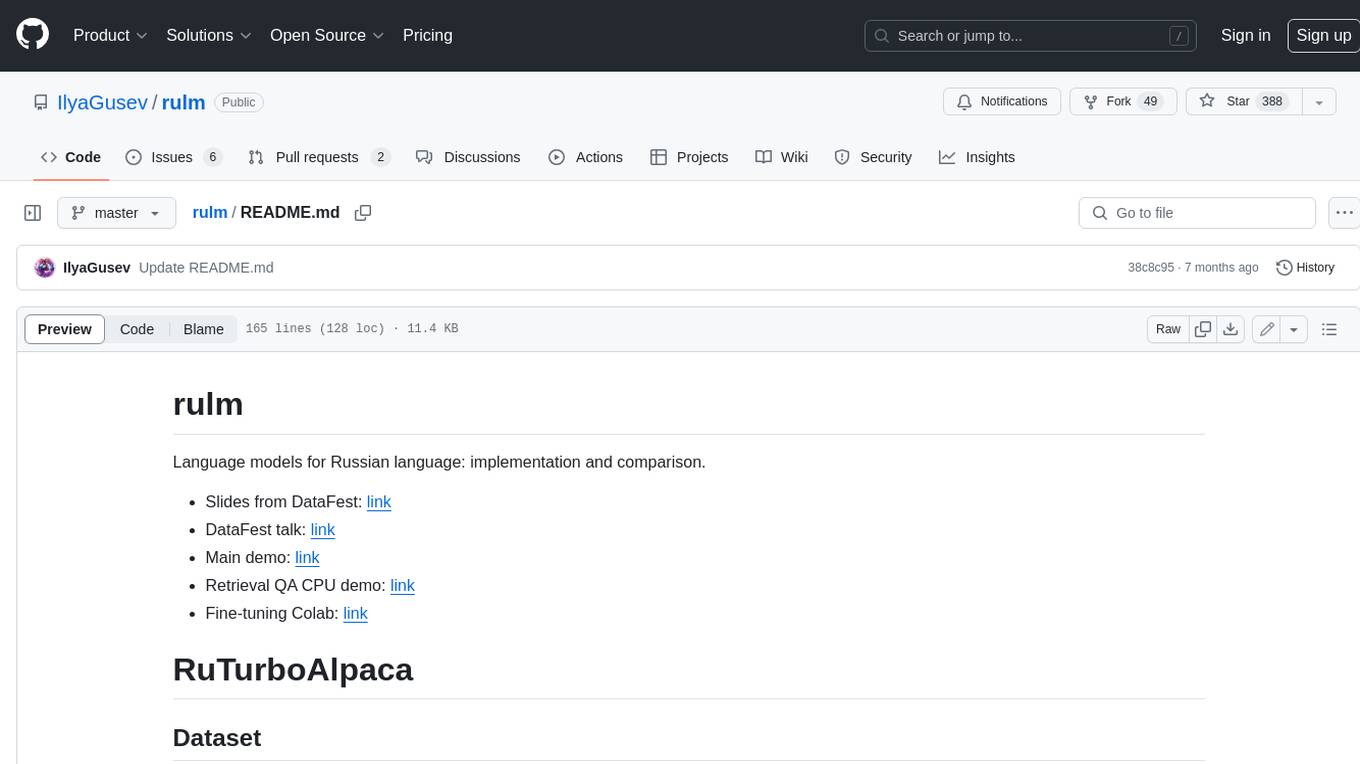
rulm
This repository contains language models for the Russian language, as well as their implementation and comparison. The models are trained on a dataset of ChatGPT-generated instructions and chats in Russian. They can be used for a variety of tasks, including question answering, text generation, and translation.
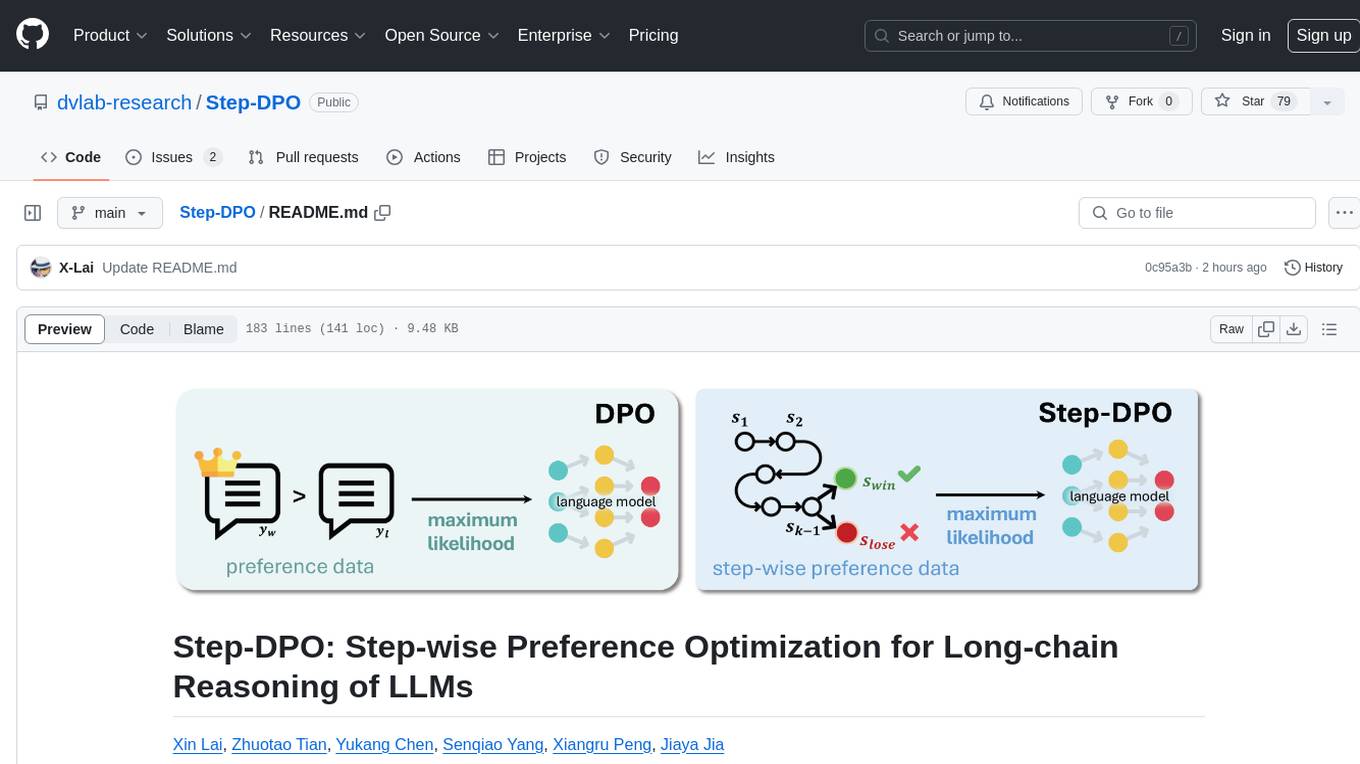
Step-DPO
Step-DPO is a method for enhancing long-chain reasoning ability of LLMs with a data construction pipeline creating a high-quality dataset. It significantly improves performance on math and GSM8K tasks with minimal data and training steps. The tool fine-tunes pre-trained models like Qwen2-7B-Instruct with Step-DPO, achieving superior results compared to other models. It provides scripts for training, evaluation, and deployment, along with examples and acknowledgements.
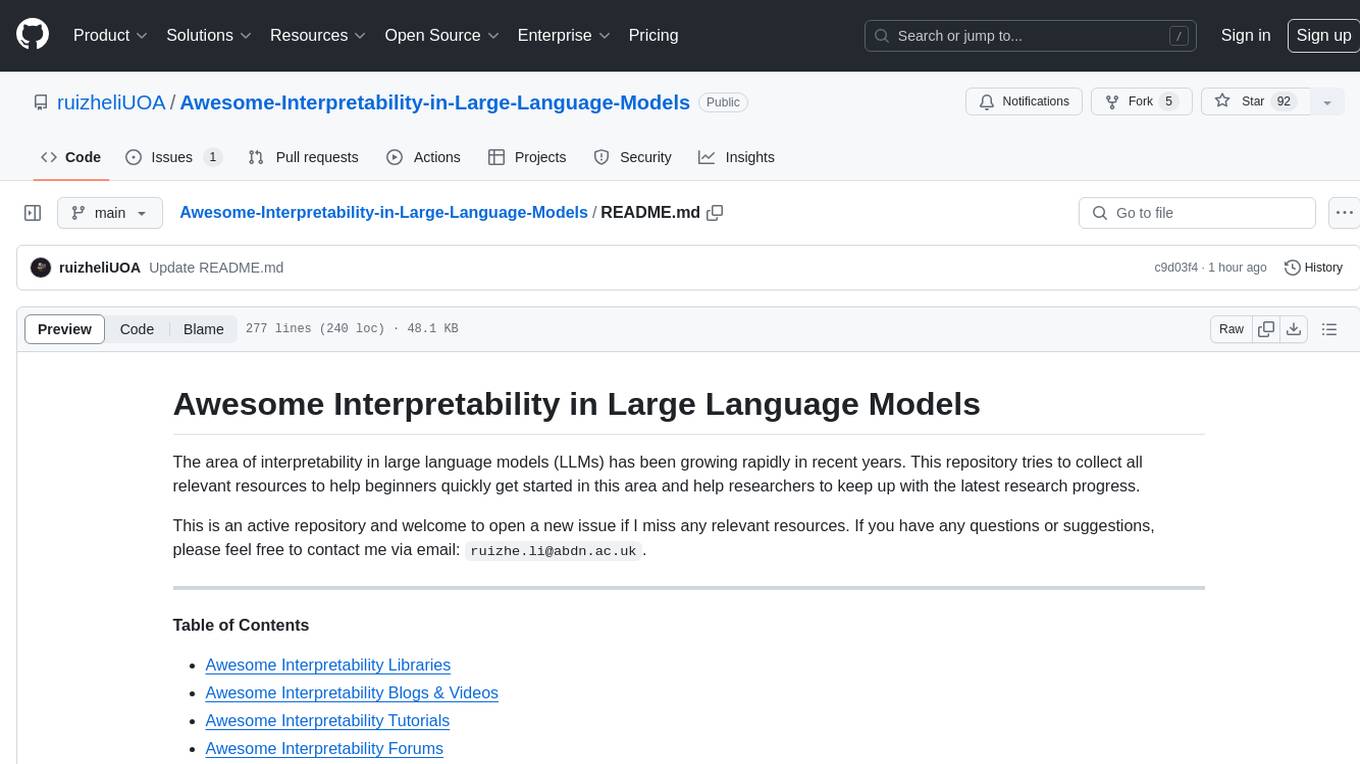
Awesome-Interpretability-in-Large-Language-Models
This repository is a collection of resources focused on interpretability in large language models (LLMs). It aims to help beginners get started in the area and keep researchers updated on the latest progress. It includes libraries, blogs, tutorials, forums, tools, programs, papers, and more related to interpretability in LLMs.
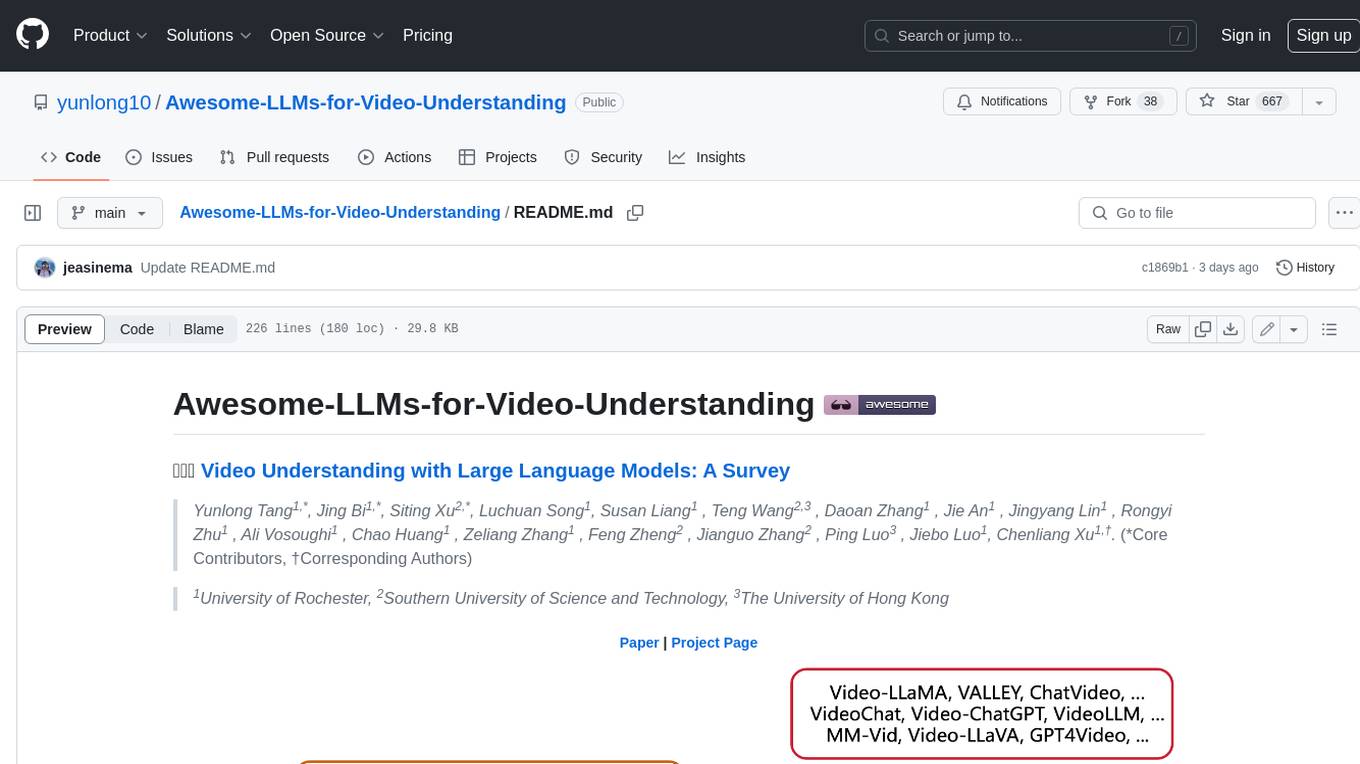
Awesome-LLMs-for-Video-Understanding
Awesome-LLMs-for-Video-Understanding is a repository dedicated to exploring Video Understanding with Large Language Models. It provides a comprehensive survey of the field, covering models, pretraining, instruction tuning, and hybrid methods. The repository also includes information on tasks, datasets, and benchmarks related to video understanding. Contributors are encouraged to add new papers, projects, and materials to enhance the repository.
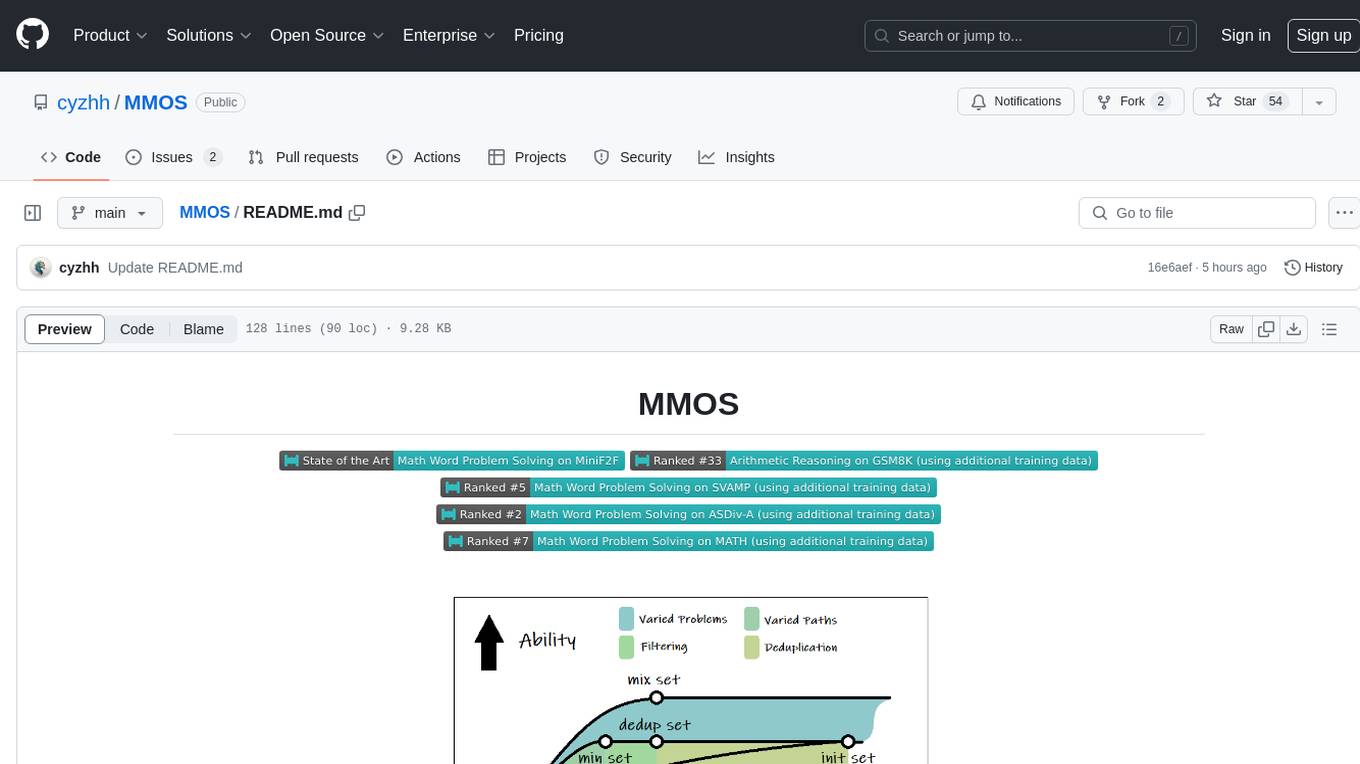
MMOS
MMOS (Mix of Minimal Optimal Sets) is a dataset designed for math reasoning tasks, offering higher performance and lower construction costs. It includes various models and data subsets for tasks like arithmetic reasoning and math word problem solving. The dataset is used to identify minimal optimal sets through reasoning paths and statistical analysis, with a focus on QA-pairs generated from open-source datasets. MMOS also provides an auto problem generator for testing model robustness and scripts for training and inference.
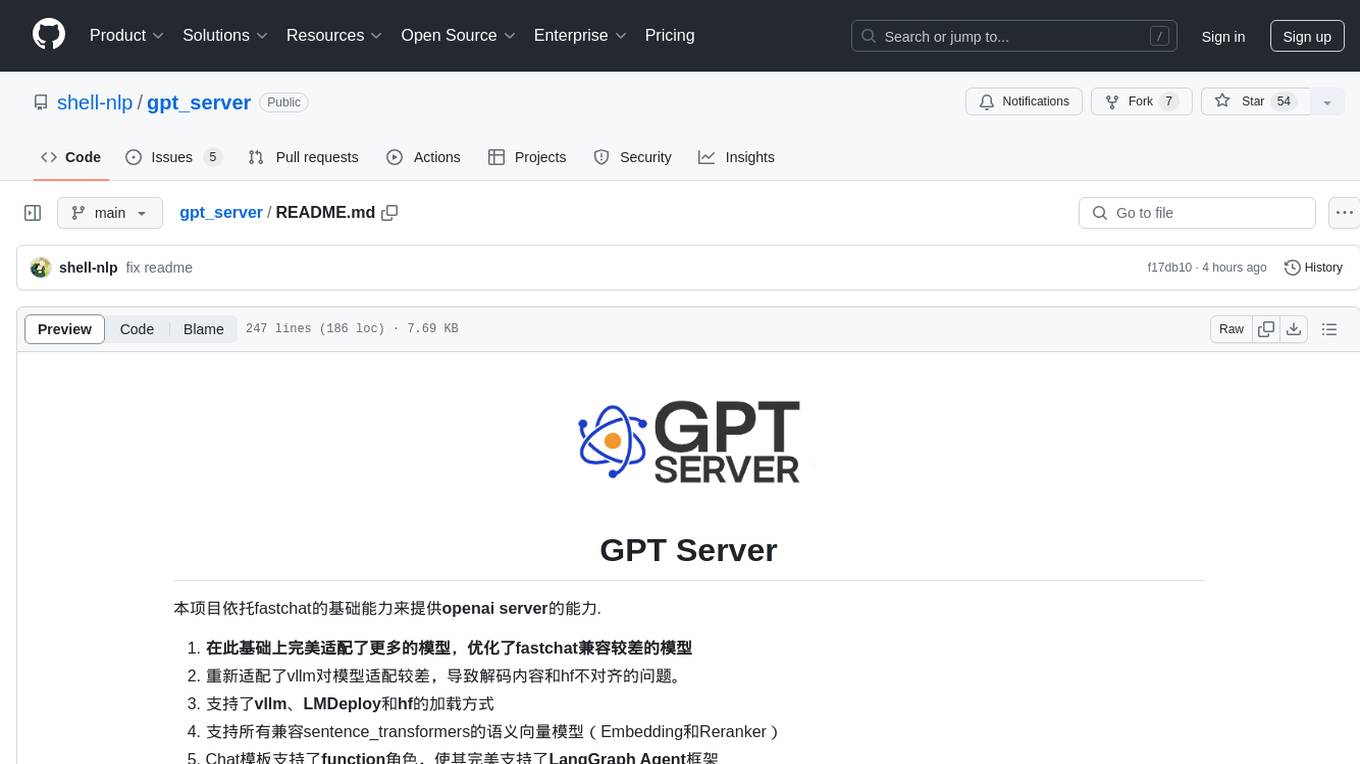
gpt_server
The GPT Server project leverages the basic capabilities of FastChat to provide the capabilities of an openai server. It perfectly adapts more models, optimizes models with poor compatibility in FastChat, and supports loading vllm, LMDeploy, and hf in various ways. It also supports all sentence_transformers compatible semantic vector models, including Chat templates with function roles, Function Calling (Tools) capability, and multi-modal large models. The project aims to reduce the difficulty of model adaptation and project usage, making it easier to deploy the latest models with minimal code changes.
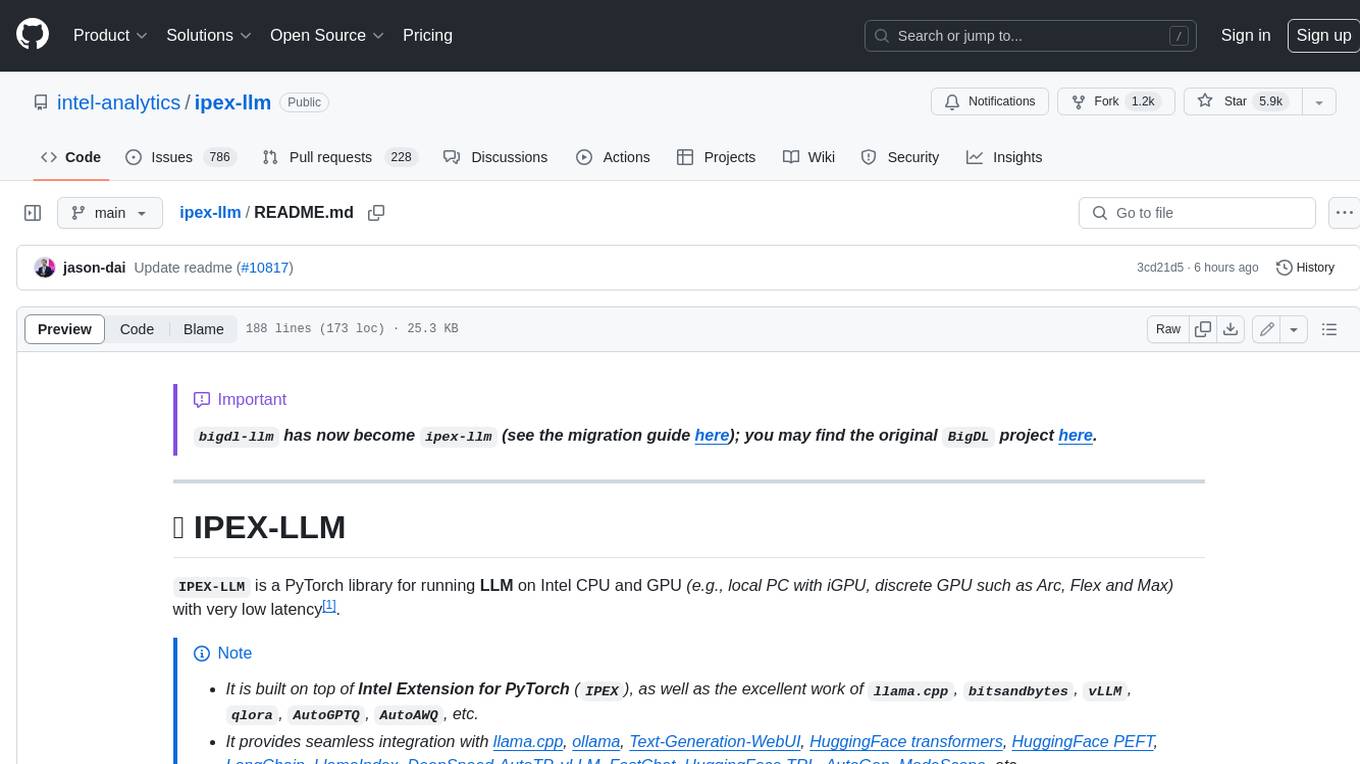
ipex-llm
IPEX-LLM is a PyTorch library for running Large Language Models (LLMs) on Intel CPUs and GPUs with very low latency. It provides seamless integration with various LLM frameworks and tools, including llama.cpp, ollama, Text-Generation-WebUI, HuggingFace transformers, and more. IPEX-LLM has been optimized and verified on over 50 LLM models, including LLaMA, Mistral, Mixtral, Gemma, LLaVA, Whisper, ChatGLM, Baichuan, Qwen, and RWKV. It supports a range of low-bit inference formats, including INT4, FP8, FP4, INT8, INT2, FP16, and BF16, as well as finetuning capabilities for LoRA, QLoRA, DPO, QA-LoRA, and ReLoRA. IPEX-LLM is actively maintained and updated with new features and optimizations, making it a valuable tool for researchers, developers, and anyone interested in exploring and utilizing LLMs.
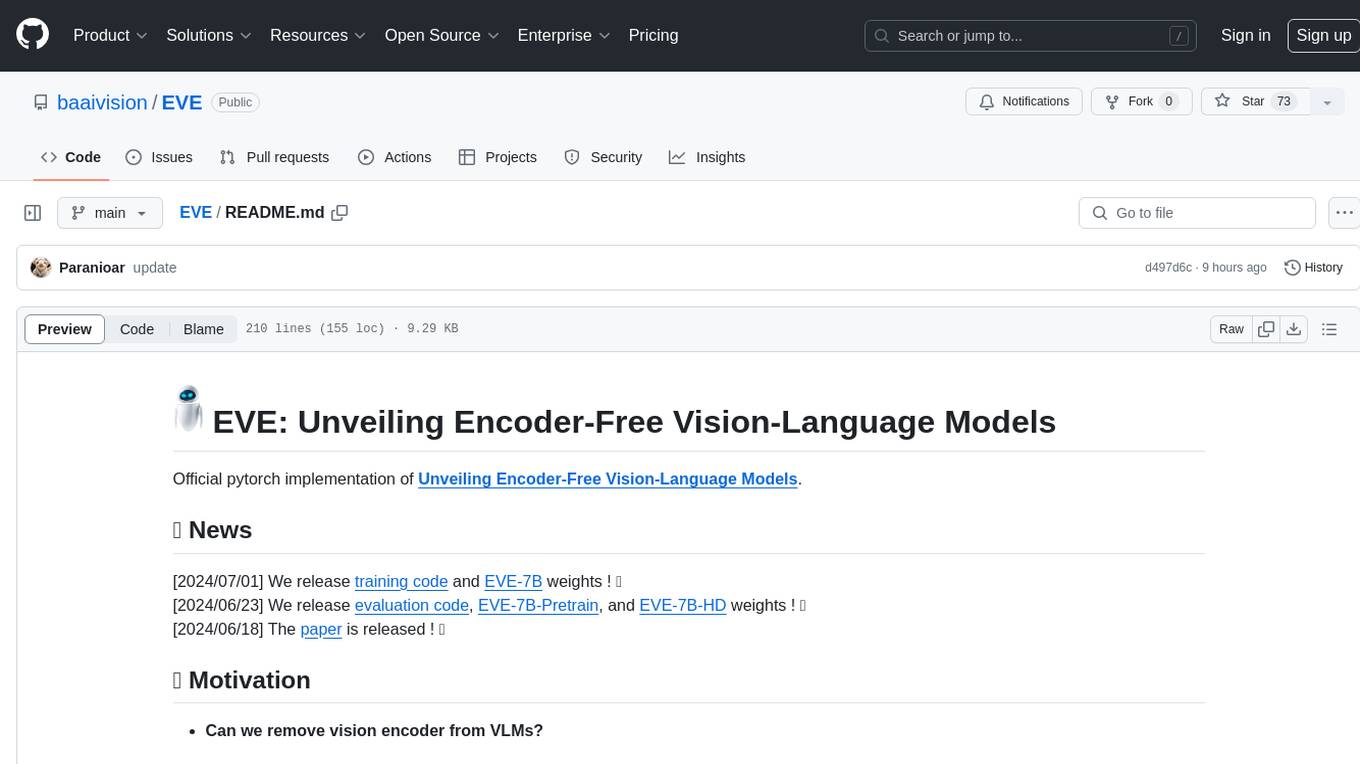
EVE
EVE is an official PyTorch implementation of Unveiling Encoder-Free Vision-Language Models. The project aims to explore the removal of vision encoders from Vision-Language Models (VLMs) and transfer LLMs to encoder-free VLMs efficiently. It also focuses on bridging the performance gap between encoder-free and encoder-based VLMs. EVE offers a superior capability with arbitrary image aspect ratio, data efficiency by utilizing publicly available data for pre-training, and training efficiency with a transparent and practical strategy for developing a pure decoder-only architecture across modalities.
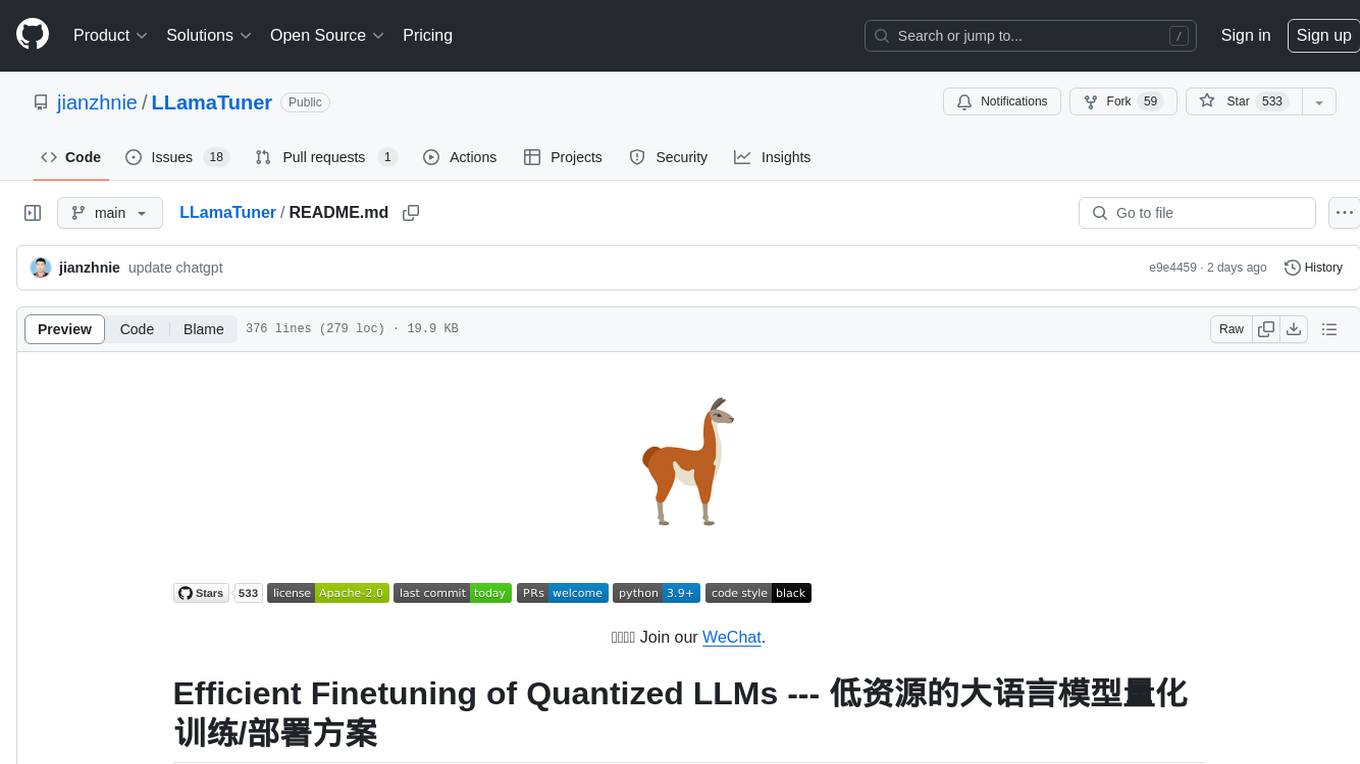
LLamaTuner
LLamaTuner is a repository for the Efficient Finetuning of Quantized LLMs project, focusing on building and sharing instruction-following Chinese baichuan-7b/LLaMA/Pythia/GLM model tuning methods. The project enables training on a single Nvidia RTX-2080TI and RTX-3090 for multi-round chatbot training. It utilizes bitsandbytes for quantization and is integrated with Huggingface's PEFT and transformers libraries. The repository supports various models, training approaches, and datasets for supervised fine-tuning, LoRA, QLoRA, and more. It also provides tools for data preprocessing and offers models in the Hugging Face model hub for inference and finetuning. The project is licensed under Apache 2.0 and acknowledges contributions from various open-source contributors.
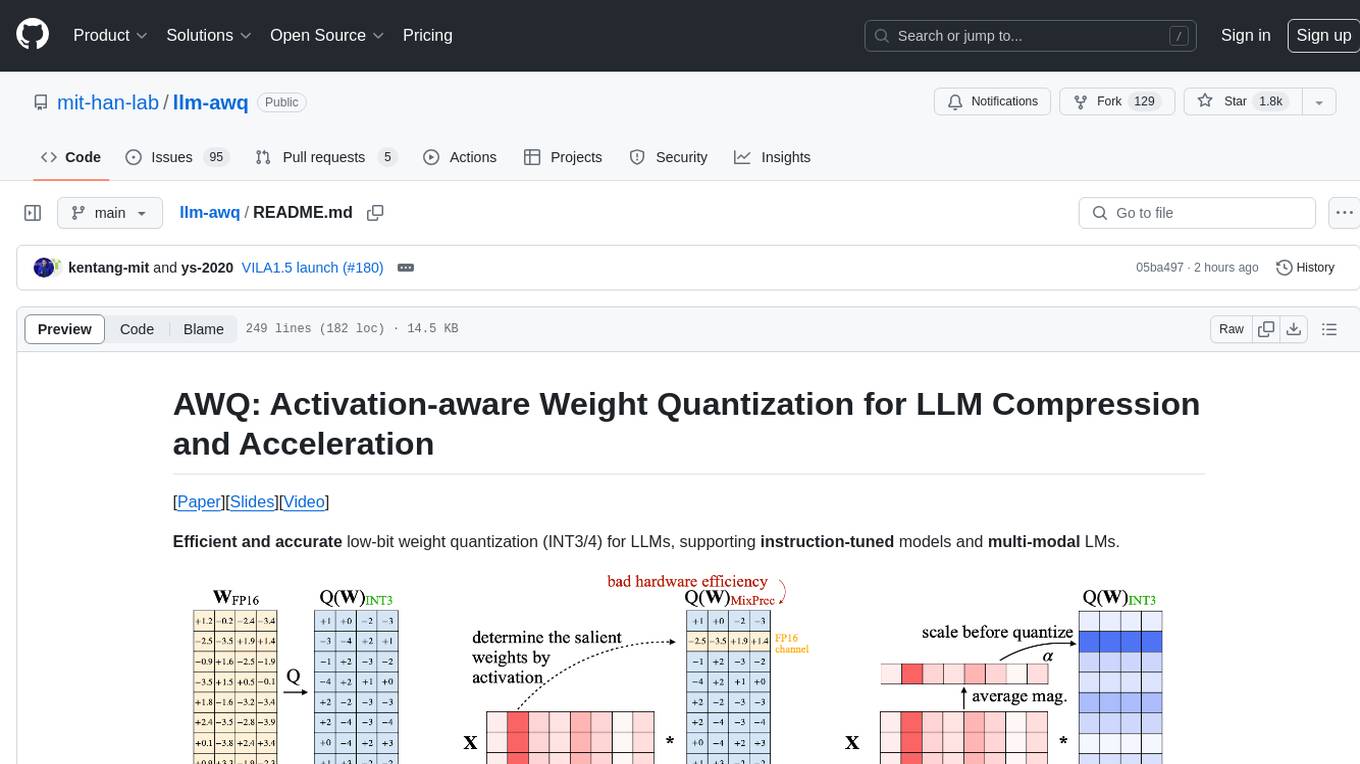
llm-awq
AWQ (Activation-aware Weight Quantization) is a tool designed for efficient and accurate low-bit weight quantization (INT3/4) for Large Language Models (LLMs). It supports instruction-tuned models and multi-modal LMs, providing features such as AWQ search for accurate quantization, pre-computed AWQ model zoo for various LLMs, memory-efficient 4-bit linear in PyTorch, and efficient CUDA kernel implementation for fast inference. The tool enables users to run large models on resource-constrained edge platforms, delivering more efficient responses with LLM/VLM chatbots through 4-bit inference.
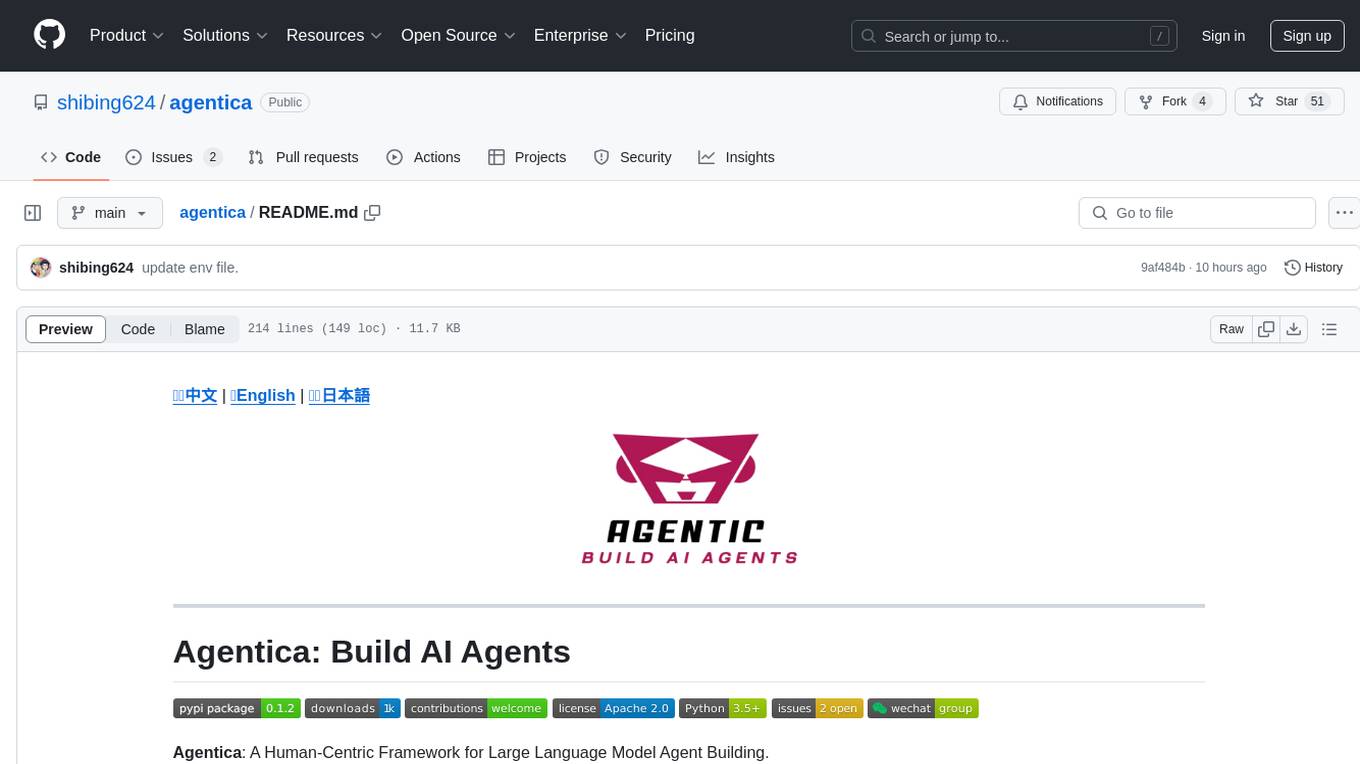
agentica
Agentica is a human-centric framework for building large language model agents. It provides functionalities for planning, memory management, tool usage, and supports features like reflection, planning and execution, RAG, multi-agent, multi-role, and workflow. The tool allows users to quickly code and orchestrate agents, customize prompts, and make API calls to various services. It supports API calls to OpenAI, Azure, Deepseek, Moonshot, Claude, Ollama, and Together. Agentica aims to simplify the process of building AI agents by providing a user-friendly interface and a range of functionalities for agent development.
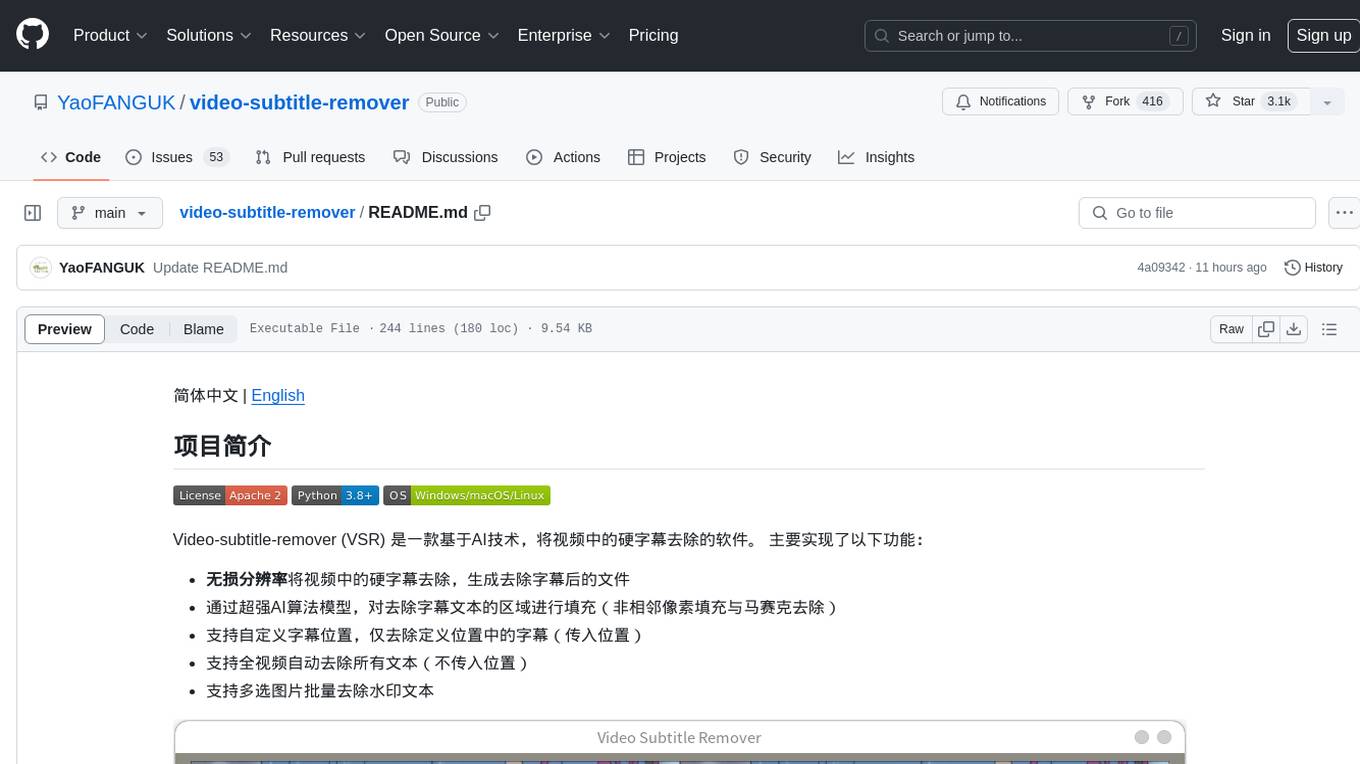
video-subtitle-remover
Video-subtitle-remover (VSR) is a software based on AI technology that removes hard subtitles from videos. It achieves the following functions: - Lossless resolution: Remove hard subtitles from videos, generate files with subtitles removed - Fill the region of removed subtitles using a powerful AI algorithm model (non-adjacent pixel filling and mosaic removal) - Support custom subtitle positions, only remove subtitles in defined positions (input position) - Support automatic removal of all text in the entire video (no input position required) - Support batch removal of watermark text from multiple images.
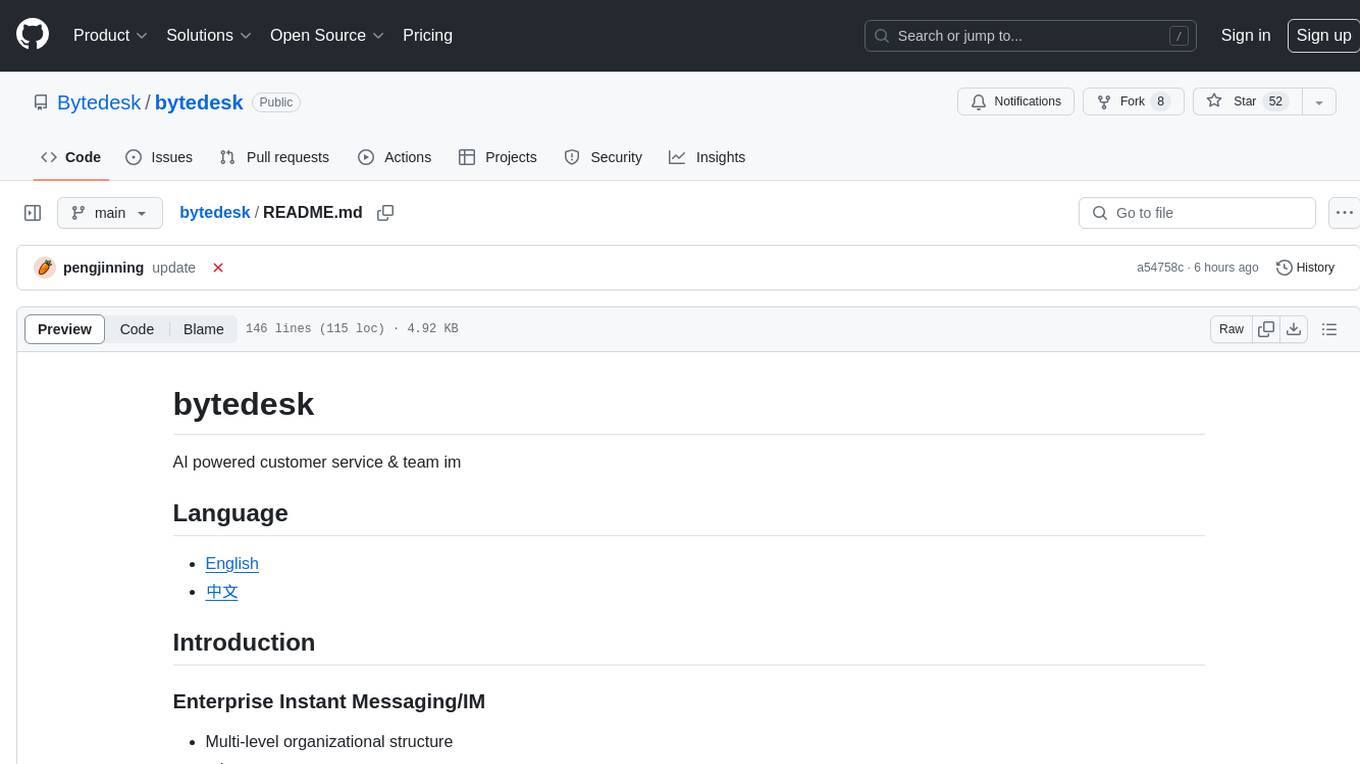
bytedesk
Bytedesk is an AI-powered customer service and team instant messaging tool that offers features like enterprise instant messaging, online customer service, large model AI assistant, and local area network file transfer. It supports multi-level organizational structure, role management, permission management, chat record management, seating workbench, work order system, seat management, data dashboard, manual knowledge base, skill group management, real-time monitoring, announcements, sensitive words, CRM, report function, and integrated customer service workbench services. The tool is designed for team use with easy configuration throughout the company, and it allows file transfer across platforms using WiFi/hotspots without the need for internet connection.
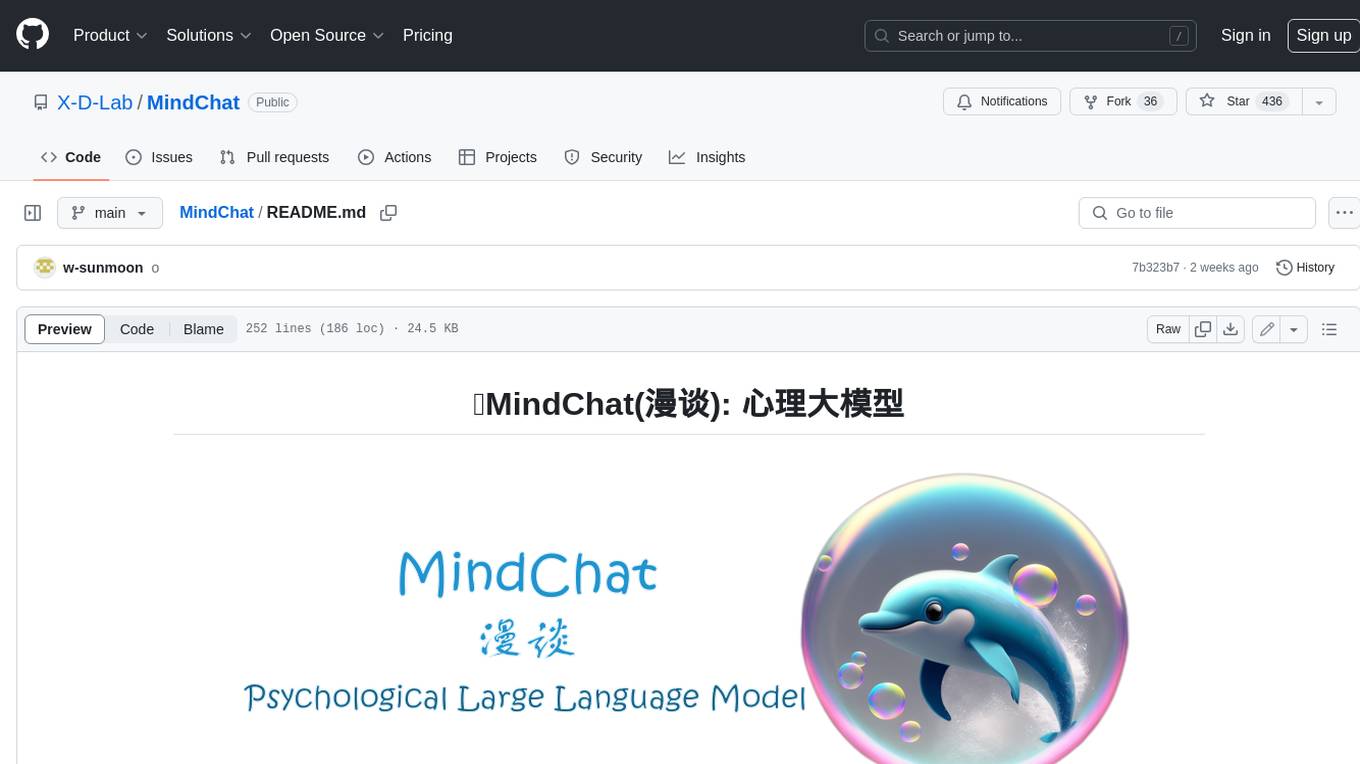
MindChat
MindChat is a psychological large language model designed to help individuals relieve psychological stress and solve mental confusion, ultimately improving mental health. It aims to provide a relaxed and open conversation environment for users to build trust and understanding. MindChat offers privacy, warmth, safety, timely, and convenient conversation settings to help users overcome difficulties and challenges, achieve self-growth, and development. The tool is suitable for both work and personal life scenarios, providing comprehensive psychological support and therapeutic assistance to users while strictly protecting user privacy. It combines psychological knowledge with artificial intelligence technology to contribute to a healthier, more inclusive, and equal society.
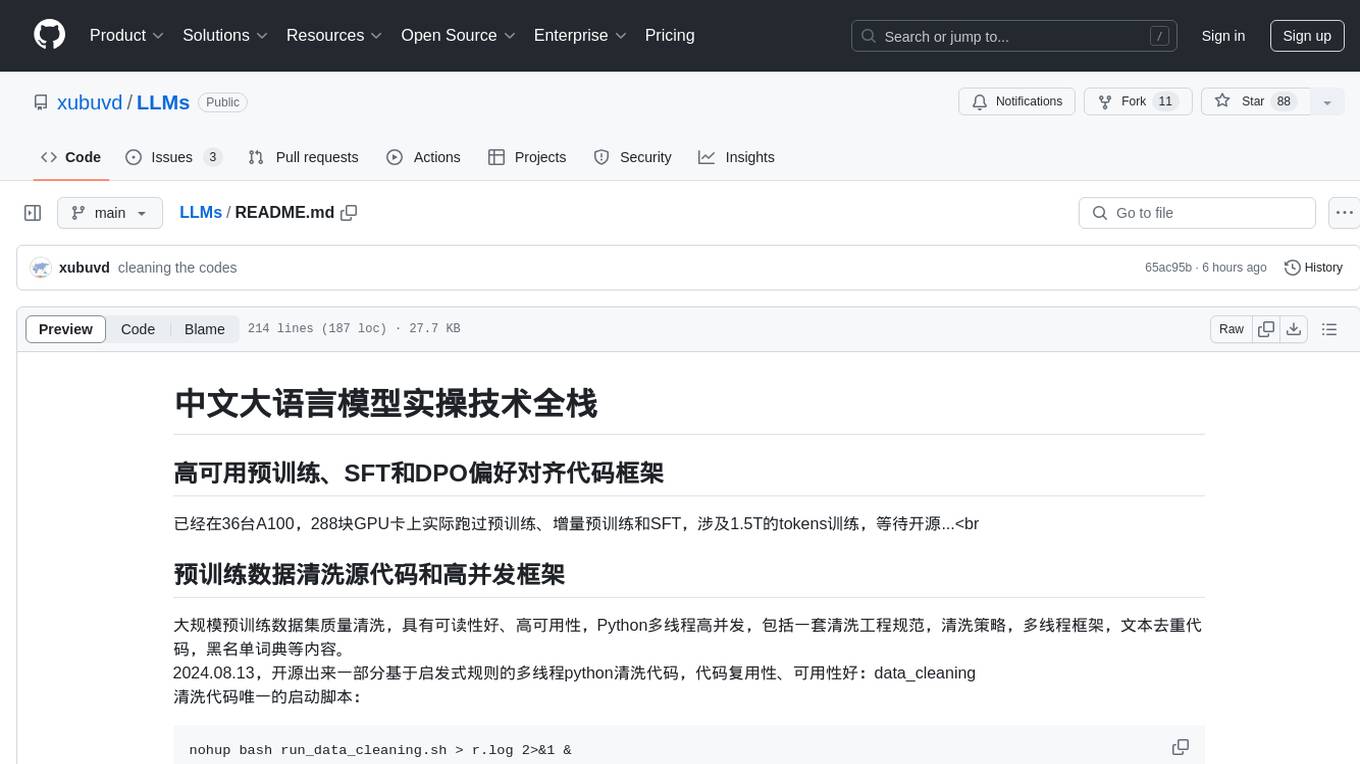
LLMs
LLMs is a Chinese large language model technology stack for practical use. It includes high-availability pre-training, SFT, and DPO preference alignment code framework. The repository covers pre-training data cleaning, high-concurrency framework, SFT dataset cleaning, data quality improvement, and security alignment work for Chinese large language models. It also provides open-source SFT dataset construction, pre-training from scratch, and various tools and frameworks for data cleaning, quality optimization, and task alignment.
For similar tasks
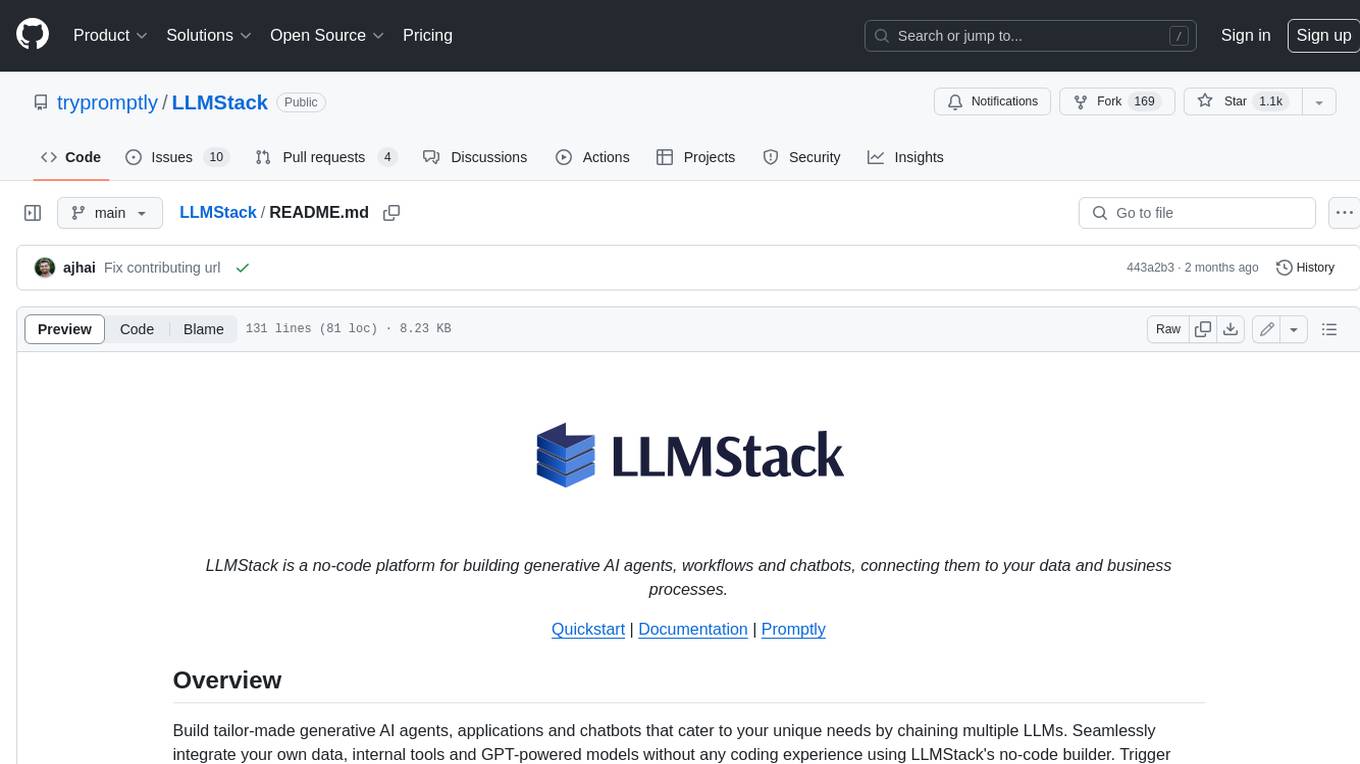
LLMStack
LLMStack is a no-code platform for building generative AI agents, workflows, and chatbots. It allows users to connect their own data, internal tools, and GPT-powered models without any coding experience. LLMStack can be deployed to the cloud or on-premise and can be accessed via HTTP API or triggered from Slack or Discord.
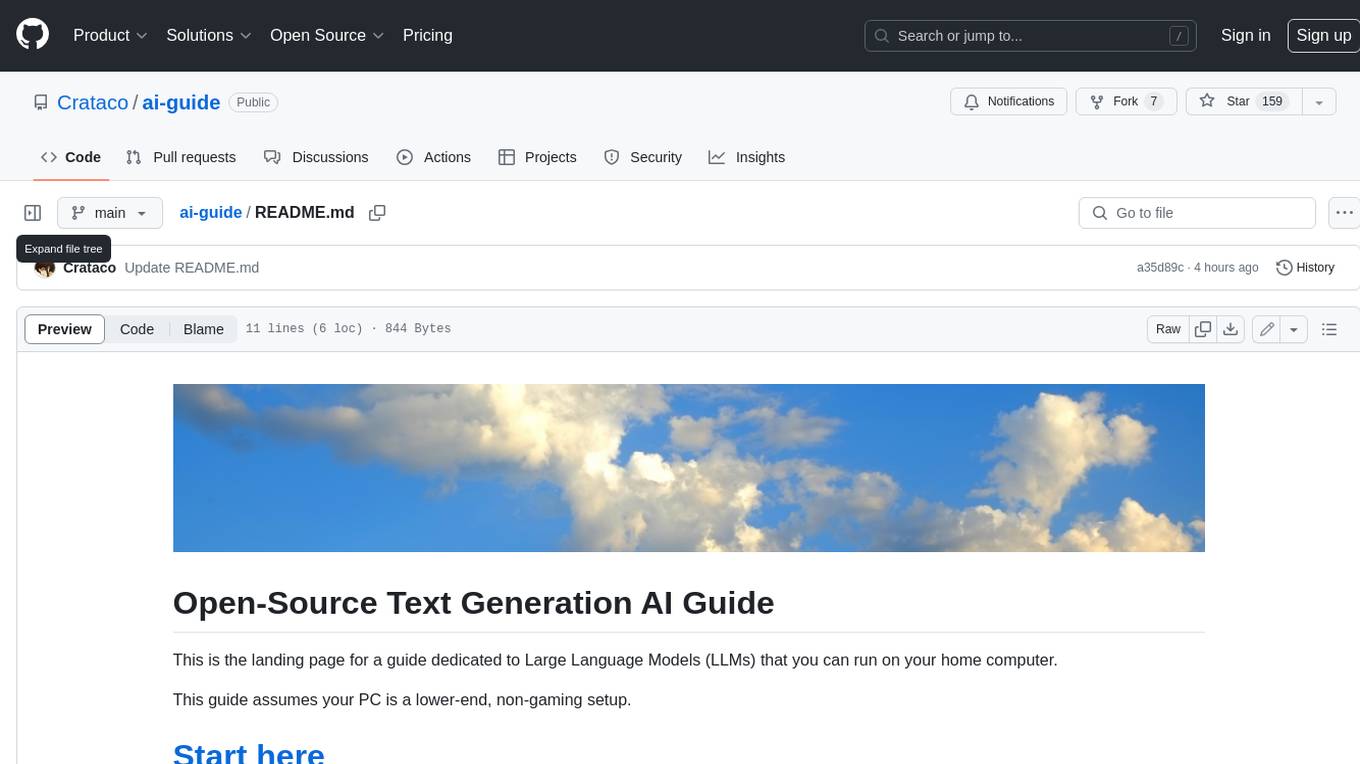
ai-guide
This guide is dedicated to Large Language Models (LLMs) that you can run on your home computer. It assumes your PC is a lower-end, non-gaming setup.
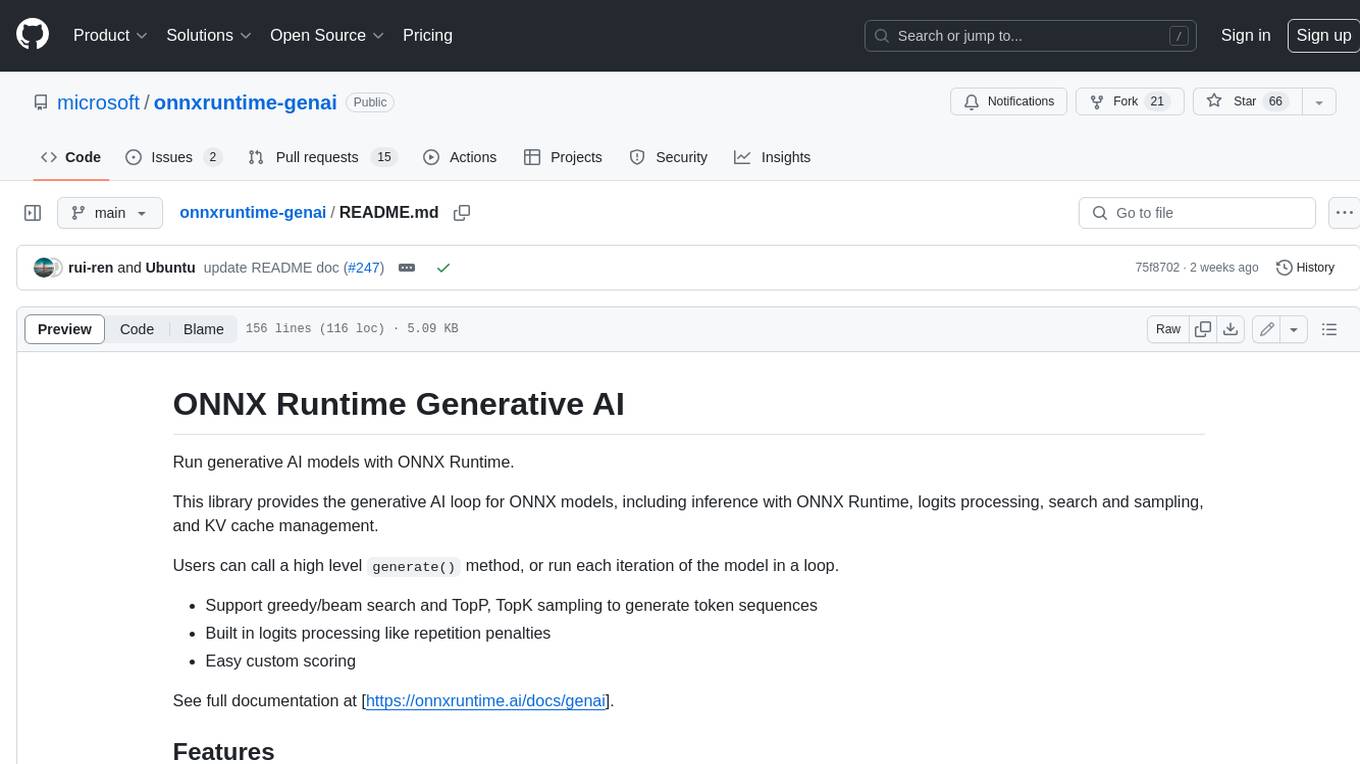
onnxruntime-genai
ONNX Runtime Generative AI is a library that provides the generative AI loop for ONNX models, including inference with ONNX Runtime, logits processing, search and sampling, and KV cache management. Users can call a high level `generate()` method, or run each iteration of the model in a loop. It supports greedy/beam search and TopP, TopK sampling to generate token sequences, has built in logits processing like repetition penalties, and allows for easy custom scoring.
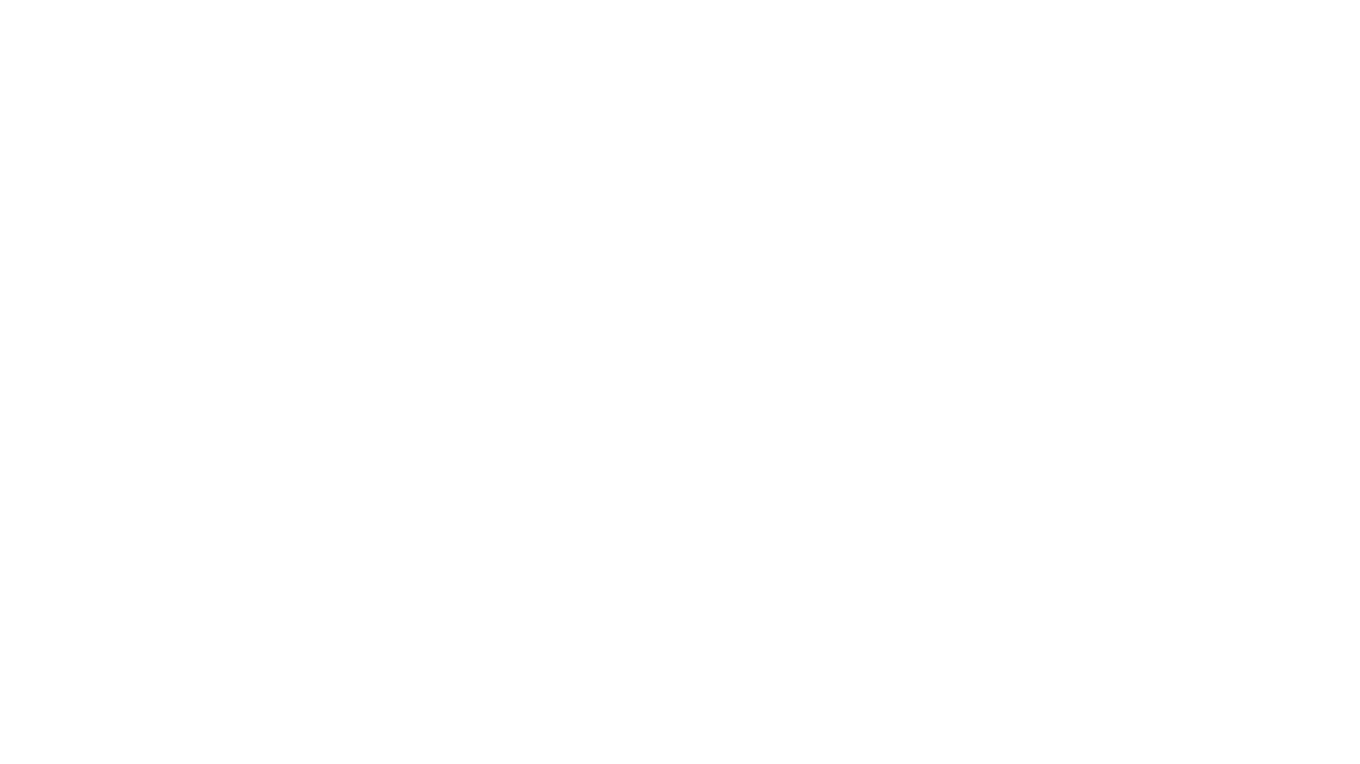
jupyter-ai
Jupyter AI connects generative AI with Jupyter notebooks. It provides a user-friendly and powerful way to explore generative AI models in notebooks and improve your productivity in JupyterLab and the Jupyter Notebook. Specifically, Jupyter AI offers: * An `%%ai` magic that turns the Jupyter notebook into a reproducible generative AI playground. This works anywhere the IPython kernel runs (JupyterLab, Jupyter Notebook, Google Colab, Kaggle, VSCode, etc.). * A native chat UI in JupyterLab that enables you to work with generative AI as a conversational assistant. * Support for a wide range of generative model providers, including AI21, Anthropic, AWS, Cohere, Gemini, Hugging Face, NVIDIA, and OpenAI. * Local model support through GPT4All, enabling use of generative AI models on consumer grade machines with ease and privacy.
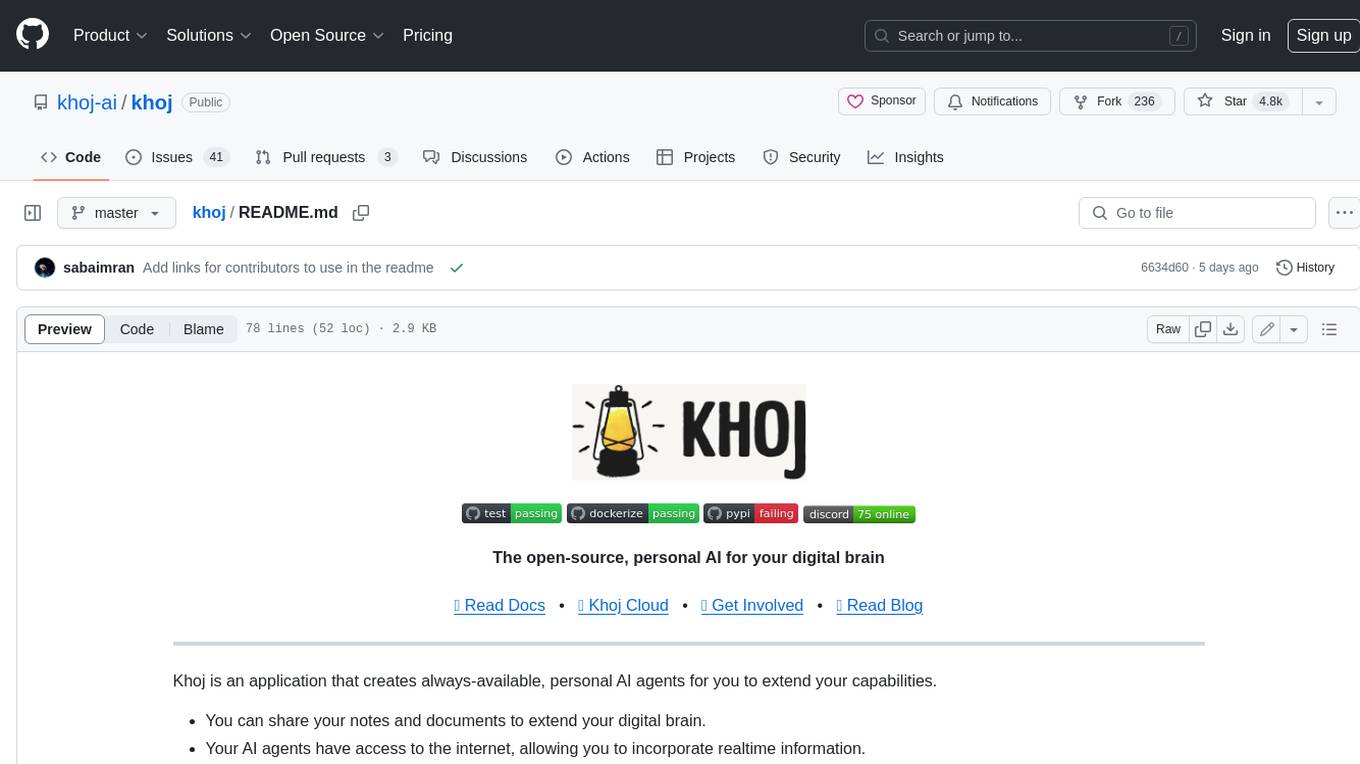
khoj
Khoj is an open-source, personal AI assistant that extends your capabilities by creating always-available AI agents. You can share your notes and documents to extend your digital brain, and your AI agents have access to the internet, allowing you to incorporate real-time information. Khoj is accessible on Desktop, Emacs, Obsidian, Web, and Whatsapp, and you can share PDF, markdown, org-mode, notion files, and GitHub repositories. You'll get fast, accurate semantic search on top of your docs, and your agents can create deeply personal images and understand your speech. Khoj is self-hostable and always will be.
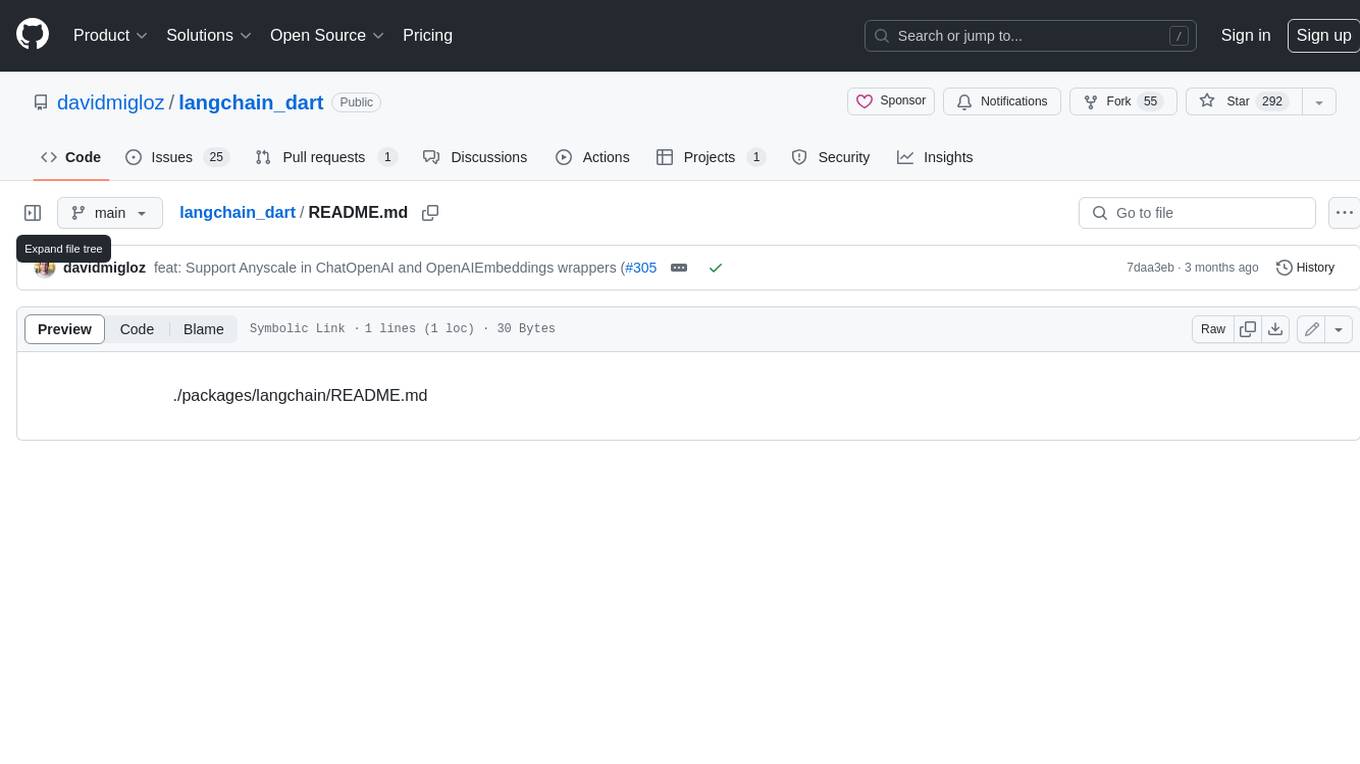
langchain_dart
LangChain.dart is a Dart port of the popular LangChain Python framework created by Harrison Chase. LangChain provides a set of ready-to-use components for working with language models and a standard interface for chaining them together to formulate more advanced use cases (e.g. chatbots, Q&A with RAG, agents, summarization, extraction, etc.). The components can be grouped into a few core modules: * **Model I/O:** LangChain offers a unified API for interacting with various LLM providers (e.g. OpenAI, Google, Mistral, Ollama, etc.), allowing developers to switch between them with ease. Additionally, it provides tools for managing model inputs (prompt templates and example selectors) and parsing the resulting model outputs (output parsers). * **Retrieval:** assists in loading user data (via document loaders), transforming it (with text splitters), extracting its meaning (using embedding models), storing (in vector stores) and retrieving it (through retrievers) so that it can be used to ground the model's responses (i.e. Retrieval-Augmented Generation or RAG). * **Agents:** "bots" that leverage LLMs to make informed decisions about which available tools (such as web search, calculators, database lookup, etc.) to use to accomplish the designated task. The different components can be composed together using the LangChain Expression Language (LCEL).
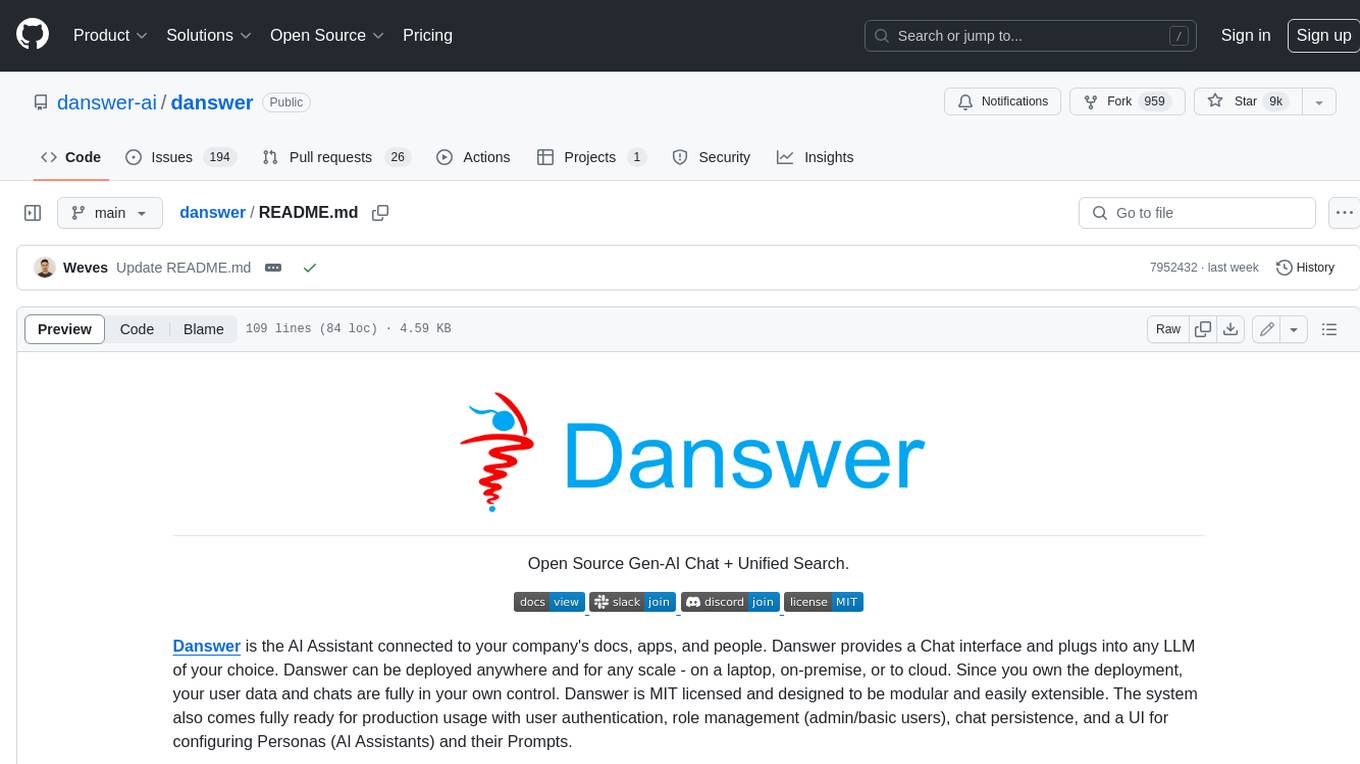
danswer
Danswer is an open-source Gen-AI Chat and Unified Search tool that connects to your company's docs, apps, and people. It provides a Chat interface and plugs into any LLM of your choice. Danswer can be deployed anywhere and for any scale - on a laptop, on-premise, or to cloud. Since you own the deployment, your user data and chats are fully in your own control. Danswer is MIT licensed and designed to be modular and easily extensible. The system also comes fully ready for production usage with user authentication, role management (admin/basic users), chat persistence, and a UI for configuring Personas (AI Assistants) and their Prompts. Danswer also serves as a Unified Search across all common workplace tools such as Slack, Google Drive, Confluence, etc. By combining LLMs and team specific knowledge, Danswer becomes a subject matter expert for the team. Imagine ChatGPT if it had access to your team's unique knowledge! It enables questions such as "A customer wants feature X, is this already supported?" or "Where's the pull request for feature Y?"
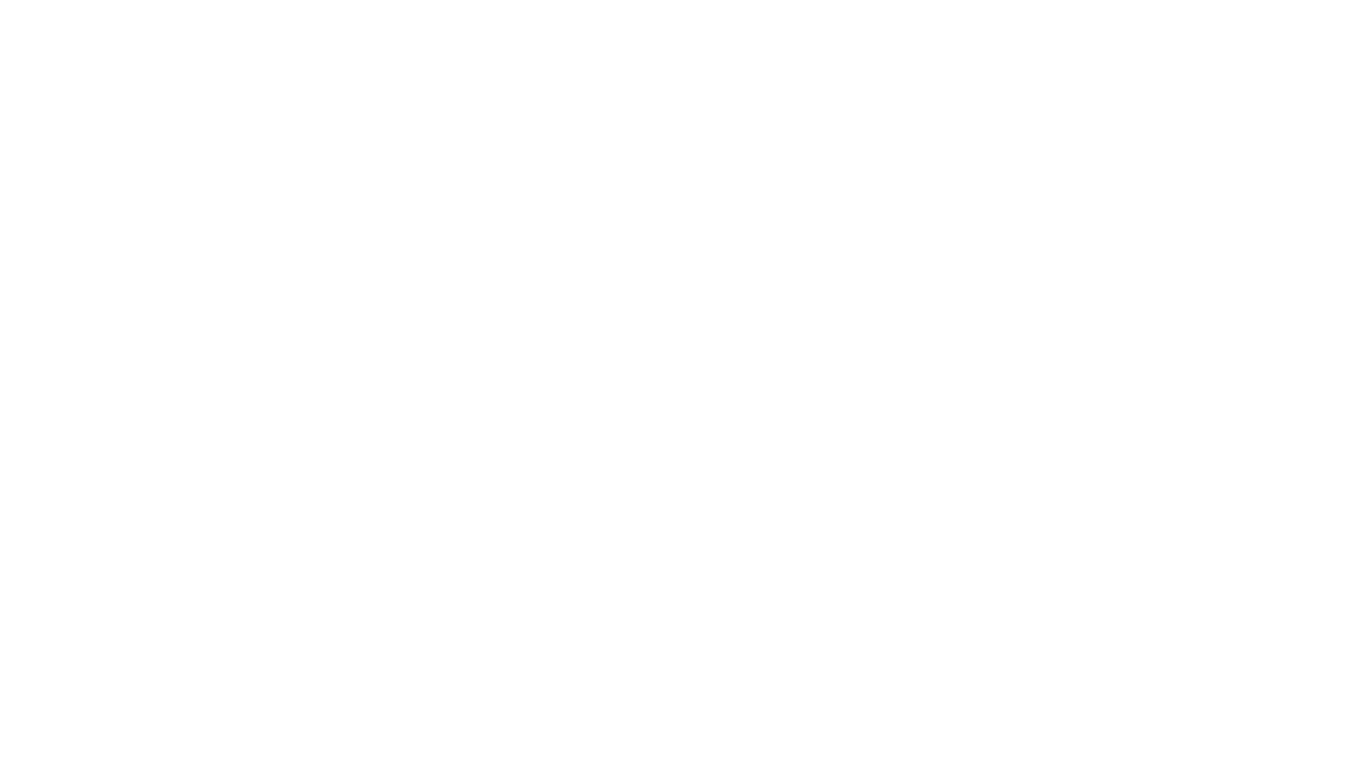
infinity
Infinity is an AI-native database designed for LLM applications, providing incredibly fast full-text and vector search capabilities. It supports a wide range of data types, including vectors, full-text, and structured data, and offers a fused search feature that combines multiple embeddings and full text. Infinity is easy to use, with an intuitive Python API and a single-binary architecture that simplifies deployment. It achieves high performance, with 0.1 milliseconds query latency on million-scale vector datasets and up to 15K QPS.
For similar jobs
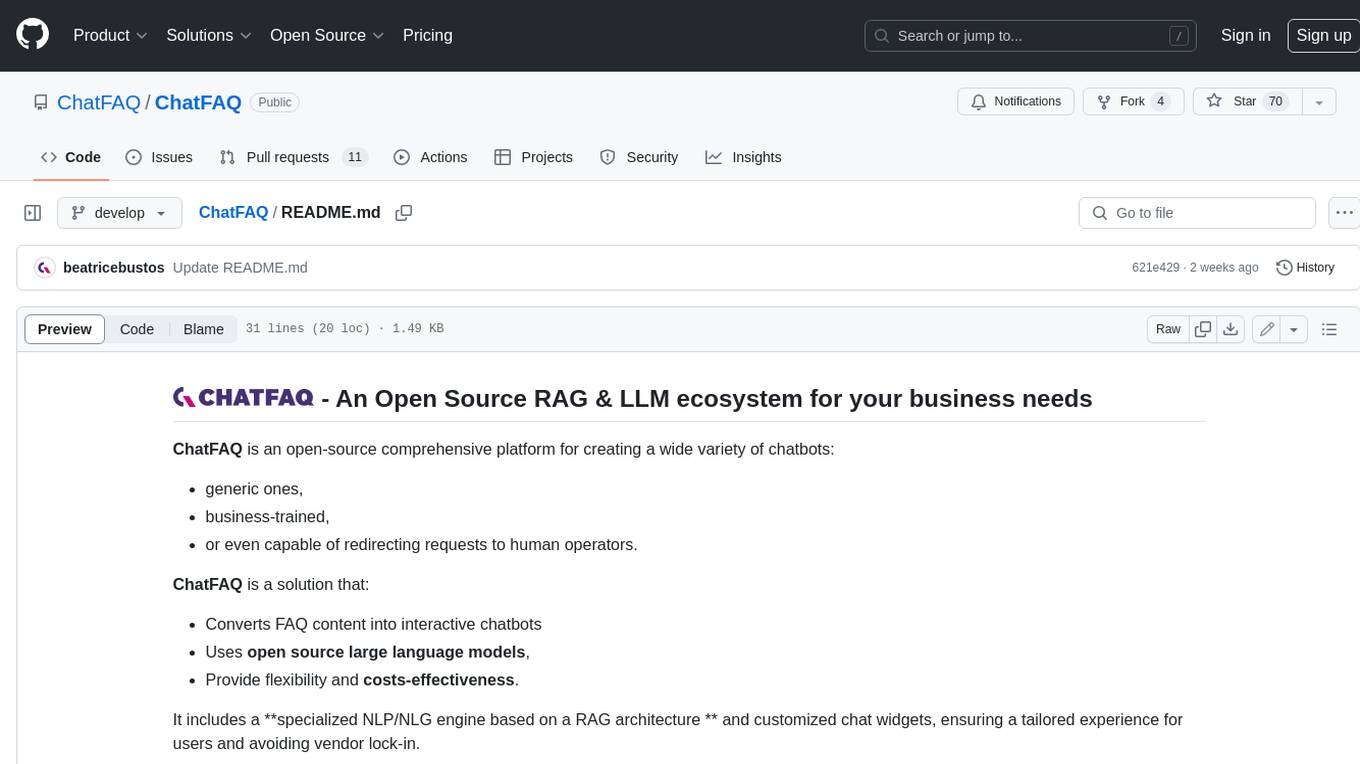
ChatFAQ
ChatFAQ is an open-source comprehensive platform for creating a wide variety of chatbots: generic ones, business-trained, or even capable of redirecting requests to human operators. It includes a specialized NLP/NLG engine based on a RAG architecture and customized chat widgets, ensuring a tailored experience for users and avoiding vendor lock-in.
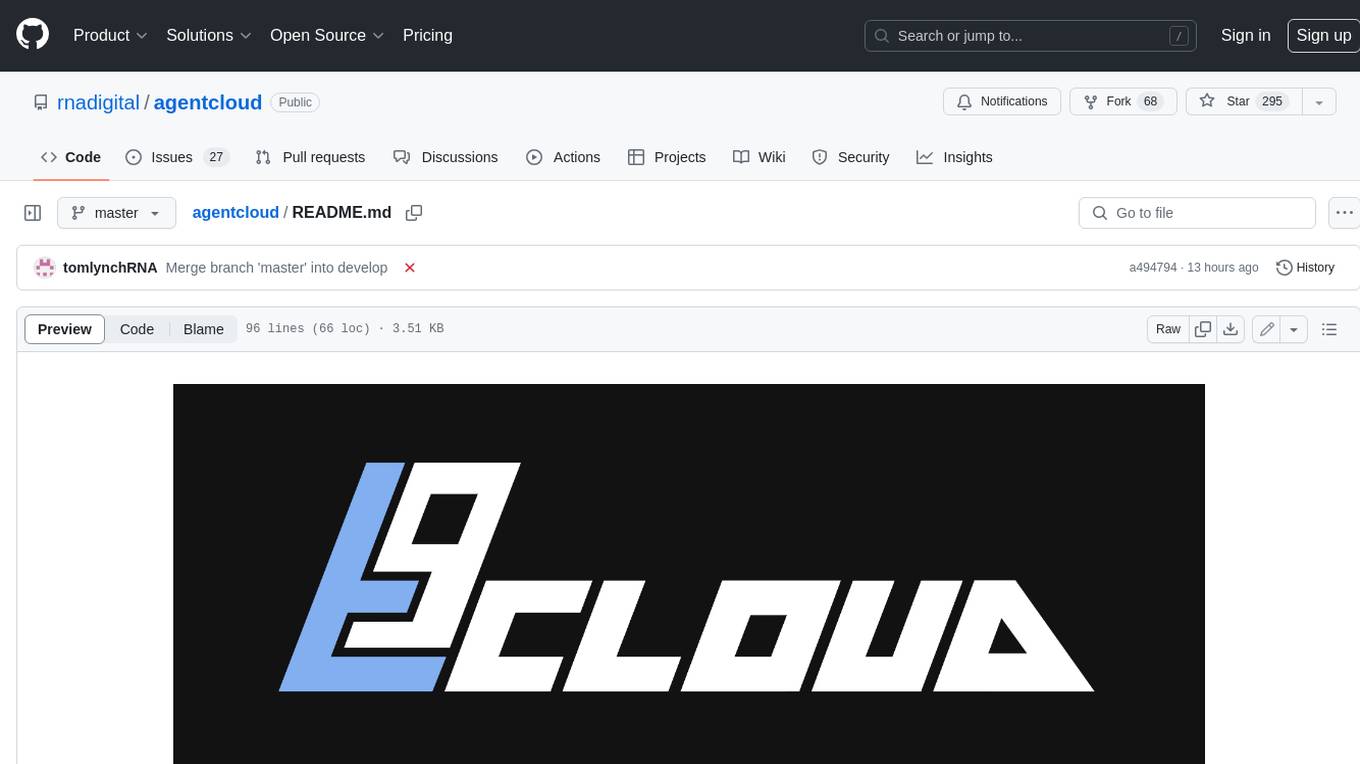
agentcloud
AgentCloud is an open-source platform that enables companies to build and deploy private LLM chat apps, empowering teams to securely interact with their data. It comprises three main components: Agent Backend, Webapp, and Vector Proxy. To run this project locally, clone the repository, install Docker, and start the services. The project is licensed under the GNU Affero General Public License, version 3 only. Contributions and feedback are welcome from the community.
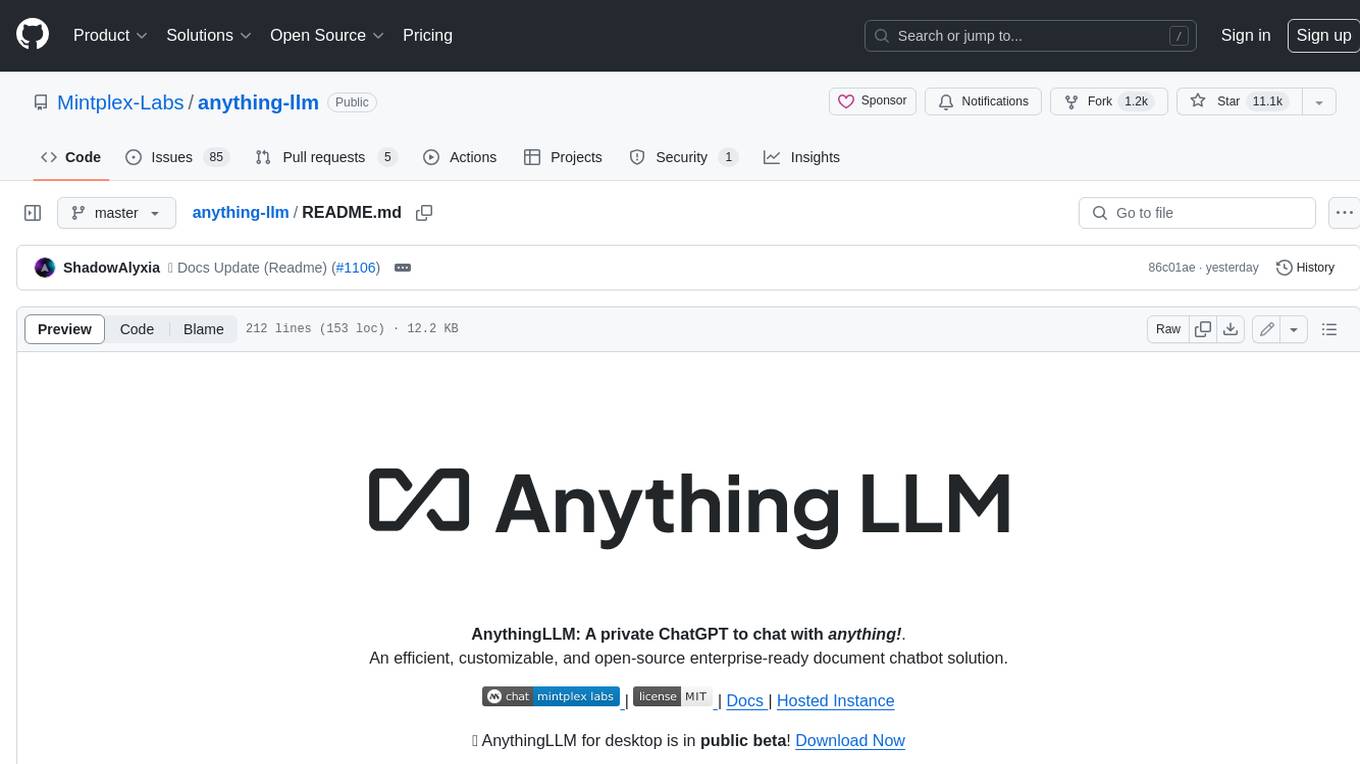
anything-llm
AnythingLLM is a full-stack application that enables you to turn any document, resource, or piece of content into context that any LLM can use as references during chatting. This application allows you to pick and choose which LLM or Vector Database you want to use as well as supporting multi-user management and permissions.
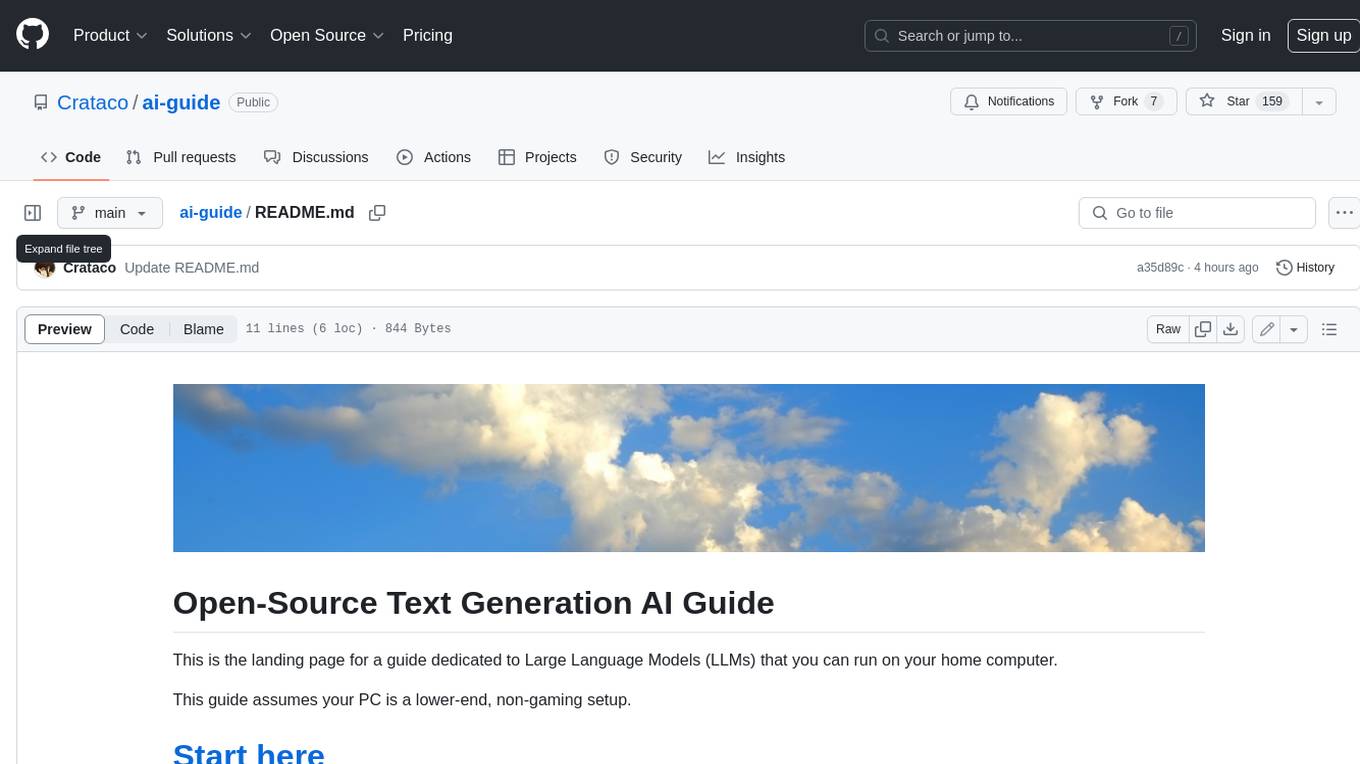
ai-guide
This guide is dedicated to Large Language Models (LLMs) that you can run on your home computer. It assumes your PC is a lower-end, non-gaming setup.
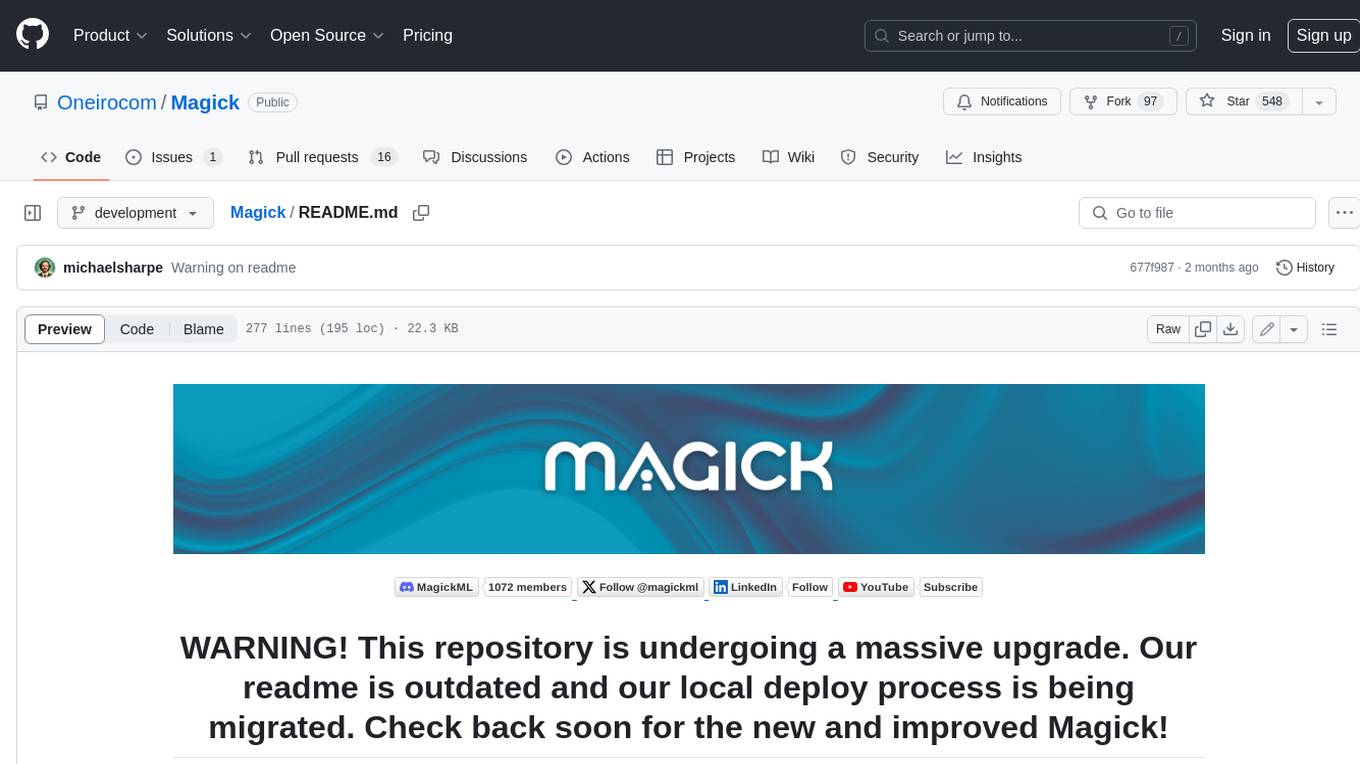
Magick
Magick is a groundbreaking visual AIDE (Artificial Intelligence Development Environment) for no-code data pipelines and multimodal agents. Magick can connect to other services and comes with nodes and templates well-suited for intelligent agents, chatbots, complex reasoning systems and realistic characters.
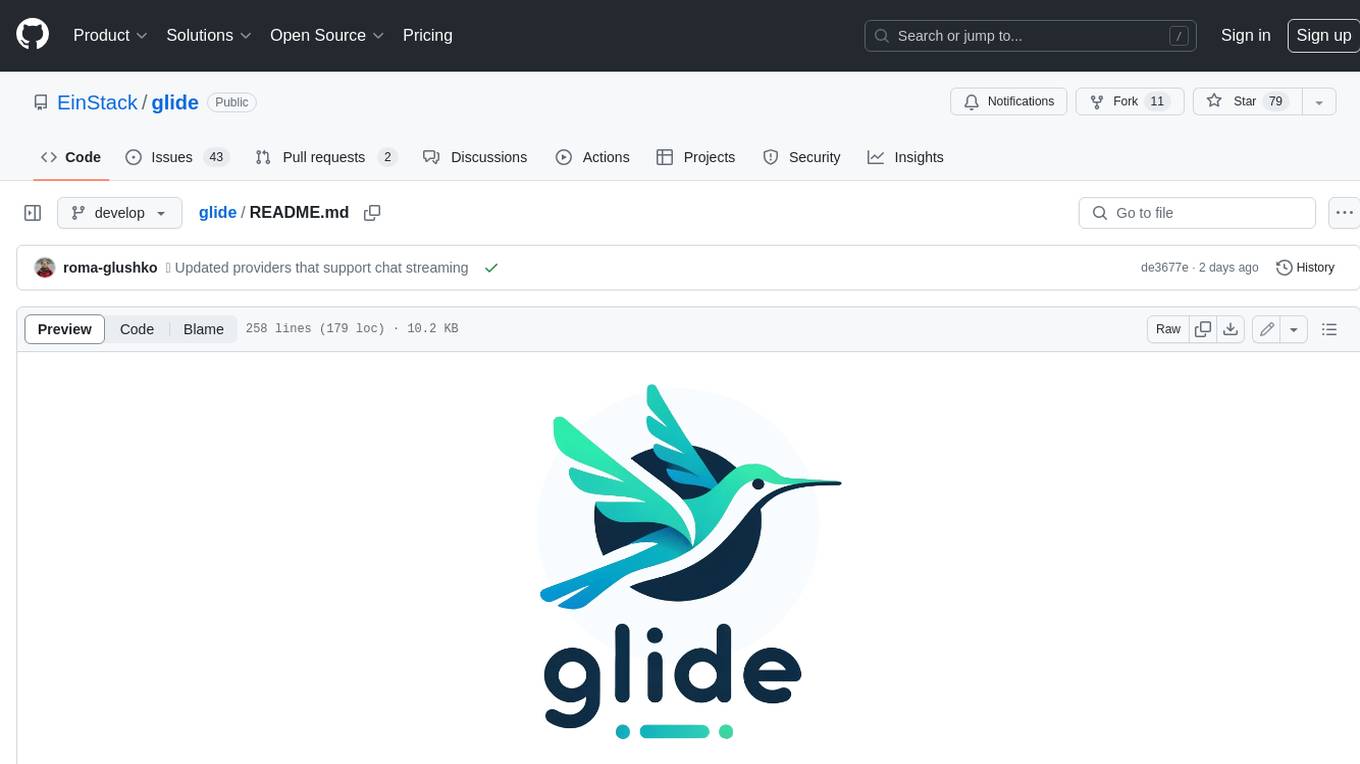
glide
Glide is a cloud-native LLM gateway that provides a unified REST API for accessing various large language models (LLMs) from different providers. It handles LLMOps tasks such as model failover, caching, key management, and more, making it easy to integrate LLMs into applications. Glide supports popular LLM providers like OpenAI, Anthropic, Azure OpenAI, AWS Bedrock (Titan), Cohere, Google Gemini, OctoML, and Ollama. It offers high availability, performance, and observability, and provides SDKs for Python and NodeJS to simplify integration.
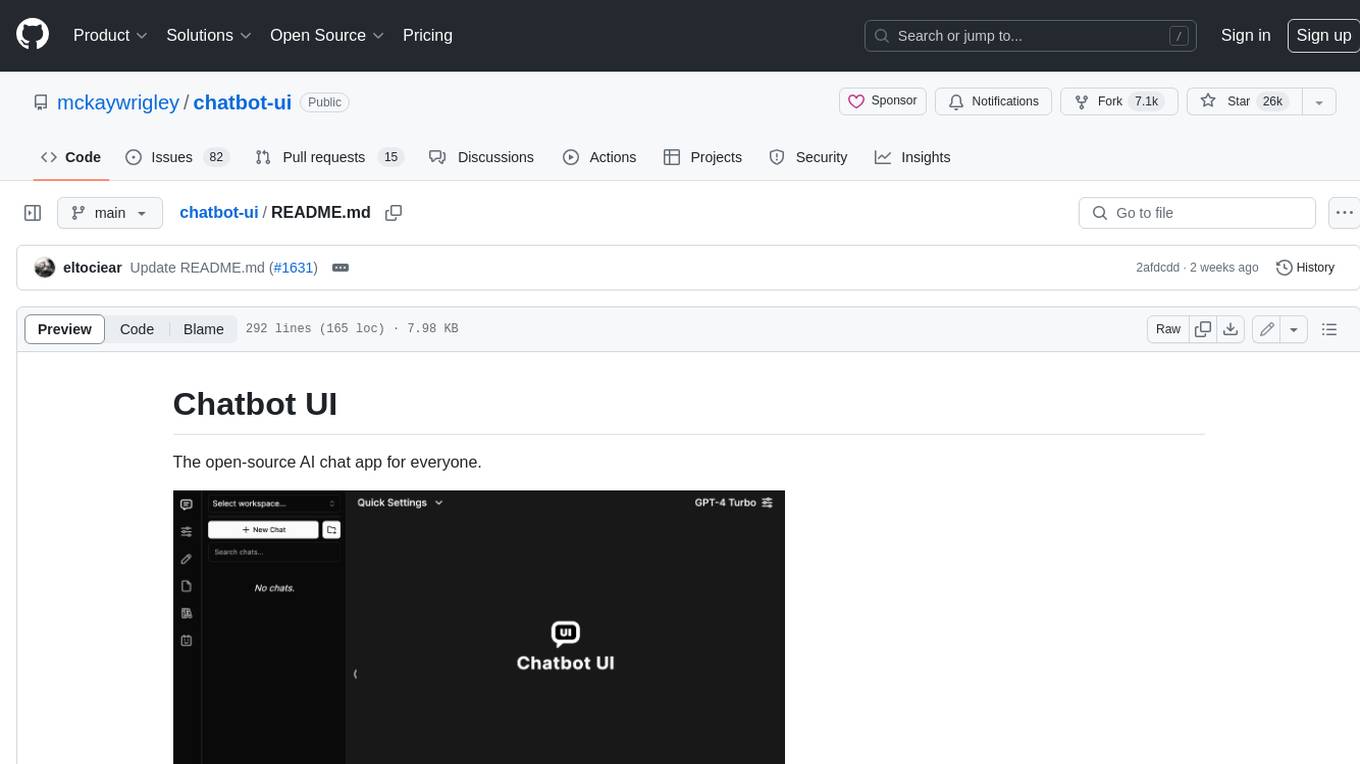
chatbot-ui
Chatbot UI is an open-source AI chat app that allows users to create and deploy their own AI chatbots. It is easy to use and can be customized to fit any need. Chatbot UI is perfect for businesses, developers, and anyone who wants to create a chatbot.
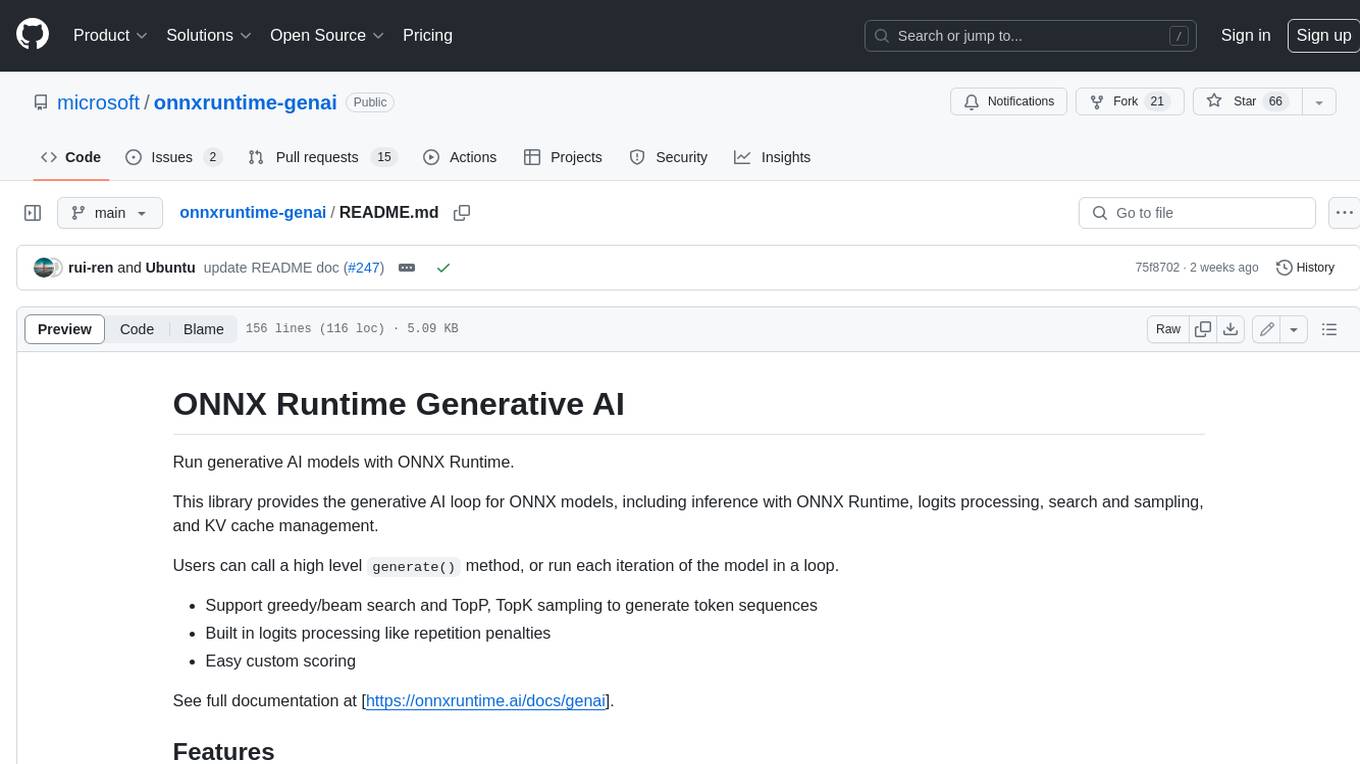
onnxruntime-genai
ONNX Runtime Generative AI is a library that provides the generative AI loop for ONNX models, including inference with ONNX Runtime, logits processing, search and sampling, and KV cache management. Users can call a high level `generate()` method, or run each iteration of the model in a loop. It supports greedy/beam search and TopP, TopK sampling to generate token sequences, has built in logits processing like repetition penalties, and allows for easy custom scoring.