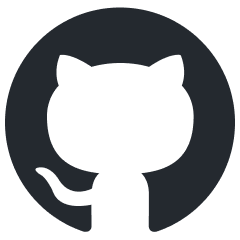
CALF
An official implementation of "CALF: Aligning LLMs for Time Series Forecasting via Cross-modal Fine-Tuning"
Stars: 60
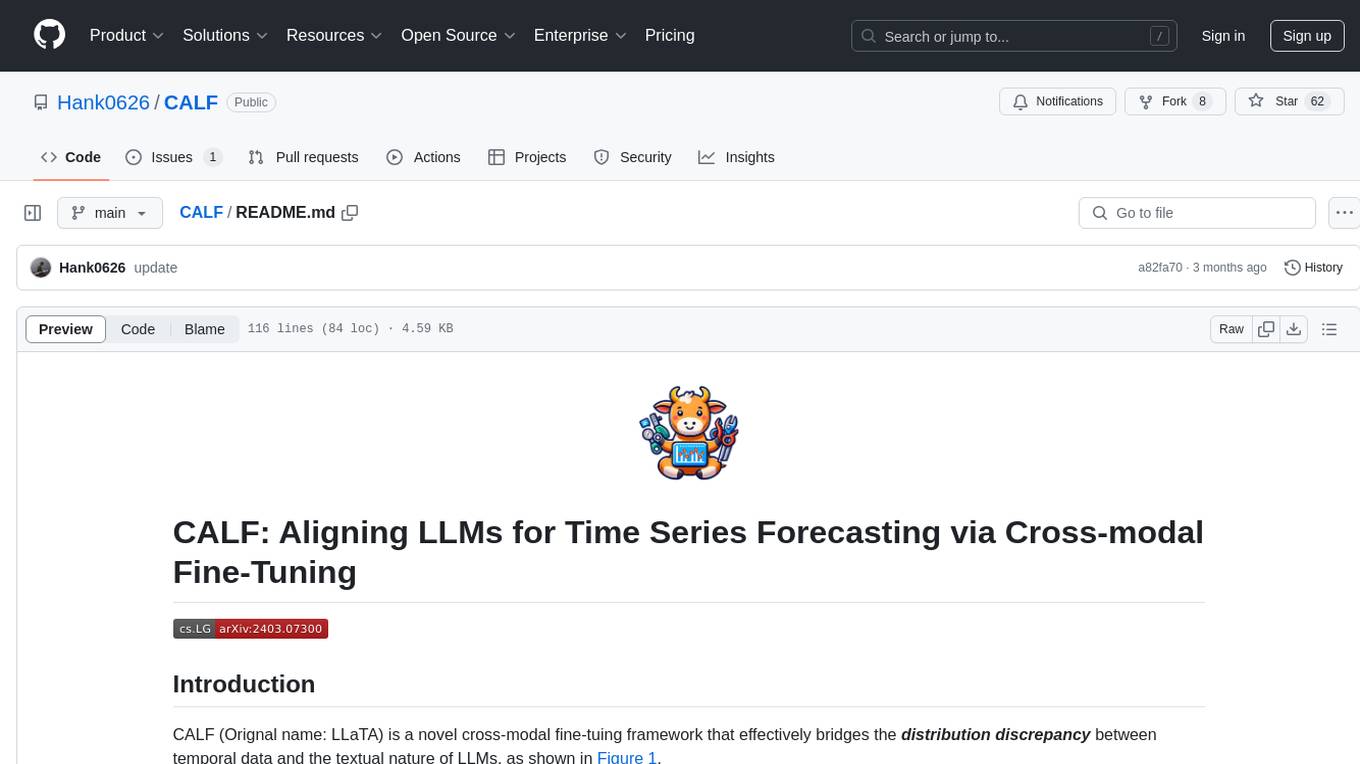
CALF (LLaTA) is a cross-modal fine-tuning framework that bridges the distribution discrepancy between temporal data and the textual nature of LLMs. It introduces three cross-modal fine-tuning techniques: Cross-Modal Match Module, Feature Regularization Loss, and Output Consistency Loss. The framework aligns time series and textual inputs, ensures effective weight updates, and maintains consistent semantic context for time series data. CALF provides scripts for long-term and short-term forecasting, requires Python 3.9, and utilizes word token embeddings for model training.
README:
CALF (Orignal name: LLaTA) is a novel cross-modal fine-tuing framework that effectively bridges the distribution discrepancy between temporal data and the textual nature of LLMs, as shown in Figure 1.
Figure 1: The t-SNE visualization of pre-trained word token embeddings of LLM with temporal tokens from GPT4TS (Left) and our method (Right). Our method shows more cohesive integration, indicating effective modality alignment.
To bridge the modality gap between textual and temporal data, we introduce three meticulously designed cross-modal fine-tuning techniques (see Figure 2):
- Cross-Modal Match Module integrates time series and textual inputs through principal word embedding extraction and a cross-attention mechanism, ensuring efficient alignment of the marginal input distribution between time series and text.
- Feature Regularization Loss aligns the outputs of each intermediate layer, ensuring that gradients at every layer are more effectively guided for better weight updates.
- Output Consistency Loss ensures that the output representations of textual and temporal series modalities correspond effectively, resolving discrepancies in the representation space and maintaining consistent semantic context for time series data.
Figure 2: Conceptual illustration of cross-modal fine-tuning technique.
Before proceeding, ensure Python 3.9 is installed. Install the required dependencies with the following command:
pip install -r requirements.txt
Acquire datasets from Autoformer. Organize them in the ./datasets
directory as shown below:
datasets
├── electricity
│ └── electricity.csv
├── ETT-small
│ ├── ETTh1.csv
│ ├── ETTh2.csv
│ ├── ETTm1.csv
│ └── ETTm2.csv
├── traffic
│ └── traffic.csv
└── weather
└── weather.csv
For short-term forecasting, download the M4 datasets from Time-Series-Library. Place the m4
folder within ./datasets
.
Execute the command below to extract principal components from the word token embeddings:
python pca.py
These embeddings will be saved in ./wte_pca_500.pt
.
Training scripts are located in the ./scripts
folder. For instance, to train the CALF model on the ETTh2 dataset for long-term forecasting, execute:
sh scripts/long_term_forecasting/ETTh2.sh
For short-term forecasting, use:
sh scripts/short_term_forecasting/m4.sh
Post-Training:
- Trained models will be saved in
./checkpoints
. - Numerical results are available in
.npy
format under./results
. - Detailed summaries of performance metrics can be found in
./results_{task_name}.txt
.
If this repository contributes to your research, please consider citing our work:
@article{liu2024taming,
title={CALF: Aligning LLMs for Time Series Forecasting via Cross-modal Fine-Tuning},
author={Liu, Peiyuan and Guo, Hang and Dai, Tao and Li, Naiqi and Bao, Jigang and Ren, Xudong and Jiang, Yong and Xia, Shu-Tao},
journal={arXiv preprint arXiv:2403.07300},
year={2024},
arxiv={2403.07300}
}
Our gratitude extends to the authors of the following repositories for their foundational model implementations:
For inquiries or further assistance, contact us at [email protected] or open an issue on this repository.
For Tasks:
Click tags to check more tools for each tasksFor Jobs:
Alternative AI tools for CALF
Similar Open Source Tools
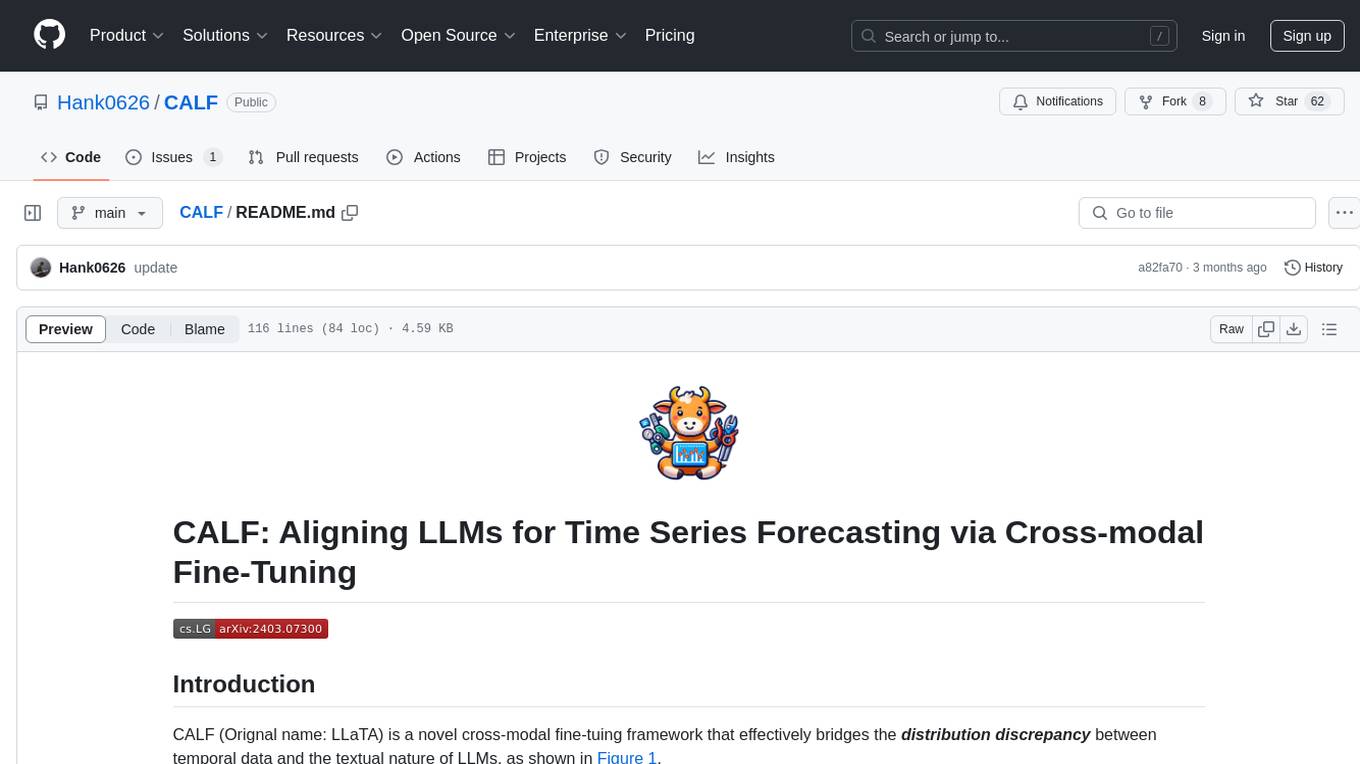
CALF
CALF (LLaTA) is a cross-modal fine-tuning framework that bridges the distribution discrepancy between temporal data and the textual nature of LLMs. It introduces three cross-modal fine-tuning techniques: Cross-Modal Match Module, Feature Regularization Loss, and Output Consistency Loss. The framework aligns time series and textual inputs, ensures effective weight updates, and maintains consistent semantic context for time series data. CALF provides scripts for long-term and short-term forecasting, requires Python 3.9, and utilizes word token embeddings for model training.
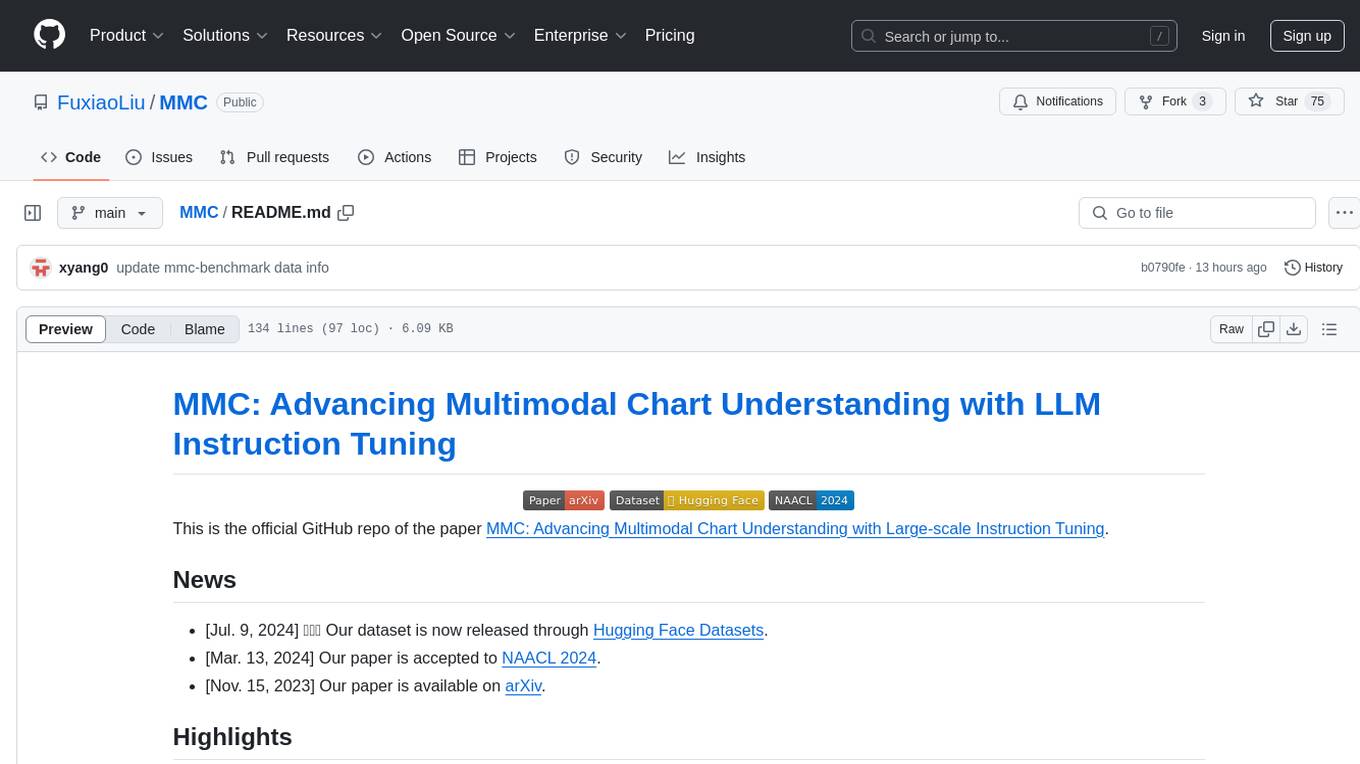
MMC
This repository, MMC, focuses on advancing multimodal chart understanding through large-scale instruction tuning. It introduces a dataset supporting various tasks and chart types, a benchmark for evaluating reasoning capabilities over charts, and an assistant achieving state-of-the-art performance on chart QA benchmarks. The repository provides data for chart-text alignment, benchmarking, and instruction tuning, along with existing datasets used in experiments. Additionally, it offers a Gradio demo for the MMCA model.
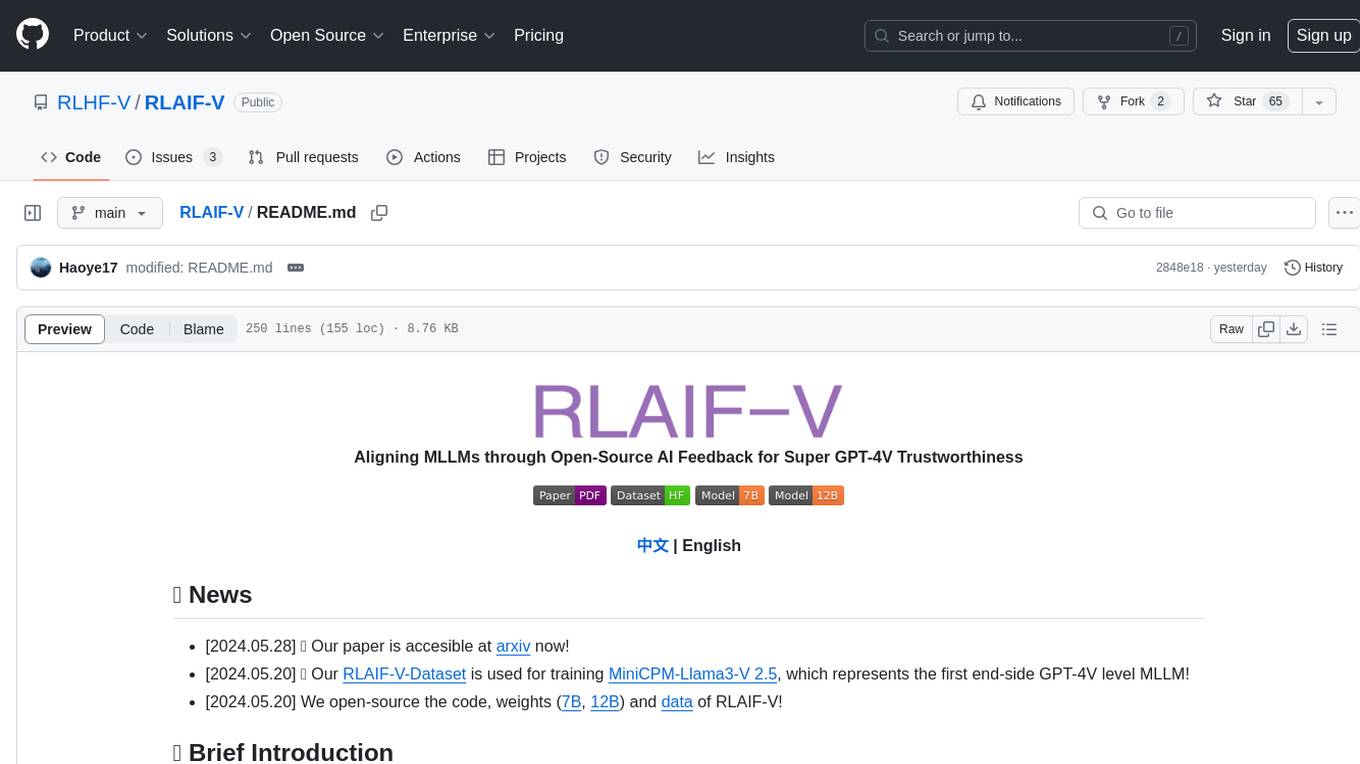
RLAIF-V
RLAIF-V is a novel framework that aligns MLLMs in a fully open-source paradigm for super GPT-4V trustworthiness. It maximally exploits open-source feedback from high-quality feedback data and online feedback learning algorithm. Notable features include achieving super GPT-4V trustworthiness in both generative and discriminative tasks, using high-quality generalizable feedback data to reduce hallucination of different MLLMs, and exhibiting better learning efficiency and higher performance through iterative alignment.
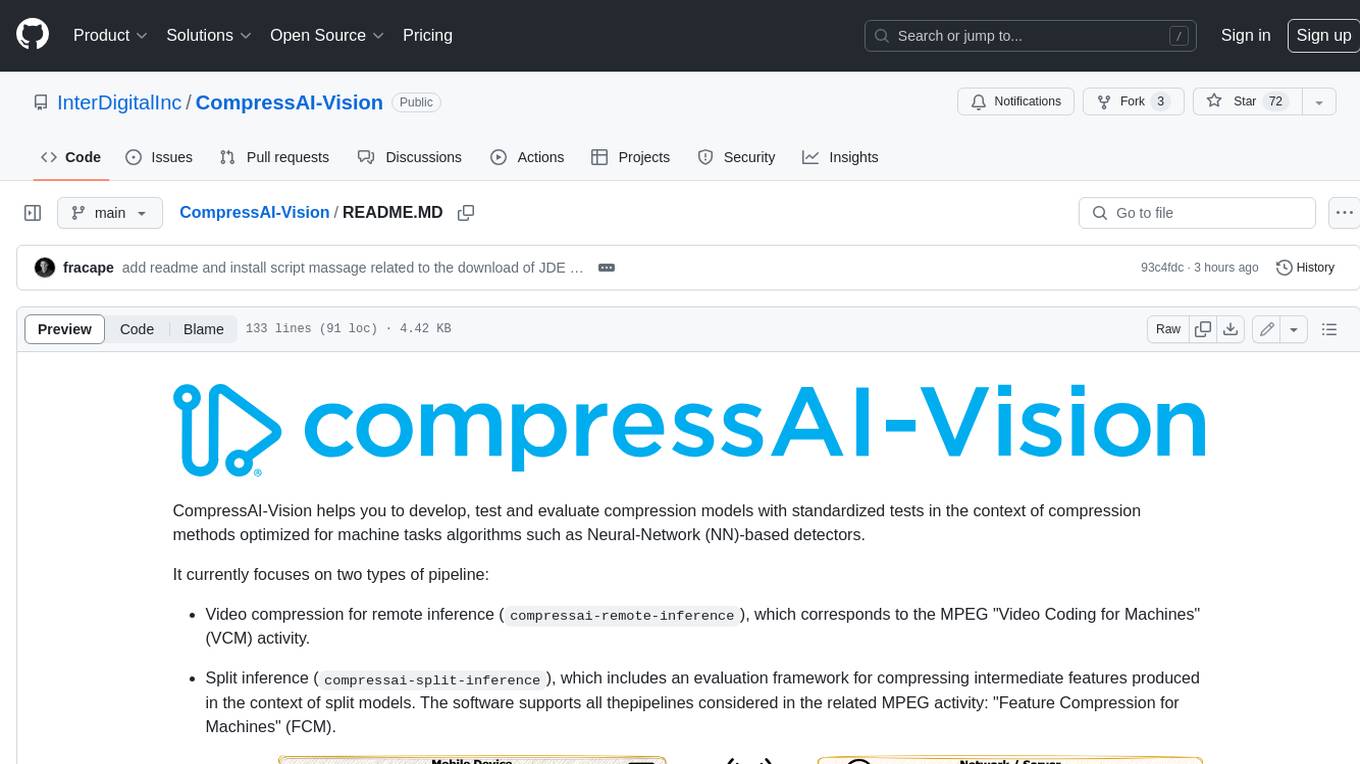
CompressAI-Vision
CompressAI-Vision is a tool that helps you develop, test, and evaluate compression models with standardized tests in the context of compression methods optimized for machine tasks algorithms such as Neural-Network (NN)-based detectors. It currently focuses on two types of pipeline: Video compression for remote inference (`compressai-remote-inference`), which corresponds to the MPEG "Video Coding for Machines" (VCM) activity. Split inference (`compressai-split-inference`), which includes an evaluation framework for compressing intermediate features produced in the context of split models. The software supports all the pipelines considered in the related MPEG activity: "Feature Compression for Machines" (FCM).
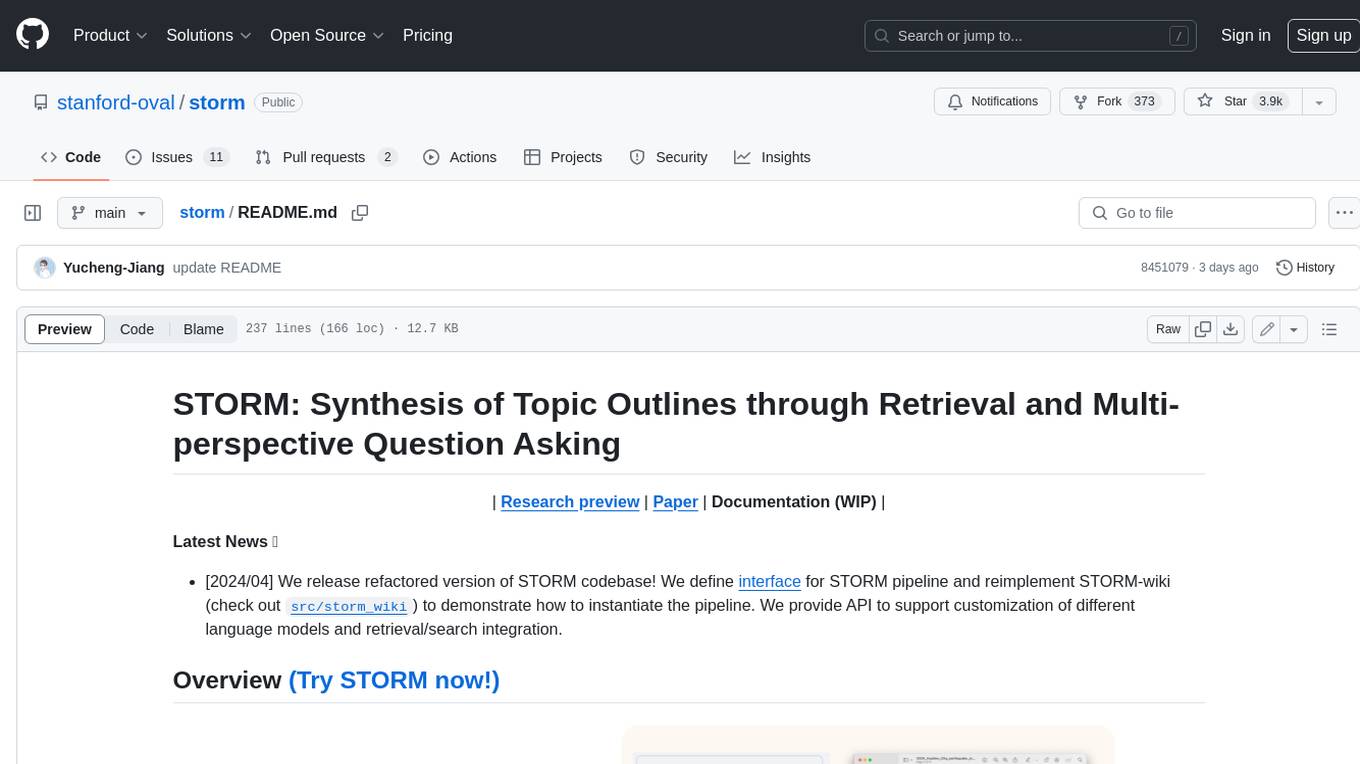
storm
STORM is a LLM system that writes Wikipedia-like articles from scratch based on Internet search. While the system cannot produce publication-ready articles that often require a significant number of edits, experienced Wikipedia editors have found it helpful in their pre-writing stage. **Try out our [live research preview](https://storm.genie.stanford.edu/) to see how STORM can help your knowledge exploration journey and please provide feedback to help us improve the system 🙏!**
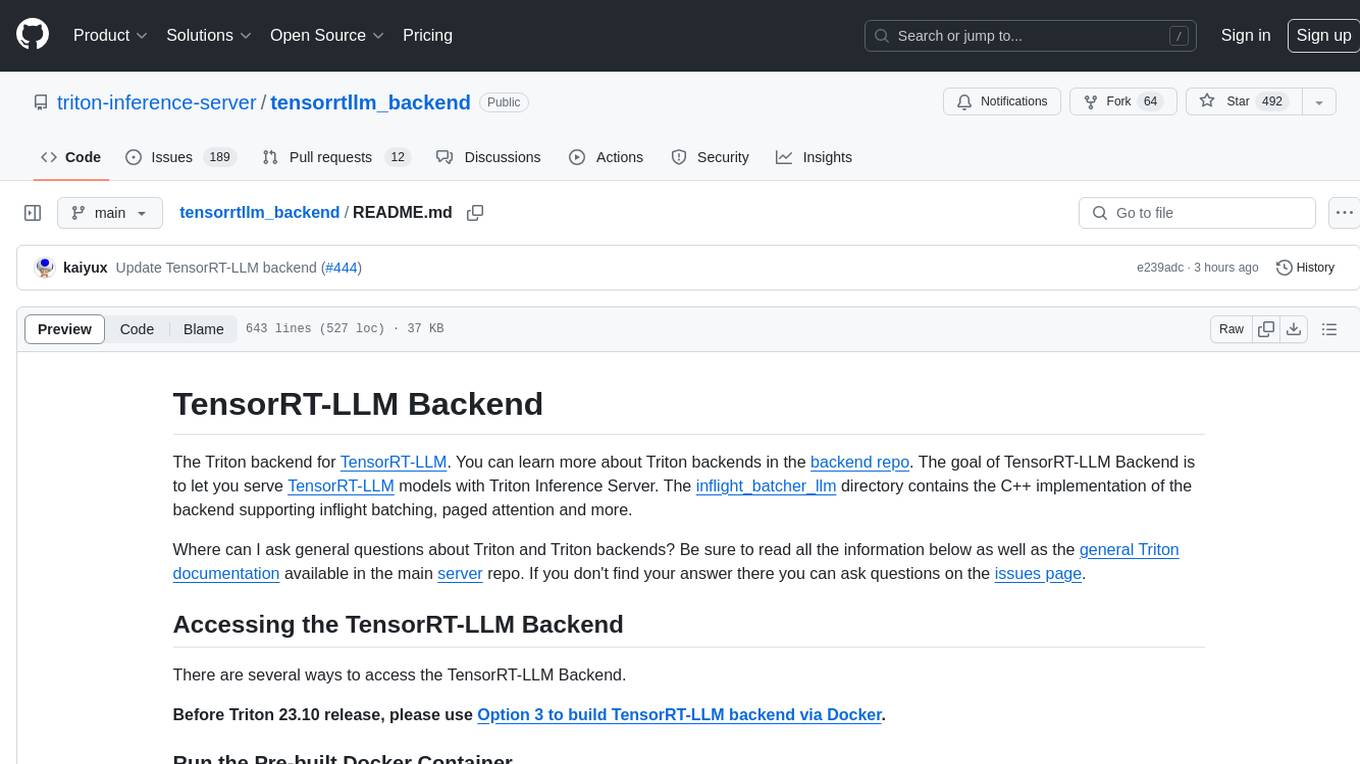
tensorrtllm_backend
The TensorRT-LLM Backend is a Triton backend designed to serve TensorRT-LLM models with Triton Inference Server. It supports features like inflight batching, paged attention, and more. Users can access the backend through pre-built Docker containers or build it using scripts provided in the repository. The backend can be used to create models for tasks like tokenizing, inferencing, de-tokenizing, ensemble modeling, and more. Users can interact with the backend using provided client scripts and query the server for metrics related to request handling, memory usage, KV cache blocks, and more. Testing for the backend can be done following the instructions in the 'ci/README.md' file.
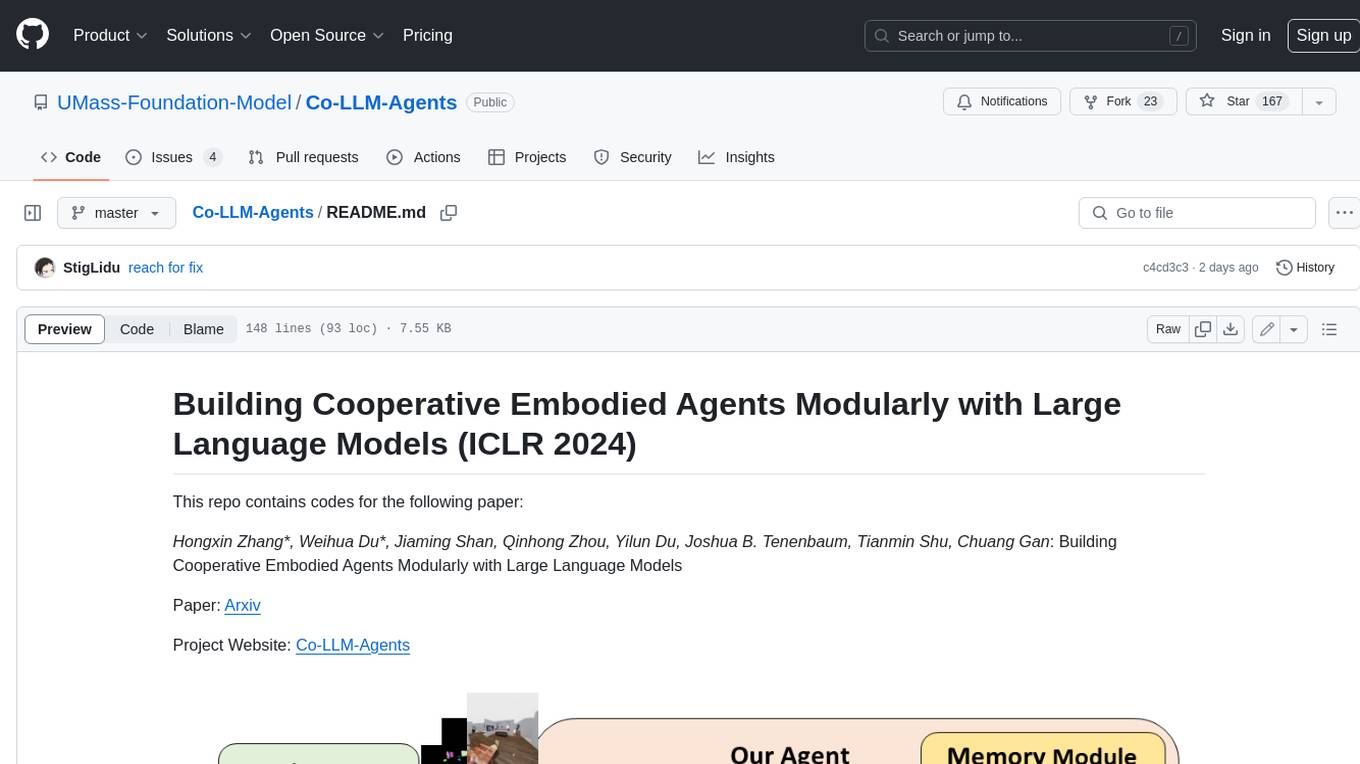
Co-LLM-Agents
This repository contains code for building cooperative embodied agents modularly with large language models. The agents are trained to perform tasks in two different environments: ThreeDWorld Multi-Agent Transport (TDW-MAT) and Communicative Watch-And-Help (C-WAH). TDW-MAT is a multi-agent environment where agents must transport objects to a goal position using containers. C-WAH is an extension of the Watch-And-Help challenge, which enables agents to send messages to each other. The code in this repository can be used to train agents to perform tasks in both of these environments.
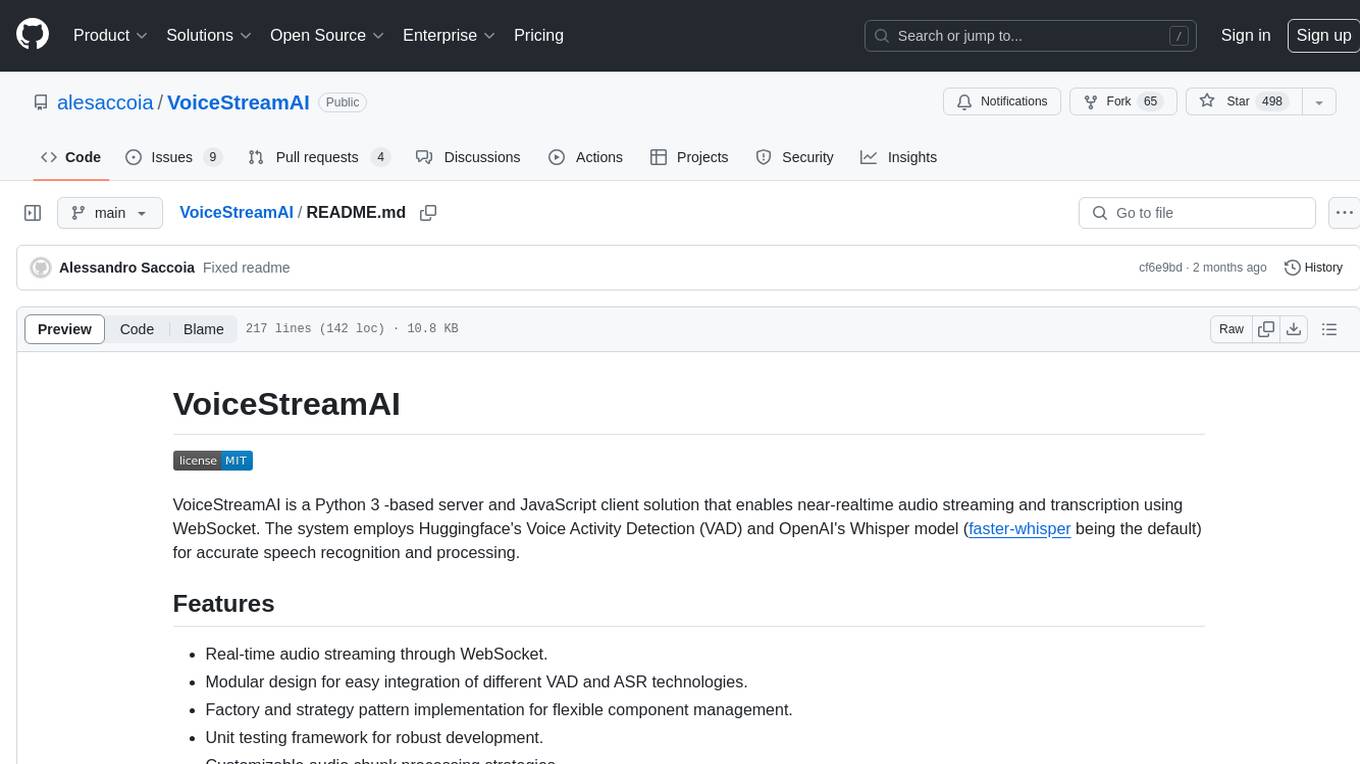
VoiceStreamAI
VoiceStreamAI is a Python 3-based server and JavaScript client solution for near-realtime audio streaming and transcription using WebSocket. It employs Huggingface's Voice Activity Detection (VAD) and OpenAI's Whisper model for accurate speech recognition. The system features real-time audio streaming, modular design for easy integration of VAD and ASR technologies, customizable audio chunk processing strategies, support for multilingual transcription, and secure sockets support. It uses a factory and strategy pattern implementation for flexible component management and provides a unit testing framework for robust development.
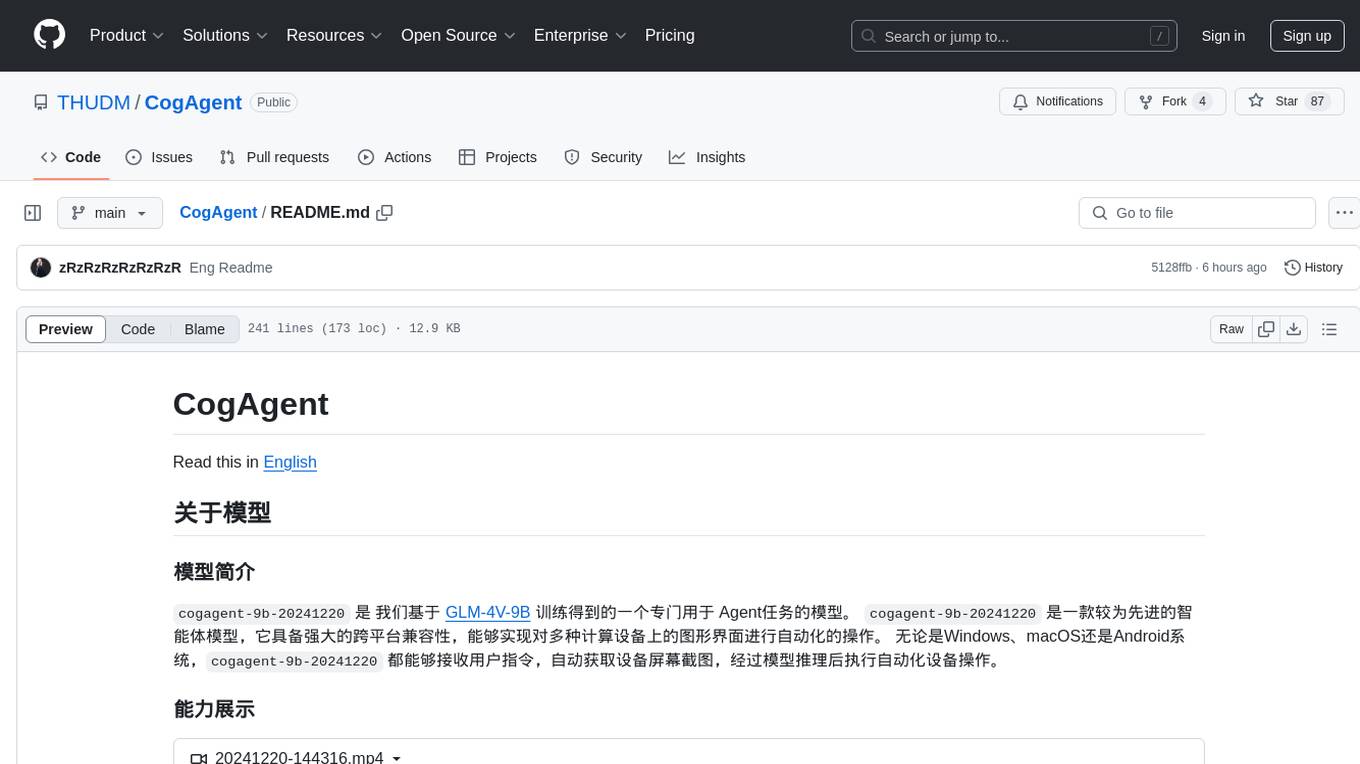
CogAgent
CogAgent is an advanced intelligent agent model designed for automating operations on graphical interfaces across various computing devices. It supports platforms like Windows, macOS, and Android, enabling users to issue commands, capture device screenshots, and perform automated operations. The model requires a minimum of 29GB of GPU memory for inference at BF16 precision and offers capabilities for executing tasks like sending Christmas greetings and sending emails. Users can interact with the model by providing task descriptions, platform specifications, and desired output formats.
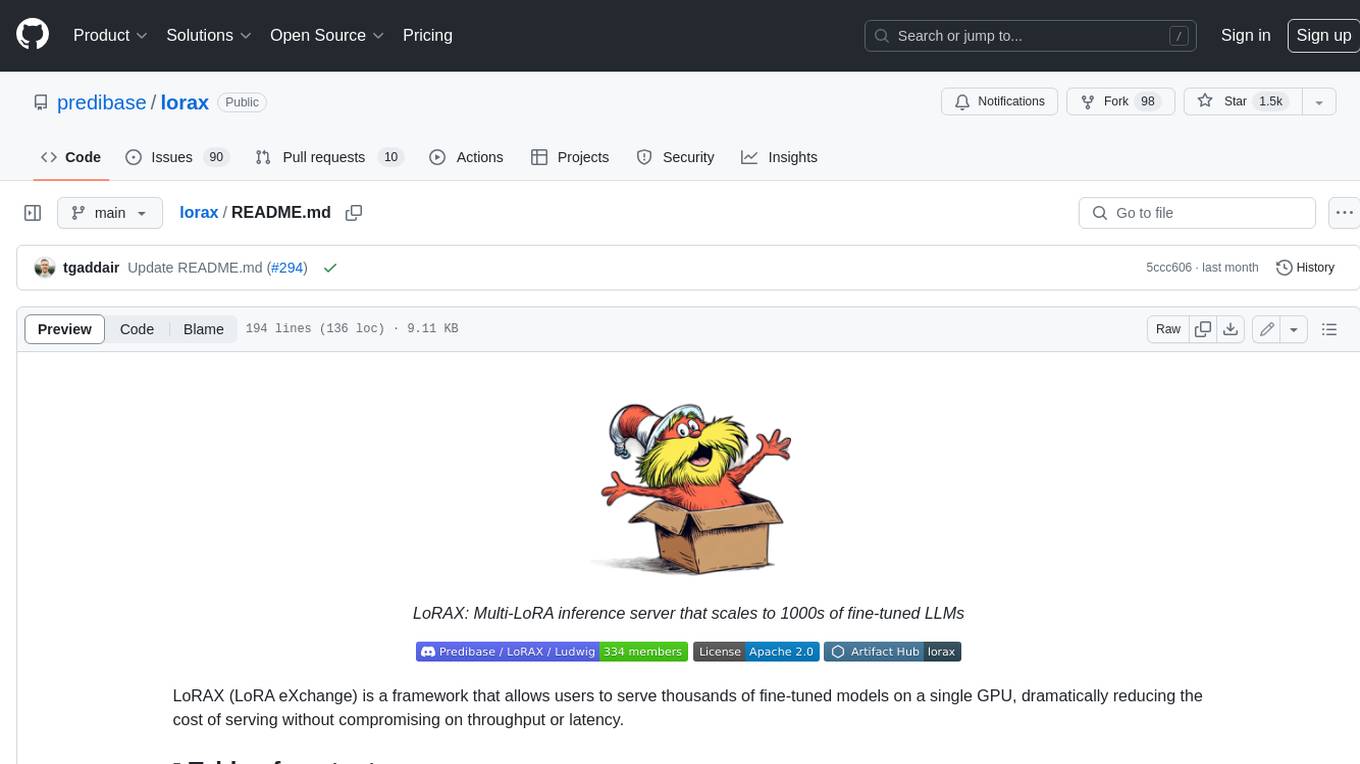
lorax
LoRAX is a framework that allows users to serve thousands of fine-tuned models on a single GPU, dramatically reducing the cost of serving without compromising on throughput or latency. It features dynamic adapter loading, heterogeneous continuous batching, adapter exchange scheduling, optimized inference, and is ready for production with prebuilt Docker images, Helm charts for Kubernetes, Prometheus metrics, and distributed tracing with Open Telemetry. LoRAX supports a number of Large Language Models as the base model including Llama, Mistral, and Qwen, and any of the linear layers in the model can be adapted via LoRA and loaded in LoRAX.
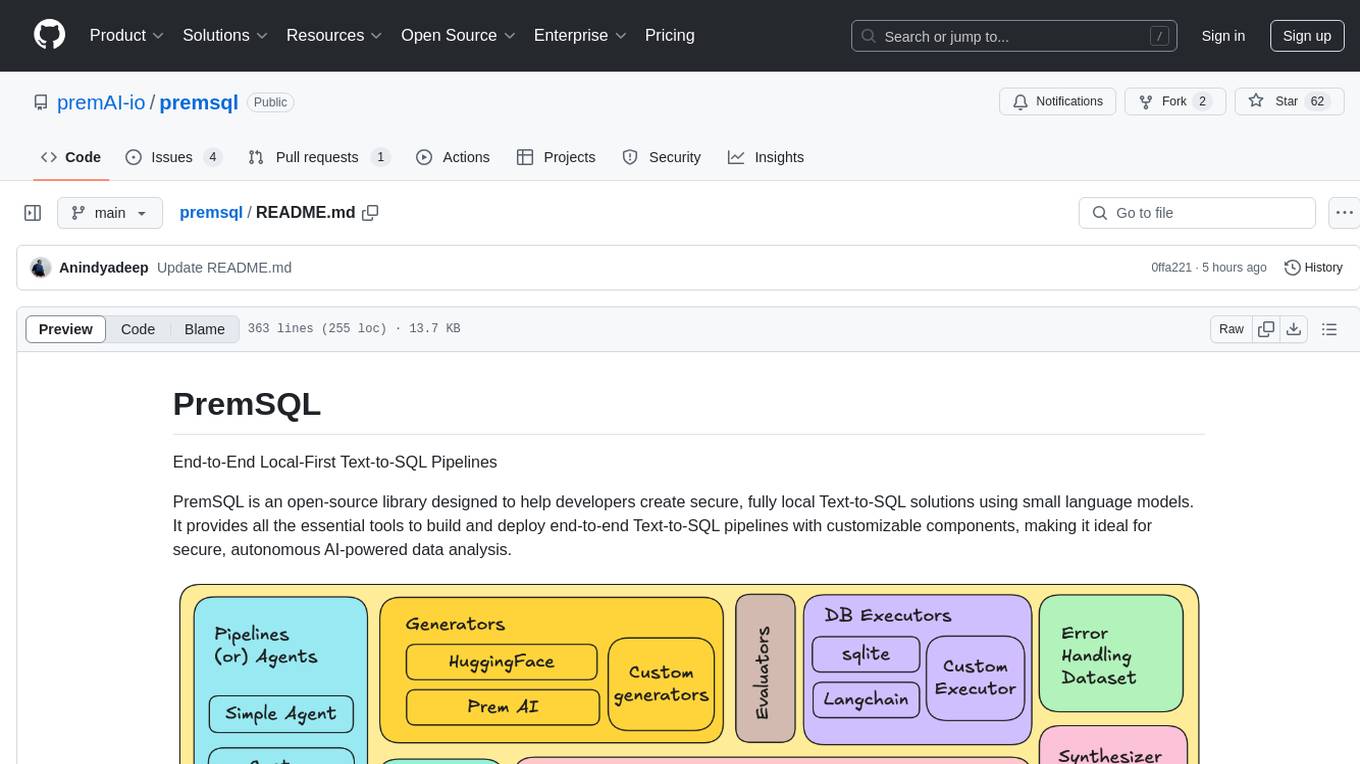
premsql
PremSQL is an open-source library designed to help developers create secure, fully local Text-to-SQL solutions using small language models. It provides essential tools for building and deploying end-to-end Text-to-SQL pipelines with customizable components, ideal for secure, autonomous AI-powered data analysis. The library offers features like Local-First approach, Customizable Datasets, Robust Executors and Evaluators, Advanced Generators, Error Handling and Self-Correction, Fine-Tuning Support, and End-to-End Pipelines. Users can fine-tune models, generate SQL queries from natural language inputs, handle errors, and evaluate model performance against predefined metrics. PremSQL is extendible for customization and private data usage.
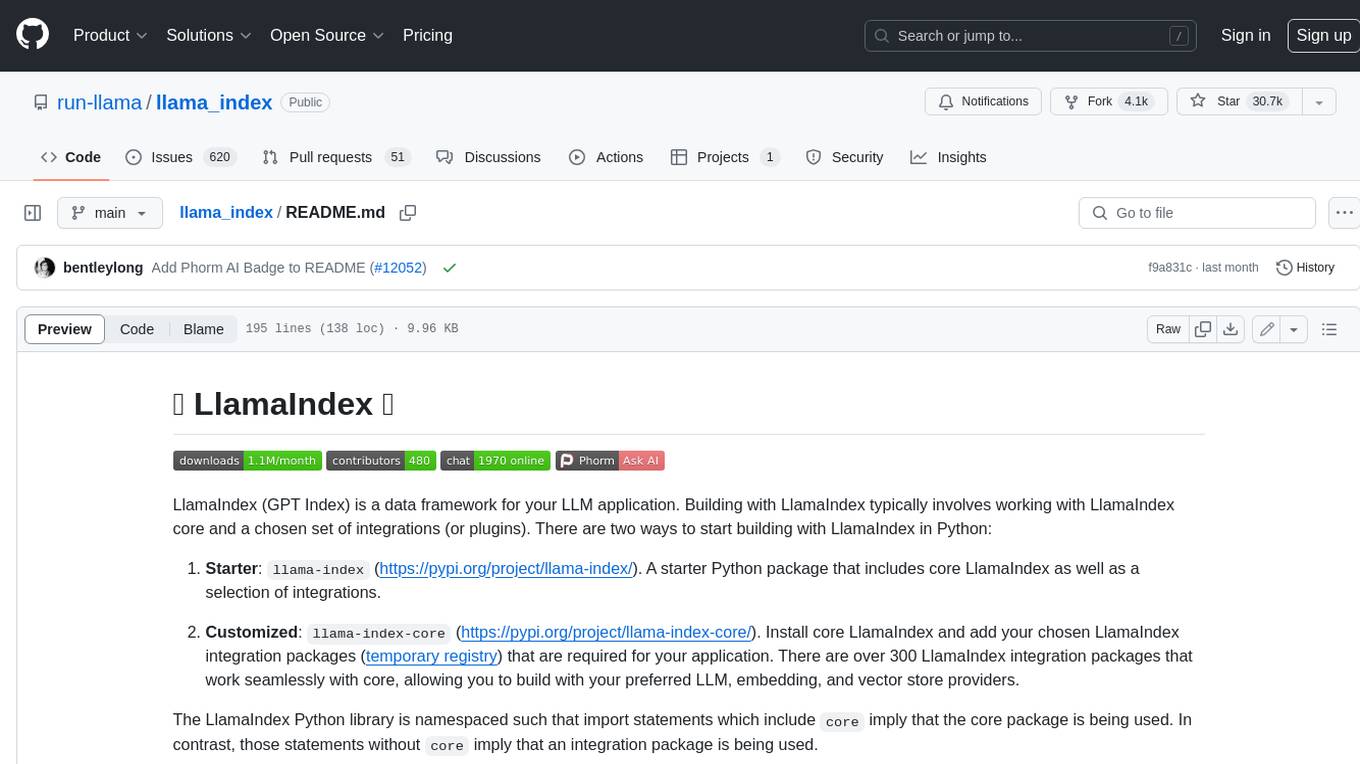
llama_index
LlamaIndex is a data framework for building LLM applications. It provides tools for ingesting, structuring, and querying data, as well as integrating with LLMs and other tools. LlamaIndex is designed to be easy to use for both beginner and advanced users, and it provides a comprehensive set of features for building LLM applications.
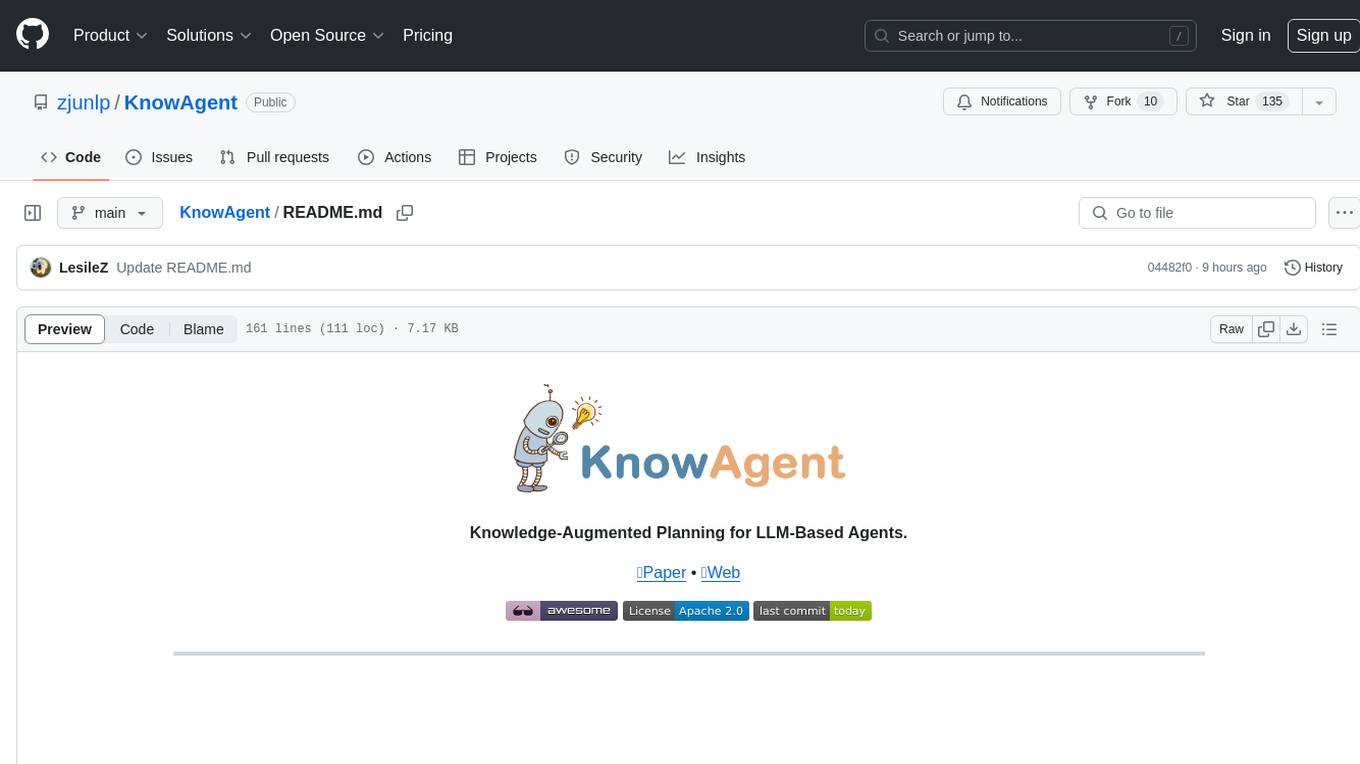
KnowAgent
KnowAgent is a tool designed for Knowledge-Augmented Planning for LLM-Based Agents. It involves creating an action knowledge base, converting action knowledge into text for model understanding, and a knowledgeable self-learning phase to continually improve the model's planning abilities. The tool aims to enhance agents' potential for application in complex situations by leveraging external reservoirs of information and iterative processes.
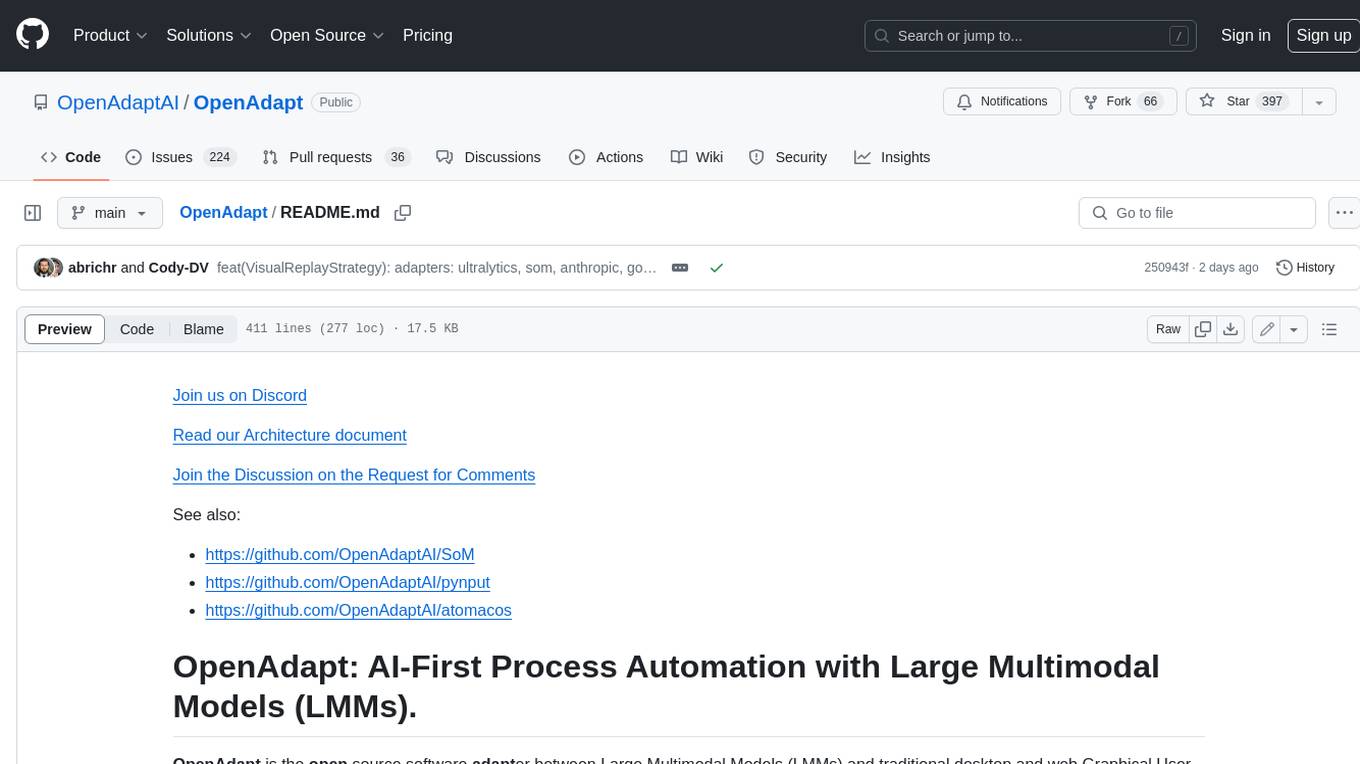
OpenAdapt
OpenAdapt is an open-source software adapter between Large Multimodal Models (LMMs) and traditional desktop and web Graphical User Interfaces (GUIs). It aims to automate repetitive GUI workflows by leveraging the power of LMMs. OpenAdapt records user input and screenshots, converts them into tokenized format, and generates synthetic input via transformer model completions. It also analyzes recordings to generate task trees and replay synthetic input to complete tasks. OpenAdapt is model agnostic and generates prompts automatically by learning from human demonstration, ensuring that agents are grounded in existing processes and mitigating hallucinations. It works with all types of desktop GUIs, including virtualized and web, and is open source under the MIT license.
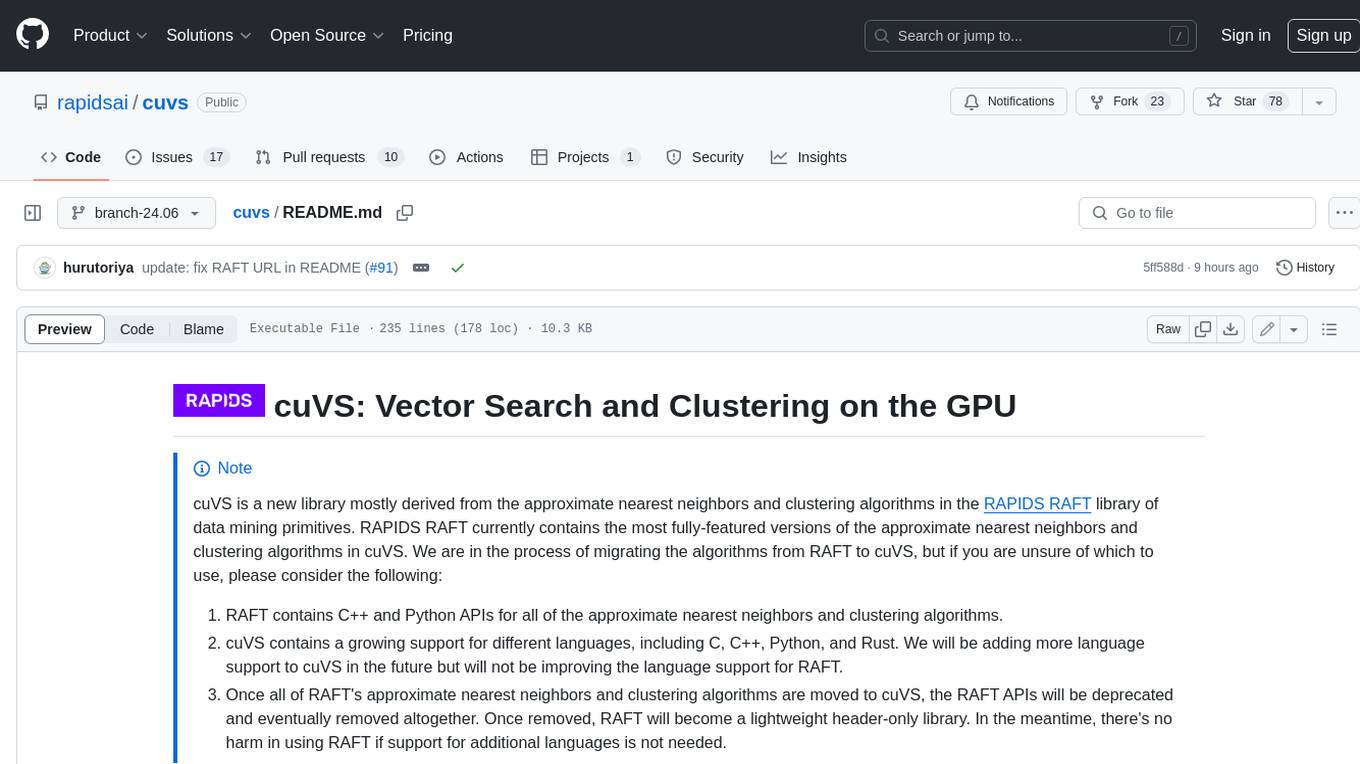
cuvs
cuVS is a library that contains state-of-the-art implementations of several algorithms for running approximate nearest neighbors and clustering on the GPU. It can be used directly or through the various databases and other libraries that have integrated it. The primary goal of cuVS is to simplify the use of GPUs for vector similarity search and clustering.
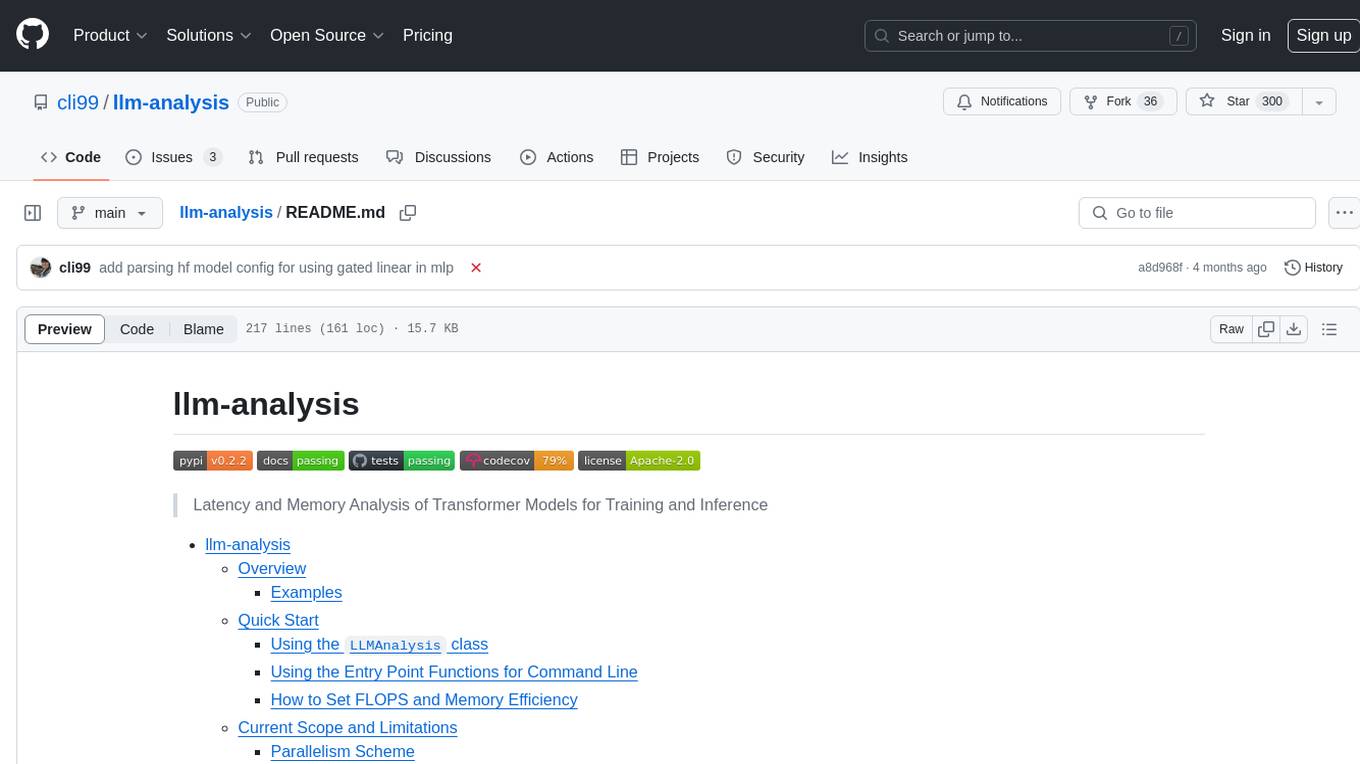
llm-analysis
llm-analysis is a tool designed for Latency and Memory Analysis of Transformer Models for Training and Inference. It automates the calculation of training or inference latency and memory usage for Large Language Models (LLMs) or Transformers based on specified model, GPU, data type, and parallelism configurations. The tool helps users to experiment with different setups theoretically, understand system performance, and optimize training/inference scenarios. It supports various parallelism schemes, communication methods, activation recomputation options, data types, and fine-tuning strategies. Users can integrate llm-analysis in their code using the `LLMAnalysis` class or use the provided entry point functions for command line interface. The tool provides lower-bound estimations of memory usage and latency, and aims to assist in achieving feasible and optimal setups for training or inference.
For similar tasks
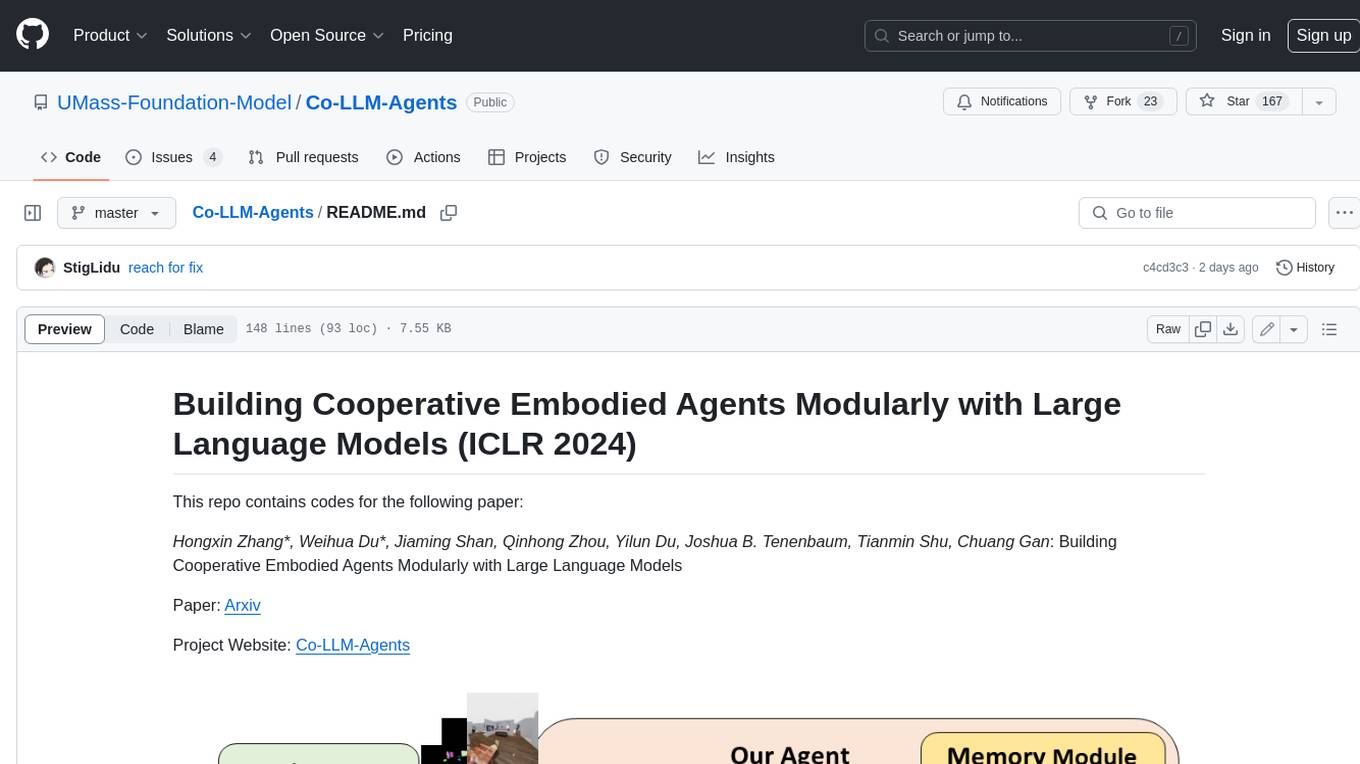
Co-LLM-Agents
This repository contains code for building cooperative embodied agents modularly with large language models. The agents are trained to perform tasks in two different environments: ThreeDWorld Multi-Agent Transport (TDW-MAT) and Communicative Watch-And-Help (C-WAH). TDW-MAT is a multi-agent environment where agents must transport objects to a goal position using containers. C-WAH is an extension of the Watch-And-Help challenge, which enables agents to send messages to each other. The code in this repository can be used to train agents to perform tasks in both of these environments.
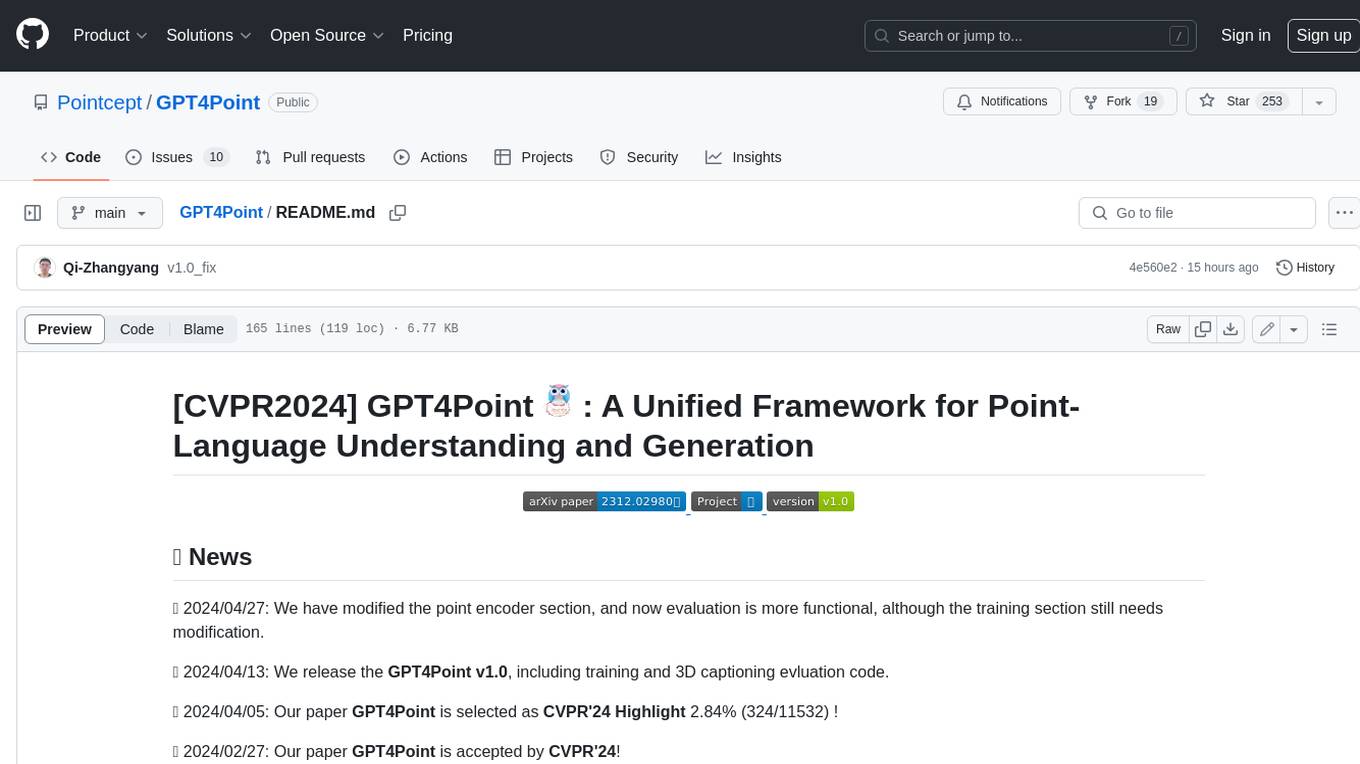
GPT4Point
GPT4Point is a unified framework for point-language understanding and generation. It aligns 3D point clouds with language, providing a comprehensive solution for tasks such as 3D captioning and controlled 3D generation. The project includes an automated point-language dataset annotation engine, a novel object-level point cloud benchmark, and a 3D multi-modality model. Users can train and evaluate models using the provided code and datasets, with a focus on improving models' understanding capabilities and facilitating the generation of 3D objects.
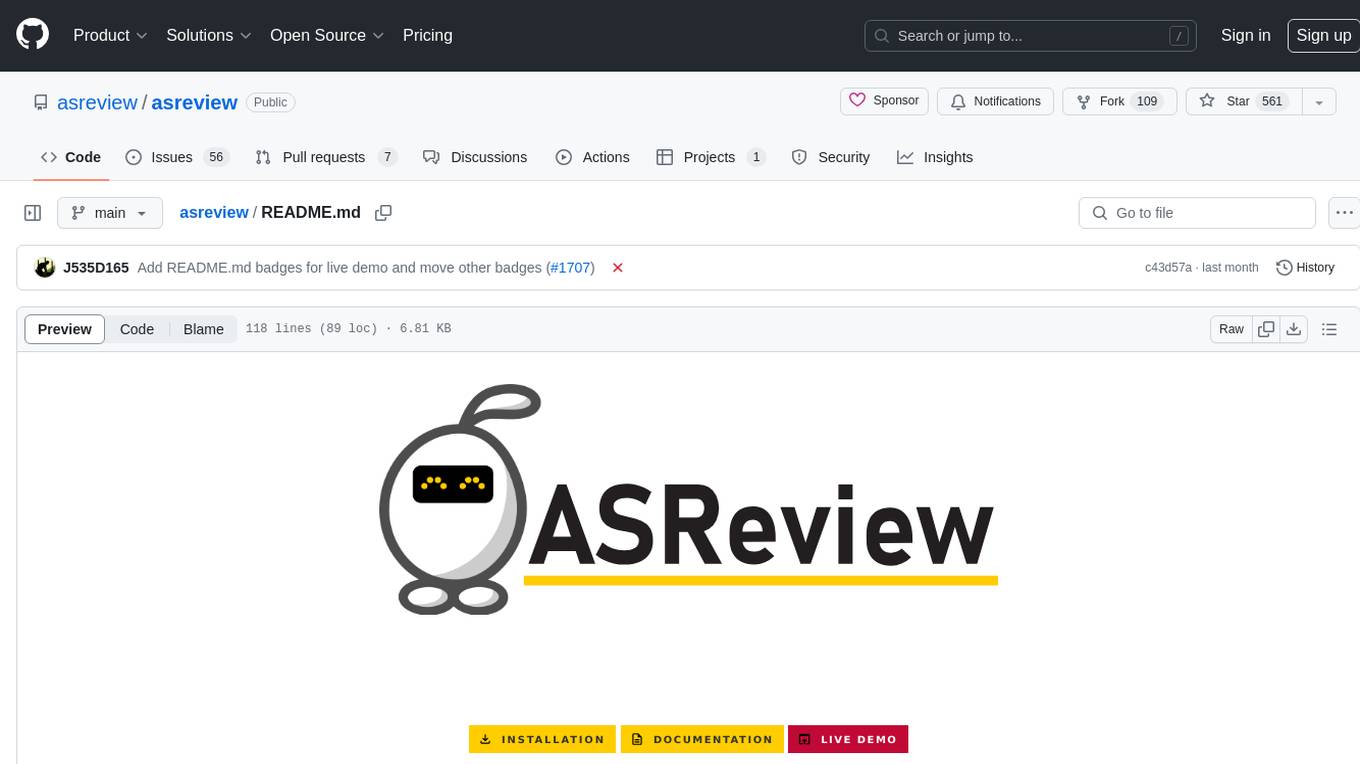
asreview
The ASReview project implements active learning for systematic reviews, utilizing AI-aided pipelines to assist in finding relevant texts for search tasks. It accelerates the screening of textual data with minimal human input, saving time and increasing output quality. The software offers three modes: Oracle for interactive screening, Exploration for teaching purposes, and Simulation for evaluating active learning models. ASReview LAB is designed to support decision-making in any discipline or industry by improving efficiency and transparency in screening large amounts of textual data.
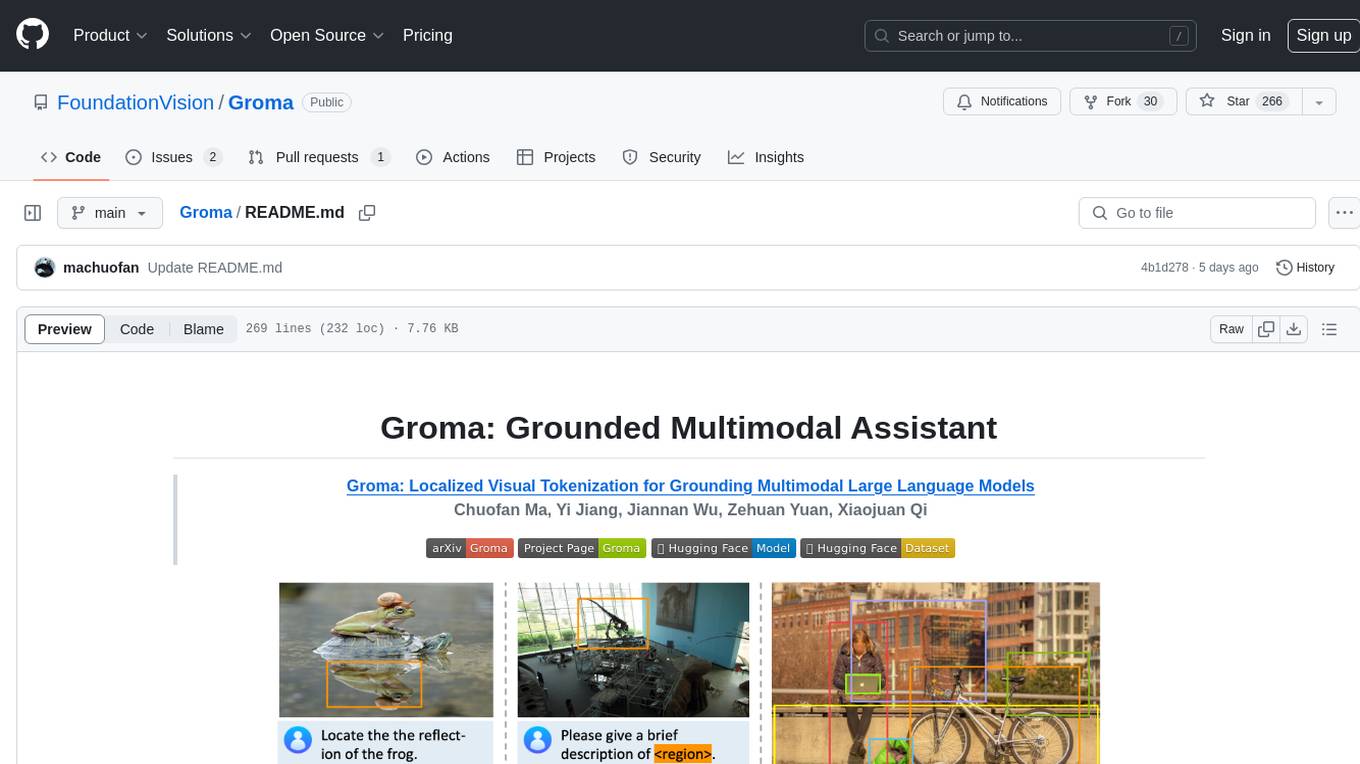
Groma
Groma is a grounded multimodal assistant that excels in region understanding and visual grounding. It can process user-defined region inputs and generate contextually grounded long-form responses. The tool presents a unique paradigm for multimodal large language models, focusing on visual tokenization for localization. Groma achieves state-of-the-art performance in referring expression comprehension benchmarks. The tool provides pretrained model weights and instructions for data preparation, training, inference, and evaluation. Users can customize training by starting from intermediate checkpoints. Groma is designed to handle tasks related to detection pretraining, alignment pretraining, instruction finetuning, instruction following, and more.
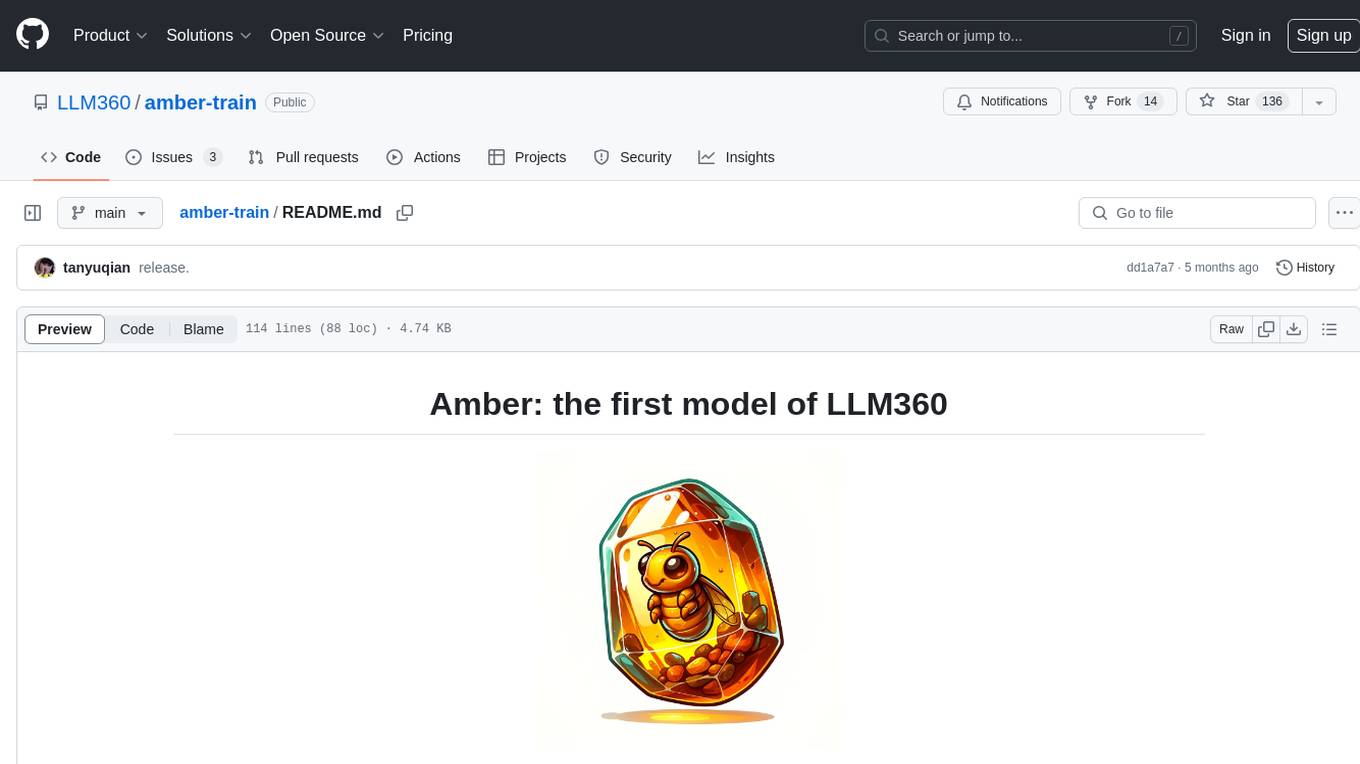
amber-train
Amber is the first model in the LLM360 family, an initiative for comprehensive and fully open-sourced LLMs. It is a 7B English language model with the LLaMA architecture. The model type is a language model with the same architecture as LLaMA-7B. It is licensed under Apache 2.0. The resources available include training code, data preparation, metrics, and fully processed Amber pretraining data. The model has been trained on various datasets like Arxiv, Book, C4, Refined-Web, StarCoder, StackExchange, and Wikipedia. The hyperparameters include a total of 6.7B parameters, hidden size of 4096, intermediate size of 11008, 32 attention heads, 32 hidden layers, RMSNorm ε of 1e^-6, max sequence length of 2048, and a vocabulary size of 32000.
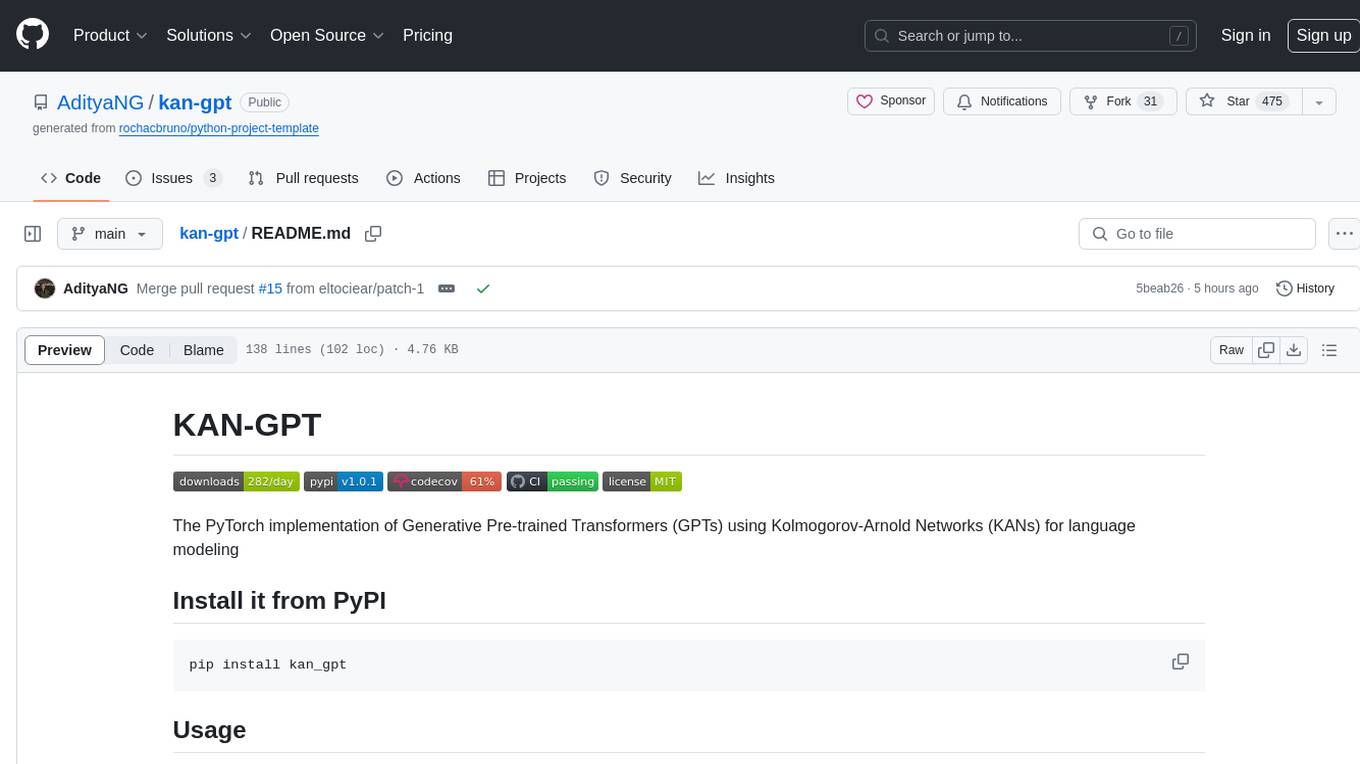
kan-gpt
The KAN-GPT repository is a PyTorch implementation of Generative Pre-trained Transformers (GPTs) using Kolmogorov-Arnold Networks (KANs) for language modeling. It provides a model for generating text based on prompts, with a focus on improving performance compared to traditional MLP-GPT models. The repository includes scripts for training the model, downloading datasets, and evaluating model performance. Development tasks include integrating with other libraries, testing, and documentation.
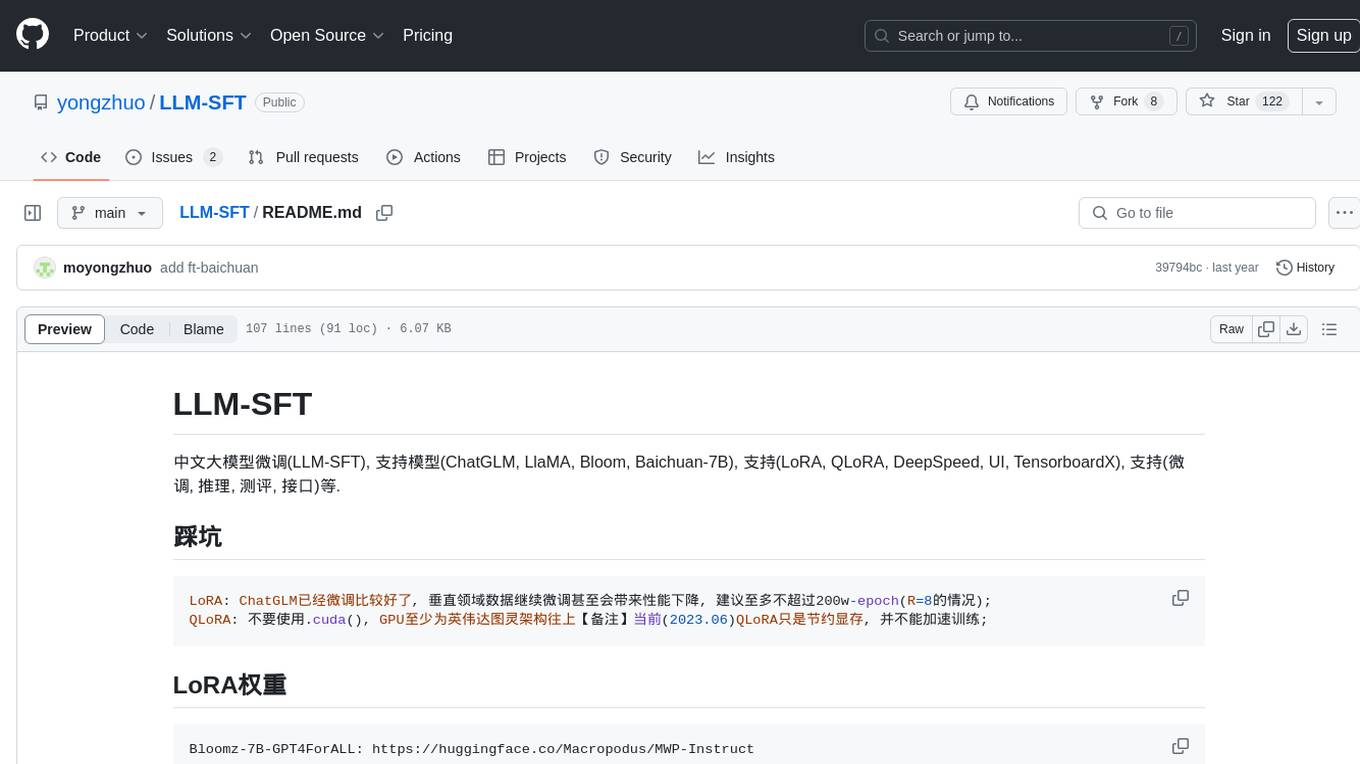
LLM-SFT
LLM-SFT is a Chinese large model fine-tuning tool that supports models such as ChatGLM, LlaMA, Bloom, Baichuan-7B, and frameworks like LoRA, QLoRA, DeepSpeed, UI, and TensorboardX. It facilitates tasks like fine-tuning, inference, evaluation, and API integration. The tool provides pre-trained weights for various models and datasets for Chinese language processing. It requires specific versions of libraries like transformers and torch for different functionalities.
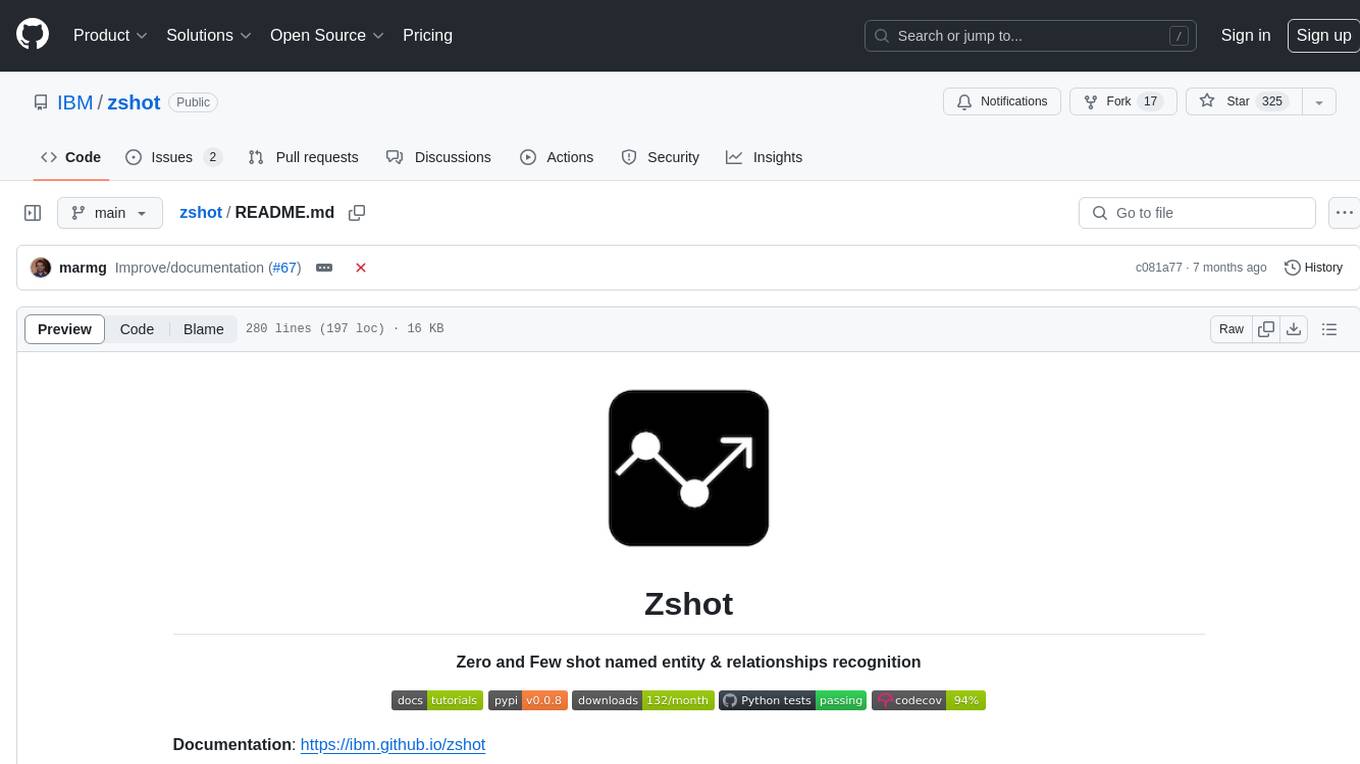
zshot
Zshot is a highly customizable framework for performing Zero and Few shot named entity and relationships recognition. It can be used for mentions extraction, wikification, zero and few shot named entity recognition, zero and few shot named relationship recognition, and visualization of zero-shot NER and RE extraction. The framework consists of two main components: the mentions extractor and the linker. There are multiple mentions extractors and linkers available, each serving a specific purpose. Zshot also includes a relations extractor and a knowledge extractor for extracting relations among entities and performing entity classification. The tool requires Python 3.6+ and dependencies like spacy, torch, transformers, evaluate, and datasets for evaluation over datasets like OntoNotes. Optional dependencies include flair and blink for additional functionalities. Zshot provides examples, tutorials, and evaluation methods to assess the performance of the components.
For similar jobs
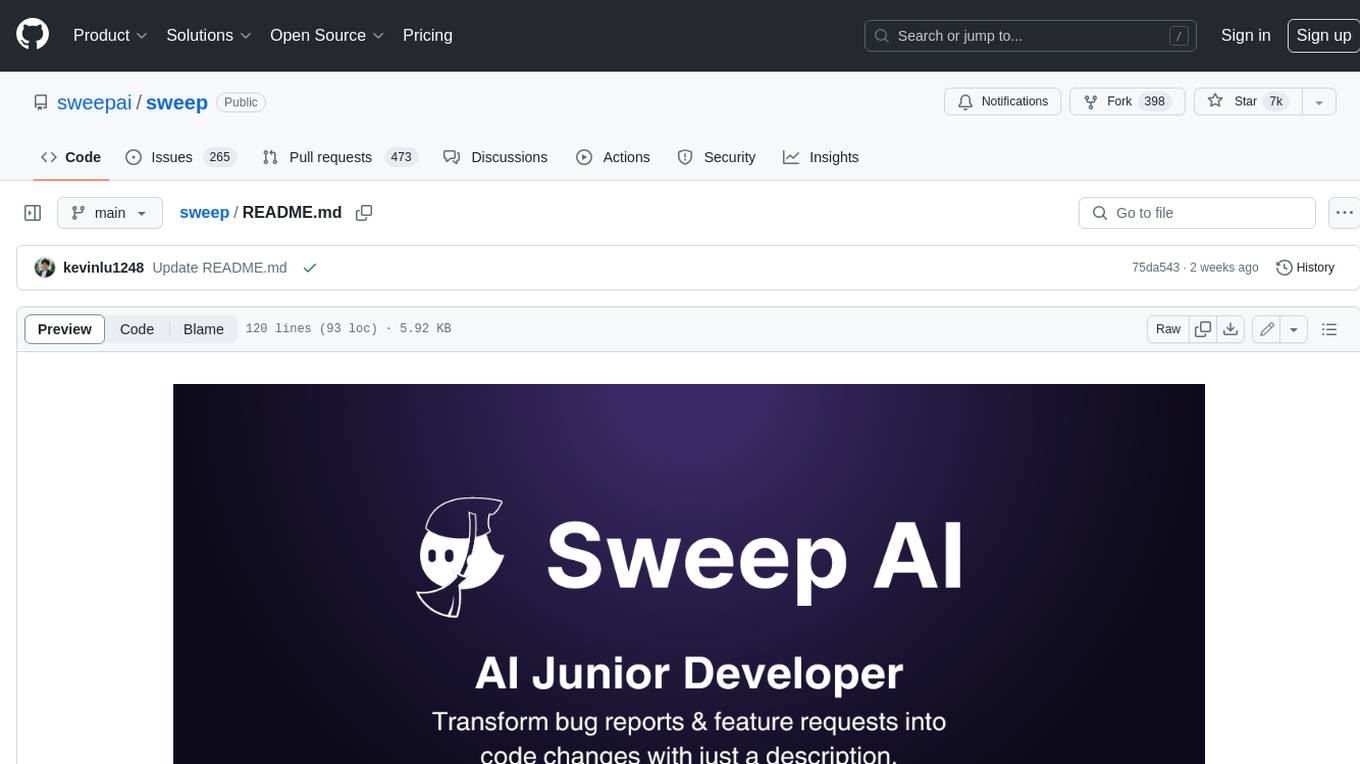
sweep
Sweep is an AI junior developer that turns bugs and feature requests into code changes. It automatically handles developer experience improvements like adding type hints and improving test coverage.
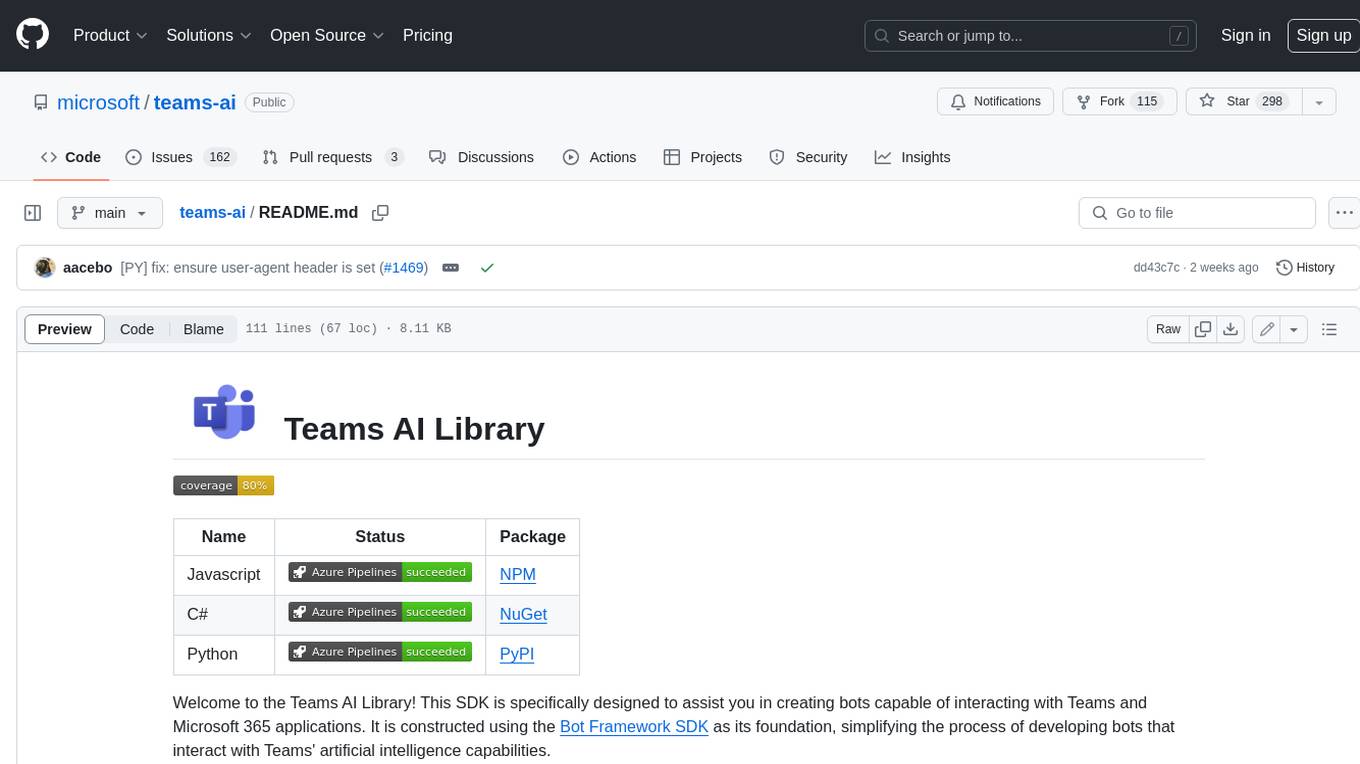
teams-ai
The Teams AI Library is a software development kit (SDK) that helps developers create bots that can interact with Teams and Microsoft 365 applications. It is built on top of the Bot Framework SDK and simplifies the process of developing bots that interact with Teams' artificial intelligence capabilities. The SDK is available for JavaScript/TypeScript, .NET, and Python.
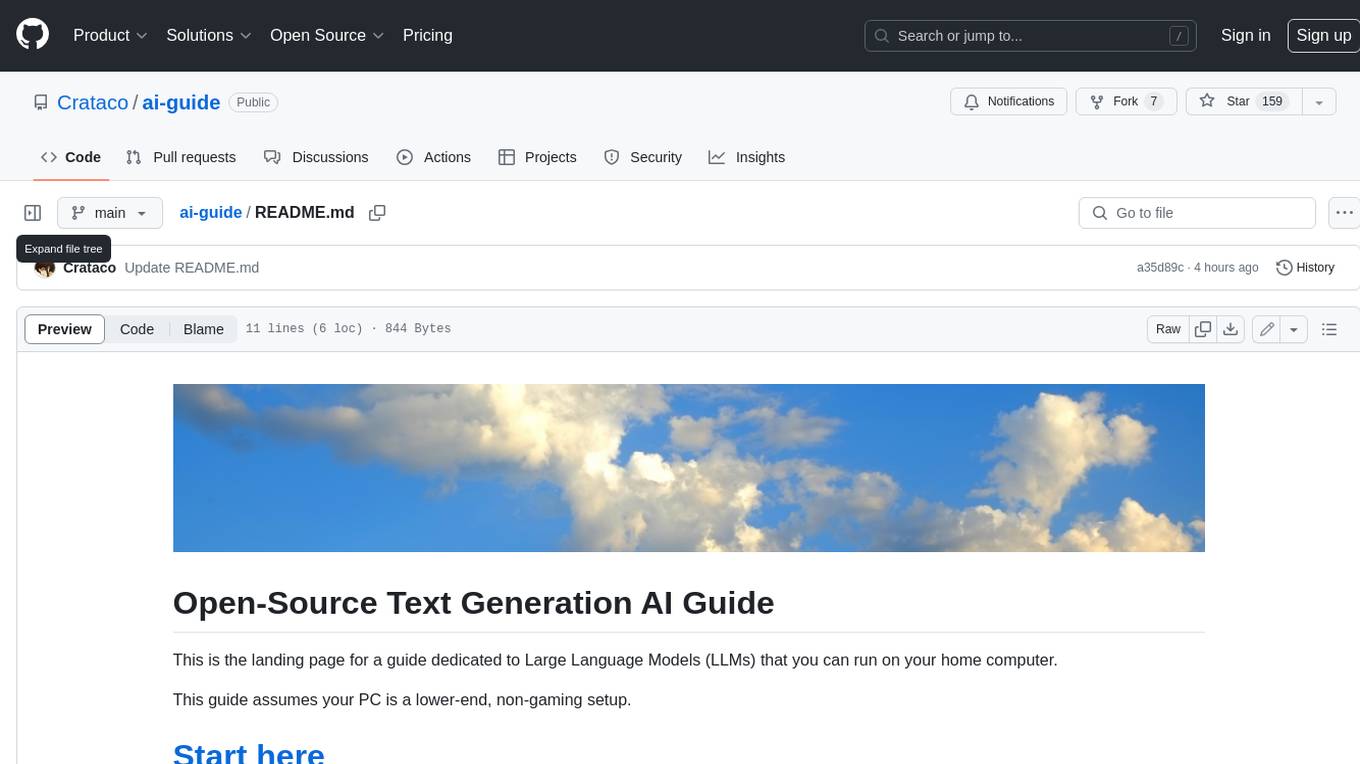
ai-guide
This guide is dedicated to Large Language Models (LLMs) that you can run on your home computer. It assumes your PC is a lower-end, non-gaming setup.
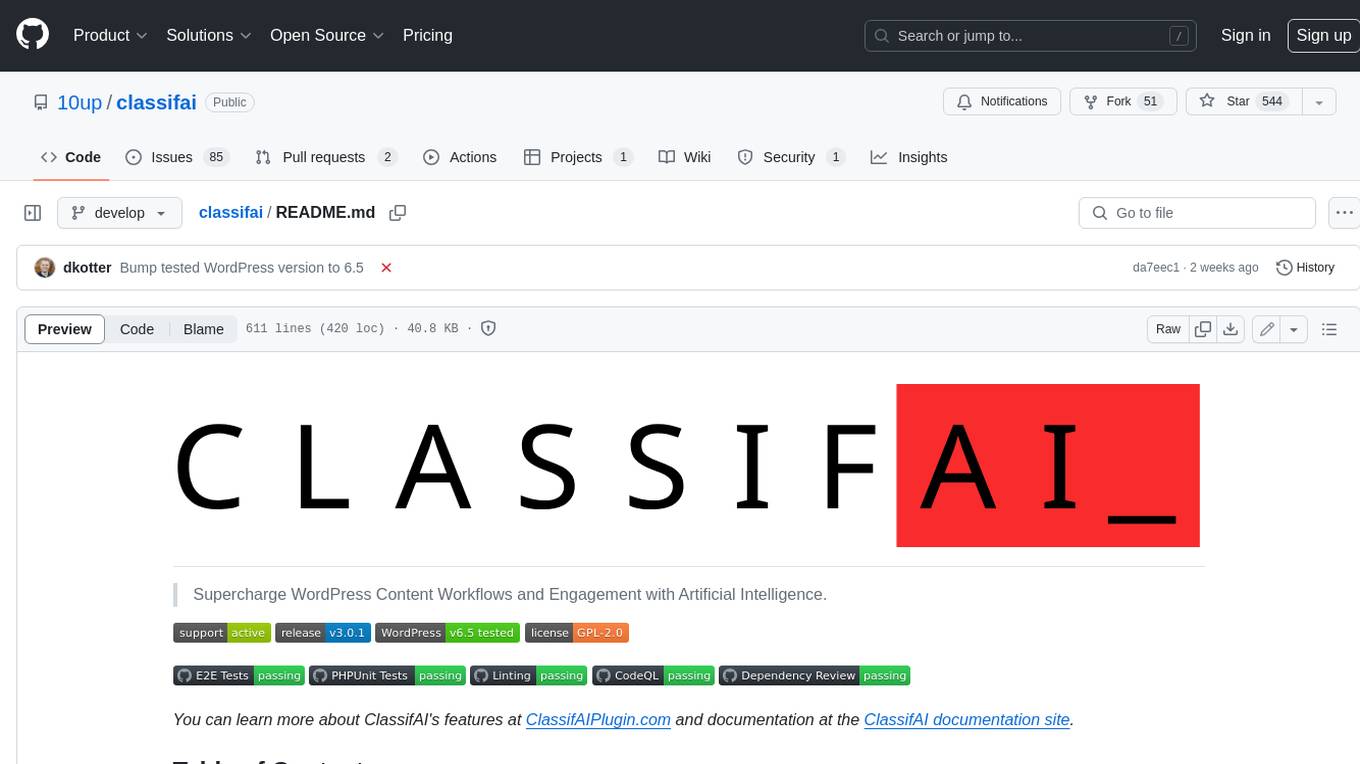
classifai
Supercharge WordPress Content Workflows and Engagement with Artificial Intelligence. Tap into leading cloud-based services like OpenAI, Microsoft Azure AI, Google Gemini and IBM Watson to augment your WordPress-powered websites. Publish content faster while improving SEO performance and increasing audience engagement. ClassifAI integrates Artificial Intelligence and Machine Learning technologies to lighten your workload and eliminate tedious tasks, giving you more time to create original content that matters.
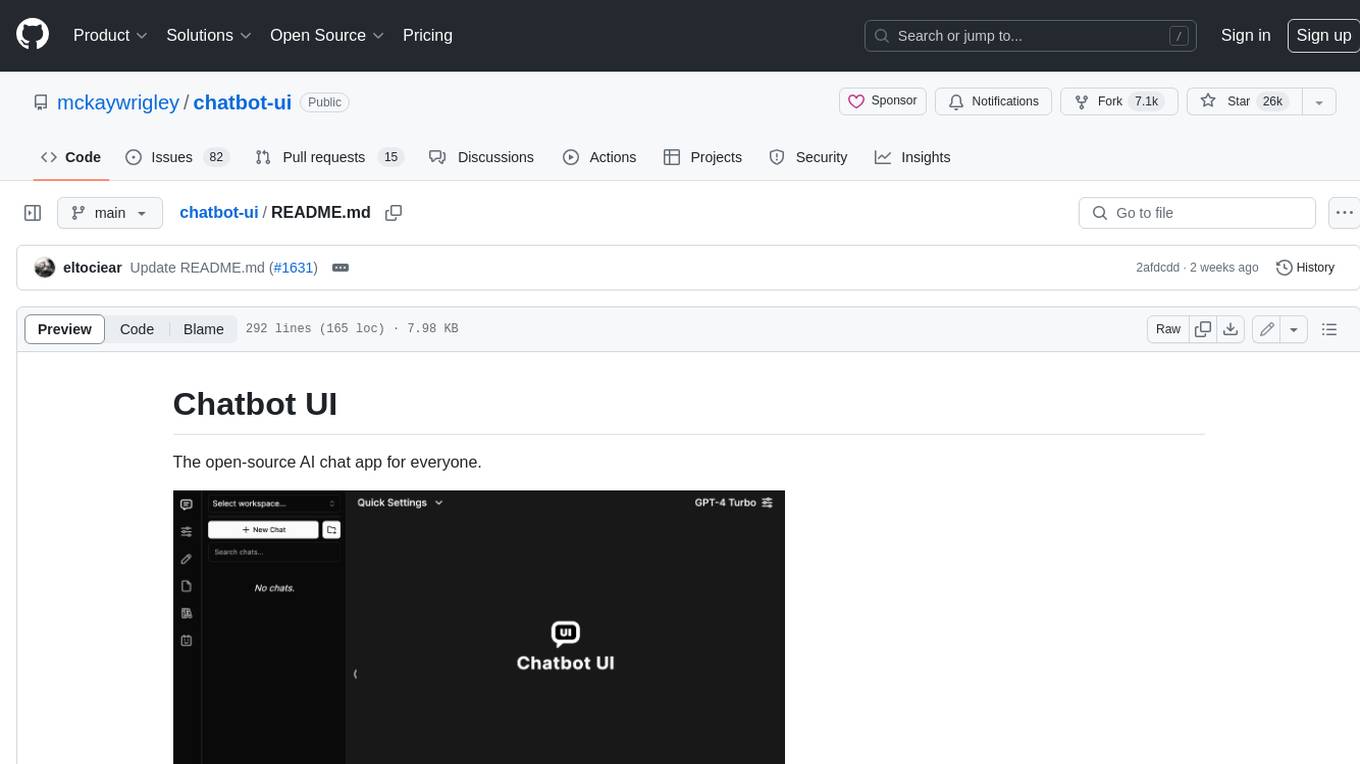
chatbot-ui
Chatbot UI is an open-source AI chat app that allows users to create and deploy their own AI chatbots. It is easy to use and can be customized to fit any need. Chatbot UI is perfect for businesses, developers, and anyone who wants to create a chatbot.
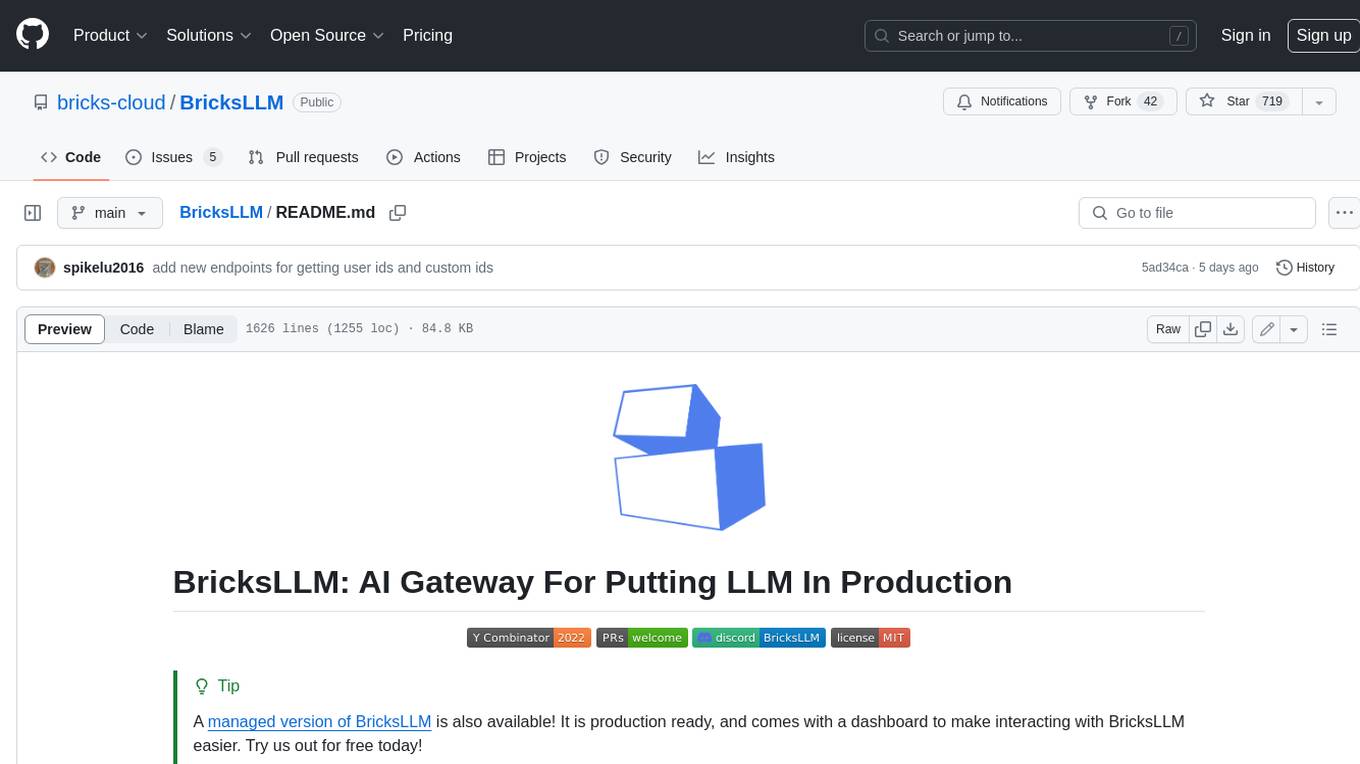
BricksLLM
BricksLLM is a cloud native AI gateway written in Go. Currently, it provides native support for OpenAI, Anthropic, Azure OpenAI and vLLM. BricksLLM aims to provide enterprise level infrastructure that can power any LLM production use cases. Here are some use cases for BricksLLM: * Set LLM usage limits for users on different pricing tiers * Track LLM usage on a per user and per organization basis * Block or redact requests containing PIIs * Improve LLM reliability with failovers, retries and caching * Distribute API keys with rate limits and cost limits for internal development/production use cases * Distribute API keys with rate limits and cost limits for students
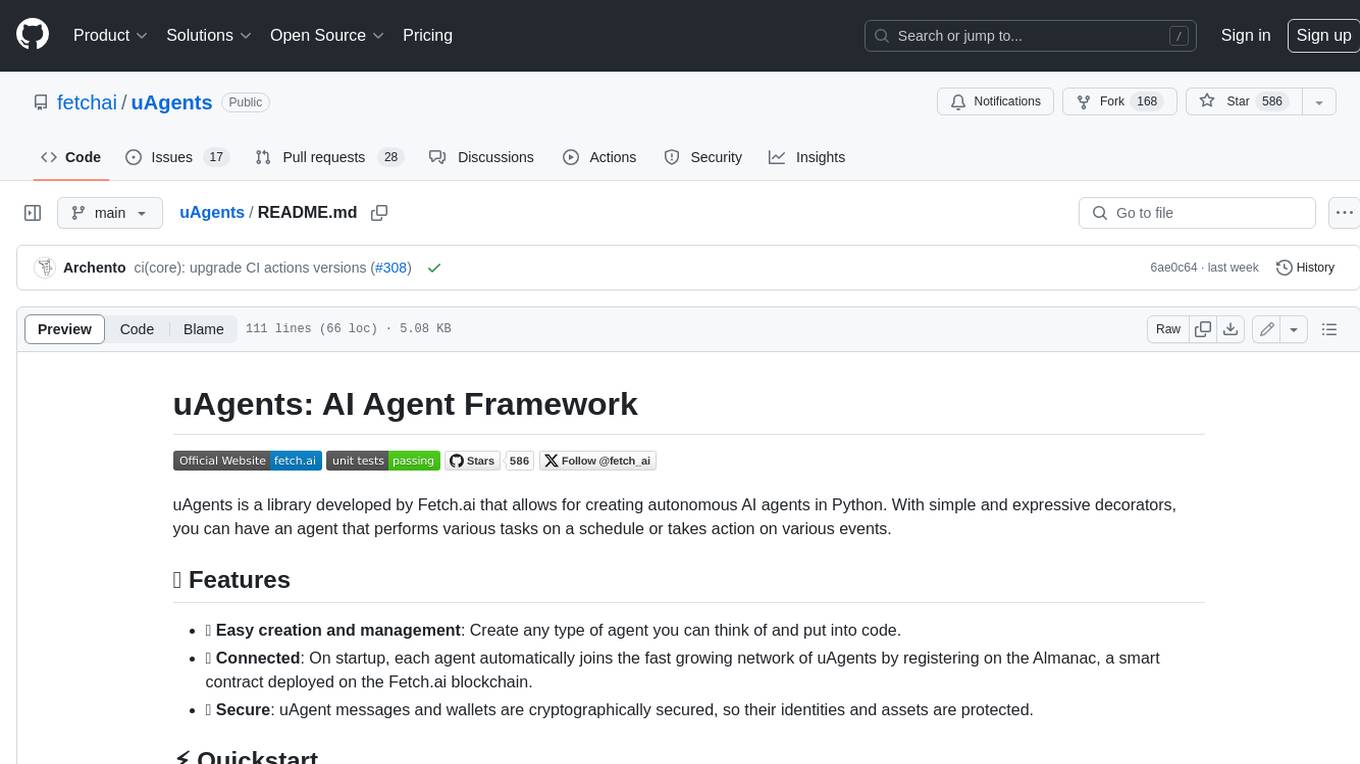
uAgents
uAgents is a Python library developed by Fetch.ai that allows for the creation of autonomous AI agents. These agents can perform various tasks on a schedule or take action on various events. uAgents are easy to create and manage, and they are connected to a fast-growing network of other uAgents. They are also secure, with cryptographically secured messages and wallets.
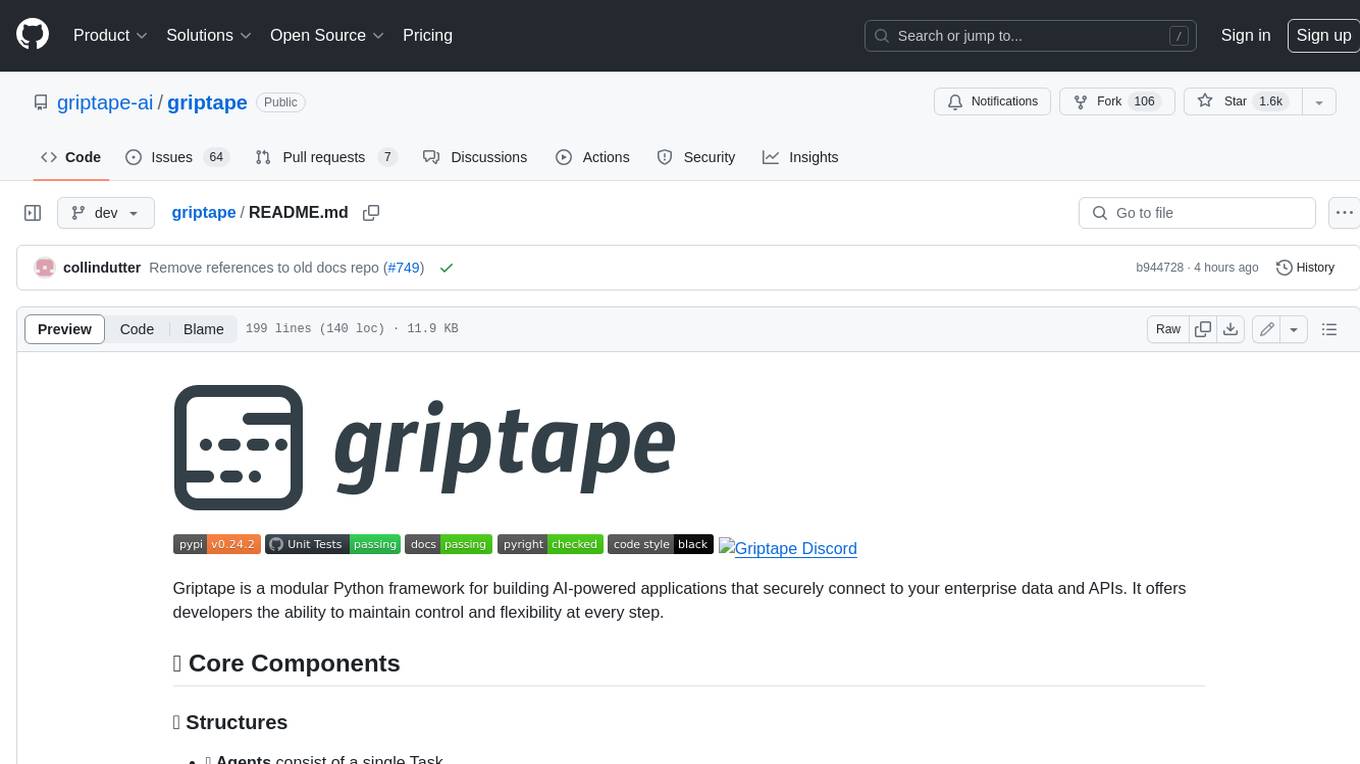
griptape
Griptape is a modular Python framework for building AI-powered applications that securely connect to your enterprise data and APIs. It offers developers the ability to maintain control and flexibility at every step. Griptape's core components include Structures (Agents, Pipelines, and Workflows), Tasks, Tools, Memory (Conversation Memory, Task Memory, and Meta Memory), Drivers (Prompt and Embedding Drivers, Vector Store Drivers, Image Generation Drivers, Image Query Drivers, SQL Drivers, Web Scraper Drivers, and Conversation Memory Drivers), Engines (Query Engines, Extraction Engines, Summary Engines, Image Generation Engines, and Image Query Engines), and additional components (Rulesets, Loaders, Artifacts, Chunkers, and Tokenizers). Griptape enables developers to create AI-powered applications with ease and efficiency.