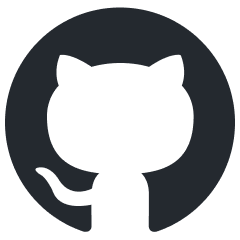
dodari
AI 영한-한영 번역기, 도다리 en-ko/ ko-en AI translator
Stars: 168
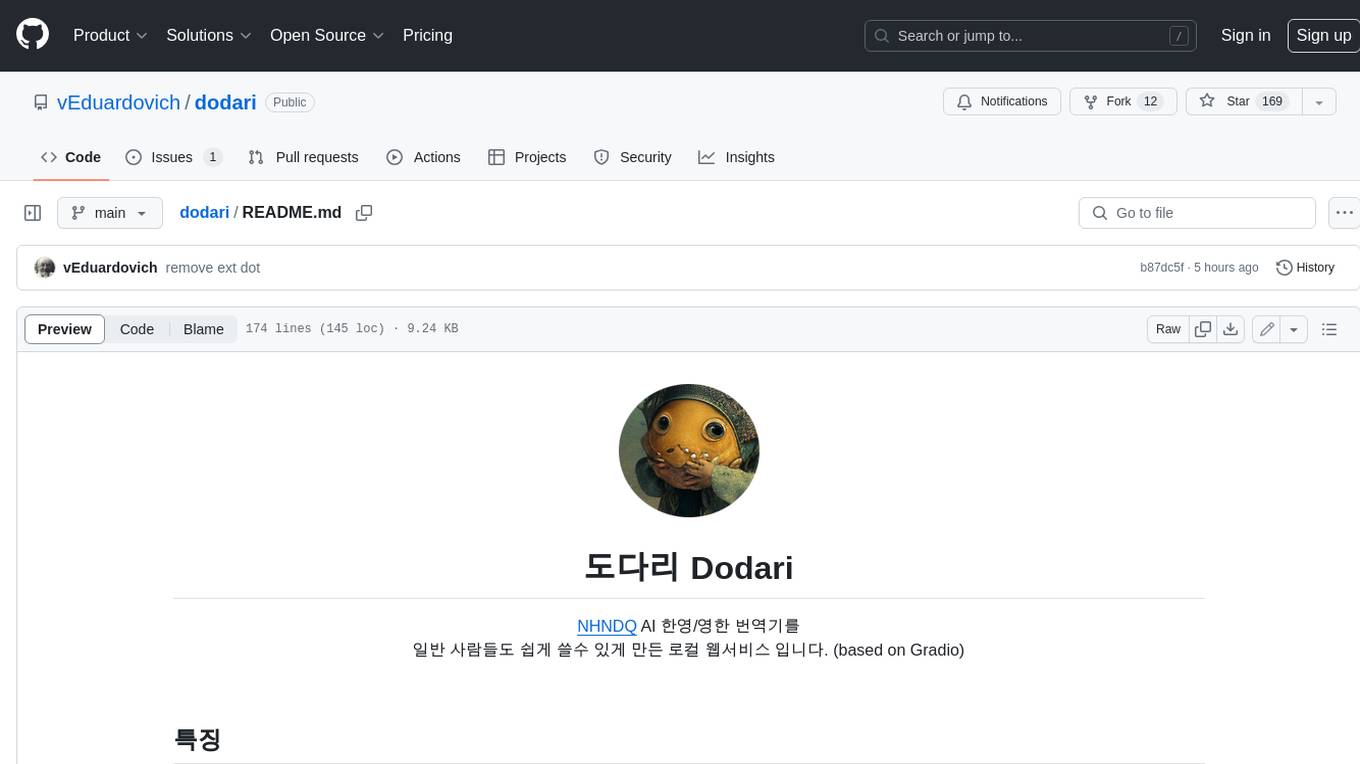
Dodari is a local web service that makes NHNDQ AI Korean-English/English-Korean translation easy for everyday people to use (based on Gradio).
README:
NHNDQ
AI 한영/영한 번역기를
일반 사람들도 쉽게 쓸수 있게 만든 로컬 웹서비스 입니다. (based on Gradio)
자신의 컴퓨터에서 제한없이 한영
-영한
AI 번역이 가능합니다.
-
일반 기계번역에 비해 품질이 우수합니다.
-
txt와 epub파일 번역이 가능합니다. epub은 제목이나 목차등을 제외한 본문의 내용만 번역하였습니다.
-
번역문(원문)
파일과번역문
파일, 이렇게 두가지 파일로 출력됩니다. 번역이 이상할 경우 원문과 바로 비교할수 있습니다. -
사용이 아주 쉽습니다. 번역이 필요한 파일들을 드래그한 후
번역 실행하기
버튼만 클릭하면 됩니다. 알아서한↔영
으로 번역해 줍니다. -
번역 성능이 뛰어난 모델로 최신 업데이트가 가능합니다 - 현재는 가성비가 가장 좋은 NHNDQ만 사용합니다.
아래와 같이 두개의 파일이 만들어집니다.
도다리 번역 - 번역문(원문)파일
도다리 번역 - 번역문파일
(참고) DeepL 번역
"토끼굴 아래로 앨리스는 언니 옆에 앉아서 할 일이 없는 것에 매우 지치기 시작했고, 언니가 읽고 있는 책을 한두 번 들여다봤지만, 그 책에는 그림이나 대화가 없었습니다."그림이나 대화가 없는 책이 무슨 소용이 있을까?"앨리스는 생각했습니다. "그래서 그녀는 데이지 사슬을 만드는 즐거움이 일어나서 데이지를 따는 수고로움의 가치가 있는지, (더운 날이 그녀를 매우 졸리고 멍청하게 만들었 기 때문에 가능한 한 잘 생각했습니다) 마음 속으로 생각하고 있었는데 갑자기 분홍색 눈을 가진 하얀 토끼가 그녀 곁으로 달려갔습니다."거기에는 그렇게 놀라운 것도 없었고 앨리스도 토끼가 스스로 말하는 것을 듣는 것이 그렇게 매우 이상하게 생각하지 않았습니다."오 맙소사! 오, 이런! 너무 늦겠어!" (나중에 곰곰이 생각해보니 앨리스가 이걸 궁금해했어야 했다는 생각이 들었지만, 그 당시에는 모든 것이 아주 자연스러워 보였습니다); "그러나 토끼가 실제로 양복 조끼 주머니에서 시계를 꺼내어 그것을 보고는 서둘러 가자 앨리스는 발걸음을 재촉했습니다. 왜냐하면 양복 조끼 주머니를 가진 토끼나 시계를 꺼내는 토끼를 본 적이 없다는 생각이 스쳐 지나갔고, 호기심에 불타서 그 토끼를 따라 들판을 가로질러 뛰어갔을 때 마침 울타리 아래 커다란 토끼 구멍으로 토끼가 튀어 나오는 것을 보았기 때문입니다." "앨리스는 도대체 어떻게 다시 빠져나올 수 있을지 한 번도 생각하지 않은 채 토끼굴을 따라 내려갔고, 토끼굴은 터널처럼 곧장 이어지다가 갑자기 아래로 가라앉아 앨리스는 멈출 생각을 할 틈도 없이 아주 깊은 우물 속으로 떨어지는 자신을 발견했다."
(참고) 구글 번역
“앨리스는 은행에서 여동생 옆에 앉아 있고 할 일이 없는 것에 매우 지치기 시작했습니다. 한두 번 그녀는 여동생이 읽고 있는 책을 들여다 보았지만 그 안에 그림이나 대화가 전혀 없었습니다. "그림이나 대화가 없는 책이 무슨 소용이 있겠는가?"라고 앨리스는 생각했습니다. 데이지 체인을 만드는 즐거움이 일어나서 데이지를 따는 수고를 할 만큼 가치가 있을지에 대해 그녀 자신의 마음 속에서 (그리고 그녀는 할 수 있는 한, 더운 날 때문에 그녀는 매우 졸리고 멍청하다고 느꼈다) 갑자기 백인이 분홍색 눈을 가진 토끼가 그녀 옆으로 달려왔습니다. 그다지 주목할만한 점은 없었습니다. 앨리스는 토끼가 혼잣말하는 것을 듣고도 별로 이상하다고 생각하지 않았습니다. “오 이런! 이런! 너무 늦을 것 같아요!” (나중에 그녀가 곰곰이 생각해보니, 그녀는 이것에 대해 궁금해했어야 했다는 생각이 들었지만, 당시에는 모든 것이 아주 자연스러워 보였습니다.) “그러나 토끼가 실제로 양복 조끼 주머니에서 시계를 꺼내서 살펴보더니 서둘러 갔을 때, 앨리스는 일어서기 시작했습니다. 그녀는 양복 조끼 주머니나 시계를 꺼내려고 호기심에 불타서 그것을 쫓아 들판을 가로질러 달려갔고, 때맞춰 그것이 울타리 아래 커다란 토끼 굴로 떨어지는 것을 보았습니다.” "또 다른 순간에 앨리스는 그 뒤를 따라 내려갔습니다. 도대체 그녀가 다시 나갈 수 있다는 생각은 단 한 번도 하지 않았습니다. 토끼굴은 어떤 식으로든 터널처럼 곧게 이어지다가 갑자기 아래로 내려갔습니다. 너무 갑자기 내려가서 앨리스는 더 이상 빠져나갈 수 없었습니다. 그녀가 아주 깊은 우물처럼 보이는 곳으로 떨어지기 전에 자신을 멈추는 것에 대해 생각할 순간이었습니다.”
공통,
- 먼저 파이썬이 설치되어 있어야 합니다. 윈도우에는 파이썬이 기본으로 설치되어 있지 않습니다.
- https://wikidocs.net/8 를 참고하여 쉽게 설치하실수 있습니다.
초보자라면,
- 압축 파일 다운로드 클릭
- 압축해제 후
- 윈도우 사용자는 start_windows.bat 더블 클릭
- 맥이나 우분투 사용자는 터미널 창에서 sh start_mac.sh 실행
- 처음 실행이라면 프로그램을 자동으로 설치한 후 실행합니다. 이미 설치가 되었다면 바로 실행합니다.
고급 사용자라면,
- git clone https://github.com/vEduardovich/dodari.git
- cd dodari
- 아래 방법으로 실행하기
- 윈도우는 start_windows.bat 실행
- 맥, 우분투는 sh start_mac.sh 실행
첫 실행시 관련 프로그램 설치와 AI 모델을 다운로드 하는데 아주 오랜 시간이 걸립니다!
헤르만 헤세의 싯다르타 text과 이상한 나라의 앨리스(영문판).epub을 번역해봤습니다.
사양 | 운영체제 | CPU | GPU | 문장수 | 싯다르타.txt | 이상한나라의 앨리스.epub |
---|---|---|---|---|---|---|
LG전자 2020 그램 17 | 윈도우10 | i7 (1.3GHz) | 내장그래픽 | 378KB 2577개 |
2시간 55분 24초 | 3시간 45분 6초 |
Mac Pro M1 | iOS | 10코어 | 16코어 | 54분 23초 | 1시간 13분 5초 | |
데스크탑 | Ubuntu22.04 | i9-13900k | RTX4090 24GB | 5분 25초 | 7분 20초 | |
데스크탑 | 윈도우11 | i9-13900k | RTX4090 24GB | 11분 49초 | 14분 36초 |
이상한나라의 앨리스.epub, RTX 4090으로 테스트
상태 | 걸린시간 |
---|---|
vllm 미적용시 | 4시간 7분 26초 |
vllm 적용시 | 40분 5초 |
dodari
├⎯ models : AI가 다운로드되는 폴더
├⎯ venv : 도다리 실행을 위한 관련 파일들이 설치되는 폴더
├⎯ imgs : 도다리 이미지
초보자라면,
- 위 압축파일을 다시 다운로드하고 압축을 푼후
- 기존 도다리 폴더안에 덮어쓰면 됩니다.
고급 사용자라면,
- git pull
- 폴더 전체를 지우면 깨끗하게 지워집니다.
- ext dot 중복제거
- 주요 라이브러리 버전 fix
For Tasks:
Click tags to check more tools for each tasksFor Jobs:
Alternative AI tools for dodari
Similar Open Source Tools
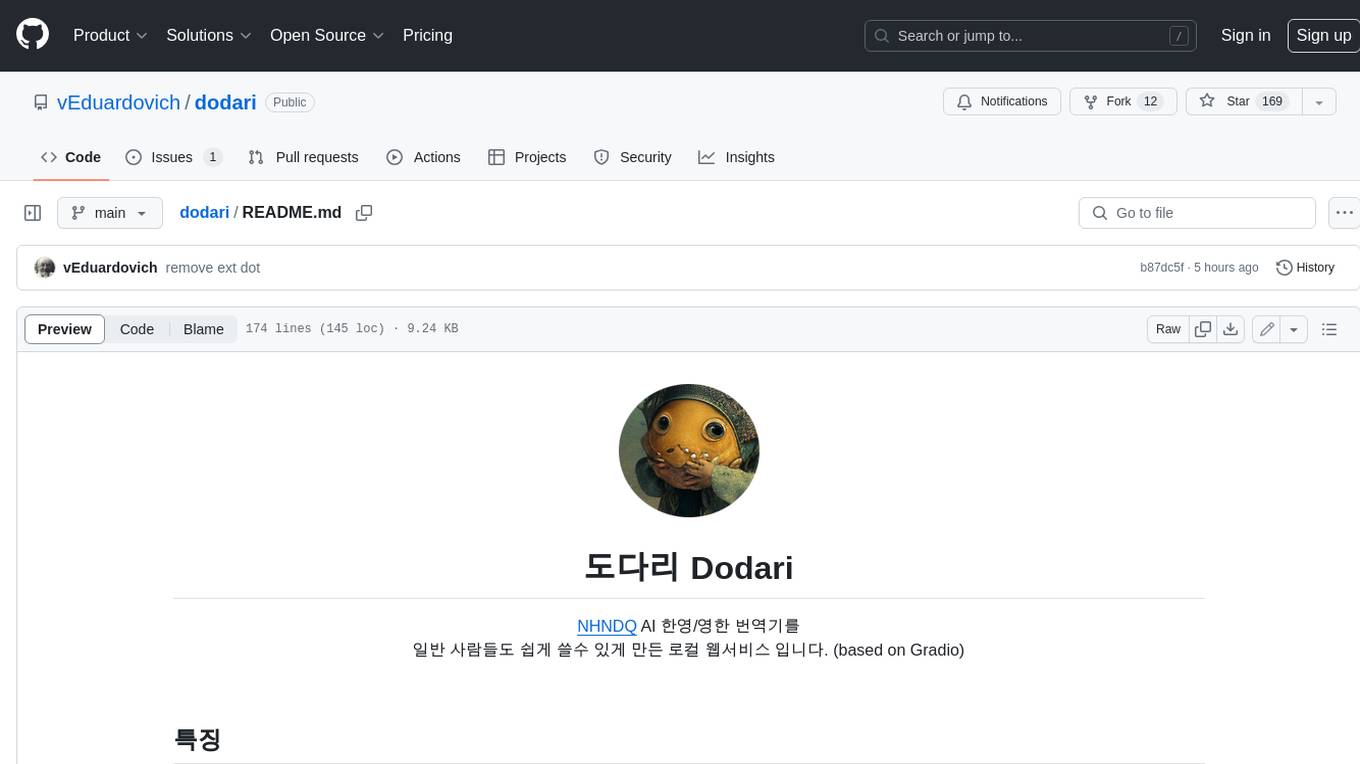
dodari
Dodari is a local web service that makes NHNDQ AI Korean-English/English-Korean translation easy for everyday people to use (based on Gradio).
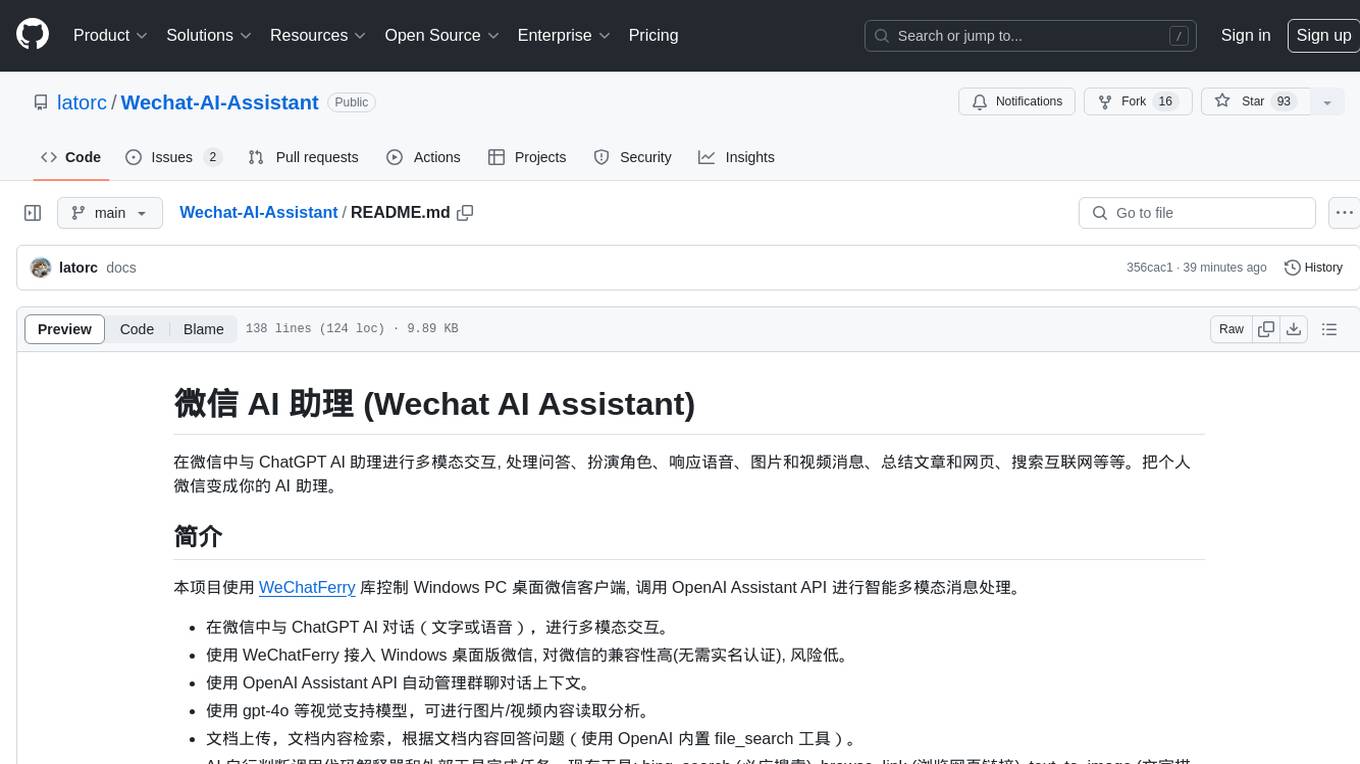
Wechat-AI-Assistant
Wechat AI Assistant is a project that enables multi-modal interaction with ChatGPT AI assistant within WeChat. It allows users to engage in conversations, role-playing, respond to voice messages, analyze images and videos, summarize articles and web links, and search the internet. The project utilizes the WeChatFerry library to control the Windows PC desktop WeChat client and leverages the OpenAI Assistant API for intelligent multi-modal message processing. Users can interact with ChatGPT AI in WeChat through text or voice, access various tools like bing_search, browse_link, image_to_text, text_to_image, text_to_speech, video_analysis, and more. The AI autonomously determines which code interpreter and external tools to use to complete tasks. Future developments include file uploads for AI to reference content, integration with other APIs, and login support for enterprise WeChat and WeChat official accounts.
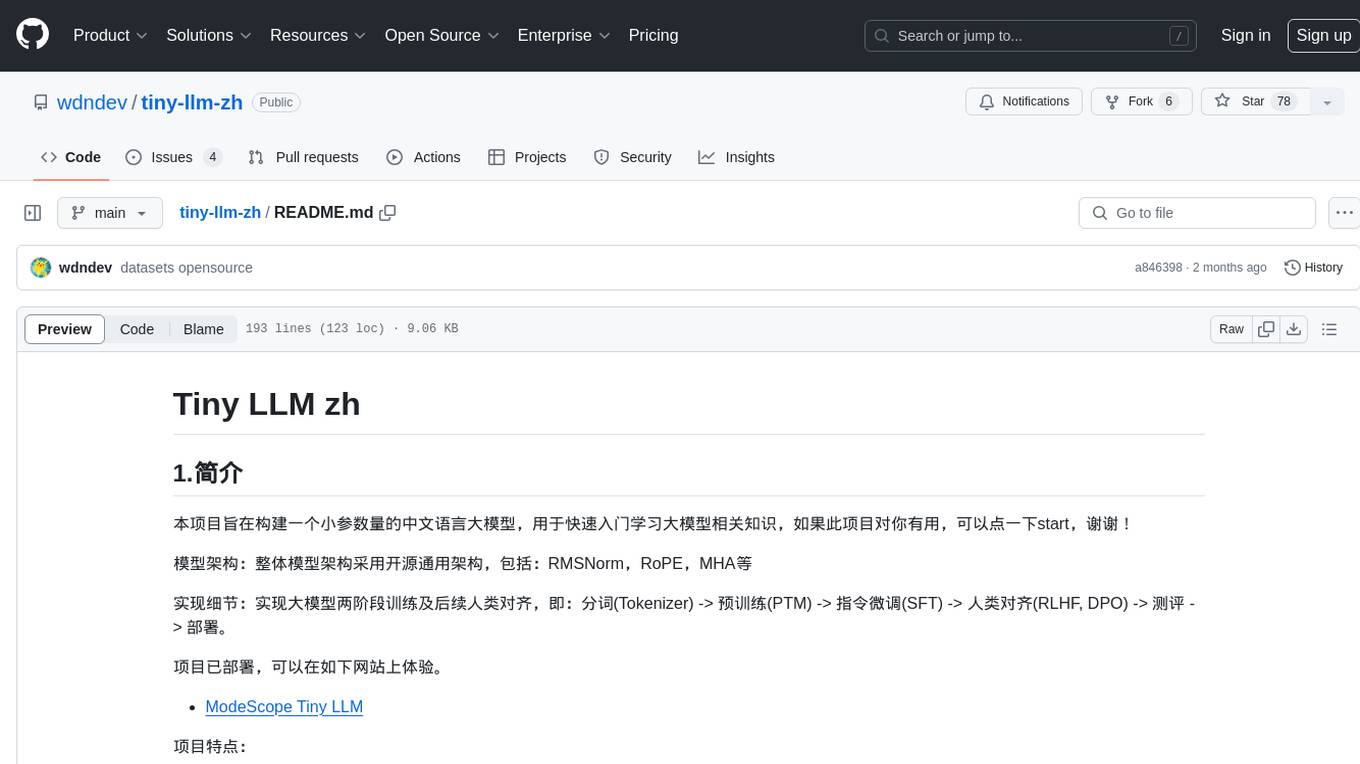
tiny-llm-zh
Tiny LLM zh is a project aimed at building a small-parameter Chinese language large model for quick entry into learning large model-related knowledge. The project implements a two-stage training process for large models and subsequent human alignment, including tokenization, pre-training, instruction fine-tuning, human alignment, evaluation, and deployment. It is deployed on ModeScope Tiny LLM website and features open access to all data and code, including pre-training data and tokenizer. The project trains a tokenizer using 10GB of Chinese encyclopedia text to build a Tiny LLM vocabulary. It supports training with Transformers deepspeed, multiple machine and card support, and Zero optimization techniques. The project has three main branches: llama2_torch, main tiny_llm, and tiny_llm_moe, each with specific modifications and features.
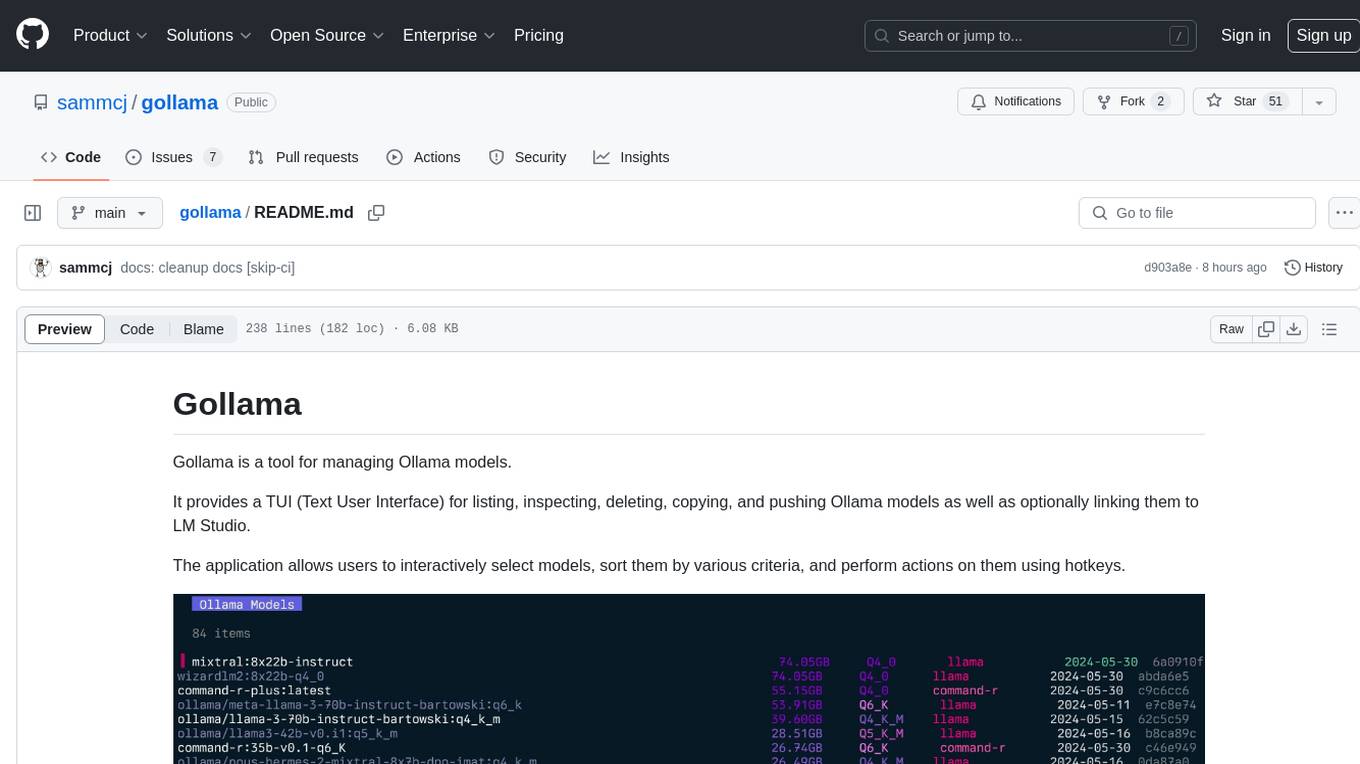
gollama
Gollama is a tool designed for managing Ollama models through a Text User Interface (TUI). Users can list, inspect, delete, copy, and push Ollama models, as well as link them to LM Studio. The application offers interactive model selection, sorting by various criteria, and actions using hotkeys. It provides features like sorting and filtering capabilities, displaying model metadata, model linking, copying, pushing, and more. Gollama aims to be user-friendly and useful for managing models, especially for cleaning up old models.
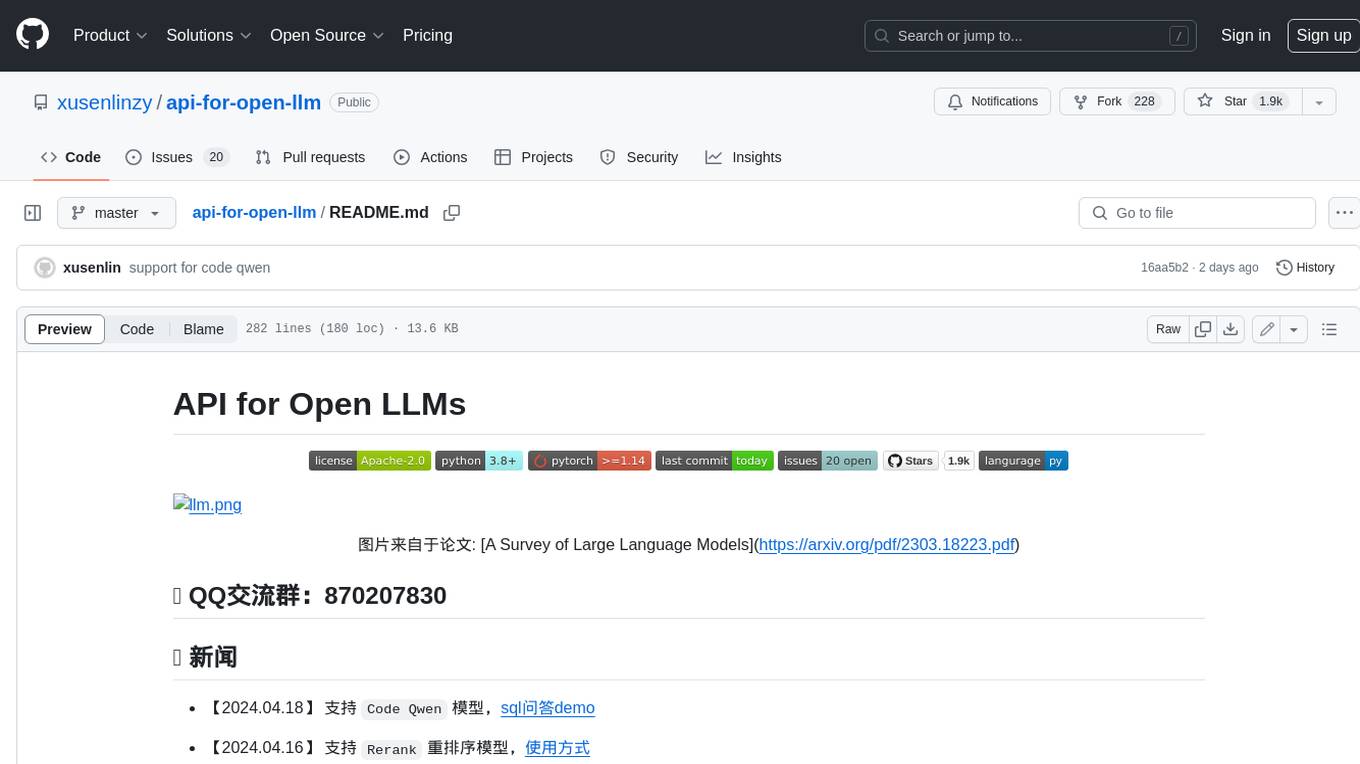
api-for-open-llm
This project provides a unified backend interface for open large language models (LLMs), offering a consistent experience with OpenAI's ChatGPT API. It supports various open-source LLMs, enabling developers to seamlessly integrate them into their applications. The interface features streaming responses, text embedding capabilities, and support for LangChain, a tool for developing LLM-based applications. By modifying environment variables, developers can easily use open-source models as alternatives to ChatGPT, providing a cost-effective and customizable solution for various use cases.
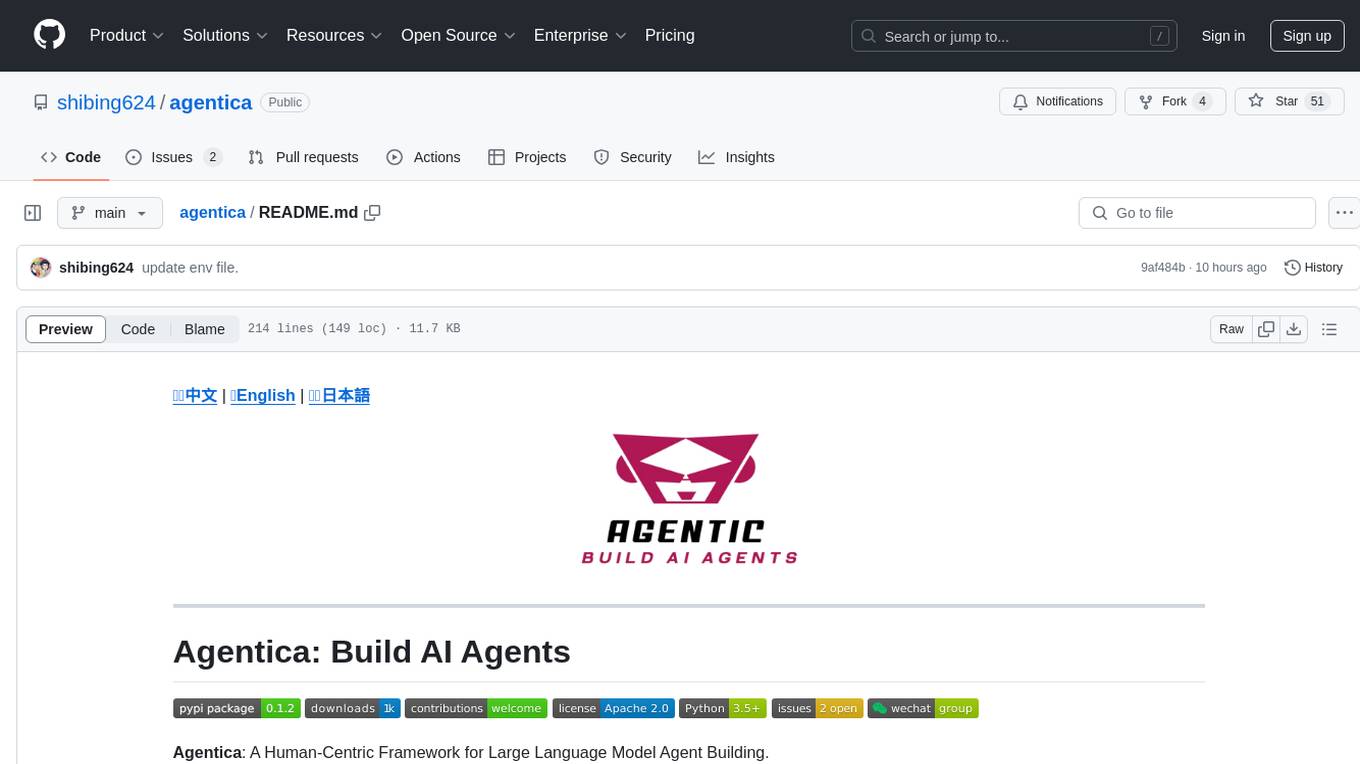
agentica
Agentica is a human-centric framework for building large language model agents. It provides functionalities for planning, memory management, tool usage, and supports features like reflection, planning and execution, RAG, multi-agent, multi-role, and workflow. The tool allows users to quickly code and orchestrate agents, customize prompts, and make API calls to various services. It supports API calls to OpenAI, Azure, Deepseek, Moonshot, Claude, Ollama, and Together. Agentica aims to simplify the process of building AI agents by providing a user-friendly interface and a range of functionalities for agent development.
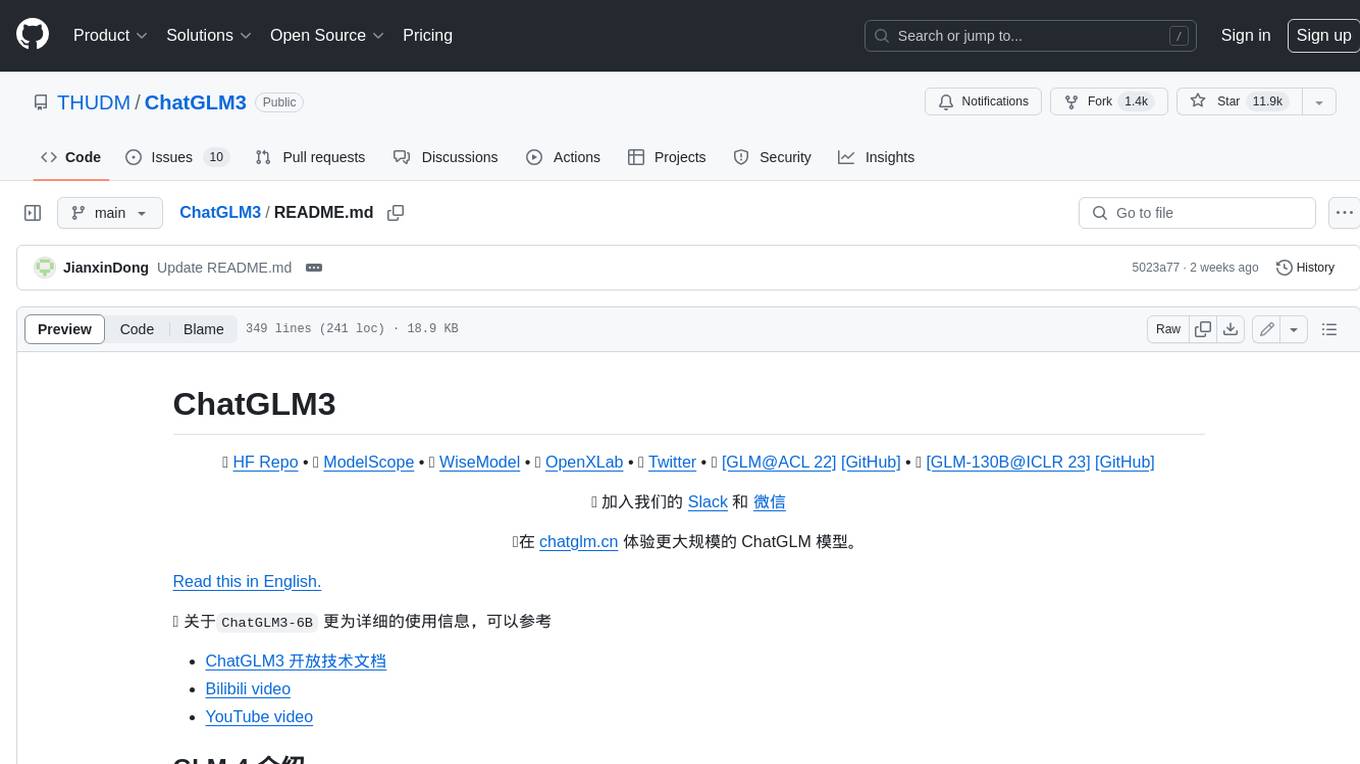
ChatGLM3
ChatGLM3 is a conversational pretrained model jointly released by Zhipu AI and THU's KEG Lab. ChatGLM3-6B is the open-sourced model in the ChatGLM3 series. It inherits the advantages of its predecessors, such as fluent conversation and low deployment threshold. In addition, ChatGLM3-6B introduces the following features: 1. A stronger foundation model: ChatGLM3-6B's foundation model ChatGLM3-6B-Base employs more diverse training data, more sufficient training steps, and more reasonable training strategies. Evaluation on datasets from different perspectives, such as semantics, mathematics, reasoning, code, and knowledge, shows that ChatGLM3-6B-Base has the strongest performance among foundation models below 10B parameters. 2. More complete functional support: ChatGLM3-6B adopts a newly designed prompt format, which supports not only normal multi-turn dialogue, but also complex scenarios such as tool invocation (Function Call), code execution (Code Interpreter), and Agent tasks. 3. A more comprehensive open-source sequence: In addition to the dialogue model ChatGLM3-6B, the foundation model ChatGLM3-6B-Base, the long-text dialogue model ChatGLM3-6B-32K, and ChatGLM3-6B-128K, which further enhances the long-text comprehension ability, are also open-sourced. All the above weights are completely open to academic research and are also allowed for free commercial use after filling out a questionnaire.
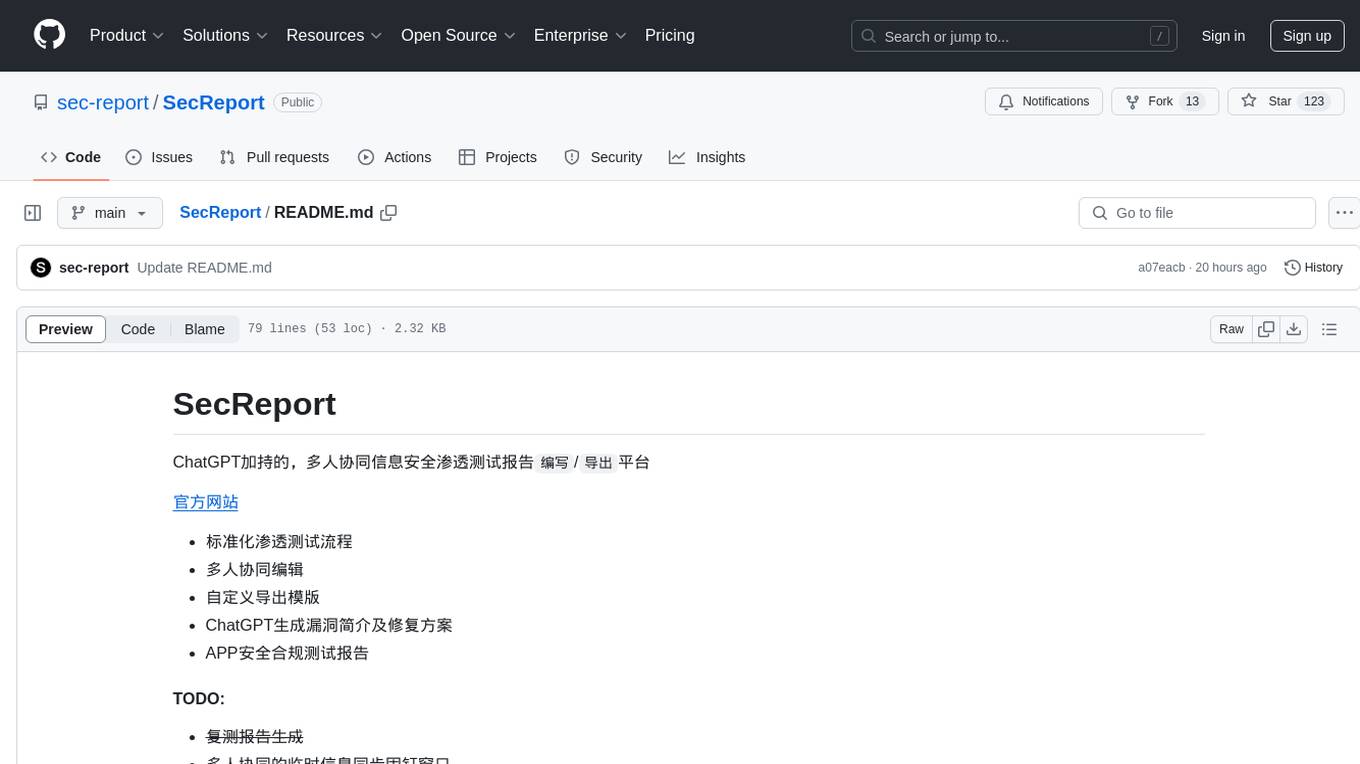
SecReport
SecReport is a platform for collaborative information security penetration testing report writing and exporting, powered by ChatGPT. It standardizes penetration testing processes, allows multiple users to edit reports, offers custom export templates, generates vulnerability summaries and fix suggestions using ChatGPT, and provides APP security compliance testing reports. The tool aims to streamline the process of creating and managing security reports for penetration testing and compliance purposes.
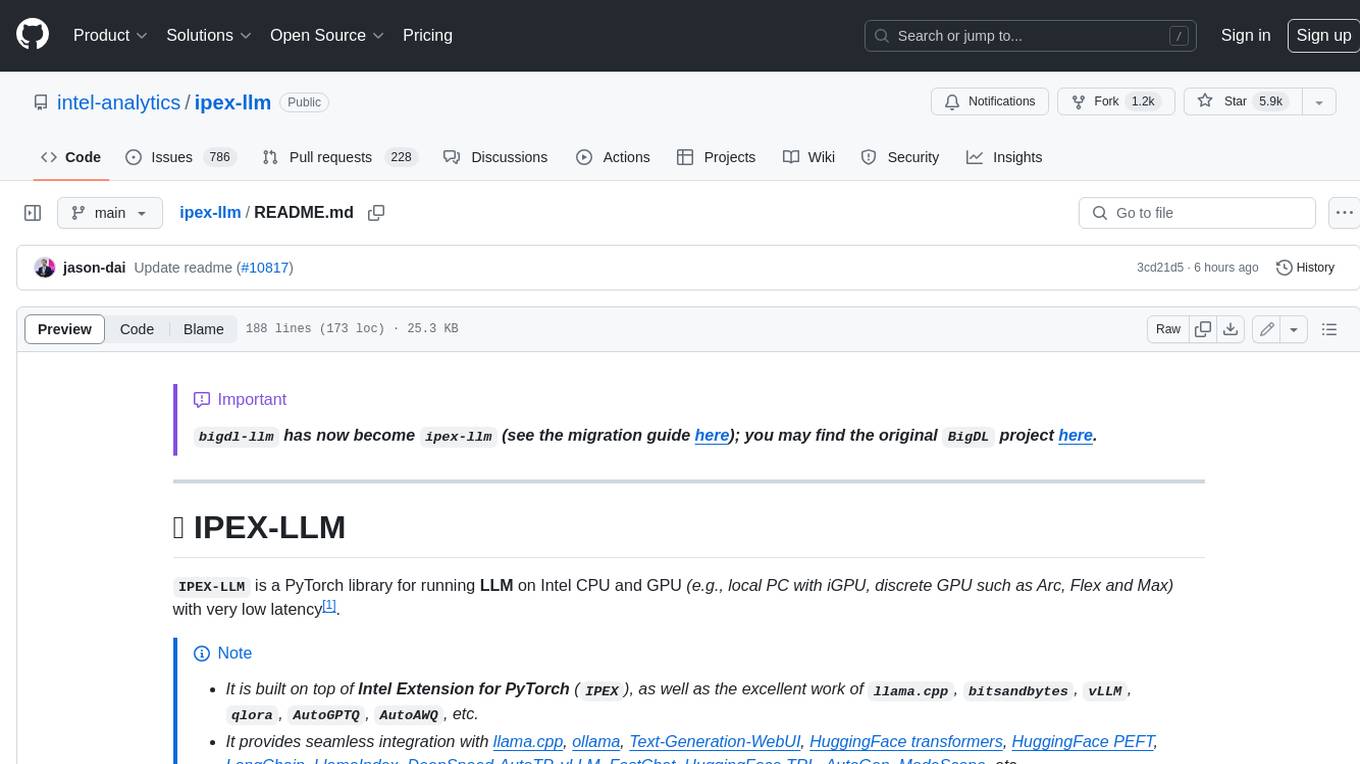
ipex-llm
IPEX-LLM is a PyTorch library for running Large Language Models (LLMs) on Intel CPUs and GPUs with very low latency. It provides seamless integration with various LLM frameworks and tools, including llama.cpp, ollama, Text-Generation-WebUI, HuggingFace transformers, and more. IPEX-LLM has been optimized and verified on over 50 LLM models, including LLaMA, Mistral, Mixtral, Gemma, LLaVA, Whisper, ChatGLM, Baichuan, Qwen, and RWKV. It supports a range of low-bit inference formats, including INT4, FP8, FP4, INT8, INT2, FP16, and BF16, as well as finetuning capabilities for LoRA, QLoRA, DPO, QA-LoRA, and ReLoRA. IPEX-LLM is actively maintained and updated with new features and optimizations, making it a valuable tool for researchers, developers, and anyone interested in exploring and utilizing LLMs.
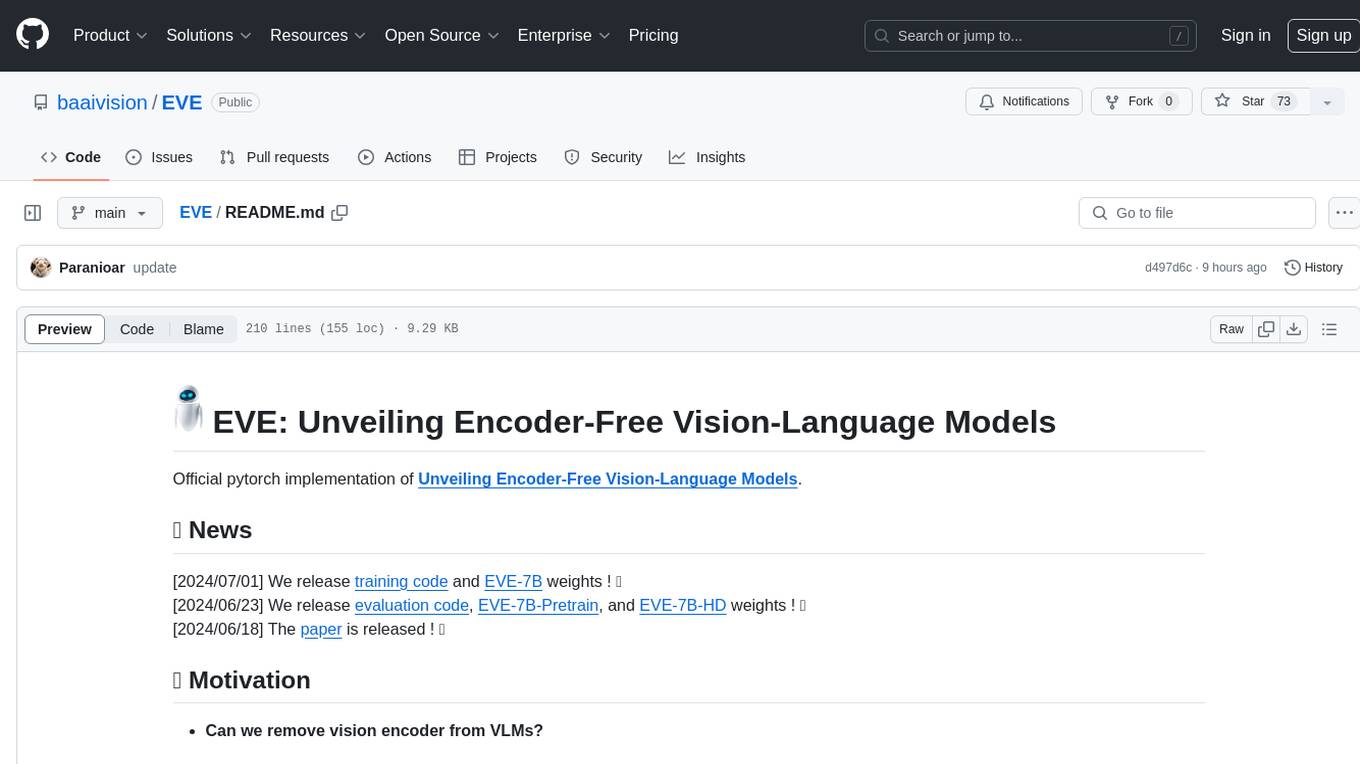
EVE
EVE is an official PyTorch implementation of Unveiling Encoder-Free Vision-Language Models. The project aims to explore the removal of vision encoders from Vision-Language Models (VLMs) and transfer LLMs to encoder-free VLMs efficiently. It also focuses on bridging the performance gap between encoder-free and encoder-based VLMs. EVE offers a superior capability with arbitrary image aspect ratio, data efficiency by utilizing publicly available data for pre-training, and training efficiency with a transparent and practical strategy for developing a pure decoder-only architecture across modalities.
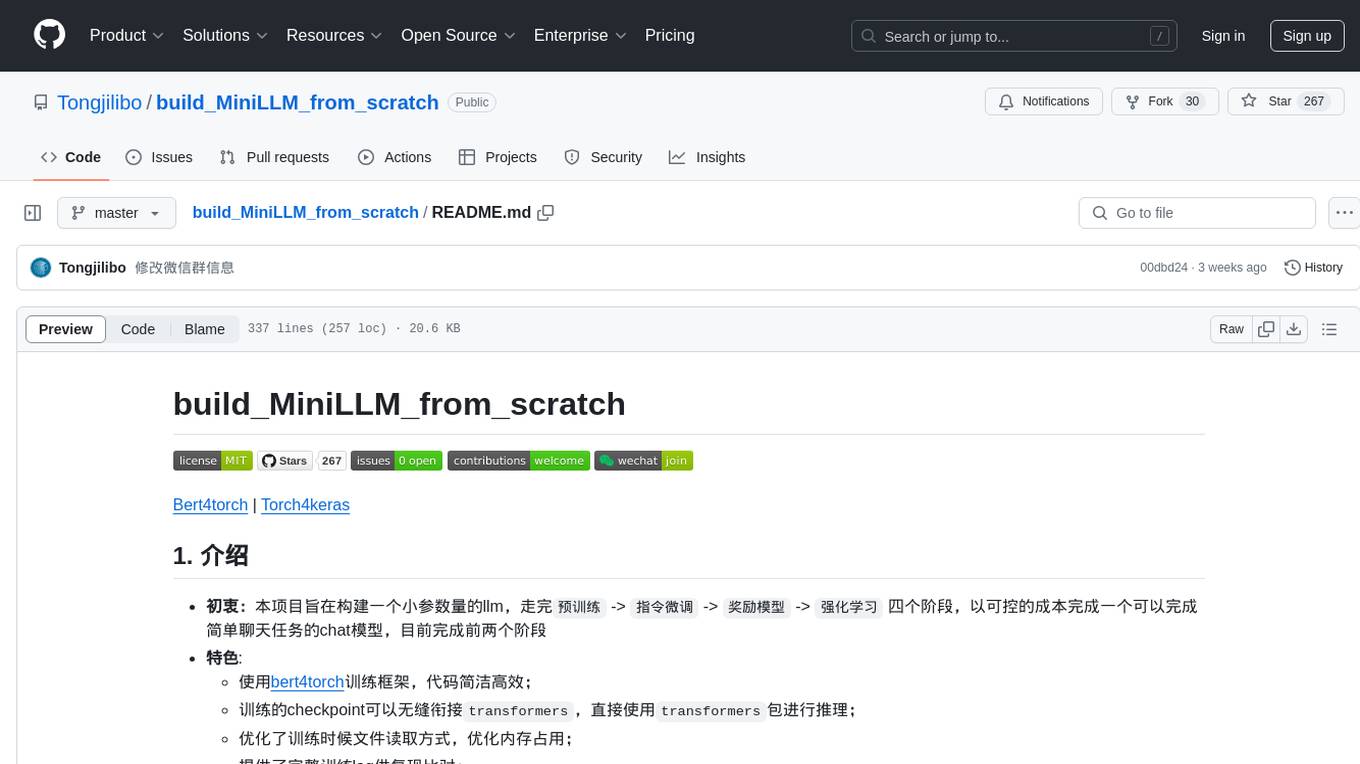
build_MiniLLM_from_scratch
This repository aims to build a low-parameter LLM model through pretraining, fine-tuning, model rewarding, and reinforcement learning stages to create a chat model capable of simple conversation tasks. It features using the bert4torch training framework, seamless integration with transformers package for inference, optimized file reading during training to reduce memory usage, providing complete training logs for reproducibility, and the ability to customize robot attributes. The chat model supports multi-turn conversations. The trained model currently only supports basic chat functionality due to limitations in corpus size, model scale, SFT corpus size, and quality.
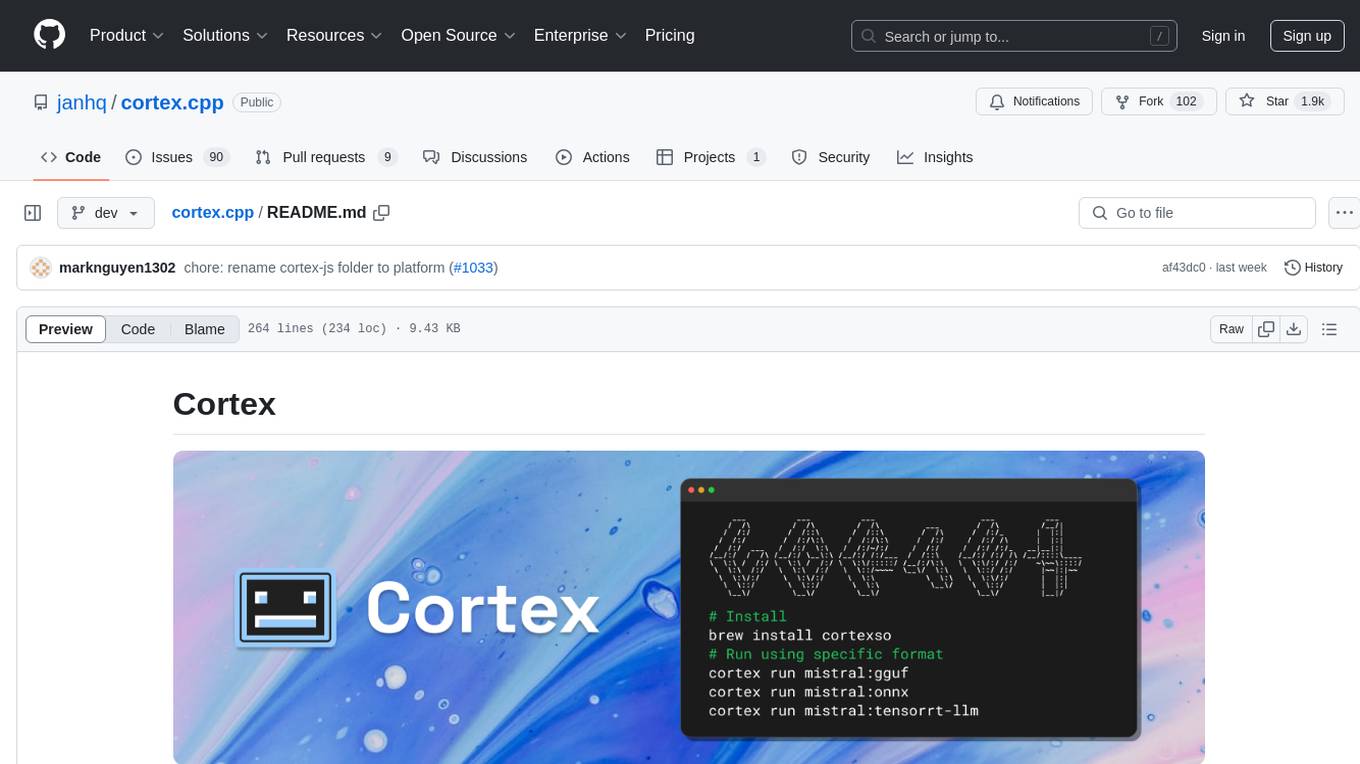
cortex.cpp
Cortex is a C++ AI engine with a Docker-like command-line interface and client libraries. It supports running AI models using ONNX, TensorRT-LLM, and llama.cpp engines. Cortex can function as a standalone server or be integrated as a library. The tool provides support for various engines and models, allowing users to easily deploy and interact with AI models. It offers a range of CLI commands for managing models, embeddings, and engines, as well as a REST API for interacting with models. Cortex is designed to simplify the deployment and usage of AI models in C++ applications.
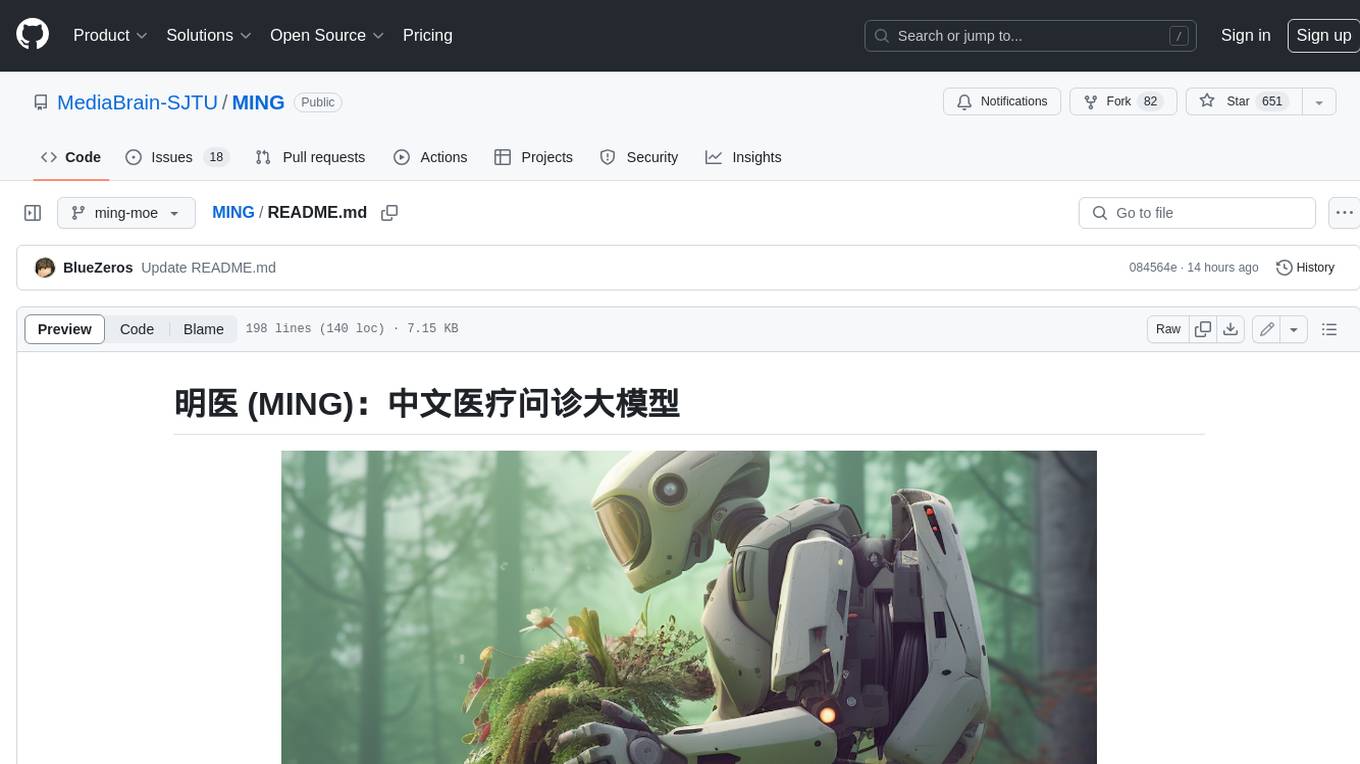
MING
MING is an open-sourced Chinese medical consultation model fine-tuned based on medical instructions. The main functions of the model are as follows: Medical Q&A: answering medical questions and analyzing cases. Intelligent consultation: giving diagnosis results and suggestions after multiple rounds of consultation.
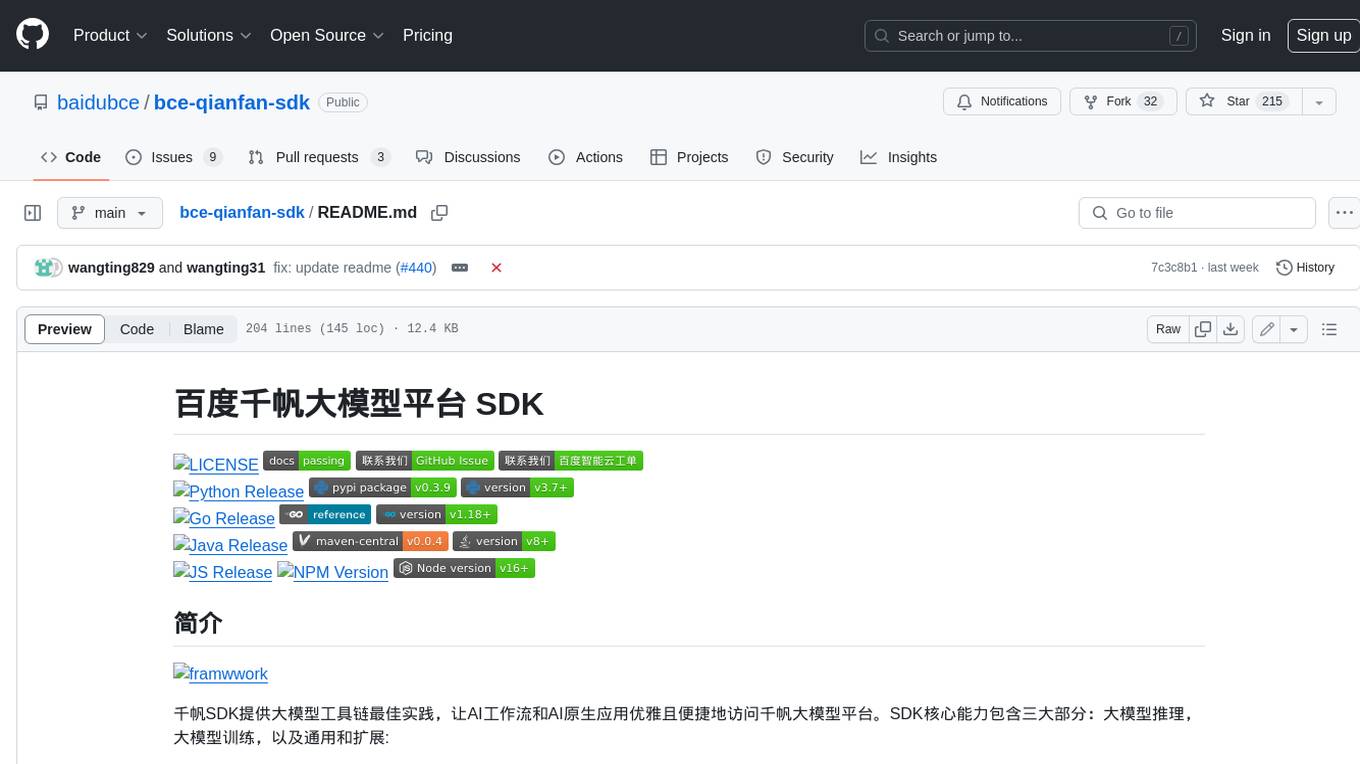
bce-qianfan-sdk
The Qianfan SDK provides best practices for large model toolchains, allowing AI workflows and AI-native applications to access the Qianfan large model platform elegantly and conveniently. The core capabilities of the SDK include three parts: large model reasoning, large model training, and general and extension: * `Large model reasoning`: Implements interface encapsulation for reasoning of Yuyan (ERNIE-Bot) series, open source large models, etc., supporting dialogue, completion, Embedding, etc. * `Large model training`: Based on platform capabilities, it supports end-to-end large model training process, including training data, fine-tuning/pre-training, and model services. * `General and extension`: General capabilities include common AI development tools such as Prompt/Debug/Client. The extension capability is based on the characteristics of Qianfan to adapt to common middleware frameworks.
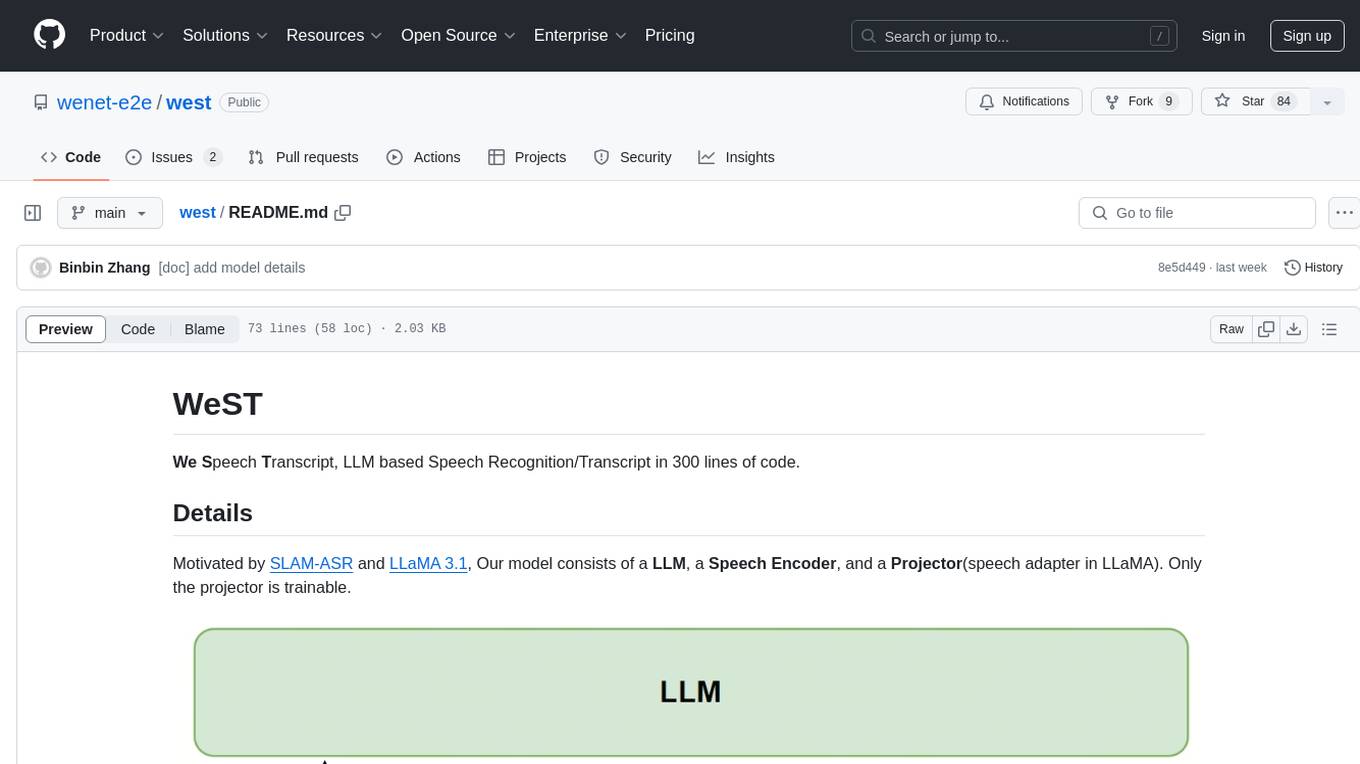
west
WeST is a Speech Recognition/Transcript tool developed in 300 lines of code, inspired by SLAM-ASR and LLaMA 3.1. The model includes a Language Model (LLM), a Speech Encoder, and a trainable Projector. It requires training data in jsonl format with 'wav' and 'txt' entries. WeST can be used for training and decoding speech recognition models.
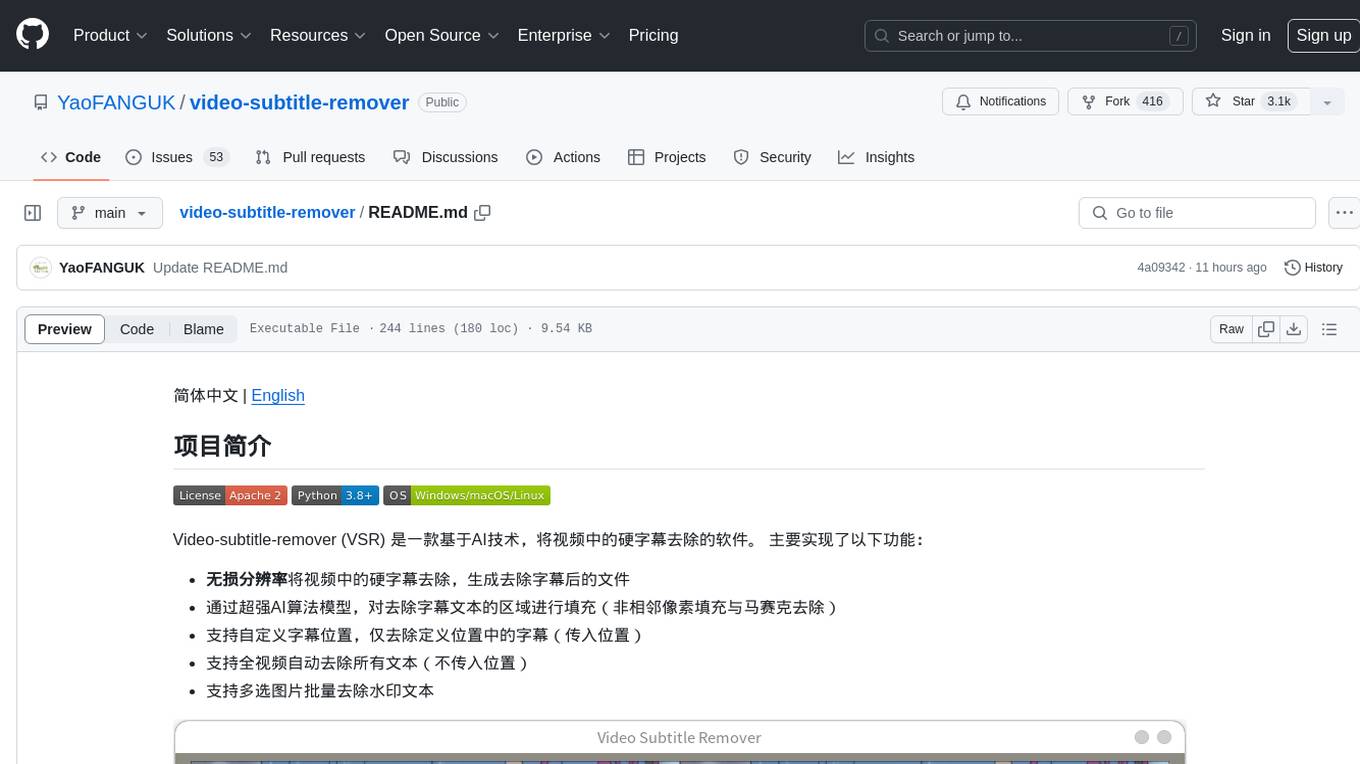
video-subtitle-remover
Video-subtitle-remover (VSR) is a software based on AI technology that removes hard subtitles from videos. It achieves the following functions: - Lossless resolution: Remove hard subtitles from videos, generate files with subtitles removed - Fill the region of removed subtitles using a powerful AI algorithm model (non-adjacent pixel filling and mosaic removal) - Support custom subtitle positions, only remove subtitles in defined positions (input position) - Support automatic removal of all text in the entire video (no input position required) - Support batch removal of watermark text from multiple images.
For similar tasks
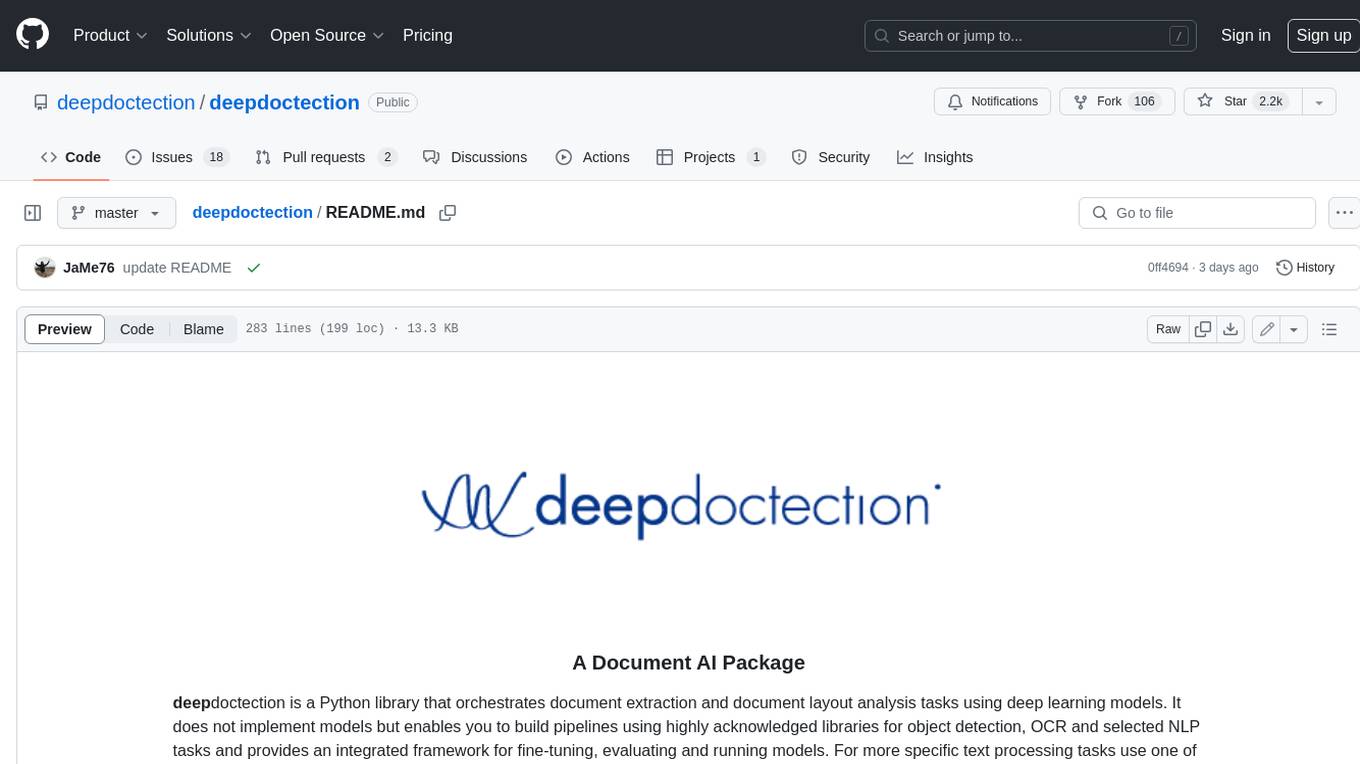
deepdoctection
**deep** doctection is a Python library that orchestrates document extraction and document layout analysis tasks using deep learning models. It does not implement models but enables you to build pipelines using highly acknowledged libraries for object detection, OCR and selected NLP tasks and provides an integrated framework for fine-tuning, evaluating and running models. For more specific text processing tasks use one of the many other great NLP libraries. **deep** doctection focuses on applications and is made for those who want to solve real world problems related to document extraction from PDFs or scans in various image formats. **deep** doctection provides model wrappers of supported libraries for various tasks to be integrated into pipelines. Its core function does not depend on any specific deep learning library. Selected models for the following tasks are currently supported: * Document layout analysis including table recognition in Tensorflow with **Tensorpack**, or PyTorch with **Detectron2**, * OCR with support of **Tesseract**, **DocTr** (Tensorflow and PyTorch implementations available) and a wrapper to an API for a commercial solution, * Text mining for native PDFs with **pdfplumber**, * Language detection with **fastText**, * Deskewing and rotating images with **jdeskew**. * Document and token classification with all LayoutLM models provided by the **Transformer library**. (Yes, you can use any LayoutLM-model with any of the provided OCR-or pdfplumber tools straight away!). * Table detection and table structure recognition with **table-transformer**. * There is a small dataset for token classification available and a lot of new tutorials to show, how to train and evaluate this dataset using LayoutLMv1, LayoutLMv2, LayoutXLM and LayoutLMv3. * Comprehensive configuration of **analyzer** like choosing different models, output parsing, OCR selection. Check this notebook or the docs for more infos. * Document layout analysis and table recognition now runs with **Torchscript** (CPU) as well and **Detectron2** is not required anymore for basic inference. * [**new**] More angle predictors for determining the rotation of a document based on **Tesseract** and **DocTr** (not contained in the built-in Analyzer). * [**new**] Token classification with **LiLT** via **transformers**. We have added a model wrapper for token classification with LiLT and added a some LiLT models to the model catalog that seem to look promising, especially if you want to train a model on non-english data. The training script for LayoutLM can be used for LiLT as well and we will be providing a notebook on how to train a model on a custom dataset soon. **deep** doctection provides on top of that methods for pre-processing inputs to models like cropping or resizing and to post-process results, like validating duplicate outputs, relating words to detected layout segments or ordering words into contiguous text. You will get an output in JSON format that you can customize even further by yourself. Have a look at the **introduction notebook** in the notebook repo for an easy start. Check the **release notes** for recent updates. **deep** doctection or its support libraries provide pre-trained models that are in most of the cases available at the **Hugging Face Model Hub** or that will be automatically downloaded once requested. For instance, you can find pre-trained object detection models from the Tensorpack or Detectron2 framework for coarse layout analysis, table cell detection and table recognition. Training is a substantial part to get pipelines ready on some specific domain, let it be document layout analysis, document classification or NER. **deep** doctection provides training scripts for models that are based on trainers developed from the library that hosts the model code. Moreover, **deep** doctection hosts code to some well established datasets like **Publaynet** that makes it easy to experiment. It also contains mappings from widely used data formats like COCO and it has a dataset framework (akin to **datasets** so that setting up training on a custom dataset becomes very easy. **This notebook** shows you how to do this. **deep** doctection comes equipped with a framework that allows you to evaluate predictions of a single or multiple models in a pipeline against some ground truth. Check again **here** how it is done. Having set up a pipeline it takes you a few lines of code to instantiate the pipeline and after a for loop all pages will be processed through the pipeline.
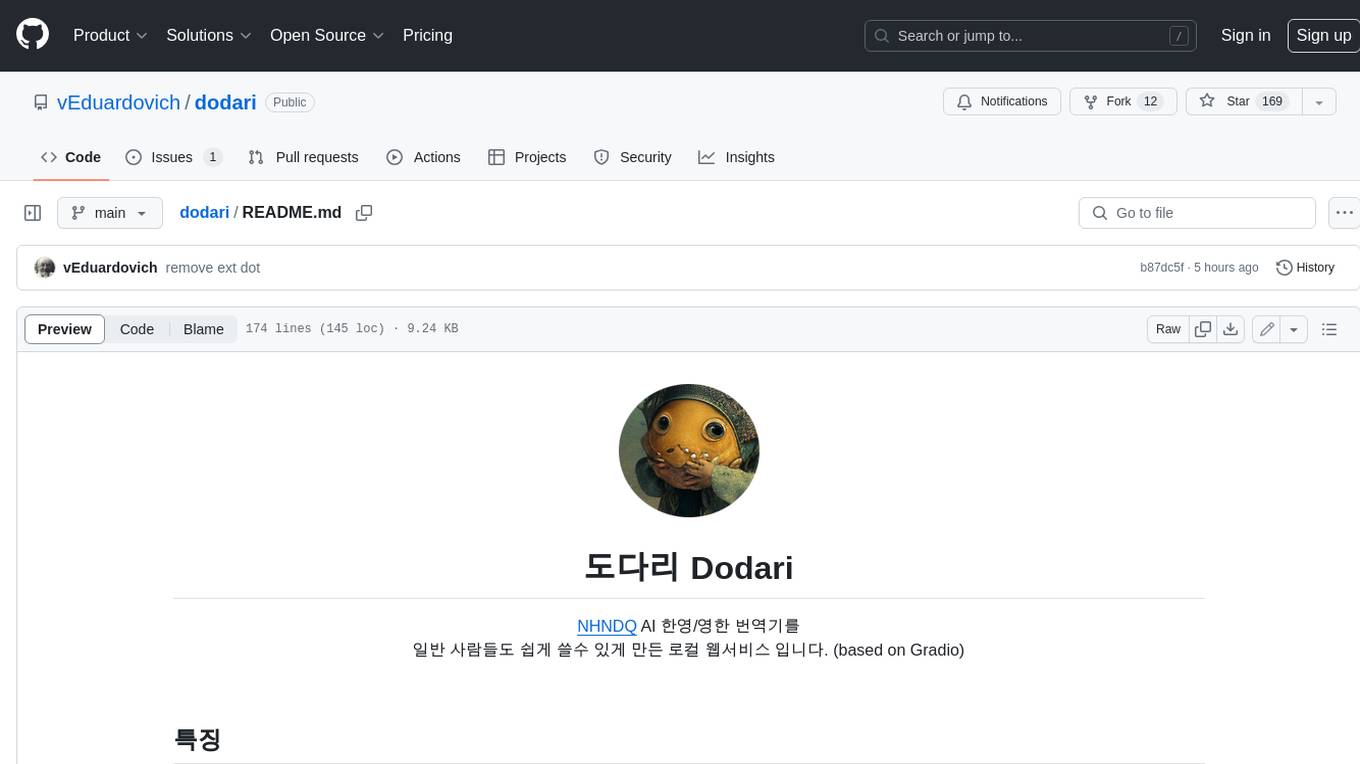
dodari
Dodari is a local web service that makes NHNDQ AI Korean-English/English-Korean translation easy for everyday people to use (based on Gradio).
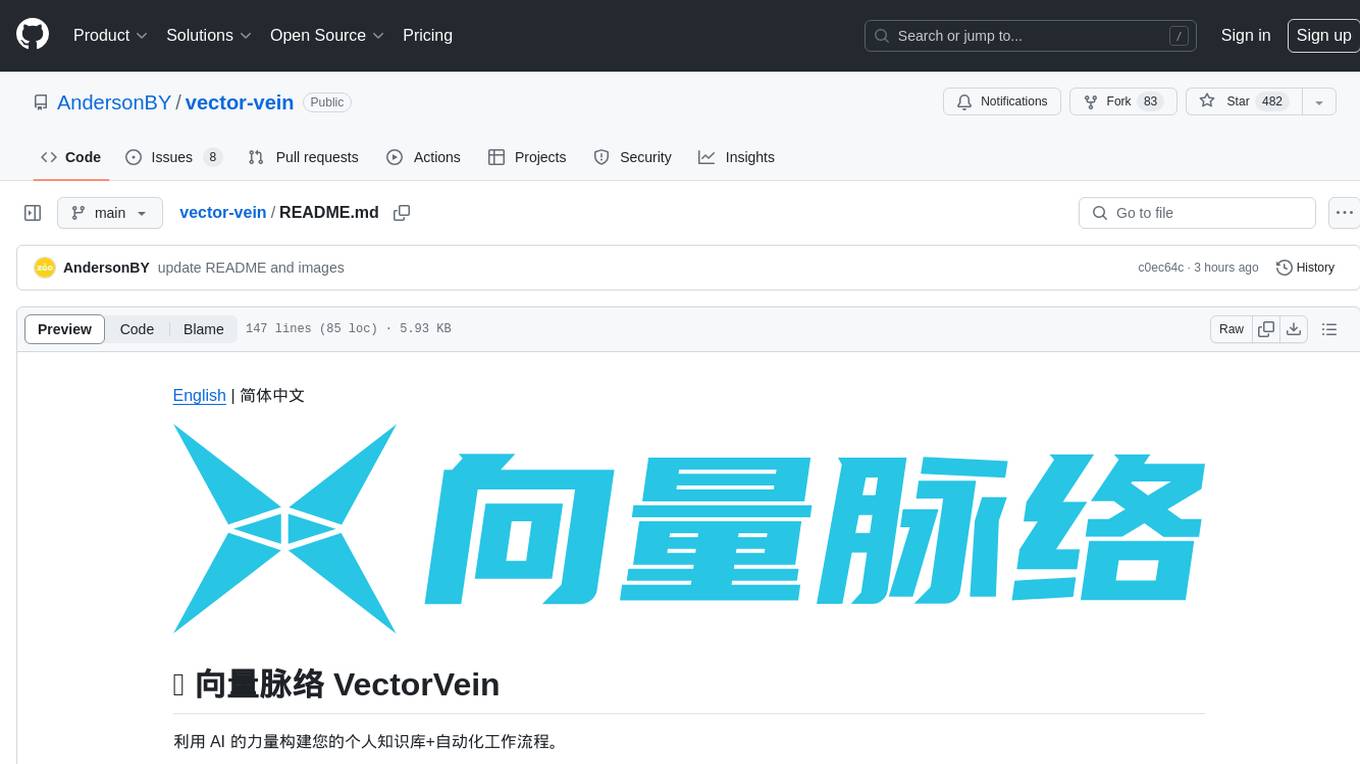
vector-vein
VectorVein is a no-code AI workflow software inspired by LangChain and langflow, aiming to combine the powerful capabilities of large language models and enable users to achieve intelligent and automated daily workflows through simple drag-and-drop actions. Users can create powerful workflows without the need for programming, automating all tasks with ease. The software allows users to define inputs, outputs, and processing methods to create customized workflow processes for various tasks such as translation, mind mapping, summarizing web articles, and automatic categorization of customer reviews.
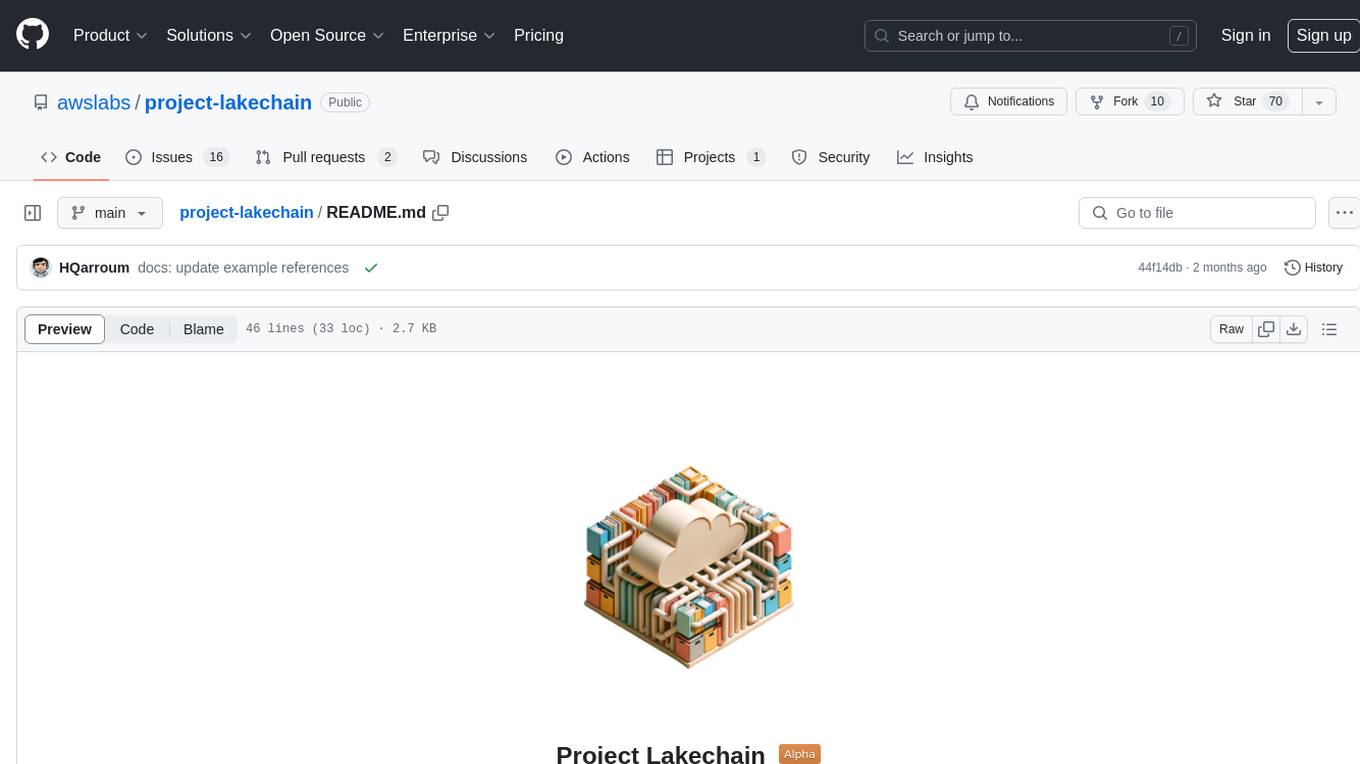
project-lakechain
Project Lakechain is a cloud-native, AI-powered framework for building document processing pipelines on AWS. It provides a composable API with built-in middlewares for common tasks, scalable architecture, cost efficiency, GPU and CPU support, and the ability to create custom transform middlewares. With ready-made examples and emphasis on modularity, Lakechain simplifies the deployment of scalable document pipelines for tasks like metadata extraction, NLP analysis, text summarization, translations, audio transcriptions, computer vision, and more.
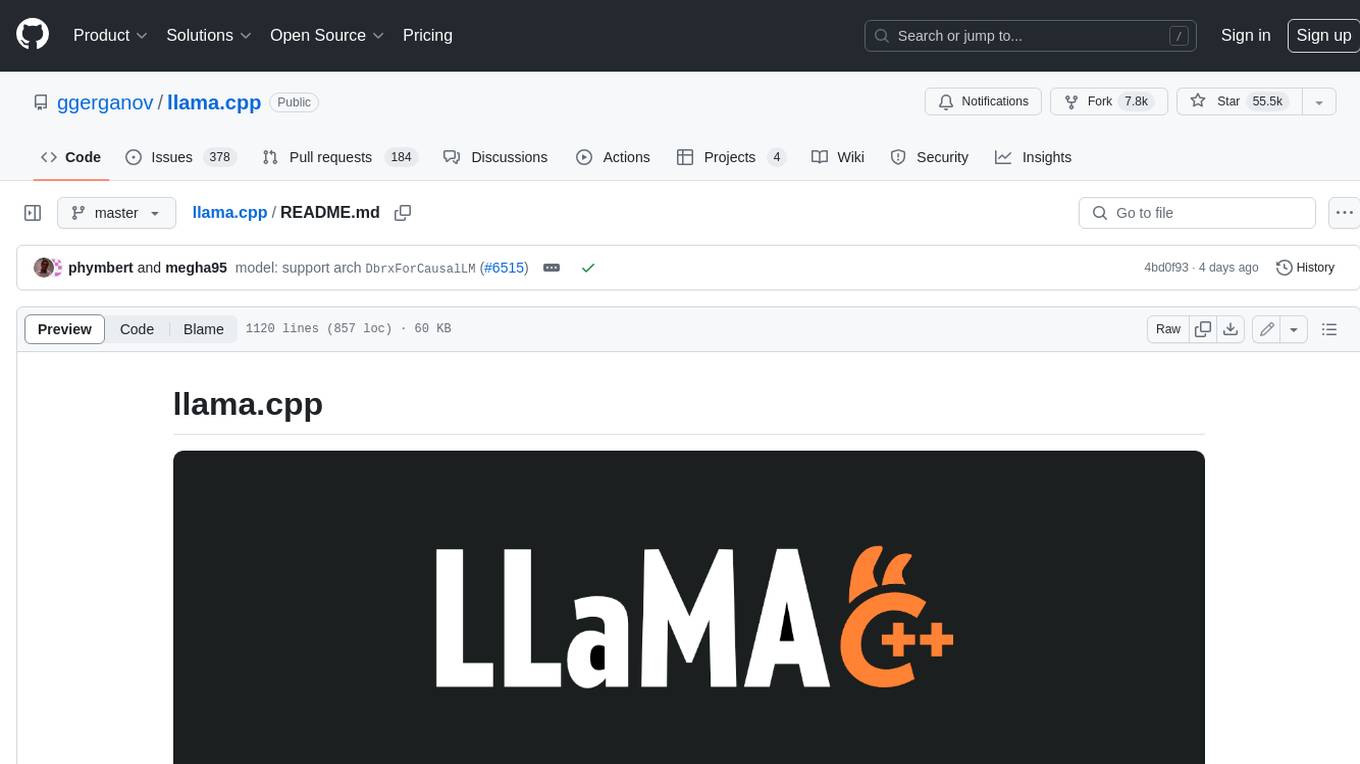
llama.cpp
llama.cpp is a C++ implementation of LLaMA, a large language model from Meta. It provides a command-line interface for inference and can be used for a variety of tasks, including text generation, translation, and question answering. llama.cpp is highly optimized for performance and can be run on a variety of hardware, including CPUs, GPUs, and TPUs.
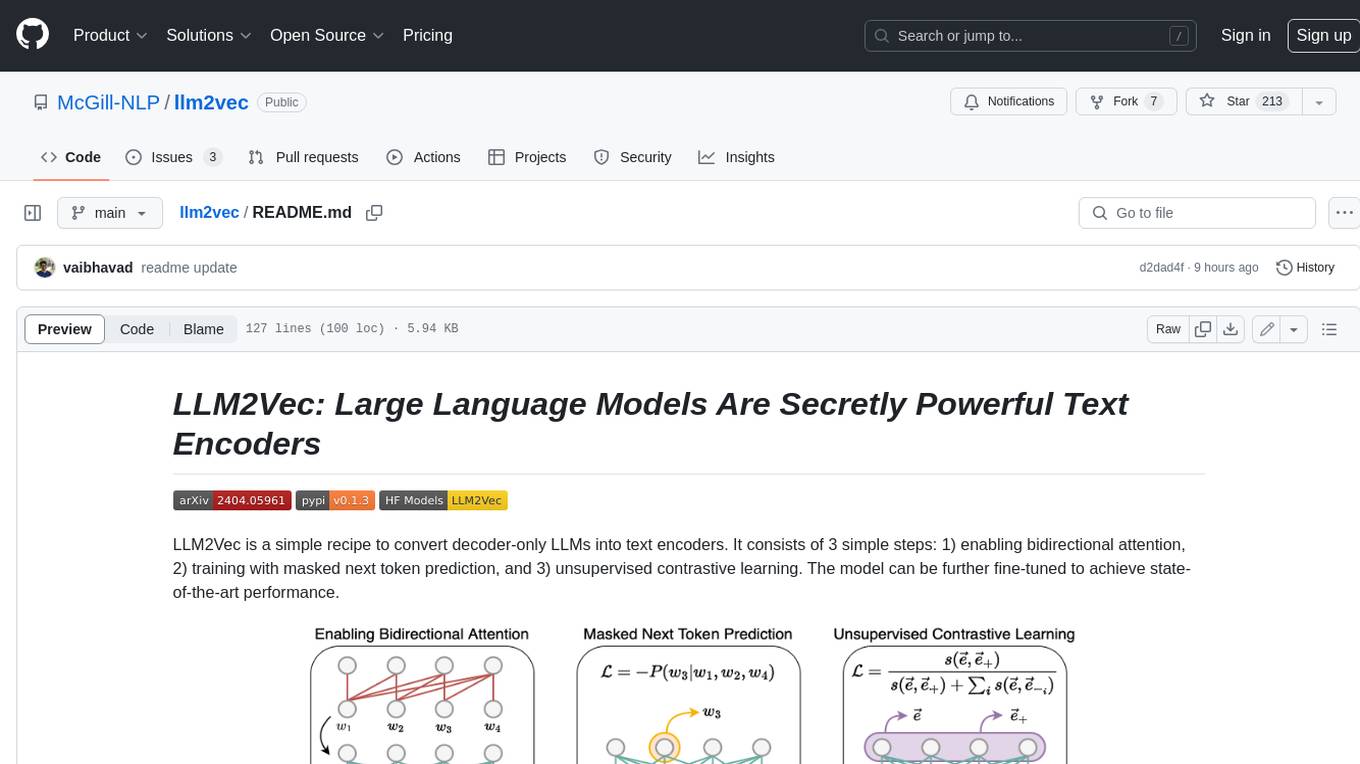
llm2vec
LLM2Vec is a simple recipe to convert decoder-only LLMs into text encoders. It consists of 3 simple steps: 1) enabling bidirectional attention, 2) training with masked next token prediction, and 3) unsupervised contrastive learning. The model can be further fine-tuned to achieve state-of-the-art performance.
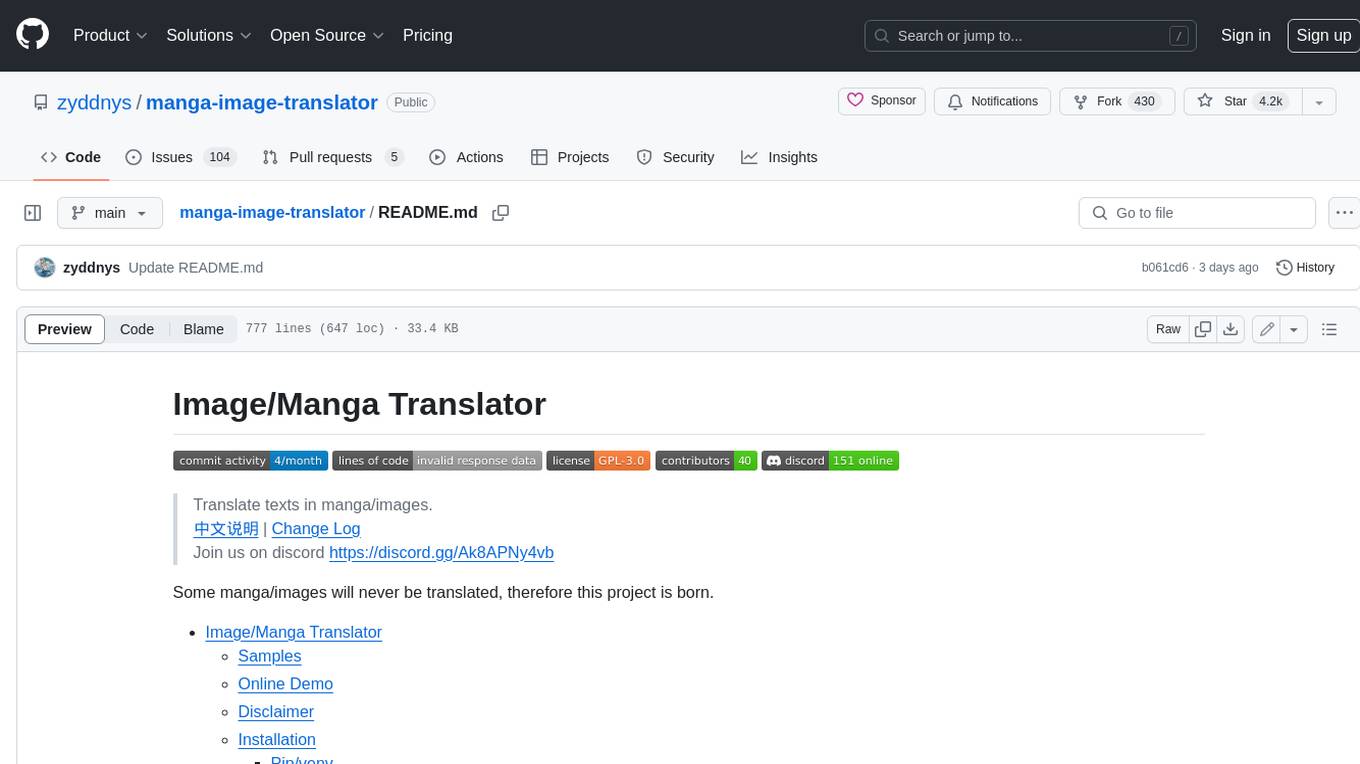
manga-image-translator
Translate texts in manga/images. Some manga/images will never be translated, therefore this project is born. * Image/Manga Translator * Samples * Online Demo * Disclaimer * Installation * Pip/venv * Poetry * Additional instructions for **Windows** * Docker * Hosting the web server * Using as CLI * Setting Translation Secrets * Using with Nvidia GPU * Building locally * Usage * Batch mode (default) * Demo mode * Web Mode * Api Mode * Related Projects * Docs * Recommended Modules * Tips to improve translation quality * Options * Language Code Reference * Translators Reference * GPT Config Reference * Using Gimp for rendering * Api Documentation * Synchronous mode * Asynchronous mode * Manual translation * Next steps * Support Us * Thanks To All Our Contributors :
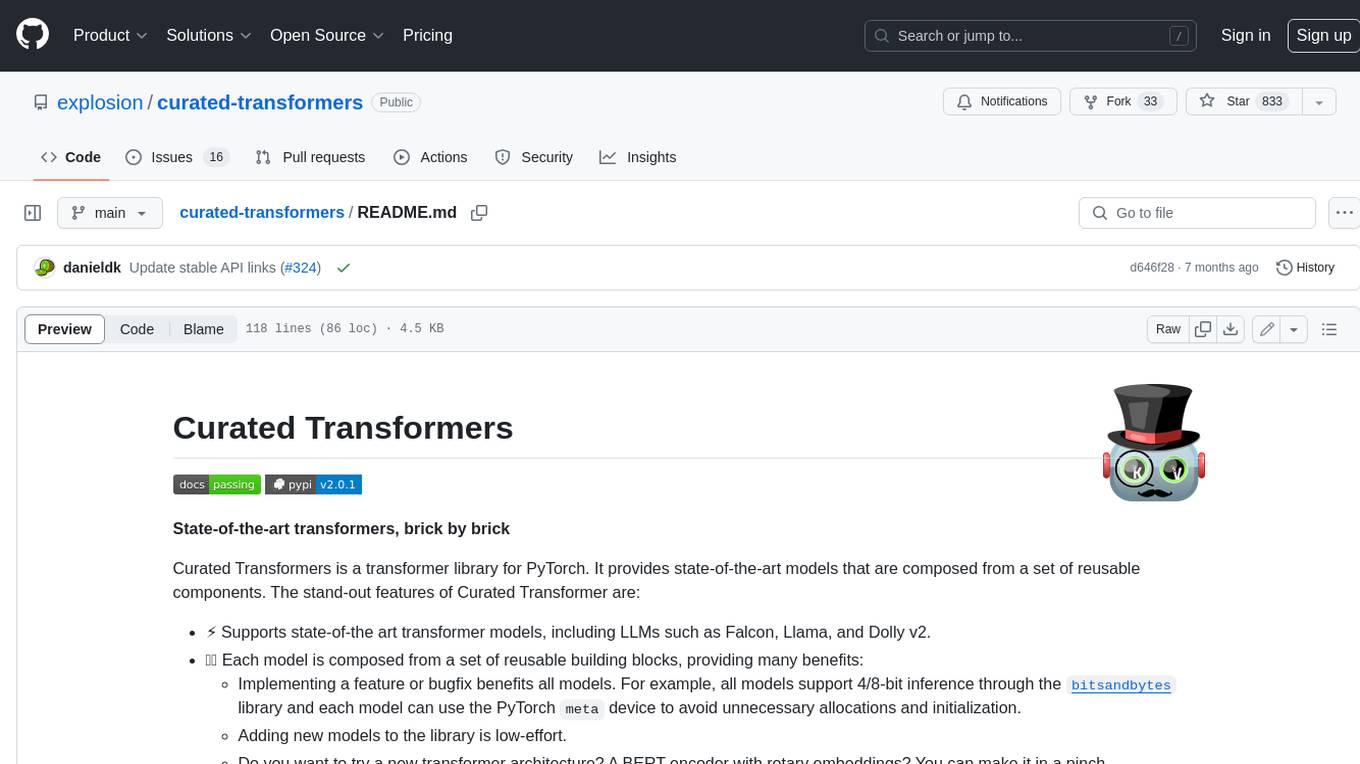
curated-transformers
Curated Transformers is a transformer library for PyTorch that provides state-of-the-art models composed of reusable components. It supports various transformer architectures, including encoders like ALBERT, BERT, and RoBERTa, and decoders like Falcon, Llama, and MPT. The library emphasizes consistent type annotations, minimal dependencies, and ease of use for education and research. It has been production-tested by Explosion and will be the default transformer implementation in spaCy 3.7.
For similar jobs
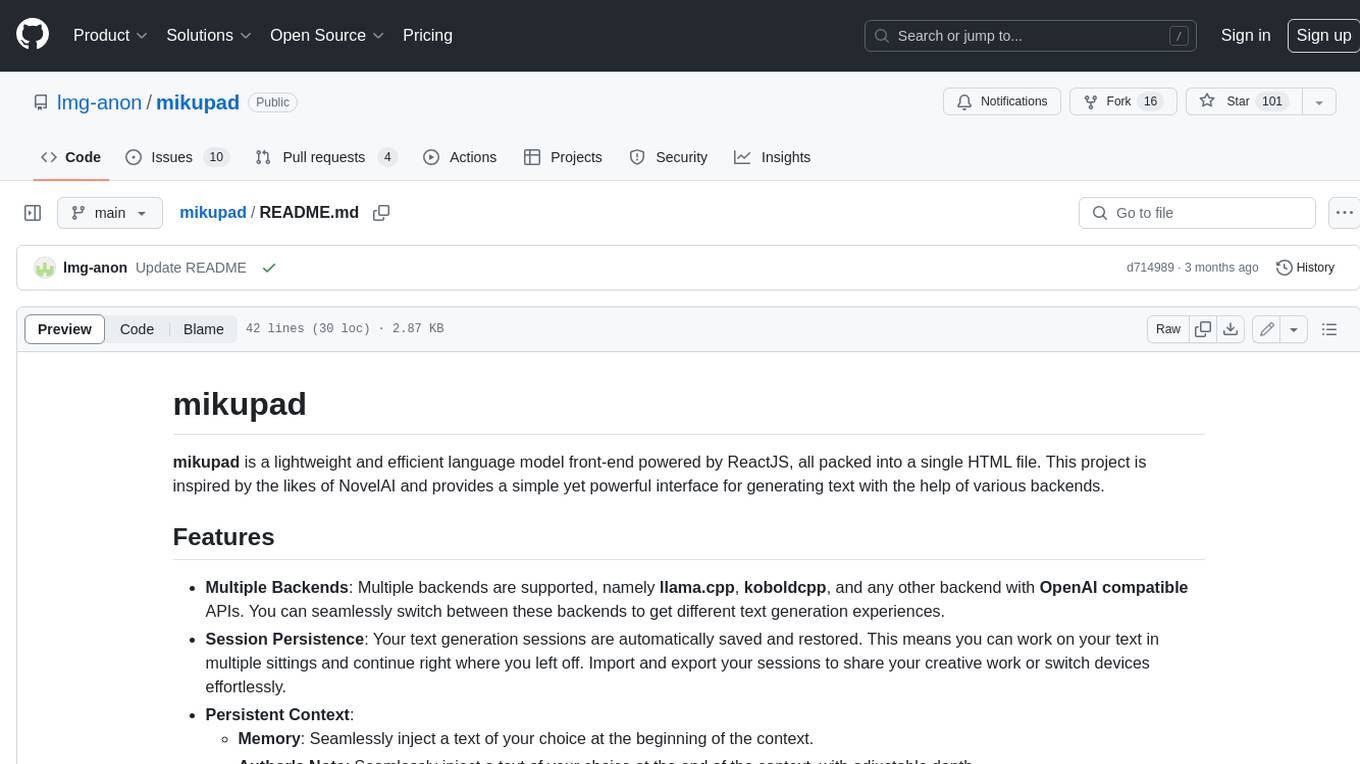
mikupad
mikupad is a lightweight and efficient language model front-end powered by ReactJS, all packed into a single HTML file. Inspired by the likes of NovelAI, it provides a simple yet powerful interface for generating text with the help of various backends.
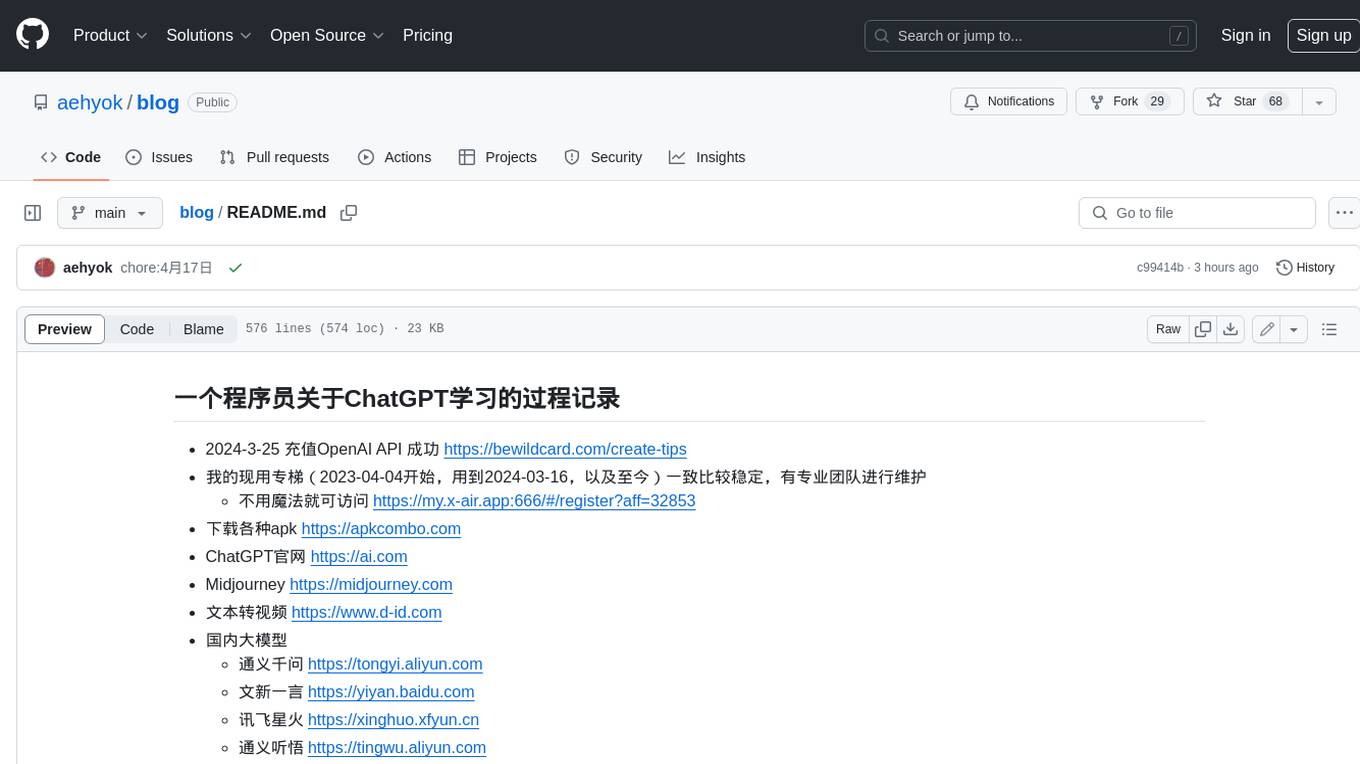
blog
这是一个程序员关于 ChatGPT 学习过程的记录,其中包括了 ChatGPT 的使用技巧、相关工具和资源的整理,以及一些个人见解和思考。 **使用技巧** * **充值 OpenAI API**:可以通过 https://beta.openai.com/account/api-keys 进行充值,支持信用卡和 PayPal。 * **使用专梯**:推荐使用稳定的专梯,可以有效提高 ChatGPT 的访问速度和稳定性。 * **使用魔法**:可以通过 https://my.x-air.app:666/#/register?aff=32853 访问 ChatGPT,无需魔法即可访问。 * **下载各种 apk**:可以通过 https://apkcombo.com 下载各种安卓应用的 apk 文件。 * **ChatGPT 官网**:ChatGPT 的官方网站是 https://ai.com。 * **Midjourney**:Midjourney 是一个生成式 AI 图像平台,可以通过 https://midjourney.com 访问。 * **文本转视频**:可以通过 https://www.d-id.com 将文本转换为视频。 * **国内大模型**:国内也有很多大模型,如阿里巴巴的通义千问、百度文心一言、讯飞星火、阿里巴巴通义听悟等。 * **查看 OpenAI 状态**:可以通过 https://status.openai.com/ 查看 OpenAI 的服务状态。 * **Canva 画图**:Canva 是一个在线平面设计平台,可以通过 https://www.canva.cn 进行画图。 **相关工具和资源** * **文字转语音**:可以通过 https://modelscope.cn/models?page=1&tasks=text-to-speech&type=audio 找到文字转语音的模型。 * **可好好玩玩的项目**: * https://github.com/sunner/ChatALL * https://github.com/labring/FastGPT * https://github.com/songquanpeng/one-api * **个人博客**: * https://baoyu.io/ * https://gorden-sun.notion.site/527689cd2b294e60912f040095e803c5?v=4f6cc12006c94f47aee4dc909511aeb5 * **srt 2 lrc 歌词**:可以通过 https://gotranscript.com/subtitle-converter 将 srt 格式的字幕转换为 lrc 格式的歌词。 * **5 种速率限制**:OpenAI API 有 5 种速率限制:RPM(每分钟请求数)、RPD(每天请求数)、TPM(每分钟 tokens 数量)、TPD(每天 tokens 数量)、IPM(每分钟图像数量)。 * **扣子平台**:coze.cn 是一个扣子平台,可以提供各种扣子。 * **通过云函数免费使用 GPT-3.5**:可以通过 https://juejin.cn/post/7353849549540589587 免费使用 GPT-3.5。 * **不蒜子 统计网页基数**:可以通过 https://busuanzi.ibruce.info/ 统计网页的基数。 * **视频总结和翻译网页**:可以通过 https://glarity.app/zh-CN 总结和翻译视频。 * **视频翻译和配音工具**:可以通过 https://github.com/jianchang512/pyvideotrans 翻译和配音视频。 * **文字生成音频**:可以通过 https://www.cnblogs.com/jijunjian/p/18118366 将文字生成音频。 * **memo ai**:memo.ac 是一个多模态 AI 平台,可以将视频链接、播客链接、本地音视频转换为文字,支持多语言转录后翻译,还可以将文字转换为新的音频。 * **视频总结工具**:可以通过 https://summarize.ing/ 总结视频。 * **可每天免费玩玩**:可以通过 https://www.perplexity.ai/ 每天免费玩玩。 * **Suno.ai**:Suno.ai 是一个 AI 语言模型,可以通过 https://bibigpt.co/ 访问。 * **CapCut**:CapCut 是一个视频编辑软件,可以通过 https://www.capcut.cn/ 下载。 * **Valla.ai**:Valla.ai 是一个多模态 AI 模型,可以通过 https://www.valla.ai/ 访问。 * **Viggle.ai**:Viggle.ai 是一个 AI 视频生成平台,可以通过 https://viggle.ai 访问。 * **使用免费的 GPU 部署文生图大模型**:可以通过 https://www.cnblogs.com/xuxiaona/p/18088404 部署文生图大模型。 * **语音转文字**:可以通过 https://speech.microsoft.com/portal 将语音转换为文字。 * **投资界的 ai**:可以通过 https://reportify.cc/ 了解投资界的 ai。 * **抓取小视频 app 的各种信息**:可以通过 https://github.com/NanmiCoder/MediaCrawler 抓取小视频 app 的各种信息。 * **马斯克 Grok1 开源**:马斯克的 Grok1 模型已经开源,可以通过 https://github.com/xai-org/grok-1 访问。 * **ChatALL**:ChatALL 是一个跨端支持的聊天机器人,可以通过 https://github.com/sunner/ChatALL 访问。 * **零一万物**:零一万物是一个 AI 平台,可以通过 https://www.01.ai/cn 访问。 * **智普**:智普是一个 AI 语言模型,可以通过 https://chatglm.cn/ 访问。 * **memo ai 下载**:可以通过 https://memo.ac/ 下载 memo ai。 * **ffmpeg 学习**:可以通过 https://www.ruanyifeng.com/blog/2020/01/ffmpeg.html 学习 ffmpeg。 * **自动生成文章小工具**:可以通过 https://www.cognition-labs.com/blog 生成文章。 * **简易商城**:可以通过 https://www.cnblogs.com/whuanle/p/18086537 搭建简易商城。 * **物联网**:可以通过 https://www.cnblogs.com/xuxiaona/p/18088404 学习物联网。 * **自定义表单、自定义列表、自定义上传和下载、自定义流程、自定义报表**:可以通过 https://www.cnblogs.com/whuanle/p/18086537 实现自定义表单、自定义列表、自定义上传和下载、自定义流程、自定义报表。 **个人见解和思考** * ChatGPT 是一个强大的工具,可以用来提高工作效率和创造力。 * ChatGPT 的使用门槛较低,即使是非技术人员也可以轻松上手。 * ChatGPT 的发展速度非常快,未来可能会对各个行业产生深远的影响。 * 我们应该理性看待 ChatGPT,既要看到它的优点,也要意识到它的局限性。 * 我们应该积极探索 ChatGPT 的应用场景,为社会创造价值。
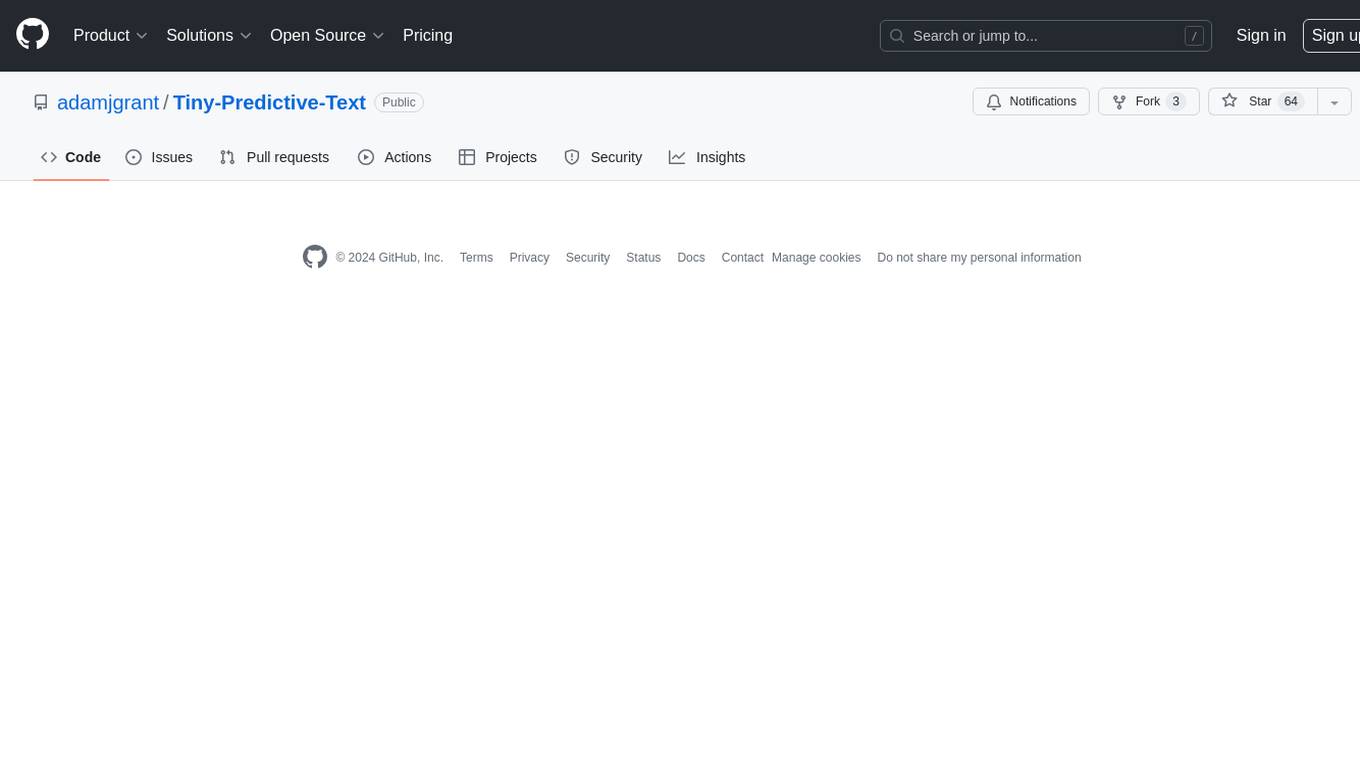
Tiny-Predictive-Text
Tiny-Predictive-Text is a demonstration of predictive text without an LLM, using permy.link. It provides a detailed description of the tool, including its features, benefits, and how to use it. The tool is suitable for a variety of jobs, including content writers, editors, and researchers. It can be used to perform a variety of tasks, such as generating text, completing sentences, and correcting errors.
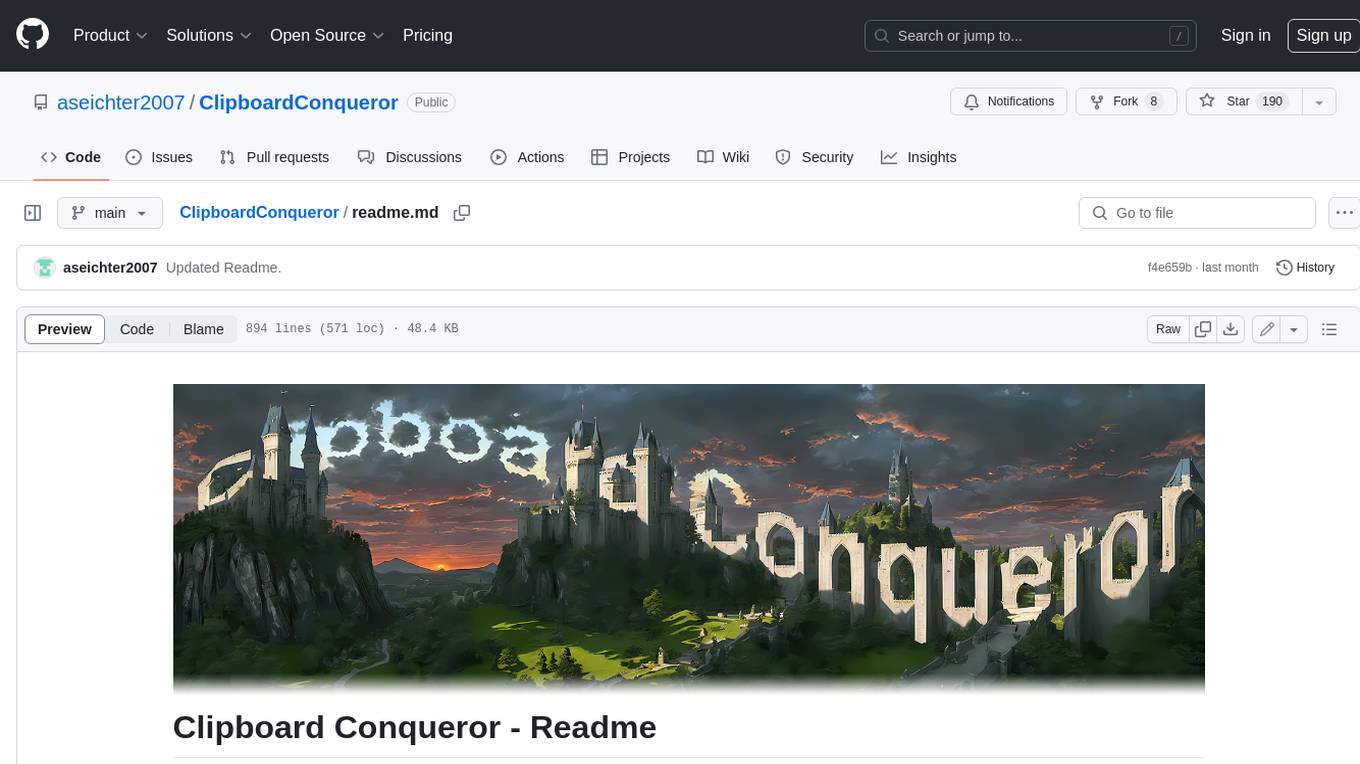
ClipboardConqueror
Clipboard Conqueror is a multi-platform omnipresent copilot alternative. Currently requiring a kobold united or openAI compatible back end, this software brings powerful LLM based tools to any text field, the universal copilot you deserve. It simply works anywhere. No need to sign in, no required key. Provided you are using local AI, CC is a data secure alternative integration provided you trust whatever backend you use. *Special thank you to the creators of KoboldAi, KoboldCPP, llamma, openAi, and the communities that made all this possible to figure out.
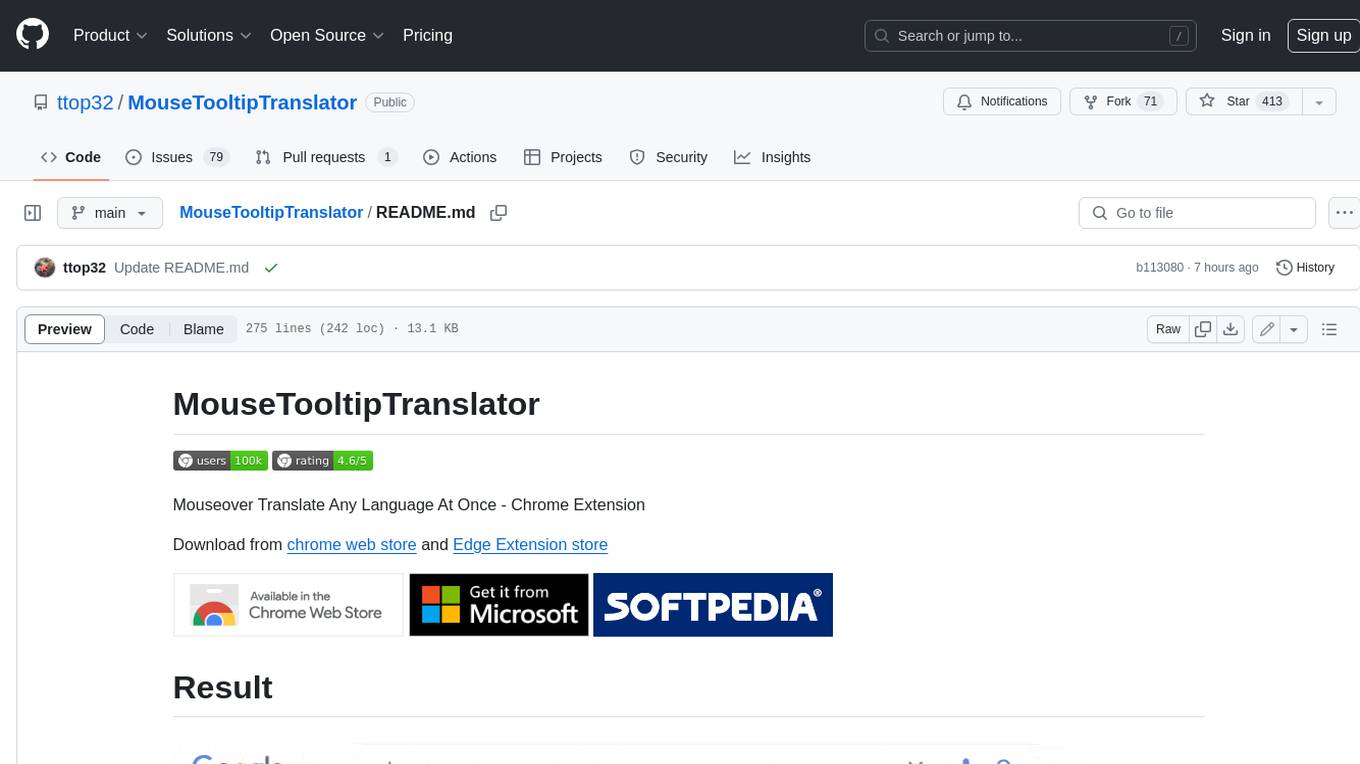
MouseTooltipTranslator
MouseTooltipTranslator is a Chrome extension that allows users to translate any text on a webpage by simply hovering over it. It supports both Google Translate and Bing Translate, and can also be used to listen to the pronunciation of words and phrases. Additionally, the extension can be used to translate text in input boxes and highlighted text, and to display translated tooltips for PDFs and YouTube videos. It also supports OCR, allowing users to translate text in images by holding down the left shift key and hovering over the image.
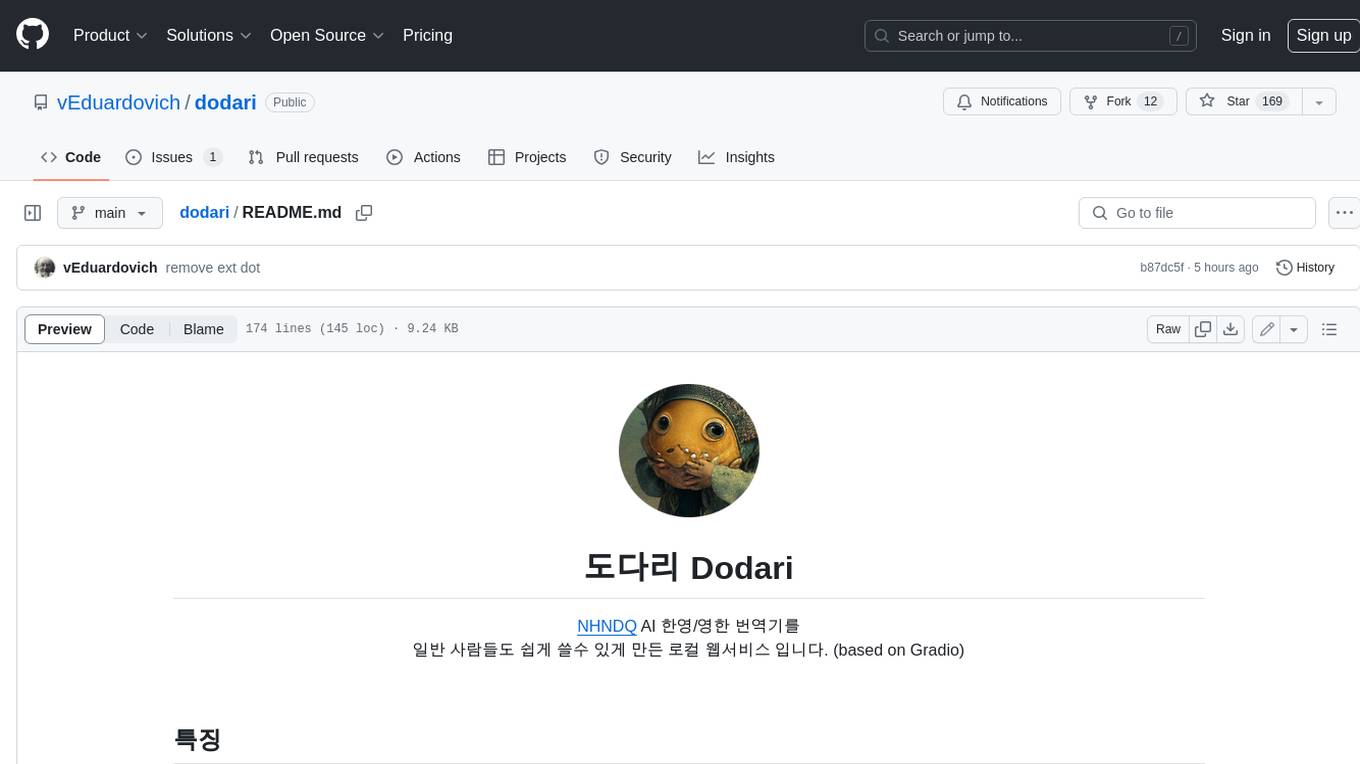
dodari
Dodari is a local web service that makes NHNDQ AI Korean-English/English-Korean translation easy for everyday people to use (based on Gradio).
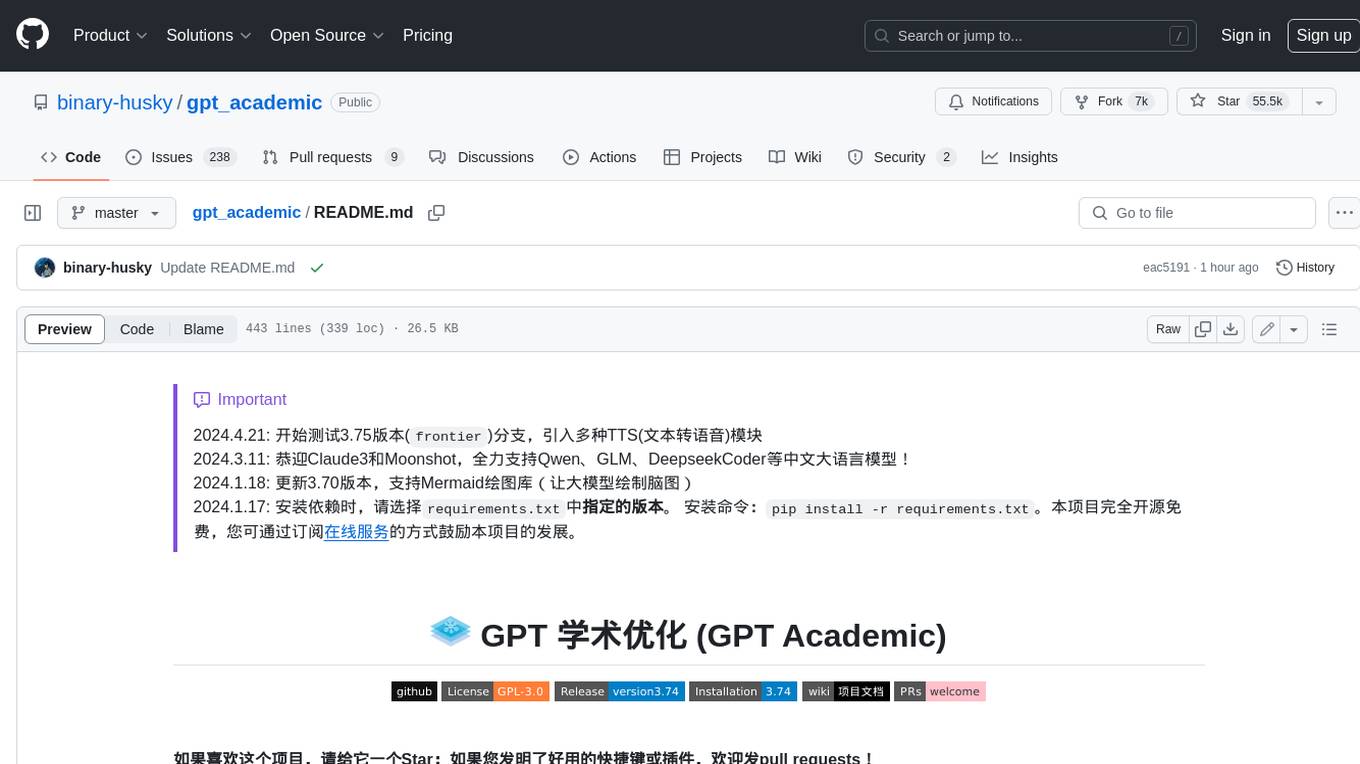
gpt_academic
GPT Academic is a powerful tool that leverages the capabilities of large language models (LLMs) to enhance academic research and writing. It provides a user-friendly interface that allows researchers, students, and professionals to interact with LLMs and utilize their abilities for various academic tasks. With GPT Academic, users can access a wide range of features and functionalities, including: * **Summarization and Paraphrasing:** GPT Academic can summarize complex texts, articles, and research papers into concise and informative summaries. It can also paraphrase text to improve clarity and readability. * **Question Answering:** Users can ask GPT Academic questions related to their research or studies, and the tool will provide comprehensive and well-informed answers based on its knowledge and understanding of the relevant literature. * **Code Generation and Explanation:** GPT Academic can generate code snippets and provide explanations for complex coding concepts. It can also help debug code and suggest improvements. * **Translation:** GPT Academic supports translation of text between multiple languages, making it a valuable tool for researchers working with international collaborations or accessing resources in different languages. * **Citation and Reference Management:** GPT Academic can help users manage their citations and references by automatically generating citations in various formats and providing suggestions for relevant references based on the user's research topic. * **Collaboration and Note-Taking:** GPT Academic allows users to collaborate on projects and take notes within the tool. They can share their work with others and access a shared workspace for real-time collaboration. * **Customizable Interface:** GPT Academic offers a customizable interface that allows users to tailor the tool to their specific needs and preferences. They can choose from a variety of themes, adjust the layout, and add or remove features to create a personalized workspace. Overall, GPT Academic is a versatile and powerful tool that can significantly enhance the productivity and efficiency of academic research and writing. It empowers users to leverage the capabilities of LLMs and unlock new possibilities for academic exploration and knowledge creation.
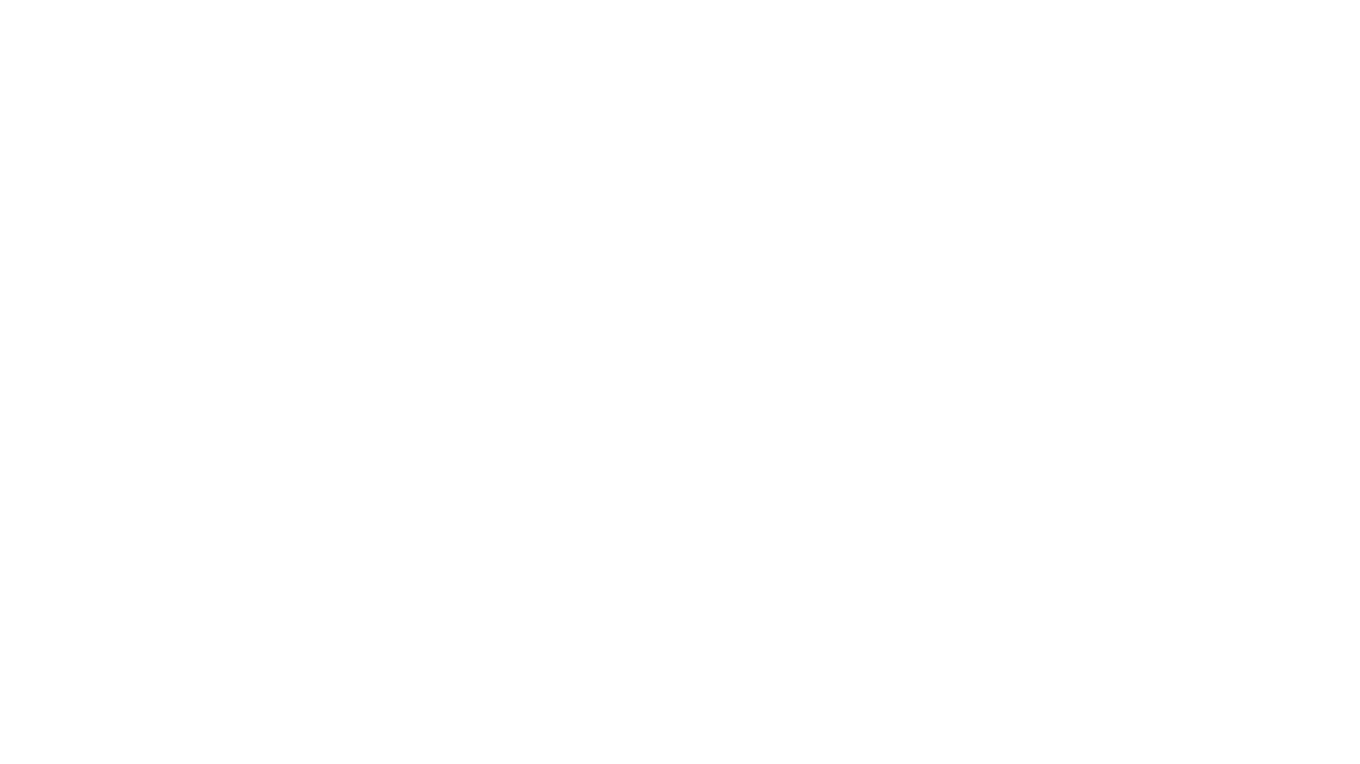
Chinese-LLaMA-Alpaca-2
Chinese-LLaMA-Alpaca-2 is a large Chinese language model developed by Meta AI. It is based on the Llama-2 model and has been further trained on a large dataset of Chinese text. Chinese-LLaMA-Alpaca-2 can be used for a variety of natural language processing tasks, including text generation, question answering, and machine translation. Here are some of the key features of Chinese-LLaMA-Alpaca-2: * It is the largest Chinese language model ever trained, with 13 billion parameters. * It is trained on a massive dataset of Chinese text, including books, news articles, and social media posts. * It can be used for a variety of natural language processing tasks, including text generation, question answering, and machine translation. * It is open-source and available for anyone to use. Chinese-LLaMA-Alpaca-2 is a powerful tool that can be used to improve the performance of a wide range of natural language processing tasks. It is a valuable resource for researchers and developers working in the field of artificial intelligence.