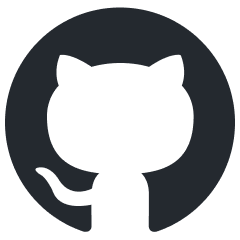
grps_trtllm
Higher performance OpenAI LLM service than vLLM serve: A pure C++ high-performance OpenAI LLM service implemented with GPRS+TensorRT-LLM+Tokenizers.cpp, supporting chat and function call, AI agents, distributed multi-GPU inference, multimodal capabilities, and a Gradio chat interface.
Stars: 122
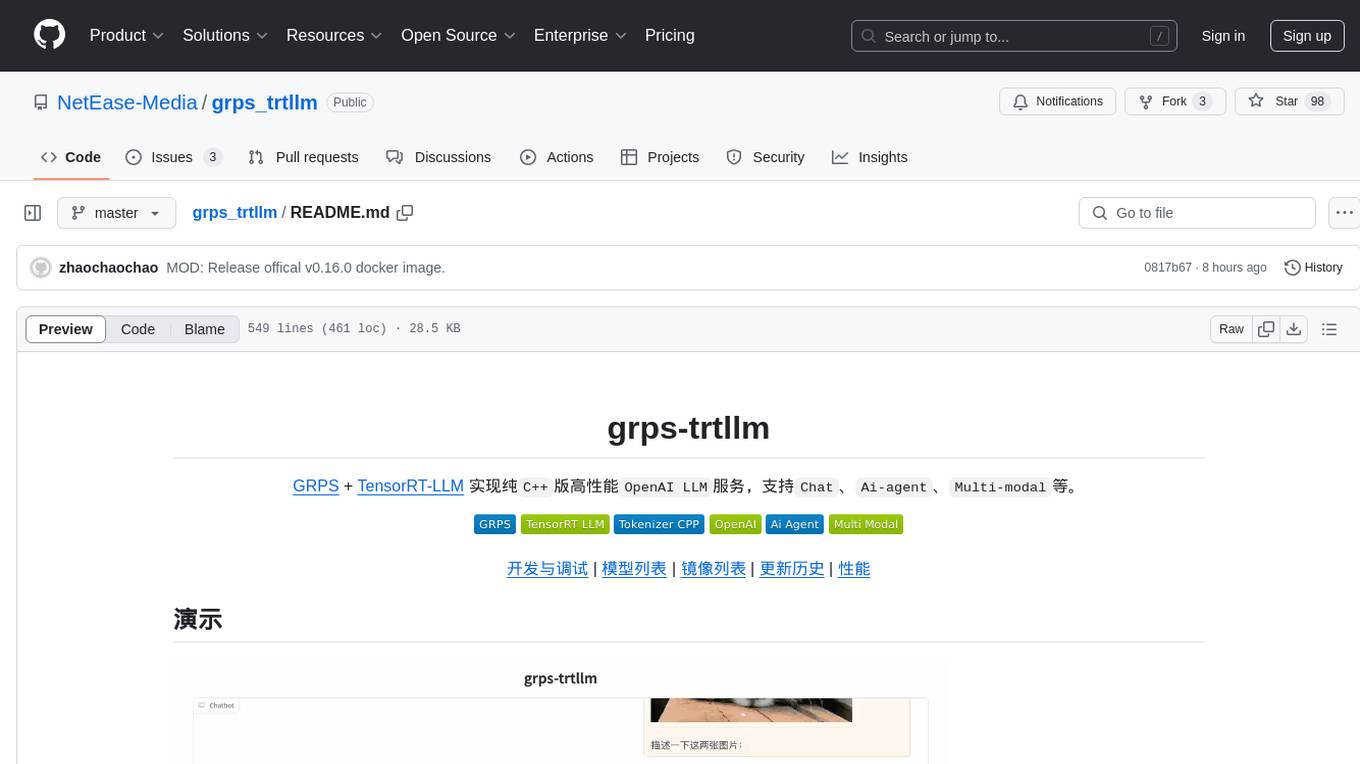
The grps-trtllm repository is a C++ implementation of a high-performance OpenAI LLM service, combining GRPS and TensorRT-LLM. It supports functionalities like Chat, Ai-agent, and Multi-modal. The repository offers advantages over triton-trtllm, including a complete LLM service implemented in pure C++, integrated tokenizer supporting huggingface and sentencepiece, custom HTTP functionality for OpenAI interface, support for different LLM prompt styles and result parsing styles, integration with tensorrt backend and opencv library for multi-modal LLM, and stable performance improvement compared to triton-trtllm.
README:
GRPS + TensorRT-LLM
实现纯C++
版,相比vllm serve
更优性能的OpenAI LLM
服务,支持Chat
、Ai-agent
、Multi-modal
、多卡推理等。
快速开始 | 模型列表 | 镜像列表 | 性能 | 更新历史 | 预告

grps接入trtllm
实现更高性能的、支持OpenAI
模式访问、支持Ai-agent
以及多模态的LLM
服务,相比较triton tensorrtllm_backend
实现服务。有如下优势:
- 通过纯
C++
实现完整LLM
服务。包含tokenizer
部分,支持huggingface
,sentencepiece
tokenizer。 - 不存在
triton_server <--> tokenizer_backend <--> trtllm_backend
之间的进程间通信。 - 通过
grps
的自定义http
功能实现OpenAI
接口协议,支持chat
和function call
模式。 - 支持扩展不同
LLM
的prompt
构建风格以及生成结果的解析风格,以实现不同LLM
的chat
和function call
模式,支持llama-indexai-agent
。 - 通过集成
tensorrt
推理后端与opencv
库,支持多模态LLM
。 - 通过测试,
grps-trtllm
相比较triton-trtllm
性能有稳定的提升。
欢迎各位使用和提issue ,欢迎提交pr支持新的模型,感谢star⭐️。也可以添加微信沟通:zhaocc1218。
支持的文本LLM:
supported model | llm_styler | chat | function_call | doc |
---|---|---|---|---|
DeepSeek-R1-Distill TinyR1-32B-Preview |
deepseek-r1 | ✅ | ❌ | deepseek-r1-distill |
QwQ-32B QwQ-32B-AWQ |
qwq | ✅ | ✅ | qwq |
QwQ-32B-Preview | qwq-preview | ✅ | ❌ | qwq-preview |
Qwen2.5-1M Qwen2.5-Coder Qwen2.5-Math Qwen2.5 |
qwen2.5 | ✅ | ✅ | qwen2.5 |
Qwen1.5-Chat Qwen1.5-Moe-Chat Qwen2-Instruct Qwen2-Moe-Instruct |
qwen | ✅ | ✅ | qwen2 |
chatglm3 | chatglm3 | ✅ | ✅ | chatglm3 |
glm4 | glm4 | ✅ | ✅ | glm4 |
internlm2_5-chat internlm2-chat |
internlm2 | ✅ | ✅ | internlm2.5 |
llama-3-instruct llama-3.1-instruct |
llama3 | ✅ | ❌ | llama3 |
phi-4 | phi4 | ✅ | ❌ | phi4 |
Phi-3, Phi-3.5 | phi3 | ✅ | ❌ | phi3 |
gemma-3(experimental) | gemma3 | ✅ | ❌ | gemma-3 |
支持的多模态LLM:
supported model | llm_styler | vit | vit_type | chat | function_call | doc |
---|---|---|---|---|---|---|
MiniCPM-V-2_6 | minicpmv | minicpmv | py | ✅ | ❌ | minicpmv |
Janus-Pro | janus-pro | janus-pro | c++ | ✅ | ❌ | janus-pro |
InternVideo2.5 | intern-video2.5 | intern-video2.5 | py | ✅ | ❌ | intern-video2.5 |
InternVL2_5 InternVL2_5-MPO |
internvl2.5 | internvl2 | c++ | ✅ | ❌ | internvl2.5 |
InternVL2-2B InternVL2-8B InternVL2-26B |
internvl2-internlm2 | internvl2 | c++ | ✅ | ❌ | internvl2 |
InternVL2-1B | internvl2-qwen2 | internvl2 | c++ | ✅ | ❌ | internvl2 |
InternVL2-4B | internvl2-phi3 | internvl2 | c++ | ✅ | ❌ | internvl2 |
olmOCR | qwen2vl | qwen2vl | c++ | ✅ | ❌ | olm-ocr |
Qwen2-VL-Instruct | qwen2vl | qwen2vl | c++ | ✅ | ❌ | qwen2vl |
Qwen-VL-Chat Qwen-VL |
qwenvl | qwenvl | c++ | ✅ | ❌ | qwenvl |
|-- client # 客户端样例
|-- conf # 配置文件
| |-- inference*.yml # 各类llm推理配置
| |-- server.yml # 服务配置
|-- data # 数据文件
|-- docker # docker镜像构建
|-- docs # 文档
|-- processors # 远程处理器
|-- second_party # grps框架依赖
|-- src # 自定义源码
| |-- tensorrt # tensorrt推理后端
| |-- vit # vit实现
| |-- constants.cc/.h # 常量定义
| |-- customized_inferer.cc/.h # 自定义推理器
| |-- llm_styler.cc/.h # LLM风格定义,prompt构建,结果解析
| |-- tokenizer.cc/.h # Tokenizer实现
| |-- trtllm_model_instance.cc/.h # TensorRT-LLM模型实例
| |-- trtllm_model_state.cc/.h # TensorRT-LLM模型状态
| |-- utils.cc/.h # 工具
| |-- main.cc # 本地单元测试
|-- third_party # 第三方依赖
|-- tools # 工具
|-- build.sh # 构建脚本
|-- CMakelists.txt # 工程构建文件
|-- .clang-format # 代码格式化配置文件
|-- .config # 工程配置文件,包含一些工程配置开关
以qwen2.5-instruct为例。更多llm示例见模型列表,拉取代码与创建容器步骤相同。
git clone https://github.com/NetEase-Media/grps_trtllm.git
cd grps_trtllm
git submodule update --init --recursive
使用registry.cn-hangzhou.aliyuncs.com/opengrps/grps_gpu:grps1.1.0_cuda12.6_cudnn9.6_trtllm0.16.0_py3.12
镜像。
这里挂载了当前目录用于构建工程并保留构建产物,挂载/tmp目录用于保存构建的trtllm引擎文件。参考triton-trtllm
设置共享内存大小,解除物理内存锁定限制,设置栈大小,配置参数
--shm-size=2g --ulimit memlock=-1 --ulimit stack=67108864
。
# 创建容器
docker run -itd --name grps_trtllm_dev --runtime=nvidia --network host --shm-size=2g --ulimit memlock=-1 --ulimit stack=67108864 \
-v $(pwd):/grps_dev -v /tmp:/tmp -w /grps_dev \
registry.cn-hangzhou.aliyuncs.com/opengrps/grps_gpu:grps1.1.0_cuda12.6_cudnn9.6_trtllm0.16.0_py3.12 bash
# 进入开发容器
docker exec -it grps_trtllm_dev bash
# 下载Qwen2.5-7B-Instruct模型
apt update && apt install git-lfs
git lfs install
git clone https://huggingface.co/Qwen/Qwen2.5-7B-Instruct /tmp/Qwen2.5-7B-Instruct
# 进入TensorRT-LLM/examples/qwen目录,参考README进行构建trtllm引擎。
cd third_party/TensorRT-LLM/examples/qwen
# 转换ckpt
rm -rf /tmp/Qwen2.5-7B-Instruct/tllm_checkpoint/
python3 convert_checkpoint.py --model_dir /tmp/Qwen2.5-7B-Instruct \
--output_dir /tmp/Qwen2.5-7B-Instruct/tllm_checkpoint/ --dtype bfloat16 --load_model_on_cpu
# 构建引擎
rm -rf /tmp/Qwen2.5-7B-Instruct/trt_engines/
trtllm-build --checkpoint_dir /tmp/Qwen2.5-7B-Instruct/tllm_checkpoint/ \
--output_dir /tmp/Qwen2.5-7B-Instruct/trt_engines/ \
--gemm_plugin bfloat16 --max_batch_size 16 --paged_kv_cache enable --use_paged_context_fmha enable \
--max_input_len 32256 --max_seq_len 32768 --max_num_tokens 32256
# 运行测试
python3 ../run.py --input_text "你好,你是谁?" --max_output_len=50 \
--tokenizer_dir /tmp/Qwen2.5-7B-Instruct/ \
--engine_dir=/tmp/Qwen2.5-7B-Instruct/trt_engines/
# 回到工程根目录
cd ../../../../
修改llm对应的conf/inference*.yml中inferer_args
相关参数。注意修改tokenizer_path
和gpt_model_path
为新路径,更多核心参数见如下:
models:
- name: trtllm_model
...
inferer_args:
# llm style used to build prompt(chat or function call) and parse generated response for openai interface.
# Support llm_style see README.md.
llm_style: qwen2.5
# tokenizer config.
tokenizer_type: huggingface # can be `huggingface`, `sentencepiece`. Must be set.
tokenizer_path: /tmp/Qwen2.5-7B-Instruct/ # path of tokenizer. Must be set.
tokenizer_parallelism: 16 # tokenizers count for parallel tokenization. Will be set to 1 if not set.
end_token_id: 151645 # end token id of tokenizer. Null if not set.
pad_token_id: 151643 # pad token id of tokenizer. Null if not set.
skip_special_tokens: # skip special tokens when decoding. Empty if not set.
- 151643 # "<|endoftext|>"
- 151644 # "<|im_start|>"
- 151645 # "<|im_end|>"
...
force_tokens_dict: # will be used to force map tokens to ids when encode and decode instead of using tokenizer. Empty if not set.
# - token: "<|endoftext|>"
# id: 151643
prefix_tokens_id: # prefix tokens id will be added to the beginning of the input ids. Empty if not set.
suffix_tokens_id: # suffix tokens id will be added to the end of the input ids. Empty if not set.
# default sampling config, sampling param in request will overwrite these. Support sampling params see
# @ref(src/constants.h - SamplingConfig)
sampling:
top_k: 50
top_p: 1.0
# trtllm config.
gpt_model_type: inflight_fused_batching # must be `V1`(==`v1`) or `inflight_batching`(==`inflight_fused_batching`).
gpt_model_path: /tmp/Qwen2.5-7B-Instruct/trt_engines/ # path of decoder model. Must be set.
encoder_model_path: # path of encoder model. Null if not set.
stop_words: # additional stop words. Empty if not set.
- "<|im_start|>"
- "<|im_end|>"
- "<|endoftext|>"
bad_words: # additional bad words. Empty if not set.
batch_scheduler_policy: guaranteed_no_evict # must be `max_utilization` or `guaranteed_no_evict`.
kv_cache_free_gpu_mem_fraction: 0.9 # will be set to 0.9 or `max_tokens_in_paged_kv_cache` if not set.
exclude_input_in_output: true # will be set to false if not set.
# 构建
grpst archive .
# 部署,
# 通过--inference_conf参数指定模型对应的inference.yml配置文件启动服务。
# 如需修改服务端口,并发限制等,可以修改conf/server.yml文件,然后启动时指定--server_conf参数指定新的server.yml文件。
# 注意如果使用多卡推理,需要使用mpi方式启动,--mpi_np参数为并行推理的GPU数量。
grpst start ./server.mar --inference_conf=conf/inference_qwen2.5.yml
# 查看服务状态
grpst ps
# 如下输出
PORT(HTTP,RPC) NAME PID DEPLOY_PATH
9997 my_grps 65322 /home/appops/.grps/my_grps
# curl命令非stream请求
curl --no-buffer http://127.0.0.1:9997/v1/chat/completions \
-H "Content-Type: application/json" \
-d '{
"model": "qwen2.5-instruct",
"messages": [
{
"role": "user",
"content": "你好,你是谁?"
}
]
}'
# 返回如下:
: '
{
"id": "chatcmpl-7",
"object": "chat.completion",
"created": 1726733862,
"model": "qwen2.5-instruct",
"system_fingerprint": "grps-trtllm-server",
"choices": [
{
"index": 0,
"message": {
"role": "assistant",
"content": "你好!我是Qwen,由阿里云开发的人工智能模型。我被设计用来提供信息、回答问题和进行各种对话任务。有什么我可以帮助你的吗?"
},
"logprobs": null,
"finish_reason": "stop"
}
],
"usage": {
"prompt_tokens": 34,
"completion_tokens": 36,
"total_tokens": 70
}
}
'
# curl命令stream请求
curl --no-buffer http://127.0.0.1:9997/v1/chat/completions \
-H "Content-Type: application/json" \
-d '{
"model": "qwen2.5-instruct",
"messages": [
{
"role": "user",
"content": "你好,你是谁?"
}
],
"stream": true
}'
# 返回如下:
: '
data: {"id":"chatcmpl-8","object":"chat.completion.chunk","created":1726733878,"model":"qwen2.5-instruct","system_fingerprint":"grps-trtllm-server","choices":[{"index":0,"delta":{"role":"assistant","content":"你好"},"logprobs":null,"finish_reason":null}]}
data: {"id":"chatcmpl-8","object":"chat.completion.chunk","created":1726733878,"model":"qwen2.5-instruct","system_fingerprint":"grps-trtllm-server","choices":[{"index":0,"delta":{"content":"!"},"logprobs":null,"finish_reason":null}]}
data: {"id":"chatcmpl-8","object":"chat.completion.chunk","created":1726733878,"model":"qwen2.5-instruct","system_fingerprint":"grps-trtllm-server","choices":[{"index":0,"delta":{"content":"我是"},"logprobs":null,"finish_reason":null}]}
'
# 测试stop参数
curl --no-buffer http://127.0.0.1:9997/v1/chat/completions \
-H "Content-Type: application/json" \
-d '{
"model": "qwen2.5-instruct",
"messages": [
{
"role": "user",
"content": "重复1234#END#5678"
}
],
"stop": ["#END#"]
}'
# 返回如下:
: '
{
"id": "chatcmpl-2",
"object": "chat.completion",
"created": 1727433345,
"model": "qwen2.5-instruct",
"system_fingerprint": "grps-trtllm-server",
"choices": [
{
"index": 0,
"message": {
"role": "assistant",
"content": "1234#END#"
},
"logprobs": null,
"finish_reason": "stop"
}
],
"usage": {
"prompt_tokens": 41,
"completion_tokens": 7,
"total_tokens": 48
}
}
'
# openai_cli.py 非stream请求
python3 client/openai_cli.py 127.0.0.1:9997 "你好,你是谁?" false
# 返回如下:
: '
ChatCompletion(id='chatcmpl-9', choices=[Choice(finish_reason='stop', index=0, logprobs=None, message=ChatCompletionMessage(content='你好!我是Qwen,由阿里云开发的人工智能模型。我被设计用来提供信息、回答问题和进行各种对话任务。有什么我可以帮助你的吗?', refusal=None, role='assistant', function_call=None, tool_calls=None))], created=1726733895, model='', object='chat.completion', service_tier=None, system_fingerprint='grps-trtllm-server', usage=CompletionUsage(completion_tokens=36, prompt_tokens=34, total_tokens=70, completion_tokens_details=None))
'
# openai_cli.py stream请求
python3 client/openai_cli.py 127.0.0.1:9997 "你好,你是谁?" true
# 返回如下:
: '
ChatCompletionChunk(id='chatcmpl-10', choices=[Choice(delta=ChoiceDelta(content='你好', function_call=None, refusal=None, role='assistant', tool_calls=None), finish_reason=None, index=0, logprobs=None)], created=1726733914, model='', object='chat.completion.chunk', service_tier=None, system_fingerprint='grps-trtllm-server', usage=None)
ChatCompletionChunk(id='chatcmpl-10', choices=[Choice(delta=ChoiceDelta(content='!', function_call=None, refusal=None, role=None, tool_calls=None), finish_reason=None, index=0, logprobs=None)], created=1726733914, model='', object='chat.completion.chunk', service_tier=None, system_fingerprint='grps-trtllm-server', usage=None)
ChatCompletionChunk(id='chatcmpl-10', choices=[Choice(delta=ChoiceDelta(content='我是', function_call=None, refusal=None, role=None, tool_calls=None), finish_reason=None, index=0, logprobs=None)], created=1726733914, model='', object='chat.completion.chunk', service_tier=None, system_fingerprint='grps-trtllm-server', usage=None)
'
# 输入32k长文本小说验证长文本的支持
python3 client/openai_txt_cli.py 127.0.0.1:9997 ./data/32k_novel.txt "上面这篇小说作者是谁?" false
# 返回如下:
: '
ChatCompletion(id='chatcmpl-11', choices=[Choice(finish_reason='stop', index=0, logprobs=None, message=ChatCompletionMessage(content='这篇小说的作者是弦三千。', refusal=None, role='assistant', function_call=None, tool_calls=None))], created=1726733931, model='', object='chat.completion', service_tier=None, system_fingerprint='grps-trtllm-server', usage=CompletionUsage(completion_tokens=8, prompt_tokens=31615, total_tokens=31623, completion_tokens_details=None))
'
# 输入32k长文本小说进行总结
python3 client/openai_txt_cli.py 127.0.0.1:9997 ./data/32k_novel.txt "简述一下上面这篇小说的前几章内容。" false
# 返回如下:
: '
ChatCompletion(id='chatcmpl-12', choices=[Choice(finish_reason='stop', index=0, logprobs=None, message=ChatCompletionMessage(content='以下是《拜托,只想干饭的北极熊超酷的!》前几章的主要内容概述:\n\n1. **第一章**:楚云霁意外穿越成了一只北极熊,他发现了一群科考队,并用鱼与他们交流。楚云霁在暴风雪中艰难生存,通过抓鱼和捕猎海豹来获取食物。\n\n2. **第二章**:楚云霁在暴风雪后继续捕猎,遇到了一只北极白狼。白狼似乎对楚云霁很友好,甚至带他去捕猎海豹。楚云霁吃了一顿饱饭后,与白狼一起回到白狼的洞穴休息。\n\n3. **第三章**:楚云霁在白狼的洞穴中休息,醒来后发现白狼已经离开。他继续捕猎,遇到了一群海豹,但海豹很快被一只成年北极熊吓跑。楚云霁在冰面上发现了一群生蚝,但白狼对生蚝不感兴趣,楚云霁只好自己吃了。\n\n4. **第四章**:楚云霁在捕猎时遇到了一只成年北极熊,成年北极熊似乎在挑衅他。楚云霁和白狼一起捕猎了一只驯鹿,分享了食物。直播设备记录下了这一幕,引起了观众的热议。\n\n5. **第五章**:楚云霁和白狼一起捕猎了一只驯鹿,分享了食物。楚云霁在捕猎时遇到了一只北极狐,但北极狐被北极熊吓跑。楚云霁还遇到了一只海鸟,海鸟试图抢食,但被白狼赶走。楚云霁和白狼一起处理了一只驯鹿,白狼还帮助楚云霁取下了鹿角。\n\n6. **第六章**:楚云霁和白狼一起捕猎,楚云霁在冰面上睡觉时被冰面漂走。醒来后,楚云霁发现白狼还在身边,感到非常高兴。他们一起捕猎了一只海象,但海象偷走了鱼竿。楚云霁和白狼一起追捕海象,最终成功捕获了海象。\n\n7. **第七章**:楚云霁和白狼一起捕猎,楚云霁发现了一根鱼竿。他们一起用鱼竿钓鱼,但鱼竿被海象带走。楚云霁和白狼一起追捕海象,最终成功捕获了海象。楚云霁和白狼一起分享了海象肉。\n\n8. **第八章**:楚云霁和白狼一起捕猎,楚云霁发现了一根鱼竿。他们一起用鱼竿钓鱼,但鱼竿被海象带走。楚云霁和白狼一起追捕海象,最终成功捕获了海象。楚云霁和白狼一起分享了海象肉。\n\n9. **第九章**:楚云霁和白狼一起捕猎,楚云霁发现了一根鱼竿。他们一起用鱼竿钓鱼,但鱼竿被海象带走。楚云霁和白狼一起追捕海象,最终成功捕获了海象。楚云霁和白狼一起分享了海象肉。\n\n10. **第十章**:楚云霁和白狼一起捕猎,楚云霁发现了一根鱼竿。他们一起用鱼竿钓鱼,但鱼竿被海象带走。楚云霁和白狼一起追捕海象,最终成功捕获了海象。楚云霁和白狼一起分享了海象肉。\n\n11. **第十一章**:楚云霁在白狼的洞穴中发现了一个背包,背包里装满了各种食物和补给品。楚云霁和白狼一起分享了这些食物,包括罐头和海带。楚云霁还和白狼一起出去捕猎,但没有成功。\n\n12. **第十二章**:楚云霁和白狼一起出去捕猎,楚云霁发现了一根鱼竿。他们一起用鱼竿钓鱼,但鱼竿被海象带走。楚云霁和白狼一起追捕海象,最终成功捕获了海象。楚云霁和白狼一起分享了海象肉,并一起出去探索周围的环境。楚云霁还发现了一个背包,背包里装满了各种食物和补给品。楚云霁和白狼一起分享了这些食物,包括罐头和海带。楚云霁还和白狼一起出去捕猎,但没有成功。', refusal=None, role='assistant', function_call=None, tool_calls=None))], created=1726733966, model='', object='chat.completion', service_tier=None, system_fingerprint='grps-trtllm-server', usage=CompletionUsage(completion_tokens=959, prompt_tokens=31621, total_tokens=32580, completion_tokens_details=None))
'
# openai_func_call.py进行function call模拟
python3 client/openai_func_call.py 127.0.0.1:9997
# 返回如下:
: '
Query server with question: What's the weather like in Boston today? ...
Server response: thought: None, call local function(get_current_weather) with arguments: location=Boston, MA, unit=fahrenheit
Send the result back to the server with function result(59.0) ...
Final server response: The current temperature in Boston today is 59°F.
'
# openai_func_call2.py进行一次两个函数的function call模拟
python3 client/openai_func_call2.py 127.0.0.1:9997
# 返回如下:
: '
Query server with question: What's the postcode of Boston and what's the weather like in Boston today? ...
Server response: thought: None, call local function(get_postcode) with arguments: location=Boston, MA
Server response: thought: None, call local function(get_current_weather) with arguments: location=Boston, MA, unit=fahrenheit
Send the result back to the server with function result ...
Final server response: The postcode for Boston, MA is 02138. The current temperature in Boston today is 59.0°F.
'
# llama-index ai agent模拟
pip install llama_index llama_index.llms.openai_like
python3 client/llamaindex_ai_agent.py 127.0.0.1:9997
# 返回如下:
: '
Query: What is the weather in Boston today?
Added user message to memory: What is the weather in Boston today?
=== Calling Function ===
Calling function: get_weather with args: {"location":"Boston, MA","unit":"fahrenheit"}
Got output: 59.0
========================
Response: The current temperature in Boston is 59.0 degrees Fahrenheit.
'
通过访问http://ip:9997/
可以查看服务的指标信息。如下指标:
# 关闭服务
grpst stop my_grps
- 当前基于
tensorrt-llm v0.10.0
之后的版本进行的实现,最新支持到v0.16.0
(主分支),具体见仓库的分支信息。由于人力受限,一些bug不能及时在每一个分支修复,请尽量使用最新版本分支。 - 由于不同家族系的
LLM
的chat
和function call
的prompt
构建以及结果解析风格不同,所以需要实现不同LLM
家族的styler
,见src/llm_styler.cc/.h
,用户可以自行扩展。拓展后需要修改conf/inference.yml
的llm_style
为对应的家族名。 不同家族的styler
持续开发中...。 - 不同多模态模型的
vit
实现不同,见src/vit
,用户可以自行扩展。拓展后需要修改conf/inference.yml
的vit_type
为对应的类型名。 不同多模态模型的vit
持续开发中...。 - 书写用户自定义拓展
llm_styler
与vit
开发文档。
For Tasks:
Click tags to check more tools for each tasksFor Jobs:
Alternative AI tools for grps_trtllm
Similar Open Source Tools
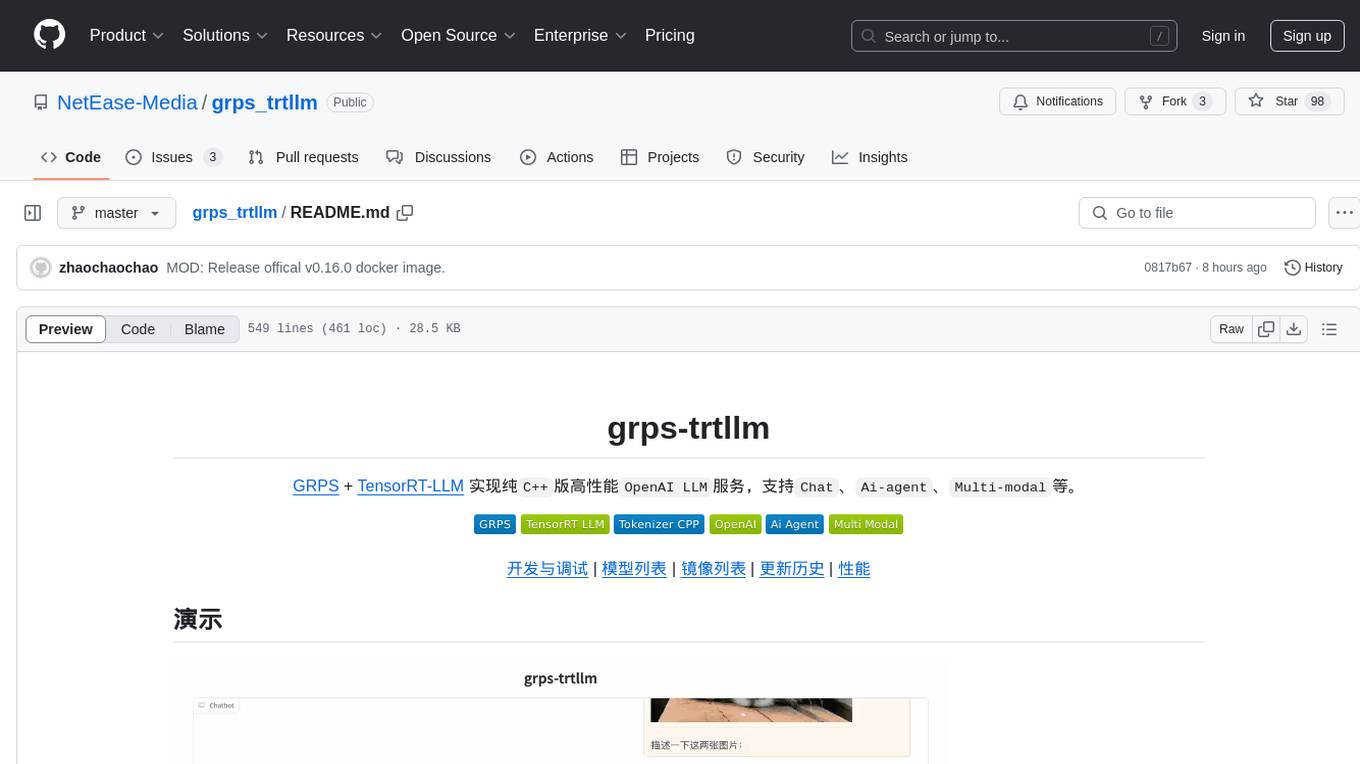
grps_trtllm
The grps-trtllm repository is a C++ implementation of a high-performance OpenAI LLM service, combining GRPS and TensorRT-LLM. It supports functionalities like Chat, Ai-agent, and Multi-modal. The repository offers advantages over triton-trtllm, including a complete LLM service implemented in pure C++, integrated tokenizer supporting huggingface and sentencepiece, custom HTTP functionality for OpenAI interface, support for different LLM prompt styles and result parsing styles, integration with tensorrt backend and opencv library for multi-modal LLM, and stable performance improvement compared to triton-trtllm.
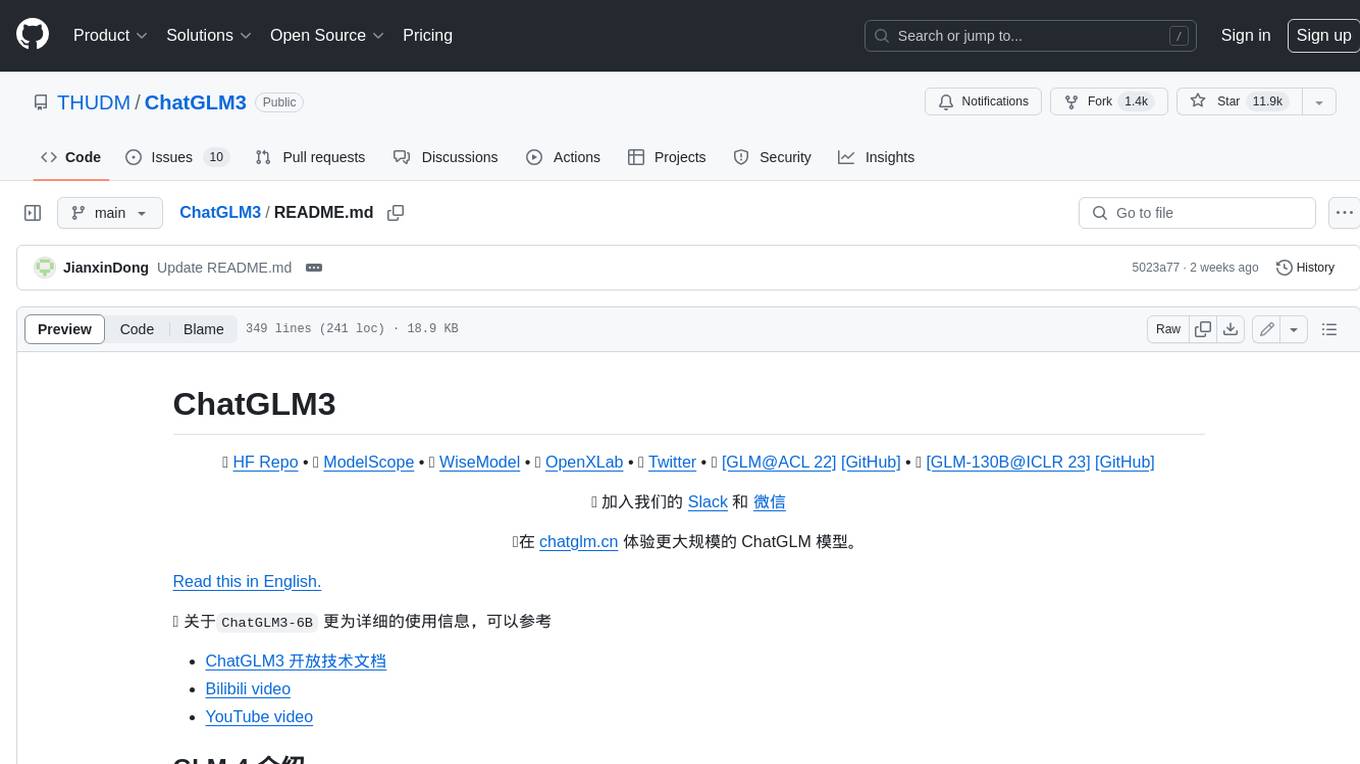
ChatGLM3
ChatGLM3 is a conversational pretrained model jointly released by Zhipu AI and THU's KEG Lab. ChatGLM3-6B is the open-sourced model in the ChatGLM3 series. It inherits the advantages of its predecessors, such as fluent conversation and low deployment threshold. In addition, ChatGLM3-6B introduces the following features: 1. A stronger foundation model: ChatGLM3-6B's foundation model ChatGLM3-6B-Base employs more diverse training data, more sufficient training steps, and more reasonable training strategies. Evaluation on datasets from different perspectives, such as semantics, mathematics, reasoning, code, and knowledge, shows that ChatGLM3-6B-Base has the strongest performance among foundation models below 10B parameters. 2. More complete functional support: ChatGLM3-6B adopts a newly designed prompt format, which supports not only normal multi-turn dialogue, but also complex scenarios such as tool invocation (Function Call), code execution (Code Interpreter), and Agent tasks. 3. A more comprehensive open-source sequence: In addition to the dialogue model ChatGLM3-6B, the foundation model ChatGLM3-6B-Base, the long-text dialogue model ChatGLM3-6B-32K, and ChatGLM3-6B-128K, which further enhances the long-text comprehension ability, are also open-sourced. All the above weights are completely open to academic research and are also allowed for free commercial use after filling out a questionnaire.
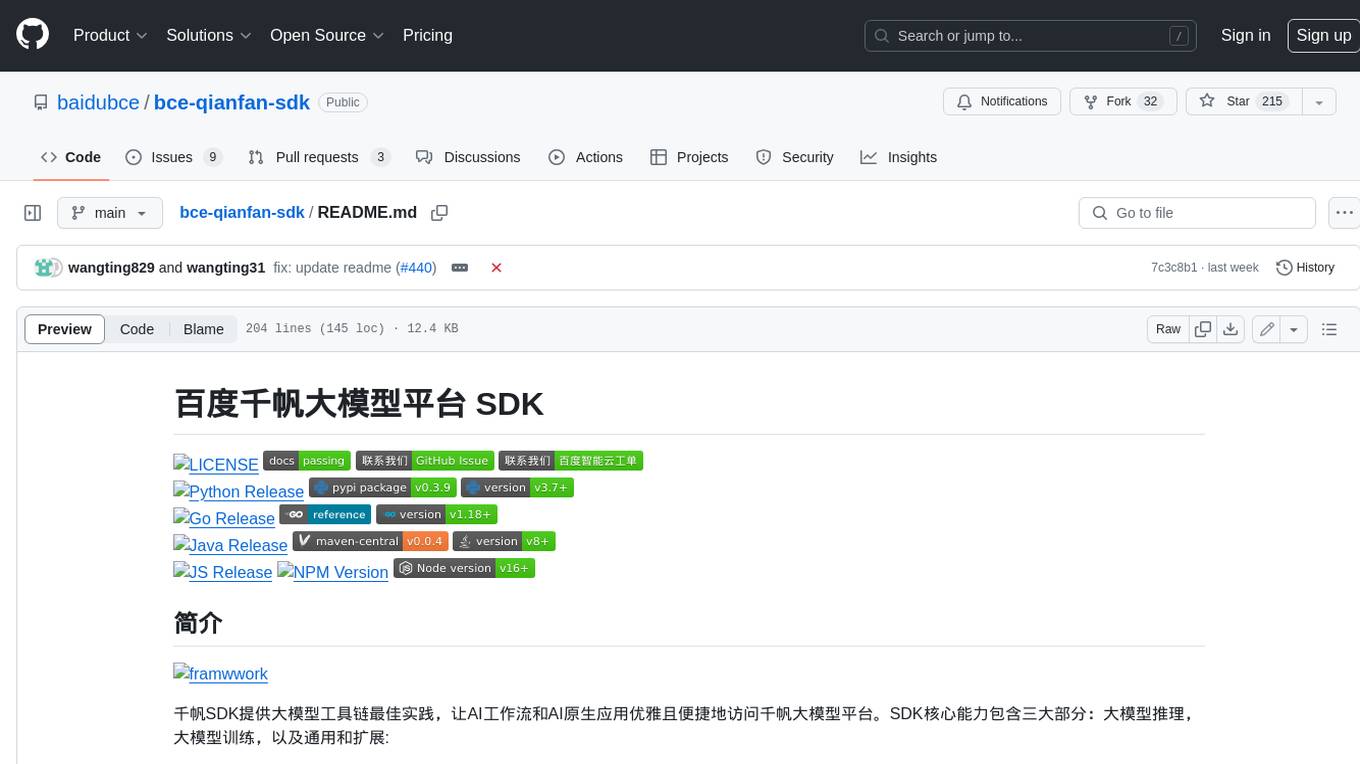
bce-qianfan-sdk
The Qianfan SDK provides best practices for large model toolchains, allowing AI workflows and AI-native applications to access the Qianfan large model platform elegantly and conveniently. The core capabilities of the SDK include three parts: large model reasoning, large model training, and general and extension: * `Large model reasoning`: Implements interface encapsulation for reasoning of Yuyan (ERNIE-Bot) series, open source large models, etc., supporting dialogue, completion, Embedding, etc. * `Large model training`: Based on platform capabilities, it supports end-to-end large model training process, including training data, fine-tuning/pre-training, and model services. * `General and extension`: General capabilities include common AI development tools such as Prompt/Debug/Client. The extension capability is based on the characteristics of Qianfan to adapt to common middleware frameworks.
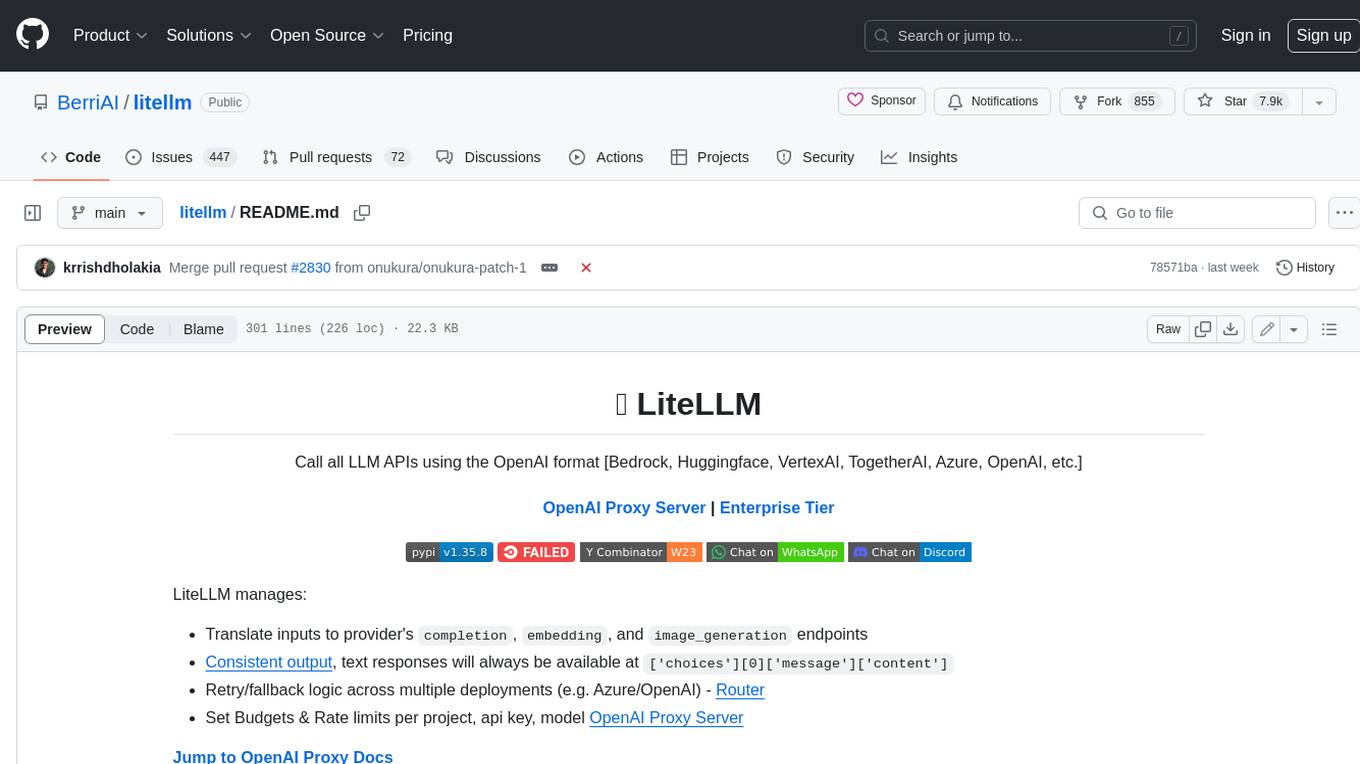
litellm
LiteLLM is a tool that allows you to call all LLM APIs using the OpenAI format. This includes Bedrock, Huggingface, VertexAI, TogetherAI, Azure, OpenAI, and more. LiteLLM manages translating inputs to provider's `completion`, `embedding`, and `image_generation` endpoints, providing consistent output, and retry/fallback logic across multiple deployments. It also supports setting budgets and rate limits per project, api key, and model.
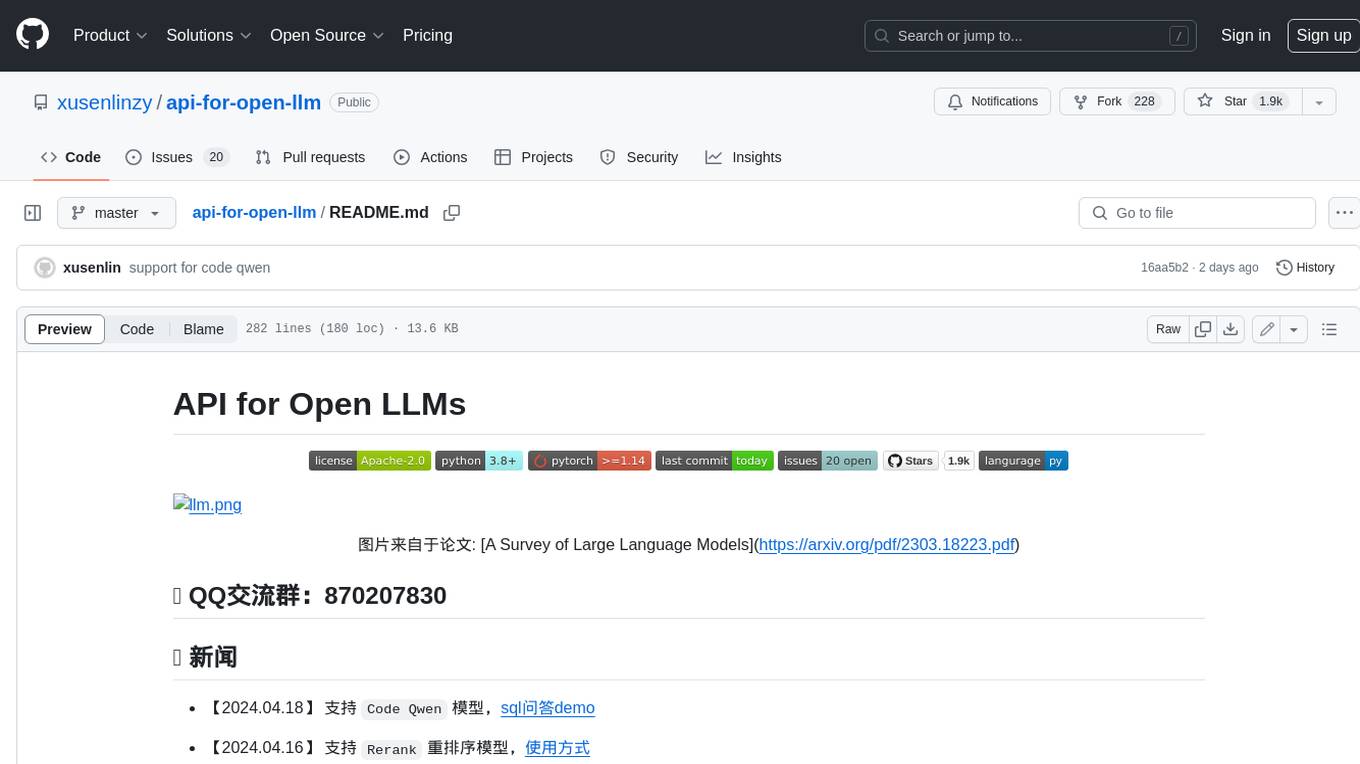
api-for-open-llm
This project provides a unified backend interface for open large language models (LLMs), offering a consistent experience with OpenAI's ChatGPT API. It supports various open-source LLMs, enabling developers to seamlessly integrate them into their applications. The interface features streaming responses, text embedding capabilities, and support for LangChain, a tool for developing LLM-based applications. By modifying environment variables, developers can easily use open-source models as alternatives to ChatGPT, providing a cost-effective and customizable solution for various use cases.
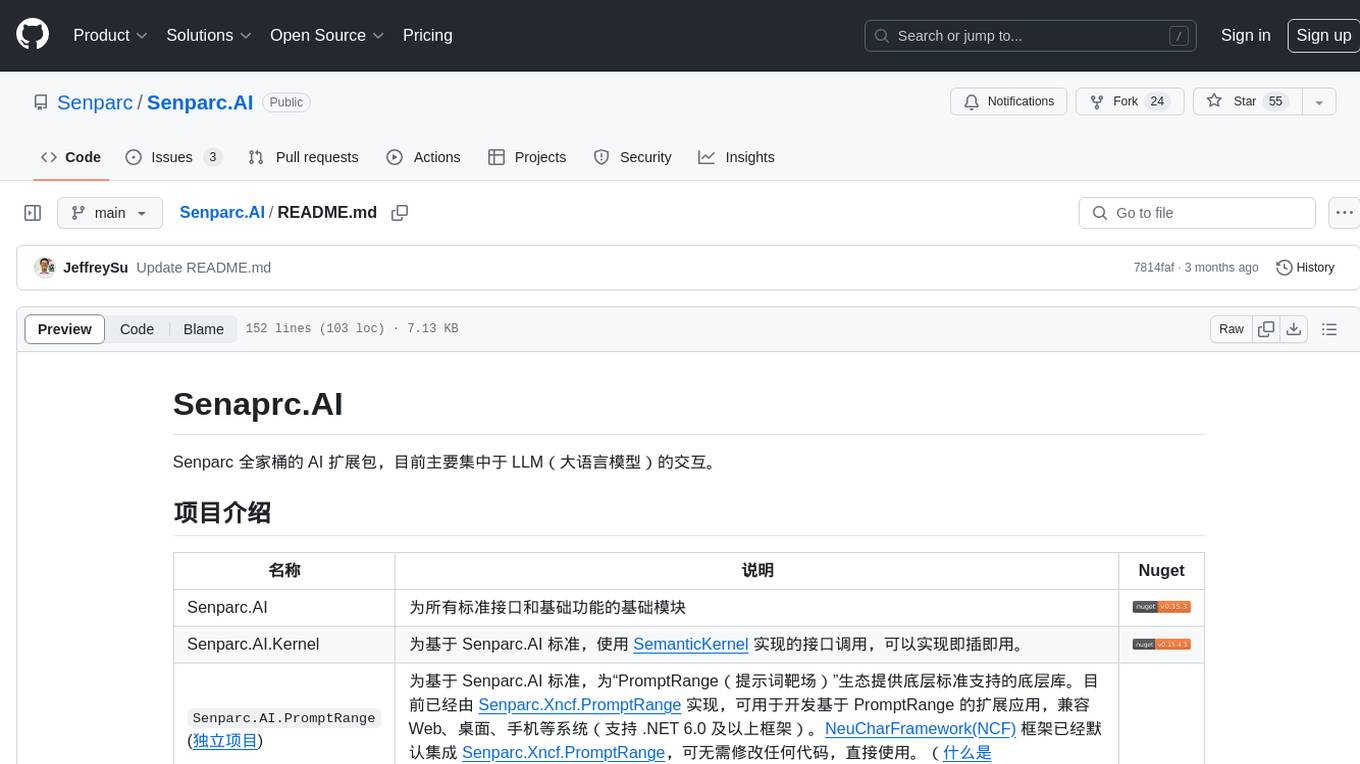
Senparc.AI
Senparc.AI is an AI extension package for the Senparc ecosystem, focusing on LLM (Large Language Models) interaction. It provides modules for standard interfaces and basic functionalities, as well as interfaces using SemanticKernel for plug-and-play capabilities. The package also includes a library for supporting the 'PromptRange' ecosystem, compatible with various systems and frameworks. Users can configure different AI platforms and models, define AI interface parameters, and run AI functions easily. The package offers examples and commands for dialogue, embedding, and DallE drawing operations.
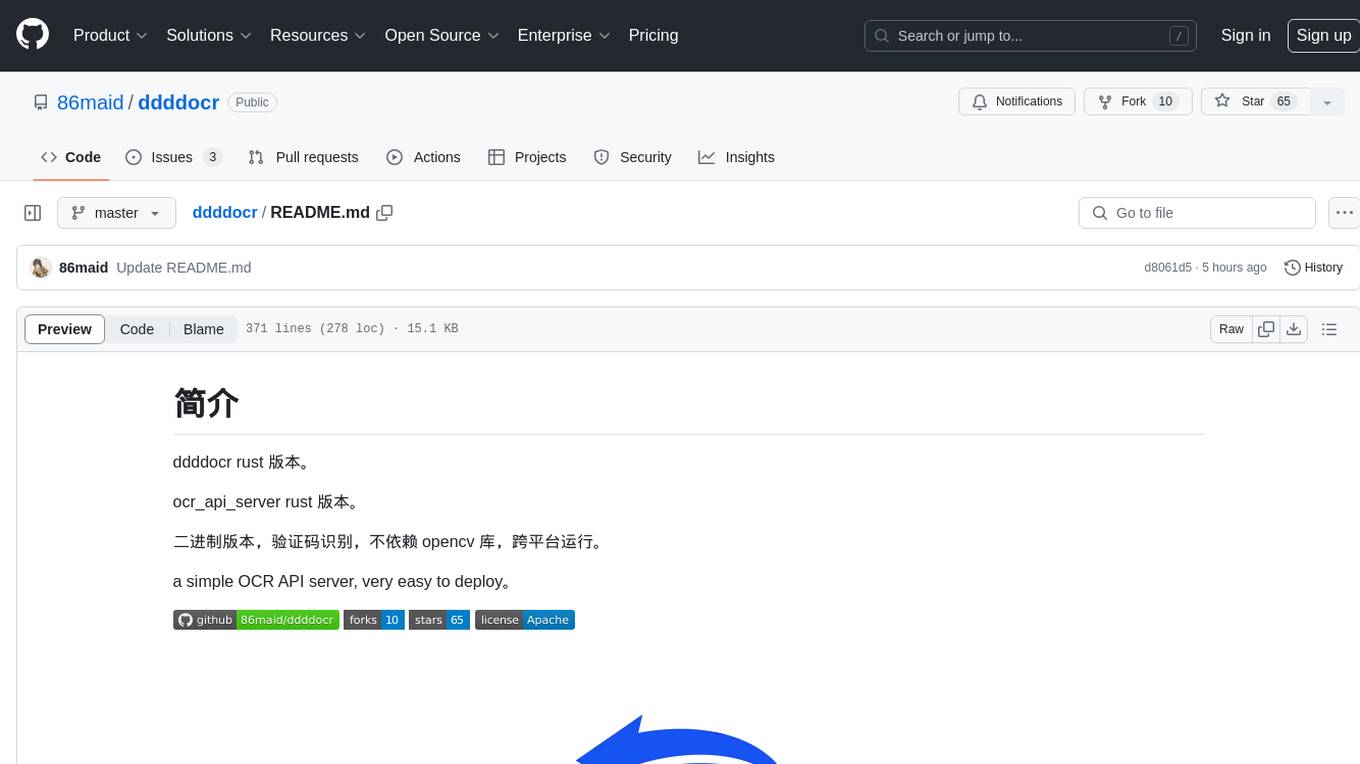
ddddocr
ddddocr is a Rust version of a simple OCR API server that provides easy deployment for captcha recognition without relying on the OpenCV library. It offers a user-friendly general-purpose captcha recognition Rust library. The tool supports recognizing various types of captchas, including single-line text, transparent black PNG images, target detection, and slider matching algorithms. Users can also import custom OCR training models and utilize the OCR API server for flexible OCR result control and range limitation. The tool is cross-platform and can be easily deployed.
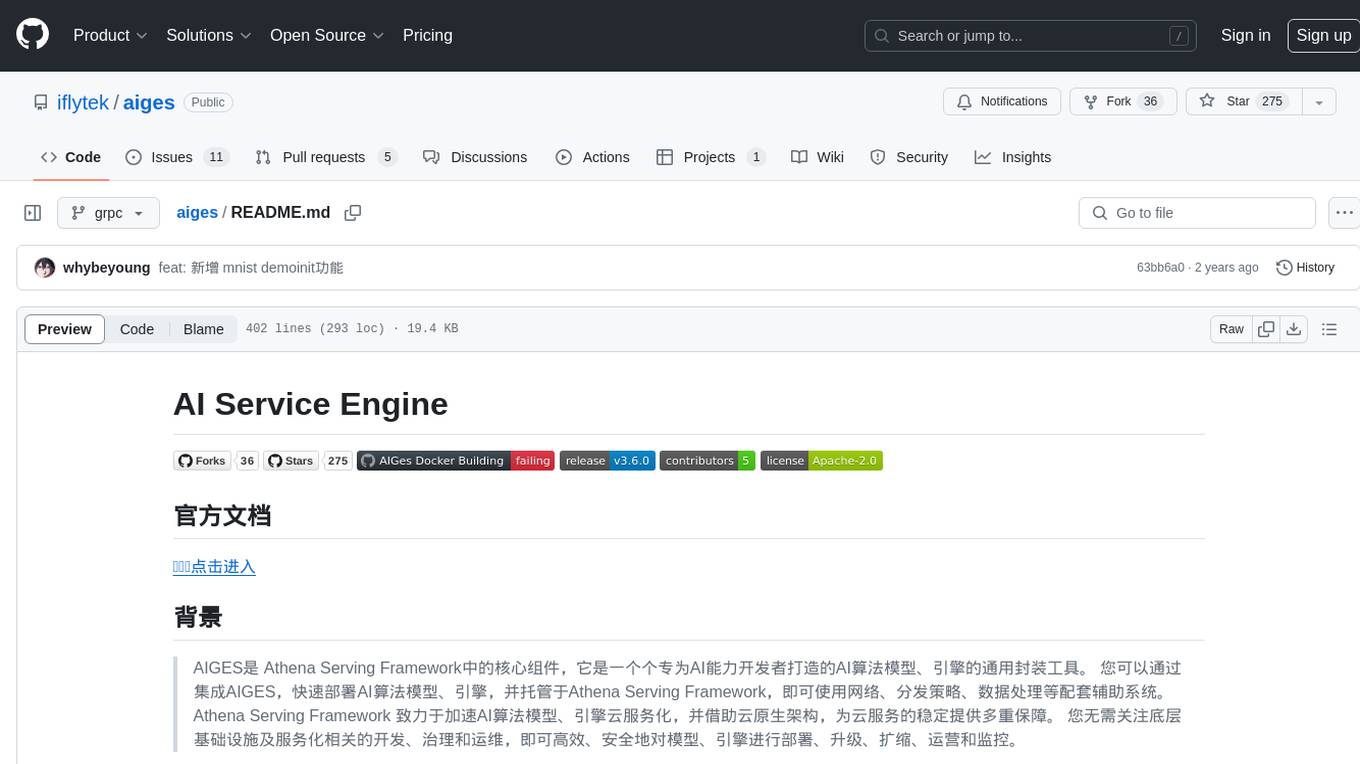
aiges
AIGES is a core component of the Athena Serving Framework, designed as a universal encapsulation tool for AI developers to deploy AI algorithm models and engines quickly. By integrating AIGES, you can deploy AI algorithm models and engines rapidly and host them on the Athena Serving Framework, utilizing supporting auxiliary systems for networking, distribution strategies, data processing, etc. The Athena Serving Framework aims to accelerate the cloud service of AI algorithm models and engines, providing multiple guarantees for cloud service stability through cloud-native architecture. You can efficiently and securely deploy, upgrade, scale, operate, and monitor models and engines without focusing on underlying infrastructure and service-related development, governance, and operations.
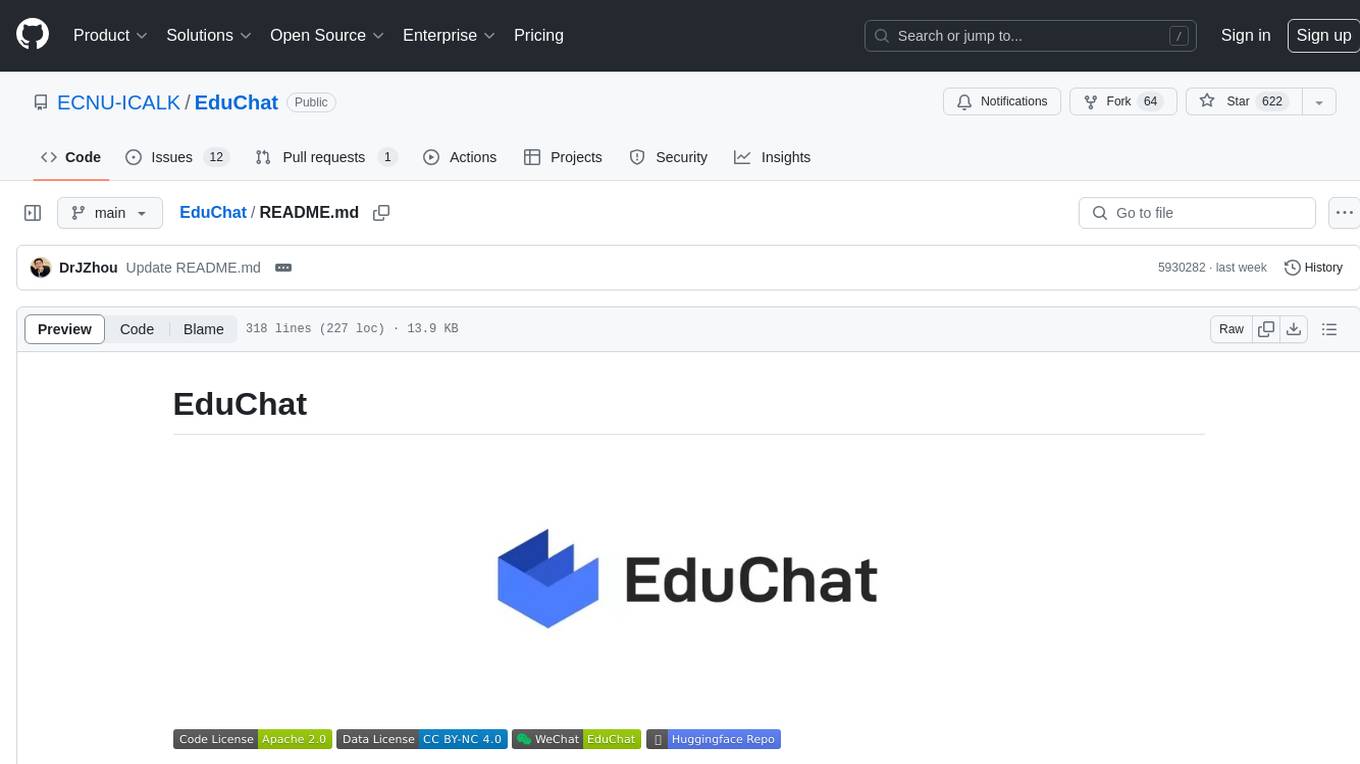
EduChat
EduChat is a large-scale language model-based chatbot system designed for intelligent education by the EduNLP team at East China Normal University. The project focuses on developing a dialogue-based language model for the education vertical domain, integrating diverse education vertical domain data, and providing functions such as automatic question generation, homework correction, emotional support, course guidance, and college entrance examination consultation. The tool aims to serve teachers, students, and parents to achieve personalized, fair, and warm intelligent education.
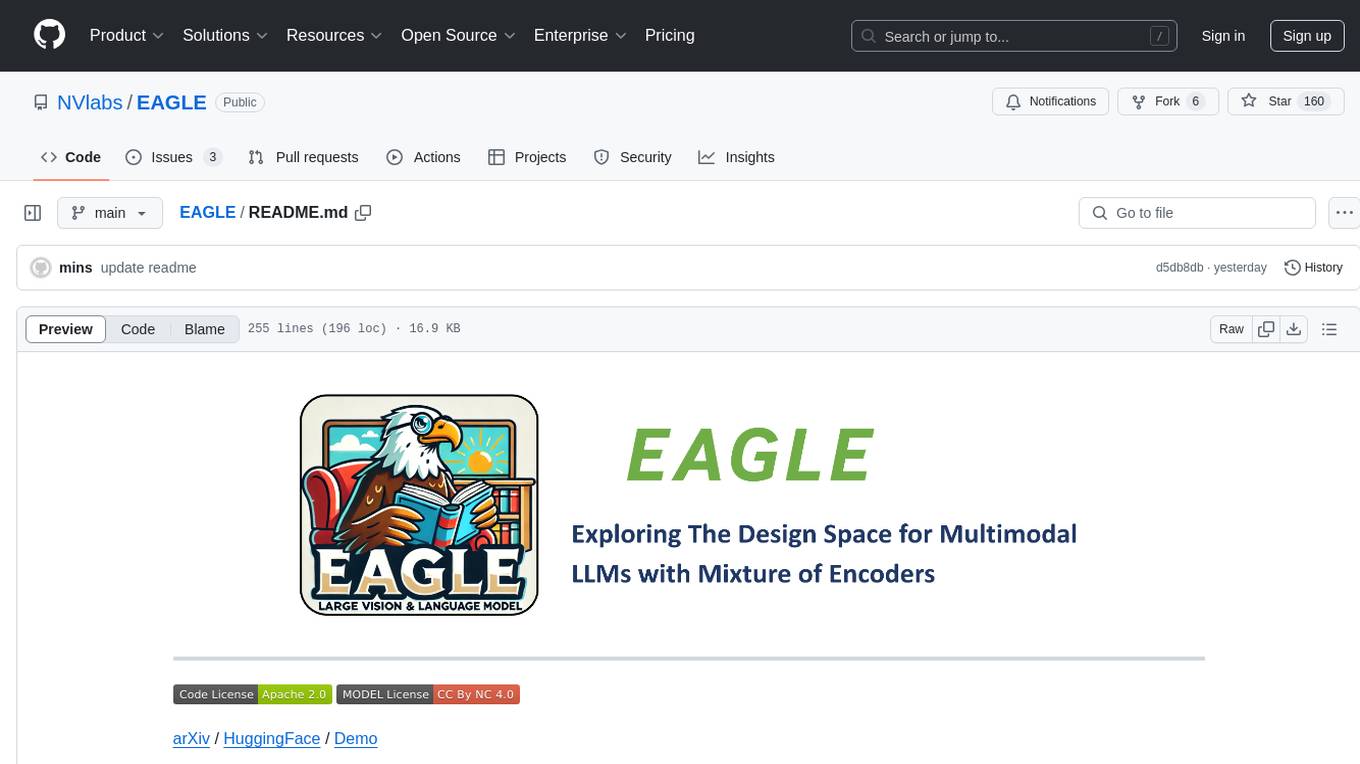
EAGLE
Eagle is a family of Vision-Centric High-Resolution Multimodal LLMs that enhance multimodal LLM perception using a mix of vision encoders and various input resolutions. The model features a channel-concatenation-based fusion for vision experts with different architectures and knowledge, supporting up to over 1K input resolution. It excels in resolution-sensitive tasks like optical character recognition and document understanding.
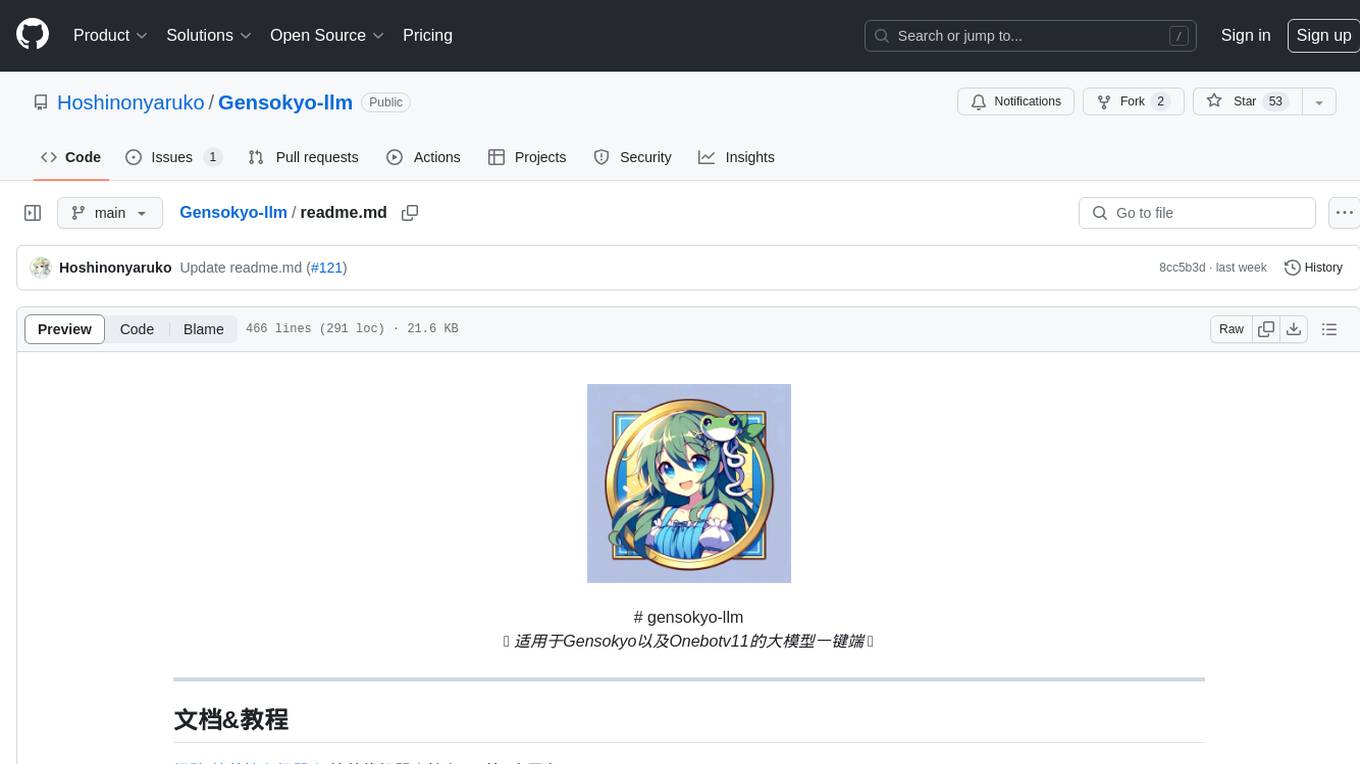
Gensokyo-llm
Gensokyo-llm is a tool designed for Gensokyo and Onebotv11, providing a one-click solution for large models. It supports various Onebotv11 standard frameworks, HTTP-API, and reverse WS. The tool is lightweight, with built-in SQLite for context maintenance and proxy support. It allows easy integration with the Gensokyo framework by configuring reverse HTTP and forward HTTP addresses. Users can set system settings, role cards, and context length. Additionally, it offers an openai original flavor API with automatic context. The tool can be used as an API or integrated with QQ channel robots. It supports converting GPT's SSE type and ensures memory safety in concurrent SSE environments. The tool also supports multiple users simultaneously transmitting SSE bidirectionally.
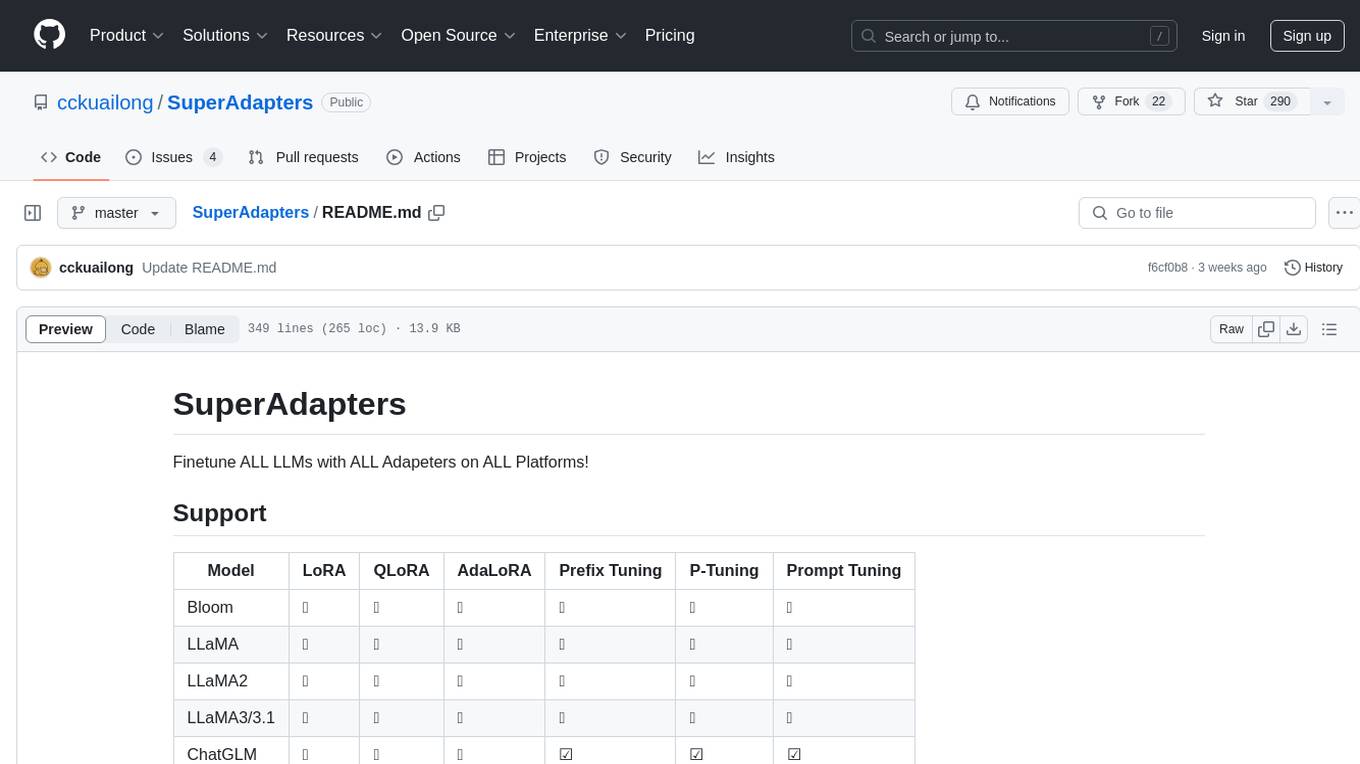
SuperAdapters
SuperAdapters is a tool designed to finetune Large Language Models (LLMs) with various adapters on different platforms. It supports models like Bloom, LLaMA, ChatGLM, Qwen, Baichuan, Mixtral, Phi, and more. Users can finetune LLMs on Windows, Linux, and Mac M1/2, handle train/test data with Terminal, File, or DataBase, and perform tasks like CausalLM and SequenceClassification. The tool provides detailed instructions on how to use different models with specific adapters for tasks like finetuning and inference. It also includes requirements for CentOS, Ubuntu, and MacOS, along with information on LLM downloads and data formats. Additionally, it offers parameters for finetuning and inference, as well as options for web and API-based inference.
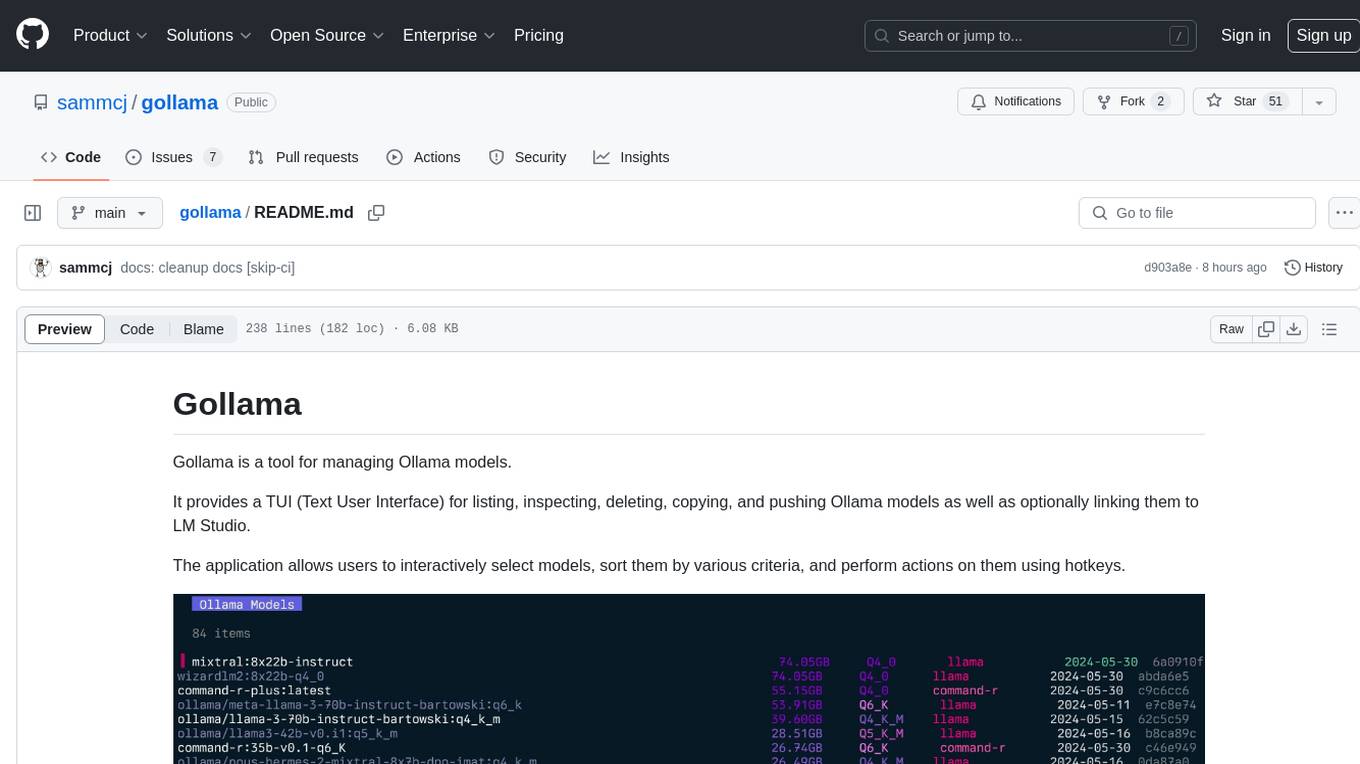
gollama
Gollama is a tool designed for managing Ollama models through a Text User Interface (TUI). Users can list, inspect, delete, copy, and push Ollama models, as well as link them to LM Studio. The application offers interactive model selection, sorting by various criteria, and actions using hotkeys. It provides features like sorting and filtering capabilities, displaying model metadata, model linking, copying, pushing, and more. Gollama aims to be user-friendly and useful for managing models, especially for cleaning up old models.
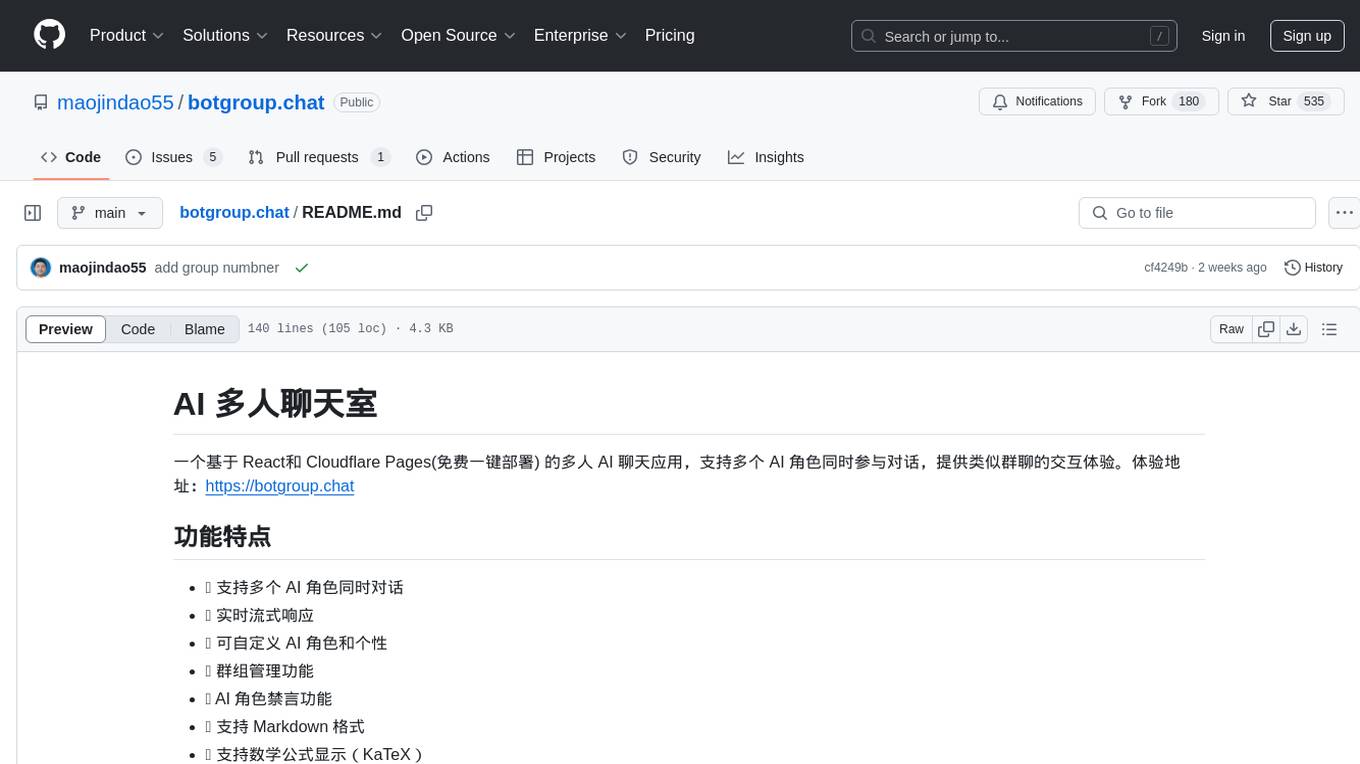
botgroup.chat
botgroup.chat is a multi-person AI chat application based on React and Cloudflare Pages for free one-click deployment. It supports multiple AI roles participating in conversations simultaneously, providing an interactive experience similar to group chat. The application features real-time streaming responses, customizable AI roles and personalities, group management functionality, AI role mute function, Markdown format support, mathematical formula display with KaTeX, aesthetically pleasing UI design, and responsive design for mobile devices.
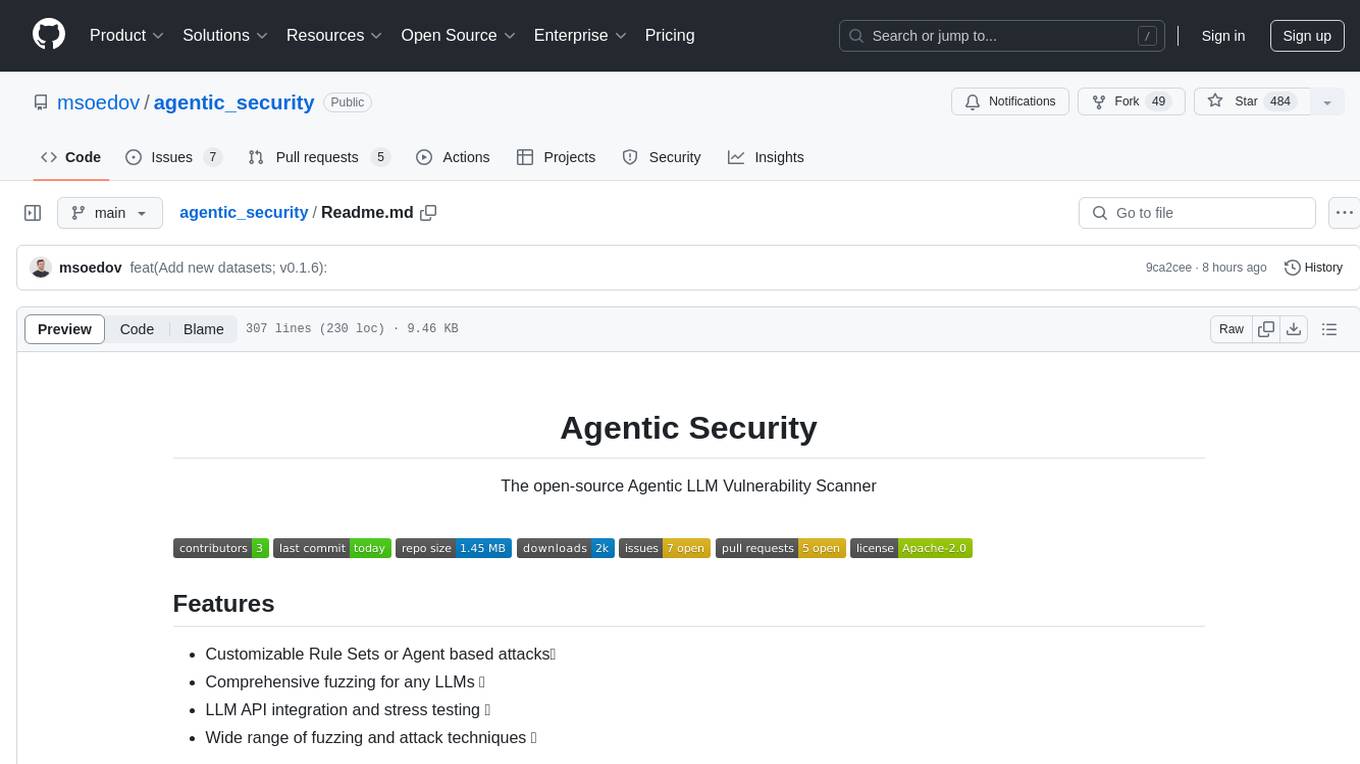
agentic_security
Agentic Security is an open-source vulnerability scanner designed for safety scanning, offering customizable rule sets and agent-based attacks. It provides comprehensive fuzzing for any LLMs, LLM API integration, and stress testing with a wide range of fuzzing and attack techniques. The tool is not a foolproof solution but aims to enhance security measures against potential threats. It offers installation via pip and supports quick start commands for easy setup. Users can utilize the tool for LLM integration, adding custom datasets, running CI checks, extending dataset collections, and dynamic datasets with mutations. The tool also includes a probe endpoint for integration testing. The roadmap includes expanding dataset variety, introducing new attack vectors, developing an attacker LLM, and integrating OWASP Top 10 classification.
For similar tasks
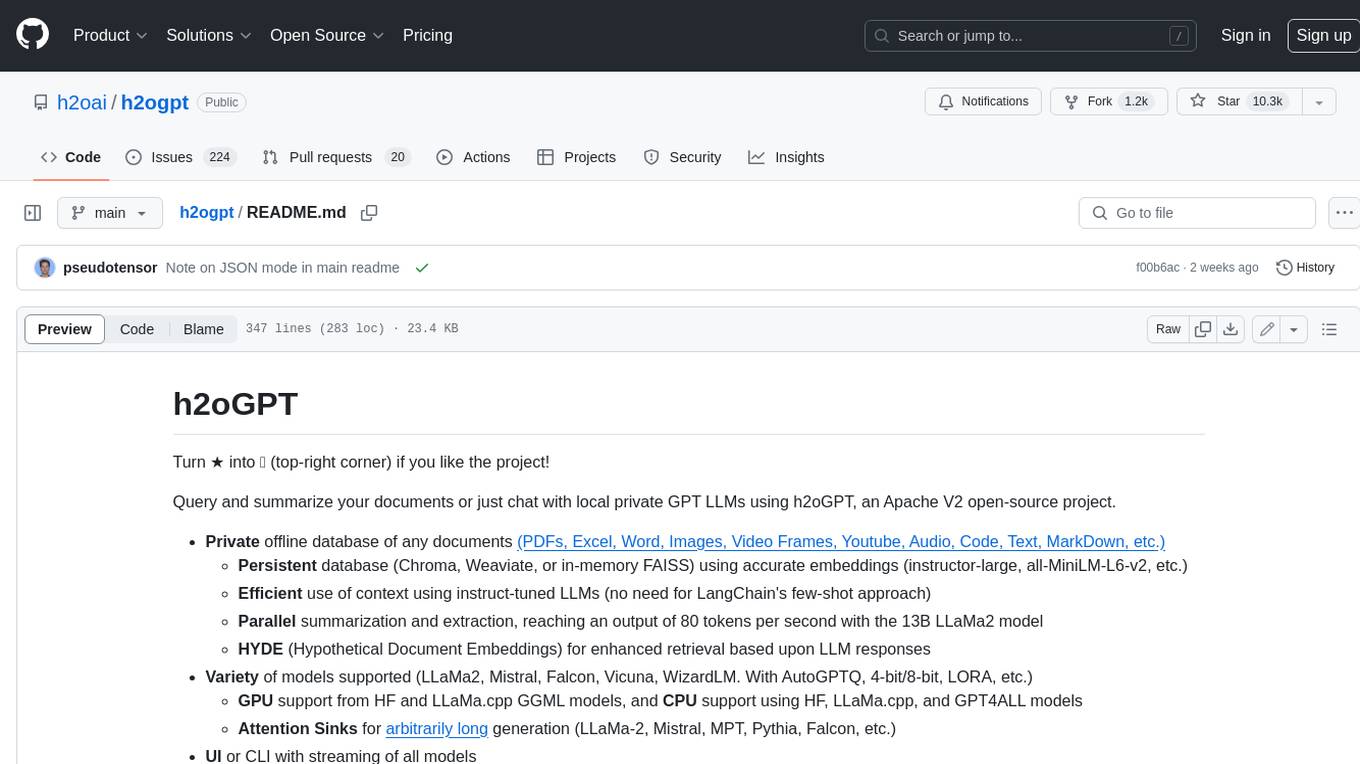
h2ogpt
h2oGPT is an Apache V2 open-source project that allows users to query and summarize documents or chat with local private GPT LLMs. It features a private offline database of any documents (PDFs, Excel, Word, Images, Video Frames, Youtube, Audio, Code, Text, MarkDown, etc.), a persistent database (Chroma, Weaviate, or in-memory FAISS) using accurate embeddings (instructor-large, all-MiniLM-L6-v2, etc.), and efficient use of context using instruct-tuned LLMs (no need for LangChain's few-shot approach). h2oGPT also offers parallel summarization and extraction, reaching an output of 80 tokens per second with the 13B LLaMa2 model, HYDE (Hypothetical Document Embeddings) for enhanced retrieval based upon LLM responses, a variety of models supported (LLaMa2, Mistral, Falcon, Vicuna, WizardLM. With AutoGPTQ, 4-bit/8-bit, LORA, etc.), GPU support from HF and LLaMa.cpp GGML models, and CPU support using HF, LLaMa.cpp, and GPT4ALL models. Additionally, h2oGPT provides Attention Sinks for arbitrarily long generation (LLaMa-2, Mistral, MPT, Pythia, Falcon, etc.), a UI or CLI with streaming of all models, the ability to upload and view documents through the UI (control multiple collaborative or personal collections), Vision Models LLaVa, Claude-3, Gemini-Pro-Vision, GPT-4-Vision, Image Generation Stable Diffusion (sdxl-turbo, sdxl) and PlaygroundAI (playv2), Voice STT using Whisper with streaming audio conversion, Voice TTS using MIT-Licensed Microsoft Speech T5 with multiple voices and Streaming audio conversion, Voice TTS using MPL2-Licensed TTS including Voice Cloning and Streaming audio conversion, AI Assistant Voice Control Mode for hands-free control of h2oGPT chat, Bake-off UI mode against many models at the same time, Easy Download of model artifacts and control over models like LLaMa.cpp through the UI, Authentication in the UI by user/password via Native or Google OAuth, State Preservation in the UI by user/password, Linux, Docker, macOS, and Windows support, Easy Windows Installer for Windows 10 64-bit (CPU/CUDA), Easy macOS Installer for macOS (CPU/M1/M2), Inference Servers support (oLLaMa, HF TGI server, vLLM, Gradio, ExLLaMa, Replicate, OpenAI, Azure OpenAI, Anthropic), OpenAI-compliant, Server Proxy API (h2oGPT acts as drop-in-replacement to OpenAI server), Python client API (to talk to Gradio server), JSON Mode with any model via code block extraction. Also supports MistralAI JSON mode, Claude-3 via function calling with strict Schema, OpenAI via JSON mode, and vLLM via guided_json with strict Schema, Web-Search integration with Chat and Document Q/A, Agents for Search, Document Q/A, Python Code, CSV frames (Experimental, best with OpenAI currently), Evaluate performance using reward models, and Quality maintained with over 1000 unit and integration tests taking over 4 GPU-hours.
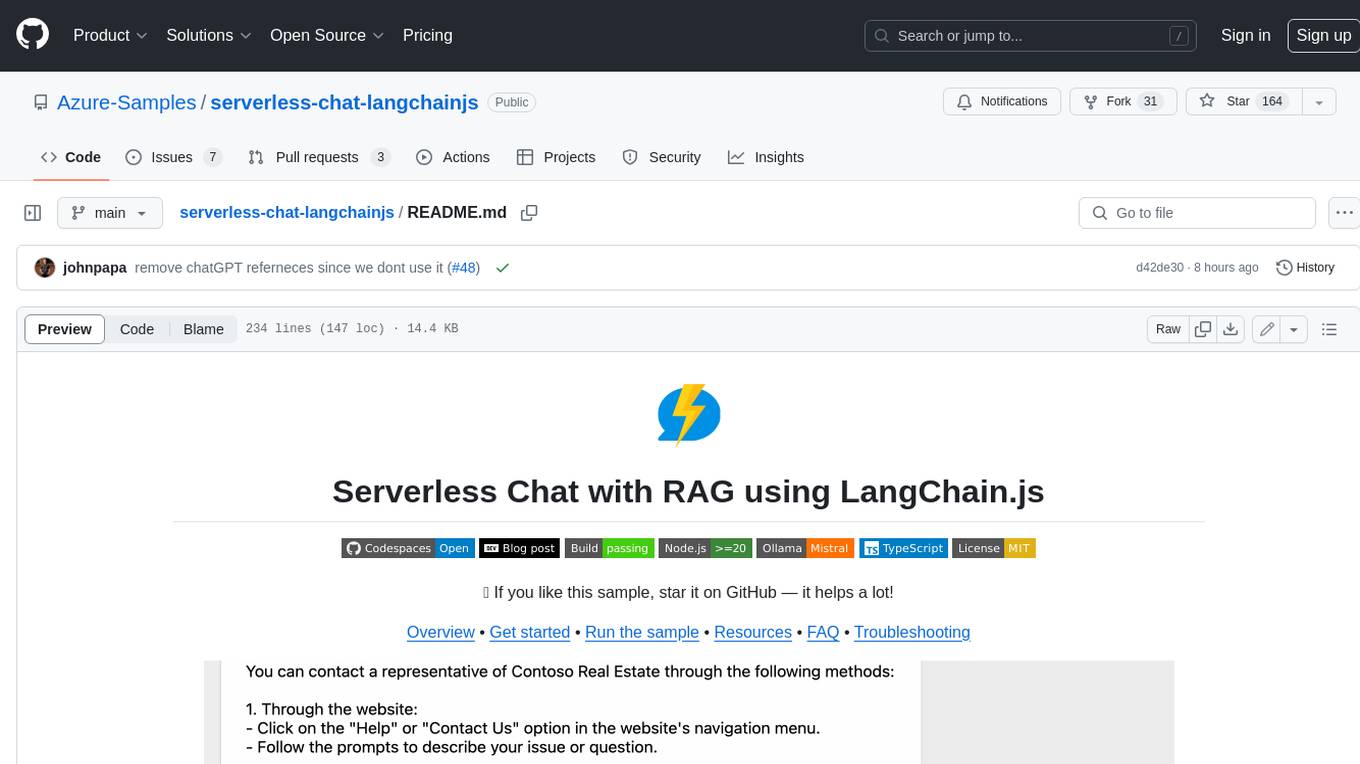
serverless-chat-langchainjs
This sample shows how to build a serverless chat experience with Retrieval-Augmented Generation using LangChain.js and Azure. The application is hosted on Azure Static Web Apps and Azure Functions, with Azure Cosmos DB for MongoDB vCore as the vector database. You can use it as a starting point for building more complex AI applications.
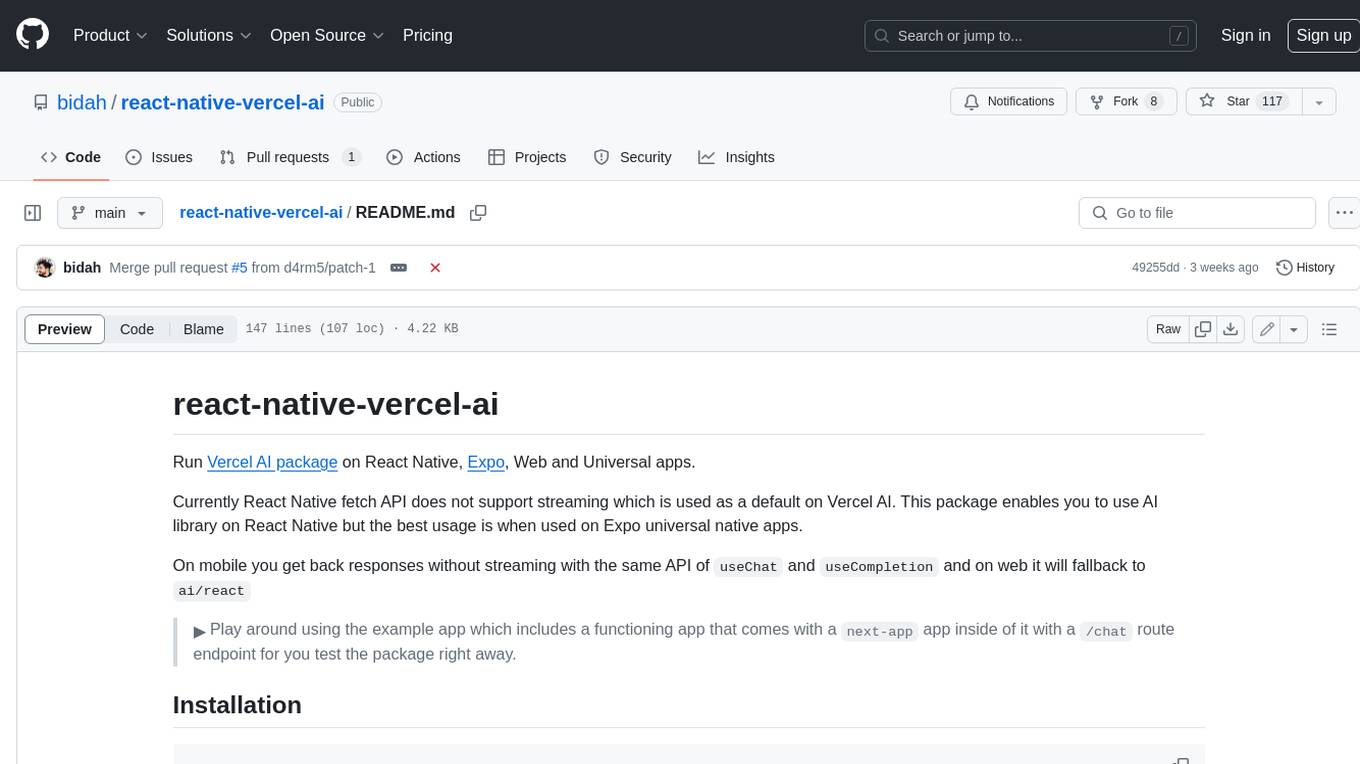
react-native-vercel-ai
Run Vercel AI package on React Native, Expo, Web and Universal apps. Currently React Native fetch API does not support streaming which is used as a default on Vercel AI. This package enables you to use AI library on React Native but the best usage is when used on Expo universal native apps. On mobile you get back responses without streaming with the same API of `useChat` and `useCompletion` and on web it will fallback to `ai/react`
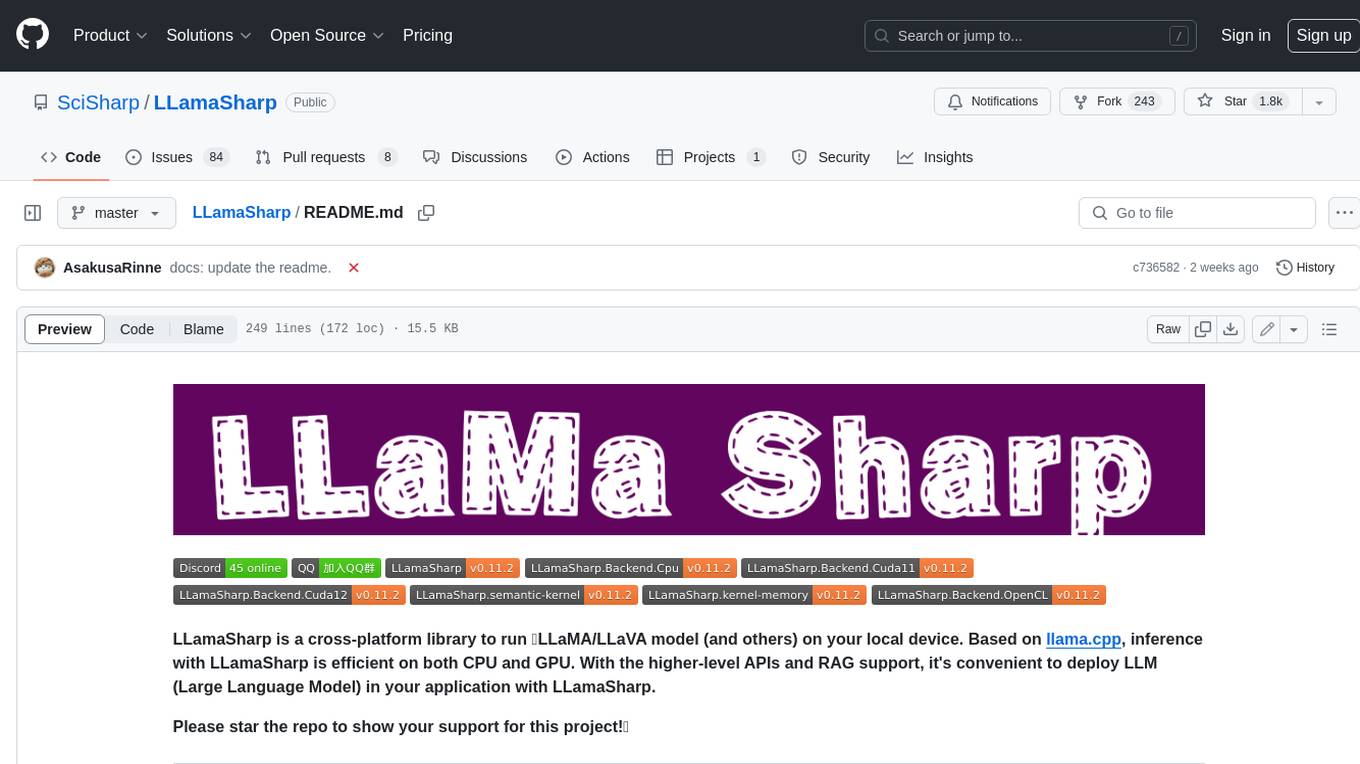
LLamaSharp
LLamaSharp is a cross-platform library to run 🦙LLaMA/LLaVA model (and others) on your local device. Based on llama.cpp, inference with LLamaSharp is efficient on both CPU and GPU. With the higher-level APIs and RAG support, it's convenient to deploy LLM (Large Language Model) in your application with LLamaSharp.
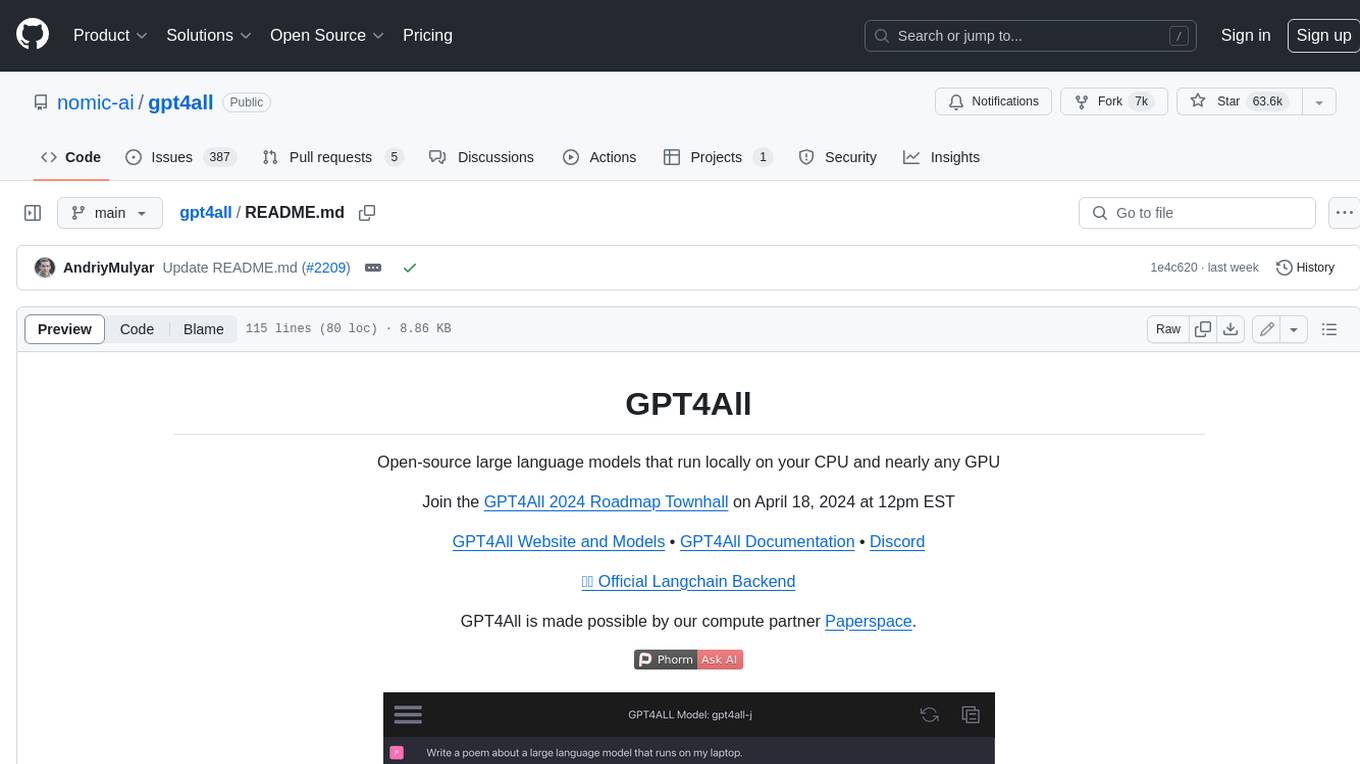
gpt4all
GPT4All is an ecosystem to run powerful and customized large language models that work locally on consumer grade CPUs and any GPU. Note that your CPU needs to support AVX or AVX2 instructions. Learn more in the documentation. A GPT4All model is a 3GB - 8GB file that you can download and plug into the GPT4All open-source ecosystem software. Nomic AI supports and maintains this software ecosystem to enforce quality and security alongside spearheading the effort to allow any person or enterprise to easily train and deploy their own on-edge large language models.
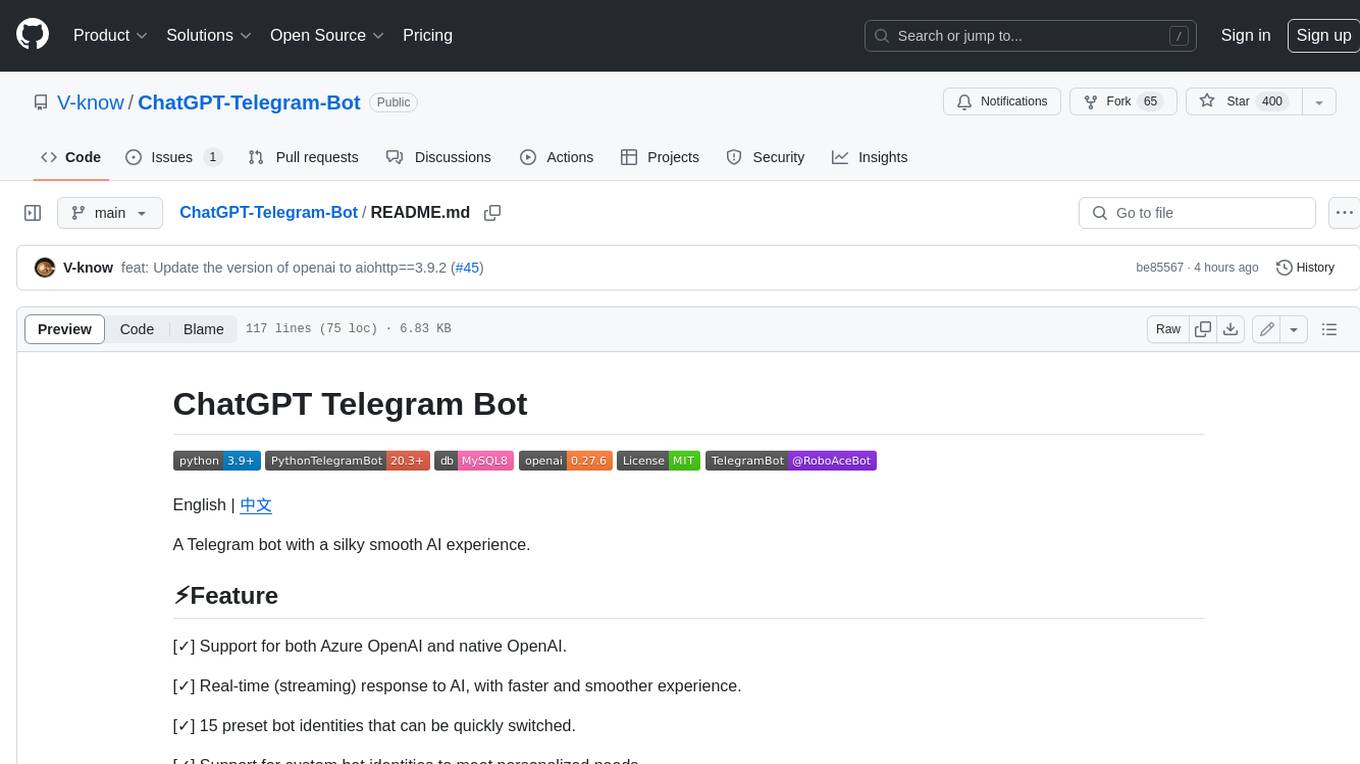
ChatGPT-Telegram-Bot
ChatGPT Telegram Bot is a Telegram bot that provides a smooth AI experience. It supports both Azure OpenAI and native OpenAI, and offers real-time (streaming) response to AI, with a faster and smoother experience. The bot also has 15 preset bot identities that can be quickly switched, and supports custom bot identities to meet personalized needs. Additionally, it supports clearing the contents of the chat with a single click, and restarting the conversation at any time. The bot also supports native Telegram bot button support, making it easy and intuitive to implement required functions. User level division is also supported, with different levels enjoying different single session token numbers, context numbers, and session frequencies. The bot supports English and Chinese on UI, and is containerized for easy deployment.
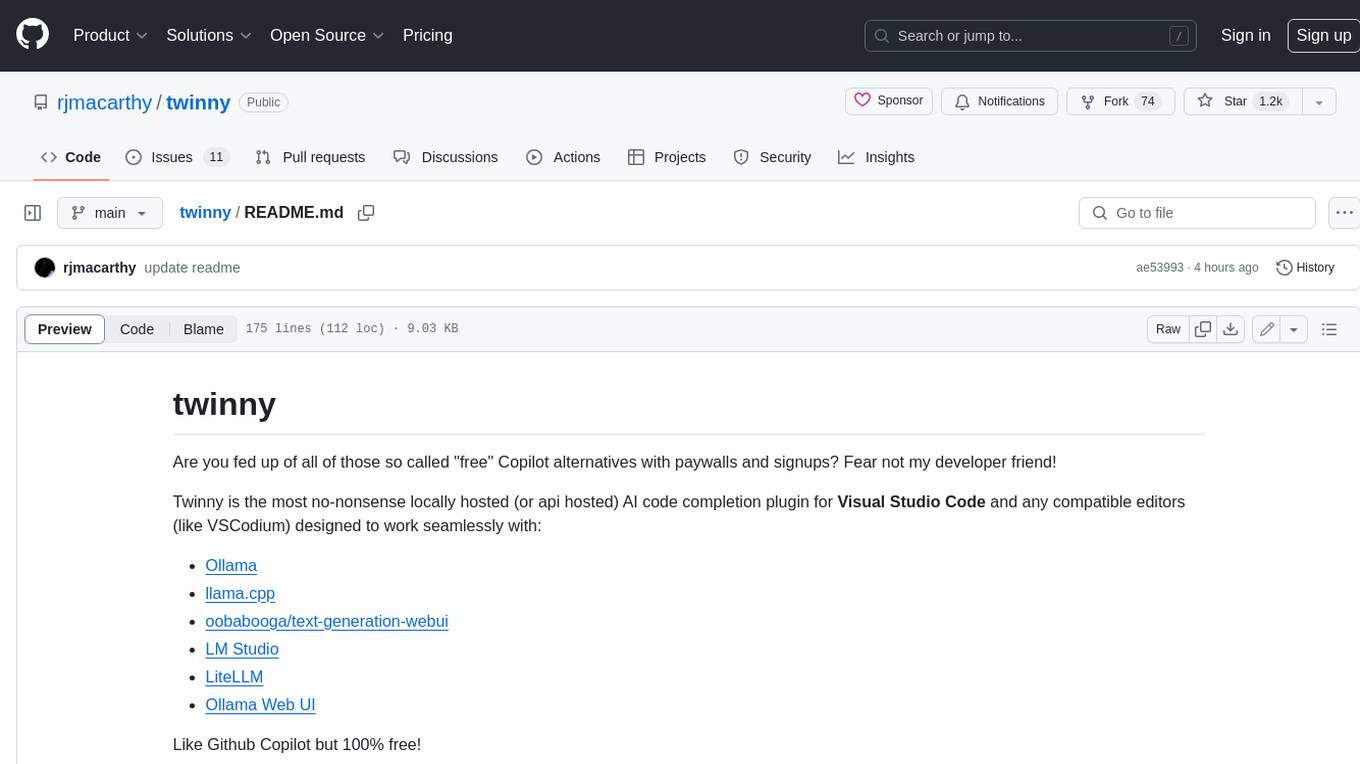
twinny
Twinny is a free and open-source AI code completion plugin for Visual Studio Code and compatible editors. It integrates with various tools and frameworks, including Ollama, llama.cpp, oobabooga/text-generation-webui, LM Studio, LiteLLM, and Open WebUI. Twinny offers features such as fill-in-the-middle code completion, chat with AI about your code, customizable API endpoints, and support for single or multiline fill-in-middle completions. It is easy to install via the Visual Studio Code extensions marketplace and provides a range of customization options. Twinny supports both online and offline operation and conforms to the OpenAI API standard.
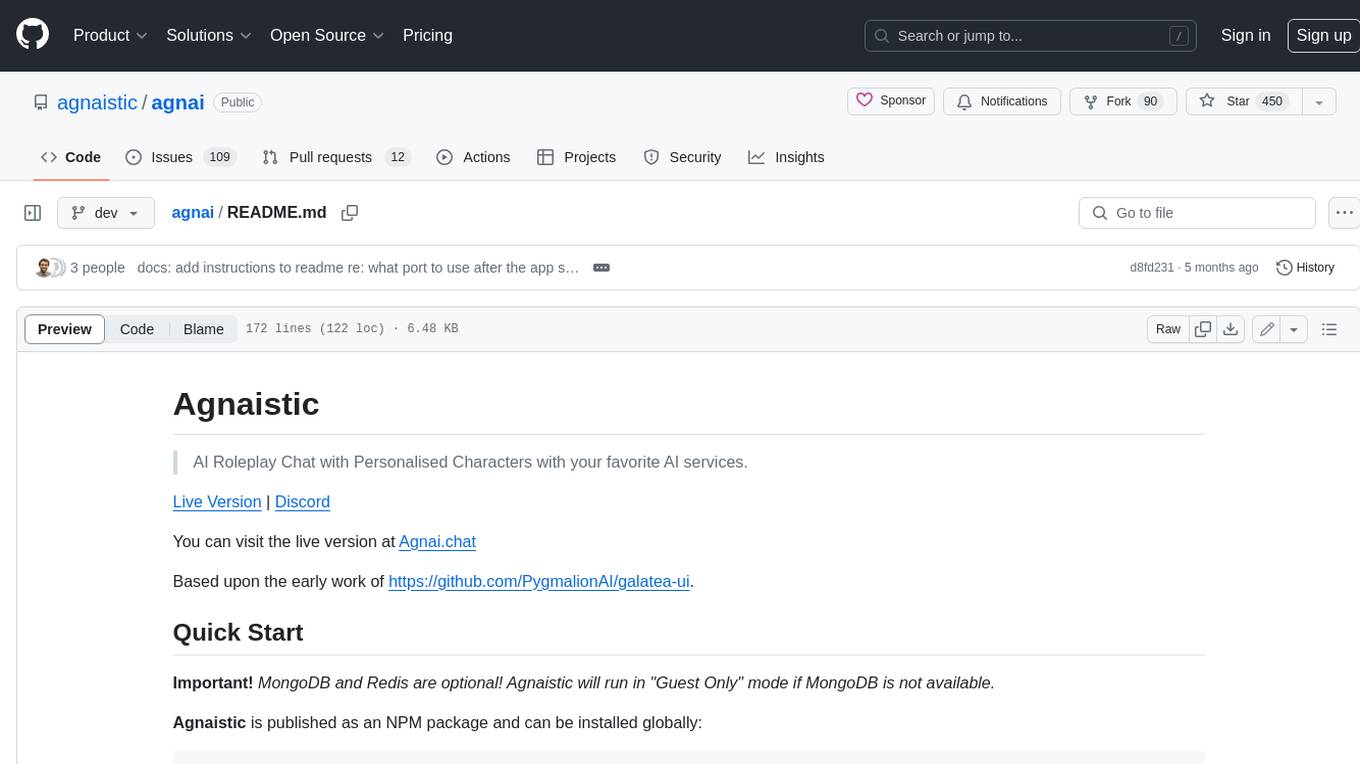
agnai
Agnaistic is an AI roleplay chat tool that allows users to interact with personalized characters using their favorite AI services. It supports multiple AI services, persona schema formats, and features such as group conversations, user authentication, and memory/lore books. Agnaistic can be self-hosted or run using Docker, and it provides a range of customization options through its settings.json file. The tool is designed to be user-friendly and accessible, making it suitable for both casual users and developers.
For similar jobs
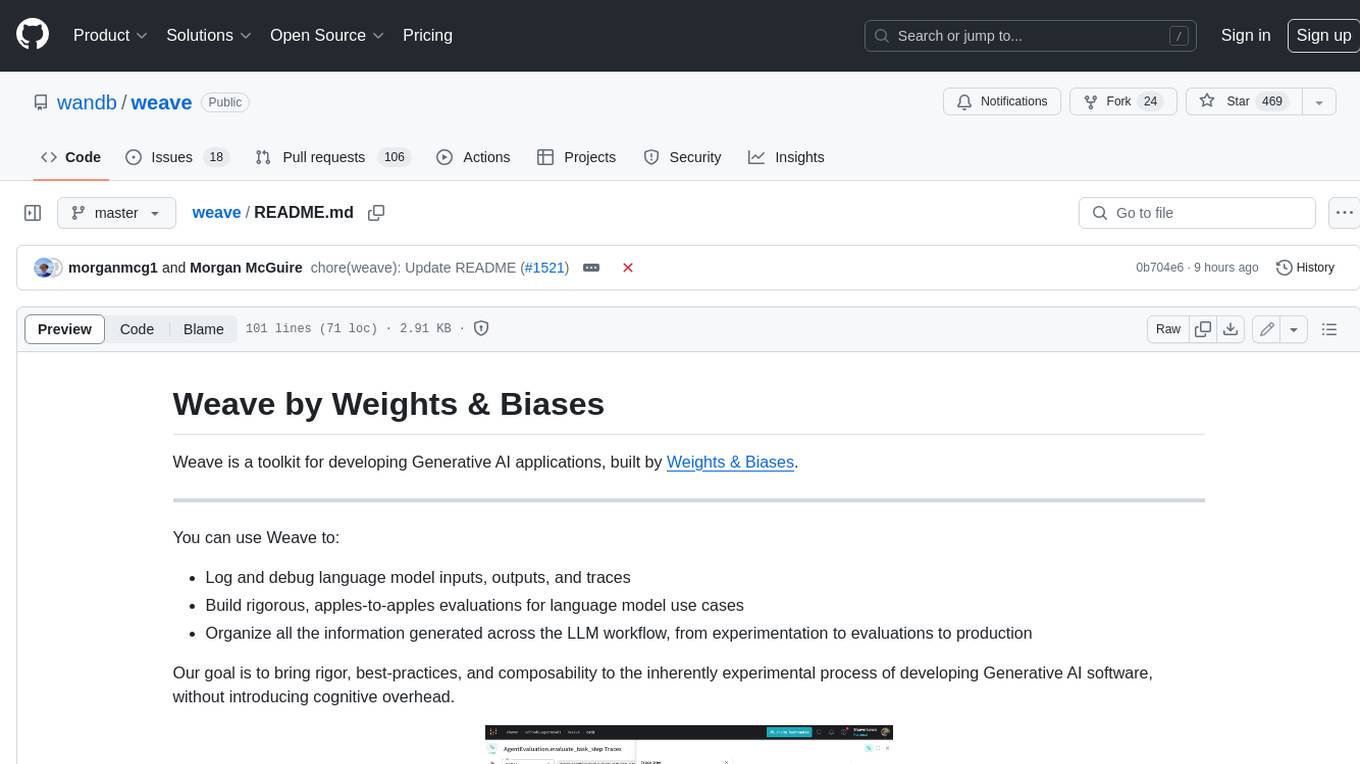
weave
Weave is a toolkit for developing Generative AI applications, built by Weights & Biases. With Weave, you can log and debug language model inputs, outputs, and traces; build rigorous, apples-to-apples evaluations for language model use cases; and organize all the information generated across the LLM workflow, from experimentation to evaluations to production. Weave aims to bring rigor, best-practices, and composability to the inherently experimental process of developing Generative AI software, without introducing cognitive overhead.
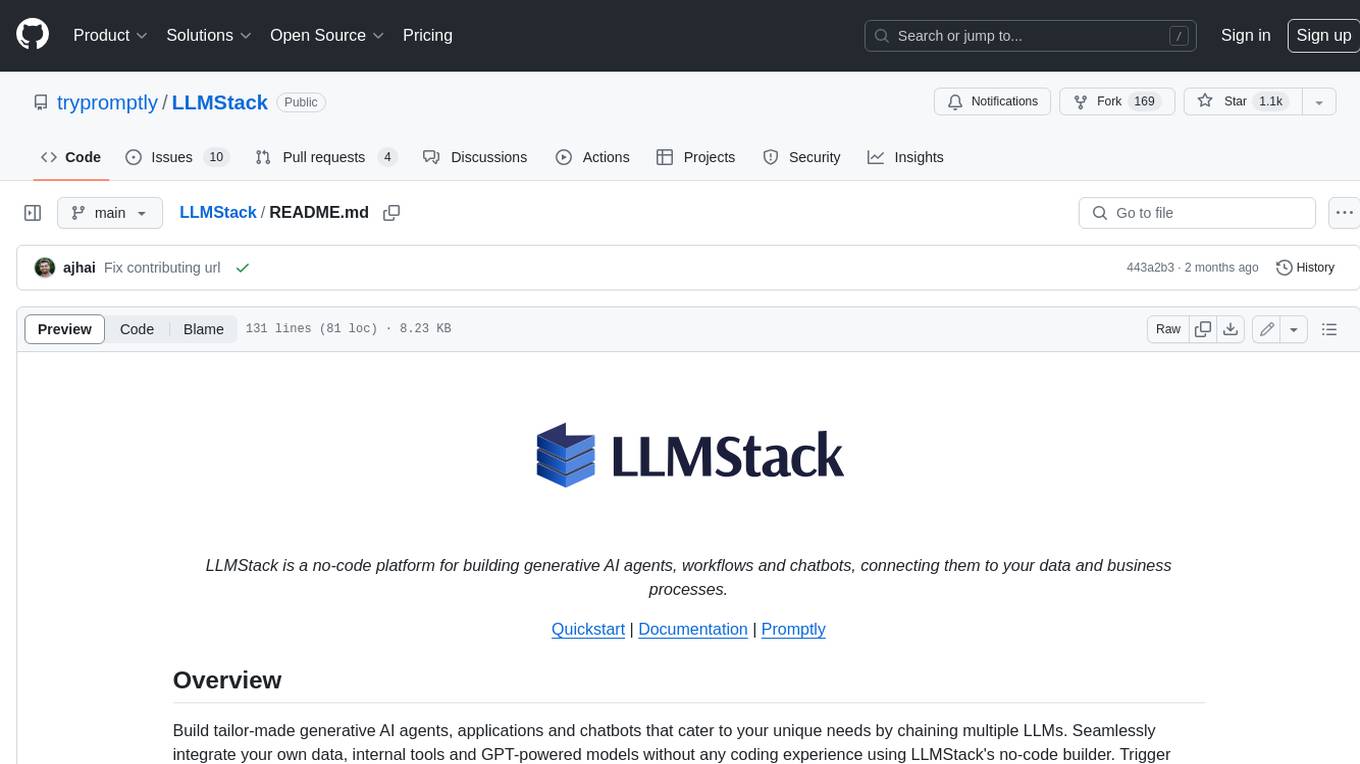
LLMStack
LLMStack is a no-code platform for building generative AI agents, workflows, and chatbots. It allows users to connect their own data, internal tools, and GPT-powered models without any coding experience. LLMStack can be deployed to the cloud or on-premise and can be accessed via HTTP API or triggered from Slack or Discord.
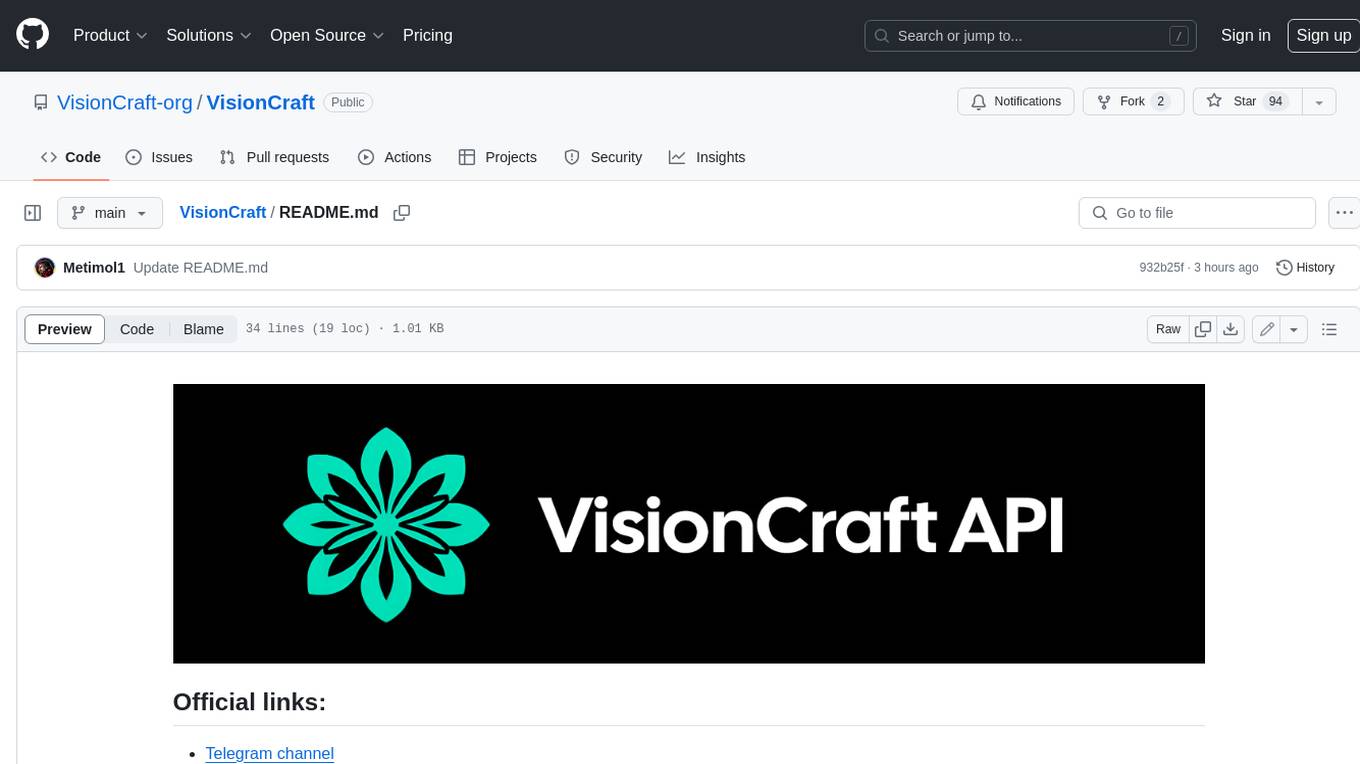
VisionCraft
The VisionCraft API is a free API for using over 100 different AI models. From images to sound.
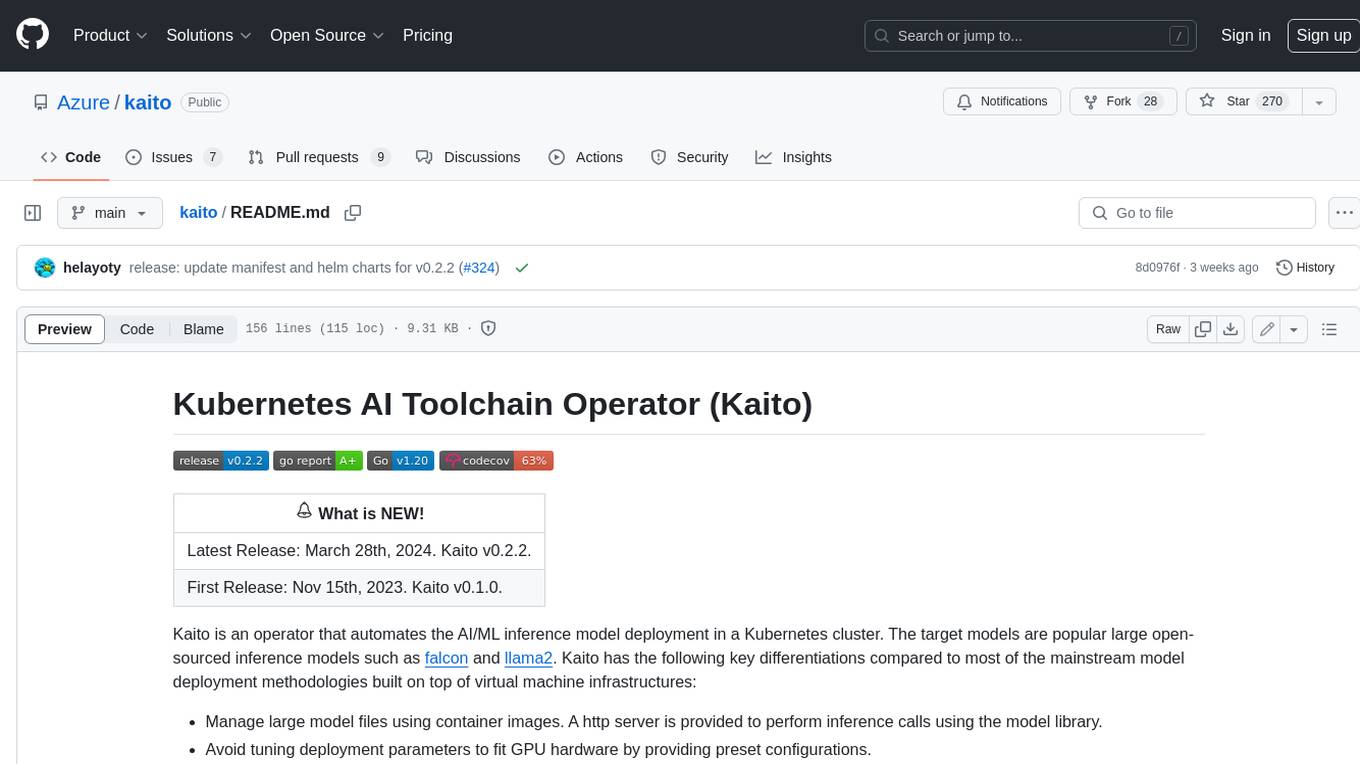
kaito
Kaito is an operator that automates the AI/ML inference model deployment in a Kubernetes cluster. It manages large model files using container images, avoids tuning deployment parameters to fit GPU hardware by providing preset configurations, auto-provisions GPU nodes based on model requirements, and hosts large model images in the public Microsoft Container Registry (MCR) if the license allows. Using Kaito, the workflow of onboarding large AI inference models in Kubernetes is largely simplified.
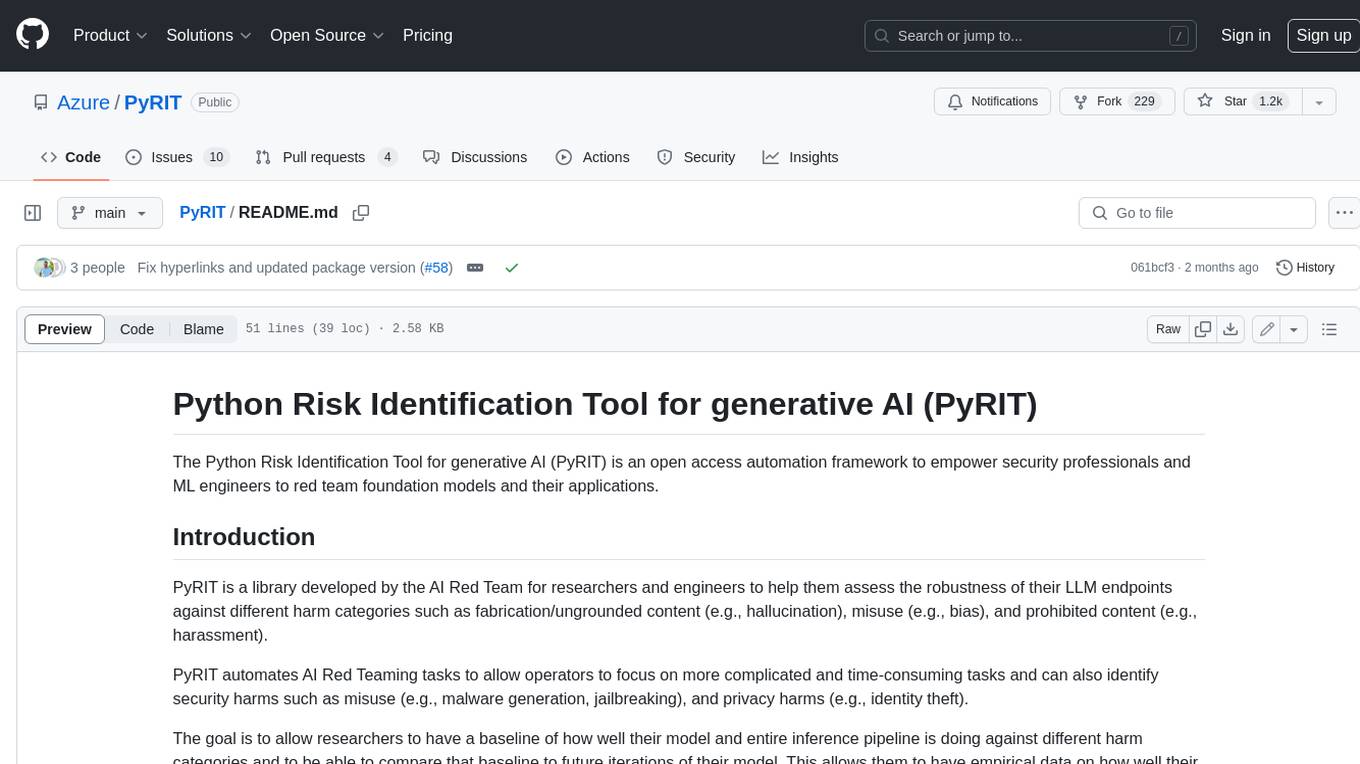
PyRIT
PyRIT is an open access automation framework designed to empower security professionals and ML engineers to red team foundation models and their applications. It automates AI Red Teaming tasks to allow operators to focus on more complicated and time-consuming tasks and can also identify security harms such as misuse (e.g., malware generation, jailbreaking), and privacy harms (e.g., identity theft). The goal is to allow researchers to have a baseline of how well their model and entire inference pipeline is doing against different harm categories and to be able to compare that baseline to future iterations of their model. This allows them to have empirical data on how well their model is doing today, and detect any degradation of performance based on future improvements.
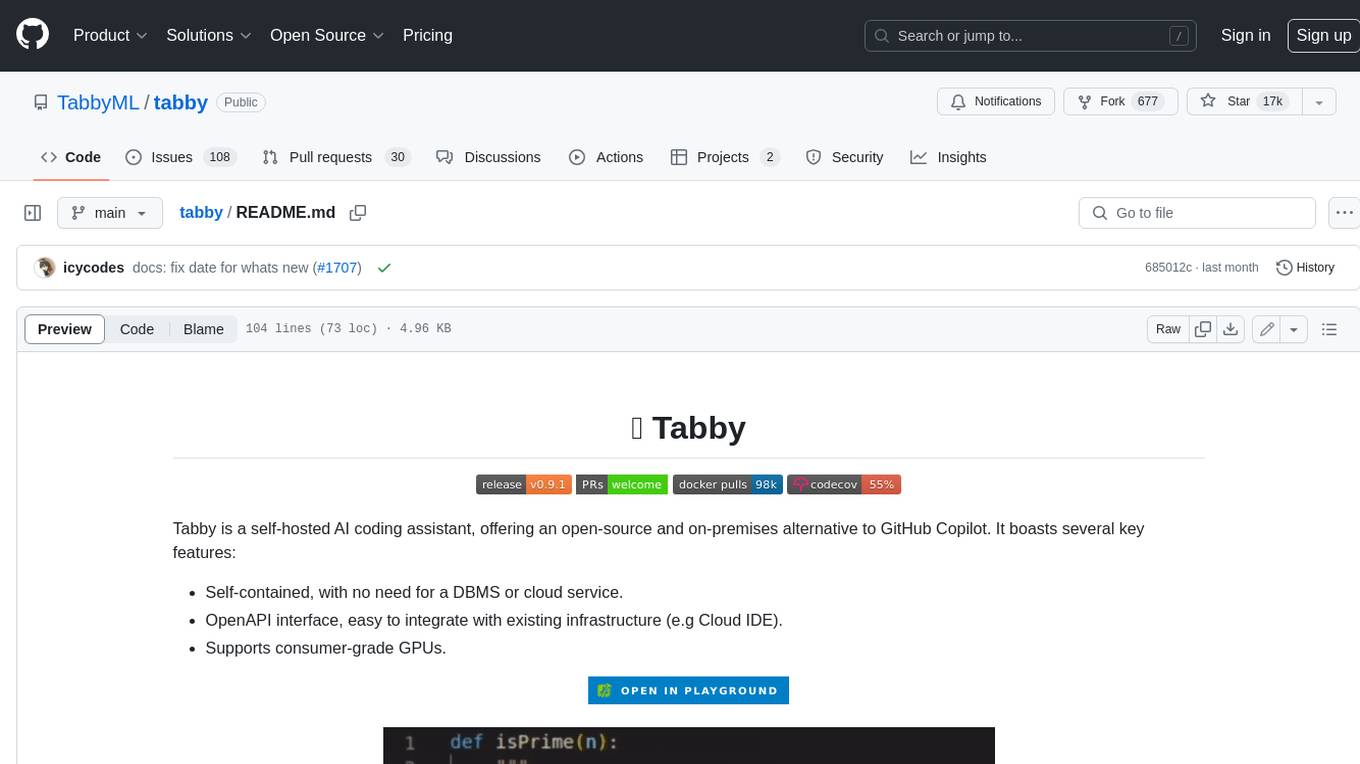
tabby
Tabby is a self-hosted AI coding assistant, offering an open-source and on-premises alternative to GitHub Copilot. It boasts several key features: * Self-contained, with no need for a DBMS or cloud service. * OpenAPI interface, easy to integrate with existing infrastructure (e.g Cloud IDE). * Supports consumer-grade GPUs.
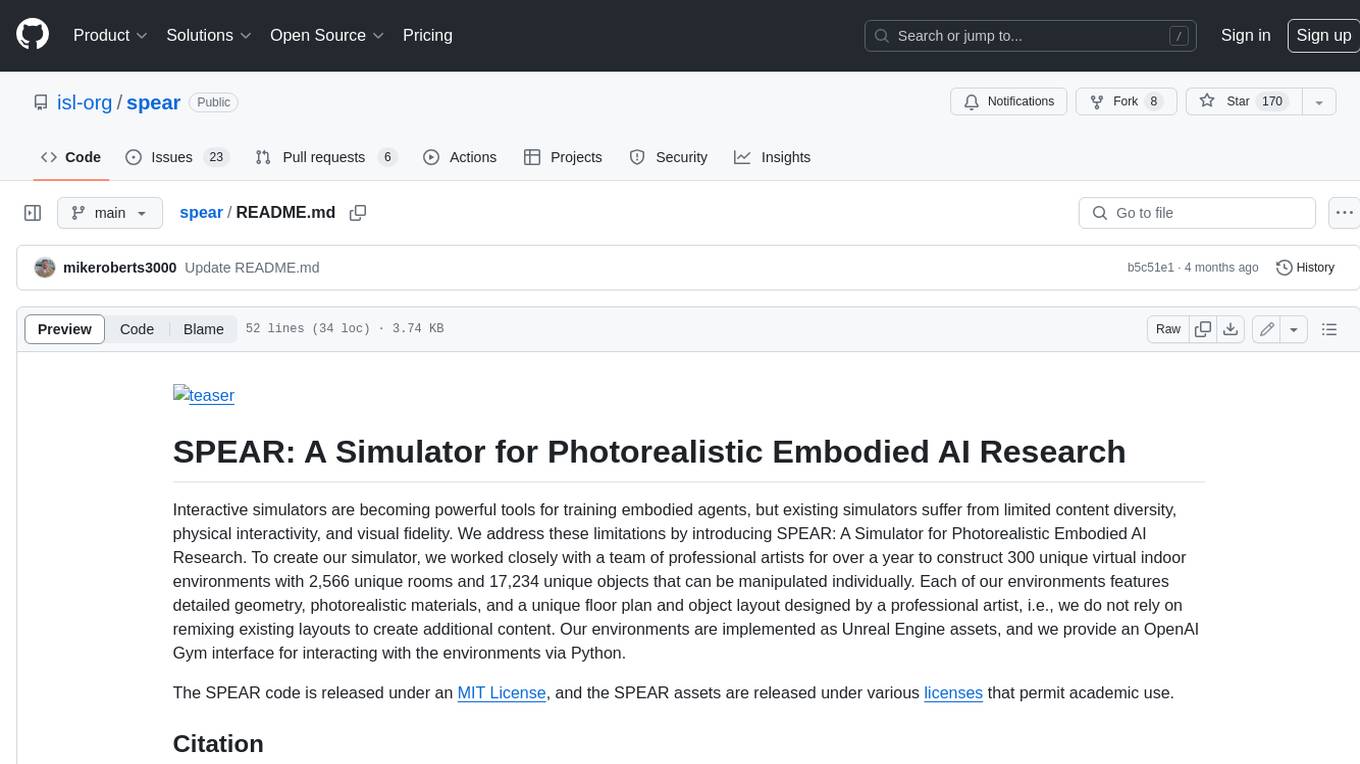
spear
SPEAR (Simulator for Photorealistic Embodied AI Research) is a powerful tool for training embodied agents. It features 300 unique virtual indoor environments with 2,566 unique rooms and 17,234 unique objects that can be manipulated individually. Each environment is designed by a professional artist and features detailed geometry, photorealistic materials, and a unique floor plan and object layout. SPEAR is implemented as Unreal Engine assets and provides an OpenAI Gym interface for interacting with the environments via Python.
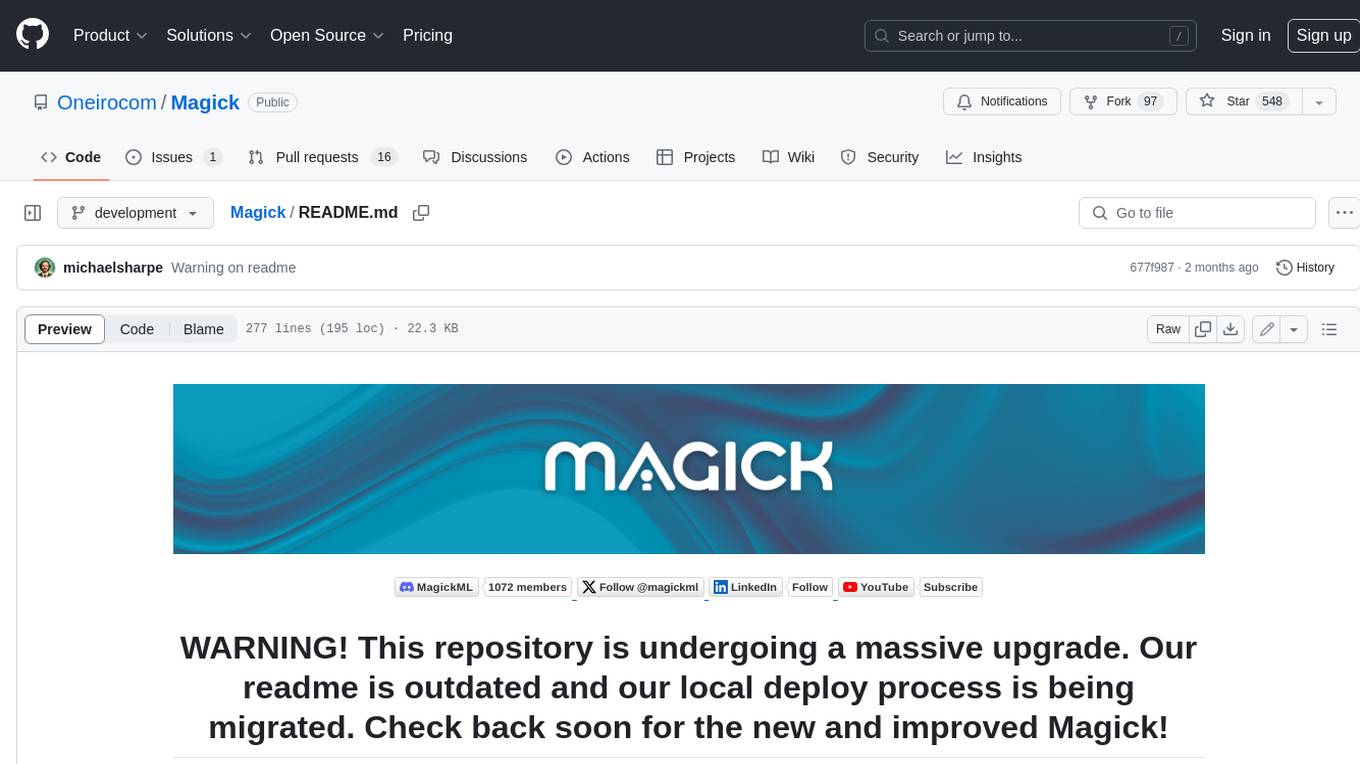
Magick
Magick is a groundbreaking visual AIDE (Artificial Intelligence Development Environment) for no-code data pipelines and multimodal agents. Magick can connect to other services and comes with nodes and templates well-suited for intelligent agents, chatbots, complex reasoning systems and realistic characters.